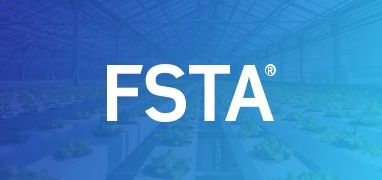
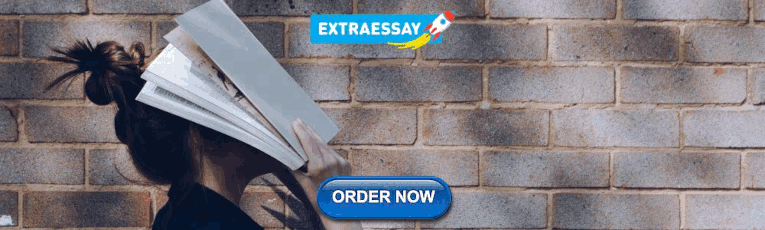
Start your free trial
Arrange a trial for your organisation and discover why FSTA is the leading database for reliable research on the sciences of food and health.
REQUEST A FREE TRIAL
- Research Skills Blog
5 software tools to support your systematic review processes
By Dr. Mina Kalantar on 19-Jan-2021 13:01:01
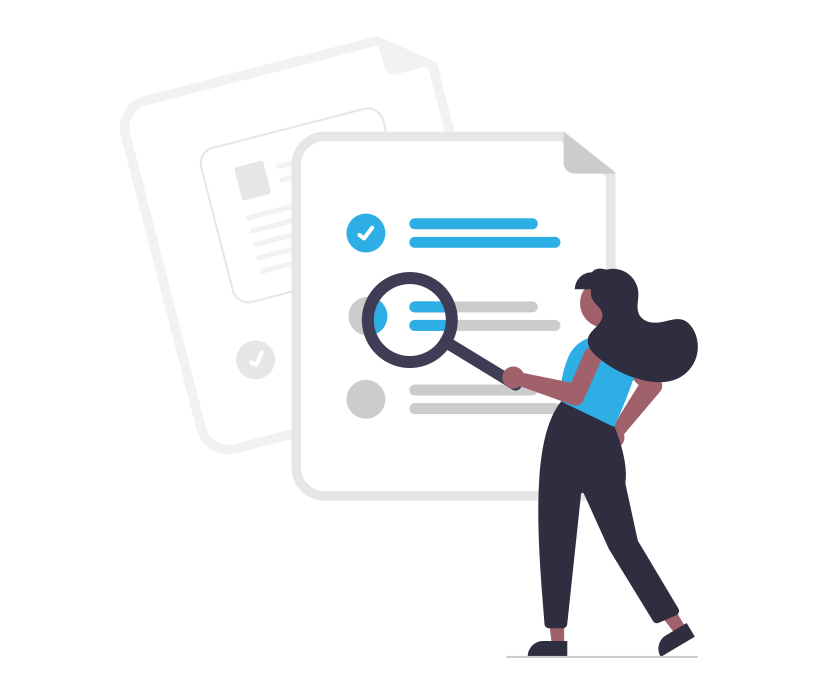
Systematic reviews are a reassessment of scholarly literature to facilitate decision making. This methodical approach of re-evaluating evidence was initially applied in healthcare, to set policies, create guidelines and answer medical questions.
Systematic reviews are large, complex projects and, depending on the purpose, they can be quite expensive to conduct. A team of researchers, data analysts and experts from various fields may collaborate to review and examine incredibly large numbers of research articles for evidence synthesis. Depending on the spectrum, systematic reviews often take at least 6 months, and sometimes upwards of 18 months to complete.
The main principles of transparency and reproducibility require a pragmatic approach in the organisation of the required research activities and detailed documentation of the outcomes. As a result, many software tools have been developed to help researchers with some of the tedious tasks required as part of the systematic review process.
hbspt.cta._relativeUrls=true;hbspt.cta.load(97439, 'ccc20645-09e2-4098-838f-091ed1bf1f4e', {"useNewLoader":"true","region":"na1"});
The first generation of these software tools were produced to accommodate and manage collaborations, but gradually developed to help with screening literature and reporting outcomes. Some of these software packages were initially designed for medical and healthcare studies and have specific protocols and customised steps integrated for various types of systematic reviews. However, some are designed for general processing, and by extending the application of the systematic review approach to other fields, they are being increasingly adopted and used in software engineering, health-related nutrition, agriculture, environmental science, social sciences and education.
Software tools
There are various free and subscription-based tools to help with conducting a systematic review. Many of these tools are designed to assist with the key stages of the process, including title and abstract screening, data synthesis, and critical appraisal. Some are designed to facilitate the entire process of review, including protocol development, reporting of the outcomes and help with fast project completion.
As time goes on, more functions are being integrated into such software tools. Technological advancement has allowed for more sophisticated and user-friendly features, including visual graphics for pattern recognition and linking multiple concepts. The idea is to digitalise the cumbersome parts of the process to increase efficiency, thus allowing researchers to focus their time and efforts on assessing the rigorousness and robustness of the research articles.
This article introduces commonly used systematic review tools that are relevant to food research and related disciplines, which can be used in a similar context to the process in healthcare disciplines.
These reviews are based on IFIS' internal research, thus are unbiased and not affiliated with the companies.
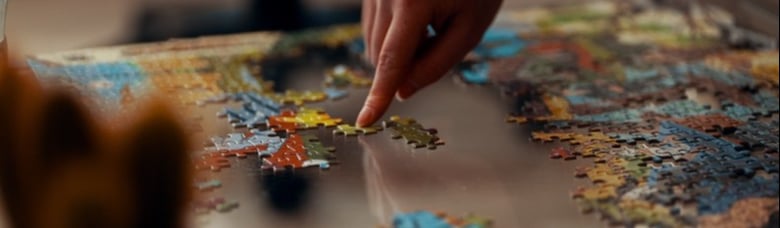
This online platform is a core component of the Cochrane toolkit, supporting parts of the systematic review process, including title/abstract and full-text screening, documentation, and reporting.
The Covidence platform enables collaboration of the entire systematic reviews team and is suitable for researchers and students at all levels of experience.
From a user perspective, the interface is intuitive, and the citation screening is directed step-by-step through a well-defined workflow. Imports and exports are straightforward, with easy export options to Excel and CVS.
Access is free for Cochrane authors (a single reviewer), and Cochrane provides a free trial to other researchers in healthcare. Universities can also subscribe on an institutional basis.
Rayyan is a free and open access web-based platform funded by the Qatar Foundation, a non-profit organisation supporting education and community development initiative . Rayyan is used to screen and code literature through a systematic review process.
Unlike Covidence, Rayyan does not follow a standard SR workflow and simply helps with citation screening. It is accessible through a mobile application with compatibility for offline screening. The web-based platform is known for its accessible user interface, with easy and clear export options.
Function comparison of 5 software tools to support the systematic review process
Eppi-reviewer.
EPPI-Reviewer is a web-based software programme developed by the Evidence for Policy and Practice Information and Co-ordinating Centre (EPPI) at the UCL Institute for Education, London .
It provides comprehensive functionalities for coding and screening. Users can create different levels of coding in a code set tool for clustering, screening, and administration of documents. EPPI-Reviewer allows direct search and import from PubMed. The import of search results from other databases is feasible in different formats. It stores, references, identifies and removes duplicates automatically. EPPI-Reviewer allows full-text screening, text mining, meta-analysis and the export of data into different types of reports.
There is no limit for concurrent use of the software and the number of articles being reviewed. Cochrane reviewers can access EPPI reviews using their Cochrane subscription details.
EPPI-Centre has other tools for facilitating the systematic review process, including coding guidelines and data management tools.
CADIMA is a free, online, open access review management tool, developed to facilitate research synthesis and structure documentation of the outcomes.
The Julius Institute and the Collaboration for Environmental Evidence established the software programme to support and guide users through the entire systematic review process, including protocol development, literature searching, study selection, critical appraisal, and documentation of the outcomes. The flexibility in choosing the steps also makes CADIMA suitable for conducting systematic mapping and rapid reviews.
CADIMA was initially developed for research questions in agriculture and environment but it is not limited to these, and as such, can be used for managing review processes in other disciplines. It enables users to export files and work offline.
The software allows for statistical analysis of the collated data using the R statistical software. Unlike EPPI-Reviewer, CADIMA does not have a built-in search engine to allow for searching in literature databases like PubMed.
DistillerSR
DistillerSR is an online software maintained by the Canadian company, Evidence Partners which specialises in literature review automation. DistillerSR provides a collaborative platform for every stage of literature review management. The framework is flexible and can accommodate literature reviews of different sizes. It is configurable to different data curation procedures, workflows and reporting standards. The platform integrates necessary features for screening, quality assessment, data extraction and reporting. The software uses Artificial Learning (AL)-enabled technologies in priority screening. It is to cut the screening process short by reranking the most relevant references nearer to the top. It can also use AL, as a second reviewer, in quality control checks of screened studies by human reviewers. DistillerSR is used to manage systematic reviews in various medical disciplines, surveillance, pharmacovigilance and public health reviews including food and nutrition topics. The software does not support statistical analyses. It provides configurable forms in standard formats for data extraction.
DistillerSR allows direct search and import of references from PubMed. It provides an add on feature called LitConnect which can be set to automatically import newly published references from data providers to keep reviews up to date during their progress.
The systematic review Toolbox is a web-based catalogue of various tools, including software packages which can assist with single or multiple tasks within the evidence synthesis process. Researchers can run a quick search or tailor a more sophisticated search by choosing their approach, budget, discipline, and preferred support features, to find the right tools for their research.
If you enjoyed this blog post, you may also be interested in our recently published blog post addressing the difference between a systematic review and a systematic literature review.
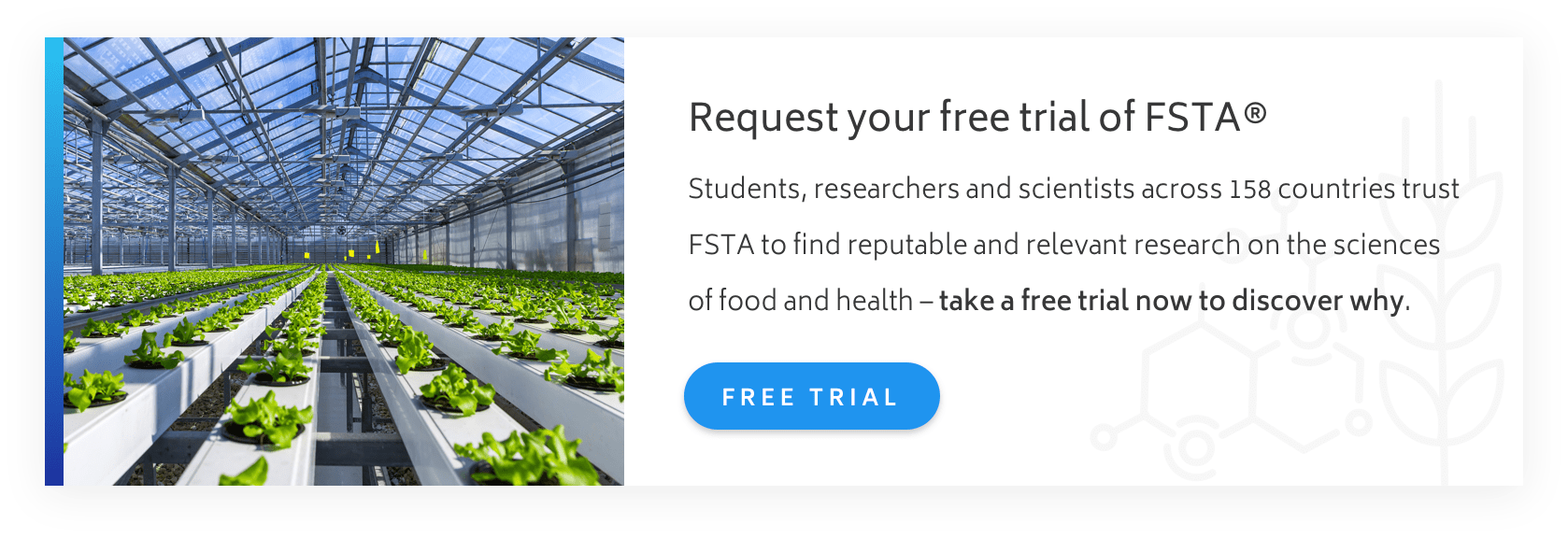
- FSTA - Food Science & Technology Abstracts
- IFIS Collections
- Resources Hub
- Diversity Statement
- Sustainability Commitment
- Company news
- Frequently Asked Questions
- Privacy Policy
- Terms of Use for IFIS Collections
Ground Floor, 115 Wharfedale Road, Winnersh Triangle, Wokingham, Berkshire RG41 5RB
Get in touch with IFIS
© International Food Information Service (IFIS Publishing) operating as IFIS – All Rights Reserved | Charity Reg. No. 1068176 | Limited Company No. 3507902 | Designed by Blend
Covidence website will be inaccessible as we upgrading our platform on Monday 23rd August at 10am AEST, / 2am CEST/1am BST (Sunday, 15th August 8pm EDT/5pm PDT)
The World's #1 Systematic Review Tool
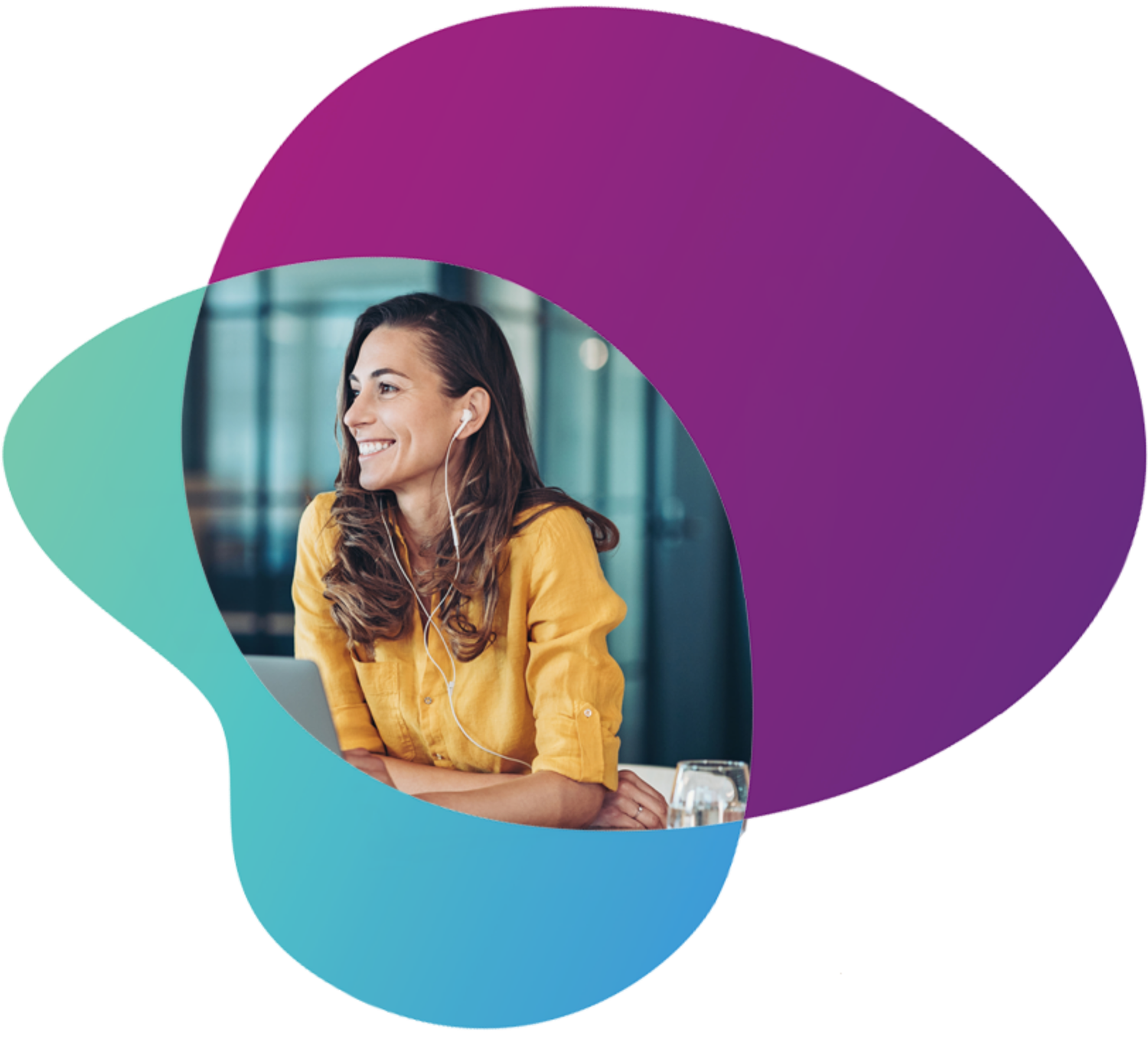
See your systematic reviews like never before
Faster reviews.
An average 35% reduction in time spent per review, saving an average of 71 hours per review.
Expert, online support
Easy to learn and use, with 24/7 support from product experts who are also seasoned reviewers!
Seamless collaboration
Enable the whole review team to collaborate from anywhere.
Suits all levels of experience and sectors
Suitable for reviewers in a variety of sectors including health, education, social science and many others.
Supporting the world's largest systematic review community
See how it works.
Step inside Covidence to see a more intuitive, streamlined way to manage systematic reviews.
Unlimited use for every organization
With no restrictions on reviews and users, Covidence gets out of the way so you can bring the best evidence to the world, more quickly.
Covidence is used by world-leading evidence organizations
Whether you’re an academic institution, a hospital or society, Covidence is working for organizations like yours right now.
See a list of organizations already using Covidence →
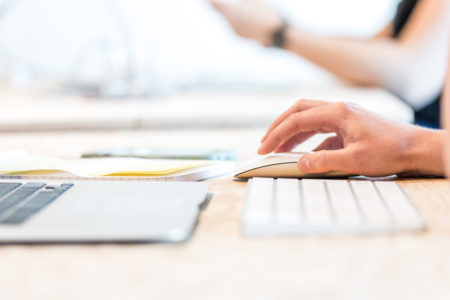
How Covidence has enabled living guidelines for Australians impacted by stroke
Clinical guidelines took 7 years to update prior to moving to a living evidence approach. Learn how Covidence streamlined workflows and created real time savings for the guidelines team.
University of Ottawa Drives Systematic Review Excellence Across Many Academic Disciplines
University of Ottawa
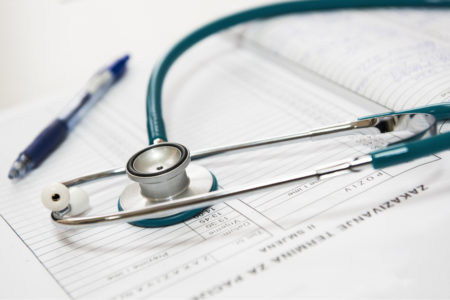
Top Ranked U.S. Teaching Hospital Delivers Effective Systematic Review Management
Top Ranked U.S. Teaching Hospital
See more Case Studies
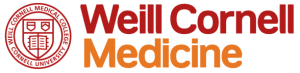
Better systematic review management
Head office, working for an institution or organisation.
Find out why over 350 of the world’s leading institutions are seeing a surge in publications since using Covidence!
Request a consultation with one of our team members and start empowering your researchers:
By using our site you consent to our use of cookies to measure and improve our site’s performance. Please see our Privacy Policy for more information.
- Open access
- Published: 08 June 2023
Guidance to best tools and practices for systematic reviews
- Kat Kolaski 1 ,
- Lynne Romeiser Logan 2 &
- John P. A. Ioannidis 3
Systematic Reviews volume 12 , Article number: 96 ( 2023 ) Cite this article
18k Accesses
14 Citations
76 Altmetric
Metrics details
Data continue to accumulate indicating that many systematic reviews are methodologically flawed, biased, redundant, or uninformative. Some improvements have occurred in recent years based on empirical methods research and standardization of appraisal tools; however, many authors do not routinely or consistently apply these updated methods. In addition, guideline developers, peer reviewers, and journal editors often disregard current methodological standards. Although extensively acknowledged and explored in the methodological literature, most clinicians seem unaware of these issues and may automatically accept evidence syntheses (and clinical practice guidelines based on their conclusions) as trustworthy.
A plethora of methods and tools are recommended for the development and evaluation of evidence syntheses. It is important to understand what these are intended to do (and cannot do) and how they can be utilized. Our objective is to distill this sprawling information into a format that is understandable and readily accessible to authors, peer reviewers, and editors. In doing so, we aim to promote appreciation and understanding of the demanding science of evidence synthesis among stakeholders. We focus on well-documented deficiencies in key components of evidence syntheses to elucidate the rationale for current standards. The constructs underlying the tools developed to assess reporting, risk of bias, and methodological quality of evidence syntheses are distinguished from those involved in determining overall certainty of a body of evidence. Another important distinction is made between those tools used by authors to develop their syntheses as opposed to those used to ultimately judge their work.
Exemplar methods and research practices are described, complemented by novel pragmatic strategies to improve evidence syntheses. The latter include preferred terminology and a scheme to characterize types of research evidence. We organize best practice resources in a Concise Guide that can be widely adopted and adapted for routine implementation by authors and journals. Appropriate, informed use of these is encouraged, but we caution against their superficial application and emphasize their endorsement does not substitute for in-depth methodological training. By highlighting best practices with their rationale, we hope this guidance will inspire further evolution of methods and tools that can advance the field.
Part 1. The state of evidence synthesis
Evidence syntheses are commonly regarded as the foundation of evidence-based medicine (EBM). They are widely accredited for providing reliable evidence and, as such, they have significantly influenced medical research and clinical practice. Despite their uptake throughout health care and ubiquity in contemporary medical literature, some important aspects of evidence syntheses are generally overlooked or not well recognized. Evidence syntheses are mostly retrospective exercises, they often depend on weak or irreparably flawed data, and they may use tools that have acknowledged or yet unrecognized limitations. They are complicated and time-consuming undertakings prone to bias and errors. Production of a good evidence synthesis requires careful preparation and high levels of organization in order to limit potential pitfalls [ 1 ]. Many authors do not recognize the complexity of such an endeavor and the many methodological challenges they may encounter. Failure to do so is likely to result in research and resource waste.
Given their potential impact on people’s lives, it is crucial for evidence syntheses to correctly report on the current knowledge base. In order to be perceived as trustworthy, reliable demonstration of the accuracy of evidence syntheses is equally imperative [ 2 ]. Concerns about the trustworthiness of evidence syntheses are not recent developments. From the early years when EBM first began to gain traction until recent times when thousands of systematic reviews are published monthly [ 3 ] the rigor of evidence syntheses has always varied. Many systematic reviews and meta-analyses had obvious deficiencies because original methods and processes had gaps, lacked precision, and/or were not widely known. The situation has improved with empirical research concerning which methods to use and standardization of appraisal tools. However, given the geometrical increase in the number of evidence syntheses being published, a relatively larger pool of unreliable evidence syntheses is being published today.
Publication of methodological studies that critically appraise the methods used in evidence syntheses is increasing at a fast pace. This reflects the availability of tools specifically developed for this purpose [ 4 , 5 , 6 ]. Yet many clinical specialties report that alarming numbers of evidence syntheses fail on these assessments. The syntheses identified report on a broad range of common conditions including, but not limited to, cancer, [ 7 ] chronic obstructive pulmonary disease, [ 8 ] osteoporosis, [ 9 ] stroke, [ 10 ] cerebral palsy, [ 11 ] chronic low back pain, [ 12 ] refractive error, [ 13 ] major depression, [ 14 ] pain, [ 15 ] and obesity [ 16 , 17 ]. The situation is even more concerning with regard to evidence syntheses included in clinical practice guidelines (CPGs) [ 18 , 19 , 20 ]. Astonishingly, in a sample of CPGs published in 2017–18, more than half did not apply even basic systematic methods in the evidence syntheses used to inform their recommendations [ 21 ].
These reports, while not widely acknowledged, suggest there are pervasive problems not limited to evidence syntheses that evaluate specific kinds of interventions or include primary research of a particular study design (eg, randomized versus non-randomized) [ 22 ]. Similar concerns about the reliability of evidence syntheses have been expressed by proponents of EBM in highly circulated medical journals [ 23 , 24 , 25 , 26 ]. These publications have also raised awareness about redundancy, inadequate input of statistical expertise, and deficient reporting. These issues plague primary research as well; however, there is heightened concern for the impact of these deficiencies given the critical role of evidence syntheses in policy and clinical decision-making.
Methods and guidance to produce a reliable evidence synthesis
Several international consortiums of EBM experts and national health care organizations currently provide detailed guidance (Table 1 ). They draw criteria from the reporting and methodological standards of currently recommended appraisal tools, and regularly review and update their methods to reflect new information and changing needs. In addition, they endorse the Grading of Recommendations Assessment, Development and Evaluation (GRADE) system for rating the overall quality of a body of evidence [ 27 ]. These groups typically certify or commission systematic reviews that are published in exclusive databases (eg, Cochrane, JBI) or are used to develop government or agency sponsored guidelines or health technology assessments (eg, National Institute for Health and Care Excellence [NICE], Scottish Intercollegiate Guidelines Network [SIGN], Agency for Healthcare Research and Quality [AHRQ]). They offer developers of evidence syntheses various levels of methodological advice, technical and administrative support, and editorial assistance. Use of specific protocols and checklists are required for development teams within these groups, but their online methodological resources are accessible to any potential author.
Notably, Cochrane is the largest single producer of evidence syntheses in biomedical research; however, these only account for 15% of the total [ 28 ]. The World Health Organization requires Cochrane standards be used to develop evidence syntheses that inform their CPGs [ 29 ]. Authors investigating questions of intervention effectiveness in syntheses developed for Cochrane follow the Methodological Expectations of Cochrane Intervention Reviews [ 30 ] and undergo multi-tiered peer review [ 31 , 32 ]. Several empirical evaluations have shown that Cochrane systematic reviews are of higher methodological quality compared with non-Cochrane reviews [ 4 , 7 , 9 , 11 , 14 , 32 , 33 , 34 , 35 ]. However, some of these assessments have biases: they may be conducted by Cochrane-affiliated authors, and they sometimes use scales and tools developed and used in the Cochrane environment and by its partners. In addition, evidence syntheses published in the Cochrane database are not subject to space or word restrictions, while non-Cochrane syntheses are often limited. As a result, information that may be relevant to the critical appraisal of non-Cochrane reviews is often removed or is relegated to online-only supplements that may not be readily or fully accessible [ 28 ].
Influences on the state of evidence synthesis
Many authors are familiar with the evidence syntheses produced by the leading EBM organizations but can be intimidated by the time and effort necessary to apply their standards. Instead of following their guidance, authors may employ methods that are discouraged or outdated 28]. Suboptimal methods described in in the literature may then be taken up by others. For example, the Newcastle–Ottawa Scale (NOS) is a commonly used tool for appraising non-randomized studies [ 36 ]. Many authors justify their selection of this tool with reference to a publication that describes the unreliability of the NOS and recommends against its use [ 37 ]. Obviously, the authors who cite this report for that purpose have not read it. Authors and peer reviewers have a responsibility to use reliable and accurate methods and not copycat previous citations or substandard work [ 38 , 39 ]. Similar cautions may potentially extend to automation tools. These have concentrated on evidence searching [ 40 ] and selection given how demanding it is for humans to maintain truly up-to-date evidence [ 2 , 41 ]. Cochrane has deployed machine learning to identify randomized controlled trials (RCTs) and studies related to COVID-19, [ 2 , 42 ] but such tools are not yet commonly used [ 43 ]. The routine integration of automation tools in the development of future evidence syntheses should not displace the interpretive part of the process.
Editorials about unreliable or misleading systematic reviews highlight several of the intertwining factors that may contribute to continued publication of unreliable evidence syntheses: shortcomings and inconsistencies of the peer review process, lack of endorsement of current standards on the part of journal editors, the incentive structure of academia, industry influences, publication bias, and the lure of “predatory” journals [ 44 , 45 , 46 , 47 , 48 ]. At this juncture, clarification of the extent to which each of these factors contribute remains speculative, but their impact is likely to be synergistic.
Over time, the generalized acceptance of the conclusions of systematic reviews as incontrovertible has affected trends in the dissemination and uptake of evidence. Reporting of the results of evidence syntheses and recommendations of CPGs has shifted beyond medical journals to press releases and news headlines and, more recently, to the realm of social media and influencers. The lay public and policy makers may depend on these outlets for interpreting evidence syntheses and CPGs. Unfortunately, communication to the general public often reflects intentional or non-intentional misrepresentation or “spin” of the research findings [ 49 , 50 , 51 , 52 ] News and social media outlets also tend to reduce conclusions on a body of evidence and recommendations for treatment to binary choices (eg, “do it” versus “don’t do it”) that may be assigned an actionable symbol (eg, red/green traffic lights, smiley/frowning face emoji).
Strategies for improvement
Many authors and peer reviewers are volunteer health care professionals or trainees who lack formal training in evidence synthesis [ 46 , 53 ]. Informing them about research methodology could increase the likelihood they will apply rigorous methods [ 25 , 33 , 45 ]. We tackle this challenge, from both a theoretical and a practical perspective, by offering guidance applicable to any specialty. It is based on recent methodological research that is extensively referenced to promote self-study. However, the information presented is not intended to be substitute for committed training in evidence synthesis methodology; instead, we hope to inspire our target audience to seek such training. We also hope to inform a broader audience of clinicians and guideline developers influenced by evidence syntheses. Notably, these communities often include the same members who serve in different capacities.
In the following sections, we highlight methodological concepts and practices that may be unfamiliar, problematic, confusing, or controversial. In Part 2, we consider various types of evidence syntheses and the types of research evidence summarized by them. In Part 3, we examine some widely used (and misused) tools for the critical appraisal of systematic reviews and reporting guidelines for evidence syntheses. In Part 4, we discuss how to meet methodological conduct standards applicable to key components of systematic reviews. In Part 5, we describe the merits and caveats of rating the overall certainty of a body of evidence. Finally, in Part 6, we summarize suggested terminology, methods, and tools for development and evaluation of evidence syntheses that reflect current best practices.
Part 2. Types of syntheses and research evidence
A good foundation for the development of evidence syntheses requires an appreciation of their various methodologies and the ability to correctly identify the types of research potentially available for inclusion in the synthesis.
Types of evidence syntheses
Systematic reviews have historically focused on the benefits and harms of interventions; over time, various types of systematic reviews have emerged to address the diverse information needs of clinicians, patients, and policy makers [ 54 ] Systematic reviews with traditional components have become defined by the different topics they assess (Table 2.1 ). In addition, other distinctive types of evidence syntheses have evolved, including overviews or umbrella reviews, scoping reviews, rapid reviews, and living reviews. The popularity of these has been increasing in recent years [ 55 , 56 , 57 , 58 ]. A summary of the development, methods, available guidance, and indications for these unique types of evidence syntheses is available in Additional File 2 A.
Both Cochrane [ 30 , 59 ] and JBI [ 60 ] provide methodologies for many types of evidence syntheses; they describe these with different terminology, but there is obvious overlap (Table 2.2 ). The majority of evidence syntheses published by Cochrane (96%) and JBI (62%) are categorized as intervention reviews. This reflects the earlier development and dissemination of their intervention review methodologies; these remain well-established [ 30 , 59 , 61 ] as both organizations continue to focus on topics related to treatment efficacy and harms. In contrast, intervention reviews represent only about half of the total published in the general medical literature, and several non-intervention review types contribute to a significant proportion of the other half.
Types of research evidence
There is consensus on the importance of using multiple study designs in evidence syntheses; at the same time, there is a lack of agreement on methods to identify included study designs. Authors of evidence syntheses may use various taxonomies and associated algorithms to guide selection and/or classification of study designs. These tools differentiate categories of research and apply labels to individual study designs (eg, RCT, cross-sectional). A familiar example is the Design Tree endorsed by the Centre for Evidence-Based Medicine [ 70 ]. Such tools may not be helpful to authors of evidence syntheses for multiple reasons.
Suboptimal levels of agreement and accuracy even among trained methodologists reflect challenges with the application of such tools [ 71 , 72 ]. Problematic distinctions or decision points (eg, experimental or observational, controlled or uncontrolled, prospective or retrospective) and design labels (eg, cohort, case control, uncontrolled trial) have been reported [ 71 ]. The variable application of ambiguous study design labels to non-randomized studies is common, making them especially prone to misclassification [ 73 ]. In addition, study labels do not denote the unique design features that make different types of non-randomized studies susceptible to different biases, including those related to how the data are obtained (eg, clinical trials, disease registries, wearable devices). Given this limitation, it is important to be aware that design labels preclude the accurate assignment of non-randomized studies to a “level of evidence” in traditional hierarchies [ 74 ].
These concerns suggest that available tools and nomenclature used to distinguish types of research evidence may not uniformly apply to biomedical research and non-health fields that utilize evidence syntheses (eg, education, economics) [ 75 , 76 ]. Moreover, primary research reports often do not describe study design or do so incompletely or inaccurately; thus, indexing in PubMed and other databases does not address the potential for misclassification [ 77 ]. Yet proper identification of research evidence has implications for several key components of evidence syntheses. For example, search strategies limited by index terms using design labels or study selection based on labels applied by the authors of primary studies may cause inconsistent or unjustified study inclusions and/or exclusions [ 77 ]. In addition, because risk of bias (RoB) tools consider attributes specific to certain types of studies and study design features, results of these assessments may be invalidated if an inappropriate tool is used. Appropriate classification of studies is also relevant for the selection of a suitable method of synthesis and interpretation of those results.
An alternative to these tools and nomenclature involves application of a few fundamental distinctions that encompass a wide range of research designs and contexts. While these distinctions are not novel, we integrate them into a practical scheme (see Fig. 1 ) designed to guide authors of evidence syntheses in the basic identification of research evidence. The initial distinction is between primary and secondary studies. Primary studies are then further distinguished by: 1) the type of data reported (qualitative or quantitative); and 2) two defining design features (group or single-case and randomized or non-randomized). The different types of studies and study designs represented in the scheme are described in detail in Additional File 2 B. It is important to conceptualize their methods as complementary as opposed to contrasting or hierarchical [ 78 ]; each offers advantages and disadvantages that determine their appropriateness for answering different kinds of research questions in an evidence synthesis.
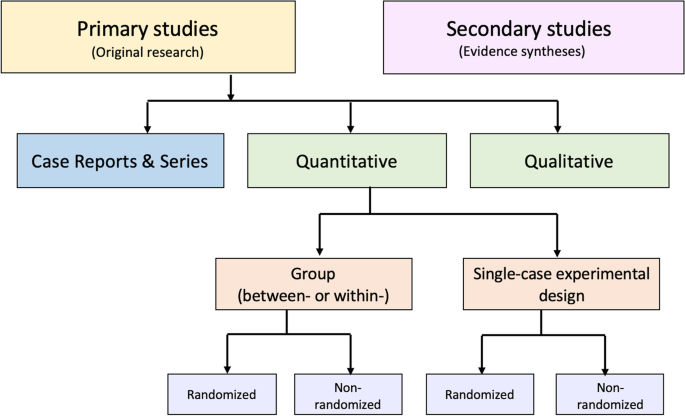
Distinguishing types of research evidence
Application of these basic distinctions may avoid some of the potential difficulties associated with study design labels and taxonomies. Nevertheless, debatable methodological issues are raised when certain types of research identified in this scheme are included in an evidence synthesis. We briefly highlight those associated with inclusion of non-randomized studies, case reports and series, and a combination of primary and secondary studies.
Non-randomized studies
When investigating an intervention’s effectiveness, it is important for authors to recognize the uncertainty of observed effects reported by studies with high RoB. Results of statistical analyses that include such studies need to be interpreted with caution in order to avoid misleading conclusions [ 74 ]. Review authors may consider excluding randomized studies with high RoB from meta-analyses. Non-randomized studies of intervention (NRSI) are affected by a greater potential range of biases and thus vary more than RCTs in their ability to estimate a causal effect [ 79 ]. If data from NRSI are synthesized in meta-analyses, it is helpful to separately report their summary estimates [ 6 , 74 ].
Nonetheless, certain design features of NRSI (eg, which parts of the study were prospectively designed) may help to distinguish stronger from weaker ones. Cochrane recommends that authors of a review including NRSI focus on relevant study design features when determining eligibility criteria instead of relying on non-informative study design labels [ 79 , 80 ] This process is facilitated by a study design feature checklist; guidance on using the checklist is included with developers’ description of the tool [ 73 , 74 ]. Authors collect information about these design features during data extraction and then consider it when making final study selection decisions and when performing RoB assessments of the included NRSI.
Case reports and case series
Correctly identified case reports and case series can contribute evidence not well captured by other designs [ 81 ]; in addition, some topics may be limited to a body of evidence that consists primarily of uncontrolled clinical observations. Murad and colleagues offer a framework for how to include case reports and series in an evidence synthesis [ 82 ]. Distinguishing between cohort studies and case series in these syntheses is important, especially for those that rely on evidence from NRSI. Additional data obtained from studies misclassified as case series can potentially increase the confidence in effect estimates. Mathes and Pieper provide authors of evidence syntheses with specific guidance on distinguishing between cohort studies and case series, but emphasize the increased workload involved [ 77 ].
Primary and secondary studies
Synthesis of combined evidence from primary and secondary studies may provide a broad perspective on the entirety of available literature on a topic. This is, in fact, the recommended strategy for scoping reviews that may include a variety of sources of evidence (eg, CPGs, popular media). However, except for scoping reviews, the synthesis of data from primary and secondary studies is discouraged unless there are strong reasons to justify doing so.
Combining primary and secondary sources of evidence is challenging for authors of other types of evidence syntheses for several reasons [ 83 ]. Assessments of RoB for primary and secondary studies are derived from conceptually different tools, thus obfuscating the ability to make an overall RoB assessment of a combination of these study types. In addition, authors who include primary and secondary studies must devise non-standardized methods for synthesis. Note this contrasts with well-established methods available for updating existing evidence syntheses with additional data from new primary studies [ 84 , 85 , 86 ]. However, a new review that synthesizes data from primary and secondary studies raises questions of validity and may unintentionally support a biased conclusion because no existing methodological guidance is currently available [ 87 ].
Recommendations
We suggest that journal editors require authors to identify which type of evidence synthesis they are submitting and reference the specific methodology used for its development. This will clarify the research question and methods for peer reviewers and potentially simplify the editorial process. Editors should announce this practice and include it in the instructions to authors. To decrease bias and apply correct methods, authors must also accurately identify the types of research evidence included in their syntheses.
Part 3. Conduct and reporting
The need to develop criteria to assess the rigor of systematic reviews was recognized soon after the EBM movement began to gain international traction [ 88 , 89 ]. Systematic reviews rapidly became popular, but many were very poorly conceived, conducted, and reported. These problems remain highly prevalent [ 23 ] despite development of guidelines and tools to standardize and improve the performance and reporting of evidence syntheses [ 22 , 28 ]. Table 3.1 provides some historical perspective on the evolution of tools developed specifically for the evaluation of systematic reviews, with or without meta-analysis.
These tools are often interchangeably invoked when referring to the “quality” of an evidence synthesis. However, quality is a vague term that is frequently misused and misunderstood; more precisely, these tools specify different standards for evidence syntheses. Methodological standards address how well a systematic review was designed and performed [ 5 ]. RoB assessments refer to systematic flaws or limitations in the design, conduct, or analysis of research that distort the findings of the review [ 4 ]. Reporting standards help systematic review authors describe the methodology they used and the results of their synthesis in sufficient detail [ 92 ]. It is essential to distinguish between these evaluations: a systematic review may be biased, it may fail to report sufficient information on essential features, or it may exhibit both problems; a thoroughly reported systematic evidence synthesis review may still be biased and flawed while an otherwise unbiased one may suffer from deficient documentation.
We direct attention to the currently recommended tools listed in Table 3.1 but concentrate on AMSTAR-2 (update of AMSTAR [A Measurement Tool to Assess Systematic Reviews]) and ROBIS (Risk of Bias in Systematic Reviews), which evaluate methodological quality and RoB, respectively. For comparison and completeness, we include PRISMA 2020 (update of the 2009 Preferred Reporting Items for Systematic Reviews of Meta-Analyses statement), which offers guidance on reporting standards. The exclusive focus on these three tools is by design; it addresses concerns related to the considerable variability in tools used for the evaluation of systematic reviews [ 28 , 88 , 96 , 97 ]. We highlight the underlying constructs these tools were designed to assess, then describe their components and applications. Their known (or potential) uptake and impact and limitations are also discussed.
Evaluation of conduct
Development.
AMSTAR [ 5 ] was in use for a decade prior to the 2017 publication of AMSTAR-2; both provide a broad evaluation of methodological quality of intervention systematic reviews, including flaws arising through poor conduct of the review [ 6 ]. ROBIS, published in 2016, was developed to specifically assess RoB introduced by the conduct of the review; it is applicable to systematic reviews of interventions and several other types of reviews [ 4 ]. Both tools reflect a shift to a domain-based approach as opposed to generic quality checklists. There are a few items unique to each tool; however, similarities between items have been demonstrated [ 98 , 99 ]. AMSTAR-2 and ROBIS are recommended for use by: 1) authors of overviews or umbrella reviews and CPGs to evaluate systematic reviews considered as evidence; 2) authors of methodological research studies to appraise included systematic reviews; and 3) peer reviewers for appraisal of submitted systematic review manuscripts. For authors, these tools may function as teaching aids and inform conduct of their review during its development.
Description
Systematic reviews that include randomized and/or non-randomized studies as evidence can be appraised with AMSTAR-2 and ROBIS. Other characteristics of AMSTAR-2 and ROBIS are summarized in Table 3.2 . Both tools define categories for an overall rating; however, neither tool is intended to generate a total score by simply calculating the number of responses satisfying criteria for individual items [ 4 , 6 ]. AMSTAR-2 focuses on the rigor of a review’s methods irrespective of the specific subject matter. ROBIS places emphasis on a review’s results section— this suggests it may be optimally applied by appraisers with some knowledge of the review’s topic as they may be better equipped to determine if certain procedures (or lack thereof) would impact the validity of a review’s findings [ 98 , 100 ]. Reliability studies show AMSTAR-2 overall confidence ratings strongly correlate with the overall RoB ratings in ROBIS [ 100 , 101 ].
Interrater reliability has been shown to be acceptable for AMSTAR-2 [ 6 , 11 , 102 ] and ROBIS [ 4 , 98 , 103 ] but neither tool has been shown to be superior in this regard [ 100 , 101 , 104 , 105 ]. Overall, variability in reliability for both tools has been reported across items, between pairs of raters, and between centers [ 6 , 100 , 101 , 104 ]. The effects of appraiser experience on the results of AMSTAR-2 and ROBIS require further evaluation [ 101 , 105 ]. Updates to both tools should address items shown to be prone to individual appraisers’ subjective biases and opinions [ 11 , 100 ]; this may involve modifications of the current domains and signaling questions as well as incorporation of methods to make an appraiser’s judgments more explicit. Future revisions of these tools may also consider the addition of standards for aspects of systematic review development currently lacking (eg, rating overall certainty of evidence, [ 99 ] methods for synthesis without meta-analysis [ 105 ]) and removal of items that assess aspects of reporting that are thoroughly evaluated by PRISMA 2020.
Application
A good understanding of what is required to satisfy the standards of AMSTAR-2 and ROBIS involves study of the accompanying guidance documents written by the tools’ developers; these contain detailed descriptions of each item’s standards. In addition, accurate appraisal of a systematic review with either tool requires training. Most experts recommend independent assessment by at least two appraisers with a process for resolving discrepancies as well as procedures to establish interrater reliability, such as pilot testing, a calibration phase or exercise, and development of predefined decision rules [ 35 , 99 , 100 , 101 , 103 , 104 , 106 ]. These methods may, to some extent, address the challenges associated with the diversity in methodological training, subject matter expertise, and experience using the tools that are likely to exist among appraisers.
The standards of AMSTAR, AMSTAR-2, and ROBIS have been used in many methodological studies and epidemiological investigations. However, the increased publication of overviews or umbrella reviews and CPGs has likely been a greater influence on the widening acceptance of these tools. Critical appraisal of the secondary studies considered evidence is essential to the trustworthiness of both the recommendations of CPGs and the conclusions of overviews. Currently both Cochrane [ 55 ] and JBI [ 107 ] recommend AMSTAR-2 and ROBIS in their guidance for authors of overviews or umbrella reviews. However, ROBIS and AMSTAR-2 were released in 2016 and 2017, respectively; thus, to date, limited data have been reported about the uptake of these tools or which of the two may be preferred [ 21 , 106 ]. Currently, in relation to CPGs, AMSTAR-2 appears to be overwhelmingly popular compared to ROBIS. A Google Scholar search of this topic (search terms “AMSTAR 2 AND clinical practice guidelines,” “ROBIS AND clinical practice guidelines” 13 May 2022) found 12,700 hits for AMSTAR-2 and 1,280 for ROBIS. The apparent greater appeal of AMSTAR-2 may relate to its longer track record given the original version of the tool was in use for 10 years prior to its update in 2017.
Barriers to the uptake of AMSTAR-2 and ROBIS include the real or perceived time and resources necessary to complete the items they include and appraisers’ confidence in their own ratings [ 104 ]. Reports from comparative studies available to date indicate that appraisers find AMSTAR-2 questions, responses, and guidance to be clearer and simpler compared with ROBIS [ 11 , 101 , 104 , 105 ]. This suggests that for appraisal of intervention systematic reviews, AMSTAR-2 may be a more practical tool than ROBIS, especially for novice appraisers [ 101 , 103 , 104 , 105 ]. The unique characteristics of each tool, as well as their potential advantages and disadvantages, should be taken into consideration when deciding which tool should be used for an appraisal of a systematic review. In addition, the choice of one or the other may depend on how the results of an appraisal will be used; for example, a peer reviewer’s appraisal of a single manuscript versus an appraisal of multiple systematic reviews in an overview or umbrella review, CPG, or systematic methodological study.
Authors of overviews and CPGs report results of AMSTAR-2 and ROBIS appraisals for each of the systematic reviews they include as evidence. Ideally, an independent judgment of their appraisals can be made by the end users of overviews and CPGs; however, most stakeholders, including clinicians, are unlikely to have a sophisticated understanding of these tools. Nevertheless, they should at least be aware that AMSTAR-2 and ROBIS ratings reported in overviews and CPGs may be inaccurate because the tools are not applied as intended by their developers. This can result from inadequate training of the overview or CPG authors who perform the appraisals, or to modifications of the appraisal tools imposed by them. The potential variability in overall confidence and RoB ratings highlights why appraisers applying these tools need to support their judgments with explicit documentation; this allows readers to judge for themselves whether they agree with the criteria used by appraisers [ 4 , 108 ]. When these judgments are explicit, the underlying rationale used when applying these tools can be assessed [ 109 ].
Theoretically, we would expect an association of AMSTAR-2 with improved methodological rigor and an association of ROBIS with lower RoB in recent systematic reviews compared to those published before 2017. To our knowledge, this has not yet been demonstrated; however, like reports about the actual uptake of these tools, time will tell. Additional data on user experience is also needed to further elucidate the practical challenges and methodological nuances encountered with the application of these tools. This information could potentially inform the creation of unifying criteria to guide and standardize the appraisal of evidence syntheses [ 109 ].
Evaluation of reporting
Complete reporting is essential for users to establish the trustworthiness and applicability of a systematic review’s findings. Efforts to standardize and improve the reporting of systematic reviews resulted in the 2009 publication of the PRISMA statement [ 92 ] with its accompanying explanation and elaboration document [ 110 ]. This guideline was designed to help authors prepare a complete and transparent report of their systematic review. In addition, adherence to PRISMA is often used to evaluate the thoroughness of reporting of published systematic reviews [ 111 ]. The updated version, PRISMA 2020 [ 93 ], and its guidance document [ 112 ] were published in 2021. Items on the original and updated versions of PRISMA are organized by the six basic review components they address (title, abstract, introduction, methods, results, discussion). The PRISMA 2020 update is a considerably expanded version of the original; it includes standards and examples for the 27 original and 13 additional reporting items that capture methodological advances and may enhance the replicability of reviews [ 113 ].
The original PRISMA statement fostered the development of various PRISMA extensions (Table 3.3 ). These include reporting guidance for scoping reviews and reviews of diagnostic test accuracy and for intervention reviews that report on the following: harms outcomes, equity issues, the effects of acupuncture, the results of network meta-analyses and analyses of individual participant data. Detailed reporting guidance for specific systematic review components (abstracts, protocols, literature searches) is also available.
Uptake and impact
The 2009 PRISMA standards [ 92 ] for reporting have been widely endorsed by authors, journals, and EBM-related organizations. We anticipate the same for PRISMA 2020 [ 93 ] given its co-publication in multiple high-impact journals. However, to date, there is a lack of strong evidence for an association between improved systematic review reporting and endorsement of PRISMA 2009 standards [ 43 , 111 ]. Most journals require a PRISMA checklist accompany submissions of systematic review manuscripts. However, the accuracy of information presented on these self-reported checklists is not necessarily verified. It remains unclear which strategies (eg, authors’ self-report of checklists, peer reviewer checks) might improve adherence to the PRISMA reporting standards; in addition, the feasibility of any potentially effective strategies must be taken into consideration given the structure and limitations of current research and publication practices [ 124 ].
Pitfalls and limitations of PRISMA, AMSTAR-2, and ROBIS
Misunderstanding of the roles of these tools and their misapplication may be widespread problems. PRISMA 2020 is a reporting guideline that is most beneficial if consulted when developing a review as opposed to merely completing a checklist when submitting to a journal; at that point, the review is finished, with good or bad methodological choices. However, PRISMA checklists evaluate how completely an element of review conduct was reported, but do not evaluate the caliber of conduct or performance of a review. Thus, review authors and readers should not think that a rigorous systematic review can be produced by simply following the PRISMA 2020 guidelines. Similarly, it is important to recognize that AMSTAR-2 and ROBIS are tools to evaluate the conduct of a review but do not substitute for conceptual methodological guidance. In addition, they are not intended to be simple checklists. In fact, they have the potential for misuse or abuse if applied as such; for example, by calculating a total score to make a judgment about a review’s overall confidence or RoB. Proper selection of a response for the individual items on AMSTAR-2 and ROBIS requires training or at least reference to their accompanying guidance documents.
Not surprisingly, it has been shown that compliance with the PRISMA checklist is not necessarily associated with satisfying the standards of ROBIS [ 125 ]. AMSTAR-2 and ROBIS were not available when PRISMA 2009 was developed; however, they were considered in the development of PRISMA 2020 [ 113 ]. Therefore, future studies may show a positive relationship between fulfillment of PRISMA 2020 standards for reporting and meeting the standards of tools evaluating methodological quality and RoB.
Choice of an appropriate tool for the evaluation of a systematic review first involves identification of the underlying construct to be assessed. For systematic reviews of interventions, recommended tools include AMSTAR-2 and ROBIS for appraisal of conduct and PRISMA 2020 for completeness of reporting. All three tools were developed rigorously and provide easily accessible and detailed user guidance, which is necessary for their proper application and interpretation. When considering a manuscript for publication, training in these tools can sensitize peer reviewers and editors to major issues that may affect the review’s trustworthiness and completeness of reporting. Judgment of the overall certainty of a body of evidence and formulation of recommendations rely, in part, on AMSTAR-2 or ROBIS appraisals of systematic reviews. Therefore, training on the application of these tools is essential for authors of overviews and developers of CPGs. Peer reviewers and editors considering an overview or CPG for publication must hold their authors to a high standard of transparency regarding both the conduct and reporting of these appraisals.
Part 4. Meeting conduct standards
Many authors, peer reviewers, and editors erroneously equate fulfillment of the items on the PRISMA checklist with superior methodological rigor. For direction on methodology, we refer them to available resources that provide comprehensive conceptual guidance [ 59 , 60 ] as well as primers with basic step-by-step instructions [ 1 , 126 , 127 ]. This section is intended to complement study of such resources by facilitating use of AMSTAR-2 and ROBIS, tools specifically developed to evaluate methodological rigor of systematic reviews. These tools are widely accepted by methodologists; however, in the general medical literature, they are not uniformly selected for the critical appraisal of systematic reviews [ 88 , 96 ].
To enable their uptake, Table 4.1 links review components to the corresponding appraisal tool items. Expectations of AMSTAR-2 and ROBIS are concisely stated, and reasoning provided.
Issues involved in meeting the standards for seven review components (identified in bold in Table 4.1 ) are addressed in detail. These were chosen for elaboration for one (or both) of two reasons: 1) the component has been identified as potentially problematic for systematic review authors based on consistent reports of their frequent AMSTAR-2 or ROBIS deficiencies [ 9 , 11 , 15 , 88 , 128 , 129 ]; and/or 2) the review component is judged by standards of an AMSTAR-2 “critical” domain. These have the greatest implications for how a systematic review will be appraised: if standards for any one of these critical domains are not met, the review is rated as having “critically low confidence.”
Research question
Specific and unambiguous research questions may have more value for reviews that deal with hypothesis testing. Mnemonics for the various elements of research questions are suggested by JBI and Cochrane (Table 2.1 ). These prompt authors to consider the specialized methods involved for developing different types of systematic reviews; however, while inclusion of the suggested elements makes a review compliant with a particular review’s methods, it does not necessarily make a research question appropriate. Table 4.2 lists acronyms that may aid in developing the research question. They include overlapping concepts of importance in this time of proliferating reviews of uncertain value [ 130 ]. If these issues are not prospectively contemplated, systematic review authors may establish an overly broad scope, or develop runaway scope allowing them to stray from predefined choices relating to key comparisons and outcomes.
Once a research question is established, searching on registry sites and databases for existing systematic reviews addressing the same or a similar topic is necessary in order to avoid contributing to research waste [ 131 ]. Repeating an existing systematic review must be justified, for example, if previous reviews are out of date or methodologically flawed. A full discussion on replication of intervention systematic reviews, including a consensus checklist, can be found in the work of Tugwell and colleagues [ 84 ].
Protocol development is considered a core component of systematic reviews [ 125 , 126 , 132 ]. Review protocols may allow researchers to plan and anticipate potential issues, assess validity of methods, prevent arbitrary decision-making, and minimize bias that can be introduced by the conduct of the review. Registration of a protocol that allows public access promotes transparency of the systematic review’s methods and processes and reduces the potential for duplication [ 132 ]. Thinking early and carefully about all the steps of a systematic review is pragmatic and logical and may mitigate the influence of the authors’ prior knowledge of the evidence [ 133 ]. In addition, the protocol stage is when the scope of the review can be carefully considered by authors, reviewers, and editors; this may help to avoid production of overly ambitious reviews that include excessive numbers of comparisons and outcomes or are undisciplined in their study selection.
An association with attainment of AMSTAR standards in systematic reviews with published prospective protocols has been reported [ 134 ]. However, completeness of reporting does not seem to be different in reviews with a protocol compared to those without one [ 135 ]. PRISMA-P [ 116 ] and its accompanying elaboration and explanation document [ 136 ] can be used to guide and assess the reporting of protocols. A final version of the review should fully describe any protocol deviations. Peer reviewers may compare the submitted manuscript with any available pre-registered protocol; this is required if AMSTAR-2 or ROBIS are used for critical appraisal.
There are multiple options for the recording of protocols (Table 4.3 ). Some journals will peer review and publish protocols. In addition, many online sites offer date-stamped and publicly accessible protocol registration. Some of these are exclusively for protocols of evidence syntheses; others are less restrictive and offer researchers the capacity for data storage, sharing, and other workflow features. These sites document protocol details to varying extents and have different requirements [ 137 ]. The most popular site for systematic reviews, the International Prospective Register of Systematic Reviews (PROSPERO), for example, only registers reviews that report on an outcome with direct relevance to human health. The PROSPERO record documents protocols for all types of reviews except literature and scoping reviews. Of note, PROSPERO requires authors register their review protocols prior to any data extraction [ 133 , 138 ]. The electronic records of most of these registry sites allow authors to update their protocols and facilitate transparent tracking of protocol changes, which are not unexpected during the progress of the review [ 139 ].
Study design inclusion
For most systematic reviews, broad inclusion of study designs is recommended [ 126 ]. This may allow comparison of results between contrasting study design types [ 126 ]. Certain study designs may be considered preferable depending on the type of review and nature of the research question. However, prevailing stereotypes about what each study design does best may not be accurate. For example, in systematic reviews of interventions, randomized designs are typically thought to answer highly specific questions while non-randomized designs often are expected to reveal greater information about harms or real-word evidence [ 126 , 140 , 141 ]. This may be a false distinction; randomized trials may be pragmatic [ 142 ], they may offer important (and more unbiased) information on harms [ 143 ], and data from non-randomized trials may not necessarily be more real-world-oriented [ 144 ].
Moreover, there may not be any available evidence reported by RCTs for certain research questions; in some cases, there may not be any RCTs or NRSI. When the available evidence is limited to case reports and case series, it is not possible to test hypotheses nor provide descriptive estimates or associations; however, a systematic review of these studies can still offer important insights [ 81 , 145 ]. When authors anticipate that limited evidence of any kind may be available to inform their research questions, a scoping review can be considered. Alternatively, decisions regarding inclusion of indirect as opposed to direct evidence can be addressed during protocol development [ 146 ]. Including indirect evidence at an early stage of intervention systematic review development allows authors to decide if such studies offer any additional and/or different understanding of treatment effects for their population or comparison of interest. Issues of indirectness of included studies are accounted for later in the process, during determination of the overall certainty of evidence (see Part 5 for details).
Evidence search
Both AMSTAR-2 and ROBIS require systematic and comprehensive searches for evidence. This is essential for any systematic review. Both tools discourage search restrictions based on language and publication source. Given increasing globalism in health care, the practice of including English-only literature should be avoided [ 126 ]. There are many examples in which language bias (different results in studies published in different languages) has been documented [ 147 , 148 ]. This does not mean that all literature, in all languages, is equally trustworthy [ 148 ]; however, the only way to formally probe for the potential of such biases is to consider all languages in the initial search. The gray literature and a search of trials may also reveal important details about topics that would otherwise be missed [ 149 , 150 , 151 ]. Again, inclusiveness will allow review authors to investigate whether results differ in gray literature and trials [ 41 , 151 , 152 , 153 ].
Authors should make every attempt to complete their review within one year as that is the likely viable life of a search. (1) If that is not possible, the search should be updated close to the time of completion [ 154 ]. Different research topics may warrant less of a delay, for example, in rapidly changing fields (as in the case of the COVID-19 pandemic), even one month may radically change the available evidence.
Excluded studies
AMSTAR-2 requires authors to provide references for any studies excluded at the full text phase of study selection along with reasons for exclusion; this allows readers to feel confident that all relevant literature has been considered for inclusion and that exclusions are defensible.
Risk of bias assessment of included studies
The design of the studies included in a systematic review (eg, RCT, cohort, case series) should not be equated with appraisal of its RoB. To meet AMSTAR-2 and ROBIS standards, systematic review authors must examine RoB issues specific to the design of each primary study they include as evidence. It is unlikely that a single RoB appraisal tool will be suitable for all research designs. In addition to tools for randomized and non-randomized studies, specific tools are available for evaluation of RoB in case reports and case series [ 82 ] and single-case experimental designs [ 155 , 156 ]. Note the RoB tools selected must meet the standards of the appraisal tool used to judge the conduct of the review. For example, AMSTAR-2 identifies four sources of bias specific to RCTs and NRSI that must be addressed by the RoB tool(s) chosen by the review authors. The Cochrane RoB-2 [ 157 ] tool for RCTs and ROBINS-I [ 158 ] for NRSI for RoB assessment meet the AMSTAR-2 standards. Appraisers on the review team should not modify any RoB tool without complete transparency and acknowledgment that they have invalidated the interpretation of the tool as intended by its developers [ 159 ]. Conduct of RoB assessments is not addressed AMSTAR-2; to meet ROBIS standards, two independent reviewers should complete RoB assessments of included primary studies.
Implications of the RoB assessments must be explicitly discussed and considered in the conclusions of the review. Discussion of the overall RoB of included studies may consider the weight of the studies at high RoB, the importance of the sources of bias in the studies being summarized, and if their importance differs in relationship to the outcomes reported. If a meta-analysis is performed, serious concerns for RoB of individual studies should be accounted for in these results as well. If the results of the meta-analysis for a specific outcome change when studies at high RoB are excluded, readers will have a more accurate understanding of this body of evidence. However, while investigating the potential impact of specific biases is a useful exercise, it is important to avoid over-interpretation, especially when there are sparse data.
Synthesis methods for quantitative data
Syntheses of quantitative data reported by primary studies are broadly categorized as one of two types: meta-analysis, and synthesis without meta-analysis (Table 4.4 ). Before deciding on one of these methods, authors should seek methodological advice about whether reported data can be transformed or used in other ways to provide a consistent effect measure across studies [ 160 , 161 ].
Meta-analysis
Systematic reviews that employ meta-analysis should not be referred to simply as “meta-analyses.” The term meta-analysis strictly refers to a specific statistical technique used when study effect estimates and their variances are available, yielding a quantitative summary of results. In general, methods for meta-analysis involve use of a weighted average of effect estimates from two or more studies. If considered carefully, meta-analysis increases the precision of the estimated magnitude of effect and can offer useful insights about heterogeneity and estimates of effects. We refer to standard references for a thorough introduction and formal training [ 165 , 166 , 167 ].
There are three common approaches to meta-analysis in current health care–related systematic reviews (Table 4.4 ). Aggregate meta-analyses is the most familiar to authors of evidence syntheses and their end users. This standard meta-analysis combines data on effect estimates reported by studies that investigate similar research questions involving direct comparisons of an intervention and comparator. Results of these analyses provide a single summary intervention effect estimate. If the included studies in a systematic review measure an outcome differently, their reported results may be transformed to make them comparable [ 161 ]. Forest plots visually present essential information about the individual studies and the overall pooled analysis (see Additional File 4 for details).
Less familiar and more challenging meta-analytical approaches used in secondary research include individual participant data (IPD) and network meta-analyses (NMA); PRISMA extensions provide reporting guidelines for both [ 117 , 118 ]. In IPD, the raw data on each participant from each eligible study are re-analyzed as opposed to the study-level data analyzed in aggregate data meta-analyses [ 168 ]. This may offer advantages, including the potential for limiting concerns about bias and allowing more robust analyses [ 163 ]. As suggested by the description in Table 4.4 , NMA is a complex statistical approach. It combines aggregate data [ 169 ] or IPD [ 170 ] for effect estimates from direct and indirect comparisons reported in two or more studies of three or more interventions. This makes it a potentially powerful statistical tool; while multiple interventions are typically available to treat a condition, few have been evaluated in head-to-head trials [ 171 ]. Both IPD and NMA facilitate a broader scope, and potentially provide more reliable and/or detailed results; however, compared with standard aggregate data meta-analyses, their methods are more complicated, time-consuming, and resource-intensive, and they have their own biases, so one needs sufficient funding, technical expertise, and preparation to employ them successfully [ 41 , 172 , 173 ].
Several items in AMSTAR-2 and ROBIS address meta-analysis; thus, understanding the strengths, weaknesses, assumptions, and limitations of methods for meta-analyses is important. According to the standards of both tools, plans for a meta-analysis must be addressed in the review protocol, including reasoning, description of the type of quantitative data to be synthesized, and the methods planned for combining the data. This should not consist of stock statements describing conventional meta-analysis techniques; rather, authors are expected to anticipate issues specific to their research questions. Concern for the lack of training in meta-analysis methods among systematic review authors cannot be overstated. For those with training, the use of popular software (eg, RevMan [ 174 ], MetaXL [ 175 ], JBI SUMARI [ 176 ]) may facilitate exploration of these methods; however, such programs cannot substitute for the accurate interpretation of the results of meta-analyses, especially for more complex meta-analytical approaches.
Synthesis without meta-analysis
There are varied reasons a meta-analysis may not be appropriate or desirable [ 160 , 161 ]. Syntheses that informally use statistical methods other than meta-analysis are variably referred to as descriptive, narrative, or qualitative syntheses or summaries; these terms are also applied to syntheses that make no attempt to statistically combine data from individual studies. However, use of such imprecise terminology is discouraged; in order to fully explore the results of any type of synthesis, some narration or description is needed to supplement the data visually presented in tabular or graphic forms [ 63 , 177 ]. In addition, the term “qualitative synthesis” is easily confused with a synthesis of qualitative data in a qualitative or mixed methods review. “Synthesis without meta-analysis” is currently the preferred description of other ways to combine quantitative data from two or more studies. Use of this specific terminology when referring to these types of syntheses also implies the application of formal methods (Table 4.4 ).
Methods for syntheses without meta-analysis involve structured presentations of the data in any tables and plots. In comparison to narrative descriptions of each study, these are designed to more effectively and transparently show patterns and convey detailed information about the data; they also allow informal exploration of heterogeneity [ 178 ]. In addition, acceptable quantitative statistical methods (Table 4.4 ) are formally applied; however, it is important to recognize these methods have significant limitations for the interpretation of the effectiveness of an intervention [ 160 ]. Nevertheless, when meta-analysis is not possible, the application of these methods is less prone to bias compared with an unstructured narrative description of included studies [ 178 , 179 ].
Vote counting is commonly used in systematic reviews and involves a tally of studies reporting results that meet some threshold of importance applied by review authors. Until recently, it has not typically been identified as a method for synthesis without meta-analysis. Guidance on an acceptable vote counting method based on direction of effect is currently available [ 160 ] and should be used instead of narrative descriptions of such results (eg, “more than half the studies showed improvement”; “only a few studies reported adverse effects”; “7 out of 10 studies favored the intervention”). Unacceptable methods include vote counting by statistical significance or magnitude of effect or some subjective rule applied by the authors.
AMSTAR-2 and ROBIS standards do not explicitly address conduct of syntheses without meta-analysis, although AMSTAR-2 items 13 and 14 might be considered relevant. Guidance for the complete reporting of syntheses without meta-analysis for systematic reviews of interventions is available in the Synthesis without Meta-analysis (SWiM) guideline [ 180 ] and methodological guidance is available in the Cochrane Handbook [ 160 , 181 ].
Familiarity with AMSTAR-2 and ROBIS makes sense for authors of systematic reviews as these appraisal tools will be used to judge their work; however, training is necessary for authors to truly appreciate and apply methodological rigor. Moreover, judgment of the potential contribution of a systematic review to the current knowledge base goes beyond meeting the standards of AMSTAR-2 and ROBIS. These tools do not explicitly address some crucial concepts involved in the development of a systematic review; this further emphasizes the need for author training.
We recommend that systematic review authors incorporate specific practices or exercises when formulating a research question at the protocol stage, These should be designed to raise the review team’s awareness of how to prevent research and resource waste [ 84 , 130 ] and to stimulate careful contemplation of the scope of the review [ 30 ]. Authors’ training should also focus on justifiably choosing a formal method for the synthesis of quantitative and/or qualitative data from primary research; both types of data require specific expertise. For typical reviews that involve syntheses of quantitative data, statistical expertise is necessary, initially for decisions about appropriate methods, [ 160 , 161 ] and then to inform any meta-analyses [ 167 ] or other statistical methods applied [ 160 ].
Part 5. Rating overall certainty of evidence
Report of an overall certainty of evidence assessment in a systematic review is an important new reporting standard of the updated PRISMA 2020 guidelines [ 93 ]. Systematic review authors are well acquainted with assessing RoB in individual primary studies, but much less familiar with assessment of overall certainty across an entire body of evidence. Yet a reliable way to evaluate this broader concept is now recognized as a vital part of interpreting the evidence.
Historical systems for rating evidence are based on study design and usually involve hierarchical levels or classes of evidence that use numbers and/or letters to designate the level/class. These systems were endorsed by various EBM-related organizations. Professional societies and regulatory groups then widely adopted them, often with modifications for application to the available primary research base in specific clinical areas. In 2002, a report issued by the AHRQ identified 40 systems to rate quality of a body of evidence [ 182 ]. A critical appraisal of systems used by prominent health care organizations published in 2004 revealed limitations in sensibility, reproducibility, applicability to different questions, and usability to different end users [ 183 ]. Persistent use of hierarchical rating schemes to describe overall quality continues to complicate the interpretation of evidence. This is indicated by recent reports of poor interpretability of systematic review results by readers [ 184 , 185 , 186 ] and misleading interpretations of the evidence related to the “spin” systematic review authors may put on their conclusions [ 50 , 187 ].
