- Skip to main content
- Skip to primary sidebar
- Skip to footer
- QuestionPro

- Solutions Industries Gaming Automotive Sports and events Education Government Travel & Hospitality Financial Services Healthcare Cannabis Technology Use Case NPS+ Communities Audience Contactless surveys Mobile LivePolls Member Experience GDPR Positive People Science 360 Feedback Surveys
- Resources Blog eBooks Survey Templates Case Studies Training Help center

Home Market Research
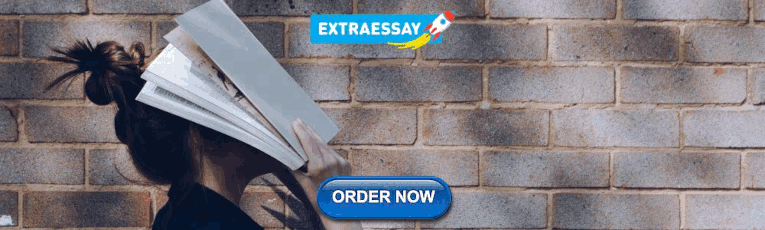
Empirical Research: Definition, Methods, Types and Examples
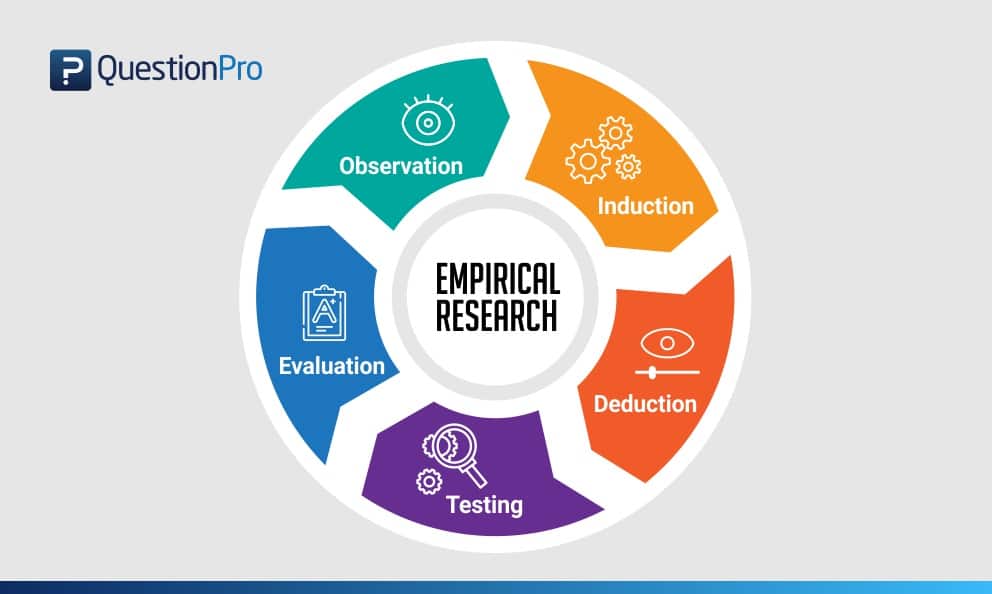
Content Index
Empirical research: Definition
Empirical research: origin, quantitative research methods, qualitative research methods, steps for conducting empirical research, empirical research methodology cycle, advantages of empirical research, disadvantages of empirical research, why is there a need for empirical research.
Empirical research is defined as any research where conclusions of the study is strictly drawn from concretely empirical evidence, and therefore “verifiable” evidence.
This empirical evidence can be gathered using quantitative market research and qualitative market research methods.
For example: A research is being conducted to find out if listening to happy music in the workplace while working may promote creativity? An experiment is conducted by using a music website survey on a set of audience who are exposed to happy music and another set who are not listening to music at all, and the subjects are then observed. The results derived from such a research will give empirical evidence if it does promote creativity or not.
LEARN ABOUT: Behavioral Research
You must have heard the quote” I will not believe it unless I see it”. This came from the ancient empiricists, a fundamental understanding that powered the emergence of medieval science during the renaissance period and laid the foundation of modern science, as we know it today. The word itself has its roots in greek. It is derived from the greek word empeirikos which means “experienced”.
In today’s world, the word empirical refers to collection of data using evidence that is collected through observation or experience or by using calibrated scientific instruments. All of the above origins have one thing in common which is dependence of observation and experiments to collect data and test them to come up with conclusions.
LEARN ABOUT: Causal Research
Types and methodologies of empirical research
Empirical research can be conducted and analysed using qualitative or quantitative methods.
- Quantitative research : Quantitative research methods are used to gather information through numerical data. It is used to quantify opinions, behaviors or other defined variables . These are predetermined and are in a more structured format. Some of the commonly used methods are survey, longitudinal studies, polls, etc
- Qualitative research: Qualitative research methods are used to gather non numerical data. It is used to find meanings, opinions, or the underlying reasons from its subjects. These methods are unstructured or semi structured. The sample size for such a research is usually small and it is a conversational type of method to provide more insight or in-depth information about the problem Some of the most popular forms of methods are focus groups, experiments, interviews, etc.
Data collected from these will need to be analysed. Empirical evidence can also be analysed either quantitatively and qualitatively. Using this, the researcher can answer empirical questions which have to be clearly defined and answerable with the findings he has got. The type of research design used will vary depending on the field in which it is going to be used. Many of them might choose to do a collective research involving quantitative and qualitative method to better answer questions which cannot be studied in a laboratory setting.
LEARN ABOUT: Qualitative Research Questions and Questionnaires
Quantitative research methods aid in analyzing the empirical evidence gathered. By using these a researcher can find out if his hypothesis is supported or not.
- Survey research: Survey research generally involves a large audience to collect a large amount of data. This is a quantitative method having a predetermined set of closed questions which are pretty easy to answer. Because of the simplicity of such a method, high responses are achieved. It is one of the most commonly used methods for all kinds of research in today’s world.
Previously, surveys were taken face to face only with maybe a recorder. However, with advancement in technology and for ease, new mediums such as emails , or social media have emerged.
For example: Depletion of energy resources is a growing concern and hence there is a need for awareness about renewable energy. According to recent studies, fossil fuels still account for around 80% of energy consumption in the United States. Even though there is a rise in the use of green energy every year, there are certain parameters because of which the general population is still not opting for green energy. In order to understand why, a survey can be conducted to gather opinions of the general population about green energy and the factors that influence their choice of switching to renewable energy. Such a survey can help institutions or governing bodies to promote appropriate awareness and incentive schemes to push the use of greener energy.
Learn more: Renewable Energy Survey Template Descriptive Research vs Correlational Research
- Experimental research: In experimental research , an experiment is set up and a hypothesis is tested by creating a situation in which one of the variable is manipulated. This is also used to check cause and effect. It is tested to see what happens to the independent variable if the other one is removed or altered. The process for such a method is usually proposing a hypothesis, experimenting on it, analyzing the findings and reporting the findings to understand if it supports the theory or not.
For example: A particular product company is trying to find what is the reason for them to not be able to capture the market. So the organisation makes changes in each one of the processes like manufacturing, marketing, sales and operations. Through the experiment they understand that sales training directly impacts the market coverage for their product. If the person is trained well, then the product will have better coverage.
- Correlational research: Correlational research is used to find relation between two set of variables . Regression analysis is generally used to predict outcomes of such a method. It can be positive, negative or neutral correlation.
LEARN ABOUT: Level of Analysis
For example: Higher educated individuals will get higher paying jobs. This means higher education enables the individual to high paying job and less education will lead to lower paying jobs.
- Longitudinal study: Longitudinal study is used to understand the traits or behavior of a subject under observation after repeatedly testing the subject over a period of time. Data collected from such a method can be qualitative or quantitative in nature.
For example: A research to find out benefits of exercise. The target is asked to exercise everyday for a particular period of time and the results show higher endurance, stamina, and muscle growth. This supports the fact that exercise benefits an individual body.
- Cross sectional: Cross sectional study is an observational type of method, in which a set of audience is observed at a given point in time. In this type, the set of people are chosen in a fashion which depicts similarity in all the variables except the one which is being researched. This type does not enable the researcher to establish a cause and effect relationship as it is not observed for a continuous time period. It is majorly used by healthcare sector or the retail industry.
For example: A medical study to find the prevalence of under-nutrition disorders in kids of a given population. This will involve looking at a wide range of parameters like age, ethnicity, location, incomes and social backgrounds. If a significant number of kids coming from poor families show under-nutrition disorders, the researcher can further investigate into it. Usually a cross sectional study is followed by a longitudinal study to find out the exact reason.
- Causal-Comparative research : This method is based on comparison. It is mainly used to find out cause-effect relationship between two variables or even multiple variables.
For example: A researcher measured the productivity of employees in a company which gave breaks to the employees during work and compared that to the employees of the company which did not give breaks at all.
LEARN ABOUT: Action Research
Some research questions need to be analysed qualitatively, as quantitative methods are not applicable there. In many cases, in-depth information is needed or a researcher may need to observe a target audience behavior, hence the results needed are in a descriptive analysis form. Qualitative research results will be descriptive rather than predictive. It enables the researcher to build or support theories for future potential quantitative research. In such a situation qualitative research methods are used to derive a conclusion to support the theory or hypothesis being studied.
LEARN ABOUT: Qualitative Interview
- Case study: Case study method is used to find more information through carefully analyzing existing cases. It is very often used for business research or to gather empirical evidence for investigation purpose. It is a method to investigate a problem within its real life context through existing cases. The researcher has to carefully analyse making sure the parameter and variables in the existing case are the same as to the case that is being investigated. Using the findings from the case study, conclusions can be drawn regarding the topic that is being studied.
For example: A report mentioning the solution provided by a company to its client. The challenges they faced during initiation and deployment, the findings of the case and solutions they offered for the problems. Such case studies are used by most companies as it forms an empirical evidence for the company to promote in order to get more business.
- Observational method: Observational method is a process to observe and gather data from its target. Since it is a qualitative method it is time consuming and very personal. It can be said that observational research method is a part of ethnographic research which is also used to gather empirical evidence. This is usually a qualitative form of research, however in some cases it can be quantitative as well depending on what is being studied.
For example: setting up a research to observe a particular animal in the rain-forests of amazon. Such a research usually take a lot of time as observation has to be done for a set amount of time to study patterns or behavior of the subject. Another example used widely nowadays is to observe people shopping in a mall to figure out buying behavior of consumers.
- One-on-one interview: Such a method is purely qualitative and one of the most widely used. The reason being it enables a researcher get precise meaningful data if the right questions are asked. It is a conversational method where in-depth data can be gathered depending on where the conversation leads.
For example: A one-on-one interview with the finance minister to gather data on financial policies of the country and its implications on the public.
- Focus groups: Focus groups are used when a researcher wants to find answers to why, what and how questions. A small group is generally chosen for such a method and it is not necessary to interact with the group in person. A moderator is generally needed in case the group is being addressed in person. This is widely used by product companies to collect data about their brands and the product.
For example: A mobile phone manufacturer wanting to have a feedback on the dimensions of one of their models which is yet to be launched. Such studies help the company meet the demand of the customer and position their model appropriately in the market.
- Text analysis: Text analysis method is a little new compared to the other types. Such a method is used to analyse social life by going through images or words used by the individual. In today’s world, with social media playing a major part of everyone’s life, such a method enables the research to follow the pattern that relates to his study.
For example: A lot of companies ask for feedback from the customer in detail mentioning how satisfied are they with their customer support team. Such data enables the researcher to take appropriate decisions to make their support team better.
Sometimes a combination of the methods is also needed for some questions that cannot be answered using only one type of method especially when a researcher needs to gain a complete understanding of complex subject matter.
We recently published a blog that talks about examples of qualitative data in education ; why don’t you check it out for more ideas?
Since empirical research is based on observation and capturing experiences, it is important to plan the steps to conduct the experiment and how to analyse it. This will enable the researcher to resolve problems or obstacles which can occur during the experiment.
Step #1: Define the purpose of the research
This is the step where the researcher has to answer questions like what exactly do I want to find out? What is the problem statement? Are there any issues in terms of the availability of knowledge, data, time or resources. Will this research be more beneficial than what it will cost.
Before going ahead, a researcher has to clearly define his purpose for the research and set up a plan to carry out further tasks.
Step #2 : Supporting theories and relevant literature
The researcher needs to find out if there are theories which can be linked to his research problem . He has to figure out if any theory can help him support his findings. All kind of relevant literature will help the researcher to find if there are others who have researched this before, or what are the problems faced during this research. The researcher will also have to set up assumptions and also find out if there is any history regarding his research problem
Step #3: Creation of Hypothesis and measurement
Before beginning the actual research he needs to provide himself a working hypothesis or guess what will be the probable result. Researcher has to set up variables, decide the environment for the research and find out how can he relate between the variables.
Researcher will also need to define the units of measurements, tolerable degree for errors, and find out if the measurement chosen will be acceptable by others.
Step #4: Methodology, research design and data collection
In this step, the researcher has to define a strategy for conducting his research. He has to set up experiments to collect data which will enable him to propose the hypothesis. The researcher will decide whether he will need experimental or non experimental method for conducting the research. The type of research design will vary depending on the field in which the research is being conducted. Last but not the least, the researcher will have to find out parameters that will affect the validity of the research design. Data collection will need to be done by choosing appropriate samples depending on the research question. To carry out the research, he can use one of the many sampling techniques. Once data collection is complete, researcher will have empirical data which needs to be analysed.
LEARN ABOUT: Best Data Collection Tools
Step #5: Data Analysis and result
Data analysis can be done in two ways, qualitatively and quantitatively. Researcher will need to find out what qualitative method or quantitative method will be needed or will he need a combination of both. Depending on the unit of analysis of his data, he will know if his hypothesis is supported or rejected. Analyzing this data is the most important part to support his hypothesis.
Step #6: Conclusion
A report will need to be made with the findings of the research. The researcher can give the theories and literature that support his research. He can make suggestions or recommendations for further research on his topic.
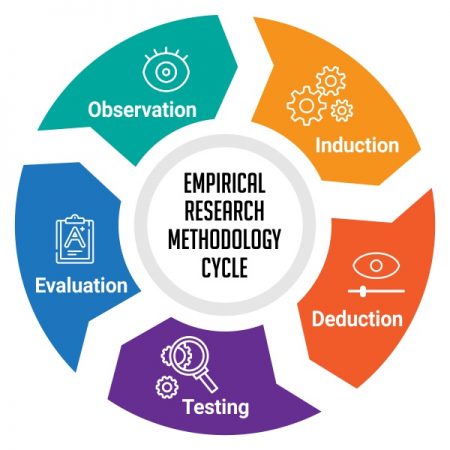
A.D. de Groot, a famous dutch psychologist and a chess expert conducted some of the most notable experiments using chess in the 1940’s. During his study, he came up with a cycle which is consistent and now widely used to conduct empirical research. It consists of 5 phases with each phase being as important as the next one. The empirical cycle captures the process of coming up with hypothesis about how certain subjects work or behave and then testing these hypothesis against empirical data in a systematic and rigorous approach. It can be said that it characterizes the deductive approach to science. Following is the empirical cycle.
- Observation: At this phase an idea is sparked for proposing a hypothesis. During this phase empirical data is gathered using observation. For example: a particular species of flower bloom in a different color only during a specific season.
- Induction: Inductive reasoning is then carried out to form a general conclusion from the data gathered through observation. For example: As stated above it is observed that the species of flower blooms in a different color during a specific season. A researcher may ask a question “does the temperature in the season cause the color change in the flower?” He can assume that is the case, however it is a mere conjecture and hence an experiment needs to be set up to support this hypothesis. So he tags a few set of flowers kept at a different temperature and observes if they still change the color?
- Deduction: This phase helps the researcher to deduce a conclusion out of his experiment. This has to be based on logic and rationality to come up with specific unbiased results.For example: In the experiment, if the tagged flowers in a different temperature environment do not change the color then it can be concluded that temperature plays a role in changing the color of the bloom.
- Testing: This phase involves the researcher to return to empirical methods to put his hypothesis to the test. The researcher now needs to make sense of his data and hence needs to use statistical analysis plans to determine the temperature and bloom color relationship. If the researcher finds out that most flowers bloom a different color when exposed to the certain temperature and the others do not when the temperature is different, he has found support to his hypothesis. Please note this not proof but just a support to his hypothesis.
- Evaluation: This phase is generally forgotten by most but is an important one to keep gaining knowledge. During this phase the researcher puts forth the data he has collected, the support argument and his conclusion. The researcher also states the limitations for the experiment and his hypothesis and suggests tips for others to pick it up and continue a more in-depth research for others in the future. LEARN MORE: Population vs Sample
LEARN MORE: Population vs Sample
There is a reason why empirical research is one of the most widely used method. There are a few advantages associated with it. Following are a few of them.
- It is used to authenticate traditional research through various experiments and observations.
- This research methodology makes the research being conducted more competent and authentic.
- It enables a researcher understand the dynamic changes that can happen and change his strategy accordingly.
- The level of control in such a research is high so the researcher can control multiple variables.
- It plays a vital role in increasing internal validity .
Even though empirical research makes the research more competent and authentic, it does have a few disadvantages. Following are a few of them.
- Such a research needs patience as it can be very time consuming. The researcher has to collect data from multiple sources and the parameters involved are quite a few, which will lead to a time consuming research.
- Most of the time, a researcher will need to conduct research at different locations or in different environments, this can lead to an expensive affair.
- There are a few rules in which experiments can be performed and hence permissions are needed. Many a times, it is very difficult to get certain permissions to carry out different methods of this research.
- Collection of data can be a problem sometimes, as it has to be collected from a variety of sources through different methods.
LEARN ABOUT: Social Communication Questionnaire
Empirical research is important in today’s world because most people believe in something only that they can see, hear or experience. It is used to validate multiple hypothesis and increase human knowledge and continue doing it to keep advancing in various fields.
For example: Pharmaceutical companies use empirical research to try out a specific drug on controlled groups or random groups to study the effect and cause. This way, they prove certain theories they had proposed for the specific drug. Such research is very important as sometimes it can lead to finding a cure for a disease that has existed for many years. It is useful in science and many other fields like history, social sciences, business, etc.
LEARN ABOUT: 12 Best Tools for Researchers
With the advancement in today’s world, empirical research has become critical and a norm in many fields to support their hypothesis and gain more knowledge. The methods mentioned above are very useful for carrying out such research. However, a number of new methods will keep coming up as the nature of new investigative questions keeps getting unique or changing.
Create a single source of real data with a built-for-insights platform. Store past data, add nuggets of insights, and import research data from various sources into a CRM for insights. Build on ever-growing research with a real-time dashboard in a unified research management platform to turn insights into knowledge.
LEARN MORE FREE TRIAL
MORE LIKE THIS
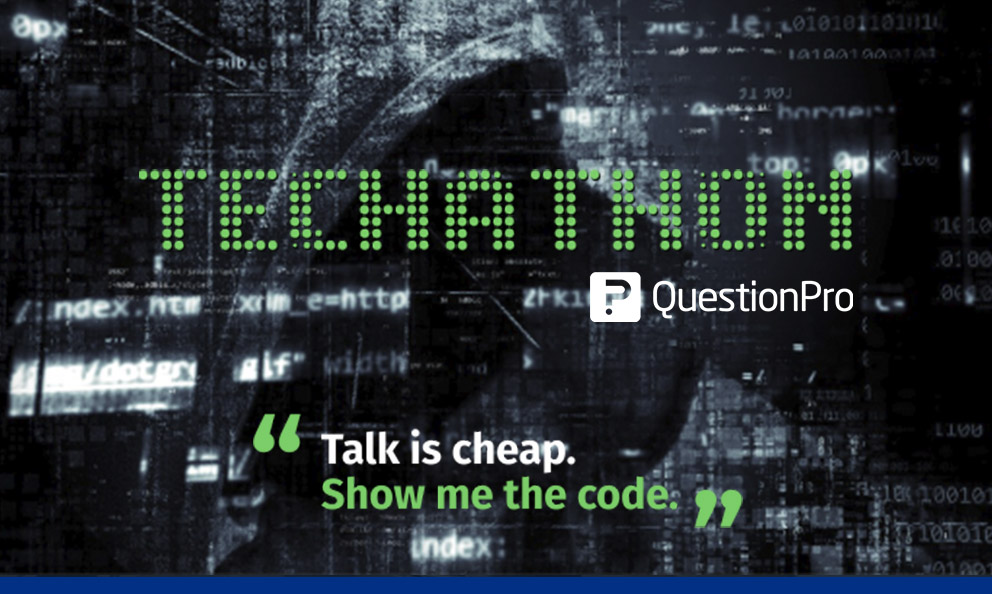
Techathon by QuestionPro: An Amazing Showcase of Tech Brilliance
Jul 3, 2024
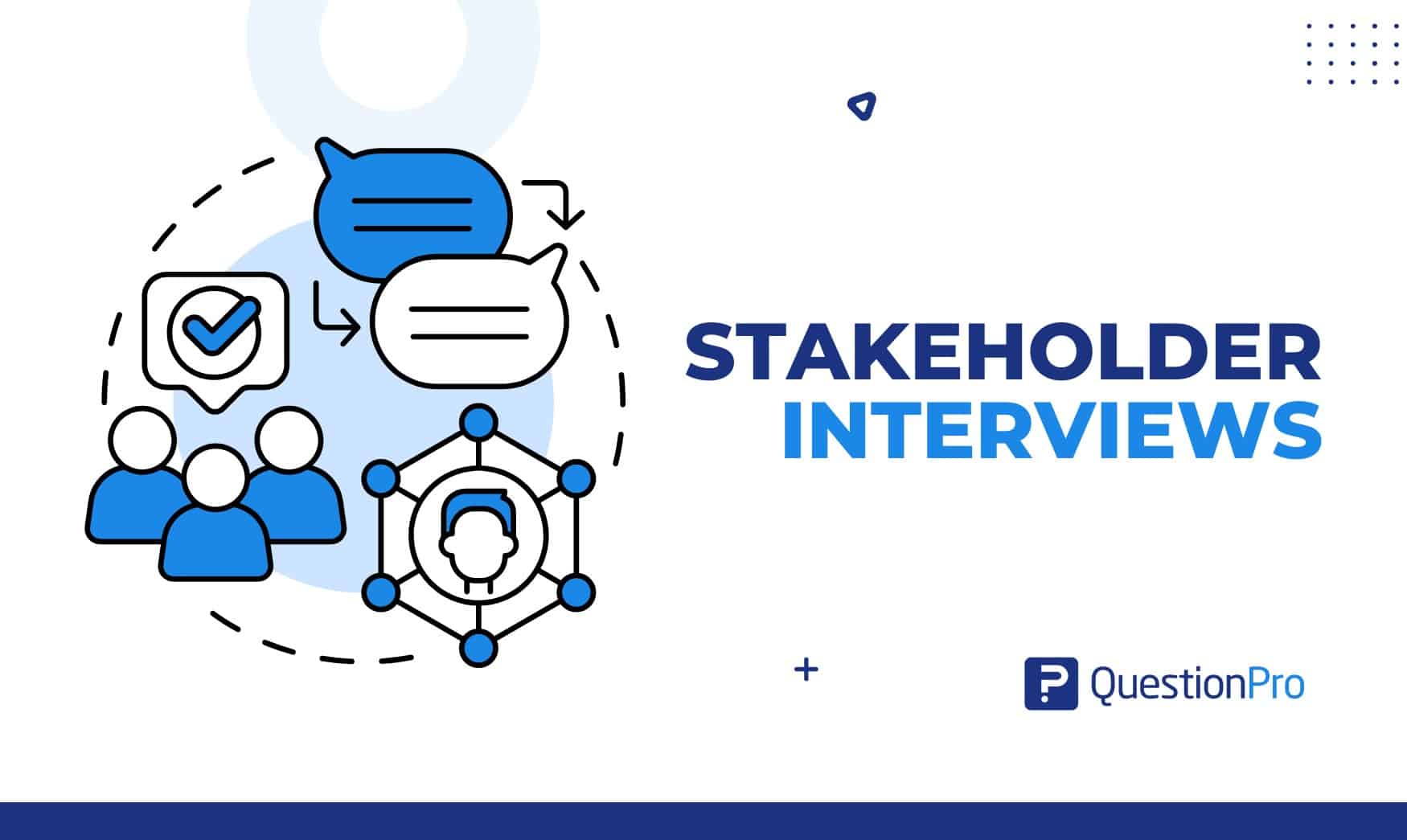
Stakeholder Interviews: A Guide to Effective Engagement
Jul 2, 2024
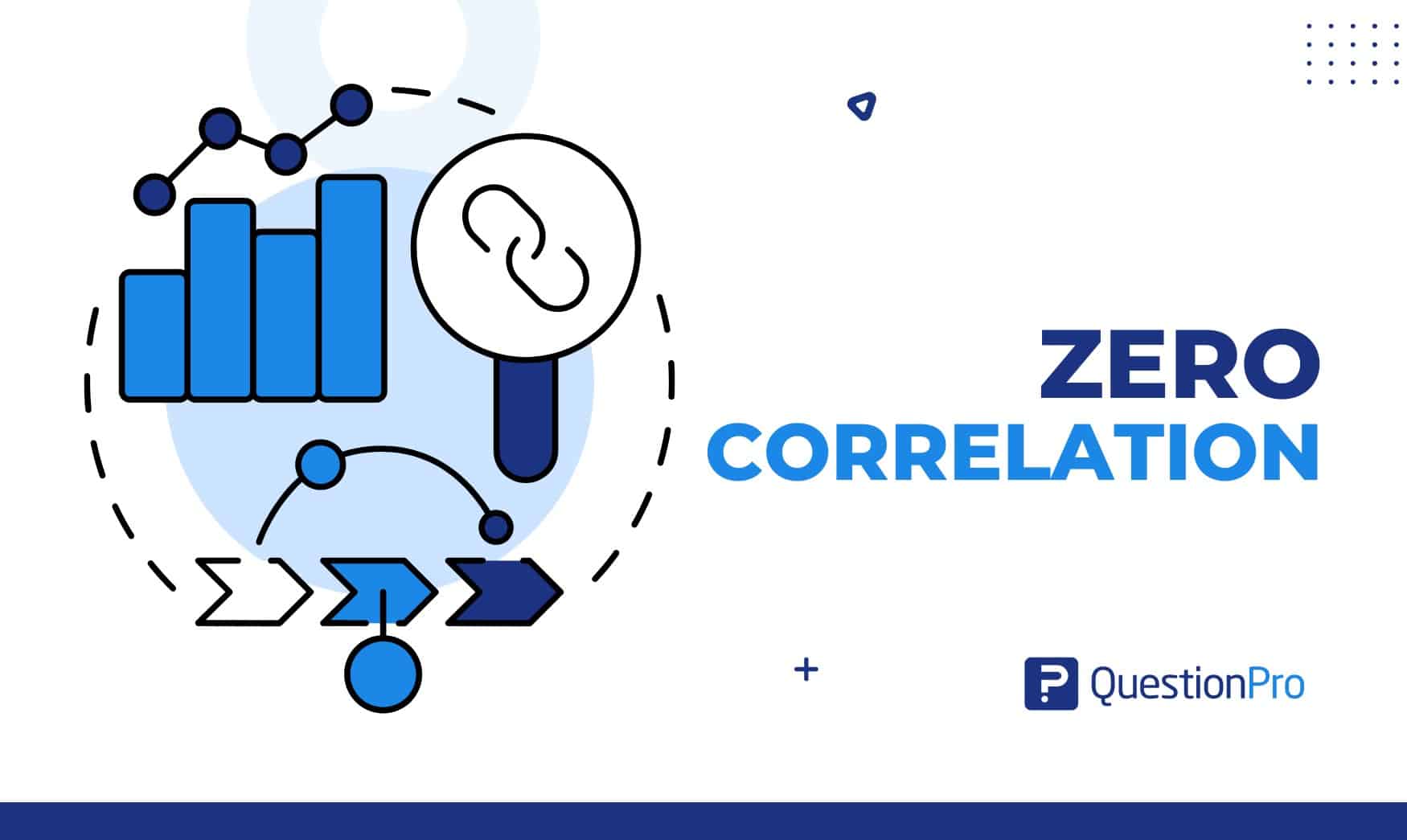
Zero Correlation: Definition, Examples + How to Determine It
Jul 1, 2024
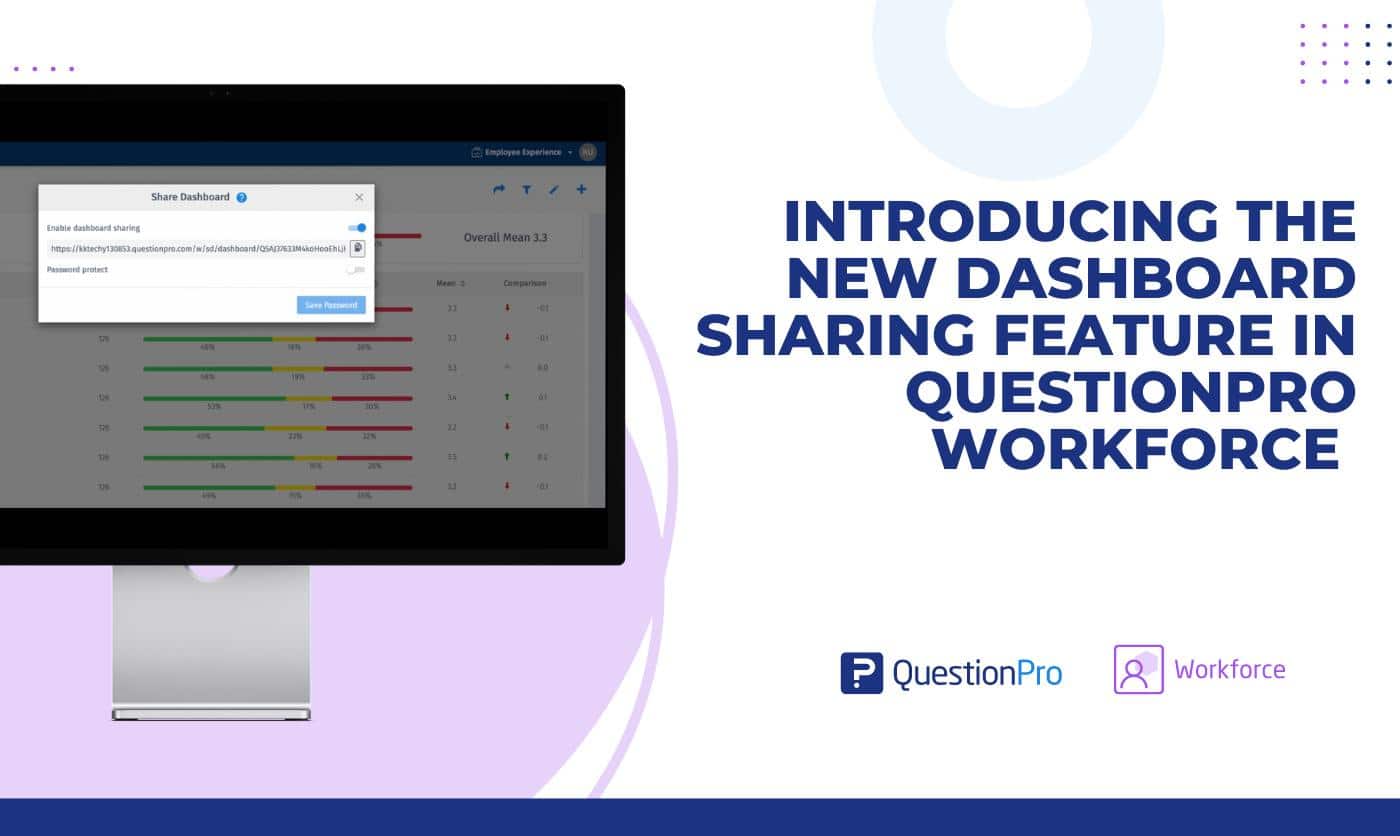
When You Have Something Important to Say, You want to Shout it From the Rooftops
Jun 28, 2024
Other categories
- Academic Research
- Artificial Intelligence
- Assessments
- Brand Awareness
- Case Studies
- Communities
- Consumer Insights
- Customer effort score
- Customer Engagement
- Customer Experience
- Customer Loyalty
- Customer Research
- Customer Satisfaction
- Employee Benefits
- Employee Engagement
- Employee Retention
- Friday Five
- General Data Protection Regulation
- Insights Hub
- Life@QuestionPro
- Market Research
- Mobile diaries
- Mobile Surveys
- New Features
- Online Communities
- Question Types
- Questionnaire
- QuestionPro Products
- Release Notes
- Research Tools and Apps
- Revenue at Risk
- Survey Templates
- Training Tips
- Tuesday CX Thoughts (TCXT)
- Uncategorized
- Video Learning Series
- What’s Coming Up
- Workforce Intelligence

