- Open access
- Published: 01 August 2019
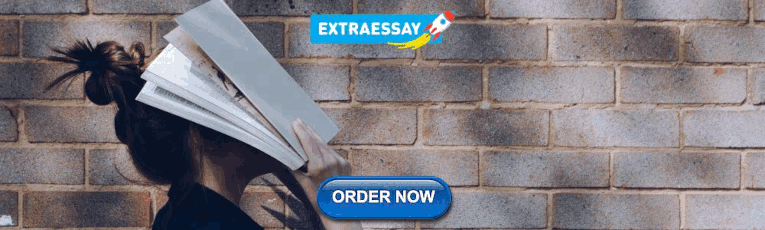
A step by step guide for conducting a systematic review and meta-analysis with simulation data
- Gehad Mohamed Tawfik 1 , 2 ,
- Kadek Agus Surya Dila 2 , 3 ,
- Muawia Yousif Fadlelmola Mohamed 2 , 4 ,
- Dao Ngoc Hien Tam 2 , 5 ,
- Nguyen Dang Kien 2 , 6 ,
- Ali Mahmoud Ahmed 2 , 7 &
- Nguyen Tien Huy 8 , 9 , 10
Tropical Medicine and Health volume 47 , Article number: 46 ( 2019 ) Cite this article
780k Accesses
286 Citations
93 Altmetric
Metrics details
The massive abundance of studies relating to tropical medicine and health has increased strikingly over the last few decades. In the field of tropical medicine and health, a well-conducted systematic review and meta-analysis (SR/MA) is considered a feasible solution for keeping clinicians abreast of current evidence-based medicine. Understanding of SR/MA steps is of paramount importance for its conduction. It is not easy to be done as there are obstacles that could face the researcher. To solve those hindrances, this methodology study aimed to provide a step-by-step approach mainly for beginners and junior researchers, in the field of tropical medicine and other health care fields, on how to properly conduct a SR/MA, in which all the steps here depicts our experience and expertise combined with the already well-known and accepted international guidance.
We suggest that all steps of SR/MA should be done independently by 2–3 reviewers’ discussion, to ensure data quality and accuracy.
SR/MA steps include the development of research question, forming criteria, search strategy, searching databases, protocol registration, title, abstract, full-text screening, manual searching, extracting data, quality assessment, data checking, statistical analysis, double data checking, and manuscript writing.
Introduction
The amount of studies published in the biomedical literature, especially tropical medicine and health, has increased strikingly over the last few decades. This massive abundance of literature makes clinical medicine increasingly complex, and knowledge from various researches is often needed to inform a particular clinical decision. However, available studies are often heterogeneous with regard to their design, operational quality, and subjects under study and may handle the research question in a different way, which adds to the complexity of evidence and conclusion synthesis [ 1 ].
Systematic review and meta-analyses (SR/MAs) have a high level of evidence as represented by the evidence-based pyramid. Therefore, a well-conducted SR/MA is considered a feasible solution in keeping health clinicians ahead regarding contemporary evidence-based medicine.
Differing from a systematic review, unsystematic narrative review tends to be descriptive, in which the authors select frequently articles based on their point of view which leads to its poor quality. A systematic review, on the other hand, is defined as a review using a systematic method to summarize evidence on questions with a detailed and comprehensive plan of study. Furthermore, despite the increasing guidelines for effectively conducting a systematic review, we found that basic steps often start from framing question, then identifying relevant work which consists of criteria development and search for articles, appraise the quality of included studies, summarize the evidence, and interpret the results [ 2 , 3 ]. However, those simple steps are not easy to be reached in reality. There are many troubles that a researcher could be struggled with which has no detailed indication.
Conducting a SR/MA in tropical medicine and health may be difficult especially for young researchers; therefore, understanding of its essential steps is crucial. It is not easy to be done as there are obstacles that could face the researcher. To solve those hindrances, we recommend a flow diagram (Fig. 1 ) which illustrates a detailed and step-by-step the stages for SR/MA studies. This methodology study aimed to provide a step-by-step approach mainly for beginners and junior researchers, in the field of tropical medicine and other health care fields, on how to properly and succinctly conduct a SR/MA; all the steps here depicts our experience and expertise combined with the already well known and accepted international guidance.
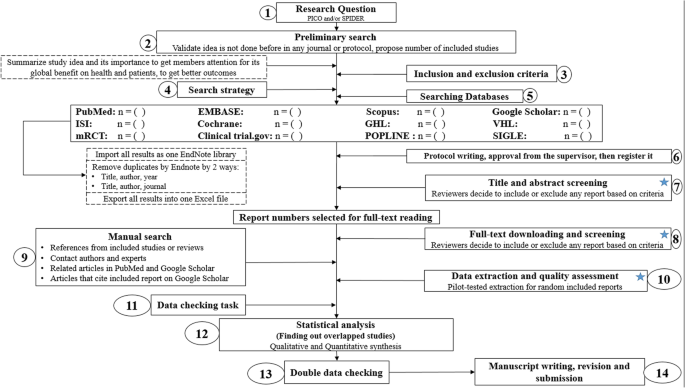
Detailed flow diagram guideline for systematic review and meta-analysis steps. Note : Star icon refers to “2–3 reviewers screen independently”
Methods and results
Detailed steps for conducting any systematic review and meta-analysis.
We searched the methods reported in published SR/MA in tropical medicine and other healthcare fields besides the published guidelines like Cochrane guidelines {Higgins, 2011 #7} [ 4 ] to collect the best low-bias method for each step of SR/MA conduction steps. Furthermore, we used guidelines that we apply in studies for all SR/MA steps. We combined these methods in order to conclude and conduct a detailed flow diagram that shows the SR/MA steps how being conducted.
Any SR/MA must follow the widely accepted Preferred Reporting Items for Systematic Review and Meta-analysis statement (PRISMA checklist 2009) (Additional file 5 : Table S1) [ 5 ].
We proposed our methods according to a valid explanatory simulation example choosing the topic of “evaluating safety of Ebola vaccine,” as it is known that Ebola is a very rare tropical disease but fatal. All the explained methods feature the standards followed internationally, with our compiled experience in the conduct of SR beside it, which we think proved some validity. This is a SR under conduct by a couple of researchers teaming in a research group, moreover, as the outbreak of Ebola which took place (2013–2016) in Africa resulted in a significant mortality and morbidity. Furthermore, since there are many published and ongoing trials assessing the safety of Ebola vaccines, we thought this would provide a great opportunity to tackle this hotly debated issue. Moreover, Ebola started to fire again and new fatal outbreak appeared in the Democratic Republic of Congo since August 2018, which caused infection to more than 1000 people according to the World Health Organization, and 629 people have been killed till now. Hence, it is considered the second worst Ebola outbreak, after the first one in West Africa in 2014 , which infected more than 26,000 and killed about 11,300 people along outbreak course.
Research question and objectives
Like other study designs, the research question of SR/MA should be feasible, interesting, novel, ethical, and relevant. Therefore, a clear, logical, and well-defined research question should be formulated. Usually, two common tools are used: PICO or SPIDER. PICO (Population, Intervention, Comparison, Outcome) is used mostly in quantitative evidence synthesis. Authors demonstrated that PICO holds more sensitivity than the more specific SPIDER approach [ 6 ]. SPIDER (Sample, Phenomenon of Interest, Design, Evaluation, Research type) was proposed as a method for qualitative and mixed methods search.
We here recommend a combined approach of using either one or both the SPIDER and PICO tools to retrieve a comprehensive search depending on time and resources limitations. When we apply this to our assumed research topic, being of qualitative nature, the use of SPIDER approach is more valid.
PICO is usually used for systematic review and meta-analysis of clinical trial study. For the observational study (without intervention or comparator), in many tropical and epidemiological questions, it is usually enough to use P (Patient) and O (outcome) only to formulate a research question. We must indicate clearly the population (P), then intervention (I) or exposure. Next, it is necessary to compare (C) the indicated intervention with other interventions, i.e., placebo. Finally, we need to clarify which are our relevant outcomes.
To facilitate comprehension, we choose the Ebola virus disease (EVD) as an example. Currently, the vaccine for EVD is being developed and under phase I, II, and III clinical trials; we want to know whether this vaccine is safe and can induce sufficient immunogenicity to the subjects.
An example of a research question for SR/MA based on PICO for this issue is as follows: How is the safety and immunogenicity of Ebola vaccine in human? (P: healthy subjects (human), I: vaccination, C: placebo, O: safety or adverse effects)
Preliminary research and idea validation
We recommend a preliminary search to identify relevant articles, ensure the validity of the proposed idea, avoid duplication of previously addressed questions, and assure that we have enough articles for conducting its analysis. Moreover, themes should focus on relevant and important health-care issues, consider global needs and values, reflect the current science, and be consistent with the adopted review methods. Gaining familiarity with a deep understanding of the study field through relevant videos and discussions is of paramount importance for better retrieval of results. If we ignore this step, our study could be canceled whenever we find out a similar study published before. This means we are wasting our time to deal with a problem that has been tackled for a long time.
To do this, we can start by doing a simple search in PubMed or Google Scholar with search terms Ebola AND vaccine. While doing this step, we identify a systematic review and meta-analysis of determinant factors influencing antibody response from vaccination of Ebola vaccine in non-human primate and human [ 7 ], which is a relevant paper to read to get a deeper insight and identify gaps for better formulation of our research question or purpose. We can still conduct systematic review and meta-analysis of Ebola vaccine because we evaluate safety as a different outcome and different population (only human).
Inclusion and exclusion criteria
Eligibility criteria are based on the PICO approach, study design, and date. Exclusion criteria mostly are unrelated, duplicated, unavailable full texts, or abstract-only papers. These exclusions should be stated in advance to refrain the researcher from bias. The inclusion criteria would be articles with the target patients, investigated interventions, or the comparison between two studied interventions. Briefly, it would be articles which contain information answering our research question. But the most important is that it should be clear and sufficient information, including positive or negative, to answer the question.
For the topic we have chosen, we can make inclusion criteria: (1) any clinical trial evaluating the safety of Ebola vaccine and (2) no restriction regarding country, patient age, race, gender, publication language, and date. Exclusion criteria are as follows: (1) study of Ebola vaccine in non-human subjects or in vitro studies; (2) study with data not reliably extracted, duplicate, or overlapping data; (3) abstract-only papers as preceding papers, conference, editorial, and author response theses and books; (4) articles without available full text available; and (5) case reports, case series, and systematic review studies. The PRISMA flow diagram template that is used in SR/MA studies can be found in Fig. 2 .
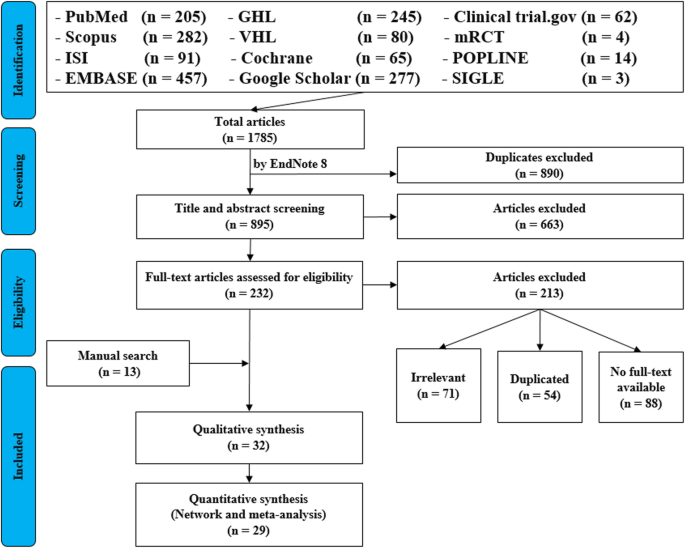
PRISMA flow diagram of studies’ screening and selection
Search strategy
A standard search strategy is used in PubMed, then later it is modified according to each specific database to get the best relevant results. The basic search strategy is built based on the research question formulation (i.e., PICO or PICOS). Search strategies are constructed to include free-text terms (e.g., in the title and abstract) and any appropriate subject indexing (e.g., MeSH) expected to retrieve eligible studies, with the help of an expert in the review topic field or an information specialist. Additionally, we advise not to use terms for the Outcomes as their inclusion might hinder the database being searched to retrieve eligible studies because the used outcome is not mentioned obviously in the articles.
The improvement of the search term is made while doing a trial search and looking for another relevant term within each concept from retrieved papers. To search for a clinical trial, we can use these descriptors in PubMed: “clinical trial”[Publication Type] OR “clinical trials as topic”[MeSH terms] OR “clinical trial”[All Fields]. After some rounds of trial and refinement of search term, we formulate the final search term for PubMed as follows: (ebola OR ebola virus OR ebola virus disease OR EVD) AND (vaccine OR vaccination OR vaccinated OR immunization) AND (“clinical trial”[Publication Type] OR “clinical trials as topic”[MeSH Terms] OR “clinical trial”[All Fields]). Because the study for this topic is limited, we do not include outcome term (safety and immunogenicity) in the search term to capture more studies.
Search databases, import all results to a library, and exporting to an excel sheet
According to the AMSTAR guidelines, at least two databases have to be searched in the SR/MA [ 8 ], but as you increase the number of searched databases, you get much yield and more accurate and comprehensive results. The ordering of the databases depends mostly on the review questions; being in a study of clinical trials, you will rely mostly on Cochrane, mRCTs, or International Clinical Trials Registry Platform (ICTRP). Here, we propose 12 databases (PubMed, Scopus, Web of Science, EMBASE, GHL, VHL, Cochrane, Google Scholar, Clinical trials.gov , mRCTs, POPLINE, and SIGLE), which help to cover almost all published articles in tropical medicine and other health-related fields. Among those databases, POPLINE focuses on reproductive health. Researchers should consider to choose relevant database according to the research topic. Some databases do not support the use of Boolean or quotation; otherwise, there are some databases that have special searching way. Therefore, we need to modify the initial search terms for each database to get appreciated results; therefore, manipulation guides for each online database searches are presented in Additional file 5 : Table S2. The detailed search strategy for each database is found in Additional file 5 : Table S3. The search term that we created in PubMed needs customization based on a specific characteristic of the database. An example for Google Scholar advanced search for our topic is as follows:
With all of the words: ebola virus
With at least one of the words: vaccine vaccination vaccinated immunization
Where my words occur: in the title of the article
With all of the words: EVD
Finally, all records are collected into one Endnote library in order to delete duplicates and then to it export into an excel sheet. Using remove duplicating function with two options is mandatory. All references which have (1) the same title and author, and published in the same year, and (2) the same title and author, and published in the same journal, would be deleted. References remaining after this step should be exported to an excel file with essential information for screening. These could be the authors’ names, publication year, journal, DOI, URL link, and abstract.
Protocol writing and registration
Protocol registration at an early stage guarantees transparency in the research process and protects from duplication problems. Besides, it is considered a documented proof of team plan of action, research question, eligibility criteria, intervention/exposure, quality assessment, and pre-analysis plan. It is recommended that researchers send it to the principal investigator (PI) to revise it, then upload it to registry sites. There are many registry sites available for SR/MA like those proposed by Cochrane and Campbell collaborations; however, we recommend registering the protocol into PROSPERO as it is easier. The layout of a protocol template, according to PROSPERO, can be found in Additional file 5 : File S1.
Title and abstract screening
Decisions to select retrieved articles for further assessment are based on eligibility criteria, to minimize the chance of including non-relevant articles. According to the Cochrane guidance, two reviewers are a must to do this step, but as for beginners and junior researchers, this might be tiresome; thus, we propose based on our experience that at least three reviewers should work independently to reduce the chance of error, particularly in teams with a large number of authors to add more scrutiny and ensure proper conduct. Mostly, the quality with three reviewers would be better than two, as two only would have different opinions from each other, so they cannot decide, while the third opinion is crucial. And here are some examples of systematic reviews which we conducted following the same strategy (by a different group of researchers in our research group) and published successfully, and they feature relevant ideas to tropical medicine and disease [ 9 , 10 , 11 ].
In this step, duplications will be removed manually whenever the reviewers find them out. When there is a doubt about an article decision, the team should be inclusive rather than exclusive, until the main leader or PI makes a decision after discussion and consensus. All excluded records should be given exclusion reasons.
Full text downloading and screening
Many search engines provide links for free to access full-text articles. In case not found, we can search in some research websites as ResearchGate, which offer an option of direct full-text request from authors. Additionally, exploring archives of wanted journals, or contacting PI to purchase it if available. Similarly, 2–3 reviewers work independently to decide about included full texts according to eligibility criteria, with reporting exclusion reasons of articles. In case any disagreement has occurred, the final decision has to be made by discussion.
Manual search
One has to exhaust all possibilities to reduce bias by performing an explicit hand-searching for retrieval of reports that may have been dropped from first search [ 12 ]. We apply five methods to make manual searching: searching references from included studies/reviews, contacting authors and experts, and looking at related articles/cited articles in PubMed and Google Scholar.
We describe here three consecutive methods to increase and refine the yield of manual searching: firstly, searching reference lists of included articles; secondly, performing what is known as citation tracking in which the reviewers track all the articles that cite each one of the included articles, and this might involve electronic searching of databases; and thirdly, similar to the citation tracking, we follow all “related to” or “similar” articles. Each of the abovementioned methods can be performed by 2–3 independent reviewers, and all the possible relevant article must undergo further scrutiny against the inclusion criteria, after following the same records yielded from electronic databases, i.e., title/abstract and full-text screening.
We propose an independent reviewing by assigning each member of the teams a “tag” and a distinct method, to compile all the results at the end for comparison of differences and discussion and to maximize the retrieval and minimize the bias. Similarly, the number of included articles has to be stated before addition to the overall included records.
Data extraction and quality assessment
This step entitles data collection from included full-texts in a structured extraction excel sheet, which is previously pilot-tested for extraction using some random studies. We recommend extracting both adjusted and non-adjusted data because it gives the most allowed confounding factor to be used in the analysis by pooling them later [ 13 ]. The process of extraction should be executed by 2–3 independent reviewers. Mostly, the sheet is classified into the study and patient characteristics, outcomes, and quality assessment (QA) tool.
Data presented in graphs should be extracted by software tools such as Web plot digitizer [ 14 ]. Most of the equations that can be used in extraction prior to analysis and estimation of standard deviation (SD) from other variables is found inside Additional file 5 : File S2 with their references as Hozo et al. [ 15 ], Xiang et al. [ 16 ], and Rijkom et al. [ 17 ]. A variety of tools are available for the QA, depending on the design: ROB-2 Cochrane tool for randomized controlled trials [ 18 ] which is presented as Additional file 1 : Figure S1 and Additional file 2 : Figure S2—from a previous published article data—[ 19 ], NIH tool for observational and cross-sectional studies [ 20 ], ROBINS-I tool for non-randomize trials [ 21 ], QUADAS-2 tool for diagnostic studies, QUIPS tool for prognostic studies, CARE tool for case reports, and ToxRtool for in vivo and in vitro studies. We recommend that 2–3 reviewers independently assess the quality of the studies and add to the data extraction form before the inclusion into the analysis to reduce the risk of bias. In the NIH tool for observational studies—cohort and cross-sectional—as in this EBOLA case, to evaluate the risk of bias, reviewers should rate each of the 14 items into dichotomous variables: yes, no, or not applicable. An overall score is calculated by adding all the items scores as yes equals one, while no and NA equals zero. A score will be given for every paper to classify them as poor, fair, or good conducted studies, where a score from 0–5 was considered poor, 6–9 as fair, and 10–14 as good.
In the EBOLA case example above, authors can extract the following information: name of authors, country of patients, year of publication, study design (case report, cohort study, or clinical trial or RCT), sample size, the infected point of time after EBOLA infection, follow-up interval after vaccination time, efficacy, safety, adverse effects after vaccinations, and QA sheet (Additional file 6 : Data S1).
Data checking
Due to the expected human error and bias, we recommend a data checking step, in which every included article is compared with its counterpart in an extraction sheet by evidence photos, to detect mistakes in data. We advise assigning articles to 2–3 independent reviewers, ideally not the ones who performed the extraction of those articles. When resources are limited, each reviewer is assigned a different article than the one he extracted in the previous stage.
Statistical analysis
Investigators use different methods for combining and summarizing findings of included studies. Before analysis, there is an important step called cleaning of data in the extraction sheet, where the analyst organizes extraction sheet data in a form that can be read by analytical software. The analysis consists of 2 types namely qualitative and quantitative analysis. Qualitative analysis mostly describes data in SR studies, while quantitative analysis consists of two main types: MA and network meta-analysis (NMA). Subgroup, sensitivity, cumulative analyses, and meta-regression are appropriate for testing whether the results are consistent or not and investigating the effect of certain confounders on the outcome and finding the best predictors. Publication bias should be assessed to investigate the presence of missing studies which can affect the summary.
To illustrate basic meta-analysis, we provide an imaginary data for the research question about Ebola vaccine safety (in terms of adverse events, 14 days after injection) and immunogenicity (Ebola virus antibodies rise in geometric mean titer, 6 months after injection). Assuming that from searching and data extraction, we decided to do an analysis to evaluate Ebola vaccine “A” safety and immunogenicity. Other Ebola vaccines were not meta-analyzed because of the limited number of studies (instead, it will be included for narrative review). The imaginary data for vaccine safety meta-analysis can be accessed in Additional file 7 : Data S2. To do the meta-analysis, we can use free software, such as RevMan [ 22 ] or R package meta [ 23 ]. In this example, we will use the R package meta. The tutorial of meta package can be accessed through “General Package for Meta-Analysis” tutorial pdf [ 23 ]. The R codes and its guidance for meta-analysis done can be found in Additional file 5 : File S3.
For the analysis, we assume that the study is heterogenous in nature; therefore, we choose a random effect model. We did an analysis on the safety of Ebola vaccine A. From the data table, we can see some adverse events occurring after intramuscular injection of vaccine A to the subject of the study. Suppose that we include six studies that fulfill our inclusion criteria. We can do a meta-analysis for each of the adverse events extracted from the studies, for example, arthralgia, from the results of random effect meta-analysis using the R meta package.
From the results shown in Additional file 3 : Figure S3, we can see that the odds ratio (OR) of arthralgia is 1.06 (0.79; 1.42), p value = 0.71, which means that there is no association between the intramuscular injection of Ebola vaccine A and arthralgia, as the OR is almost one, and besides, the P value is insignificant as it is > 0.05.
In the meta-analysis, we can also visualize the results in a forest plot. It is shown in Fig. 3 an example of a forest plot from the simulated analysis.
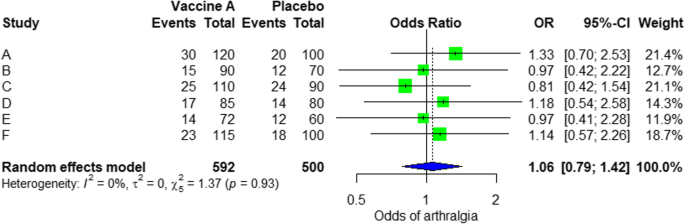
Random effect model forest plot for comparison of vaccine A versus placebo
From the forest plot, we can see six studies (A to F) and their respective OR (95% CI). The green box represents the effect size (in this case, OR) of each study. The bigger the box means the study weighted more (i.e., bigger sample size). The blue diamond shape represents the pooled OR of the six studies. We can see the blue diamond cross the vertical line OR = 1, which indicates no significance for the association as the diamond almost equalized in both sides. We can confirm this also from the 95% confidence interval that includes one and the p value > 0.05.
For heterogeneity, we see that I 2 = 0%, which means no heterogeneity is detected; the study is relatively homogenous (it is rare in the real study). To evaluate publication bias related to the meta-analysis of adverse events of arthralgia, we can use the metabias function from the R meta package (Additional file 4 : Figure S4) and visualization using a funnel plot. The results of publication bias are demonstrated in Fig. 4 . We see that the p value associated with this test is 0.74, indicating symmetry of the funnel plot. We can confirm it by looking at the funnel plot.
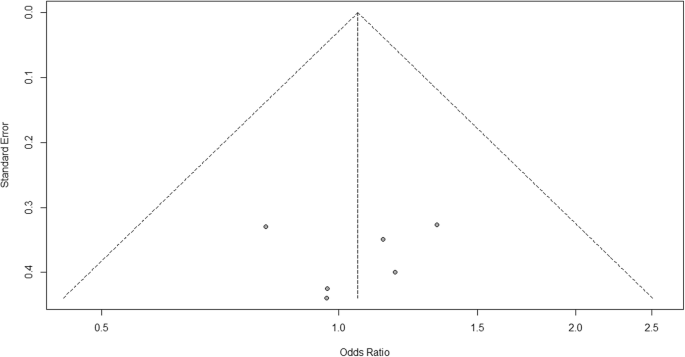
Publication bias funnel plot for comparison of vaccine A versus placebo
Looking at the funnel plot, the number of studies at the left and right side of the funnel plot is the same; therefore, the plot is symmetry, indicating no publication bias detected.
Sensitivity analysis is a procedure used to discover how different values of an independent variable will influence the significance of a particular dependent variable by removing one study from MA. If all included study p values are < 0.05, hence, removing any study will not change the significant association. It is only performed when there is a significant association, so if the p value of MA done is 0.7—more than one—the sensitivity analysis is not needed for this case study example. If there are 2 studies with p value > 0.05, removing any of the two studies will result in a loss of the significance.
Double data checking
For more assurance on the quality of results, the analyzed data should be rechecked from full-text data by evidence photos, to allow an obvious check for the PI of the study.
Manuscript writing, revision, and submission to a journal
Writing based on four scientific sections: introduction, methods, results, and discussion, mostly with a conclusion. Performing a characteristic table for study and patient characteristics is a mandatory step which can be found as a template in Additional file 5 : Table S3.
After finishing the manuscript writing, characteristics table, and PRISMA flow diagram, the team should send it to the PI to revise it well and reply to his comments and, finally, choose a suitable journal for the manuscript which fits with considerable impact factor and fitting field. We need to pay attention by reading the author guidelines of journals before submitting the manuscript.
The role of evidence-based medicine in biomedical research is rapidly growing. SR/MAs are also increasing in the medical literature. This paper has sought to provide a comprehensive approach to enable reviewers to produce high-quality SR/MAs. We hope that readers could gain general knowledge about how to conduct a SR/MA and have the confidence to perform one, although this kind of study requires complex steps compared to narrative reviews.
Having the basic steps for conduction of MA, there are many advanced steps that are applied for certain specific purposes. One of these steps is meta-regression which is performed to investigate the association of any confounder and the results of the MA. Furthermore, there are other types rather than the standard MA like NMA and MA. In NMA, we investigate the difference between several comparisons when there were not enough data to enable standard meta-analysis. It uses both direct and indirect comparisons to conclude what is the best between the competitors. On the other hand, mega MA or MA of patients tend to summarize the results of independent studies by using its individual subject data. As a more detailed analysis can be done, it is useful in conducting repeated measure analysis and time-to-event analysis. Moreover, it can perform analysis of variance and multiple regression analysis; however, it requires homogenous dataset and it is time-consuming in conduct [ 24 ].
Conclusions
Systematic review/meta-analysis steps include development of research question and its validation, forming criteria, search strategy, searching databases, importing all results to a library and exporting to an excel sheet, protocol writing and registration, title and abstract screening, full-text screening, manual searching, extracting data and assessing its quality, data checking, conducting statistical analysis, double data checking, manuscript writing, revising, and submitting to a journal.
Availability of data and materials
Not applicable.
Abbreviations
Network meta-analysis
Principal investigator
Population, Intervention, Comparison, Outcome
Preferred Reporting Items for Systematic Review and Meta-analysis statement
Quality assessment
Sample, Phenomenon of Interest, Design, Evaluation, Research type
Systematic review and meta-analyses
Bello A, Wiebe N, Garg A, Tonelli M. Evidence-based decision-making 2: systematic reviews and meta-analysis. Methods Mol Biol (Clifton, NJ). 2015;1281:397–416.
Article Google Scholar
Khan KS, Kunz R, Kleijnen J, Antes G. Five steps to conducting a systematic review. J R Soc Med. 2003;96(3):118–21.
Rys P, Wladysiuk M, Skrzekowska-Baran I, Malecki MT. Review articles, systematic reviews and meta-analyses: which can be trusted? Polskie Archiwum Medycyny Wewnetrznej. 2009;119(3):148–56.
PubMed Google Scholar
Higgins JPT, Green S. Cochrane Handbook for Systematic Reviews of Interventions Version 5.1.0 [updated March 2011]. 2011.
Moher D, Liberati A, Tetzlaff J, Altman DG. Preferred reporting items for systematic reviews and meta-analyses: the PRISMA statement. BMJ. 2009;339:b2535.
Methley AM, Campbell S, Chew-Graham C, McNally R, Cheraghi-Sohi S. PICO, PICOS and SPIDER: a comparison study of specificity and sensitivity in three search tools for qualitative systematic reviews. BMC Health Serv Res. 2014;14:579.
Gross L, Lhomme E, Pasin C, Richert L, Thiebaut R. Ebola vaccine development: systematic review of pre-clinical and clinical studies, and meta-analysis of determinants of antibody response variability after vaccination. Int J Infect Dis. 2018;74:83–96.
Article CAS Google Scholar
Shea BJ, Reeves BC, Wells G, Thuku M, Hamel C, Moran J, ... Henry DA. AMSTAR 2: a critical appraisal tool for systematic reviews that include randomised or non-randomised studies of healthcare interventions, or both. BMJ. 2017;358:j4008.
Giang HTN, Banno K, Minh LHN, Trinh LT, Loc LT, Eltobgy A, et al. Dengue hemophagocytic syndrome: a systematic review and meta-analysis on epidemiology, clinical signs, outcomes, and risk factors. Rev Med Virol. 2018;28(6):e2005.
Morra ME, Altibi AMA, Iqtadar S, Minh LHN, Elawady SS, Hallab A, et al. Definitions for warning signs and signs of severe dengue according to the WHO 2009 classification: systematic review of literature. Rev Med Virol. 2018;28(4):e1979.
Morra ME, Van Thanh L, Kamel MG, Ghazy AA, Altibi AMA, Dat LM, et al. Clinical outcomes of current medical approaches for Middle East respiratory syndrome: a systematic review and meta-analysis. Rev Med Virol. 2018;28(3):e1977.
