Thank you for visiting nature.com. You are using a browser version with limited support for CSS. To obtain the best experience, we recommend you use a more up to date browser (or turn off compatibility mode in Internet Explorer). In the meantime, to ensure continued support, we are displaying the site without styles and JavaScript.
- View all journals
- ADVERTISEMENT FEATURE Advertiser retains sole responsibility for the content of this article
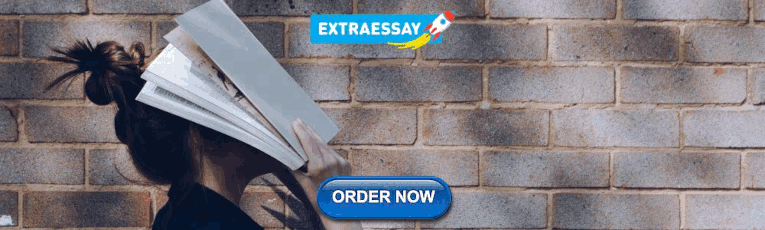
Innovations in digital twin research
Produced by
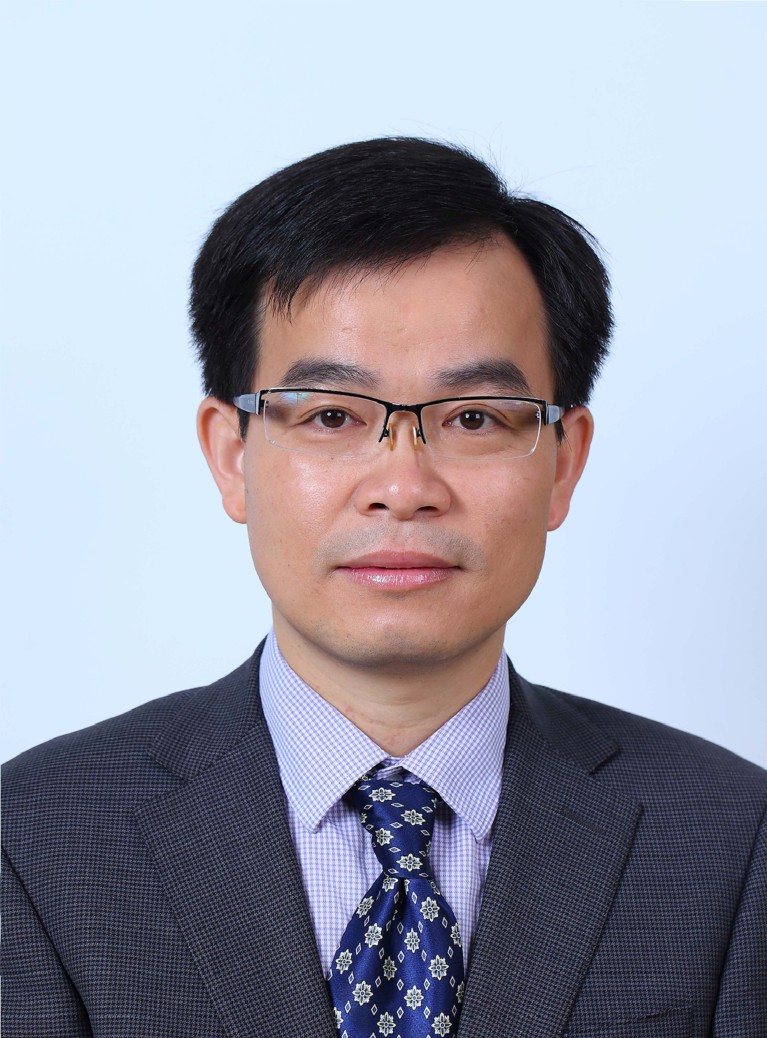
Fei Tao, Vice Dean of the Institute of Science and Technology, Beihang University Credit: Beihang University
Digital twins, comprising five dimensions including physical entities, virtual models, data, services, and connections, can be used to improve aspects of production, operations and business processes. Here, Fei Tao, discusses the Digital Twin Project, a collaboration between Beihang University and the First Research Institute of China Aerospace Science and Technology Corporation.
Can you tell us a little about the digital twin research field?
Digital twin is an interdisciplinary research field which includes engineering, computer science, automation and control, and so on. But due to the multidisciplinary nature of the field, it also touches on materials science, communication, operations management, robotics, medicine and other disciplines. A keyword analysis indicates that digital twin, ‘smart manufacturing’, ‘big data’, ‘cyber-physical system’, and ‘digital economy’ are closely related fields.
Digital twin publications have been produced by researchers from more than 80 countries. And according to a literature review conducted using Scopus, the field started a period of rapid growth around 2016, with the number of digital twin publications doubling every year thereafter. Due to its wide range of applications, we expect that digital twins will attract more scholars to conduct more in-depth research in the future.
How can digital twins be used by industry?
Based on more refined and dynamic models as well as richer data drives, digital twins have played an important role in operation monitoring, simulation, optimization, predictive maintenance, and supply chain optimization. Take the digital twin shop-floor, proposed in 2016 by our group, as a specific example. It can improve the efficiency of operation, control, and management by achieving a deep understanding, correct reasoning and precise operation of objects in the physical space and digital space of a shop-floor.
Now, more and more companies are using digital twins to improve their efficiency–Siemens, ANSYS, Dassault, Airbus, Lockheed Martin, and GE, for example. And according to a survey published by Gartner in 2019, 75% of organizations involved in IoT projects were either already using digital twins or planning to within a year.
The future development prospects of digital twins are broad. They have been applied to 15 fields including aerospace, smart manufacturing, smart cities, construction, medical, robotics, ships, automobiles, rail transit, industrial engineering, agriculture, mining, energy, and the environment. Indeed, a report released by MarketsandMarkets predicts that by 2026, the digital twin market will reach US$ 48.2 billion.
What are the scientific challenges of digital twins?
The Beihang Digital Twin Research Group proposed a digital twin five-dimensional model in 2018, the dimensions being: physical entities, virtual models, data, connection and interaction, and service/ application. At present, the digital twin five-dimensional model has been applied in ten fields including manufacturing, satellites, automobiles, ships, aircraft, smart cities, etc. by ten group enterprises.
The most notable challenges in the physical entities dimension include realizing the intelligent perception and interconnection of multi-source heterogeneous physical entities; and obtaining multi-dimensional data of physical entities in real time.
Challenges in the virtual model dimension include building a dynamic multi-dimensional, multi-space-time scale high- fidelity model; ensuring and verifying the consistency, validity, and reliability of the model; and realizing the assembly and integration of a multi-source, multi-discipline and multi- dimensional model.
Realizing both the real-time collection and efficient transmission of multi-source heterogeneous data and the deep integration and comprehensive processing of cyber-physical data are the main problems in the data dimension. Whereas cross-protocol/cross-interface/cross-platform real-time interactions and realizing the iterative interaction and dynamic evolution of data-model-application are challenges being investigated in the connection and interaction dimension.
The most challenging problems in the service/ application dimension are providing services that meet the needs of different fields, different levels of users, and different business applications based on multi-dimensional models and data, and realizing the value-added efficiency of the on-demand use of services.
For those interested in the practical bottlenecks in the field, in a comment published in Nature in 2019 we discussed how to facilitate the collaboration of experts in different fields and disciplines, and how to combine industry, universities, and researchers to encourage innovation.
How can digital twin research and applications be promoted?
Mostly through dedicated journals and international conferences. In 2017, myself and scholars from 12 universities initiated a yearly conference on digital twin and smart manufacturing service, which is the first of its kind. The conference, which has been held four times, gathers world- renowned scholars to build a high-level international exchange platform and lead the innovation and development of science, technology and engineering in digital twins. The 5th event is scheduled for July 16-18, 2021, in Shanghai. In April 2021, we also launched an international, comprehensive journal called Digital Twin with Taylor & Francis, which will cover theoretical, technological and application innovations of digital twins.
Digitization Solutions
Founded in 2001, Beijing Aero-Top Hi-Tech Co Ltd. is committed to high-end intelligent manufacturing and system integration, and can provide first-class products and system integration solutions in the energy digitization and factory digitization fields.
Digital twin energy capabilities
• Energy operation and dispatch management, energy transmission and distribution dispatch management
• Energy emergency maintenance scheduling management, energy emergency command management
• Energy pipeline network industrial interconnection, energy pipeline network monitoring and control
• Energy pipeline network twin information, energy pipeline network inspection and security management
• Energy pipeline network simulation and prediction, energy life cycle management
Digital twin factory capabilities
• Factory industrial interconnection, factory data collection
• Factory centralized monitoring, factory intelligent scheduling
• Factory twin modelling, factory production visualization
• Factory big data, factory data mining
• Factory process self-driven engine, factory equipment AI technology
• Factory scene simulation, smart stand-alone
• Factory process coordination, paperless operation
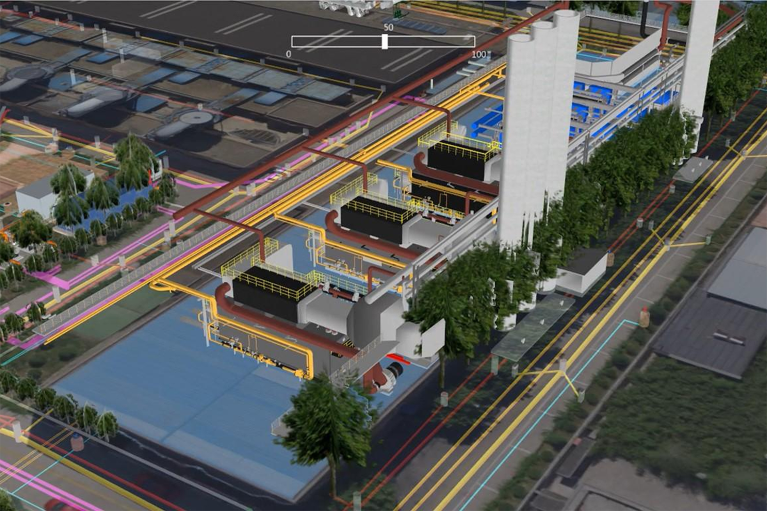
Credit: Beijing Aero-Top Hi-Tech Co Ltd
The digital twin gas pipeline network regulation and control system provides digital support for production and operation by performing data collection, display, storage, release and intelligent analysis at each site of the urban pipeline network. The system integrates various subsystems such as production dispatching command system, pipeline integrity, hazard source prevention and control, and emergency command. It digitizes pipeline production management, pipeline information management, pipeline safety management, pipeline risk management, daily production and operation business processing, learning and exchange training platform. A real-time, safe, complete and efficient closed-loop management of the gas pipeline network is formed to enhance operation efficiency and reduce operating costs.
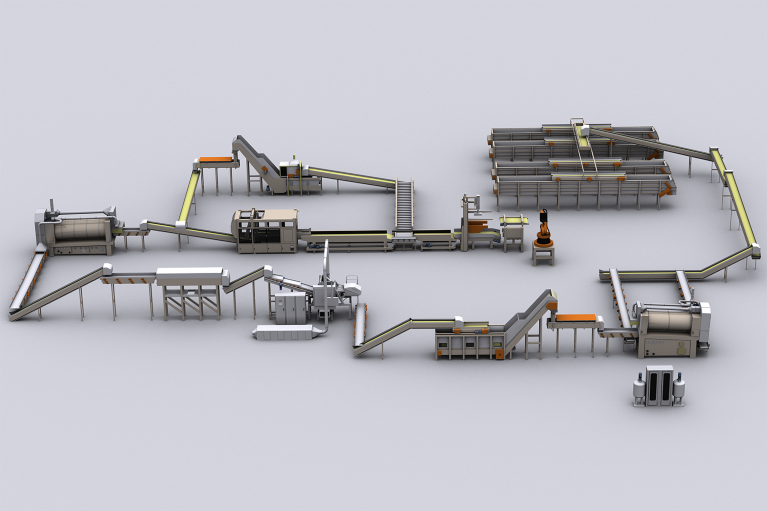
The digital twin factory production line control system undertakes data collection, automatic control, status display and management scheduling of material flow, information flow and energy flow in the production and processing process. Using big data analysis, artificial intelligence and other technical means, it performs real-time optimization analysis and makes intelligent decisions on process quality, production efficiency, energy consumption and other information to create an intelligent production scheduling operations and maintenance management platform.
An award-winning solutions provider
Thanks to its advanced technology, excellent team and high-quality service, Beijing Aero-Top Hi-Tech Co Ltd has won several national and provincial technical awards, and its market share ranks among the top of its domestic counterparts.
Contact information
86-010-58089996
www.aerotop.com.cn
Quick links
- Explore articles by subject
- Guide to authors
- Editorial policies
The Economics of Digital Twins
Ieee account.
- Change Username/Password
- Update Address
Purchase Details
- Payment Options
- Order History
- View Purchased Documents
Profile Information
- Communications Preferences
- Profession and Education
- Technical Interests
- US & Canada: +1 800 678 4333
- Worldwide: +1 732 981 0060
- Contact & Support
- About IEEE Xplore
- Accessibility
- Terms of Use
- Nondiscrimination Policy
- Privacy & Opting Out of Cookies
A not-for-profit organization, IEEE is the world's largest technical professional organization dedicated to advancing technology for the benefit of humanity. © Copyright 2024 IEEE - All rights reserved. Use of this web site signifies your agreement to the terms and conditions.

An official website of the United States government
The .gov means it’s official. Federal government websites often end in .gov or .mil. Before sharing sensitive information, make sure you’re on a federal government site.
The site is secure. The https:// ensures that you are connecting to the official website and that any information you provide is encrypted and transmitted securely.
- Publications
- Account settings
Preview improvements coming to the PMC website in October 2024. Learn More or Try it out now .