Recognition of the shortcomings of hierarchical rating systems raised concerns that misleading clinical recommendations could result even if based on a rigorous systematic review. In addition, the number and variability of these systems were considered obstacles to quick and accurate interpretations of the evidence by clinicians, patients, and policymakers [ 183 ]. These issues contributed to the development of the GRADE approach. An international working group, that continues to actively evaluate and refine it, first introduced GRADE in 2004 [ 188 ]. Currently more than 110 organizations from 19 countries around the world have endorsed or are using GRADE [ 189 ].
GRADE approach to rating overall certainty
GRADE offers a consistent and sensible approach for two separate processes: rating the overall certainty of a body of evidence and the strength of recommendations. The former is the expected conclusion of a systematic review, while the latter is pertinent to the development of CPGs. As such, GRADE provides a mechanism to bridge the gap from evidence synthesis to application of the evidence for informed clinical decision-making [ 27 , 190 ]. We briefly examine the GRADE approach but only as it applies to rating overall certainty of evidence in systematic reviews.
In GRADE, use of “certainty” of a body of evidence is preferred over the term “quality.” [ 191 ] Certainty refers to the level of confidence systematic review authors have that, for each outcome, an effect estimate represents the true effect. The GRADE approach to rating confidence in estimates begins with identifying the study type (RCT or NRSI) and then systematically considers criteria to rate the certainty of evidence up or down (Table 5.1 ).
This process results in assignment of one of the four GRADE certainty ratings to each outcome; these are clearly conveyed with the use of basic interpretation symbols (Table 5.2 ) [ 192 ]. Notably, when multiple outcomes are reported in a systematic review, each outcome is assigned a unique certainty rating; thus different levels of certainty may exist in the body of evidence being examined.
GRADE’s developers acknowledge some subjectivity is involved in this process [ 193 ]. In addition, they emphasize that both the criteria for rating evidence up and down (Table 5.1 ) as well as the four overall certainty ratings (Table 5.2 ) reflect a continuum as opposed to discrete categories [ 194 ]. Consequently, deciding whether a study falls above or below the threshold for rating up or down may not be straightforward, and preliminary overall certainty ratings may be intermediate (eg, between low and moderate). Thus, the proper application of GRADE requires systematic review authors to take an overall view of the body of evidence and explicitly describe the rationale for their final ratings.
Advantages of GRADE
Outcomes important to the individuals who experience the problem of interest maintain a prominent role throughout the GRADE process [ 191 ]. These outcomes must inform the research questions (eg, PICO [population, intervention, comparator, outcome]) that are specified a priori in a systematic review protocol. Evidence for these outcomes is then investigated and each critical or important outcome is ultimately assigned a certainty of evidence as the end point of the review. Notably, limitations of the included studies have an impact at the outcome level. Ultimately, the certainty ratings for each outcome reported in a systematic review are considered by guideline panels. They use a different process to formulate recommendations that involves assessment of the evidence across outcomes [ 201 ]. It is beyond our scope to describe the GRADE process for formulating recommendations; however, it is critical to understand how these two outcome-centric concepts of certainty of evidence in the GRADE framework are related and distinguished. An in-depth illustration using examples from recently published evidence syntheses and CPGs is provided in Additional File 5 A (Table AF5A-1).
The GRADE approach is applicable irrespective of whether the certainty of the primary research evidence is high or very low; in some circumstances, indirect evidence of higher certainty may be considered if direct evidence is unavailable or of low certainty [ 27 ]. In fact, most interventions and outcomes in medicine have low or very low certainty of evidence based on GRADE and there seems to be no major improvement over time [ 202 , 203 ]. This is still a very important (even if sobering) realization for calibrating our understanding of medical evidence. A major appeal of the GRADE approach is that it offers a common framework that enables authors of evidence syntheses to make complex judgments about evidence certainty and to convey these with unambiguous terminology. This prevents some common mistakes made by review authors, including overstating results (or under-reporting harms) [ 187 ] and making recommendations for treatment. This is illustrated in Table AF5A-2 (Additional File 5 A), which compares the concluding statements made about overall certainty in a systematic review with and without application of the GRADE approach.
Theoretically, application of GRADE should improve consistency of judgments about certainty of evidence, both between authors and across systematic reviews. In one empirical evaluation conducted by the GRADE Working Group, interrater reliability of two individual raters assessing certainty of the evidence for a specific outcome increased from ~ 0.3 without using GRADE to ~ 0.7 by using GRADE [ 204 ]. However, others report variable agreement among those experienced in GRADE assessments of evidence certainty [ 190 ]. Like any other tool, GRADE requires training in order to be properly applied. The intricacies of the GRADE approach and the necessary subjectivity involved suggest that improving agreement may require strict rules for its application; alternatively, use of general guidance and consensus among review authors may result in less consistency but provide important information for the end user [ 190 ].
GRADE caveats
Simply invoking “the GRADE approach” does not automatically ensure GRADE methods were employed by authors of a systematic review (or developers of a CPG). Table 5.3 lists the criteria the GRADE working group has established for this purpose. These criteria highlight the specific terminology and methods that apply to rating the certainty of evidence for outcomes reported in a systematic review [ 191 ], which is different from rating overall certainty across outcomes considered in the formulation of recommendations [ 205 ]. Modifications of standard GRADE methods and terminology are discouraged as these may detract from GRADE’s objectives to minimize conceptual confusion and maximize clear communication [ 206 ].
Nevertheless, GRADE is prone to misapplications [ 207 , 208 ], which can distort a systematic review’s conclusions about the certainty of evidence. Systematic review authors without proper GRADE training are likely to misinterpret the terms “quality” and “grade” and to misunderstand the constructs assessed by GRADE versus other appraisal tools. For example, review authors may reference the standard GRADE certainty ratings (Table 5.2 ) to describe evidence for their outcome(s) of interest. However, these ratings are invalidated if authors omit or inadequately perform RoB evaluations of each included primary study. Such deficiencies in RoB assessments are unacceptable but not uncommon, as reported in methodological studies of systematic reviews and overviews [ 104 , 186 , 209 , 210 ]. GRADE ratings are also invalidated if review authors do not formally address and report on the other criteria (Table 5.1 ) necessary for a GRADE certainty rating.
Other caveats pertain to application of a GRADE certainty of evidence rating in various types of evidence syntheses. Current adaptations of GRADE are described in Additional File 5 B and included on Table 6.3 , which is introduced in the next section.
The expected culmination of a systematic review should be a rating of overall certainty of a body of evidence for each outcome reported. The GRADE approach is recommended for making these judgments for outcomes reported in systematic reviews of interventions and can be adapted for other types of reviews. This represents the initial step in the process of making recommendations based on evidence syntheses. Peer reviewers should ensure authors meet the minimal criteria for supporting the GRADE approach when reviewing any evidence synthesis that reports certainty ratings derived using GRADE. Authors and peer reviewers of evidence syntheses unfamiliar with GRADE are encouraged to seek formal training and take advantage of the resources available on the GRADE website [ 211 , 212 ].
Part 6. Concise Guide to best practices
Accumulating data in recent years suggest that many evidence syntheses (with or without meta-analysis) are not reliable. This relates in part to the fact that their authors, who are often clinicians, can be overwhelmed by the plethora of ways to evaluate evidence. They tend to resort to familiar but often inadequate, inappropriate, or obsolete methods and tools and, as a result, produce unreliable reviews. These manuscripts may not be recognized as such by peer reviewers and journal editors who may disregard current standards. When such a systematic review is published or included in a CPG, clinicians and stakeholders tend to believe that it is trustworthy. A vicious cycle in which inadequate methodology is rewarded and potentially misleading conclusions are accepted is thus supported. There is no quick or easy way to break this cycle; however, increasing awareness of best practices among all these stakeholder groups, who often have minimal (if any) training in methodology, may begin to mitigate it. This is the rationale for inclusion of Parts 2 through 5 in this guidance document. These sections present core concepts and important methodological developments that inform current standards and recommendations. We conclude by taking a direct and practical approach.
Inconsistent and imprecise terminology used in the context of development and evaluation of evidence syntheses is problematic for authors, peer reviewers and editors, and may lead to the application of inappropriate methods and tools. In response, we endorse use of the basic terms (Table 6.1 ) defined in the PRISMA 2020 statement [ 93 ]. In addition, we have identified several problematic expressions and nomenclature. In Table 6.2 , we compile suggestions for preferred terms less likely to be misinterpreted.
We also propose a Concise Guide (Table 6.3 ) that summarizes the methods and tools recommended for the development and evaluation of nine types of evidence syntheses. Suggestions for specific tools are based on the rigor of their development as well as the availability of detailed guidance from their developers to ensure their proper application. The formatting of the Concise Guide addresses a well-known source of confusion by clearly distinguishing the underlying methodological constructs that these tools were designed to assess. Important clarifications and explanations follow in the guide’s footnotes; associated websites, if available, are listed in Additional File 6 .
To encourage uptake of best practices, journal editors may consider adopting or adapting the Concise Guide in their instructions to authors and peer reviewers of evidence syntheses. Given the evolving nature of evidence synthesis methodology, the suggested methods and tools are likely to require regular updates. Authors of evidence syntheses should monitor the literature to ensure they are employing current methods and tools. Some types of evidence syntheses (eg, rapid, economic, methodological) are not included in the Concise Guide; for these, authors are advised to obtain recommendations for acceptable methods by consulting with their target journal.
We encourage the appropriate and informed use of the methods and tools discussed throughout this commentary and summarized in the Concise Guide (Table 6.3 ). However, we caution against their application in a perfunctory or superficial fashion. This is a common pitfall among authors of evidence syntheses, especially as the standards of such tools become associated with acceptance of a manuscript by a journal. Consequently, published evidence syntheses may show improved adherence to the requirements of these tools without necessarily making genuine improvements in their performance.
In line with our main objective, the suggested tools in the Concise Guide address the reliability of evidence syntheses; however, we recognize that the utility of systematic reviews is an equally important concern. An unbiased and thoroughly reported evidence synthesis may still not be highly informative if the evidence itself that is summarized is sparse, weak and/or biased [ 24 ]. Many intervention systematic reviews, including those developed by Cochrane [ 203 ] and those applying GRADE [ 202 ], ultimately find no evidence, or find the evidence to be inconclusive (eg, “weak,” “mixed,” or of “low certainty”). This often reflects the primary research base; however, it is important to know what is known (or not known) about a topic when considering an intervention for patients and discussing treatment options with them.
Alternatively, the frequency of “empty” and inconclusive reviews published in the medical literature may relate to limitations of conventional methods that focus on hypothesis testing; these have emphasized the importance of statistical significance in primary research and effect sizes from aggregate meta-analyses [ 183 ]. It is becoming increasingly apparent that this approach may not be appropriate for all topics [ 130 ]. Development of the GRADE approach has facilitated a better understanding of significant factors (beyond effect size) that contribute to the overall certainty of evidence. Other notable responses include the development of integrative synthesis methods for the evaluation of complex interventions [ 230 , 231 ], the incorporation of crowdsourcing and machine learning into systematic review workflows (eg the Cochrane Evidence Pipeline) [ 2 ], the shift in paradigm to living systemic review and NMA platforms [ 232 , 233 ] and the proposal of a new evidence ecosystem that fosters bidirectional collaborations and interactions among a global network of evidence synthesis stakeholders [ 234 ]. These evolutions in data sources and methods may ultimately make evidence syntheses more streamlined, less duplicative, and more importantly, they may be more useful for timely policy and clinical decision-making; however, that will only be the case if they are rigorously reported and conducted.
We look forward to others’ ideas and proposals for the advancement of methods for evidence syntheses. For now, we encourage dissemination and uptake of the currently accepted best tools and practices for their development and evaluation; at the same time, we stress that uptake of appraisal tools, checklists, and software programs cannot substitute for proper education in the methodology of evidence syntheses and meta-analysis. Authors, peer reviewers, and editors must strive to make accurate and reliable contributions to the present evidence knowledge base; online alerts, upcoming technology, and accessible education may make this more feasible than ever before. Our intention is to improve the trustworthiness of evidence syntheses across disciplines, topics, and types of evidence syntheses. All of us must continue to study, teach, and act cooperatively for that to happen.
Muka T, Glisic M, Milic J, Verhoog S, Bohlius J, Bramer W, et al. A 24-step guide on how to design, conduct, and successfully publish a systematic review and meta-analysis in medical research. Eur J Epidemiol. 2020;35(1):49–60.
Article PubMed Google Scholar
Thomas J, McDonald S, Noel-Storr A, Shemilt I, Elliott J, Mavergames C, et al. Machine learning reduced workload with minimal risk of missing studies: development and evaluation of a randomized controlled trial classifier for cochrane reviews. J Clin Epidemiol. 2021;133:140–51.
Article PubMed PubMed Central Google Scholar
Fontelo P, Liu F. A review of recent publication trends from top publishing countries. Syst Rev. 2018;7(1):147.
Whiting P, Savović J, Higgins JPT, Caldwell DM, Reeves BC, Shea B, et al. ROBIS: a new tool to assess risk of bias in systematic reviews was developed. J Clin Epidemiol. 2016;69:225–34.
Shea BJ, Grimshaw JM, Wells GA, Boers M, Andersson N, Hamel C, et al. Development of AMSTAR: a measurement tool to assess the methodological quality of systematic reviews. BMC Med Res Methodol. 2007;7:1–7.
Article Google Scholar
Shea BJ, Reeves BC, Wells G, Thuku M, Hamel C, Moran J, et al. AMSTAR 2: a critical appraisal tool for systematic reviews that include randomised or non-randomised studies of healthcare interventions, or both. BMJ. 2017;358: j4008.
Goldkuhle M, Narayan VM, Weigl A, Dahm P, Skoetz N. A systematic assessment of Cochrane reviews and systematic reviews published in high-impact medical journals related to cancer. BMJ Open. 2018;8(3): e020869.
Ho RS, Wu X, Yuan J, Liu S, Lai X, Wong SY, et al. Methodological quality of meta-analyses on treatments for chronic obstructive pulmonary disease: a cross-sectional study using the AMSTAR (Assessing the Methodological Quality of Systematic Reviews) tool. NPJ Prim Care Respir Med. 2015;25:14102.
Tsoi AKN, Ho LTF, Wu IXY, Wong CHL, Ho RST, Lim JYY, et al. Methodological quality of systematic reviews on treatments for osteoporosis: a cross-sectional study. Bone. 2020;139(June): 115541.
Arienti C, Lazzarini SG, Pollock A, Negrini S. Rehabilitation interventions for improving balance following stroke: an overview of systematic reviews. PLoS ONE. 2019;14(7):1–23.
Kolaski K, Romeiser Logan L, Goss KD, Butler C. Quality appraisal of systematic reviews of interventions for children with cerebral palsy reveals critically low confidence. Dev Med Child Neurol. 2021;63(11):1316–26.
Almeida MO, Yamato TP, Parreira PCS, do Costa LOP, Kamper S, Saragiotto BT. Overall confidence in the results of systematic reviews on exercise therapy for chronic low back pain: a cross-sectional analysis using the Assessing the Methodological Quality of Systematic Reviews (AMSTAR) 2 tool. Braz J Phys Ther. 2020;24(2):103–17.
Mayo-Wilson E, Ng SM, Chuck RS, Li T. The quality of systematic reviews about interventions for refractive error can be improved: a review of systematic reviews. BMC Ophthalmol. 2017;17(1):1–10.
Matthias K, Rissling O, Pieper D, Morche J, Nocon M, Jacobs A, et al. The methodological quality of systematic reviews on the treatment of adult major depression needs improvement according to AMSTAR 2: a cross-sectional study. Heliyon. 2020;6(9): e04776.
Article CAS PubMed PubMed Central Google Scholar
Riado Minguez D, Kowalski M, Vallve Odena M, Longin Pontzen D, Jelicic Kadic A, Jeric M, et al. Methodological and reporting quality of systematic reviews published in the highest ranking journals in the field of pain. Anesth Analg. 2017;125(4):1348–54.
Churuangsuk C, Kherouf M, Combet E, Lean M. Low-carbohydrate diets for overweight and obesity: a systematic review of the systematic reviews. Obes Rev. 2018;19(12):1700–18.
Article CAS PubMed Google Scholar
Storman M, Storman D, Jasinska KW, Swierz MJ, Bala MM. The quality of systematic reviews/meta-analyses published in the field of bariatrics: a cross-sectional systematic survey using AMSTAR 2 and ROBIS. Obes Rev. 2020;21(5):1–11.
Franco JVA, Arancibia M, Meza N, Madrid E, Kopitowski K. [Clinical practice guidelines: concepts, limitations and challenges]. Medwave. 2020;20(3):e7887 ([Spanish]).
Brito JP, Tsapas A, Griebeler ML, Wang Z, Prutsky GJ, Domecq JP, et al. Systematic reviews supporting practice guideline recommendations lack protection against bias. J Clin Epidemiol. 2013;66(6):633–8.
Zhou Q, Wang Z, Shi Q, Zhao S, Xun Y, Liu H, et al. Clinical epidemiology in China series. Paper 4: the reporting and methodological quality of Chinese clinical practice guidelines published between 2014 and 2018: a systematic review. J Clin Epidemiol. 2021;140:189–99.
Lunny C, Ramasubbu C, Puil L, Liu T, Gerrish S, Salzwedel DM, et al. Over half of clinical practice guidelines use non-systematic methods to inform recommendations: a methods study. PLoS ONE. 2021;16(4):1–21.
Faber T, Ravaud P, Riveros C, Perrodeau E, Dechartres A. Meta-analyses including non-randomized studies of therapeutic interventions: a methodological review. BMC Med Res Methodol. 2016;16(1):1–26.
Ioannidis JPA. The mass production of redundant, misleading, and conflicted systematic reviews and meta-analyses. Milbank Q. 2016;94(3):485–514.
Møller MH, Ioannidis JPA, Darmon M. Are systematic reviews and meta-analyses still useful research? We are not sure. Intensive Care Med. 2018;44(4):518–20.
Moher D, Glasziou P, Chalmers I, Nasser M, Bossuyt PMM, Korevaar DA, et al. Increasing value and reducing waste in biomedical research: who’s listening? Lancet. 2016;387(10027):1573–86.
Barnard ND, Willet WC, Ding EL. The misuse of meta-analysis in nutrition research. JAMA. 2017;318(15):1435–6.
Guyatt G, Oxman AD, Akl EA, Kunz R, Vist G, Brozek J, et al. GRADE guidelines: 1. Introduction - GRADE evidence profiles and summary of findings tables. J Clin Epidemiol. 2011;64(4):383–94.
Page MJ, Shamseer L, Altman DG, Tetzlaff J, Sampson M, Tricco AC, et al. Epidemiology and reporting characteristics of systematic reviews of biomedical research: a cross-sectional study. PLoS Med. 2016;13(5):1–31.
World Health Organization. WHO handbook for guideline development, 2nd edn. WHO; 2014. Available from: https://www.who.int/publications/i/item/9789241548960 . Cited 2022 Jan 20
Higgins J, Lasserson T, Chandler J, Tovey D, Thomas J, Flemying E, et al. Methodological expectations of Cochrane intervention reviews. Cochrane; 2022. Available from: https://community.cochrane.org/mecir-manual/key-points-and-introduction . Cited 2022 Jul 19
Cumpston M, Chandler J. Chapter II: Planning a Cochrane review. In: Higgins J, Thomas J, Chandler J, Cumpston M, Li T, Page M, et al., editors. Cochrane handbook for systematic reviews of interventions. Cochrane; 2022. Available from: https://training.cochrane.org/handbook . Cited 2022 Jan 30
Henderson LK, Craig JC, Willis NS, Tovey D, Webster AC. How to write a cochrane systematic review. Nephrology. 2010;15(6):617–24.
Page MJ, Altman DG, Shamseer L, McKenzie JE, Ahmadzai N, Wolfe D, et al. Reproducible research practices are underused in systematic reviews of biomedical interventions. J Clin Epidemiol. 2018;94:8–18.
Lorenz RC, Matthias K, Pieper D, Wegewitz U, Morche J, Nocon M, et al. AMSTAR 2 overall confidence rating: lacking discriminating capacity or requirement of high methodological quality? J Clin Epidemiol. 2020;119:142–4.
Posadzki P, Pieper D, Bajpai R, Makaruk H, Könsgen N, Neuhaus AL, et al. Exercise/physical activity and health outcomes: an overview of Cochrane systematic reviews. BMC Public Health. 2020;20(1):1–12.
Wells G, Shea B, O’Connell D, Peterson J, Welch V, Losos M. The Newcastile-Ottawa Scale (NOS) for assessing the quality of nonrandomized studies in meta-analyses. The Ottawa Hospital; 2009. Available from: https://www.ohri.ca/programs/clinical_epidemiology/oxford.asp . Cited 2022 Jul 19
Stang A. Critical evaluation of the Newcastle-Ottawa scale for the assessment of the quality of nonrandomized studies in meta-analyses. Eur J Epidemiol. 2010;25(9):603–5.
Stang A, Jonas S, Poole C. Case study in major quotation errors: a critical commentary on the Newcastle-Ottawa scale. Eur J Epidemiol. 2018;33(11):1025–31.
Ioannidis JPA. Massive citations to misleading methods and research tools: Matthew effect, quotation error and citation copying. Eur J Epidemiol. 2018;33(11):1021–3.
Khalil H, Ameen D, Zarnegar A. Tools to support the automation of systematic reviews: a scoping review. J Clin Epidemiol. 2022;144:22–42.
Crequit P, Boutron I, Meerpohl J, Williams H, Craig J, Ravaud P. Future of evidence ecosystem series: 2. Current opportunities and need for better tools and methods. J Clin Epidemiol. 2020;123:143–52.
Shemilt I, Noel-Storr A, Thomas J, Featherstone R, Mavergames C. Machine learning reduced workload for the cochrane COVID-19 study register: development and evaluation of the cochrane COVID-19 study classifier. Syst Rev. 2022;11(1):15.
Nguyen P-Y, Kanukula R, McKensie J, Alqaidoom Z, Brennan SE, Haddaway N, et al. Changing patterns in reporting and sharing of review data in systematic reviews with meta-analysis of the effects of interventions: a meta-research study. medRxiv; 2022 Available from: https://doi.org/10.1101/2022.04.11.22273688v3 . Cited 2022 Nov 18
Afshari A, Møller MH. Broken science and the failure of academics—resignation or reaction? Acta Anaesthesiol Scand. 2018;62(8):1038–40.
Butler E, Granholm A, Aneman A. Trustworthy systematic reviews–can journals do more? Acta Anaesthesiol Scand. 2019;63(4):558–9.
Negrini S, Côté P, Kiekens C. Methodological quality of systematic reviews on interventions for children with cerebral palsy: the evidence pyramid paradox. Dev Med Child Neurol. 2021;63(11):1244–5.
Page MJ, Moher D. Mass production of systematic reviews and meta-analyses: an exercise in mega-silliness? Milbank Q. 2016;94(3):515–9.
Clarke M, Chalmers I. Reflections on the history of systematic reviews. BMJ Evid Based Med. 2018;23(4):121–2.
Alnemer A, Khalid M, Alhuzaim W, Alnemer A, Ahmed B, Alharbi B, et al. Are health-related tweets evidence based? Review and analysis of health-related tweets on twitter. J Med Internet Res. 2015;17(10): e246.
PubMed PubMed Central Google Scholar
Haber N, Smith ER, Moscoe E, Andrews K, Audy R, Bell W, et al. Causal language and strength of inference in academic and media articles shared in social media (CLAIMS): a systematic review. PLoS ONE. 2018;13(5): e196346.
Swetland SB, Rothrock AN, Andris H, Davis B, Nguyen L, Davis P, et al. Accuracy of health-related information regarding COVID-19 on Twitter during a global pandemic. World Med Heal Policy. 2021;13(3):503–17.
Nascimento DP, Almeida MO, Scola LFC, Vanin AA, Oliveira LA, Costa LCM, et al. Letter to the editor – not even the top general medical journals are free of spin: a wake-up call based on an overview of reviews. J Clin Epidemiol. 2021;139:232–4.
Ioannidis JPA, Fanelli D, Dunne DD, Goodman SN. Meta-research: evaluation and improvement of research methods and practices. PLoS Biol. 2015;13(10):1–7.
Munn Z, Stern C, Aromataris E, Lockwood C, Jordan Z. What kind of systematic review should I conduct? A proposed typology and guidance for systematic reviewers in the medical and health sciences. BMC Med Res Methodol. 2018;18(1):1–9.
Pollock M, Fernandez R, Becker LA, Pieper D, Hartling L. Chapter V: overviews of reviews. Cochrane handbook for systematic reviews of interventions. In: Higgins J, Thomas J, Chandler J, Cumpston M, Li T, Page M, et al., editors. Cochrane; 2022. Available from: https://training.cochrane.org/handbook/current/chapter-v . Cited 2022 Mar 7
Tricco AC, Lillie E, Zarin W, O’Brien K, Colquhoun H, Kastner M, et al. A scoping review on the conduct and reporting of scoping reviews. BMC Med Res Methodol. 2016;16(1):1–10.
Garritty C, Gartlehner G, Nussbaumer-Streit B, King VJ, Hamel C, Kamel C, et al. Cochrane rapid reviews methods group offers evidence-informed guidance to conduct rapid reviews. J Clin Epidemiol. 2021;130:13–22.
Elliott JH, Synnot A, Turner T, Simmonds M, Akl EA, McDonald S, et al. Living systematic review: 1. Introduction—the why, what, when, and how. J Clin Epidemiol. 2017;91:23–30.
Higgins JPT, Thomas J, Chandler J. Cochrane handbook for systematic reviews of interventions. Cochrane; 2022. Available from: https://training.cochrane.org/handbook . Cited 2022 Jan 25
Aromataris E, Munn Z. JBI Manual for Evidence Synthesis [internet]. JBI; 2020 [cited 2022 Jan 15]. Available from: https://synthesismanual.jbi.global .
Tufanaru C, Munn Z, Aromartaris E, Campbell J, Hopp L. Chapter 3: Systematic reviews of effectiveness. In Aromataris E, Munn Z, editors. JBI Manual for Evidence Synthesis [internet]. JBI; 2020 [cited 2022 Jan 25]. Available from: https://synthesismanual.jbi.global .
Leeflang MMG, Davenport C, Bossuyt PM. Defining the review question. In: Deeks JJ, Bossuyt PM, Leeflang MMG, Takwoingi Y, editors. Cochrane handbook for systematic reviews of diagnostic test accuracy [internet]. Cochrane; 2022 [cited 2022 Mar 30]. Available from: https://training.cochrane.org/6-defining-review-question .
Noyes J, Booth A, Cargo M, Flemming K, Harden A, Harris J, et al.Qualitative evidence. In: Higgins J, Tomas J, Chandler J, Cumpston M, Li T, Page M, et al., editors. Cochrane handbook for systematic reviews of interventions [internet]. Cochrane; 2022 [cited 2022 Mar 30]. Available from: https://training.cochrane.org/handbook/current/chapter-21#section-21-5 .
Lockwood C, Porritt K, Munn Z, Rittenmeyer L, Salmond S, Bjerrum M, et al. Chapter 2: Systematic reviews of qualitative evidence. In: Aromataris E, Munn Z, editors. JBI Manual for Evidence Synthesis [internet]. JBI; 2020 [cited 2022 Jul 11]. Available from: https://synthesismanual.jbi.global .
Debray TPA, Damen JAAG, Snell KIE, Ensor J, Hooft L, Reitsma JB, et al. A guide to systematic review and meta-analysis of prediction model performance. BMJ. 2017;356:i6460.
Moola S, Munn Z, Tufanaru C, Aromartaris E, Sears K, Sfetcu R, et al. Systematic reviews of etiology and risk. In: Aromataris E, Munn Z, editors. JBI Manual for Evidence Synthesis [internet]. JBI; 2020 [cited 2022 Mar 30]. Available from: https://synthesismanual.jbi.global/ .
Mokkink LB, Terwee CB, Patrick DL, Alonso J, Stratford PW, Knol DL, et al. The COSMIN checklist for assessing the methodological quality of studies on measurement properties of health status measurement instruments: an international Delphi study. Qual Life Res. 2010;19(4):539–49.
Prinsen CAC, Mokkink LB, Bouter LM, Alonso J, Patrick DL, de Vet HCW, et al. COSMIN guideline for systematic reviews of patient-reported outcome measures. Qual Life Res. 2018;27(5):1147–57.
Munn Z, Moola S, Lisy K, Riitano D, Tufanaru C. Chapter 5: Systematic reviews of prevalence and incidence. In: Aromataris E, Munn Z, editors. JBI Manual for Evidence Synthesis [internet]. JBI; 2020 [cited 2022 Mar 30]. Available from: https://synthesismanual.jbi.global/ .
Centre for Evidence-Based Medicine. Study designs. CEBM; 2016. Available from: https://www.cebm.ox.ac.uk/resources/ebm-tools/study-designs . Cited 2022 Aug 30
Hartling L, Bond K, Santaguida PL, Viswanathan M, Dryden DM. Testing a tool for the classification of study designs in systematic reviews of interventions and exposures showed moderate reliability and low accuracy. J Clin Epidemiol. 2011;64(8):861–71.
Crowe M, Sheppard L, Campbell A. Reliability analysis for a proposed critical appraisal tool demonstrated value for diverse research designs. J Clin Epidemiol. 2012;65(4):375–83.
Reeves BC, Wells GA, Waddington H. Quasi-experimental study designs series—paper 5: a checklist for classifying studies evaluating the effects on health interventions—a taxonomy without labels. J Clin Epidemiol. 2017;89:30–42.
Reeves BC, Deeks JJ, Higgins JPT, Shea B, Tugwell P, Wells GA. Chapter 24: including non-randomized studies on intervention effects. In: Higgins J, Thomas J, Chandler J, Cumpston M, Li T, Page M, et al., editors. Cochrane handbook for systematic reviews of interventions. Cochrane; 2022. Available from: https://training.cochrane.org/handbook/current/chapter-24 . Cited 2022 Mar 1
Reeves B. A framework for classifying study designs to evaluate health care interventions. Forsch Komplementarmed Kl Naturheilkd. 2004;11(Suppl 1):13–7.
Google Scholar
Rockers PC, Røttingen J, Shemilt I. Inclusion of quasi-experimental studies in systematic reviews of health systems research. Health Policy. 2015;119(4):511–21.
Mathes T, Pieper D. Clarifying the distinction between case series and cohort studies in systematic reviews of comparative studies: potential impact on body of evidence and workload. BMC Med Res Methodol. 2017;17(1):8–13.
Jhangiani R, Cuttler C, Leighton D. Single subject research. In: Jhangiani R, Cuttler C, Leighton D, editors. Research methods in psychology, 4th edn. Pressbooks KPU; 2019. Available from: https://kpu.pressbooks.pub/psychmethods4e/part/single-subject-research/ . Cited 2022 Aug 15
Higgins JP, Ramsay C, Reeves BC, Deeks JJ, Shea B, Valentine JC, et al. Issues relating to study design and risk of bias when including non-randomized studies in systematic reviews on the effects of interventions. Res Synth Methods. 2013;4(1):12–25.
Cumpston M, Lasserson T, Chandler J, Page M. 3.4.1 Criteria for considering studies for this review, Chapter III: Reporting the review. In: Higgins J, Thomas J, Chandler J, Cumpston M, Li T, Page M, et al., editors. Cochrane handbook for systematic reviews of interventions. Cochrane; 2022. Available from: https://training.cochrane.org/handbook/current/chapter-iii#section-iii-3-4-1 . Cited 2022 Oct 12
Kooistra B, Dijkman B, Einhorn TA, Bhandari M. How to design a good case series. J Bone Jt Surg. 2009;91(Suppl 3):21–6.
Murad MH, Sultan S, Haffar S, Bazerbachi F. Methodological quality and synthesis of case series and case reports. Evid Based Med. 2018;23(2):60–3.
Robinson K, Chou R, Berkman N, Newberry S, FU R, Hartling L, et al. Methods guide for comparative effectiveness reviews integrating bodies of evidence: existing systematic reviews and primary studies. AHRQ; 2015. Available from: https://archive.org/details/integrating-evidence-report-150226 . Cited 2022 Aug 7
Tugwell P, Welch VA, Karunananthan S, Maxwell LJ, Akl EA, Avey MT, et al. When to replicate systematic reviews of interventions: consensus checklist. BMJ. 2020;370: m2864.
Tsertsvadze A, Maglione M, Chou R, Garritty C, Coleman C, Lux L, et al. Updating comparative effectiveness reviews:current efforts in AHRQ’s effective health care program. J Clin Epidemiol. 2011;64(11):1208–15.
Cumpston M, Chandler J. Chapter IV: Updating a review. In: Higgins J, Thomas J, Chandler J, Cumpston M, Li T, Page M, et al., editors. Cochrane handbook for systematic reviews of interventions. Cochrane; 2022. Available from: https://training.cochrane.org/handbook . Cited 2022 Aug 2
Pollock M, Fernandes RM, Newton AS, Scott SD, Hartling L. A decision tool to help researchers make decisions about including systematic reviews in overviews of reviews of healthcare interventions. Syst Rev. 2019;8(1):1–8.
Pussegoda K, Turner L, Garritty C, Mayhew A, Skidmore B, Stevens A, et al. Identifying approaches for assessing methodological and reporting quality of systematic reviews: a descriptive study. Syst Rev. 2017;6(1):1–12.
Bhaumik S. Use of evidence for clinical practice guideline development. Trop Parasitol. 2017;7(2):65–71.
Moher D, Eastwood S, Olkin I, Drummond R, Stroup D. Improving the quality of reports of meta-analyses of randomised controlled trials: the QUOROM statement. Lancet. 1999;354:1896–900.
Stroup D, Berlin J, Morton S, Olkin I, Williamson G, Rennie D, et al. Meta-analysis of observational studies in epidemiology A proposal for reporting. JAMA. 2000;238(15):2008–12.
Moher D, Liberati A, Tetzlaff J, Altman DG. Preferred reporting items for systematic reviews and meta-analyses: the PRISMA statement. J Clin Epidemiol. 2009;62(10):1006–12.
Page MJ, McKenzie JE, Bossuyt PM, Boutron I, Hoffmann TC, Mulrow CD, et al. The PRISMA 2020 statement: an updated guideline for reporting systematic reviews. BMJ. 2021;372: n71.
Oxman AD, Guyatt GH. Validation of an index of the quality of review articles. J Clin Epidemiol. 1991;44(11):1271–8.
Centre for Evidence-Based Medicine. Critical appraisal tools. CEBM; 2015. Available from: https://www.cebm.ox.ac.uk/resources/ebm-tools/critical-appraisal-tools . Cited 2022 Apr 10
Page MJ, McKenzie JE, Higgins JPT. Tools for assessing risk of reporting biases in studies and syntheses of studies: a systematic review. BMJ Open. 2018;8(3):1–16.
Article CAS Google Scholar
Ma LL, Wang YY, Yang ZH, Huang D, Weng H, Zeng XT. Methodological quality (risk of bias) assessment tools for primary and secondary medical studies: what are they and which is better? Mil Med Res. 2020;7(1):1–11.
Banzi R, Cinquini M, Gonzalez-Lorenzo M, Pecoraro V, Capobussi M, Minozzi S. Quality assessment versus risk of bias in systematic reviews: AMSTAR and ROBIS had similar reliability but differed in their construct and applicability. J Clin Epidemiol. 2018;99:24–32.
Swierz MJ, Storman D, Zajac J, Koperny M, Weglarz P, Staskiewicz W, et al. Similarities, reliability and gaps in assessing the quality of conduct of systematic reviews using AMSTAR-2 and ROBIS: systematic survey of nutrition reviews. BMC Med Res Methodol. 2021;21(1):1–10.
Pieper D, Puljak L, González-Lorenzo M, Minozzi S. Minor differences were found between AMSTAR 2 and ROBIS in the assessment of systematic reviews including both randomized and nonrandomized studies. J Clin Epidemiol. 2019;108:26–33.
Lorenz RC, Matthias K, Pieper D, Wegewitz U, Morche J, Nocon M, et al. A psychometric study found AMSTAR 2 to be a valid and moderately reliable appraisal tool. J Clin Epidemiol. 2019;114:133–40.
Leclercq V, Hiligsmann M, Parisi G, Beaudart C, Tirelli E, Bruyère O. Best-worst scaling identified adequate statistical methods and literature search as the most important items of AMSTAR2 (A measurement tool to assess systematic reviews). J Clin Epidemiol. 2020;128:74–82.
Bühn S, Mathes T, Prengel P, Wegewitz U, Ostermann T, Robens S, et al. The risk of bias in systematic reviews tool showed fair reliability and good construct validity. J Clin Epidemiol. 2017;91:121–8.
Gates M, Gates A, Duarte G, Cary M, Becker M, Prediger B, et al. Quality and risk of bias appraisals of systematic reviews are inconsistent across reviewers and centers. J Clin Epidemiol. 2020;125:9–15.
Perry R, Whitmarsh A, Leach V, Davies P. A comparison of two assessment tools used in overviews of systematic reviews: ROBIS versus AMSTAR-2. Syst Rev. 2021;10(1):273.
Gates M, Gates A, Guitard S, Pollock M, Hartling L. Guidance for overviews of reviews continues to accumulate, but important challenges remain: a scoping review. Syst Rev. 2020;9(1):1–19.
Aromataris E, Fernandez R, Godfrey C, Holly C, Khalil H, Tungpunkom P. Chapter 10: umbrella reviews. In: Aromataris E, Munn Z, editors. JBI Manual for Evidence Synthesis. JBI; 2020. Available from: https://synthesismanual.jbi.global . Cited 2022 Jul 11
Pieper D, Lorenz RC, Rombey T, Jacobs A, Rissling O, Freitag S, et al. Authors should clearly report how they derived the overall rating when applying AMSTAR 2—a cross-sectional study. J Clin Epidemiol. 2021;129:97–103.
Franco JVA, Meza N. Authors should also report the support for judgment when applying AMSTAR 2. J Clin Epidemiol. 2021;138:240.
Liberati A, Altman DG, Tetzlaff J, Mulrow C, Gøtzsche PC, Ioannidis JPA, et al. The PRISMA statement for reporting systematic reviews and meta-analyses of studies that evaluate health care interventions: explanation and elaboration. PLoS Med. 2009;6(7): e1000100.
Page MJ, Moher D. Evaluations of the uptake and impact of the Preferred Reporting Items for Systematic reviews and Meta-Analyses (PRISMA) statement and extensions: a scoping review. Syst Rev. 2017;6(1):263.
Page MJ, Moher D, Bossuyt PM, Boutron I, Hoffmann TC, Mulrow CD, et al. PRISMA 2020 explanation and elaboration: updated guidance and exemplars for reporting systematic reviews. BMJ. 2021;372: n160.
Page MJ, McKenzie JE, Bossuyt PM, Boutron I, Hoffmann TC, Mulrow CD, et al. Updating guidance for reporting systematic reviews: development of the PRISMA 2020 statement. J Clin Epidemiol. 2021;134:103–12.
Welch V, Petticrew M, Petkovic J, Moher D, Waters E, White H, et al. Extending the PRISMA statement to equity-focused systematic reviews (PRISMA-E 2012): explanation and elaboration. J Clin Epidemiol. 2016;70:68–89.
Beller EM, Glasziou PP, Altman DG, Hopewell S, Bastian H, Chalmers I, et al. PRISMA for abstracts: reporting systematic reviews in journal and conference abstracts. PLoS Med. 2013;10(4): e1001419.
Moher D, Shamseer L, Clarke M. Preferred reporting items for systematic review and meta-analysis protocols (PRISMA-P) 2015 statement. Syst Rev. 2015;4(1):1.
Hutton B, Salanti G, Caldwell DM, Chaimani A, Schmid CH, Cameron C, et al. The PRISMA extension statement for reporting of systematic reviews incorporating network meta-analyses of health care interventions: checklist and explanations. Ann Intern Med. 2015;162(11):777–84.
Stewart LA, Clarke M, Rovers M, Riley RD, Simmonds M, Stewart G, et al. Preferred reporting items for a systematic review and meta-analysis of individual participant data: The PRISMA-IPD statement. JAMA. 2015;313(16):1657–65.
Zorzela L, Loke YK, Ioannidis JP, Golder S, Santaguida P, Altman DG, et al. PRISMA harms checklist: Improving harms reporting in systematic reviews. BMJ. 2016;352: i157.
McInnes MDF, Moher D, Thombs BD, McGrath TA, Bossuyt PM, Clifford T, et al. Preferred Reporting Items for a Systematic Review and Meta-analysis of Diagnostic Test Accuracy studies The PRISMA-DTA statement. JAMA. 2018;319(4):388–96.
Tricco AC, Lillie E, Zarin W, O’Brien KK, Colquhoun H, Levac D, et al. PRISMA extension for scoping reviews (PRISMA-ScR): checklist and explanation. Ann Intern Med. 2018;169(7):467–73.
Wang X, Chen Y, Liu Y, Yao L, Estill J, Bian Z, et al. Reporting items for systematic reviews and meta-analyses of acupuncture: the PRISMA for acupuncture checklist. BMC Complement Altern Med. 2019;19(1):1–10.
Rethlefsen ML, Kirtley S, Waffenschmidt S, Ayala AP, Moher D, Page MJ, et al. PRISMA-S: An extension to the PRISMA statement for reporting literature searches in systematic reviews. J Med Libr Assoc. 2021;109(2):174–200.
Blanco D, Altman D, Moher D, Boutron I, Kirkham JJ, Cobo E. Scoping review on interventions to improve adherence to reporting guidelines in health research. BMJ Open. 2019;9(5): e26589.
Koster TM, Wetterslev J, Gluud C, Keus F, van der Horst ICC. Systematic overview and critical appraisal of meta-analyses of interventions in intensive care medicine. Acta Anaesthesiol Scand. 2018;62(8):1041–9.
Johnson BT, Hennessy EA. Systematic reviews and meta-analyses in the health sciences: best practice methods for research syntheses. Soc Sci Med. 2019;233:237–51.
Pollock A, Berge E. How to do a systematic review. Int J Stroke. 2018;13(2):138–56.
Gagnier JJ, Kellam PJ. Reporting and methodological quality of systematic reviews in the orthopaedic literature. J Bone Jt Surg. 2013;95(11):1–7.
Martinez-Monedero R, Danielian A, Angajala V, Dinalo JE, Kezirian EJ. Methodological quality of systematic reviews and meta-analyses published in high-impact otolaryngology journals. Otolaryngol Head Neck Surg. 2020;163(5):892–905.
Boutron I, Crequit P, Williams H, Meerpohl J, Craig J, Ravaud P. Future of evidence ecosystem series 1. Introduction-evidence synthesis ecosystem needs dramatic change. J Clin Epidemiol. 2020;123:135–42.
Ioannidis JPA, Bhattacharya S, Evers JLH, Der Veen F, Van SE, Barratt CLR, et al. Protect us from poor-quality medical research. Hum Reprod. 2018;33(5):770–6.
Lasserson T, Thomas J, Higgins J. Section 1.5 Protocol development, Chapter 1: Starting a review. In: Higgins J, Thomas J, Chandler J, Cumpston M, Li T, Page M, et al., editors. Cochrane handbook for systematic reviews of interventions. Cochrane; 2022. Available from: https://training.cochrane.org/handbook/archive/v6/chapter-01#section-1-5 . Cited 2022 Mar 20
Stewart L, Moher D, Shekelle P. Why prospective registration of systematic reviews makes sense. Syst Rev. 2012;1(1):7–10.
Allers K, Hoffmann F, Mathes T, Pieper D. Systematic reviews with published protocols compared to those without: more effort, older search. J Clin Epidemiol. 2018;95:102–10.
Ge L, Tian J, Li Y, Pan J, Li G, Wei D, et al. Association between prospective registration and overall reporting and methodological quality of systematic reviews: a meta-epidemiological study. J Clin Epidemiol. 2018;93:45–55.
Shamseer L, Moher D, Clarke M, Ghersi D, Liberati A, Petticrew M, et al. Preferred Reporting Items for Systematic Review and Meta-Analysis Protocols (PRISMA-P) 2015: elaboration and explanation. BMJ. 2015;350: g7647.
Pieper D, Rombey T. Where to prospectively register a systematic review. Syst Rev. 2022;11(1):8.
PROSPERO. PROSPERO will require earlier registration. NIHR; 2022. Available from: https://www.crd.york.ac.uk/prospero/ . Cited 2022 Mar 20
Kirkham JJ, Altman DG, Williamson PR. Bias due to changes in specified outcomes during the systematic review process. PLoS ONE. 2010;5(3):3–7.
Victora CG, Habicht JP, Bryce J. Evidence-based public health: moving beyond randomized trials. Am J Public Health. 2004;94(3):400–5.
Peinemann F, Kleijnen J. Development of an algorithm to provide awareness in choosing study designs for inclusion in systematic reviews of healthcare interventions: a method study. BMJ Open. 2015;5(8): e007540.
Loudon K, Treweek S, Sullivan F, Donnan P, Thorpe KE, Zwarenstein M. The PRECIS-2 tool: designing trials that are fit for purpose. BMJ. 2015;350: h2147.
Junqueira DR, Phillips R, Zorzela L, Golder S, Loke Y, Moher D, et al. Time to improve the reporting of harms in randomized controlled trials. J Clin Epidemiol. 2021;136:216–20.
Hemkens LG, Contopoulos-Ioannidis DG, Ioannidis JPA. Routinely collected data and comparative effectiveness evidence: promises and limitations. CMAJ. 2016;188(8):E158–64.
Murad MH. Clinical practice guidelines: a primer on development and dissemination. Mayo Clin Proc. 2017;92(3):423–33.
Abdelhamid AS, Loke YK, Parekh-Bhurke S, Chen Y-F, Sutton A, Eastwood A, et al. Use of indirect comparison methods in systematic reviews: a survey of cochrane review authors. Res Synth Methods. 2012;3(2):71–9.
Jüni P, Holenstein F, Sterne J, Bartlett C, Egger M. Direction and impact of language bias in meta-analyses of controlled trials: empirical study. Int J Epidemiol. 2002;31(1):115–23.
Vickers A, Goyal N, Harland R, Rees R. Do certain countries produce only positive results? A systematic review of controlled trials. Control Clin Trials. 1998;19(2):159–66.
Jones CW, Keil LG, Weaver MA, Platts-Mills TF. Clinical trials registries are under-utilized in the conduct of systematic reviews: a cross-sectional analysis. Syst Rev. 2014;3(1):1–7.
Baudard M, Yavchitz A, Ravaud P, Perrodeau E, Boutron I. Impact of searching clinical trial registries in systematic reviews of pharmaceutical treatments: methodological systematic review and reanalysis of meta-analyses. BMJ. 2017;356: j448.
Fanelli D, Costas R, Ioannidis JPA. Meta-assessment of bias in science. Proc Natl Acad Sci USA. 2017;114(14):3714–9.
Hartling L, Featherstone R, Nuspl M, Shave K, Dryden DM, Vandermeer B. Grey literature in systematic reviews: a cross-sectional study of the contribution of non-English reports, unpublished studies and dissertations to the results of meta-analyses in child-relevant reviews. BMC Med Res Methodol. 2017;17(1):64.
Hopewell S, McDonald S, Clarke M, Egger M. Grey literature in meta-analyses of randomized trials of health care interventions. Cochrane Database Syst Rev. 2007;2:MR000010.
Shojania K, Sampson M, Ansari MT, Ji J, Garritty C, Radar T, et al. Updating systematic reviews. AHRQ Technical Reviews. 2007: Report 07–0087.
Tate RL, Perdices M, Rosenkoetter U, Wakim D, Godbee K, Togher L, et al. Revision of a method quality rating scale for single-case experimental designs and n-of-1 trials: The 15-item Risk of Bias in N-of-1 Trials (RoBiNT) Scale. Neuropsychol Rehabil. 2013;23(5):619–38.
Tate RL, Perdices M, McDonald S, Togher L, Rosenkoetter U. The design, conduct and report of single-case research: Resources to improve the quality of the neurorehabilitation literature. Neuropsychol Rehabil. 2014;24(3–4):315–31.
Sterne JAC, Savović J, Page MJ, Elbers RG, Blencowe NS, Boutron I, et al. RoB 2: a revised tool for assessing risk of bias in randomised trials. BMJ. 2019;366: l4894.
Sterne JA, Hernán MA, Reeves BC, Savović J, Berkman ND, Viswanathan M, et al. ROBINS-I: a tool for assessing risk of bias in non-randomised studies of interventions. BMJ. 2016;355: i4919.
Igelström E, Campbell M, Craig P, Katikireddi SV. Cochrane’s risk of bias tool for non-randomized studies (ROBINS-I) is frequently misapplied: a methodological systematic review. J Clin Epidemiol. 2021;140:22–32.
McKenzie JE, Brennan SE. Chapter 12: Synthesizing and presenting findings using other methods. In: Higgins J, Thomas J, Chandler J, Cumpston M, Li T, Page M, et al., editors. Cochrane handbook for systematic reviews of interventions. Cochrane; 2022. Available from: https://training.cochrane.org/handbook/current/chapter-12 . Cited 2022 Apr 10
Ioannidis J, Patsopoulos N, Rothstein H. Reasons or excuses for avoiding meta-analysis in forest plots. BMJ. 2008;336(7658):1413–5.
Stewart LA, Tierney JF. To IPD or not to IPD? Eval Health Prof. 2002;25(1):76–97.
Tierney JF, Stewart LA, Clarke M. Chapter 26: Individual participant data. In: Higgins JPT, Thomas J, Chandler J, Cumpston M, Li T, Page M, et al., editors. Cochrane handbook for systematic reviews of interventions. Cochrane; 2022. Available from: https://training.cochrane.org/handbook/current/chapter-26 . Cited 2022 Oct 12
Chaimani A, Caldwell D, Li T, Higgins J, Salanti G. Chapter 11: Undertaking network meta-analyses. In: Higgins J, Thomas J, Chandler J, Cumpston M, Li T, Page M, et al., editors. Cochrane handbook for systematic reviews of interventions. Cochrane; 2022. Available from: https://training.cochrane.org/handbook . Cited 2022 Oct 12.
Cooper H, Hedges L, Valentine J. The handbook of research synthesis and meta-analysis. 3rd ed. Russell Sage Foundation; 2019.
Sutton AJ, Abrams KR, Jones DR, Sheldon T, Song F. Methods for meta-analysis in medical research. Methods for meta-analysis in medical research; 2000.
Deeks J, Higgins JPT, Altman DG. Chapter 10: Analysing data and undertaking meta-analyses. In: Higgins JPT, Thomas J, Chandler J, Cumpston M, Li T, Page M, et al., editors. Cochrane handbook for systematic review of interventions. Cochrane; 2022. Available from: http://www.training.cochrane.org/handbook . Cited 2022 Mar 20.
Clarke MJ. Individual patient data meta-analyses. Best Pract Res Clin Obstet Gynaecol. 2005;19(1):47–55.
Catalá-López F, Tobías A, Cameron C, Moher D, Hutton B. Network meta-analysis for comparing treatment effects of multiple interventions: an introduction. Rheumatol Int. 2014;34(11):1489–96.
Debray T, Schuit E, Efthimiou O, Reitsma J, Ioannidis J, Salanti G, et al. An overview of methods for network meta-analysis using individual participant data: when do benefits arise? Stat Methods Med Res. 2016;27(5):1351–64.
Tonin FS, Rotta I, Mendes AM, Pontarolo R. Network meta-analysis : a technique to gather evidence from direct and indirect comparisons. Pharm Pract (Granada). 2017;15(1):943.
Tierney JF, Vale C, Riley R, Smith CT, Stewart L, Clarke M, et al. Individual participant data (IPD) metaanalyses of randomised controlled trials: guidance on their use. PLoS Med. 2015;12(7): e1001855.
Rouse B, Chaimani A, Li T. Network meta-analysis: an introduction for clinicians. Intern Emerg Med. 2017;12(1):103–11.
Cochrane Training. Review Manager RevMan Web. Cochrane; 2022. Available from: https://training.cochrane.org/online-learning/core-software/revman . Cited 2022 Jun 24
MetaXL. MetalXL. Epi Gear; 2016. Available from: http://epigear.com/index_files/metaxl.html . Cited 2022 Jun 24.
JBI. JBI SUMARI. JBI; 2019. Available from: https://sumari.jbi.global/ . Cited 2022 Jun 24.
Ryan R. Cochrane Consumers and Communication Review Group: data synthesis and analysis. Cochrane Consumers and Communication Review Group; 2013. Available from: http://cccrg.cochrane.org . Cited 2022 Jun 24
McKenzie JE, Beller EM, Forbes AB. Introduction to systematic reviews and meta-analysis. Respirology. 2016;21(4):626–37.
Campbell M, Katikireddi SV, Sowden A, Thomson H. Lack of transparency in reporting narrative synthesis of quantitative data: a methodological assessment of systematic reviews. J Clin Epidemiol. 2019;105:1–9.
Campbell M, McKenzie JE, Sowden A, Katikireddi SV, Brennan SE, Ellis S, et al. Synthesis without meta-analysis (SWiM) in systematic reviews: reporting guideline. BMJ. 2020;368: l6890.
McKenzie JE, Brennan S, Ryan R. Summarizing study characteristics and preparing for synthesis. In: Higgins J, Thomas J, Chandler J, Cumpston M, Li T, Page M, et al., editors. Cochrane handbook for systematic reviews of interventions. Cochrane; 2022. Available from: https://training.cochrane.org/handbook . Cited 2022 Oct 12
AHRQ. Systems to rate the strength of scientific evidence. Evidence report/technology assessment no. 47. AHRQ; 2002. Available from: https://archive.ahrq.gov/clinic/epcsums/strengthsum.htm . Cited 2022 Apr 10.
Atkins D, Eccles M, Flottorp S, Guyatt GH, Henry D, Hill S, et al. Systems for grading the quality of evidence and the strength of recommendations I: critical appraisal of existing approaches. BMC Health Serv Res. 2004;4(1):38.
Ioannidis JPA. Meta-research: the art of getting it wrong. Res Synth Methods. 2010;1(3–4):169–84.
Lai NM, Teng CL, Lee ML. Interpreting systematic reviews: are we ready to make our own conclusions? A cross sectional study. BMC Med. 2011;9(1):30.
Glenton C, Santesso N, Rosenbaum S, Nilsen ES, Rader T, Ciapponi A, et al. Presenting the results of Cochrane systematic reviews to a consumer audience: a qualitative study. Med Decis Making. 2010;30(5):566–77.
Yavchitz A, Ravaud P, Altman DG, Moher D, HrobjartssonA, Lasserson T, et al. A new classification of spin in systematic reviews and meta-analyses was developed and ranked according to the severity. J Clin Epidemiol. 2016;75:56–65.
Atkins D, Best D, Briss PA, Eccles M, Falck-Ytter Y, Flottorp S, et al. GRADE Working Group. Grading quality of evidence and strength of recommendations. BMJ. 2004;328:7454.
GRADE Working Group. Organizations. GRADE; 2022 [cited 2023 May 2]. Available from: www.gradeworkinggroup.org .
Hartling L, Fernandes RM, Seida J, Vandermeer B, Dryden DM. From the trenches: a cross-sectional study applying the grade tool in systematic reviews of healthcare interventions. PLoS One. 2012;7(4):e34697.
Hultcrantz M, Rind D, Akl EA, Treweek S, Mustafa RA, Iorio A, et al. The GRADE working group clarifies the construct of certainty of evidence. J Clin Epidemiol. 2017;87:4–13.
Schünemann H, Brozek J, Guyatt G, Oxman AD, Editors. Section 6.3.2. Symbolic representation. GRADE Handbook [internet]. GRADE; 2013 [cited 2022 Jan 27]. Available from: https://gdt.gradepro.org/app/handbook/handbook.html#h.lr8e9vq954 .
Siemieniuk R, Guyatt G What is GRADE? [internet] BMJ Best Practice; 2017 [cited 2022 Jul 20]. Available from: https://bestpractice.bmj.com/info/toolkit/learn-ebm/what-is-grade/ .
Guyatt G, Oxman AD, Sultan S, Brozek J, Glasziou P, Alonso-Coello P, et al. GRADE guidelines: 11. Making an overall rating of confidence in effect estimates for a single outcome and for all outcomes. J Clin Epidemiol. 2013;66(2):151–7.
Guyatt GH, Oxman AD, Sultan S, Glasziou P, Akl EA, Alonso-Coello P, et al. GRADE guidelines: 9. Rating up the quality of evidence. J Clin Epidemiol. 2011;64(12):1311–6.
Guyatt GH, Oxman AD, Vist G, Kunz R, Brozek J, Alonso-Coello P, et al. GRADE guidelines: 4. Rating the quality of evidence - Study limitations (risk of bias). J Clin Epidemiol. 2011;64(4):407–15.
Guyatt GH, Oxman AD, Kunz R, Brozek J, Alonso-Coello P, Rind D, et al. GRADE guidelines 6. Rating the quality of evidence - Imprecision. J Clin Epidemiol. 2011;64(12):1283–93.
Guyatt GH, Oxman AD, Kunz R, Woodcock J, Brozek J, Helfand M, et al. GRADE guidelines: 7. Rating the quality of evidence - Inconsistency. J Clin Epidemiol. 2011;64(12):1294–302.
Guyatt GH, Oxman AD, Kunz R, Woodcock J, Brozek J, Helfand M, et al. GRADE guidelines: 8. Rating the quality of evidence - Indirectness. J Clin Epidemiol. 2011;64(12):1303–10.
Guyatt GH, Oxman AD, Montori V, Vist G, Kunz R, Brozek J, et al. GRADE guidelines: 5. Rating the quality of evidence - Publication bias. J Clin Epidemiol. 2011;64(12):1277–82.
Andrews JC, Schünemann HJ, Oxman AD, Pottie K, Meerpohl JJ, Coello PA, et al. GRADE guidelines: 15. Going from evidence to recommendation - Determinants of a recommendation’s direction and strength. J Clin Epidemiol. 2013;66(7):726–35.
Fleming PS, Koletsi D, Ioannidis JPA, Pandis N. High quality of the evidence for medical and other health-related interventions was uncommon in Cochrane systematic reviews. J Clin Epidemiol. 2016;78:34–42.
Howick J, Koletsi D, Pandis N, Fleming PS, Loef M, Walach H, et al. The quality of evidence for medical interventions does not improve or worsen: a metaepidemiological study of Cochrane reviews. J Clin Epidemiol. 2020;126:154–9.
Mustafa RA, Santesso N, Brozek J, Akl EA, Walter SD, Norman G, et al. The GRADE approach is reproducible in assessing the quality of evidence of quantitative evidence syntheses. J Clin Epidemiol. 2013;66(7):736-742.e5.
Schünemann H, Brozek J, Guyatt G, Oxman A, editors. Section 5.4: Overall quality of evidence. GRADE Handbook. GRADE; 2013. Available from: https://gdt.gradepro.org/app/handbook/handbook.html#h.lr8e9vq954a . Cited 2022 Mar 25.
GRADE Working Group. Criteria for using GRADE. GRADE; 2016. Available from: https://www.gradeworkinggroup.org/docs/Criteria_for_using_GRADE_2016-04-05.pdf . Cited 2022 Jan 26
Werner SS, Binder N, Toews I, Schünemann HJ, Meerpohl JJ, Schwingshackl L. Use of GRADE in evidence syntheses published in high-impact-factor nutrition journals: a methodological survey. J Clin Epidemiol. 2021;135:54–69.
Zhang S, Wu QJ, Liu SX. A methodologic survey on use of the GRADE approach in evidence syntheses published in high-impact factor urology and nephrology journals. BMC Med Res Methodol. 2022;22(1):220.
Li L, Tian J, Tian H, Sun R, Liu Y, Yang K. Quality and transparency of overviews of systematic reviews. J Evid Based Med. 2012;5(3):166–73.
Pieper D, Buechter R, Jerinic P, Eikermann M. Overviews of reviews often have limited rigor: a systematic review. J Clin Epidemiol. 2012;65(12):1267–73.
Cochrane Editorial Unit. Appendix 1: Checklist for auditing GRADE and SoF tables in protocols of intervention reviews. Cochrane Training; 2022. Available from: https://training.cochrane.org/gomo/modules/522/resources/8307/Checklist for GRADE and SoF methods in Protocols for Gomo.pdf. Cited 2022 Mar 12
Ryan R, Hill S. How to GRADE the quality of the evidence. Cochrane Consumers and Communication Group. Cochrane; 2016. Available from: https://cccrg.cochrane.org/author-resources .
Cunningham M, France EF, Ring N, Uny I, Duncan EA, Roberts RJ, et al. Developing a reporting guideline to improve meta-ethnography in health research: the eMERGe mixed-methods study. Heal Serv Deliv Res. 2019;7(4):1–116.
Tong A, Flemming K, McInnes E, Oliver S, Craig J. Enhancing transparency in reporting the synthesis of qualitative research: ENTREQ. BMC Med Res Methodol. 2012;12:181.
Gates M, Gates G, Pieper D, Fernandes R, Tricco A, Moher D, et al. Reporting guideline for overviews of reviews of healthcare interventions: development of the PRIOR statement. BMJ. 2022;378:e070849.
Whiting PF, Reitsma JB, Leeflang MMG, Sterne JAC, Bossuyt PMM, Rutjes AWSS, et al. QUADAS-2: a revised tool for the quality assessment of diagnostic accuracy studies. Ann Intern Med. 2011;155(4):529–36.
Hayden JA, van der Windt DA, Cartwright JL, Co P. Research and reporting methods assessing bias in studies of prognostic factors. Ann Intern Med. 2013;158(4):280–6.
Critical Appraisal Skills Programme. CASP qualitative checklist. CASP; 2018. Available from: https://casp-uk.net/images/checklist/documents/CASP-Qualitative-Studies-Checklist/CASP-Qualitative-Checklist-2018_fillable_form.pdf . Cited 2022 Apr 26
Hannes K, Lockwood C, Pearson A. A comparative analysis of three online appraisal instruments’ ability to assess validity in qualitative research. Qual Health Res. 2010;20(12):1736–43.
Munn Z, Moola S, Riitano D, Lisy K. The development of a critical appraisal tool for use in systematic reviews addressing questions of prevalence. Int J Heal Policy Manag. 2014;3(3):123–8.
Lewin S, Bohren M, Rashidian A, Munthe-Kaas H, Glenton C, Colvin CJ, et al. Applying GRADE-CERQual to qualitative evidence synthesis findings-paper 2: how to make an overall CERQual assessment of confidence and create a Summary of Qualitative Findings table. Implement Sci. 2018;13(suppl 1):10.
Munn Z, Porritt K, Lockwood C, Aromataris E, Pearson A. Establishing confidence in the output of qualitative research synthesis: the ConQual approach. BMC Med Res Methodol. 2014;14(1):108.
Flemming K, Booth A, Hannes K, Cargo M, Noyes J. Cochrane Qualitative and Implementation Methods Group guidance series—paper 6: reporting guidelines for qualitative, implementation, and process evaluation evidence syntheses. J Clin Epidemiol. 2018;97:79–85.
Lockwood C, Munn Z, Porritt K. Qualitative research synthesis: methodological guidance for systematic reviewers utilizing meta-aggregation. Int J Evid Based Health. 2015;13(3):179–87.
Schünemann HJ, Mustafa RA, Brozek J, Steingart KR, Leeflang M, Murad MH, et al. GRADE guidelines: 21 part 1. Study design, risk of bias, and indirectness in rating the certainty across a body of evidence for test accuracy. J Clin Epidemiol. 2020;122:129–41.
Schünemann HJ, Mustafa RA, Brozek J, Steingart KR, Leeflang M, Murad MH, et al. GRADE guidelines: 21 part 2. Test accuracy: inconsistency, imprecision, publication bias, and other domains for rating the certainty of evidence and presenting it in evidence profiles and summary of findings tables. J Clin Epidemiol. 2020;122:142–52.
Foroutan F, Guyatt G, Zuk V, Vandvik PO, Alba AC, Mustafa R, et al. GRADE Guidelines 28: use of GRADE for the assessment of evidence about prognostic factors: rating certainty in identification of groups of patients with different absolute risks. J Clin Epidemiol. 2020;121:62–70.
Janiaud P, Agarwal A, Belbasis L, Tzoulaki I. An umbrella review of umbrella reviews for non-randomized observational evidence on putative risk and protective factors [internet]. OSF protocol; 2021 [cited 2022 May 28]. Available from: https://osf.io/xj5cf/ .
Mokkink LB, Prinsen CA, Patrick DL, Alonso J, Bouter LM, et al. COSMIN methodology for systematic reviews of Patient-Reported Outcome Measures (PROMs) - user manual. COSMIN; 2018 [cited 2022 Feb 15]. Available from: http://www.cosmin.nl/ .
Thomas J, M P, Noyes J, Chandler J, Rehfuess E, Tugwell P, et al. Chapter 17: Intervention complexity. In: Higgins J, Thomas J, Chandler J, Cumpston M, Li T, Page M, et al., editors. Cochrane handbook for systematic reviews of interventions. Cochrane; 2022. Available from: https://training.cochrane.org/handbook/current/chapter-17 . Cited 2022 Oct 12
Guise JM, Chang C, Butler M, Viswanathan M, Tugwell P. AHRQ series on complex intervention systematic reviews—paper 1: an introduction to a series of articles that provide guidance and tools for reviews of complex interventions. J Clin Epidemiol. 2017;90:6–10.
Riaz IB, He H, Ryu AJ, Siddiqi R, Naqvi SAA, Yao Y, et al. A living, interactive systematic review and network meta-analysis of first-line treatment of metastatic renal cell carcinoma [formula presented]. Eur Urol. 2021;80(6):712–23.
Créquit P, Trinquart L, Ravaud P. Live cumulative network meta-analysis: protocol for second-line treatments in advanced non-small-cell lung cancer with wild-type or unknown status for epidermal growth factor receptor. BMJ Open. 2016;6(8):e011841.
Ravaud P, Créquit P, Williams HC, Meerpohl J, Craig JC, Boutron I. Future of evidence ecosystem series: 3. From an evidence synthesis ecosystem to an evidence ecosystem. J Clin Epidemiol. 2020;123:153–61.
Download references
Acknowledgements
Michelle Oakman Hayes for her assistance with the graphics, Mike Clarke for his willingness to answer our seemingly arbitrary questions, and Bernard Dan for his encouragement of this project.
The work of John Ioannidis has been supported by an unrestricted gift from Sue and Bob O’Donnell to Stanford University.
Author information
Authors and affiliations.
Departments of Orthopaedic Surgery, Pediatrics, and Neurology, Wake Forest School of Medicine, Winston-Salem, NC, USA
Kat Kolaski
Department of Physical Medicine and Rehabilitation, SUNY Upstate Medical University, Syracuse, NY, USA
Lynne Romeiser Logan
Departments of Medicine, of Epidemiology and Population Health, of Biomedical Data Science, and of Statistics, and Meta-Research Innovation Center at Stanford (METRICS), Stanford University School of Medicine, Stanford, CA, USA
John P. A. Ioannidis
You can also search for this author in PubMed Google Scholar
Contributions
All authors participated in the development of the ideas, writing, and review of this manuscript. The author(s) read and approved the final manuscript.
Corresponding author
Correspondence to Kat Kolaski .
Ethics declarations
Competing interests.
The authors declare no competing interests.
Additional information
Publisher’ s note.
Springer Nature remains neutral with regard to jurisdictional claims in published maps and institutional affiliations.
This article has been published simultaneously in BMC Systematic Reviews, Acta Anaesthesiologica Scandinavica, BMC Infectious Diseases, British Journal of Pharmacology, JBI Evidence Synthesis, the Journal of Bone and Joint Surgery Reviews , and the Journal of Pediatric Rehabilitation Medicine .
Supplementary Information
Additional file 2a..
Overviews, scoping reviews, rapid reviews and living reviews.
Additional file 2B.
Practical scheme for distinguishing types of research evidence.
Additional file 4.
Presentation of forest plots.
Additional file 5A.
Illustrations of the GRADE approach.
Additional file 5B.
Adaptations of GRADE for evidence syntheses.
Additional file 6.
Links to Concise Guide online resources.
Rights and permissions
Open Access This article is licensed under a Creative Commons Attribution 4.0 International License, which permits use, sharing, adaptation, distribution and reproduction in any medium or format, as long as you give appropriate credit to the original author(s) and the source, provide a link to the Creative Commons licence, and indicate if changes were made. The images or other third party material in this article are included in the article's Creative Commons licence, unless indicated otherwise in a credit line to the material. If material is not included in the article's Creative Commons licence and your intended use is not permitted by statutory regulation or exceeds the permitted use, you will need to obtain permission directly from the copyright holder. To view a copy of this licence, visit http://creativecommons.org/licenses/by/4.0/ . The Creative Commons Public Domain Dedication waiver ( http://creativecommons.org/publicdomain/zero/1.0/ ) applies to the data made available in this article, unless otherwise stated in a credit line to the data.
Reprints and permissions
About this article
Cite this article.
Kolaski, K., Logan, L.R. & Ioannidis, J.P.A. Guidance to best tools and practices for systematic reviews. Syst Rev 12 , 96 (2023). https://doi.org/10.1186/s13643-023-02255-9
Download citation
Received : 03 October 2022
Accepted : 19 February 2023
Published : 08 June 2023
DOI : https://doi.org/10.1186/s13643-023-02255-9
Share this article
Anyone you share the following link with will be able to read this content:
Sorry, a shareable link is not currently available for this article.
Provided by the Springer Nature SharedIt content-sharing initiative
- Certainty of evidence
- Critical appraisal
- Methodological quality
- Risk of bias
- Systematic review
Systematic Reviews
ISSN: 2046-4053
- Submission enquiries: Access here and click Contact Us
- General enquiries: [email protected]
Cochrane RevMan
Revman: systematic review and meta-analysis tool for researchers worldwide.
Pricing & subscription
Intuitive and easy to use
High quality support and guidance, promotes high quality research, collaborate on the same project.
University of Tasmania, Australia
Systematic reviews for health: tools for systematic review.
- Handbooks / Guidelines for Systematic Reviews
- Standards for Reporting
- Registering a Protocol
- Tools for Systematic Review
- Online Tutorials & Courses
- Books and Articles about Systematic Reviews
- Finding Systematic Reviews
- Critical Appraisal
- Library Help
- Bibliographic Databases
- Grey Literature
- Handsearching
- Citation Tracking
- 1. Formulate the Research Question
- 2. Identify the Key Concepts
- 3. Develop Search Terms - Free-Text
- 4. Develop Search Terms - Controlled Vocabulary
- 5. Search Fields
- 6. Phrase Searching, Wildcards and Proximity Operators
- 7. Boolean Operators
- 8. Search Limits
- 9. Pilot Search Strategy & Monitor Its Development
- 10. Final Search Strategy
- 11. Adapt Search Syntax
- Documenting Search Strategies
- Handling Results & Storing Papers
Tools for Systematic Reviews
Managing the selection process can be challenging, particularly in a large-scale systematic review that involves multiple reviewers. There are various free and subscription-based tools available that support the study selection process ( Cochrane Handbook, 4.6.6.1 ).
This page describes various tools available to help conduct a systematic review. The University of Tasmania has access to EndNote, Covidence and JBI SUMARI.
Covidence is an online systematic review program developed by, and for, systematic reviewers. It can import citations from reference managers like EndNote, facilitate the screening of abstracts and full-text, populate risk of bias tables, assist with data extraction, and export to all common formats.
Covidence Demo video [3:24]
Covidence is a core component of Cochrane's review production toolkit and has also been endorsed by JBI.
Access to UTAS Covidence account
If you are the project leader, follow these steps to create a UTAS Covidence account:
- Create a UTAS Covidence account Step-by-step instructions on how to create at UTAS Covidence account
Once you have created your UTAS Covidence account, you can create a review and invite others to join the review.
If you are not the project leader, please wait for your invitation from your project leader to join the review (you don't need to create a UTAS Covidence account).
Covidence Training & Help
- Need some help getting started? Covidence offers regular training webinars . You also have the option to listen to the recording of a recent session.
- Work through the content of the Covidence Academy .
- Subscribe to the Covidence YouTube channel to find video tutorials and recorded webinars. The Step-by-step webinars playlist is particularly useful for in-depth guidance.
- Head to the Covidence knowledge base to get answers to FAQs.
- Contact [email protected] anytime to answer any of your questions.
Abstrackr is a software for semi-automated abstract screening for systematic reviews. At present, Abstrackr is a free, open-source tool for facilitating the citation screening process. Upload your abstracts, invite reviewers, and get to screening!
Rayyan is a free online tool that can be used for screening and coding of studies in a systematic review. It uses tagging and filtering to code and organise references.
The System for the Unified Management, Assessment and Review of Information ( SUMARI ) is JBI 's software for the systematic review of literature.
I t is designed to assist researchers to conduct systematic reviews and facilitates the entire review process. SUMARI supports 10 review types. It is especially useful for new review types and qualitative reviews.
University of Tasmania researchers have access to SUMARI via the JBI EBP Database under EBP Tools .
- JBI EBP Database via Ovid This link opens in a new window
SUMARI support:
- JBI SUMARI Video Tutorials
- JBI SUMARI Knowledge Base
RevMan 5 is the software used for preparing and maintaining Cochrane Reviews. RevMan facilitates preparation of protocols and full reviews, including text, characteristics of studies, comparison tables, and study data. It can perform meta-analysis of the data entered, and present the results graphically.
RevMan 5 is no longer being developed, but they continue to support Cochrane authors.
RevMan Web is the next generation of Cochrane's software for preparing and maintaining systematic reviews. This web-based version of RevMan works across all platforms, is installation-free, and automatically updated.
DistillerSR
DistillerSR is a systematic review software. It was designed from the ground up to provide a better review experience, faster project completion and transparent, audit-ready results.
What can you do in DistillerSR? Upload your references from any reference management software, create screening and data extraction forms, lay out workflow and assign reviewers, monitor study progress and review process, export results (incl PRISMA flowchart automation).
This software is more sophisticated and a bit harder to learn. DistillerSR attracts a fee .
The Systematic Review Toolbox is a community-driven, searchable, web-based catalogue of tools that support the systematic review process across multiple domains. The resource aims to help reviewers find appropriate tools based on how they provide support for the systematic review process. Users can perform a simple keyword search (i.e. Quick Search) to locate tools, a more detailed search (i.e. Advanced Search) allowing users to select various criteria to find specific types of tools and submit new tools to the database.
Need More Help? Book a consultation with a Learning and Research Librarian or contact [email protected] .
- << Previous: Registering a Protocol
- Next: Online Tutorials & Courses >>
- Last Updated: Apr 4, 2024 10:17 AM
- URL: https://utas.libguides.com/SystematicReviews