Vassar M, Atakpo P, Kash MJ. Manual search approaches used by systematic reviewers in dermatology. Journal of the Medical Library Association: JMLA. 2016;104(4):302.
Naunheim MR, Remenschneider AK, Scangas GA, Bunting GW, Deschler DG. The effect of initial tracheoesophageal voice prosthesis size on postoperative complications and voice outcomes. Ann Otol Rhinol Laryngol. 2016;125(6):478–84.
Rohatgi AJaiWa. Web Plot Digitizer. ht tp. 2014;2.
Hozo SP, Djulbegovic B, Hozo I. Estimating the mean and variance from the median, range, and the size of a sample. BMC Med Res Methodol. 2005;5(1):13.
Wan X, Wang W, Liu J, Tong T. Estimating the sample mean and standard deviation from the sample size, median, range and/or interquartile range. BMC Med Res Methodol. 2014;14(1):135.
Van Rijkom HM, Truin GJ, Van’t Hof MA. A meta-analysis of clinical studies on the caries-inhibiting effect of fluoride gel treatment. Carries Res. 1998;32(2):83–92.
Higgins JP, Altman DG, Gotzsche PC, Juni P, Moher D, Oxman AD, et al. The Cochrane Collaboration's tool for assessing risk of bias in randomised trials. BMJ. 2011;343:d5928.
Tawfik GM, Tieu TM, Ghozy S, Makram OM, Samuel P, Abdelaal A, et al. Speech efficacy, safety and factors affecting lifetime of voice prostheses in patients with laryngeal cancer: a systematic review and network meta-analysis of randomized controlled trials. J Clin Oncol. 2018;36(15_suppl):e18031-e.
Wannemuehler TJ, Lobo BC, Johnson JD, Deig CR, Ting JY, Gregory RL. Vibratory stimulus reduces in vitro biofilm formation on tracheoesophageal voice prostheses. Laryngoscope. 2016;126(12):2752–7.
Sterne JAC, Hernán MA, Reeves BC, Savović J, Berkman ND, Viswanathan M, et al. ROBINS-I: a tool for assessing risk of bias in non-randomised studies of interventions. BMJ. 2016;355.
RevMan The Cochrane Collaboration %J Copenhagen TNCCTCC. Review Manager (RevMan). 5.0. 2008.
Schwarzer GJRn. meta: An R package for meta-analysis. 2007;7(3):40-45.
Google Scholar
Simms LLH. Meta-analysis versus mega-analysis: is there a difference? Oral budesonide for the maintenance of remission in Crohn’s disease: Faculty of Graduate Studies, University of Western Ontario; 1998.
Download references
Acknowledgements
This study was conducted (in part) at the Joint Usage/Research Center on Tropical Disease, Institute of Tropical Medicine, Nagasaki University, Japan.
Author information
Authors and affiliations.
Faculty of Medicine, Ain Shams University, Cairo, Egypt
Gehad Mohamed Tawfik
Online research Club http://www.onlineresearchclub.org/
Gehad Mohamed Tawfik, Kadek Agus Surya Dila, Muawia Yousif Fadlelmola Mohamed, Dao Ngoc Hien Tam, Nguyen Dang Kien & Ali Mahmoud Ahmed
Pratama Giri Emas Hospital, Singaraja-Amlapura street, Giri Emas village, Sawan subdistrict, Singaraja City, Buleleng, Bali, 81171, Indonesia
Kadek Agus Surya Dila
Faculty of Medicine, University of Khartoum, Khartoum, Sudan
Muawia Yousif Fadlelmola Mohamed
Nanogen Pharmaceutical Biotechnology Joint Stock Company, Ho Chi Minh City, Vietnam
Dao Ngoc Hien Tam
Department of Obstetrics and Gynecology, Thai Binh University of Medicine and Pharmacy, Thai Binh, Vietnam
Nguyen Dang Kien
Faculty of Medicine, Al-Azhar University, Cairo, Egypt
Ali Mahmoud Ahmed
Evidence Based Medicine Research Group & Faculty of Applied Sciences, Ton Duc Thang University, Ho Chi Minh City, 70000, Vietnam
Nguyen Tien Huy
Faculty of Applied Sciences, Ton Duc Thang University, Ho Chi Minh City, 70000, Vietnam
Department of Clinical Product Development, Institute of Tropical Medicine (NEKKEN), Leading Graduate School Program, and Graduate School of Biomedical Sciences, Nagasaki University, 1-12-4 Sakamoto, Nagasaki, 852-8523, Japan
You can also search for this author in PubMed Google Scholar
Contributions
NTH and GMT were responsible for the idea and its design. The figure was done by GMT. All authors contributed to the manuscript writing and approval of the final version.
Corresponding author
Correspondence to Nguyen Tien Huy .
Ethics declarations
Ethics approval and consent to participate, consent for publication, competing interests.
The authors declare that they have no competing interests.
Additional information
Publisher’s note.
Springer Nature remains neutral with regard to jurisdictional claims in published maps and institutional affiliations.
Additional files
Additional file 1:.
Figure S1. Risk of bias assessment graph of included randomized controlled trials. (TIF 20 kb)
Additional file 2:
Figure S2. Risk of bias assessment summary. (TIF 69 kb)
Additional file 3:
Figure S3. Arthralgia results of random effect meta-analysis using R meta package. (TIF 20 kb)
Additional file 4:
Figure S4. Arthralgia linear regression test of funnel plot asymmetry using R meta package. (TIF 13 kb)
Additional file 5:
Table S1. PRISMA 2009 Checklist. Table S2. Manipulation guides for online database searches. Table S3. Detailed search strategy for twelve database searches. Table S4. Baseline characteristics of the patients in the included studies. File S1. PROSPERO protocol template file. File S2. Extraction equations that can be used prior to analysis to get missed variables. File S3. R codes and its guidance for meta-analysis done for comparison between EBOLA vaccine A and placebo. (DOCX 49 kb)
Additional file 6:
Data S1. Extraction and quality assessment data sheets for EBOLA case example. (XLSX 1368 kb)
Additional file 7:
Data S2. Imaginary data for EBOLA case example. (XLSX 10 kb)
Rights and permissions
Open Access This article is distributed under the terms of the Creative Commons Attribution 4.0 International License ( http://creativecommons.org/licenses/by/4.0/ ), which permits unrestricted use, distribution, and reproduction in any medium, provided you give appropriate credit to the original author(s) and the source, provide a link to the Creative Commons license, and indicate if changes were made. The Creative Commons Public Domain Dedication waiver ( http://creativecommons.org/publicdomain/zero/1.0/ ) applies to the data made available in this article, unless otherwise stated.
Reprints and permissions
About this article
Cite this article.
Tawfik, G.M., Dila, K.A.S., Mohamed, M.Y.F. et al. A step by step guide for conducting a systematic review and meta-analysis with simulation data. Trop Med Health 47 , 46 (2019). https://doi.org/10.1186/s41182-019-0165-6
Download citation
Received : 30 January 2019
Accepted : 24 May 2019
Published : 01 August 2019
DOI : https://doi.org/10.1186/s41182-019-0165-6
Share this article
Anyone you share the following link with will be able to read this content:
Sorry, a shareable link is not currently available for this article.
Provided by the Springer Nature SharedIt content-sharing initiative
Tropical Medicine and Health
ISSN: 1349-4147
- Submission enquiries: Access here and click Contact Us
- General enquiries: [email protected]
Systematic Reviews and Meta-Analyses: Home
- Get Started
- Exploratory Search
- Where to Search
- How to Search
- Grey Literature
- What about errata and retractions?
- Eligibility Screening
- Critical Appraisal
- Data Extraction
- Synthesis & Discussion
- Assess Certainty
- Share & Archive
Welcome! This guide is designed to help novice and experienced review teams navigate the systematic review and/or meta-analysis process.
Guide Sections - Table of Contents
If you're new to this methodology, check out the video and resources below. Each tab above contains more detail about the respective topic.
Table of Contents for this Guide
Get Started | Reporting guidelines and methodological guidance, team formation, finding existing reviews.
Define Scope | F ormation of a clear research question and eligibility criteria; contains subpage for exploratory searching .
Protocol | Introduction to protocol purpose, development, registration.
Comprehensive Search | Contains subpages where to search , how to search , grey literature , and errata/retractions .
Eligibility Screening | Title and abstract screening, full-text review, interrater reliability, and resolving disagreements.
Critical Appraisal | Risk of bias assessment purpose, tools, and presentation.
Data Extraction | Data extraction execution, and presentation.
Synthesis & Discussion | Qualitative synthesis, meta-analysis, and discussion
Assess Certainty | Assessing certainty of evidence using formal methods.
Share & Archive | Repositories to share supplemental material.
Help & Training | Evidence Synthesis Services support and events; additional support outside of the VT Libraries; contains subpage tools .
What is a systematic review and/or meta-analysis?
A review of a clearly formulated question that uses systematic and explicit methods to identify , select , and critically appraise relevant research, and to collect and analyse data from the studies that are included in the review. Statistical methods ( meta-analysis ) may or may not be used to analyse and summarise the results of the included studies.
- Cochrane Collaboration Definition
Considerations before you start.

Has the review already been done or is a review currently underway ? (no need to duplicate if a review exists or is in-progress)
Do you have the resource capacity? (e.g., a team of 3 or more people, time to commit to a months or years long review?)
If a systematic review and/or meta-analysis is not the best option, you may consider alternative evidence synthesis approaches !
Cornerstones
Cornerstones of the systematic review and/or meta-analysis.

According to Wormald & Evans (2018) , the systematic review differs from a subjective, traditional literature review approach in that:
A systematic review is a reproducible piece of observational research and should have a protocol that sets out explicitly objective methods for the conduct of the review, particularly focusing on the control of error , both from bias and the reduction of random error through meta-analysis. Especially important in a systematic review is the objective , methodologically sound and reproducible retrieval of the evidence using...search strategies devised by a trained and experienced information scientist .
Note: This site will continue to evolve and develop through community driven collaboration with information retrieval and evidence synthesis experts across many disciplines. If you've found a broken link or have suggestions for the guide, please reach out to [email protected] .

- Next: Get Started >>
- Last Updated: Mar 28, 2024 2:54 PM
- URL: https://guides.lib.vt.edu/SRMA
Systematic Reviews and Meta Analysis
- Getting Started
- Guides and Standards
- Review Protocols
- Databases and Sources
- Randomized Controlled Trials
- Controlled Clinical Trials
- Observational Designs
- Tests of Diagnostic Accuracy
- Software and Tools
- Where do I get all those articles?
- Collaborations
- EPI 233/528
- Countway Mediated Search
- Risk of Bias (RoB)
Systematic review Q & A
What is a systematic review.
A systematic review is guided filtering and synthesis of all available evidence addressing a specific, focused research question, generally about a specific intervention or exposure. The use of standardized, systematic methods and pre-selected eligibility criteria reduce the risk of bias in identifying, selecting and analyzing relevant studies. A well-designed systematic review includes clear objectives, pre-selected criteria for identifying eligible studies, an explicit methodology, a thorough and reproducible search of the literature, an assessment of the validity or risk of bias of each included study, and a systematic synthesis, analysis and presentation of the findings of the included studies. A systematic review may include a meta-analysis.
For details about carrying out systematic reviews, see the Guides and Standards section of this guide.
Is my research topic appropriate for systematic review methods?
A systematic review is best deployed to test a specific hypothesis about a healthcare or public health intervention or exposure. By focusing on a single intervention or a few specific interventions for a particular condition, the investigator can ensure a manageable results set. Moreover, examining a single or small set of related interventions, exposures, or outcomes, will simplify the assessment of studies and the synthesis of the findings.
Systematic reviews are poor tools for hypothesis generation: for instance, to determine what interventions have been used to increase the awareness and acceptability of a vaccine or to investigate the ways that predictive analytics have been used in health care management. In the first case, we don't know what interventions to search for and so have to screen all the articles about awareness and acceptability. In the second, there is no agreed on set of methods that make up predictive analytics, and health care management is far too broad. The search will necessarily be incomplete, vague and very large all at the same time. In most cases, reviews without clearly and exactly specified populations, interventions, exposures, and outcomes will produce results sets that quickly outstrip the resources of a small team and offer no consistent way to assess and synthesize findings from the studies that are identified.
If not a systematic review, then what?
You might consider performing a scoping review . This framework allows iterative searching over a reduced number of data sources and no requirement to assess individual studies for risk of bias. The framework includes built-in mechanisms to adjust the analysis as the work progresses and more is learned about the topic. A scoping review won't help you limit the number of records you'll need to screen (broad questions lead to large results sets) but may give you means of dealing with a large set of results.
This tool can help you decide what kind of review is right for your question.
Can my student complete a systematic review during her summer project?
Probably not. Systematic reviews are a lot of work. Including creating the protocol, building and running a quality search, collecting all the papers, evaluating the studies that meet the inclusion criteria and extracting and analyzing the summary data, a well done review can require dozens to hundreds of hours of work that can span several months. Moreover, a systematic review requires subject expertise, statistical support and a librarian to help design and run the search. Be aware that librarians sometimes have queues for their search time. It may take several weeks to complete and run a search. Moreover, all guidelines for carrying out systematic reviews recommend that at least two subject experts screen the studies identified in the search. The first round of screening can consume 1 hour per screener for every 100-200 records. A systematic review is a labor-intensive team effort.
How can I know if my topic has been been reviewed already?
Before starting out on a systematic review, check to see if someone has done it already. In PubMed you can use the systematic review subset to limit to a broad group of papers that is enriched for systematic reviews. You can invoke the subset by selecting if from the Article Types filters to the left of your PubMed results, or you can append AND systematic[sb] to your search. For example:
"neoadjuvant chemotherapy" AND systematic[sb]
The systematic review subset is very noisy, however. To quickly focus on systematic reviews (knowing that you may be missing some), simply search for the word systematic in the title:
"neoadjuvant chemotherapy" AND systematic[ti]
Any PRISMA-compliant systematic review will be captured by this method since including the words "systematic review" in the title is a requirement of the PRISMA checklist. Cochrane systematic reviews do not include 'systematic' in the title, however. It's worth checking the Cochrane Database of Systematic Reviews independently.
You can also search for protocols that will indicate that another group has set out on a similar project. Many investigators will register their protocols in PROSPERO , a registry of review protocols. Other published protocols as well as Cochrane Review protocols appear in the Cochrane Methodology Register, a part of the Cochrane Library .
- Next: Guides and Standards >>
- Last Updated: Feb 26, 2024 3:17 PM
- URL: https://guides.library.harvard.edu/meta-analysis
- Meta-Analysis/Meta-Synthesis
Meta Analysis
Meta-analysis is a set of statistical techniques for synthesizing data across studies. It is a statistical method for combining the findings from quantitative studies. It evaluates, synthesizes, and summarizes results. It may be conducted independently or as a specialized subset of a systematic review. A systematic review attempts to collate empirical evidence that fits predefined eligibility criteria to answer a specific research question. Meta-analysis is a quantitative, formal, epidemiological study design used to systematically assess the results of previous research to derive conclusions about that body of research (Haidrich, 2010). Rigorously conducted meta-analyses are useful tools in evidence-based medicine . Outcomes from a meta-analysis may include a more precise estimate of the effect of a treatment or risk factor for disease or other outcomes. Not all systematic reviews include meta-analysis , but all meta-analyses are found in systematic reviews (Haidrich, 2010).
A Meta analysis is appropriate when a group of studies report quantitative results rather than qualitative findings or theory, if they examine the same or similar constructs or relationships, if they are derived from similar research designs and report the simple relationships between two variables rather than relationships that have been adjusted for the effect of additional variables (siddaway, et al., 2019).
Haidich A. B. (2010). Meta-analysis in medical research. Hippokratia , 14 (Suppl 1), 29–37.
Siddaway, A. P., Wood, A. M., & Hedges, L. V. (2019). How to do a systematic review: A best practice guide for conducting and reporting narrative reviews, meta-analyses, and meta-syntheses. Annual Review of Psychology, 70 , 747–770.
Meta Synthesis
A meta synthesis is the systematic review and integration of findings from qualitative studies (Lachal et al., 2017). Reviews of qualitative information can be conducted and reported using the same replicable, rigorous, and transparent methodology and presentation. A meta-synthesis can be used when a review aims to integrate qualitative research. A meta-synthesis attempts to synthesize qualitative studies on a topic to identify key themes, concepts, or theories that provide novel or more powerful explanations for the phenomenon under review (Siddaway et al., 2019).
Lachal, J., Revah-Levy, A., Orri, M., & Moro, M. R. (2017). Metasynthesis: An original method to synthesize qualitative literature in psychiatry. Frontiers in Psychiatry, 8 , 269 .
Siddaway, A. P., Wood, A. M., & Hedges, L. V. (2019). How to do a systematic review: A best practice guide for conducting and reporting narrative reviews, meta-analyses, and meta-syntheses. Annual Review of Psychology, 70 , 747–770 .
- << Previous: Rapid Review
- Next: Selecting a Review Type >>
- Types of Questions
- Key Features and Limitations
- Is a Systematic Review Right for Your Research?
- Integrative Review
- Scoping Review
- Rapid Review
- Selecting a Review Type
- Reducing Bias
- Guidelines for Student Researchers
- Training Resources
- Register Your Protocol
- Handbooks & Manuals
- Reporting Guidelines
- PRESS 2015 Guidelines
- Search Strategies
- Selected Databases
- Grey Literature
- Handsearching
- Citation Searching
- Study Types & Terminology
- Quantitative vs. Qualitative Research
- Critical Appraisal of Studies
- Broad Functionality Programs & Tools
- Search Strategy Tools
- Deduplication Tools
- CItation Screening
- Critical Appraisal Tools
- Quality Assessment/Risk of Bias Tools
- Data Collection/Extraction
- Meta Analysis Tools
- Books on Systematic Reviews
- Finding Systematic Review Articles in the Databases
- Systematic Review Journals
- More Resources
- Evidence-Based Practice Research in Nursing
- Citation Management Programs
- Last Updated: Mar 20, 2024 2:16 PM
- URL: https://libguides.adelphi.edu/Systematic_Reviews
University of Minnesota
Digital conservancy.
- University Digital Conservancy Home
- University of Minnesota Twin Cities
- Dissertations and Theses
- Dissertations
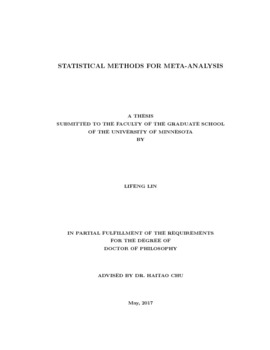
View/ Download file
Persistent link to this item, appears in collections, description, suggested citation, udc services.
- About the UDC
- How to Deposit
- Policies and Terms of Use
Related Services
- University Archives
- U of M Web Archive
- UMedia Archive
- Copyright Services
- Digital Library Services
- News & Events
- Staff Directory
- Subject Librarians
- Vision, Mission, & Goals

- Search Menu
- Browse content in Arts and Humanities
- Browse content in Archaeology
- Anglo-Saxon and Medieval Archaeology
- Archaeological Methodology and Techniques
- Archaeology by Region
- Archaeology of Religion
- Archaeology of Trade and Exchange
- Biblical Archaeology
- Contemporary and Public Archaeology
- Environmental Archaeology
- Historical Archaeology
- History and Theory of Archaeology
- Industrial Archaeology
- Landscape Archaeology
- Mortuary Archaeology
- Prehistoric Archaeology
- Underwater Archaeology
- Urban Archaeology
- Zooarchaeology
- Browse content in Architecture
- Architectural Structure and Design
- History of Architecture
- Residential and Domestic Buildings
- Theory of Architecture
- Browse content in Art
- Art Subjects and Themes
- History of Art
- Industrial and Commercial Art
- Theory of Art
- Biographical Studies
- Byzantine Studies
- Browse content in Classical Studies
- Classical History
- Classical Philosophy
- Classical Mythology
- Classical Literature
- Classical Reception
- Classical Art and Architecture
- Classical Oratory and Rhetoric
- Greek and Roman Epigraphy
- Greek and Roman Law
- Greek and Roman Papyrology
- Greek and Roman Archaeology
- Late Antiquity
- Religion in the Ancient World
- Digital Humanities
- Browse content in History
- Colonialism and Imperialism
- Diplomatic History
- Environmental History
- Genealogy, Heraldry, Names, and Honours
- Genocide and Ethnic Cleansing
- Historical Geography
- History by Period
- History of Emotions
- History of Agriculture
- History of Education
- History of Gender and Sexuality
- Industrial History
- Intellectual History
- International History
- Labour History
- Legal and Constitutional History
- Local and Family History
- Maritime History
- Military History
- National Liberation and Post-Colonialism
- Oral History
- Political History
- Public History
- Regional and National History
- Revolutions and Rebellions
- Slavery and Abolition of Slavery
- Social and Cultural History
- Theory, Methods, and Historiography
- Urban History
- World History
- Browse content in Language Teaching and Learning
- Language Learning (Specific Skills)
- Language Teaching Theory and Methods
- Browse content in Linguistics
- Applied Linguistics
- Cognitive Linguistics
- Computational Linguistics
- Forensic Linguistics
- Grammar, Syntax and Morphology
- Historical and Diachronic Linguistics
- History of English
- Language Acquisition
- Language Evolution
- Language Reference
- Language Variation
- Language Families
- Lexicography
- Linguistic Anthropology
- Linguistic Theories
- Linguistic Typology
- Phonetics and Phonology
- Psycholinguistics
- Sociolinguistics
- Translation and Interpretation
- Writing Systems
- Browse content in Literature
- Bibliography
- Children's Literature Studies
- Literary Studies (Asian)
- Literary Studies (European)
- Literary Studies (Eco-criticism)
- Literary Studies (Romanticism)
- Literary Studies (American)
- Literary Studies (Modernism)
- Literary Studies - World
- Literary Studies (1500 to 1800)
- Literary Studies (19th Century)
- Literary Studies (20th Century onwards)
- Literary Studies (African American Literature)
- Literary Studies (British and Irish)
- Literary Studies (Early and Medieval)
- Literary Studies (Fiction, Novelists, and Prose Writers)
- Literary Studies (Gender Studies)
- Literary Studies (Graphic Novels)
- Literary Studies (History of the Book)
- Literary Studies (Plays and Playwrights)
- Literary Studies (Poetry and Poets)
- Literary Studies (Postcolonial Literature)
- Literary Studies (Queer Studies)
- Literary Studies (Science Fiction)
- Literary Studies (Travel Literature)
- Literary Studies (War Literature)
- Literary Studies (Women's Writing)
- Literary Theory and Cultural Studies
- Mythology and Folklore
- Shakespeare Studies and Criticism
- Browse content in Media Studies
- Browse content in Music
- Applied Music
- Dance and Music
- Ethics in Music
- Ethnomusicology
- Gender and Sexuality in Music
- Medicine and Music
- Music Cultures
- Music and Religion
- Music and Media
- Music and Culture
- Music Education and Pedagogy
- Music Theory and Analysis
- Musical Scores, Lyrics, and Libretti
- Musical Structures, Styles, and Techniques
- Musicology and Music History
- Performance Practice and Studies
- Race and Ethnicity in Music
- Sound Studies
- Browse content in Performing Arts
- Browse content in Philosophy
- Aesthetics and Philosophy of Art
- Epistemology
- Feminist Philosophy
- History of Western Philosophy
- Metaphysics
- Moral Philosophy
- Non-Western Philosophy
- Philosophy of Science
- Philosophy of Language
- Philosophy of Mind
- Philosophy of Perception
- Philosophy of Action
- Philosophy of Law
- Philosophy of Religion
- Philosophy of Mathematics and Logic
- Practical Ethics
- Social and Political Philosophy
- Browse content in Religion
- Biblical Studies
- Christianity
- East Asian Religions
- History of Religion
- Judaism and Jewish Studies
- Qumran Studies
- Religion and Education
- Religion and Health
- Religion and Politics
- Religion and Science
- Religion and Law
- Religion and Art, Literature, and Music
- Religious Studies
- Browse content in Society and Culture
- Cookery, Food, and Drink
- Cultural Studies
- Customs and Traditions
- Ethical Issues and Debates
- Hobbies, Games, Arts and Crafts
- Lifestyle, Home, and Garden
- Natural world, Country Life, and Pets
- Popular Beliefs and Controversial Knowledge
- Sports and Outdoor Recreation
- Technology and Society
- Travel and Holiday
- Visual Culture
- Browse content in Law
- Arbitration
- Browse content in Company and Commercial Law
- Commercial Law
- Company Law
- Browse content in Comparative Law
- Systems of Law
- Competition Law
- Browse content in Constitutional and Administrative Law
- Government Powers
- Judicial Review
- Local Government Law
- Military and Defence Law
- Parliamentary and Legislative Practice
- Construction Law
- Contract Law
- Browse content in Criminal Law
- Criminal Procedure
- Criminal Evidence Law
- Sentencing and Punishment
- Employment and Labour Law
- Environment and Energy Law
- Browse content in Financial Law
- Banking Law
- Insolvency Law
- History of Law
- Human Rights and Immigration
- Intellectual Property Law
- Browse content in International Law
- Private International Law and Conflict of Laws
- Public International Law
- IT and Communications Law
- Jurisprudence and Philosophy of Law
- Law and Politics
- Law and Society
- Browse content in Legal System and Practice
- Courts and Procedure
- Legal Skills and Practice
- Primary Sources of Law
- Regulation of Legal Profession
- Medical and Healthcare Law
- Browse content in Policing
- Criminal Investigation and Detection
- Police and Security Services
- Police Procedure and Law
- Police Regional Planning
- Browse content in Property Law
- Personal Property Law
- Study and Revision
- Terrorism and National Security Law
- Browse content in Trusts Law
- Wills and Probate or Succession
- Browse content in Medicine and Health
- Browse content in Allied Health Professions
- Arts Therapies
- Clinical Science
- Dietetics and Nutrition
- Occupational Therapy
- Operating Department Practice
- Physiotherapy
- Radiography
- Speech and Language Therapy
- Browse content in Anaesthetics
- General Anaesthesia
- Neuroanaesthesia
- Browse content in Clinical Medicine
- Acute Medicine
- Cardiovascular Medicine
- Clinical Genetics
- Clinical Pharmacology and Therapeutics
- Dermatology
- Endocrinology and Diabetes
- Gastroenterology
- Genito-urinary Medicine
- Geriatric Medicine
- Infectious Diseases
- Medical Toxicology
- Medical Oncology
- Pain Medicine
- Palliative Medicine
- Rehabilitation Medicine
- Respiratory Medicine and Pulmonology
- Rheumatology
- Sleep Medicine
- Sports and Exercise Medicine
- Clinical Neuroscience
- Community Medical Services
- Critical Care
- Emergency Medicine
- Forensic Medicine
- Haematology
- History of Medicine
- Browse content in Medical Dentistry
- Oral and Maxillofacial Surgery
- Paediatric Dentistry
- Restorative Dentistry and Orthodontics
- Surgical Dentistry
- Browse content in Medical Skills
- Clinical Skills
- Communication Skills
- Nursing Skills
- Surgical Skills
- Medical Ethics
- Medical Statistics and Methodology
- Browse content in Neurology
- Clinical Neurophysiology
- Neuropathology
- Nursing Studies
- Browse content in Obstetrics and Gynaecology
- Gynaecology
- Occupational Medicine
- Ophthalmology
- Otolaryngology (ENT)
- Browse content in Paediatrics
- Neonatology
- Browse content in Pathology
- Chemical Pathology
- Clinical Cytogenetics and Molecular Genetics
- Histopathology
- Medical Microbiology and Virology
- Patient Education and Information
- Browse content in Pharmacology
- Psychopharmacology
- Browse content in Popular Health
- Caring for Others
- Complementary and Alternative Medicine
- Self-help and Personal Development
- Browse content in Preclinical Medicine
- Cell Biology
- Molecular Biology and Genetics
- Reproduction, Growth and Development
- Primary Care
- Professional Development in Medicine
- Browse content in Psychiatry
- Addiction Medicine
- Child and Adolescent Psychiatry
- Forensic Psychiatry
- Learning Disabilities
- Old Age Psychiatry
- Psychotherapy
- Browse content in Public Health and Epidemiology
- Epidemiology
- Public Health
- Browse content in Radiology
- Clinical Radiology
- Interventional Radiology
- Nuclear Medicine
- Radiation Oncology
- Reproductive Medicine
- Browse content in Surgery
- Cardiothoracic Surgery
- Gastro-intestinal and Colorectal Surgery
- General Surgery
- Neurosurgery
- Paediatric Surgery
- Peri-operative Care
- Plastic and Reconstructive Surgery
- Surgical Oncology
- Transplant Surgery
- Trauma and Orthopaedic Surgery
- Vascular Surgery
- Browse content in Science and Mathematics
- Browse content in Biological Sciences
- Aquatic Biology
- Biochemistry
- Bioinformatics and Computational Biology
- Developmental Biology
- Ecology and Conservation
- Evolutionary Biology
- Genetics and Genomics
- Microbiology
- Molecular and Cell Biology
- Natural History
- Plant Sciences and Forestry
- Research Methods in Life Sciences
- Structural Biology
- Systems Biology
- Zoology and Animal Sciences
- Browse content in Chemistry
- Analytical Chemistry
- Computational Chemistry
- Crystallography
- Environmental Chemistry
- Industrial Chemistry
- Inorganic Chemistry
- Materials Chemistry
- Medicinal Chemistry
- Mineralogy and Gems
- Organic Chemistry
- Physical Chemistry
- Polymer Chemistry
- Study and Communication Skills in Chemistry
- Theoretical Chemistry
- Browse content in Computer Science
- Artificial Intelligence
- Computer Architecture and Logic Design
- Game Studies
- Human-Computer Interaction
- Mathematical Theory of Computation
- Programming Languages
- Software Engineering
- Systems Analysis and Design
- Virtual Reality
- Browse content in Computing
- Business Applications
- Computer Security
- Computer Games
- Computer Networking and Communications
- Digital Lifestyle
- Graphical and Digital Media Applications
- Operating Systems
- Browse content in Earth Sciences and Geography
- Atmospheric Sciences
- Environmental Geography
- Geology and the Lithosphere
- Maps and Map-making
- Meteorology and Climatology
- Oceanography and Hydrology
- Palaeontology
- Physical Geography and Topography
- Regional Geography
- Soil Science
- Urban Geography
- Browse content in Engineering and Technology
- Agriculture and Farming
- Biological Engineering
- Civil Engineering, Surveying, and Building
- Electronics and Communications Engineering
- Energy Technology
- Engineering (General)
- Environmental Science, Engineering, and Technology
- History of Engineering and Technology
- Mechanical Engineering and Materials
- Technology of Industrial Chemistry
- Transport Technology and Trades
- Browse content in Environmental Science
- Applied Ecology (Environmental Science)
- Conservation of the Environment (Environmental Science)
- Environmental Sustainability
- Environmentalist Thought and Ideology (Environmental Science)
- Management of Land and Natural Resources (Environmental Science)
- Natural Disasters (Environmental Science)
- Nuclear Issues (Environmental Science)
- Pollution and Threats to the Environment (Environmental Science)
- Social Impact of Environmental Issues (Environmental Science)
- History of Science and Technology
- Browse content in Materials Science
- Ceramics and Glasses
- Composite Materials
- Metals, Alloying, and Corrosion
- Nanotechnology
- Browse content in Mathematics
- Applied Mathematics
- Biomathematics and Statistics
- History of Mathematics
- Mathematical Education
- Mathematical Finance
- Mathematical Analysis
- Numerical and Computational Mathematics
- Probability and Statistics
- Pure Mathematics
- Browse content in Neuroscience
- Cognition and Behavioural Neuroscience
- Development of the Nervous System
- Disorders of the Nervous System
- History of Neuroscience
- Invertebrate Neurobiology
- Molecular and Cellular Systems
- Neuroendocrinology and Autonomic Nervous System
- Neuroscientific Techniques
- Sensory and Motor Systems
- Browse content in Physics
- Astronomy and Astrophysics
- Atomic, Molecular, and Optical Physics
- Biological and Medical Physics
- Classical Mechanics
- Computational Physics
- Condensed Matter Physics
- Electromagnetism, Optics, and Acoustics
- History of Physics
- Mathematical and Statistical Physics
- Measurement Science
- Nuclear Physics
- Particles and Fields
- Plasma Physics
- Quantum Physics
- Relativity and Gravitation
- Semiconductor and Mesoscopic Physics
- Browse content in Psychology
- Affective Sciences
- Clinical Psychology
- Cognitive Psychology
- Cognitive Neuroscience
- Criminal and Forensic Psychology
- Developmental Psychology
- Educational Psychology
- Evolutionary Psychology
- Health Psychology
- History and Systems in Psychology
- Music Psychology
- Neuropsychology
- Organizational Psychology
- Psychological Assessment and Testing
- Psychology of Human-Technology Interaction
- Psychology Professional Development and Training
- Research Methods in Psychology
- Social Psychology
- Browse content in Social Sciences
- Browse content in Anthropology
- Anthropology of Religion
- Human Evolution
- Medical Anthropology
- Physical Anthropology
- Regional Anthropology
- Social and Cultural Anthropology
- Theory and Practice of Anthropology
- Browse content in Business and Management
- Business Strategy
- Business Ethics
- Business History
- Business and Government
- Business and Technology
- Business and the Environment
- Comparative Management
- Corporate Governance
- Corporate Social Responsibility
- Entrepreneurship
- Health Management
- Human Resource Management
- Industrial and Employment Relations
- Industry Studies
- Information and Communication Technologies
- International Business
- Knowledge Management
- Management and Management Techniques
- Operations Management
- Organizational Theory and Behaviour
- Pensions and Pension Management
- Public and Nonprofit Management
- Strategic Management
- Supply Chain Management
- Browse content in Criminology and Criminal Justice
- Criminal Justice
- Criminology
- Forms of Crime
- International and Comparative Criminology
- Youth Violence and Juvenile Justice
- Development Studies
- Browse content in Economics
- Agricultural, Environmental, and Natural Resource Economics
- Asian Economics
- Behavioural Finance
- Behavioural Economics and Neuroeconomics
- Econometrics and Mathematical Economics
- Economic Systems
- Economic History
- Economic Methodology
- Economic Development and Growth
- Financial Markets
- Financial Institutions and Services
- General Economics and Teaching
- Health, Education, and Welfare
- History of Economic Thought
- International Economics
- Labour and Demographic Economics
- Law and Economics
- Macroeconomics and Monetary Economics
- Microeconomics
- Public Economics
- Urban, Rural, and Regional Economics
- Welfare Economics
- Browse content in Education
- Adult Education and Continuous Learning
- Care and Counselling of Students
- Early Childhood and Elementary Education
- Educational Equipment and Technology
- Educational Strategies and Policy
- Higher and Further Education
- Organization and Management of Education
- Philosophy and Theory of Education
- Schools Studies
- Secondary Education
- Teaching of a Specific Subject
- Teaching of Specific Groups and Special Educational Needs
- Teaching Skills and Techniques
- Browse content in Environment
- Applied Ecology (Social Science)
- Climate Change
- Conservation of the Environment (Social Science)
- Environmentalist Thought and Ideology (Social Science)
- Natural Disasters (Environment)
- Social Impact of Environmental Issues (Social Science)
- Browse content in Human Geography
- Cultural Geography
- Economic Geography
- Political Geography
- Browse content in Interdisciplinary Studies
- Communication Studies
- Museums, Libraries, and Information Sciences
- Browse content in Politics
- African Politics
- Asian Politics
- Chinese Politics
- Comparative Politics
- Conflict Politics
- Elections and Electoral Studies
- Environmental Politics
- European Union
- Foreign Policy
- Gender and Politics
- Human Rights and Politics
- Indian Politics
- International Relations
- International Organization (Politics)
- International Political Economy
- Irish Politics
- Latin American Politics
- Middle Eastern Politics
- Political Methodology
- Political Communication
- Political Philosophy
- Political Sociology
- Political Behaviour
- Political Economy
- Political Institutions
- Political Theory
- Politics and Law
- Public Administration
- Public Policy
- Quantitative Political Methodology
- Regional Political Studies
- Russian Politics
- Security Studies
- State and Local Government
- UK Politics
- US Politics
- Browse content in Regional and Area Studies
- African Studies
- Asian Studies
- East Asian Studies
- Japanese Studies
- Latin American Studies
- Middle Eastern Studies
- Native American Studies
- Scottish Studies
- Browse content in Research and Information
- Research Methods
- Browse content in Social Work
- Addictions and Substance Misuse
- Adoption and Fostering
- Care of the Elderly
- Child and Adolescent Social Work
- Couple and Family Social Work
- Developmental and Physical Disabilities Social Work
- Direct Practice and Clinical Social Work
- Emergency Services
- Human Behaviour and the Social Environment
- International and Global Issues in Social Work
- Mental and Behavioural Health
- Social Justice and Human Rights
- Social Policy and Advocacy
- Social Work and Crime and Justice
- Social Work Macro Practice
- Social Work Practice Settings
- Social Work Research and Evidence-based Practice
- Welfare and Benefit Systems
- Browse content in Sociology
- Childhood Studies
- Community Development
- Comparative and Historical Sociology
- Economic Sociology
- Gender and Sexuality
- Gerontology and Ageing
- Health, Illness, and Medicine
- Marriage and the Family
- Migration Studies
- Occupations, Professions, and Work
- Organizations
- Population and Demography
- Race and Ethnicity
- Social Theory
- Social Movements and Social Change
- Social Research and Statistics
- Social Stratification, Inequality, and Mobility
- Sociology of Religion
- Sociology of Education
- Sport and Leisure
- Urban and Rural Studies
- Browse content in Warfare and Defence
- Defence Strategy, Planning, and Research
- Land Forces and Warfare
- Military Administration
- Military Life and Institutions
- Naval Forces and Warfare
- Other Warfare and Defence Issues
- Peace Studies and Conflict Resolution
- Weapons and Equipment

- < Previous chapter
- Next chapter >

17 Meta-analysis in Clinical Psychology Research
Andy P. Field, School of Psychology, University of Sussex
- Published: 01 August 2013
- Cite Icon Cite
- Permissions Icon Permissions
Meta-analysis is now the method of choice for assimilating research investigating the same question. This chapter is a nontechnical overview of the process of conducting meta-analysis in the context of clinical psychology. We begin with an overview of what meta-analysis aims to achieve. The process of conducting a meta-analysis is then described in six stages: (1) how to do a literature search; (2) how to decide which studies to include in the analysis (inclusion criteria); (3) how to calculate effect sizes for each study; (4) running a basic meta-analysis using the metaphor package for the free software R; (5) how to look for publication bias and moderators of effect sizes; and (6) how to write up the results for publication.