Difference between Theoretical and Empirical Research

The difference between theoretical and empirical research is fundamental to scientific, scholarly research, as it separates the development of ideas and models from their testing and validation.
These two approaches are used in many different fields of inquiry, including the natural sciences, social sciences, and humanities, and they serve different purposes and employ different methods.
Table of Contents
What is theoretical research.
Theoretical research involves the development of models, frameworks, and theories based on existing knowledge, logic, and intuition.
It aims to explain and predict phenomena, generate new ideas and insights, and provide a foundation for further research.
Theoretical research often takes place at the conceptual level and is typically based on existing knowledge, data, and assumptions.
What is Empirical Research?
In contrast, empirical research involves collecting and analysing data to test theories and models.
Empirical research is often conducted at the observational or experimental level and is based on direct or indirect observation of the world.
Empirical research involves testing theories and models, establishing cause-and-effect relationships, and refining or rejecting existing knowledge.
Theoretical vs Empirical Research
Theoretical research is often seen as the starting point for empirical research, providing the ideas and models that must be tested and validated.
Theoretical research can be qualitative or quantitative and involve mathematical models, simulations, and other computational methods.
Theoretical research is often conducted in isolation, without reference to primary data or observations.
On the other hand, empirical research is often seen as the final stage in the scientific process, as it provides evidence that supports or refutes theoretical models.
Empirical research can be qualitative or quantitative, involving surveys, experiments, observational studies, and other data collection methods.
Empirical research is often conducted in collaboration with others and is based on systematic data collection, analysis, and interpretation.
It is important to note that theoretical and empirical research are not mutually exclusive and can often complement each other.
For example, empirical data can inform the development of theories and models, and theoretical models can guide the design of empirical studies.
The most valuable research combines theoretical and empirical approaches in many fields, allowing for a comprehensive understanding of the studied phenomena.
EMPIRICAL RESEARCH | ||
---|---|---|
Purpose | To develop ideas and models based on existing knowledge, logic, and intuition | To test and validate theories and models using data and observations |
Method | Based on existing knowledge, data, and assumptions | Based on direct or indirect observation of the world |
Focus | Conceptual level, explaining and predicting phenomena | Observational or experimental level, testing and establishing cause-and-effect relationships |
Approach | Qualitative or quantitative, often mathematical or computational | Qualitative or quantitative, often involving surveys, experiments, or observational studies |
Data Collection | Often conducted in isolation, without reference to data or observations | Often conducted in collaboration with others, based on systematic data collection, analysis, and interpretation |
It is important to note that this table is not meant to be exhaustive or prescriptive but rather to provide a general overview of the main difference between theoretical and empirical research.
The boundaries between these two approaches are not always clear, and in many cases, research may involve a combination of theoretical and empirical methods.
What are the Limitations of Theoretical Research?
Assumptions and simplifications may be made that do not accurately reflect the complexity of real-world phenomena, which is one of its limitations. Theoretical research relies heavily on logic and deductive reasoning, which can sometimes be biased or limited by the researcher’s assumptions and perspectives.
Furthermore, theoretical research may not be directly applicable to real-world situations without empirical validation. Applying theoretical ideas to practical situations is difficult if no empirical evidence supports or refutes them.
Furthermore, theoretical research may be limited by the availability of data and the researcher’s ability to access and interpret it, which can further limit the validity and applicability of theories.
What are the Limitations of Empirical Research?
There are many limitations to empirical research, including the limitations of the data available and the quality of the data that can be collected. Data collection can be limited by the resources available to collect the data, accessibility to populations or individuals of interest, or ethical constraints.
The researchers or participants may also introduce biases into empirical research, resulting in inaccurate or unreliable findings.
Lastly, due to confounding variables or other methodological limitations, empirical research may be limited by the inability to establish causal relationships between variables, even when statistical associations are identified.
What Methods Are Used In Theoretical Research?
In theoretical research, deductive reasoning, logical analysis, and conceptual frameworks generate new ideas and hypotheses. To identify gaps and inconsistencies in the present understanding of a phenomenon, theoretical research may involve analyzing existing literature and theories.
To test hypotheses and generate predictions, mathematical or computational models may also be developed.
Researchers may also use thought experiments or simulations to explore the implications of their ideas and hypotheses without collecting empirical data as part of theoretical research.
Theoretical research seeks to develop a conceptual framework for empirically testing and validating phenomena.
What Methods Are Used In Empirical Research?
Methods used in empirical research depend on the research questions, type of data collected, and study design. Surveys, experiments, observations, case studies, and interviews are common methods used in empirical research.
An empirical study tests hypotheses and generates new knowledge about phenomena by systematically collecting and analyzing data.
These methods may utilize standardized instruments or protocols for data collection consistency and reliability. Statistical analysis, content analysis, or qualitative analysis may be used for the data collection type.
As a result of empirical research, the findings can inform theories, models, and practical applications.
Conclusion: Theoretical vs Empirical Research
In conclusion, theoretical and empirical research are two distinct but interrelated approaches to scientific inquiry, and they serve different purposes and employ different methods.
Theoretical research involves the development of ideas and models, while empirical research involves testing and validating these ideas.
Both approaches are essential to research and can be combined to provide a more complete understanding of the world.
- Dictionary.com. “ Empirical vs Theoretical “.
- PennState University Libraries. “ Empirical Research in the Social Sciences and Education “.
- William M. Landes and Richard A. Posner. “ Legal Precedent: A Theoretical and Empirical Analysis “, The Journal of Law and Economics, 1976.
Read more articles


- University of Memphis Libraries
- Research Guides
Empirical Research: Defining, Identifying, & Finding
Defining empirical research, what is empirical research, quantitative or qualitative.
- Introduction
- Database Tools
- Search Terms
- Image Descriptions
Calfee & Chambliss (2005) (UofM login required) describe empirical research as a "systematic approach for answering certain types of questions." Those questions are answered "[t]hrough the collection of evidence under carefully defined and replicable conditions" (p. 43).
The evidence collected during empirical research is often referred to as "data."
Characteristics of Empirical Research
Emerald Publishing's guide to conducting empirical research identifies a number of common elements to empirical research:
- A research question , which will determine research objectives.
- A particular and planned design for the research, which will depend on the question and which will find ways of answering it with appropriate use of resources.
- The gathering of primary data , which is then analysed.
- A particular methodology for collecting and analysing the data, such as an experiment or survey.
- The limitation of the data to a particular group, area or time scale, known as a sample [emphasis added]: for example, a specific number of employees of a particular company type, or all users of a library over a given time scale. The sample should be somehow representative of a wider population.
- The ability to recreate the study and test the results. This is known as reliability .
- The ability to generalize from the findings to a larger sample and to other situations.
If you see these elements in a research article, you can feel confident that you have found empirical research. Emerald's guide goes into more detail on each element.
Empirical research methodologies can be described as quantitative, qualitative, or a mix of both (usually called mixed-methods).
Ruane (2016) (UofM login required) gets at the basic differences in approach between quantitative and qualitative research:
- Quantitative research -- an approach to documenting reality that relies heavily on numbers both for the measurement of variables and for data analysis (p. 33).
- Qualitative research -- an approach to documenting reality that relies on words and images as the primary data source (p. 33).
Both quantitative and qualitative methods are empirical . If you can recognize that a research study is quantitative or qualitative study, then you have also recognized that it is empirical study.
Below are information on the characteristics of quantitative and qualitative research. This video from Scribbr also offers a good overall introduction to the two approaches to research methodology:
Characteristics of Quantitative Research
Researchers test hypotheses, or theories, based in assumptions about causality, i.e. we expect variable X to cause variable Y. Variables have to be controlled as much as possible to ensure validity. The results explain the relationship between the variables. Measures are based in pre-defined instruments.
Examples: experimental or quasi-experimental design, pretest & post-test, survey or questionnaire with closed-ended questions. Studies that identify factors that influence an outcomes, the utility of an intervention, or understanding predictors of outcomes.
Characteristics of Qualitative Research
Researchers explore “meaning individuals or groups ascribe to social or human problems (Creswell & Creswell, 2018, p3).” Questions and procedures emerge rather than being prescribed. Complexity, nuance, and individual meaning are valued. Research is both inductive and deductive. Data sources are multiple and varied, i.e. interviews, observations, documents, photographs, etc. The researcher is a key instrument and must be reflective of their background, culture, and experiences as influential of the research.
Examples: open question interviews and surveys, focus groups, case studies, grounded theory, ethnography, discourse analysis, narrative, phenomenology, participatory action research.
Calfee, R. C. & Chambliss, M. (2005). The design of empirical research. In J. Flood, D. Lapp, J. R. Squire, & J. Jensen (Eds.), Methods of research on teaching the English language arts: The methodology chapters from the handbook of research on teaching the English language arts (pp. 43-78). Routledge. http://ezproxy.memphis.edu/login?url=http://search.ebscohost.com/login.aspx?direct=true&db=nlebk&AN=125955&site=eds-live&scope=site .
Creswell, J. W., & Creswell, J. D. (2018). Research design: Qualitative, quantitative, and mixed methods approaches (5th ed.). Thousand Oaks: Sage.
How to... conduct empirical research . (n.d.). Emerald Publishing. https://www.emeraldgrouppublishing.com/how-to/research-methods/conduct-empirical-research .
Scribbr. (2019). Quantitative vs. qualitative: The differences explained [video]. YouTube. https://www.youtube.com/watch?v=a-XtVF7Bofg .
Ruane, J. M. (2016). Introducing social research methods : Essentials for getting the edge . Wiley-Blackwell. http://ezproxy.memphis.edu/login?url=http://search.ebscohost.com/login.aspx?direct=true&db=nlebk&AN=1107215&site=eds-live&scope=site .
- << Previous: Home
- Next: Identifying Empirical Research >>
- Last Updated: Apr 2, 2024 11:25 AM
- URL: https://libguides.memphis.edu/empirical-research
What is Empirical Research? Definition, Methods, Examples
Appinio Research · 09.02.2024 · 36min read
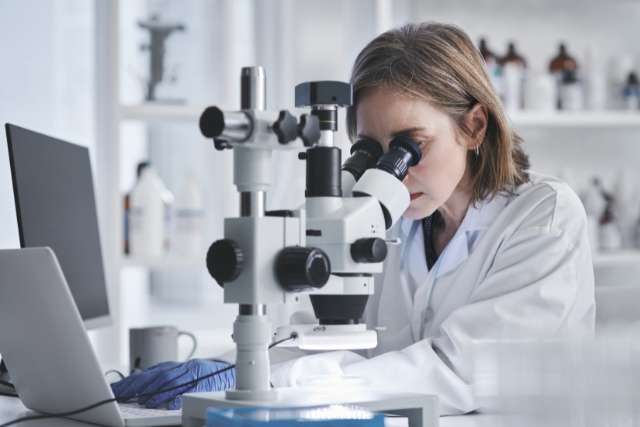
Ever wondered how we gather the facts, unveil hidden truths, and make informed decisions in a world filled with questions? Empirical research holds the key.
In this guide, we'll delve deep into the art and science of empirical research, unraveling its methods, mysteries, and manifold applications. From defining the core principles to mastering data analysis and reporting findings, we're here to equip you with the knowledge and tools to navigate the empirical landscape.
What is Empirical Research?
Empirical research is the cornerstone of scientific inquiry, providing a systematic and structured approach to investigating the world around us. It is the process of gathering and analyzing empirical or observable data to test hypotheses, answer research questions, or gain insights into various phenomena. This form of research relies on evidence derived from direct observation or experimentation, allowing researchers to draw conclusions based on real-world data rather than purely theoretical or speculative reasoning.
Characteristics of Empirical Research
Empirical research is characterized by several key features:
- Observation and Measurement : It involves the systematic observation or measurement of variables, events, or behaviors.
- Data Collection : Researchers collect data through various methods, such as surveys, experiments, observations, or interviews.
- Testable Hypotheses : Empirical research often starts with testable hypotheses that are evaluated using collected data.
- Quantitative or Qualitative Data : Data can be quantitative (numerical) or qualitative (non-numerical), depending on the research design.
- Statistical Analysis : Quantitative data often undergo statistical analysis to determine patterns , relationships, or significance.
- Objectivity and Replicability : Empirical research strives for objectivity, minimizing researcher bias . It should be replicable, allowing other researchers to conduct the same study to verify results.
- Conclusions and Generalizations : Empirical research generates findings based on data and aims to make generalizations about larger populations or phenomena.
Importance of Empirical Research
Empirical research plays a pivotal role in advancing knowledge across various disciplines. Its importance extends to academia, industry, and society as a whole. Here are several reasons why empirical research is essential:
- Evidence-Based Knowledge : Empirical research provides a solid foundation of evidence-based knowledge. It enables us to test hypotheses, confirm or refute theories, and build a robust understanding of the world.
- Scientific Progress : In the scientific community, empirical research fuels progress by expanding the boundaries of existing knowledge. It contributes to the development of theories and the formulation of new research questions.
- Problem Solving : Empirical research is instrumental in addressing real-world problems and challenges. It offers insights and data-driven solutions to complex issues in fields like healthcare, economics, and environmental science.
- Informed Decision-Making : In policymaking, business, and healthcare, empirical research informs decision-makers by providing data-driven insights. It guides strategies, investments, and policies for optimal outcomes.
- Quality Assurance : Empirical research is essential for quality assurance and validation in various industries, including pharmaceuticals, manufacturing, and technology. It ensures that products and processes meet established standards.
- Continuous Improvement : Businesses and organizations use empirical research to evaluate performance, customer satisfaction, and product effectiveness. This data-driven approach fosters continuous improvement and innovation.
- Human Advancement : Empirical research in fields like medicine and psychology contributes to the betterment of human health and well-being. It leads to medical breakthroughs, improved therapies, and enhanced psychological interventions.
- Critical Thinking and Problem Solving : Engaging in empirical research fosters critical thinking skills, problem-solving abilities, and a deep appreciation for evidence-based decision-making.
Empirical research empowers us to explore, understand, and improve the world around us. It forms the bedrock of scientific inquiry and drives progress in countless domains, shaping our understanding of both the natural and social sciences.
How to Conduct Empirical Research?
So, you've decided to dive into the world of empirical research. Let's begin by exploring the crucial steps involved in getting started with your research project.
1. Select a Research Topic
Selecting the right research topic is the cornerstone of a successful empirical study. It's essential to choose a topic that not only piques your interest but also aligns with your research goals and objectives. Here's how to go about it:
- Identify Your Interests : Start by reflecting on your passions and interests. What topics fascinate you the most? Your enthusiasm will be your driving force throughout the research process.
- Brainstorm Ideas : Engage in brainstorming sessions to generate potential research topics. Consider the questions you've always wanted to answer or the issues that intrigue you.
- Relevance and Significance : Assess the relevance and significance of your chosen topic. Does it contribute to existing knowledge? Is it a pressing issue in your field of study or the broader community?
- Feasibility : Evaluate the feasibility of your research topic. Do you have access to the necessary resources, data, and participants (if applicable)?
2. Formulate Research Questions
Once you've narrowed down your research topic, the next step is to formulate clear and precise research questions . These questions will guide your entire research process and shape your study's direction. To create effective research questions:
- Specificity : Ensure that your research questions are specific and focused. Vague or overly broad questions can lead to inconclusive results.
- Relevance : Your research questions should directly relate to your chosen topic. They should address gaps in knowledge or contribute to solving a particular problem.
- Testability : Ensure that your questions are testable through empirical methods. You should be able to gather data and analyze it to answer these questions.
- Avoid Bias : Craft your questions in a way that avoids leading or biased language. Maintain neutrality to uphold the integrity of your research.
3. Review Existing Literature
Before you embark on your empirical research journey, it's essential to immerse yourself in the existing body of literature related to your chosen topic. This step, often referred to as a literature review, serves several purposes:
- Contextualization : Understand the historical context and current state of research in your field. What have previous studies found, and what questions remain unanswered?
- Identifying Gaps : Identify gaps or areas where existing research falls short. These gaps will help you formulate meaningful research questions and hypotheses.
- Theory Development : If your study is theoretical, consider how existing theories apply to your topic. If it's empirical, understand how previous studies have approached data collection and analysis.
- Methodological Insights : Learn from the methodologies employed in previous research. What methods were successful, and what challenges did researchers face?
4. Define Variables
Variables are fundamental components of empirical research. They are the factors or characteristics that can change or be manipulated during your study. Properly defining and categorizing variables is crucial for the clarity and validity of your research. Here's what you need to know:
- Independent Variables : These are the variables that you, as the researcher, manipulate or control. They are the "cause" in cause-and-effect relationships.
- Dependent Variables : Dependent variables are the outcomes or responses that you measure or observe. They are the "effect" influenced by changes in independent variables.
- Operational Definitions : To ensure consistency and clarity, provide operational definitions for your variables. Specify how you will measure or manipulate each variable.
- Control Variables : In some studies, controlling for other variables that may influence your dependent variable is essential. These are known as control variables.
Understanding these foundational aspects of empirical research will set a solid foundation for the rest of your journey. Now that you've grasped the essentials of getting started, let's delve deeper into the intricacies of research design.
Empirical Research Design
Now that you've selected your research topic, formulated research questions, and defined your variables, it's time to delve into the heart of your empirical research journey – research design . This pivotal step determines how you will collect data and what methods you'll employ to answer your research questions. Let's explore the various facets of research design in detail.
Types of Empirical Research
Empirical research can take on several forms, each with its own unique approach and methodologies. Understanding the different types of empirical research will help you choose the most suitable design for your study. Here are some common types:
- Experimental Research : In this type, researchers manipulate one or more independent variables to observe their impact on dependent variables. It's highly controlled and often conducted in a laboratory setting.
- Observational Research : Observational research involves the systematic observation of subjects or phenomena without intervention. Researchers are passive observers, documenting behaviors, events, or patterns.
- Survey Research : Surveys are used to collect data through structured questionnaires or interviews. This method is efficient for gathering information from a large number of participants.
- Case Study Research : Case studies focus on in-depth exploration of one or a few cases. Researchers gather detailed information through various sources such as interviews, documents, and observations.
- Qualitative Research : Qualitative research aims to understand behaviors, experiences, and opinions in depth. It often involves open-ended questions, interviews, and thematic analysis.
- Quantitative Research : Quantitative research collects numerical data and relies on statistical analysis to draw conclusions. It involves structured questionnaires, experiments, and surveys.
Your choice of research type should align with your research questions and objectives. Experimental research, for example, is ideal for testing cause-and-effect relationships, while qualitative research is more suitable for exploring complex phenomena.
Experimental Design
Experimental research is a systematic approach to studying causal relationships. It's characterized by the manipulation of one or more independent variables while controlling for other factors. Here are some key aspects of experimental design:
- Control and Experimental Groups : Participants are randomly assigned to either a control group or an experimental group. The independent variable is manipulated for the experimental group but not for the control group.
- Randomization : Randomization is crucial to eliminate bias in group assignment. It ensures that each participant has an equal chance of being in either group.
- Hypothesis Testing : Experimental research often involves hypothesis testing. Researchers formulate hypotheses about the expected effects of the independent variable and use statistical analysis to test these hypotheses.
Observational Design
Observational research entails careful and systematic observation of subjects or phenomena. It's advantageous when you want to understand natural behaviors or events. Key aspects of observational design include:
- Participant Observation : Researchers immerse themselves in the environment they are studying. They become part of the group being observed, allowing for a deep understanding of behaviors.
- Non-Participant Observation : In non-participant observation, researchers remain separate from the subjects. They observe and document behaviors without direct involvement.
- Data Collection Methods : Observational research can involve various data collection methods, such as field notes, video recordings, photographs, or coding of observed behaviors.
Survey Design
Surveys are a popular choice for collecting data from a large number of participants. Effective survey design is essential to ensure the validity and reliability of your data. Consider the following:
- Questionnaire Design : Create clear and concise questions that are easy for participants to understand. Avoid leading or biased questions.
- Sampling Methods : Decide on the appropriate sampling method for your study, whether it's random, stratified, or convenience sampling.
- Data Collection Tools : Choose the right tools for data collection, whether it's paper surveys, online questionnaires, or face-to-face interviews.
Case Study Design
Case studies are an in-depth exploration of one or a few cases to gain a deep understanding of a particular phenomenon. Key aspects of case study design include:
- Single Case vs. Multiple Case Studies : Decide whether you'll focus on a single case or multiple cases. Single case studies are intensive and allow for detailed examination, while multiple case studies provide comparative insights.
- Data Collection Methods : Gather data through interviews, observations, document analysis, or a combination of these methods.
Qualitative vs. Quantitative Research
In empirical research, you'll often encounter the distinction between qualitative and quantitative research . Here's a closer look at these two approaches:
- Qualitative Research : Qualitative research seeks an in-depth understanding of human behavior, experiences, and perspectives. It involves open-ended questions, interviews, and the analysis of textual or narrative data. Qualitative research is exploratory and often used when the research question is complex and requires a nuanced understanding.
- Quantitative Research : Quantitative research collects numerical data and employs statistical analysis to draw conclusions. It involves structured questionnaires, experiments, and surveys. Quantitative research is ideal for testing hypotheses and establishing cause-and-effect relationships.
Understanding the various research design options is crucial in determining the most appropriate approach for your study. Your choice should align with your research questions, objectives, and the nature of the phenomenon you're investigating.
Data Collection for Empirical Research
Now that you've established your research design, it's time to roll up your sleeves and collect the data that will fuel your empirical research. Effective data collection is essential for obtaining accurate and reliable results.
Sampling Methods
Sampling methods are critical in empirical research, as they determine the subset of individuals or elements from your target population that you will study. Here are some standard sampling methods:
- Random Sampling : Random sampling ensures that every member of the population has an equal chance of being selected. It minimizes bias and is often used in quantitative research.
- Stratified Sampling : Stratified sampling involves dividing the population into subgroups or strata based on specific characteristics (e.g., age, gender, location). Samples are then randomly selected from each stratum, ensuring representation of all subgroups.
- Convenience Sampling : Convenience sampling involves selecting participants who are readily available or easily accessible. While it's convenient, it may introduce bias and limit the generalizability of results.
- Snowball Sampling : Snowball sampling is instrumental when studying hard-to-reach or hidden populations. One participant leads you to another, creating a "snowball" effect. This method is common in qualitative research.
- Purposive Sampling : In purposive sampling, researchers deliberately select participants who meet specific criteria relevant to their research questions. It's often used in qualitative studies to gather in-depth information.
The choice of sampling method depends on the nature of your research, available resources, and the degree of precision required. It's crucial to carefully consider your sampling strategy to ensure that your sample accurately represents your target population.
Data Collection Instruments
Data collection instruments are the tools you use to gather information from your participants or sources. These instruments should be designed to capture the data you need accurately. Here are some popular data collection instruments:
- Questionnaires : Questionnaires consist of structured questions with predefined response options. When designing questionnaires, consider the clarity of questions, the order of questions, and the response format (e.g., Likert scale , multiple-choice).
- Interviews : Interviews involve direct communication between the researcher and participants. They can be structured (with predetermined questions) or unstructured (open-ended). Effective interviews require active listening and probing for deeper insights.
- Observations : Observations entail systematically and objectively recording behaviors, events, or phenomena. Researchers must establish clear criteria for what to observe, how to record observations, and when to observe.
- Surveys : Surveys are a common data collection instrument for quantitative research. They can be administered through various means, including online surveys, paper surveys, and telephone surveys.
- Documents and Archives : In some cases, data may be collected from existing documents, records, or archives. Ensure that the sources are reliable, relevant, and properly documented.
To streamline your process and gather insights with precision and efficiency, consider leveraging innovative tools like Appinio . With Appinio's intuitive platform, you can harness the power of real-time consumer data to inform your research decisions effectively. Whether you're conducting surveys, interviews, or observations, Appinio empowers you to define your target audience, collect data from diverse demographics, and analyze results seamlessly.
By incorporating Appinio into your data collection toolkit, you can unlock a world of possibilities and elevate the impact of your empirical research. Ready to revolutionize your approach to data collection?
Book a Demo
Data Collection Procedures
Data collection procedures outline the step-by-step process for gathering data. These procedures should be meticulously planned and executed to maintain the integrity of your research.
- Training : If you have a research team, ensure that they are trained in data collection methods and protocols. Consistency in data collection is crucial.
- Pilot Testing : Before launching your data collection, conduct a pilot test with a small group to identify any potential problems with your instruments or procedures. Make necessary adjustments based on feedback.
- Data Recording : Establish a systematic method for recording data. This may include timestamps, codes, or identifiers for each data point.
- Data Security : Safeguard the confidentiality and security of collected data. Ensure that only authorized individuals have access to the data.
- Data Storage : Properly organize and store your data in a secure location, whether in physical or digital form. Back up data to prevent loss.
Ethical Considerations
Ethical considerations are paramount in empirical research, as they ensure the well-being and rights of participants are protected.
- Informed Consent : Obtain informed consent from participants, providing clear information about the research purpose, procedures, risks, and their right to withdraw at any time.
- Privacy and Confidentiality : Protect the privacy and confidentiality of participants. Ensure that data is anonymized and sensitive information is kept confidential.
- Beneficence : Ensure that your research benefits participants and society while minimizing harm. Consider the potential risks and benefits of your study.
- Honesty and Integrity : Conduct research with honesty and integrity. Report findings accurately and transparently, even if they are not what you expected.
- Respect for Participants : Treat participants with respect, dignity, and sensitivity to cultural differences. Avoid any form of coercion or manipulation.
- Institutional Review Board (IRB) : If required, seek approval from an IRB or ethics committee before conducting your research, particularly when working with human participants.
Adhering to ethical guidelines is not only essential for the ethical conduct of research but also crucial for the credibility and validity of your study. Ethical research practices build trust between researchers and participants and contribute to the advancement of knowledge with integrity.
With a solid understanding of data collection, including sampling methods, instruments, procedures, and ethical considerations, you are now well-equipped to gather the data needed to answer your research questions.
Empirical Research Data Analysis
Now comes the exciting phase of data analysis, where the raw data you've diligently collected starts to yield insights and answers to your research questions. We will explore the various aspects of data analysis, from preparing your data to drawing meaningful conclusions through statistics and visualization.
Data Preparation
Data preparation is the crucial first step in data analysis. It involves cleaning, organizing, and transforming your raw data into a format that is ready for analysis. Effective data preparation ensures the accuracy and reliability of your results.
- Data Cleaning : Identify and rectify errors, missing values, and inconsistencies in your dataset. This may involve correcting typos, removing outliers, and imputing missing data.
- Data Coding : Assign numerical values or codes to categorical variables to make them suitable for statistical analysis. For example, converting "Yes" and "No" to 1 and 0.
- Data Transformation : Transform variables as needed to meet the assumptions of the statistical tests you plan to use. Common transformations include logarithmic or square root transformations.
- Data Integration : If your data comes from multiple sources, integrate it into a unified dataset, ensuring that variables match and align.
- Data Documentation : Maintain clear documentation of all data preparation steps, as well as the rationale behind each decision. This transparency is essential for replicability.
Effective data preparation lays the foundation for accurate and meaningful analysis. It allows you to trust the results that will follow in the subsequent stages.
Descriptive Statistics
Descriptive statistics help you summarize and make sense of your data by providing a clear overview of its key characteristics. These statistics are essential for understanding the central tendencies, variability, and distribution of your variables. Descriptive statistics include:
- Measures of Central Tendency : These include the mean (average), median (middle value), and mode (most frequent value). They help you understand the typical or central value of your data.
- Measures of Dispersion : Measures like the range, variance, and standard deviation provide insights into the spread or variability of your data points.
- Frequency Distributions : Creating frequency distributions or histograms allows you to visualize the distribution of your data across different values or categories.
Descriptive statistics provide the initial insights needed to understand your data's basic characteristics, which can inform further analysis.
Inferential Statistics
Inferential statistics take your analysis to the next level by allowing you to make inferences or predictions about a larger population based on your sample data. These methods help you test hypotheses and draw meaningful conclusions. Key concepts in inferential statistics include:
- Hypothesis Testing : Hypothesis tests (e.g., t-tests , chi-squared tests ) help you determine whether observed differences or associations in your data are statistically significant or occurred by chance.
- Confidence Intervals : Confidence intervals provide a range within which population parameters (e.g., population mean) are likely to fall based on your sample data.
- Regression Analysis : Regression models (linear, logistic, etc.) help you explore relationships between variables and make predictions.
- Analysis of Variance (ANOVA) : ANOVA tests are used to compare means between multiple groups, allowing you to assess whether differences are statistically significant.
Chi-Square Calculator :
t-Test Calculator :
One-way ANOVA Calculator :
Inferential statistics are powerful tools for drawing conclusions from your data and assessing the generalizability of your findings to the broader population.
Qualitative Data Analysis
Qualitative data analysis is employed when working with non-numerical data, such as text, interviews, or open-ended survey responses. It focuses on understanding the underlying themes, patterns, and meanings within qualitative data. Qualitative analysis techniques include:
- Thematic Analysis : Identifying and analyzing recurring themes or patterns within textual data.
- Content Analysis : Categorizing and coding qualitative data to extract meaningful insights.
- Grounded Theory : Developing theories or frameworks based on emergent themes from the data.
- Narrative Analysis : Examining the structure and content of narratives to uncover meaning.
Qualitative data analysis provides a rich and nuanced understanding of complex phenomena and human experiences.
Data Visualization
Data visualization is the art of representing data graphically to make complex information more understandable and accessible. Effective data visualization can reveal patterns, trends, and outliers in your data. Common types of data visualization include:
- Bar Charts and Histograms : Used to display the distribution of categorical data or discrete data .
- Line Charts : Ideal for showing trends and changes in data over time.
- Scatter Plots : Visualize relationships and correlations between two variables.
- Pie Charts : Display the composition of a whole in terms of its parts.
- Heatmaps : Depict patterns and relationships in multidimensional data through color-coding.
- Box Plots : Provide a summary of the data distribution, including outliers.
- Interactive Dashboards : Create dynamic visualizations that allow users to explore data interactively.
Data visualization not only enhances your understanding of the data but also serves as a powerful communication tool to convey your findings to others.
As you embark on the data analysis phase of your empirical research, remember that the specific methods and techniques you choose will depend on your research questions, data type, and objectives. Effective data analysis transforms raw data into valuable insights, bringing you closer to the answers you seek.
How to Report Empirical Research Results?
At this stage, you get to share your empirical research findings with the world. Effective reporting and presentation of your results are crucial for communicating your research's impact and insights.
1. Write the Research Paper
Writing a research paper is the culmination of your empirical research journey. It's where you synthesize your findings, provide context, and contribute to the body of knowledge in your field.
- Title and Abstract : Craft a clear and concise title that reflects your research's essence. The abstract should provide a brief summary of your research objectives, methods, findings, and implications.
- Introduction : In the introduction, introduce your research topic, state your research questions or hypotheses, and explain the significance of your study. Provide context by discussing relevant literature.
- Methods : Describe your research design, data collection methods, and sampling procedures. Be precise and transparent, allowing readers to understand how you conducted your study.
- Results : Present your findings in a clear and organized manner. Use tables, graphs, and statistical analyses to support your results. Avoid interpreting your findings in this section; focus on the presentation of raw data.
- Discussion : Interpret your findings and discuss their implications. Relate your results to your research questions and the existing literature. Address any limitations of your study and suggest avenues for future research.
- Conclusion : Summarize the key points of your research and its significance. Restate your main findings and their implications.
- References : Cite all sources used in your research following a specific citation style (e.g., APA, MLA, Chicago). Ensure accuracy and consistency in your citations.
- Appendices : Include any supplementary material, such as questionnaires, data coding sheets, or additional analyses, in the appendices.
Writing a research paper is a skill that improves with practice. Ensure clarity, coherence, and conciseness in your writing to make your research accessible to a broader audience.
2. Create Visuals and Tables
Visuals and tables are powerful tools for presenting complex data in an accessible and understandable manner.
- Clarity : Ensure that your visuals and tables are clear and easy to interpret. Use descriptive titles and labels.
- Consistency : Maintain consistency in formatting, such as font size and style, across all visuals and tables.
- Appropriateness : Choose the most suitable visual representation for your data. Bar charts, line graphs, and scatter plots work well for different types of data.
- Simplicity : Avoid clutter and unnecessary details. Focus on conveying the main points.
- Accessibility : Make sure your visuals and tables are accessible to a broad audience, including those with visual impairments.
- Captions : Include informative captions that explain the significance of each visual or table.
Compelling visuals and tables enhance the reader's understanding of your research and can be the key to conveying complex information efficiently.
3. Interpret Findings
Interpreting your findings is where you bridge the gap between data and meaning. It's your opportunity to provide context, discuss implications, and offer insights. When interpreting your findings:
- Relate to Research Questions : Discuss how your findings directly address your research questions or hypotheses.
- Compare with Literature : Analyze how your results align with or deviate from previous research in your field. What insights can you draw from these comparisons?
- Discuss Limitations : Be transparent about the limitations of your study. Address any constraints, biases, or potential sources of error.
- Practical Implications : Explore the real-world implications of your findings. How can they be applied or inform decision-making?
- Future Research Directions : Suggest areas for future research based on the gaps or unanswered questions that emerged from your study.
Interpreting findings goes beyond simply presenting data; it's about weaving a narrative that helps readers grasp the significance of your research in the broader context.
With your research paper written, structured, and enriched with visuals, and your findings expertly interpreted, you are now prepared to communicate your research effectively. Sharing your insights and contributing to the body of knowledge in your field is a significant accomplishment in empirical research.
Examples of Empirical Research
To solidify your understanding of empirical research, let's delve into some real-world examples across different fields. These examples will illustrate how empirical research is applied to gather data, analyze findings, and draw conclusions.
Social Sciences
In the realm of social sciences, consider a sociological study exploring the impact of socioeconomic status on educational attainment. Researchers gather data from a diverse group of individuals, including their family backgrounds, income levels, and academic achievements.
Through statistical analysis, they can identify correlations and trends, revealing whether individuals from lower socioeconomic backgrounds are less likely to attain higher levels of education. This empirical research helps shed light on societal inequalities and informs policymakers on potential interventions to address disparities in educational access.
Environmental Science
Environmental scientists often employ empirical research to assess the effects of environmental changes. For instance, researchers studying the impact of climate change on wildlife might collect data on animal populations, weather patterns, and habitat conditions over an extended period.
By analyzing this empirical data, they can identify correlations between climate fluctuations and changes in wildlife behavior, migration patterns, or population sizes. This empirical research is crucial for understanding the ecological consequences of climate change and informing conservation efforts.
Business and Economics
In the business world, empirical research is essential for making data-driven decisions. Consider a market research study conducted by a business seeking to launch a new product. They collect data through surveys , focus groups , and consumer behavior analysis.
By examining this empirical data, the company can gauge consumer preferences, demand, and potential market size. Empirical research in business helps guide product development, pricing strategies, and marketing campaigns, increasing the likelihood of a successful product launch.
Psychological studies frequently rely on empirical research to understand human behavior and cognition. For instance, a psychologist interested in examining the impact of stress on memory might design an experiment. Participants are exposed to stress-inducing situations, and their memory performance is assessed through various tasks.
By analyzing the data collected, the psychologist can determine whether stress has a significant effect on memory recall. This empirical research contributes to our understanding of the complex interplay between psychological factors and cognitive processes.
These examples highlight the versatility and applicability of empirical research across diverse fields. Whether in medicine, social sciences, environmental science, business, or psychology, empirical research serves as a fundamental tool for gaining insights, testing hypotheses, and driving advancements in knowledge and practice.
Conclusion for Empirical Research
Empirical research is a powerful tool for gaining insights, testing hypotheses, and making informed decisions. By following the steps outlined in this guide, you've learned how to select research topics, collect data, analyze findings, and effectively communicate your research to the world. Remember, empirical research is a journey of discovery, and each step you take brings you closer to a deeper understanding of the world around you. Whether you're a scientist, a student, or someone curious about the process, the principles of empirical research empower you to explore, learn, and contribute to the ever-expanding realm of knowledge.
How to Collect Data for Empirical Research?
Introducing Appinio , the real-time market research platform revolutionizing how companies gather consumer insights for their empirical research endeavors. With Appinio, you can conduct your own market research in minutes, gaining valuable data to fuel your data-driven decisions.
Appinio is more than just a market research platform; it's a catalyst for transforming the way you approach empirical research, making it exciting, intuitive, and seamlessly integrated into your decision-making process.
Here's why Appinio is the go-to solution for empirical research:
- From Questions to Insights in Minutes : With Appinio's streamlined process, you can go from formulating your research questions to obtaining actionable insights in a matter of minutes, saving you time and effort.
- Intuitive Platform for Everyone : No need for a PhD in research; Appinio's platform is designed to be intuitive and user-friendly, ensuring that anyone can navigate and utilize it effectively.
- Rapid Response Times : With an average field time of under 23 minutes for 1,000 respondents, Appinio delivers rapid results, allowing you to gather data swiftly and efficiently.
- Global Reach with Targeted Precision : With access to over 90 countries and the ability to define target groups based on 1200+ characteristics, Appinio empowers you to reach your desired audience with precision and ease.
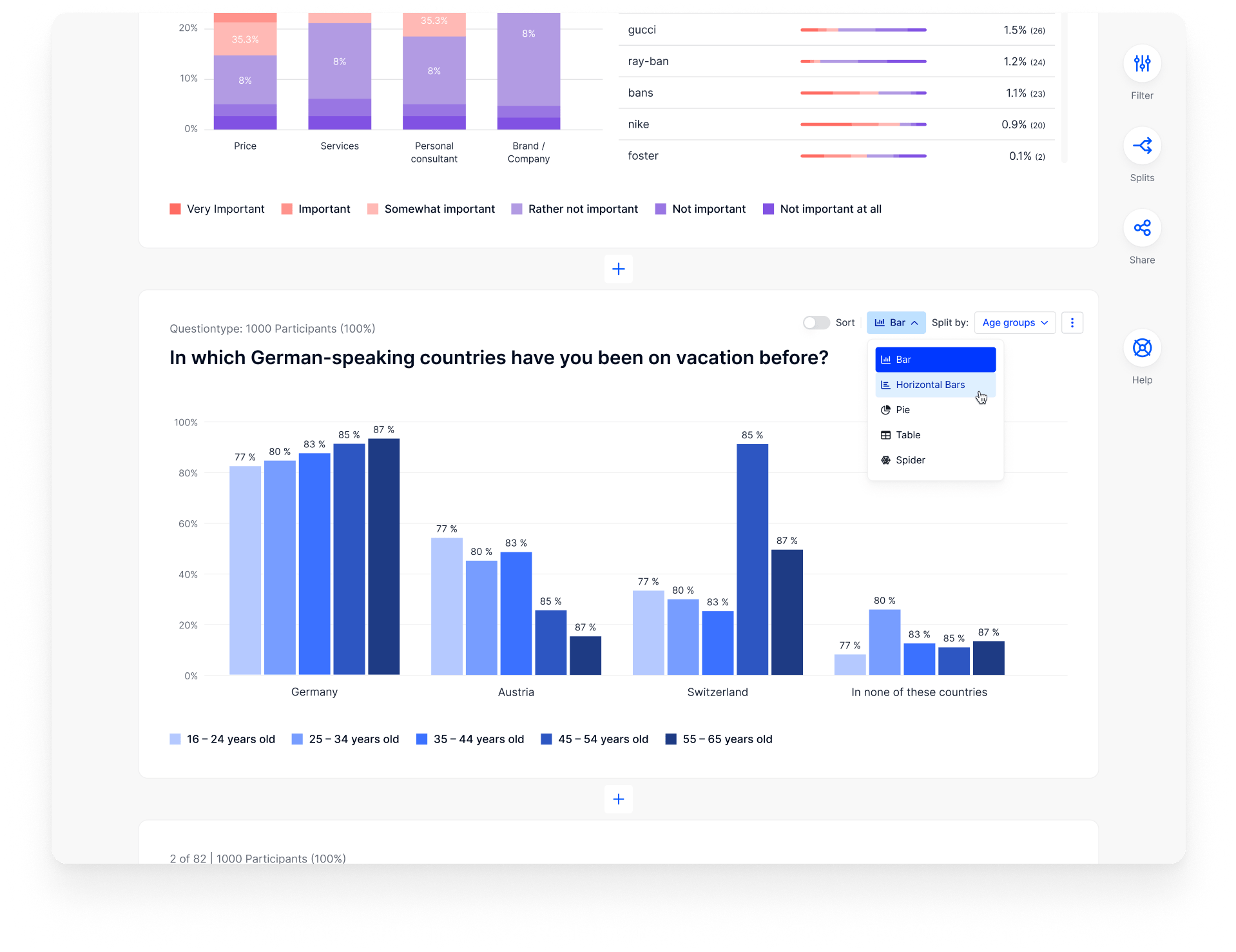
Get free access to the platform!
Join the loop 💌
Be the first to hear about new updates, product news, and data insights. We'll send it all straight to your inbox.
Get the latest market research news straight to your inbox! 💌
Wait, there's more
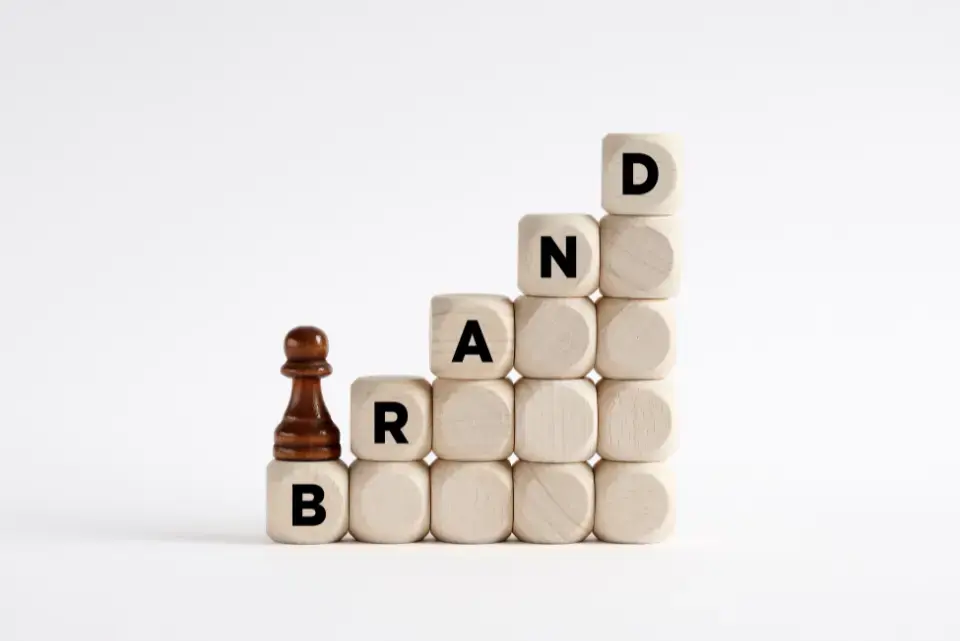
26.06.2024 | 35min read
Brand Development: Definition, Process, Strategies, Examples
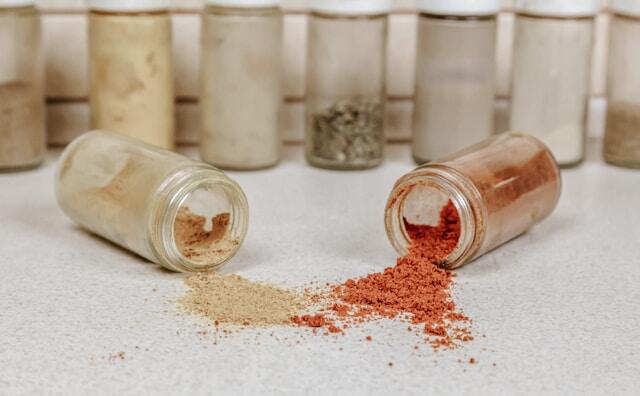
18.06.2024 | 7min read
Future Flavors: How Burger King nailed Concept Testing with Appinio's Predictive Insights
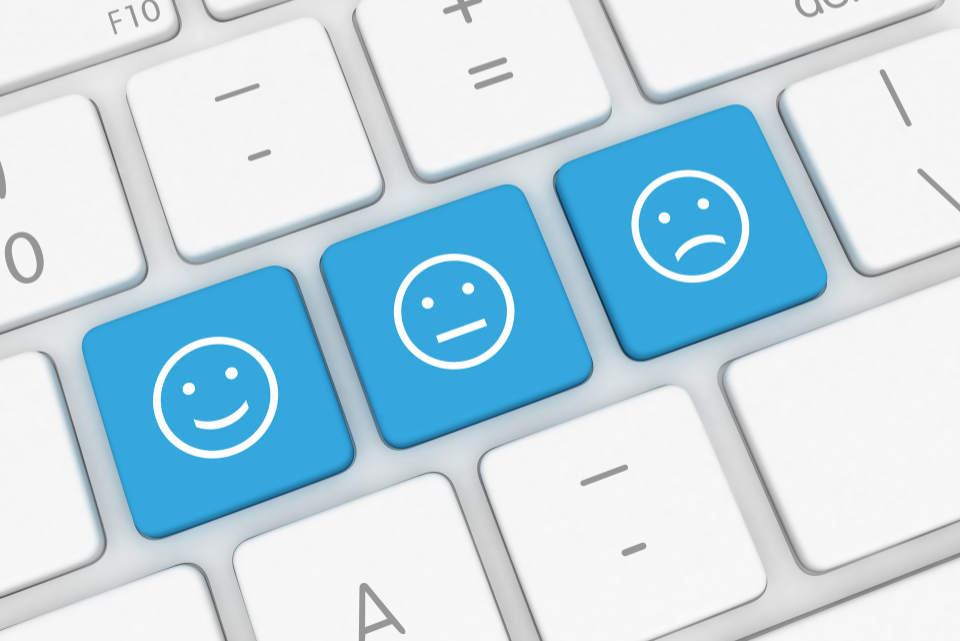
18.06.2024 | 32min read
What is a Pulse Survey? Definition, Types, Questions

