35 problem-solving techniques and methods for solving complex problems
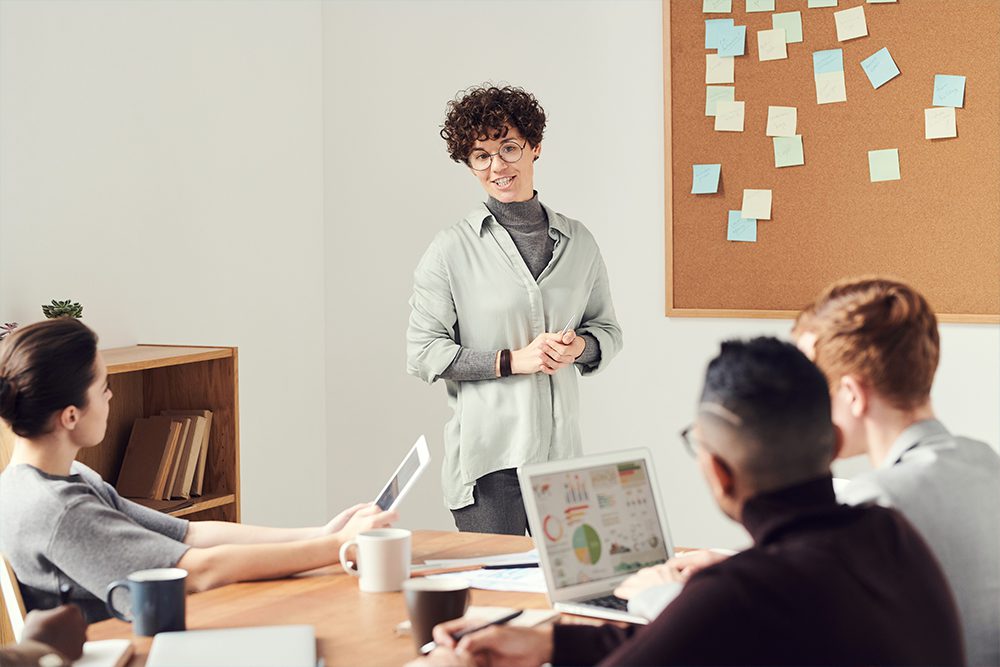
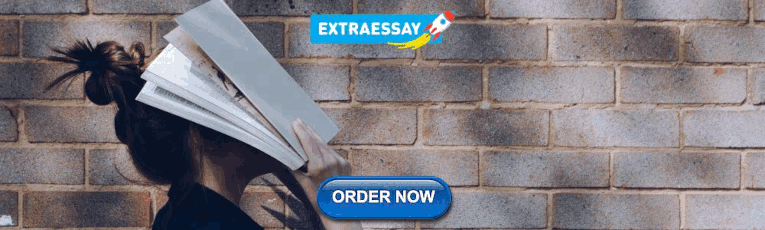
Design your next session with SessionLab
Join the 150,000+ facilitators using SessionLab.
Recommended Articles
A step-by-step guide to planning a workshop, how to create an unforgettable training session in 8 simple steps, 47 useful online tools for workshop planning and meeting facilitation.
All teams and organizations encounter challenges as they grow. There are problems that might occur for teams when it comes to miscommunication or resolving business-critical issues . You may face challenges around growth , design , user engagement, and even team culture and happiness. In short, problem-solving techniques should be part of every team’s skillset.
Problem-solving methods are primarily designed to help a group or team through a process of first identifying problems and challenges , ideating possible solutions , and then evaluating the most suitable .
Finding effective solutions to complex problems isn’t easy, but by using the right process and techniques, you can help your team be more efficient in the process.
So how do you develop strategies that are engaging, and empower your team to solve problems effectively?
In this blog post, we share a series of problem-solving tools you can use in your next workshop or team meeting. You’ll also find some tips for facilitating the process and how to enable others to solve complex problems.
Let’s get started!
How do you identify problems?
How do you identify the right solution.
- Tips for more effective problem-solving
Complete problem-solving methods
- Problem-solving techniques to identify and analyze problems
- Problem-solving techniques for developing solutions
Problem-solving warm-up activities
Closing activities for a problem-solving process.
Before you can move towards finding the right solution for a given problem, you first need to identify and define the problem you wish to solve.
Here, you want to clearly articulate what the problem is and allow your group to do the same. Remember that everyone in a group is likely to have differing perspectives and alignment is necessary in order to help the group move forward.
Identifying a problem accurately also requires that all members of a group are able to contribute their views in an open and safe manner. It can be scary for people to stand up and contribute, especially if the problems or challenges are emotive or personal in nature. Be sure to try and create a psychologically safe space for these kinds of discussions.
Remember that problem analysis and further discussion are also important. Not taking the time to fully analyze and discuss a challenge can result in the development of solutions that are not fit for purpose or do not address the underlying issue.
Successfully identifying and then analyzing a problem means facilitating a group through activities designed to help them clearly and honestly articulate their thoughts and produce usable insight.
With this data, you might then produce a problem statement that clearly describes the problem you wish to be addressed and also state the goal of any process you undertake to tackle this issue.
Finding solutions is the end goal of any process. Complex organizational challenges can only be solved with an appropriate solution but discovering them requires using the right problem-solving tool.
After you’ve explored a problem and discussed ideas, you need to help a team discuss and choose the right solution. Consensus tools and methods such as those below help a group explore possible solutions before then voting for the best. They’re a great way to tap into the collective intelligence of the group for great results!
Remember that the process is often iterative. Great problem solvers often roadtest a viable solution in a measured way to see what works too. While you might not get the right solution on your first try, the methods below help teams land on the most likely to succeed solution while also holding space for improvement.
Every effective problem solving process begins with an agenda . A well-structured workshop is one of the best methods for successfully guiding a group from exploring a problem to implementing a solution.
In SessionLab, it’s easy to go from an idea to a complete agenda . Start by dragging and dropping your core problem solving activities into place . Add timings, breaks and necessary materials before sharing your agenda with your colleagues.
The resulting agenda will be your guide to an effective and productive problem solving session that will also help you stay organized on the day!
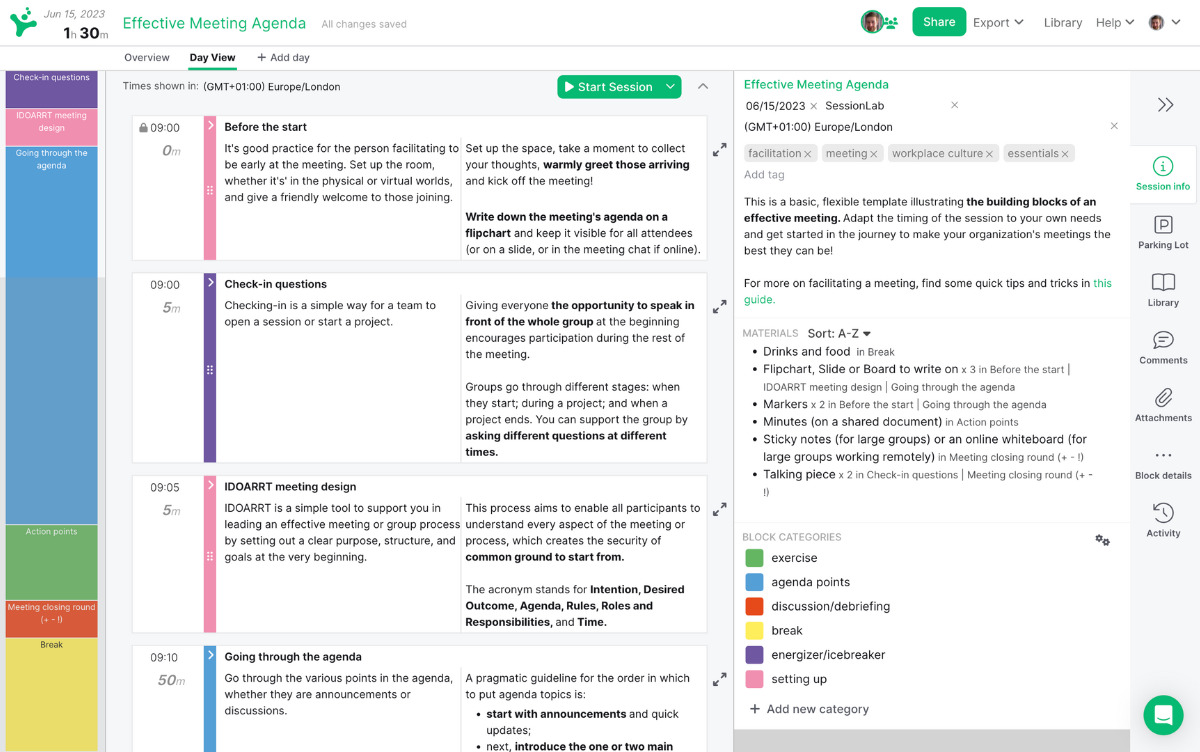
Tips for more effective problem solving
Problem-solving activities are only one part of the puzzle. While a great method can help unlock your team’s ability to solve problems, without a thoughtful approach and strong facilitation the solutions may not be fit for purpose.
Let’s take a look at some problem-solving tips you can apply to any process to help it be a success!
Clearly define the problem
Jumping straight to solutions can be tempting, though without first clearly articulating a problem, the solution might not be the right one. Many of the problem-solving activities below include sections where the problem is explored and clearly defined before moving on.
This is a vital part of the problem-solving process and taking the time to fully define an issue can save time and effort later. A clear definition helps identify irrelevant information and it also ensures that your team sets off on the right track.
Don’t jump to conclusions
It’s easy for groups to exhibit cognitive bias or have preconceived ideas about both problems and potential solutions. Be sure to back up any problem statements or potential solutions with facts, research, and adequate forethought.
The best techniques ask participants to be methodical and challenge preconceived notions. Make sure you give the group enough time and space to collect relevant information and consider the problem in a new way. By approaching the process with a clear, rational mindset, you’ll often find that better solutions are more forthcoming.
Try different approaches
Problems come in all shapes and sizes and so too should the methods you use to solve them. If you find that one approach isn’t yielding results and your team isn’t finding different solutions, try mixing it up. You’ll be surprised at how using a new creative activity can unblock your team and generate great solutions.
Don’t take it personally
Depending on the nature of your team or organizational problems, it’s easy for conversations to get heated. While it’s good for participants to be engaged in the discussions, ensure that emotions don’t run too high and that blame isn’t thrown around while finding solutions.
You’re all in it together, and even if your team or area is seeing problems, that isn’t necessarily a disparagement of you personally. Using facilitation skills to manage group dynamics is one effective method of helping conversations be more constructive.
Get the right people in the room
Your problem-solving method is often only as effective as the group using it. Getting the right people on the job and managing the number of people present is important too!
If the group is too small, you may not get enough different perspectives to effectively solve a problem. If the group is too large, you can go round and round during the ideation stages.
Creating the right group makeup is also important in ensuring you have the necessary expertise and skillset to both identify and follow up on potential solutions. Carefully consider who to include at each stage to help ensure your problem-solving method is followed and positioned for success.
Document everything
The best solutions can take refinement, iteration, and reflection to come out. Get into a habit of documenting your process in order to keep all the learnings from the session and to allow ideas to mature and develop. Many of the methods below involve the creation of documents or shared resources. Be sure to keep and share these so everyone can benefit from the work done!
Bring a facilitator
Facilitation is all about making group processes easier. With a subject as potentially emotive and important as problem-solving, having an impartial third party in the form of a facilitator can make all the difference in finding great solutions and keeping the process moving. Consider bringing a facilitator to your problem-solving session to get better results and generate meaningful solutions!
Develop your problem-solving skills
It takes time and practice to be an effective problem solver. While some roles or participants might more naturally gravitate towards problem-solving, it can take development and planning to help everyone create better solutions.
You might develop a training program, run a problem-solving workshop or simply ask your team to practice using the techniques below. Check out our post on problem-solving skills to see how you and your group can develop the right mental process and be more resilient to issues too!
Design a great agenda
Workshops are a great format for solving problems. With the right approach, you can focus a group and help them find the solutions to their own problems. But designing a process can be time-consuming and finding the right activities can be difficult.
Check out our workshop planning guide to level-up your agenda design and start running more effective workshops. Need inspiration? Check out templates designed by expert facilitators to help you kickstart your process!
In this section, we’ll look at in-depth problem-solving methods that provide a complete end-to-end process for developing effective solutions. These will help guide your team from the discovery and definition of a problem through to delivering the right solution.
If you’re looking for an all-encompassing method or problem-solving model, these processes are a great place to start. They’ll ask your team to challenge preconceived ideas and adopt a mindset for solving problems more effectively.
- Six Thinking Hats
- Lightning Decision Jam
- Problem Definition Process
- Discovery & Action Dialogue
Design Sprint 2.0
- Open Space Technology
1. Six Thinking Hats
Individual approaches to solving a problem can be very different based on what team or role an individual holds. It can be easy for existing biases or perspectives to find their way into the mix, or for internal politics to direct a conversation.
Six Thinking Hats is a classic method for identifying the problems that need to be solved and enables your team to consider them from different angles, whether that is by focusing on facts and data, creative solutions, or by considering why a particular solution might not work.
Like all problem-solving frameworks, Six Thinking Hats is effective at helping teams remove roadblocks from a conversation or discussion and come to terms with all the aspects necessary to solve complex problems.