Introduction
Meta-analysis has become an increasingly popular research methodology, with an exponential increase in papers published seen across both social sciences and science in general. Field (2009) reports data showing that up until 1990 there were very few studies published on the topic of meta-analysis, but after this date the use of this tool has been on a meteoric increase. This trend has occurred in clinical psychology too. Figure 17.1 shows the number of articles with “meta-analysis” in the title published within the domain of “clinical psychology” since the term “meta-analysis” came into common usage. The data show a clear increase in publications after the 1990s, and a staggering acceleration in the number of published meta-analyses in this area in the past 3 to 5 years. Meta-analysis has been used to draw conclusions about the causes ( Bar-Haim, Lamy, Pergamin, Bakermans-Kranenburg, & van Ijzendoorn, 2007 ; Brewin, Kleiner, Vasterling, & Field, 2007 ; Burt, 2009 ; Chan, Xu, Heinrichs, Yu, & Wang, 2010 ; Kashdan, 2007 ; Ruocco, 2005 ), diagnosis ( Bloch, Landeros-Weisenberger, Rosario, Pittenger, & Leckman, 2008 ; Cuijpers, Li, Hofmann, & Andersson, 2010 ), and preferred treatments ( Barbato & D'Avanzo, 2008 ; Bradley, Greene, Russ, Dutra, & Westen, 2005 ; Cartwright-Hatton, Roberts, Chitsabesan, Fothergill, & Harrington, 2004 ; Covin, Ouimet, Seeds, & Dozois, 2008 ; Hendriks, Voshaar, Keijsers, Hoogduin, & van Balkom, 2008 ; Kleinstaeuber, Witthoeft, & Hiller, 2011 ; Malouff, Thorsteinsson, Rooke, Bhullar, & Schutte, 2008 ; Parsons & Rizzo, 2008 ; Roberts, Kitchiner, Kenardy, Bisson, & Psych, 2009 ; Rosa-Alcazar, Sanchez-Meca, Gomez-Conesa, & Marin-Martinez, 2008 ; Singh, Singh, Kar, & Chan, 2010 ; Spreckley & Boyd, 2009 ; Stewart & Chambless, 2009 ; Villeneuve, Potvin, Lesage, & Nicole, 2010 ) of a variety of mental health problems. This illustrative selection of articles shows that meta-analysis has been used to determine the efficacy of behavioral, cognitive, couple-based, cognitive-behavioral (CBT), virtual reality, and psychopharmacological interventions and on problems as diverse as schizophrenia, anxiety disorders, depression, chronic fatigue, personality disorders, and autism. There is little in the world of clinical psychology that has not been subjected to meta-analysis.
The number of studies using meta-analysis in clinical psychology.
This chapter provides a practical introduction to meta-analysis. For mathematical details, see other sources (e.g., H. M. Cooper, 2010 ; Field, 2001 , 2005a , 2009 ; Field & Gillett, 2010 ; Hedges & Olkin, 1985 ; Hedges & Vevea, 1998 ; Hunter & Schmidt, 2004 ; Overton, 1998 ; Rosenthal & DiMatteo, 2001 ; Schulze, 2004 ). This chapter overviews the important issues when conducting meta-analysis and shows an example of how to conduct a meta-analysis using the free software R ( R Development Core Team, 2010 ).
What Is Meta-analysis?
Clinical psychologists are typically interested in reaching conclusions that can be applied generally. These questions might whether CBT is efficacious as a treatment for obsessive-compulsive disorder ( Rosa-Alcazar et al., 2008 ), whether antidepressant medication treats the negative symptoms of schizophrenia ( Singh et al., 2010 ), whether virtual reality can be effective in treating specific phobias ( Parsons & Rizzo, 2008 ), whether school-based prevention programs reduce anxiety and/or depression in youth (Mychailyszyn, Brodman, Read, & Kendall, 2011), whether there are memory deficits for emotional information in posttraumatic stress disorder (PTSD; Brewin et al., 2007 ), what the magnitude of association between exposure to disasters and youth PTSD is ( Furr, Corner, Edmunds, & Kendall, 2010 ), or what the magnitude of threat-related attentional biases in anxious individuals is ( Bar-Haim, et al., 2007 ). Although answers to these questions may be attainable in a single study, single studies have two limitations: (1) they are at the mercy of their sample size because estimates of effects in small samples will be more biased than large sample studies and (2) replication is an important means to deal with the problems created by measurement error in research ( Fisher, 1935 ). Meta-analysis pools the results from similar studies in the hope of generating more accurate estimates of the true effect in the population. A meta-analysis can tell us:
The mean and variance of underlying population effects— for example, the effects in the population of conducting CBT with depressed adolescents compared to waitlist controls. You can also compute confidence intervals for the population effects.
Variability in effects across studies. It is possible to estimate the variability between effect sizes across studies (the homogeneity of effect sizes). There is accumulating evidence that effect sizes should be heterogeneous across studies (see, e.g., National Research Council, 1992 ). Therefore, variability statistics should be reported routinely. (You will often see significance tests reported for these estimates of variability; however, these tests typically have low power and are probably best ignored.)
Moderator variables. If there is variability in effect sizes, and in most cases there is ( Field, 2005a ), this variability can be explored in terms of moderator variables ( Field, 2003b ; Overton, 1998 ). For example, we might find that attentional biases to threat in anxious individuals are stronger when picture stimuli are used to measure these biases than when words are used.
A Bit of History
More than 70 years ago, Fisher and Pearson discussed ways to combine studies to find an overall probability ( Fisher, 1938 ; Pearson, 1938 ), and over 60 years ago, Stouffer presented a method for combining effect sizes ( Stouffer, 1949 ). The roots of meta-analysis are buried deep within the psychological and statistical earth. However, clinical psychology has some claim over the popularization of the method: in 1977, Smith and Glass published an influential paper in which they combined effects from 375 studies that had looked at the effects of psychotherapy ( Smith & Glass, 1977 ). They concluded that psychotherapy was effective, and that the type of psychotherapy did not matter. A year earlier, Glass (1976) published a paper in which he coined the term “meta-analysis” (if this wasn't the first usage of the term, then it was certainly one of the first) and summarized the basic principles. Shortly after these two seminal papers, Rosenthal published an influential theoretical paper on meta-analysis, and a meta-analysis combining 345 studies to show that interpersonal expectancies affected behavior ( Rosenthal, 1978 ; Rosenthal & Rubin, 1978 ). It is probably fair to say that these papers put “meta-analysis” in the spotlight of psychology. However, it was not until the early 1980s that three books were published by Rosenthal (1984 , 1991 ), Hedges and Olkin (1985) , and Hunter and Schmidt (1990) . These books were the first to provide detailed and accessible accounts of how to conduct a meta-analysis. Given a few years for researchers to assimilate these works, it is no surprise that the use and discussion of meta-analysis accelerated after 1990 (see Fig. 17.1 ). The even more dramatic acceleration in the number of published meta-analyses in the past 5 years is almost certainly due to the widespread availability of computer software packages that make the job of meta-analysis easier than before.
Computer Software for Doing Meta-analysis
An overview of the options.
There are several standalone packages for conducting meta-analyses: for example, the Cochrane Collaboration's Review Manager (RevMan) software ( The Cochrane Collaboration, 2008 ). There is also a package called Comprehensive Meta-Analysis ( Borenstein, Hedges, Higgins, & Rothstein, 2005 ). There are two add-ins for Microsoft Excel: Mix ( Bax, Yu, Ikeda, Tsuruta, & Moons, 2006 ) and MetaEasy ( Kontopantelis & Reeves, 2009 ). These packages implement many different meta-analysis methods, convert effect sizes, and create plots of study effects. Although it is not 100 percent clear from their website, Comprehensive Meta-Analysis appears to be available only for Windows, Mix works only with Excel 2007 and 2010 in Windows, and MetaEasy works with Excel 2007 (again Windows). RevMan uses Java and so is available for Windows, Linux, and MacOS operating systems. Although RevMan and MetaEasy are free and Mix comes in a free light version, Comprehensive Meta-Analysis and the pro version of Mix are commercial products.
SPSS (a commercial statistics package commonly used by clinical psychologists) does not incorporate a menu-driven option for conducting meta-analysis, but it is possible to use its syntax to run a meta-analysis. Field and Gillett (2010) provide a tutorial on meta-analysis and also include syntax files and examples showing how to run a meta-analysis using SPSS. Other SPSS syntax files can be obtained from Lavesque (2001) and Wilson (2004) .
Meta-analysis can also be conducted with R ( R Development Core Team, 2010 ), a freely available package for conducting a staggering array of statistical procedures. R is free, open-source software available for Windows, MacOS, and Linux that is growing in popularity in the psychology community. Scripts for running a variety of meta-analysis procedures on d are available in the meta package that can be installed into R ( Schwarzer, 2005 ). However, my favorite package for conducting meta-analysis in R is metafor ( Viechtbauer, 2010 ) because it has functions to compute effect sizes from raw data, can work with a wide array of different effect sizes ( d, r , odds ratios, relative risks, risk differences, proportions, and incidence rates), produces publication-standard graphics, and implements moderator analysis and fixed- and random-effects methods (more on this later). It is a brilliant package, and given that it can be used for free across Windows, Linux, and MacOS, I have based this chapter on using this package within R .
Getting Started with R
R ( R Development Core Team, 2010 ) is an environment/language for statistical analysis and is the fastest-growing statistics software. R is a command language: we type in commands that we then execute to see the results. Clinical psychologists are likely to be familiar with the point-and-click graphical user interfaces (GUIs) of packages like SPSS and Excel, and so at first R might appear bewildering. However, I will walk through the process step by step assuming that the reader has no knowledge of R . I cannot, obviously, explain everything there is to know about R , and readers are advised to become familiar with the software by reading one of the many good introductory books (e.g., Crawley, 2007 ; Quick, 2010 ; Verzani, 2004 ; Zuur, Ieno, & Meesters, 2009 ), the best of which, in my entirely objective opinion, is Field, Miles, and Field (2012) .
Once you have installed R on your computer and opened the software, you will see the console window, which contains a prompt at which you type commands. Once a command has been typed, you press the return key to execute it. You can also write commands in a script window and execute them from there, which is my preference—see Chapter 3 of Field and colleagues (2012) . R comes with a basic set of functionality, which can be expanded by installing packages stored at a central online location that has mirrors around the globe. To install the metafor package you need to execute this command:
install.packages(“metafor”)
This command installs the package (you need to be connected to the Internet for this command to work). You need to install the package only once (although whenever you update R you will have to reinstall it), but you need to load the package every time that you want to use it. You do this by executing this command:
library(metafor)
The library() function tells R that you want to use a package (in this case metafor ) in the current session. If you close the program and restart it, then you would need to re-execute the library command to use the metafor package.
The Six Basic Steps of Meta-analysis: An Example
Broadly speaking, there are six sequential steps to conducting a quality meta-analysis: (1) Do a literature search; (2) Decide on inclusion criteria; (3) Calculate the effect sizes; (4) Do the basic meta-analysis; (5) Do some more advanced analysis; and (6) Write it up. In this chapter, to illustrate these six steps, we will use a real dataset from a meta-analysis in which I was involved ( Hanrahan, Field, Jones, & Davey, 2013 ). This meta-analysis looked at the efficacy of cognitive-based treatments for worry in generalized anxiety disorder (GAD), and the part of the analysis that we will use here simply aimed to estimate the efficacy of treatment postintervention and to see whether the type of control group used moderated the effects obtained. This meta-analysis is representative of clinical research in that relatively few studies had addressed this question (it is a small analysis) and sample sizes within each study were relatively small. These data are used as our main example, and the most benefit can be gained from reading the original meta-analysis in conjunction with this chapter. We will now look at each stage of the process of doing a meta-analysis.
Step 1: Do a Literature Search
The first step is to search the literature for studies that have addressed the core/central/same research question using electronic databases such as the ISI Web of Knowledge, PubMed, and PsycInfo. Although the obvious reason for doing this is to find articles, it is also helpful in identifying authors who might have unpublished data (see below). It is often useful to hand-search the reference sections of the articles that you have found to check for articles that you have missed, and to consult directly with noted experts in this literature to ensure that relevant papers have not been missed.
Although it is tempting to assume that meta-analysis is a wonderfully objective tool, it is not without a dash of bias. Possibly the main source of bias is the “file-drawer problem,” or publication bias ( Rosenthal, 1979 ). This bias stems from the reality that significant findings are more likely to be published than nonsignificant findings: significant findings are estimated to be eight times more likely to be submitted than nonsignificant ones ( Greenwald, 1975 ), studies with positive findings are around seven times more likely to be published than studies with results supporting the null hypothesis ( Coursol & Wagner, 1986 ), and 97 percent of articles in psychology journals report significant results ( Sterling, 1959 ). Without rigorous attempts to counteract publication bias, meta-analytic reviews could overestimate population effects because effect sizes in unpublished studies will be smaller ( McLeod & Weisz, 2004 )—up to half the size ( Shadish, 1992 )—of published studies of comparable methodological quality. The best way to minimize the bias is to extend your search to relevant conference proceedings and to contact experts in the field to see if they have or know of any unpublished data. This can be done by direct email to authors in the field, but also by posting a message to a topic-specific newsgroup or email listserv.
In our study, we gathered articles by searching PsycInfo, Web of Science, and Medline for English-language studies using keywords considered relevant. The reference lists of previous meta-analyses and retrieved articles were scanned for relevant studies. Finally, email addresses of published researchers were compiled from retrieved papers, and 52 researchers were emailed and invited to send any unpublished data fitting the inclusion criteria. This search strategy highlights the use of varied resources to ensure that all potentially relevant studies are included and to reduce bias due to the file-drawer problem.
Step 2: Decide on Inclusion Criteria
A second source of bias in a meta-analysis is the inclusion of poorly conducted research. As Field and Gillett (2010) put it:
Although meta-analysis might seem to solve the problem of variance in study quality because these differences will “come out in the wash,” even one red sock (bad study) amongst the white clothes (good studies) can ruin the laundry. (pp. 667–668)
Inclusion criteria depend on the research question being addressed and any specific methodological issues in the field, but the guiding principle is that you want to compare apples with apples, not apples with pears ( Eysenck, 1978 ). In a meta-analysis of CBT, for example, you might decide on a working definition of what constitutes CBT, and maybe exclude studies that do not have proper control groups. It is important to use a precise, reliable, set of criteria that is applied consistently to each potential study so as not to introduce subjective bias into the analysis. In your write-up, you should be explicit about your inclusion criteria and report the number of studies that were excluded at each step of the selection process.
In our analysis, at a first pass we excluded studies based on the following criteria: (a) treatments were considered too distinct to be meaningfully compared to face-to-face therapies (e.g., bibliotherapy, telephone, or computer-administered treatment); (b) subsamples of the data were already included in the meta-analysis because they were published over several papers; and (c) information was insufficient to enable us to compute effect sizes. Within this pool of studies, we set the following inclusion criteria:
Studies that included only those participants who met criteria for a diagnosis of GAD outlined by the DSM since GAD was recognized as an independent disorder; that is, the DSM-III-R, DSM-IV, or DSM-IV-TR (prior to DSM-III-R, GAD was simply a poorly characterized residual diagnostic category). This was to avoid samples being heterogeneous.
Studies in which the majority of participants were aged 18 to 65 years. This was because there may be developmental issues that affect the efficacy of therapy in younger samples.
The Penn State Worry Questionnaire (PSWQ) was used to capture symptom change.
Treatments included were defined as any treatment that used cognitive techniques, either in combination with, or without, behavioral techniques.
To ensure that the highest possible quality of data was included, only studies that used a randomized controlled design were included.
Step 3: Calculate the Effect Sizes
What are effect sizes and how do i calculate them.
Your selected studies are likely to have used different outcome measures, and of course we cannot directly compare raw change on a children's self-report inventory to that being measured using a diagnostic tool such as the Anxiety Disorders Interview Schedule (ADIS). Therefore, we need to standardize the effects within each study so that they can be combined and compared. To do this we convert each effect in each study into one of many standard effect size measures. When quantifying group differences on a continuous measure (such as the PSWQ) people tend to favor Cohen's d ; Pearson's r is used more when looking at associations between measures; and if recovery rates are the primary interest, then it is common to see odds ratios used as the effect size measure.
Once an effect size measure is chosen, you need to compute it for each effect that you want to compare for every paper you want to include in the meta-analysis. A given paper may contain several effect sizes depending on the sorts of questions you are trying to address with your meta-analysis. For example, in a meta-analysis on cognitive impairment in PTSD in which I was involved ( Brewin et al., 2007 ), impairment was measured in a variety of ways in individual studies, and so we had to compute several effect sizes within many of the studies. In this situation, we have to make a decision about how to treat the studies that have produced multiple effect sizes that address the same question. A common solution is to calculate the average effect size across all measures of the same outcome within a study ( Rosenthal, 1991 ), so that every study contributes only one effect to the main analysis (as in Brewin et al., 2007 ).
Computing effect sizes is probably the hardest part of a meta-analysis because the data contained within published articles will vary in their detail and specificity. Some articles will report effect sizes, but many will not; articles might use different effect size metrics; you will feel as though some studies have a grudge against you and are trying to make it as hard for you as possible to extract an effect size. If no effect sizes are reported, then you need to try to use the reported data to calculate one. If using d , then you can use means and standard deviations, odds ratios are easily obtained from frequency data, and most effect size measures (including r ) can be obtained from test statistics such as t , z , χ 2 , and F , or probability values for effects (by converting first to z ). A full description of the various ways in which effect sizes can be computed is beyond the present scope, but there are many freely available means to compute effect sizes from raw data and test statistics; some examples are Wilson (2001 , 2004 ) and DeCoster (1998) . To do the meta-analysis you need not just the effect size, but the corresponding value of its sampling variance ( v ) or standard error ( se ); Wilson (2001) , for example, will give you an estimate of the effect size and the sampling variance.
If a paper does not include sufficient data to calculate an effect size, contact the authors for the raw data, or relevant statistics from which an effect size can be computed. (If you are on the receiving end of such an email please be sympathetic, as attempts to get data out of researchers can be like climbing up a jelly mountain.)
Effect Sizes for Hanrahan and Colleagues’ Study
When reporting a meta-analysis it is a good idea to tabulate the effect sizes with other helpful information (such as the sample size on which the effect size is based, N ) and also to present a stem-and-leaf plot of the effect sizes. For the study conducted by Hanrahan and colleagues, we used d as the effect size measure and corrected for the known bias that d has in small samples using the adjustment described
by Hedges (1981) . In meta-analysis, a stem-and-leaf plot graphically organizes included effect sizes to visualize the shape and central tendency of the effect size distribution across studies included. Table 17.1 shows a stem-and-leaf plot of the resulting effect sizes, and this should be included in the write-up. This stem-and-leaf plot tells us the effect sizes to one decimal place, with the stem reflecting the value before the decimal point and the leaf showing the first decimal place; for example, we know the smallest effect size was d = −0.2, the largest was d = −3.2, and there were effect sizes of 1.2 and 1.4 (for example). Table 17.2 shows the studies included in the Hanrahan and colleagues’ paper, with their corresponding effect sizes (expressed as d ), the sample sizes on which these d s are based, and the standard errors associated with each effect size. Note that the d s match those reported in Table 2 of Hanrahan and colleagues (2013) .
Step 4: Do the Basic Meta-Analysis
Initial considerations.
Meta-analysis aims to estimate the effect in the population (and a confidence interval around it) by combining the effect sizes from different studies using a weighted mean of the effect sizes. The “weight” that is used is usually a value reflecting the sampling precision of the effect size, which is typically a function of sample size. As such, effect sizes with better precision are weighted more highly than effect sizes that are imprecise. There are different methods for estimating the population effects, and these methods have pros and cons. There are two related issues to consider: (1) which method to use and (2) how to conceptualize your data. There are other issues, too, but we will focus on these two because there are articles elsewhere that can be consulted as a next step (e.g., Field, 2001 , 2003a , 2003b , 2005a , 2005b ; Hall & Brannick, 2002 ; Hunter & Schmidt, 2004 ; Rosenthal & DiMatteo, 2001 ; Schulze, 2004 ).
Choosing a Model
It is tempting simply to tell you to use a random-effects model and end the discussion: however, in the interests of informed decision making I will explain why. Meta-analysis can be conceptualized in two ways: fixed- and random-effects models ( Hedges, 1992 ; Hedges & Vevea, 1998 ; Hunter & Schmidt, 2000 ). We can assume that studies in a meta-analysis are sampled from a population in which the average effect size is fixed ( Hunter & Schmidt, 2000 ). Consequently, sample effect sizes should be homogenous. This is the fixed-effects model. The alternative assumption is that the average effect size in the population varies randomly from study to study: population effect sizes can be thought of as being sampled from a “superpopulation” ( Hedges, 1992 ). In this case, because effect sizes come from populations with varying average effect sizes, they should be heterogeneous. This is the random-effects model. Essentially, the researcher using a random-effects model assumes that the studies included represent a mere random sampling of the larger population of studies that could have been conducted on the topic, whereas the researcher using a fixed-effects model assumes that the studies included are the comprehensive set of representative studies. Therefore, the fixed-effects model can be thought to characterize the scope of existing research, and the random-effects model can be thought to afford inferences about a broader population than just the sample of studies analyzed. When effect size variability is explained by a moderator variable that is treated as “fixed,” then the random-effects model becomes a mixed-effects model (see Overton, 1998 ).
Statistically speaking, fixed- and random-effects models differ in the sources of error. Fixed-effects models have error derived from sampling studies from a population of studies. Random-effects models have this error too, but in addition there is error created by sampling the populations from a superpopulation.