- Advanced Search
- Journal List
- Digit Health
- v.9; Jan-Dec 2023
Digital twin in healthcare: Recent updates and challenges
1 Department of Orthopedics, First Affiliated Hospital of Dalian Medical University, Dalian, People's Republic of China
2 Key Laboratory of Molecular Mechanism for Repair and Remodeling of Orthopedic Diseases, Dalian, People's Republic of China
3 School of Mechanical Engineering, Dalian University of Technology, Dalian, People's Republic of China
Zhonghai Li
As simulation is playing an increasingly important role in medicine, providing the individual patient with a customised diagnosis and treatment is envisaged as part of future precision medicine. Such customisation will become possible through the emergence of digital twin (DT) technology. The objective of this article is to review the progress of prominent research on DT technology in medicine and discuss the potential applications and future opportunities as well as several challenges remaining in digital healthcare. A review of the literature was conducted using PubMed, Web of Science, Google Scholar, Scopus and related bibliographic resources, in which the following terms and their derivatives were considered during the search: DT, medicine and digital health virtual healthcare. Finally, analyses of the literature yielded 465 pertinent articles, of which we selected 22 for detailed review. We summarised the application examples of DT in medicine and analysed the applications in many fields of medicine. It revealed encouraging results that DT is being increasing applied in medicine. Results from this literature review indicated that DT healthcare, as a key fusion approach of future medicine, will bring the advantages of precision diagnose and personalised treatment into reality.
Introduction
It is often said that ‘there is no disease, there is the patient’. Compared with other disciplines, medicine has inherent uncertainty, which makes medical practice challenging. Conventional drug therapy has many limitations such as medication ineffectiveness and the concept of personalised medicine has proved to play an important role in the healthcare sector. 1 – 3 Over the past few decades, more and more attention is being paid to precision medicine, which has been described as an emerging approach for disease treatment and prevention that considers variability in the genes, environment and lifestyle among individual people. 4 Some previous studies have used digital technology to construct a virtual physiological human body, with the vision of translating computational physiology into clinical practice, and have achieved certain progress. 5 , 6 The development of the technologies of big data, cloud computing, virtual-reality and the internet of things (IoT) has laid a technical foundation for the application of digital twin (DT) and thus provided clinicians and researchers with a more detailed dimension with which to study the occurrence and development of diseases and to conduct more precise diagnoses and treatments.
In recent years, the concept of DT is attracting more and more attention from researchers and engineers. With the continuous DT research by both industry and academia, however, the boundaries between DT and other related concepts have become increasingly blurred. The preliminary meaning of the DT included the physical product, virtual product and their connections. 7 The rapid development of communication technology, sensor technology, big data analysis, the IoT and simulation technology has led to the exponential growth of research on DTs. 8 , 9 Then, DT was redefined as a digital replication of living or non-living physical entities, which opened the gateway to using the DT for humans, such as in the field of health and wellbeing. 10 As a moving concept, DT is a virtual copy of the human organs, tissues, cells or micro-environment that constantly adjusts to variations in the online data and can predict the future of the corresponding counterpart. 11 , 12 However, the DT is more than just a digital model that is connected with a real-life twin through various emerging technologies. It is a living, intelligent and evolving model which can optimise the processes and continuously predicts future statuses (e.g. defects, damages and failures) through the closed-loop optimisation between DT and surrounding environment. 13 Generally speaking, the technologies required for DT can be divided into two categories, one is a statistical model driven by data, and the other is a mechanical model that integrates multi-scale knowledge and data. 14 , 15 The numerical model is used to calculate the structural performance while the analytical model is used for structural analysis. The artificial intelligence (AI) model, trained with the samples and numerical data, obtains the real-time structural performance from real-time sensor data. 16 , 17
The DT is revolutionising industry and been used by many major companies to increase efficiency and spot problems. 18 Healthcare can be another domain where DT can be applied. 19 In an application perspective, DT can treat patients as virtualised standalone assets that can be further used in environments and situations involving multiple related strategic assets of healthcare organisations. 20 The DT has great application potential to patients or hospitals to improve treatment and diagnostics. 21 The objective of this study is to make a review of the progress on DT technology in medicine and discuss the potential applications and future opportunities as well as several challenges remaining in digital healthcare.
Study design
This study aimed to review the technology in medicine and discuss the potential applications and future opportunities as well as several challenges remaining in digital healthcare. It was conducted through the academic databases PubMed, Web of Science, Google Scholar, Scopus and related bibliographic resources in which the following terms and their derivatives were considered during the search: DT, medicine, digital health and virtual healthcare. There are some important concepts and technologies in the previous research that laid the foundation for the construction of DT, such as virtual physiological human. However, as a fusion of various technologies, DT is an expansion and enrichment of previous researches. Therefore, only applications of DT technology in medicine are selected and presented, followed by a brief discussion of the advantages and limitations involved in such applications. We began the review with an overview of DT technology in the main databases. Then we continued by examining the models that meet medical characteristics and the applications of DT technology in medicine. Finally, we concluded the review by discussing the current limitations and directions for future work.
Eligibility criteria
The studies included in the review were in line with the following criteria: (1) articles in English; (2) building models of patients through DT-related technologies and (3) achieving assistance in diagnosis or personalised treatment through DT technology. However, studies that met any of the following criteria were excluded: (1) duplicated; (2) irrelevant articles; (3) proposed ideas or frameworks but not been applied yet; (4) not based on DT or medicine; (5) absence of technological support of DT during the establishment of models; (6) articles published only in the form of conference abstracts; (7) articles with the type of proposal, protocol, letter, conference or viewpoint and (8) some independent digital technologies are applied to assist diagnosis and treatment without the integration of DT technologies.
A total of 465 papers were retrieved by our search and 22 of them met the inclusion criteria and were, therefore, included in the further analysis. The flowchart of the selection procedure is shown in Figure 1 . Papers described only models or without applications were descripted in this review but excluded during eligibility screening and extraction. In general, the popularity of DT research in medicine has risen globally at an accelerating rate. There was a particularly sharp rise around 2010. The analysis of country distribution of DT publications in medicine reveals that the three countries having published the highest number of articles are the United States (35%), China (11%) and the United Kingdom (15%). Other countries like Australia (7%), Japan (5%), Canada (5%), Italy (6%) and Korea (4%) also contributed to the research in this field.

Flow diagram illustrating the screening process for papers in this study.
Figure 2 compares the time distributions of DT research in the medical field among the three main countries.

Comparison of studies on digital twin technology conducted in the United States, the United Kingdom, and China in the past 20 years.
Model construction is the core of basic research on DT in medicine, combining human anatomy and digital technology through image processing, digital collection processing, mathematical modelling and other technologies. Researchers digitally process two-dimensional (2D) cross-sectional images of the human body and use three-dimensional (3D) reconstruction technology to establish an intuitive 3D shape of the human body, allowing visualisation of the body structure. 22 The Blue Brain Project, for example, began in 2005 as a collaboration between EPFL (Ecole Polytechnique Fédérale de Lausanne) and International Business Machines and aims to model the neocortical column. 23 Schütt et al. 24 developed a DT of the Dynamic Colon Model that considered media viscosity, and peristaltic wave speed. It provided new insights for dissolution testing beyond established pharmacopoeial methods.
Modelling includes mechanics modelling, mechanical network modelling and statistical modelling. Establishing an appropriate mechanical model would make it possible to theoretically study the mass transmission of the human body and electrophysiological problems coupled with mechanics. The mechanics models can predict the emergency behaviour of biological systems in the fields of health and disease because they combine the interaction of biological components of the system. Mechanical network models usually take the form of a coupled system of ordinary differential equations, which are solved to discover how the molecular concentration evolves with time when molecules interact and respond to network inputs. Statistical models are complementary tools with which to verify the mechanics models by finding mechanistic explanations of inductive inference. Similarly, data from mechanics models need to be scrutinised quantitatively for validation. 24 , 25 To acquire and integrate real-time data or signals from a connected bioreactor, sensors including traditional sensors and newer sensors such as spectroscopic sensors are used in process analytical technology. 26 In a whole-cell project, specific types of cell and organism were modelled to better understand the molecular basis of diseases. 27
Therefore, faster solving surrogates of these models may be developed in the form of machine learning models to solve problems in real-time. 28 It can help accelerate development and improve the quality of modelling by allowing modular development and linkages that account for interactions between various sub-processes. However, the need for further validation and the lack of clinical interpretability have limited the achievements of DT technologies. 29 When used on patients from different countries or centres, the models may need recalibration and the quality of datasets may need validation before they are integrated in clinical decision making. In realising clinical translation, model synergy can be used to increase clinical interpretability, verify the versatility of results, promote effective research results and testing methods and accelerate the integration of new technologies and clinical practice. 14
Clinical application of the DT
Assisted by improvements in engineering technology, the medical industry has gradually transitioned from traditional medicine to digital medicine and then to information medicine, resulting in today's concept of smart medicine, which satisfies the individual's preventive and personalised medical needs to a greater extent. The purpose of building a DT diagnosis and treatment system is to realise precision medicine. The core of precision medicine is personalisation such that the treatment is patient centric by using AI or other cutting-edge technologies to accurately locate the cause of a patient's disease. The clinical applications of DT are shown in Table 1 .
Table 1.
Representative applications of DT in medical fields.
3D: three-dimensional; AI: artificial intelligence; CRT: Cardiac resynchronisation therapy; CT: computed tomography; DT: digital twin; FEM: finite element method; GPU: graphics processing unit; IK: inverse kinematics; IoT: internet of things.
Cardiovascular disease
The application of the DT to the cardiovascular system includes the establishment of DT heart models and the precise treatment of cardiovascular disease. Models can be used to accurately determine the most valuable diagnostic basis and reliably infer biomarkers through non-invasive procedures. The key to DT-guided diagnosis is the personalised construction of the model. This approach has been adopted for the computation of pressure drops in flow obstruction and has proved to be more accurate than following clinical guidelines. 48 Philips developed a personalised DT model based on unique computed tomography (CT) images of the heart obtained before the surgical procedure. 49 The Philips HeartNavigator tool combines CT images in a single image of the patient's heart anatomy and provides real-time 3D insight into the positioning of devices during surgery, which can simplify the prior procedure planning and help a surgeon to select the best device. Nelson et al. 31 introduced the HeartFlow Analysis technology that enables more comprehensive evaluation of coronary artery disease by providing functional information and improves the patient's outcome. As a non-invasive electrocardiographic imaging system, the Acorys Mapping system combined a biopotential amplifier and a high-density sensor with innovative image and signal processing technologies to provide electrical activity in the surface of the heart in a completely non-invasive way. 33
A DT can simulate dosage effects or the device response before a specific treatment and thus indicate whether the medical device or treatment is appropriate for patients and improve the treatment of patients with different causes of disease. After transcatheter aortic value implantation, FEops’ HEARTguide was used to predict the device–patient interactions. 50 FEops’ cutting-edge technology transforms cardiac images into DTs to improve and expand personalised management for patients with structural heart disease. 30 Combining DTs with AI-enabled anatomical analyses generates data-driven insights aspiring to enhance and improve structural heart disease management along the entire patient pathway, spanning from patient selection, procedure planning, periprocedural guidance to patient follow up. Cardiac resynchronisation therapy (CRT) is widely used for patients with prolonged QRS duration, but there remain uncertainties in the decision making in the ‘grey zone’. 32 Niederer et al. 51 used mechanical models to investigate the dependence of the CRT efficacy on cellular-scale mechanisms and organs and identify novel patient selection criteria. Such a model is used to identify the length dependence of tension as a regulator of CRT and predicts that a patient will respond less to CRT treatment if he or she has cellular-scale dyssynchronous electrical activation but effective length-dependent tension regulation. Another example is in the field of infarct-related ventricular tachycardia, where researchers use a DT to improve the ablation guidance through the accurate identification of patient-specific optimal targets before a clinical procedure. 52 Mazumder et al. 35 used a two-chambered heart, haemodynamic equations and pressure control mechanisms based on pressure reflexes to construct a DT body of the cardiovascular system. Synthetic physiological data such as photoplethysmogram (PPG) was generated from healthy and atherosclerosis models, which can generate large amounts of data on blood pressure and blood flow changes.
The idea of using the DT in the surgical field is to create a patient model for multi-disciplinary teams to plan a surgery and verify the anatomy and thus avoid inadvertent damage to structures. 34 A number of surgical specialties evaluated patient-specific simulation, including neurosurgery, vascular surgery and interventional radiology. As an example, the use of the DT in treating cardiovascular disease is growing and there is an emerging interest in the application of AI in vascular surgery. 36 , 53 The virtual model established using DT technology can used to develop diagnostic tools. Chakshu et al. 42 proposed a semi-active DT model coupled with blood flow and head vibration to detect the severity of carotid stenosis from a video of a human face. They compared the in vivo vibration against the virtual data to find the best fit between the virtual and in vivo data. Intracranial aneurysms may cause stroke and thrombosis and there may be immediate death in severe cases. Suzuki et al. 54 considered clinical, morphological and haemodynamic parameters and used computational fluid dynamics to calculate haemodynamic parameters from CT images. They obtained a rupture risk model through multivariate logistic regression and listed age, longer length, location at a bifurcation, lower pressure, loss coefficient and presence of a bleb as risk factors.