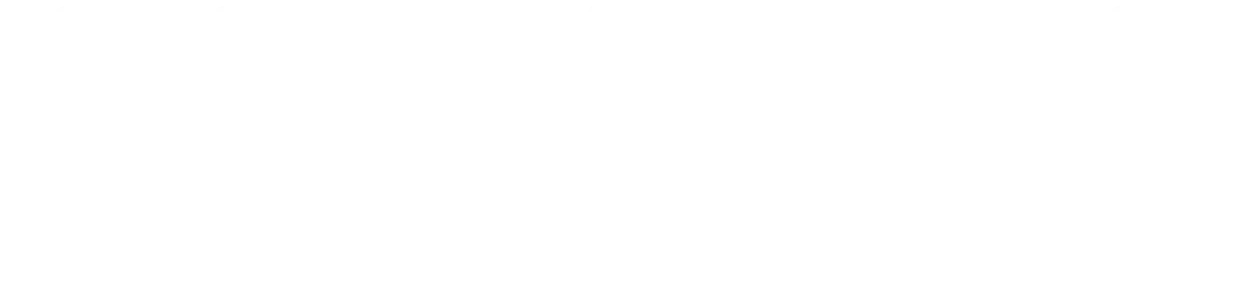
- Help Center
GET STARTED
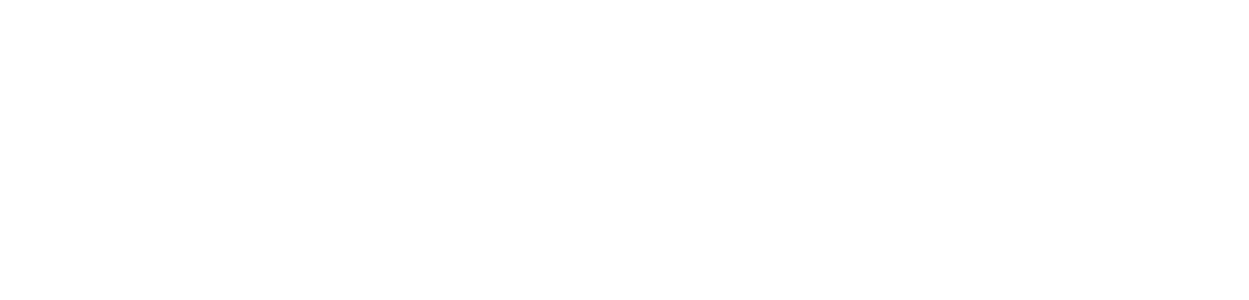
COLLABORATE ON YOUR REVIEWS WITH ANYONE, ANYWHERE, ANYTIME
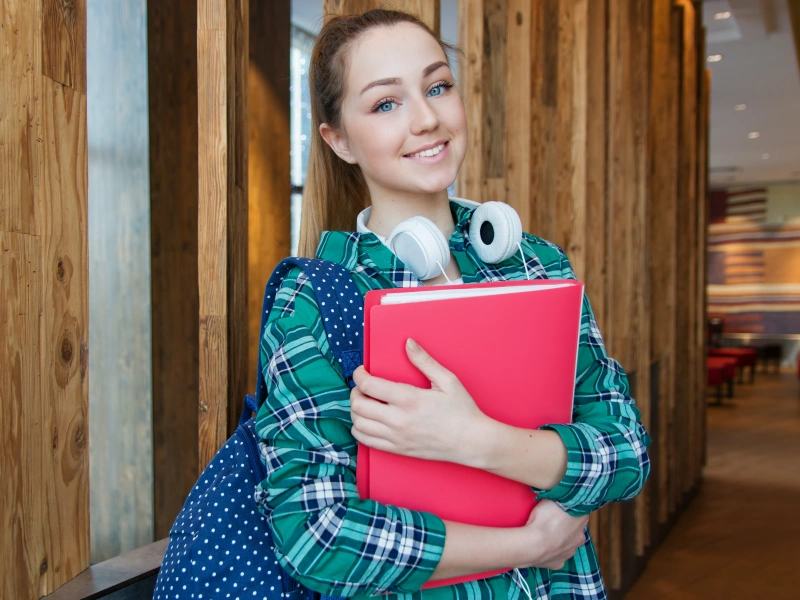
Save precious time and maximize your productivity with a Rayyan membership. Receive training, priority support, and access features to complete your systematic reviews efficiently.
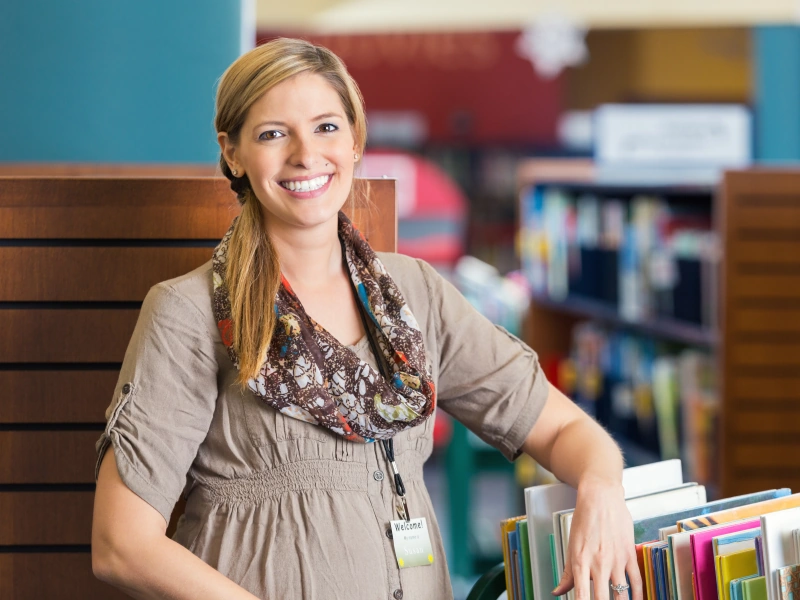
Rayyan Teams+ makes your job easier. It includes VIP Support, AI-powered in-app help, and powerful tools to create, share and organize systematic reviews, review teams, searches, and full-texts.
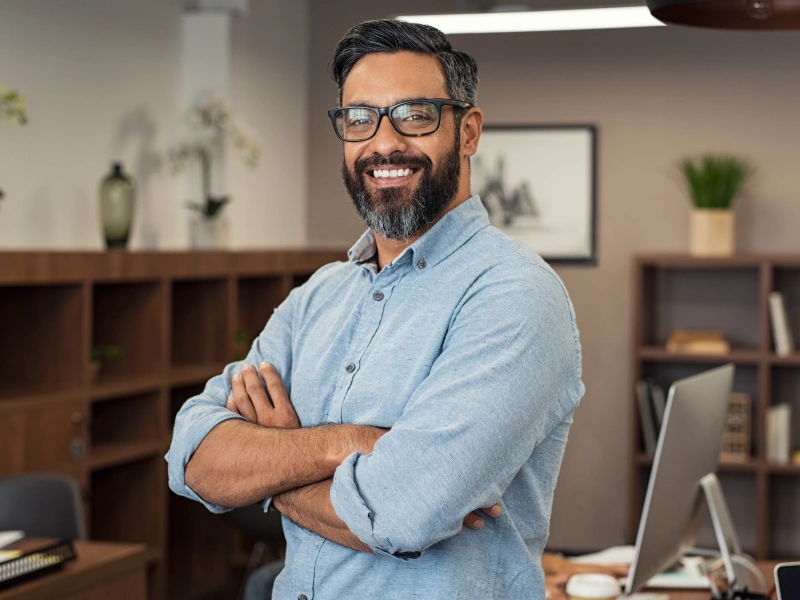
RESEARCHERS
Rayyan makes collaborative systematic reviews faster, easier, and more convenient. Training, VIP support, and access to new features maximize your productivity. Get started now!
Over 1 billion reference articles reviewed by research teams, and counting...
Intelligent, scalable and intuitive.
Rayyan understands language, learns from your decisions and helps you work quickly through even your largest systematic literature reviews.
WATCH A TUTORIAL NOW
Solutions for Organizations and Businesses
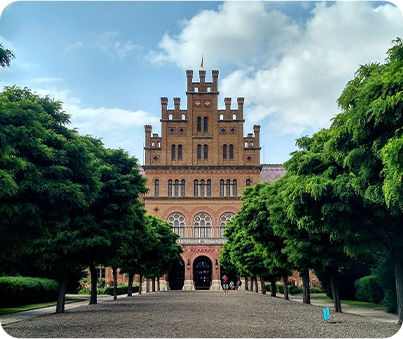
Rayyan Enterprise and Rayyan Teams+ make it faster, easier and more convenient for you to manage your research process across your organization.
- Accelerate your research across your team or organization and save valuable researcher time.
- Build and preserve institutional assets, including literature searches, systematic reviews, and full-text articles.
- Onboard team members quickly with access to group trainings for beginners and experts.
- Receive priority support to stay productive when questions arise.
- SCHEDULE A DEMO
- LEARN MORE ABOUT RAYYAN TEAMS+
RAYYAN SYSTEMATIC LITERATURE REVIEW OVERVIEW
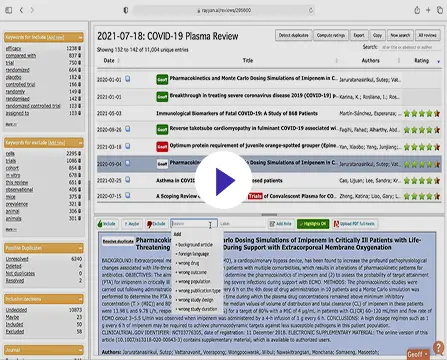
LEARN ABOUT RAYYAN’S PICO HIGHLIGHTS AND FILTERS
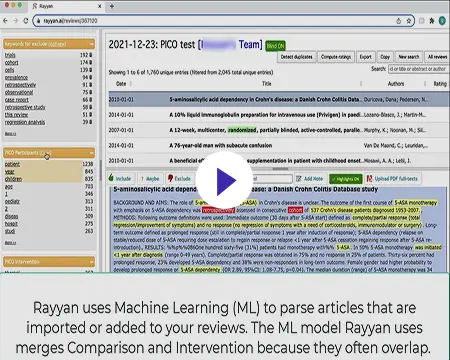
Join now to learn why Rayyan is trusted by already more than 500,000 researchers
Individual plans, teams plans.
For early career researchers just getting started with research.
Free forever
- 3 Active Reviews
- Invite Unlimited Reviewers
- Import Directly from Mendeley
- Industry Leading De-Duplication
- 5-Star Relevance Ranking
- Advanced Filtration Facets
- Mobile App Access
- 100 Decisions on Mobile App
- Standard Support
- Revoke Reviewer
- Online Training
- PICO Highlights & Filters
- PRISMA (Beta)
- Auto-Resolver
- Multiple Teams & Management Roles
- Monitor & Manage Users, Searches, Reviews, Full Texts
- Onboarding and Regular Training
Professional
For researchers who want more tools for research acceleration.
Per month billed annually
- Unlimited Active Reviews
- Unlimited Decisions on Mobile App
- Priority Support
- Auto-Resolver
For students who want more tools to accelerate their research.
Per month billed annually
Billed monthly
For a team that wants professional licenses for all members.
Per-user, per month, billed annually
- Single Team
- High Priority Support
For teams that want support and advanced tools for members.
- Multiple Teams
- Management Roles
For organizations who want access to all of their members.
Annual Subscription
Contact Sales
- Organizational Ownership
- For an organization or a company
- Access to all the premium features such as PICO Filters, Auto-Resolver, PRISMA and Mobile App
- Store and Reuse Searches and Full Texts
- A management console to view, organize and manage users, teams, review projects, searches and full texts
- Highest tier of support – Support via email, chat and AI-powered in-app help
- GDPR Compliant
- Single Sign-On
- API Integration
- Training for Experts
- Training Sessions Students Each Semester
- More options for secure access control
ANNUAL ONLY
Per-user, billed monthly
Rayyan Subscription
membership starts with 2 users. You can select the number of additional members that you’d like to add to your membership.
Total amount:
Click Proceed to get started.
Great usability and functionality. Rayyan has saved me countless hours. I even received timely feedback from staff when I did not understand the capabilities of the system, and was pleasantly surprised with the time they dedicated to my problem. Thanks again!
This is a great piece of software. It has made the independent viewing process so much quicker. The whole thing is very intuitive.
Rayyan makes ordering articles and extracting data very easy. A great tool for undertaking literature and systematic reviews!
Excellent interface to do title and abstract screening. Also helps to keep a track on the the reasons for exclusion from the review. That too in a blinded manner.
Rayyan is a fantastic tool to save time and improve systematic reviews!!! It has changed my life as a researcher!!! thanks
Easy to use, friendly, has everything you need for cooperative work on the systematic review.
Rayyan makes life easy in every way when conducting a systematic review and it is easy to use.
Literature Review Tips & Tools
- Tips & Examples
Organizational Tools
Tools for systematic reviews.
- Bubbl.us Free online brainstorming/mindmapping tool that also has a free iPad app.
- Coggle Another free online mindmapping tool.
- Organization & Structure tips from Purdue University Online Writing Lab
- Literature Reviews from The Writing Center at University of North Carolina at Chapel Hill Gives several suggestions and descriptions of ways to organize your lit review.
- Cochrane Handbook for Systematic Reviews of Interventions "The Cochrane Handbook for Systematic Reviews of Interventions is the official guide that describes in detail the process of preparing and maintaining Cochrane systematic reviews on the effects of healthcare interventions. "
- Preferred Reporting Items for Systematic Reviews and Meta-Analyses (PRISMA) website "PRISMA is an evidence-based minimum set of items for reporting in systematic reviews and meta-analyses. PRISMA focuses on the reporting of reviews evaluating randomized trials, but can also be used as a basis for reporting systematic reviews of other types of research, particularly evaluations of interventions."
- PRISMA Flow Diagram Generator Free tool that will generate a PRISMA flow diagram from a CSV file (sample CSV template provided) more... less... Please cite as: Haddaway, N. R., Page, M. J., Pritchard, C. C., & McGuinness, L. A. (2022). PRISMA2020: An R package and Shiny app for producing PRISMA 2020-compliant flow diagrams, with interactivity for optimised digital transparency and Open Synthesis Campbell Systematic Reviews, 18, e1230. https://doi.org/10.1002/cl2.1230
- Rayyan "Rayyan is a 100% FREE web application to help systematic review authors perform their job in a quick, easy and enjoyable fashion. Authors create systematic reviews, collaborate on them, maintain them over time and get suggestions for article inclusion."
- Covidence Covidence is a tool to help manage systematic reviews (and create PRISMA flow diagrams). **UMass Amherst doesn't subscribe, but Covidence offers a free trial for 1 review of no more than 500 records. It is also set up for researchers to pay for each review.
- PROSPERO - Systematic Review Protocol Registry "PROSPERO accepts registrations for systematic reviews, rapid reviews and umbrella reviews. PROSPERO does not accept scoping reviews or literature scans. Sibling PROSPERO sites registers systematic reviews of human studies and systematic reviews of animal studies."
- Critical Appraisal Tools from JBI Joanna Briggs Institute at the University of Adelaide provides these checklists to help evaluate different types of publications that could be included in a review.
- Systematic Review Toolbox "The Systematic Review Toolbox is a community-driven, searchable, web-based catalogue of tools that support the systematic review process across multiple domains. The resource aims to help reviewers find appropriate tools based on how they provide support for the systematic review process. Users can perform a simple keyword search (i.e. Quick Search) to locate tools, a more detailed search (i.e. Advanced Search) allowing users to select various criteria to find specific types of tools and submit new tools to the database. Although the focus of the Toolbox is on identifying software tools to support systematic reviews, other tools or support mechanisms (such as checklists, guidelines and reporting standards) can also be found."
- Abstrackr Free, open-source tool that "helps you upload and organize the results of a literature search for a systematic review. It also makes it possible for your team to screen, organize, and manipulate all of your abstracts in one place." -From Center for Evidence Synthesis in Health
- SRDR Plus (Systematic Review Data Repository: Plus) An open-source tool for extracting, managing,, and archiving data developed by the Center for Evidence Synthesis in Health at Brown University
- RoB 2 Tool (Risk of Bias for Randomized Trials) A revised Cochrane risk of bias tool for randomized trials
- << Previous: Tips & Examples
- Next: Writing & Citing Help >>
- Last Updated: Apr 2, 2024 4:46 PM
- URL: https://guides.library.umass.edu/litreviews
© 2022 University of Massachusetts Amherst • Site Policies • Accessibility