2. Lightning Decision Jam
Featured courtesy of Jonathan Courtney of AJ&Smart Berlin, Lightning Decision Jam is one of those strategies that should be in every facilitation toolbox. Exploring problems and finding solutions is often creative in nature, though as with any creative process, there is the potential to lose focus and get lost.
Unstructured discussions might get you there in the end, but it’s much more effective to use a method that creates a clear process and team focus.
In Lightning Decision Jam, participants are invited to begin by writing challenges, concerns, or mistakes on post-its without discussing them before then being invited by the moderator to present them to the group.
From there, the team vote on which problems to solve and are guided through steps that will allow them to reframe those problems, create solutions and then decide what to execute on.
By deciding the problems that need to be solved as a team before moving on, this group process is great for ensuring the whole team is aligned and can take ownership over the next stages.
Lightning Decision Jam (LDJ) #action #decision making #problem solving #issue analysis #innovation #design #remote-friendly The problem with anything that requires creative thinking is that it’s easy to get lost—lose focus and fall into the trap of having useless, open-ended, unstructured discussions. Here’s the most effective solution I’ve found: Replace all open, unstructured discussion with a clear process. What to use this exercise for: Anything which requires a group of people to make decisions, solve problems or discuss challenges. It’s always good to frame an LDJ session with a broad topic, here are some examples: The conversion flow of our checkout Our internal design process How we organise events Keeping up with our competition Improving sales flow
3. Problem Definition Process
While problems can be complex, the problem-solving methods you use to identify and solve those problems can often be simple in design.
By taking the time to truly identify and define a problem before asking the group to reframe the challenge as an opportunity, this method is a great way to enable change.
Begin by identifying a focus question and exploring the ways in which it manifests before splitting into five teams who will each consider the problem using a different method: escape, reversal, exaggeration, distortion or wishful. Teams develop a problem objective and create ideas in line with their method before then feeding them back to the group.
This method is great for enabling in-depth discussions while also creating space for finding creative solutions too!
Problem Definition #problem solving #idea generation #creativity #online #remote-friendly A problem solving technique to define a problem, challenge or opportunity and to generate ideas.
4. The 5 Whys
Sometimes, a group needs to go further with their strategies and analyze the root cause at the heart of organizational issues. An RCA or root cause analysis is the process of identifying what is at the heart of business problems or recurring challenges.
The 5 Whys is a simple and effective method of helping a group go find the root cause of any problem or challenge and conduct analysis that will deliver results.
By beginning with the creation of a problem statement and going through five stages to refine it, The 5 Whys provides everything you need to truly discover the cause of an issue.
The 5 Whys #hyperisland #innovation This simple and powerful method is useful for getting to the core of a problem or challenge. As the title suggests, the group defines a problems, then asks the question “why” five times, often using the resulting explanation as a starting point for creative problem solving.
5. World Cafe
World Cafe is a simple but powerful facilitation technique to help bigger groups to focus their energy and attention on solving complex problems.
World Cafe enables this approach by creating a relaxed atmosphere where participants are able to self-organize and explore topics relevant and important to them which are themed around a central problem-solving purpose. Create the right atmosphere by modeling your space after a cafe and after guiding the group through the method, let them take the lead!
Making problem-solving a part of your organization’s culture in the long term can be a difficult undertaking. More approachable formats like World Cafe can be especially effective in bringing people unfamiliar with workshops into the fold.
World Cafe #hyperisland #innovation #issue analysis World Café is a simple yet powerful method, originated by Juanita Brown, for enabling meaningful conversations driven completely by participants and the topics that are relevant and important to them. Facilitators create a cafe-style space and provide simple guidelines. Participants then self-organize and explore a set of relevant topics or questions for conversation.
6. Discovery & Action Dialogue (DAD)
One of the best approaches is to create a safe space for a group to share and discover practices and behaviors that can help them find their own solutions.
With DAD, you can help a group choose which problems they wish to solve and which approaches they will take to do so. It’s great at helping remove resistance to change and can help get buy-in at every level too!
This process of enabling frontline ownership is great in ensuring follow-through and is one of the methods you will want in your toolbox as a facilitator.
Discovery & Action Dialogue (DAD) #idea generation #liberating structures #action #issue analysis #remote-friendly DADs make it easy for a group or community to discover practices and behaviors that enable some individuals (without access to special resources and facing the same constraints) to find better solutions than their peers to common problems. These are called positive deviant (PD) behaviors and practices. DADs make it possible for people in the group, unit, or community to discover by themselves these PD practices. DADs also create favorable conditions for stimulating participants’ creativity in spaces where they can feel safe to invent new and more effective practices. Resistance to change evaporates as participants are unleashed to choose freely which practices they will adopt or try and which problems they will tackle. DADs make it possible to achieve frontline ownership of solutions.
7. Design Sprint 2.0
Want to see how a team can solve big problems and move forward with prototyping and testing solutions in a few days? The Design Sprint 2.0 template from Jake Knapp, author of Sprint, is a complete agenda for a with proven results.
Developing the right agenda can involve difficult but necessary planning. Ensuring all the correct steps are followed can also be stressful or time-consuming depending on your level of experience.
Use this complete 4-day workshop template if you are finding there is no obvious solution to your challenge and want to focus your team around a specific problem that might require a shortcut to launching a minimum viable product or waiting for the organization-wide implementation of a solution.
8. Open space technology
Open space technology- developed by Harrison Owen – creates a space where large groups are invited to take ownership of their problem solving and lead individual sessions. Open space technology is a great format when you have a great deal of expertise and insight in the room and want to allow for different takes and approaches on a particular theme or problem you need to be solved.
Start by bringing your participants together to align around a central theme and focus their efforts. Explain the ground rules to help guide the problem-solving process and then invite members to identify any issue connecting to the central theme that they are interested in and are prepared to take responsibility for.
Once participants have decided on their approach to the core theme, they write their issue on a piece of paper, announce it to the group, pick a session time and place, and post the paper on the wall. As the wall fills up with sessions, the group is then invited to join the sessions that interest them the most and which they can contribute to, then you’re ready to begin!
Everyone joins the problem-solving group they’ve signed up to, record the discussion and if appropriate, findings can then be shared with the rest of the group afterward.
Open Space Technology #action plan #idea generation #problem solving #issue analysis #large group #online #remote-friendly Open Space is a methodology for large groups to create their agenda discerning important topics for discussion, suitable for conferences, community gatherings and whole system facilitation
Techniques to identify and analyze problems
Using a problem-solving method to help a team identify and analyze a problem can be a quick and effective addition to any workshop or meeting.
While further actions are always necessary, you can generate momentum and alignment easily, and these activities are a great place to get started.
We’ve put together this list of techniques to help you and your team with problem identification, analysis, and discussion that sets the foundation for developing effective solutions.
Let’s take a look!
- The Creativity Dice
- Fishbone Analysis
- Problem Tree
- SWOT Analysis
- Agreement-Certainty Matrix
- The Journalistic Six
- LEGO Challenge
- What, So What, Now What?
- Journalists
Individual and group perspectives are incredibly important, but what happens if people are set in their minds and need a change of perspective in order to approach a problem more effectively?
Flip It is a method we love because it is both simple to understand and run, and allows groups to understand how their perspectives and biases are formed.
Participants in Flip It are first invited to consider concerns, issues, or problems from a perspective of fear and write them on a flip chart. Then, the group is asked to consider those same issues from a perspective of hope and flip their understanding.
No problem and solution is free from existing bias and by changing perspectives with Flip It, you can then develop a problem solving model quickly and effectively.
Flip It! #gamestorming #problem solving #action Often, a change in a problem or situation comes simply from a change in our perspectives. Flip It! is a quick game designed to show players that perspectives are made, not born.
10. The Creativity Dice
One of the most useful problem solving skills you can teach your team is of approaching challenges with creativity, flexibility, and openness. Games like The Creativity Dice allow teams to overcome the potential hurdle of too much linear thinking and approach the process with a sense of fun and speed.
In The Creativity Dice, participants are organized around a topic and roll a dice to determine what they will work on for a period of 3 minutes at a time. They might roll a 3 and work on investigating factual information on the chosen topic. They might roll a 1 and work on identifying the specific goals, standards, or criteria for the session.
Encouraging rapid work and iteration while asking participants to be flexible are great skills to cultivate. Having a stage for idea incubation in this game is also important. Moments of pause can help ensure the ideas that are put forward are the most suitable.
The Creativity Dice #creativity #problem solving #thiagi #issue analysis Too much linear thinking is hazardous to creative problem solving. To be creative, you should approach the problem (or the opportunity) from different points of view. You should leave a thought hanging in mid-air and move to another. This skipping around prevents premature closure and lets your brain incubate one line of thought while you consciously pursue another.
11. Fishbone Analysis
Organizational or team challenges are rarely simple, and it’s important to remember that one problem can be an indication of something that goes deeper and may require further consideration to be solved.
Fishbone Analysis helps groups to dig deeper and understand the origins of a problem. It’s a great example of a root cause analysis method that is simple for everyone on a team to get their head around.
Participants in this activity are asked to annotate a diagram of a fish, first adding the problem or issue to be worked on at the head of a fish before then brainstorming the root causes of the problem and adding them as bones on the fish.
Using abstractions such as a diagram of a fish can really help a team break out of their regular thinking and develop a creative approach.
Fishbone Analysis #problem solving ##root cause analysis #decision making #online facilitation A process to help identify and understand the origins of problems, issues or observations.
12. Problem Tree
Encouraging visual thinking can be an essential part of many strategies. By simply reframing and clarifying problems, a group can move towards developing a problem solving model that works for them.
In Problem Tree, groups are asked to first brainstorm a list of problems – these can be design problems, team problems or larger business problems – and then organize them into a hierarchy. The hierarchy could be from most important to least important or abstract to practical, though the key thing with problem solving games that involve this aspect is that your group has some way of managing and sorting all the issues that are raised.
Once you have a list of problems that need to be solved and have organized them accordingly, you’re then well-positioned for the next problem solving steps.
Problem tree #define intentions #create #design #issue analysis A problem tree is a tool to clarify the hierarchy of problems addressed by the team within a design project; it represents high level problems or related sublevel problems.
13. SWOT Analysis
Chances are you’ve heard of the SWOT Analysis before. This problem-solving method focuses on identifying strengths, weaknesses, opportunities, and threats is a tried and tested method for both individuals and teams.
Start by creating a desired end state or outcome and bare this in mind – any process solving model is made more effective by knowing what you are moving towards. Create a quadrant made up of the four categories of a SWOT analysis and ask participants to generate ideas based on each of those quadrants.
Once you have those ideas assembled in their quadrants, cluster them together based on their affinity with other ideas. These clusters are then used to facilitate group conversations and move things forward.
SWOT analysis #gamestorming #problem solving #action #meeting facilitation The SWOT Analysis is a long-standing technique of looking at what we have, with respect to the desired end state, as well as what we could improve on. It gives us an opportunity to gauge approaching opportunities and dangers, and assess the seriousness of the conditions that affect our future. When we understand those conditions, we can influence what comes next.
14. Agreement-Certainty Matrix
Not every problem-solving approach is right for every challenge, and deciding on the right method for the challenge at hand is a key part of being an effective team.
The Agreement Certainty matrix helps teams align on the nature of the challenges facing them. By sorting problems from simple to chaotic, your team can understand what methods are suitable for each problem and what they can do to ensure effective results.
If you are already using Liberating Structures techniques as part of your problem-solving strategy, the Agreement-Certainty Matrix can be an invaluable addition to your process. We’ve found it particularly if you are having issues with recurring problems in your organization and want to go deeper in understanding the root cause.
Agreement-Certainty Matrix #issue analysis #liberating structures #problem solving You can help individuals or groups avoid the frequent mistake of trying to solve a problem with methods that are not adapted to the nature of their challenge. The combination of two questions makes it possible to easily sort challenges into four categories: simple, complicated, complex , and chaotic . A problem is simple when it can be solved reliably with practices that are easy to duplicate. It is complicated when experts are required to devise a sophisticated solution that will yield the desired results predictably. A problem is complex when there are several valid ways to proceed but outcomes are not predictable in detail. Chaotic is when the context is too turbulent to identify a path forward. A loose analogy may be used to describe these differences: simple is like following a recipe, complicated like sending a rocket to the moon, complex like raising a child, and chaotic is like the game “Pin the Tail on the Donkey.” The Liberating Structures Matching Matrix in Chapter 5 can be used as the first step to clarify the nature of a challenge and avoid the mismatches between problems and solutions that are frequently at the root of chronic, recurring problems.
Organizing and charting a team’s progress can be important in ensuring its success. SQUID (Sequential Question and Insight Diagram) is a great model that allows a team to effectively switch between giving questions and answers and develop the skills they need to stay on track throughout the process.
Begin with two different colored sticky notes – one for questions and one for answers – and with your central topic (the head of the squid) on the board. Ask the group to first come up with a series of questions connected to their best guess of how to approach the topic. Ask the group to come up with answers to those questions, fix them to the board and connect them with a line. After some discussion, go back to question mode by responding to the generated answers or other points on the board.
It’s rewarding to see a diagram grow throughout the exercise, and a completed SQUID can provide a visual resource for future effort and as an example for other teams.
SQUID #gamestorming #project planning #issue analysis #problem solving When exploring an information space, it’s important for a group to know where they are at any given time. By using SQUID, a group charts out the territory as they go and can navigate accordingly. SQUID stands for Sequential Question and Insight Diagram.