The two most widely used methods of meta-analysis are those by Hunter and Schmidt (2004) , which is a random-effects method, and the method by Hedges and colleagues (e.g., Hedges, 1992 ; Hedges & Olkin, 1985 ; Hedges & Vevea, 1998 ), who provide both fixed- and random-effects methods. However, multilevel models can also be used in the context of meta-analysis (see Hox, 2002 , Chapter 8).
Your first decision is whether to conceptualize your model as fixed- or random-effects. You might consider the assumptions that can be realistically made about the populations from which your studies are sampled. There is compelling evidence that real-world data in the social sciences are likely to have variable population parameters ( Field, 2003b ; Hunter & Schmidt, 2000 , 2004 ; National Research Council, 1992 ; Osburn & Callender, 1992 ). Field (2005a) found that the standard deviations of effect sizes for all meta-analytic studies (using r ) published in Psychological Bulletin from 1997 to 2002 ranged from 0 to 0.3 and were most frequently in the region of 0.10 to 0.16; similarly, Barrick and Mount (1991) reported that the standard deviation of effect sizes ( r s) in published datasets was around 0.16.
Second, consider the inferences that you wish to make ( Hedges & Vevea, 1998 ): if you want to make inferences that extend only to the studies included in the meta-analysis ( conditional inferences ), then fixed-effect models are appropriate; however, for inferences that generalize beyond the studies in the meta-analysis ( unconditional inferences ), a random-effects model is appropriate.
Third, consider the consequences of making the “wrong” choice. The consequences of applying fixed-effects methods to random-effects data can be quite dramatic: (1) it inflates the significance tests of the estimate of the population effect from the normal 5 percent to 11 to 28 percent ( Hunter & Schmidt, 2000 ) and 43 to 80 percent ( Field, 2003b ) and (2) published fixed-effects confidence intervals around mean effect sizes have been shown to be, on average, 52 percent narrower than their actual width—these nominal 95 percent fixed-effects confidence intervals were on average 56 percent confidence intervals ( Schmidt, Oh, & Hayes, 2009 ). The consequences of applying random-effects methods to fixed-effects data are considerably less dramatic: in Hedges’ method, for example, when sample effect sizes are homogenous, the additional between-study effect size variance becomes zero, yielding the same result as the fixed-effects method.
This leads me neatly back to my opening sentence of this section: unless you can find a good reason not to, use a random-effects method because (1) social science data normally have heterogeneous effect sizes; (2) psychologists generally want to make inferences that extend beyond the studies in the meta-analysis; and (3) if you apply a random-effects method to homogenous effect sizes, it does not affect the results (certainly not as dramatically as if you apply a fixed-effects model to heterogeneous effect sizes).
Choosing a Method
Let's assume that you trust me (I have an honest face) and opt for a random-effects model. You then need to decide whether to use Hunter and Schmidt, H-S (2004) or Hedges and colleagues’ method (H-V). The technical differences between these methods have been summarized elsewhere ( Field, 2005a ) and will not be repeated here. In a series of Monte Carlo simulations comparing the performance of the Hunter and Schmidt and Hedges and Vevea (fixed- and random-effects) methods, Field (2001 ; but see Hafdahl & Williams, 2009 ) found that when comparing random-effects methods, the Hunter-Schmidt method yielded the most accurate estimates of population correlations across a variety of situations (a view echoed by Hall & Brannick, 2002 , in a similar study). Based on a more extensive set of stimulations, Field (2005a) concluded that in general both H-V and H-S random-effects methods produce accurate estimates of the population effect size. Although there were subtle differences in the accuracy of population effect size estimates across the two methods, in practical terms the bias in both methods was negligible. In terms of 95 percent confidence intervals around the population estimate, Hedges’ method was in general better at achieving these intervals (the intervals for Hunter and Schmidt's method tended to be too narrow, probably because they recommend using credibility intervals and not confidence intervals).
Hunter and Schmidt's method involves psychometric corrections for the attenuation of observed effect sizes that can be caused by measurement error ( Hunter, Schmidt, & Le, 2006 ), and these psychometric corrections can be incorporated into the H-V method if correlations are used as the effect size, but these corrections were not explored in the studies mentioned above, which limits what they can tell us. Therefore, diligent researchers might consult the various tables in Field (2005a) to assess which method might be most accurate for the given parameters of the meta-analysis that they are about to conduct; however, the small differences between the methods will probably not make a substantive impact on the conclusions that will be drawn from the analysis.
Entering the Data into R
Having computed the effect sizes, we need to enter these into R . In R , commands follow the basic structure of:
Object 〈- Instructions about how to create the object
Therefore, to create an object that is a variable, we give it a name on the left-hand side of the arrow, and on the right-hand side input the data that makes up the variable. To input data we use the c() function, which simply binds things together into a single object (in this case it binds the different values of d into a single object or variable). To enter the value of d from Table 17.2 , we would execute:
d 〈- c(1.42, 0.68, −0.17, 2.57, 0.82, 0.13, 0.45, 0.31, 2.44, 3.22, −0.08, 2.25, 0.89, 1.23, 0.27, 2.22, 0.31, 0.83, 0.26)
Executing this command creates an object that we have named “d” (we could have named it “Thelma” if we wanted to, but “d” seems like a fairly descriptive name in the circumstances). If we want to view this variable, we simply execute its name:
〉d [1] 1.42 0.68 −0.17 2.57 0.82 0.13 0.45 0.31 2.44 3.22 −0.08 2.25 0.89 1.23 0.27 2.22 0.31 0.83 0.26
We can enter the standard errors from Table 17.2 in a similar way; this time we create an object that we have decided to call “sed”:
sed 〈- c(0.278, 0.186, 0.218, 0.590, 0.313, 0.293, 0.230, 0.263, 0.468, 0.609, 0.394, 0.587, 0.284, 0.299, 0.291, 0.490, 0.343, 0.336, 0.323)
Next I'm going to create a variable that gives each effect size a label of the first author of the study from which the effect came and the year. We can do this by executing (note that to enter text strings instead of numbers, we place the text in quotes so that R knows the data are text strings):
study 〈-c(“v.d. Heiden (2010)”, “v.d. Heiden (2010)”, “Newman (2010)”, “Wells (2010)”, “Dugas (2010)”, “Dugas (2010)”, “Westra (2009)”, “Leichsenring (2009)”, “Roemer (2008)”, “Rezvan (2008)”, “Rezvan (2008)”, “Zinbarg (2007)”, “Gosselin (2006)”, “Dugas (2003)”, “Borkovec (2002)”, “Ladouceur (2000)”, “Ost (2000)”, “Borkovec (1993)”, “Borkovec (1993)”)
We also have some information about the type of control group used. We'll come back to this later, but if we want to record this information, we can do so using a coding variable. We need to enter values that represent the different types of control group, and then to tell R what these values represent. Let's imagine that we want to code non-therapy controls as 0, CT as 1, and non-CT as 2. First we can enter these values into R :
controlType 〈- c(0, 1, 1, 2, 0, 2, 1, 2, 0, 0, 1, 0, 2, 0, 1, 0, 2, 2, 2)
Next, we need to tell R that this variable is a coding variable (a.k.a. a factor), using the factor() function. Within this function we name the variable that we want to convert (in this case controlType ), we tell R what numerical values we have used to code levels of the factor by specifying levels = 0:2 (0:2 means zero to 2 inclusive, so we are specifying levels of 0, 1, 2), we then tell it what labels to attach to those levels (in the order of the numerical codes) by including labels = c( “Non-Therapy”, “CT”, “Non-CT”) . Therefore, to turn controlType into a factor based on itself, we execute:
controlType 〈- factor(controlType, levels = 0:2, labels = c(“Non-Therapy”, “CT”, “Non-CT”))
We now have four variables containing data: d (the effect sizes), sed (their standard errors), study (a string variable that identifies the study from which the effect came), and controlType (a categorical variable that defines what control group was used for each effect size). We can combine these variables into a data frame by executing:
GAD.data 〈- data.frame(study, controlType, d, sed)
This creates an object called GAD.data (note that in R you cannot use spaces when you name objects) that is a data frame made up of the four variables that we have just created. To “see” this data frame, execute its name:
〉 GAD.data study controlType d sed 1 v.d. Heiden (2010) Non-Therapy 1.42 0.278 2 v.d. Heiden (2010) CT 0.68 0.186 3 Newman (2010) CT -0.17 0.218 4 Wells (2010) Non-CT 2.57 0.590 5 Dugas (2010) Non-Therapy 0.82 0.313 6 Dugas (2010) Non-CT 0.13 0.293 7 Westra (2009) CT 0.45 0.230 8 Leichsenring (2009) Non-CT 0.31 0.263 9 Roemer (2008) Non-Therapy 2.44 0.468 10 Rezvan (2008) Non-Therapy 3.22 0.609 11 Rezvan (2008) CT -0.08 0.394 12 Zinbarg (2007) Non-Therapy 2.25 0.587 13 Gosselin (2006) Non-CT 0.89 0.284 14 Dugas (2003) Non-Therapy 1.23 0.299 15 Borkovec (2002) CT 0.27 0.291 16 Ladouceur (2000) Non-Therapy 2.22 0.490 17 Ost (2000) Non-CT 0.31 0.343 18 Borkovec (1993) Non-CT 0.83 0.336 19 Borkovec (1993) Non-CT 0.26 0.323
You can also prepare the data as a tab-delimited or comma-separated text file (using Excel, SPSS, Stata, or whatever other software you like) and read this file into R using the read.delim() or read.csv() functions. In both cases, you could use the choose.file() function to open a standard dialogue box that lets you choose the file by navigating your file system. 1 For example, to create a data frame called “GAD.data” from a tab-delimited file (.dat), you would execute:
GAD.data 〈 - read.delim(file.choose(), header = TRUE)
Similarly, to create a data frame from a comma-separated text file, you would execute:
GAD.data 〈- read.csv(file.choose(), header = TRUE)
In both cases the header = TRUE option is used if you have variable names in the first row of the data file; if you do not have variable names in the file, omit this option (the default value is false). If this data-entry section has confused you, then read Chapter 3 of Field and colleagues (2012) or your preferred introductory book on R .
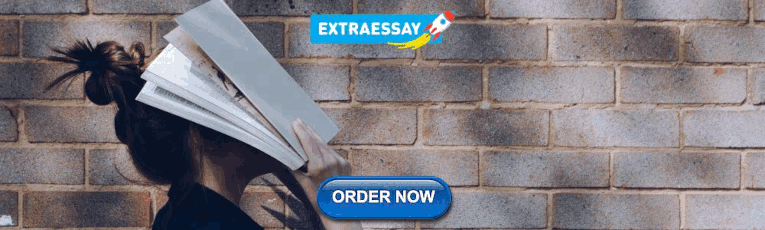
Doing the Meta-analysis
To do a basic meta-analysis you use the rma() function. This function has the following general format when using the standard error of effect sizes:
maModel 〈- rma(yi = variable containing effect sizes , sei = variable containing standard error of effect sizes , data = dataFrame , method = “DL”)
maModel is whatever name you want to give your model (but remember you can't use spaces), variable containing effect sizes is replaced with the name of the variable containing your effect sizes, variable containing standard error of effect sizes is replaced with the name of the variable that contains the standard errors, and dataFrame is the name of the data frame containing these variables.
When using the variance of effect sizes we substitute the sei option with vi :
maModel 〈- rma(yi = variable containing effect sizes , vi = variable containing variance of effect sizes , data = dataFrame , method = “DL”)
Therefore, for our GAD analysis, we can execute:
maGAD 〈- rma(yi = d, sei = sed, data = GAD.data, method = “DL”)
This creates an object called maGAD by using the rma() function. Within this function, we have told R that we want to use the object GAD.data as our data frame, and that within this data frame, the variable d contains the effect sizes ( yi = d ) and the variable sed contains the standard errors ( sei = sed ). Finally, we have set the method to be “DL,” which will use the DerSimonian-Laird estimator (which is used in the H-V random-effects method). We can change how the model is estimated by changing this option, which can be set to the following:
method = “FE”: produces a fixed-effects meta-analysis
method = “HS” = random effects using the Hunter-Schmidt estimator
method = “HE” = random effects using the Hedges estimator
method = “DL” = random effects using the DerSimonian-Laird estimator
method = “SJ” = random effects using the Sidik-Jonkman estimator
method = “ML” = random effects using the maximum-likelihood estimator
method = “REML” = random effects using the restricted maximum-likelihood estimator (this is the default if you don't specify a method at all)
method = “EB” = random effects using the empirical Bayes estimator.
To see the results of the analysis we need to use the summary() function and put the name of the model within it:
summary(maGAD)
The resulting output can be seen in Figure 17.2 . This output is fairly self-explanatory 2 . For example, we can see that for Hedges and Vevea's method, the Q statistic, which measures heterogeneity in effect sizes, is highly significant, χ 2 (18) = 100.50, p 〈 .001. The estimate of between-study variability, τ 2 = 0.44 (most important, this is not zero), and the proportion of variability due to heterogeneity, I 2 , was 82.09 percent. In other words, there was a lot of variability in study effects. The population effect size and its 95 percent confidence interval are: 0.93 (CI .95 = 0.59 (lower), 1.27 (upper)). We can also see that this population effect size is significant, z = 5.40, p 〈 .001.
R output for a basic meta-analysis.
Based on the homogeneity estimates and tests, we could say that there was considerable variation in effect sizes overall. Also, based on the estimate of population effect size and its confidence interval, we could conclude that there was a strong effect of CT for GAD compared to controls.
Creating a forest plot of the studies and their effect sizes is very easy after having created the meta-analysis model. We simply place the name of the model within the forest() command and execute:
forest(maGAD)
However, I want to add the study labels to the plot, so let's execute:
forest(maGAD, slab = GAD.data$study)
By adding slab = GAD.data$study to the command we introduce study labels (that's what slab stands for) and the labels we use are in the variable called study within the GAD.data data frame (that's what GAD.data$study means). The resulting figure is in Figure 17.3 . It shows each study with a square indicating the effect size from that study (the size of the square is proportional to the weight used in the meta-analysis, so we can see that the first three studies were weighted fairly heavily). The branches of each effect size represent the confidence interval of the effect size. Also note that because we added the slab option, our effects have been annotated using the names in the variable called study in our data frame. Looking at this plot, we can see that there are five studies that produced fairly substantially bigger effects than the rest, and two studies with effect sizes below zero (the dotted line), which therefore showed that CBT was worse than controls. The diamond at the bottom shows the population effect size based on these individual studies (it is the value of the population effect size from our analysis). The forest plot is a very useful way to summarize the studies in the meta-analysis.
Step 5: Do Some More Advanced Analysis
Estimating publication bias.
Various techniques have been developed to estimate the effect of publication bias and to correct for it. The earliest and most commonly reported estimate of publication bias is Rosenthal's (1979) fail-safe N . This was an elegant and easily understood method for estimating the number of unpublished studies that would need to exist to turn a significant population effect size estimate into a nonsignificant one. However, because significance testing the estimate of the population effect size is not really the reason for doing a meta-analysis, the fail-safe N is fairly limited.
The funnel plot ( Light & Pillerner, 1984 ) is a simple and effective graphical technique for exploring potential publication bias. A funnel plot displays effect sizes plotted against the sample size, standard error, conditional variance, or some other measure of the precision of the estimate. An unbiased sample would ideally show a cloud of data points that is symmetrical around the population effect size and has the shape of a funnel. This funnel shape reflects the greater variability in effect sizes from studies with small sample sizes/less precision, and the estimates drawn from larger/more precise studies converging around the population effect size. A sample with publication bias will lack symmetry because studies based on small samples that showed small effects will be less likely to be published than studies based on the same-sized samples that showed larger effects ( Macaskill, Walter, & Irwig, 2001 ).
Forest plot of the GAD data.
Funnel plots should be used as a first step before further analysis because factors other than publication bias can cause asymmetry. Some examples are data irregularities including fraud and poor study design ( Egger, Smith, Schneider, & Minder, 1997 ), true heterogeneity of effect sizes (in intervention studies this can happen because the intervention is more intensely delivered in smaller, more personalized studies), and English-language bias (studies with smaller effects are often found in non–English-language journals and get overlooked in the literature search).
To get a funnel plot for a meta-analysis model created in R , we simply place that model into the funnel() function and execute:
funnel(maGAD)
Figure 17.4 shows the resulting funnel plot, which is clearly not symmetrical. The studies with large standard errors (bottom right) consistently produce the largest effect sizes, and the studies are not evenly distributed around the mean effect size (or within the unshaded triangle). This graph shows clear publication bias.
Funnel plots offer no means to correct for any bias detected. Trim and fill ( Duval & Tweedie, 2000 ) is a method in which a biased funnel plot is truncated (“trimmed”) and the number ( k ) of missing studies from the truncated part is estimated. Next, k artificial studies are added (“filled”) to the negative side of the funnel plot (and therefore have small effect sizes) so that in effect the study now contains k new “studies” with effect sizes as small in magnitude as the k largest effect sizes that were trimmed. The new “filled” effects are presumed to represent the magnitude of effects identified in hypothetical unpublished studies. A new estimate of the population effect size is then calculated including these artificially small effect sizes. Vevea and Woods (2005) point out that this method can lead to overcorrection because it relies on the strict assumption that all of the “missing” studies are those with the smallest effect sizes. Vevea and Woods propose a more sophisticated correction method based on weight function models of publication bias. These methods use weights to model the process through which the likelihood of a study being published varies (usually based on a criterion such as the significance of a study). Their method can be applied to even small meta-analyses and is relatively flexible in allowing meta-analysts to specify the likely conditions of publication bias in their particular research scenario. (The downside of this flexibility is that it can be hard to know what the precise conditions are.) They specify four typical weight functions: “moderate one-tailed selection,” “severe one-tailed selection,” “moderate two-tailed selection,” and “severe two-tailed selection”; however, they recommend adapting the weight functions based on what the funnel plot reveals (see Vevea & Woods, 2005 ). These corrections can be applied in R (see Field & Gillett, 2010 , for a tutorial) but do not form part of the metafor package and are a little too technical for this introductory chapter.
Funnel plot of the GAD data.
Moderator Analysis
When there is variability in effect sizes, it can be useful to try to explain this variability using theoretically driven predictors of effect sizes. For example, in our dataset there were three different types of control group used: non-therapy (waitlist), non-CT therapy, and CT therapy. We might reasonably expect effects to be stronger if a waitlist control was used in the study compared to a CT control because the waitlist control gets no treatment at all, whereas CT controls get some treatment. We can test for this using a mixed model (i.e., a random-effects model in which we add a fixed effect).
Moderator models assume a general linear model in which each effect size can be predicted from the moderator effect (represented by β 1 ):
The within-study error variance is represented by e i . To calculate the moderator effect, β 1 , a generalized least squares (GLS) estimate is calculated. It is not necessary to know the mathematics behind the process (if you are interested, then read Field, 2003b ; Overton, 1998 ); the main thing to understand is that we're just doing a regression in which effect sizes are predicted. Like any form of regression we can, therefore, predict effect sizes from either continuous variables (such as study quality) or categorical ones (which will be dummy coded using contrast weights).
The package metafor allows both continuous and categorical predictors (moderators) to be entered into the regression model that a researcher wishes to test. Moderator variables are added by including the mods option to the basic meta-analysis command. You can enter a single moderator by specifying mods = variableName (in which variableName is the name of the moderator variable that you want to enter into the model) or enter several moderator variables by including mods = matrixName (in which matrixName is the name of a matrix that contains values of moderator variables in each of its columns). Continuous variables are treated as they are; for categorical variables, you should either dummy code them manually or use the factor() function, as we did earlier, in which case R does the dummy coding for you.
Therefore, in our example, we can add the variable controlType as a moderator by rerunning the model including mods = controlType into the command. This variable is categorical, but because we converted it to a factor earlier on, R will treat it as a dummy-coded categorical variable. The rest of the command is identical to before:
modGAD 〈- rma(yi = d, sei = sed, data = GAD.data, mods = controlType, method = “DL”) summary(modGAD)
The resulting output is shown in Figure 17.5 . This output is fairly self-explanatory; for example, we can see that for Hedges and Vevea's method, the estimate of between-study variability, χ 2 = 0.33, is less than it was before (it was 0.44), which means that our moderator variable has explained some variance. However, there is still a significant amount left to explain, χ 2 (17) = 76.35, p 〈 .001.
Output from R for moderation analysis.
The Q statistic shows that the amount of variance explained by controlType is highly significant, χ 2 (1) = 8.93, p =.0028. In other words, it is a significant predictor of effect sizes. The beta parameter for the moderator and its 95 percent confidence interval are: −0.55, CI .95 = −0.92 (lower), −0.19 (upper). We can also see that this parameter is significant, z = −3.11, p = .0028 (note that the p value is identical to the Q statistic because they're testing the same thing). In a nutshell, then, the type of control group had a significant impact on the effect that CT had on GAD (measured by the PSWQ). We could break this effect apart by running the main meta-analysis on the three control groups separately.
Step 6: Write It Up
There are several detailed guidelines on how to write up a meta-analysis. For clinical trials, the QUORUM and PRISMA guidelines are particularly useful ( Moher et al., 1999 ; Moher, Liberati, Tetzlaff, Altman, & Grp, 2009 ), and more generally the American Psychological Association (APA) has published its own Meta-Analysis Reporting Standards (MARS; H. Cooper, Maxwell, Stone, Sher, & Board, 2008 ). In addition, there are individual articles that offer advice (e.g., Field & Gillett, 2010 ; Rosenthal, 1995 ). There is a lot of overlap in these guidelines, and Table 17.3 assimilates them in an attempt to give a thorough overview of the structure and content of a meta-analysis article. This table should need no elaboration, but it is worth highlighting some of the key messages:
Introduction : Be clear about the rationale for the meta-analysis: What are the theoretical, practical, or policy drivers of the research? Why is a meta-analysis necessary? What hypotheses do you have?
Methods : Be clear about your search and inclusion criteria. How did you reach the final sample of studies? The PRISMA guidelines suggest including a flowchart of the selection process: Figure 17.6 shows the type of flowchart suggested by PRISMA, which outlines the number of studies retained and eliminated at each phase of the selection process. Also, state your computational and analytic methods in sufficient detail: Which effect size measure are you using (and did you have any issues in computing these)? Which meta-analytic technique did you apply to the data and why? Did you do a subgroup or moderator analysis?
Results : Include a graphical summary of the effect sizes included in the study. A forest plot is a very effective way to show the reader the raw data. When there are too many studies for a forest plot, consider a stem-and-leaf plot. A summary table of studies and any important study characteristics/moderator variables is helpful. If you have carried out a moderator analysis, then you might also provide stem-and-leaf plots or forest plots for subgroups of the analysis. Always report statistics relating to the variability of effect sizes (these should include the actual estimate of variability as well as statistical tests of variability), and obviously the estimate of the population effect size and its associated confidence interval (or credibility interval). Report information on publication bias (e.g., a forest plot) and preferably a sensitivity analysis (e.g., Vevea and Woods’ method).
Discussion : Pull out the key theoretical, policy, or practical messages that emerge from the analysis. Discuss any limitations or potential sources of bias within the analysis. Finally, it is helpful to make a clear statement about how the results inform the future research agenda.
The PRISMA-recommended flowchart.
This chapter offered a preliminary but comprehensive overview of the main issues when conducting a meta-analysis. We also used some real data to show how the metafor package in R can be used to conduct the analysis. The analysis begins by collecting articles about the research question you are trying to address through a variety of methods: emailing people in the field for unpublished studies, electronic searches, searches of conference abstracts, and so forth. Next, inclusion criteria should be devised that reflect the concerns pertinent to the particular research question (which might include the type of control group used, diagnostic measures, quality of outcome measure, type of treatment used, or other factors that ensure a minimum level of research quality). Statistical details are then extracted from the papers from which effect sizes can be calculated; the same effect size metric should be used for all studies, and you need to compute the variance or standard error for each effect size too. Choose the type of analysis appropriate for your particular situation (fixed- vs. random-effects, Hedges’ method or Hunter and Schmidt's, etc.), and then apply this method to the data. Describe the effect of publication bias descriptively (e.g., funnel plots), and consider investigating how to re-estimate the population effect under various publication-bias models using Vevea and Woods’ (2005) model. Finally, when reporting the results, make sure that the reader has clear information about the distribution of effect sizes (e.g., a stem-and-leaf plot), the effect size variability, the estimate of the population effect and its 95 percent confidence interval, the extent of publication bias, and whether any moderator variables were explored.
Useful Web Links
Comprehensive Meta-Analysis: http://www.meta-analysis.com/
MetaEasy: http://www.statanalysis.co.uk/meta-analysis.html
metafor package for R : http://www.metafor-project.org/
Mix: http://www.meta-analysis-made-easy.com/
PRISMA (guidelines and checklists for reporting meta-analysis): http://www.prisma-statement.org/
R : http://www.r-project.org/
Review Manager: http://ims.cochrane.org/revman
SPSS (materials accompanying Field & Gillett, 2010 ): http://www.discoveringstatistics.com/meta_analysis/how_to_do_a_meta_analysis.html
I generally find it easier to export from SPSS to a tab-delimited file because this format can also be read by software packages other than R. However, you can read SPSS data files (.sav) into R directly using the read.spss() function, but you need to first install and load a package called foreign .
There are slight differences in the decimal places between the results reported here and those on page 125 of Hanrahan and colleagues’ papers because we did not round effect sizes and their standard errors to 2 and 3 decimal places respectively before conducting the analysis.
Bar-Haim, Y. , Lamy, D. , Pergamin, L. , Bakermans-Kranenburg, M. J. , & van Ijzendoorn, M. H. ( 2007 ). Threat-related attentional bias in anxious and nonanxious individuals: A meta-analytic study. Psychological Bulletin , 133 (1), 1–24. doi: 10.1037/0033-2909.133.1.1
Google Scholar
Barbato, A. , & D'Avanzo, B. ( 2008 ). Efficacy of couple therapy as a treatment for depression: A meta-analysis. Psychiatric Quarterly , 79 (2), 121–132. doi: 10.1007/s11126-008-9068-0
Barrick, M. R. , & Mount, M. K. ( 1991 ). The big 5 personality dimensions and job-performance—a meta-analysis. Personnel Psychology , 44 (1), 1–26.
Bax, L. , Yu, L. M. , Ikeda, N. , Tsuruta, H. , & Moons, K. G. M. ( 2006 ). Development and validation of MIX: Comprehensive free software for meta-analysis of causal research data. BMC Medical Research Methodology , 6 (50). http://www.biomedcentral.com/1471-2288/6/50
Bloch, M. H. , Landeros-Weisenberger, A. , Rosario, M. C. , Pittenger, C. , & Leckman, J. F. ( 2008 ). Meta-analysis of the symptom structure of obsessive-compulsive disorder. American Journal of Psychiatry , 165 (12), 1532–1542. doi: 10.1176/appi.ajp.2008.08020320
Borenstein, M. , Hedges, L. , Higgins, J. , & Rothstein, H. ( 2005 ). Comprehensive meta-analysis (Version 2). Englewood, NJ: Biostat. Retrieved from http://www.meta-analysis.com/
Google Preview
Bradley, R. , Greene, J. , Russ, E. , Dutra, L. , & Westen, D. ( 2005 ). A multidimensional meta-analysis of psychotherapy for PTSD. American Journal of Psychiatry , 162 (2), 214–227. doi: 10.1176/appi.ajp.162.2.214
Brewin, C. R. , Kleiner, J. S. , Vasterling, J. J. , & Field, A. P. ( 2007 ). Memory for emotionally neutral information in posttraumatic stress disorder: A meta-analytic investigation. Journal of Abnormal Psychology , 116 (3), 448–463. doi: Doi 10.1037/0021-843x.116.3.448
Burt, S. A. ( 2009 ). Rethinking environmental contributions to child and adolescent psychopathology: a meta-analysis of shared environmental influences. Psychological Bulletin , 135 (4), 608–637. doi: 10.1037/a0015702
Cartwright-Hatton, S. , Roberts, C. , Chitsabesan, P. , Fothergill, C. , & Harrington, R. ( 2004 ). Systematic review of the efficacy of cognitive behaviour therapies for childhood and adolescent anxiety disorders. British Journal of Clinical Psychology , 43 , 421–436.
Chan, R. C. K. , Xu, T. , Heinrichs, R. W. , Yu, Y. , & Wang, Y. ( 2010 ). Neurological soft signs in schizophrenia: a meta-analysis. Schizophrenia Bulletin , 36 (6), 1089–1104. doi: 10.1093/schbul/sbp011
Cooper, H. , Maxwell, S. , Stone, A. , Sher, K. J. , & Board, A. P. C. ( 2008 ). Reporting standards for research in psychology: Why do we need them? What might they be? American Psychologist , 63 (9), 839–851.
Cooper, H. M. ( 2010 ). Research synthesis and meta-analysis: a step-by-step approach (4th ed.). Thousand Oaks, CA: Sage.
Coursol, A. , & Wagner, E. E. ( 1986 ). Effect of positive findings on submission and acceptance rates: A note on meta-analysis bias. Professional Psychology , 17 , 136–137.
Covin, R. , Ouimet, A. J. , Seeds, P. M. , & Dozois, D. J. A. ( 2008 ). A meta-analysis of CBT for pathological worry among clients with GAD. Journal of Anxiety Disorders , 22 (1), 108–116. doi: 10.1016/j.janxdis.2007.01.002
Crawley, M. J. ( 2007 ). The R book . Chichester: Wiley-Blackwell.
Cuijpers, P. , Li, J. , Hofmann, S. G. , & Andersson, G. ( 2010 ). Self-reported versus clinician-rated symptoms of depression as outcome measures in psychotherapy research on depression: A meta-analysis. Clinical Psychology Review , 30 (6), 768–778. doi: 10.1016/j.cpr.2010.06.001
DeCoster, J. (1998). Microsoft Excel spreadsheets: Meta-analysis . Retrieved October 1, 2006, from http://www.stat-help.com/spreadsheets.html
Duval, S. J. , & Tweedie, R. L. ( 2000 ). A nonparametric “trim and fill” method of accounting for publication bias in meta-analysis. Journal of the American Statistical Association , 95 (449), 89–98.