An official website of the United States government
The .gov means it’s official. Federal government websites often end in .gov or .mil. Before sharing sensitive information, make sure you’re on a federal government site.
The site is secure. The https:// ensures that you are connecting to the official website and that any information you provide is encrypted and transmitted securely.
- Publications
- Account settings
Preview improvements coming to the PMC website in October 2024. Learn More or Try it out now .
- Advanced Search
- Journal List
- CBE Life Sci Educ
- v.21(3); Fall 2022
Literature Reviews, Theoretical Frameworks, and Conceptual Frameworks: An Introduction for New Biology Education Researchers
Julie a. luft.
† Department of Mathematics, Social Studies, and Science Education, Mary Frances Early College of Education, University of Georgia, Athens, GA 30602-7124
Sophia Jeong
‡ Department of Teaching & Learning, College of Education & Human Ecology, Ohio State University, Columbus, OH 43210
Robert Idsardi
§ Department of Biology, Eastern Washington University, Cheney, WA 99004
Grant Gardner
∥ Department of Biology, Middle Tennessee State University, Murfreesboro, TN 37132
Associated Data
To frame their work, biology education researchers need to consider the role of literature reviews, theoretical frameworks, and conceptual frameworks as critical elements of the research and writing process. However, these elements can be confusing for scholars new to education research. This Research Methods article is designed to provide an overview of each of these elements and delineate the purpose of each in the educational research process. We describe what biology education researchers should consider as they conduct literature reviews, identify theoretical frameworks, and construct conceptual frameworks. Clarifying these different components of educational research studies can be helpful to new biology education researchers and the biology education research community at large in situating their work in the broader scholarly literature.
INTRODUCTION
Discipline-based education research (DBER) involves the purposeful and situated study of teaching and learning in specific disciplinary areas ( Singer et al. , 2012 ). Studies in DBER are guided by research questions that reflect disciplines’ priorities and worldviews. Researchers can use quantitative data, qualitative data, or both to answer these research questions through a variety of methodological traditions. Across all methodologies, there are different methods associated with planning and conducting educational research studies that include the use of surveys, interviews, observations, artifacts, or instruments. Ensuring the coherence of these elements to the discipline’s perspective also involves situating the work in the broader scholarly literature. The tools for doing this include literature reviews, theoretical frameworks, and conceptual frameworks. However, the purpose and function of each of these elements is often confusing to new education researchers. The goal of this article is to introduce new biology education researchers to these three important elements important in DBER scholarship and the broader educational literature.
The first element we discuss is a review of research (literature reviews), which highlights the need for a specific research question, study problem, or topic of investigation. Literature reviews situate the relevance of the study within a topic and a field. The process may seem familiar to science researchers entering DBER fields, but new researchers may still struggle in conducting the review. Booth et al. (2016b) highlight some of the challenges novice education researchers face when conducting a review of literature. They point out that novice researchers struggle in deciding how to focus the review, determining the scope of articles needed in the review, and knowing how to be critical of the articles in the review. Overcoming these challenges (and others) can help novice researchers construct a sound literature review that can inform the design of the study and help ensure the work makes a contribution to the field.
The second and third highlighted elements are theoretical and conceptual frameworks. These guide biology education research (BER) studies, and may be less familiar to science researchers. These elements are important in shaping the construction of new knowledge. Theoretical frameworks offer a way to explain and interpret the studied phenomenon, while conceptual frameworks clarify assumptions about the studied phenomenon. Despite the importance of these constructs in educational research, biology educational researchers have noted the limited use of theoretical or conceptual frameworks in published work ( DeHaan, 2011 ; Dirks, 2011 ; Lo et al. , 2019 ). In reviewing articles published in CBE—Life Sciences Education ( LSE ) between 2015 and 2019, we found that fewer than 25% of the research articles had a theoretical or conceptual framework (see the Supplemental Information), and at times there was an inconsistent use of theoretical and conceptual frameworks. Clearly, these frameworks are challenging for published biology education researchers, which suggests the importance of providing some initial guidance to new biology education researchers.
Fortunately, educational researchers have increased their explicit use of these frameworks over time, and this is influencing educational research in science, technology, engineering, and mathematics (STEM) fields. For instance, a quick search for theoretical or conceptual frameworks in the abstracts of articles in Educational Research Complete (a common database for educational research) in STEM fields demonstrates a dramatic change over the last 20 years: from only 778 articles published between 2000 and 2010 to 5703 articles published between 2010 and 2020, a more than sevenfold increase. Greater recognition of the importance of these frameworks is contributing to DBER authors being more explicit about such frameworks in their studies.
Collectively, literature reviews, theoretical frameworks, and conceptual frameworks work to guide methodological decisions and the elucidation of important findings. Each offers a different perspective on the problem of study and is an essential element in all forms of educational research. As new researchers seek to learn about these elements, they will find different resources, a variety of perspectives, and many suggestions about the construction and use of these elements. The wide range of available information can overwhelm the new researcher who just wants to learn the distinction between these elements or how to craft them adequately.
Our goal in writing this paper is not to offer specific advice about how to write these sections in scholarly work. Instead, we wanted to introduce these elements to those who are new to BER and who are interested in better distinguishing one from the other. In this paper, we share the purpose of each element in BER scholarship, along with important points on its construction. We also provide references for additional resources that may be beneficial to better understanding each element. Table 1 summarizes the key distinctions among these elements.
Comparison of literature reviews, theoretical frameworks, and conceptual reviews
Literature reviews | Theoretical frameworks | Conceptual frameworks | |
---|---|---|---|
Purpose | To point out the need for the study in BER and connection to the field. | To state the assumptions and orientations of the researcher regarding the topic of study | To describe the researcher’s understanding of the main concepts under investigation |
Aims | A literature review examines current and relevant research associated with the study question. It is comprehensive, critical, and purposeful. | A theoretical framework illuminates the phenomenon of study and the corresponding assumptions adopted by the researcher. Frameworks can take on different orientations. | The conceptual framework is created by the researcher(s), includes the presumed relationships among concepts, and addresses needed areas of study discovered in literature reviews. |
Connection to the manuscript | A literature review should connect to the study question, guide the study methodology, and be central in the discussion by indicating how the analyzed data advances what is known in the field. | A theoretical framework drives the question, guides the types of methods for data collection and analysis, informs the discussion of the findings, and reveals the subjectivities of the researcher. | The conceptual framework is informed by literature reviews, experiences, or experiments. It may include emergent ideas that are not yet grounded in the literature. It should be coherent with the paper’s theoretical framing. |
Additional points | A literature review may reach beyond BER and include other education research fields. | A theoretical framework does not rationalize the need for the study, and a theoretical framework can come from different fields. | A conceptual framework articulates the phenomenon under study through written descriptions and/or visual representations. |
This article is written for the new biology education researcher who is just learning about these different elements or for scientists looking to become more involved in BER. It is a result of our own work as science education and biology education researchers, whether as graduate students and postdoctoral scholars or newly hired and established faculty members. This is the article we wish had been available as we started to learn about these elements or discussed them with new educational researchers in biology.
LITERATURE REVIEWS
Purpose of a literature review.
A literature review is foundational to any research study in education or science. In education, a well-conceptualized and well-executed review provides a summary of the research that has already been done on a specific topic and identifies questions that remain to be answered, thus illustrating the current research project’s potential contribution to the field and the reasoning behind the methodological approach selected for the study ( Maxwell, 2012 ). BER is an evolving disciplinary area that is redefining areas of conceptual emphasis as well as orientations toward teaching and learning (e.g., Labov et al. , 2010 ; American Association for the Advancement of Science, 2011 ; Nehm, 2019 ). As a result, building comprehensive, critical, purposeful, and concise literature reviews can be a challenge for new biology education researchers.
Building Literature Reviews
There are different ways to approach and construct a literature review. Booth et al. (2016a) provide an overview that includes, for example, scoping reviews, which are focused only on notable studies and use a basic method of analysis, and integrative reviews, which are the result of exhaustive literature searches across different genres. Underlying each of these different review processes are attention to the s earch process, a ppraisa l of articles, s ynthesis of the literature, and a nalysis: SALSA ( Booth et al. , 2016a ). This useful acronym can help the researcher focus on the process while building a specific type of review.
However, new educational researchers often have questions about literature reviews that are foundational to SALSA or other approaches. Common questions concern determining which literature pertains to the topic of study or the role of the literature review in the design of the study. This section addresses such questions broadly while providing general guidance for writing a narrative literature review that evaluates the most pertinent studies.
The literature review process should begin before the research is conducted. As Boote and Beile (2005 , p. 3) suggested, researchers should be “scholars before researchers.” They point out that having a good working knowledge of the proposed topic helps illuminate avenues of study. Some subject areas have a deep body of work to read and reflect upon, providing a strong foundation for developing the research question(s). For instance, the teaching and learning of evolution is an area of long-standing interest in the BER community, generating many studies (e.g., Perry et al. , 2008 ; Barnes and Brownell, 2016 ) and reviews of research (e.g., Sickel and Friedrichsen, 2013 ; Ziadie and Andrews, 2018 ). Emerging areas of BER include the affective domain, issues of transfer, and metacognition ( Singer et al. , 2012 ). Many studies in these areas are transdisciplinary and not always specific to biology education (e.g., Rodrigo-Peiris et al. , 2018 ; Kolpikova et al. , 2019 ). These newer areas may require reading outside BER; fortunately, summaries of some of these topics can be found in the Current Insights section of the LSE website.
In focusing on a specific problem within a broader research strand, a new researcher will likely need to examine research outside BER. Depending upon the area of study, the expanded reading list might involve a mix of BER, DBER, and educational research studies. Determining the scope of the reading is not always straightforward. A simple way to focus one’s reading is to create a “summary phrase” or “research nugget,” which is a very brief descriptive statement about the study. It should focus on the essence of the study, for example, “first-year nonmajor students’ understanding of evolution,” “metacognitive prompts to enhance learning during biochemistry,” or “instructors’ inquiry-based instructional practices after professional development programming.” This type of phrase should help a new researcher identify two or more areas to review that pertain to the study. Focusing on recent research in the last 5 years is a good first step. Additional studies can be identified by reading relevant works referenced in those articles. It is also important to read seminal studies that are more than 5 years old. Reading a range of studies should give the researcher the necessary command of the subject in order to suggest a research question.
Given that the research question(s) arise from the literature review, the review should also substantiate the selected methodological approach. The review and research question(s) guide the researcher in determining how to collect and analyze data. Often the methodological approach used in a study is selected to contribute knowledge that expands upon what has been published previously about the topic (see Institute of Education Sciences and National Science Foundation, 2013 ). An emerging topic of study may need an exploratory approach that allows for a description of the phenomenon and development of a potential theory. This could, but not necessarily, require a methodological approach that uses interviews, observations, surveys, or other instruments. An extensively studied topic may call for the additional understanding of specific factors or variables; this type of study would be well suited to a verification or a causal research design. These could entail a methodological approach that uses valid and reliable instruments, observations, or interviews to determine an effect in the studied event. In either of these examples, the researcher(s) may use a qualitative, quantitative, or mixed methods methodological approach.
Even with a good research question, there is still more reading to be done. The complexity and focus of the research question dictates the depth and breadth of the literature to be examined. Questions that connect multiple topics can require broad literature reviews. For instance, a study that explores the impact of a biology faculty learning community on the inquiry instruction of faculty could have the following review areas: learning communities among biology faculty, inquiry instruction among biology faculty, and inquiry instruction among biology faculty as a result of professional learning. Biology education researchers need to consider whether their literature review requires studies from different disciplines within or outside DBER. For the example given, it would be fruitful to look at research focused on learning communities with faculty in STEM fields or in general education fields that result in instructional change. It is important not to be too narrow or too broad when reading. When the conclusions of articles start to sound similar or no new insights are gained, the researcher likely has a good foundation for a literature review. This level of reading should allow the researcher to demonstrate a mastery in understanding the researched topic, explain the suitability of the proposed research approach, and point to the need for the refined research question(s).
The literature review should include the researcher’s evaluation and critique of the selected studies. A researcher may have a large collection of studies, but not all of the studies will follow standards important in the reporting of empirical work in the social sciences. The American Educational Research Association ( Duran et al. , 2006 ), for example, offers a general discussion about standards for such work: an adequate review of research informing the study, the existence of sound and appropriate data collection and analysis methods, and appropriate conclusions that do not overstep or underexplore the analyzed data. The Institute of Education Sciences and National Science Foundation (2013) also offer Common Guidelines for Education Research and Development that can be used to evaluate collected studies.
Because not all journals adhere to such standards, it is important that a researcher review each study to determine the quality of published research, per the guidelines suggested earlier. In some instances, the research may be fatally flawed. Examples of such flaws include data that do not pertain to the question, a lack of discussion about the data collection, poorly constructed instruments, or an inadequate analysis. These types of errors result in studies that are incomplete, error-laden, or inaccurate and should be excluded from the review. Most studies have limitations, and the author(s) often make them explicit. For instance, there may be an instructor effect, recognized bias in the analysis, or issues with the sample population. Limitations are usually addressed by the research team in some way to ensure a sound and acceptable research process. Occasionally, the limitations associated with the study can be significant and not addressed adequately, which leaves a consequential decision in the hands of the researcher. Providing critiques of studies in the literature review process gives the reader confidence that the researcher has carefully examined relevant work in preparation for the study and, ultimately, the manuscript.
A solid literature review clearly anchors the proposed study in the field and connects the research question(s), the methodological approach, and the discussion. Reviewing extant research leads to research questions that will contribute to what is known in the field. By summarizing what is known, the literature review points to what needs to be known, which in turn guides decisions about methodology. Finally, notable findings of the new study are discussed in reference to those described in the literature review.
Within published BER studies, literature reviews can be placed in different locations in an article. When included in the introductory section of the study, the first few paragraphs of the manuscript set the stage, with the literature review following the opening paragraphs. Cooper et al. (2019) illustrate this approach in their study of course-based undergraduate research experiences (CUREs). An introduction discussing the potential of CURES is followed by an analysis of the existing literature relevant to the design of CUREs that allows for novel student discoveries. Within this review, the authors point out contradictory findings among research on novel student discoveries. This clarifies the need for their study, which is described and highlighted through specific research aims.
A literature reviews can also make up a separate section in a paper. For example, the introduction to Todd et al. (2019) illustrates the need for their research topic by highlighting the potential of learning progressions (LPs) and suggesting that LPs may help mitigate learning loss in genetics. At the end of the introduction, the authors state their specific research questions. The review of literature following this opening section comprises two subsections. One focuses on learning loss in general and examines a variety of studies and meta-analyses from the disciplines of medical education, mathematics, and reading. The second section focuses specifically on LPs in genetics and highlights student learning in the midst of LPs. These separate reviews provide insights into the stated research question.
Suggestions and Advice
A well-conceptualized, comprehensive, and critical literature review reveals the understanding of the topic that the researcher brings to the study. Literature reviews should not be so big that there is no clear area of focus; nor should they be so narrow that no real research question arises. The task for a researcher is to craft an efficient literature review that offers a critical analysis of published work, articulates the need for the study, guides the methodological approach to the topic of study, and provides an adequate foundation for the discussion of the findings.
In our own writing of literature reviews, there are often many drafts. An early draft may seem well suited to the study because the need for and approach to the study are well described. However, as the results of the study are analyzed and findings begin to emerge, the existing literature review may be inadequate and need revision. The need for an expanded discussion about the research area can result in the inclusion of new studies that support the explanation of a potential finding. The literature review may also prove to be too broad. Refocusing on a specific area allows for more contemplation of a finding.
It should be noted that there are different types of literature reviews, and many books and articles have been written about the different ways to embark on these types of reviews. Among these different resources, the following may be helpful in considering how to refine the review process for scholarly journals:
- Booth, A., Sutton, A., & Papaioannou, D. (2016a). Systemic approaches to a successful literature review (2nd ed.). Los Angeles, CA: Sage. This book addresses different types of literature reviews and offers important suggestions pertaining to defining the scope of the literature review and assessing extant studies.
- Booth, W. C., Colomb, G. G., Williams, J. M., Bizup, J., & Fitzgerald, W. T. (2016b). The craft of research (4th ed.). Chicago: University of Chicago Press. This book can help the novice consider how to make the case for an area of study. While this book is not specifically about literature reviews, it offers suggestions about making the case for your study.
- Galvan, J. L., & Galvan, M. C. (2017). Writing literature reviews: A guide for students of the social and behavioral sciences (7th ed.). Routledge. This book offers guidance on writing different types of literature reviews. For the novice researcher, there are useful suggestions for creating coherent literature reviews.
THEORETICAL FRAMEWORKS
Purpose of theoretical frameworks.
As new education researchers may be less familiar with theoretical frameworks than with literature reviews, this discussion begins with an analogy. Envision a biologist, chemist, and physicist examining together the dramatic effect of a fog tsunami over the ocean. A biologist gazing at this phenomenon may be concerned with the effect of fog on various species. A chemist may be interested in the chemical composition of the fog as water vapor condenses around bits of salt. A physicist may be focused on the refraction of light to make fog appear to be “sitting” above the ocean. While observing the same “objective event,” the scientists are operating under different theoretical frameworks that provide a particular perspective or “lens” for the interpretation of the phenomenon. Each of these scientists brings specialized knowledge, experiences, and values to this phenomenon, and these influence the interpretation of the phenomenon. The scientists’ theoretical frameworks influence how they design and carry out their studies and interpret their data.
Within an educational study, a theoretical framework helps to explain a phenomenon through a particular lens and challenges and extends existing knowledge within the limitations of that lens. Theoretical frameworks are explicitly stated by an educational researcher in the paper’s framework, theory, or relevant literature section. The framework shapes the types of questions asked, guides the method by which data are collected and analyzed, and informs the discussion of the results of the study. It also reveals the researcher’s subjectivities, for example, values, social experience, and viewpoint ( Allen, 2017 ). It is essential that a novice researcher learn to explicitly state a theoretical framework, because all research questions are being asked from the researcher’s implicit or explicit assumptions of a phenomenon of interest ( Schwandt, 2000 ).
Selecting Theoretical Frameworks
Theoretical frameworks are one of the most contemplated elements in our work in educational research. In this section, we share three important considerations for new scholars selecting a theoretical framework.
The first step in identifying a theoretical framework involves reflecting on the phenomenon within the study and the assumptions aligned with the phenomenon. The phenomenon involves the studied event. There are many possibilities, for example, student learning, instructional approach, or group organization. A researcher holds assumptions about how the phenomenon will be effected, influenced, changed, or portrayed. It is ultimately the researcher’s assumption(s) about the phenomenon that aligns with a theoretical framework. An example can help illustrate how a researcher’s reflection on the phenomenon and acknowledgment of assumptions can result in the identification of a theoretical framework.
In our example, a biology education researcher may be interested in exploring how students’ learning of difficult biological concepts can be supported by the interactions of group members. The phenomenon of interest is the interactions among the peers, and the researcher assumes that more knowledgeable students are important in supporting the learning of the group. As a result, the researcher may draw on Vygotsky’s (1978) sociocultural theory of learning and development that is focused on the phenomenon of student learning in a social setting. This theory posits the critical nature of interactions among students and between students and teachers in the process of building knowledge. A researcher drawing upon this framework holds the assumption that learning is a dynamic social process involving questions and explanations among students in the classroom and that more knowledgeable peers play an important part in the process of building conceptual knowledge.
It is important to state at this point that there are many different theoretical frameworks. Some frameworks focus on learning and knowing, while other theoretical frameworks focus on equity, empowerment, or discourse. Some frameworks are well articulated, and others are still being refined. For a new researcher, it can be challenging to find a theoretical framework. Two of the best ways to look for theoretical frameworks is through published works that highlight different frameworks.
When a theoretical framework is selected, it should clearly connect to all parts of the study. The framework should augment the study by adding a perspective that provides greater insights into the phenomenon. It should clearly align with the studies described in the literature review. For instance, a framework focused on learning would correspond to research that reported different learning outcomes for similar studies. The methods for data collection and analysis should also correspond to the framework. For instance, a study about instructional interventions could use a theoretical framework concerned with learning and could collect data about the effect of the intervention on what is learned. When the data are analyzed, the theoretical framework should provide added meaning to the findings, and the findings should align with the theoretical framework.
A study by Jensen and Lawson (2011) provides an example of how a theoretical framework connects different parts of the study. They compared undergraduate biology students in heterogeneous and homogeneous groups over the course of a semester. Jensen and Lawson (2011) assumed that learning involved collaboration and more knowledgeable peers, which made Vygotsky’s (1978) theory a good fit for their study. They predicted that students in heterogeneous groups would experience greater improvement in their reasoning abilities and science achievements with much of the learning guided by the more knowledgeable peers.
In the enactment of the study, they collected data about the instruction in traditional and inquiry-oriented classes, while the students worked in homogeneous or heterogeneous groups. To determine the effect of working in groups, the authors also measured students’ reasoning abilities and achievement. Each data-collection and analysis decision connected to understanding the influence of collaborative work.
Their findings highlighted aspects of Vygotsky’s (1978) theory of learning. One finding, for instance, posited that inquiry instruction, as a whole, resulted in reasoning and achievement gains. This links to Vygotsky (1978) , because inquiry instruction involves interactions among group members. A more nuanced finding was that group composition had a conditional effect. Heterogeneous groups performed better with more traditional and didactic instruction, regardless of the reasoning ability of the group members. Homogeneous groups worked better during interaction-rich activities for students with low reasoning ability. The authors attributed the variation to the different types of helping behaviors of students. High-performing students provided the answers, while students with low reasoning ability had to work collectively through the material. In terms of Vygotsky (1978) , this finding provided new insights into the learning context in which productive interactions can occur for students.
Another consideration in the selection and use of a theoretical framework pertains to its orientation to the study. This can result in the theoretical framework prioritizing individuals, institutions, and/or policies ( Anfara and Mertz, 2014 ). Frameworks that connect to individuals, for instance, could contribute to understanding their actions, learning, or knowledge. Institutional frameworks, on the other hand, offer insights into how institutions, organizations, or groups can influence individuals or materials. Policy theories provide ways to understand how national or local policies can dictate an emphasis on outcomes or instructional design. These different types of frameworks highlight different aspects in an educational setting, which influences the design of the study and the collection of data. In addition, these different frameworks offer a way to make sense of the data. Aligning the data collection and analysis with the framework ensures that a study is coherent and can contribute to the field.
New understandings emerge when different theoretical frameworks are used. For instance, Ebert-May et al. (2015) prioritized the individual level within conceptual change theory (see Posner et al. , 1982 ). In this theory, an individual’s knowledge changes when it no longer fits the phenomenon. Ebert-May et al. (2015) designed a professional development program challenging biology postdoctoral scholars’ existing conceptions of teaching. The authors reported that the biology postdoctoral scholars’ teaching practices became more student-centered as they were challenged to explain their instructional decision making. According to the theory, the biology postdoctoral scholars’ dissatisfaction in their descriptions of teaching and learning initiated change in their knowledge and instruction. These results reveal how conceptual change theory can explain the learning of participants and guide the design of professional development programming.
The communities of practice (CoP) theoretical framework ( Lave, 1988 ; Wenger, 1998 ) prioritizes the institutional level , suggesting that learning occurs when individuals learn from and contribute to the communities in which they reside. Grounded in the assumption of community learning, the literature on CoP suggests that, as individuals interact regularly with the other members of their group, they learn about the rules, roles, and goals of the community ( Allee, 2000 ). A study conducted by Gehrke and Kezar (2017) used the CoP framework to understand organizational change by examining the involvement of individual faculty engaged in a cross-institutional CoP focused on changing the instructional practice of faculty at each institution. In the CoP, faculty members were involved in enhancing instructional materials within their department, which aligned with an overarching goal of instituting instruction that embraced active learning. Not surprisingly, Gehrke and Kezar (2017) revealed that faculty who perceived the community culture as important in their work cultivated institutional change. Furthermore, they found that institutional change was sustained when key leaders served as mentors and provided support for faculty, and as faculty themselves developed into leaders. This study reveals the complexity of individual roles in a COP in order to support institutional instructional change.
It is important to explicitly state the theoretical framework used in a study, but elucidating a theoretical framework can be challenging for a new educational researcher. The literature review can help to identify an applicable theoretical framework. Focal areas of the review or central terms often connect to assumptions and assertions associated with the framework that pertain to the phenomenon of interest. Another way to identify a theoretical framework is self-reflection by the researcher on personal beliefs and understandings about the nature of knowledge the researcher brings to the study ( Lysaght, 2011 ). In stating one’s beliefs and understandings related to the study (e.g., students construct their knowledge, instructional materials support learning), an orientation becomes evident that will suggest a particular theoretical framework. Theoretical frameworks are not arbitrary , but purposefully selected.
With experience, a researcher may find expanded roles for theoretical frameworks. Researchers may revise an existing framework that has limited explanatory power, or they may decide there is a need to develop a new theoretical framework. These frameworks can emerge from a current study or the need to explain a phenomenon in a new way. Researchers may also find that multiple theoretical frameworks are necessary to frame and explore a problem, as different frameworks can provide different insights into a problem.
Finally, it is important to recognize that choosing “x” theoretical framework does not necessarily mean a researcher chooses “y” methodology and so on, nor is there a clear-cut, linear process in selecting a theoretical framework for one’s study. In part, the nonlinear process of identifying a theoretical framework is what makes understanding and using theoretical frameworks challenging. For the novice scholar, contemplating and understanding theoretical frameworks is essential. Fortunately, there are articles and books that can help:
- Creswell, J. W. (2018). Research design: Qualitative, quantitative, and mixed methods approaches (5th ed.). Los Angeles, CA: Sage. This book provides an overview of theoretical frameworks in general educational research.
- Ding, L. (2019). Theoretical perspectives of quantitative physics education research. Physical Review Physics Education Research , 15 (2), 020101-1–020101-13. This paper illustrates how a DBER field can use theoretical frameworks.
- Nehm, R. (2019). Biology education research: Building integrative frameworks for teaching and learning about living systems. Disciplinary and Interdisciplinary Science Education Research , 1 , ar15. https://doi.org/10.1186/s43031-019-0017-6 . This paper articulates the need for studies in BER to explicitly state theoretical frameworks and provides examples of potential studies.
- Patton, M. Q. (2015). Qualitative research & evaluation methods: Integrating theory and practice . Sage. This book also provides an overview of theoretical frameworks, but for both research and evaluation.
CONCEPTUAL FRAMEWORKS
Purpose of a conceptual framework.
A conceptual framework is a description of the way a researcher understands the factors and/or variables that are involved in the study and their relationships to one another. The purpose of a conceptual framework is to articulate the concepts under study using relevant literature ( Rocco and Plakhotnik, 2009 ) and to clarify the presumed relationships among those concepts ( Rocco and Plakhotnik, 2009 ; Anfara and Mertz, 2014 ). Conceptual frameworks are different from theoretical frameworks in both their breadth and grounding in established findings. Whereas a theoretical framework articulates the lens through which a researcher views the work, the conceptual framework is often more mechanistic and malleable.
Conceptual frameworks are broader, encompassing both established theories (i.e., theoretical frameworks) and the researchers’ own emergent ideas. Emergent ideas, for example, may be rooted in informal and/or unpublished observations from experience. These emergent ideas would not be considered a “theory” if they are not yet tested, supported by systematically collected evidence, and peer reviewed. However, they do still play an important role in the way researchers approach their studies. The conceptual framework allows authors to clearly describe their emergent ideas so that connections among ideas in the study and the significance of the study are apparent to readers.
Constructing Conceptual Frameworks
Including a conceptual framework in a research study is important, but researchers often opt to include either a conceptual or a theoretical framework. Either may be adequate, but both provide greater insight into the research approach. For instance, a research team plans to test a novel component of an existing theory. In their study, they describe the existing theoretical framework that informs their work and then present their own conceptual framework. Within this conceptual framework, specific topics portray emergent ideas that are related to the theory. Describing both frameworks allows readers to better understand the researchers’ assumptions, orientations, and understanding of concepts being investigated. For example, Connolly et al. (2018) included a conceptual framework that described how they applied a theoretical framework of social cognitive career theory (SCCT) to their study on teaching programs for doctoral students. In their conceptual framework, the authors described SCCT, explained how it applied to the investigation, and drew upon results from previous studies to justify the proposed connections between the theory and their emergent ideas.
In some cases, authors may be able to sufficiently describe their conceptualization of the phenomenon under study in an introduction alone, without a separate conceptual framework section. However, incomplete descriptions of how the researchers conceptualize the components of the study may limit the significance of the study by making the research less intelligible to readers. This is especially problematic when studying topics in which researchers use the same terms for different constructs or different terms for similar and overlapping constructs (e.g., inquiry, teacher beliefs, pedagogical content knowledge, or active learning). Authors must describe their conceptualization of a construct if the research is to be understandable and useful.
There are some key areas to consider regarding the inclusion of a conceptual framework in a study. To begin with, it is important to recognize that conceptual frameworks are constructed by the researchers conducting the study ( Rocco and Plakhotnik, 2009 ; Maxwell, 2012 ). This is different from theoretical frameworks that are often taken from established literature. Researchers should bring together ideas from the literature, but they may be influenced by their own experiences as a student and/or instructor, the shared experiences of others, or thought experiments as they construct a description, model, or representation of their understanding of the phenomenon under study. This is an exercise in intellectual organization and clarity that often considers what is learned, known, and experienced. The conceptual framework makes these constructs explicitly visible to readers, who may have different understandings of the phenomenon based on their prior knowledge and experience. There is no single method to go about this intellectual work.
Reeves et al. (2016) is an example of an article that proposed a conceptual framework about graduate teaching assistant professional development evaluation and research. The authors used existing literature to create a novel framework that filled a gap in current research and practice related to the training of graduate teaching assistants. This conceptual framework can guide the systematic collection of data by other researchers because the framework describes the relationships among various factors that influence teaching and learning. The Reeves et al. (2016) conceptual framework may be modified as additional data are collected and analyzed by other researchers. This is not uncommon, as conceptual frameworks can serve as catalysts for concerted research efforts that systematically explore a phenomenon (e.g., Reynolds et al. , 2012 ; Brownell and Kloser, 2015 ).
Sabel et al. (2017) used a conceptual framework in their exploration of how scaffolds, an external factor, interact with internal factors to support student learning. Their conceptual framework integrated principles from two theoretical frameworks, self-regulated learning and metacognition, to illustrate how the research team conceptualized students’ use of scaffolds in their learning ( Figure 1 ). Sabel et al. (2017) created this model using their interpretations of these two frameworks in the context of their teaching.