An official website of the United States government
Here's how you know
The .gov means it’s official. Federal government websites often end in .gov or .mil. Before sharing sensitive information, make sure you’re on a federal government site.
The site is secure. The https:// ensures that you are connecting to the official website and that any information you provide is encrypted and transmitted securely.
Tools & Resources
Software tools.
There are a variety of fee-based and open-source (i.e., free) tools available for conducting the various steps of your scoping or systematic review.
The NIH Library currently provides free access for NIH customers to Covidence . At least one user must be from NIH in order to request access and use Covidence. Please contact the NIH Library's Systematic Review Service to request access.
You can use Covidence to import citations from any citation management tool and then screen your citations at title and abstract and then full text levels. Covidence keeps track of who voted and manages the flow of the citations to ensure the correct number of screeners reviews each citation. It can also support single or dual screeners. In the full text screening step, you can upload PDFs into Covidence and it will keep track of your excluded citations and reasons for exclusion. Later, export this information to help you complete the PRISMA flow diagram. If you chose, you can also complete your data extraction and risk of bias assessments in Covidence by creating templates based on your needs and type of risk of bias tool. Finally, export all of your results for data management purposes or export your data into another data analysis tool for further work.
Other tools available for conducting scoping or systematic reviews are:
- DistillerSR (fee-based)
- EPPI-Reviewer (fee-based)
- JBI SUMARI (from the Joanna Briggs Institute for their reviews) (fee-based)
- LitStream (from ICF International)
- Systematic Review Data Repository (SRDR+) (from AHRQ) (free)
- Abstrackr (open source)
- Colandr (open source)
- Google Sheets and Forms
- HAWC (Health Assessment Workplace Collaborative) (free)
- Rayyan (open source)
And check out the Systematic Review Toolbox for additional software suggestions for conducting your review.
Quality Assessment Tools (i.e., risk of bias, critical appraisal)
- 2022 Repository of Quality Assessment and Risk of Bias Tools - A comprehensive resource for finding and selecting a risk of bias or quality assessment tool for evidence synthesis projects. Continually updated.
- AMSTAR 2 - AMSTAR 2 ( A MeaSurement Tool to Assess systematic Reviews). Use for critically appraising ONLY systematic reviews of healthcare interventions including randomised controlled clinical trials.
- JADAD Scale for Reporting Randomized Controlled Trials - The Jadad scale, sometimes known as Jadad scoring or the Oxford quality scoring system, is a procedure to independently assess the methodological quality of a clinical trial. Jadad et al. published a three-point questionnaire that formed the basis for a Jadad score.
- Joanna Briggs Institute Critical Appraisal Tools - includes 13 checklists to appraise a variety of different studies and publication types including qualitative studies.
- RoB 2.0: Cochrane Risk of Bias Tool for Randomized Trials Version 2 of the Cochrane RoB 2 can be used to assess the risk of bias in randomized trials.
- CASP (Critical Appraisal Skills Program ) - a number of checklists are available to appraise systematic reviews, randomised controlled trials, cohort studies, case control studies, economic evaluations, diagnostic studies, qualitative studies and clinical prediction rule.
- Newcastle-Ottawa Scale (NOS) for Assessing the Quality of Nonrandomised Studies in Meta-Analyses - Nonrandomised studies, including case-control and cohort studies, can be challenging to implement and conduct. Assessment of the quality of such studies is essential for a proper understanding of nonrandomised studies. The Newcastle-Ottawa Scale (NOS) is an ongoing collaboration between the Universities of Newcastle, Australia and Ottawa, Canada. It was developed to assess the quality of nonrandomised studies with its design, content and ease of use directed to the task of incorporating the quality assessments in the interpretation of meta-analytic results.
Background information on this important step of systematic reviews can be found at the following resources:
- Cochrane Handbook for Sysetmatic Reviews of Interventions (version 6.2) 2021 - see Chapter 7: Considering bias and conflicts of interest among the included studies , Chapter 8: Assessing risk of bias in a randomized trial , Chapter 13: Assessing risk of bias due to missing results in a synthesis and Chapter 25: Assessing risk of bias in a non-randomized study
- Aromataris E, Munn Z (Editors) . JBI Manual for Evidence Synthesis . JBI, 2020. https://doi.org/10.46658/JBIMES-20-01 - see appropriate chapter for type of review and the section on risk of bias.
- Chapter: Assessing the Risk of Bias of Individual Studies in Systematic Reviews of Healthcare Interventions from AHRQ. 2017 December. Methods Guide for Effectiveness and Comparative Effectiveness Reviews . Rockville, MD, AHRQ.
- Chapter 3: Standards for Finding and Assessing Individual Studies in Institute of Medicine. 2011. Finding What Works in Health Care: Standards for Systematic Reviews . Washington, DC: The National Academies Press.
Grading Tools
GRADE Working Group
The working group has developed a common, sensible and transparent approach to grading quality of evidence and strength of recommendations.
- Oxford Centre for Evidence Based Medicine - Levels of Evidence and Grades of Recommendations
Reporting Standards for Systematic Reviews
The Appraisal of Guidelines for Research and Evaluation (AGREE) Instrument evaluates the process of practice guideline development and the quality of reporting.
Collects guidance documents on reporting systematic reviews and other types of health research
PRISMA 2020
Preferred Reporting Items for Systematic Reviews and Meta-Analyses. PRISMA 2020 was published in 2021 with an revised checklist , flow diagram , and a new elaboration and explanation paper .
The Methodological Expectations of Cochrane Intervention Reviews (MECIR) are methodological standards to which all Cochrane Protocols, Reviews, and Updates are expected to adhere
- RAMESES publication standards: meta-narrative reviews
Online Videos on Systematic Reviews
The Campbell Collaboration
A collection of introductory and advanced videos on systematic reviews
Cochrane Introduction to Systematic Reviews
This module provides an overview to Cochrane systematic reviews, and will take approximately 45 minutes to complete.
Systematic Review and Evidence-Based Medicine
Dr. Aaron Carroll (The Incidental Economist) take on evidenced-based practice and systematic reviews
How to Critically Appraise Evidence
A collection of videos on evidence-based practice, common statistical methods in medicine, and systematic reviews
Introduction to Meta-Analysis
Dr. Michael Borenstein short introduction to meta-analysis
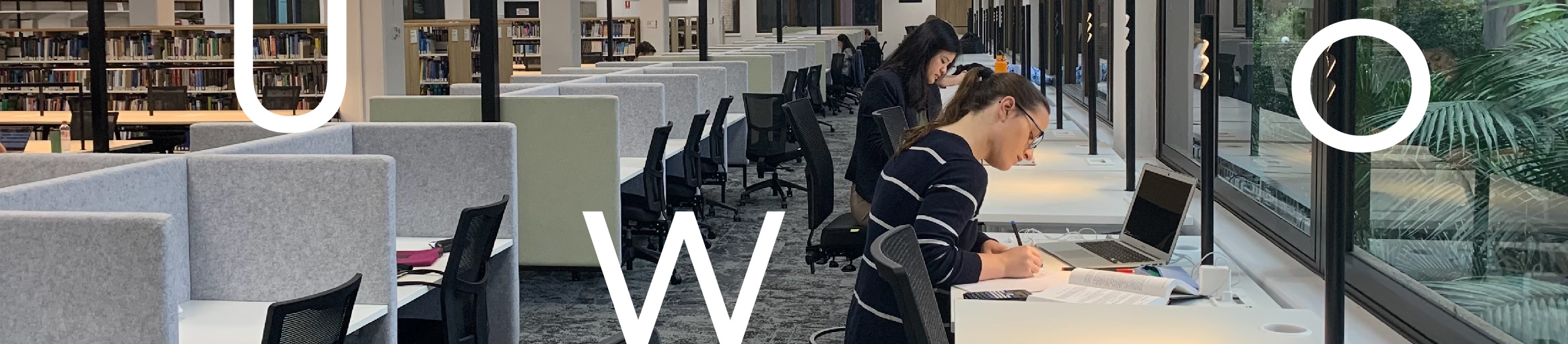
Help us improve our Library guides with this 5 minute survey . We appreciate your feedback!
- UOW Library
- Key guides for researchers
Systematic Review
- What is a systematic review?
- Five other types of systematic review
- How is a literature review different?
- Search tips for systematic reviews
- Controlled vocabularies
- Grey literature
- Transferring your search
- Documenting your results
- Support & contact
Tools for systematic reviews
There are many tools you can use when conducting a systematic review. These tools are designed to assist with the key stages of the process, including title and abstract screening, data synthesis, and critical appraisal.
Registering your review is recommended best practice and options are explored in the Register your review section of this guide.
Covidence is a web-based screening and data extraction tool for authors conducting systematic and scoping reviews. Covidence includes functions to support uploading search results, screening abstracts, conducting risk of bias assessments and more to make your review production more efficient.
How to join University of Wollongong’s Covidence account
To request access to UOW’s Covidence account, you must have an active @uow.edu.au or @uowmail.edu.au email address.
- Go to the Covidence sign-up page
- Enter your first name and UOW email address, then click “Request Invitation”
- You will receive an invitation email sent by covidence.org. Open the email and click “Accept Invitation”
- Follow the prompts to create your personal Covidence account using the same UOW email address.
Covidence support
The Covidence Knowledge Base and Getting Started with Covidence videos provide comprehensive support.
Already signed up?
Sign in with your personal account and access Covidence.
Critical appraisal tools
Critical appraisal skills enable you to systematically assess the trustworthiness, relevance and results of published papers. The Centre for Evidence Based Medicine defines critical appraisal as the systematic evaluation of clinical research papers in order to establish:
- Does this study address a clearly focused question?
- Did the study use valid methods to address this question?
- Are the valid results of this study important?
- Are these valid, important results applicable to my patient or population?
A comprehensive set of critical appraisal tools can be found on the University of South Australia’s library guide .
JBI SUMARI facilitates the entire review process, from protocol development, team management, study selection, critical appraisal, data extraction, data synthesis and writing your systematic review. This tool is developed by the Joanna Briggs Institute (JBI) .
To set up a personal OVID account and access SUMARI as UOW staff or student, follow these instructions .
Risk of bias tools
The NHMRC states that risks of bias are the likelihood that features of the study design or conduct of the study will give misleading results. This can result in wasted resources, lost opportunities for effective interventions or harm to consumers.
See riskofbias.info for details of tools you can use to asses risk of bias, including:
- RoB 2.0: Cochrane's risk of bias tool for randomised controlled trials
- ROBINS-I: evaluates the risk of bias in the studies that compare the health effects of two or more interventions
- ROBINS-E: provides a structured approach to assessing the risk of bias in observational epidemiological studies
- ROB ME: a tool for assessing risk of bias due to missing evidence in a synthesis
- Robvis: a web app designed to for visualizing risk-of-bias assessments performed as part of a systematic review.
Systematic Reviewlution
Systematic Reviewlution is a living review compiling evidence of where published systematic reviews are not being done well. Awareness of these problems will enable researchers, publishers and decision makers to conduct better systematic reviews in the future
The review includes a framework of common problems with systematic reviews, that should be considered as your develop your own review protocols.
Register your review
It is good practice to register your systematic review with PROSPERO or the International Database of Education Systematic Reviews. Scoping and rapid reviews can be registered with Figshare or Open Science Framework (OSF).
PROSPERO is an international register for prospective systematic literature reviews.
It includes protocol details for systematic reviews relevant to:
- health and public health
- social care and welfare
- crime and justice
- international development
Protocols can include any type of any study design where there is a health-related outcome.
Search PROSPERO
International Database of Education Systematic Reviews (IDESR)
IDESR is a database of published systematic reviews in Education and a clearinghouse for protocol registration of ongoing and planned systematic reviews. IDESR accepts registrations of protocols for systematic reviews in all fields of education.
- Search and register with IDESR .
Figshare is an open repository where you can make your review protocol citable, shareable and discoverable.
- Search and register with Figshare.
Open Science Framework (OSF)
Recommended by PRISMA and PRISMA-ScR, the Open Science Framework is a free, open platform to support users' research and enable collaboration.
- Search and register with OSF.
- Previous: Tools and frameworks
- Next: Frameworks
- Last Updated: Feb 22, 2024 12:34 PM
- URL: https://uow.libguides.com/systematic-review
Insert research help text here
LIBRARY RESOURCES
Library homepage
Library SEARCH
A-Z Databases
STUDY SUPPORT
Academic Skills Centre
Referencing and citing
Digital Skills Hub
MORE UOW SERVICES
UOW homepage
Student support and wellbeing
IT Services
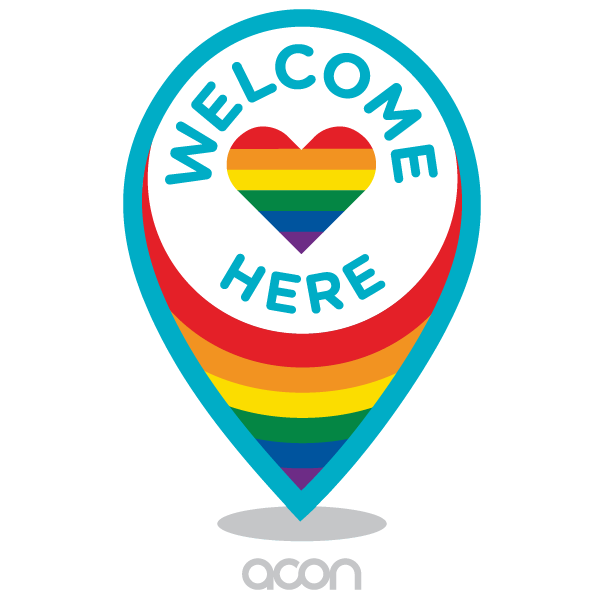
On the lands that we study, we walk, and we live, we acknowledge and respect the traditional custodians and cultural knowledge holders of these lands.
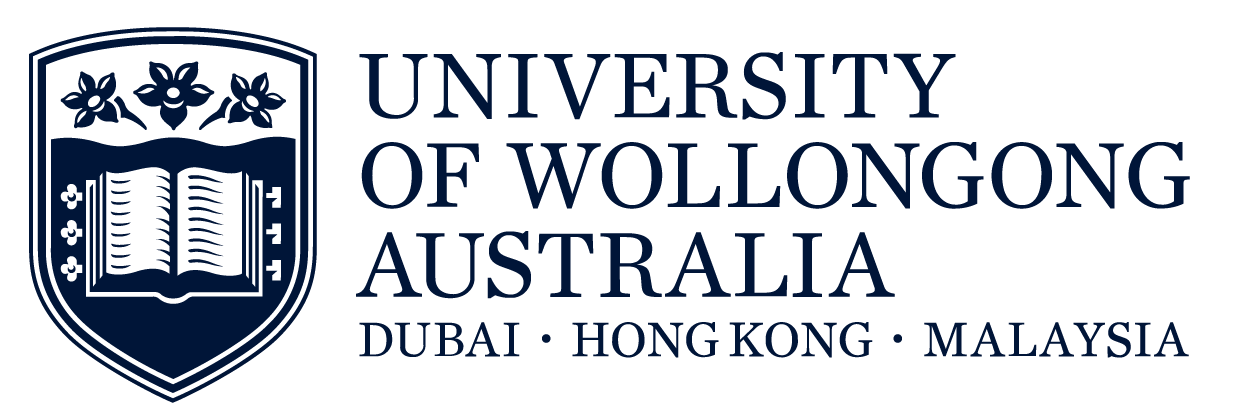
Copyright & disclaimer | Privacy & cookie usage
Have a language expert improve your writing
Run a free plagiarism check in 10 minutes, generate accurate citations for free.
- Knowledge Base
Methodology
- Systematic Review | Definition, Example, & Guide
Systematic Review | Definition, Example & Guide
Published on June 15, 2022 by Shaun Turney . Revised on November 20, 2023.
A systematic review is a type of review that uses repeatable methods to find, select, and synthesize all available evidence. It answers a clearly formulated research question and explicitly states the methods used to arrive at the answer.
They answered the question “What is the effectiveness of probiotics in reducing eczema symptoms and improving quality of life in patients with eczema?”
In this context, a probiotic is a health product that contains live microorganisms and is taken by mouth. Eczema is a common skin condition that causes red, itchy skin.
Table of contents
What is a systematic review, systematic review vs. meta-analysis, systematic review vs. literature review, systematic review vs. scoping review, when to conduct a systematic review, pros and cons of systematic reviews, step-by-step example of a systematic review, other interesting articles, frequently asked questions about systematic reviews.
A review is an overview of the research that’s already been completed on a topic.
What makes a systematic review different from other types of reviews is that the research methods are designed to reduce bias . The methods are repeatable, and the approach is formal and systematic:
- Formulate a research question
- Develop a protocol
- Search for all relevant studies
- Apply the selection criteria
- Extract the data
- Synthesize the data
- Write and publish a report
Although multiple sets of guidelines exist, the Cochrane Handbook for Systematic Reviews is among the most widely used. It provides detailed guidelines on how to complete each step of the systematic review process.
Systematic reviews are most commonly used in medical and public health research, but they can also be found in other disciplines.
Systematic reviews typically answer their research question by synthesizing all available evidence and evaluating the quality of the evidence. Synthesizing means bringing together different information to tell a single, cohesive story. The synthesis can be narrative ( qualitative ), quantitative , or both.
Here's why students love Scribbr's proofreading services
Discover proofreading & editing
Systematic reviews often quantitatively synthesize the evidence using a meta-analysis . A meta-analysis is a statistical analysis, not a type of review.
A meta-analysis is a technique to synthesize results from multiple studies. It’s a statistical analysis that combines the results of two or more studies, usually to estimate an effect size .
A literature review is a type of review that uses a less systematic and formal approach than a systematic review. Typically, an expert in a topic will qualitatively summarize and evaluate previous work, without using a formal, explicit method.
Although literature reviews are often less time-consuming and can be insightful or helpful, they have a higher risk of bias and are less transparent than systematic reviews.
Similar to a systematic review, a scoping review is a type of review that tries to minimize bias by using transparent and repeatable methods.
However, a scoping review isn’t a type of systematic review. The most important difference is the goal: rather than answering a specific question, a scoping review explores a topic. The researcher tries to identify the main concepts, theories, and evidence, as well as gaps in the current research.
Sometimes scoping reviews are an exploratory preparation step for a systematic review, and sometimes they are a standalone project.
A systematic review is a good choice of review if you want to answer a question about the effectiveness of an intervention , such as a medical treatment.
To conduct a systematic review, you’ll need the following:
- A precise question , usually about the effectiveness of an intervention. The question needs to be about a topic that’s previously been studied by multiple researchers. If there’s no previous research, there’s nothing to review.
- If you’re doing a systematic review on your own (e.g., for a research paper or thesis ), you should take appropriate measures to ensure the validity and reliability of your research.
- Access to databases and journal archives. Often, your educational institution provides you with access.
- Time. A professional systematic review is a time-consuming process: it will take the lead author about six months of full-time work. If you’re a student, you should narrow the scope of your systematic review and stick to a tight schedule.
- Bibliographic, word-processing, spreadsheet, and statistical software . For example, you could use EndNote, Microsoft Word, Excel, and SPSS.
A systematic review has many pros .
- They minimize research bias by considering all available evidence and evaluating each study for bias.
- Their methods are transparent , so they can be scrutinized by others.
- They’re thorough : they summarize all available evidence.
- They can be replicated and updated by others.
Systematic reviews also have a few cons .
- They’re time-consuming .
- They’re narrow in scope : they only answer the precise research question.
The 7 steps for conducting a systematic review are explained with an example.
Step 1: Formulate a research question
Formulating the research question is probably the most important step of a systematic review. A clear research question will:
- Allow you to more effectively communicate your research to other researchers and practitioners
- Guide your decisions as you plan and conduct your systematic review
A good research question for a systematic review has four components, which you can remember with the acronym PICO :
- Population(s) or problem(s)
- Intervention(s)
- Comparison(s)
You can rearrange these four components to write your research question:
- What is the effectiveness of I versus C for O in P ?
Sometimes, you may want to include a fifth component, the type of study design . In this case, the acronym is PICOT .
- Type of study design(s)
- The population of patients with eczema
- The intervention of probiotics
- In comparison to no treatment, placebo , or non-probiotic treatment
- The outcome of changes in participant-, parent-, and doctor-rated symptoms of eczema and quality of life
- Randomized control trials, a type of study design
Their research question was:
- What is the effectiveness of probiotics versus no treatment, a placebo, or a non-probiotic treatment for reducing eczema symptoms and improving quality of life in patients with eczema?
Step 2: Develop a protocol
A protocol is a document that contains your research plan for the systematic review. This is an important step because having a plan allows you to work more efficiently and reduces bias.
Your protocol should include the following components:
- Background information : Provide the context of the research question, including why it’s important.
- Research objective (s) : Rephrase your research question as an objective.
- Selection criteria: State how you’ll decide which studies to include or exclude from your review.
- Search strategy: Discuss your plan for finding studies.
- Analysis: Explain what information you’ll collect from the studies and how you’ll synthesize the data.
If you’re a professional seeking to publish your review, it’s a good idea to bring together an advisory committee . This is a group of about six people who have experience in the topic you’re researching. They can help you make decisions about your protocol.
It’s highly recommended to register your protocol. Registering your protocol means submitting it to a database such as PROSPERO or ClinicalTrials.gov .
Step 3: Search for all relevant studies
Searching for relevant studies is the most time-consuming step of a systematic review.
To reduce bias, it’s important to search for relevant studies very thoroughly. Your strategy will depend on your field and your research question, but sources generally fall into these four categories:
- Databases: Search multiple databases of peer-reviewed literature, such as PubMed or Scopus . Think carefully about how to phrase your search terms and include multiple synonyms of each word. Use Boolean operators if relevant.
- Handsearching: In addition to searching the primary sources using databases, you’ll also need to search manually. One strategy is to scan relevant journals or conference proceedings. Another strategy is to scan the reference lists of relevant studies.
- Gray literature: Gray literature includes documents produced by governments, universities, and other institutions that aren’t published by traditional publishers. Graduate student theses are an important type of gray literature, which you can search using the Networked Digital Library of Theses and Dissertations (NDLTD) . In medicine, clinical trial registries are another important type of gray literature.
- Experts: Contact experts in the field to ask if they have unpublished studies that should be included in your review.
At this stage of your review, you won’t read the articles yet. Simply save any potentially relevant citations using bibliographic software, such as Scribbr’s APA or MLA Generator .
- Databases: EMBASE, PsycINFO, AMED, LILACS, and ISI Web of Science
- Handsearch: Conference proceedings and reference lists of articles
- Gray literature: The Cochrane Library, the metaRegister of Controlled Trials, and the Ongoing Skin Trials Register
- Experts: Authors of unpublished registered trials, pharmaceutical companies, and manufacturers of probiotics
Step 4: Apply the selection criteria
Applying the selection criteria is a three-person job. Two of you will independently read the studies and decide which to include in your review based on the selection criteria you established in your protocol . The third person’s job is to break any ties.
To increase inter-rater reliability , ensure that everyone thoroughly understands the selection criteria before you begin.
If you’re writing a systematic review as a student for an assignment, you might not have a team. In this case, you’ll have to apply the selection criteria on your own; you can mention this as a limitation in your paper’s discussion.
You should apply the selection criteria in two phases:
- Based on the titles and abstracts : Decide whether each article potentially meets the selection criteria based on the information provided in the abstracts.
- Based on the full texts: Download the articles that weren’t excluded during the first phase. If an article isn’t available online or through your library, you may need to contact the authors to ask for a copy. Read the articles and decide which articles meet the selection criteria.
It’s very important to keep a meticulous record of why you included or excluded each article. When the selection process is complete, you can summarize what you did using a PRISMA flow diagram .
Next, Boyle and colleagues found the full texts for each of the remaining studies. Boyle and Tang read through the articles to decide if any more studies needed to be excluded based on the selection criteria.
When Boyle and Tang disagreed about whether a study should be excluded, they discussed it with Varigos until the three researchers came to an agreement.
Step 5: Extract the data
Extracting the data means collecting information from the selected studies in a systematic way. There are two types of information you need to collect from each study:
- Information about the study’s methods and results . The exact information will depend on your research question, but it might include the year, study design , sample size, context, research findings , and conclusions. If any data are missing, you’ll need to contact the study’s authors.
- Your judgment of the quality of the evidence, including risk of bias .
You should collect this information using forms. You can find sample forms in The Registry of Methods and Tools for Evidence-Informed Decision Making and the Grading of Recommendations, Assessment, Development and Evaluations Working Group .
Extracting the data is also a three-person job. Two people should do this step independently, and the third person will resolve any disagreements.
They also collected data about possible sources of bias, such as how the study participants were randomized into the control and treatment groups.
Step 6: Synthesize the data
Synthesizing the data means bringing together the information you collected into a single, cohesive story. There are two main approaches to synthesizing the data:
- Narrative ( qualitative ): Summarize the information in words. You’ll need to discuss the studies and assess their overall quality.
- Quantitative : Use statistical methods to summarize and compare data from different studies. The most common quantitative approach is a meta-analysis , which allows you to combine results from multiple studies into a summary result.
Generally, you should use both approaches together whenever possible. If you don’t have enough data, or the data from different studies aren’t comparable, then you can take just a narrative approach. However, you should justify why a quantitative approach wasn’t possible.
Boyle and colleagues also divided the studies into subgroups, such as studies about babies, children, and adults, and analyzed the effect sizes within each group.
Step 7: Write and publish a report
The purpose of writing a systematic review article is to share the answer to your research question and explain how you arrived at this answer.
Your article should include the following sections:
- Abstract : A summary of the review
- Introduction : Including the rationale and objectives
- Methods : Including the selection criteria, search method, data extraction method, and synthesis method
- Results : Including results of the search and selection process, study characteristics, risk of bias in the studies, and synthesis results
- Discussion : Including interpretation of the results and limitations of the review
- Conclusion : The answer to your research question and implications for practice, policy, or research
To verify that your report includes everything it needs, you can use the PRISMA checklist .
Once your report is written, you can publish it in a systematic review database, such as the Cochrane Database of Systematic Reviews , and/or in a peer-reviewed journal.
In their report, Boyle and colleagues concluded that probiotics cannot be recommended for reducing eczema symptoms or improving quality of life in patients with eczema. Note Generative AI tools like ChatGPT can be useful at various stages of the writing and research process and can help you to write your systematic review. However, we strongly advise against trying to pass AI-generated text off as your own work.
If you want to know more about statistics , methodology , or research bias , make sure to check out some of our other articles with explanations and examples.
- Student’s t -distribution
- Normal distribution
- Null and Alternative Hypotheses
- Chi square tests
- Confidence interval
- Quartiles & Quantiles
- Cluster sampling
- Stratified sampling
- Data cleansing
- Reproducibility vs Replicability
- Peer review
- Prospective cohort study
Research bias
- Implicit bias
- Cognitive bias
- Placebo effect
- Hawthorne effect
- Hindsight bias
- Affect heuristic
- Social desirability bias
A literature review is a survey of scholarly sources (such as books, journal articles, and theses) related to a specific topic or research question .
It is often written as part of a thesis, dissertation , or research paper , in order to situate your work in relation to existing knowledge.
A literature review is a survey of credible sources on a topic, often used in dissertations , theses, and research papers . Literature reviews give an overview of knowledge on a subject, helping you identify relevant theories and methods, as well as gaps in existing research. Literature reviews are set up similarly to other academic texts , with an introduction , a main body, and a conclusion .
An annotated bibliography is a list of source references that has a short description (called an annotation ) for each of the sources. It is often assigned as part of the research process for a paper .
A systematic review is secondary research because it uses existing research. You don’t collect new data yourself.
Cite this Scribbr article
If you want to cite this source, you can copy and paste the citation or click the “Cite this Scribbr article” button to automatically add the citation to our free Citation Generator.
Turney, S. (2023, November 20). Systematic Review | Definition, Example & Guide. Scribbr. Retrieved April 15, 2024, from https://www.scribbr.com/methodology/systematic-review/
Is this article helpful?
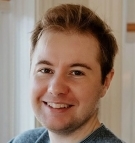
Shaun Turney
Other students also liked, how to write a literature review | guide, examples, & templates, how to write a research proposal | examples & templates, what is critical thinking | definition & examples, unlimited academic ai-proofreading.
✔ Document error-free in 5minutes ✔ Unlimited document corrections ✔ Specialized in correcting academic texts
Writing in the Health and Social Sciences: Literature Reviews and Synthesis Tools
- Journal Publishing
- Style and Writing Guides
- Readings about Writing
- Citing in APA Style This link opens in a new window
- Resources for Dissertation Authors
- Citation Management and Formatting Tools
- What are Literature Reviews?
- Conducting & Reporting Systematic Reviews
- Finding Systematic Reviews
- Tutorials & Tools for Literature Reviews
Systematic Literature Reviews: Steps & Resources
These steps for conducting a systematic literature review are listed below .
Also see subpages for more information about:
- The different types of literature reviews, including systematic reviews and other evidence synthesis methods
- Tools & Tutorials
Literature Review & Systematic Review Steps
- Develop a Focused Question
- Scope the Literature (Initial Search)
- Refine & Expand the Search
- Limit the Results
- Download Citations
- Abstract & Analyze
- Create Flow Diagram
- Synthesize & Report Results
1. Develop a Focused Question
Consider the PICO Format: Population/Problem, Intervention, Comparison, Outcome
Focus on defining the Population or Problem and Intervention (don't narrow by Comparison or Outcome just yet!)
"What are the effects of the Pilates method for patients with low back pain?"
Tools & Additional Resources:
- PICO Question Help
- Stillwell, Susan B., DNP, RN, CNE; Fineout-Overholt, Ellen, PhD, RN, FNAP, FAAN; Melnyk, Bernadette Mazurek, PhD, RN, CPNP/PMHNP, FNAP, FAAN; Williamson, Kathleen M., PhD, RN Evidence-Based Practice, Step by Step: Asking the Clinical Question, AJN The American Journal of Nursing : March 2010 - Volume 110 - Issue 3 - p 58-61 doi: 10.1097/01.NAJ.0000368959.11129.79
2. Scope the Literature
A "scoping search" investigates the breadth and/or depth of the initial question or may identify a gap in the literature.
Eligible studies may be located by searching in:
- Background sources (books, point-of-care tools)
- Article databases
- Trial registries
- Grey literature
- Cited references
- Reference lists
When searching, if possible, translate terms to controlled vocabulary of the database. Use text word searching when necessary.
Use Boolean operators to connect search terms:
- Combine separate concepts with AND (resulting in a narrower search)
- Connecting synonyms with OR (resulting in an expanded search)
Search: pilates AND ("low back pain" OR backache )
Video Tutorials - Translating PICO Questions into Search Queries
- Translate Your PICO Into a Search in PubMed (YouTube, Carrie Price, 5:11)
- Translate Your PICO Into a Search in CINAHL (YouTube, Carrie Price, 4:56)
3. Refine & Expand Your Search
Expand your search strategy with synonymous search terms harvested from:
- database thesauri
- reference lists
- relevant studies
Example:
(pilates OR exercise movement techniques) AND ("low back pain" OR backache* OR sciatica OR lumbago OR spondylosis)
As you develop a final, reproducible strategy for each database, save your strategies in a:
- a personal database account (e.g., MyNCBI for PubMed)
- Log in with your NYU credentials
- Open and "Make a Copy" to create your own tracker for your literature search strategies
4. Limit Your Results
Use database filters to limit your results based on your defined inclusion/exclusion criteria. In addition to relying on the databases' categorical filters, you may also need to manually screen results.
- Limit to Article type, e.g.,: "randomized controlled trial" OR multicenter study
- Limit by publication years, age groups, language, etc.
NOTE: Many databases allow you to filter to "Full Text Only". This filter is not recommended . It excludes articles if their full text is not available in that particular database (CINAHL, PubMed, etc), but if the article is relevant, it is important that you are able to read its title and abstract, regardless of 'full text' status. The full text is likely to be accessible through another source (a different database, or Interlibrary Loan).
- Filters in PubMed
- CINAHL Advanced Searching Tutorial
5. Download Citations
Selected citations and/or entire sets of search results can be downloaded from the database into a citation management tool. If you are conducting a systematic review that will require reporting according to PRISMA standards, a citation manager can help you keep track of the number of articles that came from each database, as well as the number of duplicate records.
In Zotero, you can create a Collection for the combined results set, and sub-collections for the results from each database you search. You can then use Zotero's 'Duplicate Items" function to find and merge duplicate records.