Egger, M. , Smith, G. D. , Schneider, M. , & Minder, C. ( 1997 ). Bias in meta-analysis detected by a simple, graphical test. British Medical Journal , 315 (7109), 629–634.
Eysenck, H. J. ( 1978 ). Exercise in mega-silliness. American Psychologist , 33 (5), 517–517.
Field, A. P. ( 2001 ). Meta-analysis of correlation coefficients: A Monte Carlo comparison of fixed- and random-effects methods. Psychological Methods , 6 (2), 161–180.
Field, A. P. ( 2003 a). Can meta-analysis be trusted? Psychologist , 16 (12), 642–645.
Field, A. P. ( 2003 b). The problems in using fixed-effects models of meta-analysis on real-world data. Understanding Statistics , 2 , 77–96.
Field, A. P. ( 2005 a). Is the meta-analysis of correlation coefficients accurate when population correlations vary? Psychological Methods , 10 (4), 444–467.
Field, A. P. ( 2005 b). Meta-analysis. In J. Miles & P. Gilbert (Eds.), A handbook of research methods in clinical and health psychology (pp. 295–308). Oxford: Oxford University Press.
Field, A. P. ( 2009 ). Meta-analysis. In R. E. Millsap & A. Maydeu-Olivares (Eds.), The SAGE handbook of quantitative methods in psychology (pp. 404–422). London: Sage.
Field, A. P. , & Gillett, R. ( 2010 ). How to do a meta-analysis. British Journal of Mathematical & Statistical Psychology , 63 , 665–694.
Field, A. P. , Miles, J. N. V. , & Field, Z. C. ( 2012 ). Discovering statistics using R: And sex and drugs and rock ‘n’ roll . London: Sage.
Fisher, R. A. ( 1935 ). The design of experiments . Edinburgh: Oliver & Boyd.
Fisher, R. A. ( 1938 ). Statistical methods for research workers (7th ed.). London: Oliver & Boyd.
Furr, J. M. , Corner, J. S. , Edmunds, J. M. , & Kendall, P. C. ( 2010 ). Disasters and youth: a meta-analytic examination of posttraumatic stress. Journal of Consulting and Clinical Psychology , 78 (6), 765–780. doi: 10.1037/A0021482
Glass, G. V. ( 1976 ). Primary, secondary, and meta-analysis of research. Educational Researcher , 5 (10), 3–8.
Greenwald, A. G. ( 1975 ). Consequences of prejudice against null hypothesis. Psychological Bulletin , 82 (1), 1–19.
Hafdahl, A. R. , & Williams, M. A. ( 2009 ). Meta-analysis of correlations revisited: Attempted replication and extension of Field's (2001) simulation studies. Psychological Methods , 14 (1), 24–42. doi: 10.1037/a0014697
Hall, S. M. , & Brannick, M. T. ( 2002 ). Comparison of two random-effects methods of meta-analysis. Journal of Applied Psychology , 87 (2), 377–389.
Hanrahan, F. , Field, A. P. , Jones, F. , & Davey, G. C. L. ( 2013 ). A meta-analysis of cognitive-behavior therapy for worry in generalized anxiety disorder. Clinical Psychology Review , 33 , 120–132..
Hedges, L. ( 1981 ). Distribution Theory for glass's estimator of effect size and related estimators. Journal of Educational Statistics , 6 , 107–128.
Hedges, L. V. ( 1992 ). Meta-analysis. Journal of Educational Statistics , 17 (4), 279–296.
Hedges, L. V. , & Olkin, I. ( 1985 ). Statistical methods for meta-analysis . Orlando, FL: Academic Press.
Hedges, L. V. , & Vevea, J. L. ( 1998 ). Fixed- and random-effects models in meta-analysis. Psychological Methods , 3 (4), 486–504.
Hendriks, G. J. , Voshaar, R. C. O. , Keijsers, G. P. J. , Hoogduin, C. A. L. , & van Balkom, A. J. L. M. ( 2008 ). Cognitive-behavioural therapy for late-life anxiety disorders: a systematic review and meta-analysis. Acta Psychiatrica Scandinavica , 117 (6), 403–411. doi: 10.1111/j.1600-0447.2008.01190.x
Hox, J. J. ( 2002 ). Multilevel analysis, techniques and applications . Mahwah, NJ: Lawrence Erlbaum Associates.
Hunter, J. E. , & Schmidt, F. L. ( 1990 ). Methods of meta-analysis: correcting error and bias in research findings . Newbury Park, CA: Sage.
Hunter, J. E. , & Schmidt, F. L. ( 2000 ). Fixed effects vs. random effects meta-analysis models: Implications for cumulative research knowledge. International Journal of Selection and Assessment , 8 (4), 275–292.
Hunter, J. E. , & Schmidt, F. L. ( 2004 ). Methods of meta-analysis: Correcting error and bias in research findings (2nd ed.). Newbury Park, CA: Sage.
Hunter, J. E. , Schmidt, F. L. , & Le, H. ( 2006 ). Implications of direct and indirect range restriction for meta-analysis methods and findings. Journal of Applied Psychology , 91 (3), 594–612. doi: 10.1037/0021-9010.91.3.594
Kashdan, T. B. ( 2007 ). Social anxiety spectrum and diminished positive experiences: Theoretical synthesis and meta-analysis. Clinical Psychology Review , 27 (3), 348–365. doi: 10.1016/j.cpr.2006.12.003
Kleinstaeuber, M. , Witthoeft, M. , & Hiller, W. ( 2011 ). Efficacy of short-term psychotherapy for multiple medically unexplained physical symptoms: A meta-analysis. Clinical Psychology Review , 31 (1), 146–160. doi: 10.1016/j.cpr.2010.09.001
Kontopantelis, E. , & Reeves, D. ( 2009 ). MetaEasy: A meta-analysis add-in for Microsoft Excel. Journal of Statistical Software , 30 (7). http://www.jstatsoft.org/v30/i07/paper
Lavesque, R. (2001). Syntax: meta-analysis . Retrieved October 1, 2006, from http://www.spsstools.net/
Light, R. J. , & Pillerner, D. B. ( 1984 ). Summing up: The science of reviewing research . Cambridge, MA: Harvard University Press.
Macaskill, P. , Walter, S. D. , & Irwig, L. ( 2001 ). A comparison of methods to detect publication bias in meta-analysis. Statistics in Medicine , 20 (4), 641–654.
Malouff, J. A. , Thorsteinsson, E. B. , Rooke, S. E. , Bhullar, N. , & Schutte, N. S. ( 2008 ). Efficacy of cognitive behavioral therapy for chronic fatigue syndrome: A meta-analysis. Clinical Psychology Review , 28 (5), 736–745. doi: 10.1016/j.cpr.2007.10.004
McLeod, B. D. , & Weisz, J. R. ( 2004 ). Using dissertations to examine potential bias in child and adolescent clinical trials. Journal of Consulting and Clinical Psychology , 72 (2), 235–251.
Moher, D. , Cook, D. J. , Eastwood, S. , Olkin, I. , Rennie, D. , Stroup, D. F. , et al. ( 1999 ). Improving the quality of reports of meta-analyses of randomised controlled trials: the QUOROM statement. Lancet , 354 (9193), 1896–1900.
Moher, D. , Liberati, A. , Tetzlaff, J. , Altman, D. G. , & Grp, P. ( 2009 ). Preferred reporting items for systematic reviews and meta-analyses: the PRISMA Statement. Journal of Clinical Epidemiology , 62 (10), 1006–1012. doi: 10.1016/J.Jclinepi.2009.06.005
Mychailyszyn, M.P. , Brodman, D. , Read, K.L. , & Kendall, P.C. ( 2012 ). Cognitive-behavioral school-based interventions for anxious and depressed youth: A meta-analysis of outcomes. Clinical Psychology: Science and Practice , 19 (2), 129–153.
National Research Council. ( 1992 ). Combining information: Statistical issues and opportunities for research . Washington, D.C.: National Academy Press.
Osburn, H. G. , & Callender, J. ( 1992 ). A note on the sampling variance of the mean uncorrected correlation in meta-analysis and validity generalization. Journal of Applied Psychology , 77 (2), 115–122.
Overton, R. C. ( 1998 ). A comparison of fixed-effects and mixed (random-effects) models for meta-analysis tests of moderator variable effects. Psychological Methods , 3 (3), 354–379.
Parsons, T. D. , & Rizzo, A. A. ( 2008 ). Affective outcomes of virtual reality exposure therapy for anxiety and specific phobias: A meta-analysis. Journal of Behavior Therapy and Experimental Psychiatry , 39 (3), 250–261. doi: 10.1016/j.jbtep.2007.07.007
Pearson, E. S. ( 1938 ). The probability integral transformation for testing goodness of fit and combining tests of significance. Biometrika , 30 , 134–148.
Quick, J. M. ( 2010 ). The statistical analysis with R beginners guide . Birmingham: Packt.
R Development Core Team. ( 2010 ). R: A language and environment for statistical computing . Vienna, Austria: R Foundation for Statistical Computing. Retrieved from http://www.R-project.org
Roberts, N. P. , Kitchiner, N. J. , Kenardy, J. , Bisson, J. I. , & Psych, F. R. C. ( 2009 ). Systematic review and meta-analysis of multiple-session early interventions following traumatic Eventse American Journal of Psychiatry , 166 (3), 293–301. doi: 10.1176/appi.ajp.2008.08040590
Rosa-Alcazar, A. I. , Sanchez-Meca, J. , Gomez-Conesa, A. , & Marin-Martinez, F. ( 2008 ). Psychological treatment of obsessive-compulsive disorder: A meta-analysis. Clinical Psychology Review , 28 (8), 1310–1325. doi: 10.1016/j.cpr.2008.07.001
Rosenthal, R. ( 1978 ). Combining results of independent studies. Psychological Bulletin , 85 (1), 185–193.
Rosenthal, R. ( 1979 ). The file drawer problem and tolerance for null results. Psychological Bulletin , 86 (3), 638–641.
Rosenthal, R. ( 1984 ). Meta-analytic procedures for social research . Beverly Hills, CA: Sage.
Rosenthal, R. ( 1991 ). Meta-analytic procedures for social research (2nd ed.). Newbury Park, CA: Sage.
Rosenthal, R. ( 1995 ). Writing meta-analytic reviews. Psychological Bulletin , 118 (2), 183–192.
Rosenthal, R. , & DiMatteo, M. R. ( 2001 ). Meta-analysis: Recent developments in quantitative methods for literature reviews. Annual Review of Psychology , 52 , 59–82.
Rosenthal, R. , & Rubin, D. B. ( 1978 ). Interpersonal expectancy effects: the first 345 studies. Behavioral and Brain Sciences , 1 (3), 377–386.
Ruocco, A. C. ( 2005 ). The neuropsychology of borderline personality disorder: A meta-analysis and review. Psychiatry Research , 137 (3), 191–202. doi: 10.1016/j.psychres.2005.07.004
Schmidt, F. L. , Oh, I. S. , & Hayes, T. L. ( 2009 ). Fixed- versus random-effects models in meta-analysis: Model properties and an empirical comparison of differences in results. British Journal of Mathematical & Statistical Psychology , 62 , 97–128. doi: 10.1348/000711007x255327
Schulze, R. ( 2004 ). Meta-analysis: a comparison of approaches . Cambridge, MA: Hogrefe & Huber.
Schwarzer, G. (2005). Meta . Retrieved October 1, 2006, from http://www.stats.bris.ac.uk/R/
Shadish, W. R. ( 1992 ). Do family and marital psychotherapies change what people do? A meta-analysis of behavioural outcomes. In T. D. Cook , H. Cooper , D. S. Cordray , H. Hartmann , L. V. Hedges , R. J. Light , T. A. Louis , & F. Mosteller (Eds.), Meta-analysis for explanation: A casebook (pp. 129–208). New York: Sage.
Singh, S. P. , Singh, V. , Kar, N. , & Chan, K. ( 2010 ). Efficacy of antidepressants in treating the negative symptoms of chronic schizophrenia: meta-analysis. British Journal of Psychiatry , 197 (3), 174–179. doi: 10.1192/bjp.bp.109.067710
Smith, M. L. , & Glass, G. V. ( 1977 ). Meta-analysis of psychotherapy outcome studies. American Psychologist , 32 (9), 752–760.
Spreckley, M. , & Boyd, R. ( 2009 ). Efficacy of applied behavioral intervention in preschool children with autism for improving cognitive, language, and adaptive behavior: a systematic review and meta-analysis. Journal of Pediatrics , 154 (3), 338–344. doi: 10.1016/j.jpeds.2008.09.012
Sterling, T. D. ( 1959 ). Publication decisions and their possible effects on inferences drawn from tests of significance—or vice versa. Journal of the American Statistical Association , 54 (285), 30–34.
Stewart, R. E. , & Chambless, D. L. ( 2009 ). Cognitive-behavioral therapy for adult anxiety disorders in clinical practice: A meta-analysis of effectiveness studies. Journal of Consulting and Clinical Psychology , 77 (4), 595–606. doi: 10.1037/a0016032
Stouffer, S. A. ( 1949 ). The American soldier: Vol. 1. Adjustment during Army life . Princeton, NJ: Princeton University Press.
The Cochrane Collaboration. ( 2008 ). Review Manager (RevMan) for Windows: Version 5.0 . Copenhagen: The Nordic Cochrane Centre. Retrieved from http://www.cc-ims.net/revman/
Verzani, J. ( 2004 ). Using R for introductory statistics . Boca Raton, FL: Chapman & Hall.
Vevea, J. L. , & Woods, C. M. ( 2005 ). Publication bias in research synthesis: Sensitivity analysis using a priori weight functions. Psychological Methods , 10 (4), 428–443.
Viechtbauer, W. ( 2010 ). Conducting meta-analyses in R with the metafor package. Journal of Statistical Software , 36 (3), 1–48.
Villeneuve, K. , Potvin, S. , Lesage, A. , & Nicole, L. ( 2010 ). Meta-analysis of rates of drop-out from psychosocial treatment among persons with schizophrenia spectrum disorder. Schizophrenia Research , 121 (1-3), 266–270. doi: 10.1016/j.schres.2010.04.003
Wilson, D. B. (2001). Practical meta-analysis effect size calculator . Retrieved August 3, 2010, from http://gunston.gmu.edu/cebcp/EffectSizeCalculator/index.html
Wilson, D. B. (2004). A spreadsheet for calculating standardized mean difference type effect sizes . Retrieved October 1, 2006, from http://mason.gmu.edu/~dwilsonb/ma.html
Zuur, A. F. , Ieno, E. N. , & Meesters, E. H. W. G. ( 2009 ). A beginner's guide to R . New York: Springer.
- About Oxford Academic
- Publish journals with us
- University press partners
- What we publish
- New features
- Open access
- Institutional account management
- Rights and permissions
- Get help with access
- Accessibility
- Advertising
- Media enquiries
- Oxford University Press
- Oxford Languages
- University of Oxford
Oxford University Press is a department of the University of Oxford. It furthers the University's objective of excellence in research, scholarship, and education by publishing worldwide
- Copyright © 2024 Oxford University Press
- Cookie settings
- Cookie policy
- Privacy policy
- Legal notice
This Feature Is Available To Subscribers Only
Sign In or Create an Account
This PDF is available to Subscribers Only
For full access to this pdf, sign in to an existing account, or purchase an annual subscription.
Thank you for visiting nature.com. You are using a browser version with limited support for CSS. To obtain the best experience, we recommend you use a more up to date browser (or turn off compatibility mode in Internet Explorer). In the meantime, to ensure continued support, we are displaying the site without styles and JavaScript.
- View all journals
- My Account Login
- Explore content
- About the journal
- Publish with us
- Sign up for alerts
- Open access
- Published: 13 July 2021
Systematic review and meta-analysis of depression, anxiety, and suicidal ideation among Ph.D. students
- Emily N. Satinsky 1 ,
- Tomoki Kimura 2 ,
- Mathew V. Kiang 3 , 4 ,
- Rediet Abebe 5 , 6 ,
- Scott Cunningham 7 ,
- Hedwig Lee 8 ,
- Xiaofei Lin 9 ,
- Cindy H. Liu 10 , 11 ,
- Igor Rudan 12 ,
- Srijan Sen 13 ,
- Mark Tomlinson 14 , 15 ,
- Miranda Yaver 16 &
- Alexander C. Tsai 1 , 11 , 17
Scientific Reports volume 11 , Article number: 14370 ( 2021 ) Cite this article
86k Accesses
66 Citations
819 Altmetric
Metrics details
- Epidemiology
- Health policy
- Quality of life
University administrators and mental health clinicians have raised concerns about depression and anxiety among Ph.D. students, yet no study has systematically synthesized the available evidence in this area. After searching the literature for studies reporting on depression, anxiety, and/or suicidal ideation among Ph.D. students, we included 32 articles. Among 16 studies reporting the prevalence of clinically significant symptoms of depression across 23,469 Ph.D. students, the pooled estimate of the proportion of students with depression was 0.24 (95% confidence interval [CI], 0.18–0.31; I 2 = 98.75%). In a meta-analysis of the nine studies reporting the prevalence of clinically significant symptoms of anxiety across 15,626 students, the estimated proportion of students with anxiety was 0.17 (95% CI, 0.12–0.23; I 2 = 98.05%). We conclude that depression and anxiety are highly prevalent among Ph.D. students. Data limitations precluded our ability to obtain a pooled estimate of suicidal ideation prevalence. Programs that systematically monitor and promote the mental health of Ph.D. students are urgently needed.
Similar content being viewed by others
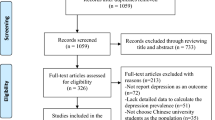
Prevalence of depression among Chinese university students: a systematic review and meta-analysis
Li Gao, Yuanchen Xie, … Wei Wang
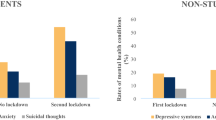
A repeated cross-sectional analysis assessing mental health conditions of adults as per student status during key periods of the COVID-19 epidemic in France
Melissa Macalli, Nathalie Texier, … Christophe Tzourio
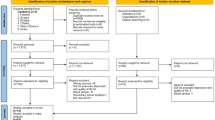
Relationship between depression and quality of life among students: a systematic review and meta-analysis
Michele da Silva Valadão Fernandes, Carolina Rodrigues Mendonça, … Matias Noll
Introduction
Mental health problems among graduate students in doctoral degree programs have received increasing attention 1 , 2 , 3 , 4 . Ph.D. students (and students completing equivalent degrees, such as the Sc.D.) face training periods of unpredictable duration, financial insecurity and food insecurity, competitive markets for tenure-track positions, and unsparing publishing and funding models 5 , 6 , 7 , 8 , 9 , 10 , 11 , 12 —all of which may have greater adverse impacts on students from marginalized and underrepresented populations 13 , 14 , 15 . Ph.D. students’ mental health problems may negatively affect their physical health 16 , interpersonal relationships 17 , academic output, and work performance 18 , 19 , and may also contribute to program attrition 20 , 21 , 22 . As many as 30 to 50% of Ph.D. students drop out of their programs, depending on the country and discipline 23 , 24 , 25 , 26 , 27 . Further, while mental health problems among Ph.D. students raise concerns for the wellbeing of the individuals themselves and their personal networks, they also have broader repercussions for their institutions and academia as a whole 22 .
Despite the potential public health significance of this problem, most evidence syntheses on student mental health have focused on undergraduate students 28 , 29 or graduate students in professional degree programs (e.g., medical students) 30 . In non-systematic summaries, estimates of the prevalence of clinically significant depressive symptoms among Ph.D. students vary considerably 31 , 32 , 33 . Reliable estimates of depression and other mental health problems among Ph.D. students are needed to inform preventive, screening, or treatment efforts. To address this gap in the literature, we conducted a systematic review and meta-analysis to explore patterns of depression, anxiety, and suicidal ideation among Ph.D. students.
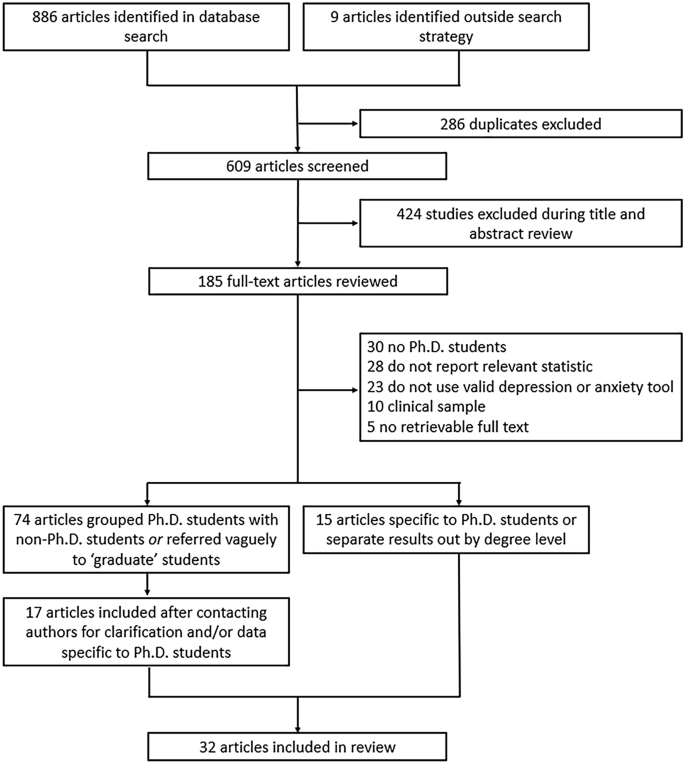
Flowchart of included articles.
The evidence search yielded 886 articles, of which 286 were excluded as duplicates (Fig. 1 ). An additional nine articles were identified through reference lists or grey literature reports published on university websites. Following a title/abstract review and subsequent full-text review, 520 additional articles were excluded.
Of the 89 remaining articles, 74 were unclear about their definition of graduate students or grouped Ph.D. and non-Ph.D. students without disaggregating the estimates by degree level. We obtained contact information for the authors of most of these articles (69 [93%]), requesting additional data. Three authors clarified that their study samples only included Ph.D. students 34 , 35 , 36 . Fourteen authors confirmed that their study samples included both Ph.D. and non-Ph.D. students but provided us with data on the subsample of Ph.D. students 37 , 38 , 39 , 40 , 41 , 42 , 43 , 44 , 45 , 46 , 47 , 48 , 49 , 50 . Where authors clarified that the sample was limited to graduate students in non-doctoral degree programs, did not provide additional data on the subsample of Ph.D. students, or did not reply to our information requests, we excluded the studies due to insufficient information (Supplementary Table S1 ).
Ultimately, 32 articles describing the findings of 29 unique studies were identified and included in the review 16 , 32 , 33 , 34 , 35 , 36 , 37 , 38 , 39 , 40 , 41 , 42 , 43 , 44 , 45 , 46 , 47 , 48 , 50 , 51 , 52 , 53 , 54 , 55 , 56 , 57 , 58 , 59 , 60 , 61 , 62 (Table 1 ). Overall, 26 studies measured depression, 19 studies measured anxiety, and six studies measured suicidal ideation. Three pairs of articles reported data on the same sample of Ph.D. students 33 , 38 , 45 , 51 , 53 , 56 and were therefore grouped in Table 1 and reported as three studies. Publication dates ranged from 1979 to 2019, but most articles (22/32 [69%]) were published after 2015. Most studies were conducted in the United States (20/29 [69%]), with additional studies conducted in Australia, Belgium, China, Iran, Mexico, and South Korea. Two studies were conducted in cross-national settings representing 48 additional countries. None were conducted in sub-Saharan Africa or South America. Most studies included students completing their degrees in a mix of disciplines (17/29 [59%]), while 12 studies were limited to students in a specific field (e.g., biomedicine, education). The median sample size was 172 students (interquartile range [IQR], 68–654; range, 6–6405). Seven studies focused on mental health outcomes in demographic subgroups, including ethnic or racialized minority students 37 , 41 , 43 , international students 47 , 50 , and sexual and gender minority students 42 , 54 .
In all, 16 studies reported the prevalence of depression among a total of 23,469 Ph.D. students (Fig. 2 ; range, 10–47%). Of these, the most widely used depression scales were the PHQ-9 (9 studies) and variants of the Center for Epidemiologic Studies-Depression scale (CES-D, 4 studies) 63 , and all studies assessed clinically significant symptoms of depression over the past one to two weeks. Three of these studies reported findings based on data from different survey years of the same parent study (the Healthy Minds Study) 40 , 42 , 43 , but due to overlap in the survey years reported across articles, these data were pooled. Most of these studies were based on data collected through online surveys (13/16 [81%]). Ten studies (63%) used random or systematic sampling, four studies (25%) used convenience sampling, and two studies (13%) used multiple sampling techniques.
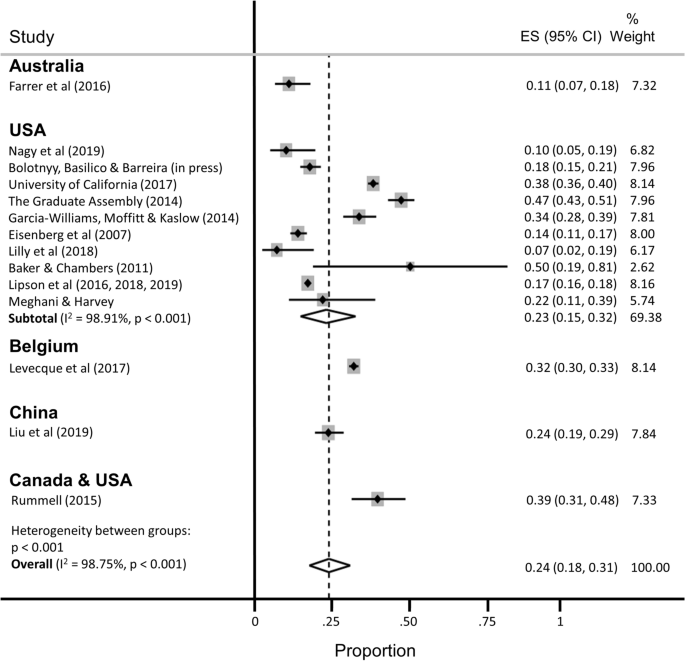
Pooled estimate of the proportion of Ph.D. students with clinically significant symptoms of depression.
The estimated proportion of Ph.D. students assessed as having clinically significant symptoms of depression was 0.24 (95% confidence interval [CI], 0.18–0.31; 95% predictive interval [PI], 0.04–0.54), with significant evidence of between-study heterogeneity (I 2 = 98.75%). A subgroup analysis restricted to the twelve studies conducted in the United States yielded similar findings (pooled estimate [ES] = 0.23; 95% CI, 0.15–0.32; 95% PI, 0.01–0.60), with no appreciable difference in heterogeneity (I 2 = 98.91%). A subgroup analysis restricted to the studies that used the PHQ-9 to assess depression yielded a slightly lower prevalence estimate and a slight reduction in heterogeneity (ES = 0.18; 95% CI, 0.14–0.22; 95% PI, 0.07–0.34; I 2 = 90.59%).
Nine studies reported the prevalence of clinically significant symptoms of anxiety among a total of 15,626 Ph.D. students (Fig. 3 ; range 4–49%). Of these, the most widely used anxiety scale was the 7-item Generalized Anxiety Disorder scale (GAD-7, 5 studies) 64 . Data from three of the Healthy Minds Study articles were pooled into two estimates, because the scale used to measure anxiety changed midway through the parent study (i.e., the Patient Health Questionnaire-Generalized Anxiety Disorder [PHQ-GAD] scale was used from 2007 to 2012 and then switched to the GAD-7 in 2013 40 ). Most studies (8/9 [89%]) assessed clinically significant symptoms of anxiety over the past two to four weeks, with the one remaining study measuring anxiety over the past year. Again, most of these studies were based on data collected through online surveys (7/9 [78%]). Five studies (56%) used random or systematic sampling, two studies (22%) used convenience sampling, and two studies (22%) used multiple sampling techniques.
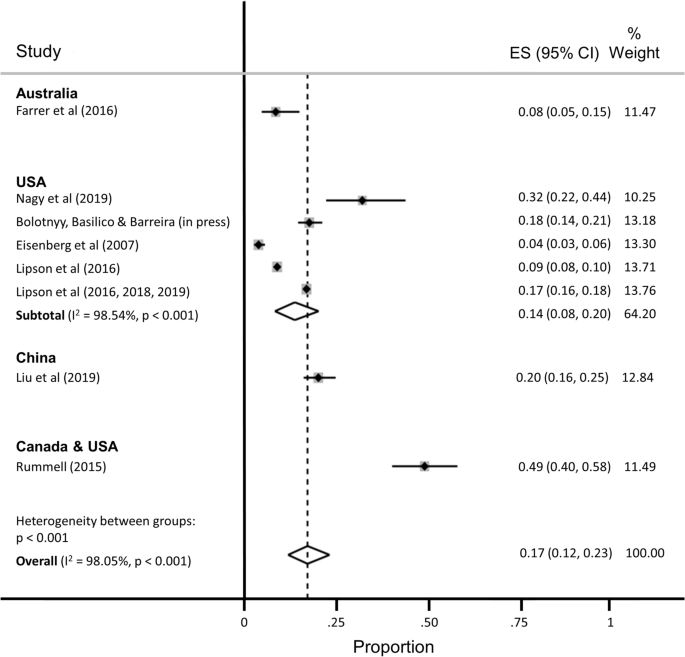
Pooled estimate of the proportion of Ph.D. students with clinically significant symptoms of anxiety.
The estimated proportion of Ph.D. students assessed as having anxiety was 0.17 (95% CI, 0.12–0.23; 95% PI, 0.02–0.41), with significant evidence of between-study heterogeneity (I 2 = 98.05%). The subgroup analysis restricted to the five studies conducted in the United States yielded a slightly lower proportion of students assessed as having anxiety (ES = 0.14; 95% CI, 0.08–0.20; 95% PI, 0.00–0.43), with no appreciable difference in heterogeneity (I 2 = 98.54%).
Six studies reported the prevalence of suicidal ideation (range, 2–12%), but the recall windows varied greatly (e.g., ideation within the past 2 weeks vs. past year), precluding pooled estimation.