Conceptual framework from Sabel et al. (2017) .
A conceptual framework should describe the relationship among components of the investigation ( Anfara and Mertz, 2014 ). These relationships should guide the researcher’s methods of approaching the study ( Miles et al. , 2014 ) and inform both the data to be collected and how those data should be analyzed. Explicitly describing the connections among the ideas allows the researcher to justify the importance of the study and the rigor of the research design. Just as importantly, these frameworks help readers understand why certain components of a system were not explored in the study. This is a challenge in education research, which is rooted in complex environments with many variables that are difficult to control.
For example, Sabel et al. (2017) stated: “Scaffolds, such as enhanced answer keys and reflection questions, can help students and instructors bridge the external and internal factors and support learning” (p. 3). They connected the scaffolds in the study to the three dimensions of metacognition and the eventual transformation of existing ideas into new or revised ideas. Their framework provides a rationale for focusing on how students use two different scaffolds, and not on other factors that may influence a student’s success (self-efficacy, use of active learning, exam format, etc.).
In constructing conceptual frameworks, researchers should address needed areas of study and/or contradictions discovered in literature reviews. By attending to these areas, researchers can strengthen their arguments for the importance of a study. For instance, conceptual frameworks can address how the current study will fill gaps in the research, resolve contradictions in existing literature, or suggest a new area of study. While a literature review describes what is known and not known about the phenomenon, the conceptual framework leverages these gaps in describing the current study ( Maxwell, 2012 ). In the example of Sabel et al. (2017) , the authors indicated there was a gap in the literature regarding how scaffolds engage students in metacognition to promote learning in large classes. Their study helps fill that gap by describing how scaffolds can support students in the three dimensions of metacognition: intelligibility, plausibility, and wide applicability. In another example, Lane (2016) integrated research from science identity, the ethic of care, the sense of belonging, and an expertise model of student success to form a conceptual framework that addressed the critiques of other frameworks. In a more recent example, Sbeglia et al. (2021) illustrated how a conceptual framework influences the methodological choices and inferences in studies by educational researchers.
Sometimes researchers draw upon the conceptual frameworks of other researchers. When a researcher’s conceptual framework closely aligns with an existing framework, the discussion may be brief. For example, Ghee et al. (2016) referred to portions of SCCT as their conceptual framework to explain the significance of their work on students’ self-efficacy and career interests. Because the authors’ conceptualization of this phenomenon aligned with a previously described framework, they briefly mentioned the conceptual framework and provided additional citations that provided more detail for the readers.
Within both the BER and the broader DBER communities, conceptual frameworks have been used to describe different constructs. For example, some researchers have used the term “conceptual framework” to describe students’ conceptual understandings of a biological phenomenon. This is distinct from a researcher’s conceptual framework of the educational phenomenon under investigation, which may also need to be explicitly described in the article. Other studies have presented a research logic model or flowchart of the research design as a conceptual framework. These constructions can be quite valuable in helping readers understand the data-collection and analysis process. However, a model depicting the study design does not serve the same role as a conceptual framework. Researchers need to avoid conflating these constructs by differentiating the researchers’ conceptual framework that guides the study from the research design, when applicable.
Explicitly describing conceptual frameworks is essential in depicting the focus of the study. We have found that being explicit in a conceptual framework means using accepted terminology, referencing prior work, and clearly noting connections between terms. This description can also highlight gaps in the literature or suggest potential contributions to the field of study. A well-elucidated conceptual framework can suggest additional studies that may be warranted. This can also spur other researchers to consider how they would approach the examination of a phenomenon and could result in a revised conceptual framework.
It can be challenging to create conceptual frameworks, but they are important. Below are two resources that could be helpful in constructing and presenting conceptual frameworks in educational research:
- Maxwell, J. A. (2012). Qualitative research design: An interactive approach (3rd ed.). Los Angeles, CA: Sage. Chapter 3 in this book describes how to construct conceptual frameworks.
- Ravitch, S. M., & Riggan, M. (2016). Reason & rigor: How conceptual frameworks guide research . Los Angeles, CA: Sage. This book explains how conceptual frameworks guide the research questions, data collection, data analyses, and interpretation of results.
CONCLUDING THOUGHTS
Literature reviews, theoretical frameworks, and conceptual frameworks are all important in DBER and BER. Robust literature reviews reinforce the importance of a study. Theoretical frameworks connect the study to the base of knowledge in educational theory and specify the researcher’s assumptions. Conceptual frameworks allow researchers to explicitly describe their conceptualization of the relationships among the components of the phenomenon under study. Table 1 provides a general overview of these components in order to assist biology education researchers in thinking about these elements.
It is important to emphasize that these different elements are intertwined. When these elements are aligned and complement one another, the study is coherent, and the study findings contribute to knowledge in the field. When literature reviews, theoretical frameworks, and conceptual frameworks are disconnected from one another, the study suffers. The point of the study is lost, suggested findings are unsupported, or important conclusions are invisible to the researcher. In addition, this misalignment may be costly in terms of time and money.
Conducting a literature review, selecting a theoretical framework, and building a conceptual framework are some of the most difficult elements of a research study. It takes time to understand the relevant research, identify a theoretical framework that provides important insights into the study, and formulate a conceptual framework that organizes the finding. In the research process, there is often a constant back and forth among these elements as the study evolves. With an ongoing refinement of the review of literature, clarification of the theoretical framework, and articulation of a conceptual framework, a sound study can emerge that makes a contribution to the field. This is the goal of BER and education research.
Supplementary Material
- Allee, V. (2000). Knowledge networks and communities of learning . OD Practitioner , 32 ( 4 ), 4–13. [ Google Scholar ]
- Allen, M. (2017). The Sage encyclopedia of communication research methods (Vols. 1–4 ). Los Angeles, CA: Sage. 10.4135/9781483381411 [ CrossRef ] [ Google Scholar ]
- American Association for the Advancement of Science. (2011). Vision and change in undergraduate biology education: A call to action . Washington, DC. [ Google Scholar ]
- Anfara, V. A., Mertz, N. T. (2014). Setting the stage . In Anfara, V. A., Mertz, N. T. (eds.), Theoretical frameworks in qualitative research (pp. 1–22). Sage. [ Google Scholar ]
- Barnes, M. E., Brownell, S. E. (2016). Practices and perspectives of college instructors on addressing religious beliefs when teaching evolution . CBE—Life Sciences Education , 15 ( 2 ), ar18. https://doi.org/10.1187/cbe.15-11-0243 [ PMC free article ] [ PubMed ] [ Google Scholar ]
- Boote, D. N., Beile, P. (2005). Scholars before researchers: On the centrality of the dissertation literature review in research preparation . Educational Researcher , 34 ( 6 ), 3–15. 10.3102/0013189x034006003 [ CrossRef ] [ Google Scholar ]
- Booth, A., Sutton, A., Papaioannou, D. (2016a). Systemic approaches to a successful literature review (2nd ed.). Los Angeles, CA: Sage. [ Google Scholar ]
- Booth, W. C., Colomb, G. G., Williams, J. M., Bizup, J., Fitzgerald, W. T. (2016b). The craft of research (4th ed.). Chicago, IL: University of Chicago Press. [ Google Scholar ]
- Brownell, S. E., Kloser, M. J. (2015). Toward a conceptual framework for measuring the effectiveness of course-based undergraduate research experiences in undergraduate biology . Studies in Higher Education , 40 ( 3 ), 525–544. https://doi.org/10.1080/03075079.2015.1004234 [ Google Scholar ]
- Connolly, M. R., Lee, Y. G., Savoy, J. N. (2018). The effects of doctoral teaching development on early-career STEM scholars’ college teaching self-efficacy . CBE—Life Sciences Education , 17 ( 1 ), ar14. https://doi.org/10.1187/cbe.17-02-0039 [ PMC free article ] [ PubMed ] [ Google Scholar ]
- Cooper, K. M., Blattman, J. N., Hendrix, T., Brownell, S. E. (2019). The impact of broadly relevant novel discoveries on student project ownership in a traditional lab course turned CURE . CBE—Life Sciences Education , 18 ( 4 ), ar57. https://doi.org/10.1187/cbe.19-06-0113 [ PMC free article ] [ PubMed ] [ Google Scholar ]
- Creswell, J. W. (2018). Research design: Qualitative, quantitative, and mixed methods approaches (5th ed.). Los Angeles, CA: Sage. [ Google Scholar ]
- DeHaan, R. L. (2011). Education research in the biological sciences: A nine decade review (Paper commissioned by the NAS/NRC Committee on the Status, Contributions, and Future Directions of Discipline Based Education Research) . Washington, DC: National Academies Press. Retrieved May 20, 2022, from www7.nationalacademies.org/bose/DBER_Mee ting2_commissioned_papers_page.html [ Google Scholar ]
- Ding, L. (2019). Theoretical perspectives of quantitative physics education research . Physical Review Physics Education Research , 15 ( 2 ), 020101. [ Google Scholar ]
- Dirks, C. (2011). The current status and future direction of biology education research . Paper presented at: Second Committee Meeting on the Status, Contributions, and Future Directions of Discipline-Based Education Research, 18–19 October (Washington, DC). Retrieved May 20, 2022, from http://sites.nationalacademies.org/DBASSE/BOSE/DBASSE_071087 [ Google Scholar ]
- Duran, R. P., Eisenhart, M. A., Erickson, F. D., Grant, C. A., Green, J. L., Hedges, L. V., Schneider, B. L. (2006). Standards for reporting on empirical social science research in AERA publications: American Educational Research Association . Educational Researcher , 35 ( 6 ), 33–40. [ Google Scholar ]
- Ebert-May, D., Derting, T. L., Henkel, T. P., Middlemis Maher, J., Momsen, J. L., Arnold, B., Passmore, H. A. (2015). Breaking the cycle: Future faculty begin teaching with learner-centered strategies after professional development . CBE—Life Sciences Education , 14 ( 2 ), ar22. https://doi.org/10.1187/cbe.14-12-0222 [ PMC free article ] [ PubMed ] [ Google Scholar ]
- Galvan, J. L., Galvan, M. C. (2017). Writing literature reviews: A guide for students of the social and behavioral sciences (7th ed.). New York, NY: Routledge. https://doi.org/10.4324/9781315229386 [ Google Scholar ]
- Gehrke, S., Kezar, A. (2017). The roles of STEM faculty communities of practice in institutional and departmental reform in higher education . American Educational Research Journal , 54 ( 5 ), 803–833. https://doi.org/10.3102/0002831217706736 [ Google Scholar ]
- Ghee, M., Keels, M., Collins, D., Neal-Spence, C., Baker, E. (2016). Fine-tuning summer research programs to promote underrepresented students’ persistence in the STEM pathway . CBE—Life Sciences Education , 15 ( 3 ), ar28. https://doi.org/10.1187/cbe.16-01-0046 [ PMC free article ] [ PubMed ] [ Google Scholar ]
- Institute of Education Sciences & National Science Foundation. (2013). Common guidelines for education research and development . Retrieved May 20, 2022, from www.nsf.gov/pubs/2013/nsf13126/nsf13126.pdf
- Jensen, J. L., Lawson, A. (2011). Effects of collaborative group composition and inquiry instruction on reasoning gains and achievement in undergraduate biology . CBE—Life Sciences Education , 10 ( 1 ), 64–73. https://doi.org/10.1187/cbe.19-05-0098 [ PMC free article ] [ PubMed ] [ Google Scholar ]
- Kolpikova, E. P., Chen, D. C., Doherty, J. H. (2019). Does the format of preclass reading quizzes matter? An evaluation of traditional and gamified, adaptive preclass reading quizzes . CBE—Life Sciences Education , 18 ( 4 ), ar52. https://doi.org/10.1187/cbe.19-05-0098 [ PMC free article ] [ PubMed ] [ Google Scholar ]
- Labov, J. B., Reid, A. H., Yamamoto, K. R. (2010). Integrated biology and undergraduate science education: A new biology education for the twenty-first century? CBE—Life Sciences Education , 9 ( 1 ), 10–16. https://doi.org/10.1187/cbe.09-12-0092 [ PMC free article ] [ PubMed ] [ Google Scholar ]
- Lane, T. B. (2016). Beyond academic and social integration: Understanding the impact of a STEM enrichment program on the retention and degree attainment of underrepresented students . CBE—Life Sciences Education , 15 ( 3 ), ar39. https://doi.org/10.1187/cbe.16-01-0070 [ PMC free article ] [ PubMed ] [ Google Scholar ]
- Lave, J. (1988). Cognition in practice: Mind, mathematics and culture in everyday life . New York, NY: Cambridge University Press. [ Google Scholar ]
- Lo, S. M., Gardner, G. E., Reid, J., Napoleon-Fanis, V., Carroll, P., Smith, E., Sato, B. K. (2019). Prevailing questions and methodologies in biology education research: A longitudinal analysis of research in CBE — Life Sciences Education and at the Society for the Advancement of Biology Education Research . CBE—Life Sciences Education , 18 ( 1 ), ar9. https://doi.org/10.1187/cbe.18-08-0164 [ PMC free article ] [ PubMed ] [ Google Scholar ]
- Lysaght, Z. (2011). Epistemological and paradigmatic ecumenism in “Pasteur’s quadrant:” Tales from doctoral research . In Official Conference Proceedings of the Third Asian Conference on Education in Osaka, Japan . Retrieved May 20, 2022, from http://iafor.org/ace2011_offprint/ACE2011_offprint_0254.pdf
- Maxwell, J. A. (2012). Qualitative research design: An interactive approach (3rd ed.). Los Angeles, CA: Sage. [ Google Scholar ]
- Miles, M. B., Huberman, A. M., Saldaña, J. (2014). Qualitative data analysis (3rd ed.). Los Angeles, CA: Sage. [ Google Scholar ]
- Nehm, R. (2019). Biology education research: Building integrative frameworks for teaching and learning about living systems . Disciplinary and Interdisciplinary Science Education Research , 1 , ar15. https://doi.org/10.1186/s43031-019-0017-6 [ Google Scholar ]
- Patton, M. Q. (2015). Qualitative research & evaluation methods: Integrating theory and practice . Los Angeles, CA: Sage. [ Google Scholar ]
- Perry, J., Meir, E., Herron, J. C., Maruca, S., Stal, D. (2008). Evaluating two approaches to helping college students understand evolutionary trees through diagramming tasks . CBE—Life Sciences Education , 7 ( 2 ), 193–201. https://doi.org/10.1187/cbe.07-01-0007 [ PMC free article ] [ PubMed ] [ Google Scholar ]
- Posner, G. J., Strike, K. A., Hewson, P. W., Gertzog, W. A. (1982). Accommodation of a scientific conception: Toward a theory of conceptual change . Science Education , 66 ( 2 ), 211–227. [ Google Scholar ]
- Ravitch, S. M., Riggan, M. (2016). Reason & rigor: How conceptual frameworks guide research . Los Angeles, CA: Sage. [ Google Scholar ]
- Reeves, T. D., Marbach-Ad, G., Miller, K. R., Ridgway, J., Gardner, G. E., Schussler, E. E., Wischusen, E. W. (2016). A conceptual framework for graduate teaching assistant professional development evaluation and research . CBE—Life Sciences Education , 15 ( 2 ), es2. https://doi.org/10.1187/cbe.15-10-0225 [ PMC free article ] [ PubMed ] [ Google Scholar ]
- Reynolds, J. A., Thaiss, C., Katkin, W., Thompson, R. J. Jr. (2012). Writing-to-learn in undergraduate science education: A community-based, conceptually driven approach . CBE—Life Sciences Education , 11 ( 1 ), 17–25. https://doi.org/10.1187/cbe.11-08-0064 [ PMC free article ] [ PubMed ] [ Google Scholar ]
- Rocco, T. S., Plakhotnik, M. S. (2009). Literature reviews, conceptual frameworks, and theoretical frameworks: Terms, functions, and distinctions . Human Resource Development Review , 8 ( 1 ), 120–130. https://doi.org/10.1177/1534484309332617 [ Google Scholar ]
- Rodrigo-Peiris, T., Xiang, L., Cassone, V. M. (2018). A low-intensity, hybrid design between a “traditional” and a “course-based” research experience yields positive outcomes for science undergraduate freshmen and shows potential for large-scale application . CBE—Life Sciences Education , 17 ( 4 ), ar53. https://doi.org/10.1187/cbe.17-11-0248 [ PMC free article ] [ PubMed ] [ Google Scholar ]
- Sabel, J. L., Dauer, J. T., Forbes, C. T. (2017). Introductory biology students’ use of enhanced answer keys and reflection questions to engage in metacognition and enhance understanding . CBE—Life Sciences Education , 16 ( 3 ), ar40. https://doi.org/10.1187/cbe.16-10-0298 [ PMC free article ] [ PubMed ] [ Google Scholar ]
- Sbeglia, G. C., Goodridge, J. A., Gordon, L. H., Nehm, R. H. (2021). Are faculty changing? How reform frameworks, sampling intensities, and instrument measures impact inferences about student-centered teaching practices . CBE—Life Sciences Education , 20 ( 3 ), ar39. https://doi.org/10.1187/cbe.20-11-0259 [ PMC free article ] [ PubMed ] [ Google Scholar ]
- Schwandt, T. A. (2000). Three epistemological stances for qualitative inquiry: Interpretivism, hermeneutics, and social constructionism . In Denzin, N. K., Lincoln, Y. S. (Eds.), Handbook of qualitative research (2nd ed., pp. 189–213). Los Angeles, CA: Sage. [ Google Scholar ]
- Sickel, A. J., Friedrichsen, P. (2013). Examining the evolution education literature with a focus on teachers: Major findings, goals for teacher preparation, and directions for future research . Evolution: Education and Outreach , 6 ( 1 ), 23. https://doi.org/10.1186/1936-6434-6-23 [ Google Scholar ]
- Singer, S. R., Nielsen, N. R., Schweingruber, H. A. (2012). Discipline-based education research: Understanding and improving learning in undergraduate science and engineering . Washington, DC: National Academies Press. [ Google Scholar ]
- Todd, A., Romine, W. L., Correa-Menendez, J. (2019). Modeling the transition from a phenotypic to genotypic conceptualization of genetics in a university-level introductory biology context . Research in Science Education , 49 ( 2 ), 569–589. https://doi.org/10.1007/s11165-017-9626-2 [ Google Scholar ]
- Vygotsky, L. S. (1978). Mind in society: The development of higher psychological processes . Cambridge, MA: Harvard University Press. [ Google Scholar ]
- Wenger, E. (1998). Communities of practice: Learning as a social system . Systems Thinker , 9 ( 5 ), 2–3. [ Google Scholar ]
- Ziadie, M. A., Andrews, T. C. (2018). Moving evolution education forward: A systematic analysis of literature to identify gaps in collective knowledge for teaching . CBE—Life Sciences Education , 17 ( 1 ), ar11. https://doi.org/10.1187/cbe.17-08-0190 [ PMC free article ] [ PubMed ] [ Google Scholar ]

How to... Conduct empirical research
Share this content
Empirical research is research that is based on observation and measurement of phenomena, as directly experienced by the researcher. The data thus gathered may be compared against a theory or hypothesis, but the results are still based on real life experience. The data gathered is all primary data, although secondary data from a literature review may form the theoretical background.
On this page
What is empirical research, the research question, the theoretical framework, sampling techniques, design of the research.
- Methods of empirical research
- Techniques of data collection & analysis
- Reporting the findings of empirical research
- Further information
Typically, empirical research embodies the following elements:
- A research question , which will determine research objectives.
- A particular and planned design for the research, which will depend on the question and which will find ways of answering it with appropriate use of resources.
- The gathering of primary data , which is then analysed.
- A particular methodology for collecting and analysing the data, such as an experiment or survey.
- The limitation of the data to a particular group, area or time scale, known as a sample: for example, a specific number of employees of a particular company type, or all users of a library over a given time scale. The sample should be somehow representative of a wider population.
- The ability to recreate the study and test the results. This is known as reliability .
- The ability to generalise from the findings to a larger sample and to other situations.
The starting point for your research should be your research question. This should be a formulation of the issue which is at the heart of the area which you are researching, which has the right degree of breadth and depth to make the research feasible within your resources. The following points are useful to remember when coming up with your research question, or RQ:
- your doctoral thesis;
- reading the relevant literature in journals, especially literature reviews which are good at giving an overview, and spotting interesting conceptual developments;
- looking at research priorities of funding bodies, professional institutes etc.;
- going to conferences;
- looking out for calls for papers;
- developing a dialogue with other researchers in your area.
- To narrow down your research topic, brainstorm ideas around it, possibly with your colleagues if you have decided to collaborate, noting all the questions down.
- Come up with a "general focus" question; then develop some other more specific ones.
- they are not too broad;
- they are not so narrow as to yield uninteresting results;
- will the research entailed be covered by your resources, i.e. will you have sufficient time and money;
- there is sufficient background literature on the topic;
- you can carry out appropriate field research;
- you have stated your question in the simplest possible way.
Let's look at some examples:
Bisking et al. examine whether or not gender has an influence on disciplinary action in their article Does the sex of the leader and subordinate influence a leader's disciplinary decisions? ( Management Decision , Volume 41 Number 10) and come up with the following series of inter-related questions:
- Given the same infraction, would a male leader impose the same disciplinary action on male and female subordinates?
- Given the same infraction, would a female leader impose the same disciplinary action on male and female subordinates?
- Given the same infraction, would a female leader impose the same disciplinary action on female subordinates as a male leader would on male subordinates?
- Given the same infraction, would a female leader impose the same disciplinary action on male subordinates as a male leader would on female subordinates?
- Given the same infraction, would a male and female leader impose the same disciplinary action on male subordinates?
- Given the same infraction, would a male and female leader impose the same disciplinary action on female subordinates?
- Do female and male leaders impose the same discipline on subordinates regardless of the type of infraction?
- Is it possible to predict how female and male leaders will impose disciplinary actions based on their respective BSRI femininity and masculinity scores?
Motion et al. examined co-branding in Equity in Corporate Co-branding ( European Journal of Marketing , Volume 37 Number 7/8) and came up with the following RQs:
RQ1: What objectives underpinned the corporate brand?
RQ2: How were brand values deployed to establish the corporate co-brand within particular discourse contexts?
RQ3: How was the desired rearticulation promoted to shareholders?
RQ4: What are the sources of corporate co-brand equity?
Note, the above two examples state the RQs very explicitly; sometimes the RQ is implicit:
Qun G. Jiao, Anthony J. Onwuegbuzie are library researchers who examined the question: "What is the relationship between library anxiety and social interdependence?" in a number of articles, see Dimensions of library anxiety and social interdependence: implications for library services ( Library Review , Volume 51 Number 2).
Or sometimes the RQ is stated as a general objective:
Ying Fan describes outsourcing in British companies in Strategic outsourcing: evidence from British companies ( Marketing Intelligence & Planning , Volume 18 Number 4) and states his research question as an objective:
The main objective of the research was to explore the two key areas in the outsourcing process, namely:
- pre-outsourcing decision process; and
- post-outsourcing supplier management.
or as a proposition:
Karin Klenke explores issues of gender in management decisions in Gender influences in decision-making processes in top management teams ( Management Decision , Volume 41 Number 10).
Given the exploratory nature of this research, no specific hypotheses were formulated. Instead, the following general propositions are postulated:
P1. Female and male members of TMTs exercise different types of power in the strategic decision making process.
P2. Female and male members of TMTs differ in the extent in which they employ political savvy in the strategic decision making process.
P3. Male and female members of TMTs manage conflict in strategic decision making situations differently.
P4. Female and male members of TMTs utilise different types of trust in the decision making process.
Sometimes, the theoretical underpinning (see next section) of the research leads you to formulate a hypothesis rather than a question:
Martin et al. explored the effect of fast-forwarding of ads (called zipping) in Remote control marketing: how ad fast-forwarding and ad repetition affect consumers ( Marketing Intelligence & Planning , Volume 20 Number 1) and his research explores the following hypotheses:
The influence of zipping H1. Individuals viewing advertisements played at normal speed will exhibit higher ad recall and recognition than those who view zipped advertisements.
Ad repetition effects H2. Individuals viewing a repeated advertisement will exhibit higher ad recall and recognition than those who see an advertisement once.
Zipping and ad repetition H3. Individuals viewing zipped, repeated advertisements will exhibit higher ad recall and recognition than those who see a normal speed advertisement that is played once.
Empirical research is not divorced from theoretical considerations; and a consideration of theory should form one of the starting points of your research. This applies particularly in the case of management research which by its very nature is practical and applied to the real world. The link between research and theory is symbiotic: theory should inform research, and the findings of research should inform theory.
There are a number of different theoretical perspectives; if you are unfamiliar with them, we suggest that you look at any good research methods textbook for a full account (see Further information), but this page will contain notes on the following:
This is the approach of the natural sciences, emphasising total objectivity and independence on the part of the researcher, a highly scientific methodology, with data being collected in a value-free manner and using quantitative techniques with some statistical measures of analysis. Assumes that there are 'independent facts' in the social world as in the natural world. The object is to generalise from what has been observed and hence add to the body of theory.
Very similar to positivism in that it has a strong reliance on objectivity and quantitative methods of data collection, but with less of a reliance on theory. There is emphasis on data and facts in their own right; they do not need to be linked to theory.
Interpretivism
This view criticises positivism as being inappropriate for the social world of business and management which is dominated by people rather than the laws of nature and hence has an inevitable subjective element as people will have different interpretations of situations and events. The business world can only be understood through people's interpretation. This view is more likely to emphasise qualitative methods such as participant observation, focus groups and semi-structured interviewing.
typically use | typically use |
are | are |
involve the researcher as ideally an | require more and on the part of the researcher. |
may focus on cause and effect. | focuses on understanding of phenomena in their social, institutional, political and economic context. |
require a hypothesis. | require a |
have the that they may force people into categories, also it cannot go into much depth about subjects and issues. | have the that they focus on a few individuals, and may therefore be difficult to generalise. |
While reality exists independently of human experience, people are not like objects in the natural world but are subject to social influences and processes. Like empiricism and positivism , this emphasises the importance of explanation, but is also concerned with the social world and with its underlying structures.
Inductive and deductive approaches
At what point in your research you bring in a theoretical perspective will depend on whether you choose an:
- Inductive approach – collect the data, then develop the theory.
- Deductive approach – assume a theoretical position then test it against the data.
is more usually linked with an approach. | is more usually linked with the approach. |
is more likely to use qualitative methods, such as interviewing, observation etc., with a more flexible structure. | is more likely to use quantitative methods, such as experiments, questionnaires etc., and a highly structured methodology with controls. |
does not simply look at cause and effect, but at people's perceptions of events, and at the context of the research. | is the more scientific method, concerned with cause and effect, and the relationship between variables. |
builds theory after collection of the data. | starts from a theoretical perspective, and develops a hypothesis which is tested against the data. |
is more likely to use an in-depth study of a smaller sample. | is more likely to use a larger sample. |
is less likely to be concerned with generalisation (a danger is that no patterns emerge). | is concerned with generalisation. |
tresses the researcher involvement. | stresses the independence of the researcher. |
It should be emphasised that none of the above approaches are mutually exclusive and can be used in combination.
Sampling may be done either:
- On a random basis – a given number is selected completely at random.
- On a systematic basis – every n th element of the population is selected.
- On a stratified random basis – the population is divided into segments, for example, in a University, you could divide the population into academic, administrators, and academic related. A random number of each group is then selected.
- On a cluster basis – a particular subgroup is chosen at random.
- Convenience – being present at a particular time e.g. at lunch in the canteen.
- Purposive – people can be selected deliberately because their views are relevant to the issue concerned.
- Quota – the assumption is made that there are subgroups in the population, and a quota of respondents is chosen to reflect this diversity.
Useful articles
Richard Laughlin in Empirical research in accounting: alternative approaches and a case for "middle-range" thinking provides an interesting general overview of the different perspectives on theory and methodology as applied to accounting. ( Accounting, Auditing & Accountability Journal, Volume 8 Number 1).
D. Tranfield and K. Starkey in The Nature, Social Organization and Promotion of Management Research: Towards Policy look at the relationship between theory and practice in management research, and develop a number of analytical frameworks, including looking at Becher's conceptual schema for disciplines and Gibbons et al.'s taxonomy of knowledge production systems. ( British Journal of Management , vol. 9, no. 4 – abstract only).
Research design is about how you go about answering your question: what strategy you adopt, and what methods do you use to achieve your results. In particular you should ask yourself...
There's a lot more to this article; just fill in the form below to instantly see the complete content.
Read the complete article
What's in the rest?
- Continuation of 'Design of the research'
- Books & websites for further information
Your data will be used, alongside feedback we may request, only to help inform and improve our 'How to' section – thank you.

Empirical Research: What is empirical research?
What is empirical research.
- How do I find empirical research in databases?
- What does empirical research look like?
- How is empirical research conducted?
- What is Empirical Research?
- How do I Find Empirical Research in Databases?
- How is Empirical Research Conducted?
Ask a Librarian
Contact the reference desk.
[email protected] | |
(603) 556-8883 | |
(603) 641-7306 | |
Empirical research is based on observed and measured phenomena and derives knowledge from actual experience rather than from theory or belief.
How do you know if a study is empirical? Read the subheadings within the article, book, or report and look for a description of the research "methodology." Ask yourself: Could I recreate this study and test these results?
Key characteristics to look for:
- Specific research questions to be answered
- Definition of the population, behavior, or phenomena being studied
- Description of the process used to study this population or phenomena, including selection criteria, controls, and testing instruments (such as surveys)
Another hint: some scholarly journals use a specific layout, called the "IMRaD" format, to communicate empirical research findings. Such articles typically have 4 components:
- Introduction : sometimes called "literature review" -- what is currently known about the topic -- usually includes a theoretical framework and/or discussion of previous studies
- Methodology: sometimes called "research design" -- how to recreate the study -- usually describes the population, research process, and analytical tools
- Results : sometimes called "findings" -- what was learned through the study -- usually appears as statistical data or as substantial quotations from research participants
- Discussion : sometimes called "conclusion" or "implications" -- why the study is important -- usually describes how the research results influence professional practices or future studies
What about when research is not empirical?
Many humanities scholars do not use empirical methods. if you are looking for empirical articles in one of these subject areas, try including keywords like:.
- quantitative
- qualitative
Also, look for opportunities to narrow your search to scholarly, academic, or peer-reviewed journals articles in the database.
Adapted from " Research Methods: Finding Empirical Articles " by Jill Anderson at Georgia State University Library.
See the complete A-Z databases list for more resources
The primary content of this guide was originally created by Ellysa Cahoy at Penn State Libraries .
- Next: How do I find empirical research in databases? >>
- Last Updated: Apr 12, 2024 8:07 AM
- URL: https://geiselguides.anselm.edu/Empirical-Research
- Connelly Library
Qualitative and Quantitative Research
What is "empirical research".
- empirical research
- Locating Articles in Cinahl and PsycInfo
- Locating Articles in PubMed
- Getting the Articles
Empirical research is based on observed and measured phenomena and derives knowledge from actual experience rather than from theory or belief.
How do you know if a study is empirical? Read the subheadings within the article, book, or report and look for a description of the research "methodology." Ask yourself: Could I recreate this study and test these results?
Key characteristics to look for:
- Specific research questions to be answered
- Definition of the population, behavior, or phenomena being studied
- Description of the process used to study this population or phenomena, including selection criteria, controls, and testing instruments (such as surveys)
Another hint: some scholarly journals use a specific layout, called the "IMRaD" format, to communicate empirical research findings. Such articles typically have 4 components:
- Introduction : sometimes called "literature review" -- what is currently known about the topic -- usually includes a theoretical framework and/or discussion of previous studies
- Methodology: sometimes called "research design" -- how to recreate the study -- usually describes the population, research process, and analytical tools
- Results : sometimes called "findings" -- what was learned through the study -- usually appears as statistical data or as substantial quotations from research participants
- Discussion : sometimes called "conclusion" or "implications" -- why the study is important -- usually describes how the research results influence professional practices or future studies
- << Previous: Home
- Next: Locating Articles in Cinahl and PsycInfo >>

© Copyright La Salle University. All rights reserved.
Colorado College
Research guides.
Tutt Library Research Guides

- Find Articles
- Search Tips
- Empirical v. Theoretical
- Credibility
- Position Papers
- Curricular Education Databases
- Statistics & Data
- Streaming Films & DVDs
- Print Books
- Professional Development & Careers
- Citation Guide This link opens in a new window
- ED 101 Information
Empirical or Theoretical?
Empirical: Based on data gathered by original experiments or observations.
Theoretical: Analyzes and makes connections between empirical studies to define or advance a theoretical position.
Videos on Finding Empirical Articles
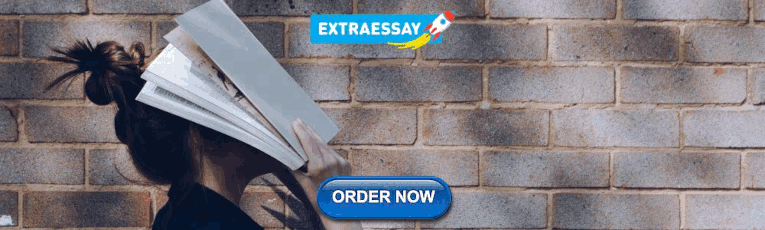
Where Can I Find Empirically-Based Education Articles?