16. Speed Boat
To continue with our nautical theme, Speed Boat is a short and sweet activity that can help a team quickly identify what employees, clients or service users might have a problem with and analyze what might be standing in the way of achieving a solution.
Methods that allow for a group to make observations, have insights and obtain those eureka moments quickly are invaluable when trying to solve complex problems.
In Speed Boat, the approach is to first consider what anchors and challenges might be holding an organization (or boat) back. Bonus points if you are able to identify any sharks in the water and develop ideas that can also deal with competitors!
Speed Boat #gamestorming #problem solving #action Speedboat is a short and sweet way to identify what your employees or clients don’t like about your product/service or what’s standing in the way of a desired goal.
17. The Journalistic Six
Some of the most effective ways of solving problems is by encouraging teams to be more inclusive and diverse in their thinking.
Based on the six key questions journalism students are taught to answer in articles and news stories, The Journalistic Six helps create teams to see the whole picture. By using who, what, when, where, why, and how to facilitate the conversation and encourage creative thinking, your team can make sure that the problem identification and problem analysis stages of the are covered exhaustively and thoughtfully. Reporter’s notebook and dictaphone optional.
The Journalistic Six – Who What When Where Why How #idea generation #issue analysis #problem solving #online #creative thinking #remote-friendly A questioning method for generating, explaining, investigating ideas.
18. LEGO Challenge
Now for an activity that is a little out of the (toy) box. LEGO Serious Play is a facilitation methodology that can be used to improve creative thinking and problem-solving skills.
The LEGO Challenge includes giving each member of the team an assignment that is hidden from the rest of the group while they create a structure without speaking.
What the LEGO challenge brings to the table is a fun working example of working with stakeholders who might not be on the same page to solve problems. Also, it’s LEGO! Who doesn’t love LEGO!
LEGO Challenge #hyperisland #team A team-building activity in which groups must work together to build a structure out of LEGO, but each individual has a secret “assignment” which makes the collaborative process more challenging. It emphasizes group communication, leadership dynamics, conflict, cooperation, patience and problem solving strategy.
19. What, So What, Now What?
If not carefully managed, the problem identification and problem analysis stages of the problem-solving process can actually create more problems and misunderstandings.
The What, So What, Now What? problem-solving activity is designed to help collect insights and move forward while also eliminating the possibility of disagreement when it comes to identifying, clarifying, and analyzing organizational or work problems.
Facilitation is all about bringing groups together so that might work on a shared goal and the best problem-solving strategies ensure that teams are aligned in purpose, if not initially in opinion or insight.
Throughout the three steps of this game, you give everyone on a team to reflect on a problem by asking what happened, why it is important, and what actions should then be taken.
This can be a great activity for bringing our individual perceptions about a problem or challenge and contextualizing it in a larger group setting. This is one of the most important problem-solving skills you can bring to your organization.
W³ – What, So What, Now What? #issue analysis #innovation #liberating structures You can help groups reflect on a shared experience in a way that builds understanding and spurs coordinated action while avoiding unproductive conflict. It is possible for every voice to be heard while simultaneously sifting for insights and shaping new direction. Progressing in stages makes this practical—from collecting facts about What Happened to making sense of these facts with So What and finally to what actions logically follow with Now What . The shared progression eliminates most of the misunderstandings that otherwise fuel disagreements about what to do. Voila!
20. Journalists
Problem analysis can be one of the most important and decisive stages of all problem-solving tools. Sometimes, a team can become bogged down in the details and are unable to move forward.
Journalists is an activity that can avoid a group from getting stuck in the problem identification or problem analysis stages of the process.
In Journalists, the group is invited to draft the front page of a fictional newspaper and figure out what stories deserve to be on the cover and what headlines those stories will have. By reframing how your problems and challenges are approached, you can help a team move productively through the process and be better prepared for the steps to follow.
Journalists #vision #big picture #issue analysis #remote-friendly This is an exercise to use when the group gets stuck in details and struggles to see the big picture. Also good for defining a vision.
Problem-solving techniques for developing solutions
The success of any problem-solving process can be measured by the solutions it produces. After you’ve defined the issue, explored existing ideas, and ideated, it’s time to narrow down to the correct solution.
Use these problem-solving techniques when you want to help your team find consensus, compare possible solutions, and move towards taking action on a particular problem.
- Improved Solutions
- Four-Step Sketch
- 15% Solutions
- How-Now-Wow matrix
- Impact Effort Matrix
21. Mindspin
Brainstorming is part of the bread and butter of the problem-solving process and all problem-solving strategies benefit from getting ideas out and challenging a team to generate solutions quickly.
With Mindspin, participants are encouraged not only to generate ideas but to do so under time constraints and by slamming down cards and passing them on. By doing multiple rounds, your team can begin with a free generation of possible solutions before moving on to developing those solutions and encouraging further ideation.
This is one of our favorite problem-solving activities and can be great for keeping the energy up throughout the workshop. Remember the importance of helping people become engaged in the process – energizing problem-solving techniques like Mindspin can help ensure your team stays engaged and happy, even when the problems they’re coming together to solve are complex.
MindSpin #teampedia #idea generation #problem solving #action A fast and loud method to enhance brainstorming within a team. Since this activity has more than round ideas that are repetitive can be ruled out leaving more creative and innovative answers to the challenge.
22. Improved Solutions
After a team has successfully identified a problem and come up with a few solutions, it can be tempting to call the work of the problem-solving process complete. That said, the first solution is not necessarily the best, and by including a further review and reflection activity into your problem-solving model, you can ensure your group reaches the best possible result.
One of a number of problem-solving games from Thiagi Group, Improved Solutions helps you go the extra mile and develop suggested solutions with close consideration and peer review. By supporting the discussion of several problems at once and by shifting team roles throughout, this problem-solving technique is a dynamic way of finding the best solution.
Improved Solutions #creativity #thiagi #problem solving #action #team You can improve any solution by objectively reviewing its strengths and weaknesses and making suitable adjustments. In this creativity framegame, you improve the solutions to several problems. To maintain objective detachment, you deal with a different problem during each of six rounds and assume different roles (problem owner, consultant, basher, booster, enhancer, and evaluator) during each round. At the conclusion of the activity, each player ends up with two solutions to her problem.
23. Four Step Sketch
Creative thinking and visual ideation does not need to be confined to the opening stages of your problem-solving strategies. Exercises that include sketching and prototyping on paper can be effective at the solution finding and development stage of the process, and can be great for keeping a team engaged.
By going from simple notes to a crazy 8s round that involves rapidly sketching 8 variations on their ideas before then producing a final solution sketch, the group is able to iterate quickly and visually. Problem-solving techniques like Four-Step Sketch are great if you have a group of different thinkers and want to change things up from a more textual or discussion-based approach.
Four-Step Sketch #design sprint #innovation #idea generation #remote-friendly The four-step sketch is an exercise that helps people to create well-formed concepts through a structured process that includes: Review key information Start design work on paper, Consider multiple variations , Create a detailed solution . This exercise is preceded by a set of other activities allowing the group to clarify the challenge they want to solve. See how the Four Step Sketch exercise fits into a Design Sprint
24. 15% Solutions
Some problems are simpler than others and with the right problem-solving activities, you can empower people to take immediate actions that can help create organizational change.
Part of the liberating structures toolkit, 15% solutions is a problem-solving technique that focuses on finding and implementing solutions quickly. A process of iterating and making small changes quickly can help generate momentum and an appetite for solving complex problems.
Problem-solving strategies can live and die on whether people are onboard. Getting some quick wins is a great way of getting people behind the process.
It can be extremely empowering for a team to realize that problem-solving techniques can be deployed quickly and easily and delineate between things they can positively impact and those things they cannot change.
15% Solutions #action #liberating structures #remote-friendly You can reveal the actions, however small, that everyone can do immediately. At a minimum, these will create momentum, and that may make a BIG difference. 15% Solutions show that there is no reason to wait around, feel powerless, or fearful. They help people pick it up a level. They get individuals and the group to focus on what is within their discretion instead of what they cannot change. With a very simple question, you can flip the conversation to what can be done and find solutions to big problems that are often distributed widely in places not known in advance. Shifting a few grains of sand may trigger a landslide and change the whole landscape.
25. How-Now-Wow Matrix
The problem-solving process is often creative, as complex problems usually require a change of thinking and creative response in order to find the best solutions. While it’s common for the first stages to encourage creative thinking, groups can often gravitate to familiar solutions when it comes to the end of the process.
When selecting solutions, you don’t want to lose your creative energy! The How-Now-Wow Matrix from Gamestorming is a great problem-solving activity that enables a group to stay creative and think out of the box when it comes to selecting the right solution for a given problem.
Problem-solving techniques that encourage creative thinking and the ideation and selection of new solutions can be the most effective in organisational change. Give the How-Now-Wow Matrix a go, and not just for how pleasant it is to say out loud.
How-Now-Wow Matrix #gamestorming #idea generation #remote-friendly When people want to develop new ideas, they most often think out of the box in the brainstorming or divergent phase. However, when it comes to convergence, people often end up picking ideas that are most familiar to them. This is called a ‘creative paradox’ or a ‘creadox’. The How-Now-Wow matrix is an idea selection tool that breaks the creadox by forcing people to weigh each idea on 2 parameters.
26. Impact and Effort Matrix
All problem-solving techniques hope to not only find solutions to a given problem or challenge but to find the best solution. When it comes to finding a solution, groups are invited to put on their decision-making hats and really think about how a proposed idea would work in practice.
The Impact and Effort Matrix is one of the problem-solving techniques that fall into this camp, empowering participants to first generate ideas and then categorize them into a 2×2 matrix based on impact and effort.
Activities that invite critical thinking while remaining simple are invaluable. Use the Impact and Effort Matrix to move from ideation and towards evaluating potential solutions before then committing to them.
Impact and Effort Matrix #gamestorming #decision making #action #remote-friendly In this decision-making exercise, possible actions are mapped based on two factors: effort required to implement and potential impact. Categorizing ideas along these lines is a useful technique in decision making, as it obliges contributors to balance and evaluate suggested actions before committing to them.
27. Dotmocracy
If you’ve followed each of the problem-solving steps with your group successfully, you should move towards the end of your process with heaps of possible solutions developed with a specific problem in mind. But how do you help a group go from ideation to putting a solution into action?
Dotmocracy – or Dot Voting -is a tried and tested method of helping a team in the problem-solving process make decisions and put actions in place with a degree of oversight and consensus.
One of the problem-solving techniques that should be in every facilitator’s toolbox, Dot Voting is fast and effective and can help identify the most popular and best solutions and help bring a group to a decision effectively.
Dotmocracy #action #decision making #group prioritization #hyperisland #remote-friendly Dotmocracy is a simple method for group prioritization or decision-making. It is not an activity on its own, but a method to use in processes where prioritization or decision-making is the aim. The method supports a group to quickly see which options are most popular or relevant. The options or ideas are written on post-its and stuck up on a wall for the whole group to see. Each person votes for the options they think are the strongest, and that information is used to inform a decision.
All facilitators know that warm-ups and icebreakers are useful for any workshop or group process. Problem-solving workshops are no different.
Use these problem-solving techniques to warm up a group and prepare them for the rest of the process. Activating your group by tapping into some of the top problem-solving skills can be one of the best ways to see great outcomes from your session.
- Check-in/Check-out
- Doodling Together
- Show and Tell
- Constellations
- Draw a Tree
28. Check-in / Check-out
Solid processes are planned from beginning to end, and the best facilitators know that setting the tone and establishing a safe, open environment can be integral to a successful problem-solving process.
Check-in / Check-out is a great way to begin and/or bookend a problem-solving workshop. Checking in to a session emphasizes that everyone will be seen, heard, and expected to contribute.
If you are running a series of meetings, setting a consistent pattern of checking in and checking out can really help your team get into a groove. We recommend this opening-closing activity for small to medium-sized groups though it can work with large groups if they’re disciplined!
Check-in / Check-out #team #opening #closing #hyperisland #remote-friendly Either checking-in or checking-out is a simple way for a team to open or close a process, symbolically and in a collaborative way. Checking-in/out invites each member in a group to be present, seen and heard, and to express a reflection or a feeling. Checking-in emphasizes presence, focus and group commitment; checking-out emphasizes reflection and symbolic closure.
29. Doodling Together
Thinking creatively and not being afraid to make suggestions are important problem-solving skills for any group or team, and warming up by encouraging these behaviors is a great way to start.
Doodling Together is one of our favorite creative ice breaker games – it’s quick, effective, and fun and can make all following problem-solving steps easier by encouraging a group to collaborate visually. By passing cards and adding additional items as they go, the workshop group gets into a groove of co-creation and idea development that is crucial to finding solutions to problems.
Doodling Together #collaboration #creativity #teamwork #fun #team #visual methods #energiser #icebreaker #remote-friendly Create wild, weird and often funny postcards together & establish a group’s creative confidence.
30. Show and Tell
You might remember some version of Show and Tell from being a kid in school and it’s a great problem-solving activity to kick off a session.
Asking participants to prepare a little something before a workshop by bringing an object for show and tell can help them warm up before the session has even begun! Games that include a physical object can also help encourage early engagement before moving onto more big-picture thinking.
By asking your participants to tell stories about why they chose to bring a particular item to the group, you can help teams see things from new perspectives and see both differences and similarities in the way they approach a topic. Great groundwork for approaching a problem-solving process as a team!