Additional stratified pooled estimates could not be obtained. One study of Ph.D. students across 54 countries found that phase of study was a significant moderator of mental health, with students in the comprehensive examination and dissertation phases more likely to experience distress compared with students primarily engaged in coursework 59 . Other studies identified a higher prevalence of mental ill-health among women 54 ; lesbian, gay, bisexual, transgender, and queer (LGBTQ) students 42 , 54 , 60 ; and students with multiple intersecting identities 54 .
Several studies identified correlates of mental health problems including: project- and supervisor-related issues, stress about productivity, and self-doubt 53 , 62 ; uncertain career prospects, poor living conditions, financial stressors, lack of sleep, feeling devalued, social isolation, and advisor relationships 61 ; financial challenges 38 ; difficulties with work-life balance 58 ; and feelings of isolation and loneliness 52 . Despite these challenges, help-seeking appeared to be limited, with only about one-quarter of Ph.D. students reporting mental health problems also reporting that they were receiving treatment 40 , 52 .
Risk of bias
Twenty-one of 32 articles were assessed as having low risk of bias (Supplementary Table S2 ). Five articles received one point for all five categories on the risk of bias assessment (lowest risk of bias), and one article received no points (highest risk). The mean risk of bias score was 3.22 (standard deviation, 1.34; median, 4; IQR, 2–4). Restricting the estimation sample to 12 studies assessed as having low risk of bias, the estimated proportion of Ph.D. students with depression was 0.25 (95% CI, 0.18–0.33; 95% PI, 0.04–0.57; I 2 = 99.11%), nearly identical to the primary estimate, with no reduction in heterogeneity. The estimated proportion of Ph.D. students with anxiety, among the 7 studies assessed as having low risk of bias, was 0.12 (95% CI, 0.07–0.17; 95% PI, 0.01–0.34; I 2 = 98.17%), again with no appreciable reduction in heterogeneity.
In our meta-analysis of 16 studies representing 23,469 Ph.D. students, we estimated that the pooled prevalence of clinically significant symptoms of depression was 24%. This estimate is consistent with estimated prevalence rates in other high-stress biomedical trainee populations, including medical students (27%) 30 , resident physicians (29%) 65 , and postdoctoral research fellows (29%) 66 . In the sample of nine studies representing 15,626 Ph.D. students, we estimated that the pooled prevalence of clinically significant symptoms of anxiety was 17%. While validated screening instruments tend to over-identify cases of depression (relative to structured clinical interviews) by approximately a factor of two 67 , 68 , our findings nonetheless point to a major public health problem among Ph.D. students. Available data suggest that the prevalence of depressive and anxiety disorders in the general population ranges from 5 to 7% worldwide 69 , 70 . In contrast, prevalence estimates of major depressive disorder among young adults have ranged from 13% (for young adults between the ages of 18 and 29 years in the 2012–2013 National Epidemiologic Survey on Alcohol and Related Conditions III 71 ) to 15% (for young adults between the ages of 18 and 25 in the 2019 U.S. National Survey on Drug Use and Health 72 ). Likewise, the prevalence of generalized anxiety disorder was estimated at 4% among young adults between the ages of 18 and 29 in the 2001–03 U.S. National Comorbidity Survey Replication 73 . Thus, even accounting for potential upward bias inherent in these studies’ use of screening instruments, our estimates suggest that the rates of recent clinically significant symptoms of depression and anxiety are greater among Ph.D. students compared with young adults in the general population.
Further underscoring the importance of this public health issue, Ph.D. students face unique stressors and uncertainties that may put them at increased risk for mental health and substance use problems. Students grapple with competing responsibilities, including coursework, teaching, and research, while also managing interpersonal relationships, social isolation, caregiving, and financial insecurity 3 , 10 . Increasing enrollment in doctoral degree programs has not been matched with a commensurate increase in tenure-track academic job opportunities, intensifying competition and pressure to find employment post-graduation 5 . Advisor-student power relations rarely offer options for recourse if and when such relationships become strained, particularly in the setting of sexual harassment, unwanted sexual attention, sexual coercion, and rape 74 , 75 , 76 , 77 , 78 . All of these stressors may be magnified—and compounded by stressors unrelated to graduate school—for subgroups of students who are underrepresented in doctoral degree programs and among whom mental health problems are either more prevalent and/or undertreated compared with the general population, including Black, indigenous, and other people of color 13 , 79 , 80 ; women 81 , 82 ; first-generation students 14 , 15 ; people who identify as LGBTQ 83 , 84 , 85 ; people with disabilities; and people with multiple intersecting identities.
Structural- and individual-level interventions will be needed to reduce the burden of mental ill-health among Ph.D. students worldwide 31 , 86 . Despite the high prevalence of mental health and substance use problems 87 , Ph.D. students demonstrate low rates of help-seeking 40 , 52 , 88 . Common barriers to help-seeking include fears of harming one’s academic career, financial insecurity, lack of time, and lack of awareness 89 , 90 , 91 , as well as health care systems-related barriers, including insufficient numbers of culturally competent counseling staff, limited access to psychological services beyond time-limited psychotherapies, and lack of programs that address the specific needs either of Ph.D. students in general 92 or of Ph.D. students belonging to marginalized groups 93 , 94 . Structural interventions focused solely on enhancing student resilience might include programs aimed at reducing stigma, fostering social cohesion, and reducing social isolation, while changing norms around help-seeking behavior 95 , 96 . However, structural interventions focused on changing stressogenic aspects of the graduate student environment itself are also needed 97 , beyond any enhancements to Ph.D. student resilience, including: undercutting power differentials between graduate students and individual faculty advisors, e.g., by diffusing power among multiple faculty advisors; eliminating racist, sexist, and other discriminatory behaviors by faculty advisors 74 , 75 , 98 ; valuing mentorship and other aspects of “invisible work” that are often disproportionately borne by women faculty and faculty of color 99 , 100 ; and training faculty members to emphasize the dignity of, and adequately prepare Ph.D. students for, non-academic careers 101 , 102 .
Our findings should be interpreted with several limitations in mind. First, the pooled estimates are characterized by a high degree of heterogeneity, similar to meta-analyses of depression prevalence in other populations 30 , 65 , 103 , 104 , 105 . Second, we were only able to aggregate depression prevalence across 16 studies and anxiety prevalence across nine studies (the majority of which were conducted in the U.S.) – far fewer than the 183 studies included in a meta-analysis of depression prevalence among medical students 30 and the 54 studies included in a meta-analysis of resident physicians 65 . These differences underscore the need for more rigorous study in this critical area. Many articles were either excluded from the review or from the meta-analyses for not meeting inclusion criteria or not reporting relevant statistics. Future research in this area should ensure the systematic collection of high-quality, clinically relevant data from a comprehensive set of institutions, across disciplines and countries, and disaggregated by graduate student type. As part of conducting research and addressing student mental health and wellbeing, university deans, provosts, and chancellors should partner with national survey and program institutions (e.g., Graduate Student Experience in the Research University [gradSERU] 106 , the American College Health Association National College Health Assessment [ACHA-NCHA], and HealthyMinds). Furthermore, federal agencies that oversee health and higher education should provide resources for these efforts, and accreditation agencies should require monitoring of mental health and programmatic responses to stressors among Ph.D. students.
Third, heterogeneity in reporting precluded a meta-analysis of the suicidality outcomes among the few studies that reported such data. While reducing the burden of mental health problems among graduate students is an important public health aim in itself, more research into understanding non-suicidal self-injurious behavior, suicide attempts, and completed suicide among Ph.D. students is warranted. Fourth, it is possible that the grey literature reports included in our meta-analysis are more likely to be undertaken at research-intensive institutions 52 , 60 , 61 . However, the direction of bias is unpredictable: mental health problems among Ph.D. students in research-intensive environments may be more prevalent due to detection bias, but such institutions may also have more resources devoted to preventive, screening, or treatment efforts 92 . Fifth, inclusion in this meta-analysis and systematic review was limited to those based on community samples. Inclusion of clinic-based samples, or of studies conducted before or after specific milestones (e.g., the qualifying examination or dissertation prospectus defense), likely would have yielded even higher pooled prevalence estimates of mental health problems. And finally, few studies provided disaggregated data according to sociodemographic factors, stage of training (e.g., first year, pre-prospectus defense, all-but-dissertation), or discipline of study. These factors might be investigated further for differences in mental health outcomes.
Clinically significant symptoms of depression and anxiety are pervasive among graduate students in doctoral degree programs, but these are understudied relative to other trainee populations. Structural and clinical interventions to systematically monitor and promote the mental health and wellbeing of Ph.D. students are urgently needed.
This systematic review and meta-analysis follows the Preferred Reporting Items for Systematic Reviews and Meta-Analyses (PRISMA) approach (Supplementary Table S3 ) 107 . This study was based on data collected from publicly available bibliometric databases and did not require ethical approval from our institutional review boards.
Eligibility criteria
Studies were included if they provided data on either: (a) the number or proportion of Ph.D. students with clinically significant symptoms of depression or anxiety, ascertained using a validated scale; or (b) the mean depression or anxiety symptom severity score and its standard deviation among Ph.D. students. Suicidal ideation was examined as a secondary outcome.
We excluded studies that focused on graduate students in non-doctoral degree programs (e.g., Master of Public Health) or professional degree programs (e.g., Doctor of Medicine, Juris Doctor) because more is known about mental health problems in these populations 30 , 108 , 109 , 110 and because Ph.D. students face unique uncertainties. To minimize the potential for upward bias in our pooled prevalence estimates, we excluded studies that recruited students from campus counseling centers or other clinic-based settings. Studies that measured affective states, or state anxiety, before or after specific events (e.g., terrorist attacks, qualifying examinations) were also excluded.
If articles described the study sample in general terms (i.e., without clarifying the degree level of the participants), we contacted the authors by email for clarification. Similarly, if articles pooled results across graduate students in doctoral and non-doctoral degree programs (e.g., reporting a single estimate for a mixed sample of graduate students), we contacted the authors by email to request disaggregated data on the subsample of Ph.D. students. If authors did not reply after two contact attempts spaced over 2 months, or were unable to provide these data, we excluded these studies from further consideration.
Search strategy and data extraction
PubMed, Embase, PsycINFO, ERIC, and Business Source Complete were searched from inception of each database to November 5, 2019. The search strategy included terms related to mental health symptoms (e.g., depression, anxiety, suicide), the study population (e.g., graduate, doctoral), and measurement category (e.g., depression, Columbia-Suicide Severity Rating Scale) (Supplementary Table S4 ). In addition, we searched the reference lists and the grey literature.
After duplicates were removed, we screened the remaining titles and abstracts, followed by a full-text review. We excluded articles following the eligibility criteria listed above (i.e., those that were not focused on Ph.D. students; those that did not assess depression and/or anxiety using a validated screening tool; those that did not report relevant statistics of depression and/or anxiety; and those that recruited students from clinic-based settings). Reasons for exclusion were tracked at each stage. Following selection of included articles, two members of the research team extracted data and conducted risk of bias assessments. Discrepancies were discussed with a third member of the research team. Key extraction variables included: study design, geographic region, sample size, response rate, demographic characteristics of the sample, screening instrument(s) used for assessment, mean depression or anxiety symptom severity score (and its standard deviation), and the number (or proportion) of students experiencing clinically significant symptoms of depression or anxiety.
Risk of bias assessment
Following prior work 30 , 65 , the Newcastle–Ottawa Scale 111 was adapted and used to assess risk of bias in the included studies. Each study was assessed across 5 categories: sample representativeness, sample size, non-respondents, ascertainment of outcomes, and quality of descriptive statistics reporting (Supplementary Information S5 ). Studies were judged as having either low risk of bias (≥ 3 points) or high risk of bias (< 3 points).
Analysis and synthesis
Before pooling the estimated prevalence rates across studies, we first transformed the proportions using a variance-stabilizing double arcsine transformation 112 . We then computed pooled estimates of prevalence using a random effects model 113 . Study specific confidence intervals were estimated using the score method 114 , 115 . We estimated between-study heterogeneity using the I 2 statistic 116 . In an attempt to reduce the extent of heterogeneity, we re-estimated pooled prevalence restricting the analysis to studies conducted in the United States and to studies in which depression assessment was based on the 9-item Patient Health Questionnaire (PHQ-9) 117 . All analyses were conducted using Stata (version 16; StataCorp LP, College Station, Tex.). Where heterogeneity limited our ability to summarize the findings using meta-analysis, we synthesized the data using narrative review.
Woolston, C. Why mental health matters. Nature 557 , 129–131 (2018).
Article ADS CAS Google Scholar
Woolston, C. A love-hurt relationship. Nature 550 , 549–552 (2017).
Article Google Scholar
Woolston, C. PhD poll reveals fear and joy, contentment and anguish. Nature 575 , 403–406 (2019).
Article ADS CAS PubMed Google Scholar
Byrom, N. COVID-19 and the research community: The challenges of lockdown for early-career researchers. Elife 9 , e59634 (2020).
Article PubMed PubMed Central Google Scholar
Alberts, B., Kirschner, M. W., Tilghman, S. & Varmus, H. Rescuing US biomedical research from its systemic flaws. Proc. Natl. Acad. Sci. USA 111 , 5773–5777 (2014).
Article ADS CAS PubMed PubMed Central Google Scholar
McDowell, G. S. et al. Shaping the future of research: A perspective from junior scientists. F1000Res 3 , 291 (2014).
Article PubMed Google Scholar
Petersen, A. M., Riccaboni, M., Stanley, H. E. & Pammoli, F. Persistence and uncertainty in the academic career. Proc. Natl. Acad. Sci. USA 109 , 5213–5218 (2012).
Leshner, A. I. Rethinking graduate education. Science 349 , 349 (2015).
National Academies of Sciences Engineering and Medicine. Graduate STEM Education for the 21st Century (National Academies Press, 2018).
Google Scholar
Charles, S. T., Karnaze, M. M. & Leslie, F. M. Positive factors related to graduate student mental health. J. Am. Coll. Health https://doi.org/10.1080/07448481.2020.1841207 (2021).
Riddle, E. S., Niles, M. T. & Nickerson, A. Prevalence and factors associated with food insecurity across an entire campus population. PLoS ONE 15 , e0237637 (2020).
Article CAS PubMed PubMed Central Google Scholar
Soldavini, J., Berner, M. & Da Silva, J. Rates of and characteristics associated with food insecurity differ among undergraduate and graduate students at a large public university in the Southeast United States. Prev. Med. Rep. 14 , 100836 (2019).
Clark, U. S. & Hurd, Y. L. Addressing racism and disparities in the biomedical sciences. Nat. Hum. Behav. 4 , 774–777 (2020).
Gardner, S. K. The challenges of first-generation doctoral students. New Dir. High. Educ. 2013 , 43–54 (2013).
Seay, S. E., Lifton, D. E., Wuensch, K. L., Bradshaw, L. K. & McDowelle, J. O. First-generation graduate students and attrition risks. J. Contin. High. Educ. 56 , 11–25 (2008).
Rummell, C. M. An exploratory study of psychology graduate student workload, health, and program satisfaction. Prof. Psychol. Res. Pract. 46 , 391–399 (2015).
Salzer, M. S. A comparative study of campus experiences of college students with mental illnesses versus a general college sample. J. Am. Coll. Health 60 , 1–7 (2012).
Hysenbegasi, A., Hass, S. & Rowland, C. The impact of depression on the academic productivity of university students. J. Ment. Health Policy Econ. 8 , 145–151 (2005).
PubMed Google Scholar
Harvey, S. et al. Depression and work performance: An ecological study using web-based screening. Occup. Med. (Lond.) 61 , 209–211 (2011).
Article CAS Google Scholar
Eisenberg, D., Golberstein, E. & Hunt, J. B. Mental health and academic success in college. BE J. Econ. Anal. Policy 9 , 40 (2009).
Lovitts, B. E. Who is responsible for graduate student attrition--the individual or the institution? Toward an explanation of the high and persistent rate of attrition. In: Annual Meeting of the American Education Research Association (New York, 1996). Available at: https://eric.ed.gov/?id=ED399878.
Gardner, S. K. Student and faculty attributions of attrition in high and low-completing doctoral programs in the United States. High. Educ. 58 , 97–112 (2009).
Lovitts, B. E. Leaving the Ivory Tower: The Causes and Consequences of Departure from Doctoral Study (Rowman & Littlefield Publishers, 2001).
Rigler Jr, K. L., Bowlin, L. K., Sweat, K., Watts, S. & Throne, R. Agency, socialization, and support: a critical review of doctoral student attrition. In: Proceedings of the Third International Conference on Doctoral Education: Organizational Leadership and Impact , University of Central Florida, Orlando, (2017).
Golde, C. M. The role of the department and discipline in doctoral student attrition: Lessons from four departments. J. High. Educ. 76 , 669–700 (2005).
Council of Graduate Schools. PhD Completion and Attrition: Analysis of Baseline Program Data from the PhD Completion Project (Council of Graduate Schools, 2008).
National Research Council. A Data-Based Assessment of Research-Doctorate Programs in the United States (The National Academies Press, 2011).
Akhtar, P. et al. Prevalence of depression among university students in low and middle income countries (LMICs): A systematic review and meta-analysis. J. Affect. Disord. 274 , 911–919 (2020).
Mortier, P. et al. The prevalence of suicidal thoughts and behaviours among college students: A meta-analysis. Psychol. Med. 48 , 554–565 (2018).
Article CAS PubMed Google Scholar
Rotenstein, L. S. et al. Prevalence of depression, depressive symptoms, and suicidal ideation among medical students: A systematic review and meta-analysis. JAMA 316 , 2214–2236 (2016).
Tsai, J. W. & Muindi, F. Towards sustaining a culture of mental health and wellness for trainees in the biosciences. Nat. Biotechnol. 34 , 353–355 (2016).
Levecque, K., Anseel, F., De Beuckelaer, A., Van der Heyden, J. & Gisle, L. Work organization and mental health problems in PhD students. Res. Policy 46 , 868–879 (2017).
Nagy, G. A. et al. Burnout and mental health problems in biomedical doctoral students. CBE Life Sci. Educ. 18 , 1–14 (2019).
Garcia-Williams, A., Moffitt, L. & Kaslow, N. J. Mental health and suicidal behavior among graduate students. Acad. Psychiatry 28 , 554–560 (2014).
Sheldon, K. M. Emotionality differences between artists and scientists. J. Res. Pers. 28 , 481–491 (1994).
Lightstone, S. N., Swencionis, C. & Cohen, H. W. The effect of bioterrorism messages on anxiety levels. Int. Q. Community Health Educ. 24 , 111–122 (2006).
Clark, C. R., Mercer, S. H., Zeigler-Hill, V. & Dufrene, B. A. Barriers to the success of ethnic minority students in school psychology graduate programs. School Psych. Rev. 41 , 176–192 (2012).
Eisenberg, D., Gollust, S. E., Golberstein, E. & Hefner, J. L. Prevalence and correlates of depression, anxiety, and suicidality among university students. Am. J. Orthopsychiatry 77 , 534–542 (2007).
Farrer, L. M., Gulliver, A., Bennett, K., Fassnacht, D. B. & Griffiths, K. M. Demographic and psychosocial predictors of major depression and generalised anxiety disorder in Australian university students. BMC Psychiatry 16 , 241 (2016).
Lipson, S. K., Zhou, S., Wagner, B. III., Beck, K. & Eisenberg, D. Major differences: Variations in undergraduate and graduate student mental health and treatment utilization across academic disciplines. J. Coll. Stud. Psychother. 30 , 23–41 (2016).
Lilly, F. R. W. et al. The influence of racial microaggressions and social rank on risk for depression among minority graduate and professional students. Coll. Stud. J. 52 , 86–104 (2018).
Lipson, S. K., Raifman, J., Abelson, S. & Reisner, S. L. Gender minority mental health in the U.S.: Results of a national survey on college campuses. Am. J. Prev. Med. 57 , 293–301 (2019).
Lipson, S. K., Kern, A., Eisenberg, D. & Breland-Noble, A. M. Mental health disparities among college students of color. J. Adolesc. Health 63 , 348–356 (2018).
Baker, A. J. L. & Chambers, J. Adult recall of childhood exposure to parental conflict: Unpacking the black box of parental alienation. J. Divorce Remarriage 52 , 55–76 (2011).
Golberstein, E., Eisenberg, D. & Gollust, S. E. Perceived stigma and mental health care seeking. Psychiatr. Serv. 59 , 392–399 (2008).
Hindman, R. K., Glass, C. R., Arnkoff, D. B. & Maron, D. D. A comparison of formal and informal mindfulness programs for stress reduction in university students. Mindfulness 6 , 873–884 (2015).
Hirai, R., Frazier, P. & Syed, M. Psychological and sociocultural adjustment of first-year international students: Trajectories and predictors. J. Couns. Psychol. 62 , 438–452 (2015).
Lee, J. S. & Jeong, B. Having mentors and campus social networks moderates the impact of worries and video gaming on depressive symptoms: A moderated mediation analysis. BMC Public Health 14 , 1–12 (2014).
Corral-Frias, N. S., Velardez Soto, S. N., Frias-Armenta, M., Corona-Espinosa, A. & Watson, D. Concurrent validity and reliability of two short forms of the mood and anxiety symptom questionnaire in a student sample from Northwest Mexico. J. Psychopathol. Behav. Assess. 41 , 304–316 (2019).
Meghani, D. T. & Harvey, E. A. Asian Indian international students’ trajectories of depression, acculturation, and enculturation. Asian Am. J. Psychol. 7 , 1–14 (2016).
Barry, K. M., Woods, M., Martin, A., Stirling, C. & Warnecke, E. A randomized controlled trial of the effects of mindfulness practice on doctoral candidate psychological status. J. Am. Coll. Health 67 , 299–307 (2019).
Bolotnyy, V., Basilico, M. & Barreira, P. Graduate student mental health: lessons from American economics departments. J. Econ. Lit. (in press).
Barry, K. M., Woods, M., Warnecke, E., Stirling, C. & Martin, A. Psychological health of doctoral candidates, study-related challenges and perceived performance. High. Educ. Res. Dev. 37 , 468–483 (2018).
Boyle, K. M. & McKinzie, A. E. The prevalence and psychological cost of interpersonal violence in graduate and law school. J. Interpers. Violence 36 , 6319-6350 (2021).
Heinrich, D. L. The causal influence of anxiety on academic achievement for students of differing intellectual ability. Appl. Psychol. Meas. 3 , 351–359 (1979).
Hish, A. J. et al. Applying the stress process model to stress-burnout and stress-depression relationships in biomedical doctoral students: A cross-sectional pilot study. CBE Life Sci. Educ. 18 , 1–11 (2019).
Jamshidi, F. et al. A cross-sectional study of psychiatric disorders in medical sciences students. Mater. Sociomed. 29 , 188–191 (2017).
Liu, C. et al. Prevalence and associated factors of depression and anxiety among doctoral students: The mediating effect of mentoring relationships on the association between research self-efficacy and depression/anxiety. Psychol. Res. Behav. Manag. 12 , 195–208 (2019).
Sverdlik, A. & Hall, N. C. Not just a phase: Exploring the role of program stage on well-being and motivation in doctoral students. J. Adult Contin. Educ. 26 , 1–28 (2019).
University of California Office of the President. The University of California Graduate student Well-Being Survey Report (University of California, 2017).
The Graduate Assembly. Graduate Student Happiness & Well-Being Report (University of California at Berkeley, 2014).
Richardson, C. M., Trusty, W. T. & George, K. A. Trainee wellness: Self-critical perfectionism, self-compassion, depression, and burnout among doctoral trainees in psychology. Couns. Psychol. Q. 33 , 187-198 (2020).
Radloff, L. S. The CES-D Scale: A self-report depression scale for research in the general population. Appl. Psychol. Meas. 1 , 385–401 (1977).
Spitzer, R. L., Kroenke, K., Williams, J. B. W. & Lowe, B. A brief measure for assessing generalized anxiety disorder: The GAD-7. Arch. Intern. Med. 166 , 1092–1097 (2006).
Mata, D. A. et al. Prevalence of depression and depressive symptoms among residents physicians: A systematic review and meta-analysis. JAMA 314 , 2373–2383 (2015).
Gloria, C. T. & Steinhardt, M. A. Flourishing, languishing, and depressed postdoctoral fellows: Differences in stress, anxiety, and depressive symptoms. J. Postdoct. Aff. 3 , 1–9 (2013).
Levis, B. et al. Patient Health Questionnaire-9 scores do not accurately estimate depression prevalence: Individual participant data meta-analysis. J. Clin. Epidemiol. 122 , 115-128.e111 (2020).
Tsai, A. C. Reliability and validity of depression assessment among persons with HIV in sub-Saharan Africa: Systematic review and meta-analysis. J. Acquir. Immune Defic. Syndr. 66 , 503–511 (2014).
Baxter, A. J., Scott, K. M., Vos, T. & Whiteford, H. A. Global prevalence of anxiety disorders: A systematic review and meta-regression. Psychol. Med. 43 , 897–910 (2013).
Ferrari, A. et al. Global variation in the prevalence and incidence of major depressive disorder: A systematic review of the epidemiological literature. Psychol. Med. 43 , 471–481 (2013).
Hasin, D. S. et al. Epidemiology of adult DSM-5 major depressive disorder and its specifiers in the United States. JAMA Psychiatry 75 , 336–346 (2018).
US Substance Abuse and Mental Health Services Administration. Key Substance Use and Mental Health Indicators in the United States: Results from the 2019 National Survey on Drug Use and Health (Center for Behavioral Health Statistics and Quality, Substance Abuse and Mental Health Services Administration, 2020).
Kessler, R. C. et al. Lifetime prevalence and age-of-onset distributions of DSM-IV disorders in the National Comorbidity Survey Replication. Arch. Gen. Psychiatry 62 , 593–602 (2005).
Working Group report to the Advisory Committee to the NIH Director. Changing the Culture to End Sexual Harassment (U. S. National Institutes of Health, 2019).
National Academies of Sciences Engineering and Medicine. Sexual Harassment of Women: Climate, Culture, and Consequences in Academic Sciences, Engineering, and Medicine (The National Academies Press, 2018).
Wadman, M. A hidden history. Science 360 , 480–485 (2018).
Hockfield, S., Magley, V. & Yoshino, K. Report of the External Review Committee to Review Sexual Harassment at Harvard University (External Review Committee to Review Sexual Harassment at Harvard University, 2021).
Bartlett, T. & Gluckman, N. She left Harvard. He got to stay. Chronicle High. Educ. 64 , A14 (2021). Available at: https://www.chronicle.com/article/she-left-harvard-he-got-to-stay/.
Tseng, M. et al. Strategies and support for Black, Indigenous, and people of colour in ecology and evolutionary biology. Nat. Ecol. Evol. 4 , 1288–1290 (2020).
Williams, D. R. et al. Prevalence and distribution of major depressive disorder in African Americans, Caribbean blacks, and non-Hispanic whites: Results from the National Survey of American Life. Arch. Gen. Psychiatry 64 , 305–315 (2007).
Wu, A. H. Gender bias in rumors among professionals: An identity-based interpretation. Rev. Econ. Stat. 102 , 867–880 (2020).
Kessler, R. C. Epidemiology of women and depression. J. Affect. Disord. 74 , 5–13 (2003).
Mattheis, A., Cruz-Ramirez De Arellano, D. & Yoder, J. B. A model of queer STEM identity in the workplace. J. Homosex 67 , 1839–1863 (2020).
Semlyen, J., King, M., Varney, J. & Hagger-Johnson, G. Sexual orientation and symptoms of common mental disorder or low wellbeing: Combined meta-analysis of 12 UK population health surveys. BMC Psychiatry 16 , 1–19 (2016).
Lark, J. S. & Croteau, J. M. Lesbian, gay, and bisexual doctoral students’ mentoring relationships with faculty in counseling psychology: A qualitative study. Couns. Psychol. 26 , 754–776 (1998).
Jaremka, L. M. et al. Common academic experiences no one talks about: Repeated rejection, imposter syndrome, and burnout. Perspect Psychol Sci 15 , 519–543 (2020).
Allen, H. K. et al. Substance use and mental health problems among graduate students: Individual and program-level correlates. J. Am. Coll. Health https://doi.org/10.1080/07448481.2020.1725020 (2020).
Turner, A. & Berry, T. Counseling center contributions to student retention and graduation: A longitudinal assessment. J. Coll. Stud. Dev. 41 , 627–636 (2000).
Dyrbye, L. N., Thomas, M. R. & Shanafelt, T. D. Medical student distress: Causes, consequences, and proposed solutions. Mayo Clin. Proc. 80 , 1613–1622 (2005).
Tija, J., Givens, J. L. & Shea, J. A. Factors associated with undertreatment of medical student depression. J. Am. Coll. Health 53 , 219–224 (2005).
Dearing, R., Maddux, J. & Tangney, J. Predictors of psychological help seeking in clinical and counseling psychology graduate students. Prof. Psychol. Res. Pract. 36 , 323–329 (2005).
Langin, K. Amid concerns about grad student mental health, one university takes a novel approach. Science https://doi.org/10.1126/science.caredit.aay7113 (2019).
Guillory, D. Combating anti-blackness in the AI community. arXiv , arXiv:2006.16879 (2020).
Galán, C. A. et al. A call to action for an antiracist clinical science. J. Clin. Child Adolesc. Psychol 50 , 12-57 (2021).
Wyman, P. A. et al. Effect of the Wingman-Connect upstream suicide prevention program for air force personnel in training: A cluster randomized clinical trial. JAMA Netw Open 3 , e2022532 (2020).
Knox, K. L. et al. The US Air Force Suicide Prevention Program: Implications for public health policy. Am. J. Public Health 100 , 2457–2463 (2010).
Inclusive Climate Subcommittee of the Government Department Climate Change Committee. Government Department Climate Change: Final Report and Recommendations (Government Department, Harvard University, 2019).
Inclusive Climate Subcommittee of the Government Department Climate Change Committee. Government Department Climate Survey Report (Government Department, Harvard University, 2019).
Magoqwana, B., Maqabuka, Q. & Tshoaedi, M. “Forced to care” at the neoliberal university: Invisible labour as academic labour performed by Black women academics in the South African university. S. Afr. Rev. Sociol. 50 , 6–21 (2019).