Owing to the extremely high risk of surgery, doctors usually use shunts to guide the blood flow away from the aneurysm sac. This method is less invasive, but the procedure is more complicated because the implant may cause additional damage to the artery. Sim&Cure adopted 3D rotational angiography to create a 3D model of an aneurysm and surrounding vessels before surgery, with the model being presented to the surgeon to complete the simulation and help choose points defining the ideal end position and the size of implants. 55 As a surgical-augmented intelligence company, Cydar's Intelligent Maps applied the latest in cloud graphics processing unit computing, computer vision and machine learning technology to advance surgical visualisation and decision making across the surgical pathway. 37 Golse et al. built a mathematical model of the entire blood circulation, which is automatically calibrated from patient characteristics. They demonstrated that a DT model could correctly predict post-operative portal hypertension, using estimated hepatic flow rate as input data. 38 Combined simulation with virtual-reality platforms, DT can be used to teach foundational technical skills in various surgical specialties and improve the surgical training of residents and provide a realistic account of the performance at the same time. 43 , 56
The medicine used in treatment should be personalised as human bodies and physiology are different. DT models created using genetic code can reveal changes in the performance of the body and provide researchers a more cost-effective opportunity to evaluate new compounds with a more accurate result. Takeda Pharmaceuticals has switched to DT technology in production to offer transformative therapies around the world. The creation of DT models can shorten pharmaceutical processes and allow realistic input–output predictions of biochemical reactions. 45 Additionally, Atos and Siemens worked with the pharmaceutical industry to improve the manufacturing process using physical DT models, which were created to overcome difficulties in terms of efficiency and production. 46
In the phase of operation, DT can constantly provide real-time process control and optimisation information in order to support the product development and risk analysis. 44 Subramanian proposed a top-down approach for creating a DT that integrated information from scientific and clinical sources to help drug discovery and research, biomarkers identification, test development, screening and clinical trial optimisation. 41 The DT models have been shown to work well and are supported by the IoT, AI and many other advanced technologies.
Orthopaedics
With the development of numerical simulation and worn devices, the use of a DT for the real-time monitoring and analysis of the lumbar spine has become a highly promising cutting-edge technology in the biomechanical field. In applying the DT in the field of orthopaedics, it is important to develop physics-based experimental models and data-driven numerical models, which have the advantages of low cost and high integrity. Croatti et al. discussed the integration of DTs with agents and multi-agent systems technologies in healthcare and presented a first case study about the application of agent-based DT to the management of severe traumas. 40
Our team firstly tried the application of DT in the human lumbar spine. Through the collection of customised information on the lumbar spine bones of a specific experimenter, we built a shape-performance integrated DT body for the prediction of the biomechanical properties of a real lumbar spine for different human postures. 57 The real-time motion posture and spatial position of the human body were obtained using human-motion-capture technology. The lumbar posture of the corresponding human body was calculated using a wearable virtual-reality device and a small volume of sensor data. Using the information of the inverse kinematics system and adopting the FE method and Gaussian process regression, a DT body of the lumbar spine was established, so as to realise various motion postures of the human body. In addition, the biomechanical properties of the lumbar spine were evaluated and predicted in real-time. Using the proxy model, the mechanical properties of the DT of the lumbar spine were dynamically calculated for real-time monitoring and prediction. Finally, a 3D virtual-reality system was developed using Unity3D software to record the real-time biomechanical performance of the lumbar spine during the movement of the body, which provided a new and effective method of warning and real-time planning in the field of orthopaedic treatments, especially in spinal rehabilitation.
Subsequently, several other studies have constructed DTs based on orthopaedic surgical models to provide quantitative and valuable information for clinical decision making and to evaluate reasonable parameters such as mechanical strength and interfragmentary strain to quantify the risk of recurrent fractures. 47 , 58 , 59 Aubert et al. 47 created a DT using post-operative 3D X-ray images of the patient, from which 4 stabilisation methods and 3 bone healing conditions resulted in a simulation of 12 scenarios from the DT. The risk of recurrent fractures was assessed by applying the maximum load during gait to assess mechanical strength, stress distribution, interfragmentary strain and fragment kinematics. Hernigou et al. built a patient model based on CT, AI and DT technology, which minimises the inaccuracy of manual selection of anatomical landmarks by imaging systems, and improved the accuracy of the model. 59 They identified the orientation and evaluated the compensation of the axis of subtalar joints.
The COVID-19 pandemic has generated enormous interest in the modelling and simulation of infectious diseases. A coronavirus spreads through person-to-person contact via respiratory droplets, especially when an infected patient coughs or sneezes. Zohdi built a rapidly computable respiratory emission model and developed a combined DT and machine learning framework with which to optimise ventilation systems. 60 , 61 The framework is based on a genomic algorithm and coupled with simplified equations for the relationship between the particles and the fluid to ascertain the placement and flow rates of multiple ventilation units, so as to optimally sequester particles that are released from patients who are coughing or sneezing.
During the pandemic, it has been necessary to find a way to vaccinate more people in a shorter time period, especially when healthcare workers are scarce. Pilati et al. 39 developed a DT system for the vaccination process and tested it in a clinic. The system allows a real-time simulation of patients and creates a dynamic vaccination centre. The virtual model can be run to find problems and address them in the real system, thus improving the efficiency of vaccination.
Other fields of application
The use of the DT in medicine is mainly focused on the personal health management, especially for the elderly. For example, Gkouskou et al. 62 described the virtual DT for precision nutrition, combining the information extracted from the genome with immunity, longitudinal and biological variables. Such a model can improve dietary choices and provide highly individualised lifestyle recommendations. It may revolutionise the management of obesity and its comorbidities, and provide a pillar for healthy ageing. Moreover, Laybenbacher et al. 63 outlined a roadmap for building an immune DT to capture key features of the immune system, which was to structure in four stages: specification, model constructions, personalisation and continued improvement. It would help to change the nature of biomedical research and accelerate the clinical translation of basic research.
In an artificial pancreas model for patients with type-1 diabetes, mathematical models of the human glucose metabolism and data algorithms that simulate insulin delivery have been customised as a patient-specific DT model, which continuously calculates the insulin requirement and regulates the blood insulin concentration. 64 , 65 For example, Grosman et al. used the run-in data from system users to generate a DT that personalised system settings during the study phase. 66 The result showed that personalised auto mode reduced the mean of sensor glucose. It is the first demonstration that DT can successfully preform the insulin pump therapy with automation and personalisation.
The main finding of this review is that DT has achieved initial applications in medicine, such as cardiovascular disease, orthopaedics, surgery, pharmacy, etc. Using this new technology successfully helped solve several problems, such as real-time monitoring, dynamic analysis and precise treatment for diseases, which were difficult to deal with traditional methods. DT has the advantages of FE methods, such as non-invasiveness, controllability, repeatability, etc. At the same time, it can obtain the real-time health data of patients through wearable devices or sensors to perform real-time analysis, so as to continuously monitor and prevent the development or further deterioration of medical conditions. Further, DT makes it possible to select a more precise treatment options for the individual patient by using computer algorithm-based methods and principles in bioinformatics. It can also enable patients to exercise a greater degree of autonomy and achieve equitable treatment for patients irrespective of race or gender. The DT technology can be used both in patient healthcare and in hospital management. Using DT, scenarios can be predicted and evaluated in the virtual environment for scheduling and implementation in the real environment, which will reduce risk and save costs. It can also transmit information of treatment methods and drugs to the DT models for validation, which can optimise treatment options and ultimately enable early diagnosis or prevention of disease.
However, the effectiveness of the technology largely depends to a large extent on the accuracy of simulation. Current methods for validation are mainly based on comparison with cadaveric data or previous studies, which lack individualised assessment and accurate validation. There are several technical hurdles to creating high-fidelity models, including the multi-scale and multi-physics nature of the models, the difficulty in linking sub-process models across scales and physics, and the scarcity of experimental data. How to solve the gap between the results calculated by the DT and the real situation is another difficulty for DT. For example, the risks which were not predicted or estimated during the modelling for a surgery, while this risk occurred when the surgery was performed. There are also socio-ethical risks in DT healthcare. Privacy, seems to be the most important socio-ethical risk, is the main reason why DT may be disadvantageous. To some extent, DT healthcare faces legal and economic problems. The government and international legislatures should conduct strict supervision and establish standards that are valid and feasible for their own countries or regions. For example, service providers ought to seek explicit informed consent from the users and convey their plans for the secondary use of the entrusted data to relevant parties transparently. At the same time, more investment is needed to support the development of DTs. Obviously, a person's DT can cause discrimination or interference with the real person, which may cause trouble to his work and life. Furthermore, the high cost of DT healthcare may lead to inequality and injustice and thus widen the existing socio-economical gap. 67 Due to the promise of DT for personalised healthcare is built on extensive health-related data and the data of the sensor is transmitted to the cloud through the 5G network, so that the safety system during the operation of DT is crucial. Besides, real-time data collection will increase the accuracy of the simulation, some invasive examinations also increase the risk of the technology itself.
DT in future medicine
The number of studies on the application of DTs in the field medicine will increase with the exploration of the DT and advances of the technologies of the IoT, big data and AI. According to a Health Market report, global spending on the IoT in the field of healthcare is expected to reach USD 188.2 billion by 2025 with a growth rate of 21.0% and national health expenditures will reach 1795 per capita with a growth rate of 1.50% from 2012. 68 Ideally, the DT is a solution for precision medicine, which requires the integrating and processing of a large volume of the data. The IoT provides technical supports for the overall perception of the physical entity through data collection methods such as 2D codes, data acquisition cards and sensors. They are necessary for real-time data collecting, and then feedback on the processed data to optimise the models and regulate the operation through communication technology. It is envisaged that individuals will have their own DTs. Combining the DT of medical equipment and the DT of medical auxiliary equipment will provide a new platform and new experimental method for personal health management and healthcare services. Furthermore, adopting DTs and big data processing, simulations can be performed using high-resolution models of patients to find accurate treatment targets and suitable drugs or treatment methods for patients in realising precision medical treatment. Finally, establishing DTs at hospitals or in departments of hospitals allows the efficient management of medical resources and the planning of demand-oriented medical activities. Figure 3 depicts the application process of DTs in medicine and considers future possibilities.

Application and prospects of digital twin technology in the medical field.
Health monitoring
Generally, chronic diseases have the characteristics of a long duration, high incidence rate and large variety and are difficult to cure. Meanwhile, seniors have weak bodies and poor memories and do not have enough knowledge or information about the medical treatment. Hence, seniors need more care and community medical services, which can be effective solutions for real-time monitoring, medical guidance and crisis warnings. The healthcare service platform can build a DT model based on the physiological parameters of seniors and obtain the real-time health data of seniors using wearable devices and mobile phones. Possible abnormal conditions can then be calculated and analysed in a timely manner to realise crisis warnings. Integrating DT can better support the care of chronic diseases, such as dementia, thus ensuring greater precision and personalisation. 69 It will also be possible to transmit information of treatment methods and drugs to the model for verification in optimising the treatment plan and finally realise the early diagnosis or prevention of diseases in seniors. 70 The DT will track an individual's life journey, using data collected by wearable sensors and the lifestyle registered by the individual for the transition from clinical medicine to preventive medicine.
It is envisaged that individuals will have a full-lifecycle DT body, where data are collected from birth to form a virtual twin, which will grow with the child and serve as a life-long health record or medical experiment object. Medical institutions can obtain data of the human body in various perceptual ways with the real-time connection of data being the basis of a full-lifecycle DT body, which will ensure the accuracy of the DT model. People can obtain information of their physical condition in a timely manner to predict the occurrence of risk and adjust their diet or schedule appropriately. Therefore, using the DT body and epidemiological big data, the medical system can perform the real-time monitoring of the patient's health status and predict the risk of disease that may occur at any time for that person. Furthermore, suggestions regarding the lifestyle and prevention methods for the individual can be given in a timely manner.
Precise diagnosis
The DT patient can be well developed using multi-source data, which can be collected using various medical scanning and wearable instruments, such as the instruments of CT, magnetic resonance imaging, emission CT and colour ultrasound. There are also biochemical data from routine blood tests, urine tests and tests of enzymes to simulate the micro-environment and basic vital signs, making the virtual patient more accurate. The ideal DT model should integrate all data of patients and all types of pathogenesis, to form multi-layer modules. 71 Data of different types can be connected through the mapping of the relationship between the different types of modules, such as messenger RNAs and proteins. 72 Additionally, network tools can link interactions between cells from different types of tissue model, thereby creating a realistically complex DT patient. 73 However, DT patients cannot be restricted to being molecular profiles. We should also consider environmental factors when it comes to a patient with severe asthma, who must avoid allergens. In addition, there are historical data, medical records, health prediction data, surgical simulation data and virtual drug test data. The collected data must be complete and made suitable for analysis in modelling and decision making. Therefore, using the DT of the human body, the medical system can predict an individual's immune response to infection or injury, which could make a precise diagnosis of disease and be lifesaving in many ways.
Precise treatment
When a person is attacked by a disease, the specialists do not need to have face-to-face consultations with the patient. Just remote visual consultations with the help of data and models are required to determine the cause of the disease or prevent it. Before an operation, a DT can assist in the drawing up of a surgical procedure plan and the surgeon can use a virtual display to evaluate the operation plan on the virtual human body. The surgeon can trial an operational process from multiple angles and for multiple modules to verify safety and feasibility and make improvements to the operational process until they are satisfied. During the operation, the DT can broaden the surgical perspective, warn of the danger of blind spots, predict hidden bleeding, and help prepare or respond to an action according to circumstance. Additionally, the DT can be used as a reference to verify the anatomy and avoid unnecessary damage to structures. Furthermore, including virtual data at the molecular cell level in conducting virtual experiments and clinical trials of drugs can greatly shorten the development period of drugs and reduce the side effects of drugs on the human body. In summary, the DT can help in realising personalised medicine including targeted intervention before the disease worsens and in performing accurate predictions, accurate detections and precise treatments.
As an example, the DT plays a vital role when precision medical methods are adopted to treat malignant tumours. 74 Using computer algorithm-based methods and the principles of bioinformatics, it is possible to choose treatment options that are more effective for malignant tumours according to the DT model of the individual patient, thereby improving the survival rate and quality of the patient's life. The patient's genotype data can be put into the calculation model for predicting the effect of anti-cancer drugs, with the model outputting the patient's sensitivity to single or multiple drugs and helping doctors to determine the most suitable therapeutic drugs for a cancer patient. All the above can help realise the precise treatment of cancer patients.