- Citation Managers - General Guide
6. Abstract and Analyze
- Migrate citations to data collection/extraction tool
- Screen Title/Abstracts for inclusion/exclusion
- Screen and appraise full text for relevance, methods,
- Resolve disagreements by consensus
Covidence is a web-based tool that enables you to work with a team to screen titles/abstracts and full text for inclusion in your review, as well as extract data from the included studies.

- Covidence Support
- Critical Appraisal Tools
- Data Extraction Tools
7. Create Flow Diagram
The PRISMA (Preferred Reporting Items for Systematic reviews and Meta-Analyses) flow diagram is a visual representation of the flow of records through different phases of a systematic review. It depicts the number of records identified, included and excluded. It is best used in conjunction with the PRISMA checklist .

Example from: Stotz, S. A., McNealy, K., Begay, R. L., DeSanto, K., Manson, S. M., & Moore, K. R. (2021). Multi-level diabetes prevention and treatment interventions for Native people in the USA and Canada: A scoping review. Current Diabetes Reports, 2 (11), 46. https://doi.org/10.1007/s11892-021-01414-3
- PRISMA Flow Diagram Generator (ShinyApp.io, Haddaway et al. )
- PRISMA Diagram Templates (Word and PDF)
- Make a copy of the file to fill out the template
- Image can be downloaded as PDF, PNG, JPG, or SVG
- Covidence generates a PRISMA diagram that is automatically updated as records move through the review phases
8. Synthesize & Report Results
There are a number of reporting guideline available to guide the synthesis and reporting of results in systematic literature reviews.
It is common to organize findings in a matrix, also known as a Table of Evidence (ToE).
- Reporting Guidelines for Systematic Reviews
- Download a sample template of a health sciences review matrix (GoogleSheets)
Steps modified from:
Cook, D. A., & West, C. P. (2012). Conducting systematic reviews in medical education: a stepwise approach. Medical Education , 46 (10), 943–952.
- << Previous: Citation Management and Formatting Tools
- Next: What are Literature Reviews? >>
- Last Updated: Apr 13, 2024 9:10 PM
- URL: https://guides.nyu.edu/healthwriting

An official website of the United States government
The .gov means it’s official. Federal government websites often end in .gov or .mil. Before sharing sensitive information, make sure you’re on a federal government site.
The site is secure. The https:// ensures that you are connecting to the official website and that any information you provide is encrypted and transmitted securely.
- Publications
- Account settings
Preview improvements coming to the PMC website in October 2024. Learn More or Try it out now .
- Advanced Search
- Journal List
- Korean J Anesthesiol
- v.71(2); 2018 Apr
Introduction to systematic review and meta-analysis
1 Department of Anesthesiology and Pain Medicine, Inje University Seoul Paik Hospital, Seoul, Korea
2 Department of Anesthesiology and Pain Medicine, Chung-Ang University College of Medicine, Seoul, Korea
Systematic reviews and meta-analyses present results by combining and analyzing data from different studies conducted on similar research topics. In recent years, systematic reviews and meta-analyses have been actively performed in various fields including anesthesiology. These research methods are powerful tools that can overcome the difficulties in performing large-scale randomized controlled trials. However, the inclusion of studies with any biases or improperly assessed quality of evidence in systematic reviews and meta-analyses could yield misleading results. Therefore, various guidelines have been suggested for conducting systematic reviews and meta-analyses to help standardize them and improve their quality. Nonetheless, accepting the conclusions of many studies without understanding the meta-analysis can be dangerous. Therefore, this article provides an easy introduction to clinicians on performing and understanding meta-analyses.
Introduction
A systematic review collects all possible studies related to a given topic and design, and reviews and analyzes their results [ 1 ]. During the systematic review process, the quality of studies is evaluated, and a statistical meta-analysis of the study results is conducted on the basis of their quality. A meta-analysis is a valid, objective, and scientific method of analyzing and combining different results. Usually, in order to obtain more reliable results, a meta-analysis is mainly conducted on randomized controlled trials (RCTs), which have a high level of evidence [ 2 ] ( Fig. 1 ). Since 1999, various papers have presented guidelines for reporting meta-analyses of RCTs. Following the Quality of Reporting of Meta-analyses (QUORUM) statement [ 3 ], and the appearance of registers such as Cochrane Library’s Methodology Register, a large number of systematic literature reviews have been registered. In 2009, the Preferred Reporting Items for Systematic Reviews and Meta-Analyses (PRISMA) statement [ 4 ] was published, and it greatly helped standardize and improve the quality of systematic reviews and meta-analyses [ 5 ].

Levels of evidence.
In anesthesiology, the importance of systematic reviews and meta-analyses has been highlighted, and they provide diagnostic and therapeutic value to various areas, including not only perioperative management but also intensive care and outpatient anesthesia [6–13]. Systematic reviews and meta-analyses include various topics, such as comparing various treatments of postoperative nausea and vomiting [ 14 , 15 ], comparing general anesthesia and regional anesthesia [ 16 – 18 ], comparing airway maintenance devices [ 8 , 19 ], comparing various methods of postoperative pain control (e.g., patient-controlled analgesia pumps, nerve block, or analgesics) [ 20 – 23 ], comparing the precision of various monitoring instruments [ 7 ], and meta-analysis of dose-response in various drugs [ 12 ].
Thus, literature reviews and meta-analyses are being conducted in diverse medical fields, and the aim of highlighting their importance is to help better extract accurate, good quality data from the flood of data being produced. However, a lack of understanding about systematic reviews and meta-analyses can lead to incorrect outcomes being derived from the review and analysis processes. If readers indiscriminately accept the results of the many meta-analyses that are published, incorrect data may be obtained. Therefore, in this review, we aim to describe the contents and methods used in systematic reviews and meta-analyses in a way that is easy to understand for future authors and readers of systematic review and meta-analysis.
Study Planning
It is easy to confuse systematic reviews and meta-analyses. A systematic review is an objective, reproducible method to find answers to a certain research question, by collecting all available studies related to that question and reviewing and analyzing their results. A meta-analysis differs from a systematic review in that it uses statistical methods on estimates from two or more different studies to form a pooled estimate [ 1 ]. Following a systematic review, if it is not possible to form a pooled estimate, it can be published as is without progressing to a meta-analysis; however, if it is possible to form a pooled estimate from the extracted data, a meta-analysis can be attempted. Systematic reviews and meta-analyses usually proceed according to the flowchart presented in Fig. 2 . We explain each of the stages below.

Flowchart illustrating a systematic review.
Formulating research questions
A systematic review attempts to gather all available empirical research by using clearly defined, systematic methods to obtain answers to a specific question. A meta-analysis is the statistical process of analyzing and combining results from several similar studies. Here, the definition of the word “similar” is not made clear, but when selecting a topic for the meta-analysis, it is essential to ensure that the different studies present data that can be combined. If the studies contain data on the same topic that can be combined, a meta-analysis can even be performed using data from only two studies. However, study selection via a systematic review is a precondition for performing a meta-analysis, and it is important to clearly define the Population, Intervention, Comparison, Outcomes (PICO) parameters that are central to evidence-based research. In addition, selection of the research topic is based on logical evidence, and it is important to select a topic that is familiar to readers without clearly confirmed the evidence [ 24 ].
Protocols and registration
In systematic reviews, prior registration of a detailed research plan is very important. In order to make the research process transparent, primary/secondary outcomes and methods are set in advance, and in the event of changes to the method, other researchers and readers are informed when, how, and why. Many studies are registered with an organization like PROSPERO ( http://www.crd.york.ac.uk/PROSPERO/ ), and the registration number is recorded when reporting the study, in order to share the protocol at the time of planning.
Defining inclusion and exclusion criteria
Information is included on the study design, patient characteristics, publication status (published or unpublished), language used, and research period. If there is a discrepancy between the number of patients included in the study and the number of patients included in the analysis, this needs to be clearly explained while describing the patient characteristics, to avoid confusing the reader.
Literature search and study selection
In order to secure proper basis for evidence-based research, it is essential to perform a broad search that includes as many studies as possible that meet the inclusion and exclusion criteria. Typically, the three bibliographic databases Medline, Embase, and Cochrane Central Register of Controlled Trials (CENTRAL) are used. In domestic studies, the Korean databases KoreaMed, KMBASE, and RISS4U may be included. Effort is required to identify not only published studies but also abstracts, ongoing studies, and studies awaiting publication. Among the studies retrieved in the search, the researchers remove duplicate studies, select studies that meet the inclusion/exclusion criteria based on the abstracts, and then make the final selection of studies based on their full text. In order to maintain transparency and objectivity throughout this process, study selection is conducted independently by at least two investigators. When there is a inconsistency in opinions, intervention is required via debate or by a third reviewer. The methods for this process also need to be planned in advance. It is essential to ensure the reproducibility of the literature selection process [ 25 ].
Quality of evidence
However, well planned the systematic review or meta-analysis is, if the quality of evidence in the studies is low, the quality of the meta-analysis decreases and incorrect results can be obtained [ 26 ]. Even when using randomized studies with a high quality of evidence, evaluating the quality of evidence precisely helps determine the strength of recommendations in the meta-analysis. One method of evaluating the quality of evidence in non-randomized studies is the Newcastle-Ottawa Scale, provided by the Ottawa Hospital Research Institute 1) . However, we are mostly focusing on meta-analyses that use randomized studies.
If the Grading of Recommendations, Assessment, Development and Evaluations (GRADE) system ( http://www.gradeworkinggroup.org/ ) is used, the quality of evidence is evaluated on the basis of the study limitations, inaccuracies, incompleteness of outcome data, indirectness of evidence, and risk of publication bias, and this is used to determine the strength of recommendations [ 27 ]. As shown in Table 1 , the study limitations are evaluated using the “risk of bias” method proposed by Cochrane 2) . This method classifies bias in randomized studies as “low,” “high,” or “unclear” on the basis of the presence or absence of six processes (random sequence generation, allocation concealment, blinding participants or investigators, incomplete outcome data, selective reporting, and other biases) [ 28 ].
The Cochrane Collaboration’s Tool for Assessing the Risk of Bias [ 28 ]
Data extraction
Two different investigators extract data based on the objectives and form of the study; thereafter, the extracted data are reviewed. Since the size and format of each variable are different, the size and format of the outcomes are also different, and slight changes may be required when combining the data [ 29 ]. If there are differences in the size and format of the outcome variables that cause difficulties combining the data, such as the use of different evaluation instruments or different evaluation timepoints, the analysis may be limited to a systematic review. The investigators resolve differences of opinion by debate, and if they fail to reach a consensus, a third-reviewer is consulted.
Data Analysis
The aim of a meta-analysis is to derive a conclusion with increased power and accuracy than what could not be able to achieve in individual studies. Therefore, before analysis, it is crucial to evaluate the direction of effect, size of effect, homogeneity of effects among studies, and strength of evidence [ 30 ]. Thereafter, the data are reviewed qualitatively and quantitatively. If it is determined that the different research outcomes cannot be combined, all the results and characteristics of the individual studies are displayed in a table or in a descriptive form; this is referred to as a qualitative review. A meta-analysis is a quantitative review, in which the clinical effectiveness is evaluated by calculating the weighted pooled estimate for the interventions in at least two separate studies.
The pooled estimate is the outcome of the meta-analysis, and is typically explained using a forest plot ( Figs. 3 and and4). 4 ). The black squares in the forest plot are the odds ratios (ORs) and 95% confidence intervals in each study. The area of the squares represents the weight reflected in the meta-analysis. The black diamond represents the OR and 95% confidence interval calculated across all the included studies. The bold vertical line represents a lack of therapeutic effect (OR = 1); if the confidence interval includes OR = 1, it means no significant difference was found between the treatment and control groups.

Forest plot analyzed by two different models using the same data. (A) Fixed-effect model. (B) Random-effect model. The figure depicts individual trials as filled squares with the relative sample size and the solid line as the 95% confidence interval of the difference. The diamond shape indicates the pooled estimate and uncertainty for the combined effect. The vertical line indicates the treatment group shows no effect (OR = 1). Moreover, if the confidence interval includes 1, then the result shows no evidence of difference between the treatment and control groups.

Forest plot representing homogeneous data.
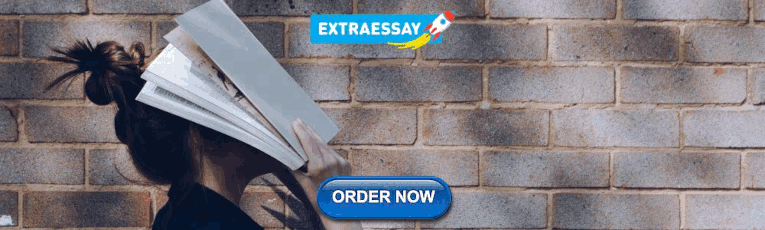
Dichotomous variables and continuous variables
In data analysis, outcome variables can be considered broadly in terms of dichotomous variables and continuous variables. When combining data from continuous variables, the mean difference (MD) and standardized mean difference (SMD) are used ( Table 2 ).
Summary of Meta-analysis Methods Available in RevMan [ 28 ]
The MD is the absolute difference in mean values between the groups, and the SMD is the mean difference between groups divided by the standard deviation. When results are presented in the same units, the MD can be used, but when results are presented in different units, the SMD should be used. When the MD is used, the combined units must be shown. A value of “0” for the MD or SMD indicates that the effects of the new treatment method and the existing treatment method are the same. A value lower than “0” means the new treatment method is less effective than the existing method, and a value greater than “0” means the new treatment is more effective than the existing method.
When combining data for dichotomous variables, the OR, risk ratio (RR), or risk difference (RD) can be used. The RR and RD can be used for RCTs, quasi-experimental studies, or cohort studies, and the OR can be used for other case-control studies or cross-sectional studies. However, because the OR is difficult to interpret, using the RR and RD, if possible, is recommended. If the outcome variable is a dichotomous variable, it can be presented as the number needed to treat (NNT), which is the minimum number of patients who need to be treated in the intervention group, compared to the control group, for a given event to occur in at least one patient. Based on Table 3 , in an RCT, if x is the probability of the event occurring in the control group and y is the probability of the event occurring in the intervention group, then x = c/(c + d), y = a/(a + b), and the absolute risk reduction (ARR) = x − y. NNT can be obtained as the reciprocal, 1/ARR.
Calculation of the Number Needed to Treat in the Dichotomous table
Fixed-effect models and random-effect models
In order to analyze effect size, two types of models can be used: a fixed-effect model or a random-effect model. A fixed-effect model assumes that the effect of treatment is the same, and that variation between results in different studies is due to random error. Thus, a fixed-effect model can be used when the studies are considered to have the same design and methodology, or when the variability in results within a study is small, and the variance is thought to be due to random error. Three common methods are used for weighted estimation in a fixed-effect model: 1) inverse variance-weighted estimation 3) , 2) Mantel-Haenszel estimation 4) , and 3) Peto estimation 5) .
A random-effect model assumes heterogeneity between the studies being combined, and these models are used when the studies are assumed different, even if a heterogeneity test does not show a significant result. Unlike a fixed-effect model, a random-effect model assumes that the size of the effect of treatment differs among studies. Thus, differences in variation among studies are thought to be due to not only random error but also between-study variability in results. Therefore, weight does not decrease greatly for studies with a small number of patients. Among methods for weighted estimation in a random-effect model, the DerSimonian and Laird method 6) is mostly used for dichotomous variables, as the simplest method, while inverse variance-weighted estimation is used for continuous variables, as with fixed-effect models. These four methods are all used in Review Manager software (The Cochrane Collaboration, UK), and are described in a study by Deeks et al. [ 31 ] ( Table 2 ). However, when the number of studies included in the analysis is less than 10, the Hartung-Knapp-Sidik-Jonkman method 7) can better reduce the risk of type 1 error than does the DerSimonian and Laird method [ 32 ].
Fig. 3 shows the results of analyzing outcome data using a fixed-effect model (A) and a random-effect model (B). As shown in Fig. 3 , while the results from large studies are weighted more heavily in the fixed-effect model, studies are given relatively similar weights irrespective of study size in the random-effect model. Although identical data were being analyzed, as shown in Fig. 3 , the significant result in the fixed-effect model was no longer significant in the random-effect model. One representative example of the small study effect in a random-effect model is the meta-analysis by Li et al. [ 33 ]. In a large-scale study, intravenous injection of magnesium was unrelated to acute myocardial infarction, but in the random-effect model, which included numerous small studies, the small study effect resulted in an association being found between intravenous injection of magnesium and myocardial infarction. This small study effect can be controlled for by using a sensitivity analysis, which is performed to examine the contribution of each of the included studies to the final meta-analysis result. In particular, when heterogeneity is suspected in the study methods or results, by changing certain data or analytical methods, this method makes it possible to verify whether the changes affect the robustness of the results, and to examine the causes of such effects [ 34 ].
Heterogeneity
Homogeneity test is a method whether the degree of heterogeneity is greater than would be expected to occur naturally when the effect size calculated from several studies is higher than the sampling error. This makes it possible to test whether the effect size calculated from several studies is the same. Three types of homogeneity tests can be used: 1) forest plot, 2) Cochrane’s Q test (chi-squared), and 3) Higgins I 2 statistics. In the forest plot, as shown in Fig. 4 , greater overlap between the confidence intervals indicates greater homogeneity. For the Q statistic, when the P value of the chi-squared test, calculated from the forest plot in Fig. 4 , is less than 0.1, it is considered to show statistical heterogeneity and a random-effect can be used. Finally, I 2 can be used [ 35 ].
I 2 , calculated as shown above, returns a value between 0 and 100%. A value less than 25% is considered to show strong homogeneity, a value of 50% is average, and a value greater than 75% indicates strong heterogeneity.
Even when the data cannot be shown to be homogeneous, a fixed-effect model can be used, ignoring the heterogeneity, and all the study results can be presented individually, without combining them. However, in many cases, a random-effect model is applied, as described above, and a subgroup analysis or meta-regression analysis is performed to explain the heterogeneity. In a subgroup analysis, the data are divided into subgroups that are expected to be homogeneous, and these subgroups are analyzed. This needs to be planned in the predetermined protocol before starting the meta-analysis. A meta-regression analysis is similar to a normal regression analysis, except that the heterogeneity between studies is modeled. This process involves performing a regression analysis of the pooled estimate for covariance at the study level, and so it is usually not considered when the number of studies is less than 10. Here, univariate and multivariate regression analyses can both be considered.
Publication bias
Publication bias is the most common type of reporting bias in meta-analyses. This refers to the distortion of meta-analysis outcomes due to the higher likelihood of publication of statistically significant studies rather than non-significant studies. In order to test the presence or absence of publication bias, first, a funnel plot can be used ( Fig. 5 ). Studies are plotted on a scatter plot with effect size on the x-axis and precision or total sample size on the y-axis. If the points form an upside-down funnel shape, with a broad base that narrows towards the top of the plot, this indicates the absence of a publication bias ( Fig. 5A ) [ 29 , 36 ]. On the other hand, if the plot shows an asymmetric shape, with no points on one side of the graph, then publication bias can be suspected ( Fig. 5B ). Second, to test publication bias statistically, Begg and Mazumdar’s rank correlation test 8) [ 37 ] or Egger’s test 9) [ 29 ] can be used. If publication bias is detected, the trim-and-fill method 10) can be used to correct the bias [ 38 ]. Fig. 6 displays results that show publication bias in Egger’s test, which has then been corrected using the trim-and-fill method using Comprehensive Meta-Analysis software (Biostat, USA).

Funnel plot showing the effect size on the x-axis and sample size on the y-axis as a scatter plot. (A) Funnel plot without publication bias. The individual plots are broader at the bottom and narrower at the top. (B) Funnel plot with publication bias. The individual plots are located asymmetrically.

Funnel plot adjusted using the trim-and-fill method. White circles: comparisons included. Black circles: inputted comparisons using the trim-and-fill method. White diamond: pooled observed log risk ratio. Black diamond: pooled inputted log risk ratio.
Result Presentation
When reporting the results of a systematic review or meta-analysis, the analytical content and methods should be described in detail. First, a flowchart is displayed with the literature search and selection process according to the inclusion/exclusion criteria. Second, a table is shown with the characteristics of the included studies. A table should also be included with information related to the quality of evidence, such as GRADE ( Table 4 ). Third, the results of data analysis are shown in a forest plot and funnel plot. Fourth, if the results use dichotomous data, the NNT values can be reported, as described above.
The GRADE Evidence Quality for Each Outcome
N: number of studies, ROB: risk of bias, PON: postoperative nausea, POV: postoperative vomiting, PONV: postoperative nausea and vomiting, CI: confidence interval, RR: risk ratio, AR: absolute risk.
When Review Manager software (The Cochrane Collaboration, UK) is used for the analysis, two types of P values are given. The first is the P value from the z-test, which tests the null hypothesis that the intervention has no effect. The second P value is from the chi-squared test, which tests the null hypothesis for a lack of heterogeneity. The statistical result for the intervention effect, which is generally considered the most important result in meta-analyses, is the z-test P value.
A common mistake when reporting results is, given a z-test P value greater than 0.05, to say there was “no statistical significance” or “no difference.” When evaluating statistical significance in a meta-analysis, a P value lower than 0.05 can be explained as “a significant difference in the effects of the two treatment methods.” However, the P value may appear non-significant whether or not there is a difference between the two treatment methods. In such a situation, it is better to announce “there was no strong evidence for an effect,” and to present the P value and confidence intervals. Another common mistake is to think that a smaller P value is indicative of a more significant effect. In meta-analyses of large-scale studies, the P value is more greatly affected by the number of studies and patients included, rather than by the significance of the results; therefore, care should be taken when interpreting the results of a meta-analysis.
When performing a systematic literature review or meta-analysis, if the quality of studies is not properly evaluated or if proper methodology is not strictly applied, the results can be biased and the outcomes can be incorrect. However, when systematic reviews and meta-analyses are properly implemented, they can yield powerful results that could usually only be achieved using large-scale RCTs, which are difficult to perform in individual studies. As our understanding of evidence-based medicine increases and its importance is better appreciated, the number of systematic reviews and meta-analyses will keep increasing. However, indiscriminate acceptance of the results of all these meta-analyses can be dangerous, and hence, we recommend that their results be received critically on the basis of a more accurate understanding.
1) http://www.ohri.ca .
2) http://methods.cochrane.org/bias/assessing-risk-bias-included-studies .
3) The inverse variance-weighted estimation method is useful if the number of studies is small with large sample sizes.
4) The Mantel-Haenszel estimation method is useful if the number of studies is large with small sample sizes.
5) The Peto estimation method is useful if the event rate is low or one of the two groups shows zero incidence.
6) The most popular and simplest statistical method used in Review Manager and Comprehensive Meta-analysis software.
7) Alternative random-effect model meta-analysis that has more adequate error rates than does the common DerSimonian and Laird method, especially when the number of studies is small. However, even with the Hartung-Knapp-Sidik-Jonkman method, when there are less than five studies with very unequal sizes, extra caution is needed.
8) The Begg and Mazumdar rank correlation test uses the correlation between the ranks of effect sizes and the ranks of their variances [ 37 ].
9) The degree of funnel plot asymmetry as measured by the intercept from the regression of standard normal deviates against precision [ 29 ].
10) If there are more small studies on one side, we expect the suppression of studies on the other side. Trimming yields the adjusted effect size and reduces the variance of the effects by adding the original studies back into the analysis as a mirror image of each study.
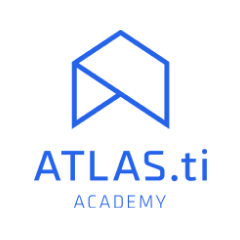
Accelerate your research with the best systematic literature review tools
The ideal literature review tool helps you make sense of the most important insights in your research field. ATLAS.ti empowers researchers to perform powerful and collaborative analysis using the leading software for literature review.
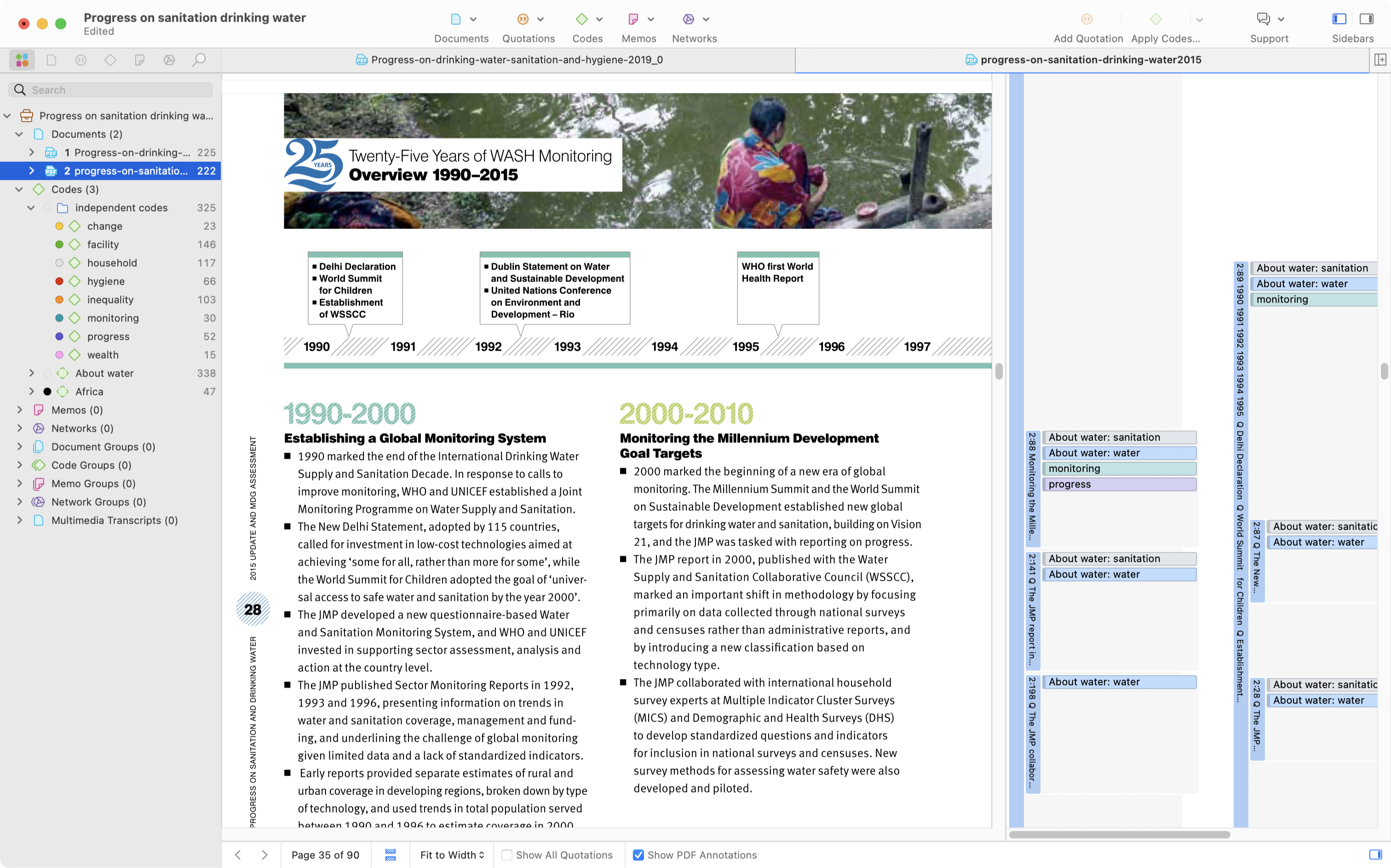
Finalize your literature review faster with comfort
ATLAS.ti makes it easy to manage, organize, and analyze articles, PDFs, excerpts, and more for your projects. Conduct a deep systematic literature review and get the insights you need with a comprehensive toolset built specifically for your research projects.
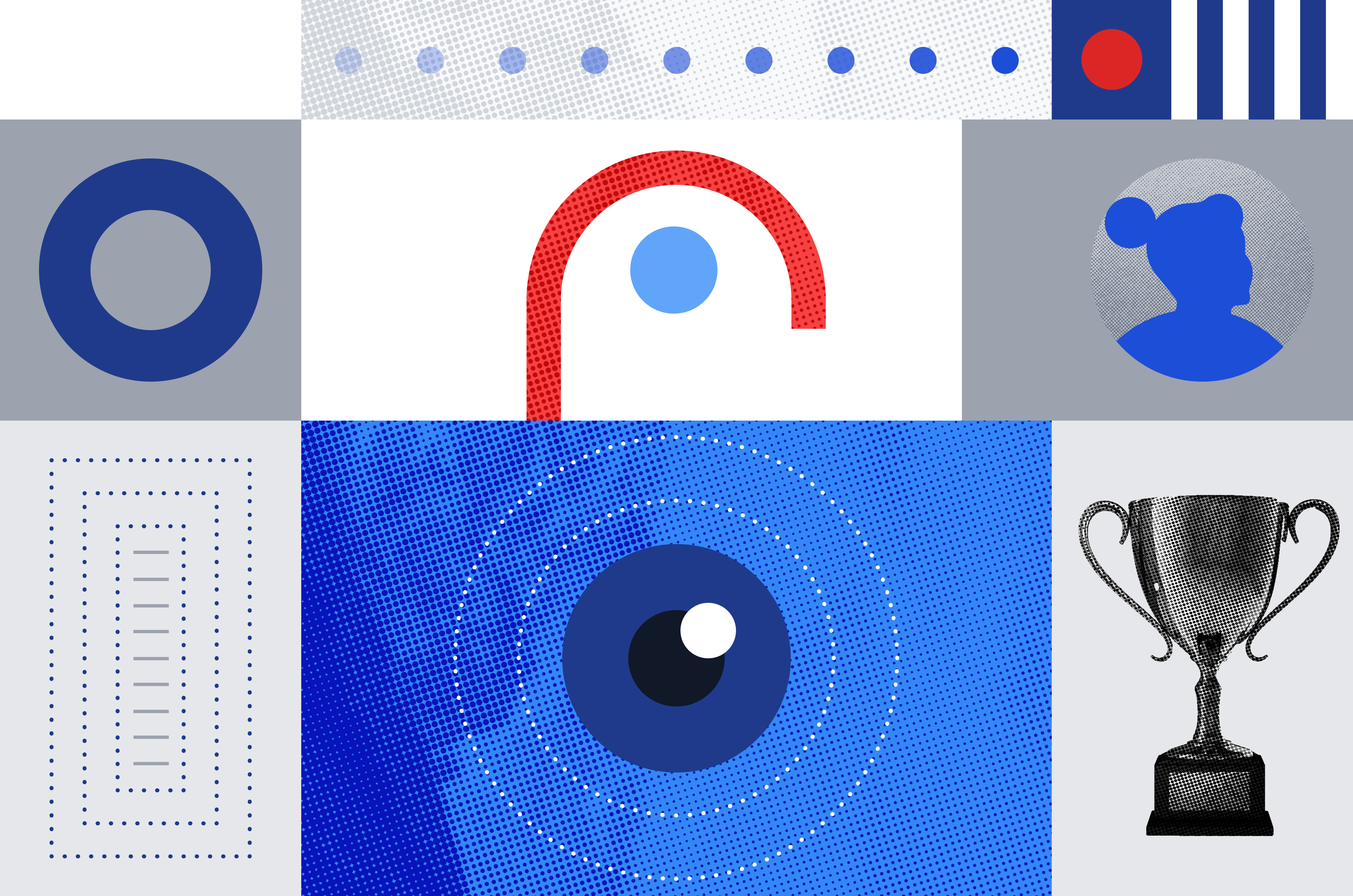
Figure out the "why" behind your participant's motivations
Understand the behaviors and emotions that are driving your focus group participants. With ATLAS.ti, you can transform your raw data and turn it into qualitative insights you can learn from. Easily determine user intent in the same spot you're deciphering your overall focus group data.
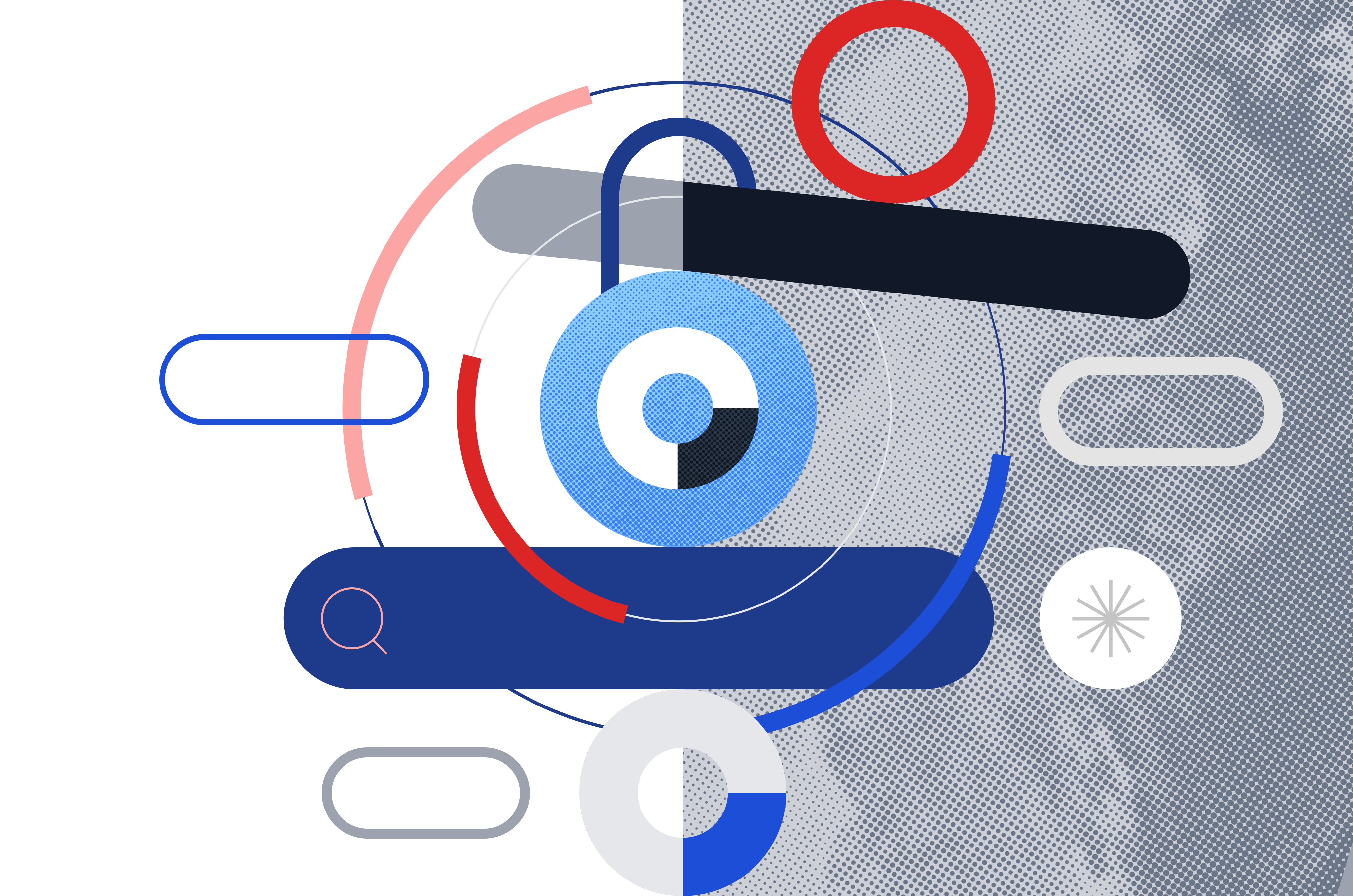
Visualize your research findings like never before
We make it simple to present your analysis results with meaningful charts, networks, and diagrams. Instead of figuring out how to communicate the insights you just unlocked, we enable you to leverage easy-to-use visualizations that support your goals.
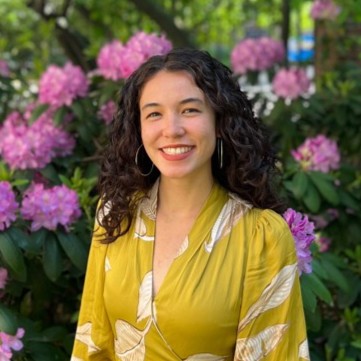
Everything you need to elevate your literature review
Import and organize literature data.
Import and analyze any type of text content – ATLAS.ti supports all standard text and transcription files such as Word and PDF.
Analyze with ease and speed
Utilize easy-to-learn workflows that save valuable time, such as auto coding, sentiment analysis, team collaboration, and more.
Leverage AI-driven tools
Make efficiency a priority and let ATLAS.ti do your work with AI-powered research tools and features for faster results.
Visualize and present findings
With just a few clicks, you can create meaningful visualizations like charts, word clouds, tables, networks, among others for your literature data.
The faster way to make sense of your literature review. Try it for free, today.
A literature review analyzes the most current research within a research area. A literature review consists of published studies from many sources:
- Peer-reviewed academic publications
- Full-length books
- University bulletins
- Conference proceedings
- Dissertations and theses
Literature reviews allow researchers to:
- Summarize the state of the research
- Identify unexplored research inquiries
- Recommend practical applications
- Critique currently published research
Literature reviews are either standalone publications or part of a paper as background for an original research project. A literature review, as a section of a more extensive research article, summarizes the current state of the research to justify the primary research described in the paper.
For example, a researcher may have reviewed the literature on a new supplement's health benefits and concluded that more research needs to be conducted on those with a particular condition. This research gap warrants a study examining how this understudied population reacted to the supplement. Researchers need to establish this research gap through a literature review to persuade journal editors and reviewers of the value of their research.
Consider a literature review as a typical research publication presenting a study, its results, and the salient points scholars can infer from the study. The only significant difference with a literature review treats existing literature as the research data to collect and analyze. From that analysis, a literature review can suggest new inquiries to pursue.
Identify a focus
Similar to a typical study, a literature review should have a research question or questions that analysis can answer. This sort of inquiry typically targets a particular phenomenon, population, or even research method to examine how different studies have looked at the same thing differently. A literature review, then, should center the literature collection around that focus.
Collect and analyze the literature
With a focus in mind, a researcher can collect studies that provide relevant information for that focus. They can then analyze the collected studies by finding and identifying patterns or themes that occur frequently. This analysis allows the researcher to point out what the field has frequently explored or, on the other hand, overlooked.
Suggest implications
The literature review allows the researcher to argue a particular point through the evidence provided by the analysis. For example, suppose the analysis makes it apparent that the published research on people's sleep patterns has not adequately explored the connection between sleep and a particular factor (e.g., television-watching habits, indoor air quality). In that case, the researcher can argue that further study can address this research gap.
External requirements aside (e.g., many academic journals have a word limit of 6,000-8,000 words), a literature review as a standalone publication is as long as necessary to allow readers to understand the current state of the field. Even if it is just a section in a larger paper, a literature review is long enough to allow the researcher to justify the study that is the paper's focus.
Note that a literature review needs only to incorporate a representative number of studies relevant to the research inquiry. For term papers in university courses, 10 to 20 references might be appropriate for demonstrating analytical skills. Published literature reviews in peer-reviewed journals might have 40 to 50 references. One of the essential goals of a literature review is to persuade readers that you have analyzed a representative segment of the research you are reviewing.
Researchers can find published research from various online sources:
- Journal websites
- Research databases
- Search engines (Google Scholar, Semantic Scholar)
- Research repositories
- Social networking sites (Academia, ResearchGate)
Many journals make articles freely available under the term "open access," meaning that there are no restrictions to viewing and downloading such articles. Otherwise, collecting research articles from restricted journals usually requires access from an institution such as a university or a library.
Evidence of a rigorous literature review is more important than the word count or the number of articles that undergo data analysis. Especially when writing for a peer-reviewed journal, it is essential to consider how to demonstrate research rigor in your literature review to persuade reviewers of its scholarly value.
Select field-specific journals
The most significant research relevant to your field focuses on a narrow set of journals similar in aims and scope. Consider who the most prominent scholars in your field are and determine which journals publish their research or have them as editors or reviewers. Journals tend to look favorably on systematic reviews that include articles they have published.
Incorporate recent research
Recently published studies have greater value in determining the gaps in the current state of research. Older research is likely to have encountered challenges and critiques that may render their findings outdated or refuted. What counts as recent differs by field; start by looking for research published within the last three years and gradually expand to older research when you need to collect more articles for your review.
Consider the quality of the research
Literature reviews are only as strong as the quality of the studies that the researcher collects. You can judge any particular study by many factors, including:
- the quality of the article's journal
- the article's research rigor
- the timeliness of the research
The critical point here is that you should consider more than just a study's findings or research outputs when including research in your literature review.
Narrow your research focus
Ideally, the articles you collect for your literature review have something in common, such as a research method or research context. For example, if you are conducting a literature review about teaching practices in high school contexts, it is best to narrow your literature search to studies focusing on high school. You should consider expanding your search to junior high school and university contexts only when there are not enough studies that match your focus.
You can create a project in ATLAS.ti for keeping track of your collected literature. ATLAS.ti allows you to view and analyze full text articles and PDF files in a single project. Within projects, you can use document groups to separate studies into different categories for easier and faster analysis.
For example, a researcher with a literature review that examines studies across different countries can create document groups labeled "United Kingdom," "Germany," and "United States," among others. A researcher can also use ATLAS.ti's global filters to narrow analysis to a particular set of studies and gain insights about a smaller set of literature.
ATLAS.ti allows you to search, code, and analyze text documents and PDF files. You can treat a set of research articles like other forms of qualitative data. The codes you apply to your literature collection allow for analysis through many powerful tools in ATLAS.ti:
- Code Co-Occurrence Explorer
- Code Co-Occurrence Table
- Code-Document Table
Other tools in ATLAS.ti employ machine learning to facilitate parts of the coding process for you. Some of our software tools that are effective for analyzing literature include:
- Named Entity Recognition
- Opinion Mining
- Sentiment Analysis
As long as your documents are text documents or text-enable PDF files, ATLAS.ti's automated tools can provide essential assistance in the data analysis process.
Tools to support the automation of systematic reviews: a scoping review
Affiliations.
- 1 School of Psychology and Public Health, Department of Public Health, La Trobe University, Melbourne Campus, Victoria, Australia. Electronic address: [email protected].
- 2 Faculty of Medicine, Nursing and Health Sciences, Monash University, Wellington Road, Clayton Vic 3168, Australia.
- 3 School of Science, Computing and engineering technologies, Swinburne University of Technology, Melbourne, Australia
- PMID: 34896236
- DOI: 10.1016/j.jclinepi.2021.12.005
Objective: The objectives of this scoping review are to identify the reliability and validity of the available tools, their limitations and any recommendations to further improve the use of these tools.
Study design: A scoping review methodology was followed to map the literature published on the challenges and solutions of conducting evidence synthesis using the JBI scoping review methodology.
Results: A total of 47 publications were included in the review. The current scoping review identified that LitSuggest, Rayyan, Abstractr, BIBOT, R software, RobotAnalyst, DistillerSR, ExaCT and NetMetaXL have potential to be used for the automation of systematic reviews. However, they are not without limitations. The review also identified other studies that employed algorithms that have not yet been developed into user friendly tools. Some of these algorithms showed high validity and reliability but their use is conditional on user knowledge of computer science and algorithms.
Conclusion: Abstract screening has reached maturity; data extraction is still an active area. Developing methods to semi-automate different steps of evidence synthesis via machine learning remains an important research direction. Also, it is important to move from the research prototypes currently available to professionally maintained platforms.
Keywords: Abstract screening; Artificial intelligence; Automation; Machine learning; Reliability; Systematic review.
Copyright © 2021. Published by Elsevier Inc.
Publication types
- Machine Learning*
- Reproducibility of Results
- Systematic Reviews as Topic
- - Google Chrome
Intended for healthcare professionals
- Access provided by Google Indexer
- My email alerts
- BMA member login
- Username * Password * Forgot your log in details? Need to activate BMA Member Log In Log in via OpenAthens Log in via your institution