Jones, H. A., Perrin, P. B., Heller, M. B., Hailu, S. & Barnett, C. Black psychology graduate students’ lives matter: Using informal mentoring to create an inclusive climate amidst national race-related events. Prof. Psychol. Res. Pract. 49 , 75–82 (2018).
Mathur, A., Meyers, F. J., Chalkley, R., O’Brien, T. C. & Fuhrmann, C. N. Transforming training to reflect the workforce. Sci. Transl. Med. 7 , 285 (2015).
Scharff, V. Advice: Prepare your Ph.D.s for diverse career paths. Chronicle High. Educ. 65 , 30 (2018).
Beattie, T. S., Smilenova, B., Krishnaratne, S. & Mazzuca, A. Mental health problems among female sex workers in low- and middle-income countries: A systematic review and meta-analysis. PLoS Med. 17 , e1003297 (2020).
Ismail, Z. et al. Prevalence of depression in patients with mild cognitive impairment: A systematic review and meta-analysis. JAMA Psychiatry 74 , 58–67 (2017).
Lim, G. Y. et al. Prevalence of depression in the community from 30 countries between 1994 and 2014. Sci. Rep. 8 , 1–10 (2018).
Article ADS Google Scholar
Jones-White, D. R., Soria, K. M., Tower, E. K. B. & Horner, O. G. Factors associated with anxiety and depression among U.S. doctoral students: Evidence from the gradSERU survey. J. Am. Coll. Health https://doi.org/10.1080/07448481.2020.1865975 (2021).
Moher, D., Liberati, A., Tetzlaff, J. & Altman, D. G. Preferred reporting items for systematic reviews and meta-analyses: The PRISMA statement. Ann. Intern. Med. 151 , 264–269 (2009).
Helmers, K. F., Danoff, D., Steinert, Y., Leyton, M. & Young, S. N. Stress and depressed mood in medical students, law students, and graduate students at McGill University. Acad. Med. 72 , 708–714 (1997).
Rabkow, N. et al. Facing the truth: A report on the mental health situation of German law students. Int. J. Law Psychiatry 71 , 101599 (2020).
Bergin, A. & Pakenham, K. Law student stress: Relationships between academic demands, social isolation, career pressure, study/life imbalance and adjustment outcomes in law students. Psychiatr. Psychol. Law 22 , 388–406 (2015).
Stang, A. Critical evaluation of the Newcastle-Ottawa scale for the assessment of the quality of nonrandomized studies in meta-analyses. Eur. J. Epidemiol. 25 , 603–605 (2010).
Freeman, M. F. & Tukey, J. W. Transformations related to the angular and the square root. Ann. Math. Stat. 21 , 607–611 (1950).
Article MathSciNet MATH Google Scholar
DerSimonian, R. & Laird, N. Meta-analysis in clinical trials. Control Clin. Trials 7 , 177–188 (1986).
Wilson, E. B. Probable inference, the law of succession, and statistical inference. J. Am. Stat. Assoc. 22 , 209–212 (1927).
Newcombe, R. G. Two-sided confidence intervals for the single proportion: Comparison of seven methods. Stat. Med. 17 , 857–872 (1998).
Higgins, J. P. T. & Thompson, S. G. Quantifying heterogeneity in a meta-analysis. Stat. Med. 21 , 1539–1558 (2002).
Kroenke, K., Spitzer, R. L. & Williams, J. B. W. The PHQ-9: Validity of a brief depression severity measure. J. Gen. Intern. Med. 16 , 606–613 (2001).
Download references
Acknowledgements
We thank the following investigators for generously sharing their time and/or data: Gordon J. G. Asmundson, Ph.D., Amy J. L. Baker, Ph.D., Hillel W. Cohen, Dr.P.H., Alcir L. Dafre, Ph.D., Deborah Danoff, M.D., Daniel Eisenberg, Ph.D., Lou Farrer, Ph.D., Christy B. Fraenza, Ph.D., Patricia A. Frazier, Ph.D., Nadia Corral-Frías, Ph.D., Hanga Galfalvy, Ph.D., Edward E. Goldenberg, Ph.D., Robert K. Hindman, Ph.D., Jürgen Hoyer, Ph.D., Ayako Isato, Ph.D., Azharul Islam, Ph.D., Shanna E. Smith Jaggars, Ph.D., Bumseok Jeong, M.D., Ph.D., Ju R. Joeng, Nadine J. Kaslow, Ph.D., Rukhsana Kausar, Ph.D., Flavius R. W. Lilly, Ph.D., Sarah K. Lipson, Ph.D., Frances Meeten, D.Phil., D.Clin.Psy., Dhara T. Meghani, Ph.D., Sterett H. Mercer, Ph.D., Masaki Mori, Ph.D., Arif Musa, M.D., Shizar Nahidi, M.D., Ph.D., Arthur M. Nezu, Ph.D., D.H.L., Angelo Picardi, M.D., Nicole E. Rossi, Ph.D., Denise M. Saint Arnault, Ph.D., Sagar Sharma, Ph.D., Bryony Sheaves, D.Clin.Psy., Kennon M. Sheldon, Ph.D., Daniel Shepherd, Ph.D., Keisuke Takano, Ph.D., Sara Tement, Ph.D., Sherri Turner, Ph.D., Shawn O. Utsey, Ph.D., Ron Valle, Ph.D., Caleb Wang, B.S., Pengju Wang, Katsuyuki Yamasaki, Ph.D.
A.C.T. acknowledges funding from the Sullivan Family Foundation. This paper does not reflect an official statement or opinion from the County of San Mateo.
Author information
Authors and affiliations.
Center for Global Health, Massachusetts General Hospital, Boston, MA, USA
Emily N. Satinsky & Alexander C. Tsai
San Mateo County Behavioral Health and Recovery Services, San Mateo, CA, USA
Tomoki Kimura
Department of Epidemiology and Population Health, Stanford University, Palo Alto, CA, USA
Mathew V. Kiang
Center for Population Health Sciences, Stanford University School of Medicine, Palo Alto, CA, USA
Harvard Society of Fellows, Harvard University, Cambridge, MA, USA
Rediet Abebe
Department of Electrical Engineering and Computer Science, University of California Berkeley, Berkeley, CA, USA
Department of Economics, Hankamer School of Business, Baylor University, Waco, TX, USA
Scott Cunningham
Department of Sociology, Washington University in St. Louis, St. Louis, MO, USA
Department of Microbiology, Immunology, and Molecular Genetics, Institute for Quantitative and Computational Biosciences, University of California Los Angeles, Los Angeles, CA, USA
Xiaofei Lin
Departments of Newborn Medicine and Psychiatry, Brigham and Women’s Hospital, Boston, MA, USA
Cindy H. Liu
Harvard Medical School, Boston, MA, USA
Cindy H. Liu & Alexander C. Tsai
Centre for Global Health, Edinburgh Medical School, Usher Institute, University of Edinburgh, Edinburgh, Scotland, UK
Department of Psychiatry, University of Michigan, Ann Arbor, MI, USA
Department of Global Health, Institute for Life Course Health Research, Stellenbosch University, Cape Town, South Africa
Mark Tomlinson
School of Nursing and Midwifery, Queens University, Belfast, UK
Fielding School of Public Health, Los Angeles Area Health Services Research Training Program, University of California Los Angeles, Los Angeles, CA, USA
Miranda Yaver
Mongan Institute, Massachusetts General Hospital, Boston, MA, USA
Alexander C. Tsai
You can also search for this author in PubMed Google Scholar
Contributions
A.C.T. conceptualized the study and provided supervision. T.K. conducted the search. E.N.S. contacted authors for additional information not reported in published articles. E.N.S. and T.K. extracted data and performed the quality assessment appraisal. E.N.S. and A.C.T. conducted the statistical analysis and drafted the manuscript. T.K., M.V.K., R.A., S.C., H.L., X.L., C.H.L., I.R., S.S., M.T. and M.Y. contributed to the interpretation of the results. All authors provided critical feedback on drafts and approved the final manuscript.
Corresponding authors
Correspondence to Emily N. Satinsky or Alexander C. Tsai .
Ethics declarations
Competing interests.
The authors declare no competing interests.
Additional information
Publisher's note.
Springer Nature remains neutral with regard to jurisdictional claims in published maps and institutional affiliations.
Supplementary Information
Supplementary information., rights and permissions.
Open Access This article is licensed under a Creative Commons Attribution 4.0 International License, which permits use, sharing, adaptation, distribution and reproduction in any medium or format, as long as you give appropriate credit to the original author(s) and the source, provide a link to the Creative Commons licence, and indicate if changes were made. The images or other third party material in this article are included in the article's Creative Commons licence, unless indicated otherwise in a credit line to the material. If material is not included in the article's Creative Commons licence and your intended use is not permitted by statutory regulation or exceeds the permitted use, you will need to obtain permission directly from the copyright holder. To view a copy of this licence, visit http://creativecommons.org/licenses/by/4.0/ .
Reprints and permissions
About this article
Cite this article.
Satinsky, E.N., Kimura, T., Kiang, M.V. et al. Systematic review and meta-analysis of depression, anxiety, and suicidal ideation among Ph.D. students. Sci Rep 11 , 14370 (2021). https://doi.org/10.1038/s41598-021-93687-7
Download citation
Received : 31 March 2021
Accepted : 24 June 2021
Published : 13 July 2021
DOI : https://doi.org/10.1038/s41598-021-93687-7
Share this article
Anyone you share the following link with will be able to read this content:
Sorry, a shareable link is not currently available for this article.
Provided by the Springer Nature SharedIt content-sharing initiative
This article is cited by
How to improve academic well-being: an analysis of the leveraging factors based on the italian case.
- Alice Tontodimamma
- Emiliano del Gobbo
- Antonio Aquino
Quality & Quantity (2024)
A single-center assessment of mental health and well-being in a biomedical sciences graduate program
- Sarah K. Jachim
- Bradley S. Bowles
- Autumn J. Schulze
Nature Biotechnology (2023)
Mental Health Problems Among Graduate Students in Turkey: a Cross-Sectional Study
- Cafer Kılıç
- Faika Şanal Karahan
International Journal for the Advancement of Counselling (2023)
Suicidal affective risk among female college students: the impact of life satisfaction
- Dawei Huang
- Xianbin Wang
Current Psychology (2023)
A study in University of Ruhuna for investigating prevalence, risk factors and remedies for psychiatric illnesses among students
- Patikiri Arachchige Don Shehan Nilm Wijesekara
Scientific Reports (2022)
By submitting a comment you agree to abide by our Terms and Community Guidelines . If you find something abusive or that does not comply with our terms or guidelines please flag it as inappropriate.
Quick links
- Explore articles by subject
- Guide to authors
- Editorial policies
Sign up for the Nature Briefing newsletter — what matters in science, free to your inbox daily.


An official website of the United States government
The .gov means it’s official. Federal government websites often end in .gov or .mil. Before sharing sensitive information, make sure you’re on a federal government site.
The site is secure. The https:// ensures that you are connecting to the official website and that any information you provide is encrypted and transmitted securely.
- Publications
- Account settings
Preview improvements coming to the PMC website in October 2024. Learn More or Try it out now .
- Advanced Search
- Journal List
- Public Health Rev
- PMC10227668

A 7-Step Guideline for Qualitative Synthesis and Meta-Analysis of Observational Studies in Health Sciences
Marija glisic.
1 Institute of Social and Preventive Medicine, University of Bern, Bern, Switzerland
2 Swiss Paraplegic Research, Nottwil, Switzerland
Peter Francis Raguindin
3 Graduate School for Health Sciences, University of Bern, Bern, Switzerland
4 Faculty of Health Science and Medicine, University of Lucerne, Lucerne, Switzerland
Armin Gemperli
5 Institute of Primary and Community Care, University of Lucerne, Lucerne, Switzerland
Petek Eylul Taneri
6 HRB-Trials Methodology Research Network, National University of Ireland, Galway, Ireland
Dante Jr. Salvador
Trudy voortman.
7 Department of Epidemiology, Erasmus MC, University Medical Center, Rotterdam, Netherlands
8 Division of Human Nutrition and Health, Wageningen University and Research, Wageningen, Netherlands
Pedro Marques Vidal
9 Department of Medicine, Internal Medicine, Lausanne University Hospital (CHUV) and University of Lausanne, Lausanne, Switzerland
Stefania I. Papatheodorou
10 Department of Epidemiology, Harvard TH Chan School of Public Health, Boston, MA, United States
Setor K. Kunutsor
11 Diabetes Research Centre, University of Leicester, Leicester General Hospital, Leicester, United Kingdom
12 Translational Health Sciences, Bristol Medical School, University of Bristol, Southmead Hospital, Bristol, United Kingdom
Arjola Bano
13 Department of Cardiology, Inselspital, Bern University Hospital, University of Bern, Bern, Switzerland
John P. A. Ioannidis
14 Stanford Prevention Research Center, Department of Medicine, Stanford University School of Medicine, Stanford, CA, United States
15 Department of Epidemiology and Population Health, Stanford University School of Medicine, Stanford, CA, United States
16 Department of Statistics, Stanford University, Stanford, CA, United States
17 Meta-Research Innovation Center at Stanford (METRICS), Stanford University, Stanford, CA, United States
Taulant Muka
18 Epistudia, Bern, Switzerland
Associated Data
Objectives: To provide a step-by-step, easy-to-understand, practical guide for systematic review and meta-analysis of observational studies.
Methods: A multidisciplinary team of researchers with extensive experience in observational studies and systematic review and meta-analysis was established. Previous guidelines in evidence synthesis were considered.
Results: There is inherent variability in observational study design, population, and analysis, making evidence synthesis challenging. We provided a framework and discussed basic meta-analysis concepts to assist reviewers in making informed decisions. We also explained several statistical tools for dealing with heterogeneity, probing for bias, and interpreting findings. Finally, we briefly discussed issues and caveats for translating results into clinical and public health recommendations. Our guideline complements “A 24-step guide on how to design, conduct, and successfully publish a systematic review and meta-analysis in medical research” and addresses peculiarities for observational studies previously unexplored.
Conclusion: We provided 7 steps to synthesize evidence from observational studies. We encourage medical and public health practitioners who answer important questions to systematically integrate evidence from observational studies and contribute evidence-based decision-making in health sciences.
Introduction
Observational studies are more common than experimental studies ( 1 , 2 ). Moreover, many systematic reviews and meta-analyses (SRMA) integrate evidence from observational studies. When undertaking synthesis and MA, it is crucial to understand properties, methodologies, and limitations among different observational study designs and association estimates derived from these studies. Different study designs influence variability in results among studies, and thus heterogeneity and conclusions ( Supplementary Material S1 ). Specific study type considerations and methodological features include (among others): study participant selection and study sample representation; measurement and characterization methods for exposure and extent of information bias; potential confounders and outcomes; design-specific contributions leading to bias; and methods used to analyze the data. Furthermore, observational studies may have a wider array of selective reporting biases than randomized trials. Most observational studies are unregistered, and typically more degrees of analytical flexibility and choice of analyses report such designs compared with randomized trials, leading to more variable results and potential bias ( 3 ). These methodological components influence study design suitability and result in trustworthiness for SRMA. Indeed, evidence shows that MAs of observational studies often suffer methodologically ( 1 ), and despite statistical or other summary result significance, many observational studies demonstrate low credibility ( 2 ). Observational data often complement evidence from randomized controlled trials (RCTs) when shaping public health and clinical guidelines and recommendations. Yet, observational data for informing public health and clinical decision-making are inconsistently available in SRMAs. Therefore, we provide concise guidance for combining results in a MA of observational studies.
The current guideline was developed by a multidisciplinary team of researchers with extensive experience in SRMAs. The guide extends a previous guideline ( 4 ) and provides further recommendations for synthesizing and pooling results from observational data. For this, we considered previous guidances for SRMA of observational studies ( 5 – 7 ), and acknowledged several contentious points concerning optimal methods for MA of observational studies ( 8 ). We explicitly address such uncertainties and offer definitive recommendations for uncontested best practices. Finally, we offer guidance relevant to diverse types of observational data subject to SRMA. However, the range of observational data types, such as adverse drug events, genetic associations, effectiveness studies, nutritional associations, air pollution, and prevalence studies, is broad. Therefore, proper evidence synthesis requires knowledge of best SR practices and field-specific subject matter.
Step-by-Step Guide
The overall step-by-step guidance is visualized in Figure 1 .

The 7-Step Guide which illustrates the steps for synthesis and meta-analysis of observational studies (Bern, Switzerland. 2023).
Step 1. Decide Whether Narrative or Descriptive Data Synthesis or Meta-Analysis is Suitable
When summarizing evidence from observational studies, narrative or descriptive data synthesis is desirable when: a) the number of studies is insufficient to perform MA; b) essential information to combine results from different studies is missing across studies; or c) the evidence is judged as too heterogeneous, such as clinical heterogeneity, based on a priori decision. We provide tips for determining when clinical heterogeneity is too high in Figure 2 . We caution early, careful thinking and decision-making about handling complex patterns of bias in available evidence and pre-specified protocols. Otherwise, observed results can drive included study choices prematurely.
- a. How many studies are sufficient for MA? MA is possible if association estimates from two studies are available. However, deciding to perform a MA ( 9 )—see Step 2 for choosing statistical models—is influenced by differences in study design, exposure, adjustment, outcome assessment, study population, risk of bias, and other methodological features across studies.
- b. What information is essential for MA? To combine study results, measurements of association estimates from individual studies and standard errors or 95% confidence intervals (CIs) of the estimate are needed. For details about combining different estimates and information needed, see Step 3 and Supplementary Table S1 . We suggest contacting the corresponding authors for missing essential information.
- c. When is heterogeneity too large? Without widely accepted, automated quantitative measures to grade it, determining whether clinical or methodological heterogeneity is too high is subjective. Heterogeneity can result from methodological differences, such as different study designs, analytical assessments of exposures/outcomes, or variations among populations across different studies; it requires restricting MA based on study population, design, exposure, or outcome characteristics. To see how statistical heterogeneity is explored quantitatively using I 2 or Cochran Q statistics, see Step 6. Deciding to perform MA should not be based on statistical heterogeneity.
- d. Do “study quality” and methodological rigor determine whether to meta-analyze the evidence? “Study quality” is a complex term; it involves assessing methodological rigor (what was done) and completeness or accuracy of reporting (what is reported to have been done) within individual studies. Established and validated risk of bias tools can evaluate individual studies included in SR, which can inform the synthesis and interpretation of results. Poor methodological rigor and incomplete or inaccurate reporting of individual studies can bias synthesized results and limit MA interpretation and generalizability. Thus, potential biases across included studies should be systematically assessed. Various tools and scales can be used to assess methodological rigor and reporting. We summarize these scales in Supplementary Material S2 , Supplementary Table S2 .
- e. Does the study design determine whether to meta-analyze the evidence?

Factors to consider on whether to perform a meta-analysis or not (Bern, Switzerland. 2023).
Including all study designs in SRs reduces subjective interpretations of potential biases and inappropriate study exclusions ( 6 ); however, the decision to meta-analyze results across all study designs depends on research questions. For example, cross-sectional designs are likely inappropriate for research questions dealing with temporality but could be used to summarize prevalence estimates of diseases. If different study designs are included in SRs, address heterogeneity by study design in the MA step and perform subgroup analyses by study design otherwise, misleading results can follow ( 10 ).
Overall, when deciding to remove studies from MA due to poor methodology, it is crucial to evaluate the extent of bias across available evidence (i.e., bias in single or multiple studies). If all available studies provide biased estimates, MA simply provide a composite of these errors with low-reliability results perpetuating these biases. If only a proportion of studies are biased and subsequently included in MA, stratification by methodological features may be a solution. However, even with enough studies in the synthesis to perform subgroup analysis, it is informative only. More details are provided in Steps 6 and 7.
After carefully considering Step 1 items a–e, if MA is not feasible or meaningful, summarize findings qualitatively with narrative or descriptive data synthesis. Descriptive data synthesis is not necessarily worse or lower quality compared with MA. Depending on the number of included studies and methodological differences across studies in a descriptive synthesis, writing a narrative data summary can prove more difficult compared with MA. In Table 1 , we provide insights for simplifying the process of descriptive data synthesis. We use examples, such as grouping studies and presenting data from previously published SRs summarizing evidence without MA ( 12 – 15 ).
Steps to consider when conducting a narrative summary of evidence (Bern, Switzerland. 2023).
We suggest providing graphical summaries of important findings, especially when tables and figures amass complex, convoluted information [e.g., second figure of SR by Oliver-Williams et al. ( 12 )]. If MA is inappropriate, another graphical option is a forest plot without the overall association estimate 15 —a display that promotes reader insights on association estimate size and 95% CIs across studies. We also recommend synthesis without MA (SWiM) reporting guidelines ( 11 ) to assist in reporting findings from SRs without MA. Finally, although narrative synthesis of evidence is the default choice when performing an SR of qualitative research, it extends beyond the scope of our guidelines. Several guidelines exist on SR of qualitative studies ( 16 , 17 ).
Step 2. Understand the Concept of Meta-Analysis and Different Models
Combining results from different observational studies can lead to more comprehensive evaluations of evidence, greater external validity, and higher precision due to larger sample sizes. However, higher precision can be misleading, especially if studies are biased.
MA mathematically combines different study results ( 18 ); it computes summary statistics for each study, then further summarizes and interprets study-level summary statistics. Summary association estimates allow for overall judgments on investigated associations; however, the interpretation depends on assumptions and models used when combining data across studies. Observational studies are far more susceptible to confounding and bias; therefore, they have additional degrees of imprecision beyond observed CIs. Furthermore, many associations differ by study characteristics and exposure levels and types; thus, true effect size genuinely varies. Weighting studies in meta-analyses typically considers study imprecision and heterogeneity between studies, yet some also weigh quality scores ( 19 ). We generally discourage including quality scores because they are subjective, and it is difficult to summarize quality in a single number or weight. Nevertheless, when combining studies of different designs or identifying large discrepancies in risks of bias, additional subgroup or sensitivity analyses such as excluding studies with lower credibility and identifying influences of such studies in summary estimates. More sophisticated methods try to “correct” results for different types of bias related to internal validity and generalizability features in each study ( 20 ). Yet, they are not widely used and worthy of skepticism for claims to correct bias ( 21 ).
Fixed-Effects Model
If a single effect underlies an investigated association and all studies are homogenous, obtain a summary estimate by weighted mean by measuring that effect in fixed-effects models. The weights reflect each study’s precision. Precision is the degree of resemblance among study results if the study is repeated under similar circumstances.
Estimate precision is mainly related to variations of random error, such as sample size or the number of events of interest; measurement uncertainty—accurate and calibrated measurements—and the nature of measured phenomenon (where some events are simply more variable than others in occurrence) also affect estimate precision. The precision of estimates is expressed as the inverse variance of association estimates—or 1 divided by the square root of its standard error. Summary estimates are referred to as the fixed-effects association estimate. Fixed-effects models assume all studies have a common true (“fixed”) overall effect and any differences in observed estimates between studies are due to random error—a strong assumption typically unsuitable for most observational data.
Random-Effects Model
The random-effects model allows each study its own exposure or treatment association estimate with distributed associations varied across different individual and population characteristics, as well as dependent on exposure and treatment characteristics, such as dose or category. We expect sufficient statistical commonalities across studies when combining information; however, identical true association estimates are unnecessary for included studies. For example, the association between hormone therapy and the risk of cardiovascular disease among women depends on menopausal status and the type of hormone therapy. Although studies investigating hormone therapy and cardiovascular disease have exposure, population, and outcome in common, there are different true effects across different reproductive stages and formulations of hormone therapies ( 22 ).
The random-effects model is an extension of the fixed-effects model, where each study estimate combines the true total effect and difference from variation between studies and random errors. Therefore, an additional parameter represents variability between studies around the true common effect and distinguishes random-effects models from fixed-effects models. To simplify, random-effects models distribute true effect sizes represented across different studies. The combined random-effects estimates represent the mean of the population of true effects. Thus, we can generalize findings to broader phenotypes and populations beyond specific, well-defined phenotypes and populations analyzed in individual studies. For instance, an MA of observational studies on hormone therapy and cardiovascular disease provides an overall measure of association estimates between hormone therapy and cardiovascular disease; however, random-effects estimates are summary estimates of the overall true measure of association estimates of different types of hormone therapies and true measured of observed association estimates among different women’s reproductive stages. As a result, random-effects models incorporate higher degrees of heterogeneity between studies. It also gives proportionally higher weights to smaller studies and lower weights to larger studies than the fixed-effects association estimates, resulting in differences in summary estimates between the two models.
The random-effects model incorporates study variance and results to wider CIs. However, random and fixed-effects estimates would be similar, with no observed between-study variability and zero estimated between-study variance. There are many variants of random-effects models ( 23 ). Inverse variance and DerSimonian-Laird methods are the most widely used, yet these are not methods with the best statistical properties in most circumstances. Therefore, accurate working knowledge of alternatives and choosing the best-fit methods is essential ( 23 ).
We previously compared different characteristics of fixed-effects vs. random-effects in Supplementary Table S3 . Since observational studies typically involve variable study populations, different levels of adjustments and analyses than RCTs, and participants under different conditions, they are usually better represented by random-effects than fixed-effects models. It is even more true when different study designs are combined or when observational studies are combined with RCTs. However, random-effects models also come with several caveats. For example, estimates of between-study variance in calculations of limited numbers of studies are very uncertain; different random-effects methods yield substantially different results; in the presence of publication selection bias (mostly affecting smaller studies), random-effects models give even more importance to smaller studies and summary estimates are more biased than fixed-effects models. Some methodologists propose methods to overcome these issues, such as only combining large enough studies, using other forms of weighting, or correcting for publication and other selective reporting biases ( 24 – 26 ). Familiarity with the data at hand and the suitability of methods related to specific MAs is crucial.
Step 3. Follow the Statistical Analysis Plan
Statistical analysis plans are designed during SR protocol preparation; we describe such plans in Step 6 of our previously published guideline ( 4 ). In addition to detailed descriptions of planned analyses, SR protocols provide descriptions of research questions, study designs, inclusion and exclusion criteria, electronic databases, and preliminary search strategies. We previously discussed review protocol preparation in detail ( 4 ). Further detailed instructions on how to prepare a statistical analysis plan can be found in Supplementary Material S3 .
Step 4. Prepare Datasets for Meta-Analysis
Prior to MA, examine the results extracted from each study with either a dichotomous or continuous outcome ( Supplementary Material S4 ).
If studies use different units when reporting findings, convert units for consistency before combining. Decide units (SI or conventional units) and scales (meter, centimeter, millimeter) before mathematically combining study estimates. Resolve differences in reporting summary statistics, such as measures of central tendency (mean or median) and spread (range or standard deviation). Convert studies reporting median and interquartile range (or range) to mean and standard deviation through a priori -defined methods, such as those described by Hozo or Wan ( 27 , 28 ). Although studies not reporting summary statistics or central tendency and spread are excluded from meta-analyses, keep track of them and discuss unusable evidence and inference effects. Determine if outcomes are normally distributed. Transform values from studies reporting non-normal distributions for combination, such as log transformation.
Data reflecting risk at multiple levels of exposure, such as quantiles, present special challenges. By only extracting estimates of risk in upper versus lower levels of exposure, such as nutrient levels in nutritional associations, valuable information is lost. We suggest an interval collapsing method ( 29 ) that allows using information from all levels of exposure. Consider issues of dose-response relationships and non-linearity. Prespecify the plans for extracting and synthesizing relevant data. We advise reading and discussing articles about common MA methods on trends and dose-response ( 30 – 32 ). If studies use different cut-points to define exposure categories for continuous exposures, carefully record and consider them in the analysis ( 33 ).
Since most SR involves fewer than 100 studies, use simple spreadsheet applications to encode study details and association estimates. Use dedicated database management software, such as RedCap (free) or Microsoft Access (commercial). Recently popularized machine-learning-based software, such as Covidence (with limited validity), helps extract data, screen abstracts, and assess the quality and allows data transfer to RevMan (Cochrane Collaboration). RevMan is a multifunctional MA software performing qualitative and quantitative analyses and may be suitable for beginners. However, many MA methods are unavailable in RevMan, which limits analysis options. R (free) and Stata (commercial) are other softwares one may consider for data analysis ( Supplementary Table S4 ), We also recommend mapping adjusted variables from in each study and the analyses done (main analyses and subgroup or restricted analyses). It allows a bird’s eye view of what adjustments were made, how consistent or different adjustments considered for inclusion in the MA were across different studies, and whether different unadjusted and adjusted estimates were provided in specific studies. Adjusted and unadjusted or crude association estimates across studies are often available, and differences should be accounted and explained. When preparing data analysis plans, common dilemmas include choosing among several models and the provided variably adjusted estimates. When undertaking a synthesis or review for a particular research question using causal structures, such as through DAGs, identify confounders ideally included in studies’ adjusted models in the selection criterion. When selecting estimates for MA, limit analysis to studies adjusting for confounders defined important a priori . Alternatively, combine different covariate-conditional estimates, such as conducting minimally adjusted and maximally adjusted analyses and comparing summary results. When combining estimates from studies with estimated different covariate-conditional effects, we advise caution regarding the non-collapsibility of odds and hazard ratios, where covariate-conditional odds ratios may differ from crude odds ratios even in the absence of confounding; however, estimates of risk ratios do not exhibit this problem ( 34 ). Ultimately, compare sensitivity analysis results between meta-analyses of adjusted and unadjusted data to indicate the presence of biases.
Step 5. Run the Meta-Analysis
Meta-analysis for dichotomous outcome.
The most common measures of associations for dichotomous outcomes are proportions and prevalences, risk ratios, odds ratios, relative risks, hazard ratios, or risk differences. Mathematically transform and approximately normally distribute each of these association measures into new measures on a continuous scale. Meta-analyze transformed measures using standard tools for continuous effect sizes where derived summary effects may be finally back-transformed into its original scale. We provide an overview of study designs and common transformations in Supplementary Tables S5–S7 .