In hospitals, resource management and clinical departments can use DTs to address the lack of resources in healthcare, especially that during the COVID-19 pandemic. As an example, the radiology department of Mater Private Hospital created a DT to make various predictions and test many scenarios. Using the information provided by the DT, the hospital adjusted the planning and organisation for its radiology department and thus reduced the waiting time of patients and improved the efficiency of the hospital. The DT will be widely used in other departments of the hospital. As an example, the doctor's visit time can be adjusted according to the flow of outpatients, and the occurrence of failures can be predicted according to the DT of the equipment and repaired in a timely manner to reduce the error rate and the loss of the hospital. In addition, the virtual human body can be used to train medical staff and thus improve medical skills, the treatment success rate and the teaching level of the hospital.
This review was conducted to introduce the DT technology and summarise its applications in medicine. It will help in identifying the strengths and limitations of DT in medicine. It also showed the potential and opportunities of this technology to further application in all the medical fields. Future studies could include these aspects to expand this literature review or make more attempts of DT in medicine based on the existing researches.
Limitations
The search in this review was restricted to English articles only, which might result in the omission of some publications. Also, we restricted the search to articles that included the terms digital twin, healthcare or respective Medical Subject Heading terms. Some relevant papers which did not explicitly use these terms but dealt with relevant topics might be ignored. In addition, the application of DT in medicine is currently in the preliminary stage, and most of them are application attempts. So there is a lack of randomised controlled trials for quality assessment.
In this review, we studied the DT technology and the applications of DT in medicine. We concluded that DT will be more widely used in the medical field to solve the problems, such as real-time monitoring, dynamic analysis and precise treatment for diseases, which cannot be fully explained by traditional methods. It can model the perception and action of any relevant facility in the medical environment, coupling the observable state of the DT with the state of the physical entity (PE). Although, DT technology has technical and ethical problems in the medical field that need to be solved urgently, the progress is encouraging. The use of DT in medicine is not only limited to the diagnosis and treatment of diseases, but can also be used for the prediction of health and disease states, which provide a quantitative understanding of health and disease. DT healthcare, as a key fusion approach of future medicine, will realise precise medicine and bring the advantages of personalised treatment to reality.
Acknowledgements
We thank Liwen Bianji (Edanz) ( www.liwenbianji.cn ) for editing the language of a draft of this manuscript.
Contributorship: TS wrote the first draft of the manuscript. All authors contributed to manuscript revision, read, and approved the submitted version.
The authors declared no potential conflicts of interest with respect to the research, authorship, and/or publication of this article.
Funding: The authors disclosed receipt of the following financial support for the research, authorship, and/or publication of this article: This work was supported by the Natural Science Foundation of Liaoning Province, the Science and Technology Innovation Foundation of Dalian (grant number 2022-MS-322, 2022JJ12SN045).
ORCID iD: Zhonghai Li https://orcid.org/0000-0003-4735-1193
Digital twins and generative AI: A powerful pairing
April 11, 2024 by Alex Cosmas , Guilherme Cruz, Sebastian Cubela, Mark Huntington , Sohrab Rahimi, and Sanchit Tiwari
Many organizations across industries are separately implementing digital twins and generative AI (gen AI) —two technologies with distinct value propositions and tremendous promise—to support a wide range of use cases. Gen AI can structure inputs and synthesize outputs of digital twins, and digital twins can provide a robust test-and-learn environment for gen AI. By combining these technologies, organizations could produce synergies that reduce costs, accelerate deployment, and provide substantially more value than either could deliver on its own.
About QuantumBlack, AI by McKinsey
This blog is the first in a three-part series on digital twins. Future posts will explore when and why to use digital twins and delve into an in-depth case study on how gen AI and digital twins could be used to enhance customer experience.
Digital twins and gen AI have proven their value
Each of these technologies has demonstrated its value across a wide range of industries and use cases.
Digital twins —reusable virtual representations of assets, people, or processes and their environments that simulate strategies and optimize behaviors—are a powerful tool that can help organizations improve data-backed decision making. By constructing scenarios of real-world situations and outcomes, they can provide insights that serve as an early-warning system, predicting events and the likelihood they will occur. They also provide a risk-free digital laboratory for testing designs and options, improving efficiency and time to market, for example, by optimizing scheduling, sequencing, and maintenance.
Gen AI describes algorithms that can be used to create content, including audio, code, images, text, simulations, and videos. As with digital twins, gen AI is revolutionizing business processes and activities in myriad ways, and new applications of gen AI are being conceived daily; the impact on productivity and economic potential multiplies with each one.
Today, 75 percent of large enterprises are actively investing in digital twins to scale AI solutions. Each technology has immense potential, and together they could unlock trillions in total economic value.
Generative AI and digital twins elevate and reinforce each other
Digital twins and gen AI can be used in combination to unlock insights and elevate their respective value. Following are some examples.
A universal model
Building a digital twin, especially for highly specialized applications (such as multimachine production scheduling or vehicle routing), can be time-consuming and resource-intensive. The effort often entails designing and developing new digital-twin models, a process that can take six months or longer and incur substantial labor, computing, and server costs. By leveraging a software development platform such as GitHub, large language models (LLMs) can create code for the digital twin, accelerating the development process and increasing effectiveness. This ability to generate such output leads to an exciting prospect: LLMs could possibly be used to create a generalized digital-twin solution—a foundational, universal model—that facilitates design and serves as a starting point for developers across digital-twin projects and even industries.
The architecture of a digital twin can be represented as nodes and edges in a time-series graph, allowing graph-based LLMs (such as GNN-LM, TextGNN, GIANT, and SimTeG) to create a basic model of a twin, whose design can then be built upon and adapted for various scenarios and industries. 1 For example, in a future smart-city context, various urban elements—such as traffic systems, public utilities, and environmental sensors—could feed into LLMs, which could identify and create potential connections between nodes in a graph. This lays the groundwork for simulating and predicting interactions and impacts over time. 2
Data collection, transfer, and augmentation
Digital twins thrive on large volumes of (often real-time) data from diverse sources, which can be unwieldly to manage. LLMs offer advanced “embedding” capabilities, 3 meaning they can significantly compress data while retaining essential information. As such, they enable efficient data transfer and processing in digital twins. 4 In a manufacturing setting, for example, gen AI could organize data from maintenance logs, equipment images, and operational videos. A digital twin could analyze this data, identify patterns or anomalies that might not be evident from unstructured data alone, and inform decision making and predictive-maintenance strategies. 5
Additionally, gen AI tools can supplement data training sets used by digital twins by creating synthetic data. For example, a set of maintenance logs may not include a particular defect. Gen AI could create a synthetic data set that includes the defect so that the digital twin is trained to recognize it in the future.
Digital twins generate a large volume of data on assets, people, or processes and their environments. Multimodal LLMs (such as Gemini and GPT-4V) can efficiently analyze and interpret large data sets and synthesize insights. Additionally, LLMs can generate scenarios for the digital twin to simulate that are more comprehensive. In this way, LLMs (such as OptiGuide or Vertex) can function as an interface for simulators, allowing users to communicate with digital twins via natural language (for example, asking questions and receiving understandable insights in return). 6 This method eases interactions with a complex system, making it more accessible to users who lack extensive technical expertise, and facilitates data-backed decision making.
Checks and balances
Gen AI not only enhances digital-twin capabilities but also uses real-time data from digital twins as a source of additional context, making inputs (such as prompt engineering) far more dynamic. With stores of robust, contextualized data, digital twins provide a secure environment in which gen AI can “learn” and broaden the scope of prompts and output. Through “what if” simulations run by digital twins, users can fine-tune gen AI, enabling it to conduct predictive modeling, as opposed to the primarily backward-looking view that most LLMs provide. Last, the digital-twin constraint engine can validate gen AI capabilities and boost gen AI accuracy by limiting answers to only feasible regions, helping answers from gen AI adhere to physical limits or other constraints. For example, after gen AI copilots generate code to support a piece of machinery, the digital twin can validate the code before it is deployed to ensure it functions within preset parameters (such as machinery temperature or output rate). While this capability is still in the early stages of development, there is immense potential and substantial future value for a broad variety of use cases.
Capturing synergies requires mitigating certain risks
Leaders can mitigate risks associated with AI tools by establishing clear principles , a guiding framework and commitment to safe, secure use. Gen AI use comes with a potential for inaccuracies and bias, so it is important to ensure that tools are optimized for privacy and regulated data. Extensive diligence is necessary to assess the values and security measures of third parties prior to starting development. During the development process, it is important to carefully consider models’ assumptions and simplifications to ensure outputs are contextualized. Ongoing monitoring is also critical because inaccurate models are apt to produce misleading outcomes that the model itself is unlikely to flag. This can be avoided by ensuring that digital twins and gen AI are built on a foundation of high-volume and high-quality data . Prior to building a digital twin, it is important to have a clear business use case for the twin and safe, quality data available.
Generative AI can present ethical challenges, particularly with respect to data privacy and security. These risks are most prevalent in sensitive industries that handle personally identifiable information (PII). Addressing these challenges requires careful attention to data protection and ethically sourcing training data. Likewise, maintaining a culture of continuous learning and development of AI systems will be important as AI tools continue to evolve and advance. Regular adaptive training, improving feedback loops, and educating users are critical to success and maintaining compliance with legal and societal standards.
The symbiotic relationship between digital twins and gen AI increases their combined scalability, accessibility, and affordability. This new frontier will allow innovative and dynamic organizations to improve their advantage and allow organizations that are lagging behind to catch up to the competition.
Alex Cosmas and Guilherme Cruz are partners in McKinsey’s New York office; Sebastian Cubela is a partner in the Miami office; Mark Huntington is a partner in the Chicago office, where Sanchit Tiwari is a senior principal data scientist; and Sohrab Rahimi is an associate partner in the New Jersey office.
1 Jiadi Du and Tie Luo, “Digital twin graph: Automated domain-agnostic construction, fusion, and simulation of IoT-enabled world,” arXiv, April 20, 2023. 2 Chi Han et al., “Large language models on graphs: A comprehensive survey,” arXiv, February 1, 2024. 3 “Embeddings,” OpenAI, accessed March 25, 2024. 4 Yongming Wang et al., “Wireless network digital twin for 6G: Generative AI as a key enabler,” arXiv, November 29, 2023. 5 Nasser Jazdi et al., “Towards autonomous system: Flexible modular production system enhanced with large language model agents,” arXiv, July 24, 2023. 6 Beibin Li et al., “Large language models for supply chain optimization,” arXiv, July 13, 2023.
Home — Essay Samples — Information Science and Technology — Digital Era — Digital Twin – Rise of New Era

Digital Twin – Rise of New Era
- Categories: Digital Era
About this sample

Words: 690 |
Published: Jan 4, 2019
Words: 690 | Pages: 2 | 4 min read
Table of contents
How does digital twin work, what it means for finite element analysis, application of digital twin.
- Manufacturing is set for major change with this upcoming technology, where this has significant impact in designing the product, maintaining, optimizing which in turn reduces the throughput times.
- Automobiles: Digital Twin finds its feet in developing virtual model of the connected vehicle, capture the behavior, operational data and analyzed, which helps in improving the vehicle performance for the connected vehicle.
- Retail: Digital Twins helps in better instore planning, security implementation and energy management in an optimized manner.
- Healthcare: Digital Twins along with data from IoT can play a key role in the health care sector from cost savings to patient monitoring, preventative maintenance and providing personalized health care.
- Industrial IoT: Industrial firms with digital twin implementation can now monitor, track and control industrial systems digitally. Apart from the operational data, the digital twins capture environmental data such as location, configuration, financial models etc. which helps in predicting the future operations and anomalies
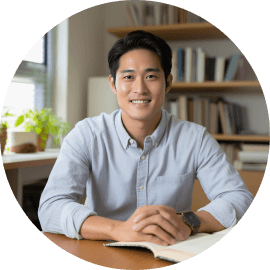
Cite this Essay
Let us write you an essay from scratch
- 450+ experts on 30 subjects ready to help
- Custom essay delivered in as few as 3 hours
Get high-quality help

Prof Ernest (PhD)
Verified writer
- Expert in: Information Science and Technology
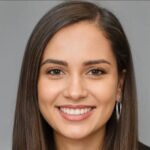
+ 120 experts online
By clicking “Check Writers’ Offers”, you agree to our terms of service and privacy policy . We’ll occasionally send you promo and account related email
No need to pay just yet!
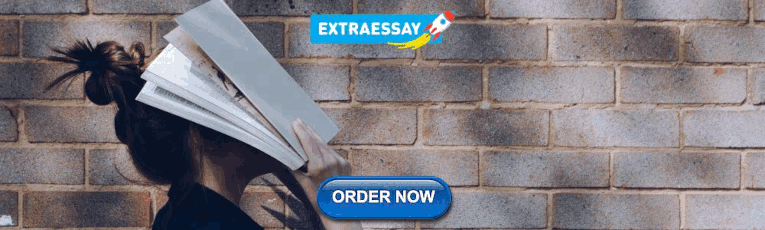
Related Essays
2 pages / 972 words
1 pages / 530 words
2 pages / 683 words
4 pages / 1802 words
Remember! This is just a sample.
You can get your custom paper by one of our expert writers.
121 writers online
Still can’t find what you need?
Browse our vast selection of original essay samples, each expertly formatted and styled
Related Essays on Digital Era
The provision of free internet access is a topic of growing importance in our increasingly digital society. The internet has transformed the way we communicate, access information, and engage with the world. However, access to [...]
The right to privacy is a fundamental human right that has evolved and adapted over time, particularly in the face of rapid technological advancements. In an era where personal information is more accessible than ever before, [...]
The question of whether students should have limited access to the internet is a complex and timely one, given the pervasive role of technology in education. While the internet offers a wealth of information and resources, [...]
In the digital age, we find ourselves immersed in a sea of information and entertainment, bombarded by a constant stream of images, videos, and messages. Neil Postman's prophetic book, "Amusing Ourselves to Death," published in [...]
1) What are the advantages and disadvantages of the new POS system? POS solutions are often customizable to suit your business needs Software is hosted on secure servers and in multiple data centers, so your information will [...]