Search form
- Advanced search
- Search responses
- Search blogs
- The PRISMA 2020...
The PRISMA 2020 statement: an updated guideline for reporting systematic reviews
PRISMA 2020 explanation and elaboration: updated guidance and exemplars for reporting systematic reviews
- Related content
- Peer review
- Matthew J Page , senior research fellow 1 ,
- Joanne E McKenzie , associate professor 1 ,
- Patrick M Bossuyt , professor 2 ,
- Isabelle Boutron , professor 3 ,
- Tammy C Hoffmann , professor 4 ,
- Cynthia D Mulrow , professor 5 ,
- Larissa Shamseer , doctoral student 6 ,
- Jennifer M Tetzlaff , research product specialist 7 ,
- Elie A Akl , professor 8 ,
- Sue E Brennan , senior research fellow 1 ,
- Roger Chou , professor 9 ,
- Julie Glanville , associate director 10 ,
- Jeremy M Grimshaw , professor 11 ,
- Asbjørn Hróbjartsson , professor 12 ,
- Manoj M Lalu , associate scientist and assistant professor 13 ,
- Tianjing Li , associate professor 14 ,
- Elizabeth W Loder , professor 15 ,
- Evan Mayo-Wilson , associate professor 16 ,
- Steve McDonald , senior research fellow 1 ,
- Luke A McGuinness , research associate 17 ,
- Lesley A Stewart , professor and director 18 ,
- James Thomas , professor 19 ,
- Andrea C Tricco , scientist and associate professor 20 ,
- Vivian A Welch , associate professor 21 ,
- Penny Whiting , associate professor 17 ,
- David Moher , director and professor 22
- 1 School of Public Health and Preventive Medicine, Monash University, Melbourne, Australia
- 2 Department of Clinical Epidemiology, Biostatistics and Bioinformatics, Amsterdam University Medical Centres, University of Amsterdam, Amsterdam, Netherlands
- 3 Université de Paris, Centre of Epidemiology and Statistics (CRESS), Inserm, F 75004 Paris, France
- 4 Institute for Evidence-Based Healthcare, Faculty of Health Sciences and Medicine, Bond University, Gold Coast, Australia
- 5 University of Texas Health Science Center at San Antonio, San Antonio, Texas, USA; Annals of Internal Medicine
- 6 Knowledge Translation Program, Li Ka Shing Knowledge Institute, Toronto, Canada; School of Epidemiology and Public Health, Faculty of Medicine, University of Ottawa, Ottawa, Canada
- 7 Evidence Partners, Ottawa, Canada
- 8 Clinical Research Institute, American University of Beirut, Beirut, Lebanon; Department of Health Research Methods, Evidence, and Impact, McMaster University, Hamilton, Ontario, Canada
- 9 Department of Medical Informatics and Clinical Epidemiology, Oregon Health & Science University, Portland, Oregon, USA
- 10 York Health Economics Consortium (YHEC Ltd), University of York, York, UK
- 11 Clinical Epidemiology Program, Ottawa Hospital Research Institute, Ottawa, Canada; School of Epidemiology and Public Health, University of Ottawa, Ottawa, Canada; Department of Medicine, University of Ottawa, Ottawa, Canada
- 12 Centre for Evidence-Based Medicine Odense (CEBMO) and Cochrane Denmark, Department of Clinical Research, University of Southern Denmark, Odense, Denmark; Open Patient data Exploratory Network (OPEN), Odense University Hospital, Odense, Denmark
- 13 Department of Anesthesiology and Pain Medicine, The Ottawa Hospital, Ottawa, Canada; Clinical Epidemiology Program, Blueprint Translational Research Group, Ottawa Hospital Research Institute, Ottawa, Canada; Regenerative Medicine Program, Ottawa Hospital Research Institute, Ottawa, Canada
- 14 Department of Ophthalmology, School of Medicine, University of Colorado Denver, Denver, Colorado, United States; Department of Epidemiology, Johns Hopkins Bloomberg School of Public Health, Baltimore, Maryland, USA
- 15 Division of Headache, Department of Neurology, Brigham and Women's Hospital, Harvard Medical School, Boston, Massachusetts, USA; Head of Research, The BMJ , London, UK
- 16 Department of Epidemiology and Biostatistics, Indiana University School of Public Health-Bloomington, Bloomington, Indiana, USA
- 17 Population Health Sciences, Bristol Medical School, University of Bristol, Bristol, UK
- 18 Centre for Reviews and Dissemination, University of York, York, UK
- 19 EPPI-Centre, UCL Social Research Institute, University College London, London, UK
- 20 Li Ka Shing Knowledge Institute of St. Michael's Hospital, Unity Health Toronto, Toronto, Canada; Epidemiology Division of the Dalla Lana School of Public Health and the Institute of Health Management, Policy, and Evaluation, University of Toronto, Toronto, Canada; Queen's Collaboration for Health Care Quality Joanna Briggs Institute Centre of Excellence, Queen's University, Kingston, Canada
- 21 Methods Centre, Bruyère Research Institute, Ottawa, Ontario, Canada; School of Epidemiology and Public Health, Faculty of Medicine, University of Ottawa, Ottawa, Canada
- 22 Centre for Journalology, Clinical Epidemiology Program, Ottawa Hospital Research Institute, Ottawa, Canada; School of Epidemiology and Public Health, Faculty of Medicine, University of Ottawa, Ottawa, Canada
- Correspondence to: M J Page matthew.page{at}monash.edu
- Accepted 4 January 2021
The Preferred Reporting Items for Systematic reviews and Meta-Analyses (PRISMA) statement, published in 2009, was designed to help systematic reviewers transparently report why the review was done, what the authors did, and what they found. Over the past decade, advances in systematic review methodology and terminology have necessitated an update to the guideline. The PRISMA 2020 statement replaces the 2009 statement and includes new reporting guidance that reflects advances in methods to identify, select, appraise, and synthesise studies. The structure and presentation of the items have been modified to facilitate implementation. In this article, we present the PRISMA 2020 27-item checklist, an expanded checklist that details reporting recommendations for each item, the PRISMA 2020 abstract checklist, and the revised flow diagrams for original and updated reviews.
Systematic reviews serve many critical roles. They can provide syntheses of the state of knowledge in a field, from which future research priorities can be identified; they can address questions that otherwise could not be answered by individual studies; they can identify problems in primary research that should be rectified in future studies; and they can generate or evaluate theories about how or why phenomena occur. Systematic reviews therefore generate various types of knowledge for different users of reviews (such as patients, healthcare providers, researchers, and policy makers). 1 2 To ensure a systematic review is valuable to users, authors should prepare a transparent, complete, and accurate account of why the review was done, what they did (such as how studies were identified and selected) and what they found (such as characteristics of contributing studies and results of meta-analyses). Up-to-date reporting guidance facilitates authors achieving this. 3
The Preferred Reporting Items for Systematic reviews and Meta-Analyses (PRISMA) statement published in 2009 (hereafter referred to as PRISMA 2009) 4 5 6 7 8 9 10 is a reporting guideline designed to address poor reporting of systematic reviews. 11 The PRISMA 2009 statement comprised a checklist of 27 items recommended for reporting in systematic reviews and an “explanation and elaboration” paper 12 13 14 15 16 providing additional reporting guidance for each item, along with exemplars of reporting. The recommendations have been widely endorsed and adopted, as evidenced by its co-publication in multiple journals, citation in over 60 000 reports (Scopus, August 2020), endorsement from almost 200 journals and systematic review organisations, and adoption in various disciplines. Evidence from observational studies suggests that use of the PRISMA 2009 statement is associated with more complete reporting of systematic reviews, 17 18 19 20 although more could be done to improve adherence to the guideline. 21
Many innovations in the conduct of systematic reviews have occurred since publication of the PRISMA 2009 statement. For example, technological advances have enabled the use of natural language processing and machine learning to identify relevant evidence, 22 23 24 methods have been proposed to synthesise and present findings when meta-analysis is not possible or appropriate, 25 26 27 and new methods have been developed to assess the risk of bias in results of included studies. 28 29 Evidence on sources of bias in systematic reviews has accrued, culminating in the development of new tools to appraise the conduct of systematic reviews. 30 31 Terminology used to describe particular review processes has also evolved, as in the shift from assessing “quality” to assessing “certainty” in the body of evidence. 32 In addition, the publishing landscape has transformed, with multiple avenues now available for registering and disseminating systematic review protocols, 33 34 disseminating reports of systematic reviews, and sharing data and materials, such as preprint servers and publicly accessible repositories. To capture these advances in the reporting of systematic reviews necessitated an update to the PRISMA 2009 statement.
Summary points
To ensure a systematic review is valuable to users, authors should prepare a transparent, complete, and accurate account of why the review was done, what they did, and what they found
The PRISMA 2020 statement provides updated reporting guidance for systematic reviews that reflects advances in methods to identify, select, appraise, and synthesise studies
The PRISMA 2020 statement consists of a 27-item checklist, an expanded checklist that details reporting recommendations for each item, the PRISMA 2020 abstract checklist, and revised flow diagrams for original and updated reviews
We anticipate that the PRISMA 2020 statement will benefit authors, editors, and peer reviewers of systematic reviews, and different users of reviews, including guideline developers, policy makers, healthcare providers, patients, and other stakeholders
Development of PRISMA 2020
A complete description of the methods used to develop PRISMA 2020 is available elsewhere. 35 We identified PRISMA 2009 items that were often reported incompletely by examining the results of studies investigating the transparency of reporting of published reviews. 17 21 36 37 We identified possible modifications to the PRISMA 2009 statement by reviewing 60 documents providing reporting guidance for systematic reviews (including reporting guidelines, handbooks, tools, and meta-research studies). 38 These reviews of the literature were used to inform the content of a survey with suggested possible modifications to the 27 items in PRISMA 2009 and possible additional items. Respondents were asked whether they believed we should keep each PRISMA 2009 item as is, modify it, or remove it, and whether we should add each additional item. Systematic review methodologists and journal editors were invited to complete the online survey (110 of 220 invited responded). We discussed proposed content and wording of the PRISMA 2020 statement, as informed by the review and survey results, at a 21-member, two-day, in-person meeting in September 2018 in Edinburgh, Scotland. Throughout 2019 and 2020, we circulated an initial draft and five revisions of the checklist and explanation and elaboration paper to co-authors for feedback. In April 2020, we invited 22 systematic reviewers who had expressed interest in providing feedback on the PRISMA 2020 checklist to share their views (via an online survey) on the layout and terminology used in a preliminary version of the checklist. Feedback was received from 15 individuals and considered by the first author, and any revisions deemed necessary were incorporated before the final version was approved and endorsed by all co-authors.
The PRISMA 2020 statement
Scope of the guideline.
The PRISMA 2020 statement has been designed primarily for systematic reviews of studies that evaluate the effects of health interventions, irrespective of the design of the included studies. However, the checklist items are applicable to reports of systematic reviews evaluating other interventions (such as social or educational interventions), and many items are applicable to systematic reviews with objectives other than evaluating interventions (such as evaluating aetiology, prevalence, or prognosis). PRISMA 2020 is intended for use in systematic reviews that include synthesis (such as pairwise meta-analysis or other statistical synthesis methods) or do not include synthesis (for example, because only one eligible study is identified). The PRISMA 2020 items are relevant for mixed-methods systematic reviews (which include quantitative and qualitative studies), but reporting guidelines addressing the presentation and synthesis of qualitative data should also be consulted. 39 40 PRISMA 2020 can be used for original systematic reviews, updated systematic reviews, or continually updated (“living”) systematic reviews. However, for updated and living systematic reviews, there may be some additional considerations that need to be addressed. Where there is relevant content from other reporting guidelines, we reference these guidelines within the items in the explanation and elaboration paper 41 (such as PRISMA-Search 42 in items 6 and 7, Synthesis without meta-analysis (SWiM) reporting guideline 27 in item 13d). Box 1 includes a glossary of terms used throughout the PRISMA 2020 statement.
Glossary of terms
Systematic review —A review that uses explicit, systematic methods to collate and synthesise findings of studies that address a clearly formulated question 43
Statistical synthesis —The combination of quantitative results of two or more studies. This encompasses meta-analysis of effect estimates (described below) and other methods, such as combining P values, calculating the range and distribution of observed effects, and vote counting based on the direction of effect (see McKenzie and Brennan 25 for a description of each method)
Meta-analysis of effect estimates —A statistical technique used to synthesise results when study effect estimates and their variances are available, yielding a quantitative summary of results 25
Outcome —An event or measurement collected for participants in a study (such as quality of life, mortality)
Result —The combination of a point estimate (such as a mean difference, risk ratio, or proportion) and a measure of its precision (such as a confidence/credible interval) for a particular outcome
Report —A document (paper or electronic) supplying information about a particular study. It could be a journal article, preprint, conference abstract, study register entry, clinical study report, dissertation, unpublished manuscript, government report, or any other document providing relevant information
Record —The title or abstract (or both) of a report indexed in a database or website (such as a title or abstract for an article indexed in Medline). Records that refer to the same report (such as the same journal article) are “duplicates”; however, records that refer to reports that are merely similar (such as a similar abstract submitted to two different conferences) should be considered unique.
Study —An investigation, such as a clinical trial, that includes a defined group of participants and one or more interventions and outcomes. A “study” might have multiple reports. For example, reports could include the protocol, statistical analysis plan, baseline characteristics, results for the primary outcome, results for harms, results for secondary outcomes, and results for additional mediator and moderator analyses
PRISMA 2020 is not intended to guide systematic review conduct, for which comprehensive resources are available. 43 44 45 46 However, familiarity with PRISMA 2020 is useful when planning and conducting systematic reviews to ensure that all recommended information is captured. PRISMA 2020 should not be used to assess the conduct or methodological quality of systematic reviews; other tools exist for this purpose. 30 31 Furthermore, PRISMA 2020 is not intended to inform the reporting of systematic review protocols, for which a separate statement is available (PRISMA for Protocols (PRISMA-P) 2015 statement 47 48 ). Finally, extensions to the PRISMA 2009 statement have been developed to guide reporting of network meta-analyses, 49 meta-analyses of individual participant data, 50 systematic reviews of harms, 51 systematic reviews of diagnostic test accuracy studies, 52 and scoping reviews 53 ; for these types of reviews we recommend authors report their review in accordance with the recommendations in PRISMA 2020 along with the guidance specific to the extension.
How to use PRISMA 2020
The PRISMA 2020 statement (including the checklists, explanation and elaboration, and flow diagram) replaces the PRISMA 2009 statement, which should no longer be used. Box 2 summarises noteworthy changes from the PRISMA 2009 statement. The PRISMA 2020 checklist includes seven sections with 27 items, some of which include sub-items ( table 1 ). A checklist for journal and conference abstracts for systematic reviews is included in PRISMA 2020. This abstract checklist is an update of the 2013 PRISMA for Abstracts statement, 54 reflecting new and modified content in PRISMA 2020 ( table 2 ). A template PRISMA flow diagram is provided, which can be modified depending on whether the systematic review is original or updated ( fig 1 ).
Noteworthy changes to the PRISMA 2009 statement
Inclusion of the abstract reporting checklist within PRISMA 2020 (see item #2 and table 2 ).
Movement of the ‘Protocol and registration’ item from the start of the Methods section of the checklist to a new Other section, with addition of a sub-item recommending authors describe amendments to information provided at registration or in the protocol (see item #24a-24c).
Modification of the ‘Search’ item to recommend authors present full search strategies for all databases, registers and websites searched, not just at least one database (see item #7).
Modification of the ‘Study selection’ item in the Methods section to emphasise the reporting of how many reviewers screened each record and each report retrieved, whether they worked independently, and if applicable, details of automation tools used in the process (see item #8).
Addition of a sub-item to the ‘Data items’ item recommending authors report how outcomes were defined, which results were sought, and methods for selecting a subset of results from included studies (see item #10a).
Splitting of the ‘Synthesis of results’ item in the Methods section into six sub-items recommending authors describe: the processes used to decide which studies were eligible for each synthesis; any methods required to prepare the data for synthesis; any methods used to tabulate or visually display results of individual studies and syntheses; any methods used to synthesise results; any methods used to explore possible causes of heterogeneity among study results (such as subgroup analysis, meta-regression); and any sensitivity analyses used to assess robustness of the synthesised results (see item #13a-13f).
Addition of a sub-item to the ‘Study selection’ item in the Results section recommending authors cite studies that might appear to meet the inclusion criteria, but which were excluded, and explain why they were excluded (see item #16b).
Splitting of the ‘Synthesis of results’ item in the Results section into four sub-items recommending authors: briefly summarise the characteristics and risk of bias among studies contributing to the synthesis; present results of all statistical syntheses conducted; present results of any investigations of possible causes of heterogeneity among study results; and present results of any sensitivity analyses (see item #20a-20d).
Addition of new items recommending authors report methods for and results of an assessment of certainty (or confidence) in the body of evidence for an outcome (see items #15 and #22).
Addition of a new item recommending authors declare any competing interests (see item #26).
Addition of a new item recommending authors indicate whether data, analytic code and other materials used in the review are publicly available and if so, where they can be found (see item #27).
PRISMA 2020 item checklist
- View inline
PRISMA 2020 for Abstracts checklist*