Originally developed as a technique for examining odds ratios with stratification in mind, the Mantel-Haenszel method was not originally developed for MA. The Mantel-Haenszel approach bypasses the need to first transform risk estimates, performs an inverse-variance weighted MA, and then back transforms summary estimators. With a weighted average of the effect sizes result, applying it directly to study risk ratios, odds ratios, or risk differences is advised. It provides robust estimates when data are sparse and produces estimates similar to the inverse variance method in other situations. Therefore, the method can be widely used. Peto’s approach is an alternative to the Mantel-Haenszel method for combining odds ratios. Peto’s summary odds ratio can be biased, especially when there are large differences in sample sizes between treatment arms; however, it generally works well in other situations. Although the Mantel-Haenszel and Peto methods pertain to raw counts with no applicability in most meta-analyses of observational data where adjusted estimates are considered, they may apply to types of observational data where raw counts are involved, such as adverse events.
When outcomes in comparison groups are either 100% or zero, computational difficulties arise in one (single-zero studies) or both (double-zero studies) comparison groups. Some studies purposely remove double-zero studies from their analyses. However, such approaches are problematic when meta-analyzing rare events, such as surgical complications and adverse medication effects. In these instances, a corrective count—typically 0.5—is added to the group with an otherwise zero count. The metan package in Stata and the metabin command from the meta library in R correct these by default. Nyaga et al. ( 35 ) provide a guide for Stata. Such arbitrary corrections possibly introduce bias or even reverse MA results, especially when the number of samples in two groups is unbalanced ( 10 ). We advise avoiding altogether or extreme caution when using methods that ignore information from double-zero studies or use continuity corrections. Beta-binomial regression methods may be the best approach for treating such studies when computing summary estimates for relative risks, odds ratios, or risk differences ( 36 ).
Meta-Analysis for Continuous Outcomes
For continuous outcomes, investigate two exposure groups (exposed vs. unexposed) or per unit increase in exposure in terms of their mean outcome level. The association is quantified as the mean difference—for example, the difference between study groups in mean weight loss—or as beta-coefficient from univariable or multivariable regression models. A MA can then directly summarize mean differences for each study. If different measurement scales, such as different instruments or different formulas to derive outcomes, are available, we advise using standardized mean differences as measures of association estimates in MA—the mean difference divided by pooled standard deviation. Use one of several ways to calculate pooled standard deviation, such as the most popular methods for standardized effect sizes: Cohen’s D, Hedge’s g, and Glass’ delta ( 37 – 39 ).
To measure standardized size effects, combine mean, standard deviation, and sample size of exposed and non-exposed groups as input with different weights. If using software, select the standardization method. Hedge’s g includes a correction factor for small sample bias; it is preferred over Cohen’s D for very small sample sizes (fewer than 20) ( 39 ). Otherwise, the two methods give very similar results. Expressing the standardized effect measure demonstrates differences between exposed and non-exposed groups by standard deviation. For example, if Hedge’s g is 1, groups differ by 1 standard deviation and so on. When standard errors are very different between study groups, Glass’s delta—a measure using only the standard deviation of the unexposed group—is usually used to measure effect size ( 38 ). If mean differences or standardized mean differences are combined, calculate with only the effect size and standard deviation of individual groups. The software calculates the differences and associated variance of differences for weighting—the standardized mean differences with appropriate variance estimation ( Supplementary Tables S6, S7 , example Supplementary Figures S1, S2 ).
95% Confidence Intervals (CIs) and Prediction Intervals
Providing 95% CIs and prediction intervals is desirable when performing a MA. CIs reflect sampling uncertainty and quantify the precision of mean summary measures of association estimates; prediction intervals reflect expected uncertainty in summary estimates when including a new study in meta-analyses. Prediction intervals—along with sampling uncertainty—reflect inherent uncertainty about specific estimates and estimate the interval of a new study if randomly selected from the same population of studies already included in meta-analyses ( 40 , 41 ). Implement prediction intervals in random-effects MA frameworks. To calculate prediction intervals, 3 studies are required; however, considering prediction intervals account for the variance of summary estimates and heterogeneity, they can be imprecise for MA of few studies.
Step 6. Explore Heterogeneity
Cochran’s Q homogeneity test and its related metric—the Higgin’s & Thompson’s I 2 index—are commonly used in most statistical software (Stata, R, and RevMan). Under the hypothesis of homogeneity among the effect sizes ( 42 ), the Q test follows a Chi-square distribution (with k-1 degrees of freedom, where k is number of studies). The Q test is used to evaluate the presence or absence of statistically significant heterogeneity based on α threshold of statistical significance ( 43 ). Calculated as [Q−df]/x 100, the I 2 measures the proportion of the total variability in effect size due to between-study heterogeneity rather than sampling error. I 2 is highly influenced by the size of the studies (within-study variability), not just the size of between-study heterogeneity. A higher percentage indicates higher heterogeneity. H is the square root of the Chi-square heterogeneity statistic divided by its degrees of freedom. It describes relative differences between observed and expected Q in the absence of heterogeneity. The H value of 1 indicates perfect homogeneity. R is the ratio of the standard error of the underlying mean from random-effects meta-analyses to standard errors of a fixed-effects meta-analytic estimate. Similar to H, the R 2 value of 1 indicates perfect homogeneity. Finally, τ 2 is the estimate of between-study variance under random-effects models. τ 2 is an absolute measure of between-study heterogeneity; in contrast to other measures (Q, I 2 , H, and R), it does not depend on study precision ( 44 ). Further information about heterogeneity can be found here ( 45 ).
Classification of Heterogeneity
Assessing heterogeneity in SRs is crucial in the synthesis of observational studies. Recall that the reliability of heterogeneity tests hinges on the number of studies. Thus, fewer studies make I 2 estimates unreliable. To classify heterogeneity, different categorizations are used across different meta-analyses. The Cochrane Collaboration recommends classifying 0%–40% as likely unimportant heterogeneity; 30%–60% as likely moderate heterogeneity; 50%–90% as likely substantial heterogeneity; and 75%–100% as likely considerable heterogeneity ( 18 ). Although there is no rule of thumb for I 2 cut-offs to classify studies as low, medium, or high heterogeneity, categorize using a priori protocol definitions. Provide CIs for I 2 since estimates of heterogeneity have large uncertainty ( 46 ) (See Supplementary Figures S1, S2 for examples).
Subgroup or Restricted Analysis
Ideally, all studies compared in meta-analyses should be similar; however, it is almost impossible for observational studies. When performing subgroup analyses, look at factors explaining between-study heterogeneity. Explore subgroups, including patient or individual characteristics, study methods, and exposure or outcome definitions. Define subgroup characteristics a priori . Group studies according to study characteristics. We outline a subgroup analysis essential guide in Supplementary Table S8 ( Supplementary Figure S3 provides example).
Meta-Regression
Meta-regression applies basic regression concepts using study-level association estimates ( 42 , 47 , 48 ). Examining the association—typically linear, yet not in all cases—between the outcome of interest and covariates determines the contribution of covariates (study characteristics) in the heterogeneity of the association estimates. In common regression analyses, patient-level information is used when comparing outcomes and exposures alongside various covariates. In meta-regression (instead of patient-level information) use population-level information, such as mean age, location, mean body mass index, percentage of females, mean follow-up time, and risk of bias, to explore association estimates. The common practice of visualizing meta-regressions is with bubble plots ( Supplementary Figure S4 ) using the metareg package in Stata ( 49 ).
In meta-regression, variables under investigation are potential effect modifiers. Beta-coefficient refers to incremental changes in outcomes with increasing levels of the covariate. Positive coefficients signify an increase in the outcome with increasing levels of the covariate variable; negative coefficients mean a decrease in the outcome.
It is important to understand that meta-regression explores consistency of findings and does not make causal inferences on associations. Meta-regression results are based on observational data across different studies. Thus, it suffers from similar pitfalls in causality and biases. A statistically significant association between an outcome and covariate (beta coefficient) may have a confounding variable that drives the association, albeit occasionally mitigated by multivariate analysis. In addition, covariates, in some cases, can be highly collinear. Since most SR involve fewer studies capable of meta-regression, power is also an issue. The number of studies is one major stumbling block when performing meta-regression. In multivariable analysis, the number of studies becomes more important since more studies are required. Based on recommendations from the Cochrane Handbook for Systematic Reviews of Diagnostic Test Accuracy, do not consider meta-regression with fewer than 10 studies in a MA. For multivariable regression, they advise at least 10 studies per covariate ( 50 ), which means multivariable analysis requires at least 20 studies ( 47 ). Meta-regression may also be subject to ecological fallacy. In meta-regression, we use average study participant characteristics; therefore, the association between average study participant characteristics and measures of association estimate may not be the same within and between analyzed studies. Common covariates prone to ecological fallacy are age and sex. Using individual-level data is the only way to avoid ecological fallacies ( 51 ). Use caution if concluding causality from meta-regression and interpreting results ( 52 ). False positive claims are common in meta-regression ( 50 ).
While the most commonly used meta-regression is the random-effects meta-regression, other models, such as fixed-effects meta-regression, control rate meta-regression, multivariate meta-regression and Bayesian hierarchical modeling, can be used. These methods will depend on the specifics of analysis, such as the type of data, the number of studies, and the research question. More information can be found elsewhere ( 53 , 54 ).
Perform Leave-One-Out Analysis (Influence Analysis)
An MA may include studies providing extreme positive or negative associations. Sometimes it is possible to identify such outliers visually by expecting the forest plot, but often the situation is more complex due to sampling variances across included studies ( 55 ). To explore whether the outlier influences the summary effect estimate, one can explore whether the exclusion of such study from the analysis leads to considerable changes in the summary effect estimate. In case of small number of studies, the exclusion may be done manually; yet the most commonly used statistical software provide a possibility to perform a leave-one-out analysis, which iteratively removes one study at a time from the analysis and provides recomputed summary association estimates ( 48 ). For instance, in STATA, use the metaninf package ( 56 ) or in R, use the metafor package to perform a leave-one-out analysis (example shown in Supplementary Figure S5 ). For further reading, we suggest the article on outlier and influence diagnostics for MA ( 55 ).
Step 7. Explore Publication Selection Bias
Selection bias related to the publication process—or publication selection bias—arises when disseminating study results influences the nature and direction of results ( 57 ). Publication selection biases include: a) classic publication bias or file drawer bias when entire studies remain unpublished; time-lag bias when rapid publication depends on results; b) duplicate publication bias when some data are published more than once; c) location bias or citation bias when citations and study visibility depend on results; d) language bias when study publication in different languages is differentially driven by results; and e) outcome reporting bias when only some outcomes and/or analyses are published preferentially.
A thorough literature search is the first step in preventing publication bias (explained in our previous publication) ( 4 ). In addition to bibliographic database search, rigorous search of the gray literature and study registries (for preliminary data or for unpublished results) should be done to identify other studies of interest. We summarized the most important databases in Supplementary Table S9 . In addition, one should consider whether highly specialized or very large numbers of studies without any special planning (e.g., when exposures and outcomes are commonly and routinely measured in datasets such as ubiquitous electronic health records) readily address the question of interest. Selective reporting bias is very easy to be introduced in the latter situation.
Several methods exist for exploring publication selection bias; however, no method definitively proves or disproves publication selection bias. We comment on several widely popular, yet often over-interpreted methods in the next two subsections and in Supplementary Table S10 and we urge caution against their misuse and misinterpretation. Based on statistical properties (sensitivity and specificity for detecting publication selection bias), newer tests, such as those based on evaluating excess statistical significance ( 26 ), may perform better. When less biased summary estimates of effects are desired, the Weighted Average of Adequately Powered Studies (WAAP) ( 24 ) (that focuses on studies with >80% power) may have the best performance. However, many MA has few studies and not well-powered studies at all; then any test for publication selection bias and attempt to adjust for such bias may be in vain. Even greater caution is needed in such circumstances.
Visual Inspection of Study Results
To help understand whether effect sizes differ systematically between small and large studies, funnel plots provide the simplest technique and a graphical representation ( Supplementary Figure S5 ). Funnel plot graphs demonstrate association sizes or estimates on the horizontal axis (x-axis) and the study precision, sample size, or the inverse of the standard error on the vertical axis (y-axis)—an inverted funnel. Ideally, symmetry around the estimates provided by larger studies (the tip of the inverted funnel) extends to the smaller studies (the foot of the inverted funnel). An asymmetrical funnel shape with larger estimates for smaller rather than larger studies hints at publication selection bias, yet other possible reasons exist for the same pattern. Draw cautious inferences ( 58 , 59 ). Since plain visual assessment is subjective, we do not recommend using it as the sole criterion to arbitrate publication bias.
In some observational studies, observed differences between large and small studies arise from methodological differences. Different study characteristics in study sizes can lead to heterogeneity in the analysis. For example, smaller studies can have more stringent disease criteria for inclusion (lower risk for misclassification bias) and more intricate methods for data collection (lower risk for recall bias) compared with larger studies. More commonly, smaller studies are subject to more selective analysis and reporting pressure with possibly more bias than well-designed large studies. There is no way to generalize a priori for all topics, and studies should be examined carefully in each case. Thus, in the context of observational studies, it holds even more than funnel plot asymmetry should not automatically indicate publication bias ( 9 , 10 ). In particular, any factor associated with both study effect and study size could confound the true association and cause an asymmetrical funnel. Contour-enhanced funnel plots may help interpret funnels and differentiate funnel plot asymmetry caused by statistical significance-related publication bias from other factors; however, most of these caveats still apply ( 60 ).
Statistical Tests to Explore Publication Selection Bias
Several tests and statistical methods are developed to detect (and potentially correct) publication selection bias. Egger’s test remains the most popular. It is based on linear regression of normalized association or effect estimates (using association estimates divided by standard errors) and study precision (inverse of the standard error) ( 61 , 62 ). The intercept of regression lines measures the asymmetry—the larger its deviation from zero, the bigger the funnel plot asymmetry. A p-value <0.05 indicates the presence of publication bias, which means estimates of smaller studies do not mimic estimates of larger studies. Egger’s test may be unreliable for fewer than 10 studies. We advise caution when interpreting estimates of fewer than 10 studies. Further, for log odds ratios, even in the absence of selective outcome reporting, the test inflates Type I errors (false positive findings) ( 58 , 63 ). When all studies have similar variances, test results have no meaning. Egger’s test (and other modifications) as small study effect tests (i.e., whether small and larger studies give different results) should be used rather than strictly as a test of publication selection bias (See Supplementary Figures S6, S7 for example).
Other methods have been developed to address the limitations of existing popular approaches, such as the three-parameter selection model ( 64 ), the proportion of statistical significance test ( 26 ), and variants thereof. The three-parameter selection model’s main assumption is the likelihood of publication is an increasing step function of the complement of a study’s p-value . Maximum likelihood methods estimate corrected effect sizes and the relative probability that insignificant results are published. Whereas the proportion of statistical significance test compares expected with observed proportions of statistically significant findings. Find detailed explanation elsewhere ( 26 ). Some methodologists propose the most reliable summary results are obtained by methods accommodating possibilities of publication selection bias. With proven, good statistical properties, some of these methods may be used more in the future ( 26 ). However, for typical meta-analyses with limited available data, mostly small studies, and no formal pre-registration, no methods are likely perfect. Even when not formally demonstrated, consider publication selection bias as a definite possibility.
Synthesizing data from high-quality observational studies, at low risk of bias, complements data from RCTs and may provide insight into prevalence, the generalizability of findings for different populations, and information on long-term effects and desirable or adverse events (harms) when dealing with interventions. SRs and MA help quantify associations not testable in RCTs, such as quantifying the association between age at menopause onset or obesity with health outcomes. For observational evidence which assess interventions, we recommend applying the grading of recommendations, assessment, development, and evaluation (GRADE) tool to translate results from SRs and MA into evidence-based recommendations for research and clinical and public health impact ( 65 ). Applying GRADE addresses a range of research questions related to diagnosing, screening, preventing, treating, and public health. A panel of experts formulates recommendations, ideally experienced information specialists and subject matter experts. For observational evidence pertaining to putative protective and risk factors, use a series of criteria focused on the amount of evidence, statistical support, the extent of heterogeneity, and hints of bias ( 66 ). Eventually, systematic reviews and meta-analyses are observational studies themselves. Therefore, always cautiously interpret and take special care when claiming causality and framing strong recommendations for policy and clinical decision-making.
Funding Statement
PFR and DS received funding from the European Union’s Horizon 2020 research and innovation programme under the Marie Skłodowska-Curie grant agreement No. 801076, through the SSPH+ Global PhD Fellowship Programme in Public Health Sciences (GlobalP3HS) of the Swiss School of Public Health.
Author Contributions
MG and TM conceptualized the study. MG, PFR, AG, PET, DS, AB, JPAI contributed to the writing of the manuscript. TV, PMV, SP, and SK provided critical inputs on the draft. JPAI and TM supervised the study conduct. All approved the final version of the manuscript.
Conflict of Interest
TM is Chief Scientific Officer at Epistudia, a start-up company on online learning and evidence synthesis. DS is a Co-founder and Director at CrunchLab Health Analytics, Inc., a health technology assessment consulting firm.
The remaining authors declare that they do not have any conflicts of interest.
Supplementary Material
The Supplementary Material for this article can be found online at: https://www.ssph-journal.org/articles/10.3389/phrs.2023.1605454/full#supplementary-material

An A.I. Researcher Takes On Election Deepfakes
Oren Etzioni was once an optimist about artificial intelligence. Now, his nonprofit, TrueMedia.org, is offering tools for fighting A.I.-manipulated content.
Oren Etzioni worries A.I.-generated fakes could overwhelm upcoming elections. Credit... Kyle Johnson for The New York Times
Supported by
- Share full article

By Cade Metz and Tiffany Hsu
Reporting from San Francisco
- April 2, 2024
For nearly 30 years, Oren Etzioni was among the most optimistic of artificial intelligence researchers.
But in 2019 Dr. Etzioni, a University of Washington professor and founding chief executive of the Allen Institute for A.I., became one of the first researchers to warn that a new breed of A.I. would accelerate the spread of disinformation online . And by the middle of last year, he said, he was distressed that A.I.-generated deepfakes would swing a major election. He founded a nonprofit, TrueMedia.org in January, hoping to fight that threat.
On Tuesday, the organization released free tools for identifying digital disinformation, with a plan to put them in the hands of journalists, fact checkers and anyone else trying to figure out what is real online.
The tools, available from the TrueMedia.org website to anyone approved by the nonprofit, are designed to detect fake and doctored images, audio and video. They review links to media files and quickly determine whether they should be trusted.
Dr. Etzioni sees these tools as an improvement over the patchwork defense currently being used to detect misleading or deceptive A.I. content. But in a year when billions of people worldwide are set to vote in elections, he continues to paint a bleak picture of what lies ahead.
“I’m terrified,” he said. “There is a very good chance we are going to see a tsunami of misinformation.”
In just the first few months of the year, A.I. technologies helped create fake voice calls from President Biden , fake Taylor Swift images and audio ads , and an entire fake interview that seemed to show a Ukrainian official claiming credit for a terrorist attack in Moscow. Detecting such disinformation is already difficult — and the tech industry continues to release increasingly powerful A.I. systems that will generate increasingly convincing deepfakes and make detection even harder.
Many artificial intelligence researchers warn that the threat is gathering steam. Last month, more than a thousand people — including Dr. Etzioni and several other prominent A.I. researchers — signed an open letter calling for laws that would make the developers and distributors of A.I. audio and visual services liable if their technology was easily used to create harmful deepfakes.
At an event hosted by Columbia University on Thursday, Hillary Clinton, the former secretary of state, interviewed Eric Schmidt, the former chief executive of Google, who warned that videos, even fake ones, could “drive voting behavior, human behavior, moods, everything.”
“I don’t think we’re ready,” Mr. Schmidt said. “This problem is going to get much worse over the next few years. Maybe or maybe not by November, but certainly in the next cycle.”
The tech industry is well aware of the threat. Even as companies race to advance generative A.I. systems, they are scrambling to limit the damage that these technologies can do. Anthropic, Google, Meta and OpenAI have all announced plans to limit or label election-related uses of their artificial intelligence services . In February, 20 tech companies — including Amazon, Microsoft, TikTok and X — signed a voluntary pledge to prevent deceptive A.I. content from disrupting voting.
That could be a challenge. Companies often release their technologies as “open source” software, meaning anyone is free to use and modify them without restriction . Experts say technology used to create deepfakes — the result of enormous investment by many of the world’s largest companies — will always outpace technology designed to detect disinformation.
Last week, during an interview with The New York Times, Dr. Etzioni showed how easy it is to create a deepfake. Using a service from a sister nonprofit, CivAI , which draws on A.I. tools readily available on the internet to demonstrate the dangers of these technologies, he instantly created photos of himself in prison — somewhere he has never been.
“When you see yourself being faked, it is extra scary,” he said.
Later, he generated a deepfake of himself in a hospital bed — the kind of image he thinks could swing an election if it is applied to Mr. Biden or former President Donald J. Trump just before the election.
TrueMedia’s tools are designed to detect forgeries like these. More than a dozen start-ups offer similar technology .
But Dr. Etzioni, while remarking on the effectiveness of his group’s tool, said no detector was perfect because they were driven by probabilities. Deepfake detection services have been fooled into declaring images of kissing robots and giant Neanderthals to be real photographs, raising concerns that such tools could further damage society’s trust in facts and evidence.
When Dr. Etzioni fed TrueMedia’s tools a known deepfake of Mr. Trump sitting on a stoop with a group of young Black men, they labeled it “highly suspicious” — their highest level of confidence. When he uploaded another known deepfake of Mr. Trump with blood on his fingers, they were “uncertain” whether it was real or fake.
“Even using the best tools, you can’t be sure,” he said.
The Federal Communications Commission recently outlawed A.I.-generated robocalls . Some companies, including OpenAI and Meta, are now labeling A.I.-generated images with watermarks. And researchers are exploring additional ways of separating the real from the fake.
The University of Maryland is developing a cryptographic system based on QR codes to authenticate unaltered live recordings. A study released last month asked dozens of adults to breathe, swallow and think while talking so their speech pause patterns could be compared with the rhythms of cloned audio.
But like many other experts, Dr. Etzioni warns that image watermarks are easily removed. And though he has dedicated his career to fighting deepfakes, he acknowledges that detection tools will struggle to surpass new generative A.I. technologies.
Since he created TrueMedia.org, OpenAI has unveiled two new technologies that promise to make his job even harder. One can recreate a person’s voice from a 15-second recording . Another can generate full-motion videos that look like something plucked from a Hollywood movie . OpenAI is not yet sharing these tools with the public, as it works to understand the potential dangers.
(The Times has sued OpenAI and its partner, Microsoft, on claims of copyright infringement involving artificial intelligence systems that generate text.)
Ultimately, Dr. Etzioni said, fighting the problem will require widespread cooperation among government regulators, the companies creating A.I. technologies, and the tech giants that control the web browsers and social media networks where disinformation is spread. He said, though, that the likelihood of that happening before the fall elections was slim.
“We are trying to give people the best technical assessment of what is in front of them,” he said. “They still need to decide if it is real.”
Cade Metz writes about artificial intelligence, driverless cars, robotics, virtual reality and other emerging areas of technology. More about Cade Metz
Tiffany Hsu reports on misinformation and disinformation and its origins, movement and consequences. She has been a journalist for more than two decades. More about Tiffany Hsu
Explore Our Coverage of Artificial Intelligence
News and Analysis
David Autor, an M.I.T. economist and tech skeptic, argues that A.I. is fundamentally different from past waves of computerization.
Economists doubt that artificial intelligence is already visible in productivity data . Big companies, however, talk often about adopting it to improve efficiency.
OpenAI unveiled Voice Engine , an A.I. technology that can recreate a person’s voice from a 15-second recording.
Amazon said it had added $2.75 billion to its investment in Anthropic , an A.I. start-up that competes with companies like OpenAI and Google.
Gov. Bill Lee of Tennessee signed a bill to prevent the use of A.I. to copy a performer’s voice. It is the first such measure in the United States.
French regulators said Google failed to notify news publishers that it was using their articles to train its A.I. algorithms, part of a wider ruling against the company for its negotiating practices with media outlets.
Advertisement
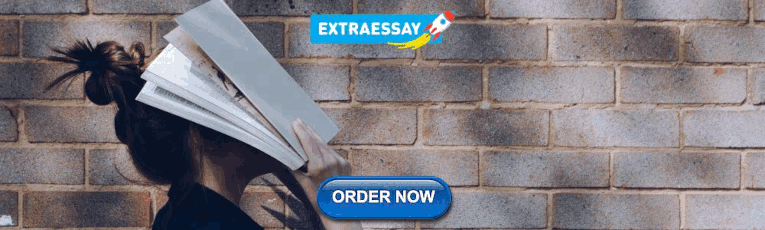
IMAGES
VIDEO
COMMENTS
2.1 Step 1: defining the research question. The first step in conducting a meta-analysis, as with any other empirical study, is the definition of the research question. Most importantly, the research question determines the realm of constructs to be considered or the type of interventions whose effects shall be analyzed.
my meta-analysis. I would also like to thank Dr. Julia Pryce for her willingness to allow me to join her in her research endeavors and provide me with support and freedom to explore the qualitative research process. Finally, I would like to extend my gratitude to Dr. Michael Borenstein for being a wonderful educator of meta-analyses. He had a way
Rule 1: Specify the topic and type of the meta-analysis. Considering that a systematic review [ 10] is fundamental for a meta-analysis, you can use the Population, Intervention, Comparison, Outcome (PICO) model to formulate the research question. It is important to verify that there are no published meta-analyses on the specific topic in order ...
The term meta-analysis was first used by Gene Glass (1976) in his presidential address at the AERA (American Educational Research Association) annual meeting, though Pearson (1904) used methods to combine results from studies on the relationship between enteric fever and mortality in 1904. The 1980s was a period of rapid development of statistical methods (Cooper & Hedges, 2009) leading to the ...
To install meta ( Figure 4 ), open RStudio (remember to install R before), (A) click Packages; (B) click Install; (C) The box for installation will open and then type the name meta. Click install and after installing, make sure that the meta package is enabled, that is, with the "check" in the box next to its name.
Meta-analysis is a central method for knowledge accumulation in many scien-tic elds (Aguinis et al. 2011c; Kepes et al. 2013). Similar to a narrative review, it serves as a synopsis of a research question or eld. However, going beyond a narra-tive summary of key ndings, a meta-analysis adds value in providing a quantitative
Meta-analysis is the quantitative, scientific synthesis of research results. Since the term and modern approaches to research synthesis were first introduced in the 1970s, meta-analysis has had a ...
Detailed steps for conducting any systematic review and meta-analysis. We searched the methods reported in published SR/MA in tropical medicine and other healthcare fields besides the published guidelines like Cochrane guidelines {Higgins, 2011 #7} [] to collect the best low-bias method for each step of SR/MA conduction steps.Furthermore, we used guidelines that we apply in studies for all SR ...
Abstract and Figures. Meta analysis refers to a process of integration of the results of many studies to arrive at evidence syn- thesis. Meta analysis is similar to systematic review; however, in ...
A review of a clearly formulated question that uses systematic and explicit methods to identify, select, and critically appraise relevant research, and to collect and analyse data from the studies that are included in the review. Statistical methods ( meta-analysis) may or may not be used to analyse and summarise the results of the included ...
It may take several weeks to complete and run a search. Moreover, all guidelines for carrying out systematic reviews recommend that at least two subject experts screen the studies identified in the search. The first round of screening can consume 1 hour per screener for every 100-200 records. A systematic review is a labor-intensive team effort.
Our thesis is that the slower adoption of meta-analytic practices in management has to do with the diverse perspectives shown by the analysis and even the way data are presented. We can synthesize the origins of the gap between the two scientific fields into four major areas: (1) limited replicability of MAs in management, mainly due to the ...
Meta-analysis provides a standardized approach for examining the existing literature on a specific, possibly controversial, issue to determine whether a conclusion can be reached regarding the effect of a treatment or exposure. Results from a meta-analysis can refute expert opinion or popular belief. For example, Nobel Laureate Linus Pauling ...
Meta-analysis is a set of statistical techniques for synthesizing data across studies. It is a statistical method for combining the findings from quantitative studies. It evaluates, synthesizes, and summarizes results. It may be conducted independently or as a specialized subset of a systematic review. A systematic review attempts to collate ...
thesis (Normand,1999). Meta analysis is essentially systematic review; however, in addition to narrative summary that is conducted in systematic review, in meta analysis, the analysts also numerically pool the results of the studies and arrive at a summary estimate. In this paper, we discuss the key steps of conducting a meta analysis.
Meta-analysis has become a widely-used tool to combine findings from independent studies in various research areas. This thesis deals with several important statistical issues in systematic reviews and meta-analyses, such as assessing heterogeneity in the presence of outliers, quantifying publication bias, and simultaneously synthesizing multiple treatments and factors.
and therefore may be a suitable approach with this population. This thesis aims to explore the efficacy of ACT in improving QoL in chronic health conditions. Methodology: A systematic literature search and analysis was undertaken utilising a meta-analysis approach. Results: A comprehensive electronic and manual search yielded a total of 1081
Meta-analysis is now the method of choice for assimilating research investigating the same question. This chapter is a nontechnical overview of the process of conducting meta-analysis in the context of clinical psychology. We begin with an overview of what meta-analysis aims to achieve. The process of conducting a meta-analysis is then ...
In a meta-analysis of the nine studies reporting the prevalence of clinically significant symptoms of anxiety across 15,626 students, the estimated proportion of students with anxiety was 0.17 (95 ...
This is a more statistical and objective way to measure publication bias in the study. The Egger's test uses a linear regression approach to measure asymmetry of the plot (Egger et al., 1997). According to this Egger's test, the regression of this meta-analysis is significant with a p-value of 0,009.
Objectives: To provide a step-by-step, easy-to-understand, practical guide for systematic review and meta-analysis of observational studies. Methods: A multidisciplinary team of researchers with extensive experience in observational studies and systematic review and meta-analysis was established. Previous guidelines in evidence synthesis were considered.
Meta Platforms is the best example of this business model. The company owns Facebook, Instagram, and WhatsApp, and this family of apps has amassed 3.98 billion monthly active users (MAUs).
An A.I. deepfake of former President Donald J. Trump sitting on a stoop with a group of young Black men was labeled "highly suspicious" by TrueMedia's tool. But a deepfake of Mr. Trump with ...