It was a time when personal computer was a set consisting of monitor and single choice of technology, but it wasn’t good like using only one thing on technology. And after that was a revolutionary change of different computing [...]
Related Topics
By clicking “Send”, you agree to our Terms of service and Privacy statement . We will occasionally send you account related emails.
Where do you want us to send this sample?
By clicking “Continue”, you agree to our terms of service and privacy policy.
Be careful. This essay is not unique
This essay was donated by a student and is likely to have been used and submitted before
Download this Sample
Free samples may contain mistakes and not unique parts
Sorry, we could not paraphrase this essay. Our professional writers can rewrite it and get you a unique paper.
Please check your inbox.
We can write you a custom essay that will follow your exact instructions and meet the deadlines. Let's fix your grades together!
Get Your Personalized Essay in 3 Hours or Less!
We use cookies to personalyze your web-site experience. By continuing we’ll assume you board with our cookie policy .
- Instructions Followed To The Letter
- Deadlines Met At Every Stage
- Unique And Plagiarism Free
Advertisement
A unified view of a human digital twin
- Review Article
- Open access
- Published: 12 March 2022
- Volume 4 , pages 23–33, ( 2022 )
Cite this article
You have full access to this open access article
- Michael E. Miller ORCID: orcid.org/0000-0001-6306-3437 1 &
- Emily Spatz 2
10k Accesses
24 Citations
3 Altmetric
Explore all metrics
The term human digital twin has recently been applied in many domains, including medical and manufacturing. This term extends the digital twin concept, which has been illustrated to provide enhanced system performance as it combines system models and analyses with real-time measurements for an individual system to improve system maintenance. Human digital twins have the potential to change the practice of human system integration as these systems employ real-time sensing and feedback to tightly couple measurements of human performance, behavior, and environmental influences throughout a product’s life cycle to human models to improve system design and performance. However, as this concept is relatively new, the literature lacks inclusive and precise definitions of this concept. The current research reviews the literature on human digital twins to provide a generalized structure of these systems, provide definitions of a human digital twin and human digital twin system, and review the potential applications of these systems within product design, development, and sustainment. This review of the existing literature suggests that components of human models sufficient to provide robust human digital twins are likely to be derived across multiple fields of study. Thus, development of these systems would benefit an open multi-disciplinary research effort.
Similar content being viewed by others
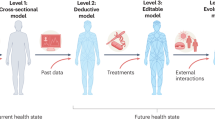
A roadmap for the development of human body digital twins
Chenyu Tang, Wentian Yi, … Luigi G. Occhipinti
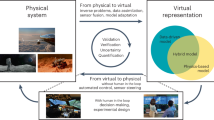
Digital twins in mechanical and aerospace engineering
Alberto Ferrari & Karen Willcox
Making Sense of Digital Twins: An Analytical Framework
Avoid common mistakes on your manuscript.
1 Introduction
An outcome of the industrial revolution and mass production is the standardization of products. This process has many advantages, including the ability to create many product copies from a single design and the ability to leverage scale to reduce overall product cost. Another advantage is the ability to use standardized parts during system operation and sustainment to reduce the cost of maintenance. While the variability between products is controlled during manufacturing, their use often varies, increasing the differences between products. If these differences are not accounted for, the variability decreases the ability to properly estimate product performance and plan maintenance cycles. Furthermore, specific products within a product family can be different, placing further stress on logistics for sustainment of these products. Digital twin technology has been developed in recent years to address the problems which arise from product and environmental differences (Tuegel et al. 2011 ). The digital twin concept includes constructing a digital representation or model of an individual product to improve the accuracy of maintenance and performance predictions for individual products (Kobryn 2020 ). Thus, a digital twin has been described as the model of a component, product, or system developed by a collection of engineering, operational, and behavioral data which support executable models, where the models evolve over the lifecycle of the system and support the derivation of solutions which assist the real time optimization of the system or service (Boschert and Rosen 2016 ).
Recently, this term has been extended to humans using the term “human digital twin.” This term has been applied in diverse fields, including medicine (Chakshu et al. 2019 ; Corral-Acero et al. 2020 ; Hirschvogel et al. 2019 ; Liu et al. 2019 ; Lutze 2020 ), sports performance (Barricelli et al. 2020 ), manufacturing ergonomics (Caputo et al. 2019 ; Greco et al. 2020 ; Sharotry et al. 2020 ), and product design (Demirel et al. 2021 )(Constantinescu et al. 2019 ; Demirel et al. 2021 ). Although the human digital twin concept may be analogous to digital twins of products, there are distinct differences, including increased underlying variability between humans and the fact that humans often employ products to achieve their goals. Commonalities can be observed in the use of the digital twin concept across the fields where it has been applied. For example, each of these fields discusses constructing and applying a model of humans which is informed by data collected from sensors which provide insight to human behavior and performance in real world settings. However, there are also differences. For example, in medicine or sports performance, the models often focus on improving the human or human performance while in manufacturing and product design, the model’s focus is on improving either the process or the artifacts with which the human interacts. As a result, the components which are modeled within each of these applications differ.
A clear example of a human digital twin from the literature pertains to manual material handling within manufacturing or warehousing applications (Greco et al. 2020 ; Sharotry et al. 2020 ). In this example, sensors are deployed with the human in their environment which monitor the human’s kinematic motion as they perform work within the environment. Other information, such as objects being lifted, or forces required to activate items within the environment are also collected. This data populates simulation models which estimate the fatigue in various muscle groups of the operator. These fatigue estimates support assessments and potential changes in work or rest schedules, handling processes, or material handling tools to improve overall worker health, safety, and productivity. Thus, a model of a human attribute is applied within a closed-loop system which fulfills the criteria for a digital twin (Boschert and Rosen 2016 ). While models of human behavior, performance, and state have been developed for decades, the key concept of human digital twins involves including these models within a tightly coupled, closed-loop system, where this system permits the model to evolve with data from a specific real-world human as they act within an environment and to provide feedback with the goal of enhancing the performance and well-being of the human to which it is coupled.
While the applications for human digital twin technologies differ, advances in one field may inform application of this technology in other fields. In fact, it is common for models which were developed to understand human performance for medical or sports applications to inform other applications, such as product design. Therefore, the current research seeks to leverage a review of existing literature regarding digital twins, and more specifically human digital twins, to derive a common definition of the human digital twin, a taxonomy of the components useful for construction of the human digital twin, and use cases of this technology within product development and sustainment across the system lifecycle. The overall goal of this paper is to support the development of this technology by proposing common terminology and a vision for applying this technology to support product development and deployment, with an emphasis on product development and deployment within the United States Department of Defense acquisition system. It is hoped that socialization of a common definition and potential applications of this technology within the human factors, ergonomics, and human systems integration communities will lead to synergistic efforts which improve the development of this technology.
The current research consisted of (1) conducting a review of literature pertaining to human digital twins, (2) developing a structural model of a human digital twin based upon the components of human digital twins from the literature, (3) applying this literature to derive an inclusive definition, and (4) synthesizing and developing use case models based upon potential applications inferred by the literature.
2.1 Literature review
The literature search included a Google Scholar search on the term “human digital twin” which was complemented by a search on “digital twin” and either “human,” “man,” or “woman.” Each search was limited to dates between 2011 and July 2021. The search on human digital twin returned 99 articles while the complementary search returned 15,000 results. Each of the human digital twin and the abstracts of the first 1000 articles on digital twin was then reviewed. As the purpose of this paper was predominantly to understand the structure and application of human digital twins to system design, development, and operation, the abstract review determined if the articles discussed the creation or application of digital twins which included a model of a human as part of the digital twin. A total of 54 unique papers which included a model of a human either as the digital twin or as a component of a digital twin were identified. For example, a model of a human may represent an athlete where performance is affected by nutrition, rest, and prescribed physical activity while a model with a human as a component may include a workstation in a factory where performance is influenced by factors such as nutrition, rest, and activity of the human but also by attributes of the system in which they are performing, such as material handling equipment and procedures.
Tables 1 and 2 show the application space and the publication year for the resulting papers. As shown, most of the papers either pertained to the manufacturing or medical domains. Four additional papers were focused specifically on understanding and modeling human biomechanics, predominantly with a focus on reducing musculoskeletal injuries in the workplace. Four additional papers focused on health and fitness applications, primarily for sports training. The remaining papers appeared to uniquely address five additional application areas, including general product design (Demirel et al. 2021 ), military application (Li et al. 2020 ), disaster management (Fan et al. 2021 ), organization design (Parmar et al. 2020 ), and identity management (Zibuschka et al. 2020 ).
As shown in Table 2 , practically all the publications appeared within the past 4 years. The earliest paper does not mention the words human digital twin, but discusses the construction of a digital athlete, which is updated based upon sensed information from the application environment (Alderson and Johnson 2016 ). The earliest papers using the term “Digital Twin” while referring to human models were published in 2018 with one of these papers appearing in the medical literature (Bruynseels et al. 2018 ) and three appearing within the manufacturing literature (Graessler and Poehler 2018a , 2018b ; Kemény et al. 2018 ).
2.2 Modeling structure and use cases
The structure and use case models of human digital twins were created using the Systems Modeling Language (SysML). This language provides a standardized language and family of diagrams for representing systems during design and development. The SysML diagrams include diagrams for representing system requirements (i.e., requirements diagram), structure (i.e., block definition and internal block diagrams), and behavior (i.e., use case, activity, state machine, and sequence diagrams) (Delligatti 2013 ; Friedenthal et al. 2014 ). Diagrams are also provided for structuring information (i.e., package diagrams) and supporting mathematical modeling (i.e., parametric diagram).
SysML was chosen to represent aspects of human digital twins as the digital twin concept has been described as a method to extend Model-Based Systems Engineering (MBSE) from the design to the operation and sustainment phase of a product (Boschert and Rosen 2016 ) and SysML has been developed to support MBSE. Furthermore, this language is an object-based language which permits the description of a generic system, sometimes through a reference architecture, and the extension of this description to specific instances or instantiations (King et al. 2020 ) and has been used to model the human’s contribution to system performance (M. Watson et al. 2017a , b ; M. E. Watson et al. 2017a , b ). Thus, the generic system description provided through the diagrams in this paper can be extended to illustrate the application of human digital twins in specific applications or instances.
Each of the articles pertaining to human digital twins were analyzed to determine the structural components and use cases they discussed. These elements were then used to compose a SysML block definition diagram to illustrate the infrastructure for a human digital twin. Furthermore, additional components were added based upon additional functions which were implied by the discussion of human digital twins. Similarly, use cases were extracted from each of the articles from the literature review. These use cases were interpreted with respect to product design and application within the United States Department of Defense (DoD). Separate use case diagrams were then developed for the application of knowledge gained from human digital twins to product design and operation. While the use cases are specific to the DoD, which acquires products for its own use, many of these use cases can be generalized to other organizations where the organization responsible for product acquisition and the organization employing the product may differ. Overall, these diagrams define the general components and product development-oriented use cases of human digital twins.
3 Structural definition of a human digital twin
As shown in the block definition diagram of Fig. 1 , digital twin systems typically include a real-world entity, i.e., the real-world twin, and a digital twin where the digital twin is a representation of one or more attributes of the real-world twin. The real-world and digital twins are linked through an interchange component such that changes in one twin can produce changes in the other (Barricelli et al. 2020 ; Grieves 2015 ; Latif and Shao 2020 ; Mendi et al. 2021 ; Sharotry et al. 2020 ; van der Valk et al. 2020 ).
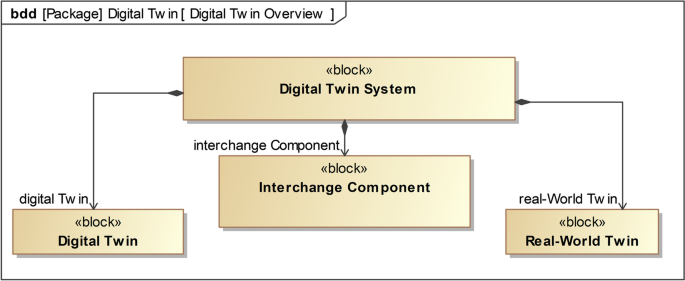
Block definition diagram showing primary components of a digital twin system
This interchange component permits the digital twin to be updated as the real-world entity acts within its environment. The digital twin then executes embedded models which are informed by the data it receives from the real-world twin to simulate one or more aspects (e.g., structure or behavior) of the real-world twin with the goal of determining changes in the real-world twin which might produce an improvement in the real-world twin.
To illustrate these components, it is useful to review examples. In the medical field, Chakshu and colleagues envision a human digital twin system in which the real-world human twin is outfit with portable sensors to characterize hemodynamic pressure as a function of time in the limbs of a patient (Chakshu et al. 2021 ). As this data is collected, it is passed to a model which uses these values together with other characteristics of the patient to estimate the pressure at other points within the circulatory system. These estimates are used to detect the presence or changes in an abdominal aortic aneurism. This system includes predominantly the human with sensors, a data processing system which collects the data and updates the model, and the model and prediction systems which compose the digital twin. However, the authors constrain the environment of use to clinical settings in which the patient is at rest.
Sharotry and colleagues discuss a digital twin system in a manufacturing environment which consists of a human conducting material handling tasks (Sharotry et al. 2020 ). The system includes a data collection module which includes motion capture, biometric suites among other sensors, a data analysis and forecasting model, and a database which contains the data which is collected for analysis. In this system, performance and operator fatigue metrics are provided to the individuals in the environment to support improved manufacturing performance and to help reduce human injury. Furthermore, this model can be used to identify material handling steps which induce substantial fatigue, permitting these steps to be evaluated and redesigned. Within this example, the real-world twin includes the manufacturing environment, the human, and the data collection subsystems. The database provides the interchange component, and the data analysis and forecasting model provides the digital representation or the digital twin.
