Operations Management
Browse operations management learning materials including case studies, simulations, and online courses. Introduce core concepts and real-world challenges to create memorable learning experiences for your students.
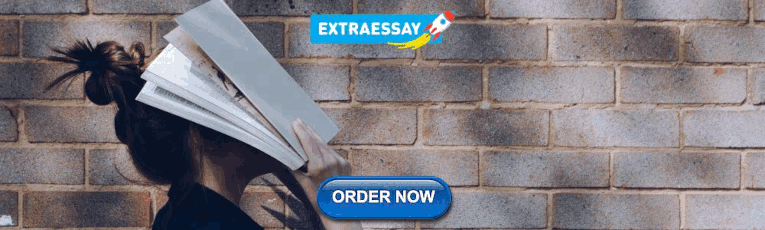
Browse by Topic
- Capacity Planning
- Demand Planning
- Inventory Management
- Process Analysis
- Process Improvement
- Production Planning
- Project Management
- Quality Management
New! Quick Cases in Operations Management
Quickly immerse students in focused and engaging business dilemmas. No student prep time required.
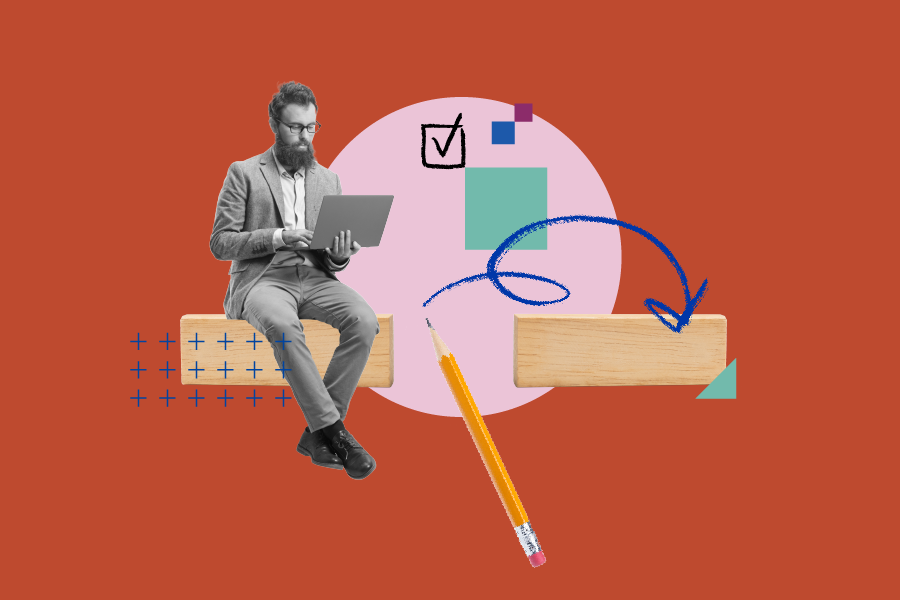
Fundamentals of Case Teaching
Our new, self-paced, online course guides you through the fundamentals for leading successful case discussions at any course level.
New in Operations Management
Explore the latest operations management learning materials
1325 word count
1563 word count
1098 word count
2320 word count
1841 word count
3547 word count
1648 word count
1840 word count
Looking for something specific?
Explore materials that align with your operations management learning objectives
Operations Management Simulations
Give your students hands-on experience making decisions.
Operations Management Cases with Female Protagonists
Explore a collection of operations management cases featuring female protagonists curated by the HBS Gender Initiative.
Operations Management Cases with Protagonists of Color
Discover operations management cases featuring protagonists of color that have been recommended by Harvard Business School faculty.
Foundational Operations Management Readings
Discover readings that cover the fundamental concepts and frameworks that business students must learn about operations management.
Bestsellers in Operations Management
Explore what other educators are using in their operations management courses
Start building your courses today
Register for a free Educator Account and get exclusive access to our entire catalog of learning materials, teaching resources, and online course planning tools.
We use cookies to understand how you use our site and to improve your experience, including personalizing content. Learn More . By continuing to use our site, you accept our use of cookies and revised Privacy Policy .
Smart. Open. Grounded. Inventive. Read our Ideas Made to Matter.
Which program is right for you?
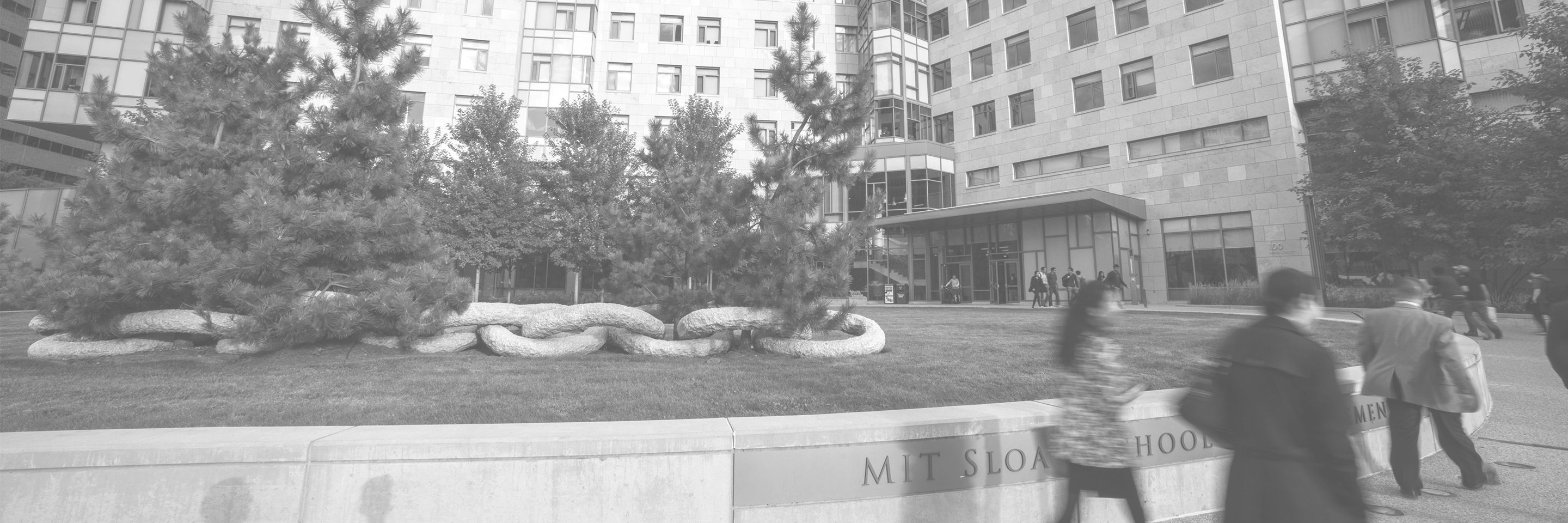
Through intellectual rigor and experiential learning, this full-time, two-year MBA program develops leaders who make a difference in the world.
A rigorous, hands-on program that prepares adaptive problem solvers for premier finance careers.
A 12-month program focused on applying the tools of modern data science, optimization and machine learning to solve real-world business problems.
Earn your MBA and SM in engineering with this transformative two-year program.
Combine an international MBA with a deep dive into management science. A special opportunity for partner and affiliate schools only.
A doctoral program that produces outstanding scholars who are leading in their fields of research.
Bring a business perspective to your technical and quantitative expertise with a bachelor’s degree in management, business analytics, or finance.
A joint program for mid-career professionals that integrates engineering and systems thinking. Earn your master’s degree in engineering and management.
An interdisciplinary program that combines engineering, management, and design, leading to a master’s degree in engineering and management.
Executive Programs
A full-time MBA program for mid-career leaders eager to dedicate one year of discovery for a lifetime of impact.
This 20-month MBA program equips experienced executives to enhance their impact on their organizations and the world.
Non-degree programs for senior executives and high-potential managers.
A non-degree, customizable program for mid-career professionals.
Teaching Resources Library
Operations Management Case Studies
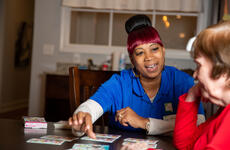
Browse Course Material
Course info, instructors.
- Prof. Charles H. Fine
- Prof. Tauhid Zaman
Departments
- Sloan School of Management
As Taught In
- Mathematics
- Social Science
Introduction to Operations Management
Cases and readings.
The required readings for this course include:
- Cases listed in the Cases/Readings column below
- Goldratt, Eliyah M., and Jeff Cox. The Goal: A Process of Ongoing Improvement . 2nd revised ed. North River Press, 1992. ISBN: 9780884270614.
- [MSD] = Cachon, Gerard, and Christian Terwiesch. Matching Supply with Demand: An Introduction to Operations Management . 3rd ed. McGraw-Hill, 2012. ISBN: 9780073525204.

You are leaving MIT OpenCourseWare
To read this content please select one of the options below:
Please note you do not have access to teaching notes, case research in operations management.
International Journal of Operations & Production Management
ISSN : 0144-3577
Article publication date: 1 February 2002
This paper reviews the use of case study research in operations management for theory development and testing. It draws on the literature on case research in a number of disciplines and uses examples drawn from operations management research. It provides guidelines and a roadmap for operations management researchers wishing to design, develop and conduct case‐based research.
- Operations management
- Methodology
- Case studies
Voss, C. , Tsikriktsis, N. and Frohlich, M. (2002), "Case research in operations management", International Journal of Operations & Production Management , Vol. 22 No. 2, pp. 195-219. https://doi.org/10.1108/01443570210414329
Copyright © 2002, MCB UP Limited
Related articles
We’re listening — tell us what you think, something didn’t work….
Report bugs here
All feedback is valuable
Please share your general feedback
Join us on our journey
Platform update page.
Visit emeraldpublishing.com/platformupdate to discover the latest news and updates
Questions & More Information
Answers to the most commonly asked questions here

- SUGGESTED TOPICS
- The Magazine
- Newsletters
- Managing Yourself
- Managing Teams
- Work-life Balance
- The Big Idea
- Data & Visuals
- Reading Lists
- Case Selections
- HBR Learning
- Topic Feeds
- Account Settings
- Email Preferences
Operations strategy
- Business management
- Operations and supply chain management
- Supply chain management

The New Human-Machine Relationship
- Ben Armstrong
- Nita A. Farahany
- Mike Seymour
- Dan Lovallo
- Alan R. Dennis
- Lingyao Ivy Yuan
- February 28, 2023

Raising Wages Is the Right Thing to Do, and Doesn’t Have to Be Bad for Your Bottom Line
- April 18, 2019
Getting Control of Just-in-Time
- Uday Karmarkar
- From the September–October 1989 Issue

Lessons from the U.S.'s Rocky Vaccine Rollout
- Robert S. Huckman
- Bradley R. Staats
- Bradley Staats
- January 28, 2021

Deep Change: How Operational Innovation Can Transform Your Company (HBR OnPoint Enhanced Edition)
- Michael Hammer
- April 01, 2004

Companies Are Working with Consumers to Reduce Waste
- Mark Esposito
- Terence Tse
- Khaled Soufani
- June 07, 2016

Hospitals Can’t Improve Without Better Management Systems
- John S. Toussaint
- October 21, 2015
How to Survive Climate Change and Still Run a Thriving Business: Checklists for Smart Leaders
- Eric Lowitt
- From the April 2014 Issue
Coupling Strategy to Operating Plans
- John M. Hobbs
- Donald F. Heany
- From the May 1977 Issue
Firms Need a Blueprint for Building Their IT Systems
- Donald A. Marchand
- Joe Peppard
- June 18, 2015
Integrate Data into Products, or Get Left Behind
- Thomas C. Redman
- June 28, 2012
The Department of Mobility
- Rex Runzheimer
- From the November 2005 Issue
Pain in the (Supply) Chain (HBR Case Study and Commentary)
- John Butman
- From the May 2002 Issue
Breaking the Trade-Off Between Efficiency and Service
- Frances X. Frei
- From the November 2006 Issue

How Loyalty Programs Are Saving Airlines
- So Yeon Chun
- Evert de Boer
- April 02, 2021

How Kenvue De-Risked Its Supply Chain
- Michael Altman
- Atalay Atasu
- Evren Özkaya
- October 18, 2023
What Every Leader Should Know About Real Estate
- Mahlon Apgar, IV
- From the November 2009 Issue
The World's Housing Crisis Doesn't Need a Revolutionary Solution
- Lola Woetzel
- Jan Mischke
- Sangeeth Ram
- December 25, 2014
Is Your Supply Chain Ready for the Congestion Crisis?
- George Stalk, Jr.
- Petros Paranikas
- June 22, 2015
Customer Intimacy and Other Value Disciplines
- Michael Treacy
- Fred Wiersema
- From the January–February 1993 Issue

FreeMarkets OnLine
- V. Kasturi Rangan
- February 27, 1998
Apple Pay and Mobile Payments in Australia (A)
- Susan Athey
- September 13, 2018
SANY: Going Global
- Stefan Lippert
- Nancy Hua Dai
- November 11, 2012
Apple Pay and Mobile Payments in Australia (B)
Ilinko: enterprise systems implementation all over again.
- January 11, 2013
Nike in China (Abridged)
- James E. Austin
- April 11, 1990
A3 Thinking
- Elliott N. Weiss
- Austin English
- June 03, 2020
The Writing Process in Systems Thinking
- Robert D. Landel
- Jennifer Corle
- March 04, 2004
Mibanco: Meeting the Mainstreaming of Microfinance
- Michael Chu
- Gustavo A. Herrero
- Jean Steege Hazell
- August 23, 2011
Booking.com
- Stefan Thomke
- Daniela Beyersdorfer
- October 15, 2018
ExtendSim (R) Simulation Exercises in Process Analysis (B2)
- Roy D. Shapiro
- September 22, 1994
Clean Core Thorium Energy and the Role of Nuclear Power in the Low-carbon Transition
- Gernot Wagner
- July 10, 2023
Rich-Con Steel
- Andrew McAfee
- January 27, 1999
Boeing 787: Manufacturing a Dream
- Rory McDonald
- Suresh Kotha
- February 12, 2015
Messer Griesheim (A)
- Josh Lerner
- Ann-Kristin Achleitner
- Eva Nathusius
- Kerry Herman
- February 18, 2009
From Correlation to Causation
- Karim R. Lakhani
- August 31, 2015
Integron, Inc.: The Integrated Components Division (ICD)
- David M. Upton
- Michelle Jarrard
- Laurie Thomas
- June 30, 1995
Southeastern Mills: The Eighth Element?
- Rebecca O. Goldberg
- Andrew Moon
- December 23, 2009
Industrial Grinders N.V.
- M. Edgar Barrett
- Rohan S. Weerasinghe
- March 01, 1975
Advanced Glass Technologies, Inc.: The ZX Project
- November 22, 2011

Whole Foods under Amazon, Teaching Note
- Dennis Campbell
- Tatiana Sandino
- Kyle Thomas
- February 22, 2019
CFNA Credit Corporation: Call Center Outsourcing, Spreadsheet
- Timothy M. Laseter
- March 28, 2011
Popular Topics
Partner center.