Show and Tell #gamestorming #action #opening #meeting facilitation Show and Tell taps into the power of metaphors to reveal players’ underlying assumptions and associations around a topic The aim of the game is to get a deeper understanding of stakeholders’ perspectives on anything—a new project, an organizational restructuring, a shift in the company’s vision or team dynamic.
31. Constellations
Who doesn’t love stars? Constellations is a great warm-up activity for any workshop as it gets people up off their feet, energized, and ready to engage in new ways with established topics. It’s also great for showing existing beliefs, biases, and patterns that can come into play as part of your session.
Using warm-up games that help build trust and connection while also allowing for non-verbal responses can be great for easing people into the problem-solving process and encouraging engagement from everyone in the group. Constellations is great in large spaces that allow for movement and is definitely a practical exercise to allow the group to see patterns that are otherwise invisible.
Constellations #trust #connection #opening #coaching #patterns #system Individuals express their response to a statement or idea by standing closer or further from a central object. Used with teams to reveal system, hidden patterns, perspectives.
32. Draw a Tree
Problem-solving games that help raise group awareness through a central, unifying metaphor can be effective ways to warm-up a group in any problem-solving model.
Draw a Tree is a simple warm-up activity you can use in any group and which can provide a quick jolt of energy. Start by asking your participants to draw a tree in just 45 seconds – they can choose whether it will be abstract or realistic.
Once the timer is up, ask the group how many people included the roots of the tree and use this as a means to discuss how we can ignore important parts of any system simply because they are not visible.
All problem-solving strategies are made more effective by thinking of problems critically and by exposing things that may not normally come to light. Warm-up games like Draw a Tree are great in that they quickly demonstrate some key problem-solving skills in an accessible and effective way.
Draw a Tree #thiagi #opening #perspectives #remote-friendly With this game you can raise awarness about being more mindful, and aware of the environment we live in.
Each step of the problem-solving workshop benefits from an intelligent deployment of activities, games, and techniques. Bringing your session to an effective close helps ensure that solutions are followed through on and that you also celebrate what has been achieved.
Here are some problem-solving activities you can use to effectively close a workshop or meeting and ensure the great work you’ve done can continue afterward.
- One Breath Feedback
- Who What When Matrix
- Response Cards
How do I conclude a problem-solving process?
All good things must come to an end. With the bulk of the work done, it can be tempting to conclude your workshop swiftly and without a moment to debrief and align. This can be problematic in that it doesn’t allow your team to fully process the results or reflect on the process.
At the end of an effective session, your team will have gone through a process that, while productive, can be exhausting. It’s important to give your group a moment to take a breath, ensure that they are clear on future actions, and provide short feedback before leaving the space.
The primary purpose of any problem-solving method is to generate solutions and then implement them. Be sure to take the opportunity to ensure everyone is aligned and ready to effectively implement the solutions you produced in the workshop.
Remember that every process can be improved and by giving a short moment to collect feedback in the session, you can further refine your problem-solving methods and see further success in the future too.
33. One Breath Feedback
Maintaining attention and focus during the closing stages of a problem-solving workshop can be tricky and so being concise when giving feedback can be important. It’s easy to incur “death by feedback” should some team members go on for too long sharing their perspectives in a quick feedback round.
One Breath Feedback is a great closing activity for workshops. You give everyone an opportunity to provide feedback on what they’ve done but only in the space of a single breath. This keeps feedback short and to the point and means that everyone is encouraged to provide the most important piece of feedback to them.
One breath feedback #closing #feedback #action This is a feedback round in just one breath that excels in maintaining attention: each participants is able to speak during just one breath … for most people that’s around 20 to 25 seconds … unless of course you’ve been a deep sea diver in which case you’ll be able to do it for longer.
34. Who What When Matrix
Matrices feature as part of many effective problem-solving strategies and with good reason. They are easily recognizable, simple to use, and generate results.
The Who What When Matrix is a great tool to use when closing your problem-solving session by attributing a who, what and when to the actions and solutions you have decided upon. The resulting matrix is a simple, easy-to-follow way of ensuring your team can move forward.
Great solutions can’t be enacted without action and ownership. Your problem-solving process should include a stage for allocating tasks to individuals or teams and creating a realistic timeframe for those solutions to be implemented or checked out. Use this method to keep the solution implementation process clear and simple for all involved.
Who/What/When Matrix #gamestorming #action #project planning With Who/What/When matrix, you can connect people with clear actions they have defined and have committed to.
35. Response cards
Group discussion can comprise the bulk of most problem-solving activities and by the end of the process, you might find that your team is talked out!
Providing a means for your team to give feedback with short written notes can ensure everyone is head and can contribute without the need to stand up and talk. Depending on the needs of the group, giving an alternative can help ensure everyone can contribute to your problem-solving model in the way that makes the most sense for them.
Response Cards is a great way to close a workshop if you are looking for a gentle warm-down and want to get some swift discussion around some of the feedback that is raised.
Response Cards #debriefing #closing #structured sharing #questions and answers #thiagi #action It can be hard to involve everyone during a closing of a session. Some might stay in the background or get unheard because of louder participants. However, with the use of Response Cards, everyone will be involved in providing feedback or clarify questions at the end of a session.
Save time and effort discovering the right solutions
A structured problem solving process is a surefire way of solving tough problems, discovering creative solutions and driving organizational change. But how can you design for successful outcomes?
With SessionLab, it’s easy to design engaging workshops that deliver results. Drag, drop and reorder blocks to build your agenda. When you make changes or update your agenda, your session timing adjusts automatically , saving you time on manual adjustments.
Collaborating with stakeholders or clients? Share your agenda with a single click and collaborate in real-time. No more sending documents back and forth over email.
Explore how to use SessionLab to design effective problem solving workshops or watch this five minute video to see the planner in action!
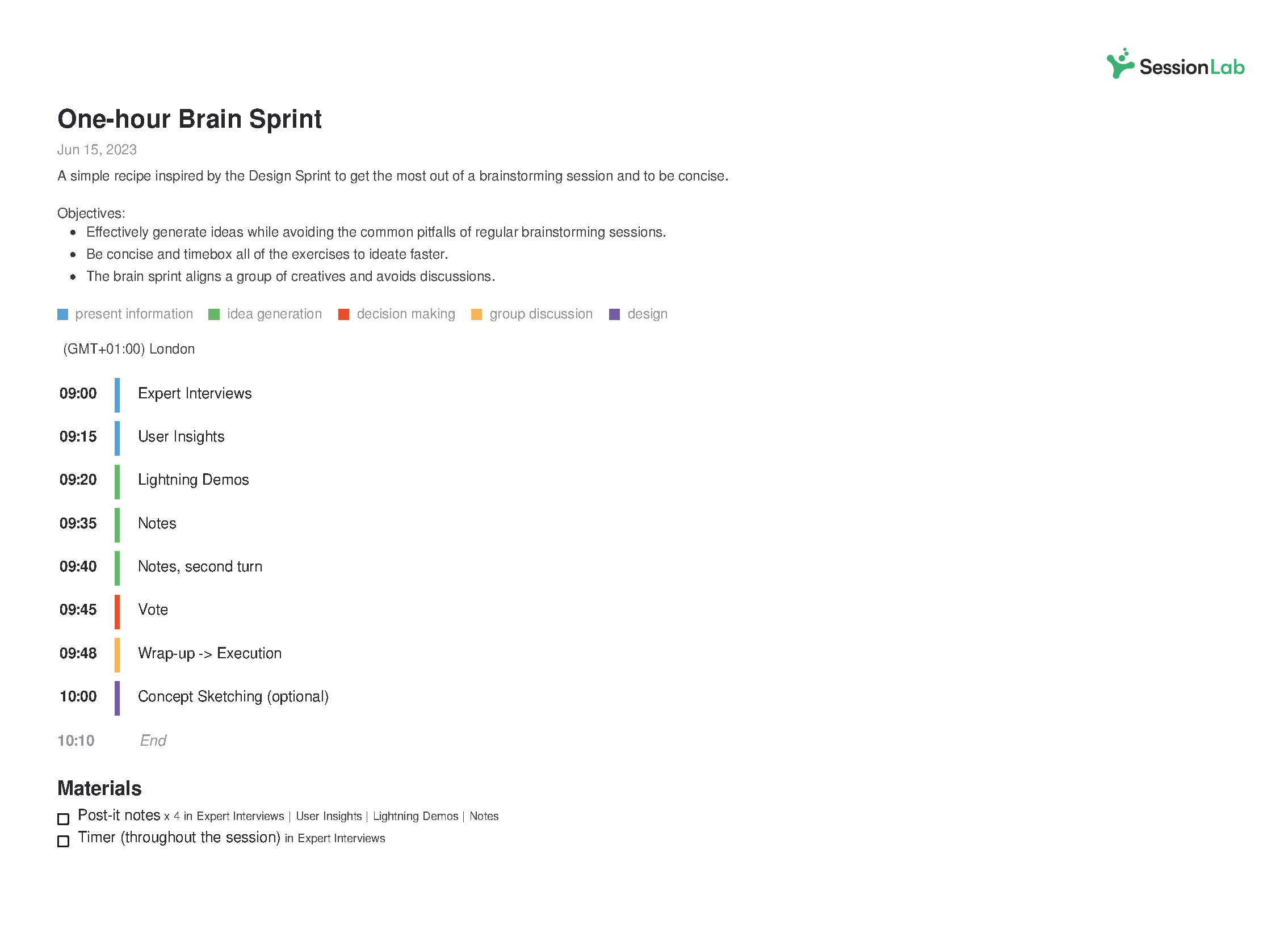
Over to you
The problem-solving process can often be as complicated and multifaceted as the problems they are set-up to solve. With the right problem-solving techniques and a mix of creative exercises designed to guide discussion and generate purposeful ideas, we hope we’ve given you the tools to find the best solutions as simply and easily as possible.
Is there a problem-solving technique that you are missing here? Do you have a favorite activity or method you use when facilitating? Let us know in the comments below, we’d love to hear from you!
thank you very much for these excellent techniques
Certainly wonderful article, very detailed. Shared!
Leave a Comment Cancel reply
Your email address will not be published. Required fields are marked *
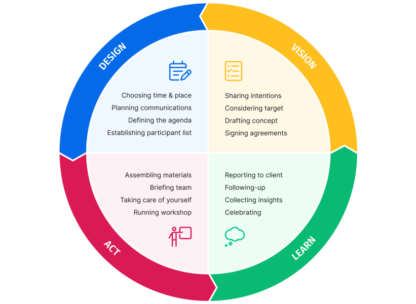
Going from a mere idea to a workshop that delivers results for your clients can feel like a daunting task. In this piece, we will shine a light on all the work behind the scenes and help you learn how to plan a workshop from start to finish. On a good day, facilitation can feel like effortless magic, but that is mostly the result of backstage work, foresight, and a lot of careful planning. Read on to learn a step-by-step approach to breaking the process of planning a workshop into small, manageable chunks. The flow starts with the first meeting with a client to define the purposes of a workshop.…
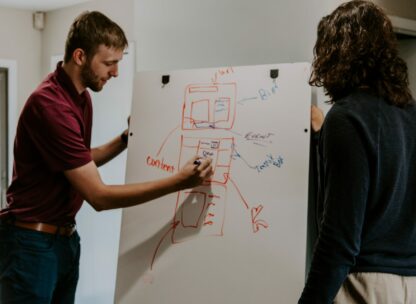
How does learning work? A clever 9-year-old once told me: “I know I am learning something new when I am surprised.” The science of adult learning tells us that, in order to learn new skills (which, unsurprisingly, is harder for adults to do than kids) grown-ups need to first get into a specific headspace. In a business, this approach is often employed in a training session where employees learn new skills or work on professional development. But how do you ensure your training is effective? In this guide, we'll explore how to create an effective training session plan and run engaging training sessions. As team leader, project manager, or consultant,…
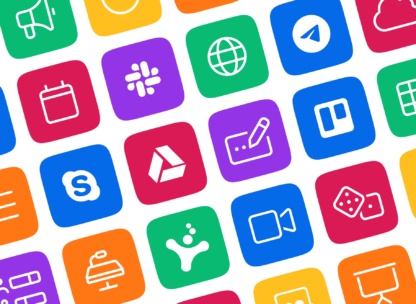
Effective online tools are a necessity for smooth and engaging virtual workshops and meetings. But how do you choose the right ones? Do you sometimes feel that the good old pen and paper or MS Office toolkit and email leaves you struggling to stay on top of managing and delivering your workshop? Fortunately, there are plenty of online tools to make your life easier when you need to facilitate a meeting and lead workshops. In this post, we’ll share our favorite online tools you can use to make your job as a facilitator easier. In fact, there are plenty of free online workshop tools and meeting facilitation software you can…
Design your next workshop with SessionLab
Join the 150,000 facilitators using SessionLab
Sign up for free
SoftwareDominos

Complex Problems: What Does the Nature of the Problem Tell Us About Its Solution
1. Overview
In The 7 Timeless Steps to Guide You Through Complex Problem Solving , we discussed a generic approach that could be systematically applied to solving complex problems. Since not all problems are complex, and many gradations of complexity exist, it is probably a good idea to start by defining what complex problem-solving involves and what categories of problems are most suitable to tackle using that approach. For this reason, understanding complex problems made the top position on the list.
Practically all living organisms deal with complex problems, from single-celled amebas to societies of Homo sapiens , and surprisingly, the solution-creation process can be very similar, at least on the conceptual level. This article will elaborate on this point further, articulating the terminology and ideas often associated with how complex adaptive systems solve complex problems. More specifically, we will answer the following questions:
This article is part of a series on complex problem-solving. The list below will guide you through the different subtopics.