As illustrated by these examples, human analysts apply the digital twin to conduct analysis, develop courses of action, and then apply the courses of action in the real world. The courses of action identify potentially useful changes in the structure or behavior of the real-world twin. In these systems, the analyst or the interchange component then causes changes in the real-world twin, which ideally improves the performance of this entity. Importantly, the two-way exchange between the digital and real-world twins provides the digital twin the ability to sense the real world, creates an understanding of the world, and acts upon it, completing a process analogous to the perceptual loop (Neisser 2014 ). However, in a digital twin system, this sequence includes a repetitive sequence with the following stages:
Sensors on the real-world twin sense the actions and performance of the real-world twin within its real-world environment, as well as relevant state information about the real world.
The interchange component conveys the sensed data to the digital twin.
An analysis is performed to determine whether the data is consistent with the digital twin’s current model projections and adjusts or builds a model to explain any differences.
The model is applied to create projections of future behavior within a virtual environment.
The projection of future behavior is compared to a goal state.
Based on this analysis, the system determines if a modification to the structure or behavior of the system is likely to move the system towards a goal state, and if so, selects a modification to the physical element or its behavior which moves the real-world twin towards the goal state.
The modification is conveyed to the real-world twin and applied to the real-world twin.
This series of steps is repeated, adjusting the digital models in the digital twin and the real-world twin to move the system towards its goal state. Ideally, the coupling of the digital and real-world twins by the interchange component provides the ability to rapidly create robust models of real-world performance, permit these models to be applied to project future real-world performance, and to improve the performance of the real-world system.
To create accurate predictions, the models represent not only the real-world entity but the environment in which the real-world twin is acting. This fact is not evident in much of the literature as the digital twin concept is applied within controlled environments. Thus, these systems were assumed to be closed; that is, they do not interact significantly with their environment. However, systems are often open, readily exchanging information, energy, and matter with their environment (Blanchard and Fabrycky 2006 ). Thus, it becomes important to model the environment to understand the entity’s interface with the environment. Specifically, the interface between these components require understanding of the spatial configuration, energy, information, and material transfer between the environment and the system (Jain et al. 2010 ). However, from an architectural standpoint, the environmental model does not necessarily need to be part of the digital twin. Instead, the environmental model may be separated from the digital twin system and simply be associated with this system. This is a significant architectural decision as the human digital twin system will not necessarily require a broad model of the environment but only requires models which reflect the information, energy, and matter that the system exchanges with the environment. Therefore, the complexity of the environmental model may be constrained if it is constructed to capture the necessary elements as opposed to the creation of a more generic and encompassing model of the environment. The real-world twin must also determine the state of the environment and communicate this state to the digital twin.
Reviewing the literature, different components of the digital twin system are illustrated by different authors. Therefore, it is possible to integrate the concepts across these papers to provide an architectural view of a generic digital twin. Figure 2 shows a more detailed block definition diagram based upon an analysis of the digital twin systems in the literature. As shown earlier, this system is comprised of a real-world twin, a digital twin, and an interchange component. However, alluded to before, the real world and a representation referred to as the virtual world are each associated with this system, where these two entities are linked by their state information.
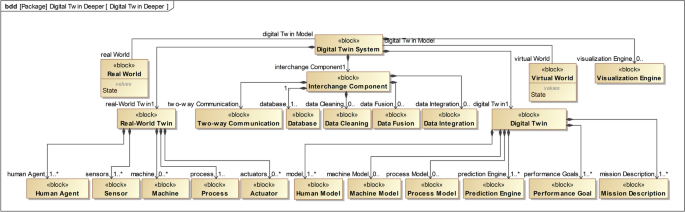
Block definition diagram showing an exploded view of a generic human digital twin system
Within the literature, the real-world twin includes a human within the environment. Sensors are used to provide real-time or near real-time information about the human and the environment (Corral-Acero et al. 2020 ; Sharotry et al. 2020 ) and this real-time feedback may be augmented with non-real time feedback, such as medical diagnoses, professional electro-cardiograms, or information from imaging devices (Corral-Acero et al. 2020 ). Additionally, the human can be queried to input subjective information (e.g., mood) or information which is difficult to track (e.g., nutrition information) into tracking applications (Barricelli et al. 2020 ). In manufacturing and product design scenarios, the human also interacts with physical devices (i.e., system), such as manufacturing equipment, material to be handled, or products which can be instrumented (Barricelli et al. 2020 ). Additionally, the real-world twin typically includes a process or procedure (Latif and Shao 2020 ). Although the process is not a physical item, it is important in understanding the behavior of the human. Finally, while not discussed explicitly in the literature, it may also be important for some mechanism to be present to modify the physical environment. The processes may be changed but to change the physical environment, it is necessary to include physical mechanisms, shown as actuators in Fig. 2 . As shown, near the head of the arrows associated with these entities, the human digital twin systems, by definition, include a human, one or more sensors, and at least 1 process. The machine and actuators are optional components as they do not exist in all systems, including the sports systems.
Of the three components, the interchange component receives the least attention within most of the published literature. Although the literature is clear that this interchange component permits two-way communication between the real-world entity and the digital entity, its components are not clearly discussed. However, beyond communications, this component can be envisioned as the warehouse for data or the database, although the database is sometimes viewed as part of the digital twin (Latif and Shao 2020 ). Furthermore, this component can be responsible for analysis to clean the data, fusing information from the various sensors associated with the real-world twin and integration of new data with the existing data (Tao et al. 2019a ).
Finally, we can review the components of the digital twin within the digital twin system. The digital twin certainly contains a model of the human. This model can include mechanistic models, which model well understood physical, chemical, biological, and physiological processes, as well as, statistical models which rely upon the data collected from the real-world twin (Corral-Acero et al. 2020 ). It can optionally contain additional models of the machine or the process which is to be performed (Latif and Shao 2020 ; Tao et al. 2019a ). Also necessary is a prediction engine which generates perturbations to the virtual world, human, machine, or process to understand how changes in these elements affect the performance of the human or the system (Tao et al. 2019a ). To assess the outcomes, a performance goal is also required such that the results produced by the prediction engine can be assessed. A mission description is also likely necessary which informs the process model of potential process steps which are considered viable.
Although not shown in Fig. 2 , it is also common for a process manager to interact with the digital twin to determine if any of the modifications evaluated by the prediction engine should be applied to the real-world twin. While these modifications may be communicated through the interchange component to the real-world twin, physical changes to the real-world twin may require human intervention.
It is worth noting that during design, the real-world twin may be under development and therefore the real-world twin or even a prototype of the real-world twin may not exist to participate in the digital twin system. Under these circumstances, and perhaps others as well, it can become useful to include the visualization engine, as shown in Fig. 2 (Havard et al. 2019 ; Ma et al. 2019 ; Wang et al. 2021 ). This visualization engine can provide a virtual or augmented reality rendition of the digital twin in the virtual or real worlds which a designer or potential user can interact with to improve insights and understanding until the real-world twin is available.
4 Defining human digital twin and human digital twin systems
Based upon the prior discussion, we can attempt to define a human digital twin and a human digital twin system. We need to consider several aspects to define the human digital twin. It is clear based upon the literature that a human digital twin system (HDTS) should include a digital representation of a real-world human. This real-world human may be an individual, whom we wish to characterize and model or a human class, where this human class represents a group of humans with common traits, characteristics, behavior, etc. The human digital twin (HDT) is then the digital representation of the real-world human or real-world twin. The HDT may exist purely as a mathematical representation of the individual or class of individuals. Alternately, the HDT may exist as a virtual entity which can be rendered within a virtual or real-world system.
The digital representation can include both first principles models which are based on fundamental understanding and statistical models. Various attributes of one or more humans can be modeled (Alexander et al. 2020 ). These include attributes in at least one of the following categories:
Physical: including anthropometric attributes, biomechanics attributes, times required for task completion, eye movements, and injuries
Physiological: measures such as heart rate, heart rate variability, galvanic skin response, muscle tension, blood oxygen level, brain electrophysiologic signals, pupillometry, blink rates and timing, peripheral blood flow, and gastronomical activity. However, these are intended to correlate to higher level physiological measures such as fatigue, circadian cycle, alertness level, and activation or engagement level.
Perceptual performance: auditory sensitivity, ability to decipher speech in different languages, visual sensitivity, color sensitivity, contrast sensitivity, pressure sensitivity, pain thresholds, temperature sensitivity, etc.
Cognitive performance: knowledge, skills, abilities or aptitudes, workload level, situation awareness, decision-making abilities, intuitive/analytic bias, etc.
Personality characteristics: attributes such as personality type, propensity to trust, and propensity towards suspicion.
Emotional state: feelings experienced by the individual within their current circumstance, including levels of depression, and anxiety.
Ethical stance: representation of the individual’s belief system, including values, beliefs, and mores.
Behavior: actions taken by the individual to interact with the system. These actions may be influenced by any of the earlier attributes.
As such, we can define a human digital twin as an integrated model which facilitates the description, prediction, or visualization of one or more characteristics of a human or class of humans as they perform within a real-world environment. A human digital twin system is a pairing of a real-world human twin and a human digital twin which includes a model of physical, physiological, personality, perception, cognitive performance, emotion, or ethics of a human; where the real-world human and human digital twin are integrated such that a change in the real-world human or its digital representation produces change(s) in the other. This human digital representation likely includes both mechanistic and statistical models of one or more attributes related to one or more of the human defined properties.
5 Human digital twin systems use cases
Besides defining HDTS and investigating their structure, it is also useful to understand how they might be applied. Within the literature, use cases are discussed within the health industry where HDTS support well-being, health care, and development of medical devices (Laamarti et al. 2020). Additionally, use cases are discussed for the design and manufacturing of products, including design of human–machine interaction, as well as the development of product service and use (Ma et al. 2019 ).
For product development and application, use cases may be considered for different product lifecycle phases; that is, they may be useful during product design, verification and validation, manufacturing, and use. Although HDTS might be useful during any phase of the lifecycle, Fig. 3 and Fig. 4 depict the use cases which apply during product development or acquisition, and operations and sustainment, respectively.
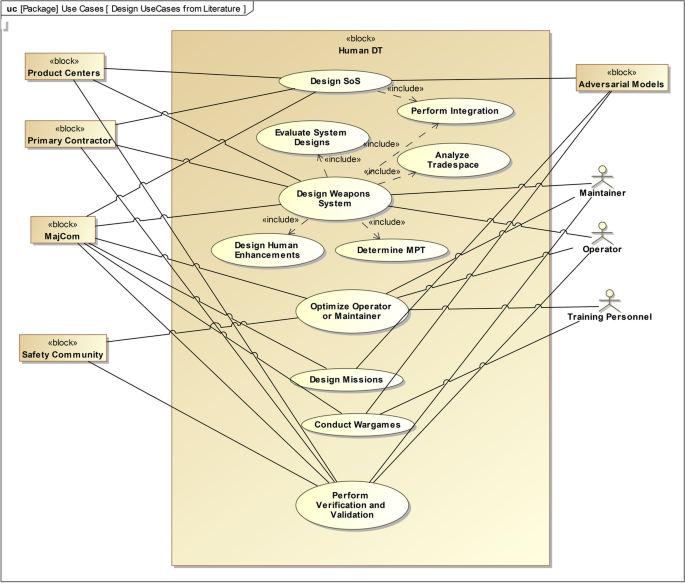
Example product development and acquisition-based use cases
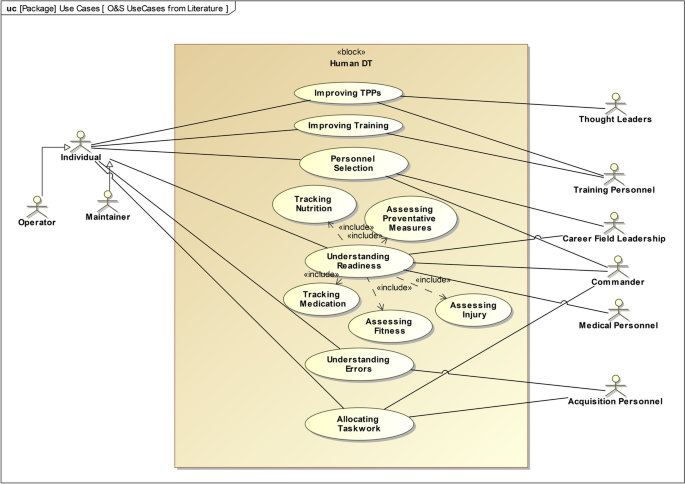
Operations and sustainment use cases imagined based upon literature
As Fig. 3 shows, during the product development and acquisition life cycle phase, HDTS can be used to aid the design of various portions of the system to be acquired. This might start with simulation of the human operating the product within the system of systems (SoS) to understand operational needs and the benefits of enhancements. As these needs are defined, modifications or new product concepts may be developed and evaluated (Laamarti 2019 ). In this use case, models which exist within the human digital twin can be applied early in system development to evaluate the utility of various designs and to analyze the impact of these differences through trade space analysis (Tao et al. 2019b ). This might include evaluation and understanding of various manpower, personnel, and training options, as well as options regarding the performance or other attributes of the system which impact important emergent behaviors, i.e., “ilities” of the system. Furthermore, these models might consider modifications to the user interface, items which enhance human physical or cognitive performance, and personal protective equipment (PPE) to prevent performance degradation. Perhaps, some models might be applied to understand the tradeoffs in operator or maintenance procedures to aid mission design or to optimize the performance of operators or maintainers within the system. As further indicated in this diagram, these models and HDTS will likely require sharing and collaboration among different communities which interact during product design and acquisition.
It should be noted that early in a development cycle of a novel product, the real-world portion of the HDTS will undergo design, and therefore, the real-world system or representative, trained operators may not exist. Thus, a representative real-world system will not be available. During these portions of the project, the digital portion of the HDTS will be required to operate in an open loop fashion. However, as prototypes of portions of the system are developed and early proxies for system operators and maintainers are used to participate in demonstrations, simulations, or evaluations, data from these events can be used to begin to close the loop from the real-world to the digital models for the system and the humans. As this data is included, the level of model fidelity and user confidence in these models should improve. Besides changes in model fidelity, the level of detail represented by the models is likely to change as one progresses through the system development and acquisition process from early needs development through acquisition. Similar models can also be applied during development of the manufacturing and logistics processes to affect human interaction as the real-world twin is developed.