- About / Contact
- Privacy Policy
- Alphabetical List of Companies
- Business Analysis Topics
Walmart’s Operations Management: 10 Strategic Decisions & Productivity
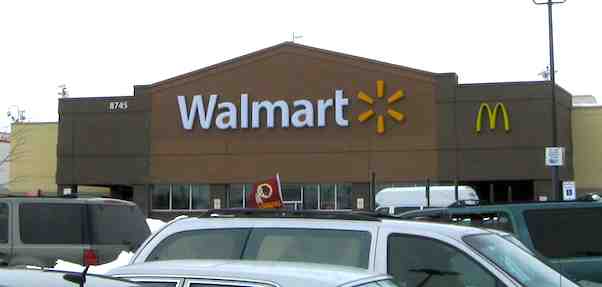
Walmart Inc.’s operations management involves a variety of approaches that are focused on managing the supply chain and inventory, as well as sales performance. The company’s success is significantly based on effective performance in retail operations management. Specifically, Walmart’s management covers all the 10 decision areas of operations management. These strategic decision areas pertain to the issues managers deal with on a daily basis as they optimize the e-commerce company’s operations. Walmart’s application of the 10 decisions of operations management reflects managers’ prioritization of business objectives. In turn, this prioritization shows the strategic significance of the different decision areas of operations management in the retail company’s business. This approach to operations aligns with Walmart’s corporate mission statement and corporate vision statement . The retail enterprise is a business case of how to achieve high efficiency in operations to ensure long-term growth and success in the global market.
The 10 decisions of operations management are effectively addressed in Walmart’s business through a combination of approaches that emphasize supply chain management, inventory management, and sales and marketing. This approach leads to strategies that strengthen the business against competitors, like Amazon and its subsidiary, Whole Foods , as well as Home Depot , eBay, Costco , Best Buy, Macy’s, Kroger, Alibaba, IKEA, Target, and Lowe’s.
The 10 Strategic Decision Areas of Operations Management at Walmart
1. Design of Goods and Services . This decision area of operations management involves the strategic characterization of the retail company’s products. In this case, the decision area covers Walmart’s goods and services. As a retailer, the company offers retail services. However, Walmart also has its own brands of goods, such as Great Value and Sam’s Choice. The company’s operations management addresses the design of retail service by emphasizing the variables of efficiency and cost-effectiveness. Walmart’s generic strategy for competitive advantage, and intensive growth strategies emphasize low costs and low selling prices. To fulfill these strategies, the firm focuses on maximum efficiency of its retail service operations. To address the design of goods in this decision area of operations management, Walmart emphasizes minimal production costs, especially for the Great Value brand. The firm’s consumer goods are designed in a way that they are easy to mass-produce. The strategic approach in this operations management area affects Walmart’s marketing mix or 4Ps and the corporation’s strategic planning for product development and retail service expansion.
2. Quality Management . Walmart approaches this decision area of operations management through three tiers of quality standards. The lowest tier specifies the minimum quality expectations of the majority of buyers. Walmart keeps this tier for most of its brands, such as Great Value. The middle tier specifies market average quality for low-cost retailers. This tier is used for some products, as well as for the job performance targets of Walmart employees, especially sales personnel. The highest tier specifies quality levels that exceed market averages in the retail industry. This tier is applied to only a minority of Walmart’s outputs, such as goods under the Sam’s Choice brand. This three-tier approach satisfies quality management objectives in the strategic decision areas of operations management throughout the retail business organization. Appropriate quality measures also contribute to the strengths identified in the SWOT analysis of Walmart Inc .
3. Process and Capacity Design . In this strategic decision area, Walmart’s operations management utilizes behavioral analysis, forecasting, and continuous monitoring. Behavioral analysis of customers and employees, such as in the brick-and-mortar stores and e-commerce operations, serves as basis for the company’s process and capacity design for optimizing space, personnel, and equipment. Forecasting is the basis for Walmart’s ever-changing capacity design for human resources. The company’s HR process and capacity design evolves as the retail business grows. Also, to satisfy concerns in this decision area of operations management, Walmart uses continuous monitoring of store capacities to inform corporate managers in keeping or changing current capacity designs.
4. Location Strategy . This decision area of operations management emphasizes efficiency of movement of materials, human resources, and business information throughout the retail organization. In this regard, Walmart’s location strategy includes stores located in or near urban centers and consumer population clusters. The company aims to maximize market reach and accessibility for consumers. Materials and goods are made available to Walmart’s employees and target customers through strategic warehouse locations. On the other hand, to address the business information aspect of this decision area of operations management, Walmart uses Internet technology and related computing systems and networks. The company has a comprehensive set of online information systems for real-time reports and monitoring that support managing individual retail stores as well as regional market operations.
5. Layout Design and Strategy . Walmart addresses this decision area of operations management by assessing shoppers’ and employees’ behaviors for the layout design of its brick-and-mortar stores, e-commerce websites, and warehouses or storage facilities. The layout design of the stores is based on consumer behavioral analysis and corporate standards. For example, Walmart’s placement of some goods in certain areas of its stores, such as near the entrance/exit, maximizes purchase likelihood. On the other hand, the layout design and strategy for the company’s warehouses are based on the need to rapidly move goods across the supply chain to the stores. Walmart’s warehouses maximize utilization and efficiency of space for the company’s trucks, suppliers’ trucks, and goods. With efficiency, cost-effectiveness, and cost-minimization, the retail company satisfies the needs in this strategic decision area of operations management.
6. Human Resources and Job Design . Walmart’s human resource management strategies involve continuous recruitment. The retail business suffers from relatively high turnover partly because of low wages, which relate to the cost-leadership generic strategy. Nonetheless, continuous recruitment addresses this strategic decision area of operations management, while maintaining Walmart’s organizational structure and corporate culture . Also, the company maintains standardized job processes, especially for positions in its stores. Walmart’s training programs support the need for standardization for the service quality standards of the business. Thus, the company satisfies concerns in this decision area of operations management despite high turnover.
7. Supply Chain Management . Walmart’s bargaining power over suppliers successfully addresses this decision area of operations management. The retailer’s supply chain is comprehensively integrated with advanced information technology, which enhances such bargaining power. For example, supply chain management information systems are directly linked to Walmart’s ability to minimize costs of operations. These systems enable managers and vendors to collaborate in deciding when to move certain amounts of merchandise across the supply chain. This condition utilizes business competitiveness with regard to competitive advantage, as shown in the Porter’s Five Forces analysis of Walmart Inc . As one of the biggest retailers in the world, the company wields its strong bargaining power to impose its demands on suppliers, as a way to address supply chain management issues in this strategic decision area of operations management. Nonetheless, considering Walmart’s stakeholders and corporate social responsibility strategy , the company balances business needs and the needs of suppliers, who are a major stakeholder group.
8. Inventory Management . In this decision area of operations management, Walmart focuses on the vendor-managed inventory model and just-in-time cross-docking. In the vendor-managed inventory model, suppliers access the company’s information systems to decide when to deliver goods based on real-time data on inventory levels. In this way, Walmart minimizes the problem of stockouts. On the other hand, in just-in-time cross-docking, the retail company minimizes the size of its inventory, thereby supporting cost-minimization efforts. These approaches help maximize the operational efficiency and performance of the retail business in this strategic decision area of operations management (See more: Walmart: Inventory Management ).
9. Scheduling . Walmart uses conventional shifts and flexible scheduling. In this decision area of operations management, the emphasis is on optimizing internal business process schedules to achieve higher efficiencies in the retail enterprise. Through optimized schedules, Walmart minimizes losses linked to overcapacity and related issues. Scheduling in the retailer’s warehouses is flexible and based on current trends. For example, based on Walmart’s approaches to inventory management and supply chain management, suppliers readily respond to changes in inventory levels. As a result, most of the company’s warehouse schedules are not fixed. On the other hand, Walmart store processes and human resources in sales and marketing use fixed conventional shifts for scheduling. Such fixed scheduling optimizes the retailer’s expenditure on human resources. However, to fully address scheduling as a strategic decision area of operations management, Walmart occasionally changes store and personnel schedules to address anticipated changes in demand, such as during Black Friday. This flexibility supports optimal retail revenues, especially during special shopping occasions.
10. Maintenance . With regard to maintenance needs, Walmart addresses this decision area of operations management through training programs to maintain human resources, dedicated personnel to maintain facilities, and dedicated personnel to maintain equipment. The retail company’s human resource management involves training programs to ensure that employees are effective and efficient. On the other hand, dedicated personnel for facility maintenance keep all of Walmart’s buildings in shape and up to corporate and regulatory standards. In relation, the company has dedicated personnel as well as third-party service providers for fixing and repairing equipment like cash registers and computers. Walmart also has personnel for maintaining its e-commerce websites and social media accounts. This combination of maintenance approaches contributes to the retail company’s effectiveness in satisfying the concerns in this strategic decision area of operations management. Effective and efficient maintenance supports business resilience against threats in the industry environment, such as the ones evaluated in the PESTEL/PESTLE Analysis of Walmart Inc .
Determining Productivity at Walmart Inc.
One of the goals of Walmart’s operations management is to maximize productivity to support the minimization of costs under the cost leadership generic strategy. There are various quantitative and qualitative criteria or measures of productivity that pertain to human resources and related internal business processes in the retail organization. Some of the most notable of these productivity measures/criteria at Walmart are:
- Revenues per sales unit
- Stockout rate
- Duration of order filling
The revenues per sales unit refers to the sales revenues per store, average sales revenues per store, and sales revenues per sales team. Walmart’s operations managers are interested in maximizing revenues per sales unit. On the other hand, the stockout rate is the frequency of stockout, which is the condition where inventories for certain products are empty or inadequate despite positive demand. Walmart’s operations management objective is to minimize stockout rates. Also, the duration of order filling is the amount of time consumed to fill inventory requests at the company’s stores. The operations management objective in this regard is to minimize the duration of order filling, as a way to enhance Walmart’s business performance.
- Reid, R. D., & Sanders, N. R. (2023). Operations Management: An Integrated Approach . John Wiley & Sons.
- Szwarc, E., Bocewicz, G., Golińska-Dawson, P., & Banaszak, Z. (2023). Proactive operations management: Staff allocation with competence maintenance constraints. Sustainability, 15 (3), 1949.
- Walmart Inc. – Form 10-K .
- Walmart Inc. – History .
- Walmart Inc. – Location Facts .
- Walmart’s E-commerce Website .
- Copyright by Panmore Institute - All rights reserved.
- This article may not be reproduced, distributed, or mirrored without written permission from Panmore Institute and its author/s.
- Educators, Researchers, and Students: You are permitted to quote or paraphrase parts of this article (not the entire article) for educational or research purposes, as long as the article is properly cited and referenced together with its URL/link.
- Browse All Articles
- Newsletter Sign-Up
ServiceOperations →
No results found in working knowledge.
- Were any results found in one of the other content buckets on the left?
- Try removing some search filters.
- Use different search filters.

Operations Management
A primary challenge for governments and organizations is to manage their resources as efficiently as possible. The teaching cases in this section challenge students to become decisive managers through a host of topics including budgeting and finance, infrastructure, regulatory policy, and transportation.
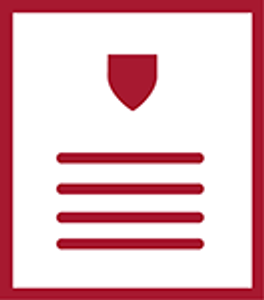
Mayoral Transitions: How Three Mayors Stepped into the Role, in Their Own Words
Publication Date: February 29, 2024
New mayors face distinct challenges as they assume office. In these vignettes depicting three types of mayoral transitions, explore how new leaders can make the most of their first one hundred days by asserting their authority and...
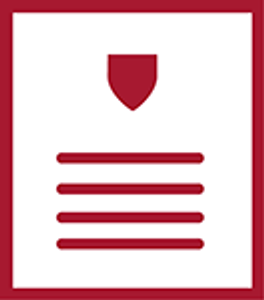
Shoring Up Child Protection in Massachusetts: Commissioner Spears & the Push to Go Fast
Publication Date: July 13, 2023
In January 2015, when incoming Massachusetts Governor Charlie Baker chose Linda Spears as his new Commissioner of the Department of Children and Families, he was looking for a reformer. Following the grizzly death of a child under DCF...
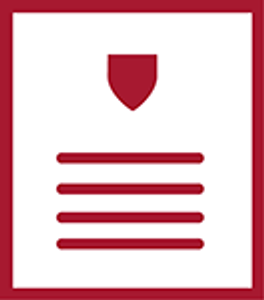
OneBlood and COVID-19: Building an Agile Supply Chain Epilogue
Publication Date: October 20, 2021
This epilogue accompanies HKS Case 2233.0. The blood supply chain is under pressure from COVID-19. How should the 3rd largest blood bank in the US, OneBlood, respond? Is adopting an agile supply chain philosophy an effective...
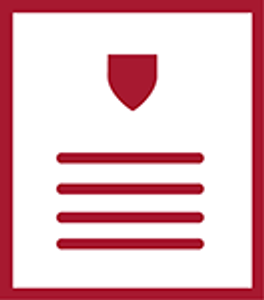
OneBlood and COVID-19: Building an Agile Supply Chain
The blood supply chain is under pressure from COVID-19. How should the 3rd largest blood bank in the US, OneBlood, respond? Is adopting an agile supply chain philosophy an effective approach? The case provides an overview of the...
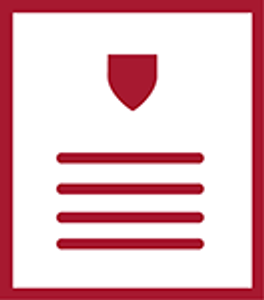
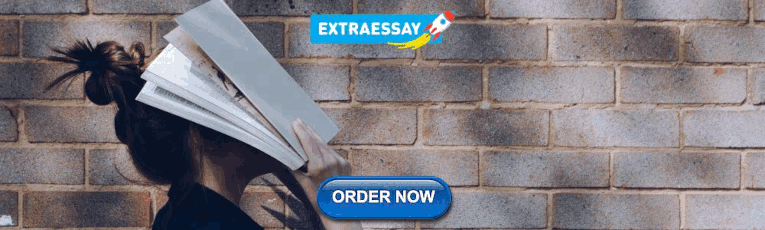
“A Difficult Lady”: Shutting Down Pollution in Kampala, Uganda Practitioner Guide
Publication Date: October 15, 2021
This practitioner guide accompanies HKS Case 2231.0. In 2011, sanitation and environmental management expert Judith Tumusiime joined the Kampala Capital City Authority (KCCA), where she and KCCA Executive Director Jennifer Musisi quickly became...
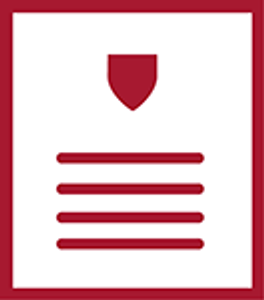
“A Difficult Lady”: Shutting Down Pollution in Kampala, Uganda
In 2011, sanitation and environmental management expert Judith Tumusiime joined the Kampala Capital City Authority (KCCA), where she and KCCA Executive Director Jennifer Musisi quickly became a dynamic team, working together to execute a mandate...
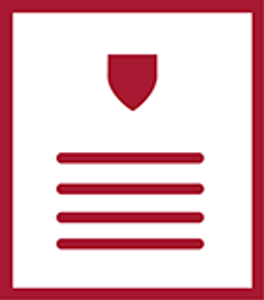
“Pressing the Right Buttons”: Jennifer Musisi for New City Leadership Epilogue
Publication Date: September 9, 2020
This epilogue accompanies HKS Case 2186.0. Jennifer Musisi, a career civil servant most recently with the Uganda Revenue Authority, was appointed by President Museveni as executive director (equivalent to city manager) of a new governing body...
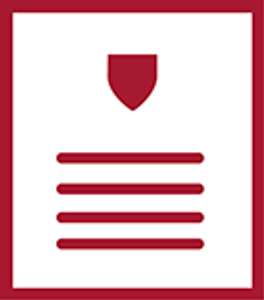
“Pressing the Right Buttons”: Jennifer Musisi for New City Leadership Practitioner Guide
This practitioner guide accompanies HKS Case 2186.0. Jennifer Musisi, a career civil servant most recently with the Uganda Revenue Authority, was appointed by President Museveni as executive director (equivalent to city manager) of a new...
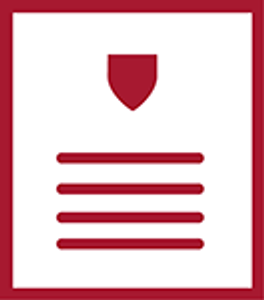
“Pressing the Right Buttons”: Jennifer Musisi for New City Leadership
Jennifer Musisi, a career civil servant most recently with the Uganda Revenue Authority, was appointed by President Museveni as executive director (equivalent to city manager) of a new governing body for Uganda’s capital, the Kampala...
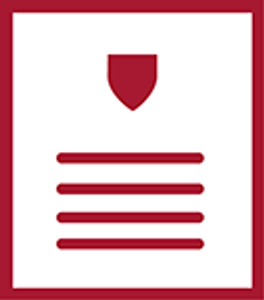
The “Garbage Lady” Cleans Up Kampala: Turning Quick Wins into Lasting Change Practitioner Guide
Publication Date: June 30, 2020
This practitioner guide accompanies HKS Case 2181.0. In 2011, at the newly formed Kampala Capital City Authority (KCCA), Judith Tumusiime, an impassioned technocrat who prided herself on operating outside of politics, was charged with...
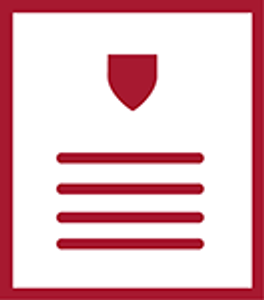
The “Garbage Lady” Cleans Up Kampala: Turning Quick Wins into Lasting Change (Epilogue)
This epilogue accompanies HKS Case 2181.0. In 2011, at the newly formed Kampala Capital City Authority (KCCA), Judith Tumusiime, an impassioned technocrat who prided herself on operating outside of politics, was charged with transforming a...
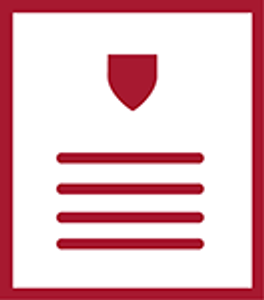
The “Garbage Lady” Cleans Up Kampala: Turning Quick Wins into Lasting Change
In 2011, at the newly formed Kampala Capital City Authority (KCCA), Judith Tumusiime, an impassioned technocrat who prided herself on operating outside of politics, was charged with transforming a “filthy city” to a clean, habitable,...