The most direct route is to search PsycInfo, linked above.
This will take you to the Advanced Search, where you can type in your key words at the top. Then scroll down through all the limiting options to the Methodology menu. Select Empirical Study.

In other databases without the Methodology limiter, such as Education Source , try keywords like empirical , study , and research .
How Can I Tell if an Article is Empirical?
Check for these components:
- Peer-reviewed
- Charts, graphs, tables, and/or statistical analyses
- More than 5 pages
- Sections with names like: Abstract, Introduction, Literature Review, Method, Data, Analysis, Results, Discussion, References
Look for visual cues of data collection and analysis:

- << Previous: Search Tips
- Next: Credibility >>
- Last Updated: Mar 27, 2024 10:27 AM
- URL: https://coloradocollege.libguides.com/education
- USC Libraries
- Research Guides
Organizing Your Social Sciences Research Paper
- Theoretical Framework
- Purpose of Guide
- Design Flaws to Avoid
- Independent and Dependent Variables
- Glossary of Research Terms
- Reading Research Effectively
- Narrowing a Topic Idea
- Broadening a Topic Idea
- Extending the Timeliness of a Topic Idea
- Academic Writing Style
- Applying Critical Thinking
- Choosing a Title
- Making an Outline
- Paragraph Development
- Research Process Video Series
- Executive Summary
- The C.A.R.S. Model
- Background Information
- The Research Problem/Question
- Citation Tracking
- Content Alert Services
- Evaluating Sources
- Primary Sources
- Secondary Sources
- Tiertiary Sources
- Scholarly vs. Popular Publications
- Qualitative Methods
- Quantitative Methods
- Insiderness
- Using Non-Textual Elements
- Limitations of the Study
- Common Grammar Mistakes
- Writing Concisely
- Avoiding Plagiarism
- Footnotes or Endnotes?
- Further Readings
- Generative AI and Writing
- USC Libraries Tutorials and Other Guides
- Bibliography
Theories are formulated to explain, predict, and understand phenomena and, in many cases, to challenge and extend existing knowledge within the limits of critical bounded assumptions or predictions of behavior. The theoretical framework is the structure that can hold or support a theory of a research study. The theoretical framework encompasses not just the theory, but the narrative explanation about how the researcher engages in using the theory and its underlying assumptions to investigate the research problem. It is the structure of your paper that summarizes concepts, ideas, and theories derived from prior research studies and which was synthesized in order to form a conceptual basis for your analysis and interpretation of meaning found within your research.
Abend, Gabriel. "The Meaning of Theory." Sociological Theory 26 (June 2008): 173–199; Kivunja, Charles. "Distinguishing between Theory, Theoretical Framework, and Conceptual Framework: A Systematic Review of Lessons from the Field." International Journal of Higher Education 7 (December 2018): 44-53; Swanson, Richard A. Theory Building in Applied Disciplines . San Francisco, CA: Berrett-Koehler Publishers 2013; Varpio, Lara, Elise Paradis, Sebastian Uijtdehaage, and Meredith Young. "The Distinctions between Theory, Theoretical Framework, and Conceptual Framework." Academic Medicine 95 (July 2020): 989-994.
Importance of Theory and a Theoretical Framework
Theories can be unfamiliar to the beginning researcher because they are rarely applied in high school social studies curriculum and, as a result, can come across as unfamiliar and imprecise when first introduced as part of a writing assignment. However, in their most simplified form, a theory is simply a set of assumptions or predictions about something you think will happen based on existing evidence and that can be tested to see if those outcomes turn out to be true. Of course, it is slightly more deliberate than that, therefore, summarized from Kivunja (2018, p. 46), here are the essential characteristics of a theory.
- It is logical and coherent
- It has clear definitions of terms or variables, and has boundary conditions [i.e., it is not an open-ended statement]
- It has a domain where it applies
- It has clearly described relationships among variables
- It describes, explains, and makes specific predictions
- It comprises of concepts, themes, principles, and constructs
- It must have been based on empirical data [i.e., it is not a guess]
- It must have made claims that are subject to testing, been tested and verified
- It must be clear and concise
- Its assertions or predictions must be different and better than those in existing theories
- Its predictions must be general enough to be applicable to and understood within multiple contexts
- Its assertions or predictions are relevant, and if applied as predicted, will result in the predicted outcome
- The assertions and predictions are not immutable, but subject to revision and improvement as researchers use the theory to make sense of phenomena
- Its concepts and principles explain what is going on and why
- Its concepts and principles are substantive enough to enable us to predict a future
Given these characteristics, a theory can best be understood as the foundation from which you investigate assumptions or predictions derived from previous studies about the research problem, but in a way that leads to new knowledge and understanding as well as, in some cases, discovering how to improve the relevance of the theory itself or to argue that the theory is outdated and a new theory needs to be formulated based on new evidence.
A theoretical framework consists of concepts and, together with their definitions and reference to relevant scholarly literature, existing theory that is used for your particular study. The theoretical framework must demonstrate an understanding of theories and concepts that are relevant to the topic of your research paper and that relate to the broader areas of knowledge being considered.
The theoretical framework is most often not something readily found within the literature . You must review course readings and pertinent research studies for theories and analytic models that are relevant to the research problem you are investigating. The selection of a theory should depend on its appropriateness, ease of application, and explanatory power.
The theoretical framework strengthens the study in the following ways :
- An explicit statement of theoretical assumptions permits the reader to evaluate them critically.
- The theoretical framework connects the researcher to existing knowledge. Guided by a relevant theory, you are given a basis for your hypotheses and choice of research methods.
- Articulating the theoretical assumptions of a research study forces you to address questions of why and how. It permits you to intellectually transition from simply describing a phenomenon you have observed to generalizing about various aspects of that phenomenon.
- Having a theory helps you identify the limits to those generalizations. A theoretical framework specifies which key variables influence a phenomenon of interest and highlights the need to examine how those key variables might differ and under what circumstances.
- The theoretical framework adds context around the theory itself based on how scholars had previously tested the theory in relation their overall research design [i.e., purpose of the study, methods of collecting data or information, methods of analysis, the time frame in which information is collected, study setting, and the methodological strategy used to conduct the research].
By virtue of its applicative nature, good theory in the social sciences is of value precisely because it fulfills one primary purpose: to explain the meaning, nature, and challenges associated with a phenomenon, often experienced but unexplained in the world in which we live, so that we may use that knowledge and understanding to act in more informed and effective ways.
The Conceptual Framework. College of Education. Alabama State University; Corvellec, Hervé, ed. What is Theory?: Answers from the Social and Cultural Sciences . Stockholm: Copenhagen Business School Press, 2013; Asher, Herbert B. Theory-Building and Data Analysis in the Social Sciences . Knoxville, TN: University of Tennessee Press, 1984; Drafting an Argument. Writing@CSU. Colorado State University; Kivunja, Charles. "Distinguishing between Theory, Theoretical Framework, and Conceptual Framework: A Systematic Review of Lessons from the Field." International Journal of Higher Education 7 (2018): 44-53; Omodan, Bunmi Isaiah. "A Model for Selecting Theoretical Framework through Epistemology of Research Paradigms." African Journal of Inter/Multidisciplinary Studies 4 (2022): 275-285; Ravitch, Sharon M. and Matthew Riggan. Reason and Rigor: How Conceptual Frameworks Guide Research . Second edition. Los Angeles, CA: SAGE, 2017; Trochim, William M.K. Philosophy of Research. Research Methods Knowledge Base. 2006; Jarvis, Peter. The Practitioner-Researcher. Developing Theory from Practice . San Francisco, CA: Jossey-Bass, 1999.
Strategies for Developing the Theoretical Framework
I. Developing the Framework
Here are some strategies to develop of an effective theoretical framework:
- Examine your thesis title and research problem . The research problem anchors your entire study and forms the basis from which you construct your theoretical framework.
- Brainstorm about what you consider to be the key variables in your research . Answer the question, "What factors contribute to the presumed effect?"
- Review related literature to find how scholars have addressed your research problem. Identify the assumptions from which the author(s) addressed the problem.
- List the constructs and variables that might be relevant to your study. Group these variables into independent and dependent categories.
- Review key social science theories that are introduced to you in your course readings and choose the theory that can best explain the relationships between the key variables in your study [note the Writing Tip on this page].
- Discuss the assumptions or propositions of this theory and point out their relevance to your research.
A theoretical framework is used to limit the scope of the relevant data by focusing on specific variables and defining the specific viewpoint [framework] that the researcher will take in analyzing and interpreting the data to be gathered. It also facilitates the understanding of concepts and variables according to given definitions and builds new knowledge by validating or challenging theoretical assumptions.
II. Purpose
Think of theories as the conceptual basis for understanding, analyzing, and designing ways to investigate relationships within social systems. To that end, the following roles served by a theory can help guide the development of your framework.
- Means by which new research data can be interpreted and coded for future use,
- Response to new problems that have no previously identified solutions strategy,
- Means for identifying and defining research problems,
- Means for prescribing or evaluating solutions to research problems,
- Ways of discerning certain facts among the accumulated knowledge that are important and which facts are not,
- Means of giving old data new interpretations and new meaning,
- Means by which to identify important new issues and prescribe the most critical research questions that need to be answered to maximize understanding of the issue,
- Means of providing members of a professional discipline with a common language and a frame of reference for defining the boundaries of their profession, and
- Means to guide and inform research so that it can, in turn, guide research efforts and improve professional practice.
Adapted from: Torraco, R. J. “Theory-Building Research Methods.” In Swanson R. A. and E. F. Holton III , editors. Human Resource Development Handbook: Linking Research and Practice . (San Francisco, CA: Berrett-Koehler, 1997): pp. 114-137; Jacard, James and Jacob Jacoby. Theory Construction and Model-Building Skills: A Practical Guide for Social Scientists . New York: Guilford, 2010; Ravitch, Sharon M. and Matthew Riggan. Reason and Rigor: How Conceptual Frameworks Guide Research . Second edition. Los Angeles, CA: SAGE, 2017; Sutton, Robert I. and Barry M. Staw. “What Theory is Not.” Administrative Science Quarterly 40 (September 1995): 371-384.
Structure and Writing Style
The theoretical framework may be rooted in a specific theory , in which case, your work is expected to test the validity of that existing theory in relation to specific events, issues, or phenomena. Many social science research papers fit into this rubric. For example, Peripheral Realism Theory, which categorizes perceived differences among nation-states as those that give orders, those that obey, and those that rebel, could be used as a means for understanding conflicted relationships among countries in Africa. A test of this theory could be the following: Does Peripheral Realism Theory help explain intra-state actions, such as, the disputed split between southern and northern Sudan that led to the creation of two nations?
However, you may not always be asked by your professor to test a specific theory in your paper, but to develop your own framework from which your analysis of the research problem is derived . Based upon the above example, it is perhaps easiest to understand the nature and function of a theoretical framework if it is viewed as an answer to two basic questions:
- What is the research problem/question? [e.g., "How should the individual and the state relate during periods of conflict?"]
- Why is your approach a feasible solution? [i.e., justify the application of your choice of a particular theory and explain why alternative constructs were rejected. I could choose instead to test Instrumentalist or Circumstantialists models developed among ethnic conflict theorists that rely upon socio-economic-political factors to explain individual-state relations and to apply this theoretical model to periods of war between nations].
The answers to these questions come from a thorough review of the literature and your course readings [summarized and analyzed in the next section of your paper] and the gaps in the research that emerge from the review process. With this in mind, a complete theoretical framework will likely not emerge until after you have completed a thorough review of the literature .
Just as a research problem in your paper requires contextualization and background information, a theory requires a framework for understanding its application to the topic being investigated. When writing and revising this part of your research paper, keep in mind the following:
- Clearly describe the framework, concepts, models, or specific theories that underpin your study . This includes noting who the key theorists are in the field who have conducted research on the problem you are investigating and, when necessary, the historical context that supports the formulation of that theory. This latter element is particularly important if the theory is relatively unknown or it is borrowed from another discipline.
- Position your theoretical framework within a broader context of related frameworks, concepts, models, or theories . As noted in the example above, there will likely be several concepts, theories, or models that can be used to help develop a framework for understanding the research problem. Therefore, note why the theory you've chosen is the appropriate one.
- The present tense is used when writing about theory. Although the past tense can be used to describe the history of a theory or the role of key theorists, the construction of your theoretical framework is happening now.
- You should make your theoretical assumptions as explicit as possible . Later, your discussion of methodology should be linked back to this theoretical framework.
- Don’t just take what the theory says as a given! Reality is never accurately represented in such a simplistic way; if you imply that it can be, you fundamentally distort a reader's ability to understand the findings that emerge. Given this, always note the limitations of the theoretical framework you've chosen [i.e., what parts of the research problem require further investigation because the theory inadequately explains a certain phenomena].
The Conceptual Framework. College of Education. Alabama State University; Conceptual Framework: What Do You Think is Going On? College of Engineering. University of Michigan; Drafting an Argument. Writing@CSU. Colorado State University; Lynham, Susan A. “The General Method of Theory-Building Research in Applied Disciplines.” Advances in Developing Human Resources 4 (August 2002): 221-241; Tavallaei, Mehdi and Mansor Abu Talib. "A General Perspective on the Role of Theory in Qualitative Research." Journal of International Social Research 3 (Spring 2010); Ravitch, Sharon M. and Matthew Riggan. Reason and Rigor: How Conceptual Frameworks Guide Research . Second edition. Los Angeles, CA: SAGE, 2017; Reyes, Victoria. Demystifying the Journal Article. Inside Higher Education; Trochim, William M.K. Philosophy of Research. Research Methods Knowledge Base. 2006; Weick, Karl E. “The Work of Theorizing.” In Theorizing in Social Science: The Context of Discovery . Richard Swedberg, editor. (Stanford, CA: Stanford University Press, 2014), pp. 177-194.
Writing Tip
Borrowing Theoretical Constructs from Other Disciplines
An increasingly important trend in the social and behavioral sciences is to think about and attempt to understand research problems from an interdisciplinary perspective. One way to do this is to not rely exclusively on the theories developed within your particular discipline, but to think about how an issue might be informed by theories developed in other disciplines. For example, if you are a political science student studying the rhetorical strategies used by female incumbents in state legislature campaigns, theories about the use of language could be derived, not only from political science, but linguistics, communication studies, philosophy, psychology, and, in this particular case, feminist studies. Building theoretical frameworks based on the postulates and hypotheses developed in other disciplinary contexts can be both enlightening and an effective way to be more engaged in the research topic.
CohenMiller, A. S. and P. Elizabeth Pate. "A Model for Developing Interdisciplinary Research Theoretical Frameworks." The Qualitative Researcher 24 (2019): 1211-1226; Frodeman, Robert. The Oxford Handbook of Interdisciplinarity . New York: Oxford University Press, 2010.
Another Writing Tip
Don't Undertheorize!
Do not leave the theory hanging out there in the introduction never to be mentioned again. Undertheorizing weakens your paper. The theoretical framework you describe should guide your study throughout the paper. Be sure to always connect theory to the review of pertinent literature and to explain in the discussion part of your paper how the theoretical framework you chose supports analysis of the research problem or, if appropriate, how the theoretical framework was found to be inadequate in explaining the phenomenon you were investigating. In that case, don't be afraid to propose your own theory based on your findings.
Yet Another Writing Tip
What's a Theory? What's a Hypothesis?
The terms theory and hypothesis are often used interchangeably in newspapers and popular magazines and in non-academic settings. However, the difference between theory and hypothesis in scholarly research is important, particularly when using an experimental design. A theory is a well-established principle that has been developed to explain some aspect of the natural world. Theories arise from repeated observation and testing and incorporates facts, laws, predictions, and tested assumptions that are widely accepted [e.g., rational choice theory; grounded theory; critical race theory].
A hypothesis is a specific, testable prediction about what you expect to happen in your study. For example, an experiment designed to look at the relationship between study habits and test anxiety might have a hypothesis that states, "We predict that students with better study habits will suffer less test anxiety." Unless your study is exploratory in nature, your hypothesis should always explain what you expect to happen during the course of your research.
The key distinctions are:
- A theory predicts events in a broad, general context; a hypothesis makes a specific prediction about a specified set of circumstances.
- A theory has been extensively tested and is generally accepted among a set of scholars; a hypothesis is a speculative guess that has yet to be tested.
Cherry, Kendra. Introduction to Research Methods: Theory and Hypothesis. About.com Psychology; Gezae, Michael et al. Welcome Presentation on Hypothesis. Slideshare presentation.
Still Yet Another Writing Tip
Be Prepared to Challenge the Validity of an Existing Theory
Theories are meant to be tested and their underlying assumptions challenged; they are not rigid or intransigent, but are meant to set forth general principles for explaining phenomena or predicting outcomes. Given this, testing theoretical assumptions is an important way that knowledge in any discipline develops and grows. If you're asked to apply an existing theory to a research problem, the analysis will likely include the expectation by your professor that you should offer modifications to the theory based on your research findings.
Indications that theoretical assumptions may need to be modified can include the following:
- Your findings suggest that the theory does not explain or account for current conditions or circumstances or the passage of time,
- The study reveals a finding that is incompatible with what the theory attempts to explain or predict, or
- Your analysis reveals that the theory overly generalizes behaviors or actions without taking into consideration specific factors revealed from your analysis [e.g., factors related to culture, nationality, history, gender, ethnicity, age, geographic location, legal norms or customs , religion, social class, socioeconomic status, etc.].
Philipsen, Kristian. "Theory Building: Using Abductive Search Strategies." In Collaborative Research Design: Working with Business for Meaningful Findings . Per Vagn Freytag and Louise Young, editors. (Singapore: Springer Nature, 2018), pp. 45-71; Shepherd, Dean A. and Roy Suddaby. "Theory Building: A Review and Integration." Journal of Management 43 (2017): 59-86.
- << Previous: The Research Problem/Question
- Next: 5. The Literature Review >>
- Last Updated: Jul 3, 2024 10:07 AM
- URL: https://libguides.usc.edu/writingguide

Social Work 3500: Methods of Social Work Research
What is empirical research, sections in empirical research.
- What to Look For
- Is this an Empirical article?
- Finding Empirical Articles
- AYSPS Online Students This link opens in a new window
- Library Services
- Citing Your Sources This link opens in a new window
- Contact Me / Online Drop-in Hours
Empirical Research is defined as research based on observed and measured phenomena. It is research that derives knowledge from actual experience rather than from theory or belief.
Requests for "Empirical" articles are usually from instructors in Education or Psychology; most other disciplines will ask for Scholarly, Peer Reviewed or Primary literature.
See if the article mentions a study, an observation, an analysis or a number of participants or subjects. Was data collected, a survey or questionnaire administered, an assessment or measurement used, an interview conducted? All of these terms indicate possible methodologies used in empirical research.
Empirical articles often contain these sections:
- Introduction
- Literature review
- Methodology
The sections may be combined, and may have different headings or no headings at all; however, the information that would fall within these sections should be present in an empirical article.
Abstract: A report of an empirical study includes an abstract that provides a very brief summary of the research.
Introduction: The introduction sets the research in a context, which provides a review of related research and develops the hypotheses for the research.
Method: The method section is a description of how the research was conducted, including who the participants were, the design of the study, what the participants did, and what measures were used.
Results: The results section describes the outcomes of the measures of the study.
Discussion : The discussion section contains the interpretations and implications of the study.
General Discussion: There may be more than one study in the report; in this case, there are usually separate Methods and Results sections for each study followed by a general discussion that ties all the research together.
References: A references section contains information about the articles and books cited in the report.
Length of Article: Empirical research articles are usually substantial (more than 1 or 2 pages) and include a bibliography or cited references section (usually at the end of the article).
Type of Publication: Empirical research articles are published in scholarly or academic journals . These publications are sometimes referred to as “peer-reviewed,” “academic” or “refereed” publications. Examples of such publications include: Social Work Research, Mental Health Practice, and Journal of Substance Abuse.
- << Previous: Welcome
- Next: What to Look For >>
- Last Updated: May 9, 2024 10:26 AM
- URL: https://research.library.gsu.edu/SW3500

Building and Using Theoretical Frameworks
- Open Access
- First Online: 03 December 2022
Cite this chapter
You have full access to this open access chapter
- James Hiebert 6 ,
- Jinfa Cai 7 ,
- Stephen Hwang 7 ,
- Anne K Morris 6 &
- Charles Hohensee 6
Part of the book series: Research in Mathematics Education ((RME))
17k Accesses
Theoretical frameworks can be confounding. They are supposed to be very important, but it is not always clear what they are or why you need them. Using ideas from Chaps. 1 and 2 , we describe them as local theories that are custom-designed for your study. Although they might use parts of larger well-known theories, they are created by individual researchers for particular studies. They are developed through the cyclic process of creating more precise and meaningful hypotheses. Building directly on constructs from the previous chapters, you can think of theoretical frameworks as equivalent to the most compelling, complete rationales you can develop for the predictions you make. Theoretical frameworks are important because they do lots of work for you. They incorporate the literature into your rationale, they explain why your study matters, they suggest how you can best test your predictions, and they help you interpret what you find. Your theoretical framework creates an essential coherence for your study and for the paper you are writing to report the study.
You have full access to this open access chapter, Download chapter PDF
Part I. What Are Theoretical Frameworks?
As the name implies, a theoretical framework is a type of theory. We will define it as the custom-made theory that focuses specifically on the hypotheses you want to test and the research questions you want to answer. It is custom-made for your study because it explains why your predictions are plausible. It does no more and no less. Building directly on Chap. 2 , as you develop more complete rationales for your predictions, you are actually building a theory to support your predictions. Our goal in this chapter is for you to become comfortable with what theoretical frameworks are, with how they relate to the general concept of theory, with what role they play in scientific inquiry, and with why and how to create one for your study.
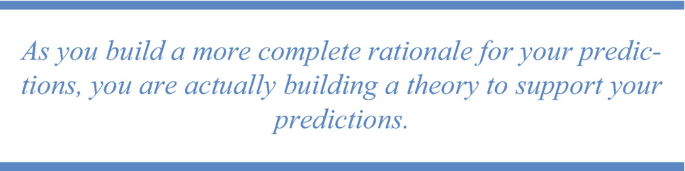
As you read this chapter, it will be helpful to remember that our definitions of terms in this book, such as theoretical framework, are based on our view of scientific inquiry as formulating, testing, and revising hypotheses. We define theoretical framework in ways that continue the coherent story we lay out across all phases of scientific inquiry and all the chapters this book. You are likely to find descriptions of theoretical frameworks in other sources that differ in some ways from our description. In addition, you are likely to see other terms that we would include as synonyms for theoretical framework, including conceptual framework. We suggest that when you encounter these special terms, make sure you understand how the authors are defining them.
|
|
Definitions of Theories
We begin by stepping back and considering how theoretical frameworks fit within the concept of theory, as usually defined. There are many definitions of theory; you can find a huge number simply by googling “theory.” Educational researchers and theorists often propose their own definitions but many of these are quite similar. Praetorius and Charalambous ( 2022 ) reviewed a number of definitions to set the stage for examining theories of teaching. Here are a few, beginning with a dictionary definition:
Lexico.com Dictionary (Oxford University Press, 2021 ): “A supposition or a system of ideas intended to explain something, especially one based on general principles independent of the thing to be explained.”
Biddle and Anderson ( 1986 ): “By scientific theory we mean the system of concepts and propositions that is used to represent, think about, and predict observable events. Within a mature science that theory is also explanatory and formalized. It does not represent ultimate ‘truth,’ however; indeed, it will be superseded by other theories presently. Instead, it represents the best explanation we have, at present, for those events we have so far observed” (p. 241).
Kerlinger ( 1964 ): “A theory is a set of interrelated constructs (concepts), definitions and propositions which presents a systematic view of phenomena by specifying relations among variables, with the purpose of explaining and predicting phenomena” (p. 11).
Colquitt and Zapata-Phelan ( 2007 ): The authors say that theories allow researchers to understand and predict outcomes of interest, describe and explain a process or sequence of events, raise consciousness about a specific set of concepts as well as prevent scholars from “being dazzled by the complexity of the empirical world by providing a linguistic tool for organizing it” (p. 1281).
For our purposes, it is important to notice two things that most definitions of theories share: They are descriptions of a connected set of facts and concepts, and they are created to predict and/or explain observed events. You can connect these ideas to Chaps. 1 and 2 by noticing that the language for the descriptors of scientific inquiry we suggested in Chap. 1 are reflected in the definitions of theories. In particular, notice in the definitions two of the descriptors: “Observing something and trying to explain why it is the way it is” and “Updating everyone’s thinking in response to more and better information.” Notice also in the definitions the emphasis on the elements of a theory similar to the elements of a rationale described in Chap. 2 : definitions, variables, and mechanisms that explain relationships.
Exercise 3.1
Before you continue reading, in your own words, write down a definition for “theoretical framework.”
Theoretical Frameworks Are Local Theories
There are strong similarities between building theories and doing scientific inquiry (formulating, testing, and revising hypotheses). In both cases, the researcher (or theorist) develops explanations for phenomena of interest. Building theories involves describing the concepts and conjectures that predict and later explain the events, and specifying the predictions by identifying the variables that will be measured. Doing scientific inquiry involves many of the same activities: formulating predictions for answers to questions about the research problem and building rationales to explain why the predictions are appropriate and reasonable.
As you move through the cycles described in Chap. 2 —cycles of asking questions, making predictions, writing out the reasons for these predictions, imagining how you would test the predictions, reading more about what scholars know and have hypothesized, revising your predictions (and maybe your questions), and so on—your theoretical rationales will become both more complete and more precise. They will become more complete as you find new arguments and new data in the literature and through talking with others, and they will become sharper as you remove parts of the rationales that originally seemed relevant but now create mostly distractions and noise. They will become increasingly customized local theories that support your predictions.
In the end, your framework should be as clean and frugal as possible without missing arguments or data that are directly relevant. In the language of mathematics, you should use an idea if and only if it makes your framework stronger, more convincing. On the one hand, including more than you need becomes a distraction and can confuse both you, as you try to conceptualize and conduct your research, and others, as they read your reports of your research. On the other hand, including less than you need means your rationale is not yet as convincing as it could be.
The set of rationales, blended together, constitute a precisely targeted custom-made theory that supports your predictions. Custom designing your rationales for your specific predictions means you probably will be drawing ideas from lots of sources and combining them in new ways. You are likely to end up with a unique local theory, a theoretical framework that has not been proposed in exactly the same way before.
A common misconception among beginning researchers is that they should borrow a theoretical framework from somewhere else, especially from well-known scholars who have theories named after them or well-known general theories of learning or teaching. You are likely to use ideas from these theories (e.g., Vygotsky’s theory of learning, Maslow’s theory of motivation, constructivist theories of learning), but you will combine specific ideas from multiple sources to create your own framework. When someone asks, “What theoretical framework are you using?” you would not say, “A Vygotskian framework.” Rather, you would say something like, “I created my framework by combining ideas from different sources so it explains why I am making these predictions.”