PRISMA 2020 flow diagram template for systematic reviews. The new design is adapted from flow diagrams proposed by Boers, 55 Mayo-Wilson et al. 56 and Stovold et al. 57 The boxes in grey should only be completed if applicable; otherwise they should be removed from the flow diagram. Note that a “report” could be a journal article, preprint, conference abstract, study register entry, clinical study report, dissertation, unpublished manuscript, government report or any other document providing relevant information.
- Download figure
- Open in new tab
- Download powerpoint
We recommend authors refer to PRISMA 2020 early in the writing process, because prospective consideration of the items may help to ensure that all the items are addressed. To help keep track of which items have been reported, the PRISMA statement website ( http://www.prisma-statement.org/ ) includes fillable templates of the checklists to download and complete (also available in the data supplement on bmj.com). We have also created a web application that allows users to complete the checklist via a user-friendly interface 58 (available at https://prisma.shinyapps.io/checklist/ and adapted from the Transparency Checklist app 59 ). The completed checklist can be exported to Word or PDF. Editable templates of the flow diagram can also be downloaded from the PRISMA statement website.
We have prepared an updated explanation and elaboration paper, in which we explain why reporting of each item is recommended and present bullet points that detail the reporting recommendations (which we refer to as elements). 41 The bullet-point structure is new to PRISMA 2020 and has been adopted to facilitate implementation of the guidance. 60 61 An expanded checklist, which comprises an abridged version of the elements presented in the explanation and elaboration paper, with references and some examples removed, is available in the data supplement on bmj.com. Consulting the explanation and elaboration paper is recommended if further clarity or information is required.
Journals and publishers might impose word and section limits, and limits on the number of tables and figures allowed in the main report. In such cases, if the relevant information for some items already appears in a publicly accessible review protocol, referring to the protocol may suffice. Alternatively, placing detailed descriptions of the methods used or additional results (such as for less critical outcomes) in supplementary files is recommended. Ideally, supplementary files should be deposited to a general-purpose or institutional open-access repository that provides free and permanent access to the material (such as Open Science Framework, Dryad, figshare). A reference or link to the additional information should be included in the main report. Finally, although PRISMA 2020 provides a template for where information might be located, the suggested location should not be seen as prescriptive; the guiding principle is to ensure the information is reported.
Use of PRISMA 2020 has the potential to benefit many stakeholders. Complete reporting allows readers to assess the appropriateness of the methods, and therefore the trustworthiness of the findings. Presenting and summarising characteristics of studies contributing to a synthesis allows healthcare providers and policy makers to evaluate the applicability of the findings to their setting. Describing the certainty in the body of evidence for an outcome and the implications of findings should help policy makers, managers, and other decision makers formulate appropriate recommendations for practice or policy. Complete reporting of all PRISMA 2020 items also facilitates replication and review updates, as well as inclusion of systematic reviews in overviews (of systematic reviews) and guidelines, so teams can leverage work that is already done and decrease research waste. 36 62 63
We updated the PRISMA 2009 statement by adapting the EQUATOR Network’s guidance for developing health research reporting guidelines. 64 We evaluated the reporting completeness of published systematic reviews, 17 21 36 37 reviewed the items included in other documents providing guidance for systematic reviews, 38 surveyed systematic review methodologists and journal editors for their views on how to revise the original PRISMA statement, 35 discussed the findings at an in-person meeting, and prepared this document through an iterative process. Our recommendations are informed by the reviews and survey conducted before the in-person meeting, theoretical considerations about which items facilitate replication and help users assess the risk of bias and applicability of systematic reviews, and co-authors’ experience with authoring and using systematic reviews.
Various strategies to increase the use of reporting guidelines and improve reporting have been proposed. They include educators introducing reporting guidelines into graduate curricula to promote good reporting habits of early career scientists 65 ; journal editors and regulators endorsing use of reporting guidelines 18 ; peer reviewers evaluating adherence to reporting guidelines 61 66 ; journals requiring authors to indicate where in their manuscript they have adhered to each reporting item 67 ; and authors using online writing tools that prompt complete reporting at the writing stage. 60 Multi-pronged interventions, where more than one of these strategies are combined, may be more effective (such as completion of checklists coupled with editorial checks). 68 However, of 31 interventions proposed to increase adherence to reporting guidelines, the effects of only 11 have been evaluated, mostly in observational studies at high risk of bias due to confounding. 69 It is therefore unclear which strategies should be used. Future research might explore barriers and facilitators to the use of PRISMA 2020 by authors, editors, and peer reviewers, designing interventions that address the identified barriers, and evaluating those interventions using randomised trials. To inform possible revisions to the guideline, it would also be valuable to conduct think-aloud studies 70 to understand how systematic reviewers interpret the items, and reliability studies to identify items where there is varied interpretation of the items.
We encourage readers to submit evidence that informs any of the recommendations in PRISMA 2020 (via the PRISMA statement website: http://www.prisma-statement.org/ ). To enhance accessibility of PRISMA 2020, several translations of the guideline are under way (see available translations at the PRISMA statement website). We encourage journal editors and publishers to raise awareness of PRISMA 2020 (for example, by referring to it in journal “Instructions to authors”), endorsing its use, advising editors and peer reviewers to evaluate submitted systematic reviews against the PRISMA 2020 checklists, and making changes to journal policies to accommodate the new reporting recommendations. We recommend existing PRISMA extensions 47 49 50 51 52 53 71 72 be updated to reflect PRISMA 2020 and advise developers of new PRISMA extensions to use PRISMA 2020 as the foundation document.
We anticipate that the PRISMA 2020 statement will benefit authors, editors, and peer reviewers of systematic reviews, and different users of reviews, including guideline developers, policy makers, healthcare providers, patients, and other stakeholders. Ultimately, we hope that uptake of the guideline will lead to more transparent, complete, and accurate reporting of systematic reviews, thus facilitating evidence based decision making.
Acknowledgments
We dedicate this paper to the late Douglas G Altman and Alessandro Liberati, whose contributions were fundamental to the development and implementation of the original PRISMA statement.
We thank the following contributors who completed the survey to inform discussions at the development meeting: Xavier Armoiry, Edoardo Aromataris, Ana Patricia Ayala, Ethan M Balk, Virginia Barbour, Elaine Beller, Jesse A Berlin, Lisa Bero, Zhao-Xiang Bian, Jean Joel Bigna, Ferrán Catalá-López, Anna Chaimani, Mike Clarke, Tammy Clifford, Ioana A Cristea, Miranda Cumpston, Sofia Dias, Corinna Dressler, Ivan D Florez, Joel J Gagnier, Chantelle Garritty, Long Ge, Davina Ghersi, Sean Grant, Gordon Guyatt, Neal R Haddaway, Julian PT Higgins, Sally Hopewell, Brian Hutton, Jamie J Kirkham, Jos Kleijnen, Julia Koricheva, Joey SW Kwong, Toby J Lasserson, Julia H Littell, Yoon K Loke, Malcolm R Macleod, Chris G Maher, Ana Marušic, Dimitris Mavridis, Jessie McGowan, Matthew DF McInnes, Philippa Middleton, Karel G Moons, Zachary Munn, Jane Noyes, Barbara Nußbaumer-Streit, Donald L Patrick, Tatiana Pereira-Cenci, Ba’ Pham, Bob Phillips, Dawid Pieper, Michelle Pollock, Daniel S Quintana, Drummond Rennie, Melissa L Rethlefsen, Hannah R Rothstein, Maroeska M Rovers, Rebecca Ryan, Georgia Salanti, Ian J Saldanha, Margaret Sampson, Nancy Santesso, Rafael Sarkis-Onofre, Jelena Savović, Christopher H Schmid, Kenneth F Schulz, Guido Schwarzer, Beverley J Shea, Paul G Shekelle, Farhad Shokraneh, Mark Simmonds, Nicole Skoetz, Sharon E Straus, Anneliese Synnot, Emily E Tanner-Smith, Brett D Thombs, Hilary Thomson, Alexander Tsertsvadze, Peter Tugwell, Tari Turner, Lesley Uttley, Jeffrey C Valentine, Matt Vassar, Areti Angeliki Veroniki, Meera Viswanathan, Cole Wayant, Paul Whaley, and Kehu Yang. We thank the following contributors who provided feedback on a preliminary version of the PRISMA 2020 checklist: Jo Abbott, Fionn Büttner, Patricia Correia-Santos, Victoria Freeman, Emily A Hennessy, Rakibul Islam, Amalia (Emily) Karahalios, Kasper Krommes, Andreas Lundh, Dafne Port Nascimento, Davina Robson, Catherine Schenck-Yglesias, Mary M Scott, Sarah Tanveer and Pavel Zhelnov. We thank Abigail H Goben, Melissa L Rethlefsen, Tanja Rombey, Anna Scott, and Farhad Shokraneh for their helpful comments on the preprints of the PRISMA 2020 papers. We thank Edoardo Aromataris, Stephanie Chang, Toby Lasserson and David Schriger for their helpful peer review comments on the PRISMA 2020 papers.
Contributors: JEM and DM are joint senior authors. MJP, JEM, PMB, IB, TCH, CDM, LS, and DM conceived this paper and designed the literature review and survey conducted to inform the guideline content. MJP conducted the literature review, administered the survey and analysed the data for both. MJP prepared all materials for the development meeting. MJP and JEM presented proposals at the development meeting. All authors except for TCH, JMT, EAA, SEB, and LAM attended the development meeting. MJP and JEM took and consolidated notes from the development meeting. MJP and JEM led the drafting and editing of the article. JEM, PMB, IB, TCH, LS, JMT, EAA, SEB, RC, JG, AH, TL, EMW, SM, LAM, LAS, JT, ACT, PW, and DM drafted particular sections of the article. All authors were involved in revising the article critically for important intellectual content. All authors approved the final version of the article. MJP is the guarantor of this work. The corresponding author attests that all listed authors meet authorship criteria and that no others meeting the criteria have been omitted.
Funding: There was no direct funding for this research. MJP is supported by an Australian Research Council Discovery Early Career Researcher Award (DE200101618) and was previously supported by an Australian National Health and Medical Research Council (NHMRC) Early Career Fellowship (1088535) during the conduct of this research. JEM is supported by an Australian NHMRC Career Development Fellowship (1143429). TCH is supported by an Australian NHMRC Senior Research Fellowship (1154607). JMT is supported by Evidence Partners Inc. JMG is supported by a Tier 1 Canada Research Chair in Health Knowledge Transfer and Uptake. MML is supported by The Ottawa Hospital Anaesthesia Alternate Funds Association and a Faculty of Medicine Junior Research Chair. TL is supported by funding from the National Eye Institute (UG1EY020522), National Institutes of Health, United States. LAM is supported by a National Institute for Health Research Doctoral Research Fellowship (DRF-2018-11-ST2-048). ACT is supported by a Tier 2 Canada Research Chair in Knowledge Synthesis. DM is supported in part by a University Research Chair, University of Ottawa. The funders had no role in considering the study design or in the collection, analysis, interpretation of data, writing of the report, or decision to submit the article for publication.
Competing interests: All authors have completed the ICMJE uniform disclosure form at http://www.icmje.org/conflicts-of-interest/ and declare: EL is head of research for the BMJ ; MJP is an editorial board member for PLOS Medicine ; ACT is an associate editor and MJP, TL, EMW, and DM are editorial board members for the Journal of Clinical Epidemiology ; DM and LAS were editors in chief, LS, JMT, and ACT are associate editors, and JG is an editorial board member for Systematic Reviews . None of these authors were involved in the peer review process or decision to publish. TCH has received personal fees from Elsevier outside the submitted work. EMW has received personal fees from the American Journal for Public Health , for which he is the editor for systematic reviews. VW is editor in chief of the Campbell Collaboration, which produces systematic reviews, and co-convenor of the Campbell and Cochrane equity methods group. DM is chair of the EQUATOR Network, IB is adjunct director of the French EQUATOR Centre and TCH is co-director of the Australasian EQUATOR Centre, which advocates for the use of reporting guidelines to improve the quality of reporting in research articles. JMT received salary from Evidence Partners, creator of DistillerSR software for systematic reviews; Evidence Partners was not involved in the design or outcomes of the statement, and the views expressed solely represent those of the author.
Provenance and peer review: Not commissioned; externally peer reviewed.
Patient and public involvement: Patients and the public were not involved in this methodological research. We plan to disseminate the research widely, including to community participants in evidence synthesis organisations.
This is an Open Access article distributed in accordance with the terms of the Creative Commons Attribution (CC BY 4.0) license, which permits others to distribute, remix, adapt and build upon this work, for commercial use, provided the original work is properly cited. See: http://creativecommons.org/licenses/by/4.0/ .
- Gurevitch J ,
- Koricheva J ,
- Nakagawa S ,
- Liberati A ,
- Tetzlaff J ,
- Altman DG ,
- PRISMA Group
- Tricco AC ,
- Sampson M ,
- Shamseer L ,
- Leoncini E ,
- de Belvis G ,
- Ricciardi W ,
- Fowler AJ ,
- Leclercq V ,
- Beaudart C ,
- Ajamieh S ,
- Rabenda V ,
- Tirelli E ,
- O’Mara-Eves A ,
- McNaught J ,
- Ananiadou S
- Marshall IJ ,
- Noel-Storr A ,
- Higgins JPT ,
- Chandler J ,
- McKenzie JE ,
- López-López JA ,
- Becker BJ ,
- Campbell M ,
- Sterne JAC ,
- Savović J ,
- Sterne JA ,
- Hernán MA ,
- Reeves BC ,
- Whiting P ,
- Higgins JP ,
- ROBIS group
- Hultcrantz M ,
- Stewart L ,
- Bossuyt PM ,
- Flemming K ,
- McInnes E ,
- France EF ,
- Cunningham M ,
- Rethlefsen ML ,
- Kirtley S ,
- Waffenschmidt S ,
- PRISMA-S Group
- ↵ Higgins JPT, Thomas J, Chandler J, et al, eds. Cochrane Handbook for Systematic Reviews of Interventions : Version 6.0. Cochrane, 2019. Available from https://training.cochrane.org/handbook .
- Dekkers OM ,
- Vandenbroucke JP ,
- Cevallos M ,
- Renehan AG ,
- ↵ Cooper H, Hedges LV, Valentine JV, eds. The Handbook of Research Synthesis and Meta-Analysis. Russell Sage Foundation, 2019.
- IOM (Institute of Medicine)
- PRISMA-P Group
- Salanti G ,
- Caldwell DM ,
- Stewart LA ,
- PRISMA-IPD Development Group
- Zorzela L ,
- Ioannidis JP ,
- PRISMAHarms Group
- McInnes MDF ,
- Thombs BD ,
- and the PRISMA-DTA Group
- Beller EM ,
- Glasziou PP ,
- PRISMA for Abstracts Group
- Mayo-Wilson E ,
- Dickersin K ,
- MUDS investigators
- Stovold E ,
- Beecher D ,
- Noel-Storr A
- McGuinness LA
- Sarafoglou A ,
- Boutron I ,
- Giraudeau B ,
- Porcher R ,
- Chauvin A ,
- Schulz KF ,
- Schroter S ,
- Stevens A ,
- Weinstein E ,
- Macleod MR ,
- IICARus Collaboration
- Kirkham JJ ,
- Petticrew M ,
- Tugwell P ,
- PRISMA-Equity Bellagio group
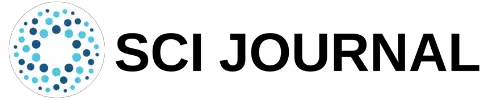
10 Best Literature Review Tools for Researchers
This post may contain affiliate links that allow us to earn a commission at no expense to you. Learn more
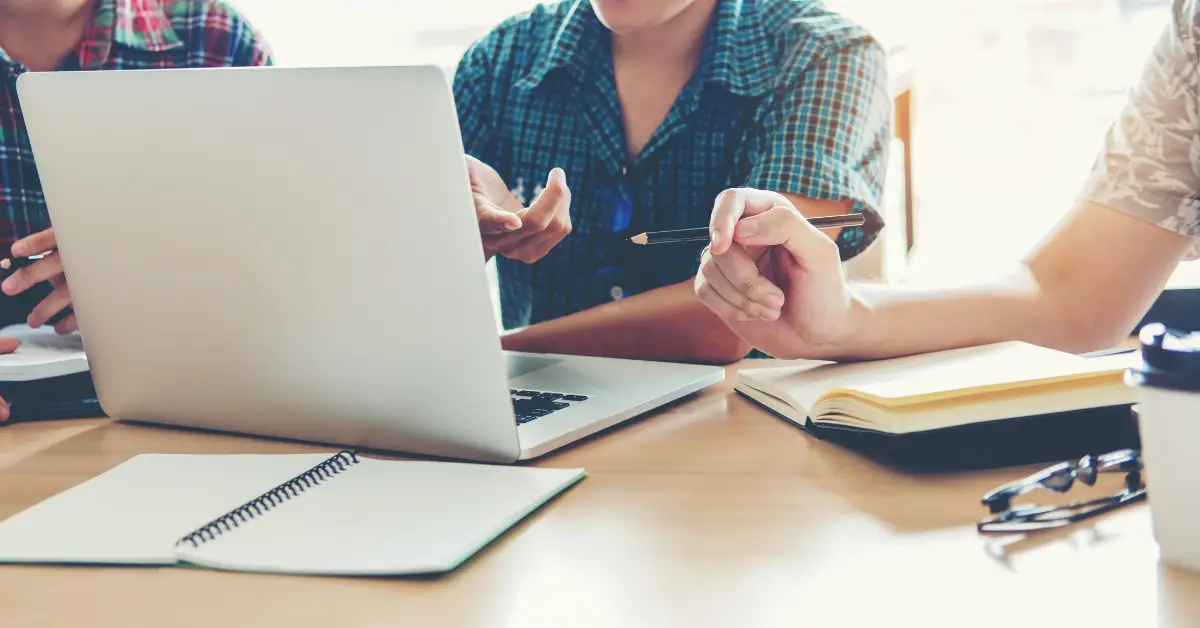
Boost your research game with these Best Literature Review Tools for Researchers! Uncover hidden gems, organize your findings, and ace your next research paper!
Conducting literature reviews poses challenges for researchers due to the overwhelming volume of information available and the lack of efficient methods to manage and analyze it.
Researchers struggle to identify key sources, extract relevant information, and maintain accuracy while manually conducting literature reviews. This leads to inefficiency, errors, and difficulty in identifying gaps or trends in existing literature.
Advancements in technology have resulted in a variety of literature review tools. These tools streamline the process, offering features like automated searching, filtering, citation management, and research data extraction. They save time, improve accuracy, and provide valuable insights for researchers.
In this article, we present a curated list of the 10 best literature review tools, empowering researchers to make informed choices and revolutionize their systematic literature review process.
Table of Contents
Top 10 Literature Review Tools for Researchers: In A Nutshell (2023)
#1. semantic scholar – a free, ai-powered research tool for scientific literature.
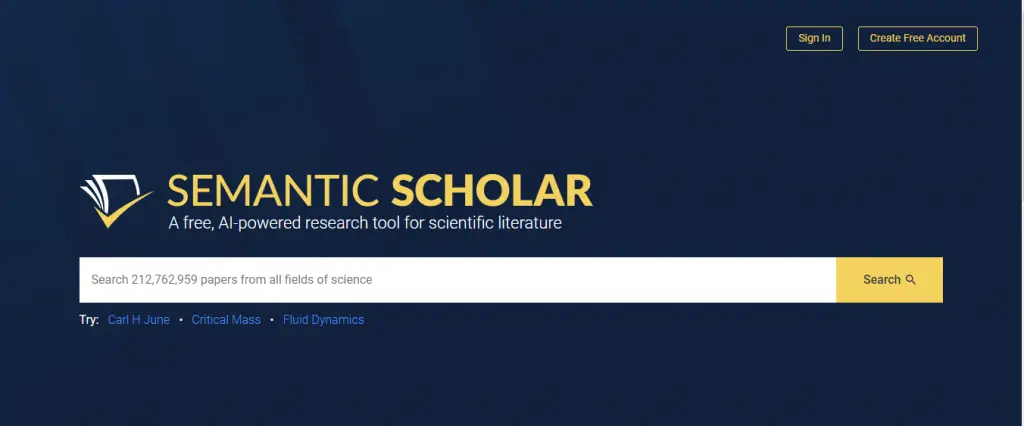
Semantic Scholar is a cutting-edge literature review tool that researchers rely on for its comprehensive access to academic publications. With its advanced AI algorithms and extensive database, it simplifies the discovery of relevant research papers.
By employing semantic analysis, users can explore scholarly articles based on context and meaning, making it a go-to resource for scholars across disciplines.
Additionally, Semantic Scholar offers personalized recommendations and alerts, ensuring researchers stay updated with the latest developments. However, users should be cautious of potential limitations.
Not all scholarly content may be indexed, and occasional false positives or inaccurate associations can occur. Furthermore, the tool primarily focuses on computer science and related fields, potentially limiting coverage in other disciplines.
Researchers should be mindful of these considerations and supplement Semantic Scholar with other reputable resources for a comprehensive literature review. Despite these caveats, Semantic Scholar remains a valuable tool for streamlining research and staying informed.
#2. Elicit – Research assistant using language models like GPT-3
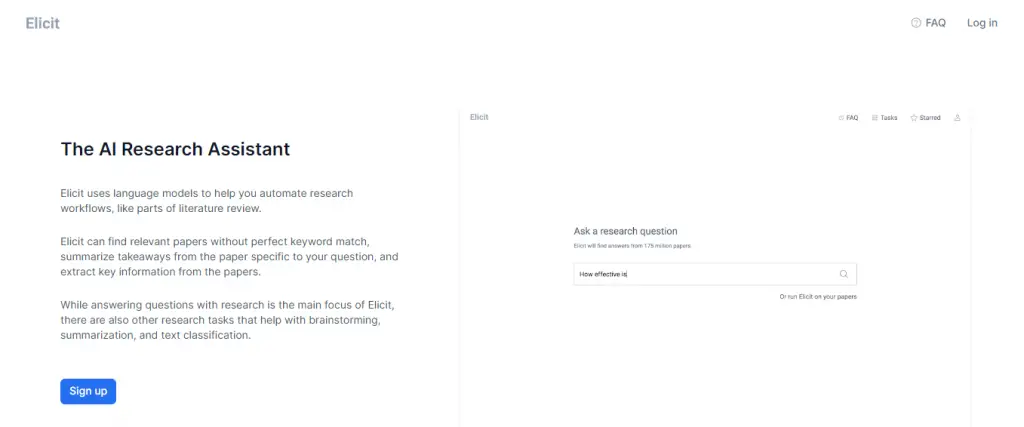
Elicit is a game-changing literature review tool that has gained popularity among researchers worldwide. With its user-friendly interface and extensive database of scholarly articles, it streamlines the research process, saving time and effort.
The tool employs advanced algorithms to provide personalized recommendations, ensuring researchers discover the most relevant studies for their field. Elicit also promotes collaboration by enabling users to create shared folders and annotate articles.
However, users should be cautious when using Elicit. It is important to verify the credibility and accuracy of the sources found through the tool, as the database encompasses a wide range of publications.
Additionally, occasional glitches in the search function have been reported, leading to incomplete or inaccurate results. While Elicit offers tremendous benefits, researchers should remain vigilant and cross-reference information to ensure a comprehensive literature review.
#3. Scite.Ai – Your personal research assistant
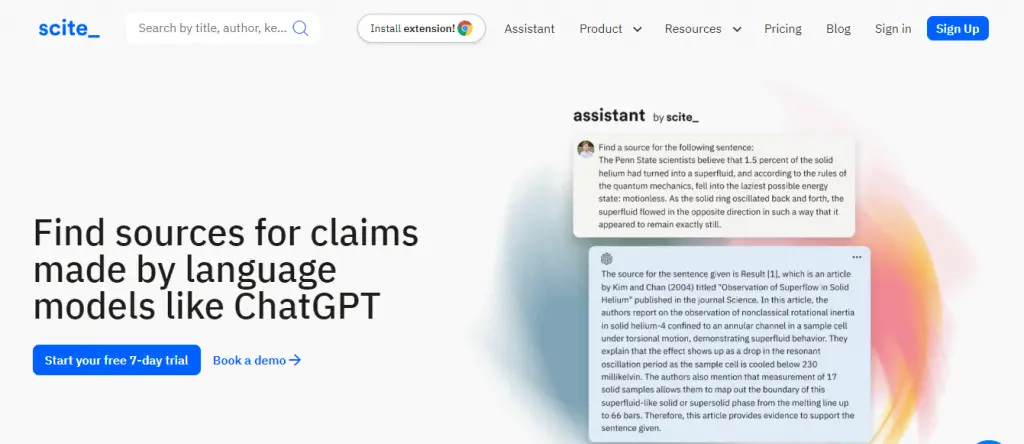
Scite.Ai is a popular literature review tool that revolutionizes the research process for scholars. With its innovative citation analysis feature, researchers can evaluate the credibility and impact of scientific articles, making informed decisions about their inclusion in their own work.
By assessing the context in which citations are used, Scite.Ai ensures that the sources selected are reliable and of high quality, enabling researchers to establish a strong foundation for their research.
However, while Scite.Ai offers numerous advantages, there are a few aspects to be cautious about. As with any data-driven tool, occasional errors or inaccuracies may arise, necessitating researchers to cross-reference and verify results with other reputable sources.
Moreover, Scite.Ai’s coverage may be limited in certain subject areas and languages, with a possibility of missing relevant studies, especially in niche fields or non-English publications.
Therefore, researchers should supplement the use of Scite.Ai with additional resources to ensure comprehensive literature coverage and avoid any potential gaps in their research.
Rayyan offers the following paid plans:
- Monthly Plan: $20
- Yearly Plan: $12
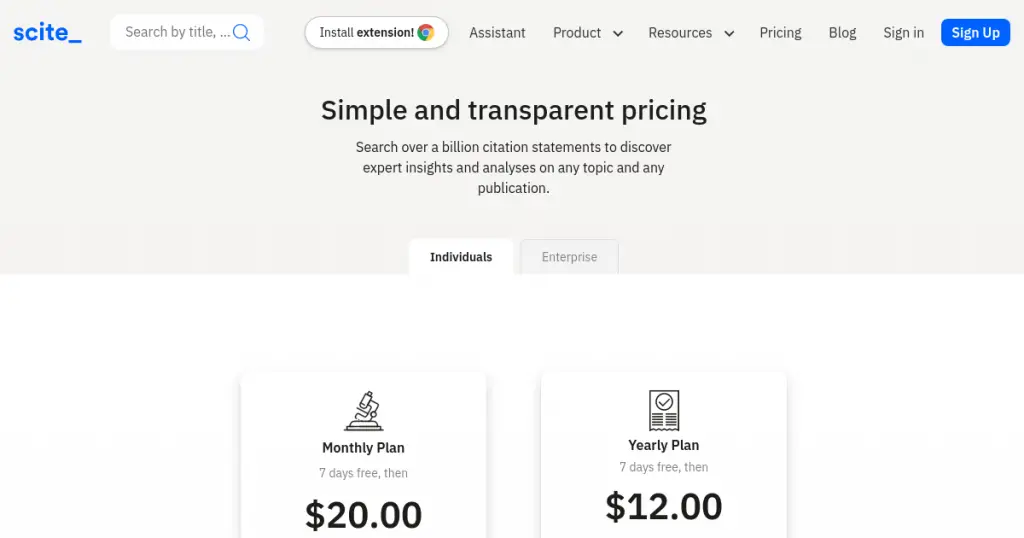
#4. DistillerSR – Literature Review Software
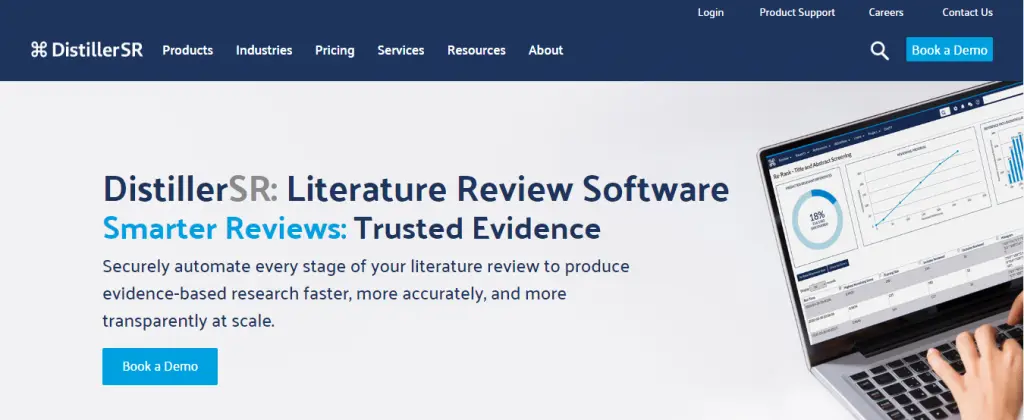
DistillerSR is a powerful literature review tool trusted by researchers for its user-friendly interface and robust features. With its advanced search capabilities, researchers can quickly find relevant studies from multiple databases, saving time and effort.
The tool offers comprehensive screening and data extraction functionalities, streamlining the review process and improving the reliability of findings. Real-time collaboration features also facilitate seamless teamwork among researchers.
While DistillerSR offers numerous advantages, there are a few considerations. Users should invest time in understanding the tool’s features and functionalities to maximize its potential. Additionally, the pricing structure may be a factor for individual researchers or small teams with limited budgets.
Despite occasional technical glitches reported by some users, the developers actively address these issues through updates and improvements, ensuring a better user experience.
Overall, DistillerSR empowers researchers to navigate the vast sea of information, enhancing the quality and efficiency of literature reviews while fostering collaboration among research teams .
#5. Rayyan – AI Powered Tool for Systematic Literature Reviews
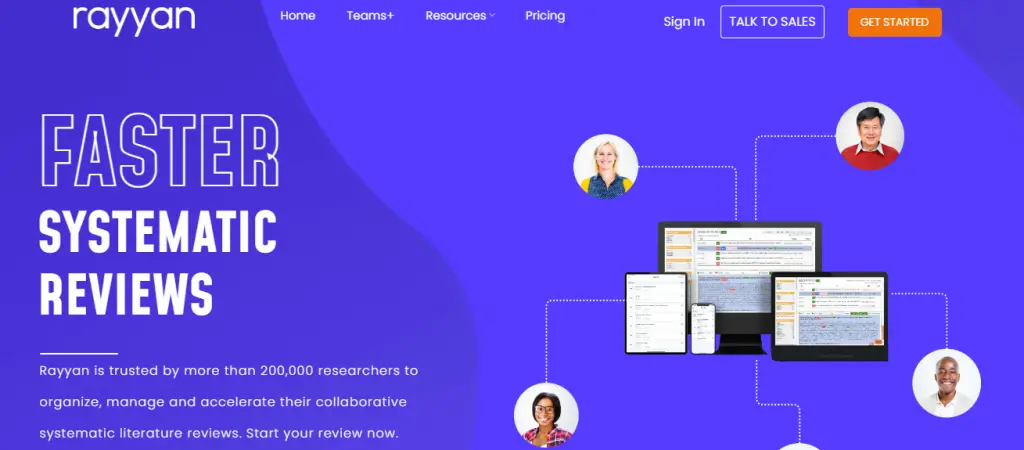
Rayyan is a powerful literature review tool that simplifies the research process for scholars and academics. With its user-friendly interface and efficient management features, Rayyan is highly regarded by researchers worldwide.
It allows users to import and organize large volumes of scholarly articles, making it easier to identify relevant studies for their research projects. The tool also facilitates seamless collaboration among team members, enhancing productivity and streamlining the research workflow.
However, it’s important to be aware of a few aspects. The free version of Rayyan has limitations, and upgrading to a premium subscription may be necessary for additional functionalities.
Users should also be mindful of occasional technical glitches and compatibility issues, promptly reporting any problems. Despite these considerations, Rayyan remains a valuable asset for researchers, providing an effective solution for literature review tasks.
Rayyan offers both free and paid plans:
- Professional: $8.25/month
- Student: $4/month
- Pro Team: $8.25/month
- Team+: $24.99/month
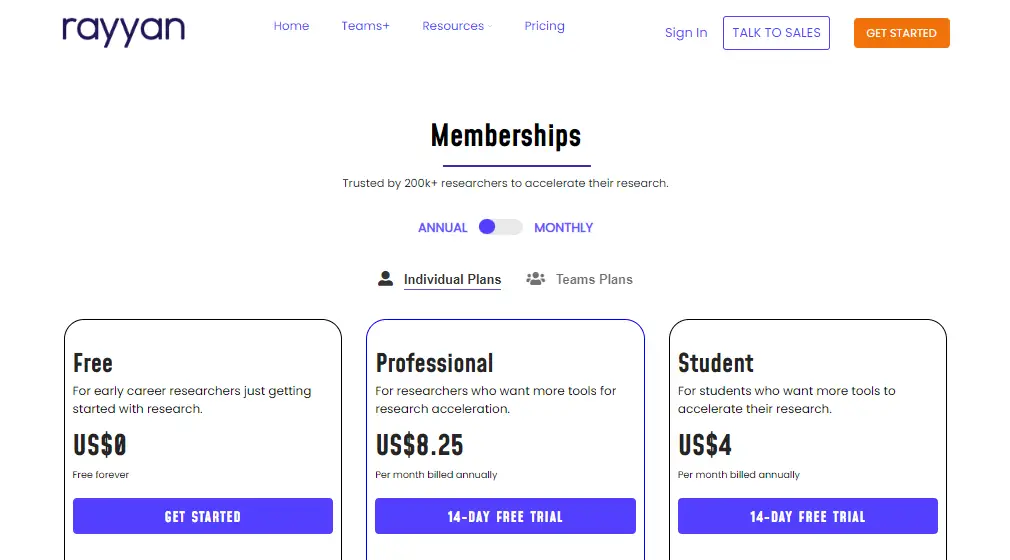
#6. Consensus – Use AI to find you answers in scientific research
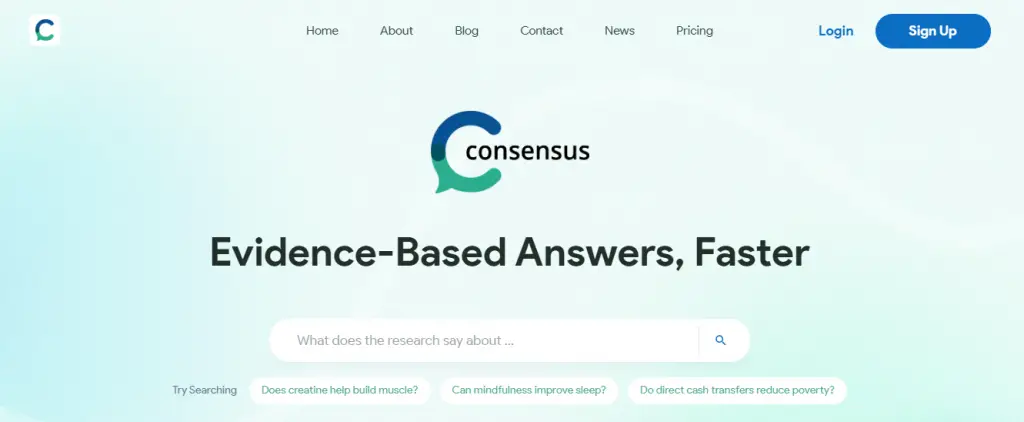
Consensus is a cutting-edge literature review tool that has become a go-to choice for researchers worldwide. Its intuitive interface and powerful capabilities make it a preferred tool for navigating and analyzing scholarly articles.
With Consensus, researchers can save significant time by efficiently organizing and accessing relevant research material.People consider Consensus for several reasons.
Its advanced search algorithms and filters help researchers sift through vast amounts of information, ensuring they focus on the most relevant articles. By streamlining the literature review process, Consensus allows researchers to extract valuable insights and accelerate their research progress.
However, there are a few factors to watch out for when using Consensus. As with any automated tool, researchers should exercise caution and independently verify the accuracy and relevance of the generated results. Complex or niche topics may present challenges, resulting in limited search results. Researchers should also supplement Consensus with manual searches to ensure comprehensive coverage of the literature.
Overall, Consensus is a valuable resource for researchers seeking to optimize their literature review process. By leveraging its features alongside critical thinking and manual searches, researchers can enhance the efficiency and effectiveness of their work, advancing their research endeavors to new heights.
Consensus offers both free and paid plans:
- Premium: $9.99/month
- Enterprise: Custom
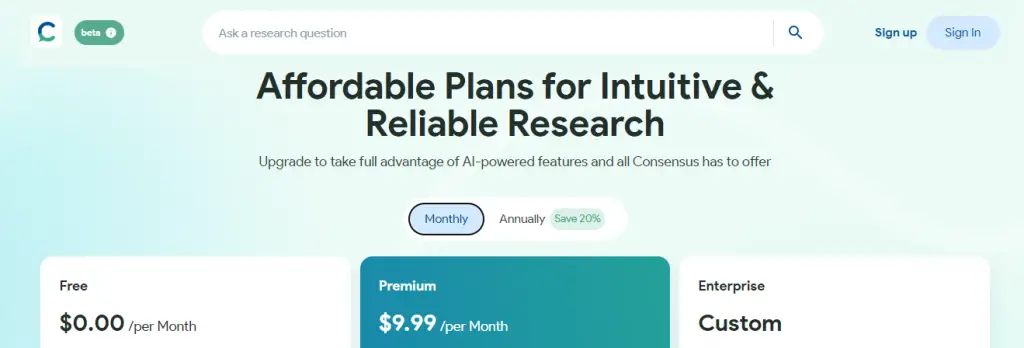
#7. RAx – AI-powered reading assistant
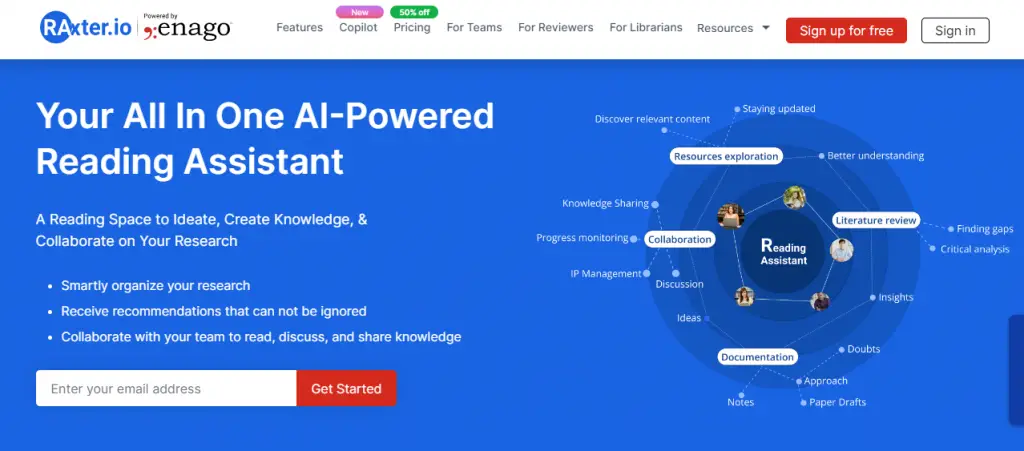
Consensus is a revolutionary literature review tool that has transformed the research process for scholars worldwide. With its user-friendly interface and advanced features, it offers a vast database of academic publications across various disciplines, providing access to relevant and up-to-date literature.
Using advanced algorithms and machine learning, Consensus delivers personalized recommendations, saving researchers time and effort in their literature search.
However, researchers should be cautious of potential biases in the recommendation system and supplement their search with manual verification to ensure a comprehensive review.
Additionally, occasional inaccuracies in metadata have been reported, making it essential for users to cross-reference information with reliable sources. Despite these considerations, Consensus remains an invaluable tool for enhancing the efficiency and quality of literature reviews.
RAx offers both free and paid plans. Currently offering 50% discounts as of July 2023:
- Premium: $6/month $3/month
- Premium with Copilot: $8/month $4/month
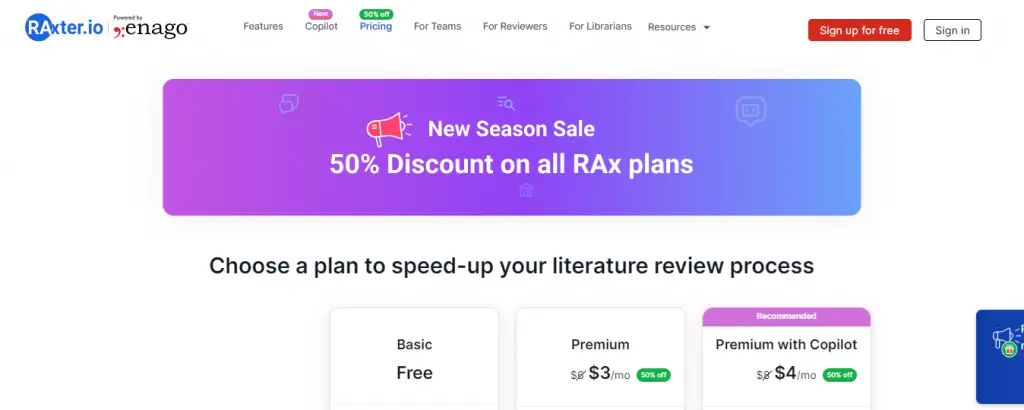
#8. Lateral – Advance your research with AI
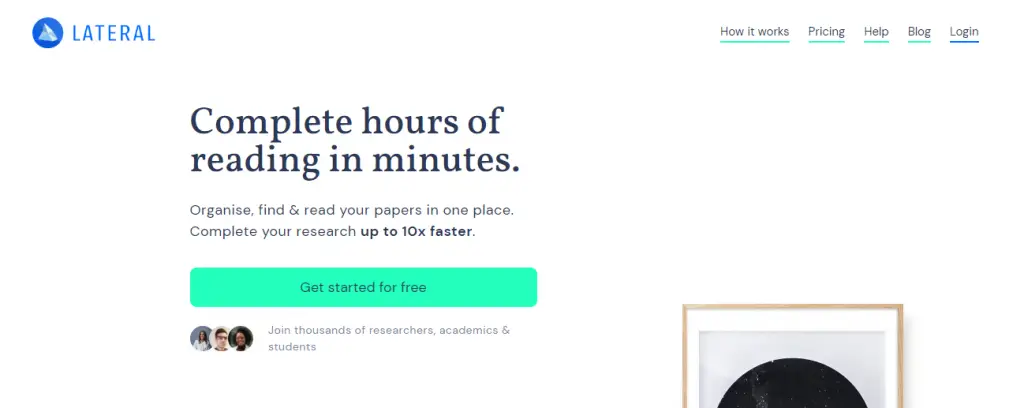
“Lateral” is a revolutionary literature review tool trusted by researchers worldwide. With its user-friendly interface and powerful search capabilities, it simplifies the process of gathering and analyzing scholarly articles.
By leveraging advanced algorithms and machine learning, Lateral saves researchers precious time by retrieving relevant articles and uncovering new connections between them, fostering interdisciplinary exploration.
While Lateral provides numerous benefits, users should exercise caution. It is advisable to cross-reference its findings with other sources to ensure a comprehensive review.
Additionally, researchers must be mindful of potential biases introduced by the tool’s algorithms and should critically evaluate and interpret the results.
Despite these considerations, Lateral remains an indispensable resource, empowering researchers to delve deeper into their fields of study and make valuable contributions to the academic community.
RAx offers both free and paid plans:
- Premium: $10.98
- Pro: $27.46
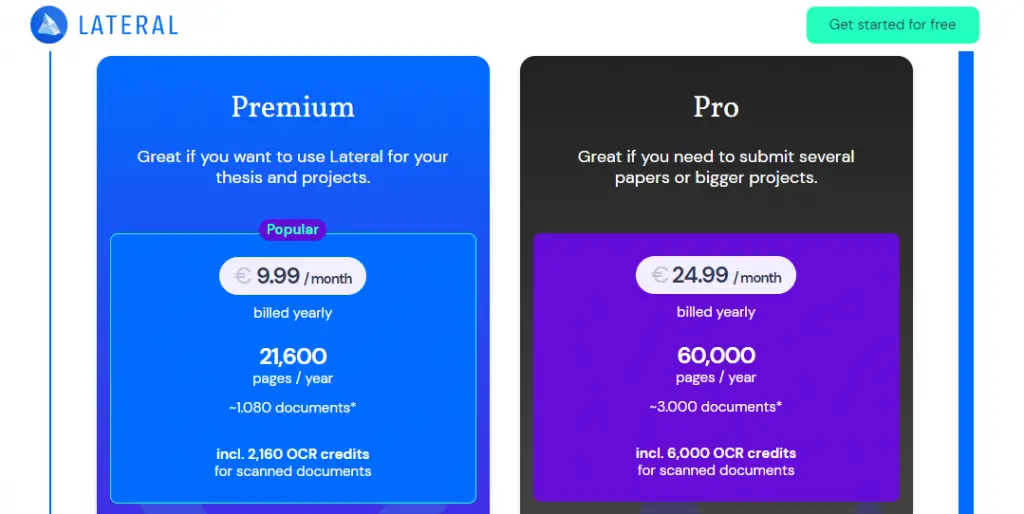
#9. Iris AI – Introducing the researcher workspace
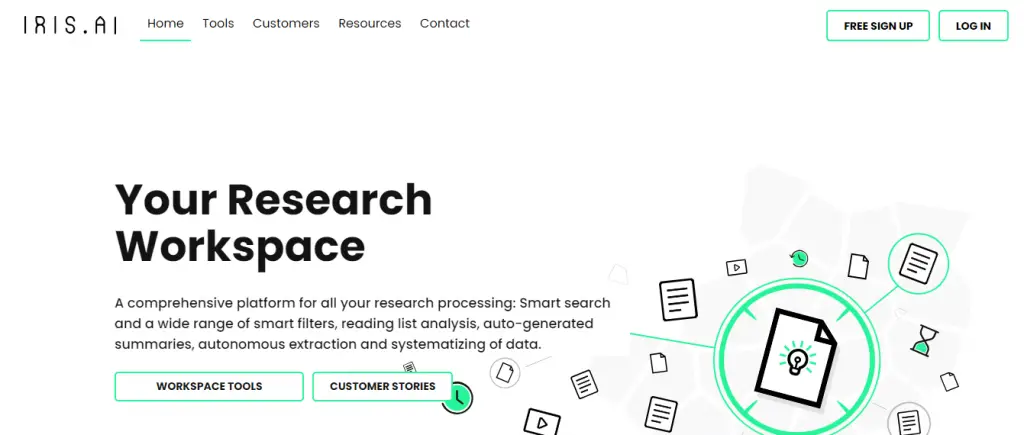
Iris AI is an innovative literature review tool that has transformed the research process for academics and scholars. With its advanced artificial intelligence capabilities, Iris AI offers a seamless and efficient way to navigate through a vast array of academic papers and publications.
Researchers are drawn to this tool because it saves valuable time by automating the tedious task of literature review and provides comprehensive coverage across multiple disciplines.
Its intelligent recommendation system suggests related articles, enabling researchers to discover hidden connections and broaden their knowledge base. However, caution should be exercised while using Iris AI.
While the tool excels at surfacing relevant papers, researchers should independently evaluate the quality and validity of the sources to ensure the reliability of their work.
It’s important to note that Iris AI may occasionally miss niche or lesser-known publications, necessitating a supplementary search using traditional methods.
Additionally, being an algorithm-based tool, there is a possibility of false positives or missed relevant articles due to the inherent limitations of automated text analysis. Nevertheless, Iris AI remains an invaluable asset for researchers, enhancing the quality and efficiency of their research endeavors.
Iris AI offers different pricing plans to cater to various user needs:
- Basic: Free
- Premium: Monthly ($82.41), Quarterly ($222.49), and Annual ($791.07)
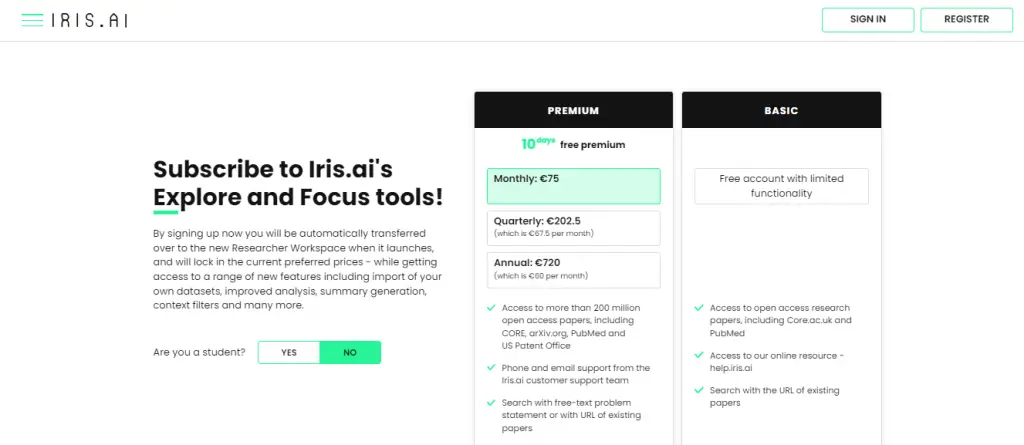
#10. Scholarcy – Summarize your literature through AI
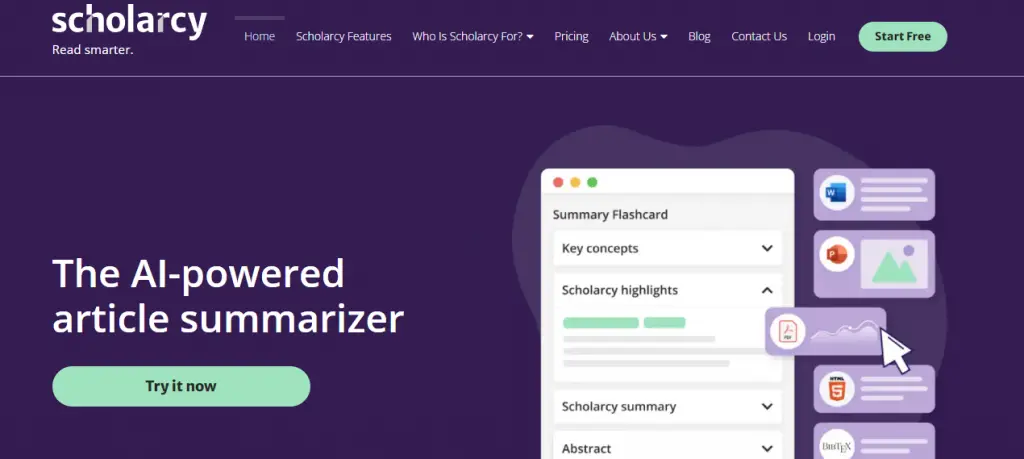
Scholarcy is a powerful literature review tool that helps researchers streamline their work. By employing advanced algorithms and natural language processing, it efficiently analyzes and summarizes academic papers, saving researchers valuable time.
Scholarcy’s ability to extract key information and generate concise summaries makes it an attractive option for scholars looking to quickly grasp the main concepts and findings of multiple papers.
However, it is important to exercise caution when relying solely on Scholarcy. While it provides a useful starting point, engaging with the original research papers is crucial to ensure a comprehensive understanding.
Scholarcy’s automated summarization may not capture the nuanced interpretations or contextual information presented in the full text.
Researchers should also be aware that certain types of documents, particularly those with heavy mathematical or technical content, may pose challenges for the tool.
Despite these considerations, Scholarcy remains a valuable resource for researchers seeking to enhance their literature review process and improve overall efficiency.
Scholarcy offer the following pricing plans:
- Browser Extension and Flashcards: Free
- Personal Library: $9.99
- Academic Institution License: $8K+
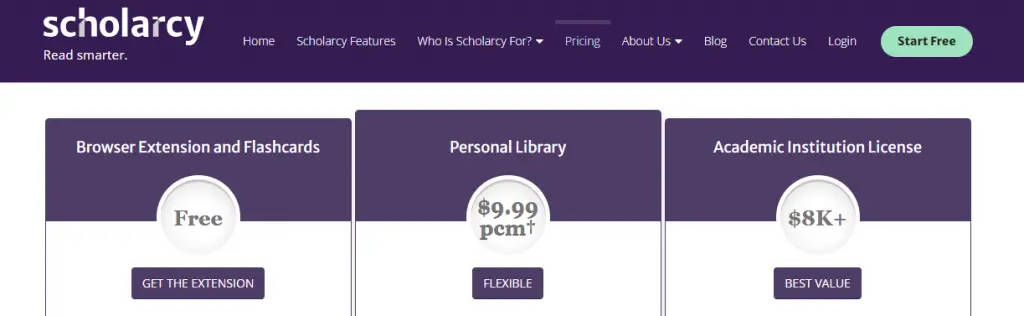
Final Thoughts
In conclusion, conducting a comprehensive literature review is a crucial aspect of any research project, and the availability of reliable and efficient tools can greatly facilitate this process for researchers. This article has explored the top 10 literature review tools that have gained popularity among researchers.
Moreover, the rise of AI-powered tools like Iris.ai and Sci.ai promises to revolutionize the literature review process by automating various tasks and enhancing research efficiency.
Ultimately, the choice of literature review tool depends on individual preferences and research needs, but the tools presented in this article serve as valuable resources to enhance the quality and productivity of research endeavors.
Researchers are encouraged to explore and utilize these tools to stay at the forefront of knowledge in their respective fields and contribute to the advancement of science and academia.
Q1. What are literature review tools for researchers?
Literature review tools for researchers are software or online platforms designed to assist researchers in efficiently conducting literature reviews. These tools help researchers find, organize, analyze, and synthesize relevant academic papers and other sources of information.
Q2. What criteria should researchers consider when choosing literature review tools?
When choosing literature review tools, researchers should consider factors such as the tool’s search capabilities, database coverage, user interface, collaboration features, citation management, annotation and highlighting options, integration with reference management software, and data extraction capabilities.
It’s also essential to consider the tool’s accessibility, cost, and technical support.
Q3. Are there any literature review tools specifically designed for systematic reviews or meta-analyses?
Yes, there are literature review tools that cater specifically to systematic reviews and meta-analyses, which involve a rigorous and structured approach to reviewing existing literature. These tools often provide features tailored to the specific needs of these methodologies, such as:
Screening and eligibility assessment: Systematic review tools typically offer functionalities for screening and assessing the eligibility of studies based on predefined inclusion and exclusion criteria. This streamlines the process of selecting relevant studies for analysis.
Data extraction and quality assessment: These tools often include templates and forms to facilitate data extraction from selected studies. Additionally, they may provide features for assessing the quality and risk of bias in individual studies.
Meta-analysis support: Some literature review tools include statistical analysis features that assist in conducting meta-analyses. These features can help calculate effect sizes, perform statistical tests, and generate forest plots or other visual representations of the meta-analytic results.
Reporting assistance: Many tools provide templates or frameworks for generating systematic review reports, ensuring compliance with established guidelines such as PRISMA (Preferred Reporting Items for Systematic Reviews and Meta-Analyses).
Q4. Can literature review tools help with organizing and annotating collected references?
Yes, literature review tools often come equipped with features to help researchers organize and annotate collected references. Some common functionalities include:
Reference management: These tools enable researchers to import references from various sources, such as databases or PDF files, and store them in a central library. They typically allow you to create folders or tags to organize references based on themes or categories.
Annotation capabilities: Many tools provide options for adding annotations, comments, or tags to individual references or specific sections of research articles. This helps researchers keep track of important information, highlight key findings, or note potential connections between different sources.
Full-text search: Literature review tools often offer full-text search functionality, allowing you to search within the content of imported articles or documents. This can be particularly useful when you need to locate specific information or keywords across multiple references.
Integration with citation managers: Some literature review tools integrate with popular citation managers like Zotero, Mendeley, or EndNote, allowing seamless transfer of references and annotations between platforms.
By leveraging these features, researchers can streamline the organization and annotation of their collected references, making it easier to retrieve relevant information during the literature review process.
Leave a Comment Cancel reply
Save my name, email, and website in this browser for the next time I comment.
We maintain and update science journals and scientific metrics. Scientific metrics data are aggregated from publicly available sources. Please note that we do NOT publish research papers on this platform. We do NOT accept any manuscript.
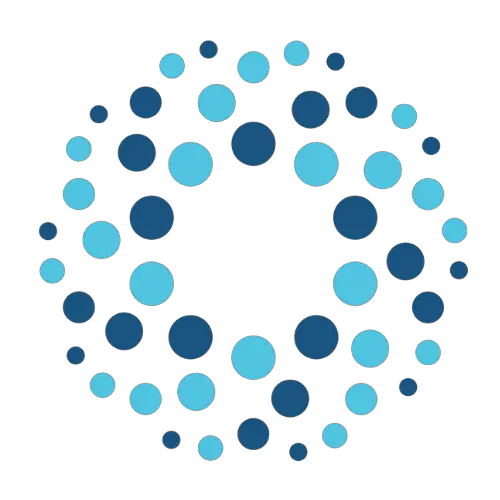
2012-2024 © scijournal.org
Thank you for visiting nature.com. You are using a browser version with limited support for CSS. To obtain the best experience, we recommend you use a more up to date browser (or turn off compatibility mode in Internet Explorer). In the meantime, to ensure continued support, we are displaying the site without styles and JavaScript.
- View all journals
- Explore content
- About the journal
- Publish with us
- Sign up for alerts
- 16 April 2024
Structure peer review to make it more robust
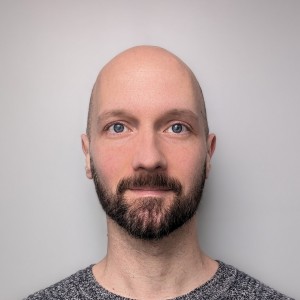
- Mario Malički 0
Mario Malički is associate director of the Stanford Program on Research Rigor and Reproducibility (SPORR) and co-editor-in-chief of the Research Integrity and Peer Review journal.
You can also search for this author in PubMed Google Scholar
You have full access to this article via your institution.
In February, I received two peer-review reports for a manuscript I’d submitted to a journal. One report contained 3 comments, the other 11. Apart from one point, all the feedback was different. It focused on expanding the discussion and some methodological details — there were no remarks about the study’s objectives, analyses or limitations.
My co-authors and I duly replied, working under two assumptions that are common in scholarly publishing: first, that anything the reviewers didn’t comment on they had found acceptable for publication; second, that they had the expertise to assess all aspects of our manuscript. But, as history has shown, those assumptions are not always accurate (see Lancet 396 , 1056; 2020 ). And through the cracks, inaccurate, sloppy and falsified research can slip.
As co-editor-in-chief of the journal Research Integrity and Peer Review (an open-access journal published by BMC, which is part of Springer Nature), I’m invested in ensuring that the scholarly peer-review system is as trustworthy as possible. And I think that to be robust, peer review needs to be more structured. By that, I mean that journals should provide reviewers with a transparent set of questions to answer that focus on methodological, analytical and interpretative aspects of a paper.
For example, editors might ask peer reviewers to consider whether the methods are described in sufficient detail to allow another researcher to reproduce the work, whether extra statistical analyses are needed, and whether the authors’ interpretation of the results is supported by the data and the study methods. Should a reviewer find anything unsatisfactory, they should provide constructive criticism to the authors. And if reviewers lack the expertise to assess any part of the manuscript, they should be asked to declare this.
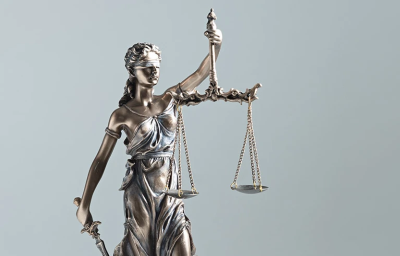
Anonymizing peer review makes the process more just
Other aspects of a study, such as novelty, potential impact, language and formatting, should be handled by editors, journal staff or even machines, reducing the workload for reviewers.
The list of questions reviewers will be asked should be published on the journal’s website, allowing authors to prepare their manuscripts with this process in mind. And, as others have argued before, review reports should be published in full. This would allow readers to judge for themselves how a paper was assessed, and would enable researchers to study peer-review practices.
To see how this works in practice, since 2022 I’ve been working with the publisher Elsevier on a pilot study of structured peer review in 23 of its journals, covering the health, life, physical and social sciences. The preliminary results indicate that, when guided by the same questions, reviewers made the same initial recommendation about whether to accept, revise or reject a paper 41% of the time, compared with 31% before these journals implemented structured peer review. Moreover, reviewers’ comments were in agreement about specific parts of a manuscript up to 72% of the time ( M. Malički and B. Mehmani Preprint at bioRxiv https://doi.org/mrdv; 2024 ). In my opinion, reaching such agreement is important for science, which proceeds mainly through consensus.
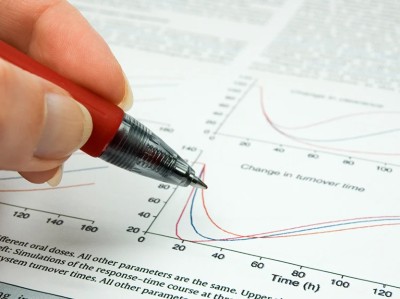
Stop the peer-review treadmill. I want to get off
I invite editors and publishers to follow in our footsteps and experiment with structured peer reviews. Anyone can trial our template questions (see go.nature.com/4ab2ppc ), or tailor them to suit specific fields or study types. For instance, mathematics journals might also ask whether referees agree with the logic or completeness of a proof. Some journals might ask reviewers if they have checked the raw data or the study code. Publications that employ editors who are less embedded in the research they handle than are academics might need to include questions about a paper’s novelty or impact.
Scientists can also use these questions, either as a checklist when writing papers or when they are reviewing for journals that don’t apply structured peer review.
Some journals — including Proceedings of the National Academy of Sciences , the PLOS family of journals, F1000 journals and some Springer Nature journals — already have their own sets of structured questions for peer reviewers. But, in general, these journals do not disclose the questions they ask, and do not make their questions consistent. This means that core peer-review checks are still not standardized, and reviewers are tasked with different questions when working for different journals.
Some might argue that, because different journals have different thresholds for publication, they should adhere to different standards of quality control. I disagree. Not every study is groundbreaking, but scientists should view quality control of the scientific literature in the same way as quality control in other sectors: as a way to ensure that a product is safe for use by the public. People should be able to see what types of check were done, and when, before an aeroplane was approved as safe for flying. We should apply the same rigour to scientific research.
Ultimately, I hope for a future in which all journals use the same core set of questions for specific study types and make all of their review reports public. I fear that a lack of standard practice in this area is delaying the progress of science.
Nature 628 , 476 (2024)
doi: https://doi.org/10.1038/d41586-024-01101-9
Reprints and permissions
Competing Interests
M.M. is co-editor-in-chief of the Research Integrity and Peer Review journal that publishes signed peer review reports alongside published articles. He is also the chair of the European Association of Science Editors Peer Review Committee.
Related Articles
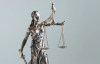
- Scientific community
- Peer review
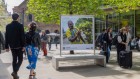
Londoners see what a scientist looks like up close in 50 photographs
Career News 18 APR 24
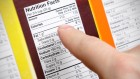
Researchers want a ‘nutrition label’ for academic-paper facts
Nature Index 17 APR 24
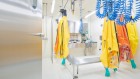
Deadly diseases and inflatable suits: how I found my niche in virology research
Spotlight 17 APR 24
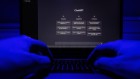
Is ChatGPT corrupting peer review? Telltale words hint at AI use
News 10 APR 24
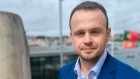
Three ways ChatGPT helps me in my academic writing
Career Column 08 APR 24
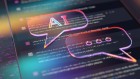
Is AI ready to mass-produce lay summaries of research articles?
Nature Index 20 MAR 24
Associate or Senior Editor, Nature Biomedical Engineering
Associate Editor or Senior Editor, Nature Biomedical Engineering Location: London, Shanghai and Madrid — Hybrid office and remote working Deadline:...
London (Central), London (Greater) (GB)
Springer Nature Ltd
FACULTY POSITION IN PATHOLOGY RESEARCH
Dallas, Texas (US)
The University of Texas Southwestern Medical Center (UT Southwestern Medical Center)
Postdoc Fellow / Senior Scientist
The Yakoub and Sulzer labs at Harvard Medical School-Brigham and Women’s Hospital and Columbia University
Boston, Massachusetts (US)
Harvard Medical School and Brigham and Women's Hospital
Postdoc in Computational Genomics – Machine Learning for Multi-Omics Profiling of Cancer Evolution
Computational Postdoc - Artificial Intelligence in Oncology and Regulatory Genomics and Cancer Evolution at the DKFZ - limited to 2 years
Heidelberg, Baden-Württemberg (DE)
German Cancer Research Center in the Helmholtz Association (DKFZ)
Computational Postdoc
The German Cancer Research Center is the largest biomedical research institution in Germany.
Sign up for the Nature Briefing newsletter — what matters in science, free to your inbox daily.
Quick links
- Explore articles by subject
- Guide to authors
- Editorial policies
Recent advances in deep learning models: a systematic literature review
- Published: 25 April 2023
- Volume 82 , pages 44977–45060, ( 2023 )
Cite this article
- Ruchika Malhotra 1 &
- Priya Singh ORCID: orcid.org/0000-0001-7656-7108 1
1645 Accesses
6 Citations
Explore all metrics
In recent years, deep learning has evolved as a rapidly growing and stimulating field of machine learning and has redefined state-of-the-art performances in a variety of applications. There are multiple deep learning models that have distinct architectures and capabilities. Up to the present, a large number of novel variants of these baseline deep learning models is proposed to address the shortcomings of the existing baseline models. This paper provides a comprehensive review of one hundred seven novel variants of six baseline deep learning models viz. Convolutional Neural Network, Recurrent Neural Network, Long Short Term Memory, Generative Adversarial Network, Autoencoder and Transformer Neural Network. The current review thoroughly examines the novel variants of each of the six baseline models to identify the advancements adopted by them to address one or more limitations of the respective baseline model. It is achieved by critically reviewing the novel variants based on their improved approach. It further provides the merits and demerits of incorporating the advancements in novel variants compared to the baseline deep learning model. Additionally, it reports the domain, datasets and performance measures exploited by the novel variants to make an overall judgment in terms of the improvements. This is because the performance of the deep learning models are subject to the application domain, type of datasets and may also vary on different performance measures. The critical findings of the review would facilitate the researchers and practitioners with the most recent progressions and advancements in the baseline deep learning models and guide them in selecting an appropriate novel variant of the baseline to solve deep learning based tasks in a similar setting.
This is a preview of subscription content, log in via an institution to check access.
Access this article
Price includes VAT (Russian Federation)
Instant access to the full article PDF.
Rent this article via DeepDyve
Institutional subscriptions
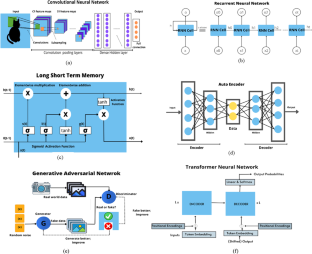
Similar content being viewed by others
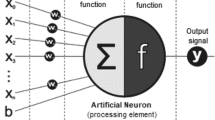
Deep Learning: A Comprehensive Overview on Techniques, Taxonomy, Applications and Research Directions
Iqbal H. Sarker
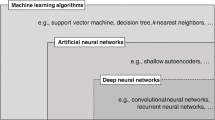
Machine learning and deep learning
Christian Janiesch, Patrick Zschech & Kai Heinrich
CBAM: Convolutional Block Attention Module
Data availability.
Data sharing is not applicable to this article as this is a review article. The detail of the selected primary studies is presented in Table 3 .
Abbreviations
Deep Leering
- Autoencoder
- Convolutional Neural Network
- Recurrent Neural Network
- Generative Adversarial Network
- Long Short-Term Memory
- Transformer Neural Network
Deep Learning Models
Systematic Literature Review
Novel Variant
Aberbour M, Mehrez H (1998) Architecture and design methodology of the RBF-DDA neural network. 1998 IEEE International Symposium on Circuits and Systems (ISCAS). 3:199–202. https://doi.org/10.1109/ISCAS.1998.703974
Ainslie J, Ontanon S, Alberti C, Cvicek V, Fisher Z, Pham P, Yang L (2020) ETC: Encoding long and structured inputs in transformers. arXiv preprint arXiv:2004.08483
Akhtar MM, Shatat RSA, Shatat ASA et al (2022) IoMT-based smart healthcare monitoring system using adaptive wavelet entropy deep feature fusion and improved RNN. Multimed Tools Appl. https://doi.org/10.1007/s11042-022-13934-5
Alexia JM (2018) The relativistic discriminator: a key element missing from standard GAN. International Conference on Learning Representations
Al-Sarem M, Boulila W, Al-Harby M, Qadir J, Alsaeedi A (2019) Deep learning-based rumor detection on microblogging platforms: A systematic review. IEEE Access 7:152788–152812. https://doi.org/10.1109/ACCESS.2019.2947855
Article Google Scholar
Alzubaidi L, Zhang J, Humaidi AJ et al (2021) Review of deep learning: concepts, CNN architectures, challenges, applications, future directions. J Big Data 8:53. https://doi.org/10.1186/s40537-021-00444-8
Amjady N (2001) Short-term hourly load forecasting using time-series modeling with peak load estimation capability. IEEE Trans Power Syst 16(4):798–805. https://doi.org/10.1109/59.962429
Arjovsky M, Chintala S, Bottou L (2017) Wasserstein generative adversarial networks. Int Conf Mach Learn 17:214–223
Google Scholar
Atassi A, Azami IEl, Sadiq A (2018) The new deep learning architecture based on GRU and word2vec. International Conference on Electronics, Control, Optimization and Computer Science (ICECOCS). https://doi.org/10.1109/ICECOCS.2018.8610611
Aygun RC, Yavuz AG (2017) Network anomaly detection with stochastically improved autoencoder based models. IEEE 4th International Conference on Cyber Security and Cloud Computing (CSCloud). IEEE, 2017. https://doi.org/10.1109/CSCloud.2017.39
Aziz MF, Mostafa SA, Mohd CF et al (2022) Integrating Elman recurrent neural network with particle swarm optimization algorithms for an improved hybrid training of multidisciplinary datasets. Expert Syst Appl 183(115441):0957–4174. https://doi.org/10.1016/j.eswa.2021.115441
Bhaskar, S, Thasleema TM. LSTM model for visual speech recognition through facial expressions. Multimed Tools Appl. https://doi.org/10.1007/s11042-022-12796-1
Biolchini L, Mian PG (2005) Systematic review in software engineering. System Engineering and Computer Science Department COPPE/UFRJ
Brock A, Jeff D, Karen S (2018) Large scale GAN training for high fidelity natural image synthesis. Large scale GAN training for high fidelity natural image synthesis
Buettner R, Bilo M, Bay N, Zubac T (2020) A systematic literature review of medical image analysis using deep learning. IEEE Symp Ind Electron Appl 1:4. https://doi.org/10.1109/ISIEA49364.2020.9188131
Cai C, Gou B, Khishe M et al (2023) Improved deep convolutional neural networks using chimp optimization algorithm for Covid19 diagnosis from the X-ray images. Expert Syst Appl 213:119206. https://doi.org/10.1016/j.eswa.2022.119206
Can Aygun R, Gokhan Yavuz A (2017) Network anomaly detection with stochastically improved autoencoder based models. 2017 IEEE 4th International Conference on Cyber Security and Cloud Computing (CSCloud). https://doi.org/10.1109/CSCloud.2017.39
Chen HF (2010) New approach to recursive identification for ARMAX systems. IEEE Trans Autom Control 55(4):868–879. https://doi.org/10.1109/TAC.2010.2041997
Article MathSciNet MATH Google Scholar
Chen G, Hu L, Zhang Q, Ren Z, Gao X, Cheng J (2020) ST-LSTM: Spatio-temporal graph based long short-term memory network for vehicle trajectory prediction. 2020 IEEE International Conference on Image Processing (ICIP). 608-612. https://doi.org/10.1109/ICIP40778.2020.9191332
Cheng Z, Wang S, Liu X, Zhu E (2021) Improved autoencoder for unsupervised anomaly detection. Int J Intell Syst 36(12):7103–7125. https://doi.org/10.1002/int.22582
Child R, Gray S, Radford A, Sutskever I (2019) Generating long sequences with sparse transformers. arXiv preprint arXiv:1904.10509
Choromanski K, Likhosherstov V, Dohan D, Song X, Gane A, Sarlos T, Weller A (2020) Rethinking attention with performers. International Conference on Learning Representations
Ciresan DC, Meier U, Gambardella LM, Schmidhuber J (2010) Deep big simple neural nets excel on hand-written digit recognition. Neural Comput 22:12. https://doi.org/10.1162/NECO_a_00052
Clauwaert J, Waegeman W (2022) Novel transformer networks for improved sequence labeling in genomics. IEEE/ACM Trans Comput Biol Bioinf 19(1):97–106. https://doi.org/10.1109/TCBB.2020.3035021
Connor JT, Martin RD, Atlas LE (1994) Recurrent neural networks and robust time series prediction. IEEE Trans Neural Networks 5(2):240–254. https://doi.org/10.1016/j.oceaneng.2020.107704
Cui J, Gao Q, Li D (2019) Improved long short-term memory network based short term load forecasting 2019 Chinese Automation Congress (CAC). 4428–4433. https://doi.org/10.1109/CAC48633.2019.8996379
Dai Z, Lai G, Yang Y, Le Q (2020) Funnel-transformer: Filtering out sequential redundancy for efficient language processing. Adv Neural Inf Process Syst 33:4271–4282
Das M, Pratama M, Ashfahani A, Samanta S (2019) FERNN: A fast and evolving recurrent neural network model for streaming data classification. International Joint Conference on Neural Networks (IJCNN), 1–8. https://doi.org/10.1109/IJCNN.2019.8851757
Dehuri S, Roy R, Cho SB, Ghosh A (2012) An improved swarm optimized functional link artificial neural network (ISO-FLANN) for classification. J Syst Softw 85(6):1333–1345. https://doi.org/10.1016/j.jss.2012.01.025
Denton E, Chintala S, Szlam A, Fergus R (2015) Deep generative image models using a Laplacian pyramid of adversarial networks. In: Proceedings of the 28th International Conference on Neural Information Processing Systems 1:1486–1494
Devlin J, Chang MW, Lee K, Toutanova K (2019) BERT: Pre-training of deep bidirectional transformers for language understanding. In: Proceedings of HLT-NAACL. Minneapolis, Minnesota. 4171–4186. https://doi.org/10.18653/v1/N19-1423
Ding Z, Liu XY, Yin M et al (2019) Tgan: Deep tensor generative adversarial nets for large image generation. International Conference on Learning Representations
Fang X, Zhang W, Guo Y, Wang J, Wang M, Li S (2022) A novel reinforced deep RNN–LSTM Algorithm: Energy management forecasting case study. IEEE Trans Industr Inf 18(8):5698–5704. https://doi.org/10.1109/TII.2021.3136562
Fetanat M, Stevens M, Jain P, Hayward C, Meijering E, Lovell NH (2022) Fully Elman Neural Network: A novel deep recurrent neural network optimized by an improved harris hawks algorithm for classification of pulmonary arterial Wedge Pressure. IEEE Trans Biomed Eng 69(5):1733–1744. https://doi.org/10.1109/TBME.2021.3129459
Gao F et al (2018) SD-CNN: A shallow-deep CNN for improved breast cancer diagnosis. Comput Med Imaging Graph 70:53–62
Gavrilescu R et al (2018) Faster R-CNN: an approach to real-time object detection. 2018 International Conference and Exposition on Electrical and Power Engineering (EPE). https://doi.org/10.1109/ICEPE.2018.8559776
Geng Z, Chen Z, Meng Q, Han Y (2022) Novel transformer based on gated convolutional neural network for dynamic soft sensor modeling of industrial processes. IEEE Trans Industr Inf 18(3):1521–1529. https://doi.org/10.1109/TII.2021.3086798
Gnanha AT, Cao W, Mao X, Wu S, Wong HS, Li Q (2022) The residual generator: An improved divergence minimization framework for GAN. Pattern Recognit 121:108222. https://doi.org/10.1016/j.patcog.2021.108222
Gong W, Chen H, Zhang Z, Zhang M, Wang R, Guan C, Wang Q (2019) A novel deep learning method for intelligent fault diagnosis of rotating machinery based on improved CNN-SVM and multichannel data fusion. Sensors 19(7):1693
Gu S, Feng Y (2019) Improving multi-head attention with capsule networks. In: Proceedings of NLPCC. 314–326. https://doi.org/10.1007/978-3-030-32233-5_25
Gu Q, Huang Z (2022) An improved convolutional neural network for wind turbine bearing fault diagnosis research method. In Proceedings of the 7th International Conference on Cyber Security and Information Engineering (ICCSIE '22). Association for Computing Machinery, New York, NY, USA, 725–729. https://doi.org/10.1145/3558819.3565179
Guan J, Pan C, Li S, Yu D (2019) Srdgan: learning the noise prior for super resolution with dual generative adversarial networks. International Conference on Learning Representations
Guo M, Zhang Y, Liu T (2019) Gaussian transformer: a lightweight approach for natural language inference. Proc AAAI Conf Artif Intell 33(1):6489–6496. https://doi.org/10.1609/aaai.v33i01.33016489
Guo Q, Qiu X, Xue X, Zhang Z (2019) Low-rank and locality constrained self-attention for sequence modeling. IEEE/ACM Trans Audio Speech Lang Process 27(12):2213–2222. https://doi.org/10.1109/TASLP.2019.2944078
Hah J, Lee W, Lee J, Par S (2018) Information-based boundary equilibrium generative adversarial networks with interpretable representation learning. Comput Intell Neurosci 2018:6465949. https://doi.org/10.1155/2018/6465949
Han L, Musunuri SH, Min MR, Gao R, Tian Y, Metaxas D (2022) AE-StyleGAN: Improved training of style-based autoencoders. IEEE/CVF Winter Conference on Applications of Computer Vision (WACV). 955–964. https://doi.org/10.1109/WACV51458.2022.00103
He W (2017) Load forecasting via deep neural networks. Procedia Comput Sci 122:308–314. https://doi.org/10.1016/j.procs.2017.11.374
He K, Zhang X, Ren S, Sun J (2016) Deep residual learning for image recognition. IEEE Conference on Computer Vision and Pattern Recognition (CVPR), 770–778. https://doi.org/10.1109/CVPR.2016.90
He Z, Shao H, Zhang X, Cheng J, Yang Y (2019) Improved deep transfer autoencoder for fault diagnosis of gearbox under variable working conditions with small training samples. IEEE Access 7:115368–115377. https://doi.org/10.1109/ACCESS.2019.2936243
He YL, Chen L, Gao Y, Ma JH, Xu Y, Zhu QX (2022) Novel double-layer bidirectional LSTM network with improved attention mechanism for predicting energy consumption. ISA Trans 127:350–360. https://doi.org/10.1016/j.isatra.2021.08.030
Heo YJ, Yeo WH, Kim BG (2022) DeepFake detection algorithm based on improved vision transformer. Appl Intell 53:7512–7527. https://doi.org/10.1007/s10489-022-03867-9
Hsu W, Zhang Y, Glass J (2016) A prioritized grid long short-term memory RNN for speech recognition. 2016 IEEE Spoken Language Technology Workshop (SLT), 467-473. https://doi.org/10.1109/SLT.2016.7846305
Hu L, Taylor G (2014) A novel hybrid technique for short-term electricity price forecasting in UK electricity markets. J Int Counc Electr Eng 4(2):114–120
Huang K, Wang X (2021) ADA-INCVAE: Improved data generation using variational autoencoder for imbalanced classification. Appl Intell 52:2838–28531. https://doi.org/10.1007/s10489-021-02566-1
Huang K, Wang X (2022) ADA-INCVAE: Improved data generation using variational autoencoder for imbalanced classification. Appl Intell 52:2838–2853. https://doi.org/10.1007/s10489-021-02566-1
Huang H, Li L, Ma H (2022) An improved cascade R-CNN-Based target detection algorithm for UAV Aerial Images, 7th International Conference on Image, Vision and Computing (ICIVC), 232–237. https://doi.org/10.1109/ICIVC55077.2022.9886321
Huang W, Gao X, Huang Y et al (2022) Improved convolutional neural network for laser welding defect prediction. Int J Precis Eng Manuf. https://doi.org/10.1007/s12541-022-00729-9
Isola P et al (2017) Image-to-image translation with conditional adversarial networks. Proceedings of the IEEE conference on computer vision and pattern recognition
Jabeen G, Rahim S, Afzal W et al (2022) Machine learning techniques for software vulnerability prediction: a comparative study. Appl Intell 52:17614–17635. https://doi.org/10.1007/s10489-022-03350-5
Joshy AA, Rajan R (2022) Automated dysarthria severity classification: A study on acoustic features and deep learning techniques. IEEE Trans Neural Syst Rehabil Eng 30:1147–1157. https://doi.org/10.1109/TNSRE.2022.3169814
Jung W et al (2019) Restructuring batch normalization to accelerate CNN training. Proc Mach Learn Syst 1:14–26
Karabayir I, Akbilgic O, Tas N (2021) A Novel Learning Algorithm to Optimize Deep Neural Networks: Evolved Gradient Direction Optimizer (EVGO). IEEE Trans Neural Netw Learn Syst 32(2):685–694. https://doi.org/10.1109/TNNLS.2020.2979121
Article MathSciNet Google Scholar
Karras T et al (2017) Progressive growing of gans for improved quality, stability, and variation. International Conference on Learning Representations
Karras T, Laine S, Aila T (2021) A Style-Based Generator Architecture for Generative Adversarial Networks. IEEE Trans Pattern Anal Mach Intell 42(12):4217–4228. https://doi.org/10.1109/TPAMI.2020.2970919
Khamparia A, Singh KM (2019) A systematic review on deep learning architectures and applications. Expert Systems 36:e12400. https://doi.org/10.1111/exsy.12400
Kisan K, Jatoth RK (2021) A new training scheme for neural network based non-linear channel equalizers in wireless communication system using Cuckoo Search Algorithm. AEU-Int J Electron Commun 138:153371. https://doi.org/10.1016/j.aeue.2020.153371
Kitchenham B, Brereton OP (2009) Systematic literature reviews in software engineering -a systematic literature review. Inf Softw Technol 51(1):7–17. https://doi.org/10.1016/j.infsof.2008.09.009
Krizhevsky A, Ilya S, Hinton GE (2012) Imagenet classification with deep convolutional neural networks. Adv Neural Inf Process Syst 60(6):84–90. https://doi.org/10.1145/3065386
Kumar A, Kumari P (2021) A pragmatic approach to face recognition using a novel deep learning algorithm. Inernational Conference on Advance Computing and Innovative Technologies in Engineering (ICACITE). 806–810. https://doi.org/10.1109/ICACITE51222.2021.9404697 .
Kumar N, Sukavanam N (2020) An improved CNN framework for detecting and tracking human body in unconstraint environment. Knowl Based Syst 193:105198. https://doi.org/10.1016/j.knosys.2019.105198
Kumar M, Mukherjee P, Verma K, Verma S, Rawat DB (2022) Improved deep convolutional neural network based malicious node detection and energy-efficient data transmission in wireless sensor networks. IEEE Trans Netw Sci Eng 9(5):3272–3281. https://doi.org/10.1109/TNSE.2021.3098011
Kuo PH, Huang CJ (2018) A high precision artificial neural networks model for short-term energy load forecasting. Energies 11(1):213. https://doi.org/10.3390/en11010213
Lata K, Dave M, Nishanth KN (2019) Image-to-Image translation using generative adversarial network. International conference on Electronics, Communication and Aerospace Technology (ICECA). 186–189. https://doi.org/10.1109/ICECA.2019.8822195
Ledig C et al (2017) Photo-realistic single image super-resolution using a generative adversarial network. IEEE Conference on Computer Vision and Pattern Recognition (CVPR), 2017, 105-114. https://doi.org/10.1109/CVPR.2017.19
Li X, Meng Y, Zhou M, Han Q, Wu F, Li J (2020) SAC: accelerating and structuring self-attention via sparse adaptive connection. In Proceedings of the 34th International Conference on Neural Information Processing Systems (NIPS'20). Curran Associates Inc., Red Hook, NY, USA. 426:16997–17008. https://doi.org/10.5555/3495724.3497150
Li Y, Zhou Z, Sun C, Chen X, Yan R (2022) Variational Attention-Based Interpretable Transformer Network for Rotary Machine Fault Diagnosis. In: IEEE Transactions on Neural Networks and Learning Systems. https://doi.org/10.1109/TNNLS.2022.3202234
Li J, Lu Y, Xu Z, Li S, Qian L (2022) MILP: A memory improved LSTM prediction algorithm for gradient transmission time in distributed deep learning. ICC 2022 - IEEE International Conference on Communications, 4462–4467
Li W, Chen J, Wang Z, Shen Z, Ma C and Cui X (2022). IFL-GAN: Improved federated learning generative adversarial network with maximum mean discrepancy model Aggregation. IEEE Trans Neural Netw Learn Syst. https://doi.org/10.1109/TNNLS.2022.3167482
Li C, Pan C, Chen F, Li J, Fu S, Zeng W (2022) A handwritten number recognition scheme based on improved convolutional neural network algorithm. Advances in artificial intelligence and security communications in computer and Information Science 1586. Springer. https://doi.org/10.1007/978-3-031-06767-9_33
Li Y, Xiao N, Ouyang W (2018) Improved boundary equilibrium generative adversarial networks. IEEE Access 6:11342–11348. https://doi.org/10.1109/ACCESS.2018.2804278
Li J, Yao P, Guo L, Zhang W (2019) Boosted transformer for image captioning. Appl Sci 9(16):3260. https://doi.org/10.3390/app9163260
Li Q, Zhao Y, Yu F (2020) A novel multichannel long short-term memory method with time series for soil temperature modeling. IEEE Access 8:182026–182043. https://doi.org/10.1109/ACCESS.2020.3028995
Li L, Kameoka H, Makino S (2022) FastMVAE2: On Improving and accelerating the fast variational autoencoder-based source separation algorithm for determined mixtures. IEEE/ACM Trans Audio Speech Lang Process 31:96–110. https://doi.org/10.1109/TASLP.2022.3214763
Li Y, Yang S, Zheng Y, Lu H (2022) Improved point-voxel region convolutional neural network: 3D object detectors for autonomous driving. IEEE Trans Intell Transp Syst 23(7):9311–9317. https://doi.org/10.1109/TITS.2021.3071790
Li X, Wei J, Jiao H (2022) Real-time tracking algorithm for aerial vehicles using improved convolutional neural network and transfer learning. IEEE Trans Intell Transp Syst 23(3):2296–2305. https://doi.org/10.1109/TITS.2021.3072872
Lin M, Qiang C, Shuicheng Y (2013) Network in network. Neural and Evolutionary Computing. https://doi.org/10.48550/arXiv.1312.4400
Lin T, Wang Y, Liu X, Qiu X (2022) A survey of transformers. AI Open 3:111–132. https://doi.org/10.1016/j.aiopen.2022.10.001
Liu B et al (2020) Traffic flow combination forecasting method based on improved LSTM and ARIMA. Int J Embedded Syst 12(1):22–30
Liu W, You J, Lee J (2021) HSIGAN: A Conditional hyperspectral image synthesis method with auxiliary classifier. IEEE J Sel Top Appl Earth Obs Remote Sens 14:330–3344. https://doi.org/10.1109/JSTARS.2021.3063911
Lodha I, Kolur L, Krishnan K, Dheenadayalan K, Sitaram D, Nandi S (2022) Cost-optimized video transfer using real-time super resolution convolutional neural networks. In: 5th Joint International Conference on Data Science & Management of Data (9th ACM IKDD CODS and 27th COMAD). Association for Computing Machinery, New York, NY, USA, 213–221. https://doi.org/10.1145/3493700.3493731
Lu S et al (2019) Psgan: A minimax game for personalized search with limited and noisy click data. Proceedings of the 42nd International ACM SIGIR Conference on Research and Development in Information Retrieval
Lu S, Dou Z, Jun X, Nie JY, Wen JR (2019) Psgan: A minimax game for personalized search with limited and noisy click data. Proceedings of the 42nd International ACM SIGIR Conference on Research and Development in Information Retrieval. 555–564. https://doi.org/10.1145/3331184.3331218
Luo X, Li J, Chen M, Yang X, Li X (2021) Ophthalmic Disease Detection via Deep Learning with a Novel Mixture Loss Function. IEEE J Biomed Health Inform 25(9):3332–3339. https://doi.org/10.1109/JBHI.2021.3083605
Lv W, Xiong J, Shi J et al (2021) A deep convolution generative adversarial networks based fuzzing framework for industry control protocols. J Intell Manuf 32:441–457. https://doi.org/10.1007/s10845-020-01584-z
Ma L, Jia X, Sun Q, Schiele B, Tuytelaars T, Van G (2017) Pose guided person image generation. Advances in neural information processing systems
Ma J et al (2013) Improved GaN-based LED grown on silicon (111) substrates using stress/dislocation-engineered interlayers. J Cryst Growth 370:265–268. https://doi.org/10.1016/j.jcrysgro.2012.10.028
Martín A, Camacho D (2022) Recent advances on effective and efficient deep learning-based solutions. Neural Comput & Applic 34:10205–10210. https://doi.org/10.1007/s00521-022-07344-9
Mashudi NA, Ahmad N, Noor NM (2022) LiWGAN: A Light Method to Improve the Performance of Generative Adversarial Network. IEEE Access 10:93155–93167. https://doi.org/10.1109/ACCESS.2022.3203065
McDowall TM, Ham FM (1997) Robust partial least-squares regression: A modular neural network approach. Appl Sci Artif Neural Netw 3077:344–355. https://doi.org/10.1117/12.271496
Article MATH Google Scholar
Mirza M, Osindero S (2014) Conditional generative adversarial nets. Computer Vision and Pattern Recognition
Mittal V, Gangodkar D, Pant B (2021) Deep Graph-Long Short-Term Memory: A Deep Learning Based Approach for Text Classification. Wireless Pers Commun 119:2287–2301. https://doi.org/10.1007/s11277-021-08331-4
Moon T, Choi H, Lee H, Song I (2015) Rnndrop: A novel dropout for rnns in asr. Automatic Speech Recognition and Understanding. 65–70, https://doi.org/10.1109/ASRU.2015.7404775
Mou L, Zhao P, Xie H, Chen H (2018) T-LSTM: A Long Short-Term Memory Neural Network Enhanced by Temporal Information for Traffic Flow Prediction. IEEE Access 7:98053–98060. https://doi.org/10.1109/ACCESS.2019.2929692
Nagabushanam P, George ST, Radha S (2020) EEG signal classification using LSTM and improved neural network algorithms. Soft Comput 24(13):9981–10003. https://doi.org/10.1007/s00500-019-04515-0
Neifar N, Mdhaffar A, Hamadou AB, Jmaiel M, and Freisleben B (2022) Disentangling temporal and amplitude variations in ECG synthesis using anchored GANs. In: Proceedings of the 37th ACM/SIGAPP Symposium on Applied Computing. https://doi.org/10.1145/3477314.3507300
Nguyen TT, Nguyen ND, Nahavandi S (2020) Deep Reinforcement Learning for Multiagent Systems: A Review of Challenges, Solutions, and Applications. IEEE Trans Cybern 50(9):3826–3839. https://doi.org/10.1109/TCYB.2020.2977374
Ni Q, Cao X (2022) MBGAN: An improved generative adversarial network with multi-head self-attention and bidirectional RNN for time series imputation. Eng Appl Artif Intell 115:105232. https://doi.org/10.1016/j.engappai.2022.105232
Odena A, Olah C, Shlens J (2017) Conditional image synthesis with auxiliary classifier gans. Int Conf Mach Learn 70(2642):2651
Ogundokun RO, Maskeliunas R, Misra S, Damaševičius R (2022). Improved CNN based on batch normalization and adam optimizer. In: Computational Science and Its Applications – ICCSA 2022 Workshops. ICCSA 2022. Lecture Notes in Computer Science, 13381. https://doi.org/10.1007/978-3-031-10548-7_43
Otter DW, Medina JR, Kalita JK (2021) A survey of the usages of deep learning for natural language processing. IEEE Trans Neural Netw Learn Syst 32(2):604–624. https://doi.org/10.1109/TNNLS.2020.2979670
Pal SK, Pramanik A, Maiti J et al (2021) Deep learning in multi-object detection and tracking: state of the art. Appl Intell 51:6400–6429. https://doi.org/10.1007/s10489-021-02293-7
Pandey A, Wang D (2022) Self-Attending RNN for speech enhancement to improve cross-corpus generalization. IEEE/ACM Trans Audio Speech Lang Process 30:1374–1385. https://doi.org/10.1109/TASLP.2022.3161143
Pandey A, Wang D (2022) Self-Attending RNN for speech enhancement to improve cross-corpus generalization. ACM Trans Audio Speech Lang Process 30:1374–1385. https://doi.org/10.1109/TASLP.2022.3161143
Playout C, Renaud D, Farida C (2018) A multitask learning architecture for simultaneous segmentation of bright and red lesions in fundus images. International Conference on Medical Image Computing and Computer-Assisted Intervention 11071. https://doi.org/10.1007/978-3-030-00934-2_12
Price SR, Steven RP, and Derek TA (2019) Introducing fuzzy layers for deep learning. IEEE International Conference on Fuzzy Systems (FUZZ-IEEE). https://doi.org/10.1109/FUZZ-IEEE.2019.8858790
Qing Y, Liu W, Feng L, Gao W (2021) Improved transformer net for hyperspectral image classification. Remote Sensing 13(11):2216. https://doi.org/10.3390/rs13112216
Qipeng G, Xipeng G, Pengfei L, Yunfan S, Xiangyang X, Zheng Z (2019) Star-transformer. In: Proceedings of HLT-NAACL. 1315–1325. https://doi.org/10.18653/v1/N19-1133
Qiu D, Cheng Y, Wang X (2022) Improved generative adversarial network for retinal image super-resolution. Comput Methods Programs Biomed 225(106995):10169–12607. https://doi.org/10.1016/j.cmpb.2022.106995
Rakotonirina NC and Rasoanaivo A (2020) ESRGAN+ : Further improving enhanced super-resolution generative adversarial network. IEEE International Conference on Acoustics, Speech and Signal Processing (ICASSP). 3637–3641. https://doi.org/10.1109/ICASSP40776.2020.9054071
Reis AFD, Medjahdi Y, Chang BS, Sublime J, Brante G, Bader CF (2022) Low Complexity LSTM-NN-Based Receiver for Vehicular Communications in the Presence of High-Power Amplifier Distortions. IEEE Access 10:121985–122000. https://doi.org/10.1109/ACCESS.2022.3223113
Roy A, Saffar M, Vaswani A, Grangier D (2021) Efficient content-based sparse attention with routing transformers. Trans Assoc Comput Linguist 9:53–68
Sermanet P, Eigen D, Zhang X, Mathieu M, Fergus R, LeCun Y (2013) Overfeat: Integrated recognition, localization and detection using convolutional networks. International Conference on Learning Representations
Serradilla O, Zugasti E, Rodriguez J et al. (2022) Deep learning models for predictive maintenance: a survey, comparison, challenges and prospects. Appl Intell. https://doi.org/10.1007/s10489-021-03004-y
She J, Gong S, Yang S, Yang H, Lu S (2022) Xigmoid: An approach to improve the gating mechanism of RNN. International Joint Conference on Neural Networks (IJCNN). 1–1. https://doi.org/10.1109/IJCNN55064.2022.9892346
Shi Y, Li Y, Fan J, Wang T, Yin T (2020) A novel network architecture of decision-making for self-driving vehicles based on long short-term memory and grasshopper optimization algorithm. IEEE Access 8:155429–155440. https://doi.org/10.1109/ACCESS.2020.3019048
Shi N, Chen Z, Chen L, Lee RST (2022) CNO-LSTM: A chaotic neural oscillatory long short-term memory model for text classification. IEEE Access 10:129564–129579. https://doi.org/10.1109/ACCESS.2022.3228600
Shrestha A, Mahmood A (2019) Review of deep learning algorithms and architectures. IEEE Access 7:53040–53065. https://doi.org/10.1109/ACCESS.2019.2912200
Simonyan K, Zisserman A (2015) Very deep convolutional networks for large-scale image recognition Very deep convolutional networks for large-scale image recognition. International Conference on Learning Representations
Singh SK, Yang R, Behjat A, Rai R, Chowdhury S, Matei I (2019) PI-LSTM: Physics-infused long short-term memory network. 18th IEEE International Conference On Machine Learning And Applications (ICMLA). 34–41. https://doi.org/10.1109/ICMLA.2019.00015
Singh R, Mary AB, Athisayamani S (2020) Banana leaf diseased image classification using novel HEAP Autoencoder (HAE) deep learning. Multimed Tools Appl 79:30601–30613. https://doi.org/10.1007/s11042-020-09521-1
Song J et al (2018) Multi-agent generative adversarial imitation learning. Advances in neural information processing systems 31. https://doi.org/10.5555/3305381.3305404
Song J et al (2018) Multi-agent generative adversarial imitation learning. Advances in neural information processing systems 31 (2018)
Song C, He Z, Yu Y, Zhang Z (2021) Low Resolution Face Recognition System Based on ESRGAN. 3rd International Conference on Applied Machine Learning (ICAML). 76–79. https://doi.org/10.1109/ICAML54311.2021.00024
Szegedy C, Liu W, Yangqing J et al (2015) Going deeper with convolutions. IEEE Conference on Computer Vision and Pattern Recognition (CVPR). 1–9. https://doi.org/10.1109/CVPR.2015.7298594
Tang X (2019) Large-scale computing systems workload prediction using parallel improved LSTM neural network. IEEE Access 7:40525–40533. https://doi.org/10.1109/ACCESS.2019.2905634
Tay Y, Bahri D, Yang L, Metzler D, Juan DC (2020). Sparse sinkhorn attention. International Conference on Machine Learning. 9438–9447. https://doi.org/10.5555/3524938.3525813
Tian C, Ma J, Zhang C, Zhan C (2018) A deep neural network model for short-term load forecast based on long short-term memory network and convolutional neural network. Energies 11:12. https://doi.org/10.3390/en11123493
Tripathi BK (2017) On the complex domain deep machine learning for face recognition. Appl Intell 47:382–396. https://doi.org/10.1007/s10489-017-0902-7
Uyar K, Taşdemir S, Ülker E, Ünlükal N, Solmaz M (2022) Improving efficiency in convolutional neural networks with 3D image filters. Biomed Signal Process Control 74(03563):1746–8094. https://doi.org/10.1016/j.bspc.2022.103563
Valliani AAA, Ranti D, Oermann EK (2019) Deep Learning and Neurology: A Systematic Review. Neurol Ther 8:351–365. https://doi.org/10.1007/s40120-019-00153-8
Venkatachalam K, Trojovský P, Pamucar D, Bacanin N, Simic V (2022) DWFH: An improved data-driven deep weather forecasting hybrid model using Transductive Long Short Term Memory (T-LSTM). Expert Syst Appl 213(119270):0957–4174. https://doi.org/10.1016/j.eswa.2022.119270
Vyas A, Katharopoulos A, Fleuret F (2020) Fast transformers with clustered attention. Adv Neural Inf Process Syst 33:21665–21674
Wang Z, Pan S (2021) An improved convolutional neural network based on noise layer. Knowledge Science, Engineering and Management . KSEM 2021. Lecture Notes in Computer Science. 12816. Springer, Cham. https://doi.org/10.1007/978-3-030-82147-0_6
Wang R, Zhang Y, Zhang J (2022) An efficient swin transformer-based method for underwater image enhancement. Multimed Tools Appl. https://doi.org/10.1007/s11042-022-14228-6
Wang H, Wu W, Su Y, Duan Y, Wang P (2019) Image super-resolution using a improved generative adversarial network. IEEE 9th International Conference on Electronics Information and Emergency Communication (ICEIEC). 312–315. https://doi.org/10.1109/ICEIEC.2019.8784610
Wang Z, Lin J, Wang Z (2017) Accelerating recurrent neural networks: A memory-efficient approach. IEEE Trans Very Large Scale Integr VLSI Syst 25(10):2763–2775. https://doi.org/10.1109/TVLSI.2017.2717950
Wang X, Yu K, Gu J et al (2018) Esrgan: Enhanced super-resolution generative adversarial networks. Proc European Conf Comput Vision (ECCV) 11133:63–79
Wang J, Zhang J, Wang X (2018) Bilateral LSTM: A two-dimensional long short-term memory model with multiply memory units for short-term cycle time forecasting in re-entrant manufacturing systems. IEEE Trans Ind Inf 14(2):748–758. https://doi.org/10.1109/TII.2017.2754641
Wang Y, Shen Y, Mao S, Chen X, Zou H (2019) LASSO and LSTM integrated temporal model for short-term solar intensity forecasting. IEEE Internet Things J 6(2):2933–2944. https://doi.org/10.1109/JIOT.2018.2877510
Wang Q, Peng RQ, Wang JQ, Li Z, Qu HB (2020) NEWLSTM: An optimized long short-term memory language model for sequence prediction. IEEE Access 8:65395–65401. https://doi.org/10.1109/ACCESS.2020.2985418
Wang J, Hou B, Ren B, Zhang Y, Yang M, Wang S, Jiao L (2022) Parameter selection of Touzi decomposition and a distribution improved autoencoder for PolSAR image classification. ISPRS J Photogramm Remote Sens 186:246–266. https://doi.org/10.1016/j.isprsjprs.2022.02.003
Wei L, Li J (2022) Short-term power load forecasting based on MA-LSTM. IEEE International Conference on Advances in Electrical Engineering and Computer Applications (AEECA). 1370–1374. https://doi.org/10.1109/AEECA55500.2022.9918996
Wei S, Zhang Y, Park SC (2021) A novel deep Autoencoder considering energy and label constraints for categorization. Expert Systems with Applications. 176:114936. https://doi.org/10.1016/j.eswa.2021.114936
Wu J, Liu J, Ma J et al (2020) Classification of power loads based on an improved denoising deconvolutional Autoencoder. Applied Soft Computing 87:105959. https://doi.org/10.1016/j.asoc.2019.105959
Chen Xi, Duan Y, Houthooft R et al (2016) Infogan: Interpretable representation learning by information maximizing generative adversarial nets. In: Proceedings of the 30th International Conference on Neural Information Processing Systems. 2180–2188
Xia L, Diao L, Jiang Z et al (2019) PAI-FCNN: FPGA based inference system for complex CNN models. 2019 IEEE 30th International Conference on Application-specific Systems, Architectures and Processors (ASAP). https://doi.org/10.1109/ASAP.2019.00-21
Xiong Y, Zeng Z, Chakraborty R, Tan M, Fung G, Li Y, Singh V (2021) Nyströmformer: A nyström-based algorithm for approximating self-attention. Proc AAAI Conf Artif Intell 35(16):14138–14148
Xu K, Shen X, Yao T, Tian X, Mei T (2018) Greedy layer-wise training of long short term memory networks. 2018 IEEE International Conference on Multimedia & Expo Workshops (ICMEW), 1–6. https://doi.org/10.1109/ICMEW.2018.8551584
Xu T, Zhang H, Li H et al (2017) Unpaired image-to-image translation using cycle-consistent adversarial networks. Proceedings of the IEEE international conference on computer vision. https://doi.org/10.1109/ICCV.2017.629
Xu X, Lu Y, Liu X et al (2020) Intelligent collision avoidance algorithms for USVs via deep reinforcement learning under COLREGs. Ocean Engineering 217:107704. https://doi.org/10.1016/j.oceaneng.2020.107704
Xu X, Xu H, Wang Y et al (2021) AENEA: A novel autoencoder-based network embedding algorithm. Peer-to-Peer Netw Appl 14:1829–1840. https://doi.org/10.1007/s12083-020-01043-9
Yang M, Xu S (2021) Orthogonal nonnegative matrix factorization using a novel deep autoencoder network. Knowledge-Based Systems 227:107236. https://doi.org/10.1016/j.knosys.2021.107236
Yang R, Xu M, Wang Z (2017) Decoder-side HEVC quality enhancement with scalable convolutional neural network. 2017 IEEE International Conference on Multimedia and Expo (ICME). 817-822. https://doi.org/10.1109/ICME.2017.8019299
Yang S, Yang J, Ge X, Wang X (2022) Medium and short-term prediction of power system load based on improved LSTM Algorithm. International Conference on Communications, Computing, Cybersecurity, and Informatics (CCCI). 1–7. https://doi.org/10.1109/CCCI55352.2022.9926584
Yang Y, Zheng K, Wu C, Yang Z (2019) Improving the classification effectiveness of intrusion detection by using improved conditional variational autoencoder and deep neural network. Sensors 19(11):2528. https://doi.org/10.3390/s19112528
Yang M, Nazir S, Xu Q et al (2020) Deep learning algorithms and multicriteria decision-making used in big data: A systematic literature review. Complexity 2020:1076–2787. https://doi.org/10.1155/2020/2836064
Yao L, Yazhuo G (2018) An improved LSTM structure for natural language processing. IEEE International Conference of Safety Produce Informatization (IICSPI). https://doi.org/10.1109/IICSPI.2018.8690387
Yin A, Zheng F, Tan J, Wang Y (2021) An improved variational autoencoder with reverse supervision for the obstacles recognition of UGVs. IEEE Sens J 21(10):11791–11798. https://doi.org/10.1109/JSEN.2020.3013668
Yuan X, He P, Zhu Q, Li X (2019) Adversarial examples: Attacks and defenses for deep learning. IEEE Trans Neural Netw Learn Syst 30(9):2805–2824. https://doi.org/10.1109/TNNLS.2018.2886017
Yuqian C, Song Y, Liu W, Zhang YJ et al (2021) CellTrack R-CNN: A novel end-to-end deep neural network for cell segmentation and tracking in microscopy images. 2021 IEEE 18th International Symposium on Biomedical Imaging. 779–782. https://doi.org/10.1109/ISBI48211.2021.9434057
Yuzhen L, and Salem FM (2017) Simplified gating in long short-term memory (lstm) recurrent neural networks. 2017 IEEE 60th International Midwest Symposium on Circuits and Systems (MWSCAS). 1601–1604. https://doi.org/10.1109/MWSCAS.2017.8053244
Zabalza J, Ren J, Zheng J et al (2016) Novel segmented stacked autoencoder for effective dimensionality reduction and feature extraction in hyperspectral imaging. Neurocomputing 185:1–10. https://doi.org/10.1016/j.neucom.2015.11.044
Zhang L, Suganthan PN (2017) Visual tracking with convolutional random vector functional link network. IEEE Trans Cybern 47:3243–3253. https://doi.org/10.1109/TCYB.2016.2588526
Zhang Z, Luo J, Liu J, Chen M, Zhang S, Zhu L (2022) DGGCNN: An improved generative grasping convolutional neural networks. 2022 Asia Conference on Advanced Robotics, Automation, and Control Engineering. 57–61. https://doi.org/10.1109/ARACE56528.2022.00019
Zhang Z, Luo J, Liu J et al (2022) DGGCNN: An improved generative grasping convolutional neural networks. 2022 Asia Conference on Advanced Robotics, Automation, and Control Engineering. 57–61. https://doi.org/10.1109/ARACE56528.2022.00019
Zhang H et al (2017) StackGAN: Text to photo-realistic image synthesis with stacked generative adversarial networks. IEEE Int Conf Comput Vision (ICCV) 2017:5908–5916. https://doi.org/10.1109/ICCV.2017.629
Zhang H, Xu T, Li H, Zhang S, Wang X, Huang X, Metaxas DN (2018) StackGAN++: Realistic image synthesis with stacked generative adversarial networks. IEEE Trans Pattern Anal Mach Intell 41(8):1947–1962. https://doi.org/10.1109/TPAMI.2018.2856256
Zhang Y, Cheng Y, Xu T, Wang G, Chen C, Yang T (2022) Fault prediction of railway turnout systems based on improved sparse autoencoder and gated recurrent unit network. IEEE Trans Intell Transp Syst 23(8):12711–12723. https://doi.org/10.1109/TITS.2021.3116966
Zhao J, Michael M, LeCun Y (2017) Energy-based generative adversarial network. 5th International Conference on Learning Representations
Zhao H, Zenget X, Zhang J et al (2011) Pipelined functional link artificial recurrent neural network with the decision feedback structure for nonlinear channel equalization. Inf Sci 181(17):3677–3692. https://doi.org/10.1016/j.ins.2011.04.033
Zhao R, Dong D, Wang Y et al (2022) Image-based crowd stability analysis using improved multi-column convolutional neural network. IEEE Trans Intell Transp Syst 23(6):5480–5489. https://doi.org/10.1109/TITS.2021.3054376
Zheng C et al (2019) A novel equivalent model of active distribution networks based on LSTM. IEEE Trans Neural Netw Learn Syst 30(9):2611–2624. https://doi.org/10.1109/TNNLS.2018.2885219
Zhong H, Wu J (2022) Image dehazing algorithm based on improved generative adversarial network. In: Proceedings of the 7th International Conference on Cyber Security and Information Engineering. 429–434. https://doi.org/10.1145/3558819.3565120
Zhou H, Zhang S, Peng J, Zhang S, Li J, Xiong H, Zhang W (2021) Informer: Beyond efficient transformer for long sequence time-series forecasting. Proc AAAI Conf Artif Intell 35(12):11106–11115
Download references
Author information
Authors and affiliations.
Department of Software Engineering, Delhi Technological University, Delhi, India
Ruchika Malhotra & Priya Singh
You can also search for this author in PubMed Google Scholar
Contributions
For the Systematic Literature Review-
1. Ruchika Malhotra proposed the idea for the article.
2. Primary Study selection was done by Priya Singh followed by a review by Dr. Ruchika Malhotra.
3. Data Extraction was done by Ruchika Malhotra and Priya Singh both separately, resolving differences where applicable at the time of merging.
4. Result Reporting was done by Priya Singh and reviewed by Ruchika Malhotra.
5. Proofreading and final review were done by Ruchika Malhotra and Priya Singh.
Corresponding author
Correspondence to Priya Singh .
Ethics declarations
Ethics approval and consent to participate.
Not applicable.
Consent for publication
The authors provide consent for publication.
Competing interests
The authors declare that they have no known competing financial interests or personal relationships that could have appeared to influence the work reported in this paper.
Conflict of interest
The authors certify that this manuscript has not been submitted to more than one journal for simultaneous consideration and it has not been published previously (partly or in full). The authors also certify that no funding has been received for the conduct of this study and the preparation of this manuscript. This article does not contain any studies with human participants or animals performed by any of the authors.
Additional information
Publisher's note.
Springer Nature remains neutral with regard to jurisdictional claims in published maps and institutional affiliations.
1.1 Quality assessment results
We provide the quality scores to 166 studies selected after Inclusion–Exclusion criteria according to 16 quality assessment questions stated in Table 2 . Table 10 reports the percentage of candidate studies that answered a given quality question as “Yes”, “Partly” or “No”.
Rights and permissions
Springer Nature or its licensor (e.g. a society or other partner) holds exclusive rights to this article under a publishing agreement with the author(s) or other rightsholder(s); author self-archiving of the accepted manuscript version of this article is solely governed by the terms of such publishing agreement and applicable law.
Reprints and permissions
About this article
Malhotra, R., Singh, P. Recent advances in deep learning models: a systematic literature review. Multimed Tools Appl 82 , 44977–45060 (2023). https://doi.org/10.1007/s11042-023-15295-z
Download citation
Received : 08 September 2022
Revised : 10 January 2023
Accepted : 06 April 2023
Published : 25 April 2023
Issue Date : December 2023
DOI : https://doi.org/10.1007/s11042-023-15295-z
Share this article
Anyone you share the following link with will be able to read this content:
Sorry, a shareable link is not currently available for this article.
Provided by the Springer Nature SharedIt content-sharing initiative
- Deep Learning
- Find a journal
- Publish with us
- Track your research
Help | Advanced Search
Computer Science > Information Retrieval
Important: e-prints posted on arXiv are not peer-reviewed by arXiv; they should not be relied upon without context to guide clinical practice or health-related behavior and should not be reported in news media as established information without consulting multiple experts in the field.
Title: Access to Library Information Resources by University Students during COVID-19 Pandemic in Africa: A Systematic Literature Review
Abstract: The study examined access to library information resources by university students during the outbreak of the COVID-19 pandemic. The study investigated measures that were adopted by academic libraries for smooth delivery of library information resources to their patrons. It also identified technological tools that were employed by libraries to facilitate access to library information resources. We also investigated the challenges faced by students in accessing library information resources. A systematic literature review approach using PRISMA guidelines was employed to investigate the relevant literature on the subject. The keyword search strategy was employed to search for relevant literature from four scholarly databases Scopus, emerald, Research4life, and Google Scholar. In this study, 23 studies that fulfilled the criteria were included. The findings revealed that the majority of the reviewed studies indicate that, during the COVID-19 pandemic many academic libraries in Africa adopted different approaches to facilitate access to library information resources by university students including expanding access to electronic resources off-campus, virtual reference services, circulation and lending services. To support access to different library services and information resources academic libraries in Africa used various digital technological tools like social media, library websites, email and video conferencing. Moreover, the study revealed that limited access to internet services and ICT devices, inadequate electronic library collection and inadequate digital and information literacy were the major challenges faced by patrons during the pandemic. This study recommends investment in ICT infrastructures and expanding electronic resource collections which are vital resources in the digital era.
Submission history
Access paper:.
- Other Formats