Top 40 Most Popular Case Studies of 2021
Two cases about Hertz claimed top spots in 2021's Top 40 Most Popular Case Studies
Two cases on the uses of debt and equity at Hertz claimed top spots in the CRDT’s (Case Research and Development Team) 2021 top 40 review of cases.
Hertz (A) took the top spot. The case details the financial structure of the rental car company through the end of 2019. Hertz (B), which ranked third in CRDT’s list, describes the company’s struggles during the early part of the COVID pandemic and its eventual need to enter Chapter 11 bankruptcy.
The success of the Hertz cases was unprecedented for the top 40 list. Usually, cases take a number of years to gain popularity, but the Hertz cases claimed top spots in their first year of release. Hertz (A) also became the first ‘cooked’ case to top the annual review, as all of the other winners had been web-based ‘raw’ cases.
Besides introducing students to the complicated financing required to maintain an enormous fleet of cars, the Hertz cases also expanded the diversity of case protagonists. Kathyrn Marinello was the CEO of Hertz during this period and the CFO, Jamere Jackson is black.
Sandwiched between the two Hertz cases, Coffee 2016, a perennial best seller, finished second. “Glory, Glory, Man United!” a case about an English football team’s IPO made a surprise move to number four. Cases on search fund boards, the future of malls, Norway’s Sovereign Wealth fund, Prodigy Finance, the Mayo Clinic, and Cadbury rounded out the top ten.
Other year-end data for 2021 showed:
- Online “raw” case usage remained steady as compared to 2020 with over 35K users from 170 countries and all 50 U.S. states interacting with 196 cases.
- Fifty four percent of raw case users came from outside the U.S..
- The Yale School of Management (SOM) case study directory pages received over 160K page views from 177 countries with approximately a third originating in India followed by the U.S. and the Philippines.
- Twenty-six of the cases in the list are raw cases.
- A third of the cases feature a woman protagonist.
- Orders for Yale SOM case studies increased by almost 50% compared to 2020.
- The top 40 cases were supervised by 19 different Yale SOM faculty members, several supervising multiple cases.
CRDT compiled the Top 40 list by combining data from its case store, Google Analytics, and other measures of interest and adoption.
All of this year’s Top 40 cases are available for purchase from the Yale Management Media store .
And the Top 40 cases studies of 2021 are:
1. Hertz Global Holdings (A): Uses of Debt and Equity
2. Coffee 2016
3. Hertz Global Holdings (B): Uses of Debt and Equity 2020
4. Glory, Glory Man United!
5. Search Fund Company Boards: How CEOs Can Build Boards to Help Them Thrive
6. The Future of Malls: Was Decline Inevitable?
7. Strategy for Norway's Pension Fund Global
8. Prodigy Finance
9. Design at Mayo
10. Cadbury
11. City Hospital Emergency Room
13. Volkswagen
14. Marina Bay Sands
15. Shake Shack IPO
16. Mastercard
17. Netflix
18. Ant Financial
19. AXA: Creating the New CR Metrics
20. IBM Corporate Service Corps
21. Business Leadership in South Africa's 1994 Reforms
22. Alternative Meat Industry
23. Children's Premier
24. Khalil Tawil and Umi (A)
25. Palm Oil 2016
26. Teach For All: Designing a Global Network
27. What's Next? Search Fund Entrepreneurs Reflect on Life After Exit
28. Searching for a Search Fund Structure: A Student Takes a Tour of Various Options
30. Project Sammaan
31. Commonfund ESG
32. Polaroid
33. Connecticut Green Bank 2018: After the Raid
34. FieldFresh Foods
35. The Alibaba Group
36. 360 State Street: Real Options
37. Herman Miller
38. AgBiome
39. Nathan Cummings Foundation
40. Toyota 2010

Case Related Links
Case studies collection.
Business Strategy Marketing Finance Human Resource Management IT and Systems Operations Economics Leadership and Entrepreneurship Project Management Business Ethics Corporate Governance Women Empowerment CSR and Sustainability Law Business Environment Enterprise Risk Management Insurance Innovation Miscellaneous Business Reports Multimedia Case Studies Cases in Other Languages Simplified Case Studies
Short Case Studies
Business Ethics Business Environment Business Strategy Consumer Behavior Human Resource Management Industrial Marketing International Marketing IT and Systems Marketing Communications Marketing Management Miscellaneous Operations Sales and Distribution Management Services Marketing More Short Case Studies >
JavaScript seems to be disabled in your browser. For the best experience on our site, be sure to turn on Javascript in your browser.
We use cookies to make your experience better. To comply with the new e-Privacy directive, we need to ask for your consent to set the cookies. Learn more .
- Compare Products
- Case Collection
- Operations Management
Items 1 - 10 of 14
- You're currently reading page 1
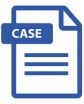
The case is centered around the timeline of the Telangana graduates’ MLC elections 2021, which were held against the backdrop of a known unknown: the COVID-19 pandemic. The electoral officials had to be mindful of the numerous security protocols and complexities involved in implementing the election process in such uncertain times. They had to incorporate additional steps and plan for contingencies to mitigate risks while executing the election process. Halfway through the election planning process, it became clear that the number of voters and candidates was unprecedentedly large. This unexpected development necessitated a revision of the prior plan for conducting the elections. Shashank Goel, Chief Electoral Officer (CEO), and M. Satyavani, Deputy CEO, were architecting the plan for conducting the elections with an unexpectedly large number of voters and candidates under pandemic-induced disruptions. Goel was also reflecting on how to develop contingency plans for these elections, given the uncertainty produced by unforeseen external factors and the associated risks. Although he had the mandate to conduct free and fair elections within the stipulated timelines and was assured that the required resources would be provided, several factors had to be considered. According to the constitutional guidelines for the graduates' MLC elections, qualified and registered graduate voters could cast their vote by ranking candidates preferentially. Paper ballots had to be used because electronic voting machines (EVMs) could not handle preferential voting. The scale and magnitude of the elections necessitated jumbo ballot boxes. To manage the process, the number of polling stations had to be increased, and manpower had to be trained. Further, the presence of healthcare workers to ensure the safety of voters and the deployed staff was imperative. The Telangana CEO’s office had to meet the increased logistical and technical requirements and ensure high voting turnouts while executing the election process.
Postponing the election was not an option for the ECI from the standpoint of the legal code of conduct. The Telangana CEO's office prepared a revised election plan. The project plan was amended to incorporate the need for additional resources and logistical support to execute the election process. As the efforts of the staff were maximized effectively, the elections could be conducted smoothly and transparently although a large number of candidates were in the fray.
Teaching and Learning Objectives:
The key case objectives are to enable students to:
- Appreciate the importance of effective project management, planning, and execution in public administration against the backdrop of uncertainties and complexities.
- Understand the importance of risk identification, risk planning, and prioritization.
- Learn strategies to manage various project risks in a real-life situation.
- Identify the characteristics of effective leadership in times of crisis and the key takeaways from such scenarios
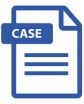
The case is designed to be used in courses on Nonprofit Operations Management, Data Analytics, Six Sigma, and Business Process Excellence/Improvement in MBA or Executive MBA programs. It is suitable for teaching students about the common problem of lower rates of volunteerism in nonprofit organizations. Further, the case study helps present the importance and application of inferential statistics (data analytics) to identify the impact of various factors on the problem (effect). The case is set in early 2021 when Shefali Sharma, the Strategy and Learning Manager with Teach For India (TFI), faced a few challenging questions from a professor at the Indian School of Business (ISB) during her presentation at an industry gathering in Hyderabad, India. Sharma was concerned about the low matriculation rate of TFI fellows, despite the rigorous recruitment, selection, and matriculation (RSM) process. A mere 50-60% matriculation rate was not a commensurate return for an investment of INR 6.5 million and the massive effort put into the RSM process. In 2017, Sharma organized focused informative and experiential events to motivate candidates to join the fellowship, but it was not very clear if these events impacted the TFI matriculation rate. After the industry gathering at ISB, Sharma followed up with the professor to seek his guidance in performing data analytics on the matriculation data. Sharma wondered if inferential data analysis could help her understand which demographic factors and events impact the matriculation rate.
Learning Objective
- Illustrate the importance of inferential statistics as a decision support system in resolving business problems
- Formulating and solving a hypothesis testing problem for attribute (discrete) data
- Visually depicting the flow of work across different stages of a process
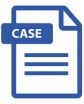
In response to the uncontrollable second wave of COVID-19 in the south Indian state of Telangana in April 2021, a few like-minded social activists in the capital city of Hyderabad came together to establish a 100-bed medical care center to treat COVID-19 patients. The project was named Ashray. Dr. Chinnababu Sunkavalli (popularly known as Chinna) was the project manager of Project Ashray. In addition to the inherent inadequacy of hospital beds to accommodate the growing number of COVID- 19 patients till March 2021, the city faced a sudden spike of infections in April that worsened the situation. Consequently, the occupancy in government and private hospitals in Hyderabad increased by 485% and 311%, respectively, from March to April. According to a prediction model, Chinna knew that hospital beds would be exhausted in several parts of the city in the next few days. The Project Ashray team was concerned about the situation. The team met on April 26, 2021, to schedule the project to establish the medical care center within the next 10 days. The case is suitable for teaching students how to approach the scheduling problem of a time- constrained project systematically. It helps as a pedagogical aid in teaching management concepts such as project visualization, estimating project duration, float, and project laddering or activity splitting, and tools such as network diagrams, critical path method, and crashing. The case exposes students to a real-time problem-solving approach under uncertainty and crises and the critical role of NGOs in supporting the governments. Alongside the Project Management and Operations Management courses, other courses like Managerial decision-making in nonprofit organizations, Health care delivery, and healthcare operations could also find support from this case.
Learning Objectives:
To learn: Time-constrained projects and associated scheduling problems Project visualization using network diagrams Activity sequencing and converting sequential activities to parallel activities Critical path method (early start, early finish, late start, late finish, forward pass, backward pass, and float) to estimate a project's overall duration Project laddering to reduce the project duration wherever possible Project crashing using linear programming
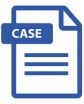
The case goes on to describe the enormous challenges involved in building the 4.94 km long Bogibeel Bridge in the North Eastern Region (NER) of India. When it was finally commissioned in 2018, it was hailed as a marvel of engineering. With two rail lines and a two-lane road over it, the bridge spanned the mighty Brahmaputra river. The Bogibeel Bridge was India's longest and Asia's second-longest road and rail bridge with fully-welded bridge technology that met European codes and welding standards. The interstate connectivity provided by the bridge enabled important socio-economic developments in the NER that included improved logistics and transportation, the growth of medical and educational facilities, higher employment, and the rise of international trade and tourism. While the outcomes of the project were significant, the efforts that went into constructing the Bogibeel Bridge were equally so. This case study is designed to teach the importance of effective risk planning in project management. Further, the case introduces students to earned value analysis and project oversight in managing large projects. The case centers on Indian Railways' need to quickly discover why the Bogibeel project was not going according to plan. The case also serves as a resource to teach public operations management where the focus is on projects and operations that result in socio-economic outcomes.
- Appreciate the importance of risk planning and risk prioritization and learn strategies to manage various project risks
- Understand earned value management (EVM) and the associated metrics and calculations for project evaluation on time and cost schedules.
- Identify social impact outcomes in public/infrastructure projects.
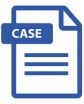
Access to clean water is so critical for development and survival that the United Nations' Sustainable Development Goal number 6 (SDG-6) was to ensure availability and sustained management of water and sanitation. The World Health Organization (WHO) in 2006 estimated that 97 million Indians lacked clean and safe water. Fluoride and total dissolvable solids (TDS) in drinking water were dangerously high at many parts of rural India, with adverse impacts. On the other hand, buying clean drinking water from commercial vendors at market rates was not a realistic alternative, a costly recurring expense that much of India's rural population could not afford. The case tracks the efforts of Huggahalli, head of the technology group of Sri Sathya Sai Seva Organisations (SSSO), to devise a sustainable solution to the drinking water problem in rural India that is low on cost, high on impact. They eventually develop a model that satisfies all these criteria and becomes the basis for a project called Premamrutha Dhaara. Funded by Sri Sathya Sai Central Trust, the project aims to install water purification plants in more than 100 villages spanning six states in India, with the ultimate goal of turning over plant operations to the beneficiary villages and setting up a welfare fund in each village from the revenue generated. Social service projects, particularly in developing countries, have their unique challenges. The case highlights the importance of performing feasibility analysis as part of the project planning in social projects. The case also describes how the financial and operational dimensions of sustainability could lead to a self-sustainable system. The social innovation framework used to deploy the water purification project to achieve broader rural welfare has wider implications for project management, social innovation and change, sustainable operations management, strategic non-profit management, and public policy.
The case offers four possibilities for central objectives:
- To perform feasibility analysis in a Project Management course
- To design a social innovation framework in a Social Innovation and Change course
- To understand the dimensions of self-sustainability in a Sustainable Operations Management course
- To measure social impact in Strategic Non-profit Management and Public Policy courses
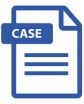
During the Indian general election of 2019, the Nizamabad constituency in Telangana state found itself in an unprecedented situation with a record 185 candidates competing for one seat. Most of these candidates were local farmers who saw the election as a platform for raising awareness about local issues, particularly the perceived lack of government support for guaranteeing minimum support prices for their crops. More than 185 candidates had in fact contested elections from a single constituency in a handful of elections in the past. The Election Commission of India (ECI) had declared them to be "special elections" where it made exceptions to the original election schedule to accommodate the large number of candidates. However, in the 2019 general election, the ECI made no such exceptions, announcing instead that polling in Nizamabad would be conducted as per the original schedule and results would be declared at the same time as the rest of the country. This presented a unique and unexpected challenge for Rajat Kumar, the Telangana Chief Electoral Officer (CEO) and his team. How were they to conduct free and fair and elections within the mandated timeframe with the largest number of electronic voting machines (EVMs) ever deployed to address the will of 185 candidates in a constituency with 1.55 million voters from rural and semi-urban areas? Case A describes the electoral process followed by the world's largest democracy to guarantee free and fair elections. It concludes by posing several situational questions, the answers to which will determine whether the polls in Nizamabad are conducted successfully or not. Case B, which should be revealed after students have had a chance to deliberate on the challenges posed in Case A, describes the decisions and actions taken by Kumar and his team in preparation for the Nizamabad polls and the events that took place on election day and afterward.
To demonstrate how a quantitative approach to decision making can be used in the public policy domain to achieve end goals. To learn how resource allocation decisions can be made by understanding the scale of the problem, the various resource constraints, and the end goals. To discover operational innovations in the face of regulatory and technical constraints and complete the required steps. To understand the multiple steps involved in conducting elections in the Indian context.
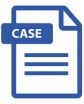
Set in April 2017, this case centers around the digital technology dilemma facing the protagonist Dr. Vimohan, the chief intensivist of Prashant Hospital. The case describes the critical challenges afflicting the intensive care unit (ICU) of the hospital. It then follows Dr. Vimohan as he visits the Bengaluru headquarters of Cloudphysician Healthcare, a Tele-ICU provider. The visit leaves Dr. Vimohan wondering whether he can leverage the Tele-ICU solution to overcome the challenges at Prashant Hospital. He instinctively knew that he would need to use a combination of qualitative and quantitative analysis to resolve this dilemma.
The case study enables critical thinking and decision-making to address the business situation. Assessing the pros and cons of a potential technology solution, examining the readiness of an organization and devising a framework for effective stakeholder and change management are some of the key concepts. Associated tools include cost-benefit analysis, net present value (NPV) analysis, force-field analysis, and change-readiness assessment, in addition to a brief discussion on SWOT analysis.
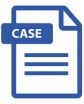
Set in 2016 in Hyderabad, India, the case follows Puvvala Yugandhar, Senior Vice President at Dr. Reddy's Laboratories (DRL), as he decides what to do about an underperforming production policy at their plants. Adopted a decade earlier, the policy, called Replenish to Consumption -Pooled (RTC-P), had not delivered the expected results. Specifically, the plants had been seeing an increase in production switchovers and creeping buffer levels for certain products, which had led to higher holding costs and lost sales for certain products. A senior consultant had suggested that DRL switch to a demand estimation-based policy called Replenish to Anticipation (RTA), which attempted to address the above concerns by segregating production capacity and updating buffer levels using demand estimates. However, Yugandhar, well aware of the challenges of changing production policies, wanted to explore a variant of RTC-P called Replenish to Consumption -Dedicated (RTC-D), which followed the same buffer update rules as RTC-P but maintained dedicated capacities for a subset of products.
By studying and solving the decision problem in the case, students should be able to better appreciate the challenges involved in making long-term operational changes. It gives them an opportunity to: (1) understand how each input might impact the final decision, and (2) how to weigh each of these inputs in arriving at the final decision.
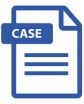
We crafted the case study "Software Acquisition for Employee Engagement at Pilot Mountain Research " for use in Business Marketing, Buyer Behavior, or Operations Management courses in undergraduate, MBA, or Executive Education programs. The Pilot Mountain Market Research (PMMR) case study provides students with the opportunity to examine how buying decisions can be made utilizing online digital tools that are increasingly available to business-to-business (B2B) purchasing managers. To do so, we created fictitious research studies and data to realistically portray the kinds of information that are publicly available to B2B purchasing managers on the Internet today. In this case study, we introduce students to fit analysis, coding quality technical assessment, sentiment analysis, and ratings & reviews analyses. Students are challenged to integrate findings from these diverse analytical tools, combining both qualitative and quantitative data into concrete employee engagement software (EES) purchasing recommendations.
1. Evolving criteria for selecting a software package for organization-wide procurement in a B2B purchase decision context 2. Appreciate increasing digitalization of businesses 3. Understand importance of employee engagement in organizations and what an organization could do to enhance employee engagement among its workforce 4. Understand decision making processes in the context of digitalisation of businesses
Academia.edu no longer supports Internet Explorer.
To browse Academia.edu and the wider internet faster and more securely, please take a few seconds to upgrade your browser .
Enter the email address you signed up with and we'll email you a reset link.
- We're Hiring!
- Help Center
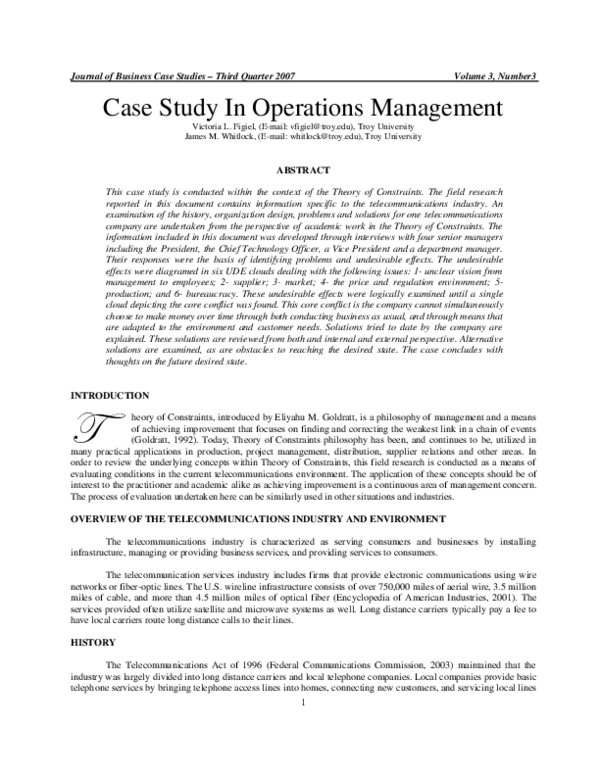
Case Study In Operations Management