Complex Problem-Solving Guide in 7 Steps
The 7 Timeless Steps to Guide You Through Complex Problem Solving
The Nature of Complex Problems
What Does the Nature of the Problem Tell Us About Its Solution
Gaussian Distributions vs Power Laws
Your Ultimate Guide to Making Sense of Natural and Social Phenomena
Complex Problem-Solving in Groups
An Exploratory Overview of ProbleSolving Processes in Groups
The Power of Critical Thinking
An Essential Guide for Personal and Professional Development
Group-Decision Making
6 Modes That Tell Us How Teams Decide
3. An Intuitive Definition of Complex Problems
We all intuitively grasp the characteristics of challenging problems, at least at their fundamental levels. For instance, we can promptly recognize that fixing a faulty washing machine is relatively simple. First, we need basic technical skills to identify the faulty part. Next, we would read the code on the back, order a spare part, and finally replace it.
In simple problems, there is no uncertainty around the root cause or the solution.
On the other hand, deciding whether or not to accept a job offer is anything but simple. Firstly, you will never have sufficient information to make an optimal decision . Secondly, you cannot predict the consequences of such a decision. Finally, whichever choice you make will change your worldview, rendering any forecasts you have made of the future almost instantly obsolete.
The following characteristics distinguish complex problems.
So, what are complex problems?
Complex problems — an intuitive guide.
Non-triviality
Complex problems generally admit non-trivial solutions. In addition to strong field expertise and solid analytical skills, they require a high cognitive load to formulate.
Uncertainty
Solutions to complex problems cannot be guaranteed as the behaviour of the system to which the solution is applied is always unpredictable.
Diagnosing complex problems is especially challenging because consensus on facts, root causes, and solutions can be difficult to obtain, especially in large groups.
3. Challenges of Working With Complex Problems
Experts like Nassim Taleb, Gerd Gigerenzer, and Daniel Kahnemann insist that solving complex problems is relatively easy once we understand which tools to apply. In their view, failures come from applying engineering methods like optimization rather than intuition , heuristics, biases, imitation, and many other techniques refined over millennia of evolution and accumulated wisdom.
4. Complex Problems in the Literature
Experts have extensively researched topics associated with intuition, cognitive psychology , risk management , organisational behaviour , and decision-making under uncertainty. This has left us with a rich body of knowledge popularized by best-selling authors such as Daniel Kahneman and Nassim Taleb, which will be reviewed next.
4.1 Fooled by Randomness (Taleb, 2001)
Fooled by Randomness is one of Taleb’s best-selling books , and its central story revolves around the hidden role of chance in our lives. In Taleb’s view, we grossly and routinely overestimate our capabilities to forecast future events ( the turkey problem ) and cope with that failure through mechanisms like the narrative fallacy and our ability to reconstruct past events based on new information.

Key takeaways from Fooled By Randomness
- In social , financial, economic, and political systems, Gaussian distributions mislead at best by providing a comforting but shifting ground for modelling events.
- Power laws like Pareto’s provide more suitable models for examining complex systems .
- Time-tested heuristics, formulated through a long knowledge acquisition and refinement period, are more valuable for decision-making under uncertainty than optimisation techniques, which require a well-behaved underlying model (such as the Gaussian).
4.2 Thinking, Fast and Slow (Kahneman, 2011)
Thinking, Fast and Slow is a best-selling book by Daniel Kahneman popularizing his work in cognitive psychology about the mechanism and efficiency of human judgment and decision-making under conditions of uncertainty. His original idea revolves around modelling the human mind as two systems, which he refers to as System 1 and System 2.

Key takeaways from Thinking, Fast and Slow
- Systems 1 and 2 perfectly cover our decision-making needs for simple and complex problems.
- System 1 is fast and inexpensive, allowing us to make critical decisions with imperfect or unreliable data .
- System 1 relies on heuristics and biases to compensate for unreliable information and processing time.
- System 2 is slow and expensive but more accurate, allowing us to make decisions requiring a high cognitive load and processing larger amounts of information.
4.3 Process Consultation (Schein, 1969)
Professor Edgar Schein is a leading authority in organizational behaviour, culture , and psychology. His short but insightful book Process Consultation: Its Role in Organizational Development dedicates a full chapter to group problem-solving and decision-making. Schein explores how leaders and their groups tackle complex problems in this chapter.

Key takeaways from Process Consultation
- Group problem-solving presents challenges and dynamics that differ from those of individuals and is a subject in its own right.
- In both cases, events that cause tension and anxiety trigger a solution-finding process that culminates in applying changes to the environment or the individual or group’s interactions with it.
- However, problem formulation, solution creation and implementation differ significantly between the two cases.
5. The Information Sufficiency Problem
5.1 how much data is enough.
During the Newtonian age, physicists believed that once the initial conditions of a physical system were precisely determined, its future evolution could be predicted with arbitrary precision. For example, the laws of dynamics allow us to calculate the infinite trajectory of a point mass given its initial position and velocity.
What happens when the system consists of innumerable particles, each with a different initial speed and position? For practical reasons, we substitute the individual particles with a unit of volume where its macro properties can be calculated by averaging over its constituent particles. For example, instead of registering the speed and position of every molecule in a gas container, we substitute those numbers with temperature and pressure calculated on a coarse-grained subvolume. This coarse-graining allows us to explore the system’s physical properties without drowning in data.
5.2 The Rise of Statistical Mechanics and Probabilistic Models
The coarse-graining method and the impracticality of precise calculations on the molecular level gave rise to statistical mechanics , which Boltzmann and others pioneered. Under statistical mechanics, physical systems are governed by the laws of thermodynamics. The second law of thermodynamics is the most famous, dictating that a system’s entropy (or disorder) must always increase.
The practical advantages of using coarse-graining came at a cost, as a probabilistic model replaced the classic view of deterministic evolution. In this new paradigm, a physical system is predisposed to evolve into one of numerous states. We can only predict the probability that it will be in a given future state, but we can never be sure which one.
But all is not lost. Even with the probabilistic model, we can still calculate a system’s future state and create contingency plans for each scenario. We might even be able to influence the outcome by applying pressure on known system levers. This assumption forms the basis of Strategic Choice Theory .
Strategic choice theory, in the realm of organizational theory, emphasizes the influence of leaders and decision-makers on an organization’s direction. It contrasts with earlier views that saw organizations solely responding to external forces.

Managing Probabilistic Systems
In probabilistic models, we assume that the system’s future states are well-defined and their probabilities are calculable. Given this information, adequate planning and optimization processes can be applied to maximize a specific utility function.
5.3 Probabilistic Models Cannot Account for Innovation
Any physical, chemical, or biological system that shows innovation cannot, by definition, be analyzed using probability models, as the latter assumes all future states are static and knowable in advance. Also, the probabilities for reaching any of those states are either fixed or vary according to well-specified rules.
Therefore, probabilistic models are not good enough to predict the future behaviour of human systems. This also spells trouble for Strategic Choice Theory, which relies on simple causal relationships between leaders’ interventions and desired consequences to achieve progress or resolve conflicts.
If a system can produce novel behaviour, it is unpredictable and, therefore, hard to manage. Ecologies of living organisms can only be understood through complexity theory and managed by principles that consider that.
Complex systems presenting complex problems will never offer sufficient information, and managers must make choices under uncertain conditions.
Even if we consider every atom (or elementary particle) in the universe , we still would not be able to predict the rich diversity of phenomena (including biodiversity on Earth) that we currently observe. Quantum mechanics and symmetry breaking ensure enough randomness is injected into the system to produce rich but unpredictable results.
The same applies when we try to understand the source of consciousness in our brains. Would it help to incorporate every neuron and synapse in a gigantic mathematical model? Even if this becomes practical someday, experts seem to believe that emerging consciousness in the inanimate matter is far away.
In summary, there seems to be a hard limit on how much useful information, in principle and practice, can be gleaned by observing a complex system .
6. Problem Classification
6.1 maximizing utility functions.
Problems can present themselves in many different ways. However, we are interested in those characterized by a utility function.
A utility function is a concept primarily used in economics, decision theory, and game theory to represent an individual’s preferences over different outcomes or states of the world. It assigns a numerical (or utility) value to each possible outcome or combination, reflecting the individual’s subjective satisfaction or preference associated with those outcomes.
How Are Utility Functions Used?

Here are some key points about utility functions:
Using utility functions, people can compare complex options involving chance or risk and make decisions based on their preferences and risk tolerance.
6.2 Ordered, Chaotic, Complex, and Random Systems
Imagine that you have the following problem. You are required to configure an air conditioning system for a data centre. The system is composed of two machines: a cooling engine and a computer connected to it. The computer has temperature and humidity sensors and various switches and dials that allow operators to set control parameters such as maximum temperature or humidity.

The engineer setting up the system must configure it to minimize power consumption while keeping the room at a given temperature and humidity level. The only issue is that the system does not have an operations guide, and the engineer has to figure out how to set it up using trial and error.
Four scenarios are possible: Ordered, Random, Complex, and Chaotic.
Ordered Systems
- Changes in the switches or dials produce a clear response in the cooling machine.
- Although some settings may impact others, the engineer can, through trial and error, understand the relationship between the controls and the outcomes.
- Ordered systems have direct causal relationships and hard constraints between their components.
- Problems in an ordered system can be resolved through the relationships between control parameters and the utility function.
- Computers, watches, and washing machines are examples of such systems.
Random Systems
- Changes in the switches or dials produce different responses every time. There seems to be no correlation between the settings and the outcomes.
- Random systems have no causal links and no constraints between their components.
- Random systems present problems that cannot be resolved; they are, by definition, unmanageable.
- A reward system based on rolling two dice is a random system.
Chaotic Systems
- Small changes in the switches or dials produce wild responses. Although the system appears random and unpredictable, it shows regular behavioural patterns over the long term.
- Chaotic systems have causal links and hard constraints between their components in addition to non-linear dynamics.
- Chaotic systems are also challenging to manage. However, causes can be linked to effects, and regularities can be leveraged.
- The weather, a turbulent water flow , and three bodies rotating around each other in gravitational fields are examples of chaotic systems.
Complex Systems
- Changes in the switches or dials produce different responses every time. Slight correlations can be measured between the settings and the outcomes.
- Complex systems have indirect causal links and loose constraints between their components.
- Complex systems come in two varieties: adaptive and non-adaptive.
- An example of a non-adaptive complex system is the Brusselator . An example of a complex adaptive system is a microbiome.
- Complex systems present problems that can be resolved through heuristics, safe-to-fail experimentation, and managing in the present rather than towards a desirable future state.
7. Small Worlds, Optimization, and Unknown Unknowns
7.1 leonard j. savage’s “small world”.
Leonard Jimmie Savage (1917-1971) was an American statistician and economist who significantly contributed to statistics , decision theory, and econometrics.
“Savage’s Small World” refers to a thought experiment proposed by the statistician and economist Leonard Jimmie Savage. This concept is often cited in discussions about subjective probability and decision theory.
Decision-Making in a Simple World
L. J. Savage’s “Small World”
- In Savage’s Small World, imagine a small society where everyone knows each other’s preferences, capabilities, and the outcomes of their decisions.
- Within this world, individuals can communicate freely and exchange information about their beliefs, desires, and experiences.
- In such a setting, decision-making becomes more transparent and informed, as individuals can access comprehensive knowledge about each other’s perspectives and choices.
The significance of Savage’s Small World lies in its implications for decision theory. It illustrates an idealized scenario where uncertainty is minimized, and individuals have perfect knowledge about the consequences of their actions. In reality, however, decision-makers often face uncertainty and incomplete information, prompting probabilistic reasoning and subjective judgment.
By contrasting Savage’s Small World with the complexities of real-world decision-making, Savage highlighted the importance of subjective probability for navigating uncertainty and making rational choices. Subjective probability allows individuals to express their beliefs and uncertainty in a formal framework, facilitating reasoned decision-making even when complete information is lacking.
7.2 Optimization Techniques
Optimization techniques can be effectively applied in a Small World scenario where all outcomes and probabilities can be precisely computed beforehand. This is because decision-makers have complete knowledge of the system, allowing them to accurately assess the consequences of their actions and choose the optimal course of action based on predetermined criteria.
In contrast, in the real world, uncertainty, complexity, and incomplete information often make it challenging to compute outcomes and probabilities beforehand precisely. As a result, optimization techniques may not be as effective, as they rely on accurate information to generate optimal solutions. Decision-makers must contend with uncertainty and imperfect knowledge, which can lead to suboptimal outcomes even when applying optimisation techniques.
One example where optimization relies on known outcomes and their probabilities is in the context of inventory management.
In inventory management, a retailer determines the optimal inventory level for each product to minimize costs while ensuring that customer demand is met. In this case, the utility function represents the retailer’s objective, which typically involves minimizing inventory holding costs and stockouts.
Optimisation Process in Inventory Management
Here’s a rigorous breakdown of the optimization process:
- 1 – Identify outcomes and probabilities :
- 2- Define the utility function :
- 3- Formulate the optimization problem :
- 4- Solve the optimization problem :
By incorporating known outcomes (demand scenarios) and their probabilities into the utility function and using optimization techniques, retailers can manage their inventory effectively, minimizing costs while ensuring customer satisfaction and maintaining adequate product availability.