As the system enters operations and sustainment, the use cases depicted in Fig. 4 become relevant. In this phase, the focus of HDTS shift from focusing on changes to the material components of the system to focusing on changes in the tactics, techniques, and procedures (TTPs) which are employed when applying the system. Similarly, these models may be used to evaluate and improve training or personnel selection methods (Ma et al. 2019 ). Additionally, the human digital twin may be used to understand readiness of personnel who interact with the systems or understand the errors or circumstances in which errors are made (Tao et al. 2019b ). Finally, the HDTS may be applied in real time to determine and allocate taskwork among team members (Ma et al. 2019 ). The corresponding use cases are shown in Fig. 4 .
As shown in Fig. 4 , as the HDTS is deployed with the system to the field, we expect that the operators will develop and employ new or revised TTPs which increase the utility and performance of the product (Cox and Szajnfarber 2017 ). The resulting innovations help to redefine taskwork and improve training. Furthermore, the models themselves may be used to explore changes to taskwork beyond those captured from observing user behavior, as observed in the manufacturing-oriented literature. Additionally, the HDTSs may be employed to analyze and understand human errors, which result in either near misses or mishaps to suggest changes in TTPs, training, or taskwork to improve safety. Furthermore, utilization of personal protective equipment, as well as environmental exposure to information, energy, or matter (e.g., radiation or known carcinogens), may be monitored to support occupational health interventions. Furthermore, the HDT may be capable of understanding attributes of the humans which enhance performance, aiding refinement of personnel selection.
Although TTPs, training, and personnel selection may all improve system performance, perhaps the greatest opportunity is understanding human readiness. However, this use case likely diverges significantly from the human digital twins that would be useful during system development. In fact, this use case likely relies upon significant interaction with the management and medical personnel who are not as likely to interact with the other use cases illustrated in Fig. 4 . Although not apparent from our earlier discussion, the HDT applied in medical and sports performance focus on understanding performance of systems which are internal to the human while HDT applied in product design or manufacturing tend to focus on human behavior and the interaction of the human with systems and the environment. As such, there is a basis for arguing that the HDT used for understanding readiness may be unique from the HDT used for practically all other use cases shown in Fig. 3 and Fig. 4 . Nevertheless, each of these HDTs relies on input from the same real-world humans and the HDT models within these two areas certainly interact. Therefore, a HDT developed to support readiness and HDT developed for other use cases will likely require some level of integration. Thus, there will be a need to integrate models of the human which are intended to understand health or health related human performance with models of the human which are intended to understand their performance when interacting with systems within an environment.
6 Summary and conclusion
This paper sought to define a common structure, definition, and set of use cases for HDTS as they pertain to systems, generally, and to product development, specifically. As discussed, the HDTS terminology has evolved in the past few years and is discussed predominantly in research papers related to medical and manufacturing systems, although other applications including fitness, product design, and military, among others, are present within this literature.
This research provides a definition, structure, and use cases for these systems, as defined within the reviewed literature. However, the development of HDTS as defined in this paper are relatively new, and therefore, the definitions, structure, and use cases are likely to evolve as these systems are employed and used. While an effort was made to capture the structure and behavior of these systems within SysML models and then to integrate these models, these activities required interpretation by the authors. Therefore, these models may not always represent the intent of the authors of the original articles. For each of these reasons, it is hoped that the definition, structure, and use cases of HDTS provided in this paper will provide an integrated view from which these definitions can evolve.
As shown, the HDTS include a real-world twin, a digital twin, and an interchange component which facilitates real-time monitoring of the real-world human as they perform in an environment using the system or product to provide robust models and predictions which facilitate system improvements. HDTS rely upon recent advances in low-cost sensors, data storage, and data mining technologies, together with the ongoing conversion to digital engineering as components. The integration of these components facilitates feedback loops which support improvements both in the design of products or systems for improved performance in real-world settings as well as improvements in TTPs and training to enhance user performance during product or system operations and sustainment.
Successful implementation of HDTS will require the development of robust models of various aspects of the human at different scales for different applications. These models will need to address aspects of the human to include physical, physiological, perceptual, cognitive, behavioral, emotional, personality, and ethical structure and performance. These models will need to describe and account for the variability among and uniqueness of individuals to be suitable for application within HDTS. The challenge, however, is the fact that component models which address, typically narrowly defined, aspects of the human are being developed within several disciplines for disparate applications as illustrated by the literature review. To accelerate the deployment of this technology, a concerted, multi-disciplinary, effort will be required to understand, define, and synthesize these models into HDTS applications.
Alderson J, Johnson W (2016) THE PERSONALISED “DIGITAL ATHLETE”: an evolving vision for the capture, modelling and simulation, of on-field athletic performance. 34th International Conference on Biomechanics in Sports, 23–26
Alexander T, Huiskamp W, Fromm L, Lewis MD, DiMatteo R, Gunzelmann G, MGroarty C, Gallant S, Blais D, Genc I (2020) Reference architecture for human behaviour modelling (STO-TR-MSG-127). https://www.sto.nato.int/publications/STOTechnical Reports/Forms/Technical Report Document Set/docsethomepage.aspx?ID=4581&FolderCTID=0x0120D5200078F9E87043356C409A0D30823AFA16F6010066D541ED10A62C40B2AB0FEBE9841A61&List=92d5819c-e6ec-4241-aa4e-57bf918681b1&RootFolder= https://www.sto.nato.int/publications/STOTechnical Reports/STO-TR-MSG-127
Barricelli BR, Casiraghi E, Gliozzo J, Petrini A, Valtolina S (2020) Human digital twin for fitness management. IEEE Access 8:26637–26664. https://doi.org/10.1109/ACCESS.2020.2971576
Article Google Scholar
Blanchard BS, Fabrycky W (2006) Systems engineering and analysis (4th edn). Pearson Education, Inc.
Boschert S, Rosen R (2016) Digital twin - the simulation aspect. In P. Hehenberger & D. Bradley (Eds.), Mechatronic Futures: Challenges and Solutions for Mechatronic Systems and Their Designers (pp. 59–74). Springer Nature. https://doi.org/10.1007/978-3-319-32156-1_1
Bruynseels K, de Sio FS, van den Hoven J (2018) Digital twins in health care: ethical implications of an emerging engineering paradigm. Front Genet 9(FEB):1–11. https://doi.org/10.3389/fgene.2018.00031
Caputo F, Greco A, Fera M, Macchiaroli R (2019) Digital twins to enhance the integration of ergonomics in the workplace design. Int J Ind Ergon 71(January):20–31. https://doi.org/10.1016/j.ergon.2019.02.001
Chakshu NK, Carson J, Sazonov I, Nithiarasu P (2019) A semi-active human digital twin model for detecting severity of carotid stenoses from head vibration—a coupled computational mechanics and computer vision method. Int J Numer Methods Biomed Eng 35(5):1–17. https://doi.org/10.1002/cnm.3180
Chakshu NK, Sazonov I, Nithiarasu P (2021) Towards enabling a cardiovascular digital twin for human systemic circulation using inverse analysis. Biomech Model Mechanobiol 20(2):449–465. https://doi.org/10.1007/s10237-020-01393-6
Constantinescu C, Rus R, Rusu CA, Popescu D (2019) Digital twins of exoskeleton-centered workplaces: challenges and development methodology. Procedia Manuf 39:58–65. https://doi.org/10.1016/j.promfg.2020.01.228
Corral-Acero J, Margara F, Marciniak M, Rodero C, Loncaric F, Feng Y, Gilbert A, Fernandes JF, Bukhari HA, Wajdan A, Martinez MV, Santos MS, Shamohammdi M, Luo H, Westphal P, Leeson P, DiAchille P, Gurev V, Mayr M, … Lamata P (2020) The “Digital Twin” to enable the vision of precision cardiology. European Heart Journal, 41(48), 4556-4564B. https://doi.org/10.1093/eurheartj/ehaa159
Cox A, Szajnfarber Z (2017) System user pathways to change. In Madni AM, Boehm B, Wrwin DA, Ghanem R, Wheaton MJ (eds) 15th Annual conference on systems engineering research disciplinary convergence: implications for systems engineering research, pp 1–9
Delligatti L (2013) SysML distilled: a brief guide to the systems modeling language. Addison-Wesley
Google Scholar
Demirel HO, Irshad L, Ahmed S, Tumer IY (2021) Digital twin-driven human-centered design frameworks for meeting sustainability objectives. J Comput Inf Sci Eng 21(3). https://doi.org/10.1115/1.4050684
Fan C, Zhang C, Yahja A, Mostafavi A (2021) Disaster city digital twin: a vision for integrating artificial and human intelligence for disaster management. Int J Inf Manag 56(2019):102049. https://doi.org/10.1016/j.ijinfomgt.2019.102049
Friedenthal S, Moore A, Steiner R (2014) A practical guide to SysML. Elseivier Science and Technology
Graessler I, Poehler A (2018a) Integration of a digital twin as human representation in a scheduling procedure of a cyber-physical production system. IEEE International Conference on Industrial Engineering and Engineering Management, 2017-Decem, 289–293. https://doi.org/10.1109/IEEM.2017.8289898
Graessler I, Poehler A (2018b) Intelligent control of an assembly station by integration of a digital twin for employees into the decentralized control system. Procedia Manuf 24:185–189. https://doi.org/10.1016/j.promfg.2018.06.041
Greco A, Caterino M, Fera M, Gerbino S (2020) Digital twin for monitoring ergonomics during manufacturing production. Appl Sci (Switzerland) 10(21):1–20. https://doi.org/10.3390/app10217758
Grieves M (2015) Digital twin: manufacturing excellence through virtual factory replication. In White paper. https://www.researchgate.net/publication/275211047_Digital_Twin_Manufacturing_Excellence_through_Virtual_Factory_Replication . Accessed Sept 2021.
Havard V, Jeanne B, Lacomblez M, Baudry D (2019) Digital twin and virtual reality: a co-simulation environment for design and assessment of industrial workstations. Prod Manuf Res 7(1):472–489. https://doi.org/10.1080/21693277.2019.1660283
Hirschvogel M, Jagschies L, Maier A, Wildhirt SM, Gee MW (2019) An in silico twin for epicardial augmentation of the failing heart. Int J Numer Methods Biomed Eng 35(10). https://doi.org/10.1002/cnm.3233
Jain R, Chandrasekaran A, Erol O (2010) A Framework for end-to-end approach to Systems Integration. Int J Ind Syst Eng 5(1):79–109. https://doi.org/10.1504/IJISE.2010.029763
Kemény Z, Beregi R, Nacsa J, Glawar R, Sihn W (2018) Expanding production perspectives by collaborating learning factories - perceived needs and possibilities. Procedia Manuf 23(2017):111–116. https://doi.org/10.1016/j.promfg.2018.04.002
King D, Jacques D, Gray J, Cheney K (2020) Design and simulation of a wide area search mission: an implementation of an autonomous systems reference architecture. In K. H. Bae, F. S. Kim, L.-M. S., Z. Zheng, T. Roeder, & R. Thiesing (Eds.), Proceedings of the 2020 Winter Simulation Conference (pp. 540–551). IEEE. https://ieeexplore.ieee.org/stamp/stamp.jsp?arnumber=9384112&casa_token=g_CYe3wx3K8AAAAA:9HIoCIeYeomnP1HptMU3kD-oI18O2W8jQPGmWXOICMTCMhhAvjAeLjYNoX0nrlTOzCPV1J-_qug&tag=1
Kobryn PA (2020) The digital twin concept. In Frontiers of Engineering: Reports on Leading-Edge Engineering from the 2019 Symposium (pp. 17–24). National Academy of Engineering. https://doi.org/10.17226/25620
Laamarti F (2019) Towards standardized digital twins for health, sport, and well-being. University of Ottawa. https://doi.org/10.20381/ruor-23746
Laamarti F, Badawi HF, Ding Y, Arafsha F, Hafidh B, Saddik AEl (2020) An ISO/IEEE 11073 standardized digital twin framework for health and well-being in smart cities. IEEE Access 8:105950–105961. https://doi.org/10.1109/ACCESS.2020.2999871
Latif H, Shao G (2020) A case study of digital twins for a manufacturing process involving human interactions. In R. Bae, K.H., Feng, B., Kim, S., Lazarova-Molnar, S., Zheng, Z., Roeder, T. and Thiesing (Ed.), Proceedings of the 2020 Winter Simulation Conference (pp. 2659–2670). http://repositorio.unan.edu.ni/2986/1/5624.pdf
Li S, Yang Q, Xing J, Yuan S (2020) Preliminary study on the application of digital twin in military engineering and equipment. Proceedings - 2020 Chinese Automation Congress, CAC 2020, 7249–7255. https://doi.org/10.1109/CAC51589.2020.9326911
Liu Y, Zhang L, Yang Y, Zhou L, Ren L, Wang F, Liu R, Pang Z, Deen MJ (2019) A novel cloud-based framework for the elderly healthcare services using digital twin. IEEE Access 7:49088–49101. https://doi.org/10.1109/ACCESS.2019.2909828
Lutze R (2020) Digital twin based software design in ehealth - a new development approach for health/ medical software products. Proceedings - 2020 IEEE International Conference on Engineering, Technology and Innovation, ICE/ITMC 2020. https://doi.org/10.1109/ICE/ITMC49519.2020.9198546
Ma X, Tao F, Zhang M, Wang T, Zuo Y (2019) Digital twin enhanced human-machine interaction in product lifecycle. Procedia CIRP 83:789–793. https://doi.org/10.1016/j.procir.2019.04.330
Mendi AF, Erol T, Dogan D (2021) Digital twin in the military field. IEEE Internet Computing 7801. https://doi.org/10.1109/mic.2021.3055153
Neisser U (2014) Cognitive psychology: classic edition. In Cognitive Psychology (Classic). Psychology Press
Parmar R, Leiponen A, Thomas LDW (2020) Building an organizational digital twin. Bus Horiz 63(6):725–736. https://doi.org/10.1016/j.bushor.2020.08.001
Sharotry A, Jimenez JA, Wierschem D, Koldenhoven RM, Valles D, Koutitas G, Aslan S (2020) A digital twin framework for real-time analysis and feedback of repetitive work in the manual material handling industry. In R. Bae, K.H., Feng, B., Kim, S., Lazarova-Molnar, S., Zheng, Z., Roeder, T. and Thiesing (Ed.), Proc. of the 2020 Winter Simulation Conference (pp. 2637–2648)
Tao F, Sui F, Liu A, Qi Q, Zhang M, Song B, Guo Z, Lu SCY, Nee AYC (2019a) Digital twin-driven product design framework. Int J Prod Res 57(12):3935–3953. https://doi.org/10.1080/00207543.2018.1443229
Tao F, Zhang H, Liu A, Nee AYC (2019b) Digital twin in industry: state-of-the-art. IEEE Trans Industr Inf 15(4):2405–2415. https://doi.org/10.1109/TII.2018.2873186
Tuegel EJ, Ingraffea AR, Eason TG, Spottswood SM (2011) Reengineering aircraft structural life prediction using a digital twin. Int J Aerospace Eng 2011. https://doi.org/10.1155/2011/154798
van der Valk H, Hunker J, Rabe M (2020) Digital twins in simulative applications: a taxonomy. In Bae R, Feng KH, Kim B, Lazarova-Molnar S, Zheng S, Roeder Z, Thiesing T (eds) Proc. of the 2020 Winter Simulation Conference, pp 2695–2706
Wang Q, Jiao W, Wang P, Zhang Y (2021) Digital twin for human-robot interactive welding and welder behavior analysis. IEEE/CAA J Automatica Sinica 8(2):334–343. https://doi.org/10.1109/JAS.2020.1003518
Watson ME, Rusnock CF, Miller ME, Colombi JM (2017a) Informing system design using human performance modeling. Syst Eng 20(2):173–187. https://doi.org/10.1002/sys
Watson M, Rusnock CF, Colombi JM, Miller ME (2017b) Human-centered design using system modeling language. J Cogn Eng Decis Making 11(3):252–269. https://doi.org/10.1177/1555343417705255
Zibuschka J, Ruff C, Horch A, Roßnagel H (2020) A human digital twin as building block of open identity management for the internet of things. Lecture Notes in Informatics (LNI), Proceedings - Series of the Gesellschaft Fur Informatik (GI), P-305, 133–142
Download references
Partial financial support was received from the Air Force Research Laboratories.