2011, Journal of Business Case Studies (JBCS)
This case study is conducted within the context of the Theory of Constraints. The field research reported in this document contains information specific to the telecommunications industry. An examination of the history, organization design, problems and solutions for one telecommunications company are undertaken from the perspective of academic work in the Theory of Constraints. The information included in this document was developed through interviews with four senior managers including the President, the Chief Technology Officer, a Vice President and a department manager. Their responses were the basis of identifying problems and undesirable effects. The undesirable effects were diagramed in six UDE clouds dealing with the following issues: 1- unclear vision from management to employees; 2- supplier; 3- market; 4- the price and regulation environment; 5- production; and 6- bureaucracy. These undesirable effects were logically examined until a single cloud depicting the core confli...
Related Papers
CHIRANJIB BHOWMIK
The aim of this paper is to implement TOC in forging area in which the constraints prevents the throughput of the system to enhance the quality and reduce errors. Many quality improvement (QI) approaches have a limited evaluation of the factors in the selection of QI projects. Theory of constraints (TOC) has been proposed as a remedy for the better selection of QI projects. The strategic Thinking Processes (TP) of Theory of constraints is designed to struggle an enormous problem faced by organizations. The paper proposes an improvement of TOC–based TP in one of the leading forging industry in India to identify and overcome the system constraints in the business. The result shows that the TOC-TP identifies the production constraints and suggests measures to improve the system. The research is applicable to any production house in which product quality reduces the throughput of the organization. This is the first time that the theory of constraints philosophy has been used to maximize...
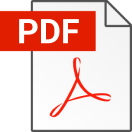
Jesus Ramon Melendez
The investigations began with the drum-buffer-rope architecture, as the basis of the Theory of Constraints (TOC). Currently, TOC has been applied in various business sectors. With the support of mathematical models and simulation, it has been possible to optimize the productive processes. The objective of this study was to determine the investigative tendencies of the TOC in the different productive sectors and its application in business management environments. The results establish that its application increases the efficiency of the process.
Nigerian Chapter of Arabian Journal of Business and Management Review
Hamed Alizadeh
In today’s economic climate, many organizations struggle with declining sales and increasing costs. Some choose to hunker down and weather the storm, hoping for better results in the future. However, layoffs and workforce reductions jeopardize future competitiveness. However, organizations that have implemented the Theory of Constraints (TOC) continue to thrive and grow in difficult times, continuing to achieve real bottom line growth, whether by improving productivity or increased revenues. In this paper, the organization dealing with the furniture manufacturing has been studied and the main constraints for the maximum throughput are identified by applying a thinking process tool called as “Theory of Constraints” (TOC). The Drum Buffer Rope (DBR) has been applied for capacity planning and the time for each identified processes is calculated and workload for each work center is calculated. Then the capacity constraint machine is identified. The proper solution has been provided to o...
Niek Du Preez
Erkam Guresen
Theory of constraints (TOC) is a technique that produces solutions for every kind of bottleneck in a short time. The philosophy of the theory is to determine the weaker part of the process chain and to eliminate this constraint point by taking action. After improvement, the next weaker part of the process chain is determined, and so on, for continuous improvement. The main goal is to apply improvement actions continuously to reach an excellent system structure. This paper describes how the five main steps of the theory of constraints were applied to eliminate waste at a supplier firm in Turkey..
Aitor Lizarralde
Purpose: The theory of constraints (TOC) drum-buffer-rope methodology is appropriate when managing a production plant in complex environments, such as make-to-order (MTO) scenarios. However, some difficulties have been detected in implementing this methodology in such changing environments. This case study analyses a MTO company to identify the key factors that influence the execution of the third step of TOC. It also aims to evaluate in more depth the research started by Lizarralde et al. (2020) and compare the results with the existing literature. Design/methodology/approach: The case study approach is selected as a research methodology because of the need to investigate a current phenomenon in a real environment. Findings: In the case study analysed, the protective capacity of non-bottleneck resources is found to the key factor when subordinating the MTO system to a bottleneck (BN). Furthermore, it coincides with one of the two key factors defined by the literature, namely protec...
Information Systems and e-Business Management
Niv Ahituv , Nitza Geri
Decision Line
Vicky Mabin
Alexei Sharpanskykh
RELATED PAPERS
João Vitor Motta Machado
Discourse and Communication for Sustainable Education
Ilga Salite
Joe Molinaro
Physical Review B
Paul Hansma
Izvestiya Wysshikh Uchebnykh Zawedeniy, Yadernaya Energetika
Dinah Changwony
Linear Algebra and its Applications
Milton Maritz
Petrus Marjono
Nanotechnology
The European Physical Journal B - Condensed Matter
Augusto Schianchi
Joseph McCarthy
Journal of Experimental Biology
Rosemary Smith
Saidon Amri
Helimarcos Pereira
ACS Catalysis
Philippe Lainé
Indian Journal of Hematology and Blood Transfusion
Yavuz Sanisoglu
Biochemical Pharmacology
Zafer Gashi
Education Sciences
Ximo Gual Arnau
Journal of Biomedical Optics
Susana Puig
Physica B+C
Wiebe Geertsma
Nepal Journal of Science and Technology
Manjusha Kulkarni
The Oncologist
Sébastien Salas
Abderraouf HILALI
Northeastern Naturalist
RELATED TOPICS
- We're Hiring!
- Help Center
- Find new research papers in:
- Health Sciences
- Earth Sciences
- Cognitive Science
- Mathematics
- Computer Science
- Academia ©2024
Machine Learning and image analysis towards improved energy management in Industry 4.0: a practical case study on quality control
- Original Article
- Open access
- Published: 13 May 2024
- Volume 17 , article number 48 , ( 2024 )
Cite this article
You have full access to this open access article
- Mattia Casini 1 ,
- Paolo De Angelis 1 ,
- Marco Porrati 2 ,
- Paolo Vigo 1 ,
- Matteo Fasano 1 ,
- Eliodoro Chiavazzo 1 &
- Luca Bergamasco ORCID: orcid.org/0000-0001-6130-9544 1
With the advent of Industry 4.0, Artificial Intelligence (AI) has created a favorable environment for the digitalization of manufacturing and processing, helping industries to automate and optimize operations. In this work, we focus on a practical case study of a brake caliper quality control operation, which is usually accomplished by human inspection and requires a dedicated handling system, with a slow production rate and thus inefficient energy usage. We report on a developed Machine Learning (ML) methodology, based on Deep Convolutional Neural Networks (D-CNNs), to automatically extract information from images, to automate the process. A complete workflow has been developed on the target industrial test case. In order to find the best compromise between accuracy and computational demand of the model, several D-CNNs architectures have been tested. The results show that, a judicious choice of the ML model with a proper training, allows a fast and accurate quality control; thus, the proposed workflow could be implemented for an ML-powered version of the considered problem. This would eventually enable a better management of the available resources, in terms of time consumption and energy usage.
Avoid common mistakes on your manuscript.
Introduction
An efficient use of energy resources in industry is key for a sustainable future (Bilgen, 2014 ; Ocampo-Martinez et al., 2019 ). The advent of Industry 4.0, and of Artificial Intelligence, have created a favorable context for the digitalisation of manufacturing processes. In this view, Machine Learning (ML) techniques have the potential for assisting industries in a better and smart usage of the available data, helping to automate and improve operations (Narciso & Martins, 2020 ; Mazzei & Ramjattan, 2022 ). For example, ML tools can be used to analyze sensor data from industrial equipment for predictive maintenance (Carvalho et al., 2019 ; Dalzochio et al., 2020 ), which allows identification of potential failures in advance, and thus to a better planning of maintenance operations with reduced downtime. Similarly, energy consumption optimization (Shen et al., 2020 ; Qin et al., 2020 ) can be achieved via ML-enabled analysis of available consumption data, with consequent adjustments of the operating parameters, schedules, or configurations to minimize energy consumption while maintaining an optimal production efficiency. Energy consumption forecast (Liu et al., 2019 ; Zhang et al., 2018 ) can also be improved, especially in industrial plants relying on renewable energy sources (Bologna et al., 2020 ; Ismail et al., 2021 ), by analysis of historical data on weather patterns and forecast, to optimize the usage of energy resources, avoid energy peaks, and leverage alternative energy sources or storage systems (Li & Zheng, 2016 ; Ribezzo et al., 2022 ; Fasano et al., 2019 ; Trezza et al., 2022 ; Mishra et al., 2023 ). Finally, ML tools can also serve for fault or anomaly detection (Angelopoulos et al., 2019 ; Md et al., 2022 ), which allows prompt corrective actions to optimize energy usage and prevent energy inefficiencies. Within this context, ML techniques for image analysis (Casini et al., 2024 ) are also gaining increasing interest (Chen et al., 2023 ), for their application to e.g. materials design and optimization (Choudhury, 2021 ), quality control (Badmos et al., 2020 ), process monitoring (Ho et al., 2021 ), or detection of machine failures by converting time series data from sensors to 2D images (Wen et al., 2017 ).
Incorporating digitalisation and ML techniques into Industry 4.0 has led to significant energy savings (Maggiore et al., 2021 ; Nota et al., 2020 ). Projects adopting these technologies can achieve an average of 15% to 25% improvement in energy efficiency in the processes where they were implemented (Arana-Landín et al., 2023 ). For instance, in predictive maintenance, ML can reduce energy consumption by optimizing the operation of machinery (Agrawal et al., 2023 ; Pan et al., 2024 ). In process optimization, ML algorithms can improve energy efficiency by 10-20% by analyzing and adjusting machine operations for optimal performance, thereby reducing unnecessary energy usage (Leong et al., 2020 ). Furthermore, the implementation of ML algorithms for optimal control can lead to energy savings of 30%, because these systems can make real-time adjustments to production lines, ensuring that machines operate at peak energy efficiency (Rahul & Chiddarwar, 2023 ).
In automotive manufacturing, ML-driven quality control can lead to energy savings by reducing the need for redoing parts or running inefficient production cycles (Vater et al., 2019 ). In high-volume production environments such as consumer electronics, novel computer-based vision models for automated detection and classification of damaged packages from intact packages can speed up operations and reduce waste (Shahin et al., 2023 ). In heavy industries like steel or chemical manufacturing, ML can optimize the energy consumption of large machinery. By predicting the optimal operating conditions and maintenance schedules, these systems can save energy costs (Mypati et al., 2023 ). Compressed air is one of the most energy-intensive processes in manufacturing. ML can optimize the performance of these systems, potentially leading to energy savings by continuously monitoring and adjusting the air compressors for peak efficiency, avoiding energy losses due to leaks or inefficient operation (Benedetti et al., 2019 ). ML can also contribute to reducing energy consumption and minimizing incorrectly produced parts in polymer processing enterprises (Willenbacher et al., 2021 ).
Here we focus on a practical industrial case study of brake caliper processing. In detail, we focus on the quality control operation, which is typically accomplished by human visual inspection and requires a dedicated handling system. This eventually implies a slower production rate, and inefficient energy usage. We thus propose the integration of an ML-based system to automatically perform the quality control operation, without the need for a dedicated handling system and thus reduced operation time. To this, we rely on ML tools able to analyze and extract information from images, that is, deep convolutional neural networks, D-CNNs (Alzubaidi et al., 2021 ; Chai et al., 2021 ).
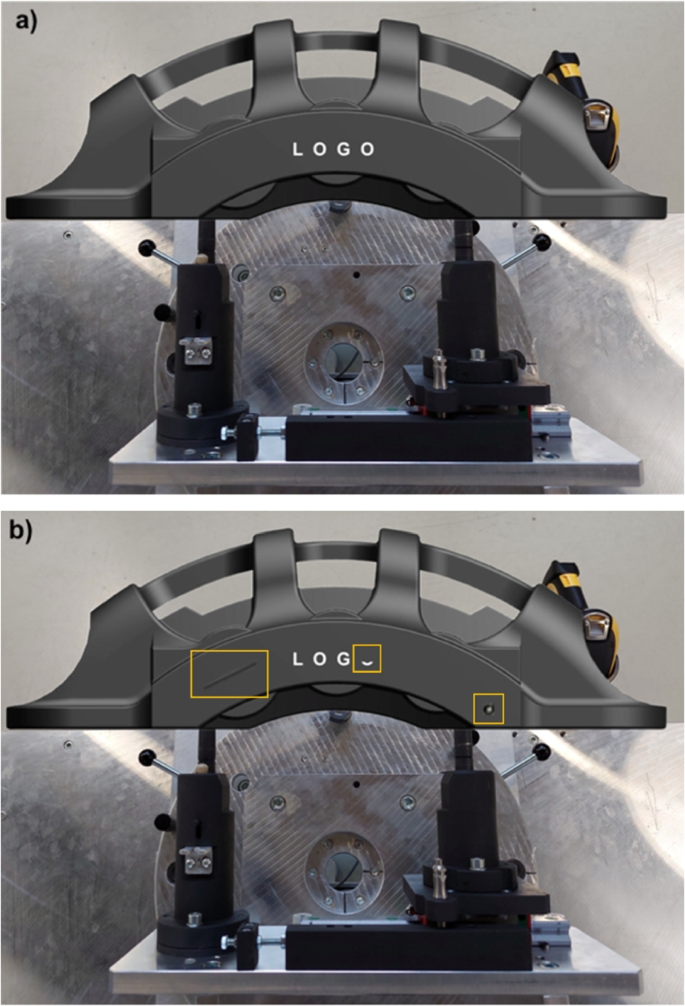
Sample 3D model (GrabCAD ) of the considered brake caliper: (a) part without defects, and (b) part with three sample defects, namely a scratch, a partially missing letter in the logo, and a circular painting defect (shown by the yellow squares, from left to right respectively)
A complete workflow for the purpose has been developed and tested on a real industrial test case. This includes: a dedicated pre-processing of the brake caliper images, their labelling and analysis using two dedicated D-CNN architectures (one for background removal, and one for defect identification), post-processing and analysis of the neural network output. Several different D-CNN architectures have been tested, in order to find the best model in terms of accuracy and computational demand. The results show that, a judicious choice of the ML model with a proper training, allows to obtain fast and accurate recognition of possible defects. The best-performing models, indeed, reach over 98% accuracy on the target criteria for quality control, and take only few seconds to analyze each image. These results make the proposed workflow compliant with the typical industrial expectations; therefore, in perspective, it could be implemented for an ML-powered version of the considered industrial problem. This would eventually allow to achieve better performance of the manufacturing process and, ultimately, a better management of the available resources in terms of time consumption and energy expense.
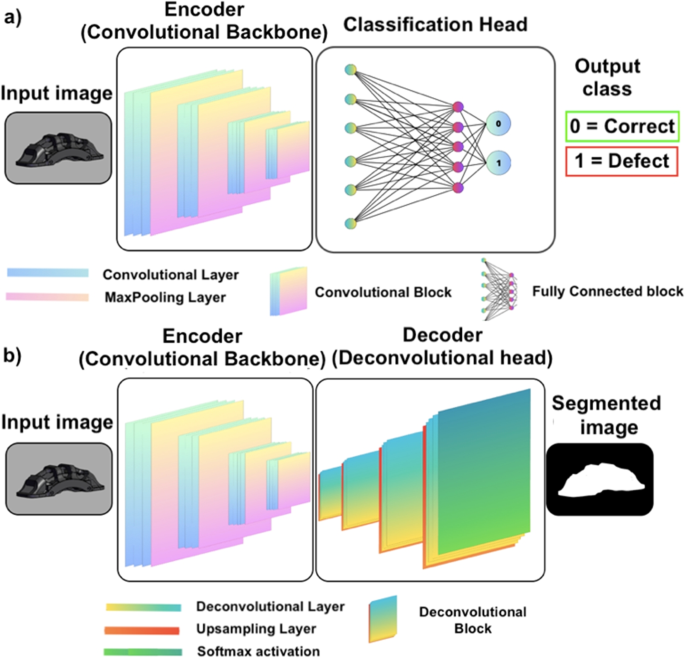
Different neural network architectures: convolutional encoder (a) and encoder-decoder (b)
The industrial quality control process that we target is the visual inspection of manufactured components, to verify the absence of possible defects. Due to industrial confidentiality reasons, a representative open-source 3D geometry (GrabCAD ) of the considered parts, similar to the original one, is shown in Fig. 1 . For illustrative purposes, the clean geometry without defects (Fig. 1 (a)) is compared to the geometry with three possible sample defects, namely: a scratch on the surface of the brake caliper, a partially missing letter in the logo, and a circular painting defect (highlighted by the yellow squares, from left to right respectively, in Fig. 1 (b)). Note that, one or multiple defects may be present on the geometry, and that other types of defects may also be considered.
Within the industrial production line, this quality control is typically time consuming, and requires a dedicated handling system with the associated slow production rate and energy inefficiencies. Thus, we developed a methodology to achieve an ML-powered version of the control process. The method relies on data analysis and, in particular, on information extraction from images of the brake calipers via Deep Convolutional Neural Networks, D-CNNs (Alzubaidi et al., 2021 ). The designed workflow for defect recognition is implemented in the following two steps: 1) removal of the background from the image of the caliper, in order to reduce noise and irrelevant features in the image, ultimately rendering the algorithms more flexible with respect to the background environment; 2) analysis of the geometry of the caliper to identify the different possible defects. These two serial steps are accomplished via two different and dedicated neural networks, whose architecture is discussed in the next section.
Convolutional Neural Networks (CNNs) pertain to a particular class of deep neural networks for information extraction from images. The feature extraction is accomplished via convolution operations; thus, the algorithms receive an image as an input, analyze it across several (deep) neural layers to identify target features, and provide the obtained information as an output (Casini et al., 2024 ). Regarding this latter output, different formats can be retrieved based on the considered architecture of the neural network. For a numerical data output, such as that required to obtain a classification of the content of an image (Bhatt et al., 2021 ), e.g. correct or defective caliper in our case, a typical layout of the network involving a convolutional backbone, and a fully-connected network can be adopted (see Fig. 2 (a)). On the other hand, if the required output is still an image, a more complex architecture with a convolutional backbone (encoder) and a deconvolutional head (decoder) can be used (see Fig. 2 (b)).