You should think of your theoretical framework as a potential contribution to the field, all on its own. Although it is unique to your study, there are elements of your framework that other researchers could draw from to construct theoretical frameworks for their studies, just as you drew from others’ frameworks. In rare cases, other researchers could use your framework as is. This might happen if they want to replicate your study or extend it in very specific ways. Usually, however, researchers borrow parts of frameworks or modify them in ways that better fit their own studies. And, just as you are doing with your own theoretical framework, those researchers will need to justify why borrowing or modifying parts of your framework will help them explain the predictions they are making.
Considering your theoretical framework as a contribution to the field means you should treat it as a central part of scientific inquiry, not just as a required step that must be completed before moving to the next phase. To be useful, the theoretical framework should be constructed as a critical part of conceptualizing and carrying out the research (Cai et al., 2019c ). This also means you should write out your framework as you are developing it. This will be a key part of your evolving research paper. Because your framework will be adjusted multiple times, your written document will go through many drafts.
If you are a graduate student, do not think of the potential audience for your written framework as only your advisor and committee members. Rather, consider your audience to be the larger community of education researchers. You will need to be sure all the key terms are defined and each part of your argument is clear, even to those who are not familiar with your study. This is one place where writing out your framework can benefit your study—it is easy to assume key terms are clear, but then you find out they are not so clear, even to you, when trying to communicate them. Failing to notice this lack of clarity can create lots of problems down the road.
Exercise 3.2
Researchers have used a number of different metaphors to describe theoretical frameworks. Maxwell (2005) referred to a theoretical framework as a “coat closet” that provides “places to ‘hang’ data, showing their relationship to other data,” although he cautioned that “a theory that neatly organizes some data will leave other data disheveled and lying on the floor, with no place to put them” (p. 49). Lester (2005) referred to a framework as a “scaffold” (p. 458), and others have called it a “blueprint” (Grant & Osanloo, 2014). Eisenhart (1991) described the framework as a “skeletal structure of justification” (p. 209). Spangler and Williams (2019) drew an analogy to the role that a house frame provides in preventing the house from collapsing in on itself. What aspects of a theoretical framework does each of these metaphors capture? What aspects does each fail to capture? Which metaphor do you find best fits your definition of a theoretical framework? Why? Can you think of another metaphor to describe a theoretical framework?
Part II. Why Do You Need Theoretical Frameworks?
Theoretical frameworks do lots of work for you. They have four primary purposes. They ensure (1) you have sound reasons to expect your predictions will be accurate, (2) you will craft appropriate methods to test your predictions, (3) you can interpret appropriately what you find, and (4) your interpretations will contribute to the accumulation of a knowledge base that can improve education. How do they do this?
Supporting Your Predictions
In previous chapters and earlier in this chapter, we described how theoretical frameworks are built along with your predictions. In fact, the rationales you develop for convincing others (and yourself) that your predictions are accurate are used to refine your predictions, and vice versa. So, it is not surprising that your refined framework provides a rationale that is fully aligned with your predictions. In fact, you could think of your theoretical framework as your best explanation, at any given moment during scientific inquiry, for why you will find what you think you will find.
Throughout this book, we are using “explanation” in a broad sense. As we noted earlier, an explanation for why your predictions are accurate includes all the concepts and definitions about mechanisms (Kerlinger’s, 1964 definition of “theory”) that help you describe why you think the predictions you are making are the best predictions possible. The explanation also identifies and describes all the variables that make up your predictions, variables that will be measured to test your predictions.
Crafting Appropriate Methods
Critical decisions you make to test your hypotheses form the methods for your scientific inquiry. As we have noted, imagining how you will test your hypotheses helps you decide whether the empirical observations you make can be compared with your predictions or whether you need to revise the methods (or your predictions). Remember, the theoretical framework is the coherent argument built from the rationales you develop as part of each hypothesis you formulate. Because each rationale explains why you make that prediction, it contains helpful cues for which methods would provide the fairest and most complete test of that prediction. In fact, your theoretical framework provides a logic against which you can check every aspect of the methods you imagine using.
You might find it helpful to ask yourself two questions as you think about which methods are best aligned with your theoretical framework. One is, “After reading my theoretical framework, will anyone be surprised by the methods I use?” If so, you should look back at your framework and make sure the predictions are clear and the rationales include all the reasons for your predictions. Your framework should telegraph the methods that make the most sense. The other question is, “Are there some predictions for which I can’t imagine appropriate methods?” If so, we recommend you return to your hypotheses—to your predictions and rationales (theoretical framework)—to make sure the predictions are phrased as precisely as possible and your framework is fully developed. In most cases, this will help you imagine methods that could be used. If not, you might need to revise your hypotheses.
Exercise 3.3
Kerlinger ( 1964 ) stated, “A theory is a set of interrelated constructs (concepts), definitions and propositions which presents a systematic view of phenomena by specifying relations among variables, with the purpose of explaining and predicting phenomena” (p. 11). What role do definitions play in a theoretical framework and how do they help in crafting appropriate methods?
Exercise 3.4
Sarah is in the beginning stages of developing a study. Her initial prediction is: There is a relationship between pedagogical content knowledge and ambitious teaching. She realizes that in order to craft appropriate measures, she needs to develop definitions of these constructs. Sarah’s original definitions are: Pedagogical content knowledge is knowledge about subject matter that is relevant to teaching. Ambitious teaching is teaching that is responsive to students’ thinking and develops a deep knowledge of content. Sarah recognizes that her prediction and her definitions are too broad and too general to work with. She wants to refine the definitions so they can guide the refinement of her prediction and the design of the study. Develop definitions of these two constructs that have clearer implications for the design and that would help Sarah to refine her prediction. (tip: Sarah may need to reduce the scope of her prediction by choosing to focus only on one aspect of pedagogical content knowledge and one aspect of ambitious teaching. Then, she can more precisely define those aspects.)
Guiding Interpretations of the Data
By providing rationales for your predictions, your theoretical framework explains why you think your predictions will be accurate. In education, researchers almost always find that if they make specific predictions (which they should), the predictions are not entirely accurate. This is a consequence of the fact that theoretical frameworks are never complete. Recall the definition of theories from Biddle and Anderson ( 1986 ): A theory “does not represent ultimate ‘truth,’ however; indeed, it will be superseded by other theories presently. Instead, it represents the best explanation we have, at present, for those events we have so far observed” (p. 241). If you have created your best developed and clearly stated theoretical framework that explains why you expected certain results, you can focus your interpretation on the ways in which your theoretical framework should be revised.
Focusing on realigning your theoretical framework with the data you collected produces the richest interpretation of your results. And it prevents you from making one of the most common errors of beginning researchers (and veteran researchers, as well): claiming that your results say more than they really do. Without this anchor to ground your interpretation of the data, it is easy to overgeneralize and make claims that go beyond the evidence.
In one of the definitions of theory presented earlier, Colquitt and Zapata-Phelan ( 2007 ) say that theories prevent scholars from “being dazzled by the complexity of the empirical world” (p. 1281). Theoretical frameworks keep researchers grounded by setting parameters within which the empirical world can be interpreted.
Exercise 3.5
Find two published articles that explicitly present theoretical frameworks (not all articles do). Where do you see evidence of the researchers using their theoretical frameworks to inform, shape, and connect other parts of their articles?
Showing the Contribution of Your Study
Theoretical frameworks contain the arguments that define the contribution of research studies. They do this in two ways, by showing how your study extends what is known and by setting the parameters for your contribution.
Showing How Your Study Extends What Is Known
Because your theoretical framework is built from what is already known or has been proposed, it situates your study in work that has occurred before. A clearly written framework shows readers how your study will take advantage of what is known to extend it further. It reveals what is new about what you are studying. The predictions that are generated from your framework are predictions that have never been made in quite the same way. They predict you will find something that has not been found previously in exactly this way. Your theoretical framework allows others to see the contributions that your study is likely to make even before the study has been conducted.
Setting the Parameters for Your Contribution
Earlier we noted that theoretical frameworks keep researchers grounded by setting parameters within which they should interpret their data. They do this by providing an initial explanation for why researchers expect to find particular results. The explanation is custom-built for each study. This means it uniquely explains the expected results. The results will almost surely turn out somewhat differently than predicted. Interpreting the data includes revising the initial explanation. So, you will end up with two versions of your theoretical framework, one that explains what you expected to find plus a second, updated framework that explains what you actually found.
The two frameworks—the initial version and the updated version—define the parameters of your study’s contribution. The difference between the two frameworks is what can be learned from your study. The first framework is a claim about what is known before you conduct your study about the phenomenon you are studying; the updated framework is a claim about how what is known has changed based on your results. It is the new aspects of the updated framework that capture the important contribution of your work.
Here is a brief example. Suppose you study the errors fourth graders make after receiving ordinary instruction on adding and subtracting decimal fractions. Based on empirical findings from past research, on theories of student learning, and on your own experience, you develop a rationale which predicts that a common error on “ragged” addition problems will be adding the wrong numerals. One of the reasons for this prediction is that students are likely to ignore the values of the digit positions and “line up” the numerals as they do with whole numbers. For instance, if they are asked to add 53.2 + .16, they are likely to answer either 5.48 or 54.8.
When you conduct your study, you present problems, handwritten, in both horizontal and vertical form. The horizontal form presents the numbers using the format shown above. The vertical form places one numeral over the other but not carefully aligned:
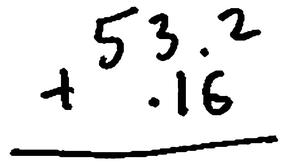
You find the predicted error occurs, but only for problems written in vertical form. To interpret these data, you look back at your theoretical framework and realize that students might ignore the value of the digits if the format reminded them of the way they lined up digits for whole number addition but might consider the value of the digits if they are forced to align the digits themselves, either by rewriting the problem or by just adding in their heads. A measure of what you (and others) learned from this study is the change in possible explanations (your theoretical frameworks). This does not mean your updated theoretical framework is “correct” or will make perfectly accurate predictions next time. But, it does mean that you are very likely moving toward more accurate predictions and toward a deeper understanding of how students think about adding decimal fractions.
Anchoring the Coherence of Your Study (and Your Evolving Research Paper)
Your theoretical framework serves as the anchor or center point around which all other aspects of your study should be aligned. This does not mean it is created first or that all other aspects are changed to align with the framework after it is created. The framework also changes as other aspects are considered. However, it is useful to always check alignment by beginning with the framework and asking whether other aspects are aligned and, if not, adjusting one or the other. This process of checking alignment is equally true when writing your evolving research paper as when planning and conducting your study.
Part III. How Do You Construct a Theoretical Framework for Your Study?
How do you start the process? Because constructing a theoretical framework is a natural extension of constructing rationales for your predictions, you already started as soon as you began formulating hypotheses: making predictions for what you will find and writing down reasons for why you are making these predictions. In Chap. 2 , we talked about beginning this process. In this section, we will explore how you can continue building out your rationales into a full-fledged theoretical framework.
Building a Theoretical Framework in Phases
Building your framework will occur in phases and proceed through cycles of clarifying your questions, making more precise and explicit your predictions, articulating reasons for making these predictions, and imagining ways of testing the predictions. The major source for ideas that will shape the framework is the research literature. That said, conversations with colleagues and other experts can help clarify your predictions and the rationales you develop to justify the predictions.
As you read relevant literature, you can ask: What have researchers found that help me predict what I will find? How have they explained their findings, and how might those explanations help me develop reasons for my predictions? Are there new ways to interpret past results so they better inform my predictions? Are there ways to look across previous results (and claims) and see new patterns that I can use to refine my predictions and enrich my rationales? How can theories that have credibility in the research community help me understand what I might find and help me explain why this is the case? As we have said, this process will go back and forth between clarifying your predictions, adjusting your rationales, reading, clarifying more, adjusting, reading, and so on.
One Researcher’s Experience Constructing a Theoretical Framework: The Continuing Case of Martha
In Chap. 2 , we followed Martha, a doctoral student in mathematics education, as she was working out the topic for her study, asking questions she wanted to answer, predicting the answers, and developing rationales for these predictions. Our story concluded with a research question, a sample set of predictions, and some reasons for Martha’s predictions. The question was: “Under what conditions do middle school teachers who lack conceptual knowledge of linear functions benefit from five 2-hour learning opportunity (LO) sessions that engage them in conceptual learning of linear functions as assessed by changes in their teaching toward a more conceptual emphasis of linear functions?” Her predictions focused on particular conditions that would affect the outcomes in particular ways. She was beginning to build rationales for these predictions by returning to the literature and identifying previous research and theory that were relevant. We continue the story here.
Imagine Martha continuing to read as she develops her theoretical framework—the rationales for her predictions. She tweaks some of her predictions based on what other researchers have already found. As she continues reading, she comes across some related literature on learning opportunities for teachers. A number of articles describe the potential of another form of LOs that might help teachers teach mathematics more conceptually—analyzing videos of mathematics lessons.
The data suggested that teachers can improve their teaching by analyzing videos of other teachers’ lessons as well as their own. However, the results were mixed so researchers did not seem to know exactly what makes the difference. Martha also read that teachers who already can analyze videos of lessons and spontaneously describe the mathematics that students are struggling with and offer useful suggestions for how to improve learning opportunities for students teach toward more conceptual learning goals, and their students learn more (Kersting et al., 2010 , 2012 ). These findings caught Martha’s attention because it is unusual to find correlates with conceptual teaching and better achievement. What is not known, realized Martha, is whether teachers who learn to analyze videos in this way, through specially designed LOs, would look like the teachers who already could analyze them. Would teachers who learned to analyze videos teach more conceptually?
It occurred to Martha she could bring these lines of research together by extending what is known along both lines. Recall our earlier suggestion of looking across the literature and noticing new patterns that can inform your work. Martha thought about studying how, exactly, these two skills are related: analyzing videos in particular ways and teaching conceptually. Would the relationships reported in the literature hold up for teachers who learn to describe the mathematics students are struggling with and make useful suggestions for improving students’ LOs?
Martha was now conflicted. She was well on her way to developing a testable hypothesis about the effects of learning about linear functions, but she was really intrigued by the work on analyzing videos of teaching. In addition, she saw several advantages of switching to this new topic:
The research question could be formulated quite easily. It would be something like: “What are the relationships between learning to analyze videos of mathematics teaching in particular ways (specified from prior research) and teaching for conceptual understanding?”
She could imagine predicting the answers to this question based directly on previous research. She would predict connections between particular kinds of analysis skills and levels of conceptual teaching of mathematics in ways that employed these skills.
The level of conceptual teaching, a challenging construct to define with her previous topic (the effects of professional development on the teaching of linear functions), was already defined in the work on analyzing videos of mathematics teaching, so that would solve a big problem. The definition foregrounded particular sets of behaviors and skills such as identifying key learning moments in a lesson and focusing on students’ thinking about the key mathematical idea during these moments. In other words, Martha saw ways to adapt a definition that had already been used and tested.
The issue of transfer—another challenging issue in her original hypothesis—was addressed more directly in this setting because the learning environment—analyzing videos of classroom teaching—is quite close to the classroom environment in which participants’ conceptual teaching would be observed.
Finally, the nature of learning opportunities, an aspect of her original idea she still needed to work through, had been explored in previous studies on this new topic, and connections were found between studying videos and changes in teaching.
Given all these advantages, Martha decided to change her topic and her research question. We applaud this decision for two major reasons. First, Martha’s interest grew as she explored this new topic. She became excited about conducting a study that might answer the research question she posed. It is always good to be passionate about what you study. Second, Martha was more likely to contribute important new insights if she could extend what is already known rather than explore a new area. Exploring something quite new requires lots of effort defining terms, creating measures, making new predictions, developing reasons for the predictions, and so on. Sometimes, exploring a new area has payoffs. But, as a beginning researcher, we suggest you take advantage of work that has already been done and extend it in creative ways.
Although Martha’s idea of extending previous work came with real advantages, she still faced a number of challenges. A first, major challenge was to decide whether she could build a rationale that would predict learning to analyze videos caused more conceptual teaching. Or, could she only build a rationale that would predict that there was a relationship between changes in analyzing videos and level of conceptual teaching? Perhaps a cause-effect relationship existed but in the opposite direction: If teachers learned to teach more conceptually, their analysis of teaching videos would improve. Although most of the literature described learning to analyze videos as the potential cause of teaching conceptually, Martha did not believe there was sufficient evidence to build a rationale for this prediction. Instead, she decided to first determine if a relationship existed and, if so, to understand the relationship. Then, if warranted, she could develop and test a hypothesis of causation in a future study. In fact, the direction of the causation might become clearer when she understood the relationship more clearly.
A second major challenge was whether to study the relationship as it existed or as one (or both) of the constructs was changing. Past research had explored the relationship as it existed, without inducing changes in either analyzing videos or teaching conceptually. So, Martha decided she could learn more about the relationship if one of the constructs was changing in a planned way. Because researchers had argued that teachers’ analysis of video could be changed with appropriate LOs, and because changing teachers’ teaching practices has resisted simple interventions, Martha decided to study the relationship as she facilitated changes in teachers’ analysis of videos. This would require gathering data on the relationship at more than one point in time.
Even after resolving these thorny issues, Martha faced many additional challenges. Should she predict a closer relationship between learning to analyze video and teaching for conceptual understanding before teachers began learning to analyze videos or after? Perhaps the relationship increases over time because conceptual teaching often changes slowly. Should she predict a closer relationship if the content of the videos teachers analyzed was the same as the content they would be teaching? Should she predict the relationship will be similar across pairs of similar topics? Should she predict that some analysis skills will show closer relationships to levels of conceptual teaching than others? These questions and others occurred to Martha as she was formulating her predictions, developing justifications for her predictions, and considering how she would test the predictions.
Based on her reading and discussions with colleagues, Martha phrased her initial predictions as follows:
There will be a significant positive correlation between teachers’ performance on analysis of videos and the extent to which they create conceptual learning opportunities for their students both before and after proposed learning experiences.
The relationship will be stronger:
Before the proposed opportunities to learn to analyze videos of teaching;
When the videos and the instruction are about similar mathematical topics; and,
When the videos analyzed display conceptual misunderstandings among students.
Of the video analysis skills that will be assessed, the two that will show the strongest relationship are spontaneously describing (1) the mathematics that students are struggling with and (2) useful suggestions for how to improve the conceptual learning opportunities for students.
Martha’s rationales for these predictions—her theoretical framework—evolved along with her predictions. We will not detail the framework here, but we will note that the rationale for the first prediction was based on findings from past research. In particular, the prediction is generated by reasoning that if there has been no special intervention, the tendency to analyze videos in particular ways and to teach conceptually develop together. This might explain Kersting’s findings described earlier. The second and third predictions were based on the literature on teachers’ learning, especially their learning from analyzing videos of teaching.
Before leaving Martha at this point in her journey, we want to make an important point about the change she made to her research topic. Changes like this occur quite often as researchers do the hard intellectual work of developing testable hypotheses that guide research studies. When this happens to you, it can feel like you have lost ground. You might feel like you wasted your time on the original topic. In Chap. 1 , we described inevitable “failure” when engaged in scientific inquiry. Failure is often associated with realizing the data you collected do not come close to supporting your predictions. But a common kind of failure occurs when researchers realize the direction they have been pursuing should change before they collect data. This happened in Martha’s case because she came across a topic that was more intriguing to her and because it helped solve some problems she was facing with the previous topic. This is an example of “failing productively” (see Chap. 1 ). Martha did not succeed in pursuing her original idea, but while she was recognizing the problems, she was also seeing new possibilities.
Constantly Improving Your Framework
We will use Martha’s experience to be more specific about the back-and-forth process in which you will engage as you flesh out your framework. We mentioned earlier your review of the literature as a major source of ideas and evidence that will affect your framework.
Reviewing Published Empirical Evidence
One of the best sources for helping you specify your predictions are studies that have been conducted on related topics. The closer to your topic, the more helpful will be the evidence for anticipating what you will find. Many beginning researchers worry they will locate a study just like the one they are planning. This (almost) never happens. Your study will be different in some ways, and a study that is very similar to yours can be extraordinarily helpful in specifying your predictions. Be excited instead of terrified when you come across a study with a title similar to yours.
Try to locate all the published research that has been conducted on your topic. What does “on your topic” mean? How widely should you cast your net? There are no rules here; you will need to use your professional judgment. However, here is a general guide: If the study does not help you clarify your predictions, change your confidence in them, or strengthen your rationale, then it falls outside your net.
In addition to helping specify your predictions, prior research studies can be a goldmine for developing and strengthening your theoretical framework. How did researchers justify their predictions or explain why they found what they did? How can you use these ideas to support (or change) your own predictions?
By reading research on similar topics, you might also imagine ways of testing your predictions. Maybe you learn of ways you could design your study, measures you could use to collect data, or strategies you could use to analyze your data. As you find helpful ideas, you will want to keep track of where you found these ideas so you can cite the appropriate sources as you write drafts of your evolving research paper.
Examining Theories
You will read a wide range of theories that provide insights into why things might work like they do. When the phenomena addressed by the theory are similar to those you will study, the associated theories can help you think through your own predictions and why you are making them. Returning to Martha’s situation, she could benefit from reading theories on adult learning, especially teacher learning, on transferring knowledge from one setting to another, on professional development for teachers, on the role of videos in learning, on the knowledge needed to teach conceptually, and so on.
Focusing on Variables and Mechanisms
As you review the literature and search for evidence and ideas that could strengthen your predictions and rationales, it is useful to keep your eyes on two components: the variables you will attend to and the mechanisms that might explain the relationships between the variables. Predictions could be considered statements about expected behaviors of the variables. The theoretical framework could be thought of as a description of all the variables that will be deliberately attended to plus the mechanisms conjectured to account for these relationships.
In Martha’s case, the most obvious variables are the responses teachers give to questions about their analysis of the videos and the features observed in their teaching practices. The mechanism of primary interest is the (mental and social) process that transforms the skills, knowledge, and attention involved in analyzing videos into particular kinds of teaching practices—or vice versa. The definition of conceptual teaching she adopted from previous studies gave her a clue about the mechanisms—about how and why learning to analyze videos might affect classroom teaching. The definition included attending to key learning moments in a lesson and tracking students’ thinking during these moments. Martha predicted that if teachers learned to attend to these aspects of teaching when viewing videos, they might attend to them when planning and implementing their own teaching.
As Martha reviewed the literature, she identified a number of variables that might affect the likelihood and extent of this translation. Here are some examples: how well teachers understand the mathematics in the videos and the mathematics they will teach; the nature of the videos themselves; the number of opportunities teachers have to analyze videos and the ways in which these opportunities are structured; teachers’ analysis of videos and their teaching practices before the learning opportunities begin; and how much time they have to apply what they learn to their own teaching.
Martha identified these additional variables because she learned they might have a direct influence on the mechanisms that could explain the relationship between analyzing videos and teaching. Some variables might support these mechanisms, and some might interfere. Martha’s task at this point in her work is to identify and describe all the variables that could play a meaningful role in the outcome of her study. This means to identify each variable for which it is possible to establish a clear and direct connection between the variable and the relationship she planned to investigate. Using the outcome of this task, Martha then needs to update her description of the mechanisms that could account for the relationships she expects to see and review her predictions and theoretical framework with these variables and mechanisms in mind.
Exercise 3.6
Review the predictions that Martha made and identify the variables that play a role in these predictions. Even though you might not be immersed in this literature, think about the alignment between the variables included in the predictions and those that could impact the relationships in which Martha is interested. Are there other missing variables that should be included in her predictions?
How Do You Know When You Have Finished Building Your Theoretical Framework?
The question of when your theoretical framework is finished could be answered in several ways. First, it is never really finished. As you continue to write your evolving research paper, you will continue strengthening your framework. You might even refine the framework as you write the final draft of your paper, after you have collected and analyzed your data. Furthermore, if you do follow-up studies, you will continue to build your framework.
A second answer is that you should invest the time and effort to build a theoretical framework that is as finished as possible at each point in the research process. As you write each draft of your evolving research paper, you should feel as if you have the strongest, most robust rationale you can have for your current predictions. In other words, you should feel that with each succeeding draft you have finished building your framework, even though you are quite sure you have not.
A third answer addresses a common, related question: “How do I know when I have included enough ideas and borrowed from enough sources? Would including another idea or citing another source be useful?” The answer is that you should include only those ideas that contribute to building a stronger framework. When you wonder whether you should include another idea or reference, ask yourself whether doing so would make your framework stronger in all the ways we described earlier.
Exercise 3.7
In 2–3 pages (single spaced), write out the plan for your study. The plan should include your research questions, your predictions of the answers, your rationale for the predictions (i.e., your theoretical framework), and your imagined plan for testing the predictions. Be as explicit and precise as you can. Be sure you have identified the critical variables and described the mechanism(s) that could explain the phenomena, the relationships, and/or the changes you predict. Look back to see if the logic connecting the parts is obvious. Ask yourself whether the tests you plan are what anyone familiar with your framework would expect (i.e., there should be no surprises).
Part IV. Refining a Theoretical Framework: A Scholarly Dialogue
As we noted above, conversations with colleagues and other experts can help you refine your theoretical framework by clarifying your predictions and digging into the details of the rationales you develop to justify those predictions. This is as true for experienced researchers as it is for beginning researchers. The dialogue below is an example of how two colleagues, Adrian (A) and Corin (C), work together to gradually formulate a testable hypothesis. Some of their conversation will look familiar as they refine their prediction through multiple steps of discussion:
Narrowing the focus of their prediction.
Making their prediction more testable.
Being more specific about what they want to study.
Engaging their prediction in cycles of refinements.
Determining the appropriate level/grainsize of their prediction (zoom in, zoom out).
Adding more predictions.
Thinking about underlying mechanisms (i.e., what explains the relationships between their variables).
Putting their predictions on a continuum (going from black and white to grey).
In addition, they construct their theoretical framework to match their hypotheses through multiple steps:
Defining and rationalizing their variables.
Re-evaluating their initial rationales in response to changes in their initial predictions.
Asking themselves “why” questions about predictions and rationales.
Finding empirical evidence and theory that better supports their evolving predictions.
Keeping in mind what they are going to be measuring.
Making sure their rationales support each link in their chain of reasoning.
Identifying underlying mechanisms.
Making sure that statements are included in their rationale if and only if they directly support their predictions and are essential to the argument.
They begin with the following hypothesis:
Prediction: Students will exhibit more persistence in mathematical course taking in high school if they work in groups.
Brief Description of Rationale: When people work in groups, they feel more competent and learn better (Cohen & Lotan, 2014 ; Jansen, 2012). When people feel more competent, they persist in additional mathematical course taking (Bandura & Schunk, 1981 ; Dweck, 1986 ).
So, do we think this hypothesis is testable?
Well actually, who these students are is probably something we need to be more specific about.
Good point, and also, since Algebra 2 is the bridge to additional course taking (i.e., the first course students don’t have to take), perhaps we should target Algebra 2. How about if we change our prediction to the following: Algebra 2 students will exhibit more mathematical persistence in mathematical course taking in high school if they work in groups in Algebra 2.
Okay, but another problem is that it would take a long time to collect data that would inform a prediction about the courses students take, and over that amount of time I’m not sure we could even tell if groupwork was responsible. What if we limited our prediction to: Algebra 2 students will exhibit more mathematical persistence in Algebra 2 if they work in groups.
Good idea! But when we talk about persistence, do we mean students don’t quit, or that they don’t drop the course, or productively struggle during class, or turn in their homework, or is it something else we mean? To me, what would be testable about mathematical persistence would be persistence at the problem level, such as when students get stuck on a problem, but they don’t give up.
I agree. So, let’s predict the following: Algebra 2 students will exhibit more mathematical persistence in Algebra 2 when they get stuck on problems if they work in groups. That’s something I think we could test.
Yes, but I think we need to be even more specific about what we mean by mathematical persistence when students get stuck on problems.
Hmm, what if we focused specifically on mathematical persistence that involves staying engaged in trying to solve a problem for the duration of a problem-solving session or until the problem gets solved? But that also makes me wonder if we want to be focusing on persistence at the individual level or at the group level?
Umm, I think we should focus on persistence at the individual level, because that’s more consistent with our original interest in persistence in course taking, which is about individual students, not about groups.
Okay, that makes sense. So then how about this for a prediction: If Algebra 2 students work in groups, they will be more likely to stay engaged in trying to solve problems for the duration of a problem-solving session or until they solve the problem.
To this point in the dialogue, Adrian and Corin are developing a theoretical framework by sharpening what they mean by their prediction and making sure their prediction is testable. In the next part, they return to their original idea to make sure they have not strayed too far by making their prediction more precise. The dialogue illustrates how making predictions should support the goal of understanding the relationship between variables and the mechanisms for change.
Yes, I’m liking the way this prediction is evolving. However, I also feel like our prediction is now so focused that we’ve lost a bit of our initial idea of competence and learning, which is what we were initially interested in. Could we do something to bring those ideas back? Perhaps we could create more predictions to get at more of those ideas?
Great idea! Okay, so to help us see what we are missing now, let’s look back at the initial links in our chain of reasoning. We initially said that Working in Groups leads to Feeling Competent & Learning Better leads to Persistence in Math Course Taking. But our chain of reasoning has changed. I think it’s more like this: Working in Groups on Problems leads to Staying Engaged in Problem Solving leads to Greater Sense of Competence and Learning Better leads to More Persistence in Course Taking.
Okay, so if that’s the case, it looks like our new prediction just tests the first link in this chain, the link between Working in Groups on Problems and Staying Engaged in Problem Solving. It looks like there are three other potential predictions we could make; we could make a prediction about the relationship between Staying Engaged in Problem Solving and having a Greater Sense of Competence, between Staying Engaged in Problem Solving and Learning Better, and between having a Greater Sense of Competence/Learning Better and More Persistence in Course Taking.
Clearly that’s too many predictions for us to tackle in one study and actually I am aware of several studies that already address the third prediction. So, we can use those studies as part of our rationale and don’t need to study that link.
I agree. Let’s just add one prediction, one about the link between Staying Engaged and Sense of Competence. In our initial prediction, we just had a vague connection between Working in Groups and Sense of Competence. But in our new prediction, we were more specific that working in groups helps students stay engaged until the end of a problem-solving session. So, I guess we could say for a second prediction then that When Algebra 2 students stay engaged in problem solving until the end of a problem-solving session, they develop a greater sense of competence.
Okay so we will have two predictions to examine with our study: Prediction 1 is: If Algebra 2 students work in groups, they will be more likely to stay engaged in trying to solve problems for the duration of a problem-solving session or until they solve the problem. This prediction deals with the first link in our chain of reasoning. And then Prediction 2 is: If Algebra 2 students try to solve problems for the duration of a problem-solving session or until they solve the problem, they will be more likely to develop a sense of competence. Oh, as soon as I finished stating that prediction, the thought just came to me, “sense of competence about what?”
How about if we focused on sense of competence in being able to solve similar problems in the future? Actually, maybe that’s too limited. Maybe we should expand our prediction a bit more so we include a sense of competence that’s at least somewhat closer to more course taking? Something like sense of competence that involves feeling capable of understanding future Algebra 2 concepts. That’s at least bigger than sense of competence at solving similar problems. If students feel they’re capable of understanding future Algebra 2 concepts, then they will probably be more likely to persist in course taking too.
Okay, that makes sense. So, then our Prediction 2 could be: If Algebra 2 students try to solve problems for the duration of a problem-solving session or until they solve the problem, they will be more likely to feel they will be capable of understanding future Algebra 2 concepts.
Oh, I just had an additional idea! What if we changed the two predictions one more time to allow for more or less of the variables? For example, Prediction 1 could be: The more Algebra 2 students work in groups, the more likely they will stay engaged in trying to solve problems for the duration of a problem-solving session or until they solve the problem.
Yes, great. So, that would mean Prediction 2 could be: The more Algebra 2 students try to solve problems for the duration of a problem-solving session or until they solve the problem, the more likely they will feel they are capable of understanding future Algebra 2 concepts.
So, I think we’re happy with our predictions for now, but I think we need to work on our rationales for those predictions because they no longer apply very well.
Okay, to recap, our original chain of reasoning was Working in Groups leads to Feeling Competent & Learning Better leads to Persistence in Math Course Taking. Our initial rationales were the following: For the link between working in groups and feeling competent, we based that link on Cohen and Lotan’s ( 2014 ) book on Designing Groupwork, in which they explain why and how all students can feel competent through their engagement in groupwork. We also based this link on that 2012 Jansen study that found that groupwork helped students enact their competence in math. Then, for the link between competence and persistence, we based that link on the Bandura and Schunk ( 1981 ) study and on the work by Carol Dweck ( 1986 ) that show that children who feel more competent in arithmetic, tend to persist more.
Corin and Adrian have looked back at their initial research idea. In doing so, they illustrated how developing a theoretical framework involves developing and refining a chain of reasoning. They continue by working on developing rationales for their predictions.
Okay, so let’s think if any of our previous rationales still work. How about Elizabeth Cohen’s work? I still think her work applies because it shows that groupwork can affect engagement. But now that I think about it, another part of her work indicates that groupwork needs particular norms in order to be effective. So maybe we should tighten up our predictions to focus just on groupwork that has particular norms?
But, on the other hand, what about Jo Boaler’s ( 1998 ) “Open and Closed Mathematics” article? In that study, students at the Phoenix Park School did not have much structure, and in spite of that, groupwork worked quite well for those students, better than individual work did for students at the Amber Hill School who had highly structured instruction.
That’s a good point. So maybe we should leave our predictions about groupwork as is (i.e., not focus on particular norms). Also, the ideas in the Boaler article would be good to add to our theoretical framework because it deals with secondary students, which aligns better with the ages of the Algebra 2 students we are planning on studying.
Okay, so we’re adding the ideas in the Boaler article. I also think we need to find literature that specifies the kind of engagement we want to focus on. Looking at the engagement literature would sharpen our thinking about the engagement we are most interested in. We should consider Brigid Barron’s ( 2003 ) study, “When Smart Groups Fail.” In her study, students produced better products if they engaged with each other and with the content. But that makes me think that we are mostly just focused on the latter, namely on how individuals engage with the content.
I agree we’re focused on individuals’ engagement with the content. Come to think of it, the fact that we’re focused on how individuals engage with content rather than how groups engage further justifies why we’re not looking at groupwork norms. But let me ask a question we need to answer. Why are we focusing on how individuals engage with content? It’s not just a preference. It’s because we think individual engagement with content is related to feeling capable. So, our decision to focus on individual engagement aligns with our predictions. And even though we’re not including Barron’s work in our framework, considering her work helped sharpen our thinking about what we’re focusing on.
You know, we are kind of in a weird space because we’re focusing on individual engagement with content at the same time as we are predicting that groupwork leads to more engagement. In other words, we are and aren’t taking a social perspective. But what this reminds me of is how, from the perspective of the theory of constructivism, even though individuals have to make sense of things for themselves, social interactions are what drives sense making. In fact, here’s a quote from von Glasersfeld ( 1995 ): “Piaget has stressed many times that the most frequent cause of accommodation is the interaction” (p. 66). So, I think we can use constructivism as a theoretical justification for predicting that the social activity of groupwork is what is related to individual engagement with content.
Interesting! Yes, makes sense. When you were describing that, I had another insight from constructivism. You know how when someone experiences a perturbation, it also creates a need in them to resolve the perturbation, right? So maybe perturbations are the mechanism explaining why groupwork leads to more individual engagement with content. Groupwork potentially generates perturbations, meaning the person engages more to try to resolve those perturbations.
Okay, now that we have brought in the idea of perturbations as potentially being the mechanism that drives how working in groups leads to staying more engaged, perhaps we need to reconsider what we will be measuring in our study. Will it be perturbations, or will it be staying engaged that we should be measuring?
I think what we are saying is that the need to resolve perturbations is part of the underlying mechanism, but measuring the need to resolve perturbations would be difficult if not impossible. So, instead, I think we should focus on measuring the variable staying engaged , a variable we can measure. And then if we find that more working in groups leads to more staying engaged, that also gives us more evidence that our theoretical framework with perturbations as a mechanism is viable. In other words, mechanisms are part of our framework and by testing our prediction, we are testing our theoretical framework (i.e., our rationales) too.
This final part of the dialogue illustrates that the rationale for a study continues to develop as the predictions continue to be refined and testability continues to be considered. In other words, the development of the predictions and rationale (i.e., the theoretical framework) should be iterative and ongoing.
Through their discussion, Adrian and Corin have refined both their predictions and their rationales. In the process, the key ideas they have drawn on contributed to their rationales and thus to constructing their theoretical framework.
Part V. Distinctions Between Rationales, Theoretical Frameworks, and Literature Reviews
We have introduced a number of terms that play critical roles in the scientific inquiry process. Because they refer to related and sometimes overlapping ideas, keeping straight their meanings and uses can be challenging. It might be helpful to revisit each of them briefly to describe how they are similar to, and different from, each other.
To distinguish between rationales, theoretical frameworks, and literature reviews, it is useful to consider the roles they play as you plan and conduct a study compared to the roles they play when you write the report of your study.
Thinking Through a Study
The chronology of the thinking process often moves through many cycles of identifying a research problem or asking a question, and then reading the literature to learn more about the problem, and then refining and narrowing the scope of a question that would add to or extend what is known, and then predicting (guessing) an answer to the question and asking yourself why you predicted this answer and writing a first draft of your rationale, and then reading the literature to improve your rationale, and then realizing you can refine the question further along with specifying a clearer and more targeted prediction, and then reading the literature to further improve your rationale, and then realizing you can refine the question further along with a clearer and more targeted prediction, and so on.
The primary activity that generates more specific and clearer hypotheses is searching and reviewing literature . You can return to the literature as often as you need to build your rationales . As your rationales develop, they morph into your theoretical framework . The theoretical framework is a coherent argument that threads together the individual rationales and explains why your predictions are the best predictions the field can make at this time.
If you have one research question and one prediction you will have one rationale. In this case, your rationale is essentially the same as your theoretical framework. If you have more than one research question, you will have multiple predictions and multiple rationales. As you develop rationales for each prediction, you might find lots of overlap. Maybe the literatures you read to refine each prediction and develop each rationale overlap, and maybe the arguments you piece together include many of the same elements. Your theoretical framework emerges from weaving the rationales together into one coherent argument. Although this process is more complicated than the thinking process for one prediction, it is more common. If you find few connections among the rationales for each prediction, we recommend stepping back and asking whether you are conducting more than one study. It might make more sense to sort the questions into two or more studies because the rationales for the predicted answers are drawing from different literatures.
Writing the Evolving Research Paper
We recommend that you write drafts of the research report as you think through your study and make decisions about how to proceed. Although your thinking will be fluid and evolving, we recommend that you follow the conventions of academic writing as you write drafts. For example, we recommend that you structure the paper using the five typical major sections of a journal article: introduction, theoretical framework, methods, results, and discussion. Each of these sections will go through multiple drafts as you plan your study, collect the data, analyze the data, and interpret the results.
In the introduction, you will present the research problem you are studying. This includes describing the problem, explaining why it is significant, defining the special terms you use, and often presenting the research questions you will address along with the answers you predict. Sometimes the questions and predictions are part of the next section—the theoretical framework.
In the theoretical framework, you will present your best arguments for expecting the predicted answers to the research questions. You will not trace the many cycles in which you engaged to get to the best versions of your arguments but rather present the latest and best version. The report of a study does not describe the chronology of the back-and-forth messiness always involved in thinking through all aspects of the study. What you learned from reviewing the literature will be an integral part of your arguments. In other words, the review of research will be included in the presentation of your theoretical framework rather than in a separate section.
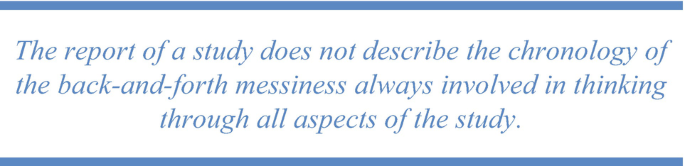
The literature you choose to include to present your theoretical framework is not all the literature you reviewed for conducting your study. Rather, the literature cited in your paper should be the literature that contributed to building your theoretical framework, and only that literature. In other words, the theoretical framework places the boundaries on what you should review in the paper.
Beginning researchers are often tempted to review much of what they read. Researchers put lots of time into reading, and leaving lots of it out when writing the paper can make all that reading feel like a waste of time. It is not a waste of time; it is always part of the research process. But, reviewing more than you need in the paper becomes a distraction and diverts the reader from the main points.
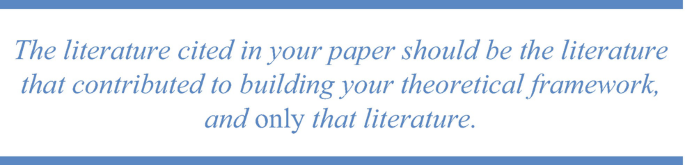
What should you do if the editor of the journal requires, or recommends, a section titled “review of research”? We recommend you create a somewhat more elaborated review for this section and then show exactly how you used the literature to build your rationale in the theoretical framework section.
Reviewers notice when the theoretical framework and the literature reviewed do not provide sufficient justification for the research questions (or the hypotheses). We found that about 13% of JRME reviews noted an especially important gap—the research questions in a paper were not sufficiently motivated. We expect the same would be true for other research journals. Reviewers also note when manuscripts either do not have an explicit theoretical framework or when they seem to be juggling more than one theoretical framework.
Part VI. Moving to Methods
A significant benefit of building rich and precise theoretical frameworks is the guidance they provide for selecting and creating the methods you will use to test your hypotheses. The next phase in the process of scientific inquiry is crafting your methods: choosing your research design, selecting your sample, developing your measures, deciding on your data analysis strategies, and so on. In Chap. 4 , we discuss how you can do this in ways that keep your story coherent.
Bandura, A., & Schunk, D. H. (1981). Cultivating competence, self-efficacy, and intrinsic interest through proximal self-motivation. Journal of Personality and Social Psychology, 41 , 586–598. https://doi.org/10.1037/0022-3514.41.3.586
Article Google Scholar
Barron, B. (2003). When smart groups fail. Journal of the Learning Sciences, 12 (3), 307–359. https://doi.org/10.1207/S15327809JLS1203_1
Biddle, B. J., & Anderson, D. S. (1986). Theory, methods, knowledge, and research on teaching. In M. C. Wittrock (Ed.), Handbook of research on teaching: A project of the American Educational Research Association . Macmillan.
Google Scholar
Boaler, J. (1998). Open and closed mathematics: Student experiences and understandings. Journal for Research in Mathematics Education, 29 , 41–62.
Cai, J., Morris, A., Hohensee, C., Hwang, S., Robison, V., Cirillo, M., Kramer, S. L., & Hiebert, J. (2019c). Theoretical framing as justifying. Journal for Research in Mathematics Education, 50 (3), 218–224. https://doi.org/10.5951/jresematheduc.50.3.0218
Cohen, E. G., & Lotan, R. A. (2014). Designing groupwork: Strategies for the heterogenous classroom (3rd ed.). Teachers College Press.
Colquitt, J. A., & Zapata-Phelan, C. P. (2007). Trends in theory building and theory testing: A five-decade study of the academy of management journal. The Academy of Management Journal, 50 (6), 1281–1303.
Dweck, C. S. (1986). Motivational processes affecting learning. American Psychologist, 41 , 1040–1048. https://doi.org/10.1037/0003-066X.41.10.1040
Kerlinger, F. N. (1964). Foundations of behavioral research: Educational and psychological inquiry . Holt, Rinehardt, & Winston.
Kersting, N. B., Givvin, K., Sotelo, F., & Stigler, J. W. (2010). Teacher’s analysis of classroom video predicts student learning of mathematics: Further explorations of a novel measure of teacher knowledge. Journal of Teacher Education , 61(1–2), 172–181. https://doi.org/10.1177/0022487109347875 , 172.
Kersting, N. B., Givvin, K. B., Thompson, B., Santagata, R., & Stigler, J. (2012). Developing measures of usable knowledge: Teachers’ analyses of mathematics classroom videos predict teaching quality and student learning. American Educational Research Journal, 49 (3), 568–590. https://doi.org/10.3102/0002831212437853
Oxford University Press. (2021). Theory. In Lexico.com dictionary . Lexico.com . https://www.lexico.com/en/definition/theory
Praetorius, A.-K., & Charalambous, C. (2022). Moving the field forward with respect to theorizing teaching: An introduction. In A.-K. Praetorius & C. Charalmabous (Eds.), Expert perspectives on theorizing teaching: Current status, problematic issues, and aspirations . Springer.
von Glasersfeld, E. (1995). Radical constructivism: A way of knowing and learning . Falmer Press.
Download references
Author information
Authors and affiliations.
School of Education, University of Delaware, Newark, DE, USA
James Hiebert, Anne K Morris & Charles Hohensee
Department of Mathematical Sciences, University of Delaware, Newark, DE, USA
Jinfa Cai & Stephen Hwang
You can also search for this author in PubMed Google Scholar
Rights and permissions
Open Access This chapter is licensed under the terms of the Creative Commons Attribution 4.0 International License ( http://creativecommons.org/licenses/by/4.0/ ), which permits use, sharing, adaptation, distribution and reproduction in any medium or format, as long as you give appropriate credit to the original author(s) and the source, provide a link to the Creative Commons license and indicate if changes were made.
The images or other third party material in this chapter are included in the chapter's Creative Commons license, unless indicated otherwise in a credit line to the material. If material is not included in the chapter's Creative Commons license and your intended use is not permitted by statutory regulation or exceeds the permitted use, you will need to obtain permission directly from the copyright holder.
Reprints and permissions
Copyright information
© 2023 The Author(s)
About this chapter
Hiebert, J., Cai, J., Hwang, S., Morris, A.K., Hohensee, C. (2023). Building and Using Theoretical Frameworks. In: Doing Research: A New Researcher’s Guide. Research in Mathematics Education. Springer, Cham. https://doi.org/10.1007/978-3-031-19078-0_3
Download citation
DOI : https://doi.org/10.1007/978-3-031-19078-0_3
Published : 03 December 2022
Publisher Name : Springer, Cham
Print ISBN : 978-3-031-19077-3
Online ISBN : 978-3-031-19078-0
eBook Packages : Education Education (R0)
Share this chapter
Anyone you share the following link with will be able to read this content:
Sorry, a shareable link is not currently available for this article.
Provided by the Springer Nature SharedIt content-sharing initiative
- Publish with us
Policies and ethics
- Find a journal
- Track your research
Want to create or adapt books like this? Learn more about how Pressbooks supports open publishing practices.
Module 2 Chapter 3: What is Empirical Literature & Where can it be Found?
In Module 1, you read about the problem of pseudoscience. Here, we revisit the issue in addressing how to locate and assess scientific or empirical literature . In this chapter you will read about:
- distinguishing between what IS and IS NOT empirical literature
- how and where to locate empirical literature for understanding diverse populations, social work problems, and social phenomena.
Probably the most important take-home lesson from this chapter is that one source is not sufficient to being well-informed on a topic. It is important to locate multiple sources of information and to critically appraise the points of convergence and divergence in the information acquired from different sources. This is especially true in emerging and poorly understood topics, as well as in answering complex questions.
What Is Empirical Literature
Social workers often need to locate valid, reliable information concerning the dimensions of a population group or subgroup, a social work problem, or social phenomenon. They might also seek information about the way specific problems or resources are distributed among the populations encountered in professional practice. Or, social workers might be interested in finding out about the way that certain people experience an event or phenomenon. Empirical literature resources may provide answers to many of these types of social work questions. In addition, resources containing data regarding social indicators may also prove helpful. Social indicators are the “facts and figures” statistics that describe the social, economic, and psychological factors that have an impact on the well-being of a community or other population group.The United Nations (UN) and the World Health Organization (WHO) are examples of organizations that monitor social indicators at a global level: dimensions of population trends (size, composition, growth/loss), health status (physical, mental, behavioral, life expectancy, maternal and infant mortality, fertility/child-bearing, and diseases like HIV/AIDS), housing and quality of sanitation (water supply, waste disposal), education and literacy, and work/income/unemployment/economics, for example.
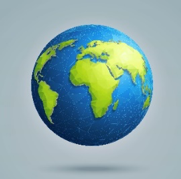
Three characteristics stand out in empirical literature compared to other types of information available on a topic of interest: systematic observation and methodology, objectivity, and transparency/replicability/reproducibility. Let’s look a little more closely at these three features.
Systematic Observation and Methodology. The hallmark of empiricism is “repeated or reinforced observation of the facts or phenomena” (Holosko, 2006, p. 6). In empirical literature, established research methodologies and procedures are systematically applied to answer the questions of interest.
Objectivity. Gathering “facts,” whatever they may be, drives the search for empirical evidence (Holosko, 2006). Authors of empirical literature are expected to report the facts as observed, whether or not these facts support the investigators’ original hypotheses. Research integrity demands that the information be provided in an objective manner, reducing sources of investigator bias to the greatest possible extent.
Transparency and Replicability/Reproducibility. Empirical literature is reported in such a manner that other investigators understand precisely what was done and what was found in a particular research study—to the extent that they could replicate the study to determine whether the findings are reproduced when repeated. The outcomes of an original and replication study may differ, but a reader could easily interpret the methods and procedures leading to each study’s findings.
What is NOT Empirical Literature
By now, it is probably obvious to you that literature based on “evidence” that is not developed in a systematic, objective, transparent manner is not empirical literature. On one hand, non-empirical types of professional literature may have great significance to social workers. For example, social work scholars may produce articles that are clearly identified as describing a new intervention or program without evaluative evidence, critiquing a policy or practice, or offering a tentative, untested theory about a phenomenon. These resources are useful in educating ourselves about possible issues or concerns. But, even if they are informed by evidence, they are not empirical literature. Here is a list of several sources of information that do not meet the standard of being called empirical literature:
- your course instructor’s lectures
- political statements
- advertisements
- newspapers & magazines (journalism)
- television news reports & analyses (journalism)
- many websites, Facebook postings, Twitter tweets, and blog postings
- the introductory literature review in an empirical article
You may be surprised to see the last two included in this list. Like the other sources of information listed, these sources also might lead you to look for evidence. But, they are not themselves sources of evidence. They may summarize existing evidence, but in the process of summarizing (like your instructor’s lectures), information is transformed, modified, reduced, condensed, and otherwise manipulated in such a manner that you may not see the entire, objective story. These are called secondary sources, as opposed to the original, primary source of evidence. In relying solely on secondary sources, you sacrifice your own critical appraisal and thinking about the original work—you are “buying” someone else’s interpretation and opinion about the original work, rather than developing your own interpretation and opinion. What if they got it wrong? How would you know if you did not examine the primary source for yourself? Consider the following as an example of “getting it wrong” being perpetuated.
Example: Bullying and School Shootings . One result of the heavily publicized April 1999 school shooting incident at Columbine High School (Colorado), was a heavy emphasis placed on bullying as a causal factor in these incidents (Mears, Moon, & Thielo, 2017), “creating a powerful master narrative about school shootings” (Raitanen, Sandberg, & Oksanen, 2017, p. 3). Naturally, with an identified cause, a great deal of effort was devoted to anti-bullying campaigns and interventions for enhancing resilience among youth who experience bullying. However important these strategies might be for promoting positive mental health, preventing poor mental health, and possibly preventing suicide among school-aged children and youth, it is a mistaken belief that this can prevent school shootings (Mears, Moon, & Thielo, 2017). Many times the accounts of the perpetrators having been bullied come from potentially inaccurate third-party accounts, rather than the perpetrators themselves; bullying was not involved in all instances of school shooting; a perpetrator’s perception of being bullied/persecuted are not necessarily accurate; many who experience severe bullying do not perpetrate these incidents; bullies are the least targeted shooting victims; perpetrators of the shooting incidents were often bullying others; and, bullying is only one of many important factors associated with perpetrating such an incident (Ioannou, Hammond, & Simpson, 2015; Mears, Moon, & Thielo, 2017; Newman &Fox, 2009; Raitanen, Sandberg, & Oksanen, 2017). While mass media reports deliver bullying as a means of explaining the inexplicable, the reality is not so simple: “The connection between bullying and school shootings is elusive” (Langman, 2014), and “the relationship between bullying and school shooting is, at best, tenuous” (Mears, Moon, & Thielo, 2017, p. 940). The point is, when a narrative becomes this publicly accepted, it is difficult to sort out truth and reality without going back to original sources of information and evidence.
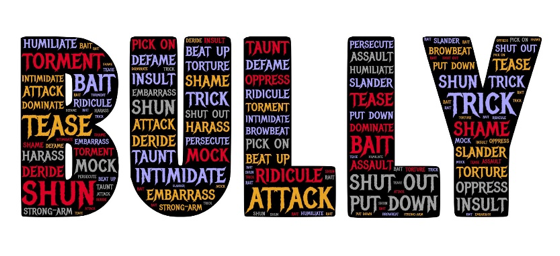
What May or May Not Be Empirical Literature: Literature Reviews
Investigators typically engage in a review of existing literature as they develop their own research studies. The review informs them about where knowledge gaps exist, methods previously employed by other scholars, limitations of prior work, and previous scholars’ recommendations for directing future research. These reviews may appear as a published article, without new study data being reported (see Fields, Anderson, & Dabelko-Schoeny, 2014 for example). Or, the literature review may appear in the introduction to their own empirical study report. These literature reviews are not considered to be empirical evidence sources themselves, although they may be based on empirical evidence sources. One reason is that the authors of a literature review may or may not have engaged in a systematic search process, identifying a full, rich, multi-sided pool of evidence reports.
There is, however, a type of review that applies systematic methods and is, therefore, considered to be more strongly rooted in evidence: the systematic review .
Systematic review of literature. A systematic reviewis a type of literature report where established methods have been systematically applied, objectively, in locating and synthesizing a body of literature. The systematic review report is characterized by a great deal of transparency about the methods used and the decisions made in the review process, and are replicable. Thus, it meets the criteria for empirical literature: systematic observation and methodology, objectivity, and transparency/reproducibility. We will work a great deal more with systematic reviews in the second course, SWK 3402, since they are important tools for understanding interventions. They are somewhat less common, but not unheard of, in helping us understand diverse populations, social work problems, and social phenomena.
Locating Empirical Evidence
Social workers have available a wide array of tools and resources for locating empirical evidence in the literature. These can be organized into four general categories.
Journal Articles. A number of professional journals publish articles where investigators report on the results of their empirical studies. However, it is important to know how to distinguish between empirical and non-empirical manuscripts in these journals. A key indicator, though not the only one, involves a peer review process . Many professional journals require that manuscripts undergo a process of peer review before they are accepted for publication. This means that the authors’ work is shared with scholars who provide feedback to the journal editor as to the quality of the submitted manuscript. The editor then makes a decision based on the reviewers’ feedback:
- Accept as is
- Accept with minor revisions
- Request that a revision be resubmitted (no assurance of acceptance)
When a “revise and resubmit” decision is made, the piece will go back through the review process to determine if it is now acceptable for publication and that all of the reviewers’ concerns have been adequately addressed. Editors may also reject a manuscript because it is a poor fit for the journal, based on its mission and audience, rather than sending it for review consideration.
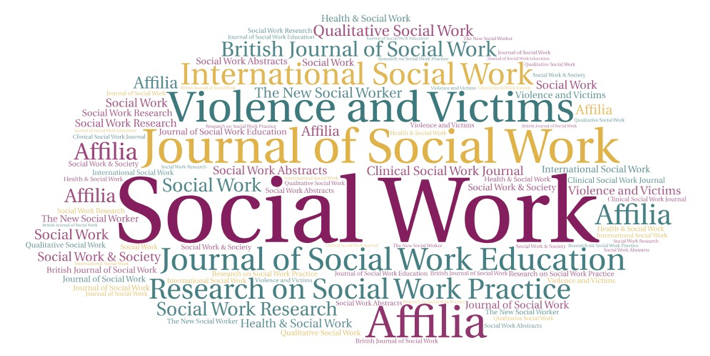
Indicators of journal relevance. Various journals are not equally relevant to every type of question being asked of the literature. Journals may overlap to a great extent in terms of the topics they might cover; in other words, a topic might appear in multiple different journals, depending on how the topic was being addressed. For example, articles that might help answer a question about the relationship between community poverty and violence exposure might appear in several different journals, some with a focus on poverty, others with a focus on violence, and still others on community development or public health. Journal titles are sometimes a good starting point but may not give a broad enough picture of what they cover in their contents.
In focusing a literature search, it also helps to review a journal’s mission and target audience. For example, at least four different journals focus specifically on poverty:
- Journal of Children & Poverty
- Journal of Poverty
- Journal of Poverty and Social Justice
- Poverty & Public Policy
Let’s look at an example using the Journal of Poverty and Social Justice . Information about this journal is located on the journal’s webpage: http://policy.bristoluniversitypress.co.uk/journals/journal-of-poverty-and-social-justice . In the section headed “About the Journal” you can see that it is an internationally focused research journal, and that it addresses social justice issues in addition to poverty alone. The research articles are peer-reviewed (there appear to be non-empirical discussions published, as well). These descriptions about a journal are almost always available, sometimes listed as “scope” or “mission.” These descriptions also indicate the sponsorship of the journal—sponsorship may be institutional (a particular university or agency, such as Smith College Studies in Social Work ), a professional organization, such as the Council on Social Work Education (CSWE) or the National Association of Social Work (NASW), or a publishing company (e.g., Taylor & Frances, Wiley, or Sage).
Indicators of journal caliber. Despite engaging in a peer review process, not all journals are equally rigorous. Some journals have very high rejection rates, meaning that many submitted manuscripts are rejected; others have fairly high acceptance rates, meaning that relatively few manuscripts are rejected. This is not necessarily the best indicator of quality, however, since newer journals may not be sufficiently familiar to authors with high quality manuscripts and some journals are very specific in terms of what they publish. Another index that is sometimes used is the journal’s impact factor . Impact factor is a quantitative number indicative of how often articles published in the journal are cited in the reference list of other journal articles—the statistic is calculated as the number of times on average each article published in a particular year were cited divided by the number of articles published (the number that could be cited). For example, the impact factor for the Journal of Poverty and Social Justice in our list above was 0.70 in 2017, and for the Journal of Poverty was 0.30. These are relatively low figures compared to a journal like the New England Journal of Medicine with an impact factor of 59.56! This means that articles published in that journal were, on average, cited more than 59 times in the next year or two.
Impact factors are not necessarily the best indicator of caliber, however, since many strong journals are geared toward practitioners rather than scholars, so they are less likely to be cited by other scholars but may have a large impact on a large readership. This may be the case for a journal like the one titled Social Work, the official journal of the National Association of Social Workers. It is distributed free to all members: over 120,000 practitioners, educators, and students of social work world-wide. The journal has a recent impact factor of.790. The journals with social work relevant content have impact factors in the range of 1.0 to 3.0 according to Scimago Journal & Country Rank (SJR), particularly when they are interdisciplinary journals (for example, Child Development , Journal of Marriage and Family , Child Abuse and Neglect , Child Maltreatmen t, Social Service Review , and British Journal of Social Work ). Once upon a time, a reader could locate different indexes comparing the “quality” of social work-related journals. However, the concept of “quality” is difficult to systematically define. These indexes have mostly been replaced by impact ratings, which are not necessarily the best, most robust indicators on which to rely in assessing journal quality. For example, new journals addressing cutting edge topics have not been around long enough to have been evaluated using this particular tool, and it takes a few years for articles to begin to be cited in other, later publications.
Beware of pseudo-, illegitimate, misleading, deceptive, and suspicious journals . Another side effect of living in the Age of Information is that almost anyone can circulate almost anything and call it whatever they wish. This goes for “journal” publications, as well. With the advent of open-access publishing in recent years (electronic resources available without subscription), we have seen an explosion of what are called predatory or junk journals . These are publications calling themselves journals, often with titles very similar to legitimate publications and often with fake editorial boards. These “publications” lack the integrity of legitimate journals. This caution is reminiscent of the discussions earlier in the course about pseudoscience and “snake oil” sales. The predatory nature of many apparent information dissemination outlets has to do with how scientists and scholars may be fooled into submitting their work, often paying to have their work peer-reviewed and published. There exists a “thriving black-market economy of publishing scams,” and at least two “journal blacklists” exist to help identify and avoid these scam journals (Anderson, 2017).
This issue is important to information consumers, because it creates a challenge in terms of identifying legitimate sources and publications. The challenge is particularly important to address when information from on-line, open-access journals is being considered. Open-access is not necessarily a poor choice—legitimate scientists may pay sizeable fees to legitimate publishers to make their work freely available and accessible as open-access resources. On-line access is also not necessarily a poor choice—legitimate publishers often make articles available on-line to provide timely access to the content, especially when publishing the article in hard copy will be delayed by months or even a year or more. On the other hand, stating that a journal engages in a peer-review process is no guarantee of quality—this claim may or may not be truthful. Pseudo- and junk journals may engage in some quality control practices, but may lack attention to important quality control processes, such as managing conflict of interest, reviewing content for objectivity or quality of the research conducted, or otherwise failing to adhere to industry standards (Laine & Winker, 2017).
One resource designed to assist with the process of deciphering legitimacy is the Directory of Open Access Journals (DOAJ). The DOAJ is not a comprehensive listing of all possible legitimate open-access journals, and does not guarantee quality, but it does help identify legitimate sources of information that are openly accessible and meet basic legitimacy criteria. It also is about open-access journals, not the many journals published in hard copy.
An additional caution: Search for article corrections. Despite all of the careful manuscript review and editing, sometimes an error appears in a published article. Most journals have a practice of publishing corrections in future issues. When you locate an article, it is helpful to also search for updates. Here is an example where data presented in an article’s original tables were erroneous, and a correction appeared in a later issue.
- Marchant, A., Hawton, K., Stewart A., Montgomery, P., Singaravelu, V., Lloyd, K., Purdy, N., Daine, K., & John, A. (2017). A systematic review of the relationship between internet use, self-harm and suicidal behaviour in young people: The good, the bad and the unknown. PLoS One, 12(8): e0181722. https://www.ncbi.nlm.nih.gov/pmc/articles/PMC5558917/
- Marchant, A., Hawton, K., Stewart A., Montgomery, P., Singaravelu, V., Lloyd, K., Purdy, N., Daine, K., & John, A. (2018).Correction—A systematic review of the relationship between internet use, self-harm and suicidal behaviour in young people: The good, the bad and the unknown. PLoS One, 13(3): e0193937. http://journals.plos.org/plosone/article?id=10.1371/journal.pone.0193937
Search Tools. In this age of information, it is all too easy to find items—the problem lies in sifting, sorting, and managing the vast numbers of items that can be found. For example, a simple Google® search for the topic “community poverty and violence” resulted in about 15,600,000 results! As a means of simplifying the process of searching for journal articles on a specific topic, a variety of helpful tools have emerged. One type of search tool has previously applied a filtering process for you: abstracting and indexing databases . These resources provide the user with the results of a search to which records have already passed through one or more filters. For example, PsycINFO is managed by the American Psychological Association and is devoted to peer-reviewed literature in behavioral science. It contains almost 4.5 million records and is growing every month. However, it may not be available to users who are not affiliated with a university library. Conducting a basic search for our topic of “community poverty and violence” in PsychINFO returned 1,119 articles. Still a large number, but far more manageable. Additional filters can be applied, such as limiting the range in publication dates, selecting only peer reviewed items, limiting the language of the published piece (English only, for example), and specified types of documents (either chapters, dissertations, or journal articles only, for example). Adding the filters for English, peer-reviewed journal articles published between 2010 and 2017 resulted in 346 documents being identified.
Just as was the case with journals, not all abstracting and indexing databases are equivalent. There may be overlap between them, but none is guaranteed to identify all relevant pieces of literature. Here are some examples to consider, depending on the nature of the questions asked of the literature:
- Academic Search Complete—multidisciplinary index of 9,300 peer-reviewed journals
- AgeLine—multidisciplinary index of aging-related content for over 600 journals
- Campbell Collaboration—systematic reviews in education, crime and justice, social welfare, international development
- Google Scholar—broad search tool for scholarly literature across many disciplines
- MEDLINE/ PubMed—National Library of medicine, access to over 15 million citations
- Oxford Bibliographies—annotated bibliographies, each is discipline specific (e.g., psychology, childhood studies, criminology, social work, sociology)
- PsycINFO/PsycLIT—international literature on material relevant to psychology and related disciplines
- SocINDEX—publications in sociology
- Social Sciences Abstracts—multiple disciplines
- Social Work Abstracts—many areas of social work are covered
- Web of Science—a “meta” search tool that searches other search tools, multiple disciplines
Placing our search for information about “community violence and poverty” into the Social Work Abstracts tool with no additional filters resulted in a manageable 54-item list. Finally, abstracting and indexing databases are another way to determine journal legitimacy: if a journal is indexed in a one of these systems, it is likely a legitimate journal. However, the converse is not necessarily true: if a journal is not indexed does not mean it is an illegitimate or pseudo-journal.
Government Sources. A great deal of information is gathered, analyzed, and disseminated by various governmental branches at the international, national, state, regional, county, and city level. Searching websites that end in.gov is one way to identify this type of information, often presented in articles, news briefs, and statistical reports. These government sources gather information in two ways: they fund external investigations through grants and contracts and they conduct research internally, through their own investigators. Here are some examples to consider, depending on the nature of the topic for which information is sought:
- Agency for Healthcare Research and Quality (AHRQ) at https://www.ahrq.gov/
- Bureau of Justice Statistics (BJS) at https://www.bjs.gov/
- Census Bureau at https://www.census.gov
- Morbidity and Mortality Weekly Report of the CDC (MMWR-CDC) at https://www.cdc.gov/mmwr/index.html
- Child Welfare Information Gateway at https://www.childwelfare.gov
- Children’s Bureau/Administration for Children & Families at https://www.acf.hhs.gov
- Forum on Child and Family Statistics at https://www.childstats.gov
- National Institutes of Health (NIH) at https://www.nih.gov , including (not limited to):
- National Institute on Aging (NIA at https://www.nia.nih.gov
- National Institute on Alcohol Abuse and Alcoholism (NIAAA) at https://www.niaaa.nih.gov
- National Institute of Child Health and Human Development (NICHD) at https://www.nichd.nih.gov
- National Institute on Drug Abuse (NIDA) at https://www.nida.nih.gov
- National Institute of Environmental Health Sciences at https://www.niehs.nih.gov
- National Institute of Mental Health (NIMH) at https://www.nimh.nih.gov
- National Institute on Minority Health and Health Disparities at https://www.nimhd.nih.gov
- National Institute of Justice (NIJ) at https://www.nij.gov
- Substance Abuse and Mental Health Services Administration (SAMHSA) at https://www.samhsa.gov/
- United States Agency for International Development at https://usaid.gov
Each state and many counties or cities have similar data sources and analysis reports available, such as Ohio Department of Health at https://www.odh.ohio.gov/healthstats/dataandstats.aspx and Franklin County at https://statisticalatlas.com/county/Ohio/Franklin-County/Overview . Data are available from international/global resources (e.g., United Nations and World Health Organization), as well.
Other Sources. The Health and Medicine Division (HMD) of the National Academies—previously the Institute of Medicine (IOM)—is a nonprofit institution that aims to provide government and private sector policy and other decision makers with objective analysis and advice for making informed health decisions. For example, in 2018 they produced reports on topics in substance use and mental health concerning the intersection of opioid use disorder and infectious disease, the legal implications of emerging neurotechnologies, and a global agenda concerning the identification and prevention of violence (see http://www.nationalacademies.org/hmd/Global/Topics/Substance-Abuse-Mental-Health.aspx ). The exciting aspect of this resource is that it addresses many topics that are current concerns because they are hoping to help inform emerging policy. The caution to consider with this resource is the evidence is often still emerging, as well.
Numerous “think tank” organizations exist, each with a specific mission. For example, the Rand Corporation is a nonprofit organization offering research and analysis to address global issues since 1948. The institution’s mission is to help improve policy and decision making “to help individuals, families, and communities throughout the world be safer and more secure, healthier and more prosperous,” addressing issues of energy, education, health care, justice, the environment, international affairs, and national security (https://www.rand.org/about/history.html). And, for example, the Robert Woods Johnson Foundation is a philanthropic organization supporting research and research dissemination concerning health issues facing the United States. The foundation works to build a culture of health across systems of care (not only medical care) and communities (https://www.rwjf.org).
While many of these have a great deal of helpful evidence to share, they also may have a strong political bias. Objectivity is often lacking in what information these organizations provide: they provide evidence to support certain points of view. That is their purpose—to provide ideas on specific problems, many of which have a political component. Think tanks “are constantly researching solutions to a variety of the world’s problems, and arguing, advocating, and lobbying for policy changes at local, state, and federal levels” (quoted from https://thebestschools.org/features/most-influential-think-tanks/ ). Helpful information about what this one source identified as the 50 most influential U.S. think tanks includes identifying each think tank’s political orientation. For example, The Heritage Foundation is identified as conservative, whereas Human Rights Watch is identified as liberal.
While not the same as think tanks, many mission-driven organizations also sponsor or report on research, as well. For example, the National Association for Children of Alcoholics (NACOA) in the United States is a registered nonprofit organization. Its mission, along with other partnering organizations, private-sector groups, and federal agencies, is to promote policy and program development in research, prevention and treatment to provide information to, for, and about children of alcoholics (of all ages). Based on this mission, the organization supports knowledge development and information gathering on the topic and disseminates information that serves the needs of this population. While this is a worthwhile mission, there is no guarantee that the information meets the criteria for evidence with which we have been working. Evidence reported by think tank and mission-driven sources must be utilized with a great deal of caution and critical analysis!
In many instances an empirical report has not appeared in the published literature, but in the form of a technical or final report to the agency or program providing the funding for the research that was conducted. One such example is presented by a team of investigators funded by the National Institute of Justice to evaluate a program for training professionals to collect strong forensic evidence in instances of sexual assault (Patterson, Resko, Pierce-Weeks, & Campbell, 2014): https://www.ncjrs.gov/pdffiles1/nij/grants/247081.pdf . Investigators may serve in the capacity of consultant to agencies, programs, or institutions, and provide empirical evidence to inform activities and planning. One such example is presented by Maguire-Jack (2014) as a report to a state’s child maltreatment prevention board: https://preventionboard.wi.gov/Documents/InvestmentInPreventionPrograming_Final.pdf .
When Direct Answers to Questions Cannot Be Found. Sometimes social workers are interested in finding answers to complex questions or questions related to an emerging, not-yet-understood topic. This does not mean giving up on empirical literature. Instead, it requires a bit of creativity in approaching the literature. A Venn diagram might help explain this process. Consider a scenario where a social worker wishes to locate literature to answer a question concerning issues of intersectionality. Intersectionality is a social justice term applied to situations where multiple categorizations or classifications come together to create overlapping, interconnected, or multiplied disadvantage. For example, women with a substance use disorder and who have been incarcerated face a triple threat in terms of successful treatment for a substance use disorder: intersectionality exists between being a woman, having a substance use disorder, and having been in jail or prison. After searching the literature, little or no empirical evidence might have been located on this specific triple-threat topic. Instead, the social worker will need to seek literature on each of the threats individually, and possibly will find literature on pairs of topics (see Figure 3-1). There exists some literature about women’s outcomes for treatment of a substance use disorder (a), some literature about women during and following incarceration (b), and some literature about substance use disorders and incarceration (c). Despite not having a direct line on the center of the intersecting spheres of literature (d), the social worker can develop at least a partial picture based on the overlapping literatures.
Figure 3-1. Venn diagram of intersecting literature sets.
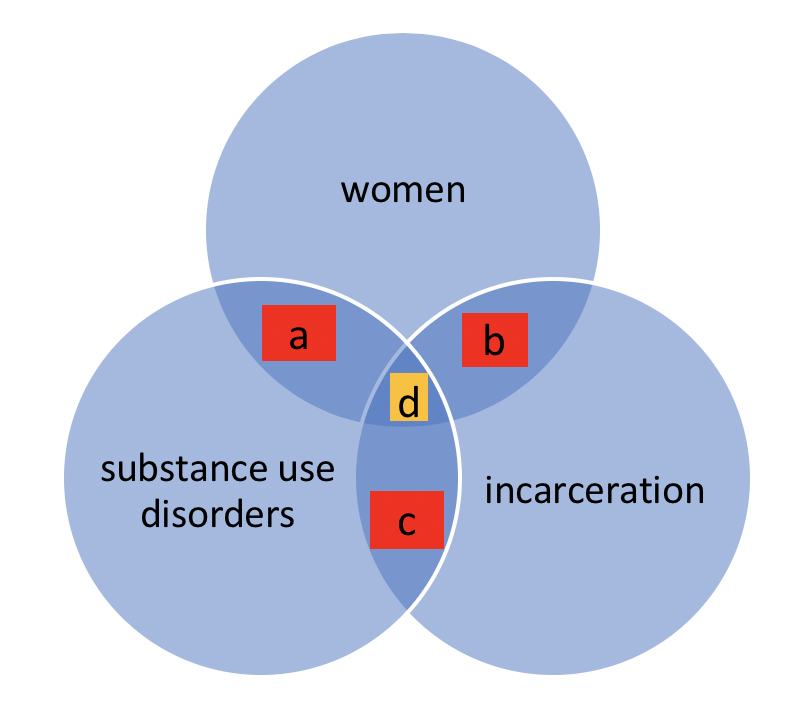
Take a moment to complete the following activity. For each statement about empirical literature, decide if it is true or false.
Social Work 3401 Coursebook Copyright © by Dr. Audrey Begun is licensed under a Creative Commons Attribution-NonCommercial-NoDerivatives 4.0 International License , except where otherwise noted.
Share This Book