Author information
Authors and affiliations.
Department of Systems Engineering and Management, Air Force Institute of Technology, 2950 Hobson Way (AFIT/ENV), Wright Patterson AFB, OH, 45433, USA
Michael E. Miller
Defense and Intel, KBR Government Solutions – U.S., 3725 Pentagon Blvd Suite #210, Beavercreek, OH, 45431, USA
Emily Spatz
You can also search for this author in PubMed Google Scholar
Contributions
Conceptualization: MEM; methodology: MEM; formal analysis and investigation: MEM, ET; writing—original draft preparation: MEM; writing—review and editing: ET; funding acquisition: MEM; resources: MEM; supervision: MEM.
Corresponding author
Correspondence to Michael E. Miller .
Ethics declarations
The views expressed in this article are those of the authors and do not necessarily reflect the official policy or position of the Department of the US Air Force, US Department of Defense, nor the US Government.
Ethics approval
Not applicable.
Consent to participate
Consent for publication.
This work was prepared by employees or contractors of the United States Government as part of their official duty, and thus, the work is not subject to copyright within the USA. The authors consent to publish this work.
Conflict of interest
The authors declare no competing interests.
Additional information
Publisher's note.
Springer Nature remains neutral with regard to jurisdictional claims in published maps and institutional affiliations.
Rights and permissions
Open Access This article is licensed under a Creative Commons Attribution 4.0 International License, which permits use, sharing, adaptation, distribution and reproduction in any medium or format, as long as you give appropriate credit to the original author(s) and the source, provide a link to the Creative Commons licence, and indicate if changes were made. The images or other third party material in this article are included in the article's Creative Commons licence, unless indicated otherwise in a credit line to the material. If material is not included in the article's Creative Commons licence and your intended use is not permitted by statutory regulation or exceeds the permitted use, you will need to obtain permission directly from the copyright holder. To view a copy of this licence, visit http://creativecommons.org/licenses/by/4.0/ .
Reprints and permissions
About this article
Miller, M.E., Spatz, E. A unified view of a human digital twin. Hum.-Intell. Syst. Integr. 4 , 23–33 (2022). https://doi.org/10.1007/s42454-022-00041-x
Download citation
Received : 16 October 2021
Accepted : 30 January 2022
Published : 12 March 2022
Issue Date : June 2022
DOI : https://doi.org/10.1007/s42454-022-00041-x
Share this article
Anyone you share the following link with will be able to read this content:
Sorry, a shareable link is not currently available for this article.
Provided by the Springer Nature SharedIt content-sharing initiative
- Human digital twin
- Model-based systems engineering
- Human performance
- Find a journal
- Publish with us
- Track your research
Subscribe to the PwC Newsletter
Join the community, edit social preview.

Add a new code entry for this paper
Remove a code repository from this paper, mark the official implementation from paper authors, add a new evaluation result row, remove a task, add a method, remove a method, edit datasets, aam-vdt: vehicle digital twin for tele-operations in advanced air mobility.
15 Apr 2024 · Tuan Anh Nguyen , Taeho Kwag , Vinh Pham , Viet Nghia Nguyen , Jeongseok Hyun , Minseok Jang , Jae-Woo Lee · Edit social preview
This study advanced tele-operations in Advanced Air Mobility (AAM) through the creation of a Vehicle Digital Twin (VDT) system for eVTOL aircraft, tailored to enhance remote control safety and efficiency, especially for Beyond Visual Line of Sight (BVLOS) operations. By synergizing digital twin technology with immersive Virtual Reality (VR) interfaces, we notably elevate situational awareness and control precision for remote operators. Our VDT framework integrates immersive tele-operation with a high-fidelity aerodynamic database, essential for authentically simulating flight dynamics and control tactics. At the heart of our methodology lies an eVTOL's high-fidelity digital replica, placed within a simulated reality that accurately reflects physical laws, enabling operators to manage the aircraft via a master-slave dynamic, substantially outperforming traditional 2D interfaces. The architecture of the designed system ensures seamless interaction between the operator, the digital twin, and the actual aircraft, facilitating exact, instantaneous feedback. Experimental assessments, involving propulsion data gathering, simulation database fidelity verification, and tele-operation testing, verify the system's capability in precise control command transmission and maintaining the digital-physical eVTOL synchronization. Our findings underscore the VDT system's potential in augmenting AAM efficiency and safety, paving the way for broader digital twin application in autonomous aerial vehicles.
Code Edit Add Remove Mark official
Tasks edit add remove, datasets edit, results from the paper edit add remove, methods edit add remove.
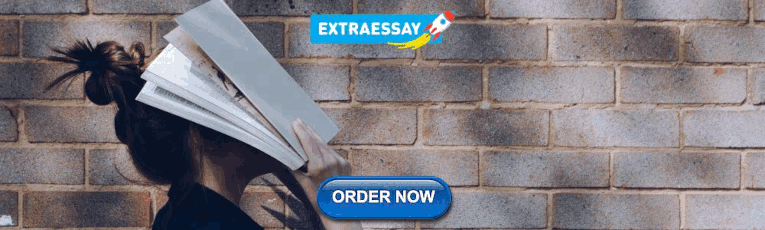
IMAGES
VIDEO
COMMENTS
The research started by developing key concepts based on a narrative literature review in the Scopus database and Google Scholar and searched for papers that included Digital Twin or Digital Twins in the title. Articles were selected from Journal articles, research articles, conference publications, research reports, or scientific encyclopedias.
Digital Twin technology is an emerging concept that has become the centre of attention for industry and, in more recent years, academia. The advancements in industry 4.0 concepts have facilitated its growth, particularly in the manufacturing industry. The Digital Twin is defined extensively but is best described as the effortless integration of data between a physical and virtual machine in ...
the many de nitions and characteristics of digital twin, several review papers have been reported in recent years, such asBarricelli et al.(2019);Jones et al.(2020);VanDerHorn and Mahadevan(2021); Liu et al.(2021b). These papers summarized vari-ous de nitions of digital twin and suggested gener-alized de nitions and characteristics to distinguish
2.1. The digital twin concept. The commonly agreed definition of a digital twin currently highlights two important features. First, a digital twin provides a connection between a physical entity and its virtual counterpart (He and Bai Citation 2021).Second, the connection between the physical entity and its virtual twin is established by sensors to provide real-time information (Tao et al ...
Health digital twins are defined as virtual representations ("digital twin") of patients ("physical twin") that are generated from multimodal patient data, population data, and real-time ...
As an emerging technology in the era of Industry 4.0, digital twin is gaining unprecedented attention because of its promise to further optimize process design, quality control, health monitoring, decision and policy making, and more, by comprehensively modeling the physical world as a group of interconnected digital models. In a two-part series of papers, we examine the fundamental role of ...
The digital twin revolution. Nature Computational Science 1 , 307-308 ( 2021) Cite this article. A uniform mathematical framework based on probabilistic graphical models drives the digital twin ...
tions of digital twin in construction engineering. Uhlemann et al. (2017) discussed the importance of digital twins in realizing cyber-physical production systems for Industry 4.0. While current review papers cover a good amount of lit-erature on different aspects of digital twin, such as defini-
Based on more refined and dynamic models as well as richer data drives, digital twins have played an important role in operation monitoring, simulation, optimization, predictive maintenance, and ...
In short, a digital twin (DT) is a virtual replica of a physical object, person, or process that can be used to increase understanding and perform tests. They are linked to real data sources in the environment and update in real time to reflect changes in the physical world. Here are two examples that can help clarify what this means.
The ever-growing interest in digital twin technology is visible in academia and industry through the increasing number of papers and patents employing the technology in different domains . Digital twin definition has evolved to reflect the advancements in the technology sector in general and in data communication, data acquisition, and the ...
Digital twin is a technology that integrates multi-physical, multi-scale, and multi-disciplinary attributes. At the same time, it has the characteristics of real-time synchronization, faithful mapping, and high fidelity. It can realize the interaction and integration of physical world and information world. In recent years, digital twin technology has attracted the attention of academic and ...
The digital twin, which can sense, diagnose and predict the state of physical entites in real time, regulate the behaviour of physical entites through collaboration and optimization decision making between twins, realize self evolution through self-learning across relevant digital models, and achieve the autonomous intelligent evolution of ...
The digital twin is ultra-realistic and may consider one or more important and interdependent vehicle systems." From the 21 papers analysed, 13 refer to this definition [24] [2] [15] [33] [31] ... of the digital twin when evaluating papers [28]. Nonetheless, when deciding if a study is a Digital Model, Digital
Very few papers address the 'true' digital twin, which, according to the authors, has an automated dataflow in both directions . The second most-cited review paper is 'A Review of the Roles of Digital Twin in CPS-based Production Systems" by Negri, Fumagalli, and Macchi, published in 2017, and cited 634 times . In this paper, a ...
Digital Twins-based practices in civil engineering provide a good conceptual framework, when analysing the impact of a data- and model-driven healthcare on concepts of health, disease, and enhancement. Unlike traditional engineering models, Digital Twins reflect the particular and individual, the idiosyncratic.
Digital twins provide a number of economic, health, social, and environmental benefits. Their value can be amplified by combining them with other technologies and tools. Published in: Computer ( Volume: 54 , Issue: 4 , April 2021 )
The digital twin concept was first proposed for the Apollo mission at the National Aeronautics and Space Administration (NASA) in the United States. Since then, manufacturing and healthcare have been using digital twins, i.e., mockups of physical objects created using real-time data, to test various what-if scenarios.
Recently, Digital Twin (DT) has a growth revolution by increasing Artificial Intelligence (AI) techniques and relative technologies as the Internet of Things (IoT). They may be considered as the panacea for DT technology for various applications in the real world such as manufacturing, healthcare, and smart cities. The integration of DT and AI is a new avenue for open research in the upcoming ...
Papers described only models or without applications were descripted in this review but excluded during eligibility screening and extraction. In general, the popularity of DT research in medicine has risen globally at an accelerating rate. ... Also, we restricted the search to articles that included the terms digital twin, healthcare or ...
Seeing is believing. Virtual twins enable the entire enterprise and value chain to focus on a common goal. More importantly, having a holistic, end-to-end view of a product can prevent many issues ...
The architecture of a digital twin can be represented as nodes and edges in a time-series graph, allowing graph-based LLMs (such as GNN-LM, TextGNN, GIANT, and SimTeG) to create a basic model of a twin, whose design can then be built upon and adapted for various scenarios and industries. 1 For example, in a future smart-city context, various ...
Digital Twin - Rise of New Era. The advancement in the technology and Internet has paved the way in bridging the digital and Physical world in Industrial 4.0. It is being echoed by many of the Industry leaders that this is going to Phase change the Manufacturing process which offers new ways to reduce costs, monitor assets, optimize ...
As shown in Table 2, practically all the publications appeared within the past 4 years.The earliest paper does not mention the words human digital twin, but discusses the construction of a digital athlete, which is updated based upon sensed information from the application environment (Alderson and Johnson 2016).The earliest papers using the term "Digital Twin" while referring to human ...
By synergizing digital twin technology with immersive Virtual Reality (VR) interfaces, we notably elevate situational awareness and control precision for remote operators. Our VDT framework integrates immersive tele-operation with a high-fidelity aerodynamic database, essential for authentically simulating flight dynamics and control tactics.