As previously introduced, our workflow targets the analysis of the brake calipers in a two-step procedure: first, the removal of the background from the input image (e.g. Fig. 1 ); second, the geometry of the caliper is analyzed and the part is classified as acceptable or not depending on the absence or presence of any defect, respectively. Thus, in the first step of the procedure, a dedicated encoder-decoder network (Minaee et al., 2021 ) is adopted to classify the pixels in the input image as brake or background. The output of this model will then be a new version of the input image, where the background pixels are blacked. This helps the algorithms in the subsequent analysis to achieve a better performance, and to avoid bias due to possible different environments in the input image. In the second step of the workflow, a dedicated encoder architecture is adopted. Here, the previous background-filtered image is fed to the convolutional network, and the geometry of the caliper is analyzed to spot possible defects and thus classify the part as acceptable or not. In this work, both deep learning models are supervised , that is, the algorithms are trained with the help of human-labeled data (LeCun et al., 2015 ). Particularly, the first algorithm for background removal is fed with the original image as well as with a ground truth (i.e. a binary image, also called mask , consisting of black and white pixels) which instructs the algorithm to learn which pixels pertain to the brake and which to the background. This latter task is usually called semantic segmentation in Machine Learning and Deep Learning (Géron, 2022 ). Analogously, the second algorithm is fed with the original image (without the background) along with an associated mask, which serves the neural networks with proper instructions to identify possible defects on the target geometry. The required pre-processing of the input images, as well as their use for training and validation of the developed algorithms, are explained in the next sections.
Image pre-processing
Machine Learning approaches rely on data analysis; thus, the quality of the final results is well known to depend strongly on the amount and quality of the available data for training of the algorithms (Banko & Brill, 2001 ; Chen et al., 2021 ). In our case, the input images should be well-representative for the target analysis and include adequate variability of the possible features to allow the neural networks to produce the correct output. In this view, the original images should include, e.g., different possible backgrounds, a different viewing angle of the considered geometry and a different light exposure (as local light reflections may affect the color of the geometry and thus the analysis). The creation of such a proper dataset for specific cases is not always straightforward; in our case, for example, it would imply a systematic acquisition of a large set of images in many different conditions. This would require, in turn, disposing of all the possible target defects on the real parts, and of an automatic acquisition system, e.g., a robotic arm with an integrated camera. Given that, in our case, the initial dataset could not be generated on real parts, we have chosen to generate a well-balanced dataset of images in silico , that is, based on image renderings of the real geometry. The key idea was that, if the rendered geometry is sufficiently close to a real photograph, the algorithms may be instructed on artificially-generated images and then tested on a few real ones. This approach, if properly automatized, clearly allows to easily produce a large amount of images in all the different conditions required for the analysis.
In a first step, starting from the CAD file of the brake calipers, we worked manually using the open-source software Blender (Blender ), to modify the material properties and achieve a realistic rendering. After that, defects were generated by means of Boolean (subtraction) operations between the geometry of the brake caliper and ad-hoc geometries for each defect. Fine tuning on the generated defects has allowed for a realistic representation of the different defects. Once the results were satisfactory, we developed an automated Python code for the procedures, to generate the renderings in different conditions. The Python code allows to: load a given CAD geometry, change the material properties, set different viewing angles for the geometry, add different types of defects (with given size, rotation and location on the geometry of the brake caliper), add a custom background, change the lighting conditions, render the scene and save it as an image.
In order to make the dataset as varied as possible, we introduced three light sources into the rendering environment: a diffuse natural lighting to simulate daylight conditions, and two additional artificial lights. The intensity of each light source and the viewing angle were then made vary randomly, to mimic different daylight conditions and illuminations of the object. This procedure was designed to provide different situations akin to real use, and to make the model invariant to lighting conditions and camera position. Moreover, to provide additional flexibility to the model, the training dataset of images was virtually expanded using data augmentation (Mumuni & Mumuni, 2022 ), where saturation, brightness and contrast were made randomly vary during training operations. This procedure has allowed to consistently increase the number and variety of the images in the training dataset.
The developed automated pre-processing steps easily allows for batch generation of thousands of different images to be used for training of the neural networks. This possibility is key for proper training of the neural networks, as the variability of the input images allows the models to learn all the possible features and details that may change during real operating conditions.
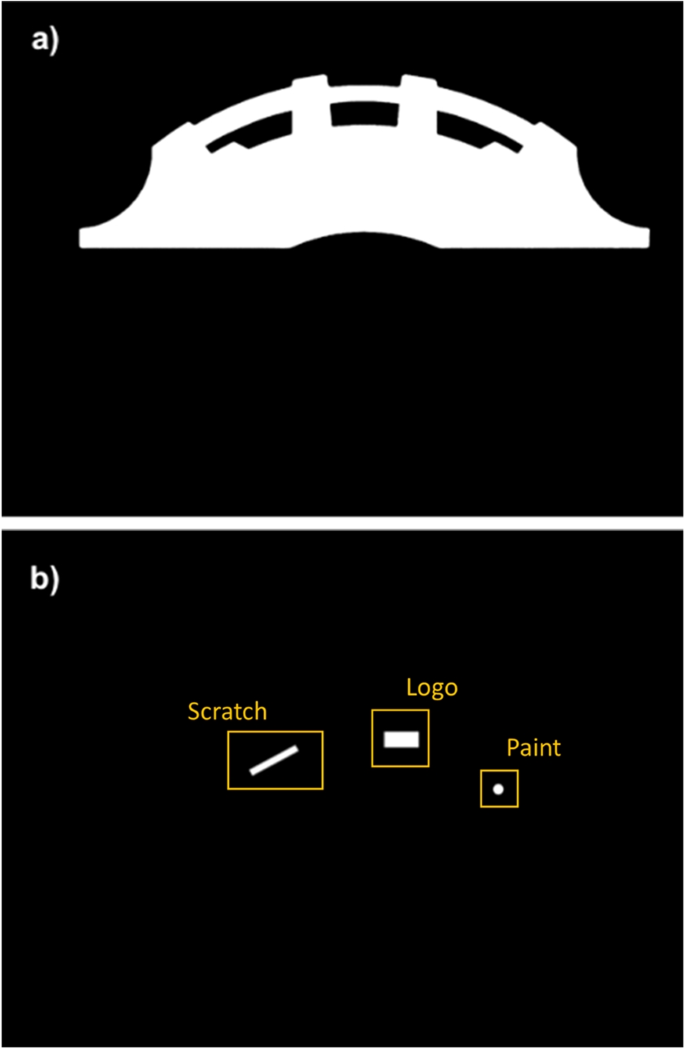
Examples of the ground truth for the two target tasks: background removal (a) and defects recognition (b)
The first tests using such virtual database have shown that, although the generated images were very similar to real photographs, the models were not able to properly recognize the target features in the real images. Thus, in a tentative to get closer to a proper set of real images, we decided to adopt a hybrid dataset, where the virtually generated images were mixed with the available few real ones. However, given that some possible defects were missing in the real images, we also decided to manipulate the images to introduce virtual defects on real images. The obtained dataset finally included more than 4,000 images, where 90% was rendered, and 10% was obtained from real images. To avoid possible bias in the training dataset, defects were present in 50% of the cases in both the rendered and real image sets. Thus, in the overall dataset, the real original images with no defects were 5% of the total.
Along with the code for the rendering and manipulation of the images, dedicated Python routines were developed to generate the corresponding data labelling for the supervised training of the networks, namely the image masks. Particularly, two masks were generated for each input image: one for the background removal operation, and one for the defect identification. In both cases, the masks consist of a binary (i.e. black and white) image where all the pixels of a target feature (i.e. the geometry or defect) are assigned unitary values (white); whereas, all the remaining pixels are blacked (zero values). An example of these masks in relation to the geometry in Fig. 1 is shown in Fig. 3 .
All the generated images were then down-sampled, that is, their resolution was reduced to avoid unnecessary large computational times and (RAM) memory usage while maintaining the required level of detail for training of the neural networks. Finally, the input images and the related masks were split into a mosaic of smaller tiles, to achieve a suitable size for feeding the images to the neural networks with even more reduced requirements on the RAM memory. All the tiles were processed, and the whole image reconstructed at the end of the process to visualize the overall final results.
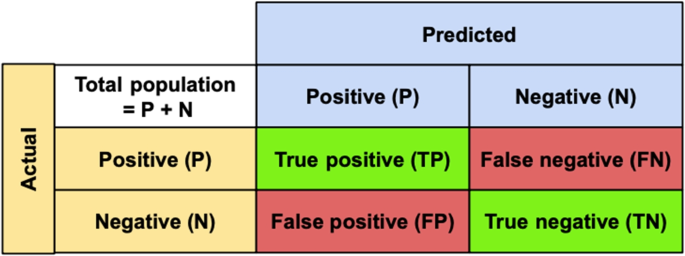
Confusion matrix for accuracy assessment of the neural networks models
Choice of the model
Within the scope of the present application, a wide range of possibly suitable models is available (Chen et al., 2021 ). In general, the choice of the best model for a given problem should be made on a case-by-case basis, considering an acceptable compromise between the achievable accuracy and computational complexity/cost. Too simple models can indeed be very fast in the response yet have a reduced accuracy. On the other hand, more complex models can generally provide more accurate results, although typically requiring larger amounts of data for training, and thus longer computational times and energy expense. Hence, testing has the crucial role to allow identification of the best trade-off between these two extreme cases. A benchmark for model accuracy can generally be defined in terms of a confusion matrix, where the model response is summarized into the following possibilities: True Positives (TP), True Negatives (TN), False Positives (FP) and False Negatives (FN). This concept can be summarized as shown in Fig. 4 . For the background removal, Positive (P) stands for pixels belonging to the brake caliper, while Negative (N) for background pixels. For the defect identification model, Positive (P) stands for non-defective geometry, whereas Negative (N) stands for defective geometries. With respect to these two cases, the True/False statements stand for correct or incorrect identification, respectively. The model accuracy can be therefore assessed as Géron ( 2022 )
Based on this metrics, the accuracy for different models can then be evaluated on a given dataset, where typically 80% of the data is used for training and the remaining 20% for validation. For the defect recognition stage, the following models were tested: VGG-16 (Simonyan & Zisserman, 2014 ), ResNet50, ResNet101, ResNet152 (He et al., 2016 ), Inception V1 (Szegedy et al., 2015 ), Inception V4 and InceptionResNet V2 (Szegedy et al., 2017 ). Details on the assessment procedure for the different models are provided in the Supplementary Information file. For the background removal stage, the DeepLabV3 \(+\) (Chen et al., 2018 ) model was chosen as the first option, and no additional models were tested as it directly provided satisfactory results in terms of accuracy and processing time. This gives preliminary indication that, from the point of view of the task complexity of the problem, the defect identification stage can be more demanding with respect to the background removal operation for the case study at hand. Besides the assessment of the accuracy according to, e.g., the metrics discussed above, additional information can be generally collected, such as too low accuracy (indicating insufficient amount of training data), possible bias of the models on the data (indicating a non-well balanced training dataset), or other specific issues related to missing representative data in the training dataset (Géron, 2022 ). This information helps both to correctly shape the training dataset, and to gather useful indications for the fine tuning of the model after its choice has been made.
Background removal
An initial bias of the model for background removal arose on the color of the original target geometry (red color). The model was indeed identifying possible red spots on the background as part of the target geometry as an unwanted output. To improve the model flexibility, and thus its accuracy on the identification of the background, the training dataset was expanded using data augmentation (Géron, 2022 ). This technique allows to artificially increase the size of the training dataset by applying various transformations to the available images, with the goal to improve the performance and generalization ability of the models. This approach typically involves applying geometric and/or color transformations to the original images; in our case, to account for different viewing angles of the geometry, different light exposures, and different color reflections and shadowing effects. These improvements of the training dataset proved to be effective on the performance for the background removal operation, with a validation accuracy finally ranging above 99% and model response time around 1-2 seconds. An example of the output of this operation for the geometry in Fig. 1 is shown in Fig. 5 .
While the results obtained were satisfactory for the original (red) color of the calipers, we decided to test the model ability to be applied on brake calipers of other colors as well. To this, the model was trained and tested on a grayscale version of the images of the calipers, which allows to completely remove any possible bias of the model on a specific color. In this case, the validation accuracy of the model was still obtained to range above 99%; thus, this approach was found to be particularly interesting to make the model suitable for background removal operation even on images including calipers of different colors.
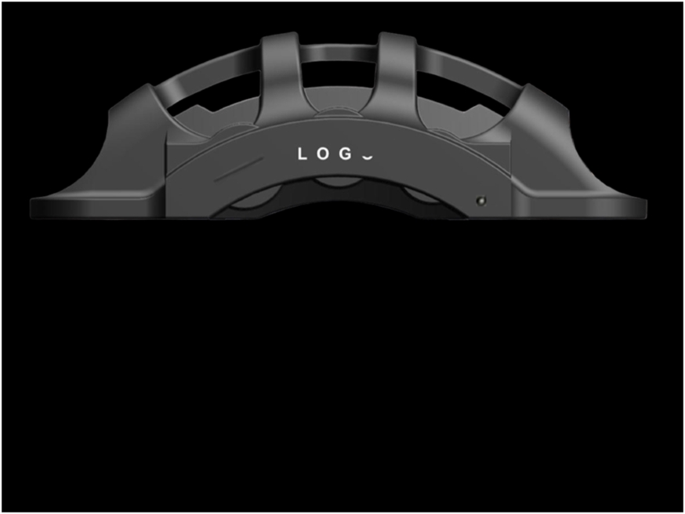
Target geometry after background removal
Defect recognition
An overview of the performance of the tested models for the defect recognition operation on the original geometry of the caliper is reported in Table 1 (see also the Supplementary Information file for more details on the assessment of different models). The results report on the achieved validation accuracy ( \(A_v\) ) and on the number of parameters ( \(N_p\) ), with this latter being the total number of parameters that can be trained for each model (Géron, 2022 ) to determine the output. Here, this quantity is adopted as an indicator of the complexity of each model.
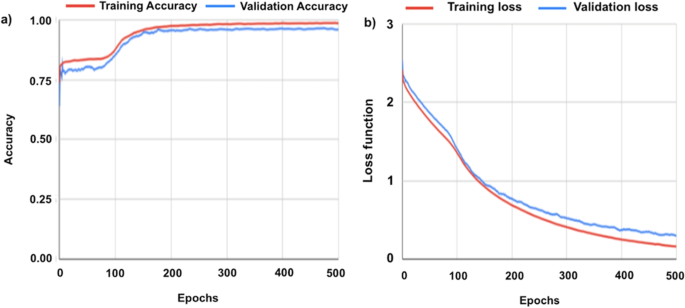
Accuracy (a) and loss function (b) curves for the Resnet101 model during training
As the results in Table 1 show, the VGG-16 model was quite unprecise for our dataset, eventually showing underfitting (Géron, 2022 ). Thus, we decided to opt for the Resnet and Inception families of models. Both these families of models have demonstrated to be suitable for handling our dataset, with slightly less accurate results being provided by the Resnet50 and InceptionV1. The best results were obtained using Resnet101 and InceptionV4, with very high final accuracy and fast processing time (in the order \(\sim \) 1 second). Finally, Resnet152 and InceptionResnetV2 models proved to be slightly too complex or slower for our case; they indeed provided excellent results but taking longer response times (in the order of \(\sim \) 3-5 seconds). The response time is indeed affected by the complexity ( \(N_p\) ) of the model itself, and by the hardware used. In our work, GPUs were used for training and testing all the models, and the hardware conditions were kept the same for all models.
Based on the results obtained, ResNet101 model was chosen as the best solution for our application, in terms of accuracy and reduced complexity. After fine-tuning operations, the accuracy that we obtained with this model reached nearly 99%, both in the validation and test datasets. This latter includes target real images, that the models have never seen before; thus, it can be used for testing of the ability of the models to generalize the information learnt during the training/validation phase.
The trend in the accuracy increase and loss function decrease during training of the Resnet101 model on the original geometry are shown in Fig. 6 (a) and (b), respectively. Particularly, the loss function quantifies the error between the predicted output during training of the model and the actual target values in the dataset. In our case, the loss function is computed using the cross-entropy function and the Adam optimiser (Géron, 2022 ). The error is expected to reduce during the training, which eventually leads to more accurate predictions of the model on previously-unseen data. The combination of accuracy and loss function trends, along with other control parameters, is typically used and monitored to evaluate the training process, and avoid e.g. under- or over-fitting problems (Géron, 2022 ). As Fig. 6 (a) shows, the accuracy experiences a sudden step increase during the very first training phase (epochs, that is, the number of times the complete database is repeatedly scrutinized by the model during its training (Géron, 2022 )). The accuracy then increases in a smooth fashion with the epochs, until an asymptotic value is reached both for training and validation accuracy. These trends in the two accuracy curves can generally be associated with a proper training; indeed, being the two curves close to each other may be interpreted as an absence of under-fitting problems. On the other hand, Fig. 6 (b) shows that the loss function curves are close to each other, with a monotonically-decreasing trend. This can be interpreted as an absence of over-fitting problems, and thus of proper training of the model.
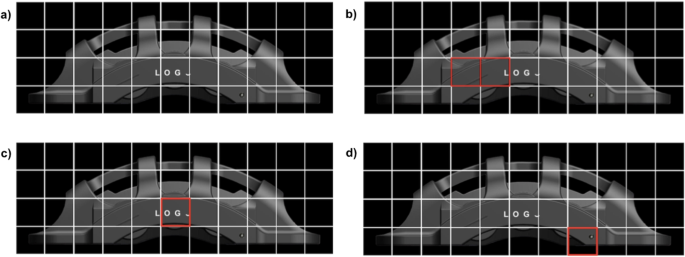
Final results of the analysis on the defect identification: (a) considered input geometry, (b), (c) and (d) identification of a scratch on the surface, partially missing logo, and painting defect respectively (highlighted in the red frames)