References & Citations
- Google Scholar
- Semantic Scholar
BibTeX formatted citation

Bibliographic and Citation Tools
Code, data and media associated with this article, recommenders and search tools.
- Institution
arXivLabs: experimental projects with community collaborators
arXivLabs is a framework that allows collaborators to develop and share new arXiv features directly on our website.
Both individuals and organizations that work with arXivLabs have embraced and accepted our values of openness, community, excellence, and user data privacy. arXiv is committed to these values and only works with partners that adhere to them.
Have an idea for a project that will add value for arXiv's community? Learn more about arXivLabs .
Log in using your username and password
- Search More Search for this keyword Advanced search
- Latest content
- For authors
- Browse by collection
- BMJ Journals More You are viewing from: Google Indexer
You are here
- Volume 14, Issue 4
- Effect of metacognitive therapy on depression in patients with chronic disease: a protocol for a systematic review and meta-analysis
- Article Text
- Article info
- Citation Tools
- Rapid Responses
- Article metrics

- http://orcid.org/0009-0001-2964-8906 Zirui Zhang ,
- Peng Wang ,
- Jinjin Gu ,
- Qiang Zhang ,
- Changqing Sun ,
- Panpan Wang
- School of Nursing and Health , Zhengzhou University , Zhengzhou , Henan , China
- Correspondence to Dr Panpan Wang; wangpanpan{at}zzu.edu.cn
Background Chronic diseases have a high prevalence worldwide, and patients with chronic diseases often suffer from depression, leading to a poor prognosis and a low quality of life. Metacognitive therapy is a transdiagnostic psychotherapy intervention focused on thinking patterns, with the advantages of reliable implementation effect, short intervention period and low cost. It can help patients change negative metacognition, alleviate depression symptoms, and has a higher implementation value compared with other cognitive interventions. Therefore, metacognitive therapy may be an effective way to improve the mental health of patients with chronic diseases.
Methods and analysis CNKI, Wanfang Database, VIP Database for Chinese Technical Periodicals, Sinomed, PubMed, SCOPUS, Embase, The Cochrane Library, Web of Science and PsycINFO will be used to select the eligible studies. As a supplement, websites (eg, the Chinese Clinical Registry, ClinicalTrials.gov) will be searched and grey literature will be included. The heterogeneity and methodological quality of the eligible studies will be independently screened and extracted by two experienced reviewers. All the data synthesis and analysis (drawing forest plots, subgroup analysis and sensitive analysis) will be conducted using RevMan 5.4.1.
Ethics and dissemination This article is a literature review that does not include patients’ identifiable information. Therefore, ethical approval is not required in this protocol. The findings of this systematic review and meta-analysis will be published in a peer-reviewed journal as well as presentations at relevant conferences.
PROSPERO registration number CRD42023411105.
- Chronic Disease
- Meta-Analysis
- Protocols & guidelines
- Depression & mood disorders
This is an open access article distributed in accordance with the Creative Commons Attribution Non Commercial (CC BY-NC 4.0) license, which permits others to distribute, remix, adapt, build upon this work non-commercially, and license their derivative works on different terms, provided the original work is properly cited, appropriate credit is given, any changes made indicated, and the use is non-commercial. See: http://creativecommons.org/licenses/by-nc/4.0/ .
https://doi.org/10.1136/bmjopen-2023-075959
Statistics from Altmetric.com
Request permissions.
If you wish to reuse any or all of this article please use the link below which will take you to the Copyright Clearance Center’s RightsLink service. You will be able to get a quick price and instant permission to reuse the content in many different ways.
STRENGTHS AND LIMITATIONS OF THIS STUDY
This systematic review and meta-analysis will evaluate the feasibility and effectiveness of metacognitive therapy (MCT) in reducing depression in patients with chronic diseases by collecting comprehensive evidence.
If heterogeneity is detected, differences in the effectiveness and durability of different types of MCTs will be determined in subgroup analysis.
Grey literature and clinical registration information will be searched manually to enrich evidence sources.
There may be a limited number of studies on the application of MCT to patients with chronic diseases, so the number of included studies may be small.
Only English and Chinese literature will be included.
Introduction
Chronic diseases, also called non-communicable diseases (NCDs), refer to diseases that do not result from infection but rather from damage by long-term accumulation. 1 Data show that billions of people suffer from chronic diseases, which has become an important public health issue. 2 3 Also, the United Nations prioritised the management of chronic diseases in the sustainable development goals. 4
Depression is one of the most common comorbidities among many chronic diseases. People with one chronic disease may have an increased risk of developing depressive symptoms by at least 20%. 5 6 Meanwhile, depression is associated with poor prognosis and increased medical costs in individuals with chronic diseases. 7 8 A literature review focused on patients with heart failure (HF) found that depression doubled the all-cause mortality in patients with HF, suggesting a possible association between depression and all-cause mortality. 9 The Lancet reported that data from 1990 to 2019 showed a high disability rate due to depression, and depression is considered one of the leading cause of burden worldwide. 10 Therefore, alleviating depression is crucial for maintaining the mental health of patients with chronic diseases. However, a systematic review comparing psychological and pharmacological interventions in patients with coronary artery disease found that neither strategy achieved the effect of alleviating depressive symptoms at the end of treatment. 11
Metacognitive therapy (MCT) was initially developed by Wells 12 and is an emerging theoretical based transdiagnostic psychotherapy that has been proven effective in alleviating depression. 13 According to Self-Regulation Executive Function model (S-REF), 14 depression will occur, persist and reoccur due to the development of unmanageable, repeated negative thinking pattern. 15 This thinking strategy called cognitive attentional syndrome (CAS), which focuses on the inner (attention, thinking and physical sensations), reflects on the past and worries about the future, accompanied by avoidance and maladaptation behaviours. The S-REF model assumes that CAS is influenced by positive or negative metacognitive beliefs. Negative metacognitive beliefs manifest as uncontrollable beliefs about contemplation and worry. Patients may express ‘My contemplation is uncontrollable’. While positive metacognitive beliefs manifest as useful beliefs about contemplation and worry, such as ‘My contemplation will help me find a solution’. 16 To alleviate depression symptoms, MCT helps patients in reducing CAS and developing healthy metacognitive beliefs. This enables patients to understand the negative effects and adverse consequences of CAS without denying the negative thinking content. 17 It includes several specific skills such as attention training technique (ATT), spatial attention control exercise, situational attention refocusing, detached mindfulness, etc. In the treatment of MCT, the ruminative thinking mode is blocked in the early stage. Patients are required to pay attention to external sounds or recognise different sound sources, helping them realise the independence of attention control from any internal and external events. 18 MCT establishes adaptive conditioned emotional response by mobilising the positive mental state of the patients, blocking the connection between conditional stimuli and negative emotions. 19
A systematic review and meta-analysis have indicated that MCT is an effective method for treating a range of psychological complaints. 13 Several clinical trials have examined the efficacy of MCT in patients with depression and chronic diseases. However, there are currently no meta-analysis related to MCT. Therefore, this protocol aims to conduct a systematic review and meta-analysis to assess the effectiveness of MCT in treating depression in patients with chronic disease.
Methods and analysis
Study registration.
This protocol was registered in the International Prospective Register of Systematic Reviews (PROSPERO) database on 4 April 2023. This protocol was reported in accordance with the Preferred Reporting Items for Systematic Reviews and Meta-analyses Statement. 20
Inclusion criteria
Types of studies.
Randomised controlled trials (RCTs) using MCT for treatment of patients with chronic disease will be included in the study. However, if <3 RCT studies will be included, we will also consider including quasi-experimental studies.
Types of participants
This study will include patients aged ≥18 years with any type of chronic disease. The NCDs considered in this study include, but are not limited to, cancer, stroke, coronary heart disease, HF, hypertension, diabetes and chronic obstructive pulmonary disease. The NCDs were diagnosed by 11th edition of the International Classification of Diseases (ICD-11). 21 There are no restrictions on gender, economic background, nationality, educational status or disease period.
Experimental and control interventions
Studies will be included if they assessed the effect of MCT or specific MCT techniques (eg, ATT) with appropriate qualified professionals are responsible for intervention delivery. According to Wells’ educational programme, 14 interventions can be lectures, self-help manuals, telephone support calls, group discussions, role plays and homework. Sessions should focus on deriving a case formulation and socialisation, practicing techniques to regulate worry and rumination, challenging metacognitive beliefs that maintain maladaptive patterns of thinking and developing a ‘helpful behaviours’ plan. The form of intervention can be adjusted according to the research objective. There is no limitation on the intervention period or intervention time. Interventions will be excluded if studies combine MCT with other psychotherapies (eg, mindfulness therapy).
In the included studies, the control group was defined as other interventions without MCT such as cognitive behavioural therapy, pharmacological treatment, wait-list control, usual care, clinical management and no interventions.
Outcome measures
The primary outcome was symptom of depression, which was evaluated using standardised and validated depressive symptom scale scores, such as The Hospital Anxiety and Depression Scale (HADS), the Beck Depression Inventory I or II, the Hamilton Depression Rating Scale and so on. We will include studies where depression is assessed as a primary or secondary outcome. If the reliability and validity of the scale used in the study are relatively low, the decision on include this study will be made through group discussion.
To comprehensively assess the effect of MCT on patients with chronic disease, our study also included anxiety, metacognitive beliefs, adverse events and traumatic stress symptoms as the secondary outcomes.
Exclusion criteria
RCTs with <10 participants.
Studies published in non-English and non-Chinese languages.
Studies that recruited ≥50% of patients with dementia or schizophrenia and it was not possible to distinguish between two groups of patients.
Studies that combine MCT techniques with other types of treatment (eg, cognitive behavioural therapy).
Studies that report similar results without further analysis or discussion.
Search methods
We will select literatures from the following four Chinese databases (CNKI, Wanfang Database, VIP Database for Chinese Technical Periodicals and Sinomed) and six English databases (PubMed, SCOPUS, Embase, The Cochrane Library, Web of Science and PsycINFO). The search time will be set from the beginning to January 2024, and the languages are limited to both Chinese and English. The Clinicaltrials.gov and Chinese Clinical Trial Registry will also be searched to obtain unpublished or ongoing trial data. The keywords of our study will be medical subject headings (MESH) terms and free-text terms corresponding to the subject heading for (1) MCT (eg, metacognitive therapy, metacognitive intervention); (2) depression (eg, depressive disorder, emotional distress, mood disorder) and (3) clinical trial. Specific searching strategy in PubMed is shown in table 1 . Appropriate modifications will be made in actual searching according to the searching methods of those databases. In addition, reference lists of the included studies will be examined to identify potentially eligible studies.
- View inline
Search strategies in PubMed
Searching other sources
Other websites will be searched as a supplement including: the Chinese Clinical Registry, the WHO International Clinical Trials Registry Platform and ClinicalTrials.gov.
Data collection and analysis
Selection of studies.
All search results will be imported into EndNote V.20 to select eligible studies, and duplicate studies will be deleted. The initial selection will be based on titles and abstracts, with two reviewers (ZZ and JG) working on separately. Those unrelated literature will be excluded. Next, full text of the remaining studies will be screened for further assessment according to the inclusion criteria. Any disagreements will be resolved through reviewers’ discussion. If an agreement cannot be reached, a third reviewer (PPW) will be consulted. The study selection flow chart is shown in figure 1 .
- Download figure
- Open in new tab
- Download powerpoint
Flow chart of the literature screening process and results.
Data extraction and management
The data extraction will be completed by two researchers (ZZ and JG) independently with a predesigned Microsoft Excel. Discrepancies in the data extraction will be resolved by consensus. If consensus cannot be reached, a third reviewer (PPW) will be consulted. The predefined items for extraction are the following: publication details (title, the first author’s name, publication year), characteristics of the research participants (sample size, gender, age, nationality, types of chronic disease, baseline data, diagnostic criteria for depression), interventions (type of MCT, number of sessions, duration of each lesson, intervention frequency), control condition (details of the treatment, including the name, dosage, frequency and course) and outcomes (outcome at each time point, adverse events in each group and numbers of dropouts). If the data are unclear or missing in our included studies, the corresponding author will be contacted through email to obtain complete data. If the data are still unattainable, only current data will be analysed, and the potential influence will be discussed.
Assessment of risk of bias
Cochrane Collaboration’s tool will be used to assess the risk of bias of included RCT studies by two authors (ZZ and JG) independently. This tool identifies bias in the following domains: random sequence generation, allocation concealment, blinding of participants and personnel, blinding of outcome assessment, incomplete outcome data, selective reporting and other bias. 22 According to the criteria, the included RCTs will be classified as low, high or unclear risk of bias. Disagreement will be resolved by discussion and the third reviewer (PW) will be consulted when necessary. The quasi-experimental studies were evaluated by the JBI Critical Appraisal Checklist for Quasi-Experimental Studies. 23 If more than 10 trials are included, a funnel plot 24 will be used to detect publication bias, and the Egger test 25 will be carried out to analyse the asymmetry in the funnel plot.
Data synthesis
Quantitative data synthesis.
RevMan V.5.4.1 will be used to conduct statistical analysis. For categorical variables, we will use risk ratio and 95% CIs as analysis indicators. For continuous variables, mean difference (MD) will be calculated. Data of same indicators measured by different scales will be converted to standardised MD and calculated as Hedges’ g with 95% CI. When heterogeneity is not obvious (p>0.1 or I 2 <50%), the fixed effect model will be used for analysis, or we will choose the random effect model (when p≤0.1 or I 2 ≥50%). If quantitative synthesis is not appropriate, we will explain the reasons and make a qualitative analysis of the research results in the Discussion section.
Assessment of heterogeneity
Following the guideline in the Cochrane Handbook, χ 2 and I 2 statistics will be chosen to evaluate the heterogeneity. High, moderate and low heterogeneity correspond to I 2 of 25%, 50% and 75%, respectively. 26 If I 2 >50%, subgroup analysis will be performed to detect the reasons of heterogeneity. If there is no reason be found, we will provide a narrative summary without conducting data synthesis.
Subgroup analysis
If significant heterogeneity is detected, we will conduct subgroup analysis according to the characteristics of researches or participants including types of MCT, intervention time, frequency of intervention, age of participants, type of chronic diseases, nationality, etc.
Sensitivity analysis
Sensitive analysis will be performed to test the stability of the results. We will remove one study at a time to identify its effect on heterogeneity and effect size. Small change of heterogeneity and effect size after each removal shows reliable stability.
Strength of recommendations and the quality of evidence
To identify the quality of included studies, two researchers (ZZ and JG) will use the Grading of Recommendations Assessment, Development and Evaluation to evaluate the strength of evidence. 27 Disagreements will be solved by discussion or consultation with a third reviewer (PPW). Confidence in the results will be graded into high, moderate, low and very low. All eligible studies will be included in the final analysis irrespective of their quality score. Correspondingly, we will analyse the impact of different quality scores in our discussion and recommendations will be drawn cautiously.
Patient and public involvement
No patients or public will be involved in the design, conduct, reporting or dissemination of this research.
Study period
Our review had started in April 2023 and will be conducted until the end of April 2024.
Due to the high prevalence rates and the impact of depression on both physical and psychosocial outcomes, there is a need for effective depression interventions in chronic disease. However, the current depression treatment takes a long time and high costs, which brings a huge burden to patients with chronic diseases. Recent studies present that MCT is a theory-based, structured treatment and is suited to addressing the psychological needs of patients with chronic disease. Therefore, we intend to perform this systematic review and meta-analysis.
In our systematic review, we hold a keen interest in delving into the efficacy of MCT for patients with chronic diseases for several reasons: (1) specific intervention strategies of MCT will regulate repetitive negative thinking cycles and other unproductive behaviours that maintain depression, helping patients realise that worry and contemplation have no advantages and can be alleviated; (2) in MCT, patients will practice new reaction methods to enhance their attention control ability to get rid of worries and contemplation 28 ; (3) contrast to other therapies, MCT does not require in-depth analysis and challenging the patients’ concerns and (4) MCT has the advantages of short intervention period, convenient implementation methods, reliable implementation effects and low cost. 16
Some studies have been published related to the application of MCT on patients with chronic diseases. Wells et al recruited 799 eligible cardiac rehabilitation patients for MCT treatment, but approximately 58% patients refused to participate. 29 Fisher et al conducted a short-term MCT treatment on cancer survivors and depression that was evaluated by the HADS. 30 The study showed an excellent effect of MCT intervention, but only 75% of patients completed the entire treatment process. 30 Zahedian et al conducted MCT intervention on 24 patients and found MCT can significantly improve depression, but the sample size was limited. 31 In summary, inconsistent measurement tools, intervention types and participant characteristics make it difficult to obtain effective clinical evidence.
To the best of our knowledge, this study is the first comprehensive evidence for the application of MCT in chronic disease patients, and plays a crucial role in clinical intervention of depression. The anticipated benefits of this research encompass: (1) exploring the clinical efficacy of MCT intervention on depression and anxiety; (2) fostering a deeper comprehension of the psychological mechanism of MCT by using metacognitive beliefs as a secondary outcome and (3) discerning if the efficacy varies in specific MCT and the reasons for the differences.
There were several limitations in this study. First, due to differences in outcome measurements, intervention intensities and types of scales, there may exist a hi g h degree of clinical and statistical heterogeneity. If so, we will conduct subgroup analysis to identify heterogeneity sources. Second, although many clinical trials of MCT have been conducted, as an emerging psychological therapy, there may be many unpublished studies, and the lack of these data may have an impact on the research results. Therefore, we will also include clinical trial data to reduce this impact. Third, only English and Chinese literature was included in the analysis, which may have an impact on the results. We will discuss the impact in the discussion section.
Ethics and dissemination
Ethical approval is not required in this study because the patients’ personal information is not involved. The findings of the systematic review and meta-analysis will be published in a peer-reviewed journal and presented at conferences. Any changes in this protocol will be updated in PROSPERO and explanations of these modifications will be stated in the paper of this review.
Ethics statements
Patient consent for publication.
Not applicable.
Ethics approval
Acknowledgments.
The authors would like to thank all participants who contributed to this study.
- Alvarez P ,
- Brown J , et al
- Jiang C-H ,
- Bussotti M ,
- Sommaruga M
- Wierenga KL ,
- Cani D , et al
- ↵ Global, regional, and national burden of 12 mental disorders in 204 countries and territories, 1990-2019: a systematic analysis for the global burden of disease study 2019 . The Lancet Psychiatry 2022 ; 9 : 137 – 50 . doi:10.1016/S2215-0366(21)00395-3 OpenUrl
- Lee EJ , et al
- Wells A. M G
- Normann N ,
- Heal C , et al
- Kaloiya G , et al
- Fergus TA ,
- Shamseer L ,
- Clarke M , et al
- Harrison JE ,
- Jakob R , et al
- Higgins JPT ,
- Altman DG ,
- Gotzsche PC , et al
- Aromataris E ,
- Sterne JAC ,
- Davey Smith G ,
- Schneider M , et al
- Thompson SG
- Cumpston M ,
- Page MJ , et al
- Capobianco L , et al
- Fisher PL ,
- Fairburn L , et al
- Zahedian E ,
- Bahreini M ,
- Ghasemi N , et al
ZZ and PW are joint first authors.
Contributors PPW, CS and QZ were responsible for the formulation of the article framework. ZZ, JG and PPW were responsible for the data collection and meta-analysis process. QZ was responsible for the content supplement. PW, PPW and CS were responsible for the feasibility analysis and improvement of the article. All authors read and approved the final manuscript.
Funding This study was supported by the funding from Science and Technology Department of Henan Province in China (232102311023), Health Commission of Henan Province (LHGJ20210496) and Zhengzhou University (XKLMJX202212).
Competing interests None declared.
Patient and public involvement Patients and/or the public were not involved in the design, or conduct, or reporting, or dissemination plans of this research.
Provenance and peer review Not commissioned; externally peer reviewed.
Read the full text or download the PDF:
- Open access
- Published: 11 May 2023
Comparative efficacy and safety of PD-1/PD-L1 inhibitors in triple negative breast cancer: a systematic review and network meta-analysis of randomized controlled trials
- Ibrahim Elmakaty 1 ,
- Ruba Abdo 1 ,
- Ahmed Elsabagh 1 ,
- Abdelrahman Elsayed 1 &
- Mohammed Imad Malki ORCID: orcid.org/0000-0002-6801-2126 2
Cancer Cell International volume 23 , Article number: 90 ( 2023 ) Cite this article
2680 Accesses
4 Citations
8 Altmetric
Metrics details
Triple-Negative Breast Cancer (TNBC) is a lethal subtype of breast cancer with limited treatment options. The purpose of this Network Meta-Analysis (NMA) is to compare the efficacy and safety of inhibitors of programmed cell death 1 (PD-1) and programmed cell death ligand 1 (PD-L1) in treating TNBC.
Our search strategy was used in six databases: PubMed, Cochrane Library, Cumulative Index to Nursing and Allied Health Literature database, Embase, Scopus, and Web of Science up to November 2nd, 2022, as well as a thorough search in the most used trial registries. We included phase II and III randomized controlled trials that looked at the efficacy of PD-1/PD-L1 inhibitors in the treatment of TNBC and reported either Overall Survival (OS), Progression-Free Survival (PFS), or pathological Complete Response (pCR). The risk of bias was assessed utilizing Cochrane's risk of bias 2 tool, and the statistical analysis was performed using a frequentist contrast-based method for NMA by employing standard pairwise meta-analysis applying random effects model.
12 trials (5324 patients) were included in our NMA including seven phase III trials. Pembrolizumab in a neoadjuvant setting achieved a pooled OS of 0.82 (95% Confidence Interval (CI) 0.65 to 1.03), a PFS of 0.82 (95% CI 0.71 to 0.94) and a pCR 2.79 (95% CI 1.07 to 7.24) compared to Atezolizumab’s OS of 0.92 (95% CI 0.74 to 1.15), PFS of 0.82 (95% CI 0.69 to 0.97), and pCR of 1.94 (95% CI 0.86 to 4.37). Atezolizumab had less grade ≥ 3 adverse events (OR 1.48, 95% CI 0.90 to 2.42) than Pembrolizumab (OR 1.90, 95% CI 1.08 to 3.33) in the neoadjuvant setting.
Conclusions
PD-1/PD-L1 inhibitors exhibited varying efficacy in terms of OS, PFS, and pCR. They were associated with an increase in immune-related adverse effects. When used early in the course of TNBC, PD-1/PD-L1 inhibitors exert their maximum benefit. Durvalumab as a maintenance treatment instead of chemotherapy has shown promising outcomes. Future studies should focus on PD-L1 expression status and TNBC subtypes, since these factors may contribute to the design of individualized TNBC therapy regimens.
Systematic review registration PROSPERO Identifier: CRD42022380712.
Breast cancer remains a major health burden, causing considerable morbidity and mortality worldwide [ 1 ]. It has surpassed lung cancer as the most frequently diagnosed malignancy overall and ranks the fifth leading cause of cancer-related mortality, with an estimated 2.3 million new cases (11.7% of all cancers), and 685,000 deaths in 2020 [ 2 ]. The incidence rate has been increasing at an alarming rate over the past years, especially in transitioning countries, and it is predicted that by 2040, this burden will grow further by over 40% to about 3 million new cases and 1 million deaths every year [ 2 , 3 ]. Triple-Negative Breast Cancer (TNBC) is a particularly aggressive subtype that accounts for approximately 15–20% of all cases and is characterized by a lack of expression of both estrogen and progesterone receptors as well as human epidermal growth factor receptor 2 [ 4 ]. The high molecular heterogeneity, great metastatic potential, and limited therapeutic options have all contributed to TNBC having a relatively poor prognosis with a 5-year overall survival rate of 77% [ 5 , 6 ]. Due to the absence of well-defined molecular targets, TNBC therapy predominantly relies on the administration of Taxane and Anthracycline-based regimens in both the neoadjuvant and the adjuvant settings [ 4 , 6 , 7 ]. More favorable response rates are shown to be achieved when using a combination rather than single-agent chemotherapy [ 8 , 9 ]. Although this can be effective initially, chemotherapy is often accompanied by resistance, relapse, and high toxicity [ 10 , 11 ]. Additionally, survival rates in those who develop metastatic disease have not changed over the past 20 years [ 9 ]. The median Overall Survival (OS) for those patients with the current treatment option is 16 months and the median Progression-Free Survival (PFS) is 5.6 months [ 12 ]. These results underscore the urgent need for more effective and less toxic therapies.
The introduction of immunotherapy has revolutionized the field of oncology over the past decade and has been successfully incorporated into the standard treatment paradigm of many malignancies including non-small cell lung cancer and renal cell cancer [ 13 , 14 ]. Whilst breast cancer has traditionally been considered immunogenically quiescent, several lines of evidence have demonstrated TNBC to be highly immunogenic and feature a microenvironment that is enriched with stromal Tumor Infiltrating Lymphocytes (TILs) with a relatively high tumor mutational burden as opposed to other subtypes [ 15 , 16 ]. The high levels of inhibitory checkpoint molecules expressed on the TILs led to the successful implementation of Immune Checkpoint Inhibitors (ICI) in TNBC treatment, particularly inhibitors of the Programmed Cell Death 1 (PD-1) and the Programmed Cell Death Ligand 1 (PD-L1) which have shown great promise in the field’s clinical trials [ 15 ]. The PD‑L1/PD-1 signaling pathway exerts a critical role in forming an adaptive immune resistance mechanism that mediates tumor invasion and metastasis [ 17 ]. Blocking this pathway would therefore restore the antitumor immune responses by reducing the inhibition of innate immunity and reactivating tumor-specific cytotoxic T cells [ 18 ].
Atezolizumab, an anti-PD-L1 antibody was the first Food and Drug Administration (FDA) approved ICI given along with nab-paclitaxel for patients with unresectable locally advanced or metastatic TNBC whose tumors express PD-L1 [ 19 ]. This accelerated approval was based on the results of the Impassion130 trial. Unfortunately, the designated confirmatory trial, IMpassion131 neither met the primary endpoint of PFS superiority nor achieved statistically significant overall OS leading to the withdrawal of this combination as an indication for treatment [ 12 ]. Alternatively, FDA granted approval to pembrolizumab, a PD-1 inhibitor to be used in combination with chemotherapy for patients with high-risk, early-stage TNBC, as well as those with locally recurrent unresectable or metastatic TNBC whose tumors have a PD-L1 Combined Positive Score (CPS) of ≥ 10 [ 12 ]. Nonetheless, there remain several additional clinical trials that have assessed the role of anti‑PD‑L1/PD‑1 agents in TNBC treatment with inconsistent results. The objective of this Network Meta-Analysis (NMA) is to evaluate the efficacy and safety of these agents, as well as compare them in order to determine the optimal therapeutic regimen for patients with TNBC.
Protocol and registration
This systematic review and meta-analysis is reported following the Preferred Reporting Items for Systematic Reviews and Meta-Analyses (PRISMA) extension for NMA Additional file 1 : (Table S1) [ 20 ]. The NMA protocol was carried in accordance with a protocol that had been registered in the International Prospective Register of Systematic Reviews (PROSPERO) online database (PROSPERO Identifier: CRD42022380712).
Search strategy
We developed our search strategy in the PubMed database using Medical Subject Headings (MeSH) that included the terms (“Immune Checkpoint Inhibitors”[MeSH] OR “programmed cell death 1 receptor/antagonists and inhibitors”[MeSH]) AND “Triple Negative Breast Neoplasms”[MeSH] AND “Randomized Controlled Trial”[Publication Type] with multiple keywords build around them. There was no date or language restriction applied to our strategy. The developed search strategy was then transferred from PubMed to five other databases by the Polyglot translator [ 21 ], namely Cochrane Library, Cumulative Index to Nursing and Allied Health Literature database, Embase, Scopus, and Web of Science. All databases were searched from the inception date until the 2nd of November 2022. The yielded studies were then exported to EndNote X7, where duplicates were identified and excluded. The remaining articles were uploaded to the Rayyan platform for screening [ 22 ]. In addition, we searched popular clinical trial registries such as ClinicalTrials.gov, EU Clinical Trials Register, International Standard Randomised Controlled Trial Number registry, International Clinical Trials Registry Platform, and breastcancertrials.org for Gery literature (unpublished trials) to ensure the comprehensiveness of our search strategy. Additional file 1 contains the complete strategy for each database and trial registries.
Eligibility criteria
We included trials that met the following criteria: (1) usage of FDA-approved PD-1/PD-L1 inhibitors, (2) phase II or III RCTs, (3) for the management of confirmed TNBC, (4) compared against a different Immune Checkpoint Inhibitors (ICIs), multiple agents’ chemotherapy regimen, single agent chemotherapy regimen or placebo (5) reported Hazard Ratios (HR) for OS, PFS or numbers of pathological Complete Response (pCR) in each both arms of the trial. We excluded review articles, non-randomized trials, quasi-randomized trials, meta-analyses and observational studies, as well as studies on animal models. We also excluded trials using non-FDA-approved immune checkpoint inhibitors.
Study selection and screening
The records obtained from applying the search strategy were evaluated on the Rayyan platform [ 22 ]. Titles and abstracts were screened independently by two reviewers either IE/RA or AhE/AbE with any disagreements were resolved by consensus among the entire team (IE, RA, AhE, AbE and MIM). The full texts of studies that were deemed potentially eligible were then retrieved and double-screened independently (IE/RA or AhE/AbE), with discrepancies dealt with through discussion with the whole team (IE, RA, AhE, AbE and MIM).
Data extraction
We extracted information from each eligible study on the first author, publication date, phase, total number of patients included, and number of patients in each arm, as well as patient demographics (median age, cancer stage), treatment given in each arm, duration of treatment, follow-up time and percentage of patients with positive PD-L1 expression at baseline defined by CPS ≥ 1. We also extracted HR values and their 95% Confidence Intervals (CI) for OS and PFS from each study, as well as the number of patients who achieved pCR in both arms. We collected data on the occurrence of common Adverse Events (AEs) in patients from each study arm. When duplicate publications were discovered, only the most recent and complete reports of RCTs were included. Two reviewers extracted all data (IE/RA or AhE/AbE), which was then summarized, discussed by the team, and compiled into an online Microsoft Excel spreadsheet accessible to all authors.
Risk of bias assessment
To assess the risk of bias, version 2 of the Cochrane Risk-Of-Bias (RoB2) assessment tool for randomized trials was used [ 23 ]. This was done independently by the reviewers (IE/RA or AhE/AbE) with disagreement being resolved by discussion and input from a third author (MIM). The RoB2 assessment tool includes five distinct domains with multiple signaling questions to aid in assessing the risk of bias. The five domains in this tool appraise bias arising from the following: randomization process, deviations from intended interventions, missing outcome data, measurement of the outcome and selection of the reported result. Accordingly, the signaling questions provided by the ROB2 tool were answered, and the two other reviewers evaluating the trial used those answers to categorize the current domain as “low risk of bias,” “some concerns,” or “high risk of bias.” The reviewer's judgment in each domain resulted in an overall risk-of-bias conclusion for the trial under consideration. The study was deemed to have a “low risk of bias” if it was judged to have a low risk of bias in all domains included in the tool, “some concerns” if it raised some concerns in at least one domain, or “high risk of bias” if it was judged to have a high risk of bias in at least one or some concerns for multiple domains, significantly lowering confidence in the result. This data for all studies was compiled in the tool's template excel sheet, which was made available to all reviewers.
As our aim is to evaluate the efficacy and safety of ICIs, we selected four different outcomes in this NMA. The first two are OS, which is defined as the time from randomization to death from any cause, and PFS, which is defined as the time from randomization to the first documented disease progression per Response Evaluation Criteria in Solid Tumors version 1.1. The HR and its 95% CI comparing the two arms of the trials in Intention-To-Treat (ITT) populations were used to generate our final effect sizes in this NMA. The third outcome is pCR, which is defined as the absence of invasive tumors in the breast and regional nodes at the time of definitive surgery (ypT0/is pN0). Finally, to assess the safety of PD-1/PD-L1 inhibitors, we estimated the likelihood of developing AEs in each arm of the ITT populations by using the number of patients who had AEs in all grades and grade 3 or higher. Both pCR and AEs were calculated using Odds Ratios (OR) and their 95% CI based on the number of reported events in each of the trial arms.
Data analysis
Our NMA used standard pairwise meta-analysis implemented in multivariate meta-analysis models using a frequentist contrast-based approach [ 24 ]. If there is no evidence of importance in transitivity, a random-effects frequentist NMA has to be performed. These models assume that direct and indirect evidence are consistent. The network meta-analysis' net evidence is a weighted average of direct and indirect evidence. For OS and PFS, we calculated the mean log HR and its standard error and entered it into the model [ 25 ], while for pCR and AEs, we entered the number of events in each arm. When the same intervention was used in both arms of an RCT, it was assumed that the effect of that intervention was cancelled out, thus we assumed that all trials used the same comparator chemotherapy, which is necessary because even within the same trial, different chemotherapy regimens were used as controls. The assumption of transitivity was tested by comparing the distribution of study and population characteristics that may act as effect modifiers across the various pairwise comparisons. If transitivity issues were present, we returned to data extraction to verify the stage of TNBC, and the type of chemotherapy regimen used. In the case of indirect evidence, inconsistency between direct and indirect evidence was investigated locally through the use of symmetrical node-splitting [ 26 ]. However, we found no head-to-head comparisons of PD-1/PD-L1 inhibitors. Visual inspection of comparison-adjusted funnel plots for NMA was used to assess publication bias [ 27 ]. Studies were expected to form an inverted funnel centred at zero in the absence of small-study effects. The Surface Under the Cumulative Ranking Curve (SUCRA) value, which represents the re-scaled mean ranking, was also calculated and summarized [ 28 ]. Where quantitative synthesis is deemed invalid due to a small number of studies using the same intervention, narrative synthesis was used to report the findings in the results section, with estimates from the original studies. For all comparisons, we adopted the network suite in Stata to perform analyses and graphs, Stata version 16 (College Station, TX, USA) [ 29 ].
Subgroup analysis
In the event of significant heterogeneity, we conducted a sensitivity analysis, removing each study and comparing its effect. In terms of the outcome of AEs, we investigated the impact of reported symptoms on AEs to check which side effects are likely to produce this effect. We performed a sensitivity analysis for NMA using the Generalized Pairwise Modelling (GPM) framework to investigate the effect of the models used [ 30 ]. The GPM framework was used to generate mixed treatment effects against a common comparator. The common comparator for all outcomes was chemotherapy. Other than transitivity, this framework requires no additional assumptions [ 30 ]. In this sensitivity analysis, the Inverse Variance Heterogeneity model was used to pool the meta-analytical estimates [ 31 ]. The H index was used to assess statistical heterogeneity across pooled direct effects, while the weighted pooled H index ( \(\overline{H }\) ) was used to examine inconsistency across the network and assess transitivity [ 30 ]. The smallest value that H and \(\overline{H }\) can take is 1, and \(\overline{H }\) <3 was thought to represent minimal inconsistency [ 32 ]. MetaXL version 5.3 was used for the GPM framework analyses (EpiGear Int Pty Ltd.; Brisbane, Australia). The results of those sensitivity analyses will be presented in the Additional file 1 .
Study selection
Figure 1 illustrates the PRISMA flow diagram of the study selection process. Our extensive database and trial registry search yielded 1583 results. 397 duplicates were automatically removed through EndNote. A total of 1186 potentially relevant articles were identified, of which 1056 were excluded after the initial review of their titles and abstracts. The full text of the remaining 130 articles was assessed for eligibility. Of those, 71 were found to be duplicate patient records, and only the most recent and inclusive records were kept. Another 31 RCTs were excluded due to a paucity of outcome measures at the time of the search. Other 16 records were similarly removed for a variety of reasons depicted in Fig. 1 . Eventually, 12 studies were eligible for inclusion in our NMA [ 33 , 34 , 35 , 36 , 37 , 38 , 39 , 40 , 41 , 42 , 43 , 44 ]. Additional file 1 : Table S2 includes all the additional information on the omitted record citations as well as full reasoning.
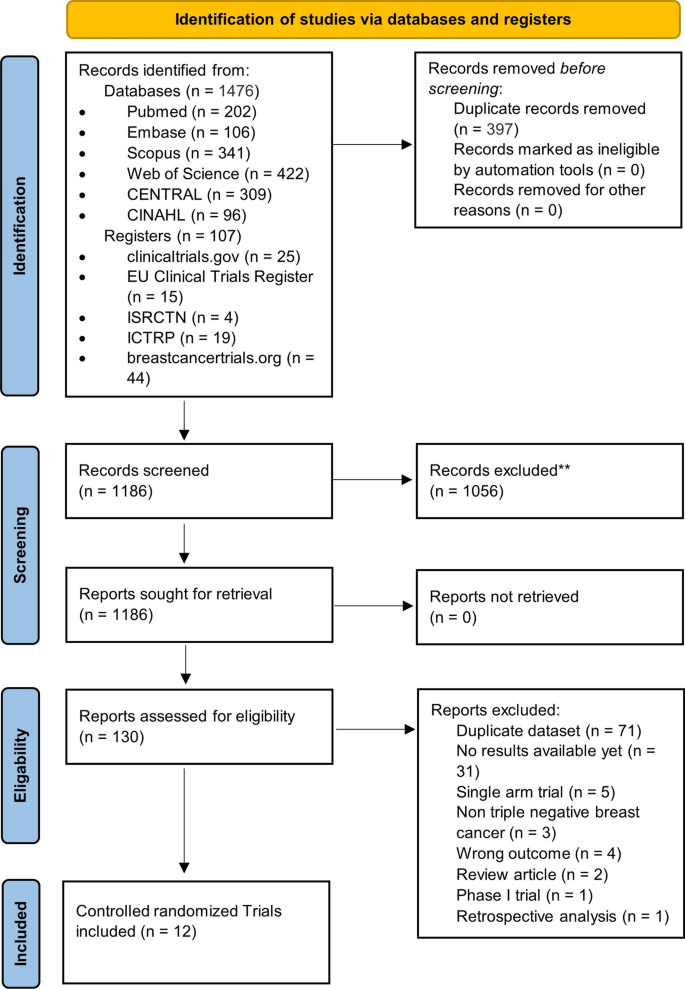
PRISMA flowchart showing the number of studies at each stage of conducting this NMA
Study characteristics and data collection
Table 1 summarizes the characteristics of the included RCTs. All 12 trials included were two-arm trials that reported results from 5324 patients with median ages ranging from 48 to 59.1 years. There were seven phase III trials and five phase II trials. Six studies looked at the effect of PD-1/PD-L1 inhibitors on unresectable, invasive, or metastatic (advanced) TNBC [ 33 , 35 , 36 , 37 , 40 , 43 ], four looked at non-metastatic/early-stage TNBC [ 39 , 41 , 42 , 44 ], and two looked at treated metastatic TNBC for maintenance therapy [ 34 , 38 ]. Atezolizumab (n = 5 trials) was the most commonly studied ICI [ 33 , 36 , 39 , 40 ], followed by Pembrolizumab (n = 4 trials) [ 34 , 35 , 41 , 42 ], Durvalumab (n = 2 trials) [ 37 , 38 ], and Nivolumab (n = 1 trial) [ 43 ]. Six trials used multiple-agent chemotherapy regimens in combination with PD-1/PD-L1 inhibitors [ 36 , 37 , 39 , 41 , 42 , 44 ], and four used mono-chemotherapy regimens with PD-1/PD-L1 inhibitors, including two Taxane-based [ 33 , 40 ], one Platinum-based [ 43 ], and one Investigator's choice chemotherapy [ 35 ]. The other two trials compared PD-1/PD-L1 inhibitors alone to chemotherapy for maintenance therapy in patients with previously treated metastatic TNBC [ 34 , 38 ]. There were some minor differences in the duration of PD-1/PD-L1 inhibitors used between studies. With the exception of one trial [ 44 ], PD-1/PD-L1 inhibitors were used for four to eight cycles with a follow-up time of more than 12 months. The PD-L1 expression in TNBC tissue samples varied significantly between the included RCTs, ranging from 39 to 87% (see Table 1 ). Table 1 is to be inserted here.
Overall, five RCTs had a low risk of bias [ 33 , 35 , 37 , 40 , 41 ], six had some concerns [ 36 , 38 , 39 , 42 , 43 , 44 ], and only one had a high risk of bias [ 34 ]. When following the intended protocol and performing ITT analysis, all included trials were of high quality. Five of the six trials that raised concerns were due to the trial being non-blinded [ 36 , 38 , 42 , 43 , 44 ], which could affect the assessment of the outcome of interest. One study found a significant difference in one of the baseline parameters [ 39 ], while the high-risk study failed to report one of the secondary outcomes in the main text [ 34 ]. Figure 2 depicts the overall risk of bias across all domains (Fig. 2 A), as well as the reviewers' judgment within each domain for all included trials (Fig. 2 B).
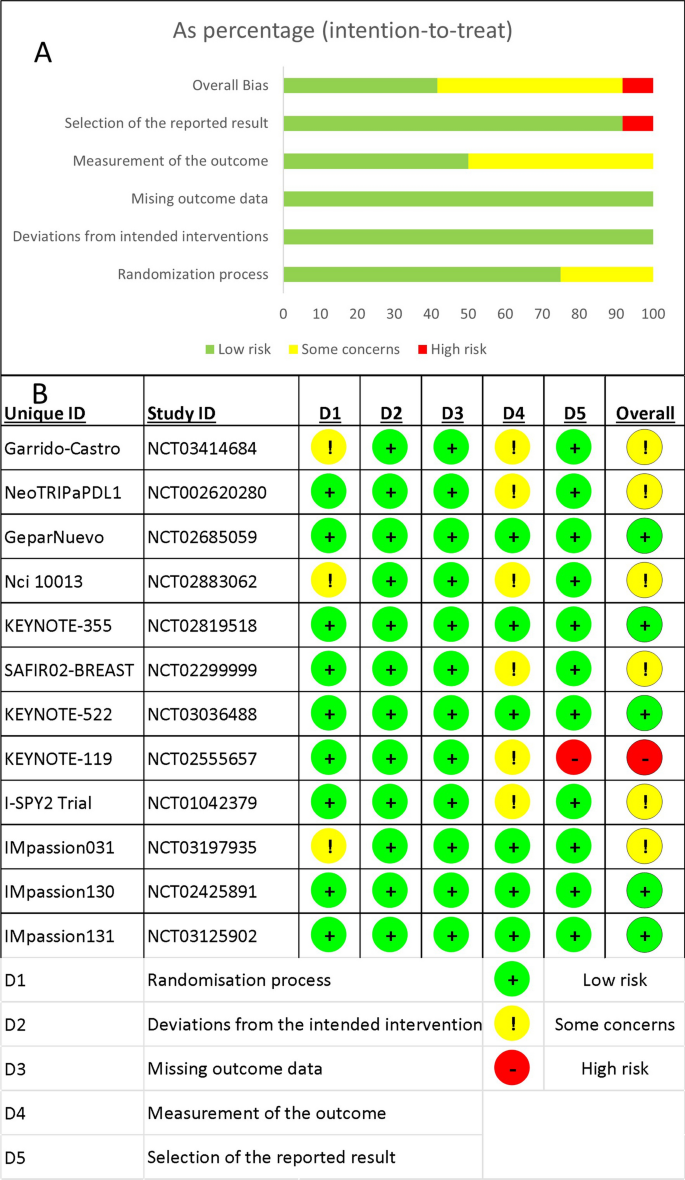
The results of the risk of bias assessment. A Stacked bar chart showing a summary of the risk of bias assessment overall and in each domain. B The detailed answers for all studies in each domain
- Overall survival
The OS was reported in nine RCTs [ 33 , 34 , 35 , 37 , 38 , 39 , 40 , 41 , 43 ], three of which used Atezolizumab [ 33 , 39 , 40 ], two used Pembrolizumab [ 35 , 41 ], and one used either Durvalumab or Nivolumab as a neoadjuvant to chemotherapy (Fig. 3 A) [ 37 , 43 ]. Pembrolizumab in a neoadjuvant setting had a pooled HR of 0.82 (95% CI 0.65 to 1.03, SUCRA = 46%, n = 2 trials, 1449 patients), which was comparable to Atezolizumab’s HR of 0.92 (95% CI 0.74 to 1.15, SUCRA = 28%, n = 3 studies, 1886 patients), demonstrating a prolonged but insignificant OS in PD-1/PD-L1 inhibitors arms (see SUCRA Additional file 1 : Table S3). GeparNuevo using Durvalumab had the only significant reported prolonged OS in PD-1/PD-L1 inhibitors in neoadjuvant settings (HR 0.24, 95% CI 0.08 to 0.72) [ 37 ]. Durvalumab also improved OS when used as a monotherapy for maintenance therapy in patients with metastatic TNBC (SAFIR02-BREAST trial, HR 0.54, 95% CI 0.30 to 0.97) [ 38 ]. This outcome's results were consistent among the studies. The rest of the analysis is shown in Fig. 3 . GPM sensitivity analysis also revealed no significant differences (Additional file 1 : Figure S1).
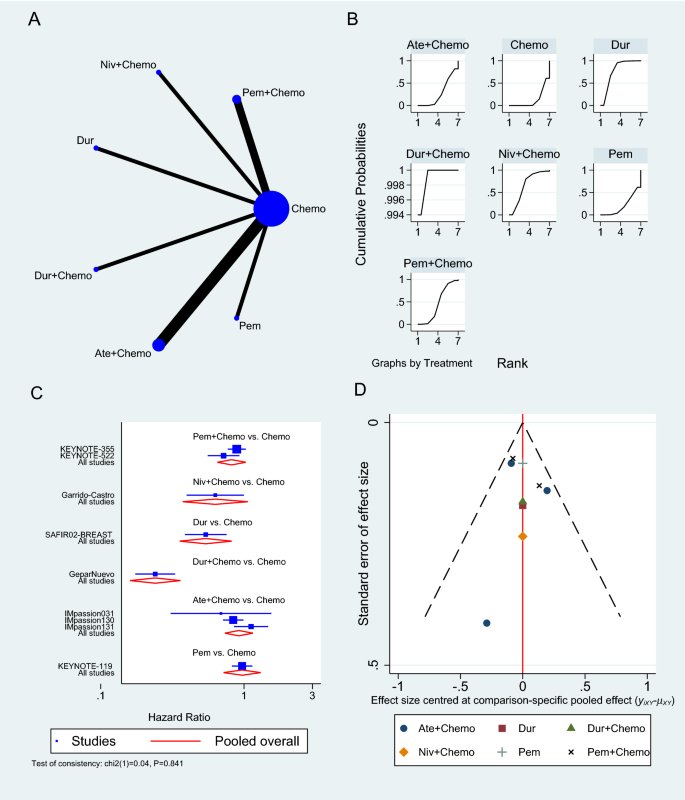
Overall survival network meta-analysis results. A Schematic diagram showing the network map for the treatments included in the analysis. B Rankogram showing the ranking probabilities for the effectiveness of each treatment. C Forest plot showing each trial effect size and confidence interval as well as the pooled effect size. D Bias-adjusted funnel plot showing each treatment separately
- Progression-free survival
Only six RCTs reported PFS [ 33 , 34 , 35 , 38 , 40 , 43 ], two of which used Atezolizumab in neoadjuvant sitting [ 33 , 40 ], as shown in Fig. 4 A. In a neoadjuvant setting along with chemotherapy, Atezolizumab achieved a pooled PFS HR of 0.82 (95% CI 0.69 to 0.97, SUCRA = 76.5%, 1553 patients) (see complete SUCRA values in Additional file 1 : Table S4), whereas Pembrolizumab can also prolong PFS as reported in KEYNOTE-355 trial when combined with chemotherapy (HR 0.82, 95% CI 0.71 to 0.94) [ 35 ]. In the SAFIR02-BREAST trial, Durvalumab had similar PFS to single-agent chemotherapy (HR 0.87, 95% CI 0.54 to 1.42, 82 patients) [ 38 ], whereas Pembrolizumab alone was associated with significantly worse PFS than chemotherapy in KEYNOTE-119 trial (HR 1.60, 95% CI 1.33 to 19.2, 622 patients) [ 34 ]. The rest of the analysis is shown in Fig. 4 , and the GPM sensitivity analysis is illustrated in the Additional file 1 : (Figure S2).
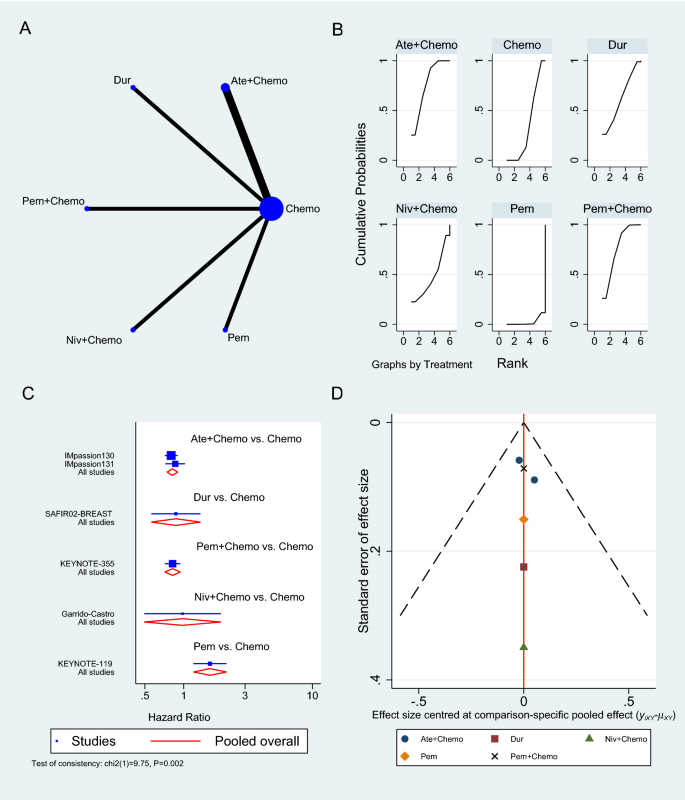
Progression-free survival network meta-analysis results. A Schematic diagram showing the network map for the treatments included in the analysis. B Rankogram showing the ranking probabilities for the effectiveness of each treatment. C Forest plot showing each trial effect size and confidence interval as well as the pooled effect size. D Bias-adjusted funnel plot showing each treatment separately
Pathologic complete response
The number of patients who achieved a complete response was reported in six trials [ 36 , 39 , 41 , 42 , 44 ]: three on Atezolizumab [ 36 , 39 , 44 ], two on Pembrolizumab [ 41 , 42 ], and one on Durvalumab [ 37 ], all in the neoadjuvant setting to chemotherapy. Pembrolizumab in combination with chemotherapy significantly increased the odds of achieving pCR compared to chemotherapy alone (OR 2.79, 95% CI 1.07 to 7.24, SUCRA = 82.1%, 2 studies, 709 patients), whereas Atezolizumab showed an insignificant increase in pCR (OR 1.94, 95% CI 0.86 to 4.37, SUCRA = 62.3, 3 studies, 674 patients) (complete SUCRA values in Additional file 1 : Table S5). In the GeparNuevo trial, the calculated OR of achieving pCR with Durvalumab and chemotherapy was 1.45 (95% CI 0.80 to 2.63) [ 37 ]. Figure 5 summarizes the results of the pCR analysis, and the GPM sensitivity analysis is presented in the Additional file 1 : Figure S3.
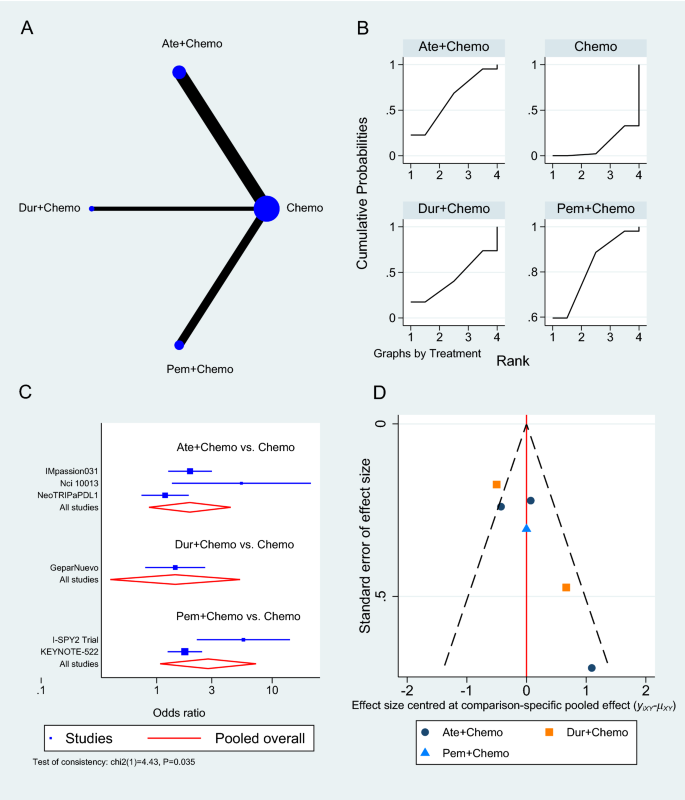
Pathologic complete response network meta-analysis results. A Schematic diagram showing the network map for the treatments included in the analysis. B Rankogram showing the ranking probabilities for the effectiveness of each treatment. C Forest plot showing each trial effect size and confidence interval as well as the pooled effect size. D Bias-adjusted funnel plot showing each treatment separately
- Adverse events
At the time of analysis, nine trials had AEs grade ≥ 3 results reported [ 33 , 34 , 35 , 36 , 39 , 40 , 41 , 42 , 44 ], the majority of which was the effect of Atezolizumab combined with chemotherapy versus chemotherapy alone (n = 5 studies) [ 33 , 36 , 39 , 40 , 44 ], followed by Pembrolizumab with chemotherapy (n = 3 studies) (Fig. 6 A) [ 35 , 41 , 42 ]. The pooled OR of Atezolizumab addition to chemotherapy causing AEs grade 3 or more compared to chemotherapy alone was 1.48 (95% CI 0.90 to 2.42, 5 studies, 2325 patients), whereas Pembrolizumab with chemotherapy showed a slightly greater risk of causing AEs grade ≥ 3 (OR 1.90, 95% CI 1.08 to 3.33, 3 studies, 2263 patients) (Fig. 6 C). Atezolizumab and Pembrolizumab achieved SUCRA values of 26.7% and 9.3% respectively compared to 64.3% for chemotherapy (Additional file 1 : Table S6). When compared to single-agent chemotherapy, the KEYNOTE-119 trial showed a significant reduction in AEs grade ≥ 3 when using Pembrolizumab alone in maintenance therapy (OR 0.29, 95% CI 0.19 to 0.43) [ 34 ].
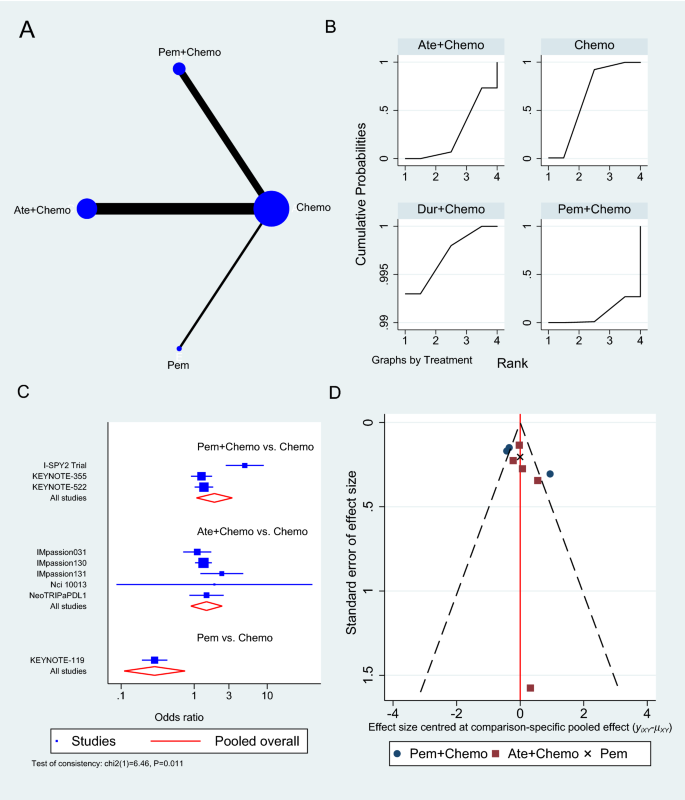
Grade ≥ 3 adverse events network meta-analysis results. A Schematic diagram showing the network map for the treatments included in the analysis. B Rankogram showing the ranking probabilities for the least odds of causing adverse events for each treatment. C Forest plot showing each trial effect size and confidence interval as well as the pooled effect size. D Bias-adjusted funnel plot showing each treatment separately
In the sensitivity analysis investigating the subtype of the reported AEs, neoadjuvant Pembrolizumab to chemotherapy showed an increase in the odds of developing adrenal insufficiency (OR 26.24, 95% CI 3.50 to 197.86, Additional file 1 : Figure S4), diarrhea (OR 1.47, 95% CI 1.14 to 1.88, Additional file 1 : Figure S5), hyperthyroidism (OR 5.22, 95% CI 2.44 to 11.15, Additional file 1 : Figure S6), hypothyroidism (OR 5.23, 95% CI 3.35 to 8.16, Additional file 1 : Figure S7), infusion reaction (OR 1.64, 95% CI 1.13 to 2.37, Additional file 1 : Figure S8) and pneumonitis (OR 5.94, 95% CI 1.29 to 27.27, Additional file 1 : Figure S9). On the other hand, Atezolizumab in the neoadjuvant settings increased the odds of developing hyperthyroidism (OR 10.91, 95% CI 1.98 to 60.15, Additional file 1 : Figure S6), hypothyroidism (OR 3.77, 95% CI 2.52 to 5.63, Additional file 1 : Figure S7) and pneumonitis (OR 2.73, 95% CI 1.41 to 5.31, Additional file 1 : Figure S9) compared to chemotherapy alone. The remaining results of the sensitivity analysis according to the type of AE developed and GPM are outlined in the Additional file 1 : (Figure S10 to Figure S17).
Principle findings and existing literature
TNBC is an aggressive form of breast cancer that is often associated with poor patient outcomes, largely due to the limited treatment options available [ 6 ]. Intensive research efforts have therefore attempted to improve the efficiency of standard-of-care chemotherapy by incorporating immunotherapeutic agents, particularly ICIs, which have emerged as a novel breakthrough in cancer treatment in the past recent years [ 15 ]. The present network meta-analysis aimed to compare the published data on the efficacy and safety of ICIs in treating TNBC. Our results showed that antiPD-1/PD-L1 therapies can be used as a neoadjuvant to chemotherapy in the first-line treatment or alone in previously treated TNBC. Multiple RCTs that were conducted on this topic have demonstrated a greater benefit of adding ICIs to chemotherapy in terms of OS, PFS, and pCR [ 45 , 46 , 47 , 48 , 49 ]. As a result, existing meta-analyses evaluating those trials were successful in achieving statistical and clinical significance. For example, Zhang et al. group reported that PD-1/PD-L1 inhibitors in combination with chemotherapy improved pCR (OR 1.59, 95% CI 1.28 to 1.98), event-free survival (HR 0.66, 95% CI 0.48 to 0.91, p = 0.01), and overall survival (HR 0.72, 95% CI 0.52 to 0.99) in TNBC patients compared to chemotherapy alone [ 45 ]. Moreover, Li et al. studied the pCR of ICIs in neoadjuvant setting in TNBC and reported that the OR significantly increased in their four included study meta-analysis (OR 2.14, 95% CI 1.37–3.35, P < 0.001) and a better event-free survival (HR 0.66, 95% CI 0.48 to 0.89, P = 0.007) [ 49 ], while similar values for pCR were reported by Rizzo et al. (OR 1.95, 95% CI 1.27 to 2.99) and Xin et al. (OR 1.91, 95% CI 1.32 to 2.78) [ 46 , 48 ]. Villacampa et al. reported that patients with PD-L1-positive tumors had a significantly better PFS with ICIs (HR 0.67, 95% CI 0.58 to 0.79) and a trend towards better OS (HR 0.79, 95% CI 0.60 to 1.03), while no benefit was observed in patients with PD-L1-negative tumors [ 47 ]. This is in contrast to Zhang et al. who found that the pCR rate was almost identical in the PD-L1-positive and negative groups [ 45 ]. However, many have reported high heterogeneity in effect estimates, indicating major systematic differences between the included RCTs [ 45 , 46 , 47 , 48 , 49 ]. Although this heterogeneity has been attributed to many factors including patient population, TNBC stage, PD-L1 levels, randomization process, and type of chemotherapy regimen, these meta-analyses have failed to acknowledge the performance differences and the distinct immunologic mechanisms by which ICIs act. Contrary to our study, they have combined all agents into a large group and regarded them as one entity, assuming they have similar efficacy and safety.
Efficacy of PD-1/PD-L1 inhibitors
In our NMA, only two trials out of nine reported statistical significance in terms of OS, both of which used Durvalumab, one as a neoadjuvant (GeparNuevo phase II trial, HR 0.24, 95% CI 0.08 to 0.72, 174 patients) and the other as maintenance (SAFIR02-BREAST trial, HR 0.54, 95% CI 0.30 to 0.97) [ 37 , 38 ]. Six of the remaining seven trials reported longer, yet statistically insignificant survival. This could be attributed to the small sample size or the lack of follow-up, yet the possibility of Durvalumab having superior efficacy remains, highlighting the need for an additional large phase III RCTs investigating Durvalumab efficacy and safety in TNBC. Five of the seven trials that used neoadjuvant PD-1/PD-L1 inhibitors and reported OS were invasive or metastatic (advanced), with only GeparNuevo achieving a significant reduction in OS HR (0.24, 95% CI 0.08 to 0.72). The remaining two neoadjuvant trials (IMpassion031 trial, HR 0.69, 95% CI 0.25 to 1.87) and (KEYNOTE-522 trial, HR 0.72, 95% CI 0.51 to 1.02) were on non-metastatic or advanced and did not show any improvement in OS.
In general, PFS prolongation followed a positive trend similar to OS when ICIs were used. The IMpassion130 trial demonstrated a significant improvement in PFS with Atezolizumab (HR 0.80, 95% CI 0.69 to 0.92) [ 40 ], as opposed to the confirmatory trial Impassion131which failed to achieve statistical significance with Atezolizumab despite extending PFS (HR 0.86, 95% CI 0.70 to 1.05) [ 33 ]. An FDA review of the discordant findings between these two trials, including chemotherapy regimens, study design, conduct and population found no single component that could be responsible for this discrepancy, as a result, the reason for this is unclear at present. It is also worth mentioning that the only two trials that reported statistical significance, KEYNOTE-355 and IMpassion130, are the ones with the largest population sample, which may have accounted for their outcome.
Alternatively, the KEYNOTE-355 trial found that Pembrolizumab is effective in prolonging PFS in the neoadjuvant setting (HR 0.82, 95% CI 0.69 to 0.97) [ 35 ], while Nivolumab appears to be less effective in improving survival PFS (HR 0.98, 95% CI 0.51 to 1.88). Both KEYNOTE-522 and IMpassion031 trials found that using ICI in the neoadjuvant setting improved disease-free survival [ 39 , 41 ]. ICIs use as maintenance therapy instead of chemotherapy in treated metastatic TNBC has also shown promising results in terms of prolonging survival using Durvalumab in the SAFIR02-BREAST trial, in contrast to Pembrolizumab that showed no significant improvement in the Keynote-119 trial (PFS HR 1.6, 95% CI 1.33 to 1.92) [ 34 , 38 ]. Nonetheless, the Keynote-119 trial demonstrated a significant reduction in AEs grade ≥ 3, negating one of chemotherapy's worst attributes [ 34 ]. Furthermore, ICIs have also been shown to improve the chances of achieving pCR in TNBC patients when compared to chemotherapy alone. According to our NMA, neoadjuvant Pembrolizumab resulted in the highest pCR (OR 2.79, 95% CI 1.07 to 7.24), followed by Atezolizumab (OR 1.94, 95% CI 0.86 to 4.37, 3 studies, 674 patients), and Durvalumab, which had the lowest pCR (1.45, 95% CI 0.80 to 2.63). However, among the six trials that reported pCR, NeoTRIPaPDL1 and GeparNuevo were the only two RCTs that did not report significant improvement in pCR [ 36 , 37 ]. This can be explained by the advanced TNBC stage both studies were conducted upon, implying that using ICIs at an earlier stage of TNBC disease progression will more likely benefit patients and improve their survival. This is supported by the fact that early-stage TNBC has a greater tumor immune microenvironment than advanced TNBC, which increases the effectiveness of ICIs with the additional stimulation to the immune response provided by chemotherapy treatment [ 46 ]. Another possibility for the negative NeoTRIPaPDL1 results could be due to the insufficient immune induction effect of the chemotherapy regimens used in the study design [ 46 ].
Safety of PD-1/PD-L1 inhibitors
In regard to safety, ICIs appear to be associated with a significant toxicity burden, especially in the form of immune-related AEs [ 50 ]. Our NMA showed that Pembrolizumab generally has a worse safety profile than Atezolizumab, causing more grade ≥ 3 AEs (OR 1.90, 95% CI 1.08 to 3.33). Despite the fact that both drugs increased the risk of hyperthyroidism, hypothyroidism, and pneumonitis, Pembrolizumab caused a significant increase in adrenal insufficiency, diarrhea, and infusion reaction, making Atezolizumab a safer option. These AEs are likely to be related to drugs’ mechanism of action. The ability of ICIs to reinvigorate exhausted T-cells in an attempt to kill the tumor may destroy the immune tolerance balance and result in autoimmune and inflammatory responses in normal tissue [ 51 , 52 ]. However, the reason why certain people or specific organs are more susceptible than others is still incompletely understood [ 51 ]. Proposed hypotheses include hereditary predisposition, environmental factors and expression of shared antigens between tumors and affected tissue [ 51 ]. Whilst most of these immune-related AEs are usually manageable and reversible, some may require long-term intervention, such as endocrinopathies [ 50 ]. Of note, close monitoring of patients and early detection of any AEs is of utmost importance to ensure patients can benefit from adding PD-1/PD-L1 inhibitors to their chemotherapy regimen. Careful follow-up care is also warranted to prevent potential later onset immune-related AEs that may present after cessation of ICIs [ 50 ].
Enhancing the benefit of PD-1/PD-L1 inhibitors
It is crucial to note that the response to ICIs as well as to the combination of other agents differs significantly among patients, highlighting the importance of predictive biomarkers [ 53 ]. A multitude of promising novel biomarkers has recently gained considerable attention including the CD274 gene and TILs, but to date, PD-L1 status remains the only biomarker approved to guide patient selection in TNBC [ 53 , 54 , 55 ]. We considered PD-L1 positivity as CPS ≥ 1 in Table 1 , yet the threshold for PD-L1 positivity and at what level ICIs become more effective remains a topic of scientific debate. Analysis of the present NMA showed that IMpassion031, Keynote-522, and GeparNuevo trials have all demonstrated PD-1/PD-L1 inhibitors to improve efficacy regardless of PD-L1 status in patients with early-stage TNBC [ 33 , 41 ]. Conversely, IMpassion130 and Keynote-355 demonstrated improved efficacy in metastatic TNBC but not in early-stage TNBC [ 35 , 40 ]. Following the outcomes of the recently published IMpassion130 and KEYNOTE-355 trials, this biomarker was validated as a predictor of response to PD-1/PD-L1 inhibitors in metastatic breast cancer [ 48 ]. Even though data from a previous meta-analysis found no correlation between pCR rates and PD-L1 expression, further investigation revealed pCR rates to be higher in PD-L1-positive patients [ 46 ]. Notably, the lack of a standardized approach for PD-L1 detection in TNBC has led to inconsistent PD-L1 prevalence, thereby hampering the precise guiding of immunotherapy [ 45 , 54 ]. Another significant challenge is that TNBC is composed of numerous heterogeneous subtypes. Biomarker research on IMpassion130 samples revealed that PD-L1 is expressed higher in basal-like immune-activated subtype (75%) and immune-inflamed tumors (63%) TNBC subtypes [ 56 , 57 ]. Another exploratory study found an improved advantage in PFS in TNBC patients with immune-inflamed tumors, basal-like immune-activated and basal-like immunosuppressed subtypes, in addition to the prolonged OS in inflamed tumors and basal-like immune-activated subtypes [ 47 , 56 , 57 ]. Certainly, the identification of predictive biomarkers of efficacy will greatly aid in optimizing personalized regimens for TNBC patients, as well as predicting the long-term effectiveness of PD-1/PD-L1 inhibitors.
Future RCTs using PD-1/PD-L1 inhibitors in TNBC
Interestingly, the majority of the currently ongoing RCTs are investigating Atezolizumab and Pembrolizumab, both of which were studied the most in nine out of the 12 RCTs included in our NMA. Hoffmann-La Roche, the sponsor of IMpassion130, IMpassion131, and Impassion031, is currently funding three additional phase III RCTs on Atezolizumab. IMpassion132 is a double-blind Phase III RCT on the efficacy and safety of neoadjuvant Atezolizumab for early relapsing TNBC (NCT03371017), while IMpassion030 is planned to be the largest RCT on ICI as it is presently recruiting 2300 patients with operable TNBC to investigate the combination of neoadjuvant Atezolizumab and chemotherapy (NCT03498716). Hoffmann-La Roche’s third RCT is looking into the combination of Atezolizumab, Ipatasertib, and Paclitaxel in patients with advanced or metastatic TNBC (NCT04177108). In another phase III double-blinded RCT, GeparDouze will investigate neoadjuvant Atezolizumab followed by adjuvant Atezolizumab in patients with high-risk TNBC (NCT03281954). The National Cancer Institute (NCI) is also funding a large phase III RCT to assess the efficacy and safety of Pembrolizumab as adjuvant therapy following neoadjuvant chemotherapy (NCT02954874). Additionally, ASCENT-04 and ASCENT-05 are both ongoing phase III RCTs investigating the PFS of Pembrolizumab in combination with Sacituzumab Govitecan versus chemotherapy in either advanced or residual invasive TNBC (NCT05382286, NCT05633654). TROPION-Breast03 is similarly a new phase III RCT looking at Datopotamab Deruxtecan (DatoDXd) with or without Durvalumab in early-stage TNBC (NCT05629585). Finally, Avelumab, another PD-L1 inhibitor, is currently being studied in a phase III RCT on high-risk TNBC patients (A-Brave trial, NCT02926196).
Limitations
There are some limitations that must be addressed in this NMA. Firstly, only 12 studies were included, in addition to the limited number of reported outcomes of interest. This is primarily due to the fact that we only included phase II and phase III RCTs because our goal was to compare the efficacy of PD-1/PD-L1 inhibitors in clinical settings. With the ongoing development of neoadjuvant ICI clinical trials, there will certainly be more comprehensive data to be analyzed in future NMA. Second, the NMA comparisons were solely based on direct evidence, with no head-to-head comparisons of neoadjuvant ICIs in TNBC. Moreover, the small number of studies has caused the limited network connectivity to produce large confidence intervals for some estimates, even when effect sizes were large. It may have also resulted in an immature investigation of heterogeneity and publication bias. We would also like to point out the differences between the included studies in terms of TNBC stage, chemotherapy backbone, ICI duration, follow-up time, and PD-L1 expression status. Different chemotherapy backbone regimens used in different studies may have influenced the interpretation of the results as they could have been added to separate groups in the NMA if the number of included studies allowed. Given this heterogeneity and the limited RCTs number, further subgroup analysis based on PD-L1 expression status and nodal involvement, as well as advanced vs early-stage, was not deemed feasible. Finally, all data in this study were derived from published literature, and no individual patient data were used. Noteworthily, the meta-analysis results could potentially be biased by two of the included RCTs that were published as abstracts, which may have relatively incomplete data, missing safety data, and unclear research methods.
Our NMA found variation in efficacy and safety among PD-1/PD-L1 inhibitors used to treat TNBC, as well as significant systematic differences between the RCTs included. To better assess those variations in efficacy, head-to-head trials between those PD-1/PD-L1 inhibitors are needed. In their use as a neoadjuvant to chemotherapy, ICIs demonstrated comparable efficacy in terms of OS, PFS, and pCR. This benefit is offset by an increase in immune-related adverse events, such as hyperthyroidism, hypothyroidism, pneumonitis, and adrenal insufficiency. We also demonstrated that Atezolizumab is safer than Pembrolizumab in the neoadjuvant setting. Only trials evaluating early-stage TNBC showed a significant improvement in pCR, implying that PD-1/PD-L1 inhibitors may be most effective when started early in the disease course. Durvalumab as a maintenance therapy instead of chemotherapy in patients with metastatic TNBC has also shown promising results in terms of survival extension. Future research should focus on PD-L1 expression status and TNBC subtypes, as these parameters may aid in the optimization of personalized treatment regimens for TNBC patients.
Availability of data and materials
Data used in this study analysis is provided in the Additional file 1 : (Table S7). Further analysis data requests and inquiries can be directed to the corresponding author.
Abbreviations
Confidence interval
Combined positive score
Food and Drug Administration
Generalized pairwise modelling
Hazard ratio
- Immune checkpoint inhibitors
Intention-to-treat
- Network meta-analysis
- Pathological complete response
Programmed cell death protein 1
Programmed cell death ligand 1
Preferred reporting items for systematic reviews and meta-analyses
International prospective register of systematic reviews
Cochrane risk-of-bias tool 2
Surface under the cumulative ranking curve
Tumor infiltrating lymphocytes
Triple-negative breast cancer
Huang J, Chan PS, Lok V, Chen X, Ding H, Jin Y, Yuan J, Lao XQ, Zheng ZJ, Wong MC. Global incidence and mortality of breast cancer: a trend analysis. Aging. 2021;13(4):5748–803.
Article PubMed PubMed Central Google Scholar
Sung H, Ferlay J, Siegel RL, Laversanne M, Soerjomataram I, Jemal A, Bray F. Global cancer statistics 2020: GLOBOCAN estimates of incidence and mortality worldwide for 36 cancers in 185 countries. CA Cancer J Clin. 2021;71(3):209–49.
Article PubMed Google Scholar
Arnold M, Morgan E, Rumgay H, Mafra A, Singh D, Laversanne M, Vignat J, Gralow JR, Cardoso F, Siesling S, et al. Current and future burden of breast cancer: global statistics for 2020 and 2040. Breast. 2022;66:15–23.
Yao H, He G, Yan S, Chen C, Song L, Rosol TJ, Deng X. Triple-negative breast cancer: is there a treatment on the horizon? Oncotarget. 2017;8(1):1913–24.
Hsu JY, Chang CJ, Cheng JS. Survival, treatment regimens and medical costs of women newly diagnosed with metastatic triple-negative breast cancer. Sci Rep. 2022;12(1):729.
Article CAS PubMed PubMed Central Google Scholar
Yin L, Duan JJ, Bian XW, Yu SC. Triple-negative breast cancer molecular subtyping and treatment progress. Breast Cancer Res. 2020;22(1):61.
Zaheed M, Wilcken N, Willson ML, O’Connell DL, Goodwin A. Sequencing of anthracyclines and taxanes in neoadjuvant and adjuvant therapy for early breast cancer. Cochrane Database Syst Rev. 2019;2(2):Cd012873.
PubMed Google Scholar
de Ruijter TC, Veeck J, de Hoon JP, van Engeland M, Tjan-Heijnen VC. Characteristics of triple-negative breast cancer. J Cancer Res Clin Oncol. 2011;137(2):183–92.
Article CAS PubMed Google Scholar
Zeichner SB, Terawaki H, Gogineni K. A review of systemic treatment in metastatic triple-negative breast cancer. Breast Cancer. 2016;10:25–36.
CAS PubMed PubMed Central Google Scholar
Nanda R, Chow LQ, Dees EC, Berger R, Gupta S, Geva R, Pusztai L, Pathiraja K, Aktan G, Cheng JD, et al. Pembrolizumab in patients with advanced triple-negative breast cancer: phase Ib KEYNOTE-012 study. J Clin Oncol. 2016;34(21):2460–7.
Manjunath M, Choudhary B. Triple-negative breast cancer: a run-through of features, classification and current therapies. Oncol Lett. 2021;22(1):512.
Abdou Y, Goudarzi A, Yu JX, Upadhaya S, Vincent B, Carey LA. Immunotherapy in triple negative breast cancer: beyond checkpoint inhibitors. NPJ Breast Cancer. 2022;8(1):121.
Pathak N, Chitikela S, Malik PS. Recent advances in lung cancer genomics: application in targeted therapy. Adv Genet. 2021;108:201–75.
Popovic M, Matovina-Brko G, Jovic M, Popovic LS. Immunotherapy: a new standard in the treatment of metastatic clear cell renal cell carcinoma. World J Clin Oncol. 2022;13(1):28–38.
Bai X, Ni J, Beretov J, Graham P, Li Y. Immunotherapy for triple-negative breast cancer: a molecular insight into the microenvironment, treatment, and resistance. J Natl Cancer Cent. 2021;1(3):75–87.
Article Google Scholar
Quinn KM. Innovative approaches to immunotherapy in breast cancer. J Thorac Dis. 2020;12(8):4536–40.
Han Y, Liu D, Li L. PD-1/PD-L1 pathway: current researches in cancer. Am J Cancer Res. 2020;10(3):727–42.
Jiang X, Wang J, Deng X, Xiong F, Ge J, Xiang B, Wu X, Ma J, Zhou M, Li X, et al. Role of the tumor microenvironment in PD-L1/PD-1-mediated tumor immune escape. Mol Cancer. 2019;18(1):10.
Narayan P, Wahby S, Gao JJ, Amiri-Kordestani L, Ibrahim A, Bloomquist E, Tang S, Xu Y, Liu J, Fu W, et al. FDA approval summary: atezolizumab plus paclitaxel protein-bound for the treatment of patients with advanced or metastatic TNBC whose tumors express PD-L1. Clin Cancer Res. 2020;26(10):2284–9.
Brian H, Georgia S, Deborah MC, Anna C, Christopher HS, Chris C, John PAI, Sharon S, Kristian T, Jeroen PJ, Cynthia M, Ferrán C-L, Peter CG, Kay D, Isabelle B, Douglas GA, David M. The PRISMA extension statement for reporting of systematic reviews incorporating network meta-analyses of health care interventions: checklist and explanations. Ann Intern Med. 2015;162(11):777–84.
Clark JM, Sanders S, Carter M, Honeyman D, Cleo G, Auld Y, Booth D, Condron P, Dalais C, Bateup S, et al. Improving the translation of search strategies using the polyglot search translator: a randomized controlled trial. J Med Libr Assoc. 2020;108(2):195–207.
Ouzzani M, Hammady H, Fedorowicz Z, Elmagarmid A. Rayyan-a web and mobile app for systematic reviews. Syst Rev. 2016;5(1):210.
Sterne JAC, Savović J, Page MJ, Elbers RG, Blencowe NS, Boutron I, Cates CJ, Cheng H-Y, Corbett MS, Eldridge SM, et al. RoB 2: a revised tool for assessing risk of bias in randomised trials. BMJ. 2019;366:l4898.
White IR, Barrett JK, Jackson D, Higgins JPT. Consistency and inconsistency in network meta-analysis: model estimation using multivariate meta-regression. Res Synth Methods. 2012;3(2):111–25.
Woods BS, Hawkins N, Scott DA. Network meta-analysis on the log-hazard scale, combining count and hazard ratio statistics accounting for multi-arm trials: a tutorial. BMC Med Res Methodol. 2010;10(1):54.
Dias S, Welton NJ, Caldwell DM, Ades AE. Checking consistency in mixed treatment comparison meta-analysis. Stat Med. 2010;29(7–8):932–44.
Chaimani A, Salanti G. Using network meta-analysis to evaluate the existence of small-study effects in a network of interventions. Res Synth Methods. 2012;3(2):161–76.
Salanti G, Ades AE, Ioannidis JP. Graphical methods and numerical summaries for presenting results from multiple-treatment meta-analysis: an overview and tutorial. J Clin Epidemiol. 2011;64(2):163–71.
White IR. Network meta-analysis. Stata J. 2015;15(4):951–85.
Doi SAR, Barendregt JJ. A generalized pairwise modelling framework for network meta-analysis. Int J Evid Based Healthc. 2018;16(4):187–94.
Doi SAR, Barendregt JJ, Khan S, Thalib L, Williams GM. Advances in the meta-analysis of heterogeneous clinical trials I: the inverse variance heterogeneity model. Contemp Clin Trial. 2015;45:130–8.
Doi SAR, Barendregt JJ. A generalized pairwise modelling framework for network meta-analysis. JBI Evid Implement. 2018;16(4):187–94.
Google Scholar
Miles D, Gligorov J, André F, Cameron D, Schneeweiss A, Barrios C, Xu B, Wardley A, Kaen D, Andrade L, et al. Primary results from IMpassion131, a double-blind, placebo-controlled, randomised phase III trial of first-line paclitaxel with or without atezolizumab for unresectable locally advanced/metastatic triple-negative breast cancer. Ann Oncol. 2021;32(8):994–1004.
Winer EP, Lipatov O, Im SA, Goncalves A, Muñoz-Couselo E, Lee KS, Schmid P, Tamura K, Testa L, Witzel I, et al. Pembrolizumab versus investigator-choice chemotherapy for metastatic triple-negative breast cancer (KEYNOTE-119): a randomised, open-label, phase 3 trial. Lancet Oncol. 2021;22(4):499–511.
Cortes J, Cescon DW, Rugo HS, Nowecki Z, Im SA, Yusof MM, Gallardo C, Lipatov O, Barrios CH, Holgado E, et al. Pembrolizumab plus chemotherapy versus placebo plus chemotherapy for previously untreated locally recurrent inoperable or metastatic triple-negative breast cancer (KEYNOTE-355): a randomised, placebo-controlled, double-blind, phase 3 clinical trial. Lancet. 2020;396(10265):1817–28.
Gianni L, Huang CS, Egle D, Bermejo B, Zamagni C, Thill M, Anton A, Zambelli S, Bianchini G, Russo S, et al. Pathologic complete response (pCR) to neoadjuvant treatment with or without atezolizumab in triple-negative, early high-risk and locally advanced breast cancer: NeoTRIP Michelangelo randomized study. Ann Oncol. 2022;33(5):534–43.
Loibl S, Schneeweiss A, Huober J, Braun M, Rey J, Blohmer JU, Furlanetto J, Zahm DM, Hanusch C, Thomalla J, et al. Neoadjuvant durvalumab improves survival in early triple-negative breast cancer independent of pathological complete response. Ann Oncol. 2022;33(11):1149–58.
Bachelot T, Filleron T, Bieche I, Arnedos M, Campone M, Dalenc F, Coussy F, Sablin MP, Debled M, Lefeuvre-Plesse C, et al. Durvalumab compared to maintenance chemotherapy in metastatic breast cancer: the randomized phase II SAFIR02-BREAST IMMUNO trial. Nat Med. 2021;27(2):250–5.
Mittendorf EA, Zhang H, Barrios CH, Saji S, Jung KH, Hegg R, Koehler A, Sohn J, Iwata H, Telli ML, et al. Neoadjuvant atezolizumab in combination with sequential nab-paclitaxel and anthracycline-based chemotherapy versus placebo and chemotherapy in patients with early-stage triple-negative breast cancer (IMpassion031): a randomised, double-blind, phase 3 trial. The Lancet. 2020;396(10257):1090–100.
Article CAS Google Scholar
Schmid P, Adams S, Rugo HS, Schneeweiss A, Barrios CH, Iwata H, Diéras V, Hegg R, Im S-A, Shaw Wright G, et al. Atezolizumab and nab-paclitaxel in advanced triple-negative breast cancer. New Engl J Med. 2018;379(22):2108–21.
Schmid P, Cortes J, Pusztai L, McArthur H, Kümmel S, Bergh J, Denkert C, Park YH, Hui R, Harbeck N, et al. Pembrolizumab for early triple-negative breast cancer. New Engl J Med. 2020;382(9):810–21.
Nanda R, Liu MC, Yau C, Shatsky R, Pusztai L, Wallace A, Chien AJ, Forero-Torres A, Ellis E, Han H, et al. Effect of pembrolizumab plus neoadjuvant chemotherapy on pathologic complete response in women with early-stage breast cancer: an analysis of the ongoing phase 2 adaptively randomized I-SPY2 trial. JAMA Oncol. 2020;6(5):676–84.
Garrido-Castro AC, Graham N, Bi K, Park J, Fu J, Keenan T, Richardson ET, Pastorello R, Lange P, Attaya V, et al. Abstract P2–14–18: A randomized phase II trial of carboplatin with or without nivolumab in metastatic triple-negative breast cancer. Cancer Res. 2022;82(4_Supplement):P2-14-18-P12-14–8.
Ademuyiwa FO, Gao F, Chen I, Northfelt DW, Wesolowski R, Arora M, Brufsky A, Dees C, Santa-Maria CA, Connolly RM, et al. Abstract PD14–09: Nci 10013—a randomized phase 2 study of neoadjuvant carboplatin and paclitaxel, with or without atezolizumab in triple negative breast cancer (TNBC). Cancer Res. 2021;81(4_Supplement):PD14-09-PD14-09.
Zhang M, Song J, Yang H, Jin F, Zheng A. Efficacy and safety of PD-1/PD-L1 inhibitors in triple-negative breast cancer: a systematic review and meta-analysis. Acta Oncol. 2022;61(9):1105–15.
Xin Y, Shen G, Zheng Y, Guan Y, Huo X, Li J, Ren D, Zhao F, Liu Z, Li Z, et al. Immune checkpoint inhibitors plus neoadjuvant chemotherapy in early triple-negative breast cancer: a systematic review and meta-analysis. BMC Cancer. 2021;21(1):1261.
Villacampa G, Tolosa P, Salvador F, Sánchez-Bayona R, Villanueva L, Dienstmann R, Ciruelos E, Pascual T. Addition of immune checkpoint inhibitors to chemotherapy versus chemotherapy alone in first-line metastatic triple-negative breast cancer: a systematic review and meta-analysis. Cancer Treat Rev. 2022;104:102352.
Rizzo A, Cusmai A, Massafra R, Bove S, Comes MC, Fanizzi A, Rinaldi L, Acquafredda S, Gadaleta-Caldarola G, Oreste D, et al. Pathological complete response to neoadjuvant chemoimmunotherapy for early triple-negative breast cancer: an updated meta-analysis. Cells. 2022;11(12):1857.
Li Y, Xing L, Li F, Liu H, Gan L, Yang D, Wang M, Yin X, Li H, Ren G. Efficacy and safety of adding immune checkpoint inhibitors to neoadjuvant chemotherapy against triple-negative breast cancer: a meta-analysis of randomized controlled trials. Front Oncol. 2021;11:657634.
Brown LC, Loi S. Immune checkpoint inhibition in the treatment of early stage triple negative breast cancer: 2021 update. Breast. 2022;62 Suppl 1(Suppl 1):S29-s33.
Wang DY, Johnson DB, Davis EJ. Toxicities associated with PD-1/PD-L1 blockade. Cancer J. 2018;24(1):36–40.
Zheng J, Cui T, Gao Y, Li T. Retrospective analysis of immune-related adverse events of the immune checkpoint inhibitors of PD-1/PD-l1 in the Fujian provincial hospital. Eur J Inflam. 2022;20:1721727X221091540.
Carretero-González A, Otero I, Lora D, Carril-Ajuria L, Castellano D, de Velasco G. Efficacy and safety of anti-PD-1/PD-L1 combinations versus standard of care in cancer: a systematic review and meta-analysis. Oncoimmunology. 2021;10(1):1878599.
Chen F, Chen N, Gao Y, Jia L, Lyu Z, Cui J. Clinical progress of PD-1/L1 inhibitors in breast cancer immunotherapy. Front Oncol. 2021;11:724424.
Leite KRM, Barrios CH, Buzaid AC, Gagliato D, Gobbi H, Soares F. Applicability of PD-L1 tests to tailor triple-negative breast cancer treatment in Brazil. Surg Exp Pathol. 2021;4(1):10.
Emens LA, Goldstein LD, Schmid P, Rugo HS, Adams S, Barrios CH, Schneeweiss A, Dieras V, Iwata H, Chang C-W, et al. The tumor microenvironment (TME) and atezolizumab + nab-paclitaxel (A+nP) activity in metastatic triple-negative breast cancer (mTNBC): IMpassion130. J Clin Oncol. 2021;39(15_suppl):1006–1006.
Emens LA, Molinero L, Loi S, Rugo HS, Schneeweiss A, Diéras V, Iwata H, Barrios CH, Nechaeva M, Nguyen-Duc A, et al. Atezolizumab and nab-paclitaxel in advanced triple-negative breast cancer: biomarker evaluation of the IMpassion130 study. J Natl Cancer Inst. 2021;113(8):1005–16.
Download references
Acknowledgements
QNL Funded the APC publication of this article.
Open Access funding provided by the Qatar National Library. This research did not receive any specific grants from funding agencies in the public, commercial, or not-for-profit sectors.
Author information
Authors and affiliations.
College of Medicine, QU Health, Qatar University, P. O. Box: 2713, Doha, Qatar
Ibrahim Elmakaty, Ruba Abdo, Ahmed Elsabagh & Abdelrahman Elsayed
Pathology Unit, Department of Basic Medical Sciences, College of Medicine, QU Health, Qatar University, Doha, Qatar
Mohammed Imad Malki
You can also search for this author in PubMed Google Scholar
Contributions
IE: Data curation, Formal analysis, Investigation, Methodology, Writing—original draft, Writing—review and editing, illustration of tables and figures. RA: Data curation, Formal analysis, Writing—original draft, Writing—review and editing. AE: Data curation, Formal analysis, Writing—original draft, Writing—review and editing. AE: Data curation, Formal analysis, Writing—review and editing. MIM: Conceptualization, Data curation, Formal analysis, Investigation, Methodology, Project administration, Supervision, Validation, Funding Acquisition, Writing—original draft, Writing—review and editing. All authors read and approved the final manuscript.
Corresponding author
Correspondence to Mohammed Imad Malki .
Ethics declarations
Ethics approval and consent to participate.
Not applicable. All the work was developed using published data.
Consent for publication
Not applicable.
Competing interests
The authors declare that they have no known competing financial interests or personal relationships that could have appeared to influence the work reported in this paper.
Additional information
Publisher's note.
Springer Nature remains neutral with regard to jurisdictional claims in published maps and institutional affiliations.
Supplementary Information
Additional file 1: table s1:.
PRISMA checklist for this network meta-analysis. Table S2: Excluded articles at full-text screening. Table S3: Overall survival treatment ranking and surface under the cumulative ranking curve. Figure S1: Overall survival using generalized pairwise modelling. Table S4: Progression free survival treatment ranking and surface under the cumulative ranking curve. Figure S2: Progression free survival using generalized pairwise modelling. Table S5: Pathologic complete response treatment ranking and surface under the cumulative ranking curve. Figure S3: Pathologic complete response using generalized pairwise modelling. Table S6: Adverse events grade ≥ 3 treatment ranking and surface under the cumulative Table. Figure S4: Adrenal insufficiency odds network meta-analysis results. A Schematic diagram showing the network map for the treatments included in the analysis. B Rankogram showing the ranking probabilities for the least odds of causing this adverse event for each treatment. C Forest plot showing each trial effect size and confidence interval as well as the pooled effect size. D Bias-adjusted funnel plot showing each treatment separately. Figure S5: Diarrhea odds network meta-analysis results. A Schematic diagram showing the network map for the treatments included in the analysis. B Rankogram showing the ranking probabilities for the least odds of causing this adverse event for each treatment. C Forest plot showing each trial effect size and confidence interval as well as the pooled effect size. D Bias-adjusted funnel plot showing each treatment separately. Figure S6: Hyperthyroidism odds network meta-analysis results. A Schematic diagram showing the network map for the treatments included in the analysis. B Rankogram showing the ranking probabilities for the least odds of causing this adverse event for each treatment. C Forest plot showing each trial effect size and confidence interval as well as the pooled effect size. D Bias-adjusted funnel plot showing each treatment separately. Figure S7: Hypothyroidism odds network meta-analysis results. A Schematic diagram showing the network map for the treatments included in the analysis. B Rankogram showing the ranking probabilities for the least odds of causing this adverse event for each treatment. C Forest plot showing each trial effect size and confidence interval as well as the pooled effect size. D Bias-adjusted funnel plot showing each treatment separately. Figure S8: Infusion reaction odds network meta-analysis results. A Schematic diagram showing the network map for the treatments included in the analysis. B Rankogram showing the ranking probabilities for the least odds of causing this adverse event for each treatment. C Forest plot showing each trial effect size and confidence interval as well as the pooled effect size. D Bias-adjusted funnel plot showing each treatment separately. Figure S9: Pneumonitis odds network meta-analysis results. A Schematic diagram showing the network map for the treatments included in the analysis. B Rankogram showing the ranking probabilities for the least odds of causing this adverse event for each treatment. C Forest plot showing each trial effect size and confidence interval as well as the pooled effect size. D Bias-adjusted funnel plot showing each treatment separately. Figure S10: Anemia odds network meta-analysis results. A Schematic diagram showing the network map for the treatments included in the analysis. B Rankogram showing the ranking probabilities for the least odds of causing this adverse event for each treatment. C Forest plot showing each trial effect size and confidence interval as well as the pooled effect size. D Bias-adjusted funnel plot showing each treatment separately. Figure S11: Colitis odds network meta-analysis results. A Schematic diagram showing the network map for the treatments included in the analysis. B Rankogram showing the ranking probabilities for the least odds of causing this adverse event for each treatment. C Forest plot showing each trial effect size and confidence interval as well as the pooled effect size. D Bias-adjusted funnel plot showing each treatment separately. Figure S12: Fatigue odds network meta-analysis results. A Schematic diagram showing the network map for the treatments included in the analysis. B Rankogram showing the ranking probabilities for the least odds of causing this adverse event for each treatment. C Forest plot showing each trial effect size and confidence interval as well as the pooled effect size. D Bias-adjusted funnel plot showing each treatment separately. Figure S13: Nausea odds network meta-analysis results. A Schematic diagram showing the network map for the treatments included in the analysis. B Rankogram showing the ranking probabilities for the least odds of causing this adverse event for each treatment. C Forest plot showing each trial effect size and confidence interval as well as the pooled effect size. D Bias-adjusted funnel plot showing each treatment separately. Figure S14: Neutropenia odds network meta-analysis results. A Schematic diagram showing the network map for the treatments included in the analysis. B Rankogram showing the ranking probabilities for the least odds of causing this adverse event for each treatment. C Forest plot showing each trial effect size and confidence interval as well as the pooled effect size. D Bias-adjusted funnel plot showing each treatment separately. Figure S15: Rash odds network meta-analysis results. A Schematic diagram showing the network map for the treatments included in the analysis. B Rankogram showing the ranking probabilities for the least odds of causing this adverse event for each treatment. C Forest plot showing each trial effect size and confidence interval as well as the pooled effect size. D Bias-adjusted funnel plot showing each treatment separately. Figure S16: Vomiting odds network meta-analysis results. A Schematic diagram showing the network map for the treatments included in the analysis. B Rankogram showing the ranking probabilities for the least odds of causing this adverse event for each treatment. C Forest plot showing each trial effect size and confidence interval as well as the pooled effect size. D Bias-adjusted funnel plot showing each treatment separately. Figure S17: Adverse events grade ≥ 3 using generalized pairwise modelling. Table S7: Extracted data used for the analysis.
Rights and permissions
Open Access This article is licensed under a Creative Commons Attribution 4.0 International License, which permits use, sharing, adaptation, distribution and reproduction in any medium or format, as long as you give appropriate credit to the original author(s) and the source, provide a link to the Creative Commons licence, and indicate if changes were made. The images or other third party material in this article are included in the article's Creative Commons licence, unless indicated otherwise in a credit line to the material. If material is not included in the article's Creative Commons licence and your intended use is not permitted by statutory regulation or exceeds the permitted use, you will need to obtain permission directly from the copyright holder. To view a copy of this licence, visit http://creativecommons.org/licenses/by/4.0/ . The Creative Commons Public Domain Dedication waiver ( http://creativecommons.org/publicdomain/zero/1.0/ ) applies to the data made available in this article, unless otherwise stated in a credit line to the data.
Reprints and permissions
About this article
Cite this article.
Elmakaty, I., Abdo, R., Elsabagh, A. et al. Comparative efficacy and safety of PD-1/PD-L1 inhibitors in triple negative breast cancer: a systematic review and network meta-analysis of randomized controlled trials. Cancer Cell Int 23 , 90 (2023). https://doi.org/10.1186/s12935-023-02941-7
Download citation
Received : 11 January 2023
Accepted : 07 May 2023
Published : 11 May 2023
DOI : https://doi.org/10.1186/s12935-023-02941-7
Share this article
Anyone you share the following link with will be able to read this content:
Sorry, a shareable link is not currently available for this article.
Provided by the Springer Nature SharedIt content-sharing initiative
- PD-1/PD-L1 inhibitors
- Systematic review
Cancer Cell International
ISSN: 1475-2867
- General enquiries: [email protected]
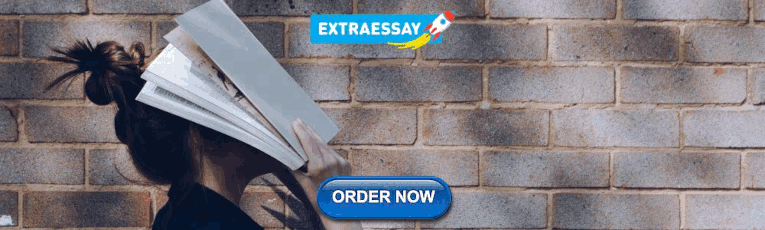
IMAGES
VIDEO
COMMENTS
Covidence. This online platform is a core component of the Cochrane toolkit, supporting parts of the systematic review process, including title/abstract and full-text screening, documentation, and reporting. The Covidence platform enables collaboration of the entire systematic reviews team and is suitable for researchers and students at all ...
Both AMSTAR-2 and ROBIS require systematic and comprehensive searches for evidence. This is essential for any systematic review. Both tools discourage search restrictions based on language and publication source. Given increasing globalism in health care, the practice of including English-only literature should be avoided .
The World's #1 Systematic Review Tool. Reviewers . Organizations. See your systematic reviews like never before. Faster reviews. An average 35% reduction in time spent per review, saving an average of 71 hours per review. Expert, online support.
Both AMSTAR-2 and ROBIS require systematic and comprehensive searches for evidence. This is essential for any systematic review. Both tools discourage search restrictions based on language and publication source. Given increasing globalism in health care, the practice of including English-only literature should be avoided .
RevMan: Systematic review and meta-analysis tool for researchers worldwide. Pricing & subscription. Intuitive and easy to use Meta-analysis has never been so easy. High quality support and guidance Up-to date how-to articles and screencasts guides you to get started. Promotes high quality research
Literature reviews establish the foundation of academic inquires. However, in the planning field, we lack rigorous systematic reviews. In this article, through a systematic search on the methodology of literature review, we categorize a typology of literature reviews, discuss steps in conducting a systematic literature review, and provide suggestions on how to enhance rigor in literature ...
A Systematic Literature Review (SLR) is a research methodology to collect, identify, and critically analyze the available research studies ... (WoS) is an international and multidisciplinary tool for accessing literature in science, technology, biomedicine, and other disciplines. Scopus is a database that today indexes 40,562 peer-reviewed ...
SR Toolbox. The Systematic Review Toolbox is a community-driven, searchable, web-based catalogue of tools that support the systematic review process across multiple domains. The resource aims to help reviewers find appropriate tools based on how they provide support for the systematic review process. Users can perform a simple keyword search (i.e. Quick Search) to locate tools, a more detailed ...
Rayyan Enterprise and Rayyan Teams+ make it faster, easier and more convenient for you to manage your research process across your organization. Accelerate your research across your team or organization and save valuable researcher time. Build and preserve institutional assets, including literature searches, systematic reviews, and full-text ...
Feature Analysis of Systematic Review Tools. ... (3/29, 10%), a search engine (1/29, 3%), and a social science literature review tool (1/29, 3%). One tool, Research Screener , was excluded owing to insufficient information available on supported features. Another tool, the Health Assessment Workspace Collaborative, was excluded because it is ...
The basic concepts of systematic literature review and related work are presented in Sect. 2. In Sect. 3, tools to support SLR through visualisation are described, and an overview of SLR activities that are supported within these tools is given. Conclusions and suggestions for future research are given in the Sect. 4.
Free, open-source tool that "helps you upload and organize the results of a literature search for a systematic review. It also makes it possible for your team to screen, organize, and manipulate all of your abstracts in one place." -From Center for Evidence Synthesis in Health. SRDR Plus (Systematic Review Data Repository: Plus) An open-source ...
And check out the Systematic Review Toolbox for additional software suggestions for conducting your review. Quality Assessment Tools (i.e., risk of bias, critical appraisal) 2022 Repository of Quality Assessment and Risk of Bias Tools - A comprehensive resource for finding and selecting a risk of bias or quality assessment tool for evidence ...
Automatic systematic review tools can be categorised into several categories: visualization tools - tools that use active learning (a combination of a Natural Language Processing (NLP) technique, machine learning classifier, and human labour) and automated tools that employ an NLP and classifier but they use labelled documents and no human interaction during the learning process (Scott et al ...
Full-Featured Software Tools for Conducting Systematic Reviews. EPPI-Reviewer 4: EPPI-Reviewer is web-based software that supports reference management, screening, coding and synthesis. It is developed by the Evidence for Policy and Practice Information and Coordinating Centre in London. Pricing is based on a subscription model.
There are many tools you can use when conducting a systematic review. These tools are designed to assist with the key stages of the process, including title and abstract screening, data synthesis, and critical appraisal. Registering your review is recommended best practice and options are explored in the Register your review section of this guide.
Systematic review vs. literature review. A literature review is a type of review that uses a less systematic and formal approach than a systematic review. Typically, an expert in a topic will qualitatively summarize and evaluate previous work, without using a formal, explicit method. ... Note Generative AI tools like ChatGPT can be useful at ...
These steps for conducting a systematic literature review are listed below. Also see subpages for more information about: What are Literature Reviews? The different types of literature reviews, including systematic reviews and other evidence synthesis methods; Conducting & Reporting Systematic Reviews; Finding Systematic Reviews; Tools & Tutorials
A systematic review collects all possible studies related to a given topic and design, and reviews and analyzes their results [ 1 ]. During the systematic review process, the quality of studies is evaluated, and a statistical meta-analysis of the study results is conducted on the basis of their quality. A meta-analysis is a valid, objective ...
Finalize your literature review faster with comfort. ATLAS.ti makes it easy to manage, organize, and analyze articles, PDFs, excerpts, and more for your projects. Conduct a deep systematic literature review and get the insights you need with a comprehensive toolset built specifically for your research projects.
Objective: The objectives of this scoping review are to identify the reliability and validity of the available tools, their limitations and any recommendations to further improve the use of these tools. Study design: A scoping review methodology was followed to map the literature published on the challenges and solutions of conducting evidence synthesis using the JBI scoping review methodology.
The Preferred Reporting Items for Systematic reviews and Meta-Analyses (PRISMA) statement, published in 2009, was designed to help systematic reviewers transparently report why the review was done, what the authors did, and what they found. Over the past decade, advances in systematic review methodology and terminology have necessitated an update to the guideline. The PRISMA 2020 statement ...
6. Consensus. Researchers to work together, annotate, and discuss research papers in real-time, fostering team collaboration and knowledge sharing. 7. RAx. Researchers to perform efficient literature search and analysis, aiding in identifying relevant articles, saving time, and improving the quality of research. 8.
In February, I received two peer-review reports for a manuscript I'd submitted to a journal. One report contained 3 comments, the other 11. Apart from one point, all the feedback was different.
In recent years, deep learning has evolved as a rapidly growing and stimulating field of machine learning and has redefined state-of-the-art performances in a variety of applications. There are multiple deep learning models that have distinct architectures and capabilities. Up to the present, a large number of novel variants of these baseline deep learning models is proposed to address the ...
It also identified technological tools that were employed by libraries to facilitate access to library information resources. We also investigated the challenges faced by students in accessing library information resources. ... A systematic literature review approach using PRISMA guidelines was employed to investigate the relevant literature on ...
Ethics and dissemination This article is a literature review that does not include patients' identifiable information. Therefore, ethical approval is not required in this protocol. The findings of this systematic review and meta-analysis will be published in a peer-reviewed journal as well as presentations at relevant conferences.
Protocol and registration. This systematic review and meta-analysis is reported following the Preferred Reporting Items for Systematic Reviews and Meta-Analyses (PRISMA) extension for NMA Additional file 1: (Table S1) [].The NMA protocol was carried in accordance with a protocol that had been registered in the International Prospective Register of Systematic Reviews (PROSPERO) online database ...
Studies that provided information based on a systematic literature review on the risk factors for non-adherence fully within the context of LT were considered to be the strongest. In addition, studies using control factors to provide results for specific subgroups, in addition to aggregate results (for example, on prevalence rates of non ...
A systematic literature review approach using PRISMA guidelines was employed to investigate the relevant literature on the subject. The keyword search strategy was employed to search for relevant literature from four scholarly databases Scopus, emerald, Research4life, and Google Scholar. ... It also identified technological tools that were ...