The difference between empirical and discussion chapters (and how to write them)
Jun 7, 2019

It is a common misconception that the empirical chapters are the place for your analysis. Often this confuses the reader. In fact, you need to split the empirical facts and discussion of those facts into two distinct sections.
In this post I want to explain why, more often that not, you need have separate empirical and discussion chapters and why you shouldn’t combine them. Then, I’ll talk you through practical steps to employ when you’re writing each up.
What is the difference between an empirical and discussion chapter?
Whilst they are closely related, they occupy two very different spaces within a PhD thesis.
We can understand a PhD as having four distinct sections :
1. Introduction – this is where you introduce and outline the entire study.
2. Background – this where you lay the groundwork for your thesis (in your literature review, theory framework and methods).
3. Core – this is where you present your findings.
4. Synthesis – this is where you relate the core to the background.
The empirical chapter(s) is/are where you present the facts of your study. They occupy the core of the thesis.
The discussion chapter though is where you interpret and discuss your findings in relation to the thesis and wider discipline. That is why is occupies the synthesis stage of the research.
Your job when writing your discussion then is to interrogate and critically engage with the findings and relate them to the research aims, objectives, research questions and gap. Most of your cutting-edge analysis and engagement with your findings will take place here.
The job of a discussion chapter is therefore to critically examine your findings with reference to the discussion in the background chapters of the thesis (introduction, literature review, theoretical framework and methods) and to make judgments as to what has been learnt in your work. In essence, the job of a discussion chapter is to tell the readers what your findings (may) mean.
The reason for this distinction between empirics and discussion is to make life easier for your examiner. They are looking at whether you are capable of both presenting observations in line with your methodology, and interpreting their significance in the context of the thesis as a whole.
However, as with everything in the PhD, there will be exceptions to this. Some theses, particularly those from within the liberal arts and social sciences, may not need a separate discussion section because the nature of their study may mean that empirics and discussion are intertwined. It is also worth pointing out that although not every thesis will have a discussion chapter, all theses will contain discussions of some sort, however short.
How do I write an empirical chapter?
It’s important to note that the form your empirical chapter will take – or indeed if you have one at all – will depend heavily on the nature of your thesis and the discipline in which you’re working. Someone working in a more theoretical space – for example, philosophers or the theoretical mathematicians – might rely less on first-hand empirical data and more on proofs. Those working on quantitative studies will have more data-driven, empiric-heavy empirical chapters.
Broadly speaking then, the emphasis in the empirical section or chapter is on factual recount and summary. You’ll be categorising your findings into particular themes and using a variety of visual elements (tables, figures, charts, and so on) to present your results. You need to show the reader what your data ‘looks like’.
You need to do it well, too. If you present data in a messy way, your examiner might think that your thinking is messy.
By the time you have finished your empirical chapter, your reader should be able to answer six questions:
1. What are the results of your investigations?
2. How do the findings relate to previous studies?
3. Was there anything surprising or that didn’t work out as planned?
4. Are there any themes or categories that emerge from the data?
5. Have you explained to the reader why you have reached particular conclusions?
6. Have you explained the results?
You are providing sufficient detail that others can draw their own inferences and construct their own explanations. Think of it as presenting the case for a jury.
That means that an empirical discussion should:
1. Tell the reader how the data was collected, with reference to the methods chapter/section
2. Tell them how they can access it if they wanted to replicate your study
3. Discuss what the results look like (using visual aids, such as tables, diagrams, graphs and so on)
4. Provide rich summaries of the findings
5. Discuss the gaps in the findings and analysis
6. Analyse the results
7. Discuss the implications of your findings
8. Discuss the limitations of the findings

Your PhD thesis. All on one page.
Use our free PhD structure template to quickly visualise every element of your thesis.
How do I write a discussion chapter?
Many students struggle to write up their discussion chapters . The reason is that they lack the confidence needed to make the kinds of knowledge claims required. The discussion chapter is where you start to develop your scholarly authority, and where you start to make truth claims about your interpretation of what’s going on. By implication, that means it is where you start to agree or disagree with existing literature and theoretical ideas.
Another reason why students struggle is that they fail to realise the significance of their findings or, put differently, they don’t think their findings are significant enough in their own right. By the time we come to write our discussion, we are so conditioned by the findings that we may not realise that they are significant and do, in fact, make a contribution. There’s often an expectation gap here; students expect their contribution to be big, whereas, more often than not, the contribution a PhD actually makes is small and specific.
As with every stage of your thesis, you must relate your discussion section/chapter to the background. Specifically, you need to relate it to the empirical chapter, aims and objectives, research questions, the gap in the literature and, if relevant, your theoretical framework. There will, therefore, be a lot of signposting to other parts of the thesis; doing so is necessary if you want to show the examiner that you can relate your findings to the broader context of your thesis and discipline.
One of the biggest obstacles is synthesising your empirical data and being able to critically discuss it in relation to this broader context. The authors of How To Write A Better Thesis offer up an effective technique you can use if you’re struggling to do this. They refer to it as a ‘mud-map’:
1. You can start by writing a long list of everything you have found.
2. See if you can sort and organise this list. Categorise each finding based on whether it is speculative or based in empirical fact. This is important because your discussion will need to be somewhat (but not too) speculative.
3. Try to categorise your different findings into themes.
4. Now try to find linkages between these themes.
5. Organise these themes into different section headings for the discussion chapter, and try to come up with sub-headings.
When it comes to writing your discussion chapter, you can start by writing a few sentences that summarise the most important results.
One danger when writing discussion sections is that they can be too wordy, offer too much interpretation and lack a clear structure. To avoid this, you should make sure that every element of your discussion section addresses one of the following questions:
- What are the relationships between observations? (The mud-map you developed earlier will help here.)
- Are there any trends and generalisations amongst the results? Are there any exceptions to these?
- What are the causes of, or mechanisms behind, the underlying patterns you have uncovered?
- Do your results agree or disagree with previous work?
- How do your findings relate to the theoretical framework you developed, if applicable?
- How do the findings relate to the hypotheses you developed, if applicable?
- What other explanations could there be for your results? This issue is more pertinent if you are engaging in theory creation/inductive reasoning.
- What do we now know as a result of your research that we didn’t know before?
- What is the significance of these findings?
- Why should we care about the findings?
When your discussion chapter is finished, your reader should be able to answer the following questions:
1. How do the findings relate to the theory and methods discussed previously?
2. Why you have reached particular conclusions?
3. How do your findings relate to the gaps in the literature you identified earlier?
4. What implications do the findings have for the discipline and for existing understanding?
5. How do the findings relate to your research questions/aims and objectives?
A particularly important theme that I want you to always bear in mind is that your interpretation and discussion of the findings needs to be done in such a way that it relates back to the aims, objectives, research questions, gap and any theory. Running through your thesis will be a central argument – your thesis statement – and it is in the empirical and, particularly, the discussion chapter, that you will present all of the evidence and logical argument necessary to support that argument.
Unsurprisingly then, these core sections of the thesis are the most important, as they are where you make your contribution. Of course, you have outlined what your contribution is in the introduction, so what you are arguing is no surprise. But, it is in the core of the thesis where you drill down into the detail and critically engage with that contribution, using your data to rigorously support your line of argument.
Hello, Doctor…
Sounds good, doesn’t it? Be able to call yourself Doctor sooner with our five-star rated How to Write A PhD email-course. Learn everything your supervisor should have taught you about planning and completing a PhD.
Now half price. Join hundreds of other students and become a better thesis writer, or your money back.
Share this:
10 comments.
Really great advice. I love simple, clear guidance. Especially when writing something that’s pretty complicated!!
Thanks for the kind words. I’m glad you found it useful.
It’s really guiding me a lot.
This is really great. I have had serious challenges putting up a convincing empirical chapter.
Glad you enjoyed it!
Very insightful guidelines for PhD candidates. So appreciative for such work.
Thanks for the kind words. I’m glad you’re finding the resources useful.
It’s really useful guidance. It helps a lot in simplifying the complex and messy ideas when starting the writing process.
Thanks for the kind words.
Very instructive.
Submit a Comment Cancel reply
Your email address will not be published. Required fields are marked *

Search The PhD Knowledge Base
Most popular articles from the phd knowlege base.