Finally, an example output of the overall analysis is shown in Fig. 7 , where the considered input geometry is shown (a), along with the identification of the defects (b), (c) and (d) obtained from the developed protocol. Note that, here the different defects have been separated in several figures for illustrative purposes; however, the analysis yields the identification of defects on one single image. In this work, a binary classification was performed on the considered brake calipers, where the output of the models allows to discriminate between defective or non-defective components based on the presence or absence of any of the considered defects. Note that, fine tuning of this discrimination is ultimately with the user’s requirements. Indeed, the model output yields as the probability (from 0 to 100%) of the possible presence of defects; thus, the discrimination between a defective or non-defective part is ultimately with the user’s choice of the acceptance threshold for the considered part (50% in our case). Therefore, stricter or looser criteria can be readily adopted. Eventually, for particularly complex cases, multiple models may also be used concurrently for the same task, and the final output defined based on a cross-comparison of the results from different models. As a last remark on the proposed procedure, note that here we adopted a binary classification based on the presence or absence of any defect; however, further classification of the different defects could also be implemented, to distinguish among different types of defects (multi-class classification) on the brake calipers.
Energy saving
Illustrative scenarios.
Given that the proposed tools have not yet been implemented and tested within a real industrial production line, we analyze here three perspective scenarios to provide a practical example of the potential for energy savings in an industrial context. To this, we consider three scenarios, which compare traditional human-based control operations and a quality control system enhanced by the proposed Machine Learning (ML) tools. Specifically, here we analyze a generic brake caliper assembly line formed by 14 stations, as outlined in Table 1 in the work by Burduk and Górnicka ( 2017 ). This assembly line features a critical inspection station dedicated to defect detection, around which we construct three distinct scenarios to evaluate the efficacy of traditional human-based control operations versus a quality control system augmented by the proposed ML-based tools, namely:
First Scenario (S1): Human-Based Inspection. The traditional approach involves a human operator responsible for the inspection tasks.
Second Scenario (S2): Hybrid Inspection. This scenario introduces a hybrid inspection system where our proposed ML-based automatic detection tool assists the human inspector. The ML tool analyzes the brake calipers and alerts the human inspector only when it encounters difficulties in identifying defects, specifically when the probability of a defect being present or absent falls below a certain threshold. This collaborative approach aims to combine the precision of ML algorithms with the experience of human inspectors, and can be seen as a possible transition scenario between the human-based and a fully-automated quality control operation.
Third Scenario (S3): Fully Automated Inspection. In the final scenario, we conceive a completely automated defect inspection station powered exclusively by our ML-based detection system. This setup eliminates the need for human intervention, relying entirely on the capabilities of the ML tools to identify defects.
For simplicity, we assume that all the stations are aligned in series without buffers, minimizing unnecessary complications in our estimations. To quantify the beneficial effects of implementing ML-based quality control, we adopt the Overall Equipment Effectiveness (OEE) as the primary metric for the analysis. OEE is a comprehensive measure derived from the product of three critical factors, as outlined by Nota et al. ( 2020 ): Availability (the ratio of operating time with respect to planned production time); Performance (the ratio of actual output with respect to the theoretical maximum output); and Quality (the ratio of the good units with respect to the total units produced). In this section, we will discuss the details of how we calculate each of these factors for the various scenarios.
To calculate Availability ( A ), we consider an 8-hour work shift ( \(t_{shift}\) ) with 30 minutes of breaks ( \(t_{break}\) ) during which we assume production stop (except for the fully automated scenario), and 30 minutes of scheduled downtime ( \(t_{sched}\) ) required for machine cleaning and startup procedures. For unscheduled downtime ( \(t_{unsched}\) ), primarily due to machine breakdowns, we assume an average breakdown probability ( \(\rho _{down}\) ) of 5% for each machine, with an average repair time of one hour per incident ( \(t_{down}\) ). Based on these assumptions, since the Availability represents the ratio of run time ( \(t_{run}\) ) to production time ( \(t_{pt}\) ), it can be calculated using the following formula:
with the unscheduled downtime being computed as follows:
where N is the number of machines in the production line and \(1-\left( 1-\rho _{down}\right) ^{N}\) represents the probability that at least one machine breaks during the work shift. For the sake of simplicity, the \(t_{down}\) is assumed constant regardless of the number of failures.
Table 2 presents the numerical values used to calculate Availability in the three scenarios. In the second scenario, we can observe that integrating the automated station leads to a decrease in the first factor of the OEE analysis, which can be attributed to the additional station for automated quality-control (and the related potential failure). This ultimately increases the estimation of the unscheduled downtime. In the third scenario, the detrimental effect of the additional station compensates the beneficial effect of the automated quality control on reducing the need for pauses during operator breaks; thus, the Availability for the third scenario yields as substantially equivalent to the first one (baseline).
The second factor of OEE, Performance ( P ), assesses the operational efficiency of production equipment relative to its maximum designed speed ( \(t_{line}\) ). This evaluation includes accounting for reductions in cycle speed and minor stoppages, collectively termed as speed losses . These losses are challenging to measure in advance, as performance is typically measured using historical data from the production line. For this analysis, we are constrained to hypothesize a reasonable estimate of 60 seconds of time lost to speed losses ( \(t_{losses}\) ) for each work cycle. Although this assumption may appear strong, it will become evident later that, within the context of this analysis – particularly regarding the impact of automated inspection on energy savings – the Performance (like the Availability) is only marginally influenced by introducing an automated inspection station. To account for the effect of automated inspection on the assembly line speed, we keep the time required by the other 13 stations ( \(t^*_{line}\) ) constant while varying the time allocated for visual inspection ( \(t_{inspect}\) ). According to Burduk and Górnicka ( 2017 ), the total operation time of the production line, excluding inspection, is 1263 seconds, with manual visual inspection taking 38 seconds. For the fully automated third scenario, we assume an inspection time of 5 seconds, which encloses the photo collection, pre-processing, ML-analysis, and post-processing steps. In the second scenario, instead, we add an additional time to the pure automatic case to consider the cases when the confidence of the ML model falls below 90%. We assume this happens once in every 10 inspections, which is a conservative estimate, higher than that we observed during model testing. This results in adding 10% of the human inspection time to the fully automated time. Thus, when \(t_{losses}\) are known, Performance can be expressed as follows:
The calculated values for Performance are presented in Table 3 , and we can note that the modification in inspection time has a negligible impact on this factor since it does not affect the speed loss or, at least to our knowledge, there is no clear evidence to suggest that the introduction of a new inspection station would alter these losses. Moreover, given the specific linear layout of the considered production line, the inspection time change has only a marginal effect on enhancing the production speed. However, this approach could potentially bias our scenario towards always favouring automation. To evaluate this hypothesis, a sensitivity analysis which explores scenarios where the production line operates at a faster pace will be discussed in the next subsection.
The last factor, Quality ( Q ), quantifies the ratio of compliant products out of the total products manufactured, effectively filtering out items that fail to meet the quality standards due to defects. Given the objective of our automated algorithm, we anticipate this factor of the OEE to be significantly enhanced by implementing the ML-based automated inspection station. To estimate it, we assume a constant defect probability for the production line ( \(\rho _{def}\) ) at 5%. Consequently, the number of defective products ( \(N_{def}\) ) during the work shift is calculated as \(N_{unit} \cdot \rho _{def}\) , where \(N_{unit}\) represents the average number of units (brake calipers) assembled on the production line, defined as:
To quantify defective units identified, we consider the inspection accuracy ( \(\rho _{acc}\) ), where for human visual inspection, the typical accuracy is 80% (Sundaram & Zeid, 2023 ), and for the ML-based station, we use the accuracy of our best model, i.e., 99%. Additionally, we account for the probability of the station mistakenly identifying a caliper as with a defect even if it is defect-free, i.e., the false negative rate ( \(\rho _{FN}\) ), defined as
In the absence of any reasonable evidence to justify a bias on one mistake over others, we assume a uniform distribution for both human and automated inspections regarding error preference, i.e. we set \(\rho ^{H}_{FN} = \rho ^{ML}_{FN} = \rho _{FN} = 50\%\) . Thus, the number of final compliant goods ( \(N_{goods}\) ), i.e., the calipers that are identified as quality-compliant, can be calculated as:
where \(N_{detect}\) is the total number of detected defective units, comprising TN (true negatives, i.e. correctly identified defective calipers) and FN (false negatives, i.e. calipers mistakenly identified as defect-free). The Quality factor can then be computed as:
Table 4 summarizes the Quality factor calculation, showcasing the substantial improvement brought by the ML-based inspection station due to its higher accuracy compared to human operators.
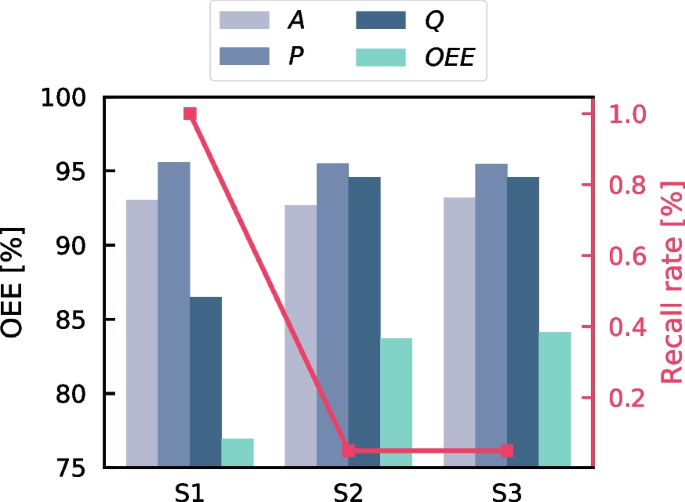
Overall Equipment Effectiveness (OEE) analysis for three scenarios (S1: Human-Based Inspection, S2: Hybrid Inspection, S3: Fully Automated Inspection). The height of the bars represents the percentage of the three factors A : Availability, P : Performance, and Q : Quality, which can be interpreted from the left axis. The green bars indicate the OEE value, derived from the product of these three factors. The red line shows the recall rate, i.e. the probability that a defective product is rejected by the client, with values displayed on the right red axis
Finally, we can determine the Overall Equipment Effectiveness by multiplying the three factors previously computed. Additionally, we can estimate the recall rate ( \(\rho _{R}\) ), which reflects the rate at which a customer might reject products. This is derived from the difference between the total number of defective units, \(N_{def}\) , and the number of units correctly identified as defective, TN , indicating the potential for defective brake calipers that may bypass the inspection process. In Fig. 8 we summarize the outcomes of the three scenarios. It is crucial to note that the scenarios incorporating the automated defect detector, S2 and S3, significantly enhance the Overall Equipment Effectiveness, primarily through substantial improvements in the Quality factor. Among these, the fully automated inspection scenario, S3, emerges as a slightly superior option, thanks to its additional benefit in removing the breaks and increasing the speed of the line. However, given the different assumptions required for this OEE study, we shall interpret these results as illustrative, and considering them primarily as comparative with the baseline scenario only. To analyze the sensitivity of the outlined scenarios on the adopted assumptions, we investigate the influence of the line speed and human accuracy on the results in the next subsection.
Sensitivity analysis
The scenarios described previously are illustrative and based on several simplifying hypotheses. One of such hypotheses is that the production chain layout operates entirely in series, with each station awaiting the arrival of the workpiece from the preceding station, resulting in a relatively slow production rate (1263 seconds). This setup can be quite different from reality, where slower operations can be accelerated by installing additional machines in parallel to balance the workload and enhance productivity. Moreover, we utilized a literature value of 80% for the accuracy of the human visual inspector operator, as reported by Sundaram and Zeid ( 2023 ). However, this accuracy can vary significantly due to factors such as the experience of the inspector and the defect type.
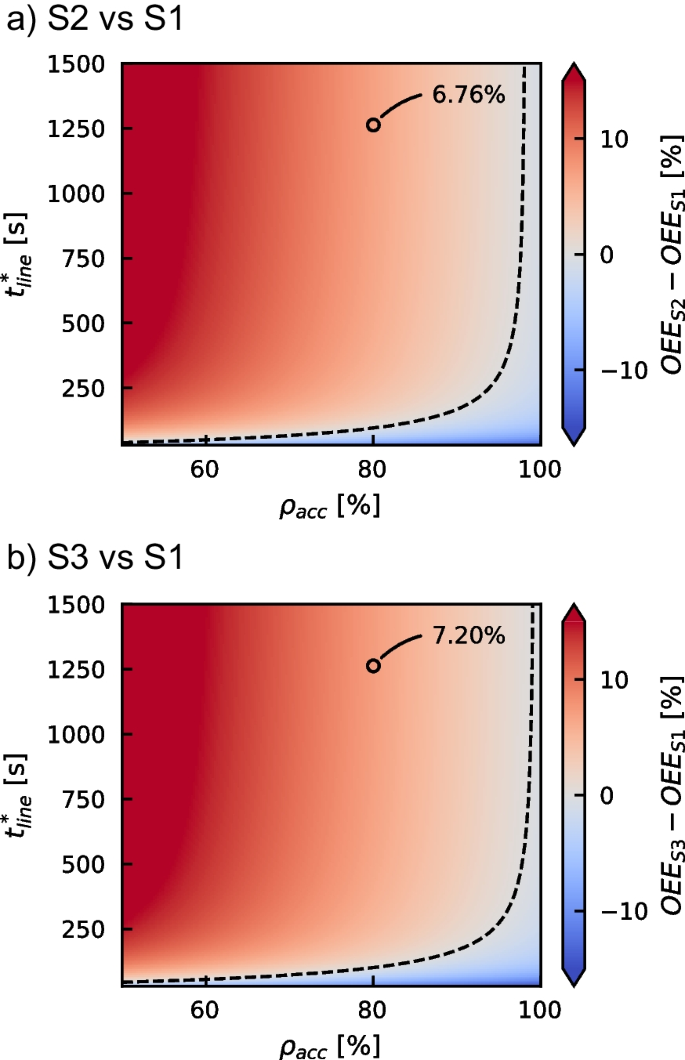
Effect of assembly time for stations (excluding visual inspection), \(t^*_{line}\) , and human inspection accuracy, \(\rho _{acc}\) , on the OEE analysis. (a) The subplot shows the difference between the scenario S2 (Hybrid Inspection) and the baseline scenario S1 (Human Inspection), while subplot (b) displays the difference between scenario S3 (Fully Automated Inspection) and the baseline. The maps indicate in red the values of \(t^*_{line}\) and \(\rho _{acc}\) where the integration of automated inspection stations can significantly improve OEE, and in blue where it may lower the score. The dashed lines denote the breakeven points, and the circled points pinpoint the values of the scenarios used in the “Illustrative scenario” Subsection.
A sensitivity analysis on these two factors was conducted to address these variations. The assembly time of the stations (excluding visual inspection), \(t^*_{line}\) , was varied from 60 s to 1500 s, and the human inspection accuracy, \(\rho _{acc}\) , ranged from 50% (akin to a random guesser) to 100% (representing an ideal visual inspector); meanwhile, the other variables were kept fixed.
The comparison of the OEE enhancement for the two scenarios employing ML-based inspection against the baseline scenario is displayed in the two maps in Fig. 9 . As the figure shows, due to the high accuracy and rapid response of the proposed automated inspection station, the area representing regions where the process may benefit energy savings in the assembly lines (indicated in red shades) is significantly larger than the areas where its introduction could degrade performance (indicated in blue shades). However, it can be also observed that the automated inspection could be superfluous or even detrimental in those scenarios where human accuracy and assembly speed are very high, indicating an already highly accurate workflow. In these cases, and particularly for very fast production lines, short times for quality control can be expected to be key (beyond accuracy) for the optimization.
Finally, it is important to remark that the blue region (areas below the dashed break-even lines) might expand if the accuracy of the neural networks for defect detection is lower when implemented in an real production line. This indicates the necessity for new rounds of active learning and an augment of the ratio of real images in the database, to eventually enhance the performance of the ML model.
Conclusions
Industrial quality control processes on manufactured parts are typically achieved by human visual inspection. This usually requires a dedicated handling system, and generally results in a slower production rate, with the associated non-optimal use of the energy resources. Based on a practical test case for quality control on brake caliper manufacturing, in this work we have reported on a developed workflow for integration of Machine Learning methods to automatize the process. The proposed approach relies on image analysis via Deep Convolutional Neural Networks. These models allow to efficiently extract information from images, thus possibly representing a valuable alternative to human inspection.
The proposed workflow relies on a two-step procedure on the images of the brake calipers: first, the background is removed from the image; second, the geometry is inspected to identify possible defects. These two steps are accomplished thanks to two dedicated neural network models, an encoder-decoder and an encoder network, respectively. Training of these neural networks typically requires a large number of representative images for the problem. Given that, one such database is not always readily available, we have presented and discussed an alternative methodology for the generation of the input database using 3D renderings. While integration of the database with real photographs was required for optimal results, this approach has allowed fast and flexible generation of a large base of representative images. The pre-processing steps required for data feeding to the neural networks and their training has been also discussed.
Several models have been tested and evaluated, and the best one for the considered case identified. The obtained accuracy for defect identification reaches \(\sim \) 99% of the tested cases. Moreover, the response of the models is fast (in the order of few seconds) on each image, which makes them compliant with the most typical industrial expectations.