7.3 Optimisation in Complex Worlds
Optimization techniques may encounter challenges in complex situations, particularly those governed by power laws (see discussion on Gaussian Distributions vs Power Laws: Your Ultimate Guide to Making Sense of Natural and Social Phenomena and their impact on our understanding of complex natural phenomena), due to several reasons:
Practical challenges of estimating model parameters in power laws versus Gaussians
Comparing the practical difficulties of estimating model parameters such as mean and variance in power laws versus Gaussians:
- Mean estimation
- Variance estimation
7.4 Unknown Unknowns
The concept of “ unknown unknowns” refers to phenomena or factors that are not only unknown but also unknowable.
In Savage’s “Small World,” which represents an idealized scenario where decision-makers have perfect knowledge of outcomes and their probabilities, the concept of “unknown unknowns” highlights the limitations of this idealization. In a Small World, nothing new ever happens, and there can be no “Unknown Unknowns”.
In contrast, complex adaptive systems constantly display emergent behaviour; patterns that could not have been anticipated. A leader managing such a system cannot list all possible outcomes, let alone assign each probability.
8. Subjectivity and the Role of the Observer
In decision-making, a leader’s subjective experience contrasts with their role as an objective observer. For example, in systems thinking and cybernetics, the leader must diagnose problems based on data and evidence and formulate a logical and rational solution.
A leader working on complex problems in a social group is an integral part of the system. As we have seen in previous sections, the leader is unable to gather sufficient information about the system in principle and practice, and whatever data they gather will be coloured by their subjective experience
Systems Thinking in a Nuntshell
Systems Thinking is a holistic approach to understanding complex systems by examining their interconnectedness, interdependencies, and dynamics. It views the leader’s role in an organization as crucial for effective strategy formulation and decision-making by emphasizing the following principles:
- Holistic Perspective :
- Interconnectedness :
- Feedback Loops :
Systems Thinking addresses the paradox of the leader being part of the system being managed by acknowledging the leader’s dual role as both a participant within the system and an external observer guiding its direction. Several key principles help resolve this conflict:
Exploring problem-solving reveals that not all problems are created equal. Distinguishing between simple and complex problems reveals the underlying nature of the systems they belong to. Simple problems are typically found within ordered systems, whereas complex problems are inherent to complex systems. These systems extend beyond biological ecologies to encompass social groups and organizations, where intricate interactions and emergent behaviours define their complexity.
One defining characteristic of complex systems is their governance by power laws, rendering traditional optimization techniques ineffective. Unlike in ordered systems, where linear solutions may suffice, complex systems defy such neat categorizations. Applying optimization strategies proves futile due to the non-linear, unpredictable dynamics governed by power laws.
Heuristics emerge as promising alternatives to optimization in navigating the labyrinth of complex systems. These intuitive, rule-of-thumb approaches allow for adaptive decision-making, acknowledging complex systems’ inherent uncertainty and non-linearity.
10. References
- Thinking, Fast and Slow — by Daniel Kahneman , 2011
- Fooled by Randomness — by Nassim Nicholas Taleb , 2001
- Process Consultation — by Edgar Schein , 1969
- The Quark and the Jaguar — by Murray Gell-Mann , 1994
Leave a Reply Cancel reply
Your email address will not be published. Required fields are marked *
Save my name, email, and website in this browser for the next time I comment.
CONCEPTUAL ANALYSIS article
Complex problem solving: what it is and what it is not.
- 1 Department of Psychology, University of Bamberg, Bamberg, Germany
- 2 Department of Psychology, Heidelberg University, Heidelberg, Germany
Computer-simulated scenarios have been part of psychological research on problem solving for more than 40 years. The shift in emphasis from simple toy problems to complex, more real-life oriented problems has been accompanied by discussions about the best ways to assess the process of solving complex problems. Psychometric issues such as reliable assessments and addressing correlations with other instruments have been in the foreground of these discussions and have left the content validity of complex problem solving in the background. In this paper, we return the focus to content issues and address the important features that define complex problems.
Succeeding in the 21st century requires many competencies, including creativity, life-long learning, and collaboration skills (e.g., National Research Council, 2011 ; Griffin and Care, 2015 ), to name only a few. One competence that seems to be of central importance is the ability to solve complex problems ( Mainzer, 2009 ). Mainzer quotes the Nobel prize winner Simon (1957) who wrote as early as 1957:
The capacity of the human mind for formulating and solving complex problems is very small compared with the size of the problem whose solution is required for objectively rational behavior in the real world or even for a reasonable approximation to such objective rationality. (p. 198)
The shift from well-defined to ill-defined problems came about as a result of a disillusion with the “general problem solver” ( Newell et al., 1959 ): The general problem solver was a computer software intended to solve all kind of problems that can be expressed through well-formed formulas. However, it soon became clear that this procedure was in fact a “special problem solver” that could only solve well-defined problems in a closed space. But real-world problems feature open boundaries and have no well-determined solution. In fact, the world is full of wicked problems and clumsy solutions ( Verweij and Thompson, 2006 ). As a result, solving well-defined problems and solving ill-defined problems requires different cognitive processes ( Schraw et al., 1995 ; but see Funke, 2010 ).
Well-defined problems have a clear set of means for reaching a precisely described goal state. For example: in a match-stick arithmetic problem, a person receives a false arithmetic expression constructed out of matchsticks (e.g., IV = III + III). According to the instructions, moving one of the matchsticks will make the equations true. Here, both the problem (find the appropriate stick to move) and the goal state (true arithmetic expression; solution is: VI = III + III) are defined clearly.
Ill-defined problems have no clear problem definition, their goal state is not defined clearly, and the means of moving towards the (diffusely described) goal state are not clear. For example: The goal state for solving the political conflict in the near-east conflict between Israel and Palestine is not clearly defined (living in peaceful harmony with each other?) and even if the conflict parties would agree on a two-state solution, this goal again leaves many issues unresolved. This type of problem is called a “complex problem” and is of central importance to this paper. All psychological processes that occur within individual persons and deal with the handling of such ill-defined complex problems will be subsumed under the umbrella term “complex problem solving” (CPS).
Systematic research on CPS started in the 1970s with observations of the behavior of participants who were confronted with computer simulated microworlds. For example, in one of those microworlds participants assumed the role of executives who were tasked to manage a company over a certain period of time (see Brehmer and Dörner, 1993 , for a discussion of this methodology). Today, CPS is an established concept and has even influenced large-scale assessments such as PISA (“Programme for International Student Assessment”), organized by the Organization for Economic Cooperation and Development ( OECD, 2014 ). According to the World Economic Forum, CPS is one of the most important competencies required in the future ( World Economic Forum, 2015 ). Numerous articles on the subject have been published in recent years, documenting the increasing research activity relating to this field. In the following collection of papers we list only those published in 2010 and later: theoretical papers ( Blech and Funke, 2010 ; Funke, 2010 ; Knauff and Wolf, 2010 ; Leutner et al., 2012 ; Selten et al., 2012 ; Wüstenberg et al., 2012 ; Greiff et al., 2013b ; Fischer and Neubert, 2015 ; Schoppek and Fischer, 2015 ), papers about measurement issues ( Danner et al., 2011a ; Greiff et al., 2012 , 2015a ; Alison et al., 2013 ; Gobert et al., 2015 ; Greiff and Fischer, 2013 ; Herde et al., 2016 ; Stadler et al., 2016 ), papers about applications ( Fischer and Neubert, 2015 ; Ederer et al., 2016 ; Tremblay et al., 2017 ), papers about differential effects ( Barth and Funke, 2010 ; Danner et al., 2011b ; Beckmann and Goode, 2014 ; Greiff and Neubert, 2014 ; Scherer et al., 2015 ; Meißner et al., 2016 ; Wüstenberg et al., 2016 ), one paper about developmental effects ( Frischkorn et al., 2014 ), one paper with a neuroscience background ( Osman, 2012 ) 1 , papers about cultural differences ( Güss and Dörner, 2011 ; Sonnleitner et al., 2014 ; Güss et al., 2015 ), papers about validity issues ( Goode and Beckmann, 2010 ; Greiff et al., 2013c ; Schweizer et al., 2013 ; Mainert et al., 2015 ; Funke et al., 2017 ; Greiff et al., 2017 , 2015b ; Kretzschmar et al., 2016 ; Kretzschmar, 2017 ), review papers and meta-analyses ( Osman, 2010 ; Stadler et al., 2015 ), and finally books ( Qudrat-Ullah, 2015 ; Csapó and Funke, 2017b ) and book chapters ( Funke, 2012 ; Hotaling et al., 2015 ; Funke and Greiff, 2017 ; Greiff and Funke, 2017 ; Csapó and Funke, 2017a ; Fischer et al., 2017 ; Molnàr et al., 2017 ; Tobinski and Fritz, 2017 ; Viehrig et al., 2017 ). In addition, a new “Journal of Dynamic Decision Making” (JDDM) has been launched ( Fischer et al., 2015 , 2016 ) to give the field an open-access outlet for research and discussion.
This paper aims to clarify aspects of validity: what should be meant by the term CPS and what not? This clarification seems necessary because misunderstandings in recent publications provide – from our point of view – a potentially misleading picture of the construct. We start this article with a historical review before attempting to systematize different positions. We conclude with a working definition.
Historical Review
The concept behind CPS goes back to the German phrase “komplexes Problemlösen” (CPS; the term “komplexes Problemlösen” was used as a book title by Funke, 1986 ). The concept was introduced in Germany by Dörner and colleagues in the mid-1970s (see Dörner et al., 1975 ; Dörner, 1975 ) for the first time. The German phrase was later translated to CPS in the titles of two edited volumes by Sternberg and Frensch (1991) and Frensch and Funke (1995a) that collected papers from different research traditions. Even though it looks as though the term was coined in the 1970s, Edwards (1962) used the term “dynamic decision making” to describe decisions that come in a sequence. He compared static with dynamic decision making, writing:
In dynamic situations, a new complication not found in the static situations arises. The environment in which the decision is set may be changing, either as a function of the sequence of decisions, or independently of them, or both. It is this possibility of an environment which changes while you collect information about it which makes the task of dynamic decision theory so difficult and so much fun. (p. 60)
The ability to solve complex problems is typically measured via dynamic systems that contain several interrelated variables that participants need to alter. Early work (see, e.g., Dörner, 1980 ) used a simulation scenario called “Lohhausen” that contained more than 2000 variables that represented the activities of a small town: Participants had to take over the role of a mayor for a simulated period of 10 years. The simulation condensed these ten years to ten hours in real time. Later, researchers used smaller dynamic systems as scenarios either based on linear equations (see, e.g., Funke, 1993 ) or on finite state automata (see, e.g., Buchner and Funke, 1993 ). In these contexts, CPS consisted of the identification and control of dynamic task environments that were previously unknown to the participants. Different task environments came along with different degrees of fidelity ( Gray, 2002 ).
According to Funke (2012) , the typical attributes of complex systems are (a) complexity of the problem situation which is usually represented by the sheer number of involved variables; (b) connectivity and mutual dependencies between involved variables; (c) dynamics of the situation, which reflects the role of time and developments within a system; (d) intransparency (in part or full) about the involved variables and their current values; and (e) polytely (greek term for “many goals”), representing goal conflicts on different levels of analysis. This mixture of features is similar to what is called VUCA (volatility, uncertainty, complexity, ambiguity) in modern approaches to management (e.g., Mack et al., 2016 ).
In his evaluation of the CPS movement, Sternberg (1995) compared (young) European approaches to CPS with (older) American research on expertise. His analysis of the differences between the European and American traditions shows advantages but also potential drawbacks for each side. He states (p. 301): “I believe that although there are problems with the European approach, it deals with some fundamental questions that American research scarcely addresses.” So, even though the echo of the European approach did not enjoy strong resonance in the US at that time, it was valued by scholars like Sternberg and others. Before attending to validity issues, we will first present a short review of different streams.
Different Approaches to CPS
In the short history of CPS research, different approaches can be identified ( Buchner, 1995 ; Fischer et al., 2017 ). To systematize, we differentiate between the following five lines of research:
(a) The search for individual differences comprises studies identifying interindividual differences that affect the ability to solve complex problems. This line of research is reflected, for example, in the early work by Dörner et al. (1983) and their “Lohhausen” study. Here, naïve student participants took over the role of the mayor of a small simulated town named Lohhausen for a simulation period of ten years. According to the results of the authors, it is not intelligence (as measured by conventional IQ tests) that predicts performance, but it is the ability to stay calm in the face of a challenging situation and the ability to switch easily between an analytic mode of processing and a more holistic one.
(b) The search for cognitive processes deals with the processes behind understanding complex dynamic systems. Representative of this line of research is, for example, Berry and Broadbent’s (1984) work on implicit and explicit learning processes when people interact with a dynamic system called “Sugar Production”. They found that those who perform best in controlling a dynamic system can do so implicitly, without explicit knowledge of details regarding the systems’ relations.
(c) The search for system factors seeks to identify the aspects of dynamic systems that determine the difficulty of complex problems and make some problems harder than others. Representative of this line of research is, for example, work by Funke (1985) , who systematically varied the number of causal effects within a dynamic system or the presence/absence of eigendynamics. He found, for example, that solution quality decreases as the number of systems relations increases.
(d) The psychometric approach develops measurement instruments that can be used as an alternative to classical IQ tests, as something that goes “beyond IQ”. The MicroDYN approach ( Wüstenberg et al., 2012 ) is representative for this line of research that presents an alternative to reasoning tests (like Raven matrices). These authors demonstrated that a small improvement in predicting school grade point average beyond reasoning is possible with MicroDYN tests.