The PhD Knowledge Base Categories
- Your PhD and Covid
- Mastering your theory and literature review chapters
- How to structure and write every chapter of the PhD
- How to stay motivated and productive
- Techniques to improve your writing and fluency
- Advice on maintaining good mental health
- Resources designed for non-native English speakers
- PhD Writing Template
- Explore our back-catalogue of motivational advice
EDITORIAL article
Editorial: theory and empirical practice in research on social and emotional skills.
- 1 Organisation for Economic Co-Operation and Development, Paris, France
- 2 Department of Developmental, Personality and Social Psychology, Faculty of Psychology and Educational Sciences, Ghent University, Ghent, Belgium
- 3 Universidade São Francisco (USF), São Paulo, Brazil
Editorial on the Research Topic Theory and empirical practice in research on social and emotional skills
Introduction to the Research Topic
Social and emotional skills—also called non-cognitive skills, soft skills, character strengths, etc.—have drawn increasing attention from policymakers, practitioners and researchers over recent years. Our ability to manage our emotions, connect with others in a respectful and meaningful way, act responsively and understand and appreciate others' points of view are essential in successfully navigating our social worlds. The importance of these capacities was further amplified by several global trends that are fundamentally reshaping the social fabrics and interpersonal relationships. These include growing inter-connectivity, population diversity, complexity and variability of job requirements, the ever-accelerating pace of technological changes, the dismantling of old social networks, etc. The unprecedented social changes introduced by the COVID-19 pandemic have also shown the critical role these skills have in people's ability to adjust to new social and professional situations. With the expected continuation of current societal and technological trends, these skills will likely become even more pivotal in the future (hence they are sometimes also called “21st-century skills”).
Research on social and emotional skills is multi-disciplinary, combining a unique mix of academic and practice-oriented research approaches. It features both theoretical and empirical research streams, with lively discussions on the conceptual foundation and structure of these skills, their origin, development, malleability, and relevance. In this Research Topic, we have tried to reflect the diversity of the field's perspectives, disciplines, and methodological approaches and its unique blend of research and policy. The Research Topic contains empirical and theoretical works representing both streams of research on social and emotional skills. It also presents both academic and practice-oriented studies on a variety of topics in the field. Still, studies presented in this Research Topic place a particular emphasis on the issue of the application of gathered academic knowledge in educational settings. Selected studies do so either through examining current states, developing policy interventions, or evaluating their effectiveness.
Studies presented in this Research Topic examine the concept of social and emotional skills and their malleability and main determinants. The effectiveness of intervention programmes designed for the improvement of these skills is also analyzed. A particular emphasis is placed on the issue of the application of gathered knowledge in educational settings. Finally, several articles investigate the issue of valid and reliable assessment of these skills and the new developments in this aspect. Overall, the Research Topic offers a rich set of approaches, perspectives, methodologies and concepts examined and discussed by some of the leading researchers in the field. We hope that this Research Topic will contribute to the current discussions and developments in this lively field of research, offering new insights and ideas for further research.
Description of published articles
Improving classroom communication: the effects of virtual social training on communication and assertion skills in middle school students.
This article ( Johnson et al. ) focuses on one of the key policy premises regarding social and emotional skills—their potential to improve students' learning in academic settings. The study examined the possibility of improving educational processes and outcomes by training students' social and emotional skills. Findings showed that the use of the training in a virtual social environment could positively impact students' social confidence and behavior in the classroom. The special importance of this study is the possibility of successful training of social skills in an age group in which anxiety, social anxiety, and difficulties in interacting with peers develop very frequently. The involvement of teachers and innovative use of digital technologies are other promising aspects of this study.
Teaching socio-emotional competencies among primary school students
This is another study exploring the possibility of developing social and emotional skills in school settings through formal training programs ( Santamaría-Villar et al. ). Such studies are badly needed in this area as robust evaluations of intervention programs that aim developing students' social and emotional skills are still relatively scarce. Nevertheless, the study findings are positive, with the designed educational program leading to improvements in social behaviors of third-grade students, accompanied by improved awareness of aggressive behaviors in school and greater coexistence among students. Another important aspect of this study is that the intervention itself-−15 lessons in the form of school classes—is applicable and practical for a wide variety of school contexts.
Social, emotional, and behavioral skills: An integrative model of the skills associated with success during adolescence and across the life span
The authors of this paper offer a new, innovative and thought-provoking understanding of social and emotional skills and how they develop and correspond with various life outcomes. The article ( Napolitano et al. ) describes a new conceptualization of social, emotional, and behavioral skills outlined in an integrative model encompassing five related functioning domains. The paper discusses some of the key questions related to these concepts, such as their relations with personality traits, the distinction between maximum performance vs. typical performance perspective, skills' developmental trajectories and milestones, their practical importance, etc. One of the interesting features of the suggested model is that it includes only those skills that are found to be malleable throughout life, thus representing a very relevant framework from the policymaking point of view.
Toward a model of personality competencies underlying social and emotional skills: Insight from the circumplex of personality metatraits
This article ( Cieciuch and Strus ) offers an interesting and thorough theoretical analysis and conceptualization of social and emotional skills. The authors engage in an in-depth examination of various theoretical issues with no hesitation and with the required knowledge and expertise. Readers have a chance to familiarize themselves with a plethora of conceptual approaches and main issues in this area. Authors introduce their model and argue convincingly for the newly introduced theoretical solutions. Interested readers will have plenty of “food for thought” after reading this paper, hopefully inspiring them to examine some of its main postulates further.
Temperament and school readiness—A literature review
The meta-analytic reviews are a very useful and increasingly used approach in summarizing empirical findings in a particular area of research. In this article ( Potmesilova and Potmesil ), the authors employ the meta-analytic approach to discern whether temperamental characteristics (here defined through concepts of executive function, effortful control and self-regulation) of children are related to their school readiness. Based on a substantive sample of empirical studies, the authors find that both positive and negative emotionality influence school behavior of children (beneficially or adversely, respectively), especially their ability to focus and, consequently, their learning outcomes. Such findings place special importance on the quality of classroom environments, as discussed in the paper.
Age-specific life skills education in school: A systematic review
Life skill programs developed for adolescents may differ in both the targeted skills and the influencing factors on health and wellbeing they have as their focus ( Kirchhoff and Keller ). This review summarizes the impact of 18 evaluation studies published between 2007 and 2020. The review is timely and critical because, from a policy perspective, one needs to know what is the most sensitive period for particular skills to be promoted at schools, taking into account adolescents' biological and psychological development and changing environmental dynamics. Most programs start in Grades 5–6 or Grades 7–9, but the development of skills was only studied in a few programs, often demonstrating zero effects but also some positive findings. Objectives of health-oriented programs vary depending on student age. The review closes by identifying several research gaps to improve evidence-based work in this flourishing field.
Impacts of social and emotional learning interventions for teachers on teachers' outcomes: A systematic review with meta-analysis
The growing consideration for the development of students' social-emotional learning also directed the attention to teachers' social-emotional skills. Teachers' professional and personal functioning should be a top priority of policymakers, given the importance of teachers for students' academic achievement and the current dearth of teachers in the labor market due to many professionals leaving the job. This meta-analysis ( Oliveira et al. ) is the first to summarize social-emotional learning interventions reported in 43 empirical studies targeting the skills of teachers. Results are promising, showing small to medium effects of interventions on both teachers' skills and mental health outcomes. These results strongly encourage the implementation of such interventions in teacher training and development programs to strengthen teachers' personal and professional outcomes.
Equity in social emotional learning (SEL) programs: A content analysis of equitable practices in PreK-5 SEL programs
Although most social-emotional learning programs in education target the development of skills in students to foster collaboration and appreciation of diversity and cultural differences, this does not guarantee that the programs and their background, content and methods reflect the values and experiences of diverse populations. This review ( Ramirez et al. ) proposes a number of key perspectives that should form the basis and can be integrated into social-emotional learning and practice to align social-emotional learning and educational equity better. It is argued that social-emotional learning should be more than just an appreciation of diversity. It also involves a reciprocal process where diversity and equity affect the core of social-emotional learning programs questioning dominant and oppressing positions also aiming for social justice advocacy and positive identity development for all.
Formative assessment of social-emotional skills using rubrics: A review of knowns and unknowns
Social-emotional learning often involves the use of formative assessment tools to examine students' development of skills. Students' standing on various skills is often monitored using rubrics describing increasing levels of mastery of a skill. This paper ( Pancorbo et al. ) discusses the requirements for skill rubrics, reviews what we know and should know, and sketches a research agenda on how the reliability and validity of rubrics can be enhanced to better serve formative assessment of social-emotional learning in education.
SENNA inventory for the assessment of social and emotional skills in public school students in Brazil: Measuring both identity and self-efficacy
Although several inventories already exist to assess social-emotional skills in students, most reflect a specific model of social-emotional learning and are developed within Western cultures. The SENNA inventory, however, was developed to assess a comprehensive framework of social-emotional skills in students attending public schools in Brazil ( Primi et al. ). The inventory assesses skills from a dual perspective, i.e., “how well students can manifest a skill (self-efficacy)” and “how typically they use that skill” (identity). Psychometric data are reported from a large-scale assessment program in Brazil, and techniques to reduce answering tendencies are demonstrated to improve the reliability and structural validity of the assessments.
Two forms of social inequality in students' socio-emotional skills: Do the levels of big five personality traits and their associations with academic achievement depend on parental socioeconomic status?
It is common for policymakers to advocate developing socio-emotional skills to accelerate the learning of disadvantaged students. However, what empirical evidence supports these links between socio-economic status (SES), social and emotional skills and academic achievement? In this paper ( Lechner et al. ), the underlying assumptions of this argument are made explicit and testable, in a unique contribution to the field. The authors report that their empirical study provides little evidence of the link between social inequality and social and emotional skills, as well as of a differentiable relationship, that is, no evidence that social and emotional skills and achievement are strongly related among socially disadvantaged children. A causal relationship such as this would justify the development of social skills as a means to reduce inequality. Curiously the authors found the opposite effect (a slightly strong association between conscientiousness and achievement for students with high SES). It is an inspirational paper to the studies investigating the interplay between SES, social and emotional skills, and children's achievement.
To score or not to score? A simulation study on the performance of test scores, plausible values, and SEM, in regression with socio-emotional skill or personality scales as predictors
This paper makes an important methodological contribution to determining the correct statistical method to use when estimating factor scores when assessing social and emotional skills. Even though all techniques generate highly correlated scores, indicating that students' order on the skills should be roughly the same regardless of the technique used, the authors demonstrated that different methods are important in terms of criterion validity. That is because some methods suppress correlations with external criteria, which have important policy consequences. A unique feature of this study ( Bhaktha and Lechner ) is the systematic use of criterion validity for determining the psychometric quality of measures. Generally, this judgment is based on internal structural properties only (reliability and item loadings on the intended factor). The authors recommended Plausible Values or the Structural Equation Method among all the tested methods. This study hopefully will encourage programs to consider criterion validity as a systematic method for studying the psychometric properties of social and emotional skill assessments.
Making space for social and emotional learning in science education
With a practical perspective, this paper offers an original contribution based on a highly arousing experience of learning about arthropods in a biology curriculum. As entomologists and educators, the authors ask, “Can you imagine bringing a tarantula or a large insect into a classroom and students NOT having an emotional response? (p. 2). This study ( Ingram et al. ) aimed to test the integration of social-emotional learning into the STEM curriculum. The program intends to use the experience to promote social and emotional skills, such as empathy (fostering sympathy not only for the arthropods but for all people with a variety of experiences), self-reflection, self-management, etc. Additionally, it seeks to improve student engagement with STEM courses. Students' qualitative responses were analyzed before and after the program. The results demonstrated that students' emotional reactions shifted from negative to positive, and their engagement with the curriculum improved. Such findings are an interesting and creative demonstration that SEL can be incorporated into a diverse curriculum not commonly studied in the field of SEL.
Social and emotional learning in preschool settings: A systematic map of systematic reviews
A very important target of the development of social and emotional skills is achieved through early childhood education. This study ( Djamnezhad et al. ) aimed to identify high-quality systematic reviews of early childhood SEL interventions. Surprisingly, the authors found only two well-designed systematic reviews, leading to the critical conclusion that there are few reviews of SEL interventions in early education. In both reviews, the effect of universal SEL interventions was reported to positively impact several outcomes. However, because of methodological limitations, the authors recommend considering these results as tentative interpretations and suggest that this field is a knowledge gap. Hopefully, this study will encourage the development of sounding reviews of SEL interventions in early childhood.
Author contributions
All authors listed have made a substantial, direct, and intellectual contribution to the work and approved it for publication.
Conflict of interest
The authors declare that the research was conducted in the absence of any commercial or financial relationships that could be construed as a potential conflict of interest.
Publisher's note
All claims expressed in this article are solely those of the authors and do not necessarily represent those of their affiliated organizations, or those of the publisher, the editors and the reviewers. Any product that may be evaluated in this article, or claim that may be made by its manufacturer, is not guaranteed or endorsed by the publisher.
Keywords: social and emotional skills, social and emotional learning (SEL), non-cognitive skills, skill development, school intervention programs, skill assessment, social and emotional behavior, 21st century skills
Citation: Kankaraš M, de Fruyt F and Primi R (2022) Editorial: Theory and empirical practice in research on social and emotional skills. Front. Educ. 7:993878. doi: 10.3389/feduc.2022.993878
Received: 14 July 2022; Accepted: 26 July 2022; Published: 11 August 2022.
Edited and reviewed by: Matteo Angelo Fabris , University of Turin, Italy
Copyright © 2022 Kankaraš, de Fruyt and Primi. This is an open-access article distributed under the terms of the Creative Commons Attribution License (CC BY) . The use, distribution or reproduction in other forums is permitted, provided the original author(s) and the copyright owner(s) are credited and that the original publication in this journal is cited, in accordance with accepted academic practice. No use, distribution or reproduction is permitted which does not comply with these terms.
*Correspondence: Miloš Kankaraš, milos306@yahoo.com
Disclaimer: All claims expressed in this article are solely those of the authors and do not necessarily represent those of their affiliated organizations, or those of the publisher, the editors and the reviewers. Any product that may be evaluated in this article or claim that may be made by its manufacturer is not guaranteed or endorsed by the publisher.
2024 Theses Doctoral
Mortality Myths?: Testing the Claims of the Theory of Deaths of Despair
Segura, Luis Esteban
A groundbreaking narrative, which would come to be known as the theory of “deaths of despair”, emerged in 2015 from a study by Case and Deaton analyzing mortality rates in the United States between 1999 and 2013. They found an increasing trend in all-cause mortality rates due to drug poisonings, alcohol-related liver disease, and suicides, which they called “deaths of despair”, among non-Hispanic (NH) white Americans aged 45 to 54—this age group was called the midlife. Case and Deaton’s findings and their narrative about the hypothetical causes of their findings garnered significant attention. The authors of this narrative hypothesized that the observed increases in mortality rates were due to white individuals in midlife increasingly suffering from “despair” and proposed a causal link between increasing “despair” rates and increased mortality rates only among white Americans in midlife. Case and Deaton did not provide a clear definition of “despair”; they presumed that white Americans in midlife were hopeless about their prospects for the future compared to what their parents had attained. This provocative narrative persisted and gained momentum because it functioned as an explanation of recent events like the 2016 U.S. presidential election, rise in white nationalism, and far right extremism. These white-related events were thought to be expressions of an agonizing, poor, under-educated generation of white Americans increasingly suffering from hypothetical feelings of "despair”, which have led them to self-destructive behaviors and premature death. However, no study has investigated the central claim of this theory: whether there is evidence of an association between increased “despair” rates and increased mortality rates only among white individuals in midlife, particularly for those with low education. Moreover, there is little evidence of their hypothesis of an increasing epidemic of “despair” affecting only white Americans in midlife, particularly those with low education. The theory of “deaths of despair” can be understood through Geoffrey Rose’s framework of causes of incidence and causes of cases, which highlights the difference between between-population and inter-individual causes of disease. Rose’s argues that causes of incidence explain the changes in outcome rates between populations, and may be uniform and imperceptible within populations. On the other hand, the causes of cases explain why some individuals within a population are susceptible or at high risk of the outcome. Like Rose’s causes of incidence, the authors of the theory of “deaths of despair” argue that “despair” increased between the midlife white American population in 1999 and in 2014, which led to increased mortality rates. Conversely, this theory does not claim that some individuals are at higher risk of death due to “despair”, which would be analogous to causes of cases. Therefore, the contrast of interest to test the central claim of Case and Deaton’s theory of “deaths of despair” is a between-population contrast (causes of incidence). As such, this dissertation aims to test the claims of the theory of “deaths of despair” proposed by Case and Deaton at the right level (causes of incidence). I began by conducting a scoping review of the current literature providing empirical support to the different elements of this theory: 1) socioeconomic causes as causes of “despair”, “diseases of despair”, “deaths of despair”, and all-cause mortality, and 2) “despair” as the cause of “diseases of despair”, “deaths of despair”, and all-cause mortality. I found 43 studies that I organized and displayed in two graphs according to Rose’s causes of cases (individual-level causes of “deaths of despair”) and causes of incidence (between-population level causes of “deaths of despair” rates). In each graph, I showed the number of studies that provided evidence for the individual- or population-level elements of the theory of “deaths of despair”. Of these 43 studies, I found that only 13 studies explicitly stated that they tested this theory. Three studies provided different definitions of “despair”, which did not align with the previous vague definition provided by Case and Deaton about white individuals’ hopeless about their prospects for the future. Most studies provided individual-level evidence for “despair” increasing the likelihood of death and despair-related outcomes, which is analogous to a type III error—a mismatch between the research question and the level at which the studies’ design and analyses were conducted to answer that question. Further, no study addressed at the right level—between populations—the central claim of the theory of “deaths of despair”. This led me to review the literature around concepts similar to “despair” and propose a suitable indicator to test the claims of the theory of “deaths of despair”. I leveraged data from the National Health Interview Survey and the Centers for Disease Control mortality data to test whether increases in the prevalence of “despair” were associated with increases in all-cause mortality rates only among white individuals in midlife and whether this effect was bigger among low educated white individuals. To obtain a valid estimate of this association, I adapted econometric methods to develop a valid estimator of the association between increasing “despair” prevalence and increased all-cause mortality rates. After adjusting for potential confounders at the between-population level, I found that the trends in the prevalence of “despair” were negligible across all race and ethnic groups and that an increasing trend could not be identified. Further, I found no evidence that increasing prevalences of “despair” were associated with increased all-cause mortality rates among NH white individuals in midlife, or that this association was more pronounced for those with low education. Lastly, I conducted a similar analysis looking at the association between increased prevalences of “despair” and increased rates of “deaths of despair”. I replicated Case and Deaton’s observed increased rates of “deaths of despair” among white individuals in midlife. However, I found no evidence that increased prevalences of “despair” were associated with increased “deaths of despair” rates among white individuals in midlife or that this association was higher for those with low education. Together, these findings suggest that the claims about the causes of increased mortality rates among white Americans in midlife are at best, questionable, and at worst, false. My aim with this work is to challenge and provide a critical examination of the theory of "deaths of despair", which has fueled the narrative of a suffering white generation and justified recent problematic events as white individuals lashing out for being forgotten to despair and die. While Case and Deaton’s observed rise in mortality rates among whites is a reproducible fact, their narrative ignores other evidence of white racial resentment as the cause of rise in mortality among white individuals. With this work, I intend to help stopping the perpetuation of narratives that favor structural whiteness by promoting an unsubstantiated narrative of psychosocial harm experienced by white Americans. Ultimately, I hope this work helps shift the focus in public health away from Case and Deaton's findings, which may overshadow and detract from the stark reality that mortality rates for Black individuals significantly exceed those for white individuals.
Geographic Areas
- United States
- Epidemiology
- Depression, Mental--Epidemiology
- White people
- Centers for Disease Control and Prevention (U.S.)
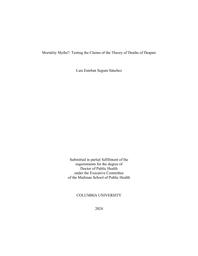
More About This Work
- DOI Copy DOI to clipboard
Agriculture and fisheries
OECD work on agriculture, food and fisheries helps governments assess the performance of their sectors, anticipate market trends, and evaluate and design policies to address the challenges they face in their transition towards sustainable and resilient food systems. The OECD facilitates dialogue through expert networks, funds international research cooperation efforts, and maintains international standards facilitating trade in seeds, produce and tractors.
Select a language
Policy issues.
- Agricultural policy monitoring Well-designed agricultural policies can help farmers meet increasing global demand for safe and nutritious food in a sustainable way. However, some current policies can have negative consequences for food security, markets, the environment, at both the domestic and global levels. The OECD’s regular monitoring of agricultural policies across 54 countries representing three-quarters of global agricultural value-added provides a comprehensive understanding of their nature, implementation and impact, with a view to helping guide governments towards more effective and efficient policy making. Learn more
- Agricultural productivity and innovation Achieving resilient, sustainable and productive agriculture and food systems will require innovation. Innovation in agriculture means learning to do things differently, to do different things, and to do more and better with less. It is an opportunity for food systems to deliver on challenging new demands, while ensuring the sustainable use of scarce natural resources. The OECD is helping support countries in developing better policies for productive, sustainable and resilient agriculture through work to benchmark the performance of agriculture and food systems, assess countries' policies and provide tailored policy advice. A focus of this work is how governments and the private sector can work together to strengthen agricultural innovation systems and foster innovative practices that increase productivity and sustainability. Learn more
- Agricultural trade and markets Agricultural trade plays a crucial role in providing livelihoods for farmers and people employed along the food supply chain and contributes to reducing global food insecurity. A growing share of agro-food trade involves global value chains (GVCs), where the different stages of agricultural and food production processes are spread over several countries. Learn more
- Agriculture and sustainability The agriculture sector faces the triple challenge of providing sufficient and nutritious food for an increasing global population, while at the same time preserving the environment and natural resources for future generations and maintaining sustainable livelihoods in rural areas. Policies have a key role to play in tackling these challenges, while addressing mounting pressures from climate change and other risk factors. Learn more
- Fisheries and aquaculture Fisheries and aquaculture provide food for billions of people and play an important role in the local economy and cultural life of coastal communities around the world. Fish products are among the most traded foods and their exports are essential for food security. But fish stocks and ecosystems are under stress from climate change, illegal fishing, excessive fishing pressure and pollution. Learn more
- Food systems Food systems worldwide face a “triple challenge”: to provide food security and nutrition for a growing population, to contribute to the livelihoods of millions of people working along the food supply chain, and to do so in an environmentally sustainable way. Moreover, food systems need to become more resilient across those dimensions. Learn more
- OECD standards for agriculture The OECD Codes and Schemes aim to facilitate and streamline international trade by simplifying procedures, enhancing transparency, reducing non-tariff barriers to trade, promoting harmonisation of standards, and enhancing environmental protection. They help to strengthen market confidence by assuring quality control, providing for inspections, and improving product traceability. Learn more
Programmes of work
- Co-operative Research Programme: Sustainable Agriculture and Food Systems The OECD's Co-operative Research Programme: Sustainable Agricultural and Food Systems (CRP) exists to strengthen scientific knowledge and provide relevant scientific information and advice that will inform future policy decisions related to the sustainability of agriculture, food, fisheries and forests. It does this through facilitating international co-operation among research scientists and institutions, by sponsoring international events (conferences, workshops) and individual research fellowships, placing a policy emphasis on all the activities it funds. It focuses on global issues such as food security, climate change, and the inter-connectedness of economies through trade and scientific co-operation. Learn more
Related publications

Subscribe to our newsletter
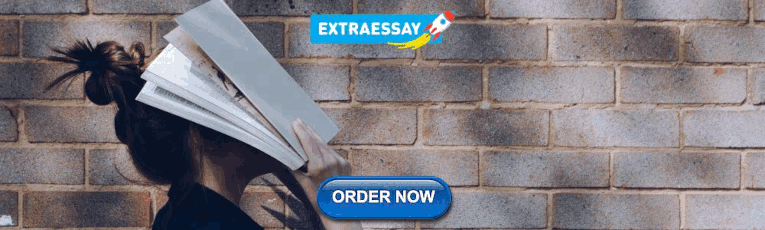
COMMENTS
Steps for conducting empirical research. Since empirical research is based on observation and capturing experiences, it is important to plan the steps to conduct the experiment and how to analyse it. This will enable the researcher to resolve problems or obstacles which can occur during the experiment. Step #1: Define the purpose of the research
Theoretical research involves the development of ideas and models, while empirical research involves testing and validating these ideas. Both approaches are essential to research and can be combined to provide a more complete understanding of the world. References. Dictionary.com. " Empirical vs Theoretical ".
A scientist gathering data for her research. Empirical research is research using empirical evidence.It is also a way of gaining knowledge by means of direct and indirect observation or experience. Empiricism values some research more than other kinds. Empirical evidence (the record of one's direct observations or experiences) can be analyzed quantitatively or qualitatively.
Empirical research methodologies can be described as quantitative, qualitative, or a mix of both (usually called mixed-methods). Ruane (2016) (UofM login required) gets at the basic differences in approach between quantitative and qualitative research: Quantitative research -- an approach to documenting reality that relies heavily on numbers both for the measurement of variables and for data ...
Empirical research is the cornerstone of scientific inquiry, providing a systematic and structured approach to investigating the world around us. It is the process of gathering and analyzing empirical or observable data to test hypotheses, answer research questions, or gain insights into various phenomena.
Empirical research is defined as any study whose conclusions are exclusively derived from concrete, verifiable evidence. The term empirical basically means that it is guided by scientific experimentation and/or evidence. Likewise, a study is empirical when it uses real-world evidence in investigating its assertions.
In empirical research, knowledge is developed from factual experience as opposed to theoretical assumption and usually involved the use of data sources like datasets or fieldwork, but can also be based on observations within a laboratory setting. Testing hypothesis or answering definite questions is a primary feature of empirical research.
Theoretical frameworks are one of the most contemplated elements in our work in educational research. In this section, we share three important considerations for new scholars selecting a theoretical framework. ... It takes time to understand the relevant research, identify a theoretical framework that provides important insights into the study ...
Jenkins-Smith et al. University of Oklahoma via University of Oklahoma Libraries. This book is concerned with the connection between theoretical claims and empirical data. It is about using statistical modeling; in particular, the tool of regression analysis, which is used to develop and refine theories.
The first part of the article provides background by presenting a brief overview of knowledge production, reflexivity, and the use of theory in qualitative research. ... Theoretical frameworks are defined, according to Anfara and Mertz, as "any empirical or quasi-empirical theory of social/ and/or psychological processes, at a variety of ...
Share this content. Empirical research is research that is based on observation and measurement of phenomena, as directly experienced by the researcher. The data thus gathered may be compared against a theory or hypothesis, but the results are still based on real life experience. The data gathered is all primary data, although secondary data ...
Definition of the population, behavior, or phenomena being studied. Description of the process used to study this population or phenomena, including selection criteria, controls, and testing instruments (such as surveys) Another hint: some scholarly journals use a specific layout, called the "IMRaD" format, to communicate empirical research ...
Another hint: some scholarly journals use a specific layout, called the "IMRaD" format, to communicate empirical research findings. Such articles typically have 4 components: Introduction : sometimes called "literature review" -- what is currently known about the topic -- usually includes a theoretical framework and/or discussion of previous ...
Abstract. This chapter constitutes a reflexive account, necessary to clarify the theoretical assumptions, the researcher's characteristics, and the methods employed. First of all, I will begin by introducing the seminal work of Erving Goffman on asylums, as well as more recent ethnographic contributions on acute mental healthcare. Then, I ...
The most direct route is to search PsycInfo, linked above. This will take you to the Advanced Search, where you can type in your key words at the top. Then scroll down through all the limiting options to the Methodology menu. Select Empirical Study. In other databases without the Methodology limiter, such as Education Source, try keywords like ...
The theoretical framework may be rooted in a specific theory, in which case, your work is expected to test the validity of that existing theory in relation to specific events, issues, or phenomena. Many social science research papers fit into this rubric. ... When writing and revising this part of your research paper, keep in mind the following ...
Abstract: A report of an empirical study includes an abstract that provides a very brief summary of the research. Introduction: The introduction sets the research in a context, which provides a review of related research and develops the hypotheses for the research. Method: The method section is a description of how the research was conducted, including who the participants were, the design of ...
routine use of research rather than aiming to create an instance of research use. As theory matured, our attention shifted to understanding ways to support agencies and other decision-making bodies in building their capacity to routinely draw upon research in their daily, weekly, and yearly work and as part of the flow of decision-making.
Exercise 3.2. Researchers have used a number of different metaphors to describe theoretical frameworks. Maxwell (2005) referred to a theoretical framework as a "coat closet" that provides "places to 'hang' data, showing their relationship to other data," although he cautioned that "a theory that neatly organizes some data will leave other data disheveled and lying on the floor ...
and the problems in undertaking field research.The epistemology of research relates to issues such as choosing a theme of research, the evolution of one's conceptual-theoretical framework, the interaction between empirical research, concept formation and theory construc tion, all of which have a dominating influence on the nature of
For example, social work scholars may produce articles that are clearly identified as describing a new intervention or program without evaluative evidence, critiquing a policy or practice, or offering a tentative, untested theory about a phenomenon. These resources are useful in educating ourselves about possible issues or concerns.
typical thesis timeline 2. Get familiar with the topic. Read 30 journal abstracts from the past 5 years. Identify key authors in field. Write down key questions researched in the field (to find where your. contribution might fit in) Narrow down your topic. Choose key papers of the relevant literature to read in more detail.
3. Core - this is where you present your findings. 4. Synthesis - this is where you relate the core to the background. The empirical chapter (s) is/are where you present the facts of your study. They occupy the core of the thesis. The discussion chapter though is where you interpret and discuss your findings in relation to the thesis and ...
Develop a rich toolkit of research methods so you can use the strengths of one method to overcome the weaknesses of another, and so you can see through one method what another cannot show. Theorize but allow for surprises. We rarely look for empirical data without a theoretical framing that informs what we decide to look for.
Research on social and emotional skills is multi-disciplinary, combining a unique mix of academic and practice-oriented research approaches. It features both theoretical and empirical research streams, with lively discussions on the conceptual foundation and structure of these skills, their origin, development, malleability, and relevance.
A groundbreaking narrative, which would come to be known as the theory of "deaths of despair", emerged in 2015 from a study by Case and Deaton analyzing mortality rates in the United States between 1999 and 2013. They found an increasing trend in all-cause mortality rates due to drug poisonings, alcohol-related liver disease, and suicides, which they called "deaths of despair", among ...
OECD work on agriculture, food and fisheries helps governments assess the performance of their sectors, anticipate market trends, and evaluate and design policies to address the challenges they face in their transition towards sustainable and resilient food systems. The OECD facilitates dialogue through expert networks, funds international research cooperation efforts, and maintains ...