In order to provide a practical example of possible energy savings when implementing the proposed ML-based methodology for quality control, we have analyzed three perspective industrial scenarios: a baseline scenario, where quality control tasks are performed by a human inspector; a hybrid scenario, where the proposed ML automatic detection tool assists the human inspector; a fully-automated scenario, where we envision a completely automated defect inspection. The results show that the proposed tools may help increasing the Overall Equipment Effectiveness up to \(\sim \) 10% with respect to the considered baseline scenario. However, a sensitivity analysis on the speed of the production line and on the accuracy of the human inspector has also shown that the automated inspection could be superfluous or even detrimental in those cases where human accuracy and assembly speed are very high. In these cases, reducing the time required for quality control can be expected to be the major controlling parameter (beyond accuracy) for optimization.
Overall the results show that, with a proper tuning, these models may represent a valuable resource for integration into production lines, with positive outcomes on the overall effectiveness, and thus ultimately leading to a better use of the energy resources. To this, while the practical implementation of the proposed tools can be expected to require contained investments (e.g. a portable camera, a dedicated workstation and an operator with proper training), in field tests on a real industrial line would be required to confirm the potential of the proposed technology.
Agrawal, R., Majumdar, A., Kumar, A., & Luthra, S. (2023). Integration of artificial intelligence in sustainable manufacturing: Current status and future opportunities. Operations Management Research, 1–22.
Alzubaidi, L., Zhang, J., Humaidi, A. J., Al-Dujaili, A., Duan, Y., Al-Shamma, O., Santamaría, J., Fadhel, M. A., Al-Amidie, M., & Farhan, L. (2021). Review of deep learning: Concepts, cnn architectures, challenges, applications, future directions. Journal of big Data, 8 , 1–74.
Article Google Scholar
Angelopoulos, A., Michailidis, E. T., Nomikos, N., Trakadas, P., Hatziefremidis, A., Voliotis, S., & Zahariadis, T. (2019). Tackling faults in the industry 4.0 era-a survey of machine—learning solutions and key aspects. Sensors, 20 (1), 109.
Arana-Landín, G., Uriarte-Gallastegi, N., Landeta-Manzano, B., & Laskurain-Iturbe, I. (2023). The contribution of lean management—industry 4.0 technologies to improving energy efficiency. Energies, 16 (5), 2124.
Badmos, O., Kopp, A., Bernthaler, T., & Schneider, G. (2020). Image-based defect detection in lithium-ion battery electrode using convolutional neural networks. Journal of Intelligent Manufacturing, 31 , 885–897. https://doi.org/10.1007/s10845-019-01484-x
Banko, M., & Brill, E. (2001). Scaling to very very large corpora for natural language disambiguation. In Proceedings of the 39th annual meeting of the association for computational linguistics (pp. 26–33).
Benedetti, M., Bonfà, F., Introna, V., Santolamazza, A., & Ubertini, S. (2019). Real time energy performance control for industrial compressed air systems: Methodology and applications. Energies, 12 (20), 3935.
Bhatt, D., Patel, C., Talsania, H., Patel, J., Vaghela, R., Pandya, S., Modi, K., & Ghayvat, H. (2021). Cnn variants for computer vision: History, architecture, application, challenges and future scope. Electronics, 10 (20), 2470.
Bilgen, S. (2014). Structure and environmental impact of global energy consumption. Renewable and Sustainable Energy Reviews, 38 , 890–902.
Blender. (2023). Open-source software. https://www.blender.org/ . Accessed 18 Apr 2023.
Bologna, A., Fasano, M., Bergamasco, L., Morciano, M., Bersani, F., Asinari, P., Meucci, L., & Chiavazzo, E. (2020). Techno-economic analysis of a solar thermal plant for large-scale water pasteurization. Applied Sciences, 10 (14), 4771.
Burduk, A., & Górnicka, D. (2017). Reduction of waste through reorganization of the component shipment logistics. Research in Logistics & Production, 7 (2), 77–90. https://doi.org/10.21008/j.2083-4950.2017.7.2.2
Carvalho, T. P., Soares, F. A., Vita, R., Francisco, R., d. P., Basto, J. P., & Alcalá, S. G. (2019). A systematic literature review of machine learning methods applied to predictive maintenance. Computers & Industrial Engineering, 137 , 106024.
Casini, M., De Angelis, P., Chiavazzo, E., & Bergamasco, L. (2024). Current trends on the use of deep learning methods for image analysis in energy applications. Energy and AI, 15 , 100330. https://doi.org/10.1016/j.egyai.2023.100330
Chai, J., Zeng, H., Li, A., & Ngai, E. W. (2021). Deep learning in computer vision: A critical review of emerging techniques and application scenarios. Machine Learning with Applications, 6 , 100134.
Chen, L. C., Zhu, Y., Papandreou, G., Schroff, F., Adam, H. (2018). Encoder-decoder with atrous separable convolution for semantic image segmentation. In Proceedings of the European conference on computer vision (ECCV) (pp. 801–818).
Chen, L., Li, S., Bai, Q., Yang, J., Jiang, S., & Miao, Y. (2021). Review of image classification algorithms based on convolutional neural networks. Remote Sensing, 13 (22), 4712.
Chen, T., Sampath, V., May, M. C., Shan, S., Jorg, O. J., Aguilar Martín, J. J., Stamer, F., Fantoni, G., Tosello, G., & Calaon, M. (2023). Machine learning in manufacturing towards industry 4.0: From ‘for now’to ‘four-know’. Applied Sciences, 13 (3), 1903. https://doi.org/10.3390/app13031903
Choudhury, A. (2021). The role of machine learning algorithms in materials science: A state of art review on industry 4.0. Archives of Computational Methods in Engineering, 28 (5), 3361–3381. https://doi.org/10.1007/s11831-020-09503-4
Dalzochio, J., Kunst, R., Pignaton, E., Binotto, A., Sanyal, S., Favilla, J., & Barbosa, J. (2020). Machine learning and reasoning for predictive maintenance in industry 4.0: Current status and challenges. Computers in Industry, 123 , 103298.
Fasano, M., Bergamasco, L., Lombardo, A., Zanini, M., Chiavazzo, E., & Asinari, P. (2019). Water/ethanol and 13x zeolite pairs for long-term thermal energy storage at ambient pressure. Frontiers in Energy Research, 7 , 148.
Géron, A. (2022). Hands-on machine learning with Scikit-Learn, Keras, and TensorFlow . O’Reilly Media, Inc.
GrabCAD. (2023). Brake caliper 3D model by Mitulkumar Sakariya from the GrabCAD free library (non-commercial public use). https://grabcad.com/library/brake-caliper-19 . Accessed 18 Apr 2023.
He, K., Zhang, X., Ren, S., & Sun, J. (2016). Deep residual learning for image recognition. In Proceedings of the IEEE conference on computer vision and pattern recognition (pp. 770–778).
Ho, S., Zhang, W., Young, W., Buchholz, M., Al Jufout, S., Dajani, K., Bian, L., & Mozumdar, M. (2021). Dlam: Deep learning based real-time porosity prediction for additive manufacturing using thermal images of the melt pool. IEEE Access, 9 , 115100–115114. https://doi.org/10.1109/ACCESS.2021.3105362
Ismail, M. I., Yunus, N. A., & Hashim, H. (2021). Integration of solar heating systems for low-temperature heat demand in food processing industry-a review. Renewable and Sustainable Energy Reviews, 147 , 111192.
LeCun, Y., Bengio, Y., & Hinton, G. (2015). Deep learning. Nature, 521 (7553), 436–444.
Leong, W. D., Teng, S. Y., How, B. S., Ngan, S. L., Abd Rahman, A., Tan, C. P., Ponnambalam, S., & Lam, H. L. (2020). Enhancing the adaptability: Lean and green strategy towards the industry revolution 4.0. Journal of cleaner production, 273 , 122870.
Liu, Z., Wang, X., Zhang, Q., & Huang, C. (2019). Empirical mode decomposition based hybrid ensemble model for electrical energy consumption forecasting of the cement grinding process. Measurement, 138 , 314–324.
Li, G., & Zheng, X. (2016). Thermal energy storage system integration forms for a sustainable future. Renewable and Sustainable Energy Reviews, 62 , 736–757.
Maggiore, S., Realini, A., Zagano, C., & Bazzocchi, F. (2021). Energy efficiency in industry 4.0: Assessing the potential of industry 4.0 to achieve 2030 decarbonisation targets. International Journal of Energy Production and Management, 6 (4), 371–381.
Mazzei, D., & Ramjattan, R. (2022). Machine learning for industry 4.0: A systematic review using deep learning-based topic modelling. Sensors, 22 (22), 8641.
Md, A. Q., Jha, K., Haneef, S., Sivaraman, A. K., & Tee, K. F. (2022). A review on data-driven quality prediction in the production process with machine learning for industry 4.0. Processes, 10 (10), 1966. https://doi.org/10.3390/pr10101966
Minaee, S., Boykov, Y., Porikli, F., Plaza, A., Kehtarnavaz, N., & Terzopoulos, D. (2021). Image segmentation using deep learning: A survey. IEEE transactions on pattern analysis and machine intelligence, 44 (7), 3523–3542.
Google Scholar
Mishra, S., Srivastava, R., Muhammad, A., Amit, A., Chiavazzo, E., Fasano, M., & Asinari, P. (2023). The impact of physicochemical features of carbon electrodes on the capacitive performance of supercapacitors: a machine learning approach. Scientific Reports, 13 (1), 6494. https://doi.org/10.1038/s41598-023-33524-1
Mumuni, A., & Mumuni, F. (2022). Data augmentation: A comprehensive survey of modern approaches. Array, 16 , 100258. https://doi.org/10.1016/j.array.2022.100258
Mypati, O., Mukherjee, A., Mishra, D., Pal, S. K., Chakrabarti, P. P., & Pal, A. (2023). A critical review on applications of artificial intelligence in manufacturing. Artificial Intelligence Review, 56 (Suppl 1), 661–768.
Narciso, D. A., & Martins, F. (2020). Application of machine learning tools for energy efficiency in industry: A review. Energy Reports, 6 , 1181–1199.
Nota, G., Nota, F. D., Peluso, D., & Toro Lazo, A. (2020). Energy efficiency in industry 4.0: The case of batch production processes. Sustainability, 12 (16), 6631. https://doi.org/10.3390/su12166631
Ocampo-Martinez, C., et al. (2019). Energy efficiency in discrete-manufacturing systems: Insights, trends, and control strategies. Journal of Manufacturing Systems, 52 , 131–145.
Pan, Y., Hao, L., He, J., Ding, K., Yu, Q., & Wang, Y. (2024). Deep convolutional neural network based on self-distillation for tool wear recognition. Engineering Applications of Artificial Intelligence, 132 , 107851.
Qin, J., Liu, Y., Grosvenor, R., Lacan, F., & Jiang, Z. (2020). Deep learning-driven particle swarm optimisation for additive manufacturing energy optimisation. Journal of Cleaner Production, 245 , 118702.
Rahul, M., & Chiddarwar, S. S. (2023). Integrating virtual twin and deep neural networks for efficient and energy-aware robotic deburring in industry 4.0. International Journal of Precision Engineering and Manufacturing, 24 (9), 1517–1534.
Ribezzo, A., Falciani, G., Bergamasco, L., Fasano, M., & Chiavazzo, E. (2022). An overview on the use of additives and preparation procedure in phase change materials for thermal energy storage with a focus on long term applications. Journal of Energy Storage, 53 , 105140.
Shahin, M., Chen, F. F., Hosseinzadeh, A., Bouzary, H., & Shahin, A. (2023). Waste reduction via image classification algorithms: Beyond the human eye with an ai-based vision. International Journal of Production Research, 1–19.
Shen, F., Zhao, L., Du, W., Zhong, W., & Qian, F. (2020). Large-scale industrial energy systems optimization under uncertainty: A data-driven robust optimization approach. Applied Energy, 259 , 114199.
Simonyan, K., & Zisserman, A. (2014). Very deep convolutional networks for large-scale image recognition. arXiv preprint arXiv:1409.1556 .
Sundaram, S., & Zeid, A. (2023). Artificial Intelligence-Based Smart Quality Inspection for Manufacturing. Micromachines, 14 (3), 570. https://doi.org/10.3390/mi14030570
Szegedy, C., Ioffe, S., Vanhoucke, V., & Alemi, A. (2017). Inception-v4, inception-resnet and the impact of residual connections on learning. In Proceedings of the AAAI conference on artificial intelligence (vol. 31).
Szegedy, C., Liu, W., Jia, Y., Sermanet, P., Reed, S., Anguelov, D., Erhan, D., Vanhoucke, V., & Rabinovich, A. (2015). Going deeper with convolutions. In Proceedings of the IEEE conference on computer vision and pattern recognition (pp. 1–9).
Trezza, G., Bergamasco, L., Fasano, M., & Chiavazzo, E. (2022). Minimal crystallographic descriptors of sorption properties in hypothetical mofs and role in sequential learning optimization. npj Computational Materials, 8 (1), 123. https://doi.org/10.1038/s41524-022-00806-7
Vater, J., Schamberger, P., Knoll, A., & Winkle, D. (2019). Fault classification and correction based on convolutional neural networks exemplified by laser welding of hairpin windings. In 2019 9th International Electric Drives Production Conference (EDPC) (pp. 1–8). IEEE.
Wen, L., Li, X., Gao, L., & Zhang, Y. (2017). A new convolutional neural network-based data-driven fault diagnosis method. IEEE Transactions on Industrial Electronics, 65 (7), 5990–5998. https://doi.org/10.1109/TIE.2017.2774777
Willenbacher, M., Scholten, J., & Wohlgemuth, V. (2021). Machine learning for optimization of energy and plastic consumption in the production of thermoplastic parts in sme. Sustainability, 13 (12), 6800.
Zhang, X. H., Zhu, Q. X., He, Y. L., & Xu, Y. (2018). Energy modeling using an effective latent variable based functional link learning machine. Energy, 162 , 883–891.
Download references
Acknowledgements
This work has been supported by GEFIT S.p.a.
Open access funding provided by Politecnico di Torino within the CRUI-CARE Agreement.
Author information
Authors and affiliations.
Department of Energy, Politecnico di Torino, Turin, Italy
Mattia Casini, Paolo De Angelis, Paolo Vigo, Matteo Fasano, Eliodoro Chiavazzo & Luca Bergamasco
R &D Department, GEFIT S.p.a., Alessandria, Italy
Marco Porrati
You can also search for this author in PubMed Google Scholar
Corresponding author
Correspondence to Luca Bergamasco .
Ethics declarations
Conflict of interest statement.
The authors declare no competing interests.
Additional information
Publisher's note.
Springer Nature remains neutral with regard to jurisdictional claims in published maps and institutional affiliations.
Supplementary Information
Below is the link to the electronic supplementary material.
Supplementary file 1 (pdf 354 KB)
Rights and permissions.
Open Access This article is licensed under a Creative Commons Attribution 4.0 International License, which permits use, sharing, adaptation, distribution and reproduction in any medium or format, as long as you give appropriate credit to the original author(s) and the source, provide a link to the Creative Commons licence, and indicate if changes were made. The images or other third party material in this article are included in the article’s Creative Commons licence, unless indicated otherwise in a credit line to the material. If material is not included in the article’s Creative Commons licence and your intended use is not permitted by statutory regulation or exceeds the permitted use, you will need to obtain permission directly from the copyright holder. To view a copy of this licence, visit http://creativecommons.org/licenses/by/4.0/ .
Reprints and permissions
About this article
Casini, M., De Angelis, P., Porrati, M. et al. Machine Learning and image analysis towards improved energy management in Industry 4.0: a practical case study on quality control. Energy Efficiency 17 , 48 (2024). https://doi.org/10.1007/s12053-024-10228-7
Download citation
Received : 22 July 2023
Accepted : 28 April 2024
Published : 13 May 2024
DOI : https://doi.org/10.1007/s12053-024-10228-7
Share this article
Anyone you share the following link with will be able to read this content:
Sorry, a shareable link is not currently available for this article.
Provided by the Springer Nature SharedIt content-sharing initiative
- Industry 4.0
- Energy management
- Artificial intelligence
- Machine learning
- Deep learning
- Convolutional neural networks
- Computer vision
- Find a journal
- Publish with us
- Track your research
- Cloud Infrastructure
VMware Cloud Foundation
Scalable, elastic private cloud IaaS solution.
Key Technologies:
vSphere | vSAN | NSX | Aria
VMware vSphere Foundation
Enterprise workload engine with intelligent operations.
vSphere | Aria
Live Recovery Private AI Foundation
- Anywhere Workspace
Access any app on any device securely.
- Workspace ONE
App Platforms
Build, deploy, manage and scale modern apps.
- VMware Tanzu
Security and Load Balancing
Zero trust lateral security and software-defined app delivery.
- VMware Avi Load Balancer
- VMware vDefend Distributed Firewall
- VMware vDefend Advanced Threat Prevention
Software-Defined Edge
Empower distributed workloads with infrastructure and management.
- Edge Compute Stack
- VeloCloud SD-WAN
- Telco Cloud
Run VMware on any Cloud. Any Environment. Anywhere.
On public & hybrid clouds.
- Alibaba Cloud VMware Service
- Azure VMware Solution
- Google Cloud VMware Engine
- IBM Cloud for VMware Solutions
- Oracle Cloud VMware Solutions
- VMware Cloud on AWS
- VMware Verified Cloud Providers
Desktop Hypervisor
Develop and test in a local virtualization sandbox.
- Fusion for Mac
- Workstation Player
- Workstation Pro
By Category
- App Platform
By Industry
- Communications Service Providers
- Federal Government
- Financial Services
- Healthcare Providers
- Manufacturing
- State and Local Government
VMware AI Solutions
Accelerate and ensure the success of your generative AI initiatives with multi-cloud flexibility, choice, privacy and control.
For Customers
- Find a Cloud Provider
- Find a Partner
- VMware Marketplace
- Work with a Partner
For Partners
- Become a Cloud Provider
- Cloud Partner Navigator
- Get Cloud Verified
- Learning and Selling Resources
- Partner Connect Login
- Partner Executive Edge
- Technology Partner Hub
- Work with VMware
Working Together with Partners for Customer Success
A new, simplified partner program to help achieve even greater opportunities for profitability.
Tools & Training
- VMware Customer Connect
- VMware Trust Center
- Learning & Certification
- Product Downloads
- Cloud Services Engagement Platform
- Hands-on Labs
- Professional Services
- Support Offerings
- Support Customer Welcome Center
Marketplace
- Cloud Marketplace
- VMware Video Library
- VMware Explore Video Library
Blogs & Communities
- News & Stories
- Communities
- Customer Stories
- VMware Explore
- All Events & Webcasts
- Products
VMware Aria Operations