(e) The experimental approach explores CPS under different experimental conditions. This approach uses CPS assessment instruments to test hypotheses derived from psychological theories and is sometimes used in research about cognitive processes (see above). Exemplary for this line of research is the work by Rohe et al. (2016) , who test the usefulness of “motto goals” in the context of complex problems compared to more traditional learning and performance goals. Motto goals differ from pure performance goals by activating positive affect and should lead to better goal attainment especially in complex situations (the mentioned study found no effect).
To be clear: these five approaches are not mutually exclusive and do overlap. But the differentiation helps to identify different research communities and different traditions. These communities had different opinions about scaling complexity.
The Race for Complexity: Use of More and More Complex Systems
In the early years of CPS research, microworlds started with systems containing about 20 variables (“Tailorshop”), soon reached 60 variables (“Moro”), and culminated in systems with about 2000 variables (“Lohhausen”). This race for complexity ended with the introduction of the concept of “minimal complex systems” (MCS; Greiff and Funke, 2009 ; Funke and Greiff, 2017 ), which ushered in a search for the lower bound of complexity instead of the higher bound, which could not be defined as easily. The idea behind this concept was that whereas the upper limits of complexity are unbound, the lower limits might be identifiable. Imagine starting with a simple system containing two variables with a simple linear connection between them; then, step by step, increase the number of variables and/or the type of connections. One soon reaches a point where the system can no longer be considered simple and has become a “complex system”. This point represents a minimal complex system. Despite some research having been conducted in this direction, the point of transition from simple to complex has not been identified clearly as of yet.
Some years later, the original “minimal complex systems” approach ( Greiff and Funke, 2009 ) shifted to the “multiple complex systems” approach ( Greiff et al., 2013a ). This shift is more than a slight change in wording: it is important because it taps into the issue of validity directly. Minimal complex systems have been introduced in the context of challenges from large-scale assessments like PISA 2012 that measure new aspects of problem solving, namely interactive problems besides static problem solving ( Greiff and Funke, 2017 ). PISA 2012 required test developers to remain within testing time constraints (given by the school class schedule). Also, test developers needed a large item pool for the construction of a broad class of problem solving items. It was clear from the beginning that MCS deal with simple dynamic situations that require controlled interaction: the exploration and control of simple ticket machines, simple mobile phones, or simple MP3 players (all of these example domains were developed within PISA 2012) – rather than really complex situations like managerial or political decision making.
As a consequence of this subtle but important shift in interpreting the letters MCS, the definition of CPS became a subject of debate recently ( Funke, 2014a ; Greiff and Martin, 2014 ; Funke et al., 2017 ). In the words of Funke (2014b , p. 495):
It is funny that problems that nowadays come under the term ‘CPS’, are less complex (in terms of the previously described attributes of complex situations) than at the beginning of this new research tradition. The emphasis on psychometric qualities has led to a loss of variety. Systems thinking requires more than analyzing models with two or three linear equations – nonlinearity, cyclicity, rebound effects, etc. are inherent features of complex problems and should show up at least in some of the problems used for research and assessment purposes. Minimal complex systems run the danger of becoming minimal valid systems.
Searching for minimal complex systems is not the same as gaining insight into the way how humans deal with complexity and uncertainty. For psychometric purposes, it is appropriate to reduce complexity to a minimum; for understanding problem solving under conditions of overload, intransparency, and dynamics, it is necessary to realize those attributes with reasonable strength. This aspect is illustrated in the next section.
Importance of the Validity Issue
The most important reason for discussing the question of what complex problem solving is and what it is not stems from its phenomenology: if we lose sight of our phenomena, we are no longer doing good psychology. The relevant phenomena in the context of complex problems encompass many important aspects. In this section, we discuss four phenomena that are specific to complex problems. We consider these phenomena as critical for theory development and for the construction of assessment instruments (i.e., microworlds). These phenomena require theories for explaining them and they require assessment instruments eliciting them in a reliable way.
The first phenomenon is the emergency reaction of the intellectual system ( Dörner, 1980 ): When dealing with complex systems, actors tend to (a) reduce their intellectual level by decreasing self-reflections, by decreasing their intentions, by stereotyping, and by reducing their realization of intentions, (b) they show a tendency for fast action with increased readiness for risk, with increased violations of rules, and with increased tendency to escape the situation, and (c) they degenerate their hypotheses formation by construction of more global hypotheses and reduced tests of hypotheses, by increasing entrenchment, and by decontextualizing their goals. This phenomenon illustrates the strong connection between cognition, emotion, and motivation that has been emphasized by Dörner (see, e.g., Dörner and Güss, 2013 ) from the beginning of his research tradition; the emergency reaction reveals a shift in the mode of information processing under the pressure of complexity.
The second phenomenon comprises cross-cultural differences with respect to strategy use ( Strohschneider and Güss, 1999 ; Güss and Wiley, 2007 ; Güss et al., 2015 ). Results from complex task environments illustrate the strong influence of context and background knowledge to an extent that cannot be found for knowledge-poor problems. For example, in a comparison between Brazilian and German participants, it turned out that Brazilians accept the given problem descriptions and are more optimistic about the results of their efforts, whereas Germans tend to inquire more about the background of the problems and take a more active approach but are less optimistic (according to Strohschneider and Güss, 1998 , p. 695).
The third phenomenon relates to failures that occur during the planning and acting stages ( Jansson, 1994 ; Ramnarayan et al., 1997 ), illustrating that rational procedures seem to be unlikely to be used in complex situations. The potential for failures ( Dörner, 1996 ) rises with the complexity of the problem. Jansson (1994) presents seven major areas for failures with complex situations: acting directly on current feedback; insufficient systematization; insufficient control of hypotheses and strategies; lack of self-reflection; selective information gathering; selective decision making; and thematic vagabonding.
The fourth phenomenon describes (a lack of) training and transfer effects ( Kretzschmar and Süß, 2015 ), which again illustrates the context dependency of strategies and knowledge (i.e., there is no strategy that is so universal that it can be used in many different problem situations). In their own experiment, the authors could show training effects only for knowledge acquisition, not for knowledge application. Only with specific feedback, performance in complex environments can be increased ( Engelhart et al., 2017 ).
These four phenomena illustrate why the type of complexity (or degree of simplicity) used in research really matters. Furthermore, they demonstrate effects that are specific for complex problems, but not for toy problems. These phenomena direct the attention to the important question: does the stimulus material used (i.e., the computer-simulated microworld) tap and elicit the manifold of phenomena described above?
Dealing with partly unknown complex systems requires courage, wisdom, knowledge, grit, and creativity. In creativity research, “little c” and “BIG C” are used to differentiate between everyday creativity and eminent creativity ( Beghetto and Kaufman, 2007 ; Kaufman and Beghetto, 2009 ). Everyday creativity is important for solving everyday problems (e.g., finding a clever fix for a broken spoke on my bicycle), eminent creativity changes the world (e.g., inventing solar cells for energy production). Maybe problem solving research should use a similar differentiation between “little p” and “BIG P” to mark toy problems on the one side and big societal challenges on the other. The question then remains: what can we learn about BIG P by studying little p? What phenomena are present in both types, and what phenomena are unique to each of the two extremes?
Discussing research on CPS requires reflecting on the field’s research methods. Even if the experimental approach has been successful for testing hypotheses (for an overview of older work, see Funke, 1995 ), other methods might provide additional and novel insights. Complex phenomena require complex approaches to understand them. The complex nature of complex systems imposes limitations on psychological experiments: The more complex the environments, the more difficult is it to keep conditions under experimental control. And if experiments have to be run in labs one should bring enough complexity into the lab to establish the phenomena mentioned, at least in part.
There are interesting options to be explored (again): think-aloud protocols , which have been discredited for many years ( Nisbett and Wilson, 1977 ) and yet are a valuable source for theory testing ( Ericsson and Simon, 1983 ); introspection ( Jäkel and Schreiber, 2013 ), which seems to be banned from psychological methods but nevertheless offers insights into thought processes; the use of life-streaming ( Wendt, 2017 ), a medium in which streamers generate a video stream of think-aloud data in computer-gaming; political decision-making ( Dhami et al., 2015 ) that demonstrates error-proneness in groups; historical case studies ( Dörner and Güss, 2011 ) that give insights into the thinking styles of political leaders; the use of the critical incident technique ( Reuschenbach, 2008 ) to construct complex scenarios; and simulations with different degrees of fidelity ( Gray, 2002 ).
The methods tool box is full of instruments that have to be explored more carefully before any individual instrument receives a ban or research narrows its focus to only one paradigm for data collection. Brehmer and Dörner (1993) discussed the tensions between “research in the laboratory and research in the field”, optimistically concluding “that the new methodology of computer-simulated microworlds will provide us with the means to bridge the gap between the laboratory and the field” (p. 183). The idea behind this optimism was that computer-simulated scenarios would bring more complexity from the outside world into the controlled lab environment. But this is not true for all simulated scenarios. In his paper on simulated environments, Gray (2002) differentiated computer-simulated environments with respect to three dimensions: (1) tractability (“the more training subjects require before they can use a simulated task environment, the less tractable it is”, p. 211), correspondence (“High correspondence simulated task environments simulate many aspects of one task environment. Low correspondence simulated task environments simulate one aspect of many task environments”, p. 214), and engagement (“A simulated task environment is engaging to the degree to which it involves and occupies the participants; that is, the degree to which they agree to take it seriously”, p. 217). But the mere fact that a task is called a “computer-simulated task environment” does not mean anything specific in terms of these three dimensions. This is one of several reasons why we should differentiate between those studies that do not address the core features of CPS and those that do.
What is not CPS?
Even though a growing number of references claiming to deal with complex problems exist (e.g., Greiff and Wüstenberg, 2015 ; Greiff et al., 2016 ), it would be better to label the requirements within these tasks “dynamic problem solving,” as it has been done adequately in earlier work ( Greiff et al., 2012 ). The dynamics behind on-off-switches ( Thimbleby, 2007 ) are remarkable but not really complex. Small nonlinear systems that exhibit stunningly complex and unstable behavior do exist – but they are not used in psychometric assessments of so-called CPS. There are other small systems (like MicroDYN scenarios: Greiff and Wüstenberg, 2014 ) that exhibit simple forms of system behavior that are completely predictable and stable. This type of simple systems is used frequently. It is even offered commercially as a complex problem-solving test called COMPRO ( Greiff and Wüstenberg, 2015 ) for business applications. But a closer look reveals that the label is not used correctly; within COMPRO, the used linear equations are far from being complex and the system can be handled properly by using only one strategy (see for more details Funke et al., 2017 ).
Why do simple linear systems not fall within CPS? At the surface, nonlinear and linear systems might appear similar because both only include 3–5 variables. But the difference is in terms of systems behavior as well as strategies and learning. If the behavior is simple (as in linear systems where more input is related to more output and vice versa), the system can be easily understood (participants in the MicroDYN world have 3 minutes to explore a complex system). If the behavior is complex (as in systems that contain strange attractors or negative feedback loops), things become more complicated and much more observation is needed to identify the hidden structure of the unknown system ( Berry and Broadbent, 1984 ; Hundertmark et al., 2015 ).
Another issue is learning. If tasks can be solved using a single (and not so complicated) strategy, steep learning curves are to be expected. The shift from problem solving to learned routine behavior occurs rapidly, as was demonstrated by Luchins (1942) . In his water jar experiments, participants quickly acquired a specific strategy (a mental set) for solving certain measurement problems that they later continued applying to problems that would have allowed for easier approaches. In the case of complex systems, learning can occur only on very general, abstract levels because it is difficult for human observers to make specific predictions. Routines dealing with complex systems are quite different from routines relating to linear systems.
What should not be studied under the label of CPS are pure learning effects, multiple-cue probability learning, or tasks that can be solved using a single strategy. This last issue is a problem for MicroDYN tasks that rely strongly on the VOTAT strategy (“vary one thing at a time”; see Tschirgi, 1980 ). In real-life, it is hard to imagine a business manager trying to solve her or his problems by means of VOTAT.
What is CPS?
In the early days of CPS research, planet Earth’s dynamics and complexities gained attention through such books as “The limits to growth” ( Meadows et al., 1972 ) and “Beyond the limits” ( Meadows et al., 1992 ). In the current decade, for example, the World Economic Forum (2016) attempts to identify the complexities and risks of our modern world. In order to understand the meaning of complexity and uncertainty, taking a look at the worlds’ most pressing issues is helpful. Searching for strategies to cope with these problems is a difficult task: surely there is no place for the simple principle of “vary-one-thing-at-a-time” (VOTAT) when it comes to global problems. The VOTAT strategy is helpful in the context of simple problems ( Wüstenberg et al., 2014 ); therefore, whether or not VOTAT is helpful in a given problem situation helps us distinguish simple from complex problems.
Because there exist no clear-cut strategies for complex problems, typical failures occur when dealing with uncertainty ( Dörner, 1996 ; Güss et al., 2015 ). Ramnarayan et al. (1997) put together a list of generic errors (e.g., not developing adequate action plans; lack of background control; learning from experience blocked by stereotype knowledge; reactive instead of proactive action) that are typical of knowledge-rich complex systems but cannot be found in simple problems.