Proactive IT Operations Management VMware Aria Operations
Enable IT operations management for your private cloud environment with a unified, high-performance VMware Cloud Foundation platform.
VMware Aria Operations is no longer sold as a standalone product. Capabilities of this product are now available as a part of VMware Cloud Foundation and VMware vSphere Foundation .
Case Studies
Boost efficiency with proactive ops management.

Maximize ROI for Cloud Operations
Minimize unplanned downtime, reduce issue-resolution workloads, and capture savings when you deploy VMware Aria in your hybrid or multi-cloud environment.

Accelerate the Journey to Cloud
Simplify your hybrid cloud management, from migration assessment and planning to operationalizing the hybrid cloud in production.

Unify Visibility Across Clouds
Automate and streamline IT management with full-stack visibility from physical, virtual and cloud infrastructure, including virtual machines (VMs) and containers , to the apps they support.

Increase Operational Efficiency
Gain proactive planning and intelligent remediation to predict, prevent and troubleshoot faster with actionable insights. Monitor heterogeneous environments and make ML-powered management decisions.

Recognized Leader in AI-Operations
Read about the latest accolade received from Enterprise Management Associates (EMA), a leading IT analyst research firm that provides deep insight across the full spectrum of IT management technologies.

Whats New in Aria Operations?
Explore the latest in VMware Aria Operations (formerly vRealize Operations and vRealize Operations Cloud).
VMware Aria Operations by the Numbers
Reduction in issue resolution time
Reduction in relative downtime
Reduction in last-minute hardware costs
VMware Aria Operations Capabilities
Continuous performance optimization.
Assure hybrid cloud performance at minimal cost. Real-time predictive analytics and AI automatically balance workloads and avoid contention.
Efficient Capacity and Cost Management
Using a forward-looking analytics engine, VMware Aria Operations predicts future demand, provides recommendations, and automates reclamation and rightsizing.
Integrated Compliance
Reduce risk and enforce regulatory standards with integrated compliance. Ensure your environments adherence to common requirements or create your own templates.

VMware [Aria Operations] works smoothly, without my influence. Im saving up to 10 hours a month on upgrades and troubleshooting. Stephan Wiechert, IT System Specialist

We saved about $1.5M with [VMware Aria Operations] helping us identify old hardware, appliances and storage thats not being used. Emilio Salguera, Principal Technical Architect

The work we've done with VMware will serve as a model for other states looking to consolidate and streamline their IT operations while improving security. Michael Allison, CTO
Learn, Evaluate, Implement
Explore technical documentation, reports, trial, communities and more.
View common question and answers about Aria Operations.

Getting started is only a few steps away!
*Required Fields
A little about you and your business will help us provide a personalized experience.
Were almost there a few more details and youre done., thank you for your interest in vmware aria operations.
A member of our team will be in touch shortly.
Ready to Get Started?
- vRealize Operations (vROps) for Horizon | VDI Monitoring
- Extend VMware Aria Operations VMware Aria Operations for Integrations
- Proactive IT Operations Management VMware Aria Operations FAQ
Case Study | DILFO streamlines document management to achieve time savings
With ProjectSight, DILFO has improved project operations with faster communication and improved visibility across construction and service teams.
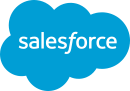
Salesforce is closed for new business in your area.
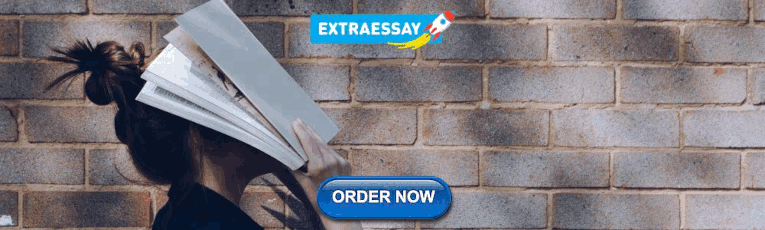
IMAGES
VIDEO
COMMENTS
This article reviews the case study research in the operations management field. In this regard, the paper's key objective is to represent a general framework to design, develop, and conduct case study research for a future operations management research by critically reviewing relevant literature and offering insights into the use of case method in particular settings.
Operations Management. Browse operations management learning materials including case studies, simulations, and online courses. Introduce core concepts and real-world challenges to create memorable learning experiences for your students.
by Rachel Layne. Many companies build their businesses on open source software, code that would cost firms $8.8 trillion to create from scratch if it weren't freely available. Research by Frank Nagle and colleagues puts a value on an economic necessity that will require investment to meet demand. 27 Feb 2024.
Master of Science in Management Studies. Combine an international MBA with a deep dive into management science. A special opportunity for partner and affiliate schools only. ... Operations Management Case Studies. Teaching Resources Library A Background Note on "Unskilled" Jobs in the United States - Past, Present, and Future
MIT Sloan Case. MIT Sloan School of Management. Case: 11-116, January 3, 2012. 7 Inventory I: EOQ & cycle stocks Reading [MSD] Chapter 7. 8 Supply chain strategy + HP DeskJet case Case. Kopczak, Laura Rock, and Hau Lee. "Hewlett-Packard Co.: DeskJet Printer Supply Chain (A)." Stanford Graduate School of Business Case. Case: GS-3A, March 8 ...
A few years ago, I wrote an editorial article like this on case studies in operations management (Childe Citation 2011), looking briefly at what can be learned from cases and encouraging researchers to publish cases in this Journal.That article proved to be surprisingly popular and after five years, it seems worthwhile revisiting the subject.
Abstract. This paper reviews the use of case study research in operations management for theory development and testing. It draws on the literature on case research in a number of disciplines and uses examples drawn from operations management research. It provides guidelines and a roadmap for operations management researchers wishing to design ...
In areas related to operations management, such as com-puter science, the term 'case study' is often used to refer to the performance of a system 'under' certain conditions. This can be the understanding in the context of simulation or optimisation, 2016 informa uK limited, trading as taylor & Francis group.
This textbook is comprised of detailed case studies covering challenging real world applications of OR techniques. Among the overall goals of the book is to provide readers with descriptions of the history and other background information on a variety of industries, service or other organizations in which decision making is an important component of their daily operations.
This article reviews the case study research in the operations management field. In this regard, the paper's key objective is to. represent a general framework to design, develop, and conduct ...
Technology & Operations Case Study. ... Jaime Giancola, an MBA student, has recently completed an operations management course in which aggregate production planning (APP) was one of the topics
The 10 Strategic Decision Areas of Operations Management at Walmart. 1. Design of Goods and Services. This decision area of operations management involves the strategic characterization of the retail company's products. In this case, the decision area covers Walmart's goods and services. As a retailer, the company offers retail services.
The answer seems to lie in the aim of the research. The widely-used paper by Voss et al. (2002) looks at case research and identifies four broad categories of research purpose - exploration, theory-building, theory-testing and theory extension/refinement. One of the interesting aspects of working with industry is that it is sometimes possible ...
by Ryan W. Buell, Kamalini Ramdas, and Nazlı Sönmez. Shared service delivery means that customers are served in groups rather than individually. Results from a large-scale study of glaucoma follow-up appointments at a major eye hospital indicate that shared service delivery can significantly improve patients' verbal and non-verbal engagement.
CASE STUDY 24 Uber Technologies, Inc. 24 VIDEO CASE STUDIES 24 Frito-Lay: Operations Management in Manufacturing 24 Hard Rock Cafe: Operations Management in Services 25 Celebrity Cruises: Operations Management at Sea 26 Endnotes 26 Bibliography 26 Chapter 1 Rapid Review 27 Self Test 28 Chapter 2 Operations Strategy in a Global Environment 29
Operations Management. A primary challenge for governments and organizations is to manage their resources as efficiently as possible. The teaching cases in this section challenge students to become decisive managers through a host of topics including budgeting and finance, infrastructure, regulatory policy, and transportation. Sort By: 1. 2. 3.
Fifty four percent of raw case users came from outside the U.S.. The Yale School of Management (SOM) case study directory pages received over 160K page views from 177 countries with approximately a third originating in India followed by the U.S. and the Philippines. Twenty-six of the cases in the list are raw cases.
This article reviews the case study research in the operations management field. In this regard, the paper's key objective is to represent a general framework to design, develop, and conduct case study research for a future operations management research by critically reviewing relevant literature and offering insights into the use of case method in particular settings.
Representing a broad range of management subjects, the ICMR Case Collection provides teachers, corporate trainers, and management professionals with a variety of teaching and reference material. The collection consists of Operations case studies and research reports on a wide range of companies and industries - both Indian and international, cases won awards in varies competitions, EFMD Case ...
The case is designed to be used in courses on Nonprofit Operations Management, Data Analytics, Six Sigma, and Business Process Excellence/Improvement in MBA or Executive MBA programs. It is suitable for teaching students about the common problem of lower rates of volunteerism in nonprofit organizations. Further, the case study helps present the ...
Download Free PDF. Journal of Business Case Studies - Third Quarter 2007 Volume 3, Number3 Case Study In Operations Management Victoria L. Figiel, (E-mail: [email protected]), Troy University James M. Whitlock, (E-mail: [email protected]), Troy University ABSTRACT This case study is conducted within the context of the Theory of Constraints.
Production and Operations Management Case Studies. Case 1: Product Development Risks. You have the opportunity to invest INR 100 billion for your company to develop a jet engine for commercial aircrafts. Development will span 5 years. The final product costing Rs. 500 million / unit could reach a sales potential, eventually of Rs. 2500 billion.
QUESTION 1 (20 Marks) 1.1. By scrutinising the key findings of the consultant's report through the lens of Wheelwright and Hayes' (1984) four-stage model of operations strategy, identify andcritically discuss the current stage of MBHE's operations strategy, and delineate actionable steps for advancing to the next stage. (10 marks) 1.2.
With the advent of Industry 4.0, Artificial Intelligence (AI) has created a favorable environment for the digitalization of manufacturing and processing, helping industries to automate and optimize operations. In this work, we focus on a practical case study of a brake caliper quality control operation, which is usually accomplished by human inspection and requires a dedicated handling system ...
VMware Aria Operations. Enable IT operations management for your private cloud environment with a unified, high-performance VMware Cloud Foundation platform. VMware Aria Operations is no longer sold as a standalone product. Capabilities of this product are now available as a part of VMware Cloud Foundation and VMware vSphere Foundation.
Case Study | DILFO streamlines document management to achieve time savings Published Date May 9, 2024 With ProjectSight, DILFO has improved project operations with faster communication and improved visibility across construction and service teams.
business document from universiti teknologi mara, 7 pages, michelle anak gilbert 2023536487 universiti teknologi mara (uitm) sarawak branch faculty of business and management bachelor in office systems management (hons.) (ba232) administrative operations system (asm553) case study (individual assignment) prepared
Elevate your field service operations with our best-in-class scheduling and optimization engine. Built on the Hyperforce platform, Enhanced Scheduling and Optimization automates scheduling while aligning with priorities and constraints. It ensures efficient resource allocation, minimizes travel time, and complies with service-level agreements.