Complex problem solving is not a one-dimensional, low-level construct. On the contrary, CPS is a multi-dimensional bundle of competencies existing at a high level of abstraction, similar to intelligence (but going beyond IQ). As Funke et al. (2018) state: “Assessment of transversal (in educational contexts: cross-curricular) competencies cannot be done with one or two types of assessment. The plurality of skills and competencies requires a plurality of assessment instruments.”
There are at least three different aspects of complex systems that are part of our understanding of a complex system: (1) a complex system can be described at different levels of abstraction; (2) a complex system develops over time, has a history, a current state, and a (potentially unpredictable) future; (3) a complex system is knowledge-rich and activates a large semantic network, together with a broad list of potential strategies (domain-specific as well as domain-general).
Complex problem solving is not only a cognitive process but is also an emotional one ( Spering et al., 2005 ; Barth and Funke, 2010 ) and strongly dependent on motivation (low-stakes versus high-stakes testing; see Hermes and Stelling, 2016 ).
Furthermore, CPS is a dynamic process unfolding over time, with different phases and with more differentiation than simply knowledge acquisition and knowledge application. Ideally, the process should entail identifying problems (see Dillon, 1982 ; Lee and Cho, 2007 ), even if in experimental settings, problems are provided to participants a priori . The more complex and open a given situation, the more options can be generated (T. S. Schweizer et al., 2016 ). In closed problems, these processes do not occur in the same way.
In analogy to the difference between formative (process-oriented) and summative (result-oriented) assessment ( Wiliam and Black, 1996 ; Bennett, 2011 ), CPS should not be reduced to the mere outcome of a solution process. The process leading up to the solution, including detours and errors made along the way, might provide a more differentiated impression of a person’s problem-solving abilities and competencies than the final result of such a process. This is one of the reasons why CPS environments are not, in fact, complex intelligence tests: research on CPS is not only about the outcome of the decision process, but it is also about the problem-solving process itself.
Complex problem solving is part of our daily life: finding the right person to share one’s life with, choosing a career that not only makes money, but that also makes us happy. Of course, CPS is not restricted to personal problems – life on Earth gives us many hard nuts to crack: climate change, population growth, the threat of war, the use and distribution of natural resources. In sum, many societal challenges can be seen as complex problems. To reduce that complexity to a one-hour lab activity on a random Friday afternoon puts it out of context and does not address CPS issues.
Theories about CPS should specify which populations they apply to. Across populations, one thing to consider is prior knowledge. CPS research with experts (e.g., Dew et al., 2009 ) is quite different from problem solving research using tasks that intentionally do not require any specific prior knowledge (see, e.g., Beckmann and Goode, 2014 ).
More than 20 years ago, Frensch and Funke (1995b) defined CPS as follows:
CPS occurs to overcome barriers between a given state and a desired goal state by means of behavioral and/or cognitive, multi-step activities. The given state, goal state, and barriers between given state and goal state are complex, change dynamically during problem solving, and are intransparent. The exact properties of the given state, goal state, and barriers are unknown to the solver at the outset. CPS implies the efficient interaction between a solver and the situational requirements of the task, and involves a solver’s cognitive, emotional, personal, and social abilities and knowledge. (p. 18)
The above definition is rather formal and does not account for content or relations between the simulation and the real world. In a sense, we need a new definition of CPS that addresses these issues. Based on our previous arguments, we propose the following working definition:
Complex problem solving is a collection of self-regulated psychological processes and activities necessary in dynamic environments to achieve ill-defined goals that cannot be reached by routine actions. Creative combinations of knowledge and a broad set of strategies are needed. Solutions are often more bricolage than perfect or optimal. The problem-solving process combines cognitive, emotional, and motivational aspects, particularly in high-stakes situations. Complex problems usually involve knowledge-rich requirements and collaboration among different persons.
The main differences to the older definition lie in the emphasis on (a) the self-regulation of processes, (b) creativity (as opposed to routine behavior), (c) the bricolage type of solution, and (d) the role of high-stakes challenges. Our new definition incorporates some aspects that have been discussed in this review but were not reflected in the 1995 definition, which focused on attributes of complex problems like dynamics or intransparency.
This leads us to the final reflection about the role of CPS for dealing with uncertainty and complexity in real life. We will distinguish thinking from reasoning and introduce the sense of possibility as an important aspect of validity.
CPS as Combining Reasoning and Thinking in an Uncertain Reality
Leading up to the Battle of Borodino in Leo Tolstoy’s novel “War and Peace”, Prince Andrei Bolkonsky explains the concept of war to his friend Pierre. Pierre expects war to resemble a game of chess: You position the troops and attempt to defeat your opponent by moving them in different directions.
“Far from it!”, Andrei responds. “In chess, you know the knight and his moves, you know the pawn and his combat strength. While in war, a battalion is sometimes stronger than a division and sometimes weaker than a company; it all depends on circumstances that can never be known. In war, you do not know the position of your enemy; some things you might be able to observe, some things you have to divine (but that depends on your ability to do so!) and many things cannot even be guessed at. In chess, you can see all of your opponent’s possible moves. In war, that is impossible. If you decide to attack, you cannot know whether the necessary conditions are met for you to succeed. Many a time, you cannot even know whether your troops will follow your orders…”
In essence, war is characterized by a high degree of uncertainty. A good commander (or politician) can add to that what he or she sees, tentatively fill in the blanks – and not just by means of logical deduction but also by intelligently bridging missing links. A bad commander extrapolates from what he sees and thus arrives at improper conclusions.
Many languages differentiate between two modes of mentalizing; for instance, the English language distinguishes between ‘thinking’ and ‘reasoning’. Reasoning denotes acute and exact mentalizing involving logical deductions. Such deductions are usually based on evidence and counterevidence. Thinking, however, is what is required to write novels. It is the construction of an initially unknown reality. But it is not a pipe dream, an unfounded process of fabrication. Rather, thinking asks us to imagine reality (“Wirklichkeitsfantasie”). In other words, a novelist has to possess a “sense of possibility” (“Möglichkeitssinn”, Robert Musil; in German, sense of possibility is often used synonymously with imagination even though imagination is not the same as sense of possibility, for imagination also encapsulates the impossible). This sense of possibility entails knowing the whole (or several wholes) or being able to construe an unknown whole that could accommodate a known part. The whole has to align with sociological and geographical givens, with the mentality of certain peoples or groups, and with the laws of physics and chemistry. Otherwise, the entire venture is ill-founded. A sense of possibility does not aim for the moon but imagines something that might be possible but has not been considered possible or even potentially possible so far.
Thinking is a means to eliminate uncertainty. This process requires both of the modes of thinking we have discussed thus far. Economic, political, or ecological decisions require us to first consider the situation at hand. Though certain situational aspects can be known, but many cannot. In fact, von Clausewitz (1832) posits that only about 25% of the necessary information is available when a military decision needs to be made. Even then, there is no way to guarantee that whatever information is available is also correct: Even if a piece of information was completely accurate yesterday, it might no longer apply today.
Once our sense of possibility has helped grasping a situation, problem solvers need to call on their reasoning skills. Not every situation requires the same action, and we may want to act this way or another to reach this or that goal. This appears logical, but it is a logic based on constantly shifting grounds: We cannot know whether necessary conditions are met, sometimes the assumptions we have made later turn out to be incorrect, and sometimes we have to revise our assumptions or make completely new ones. It is necessary to constantly switch between our sense of possibility and our sense of reality, that is, to switch between thinking and reasoning. It is an arduous process, and some people handle it well, while others do not.
If we are to believe Tuchman’s (1984) book, “The March of Folly”, most politicians and commanders are fools. According to Tuchman, not much has changed in the 3300 years that have elapsed since the misguided Trojans decided to welcome the left-behind wooden horse into their city that would end up dismantling Troy’s defensive walls. The Trojans, too, had been warned, but decided not to heed the warning. Although Laocoön had revealed the horse’s true nature to them by attacking it with a spear, making the weapons inside the horse ring, the Trojans refused to see the forest for the trees. They did not want to listen, they wanted the war to be over, and this desire ended up shaping their perception.
The objective of psychology is to predict and explain human actions and behavior as accurately as possible. However, thinking cannot be investigated by limiting its study to neatly confined fractions of reality such as the realms of propositional logic, chess, Go tasks, the Tower of Hanoi, and so forth. Within these systems, there is little need for a sense of possibility. But a sense of possibility – the ability to divine and construe an unknown reality – is at least as important as logical reasoning skills. Not researching the sense of possibility limits the validity of psychological research. All economic and political decision making draws upon this sense of possibility. By not exploring it, psychological research dedicated to the study of thinking cannot further the understanding of politicians’ competence and the reasons that underlie political mistakes. Christopher Clark identifies European diplomats’, politicians’, and commanders’ inability to form an accurate representation of reality as a reason for the outbreak of World War I. According to Clark’s (2012) book, “The Sleepwalkers”, the politicians of the time lived in their own make-believe world, wrongfully assuming that it was the same world everyone else inhabited. If CPS research wants to make significant contributions to the world, it has to acknowledge complexity and uncertainty as important aspects of it.
For more than 40 years, CPS has been a new subject of psychological research. During this time period, the initial emphasis on analyzing how humans deal with complex, dynamic, and uncertain situations has been lost. What is subsumed under the heading of CPS in modern research has lost the original complexities of real-life problems. From our point of view, the challenges of the 21st century require a return to the origins of this research tradition. We would encourage researchers in the field of problem solving to come back to the original ideas. There is enough complexity and uncertainty in the world to be studied. Improving our understanding of how humans deal with these global and pressing problems would be a worthwhile enterprise.
Author Contributions
JF drafted a first version of the manuscript, DD added further text and commented on the draft. JF finalized the manuscript.
Authors Note
After more than 40 years of controversial discussions between both authors, this is the first joint paper. We are happy to have done this now! We have found common ground!
Conflict of Interest Statement
The authors declare that the research was conducted in the absence of any commercial or financial relationships that could be construed as a potential conflict of interest.
Acknowledgments
The authors thank the Deutsche Forschungsgemeinschaft (DFG) for the continuous support of their research over many years. Thanks to Daniel Holt for his comments on validity issues, thanks to Julia Nolte who helped us by translating German text excerpts into readable English and helped us, together with Keri Hartman, to improve our style and grammar – thanks for that! We also thank the two reviewers for their helpful critical comments on earlier versions of this manuscript. Finally, we acknowledge financial support by Deutsche Forschungsgemeinschaft and Ruprecht-Karls-Universität Heidelberg within their funding programme Open Access Publishing .
- ^ The fMRI-paper from Anderson (2012) uses the term “complex problem solving” for tasks that do not fall in our understanding of CPS and is therefore excluded from this list.
Alison, L., van den Heuvel, C., Waring, S., Power, N., Long, A., O’Hara, T., et al. (2013). Immersive simulated learning environments for researching critical incidents: a knowledge synthesis of the literature and experiences of studying high-risk strategic decision making. J. Cogn. Eng. Deci. Mak. 7, 255–272. doi: 10.1177/1555343412468113
CrossRef Full Text | Google Scholar
Anderson, J. R. (2012). Tracking problem solving by multivariate pattern analysis and hidden markov model algorithms. Neuropsychologia 50, 487–498. doi: 10.1016/j.neuropsychologia.2011.07.025
PubMed Abstract | CrossRef Full Text | Google Scholar
Barth, C. M., and Funke, J. (2010). Negative affective environments improve complex solving performance. Cogn. Emot. 24, 1259–1268. doi: 10.1080/02699930903223766
Beckmann, J. F., and Goode, N. (2014). The benefit of being naïve and knowing it: the unfavourable impact of perceived context familiarity on learning in complex problem solving tasks. Instruct. Sci. 42, 271–290. doi: 10.1007/s11251-013-9280-7
Beghetto, R. A., and Kaufman, J. C. (2007). Toward a broader conception of creativity: a case for “mini-c” creativity. Psychol. Aesthetics Creat. Arts 1, 73–79. doi: 10.1037/1931-3896.1.2.73
Bennett, R. E. (2011). Formative assessment: a critical review. Assess. Educ. Princ. Policy Pract. 18, 5–25. doi: 10.1080/0969594X.2010.513678
Berry, D. C., and Broadbent, D. E. (1984). On the relationship between task performance and associated verbalizable knowledge. Q. J. Exp. Psychol. 36, 209–231. doi: 10.1080/14640748408402156
Blech, C., and Funke, J. (2010). You cannot have your cake and eat it, too: how induced goal conflicts affect complex problem solving. Open Psychol. J. 3, 42–53. doi: 10.2174/1874350101003010042
Brehmer, B., and Dörner, D. (1993). Experiments with computer-simulated microworlds: escaping both the narrow straits of the laboratory and the deep blue sea of the field study. Comput. Hum. Behav. 9, 171–184. doi: 10.1016/0747-5632(93)90005-D
Buchner, A. (1995). “Basic topics and approaches to the study of complex problem solving,” in Complex Problem Solving: The European Perspective , eds P. A. Frensch and J. Funke (Hillsdale, NJ: Erlbaum), 27–63.
Google Scholar
Buchner, A., and Funke, J. (1993). Finite state automata: dynamic task environments in problem solving research. Q. J. Exp. Psychol. 46A, 83–118. doi: 10.1080/14640749308401068
Clark, C. (2012). The Sleepwalkers: How Europe Went to War in 1914 . London: Allen Lane.
Csapó, B., and Funke, J. (2017a). “The development and assessment of problem solving in 21st-century schools,” in The Nature of Problem Solving: Using Research to Inspire 21st Century Learning , eds B. Csapó and J. Funke (Paris: OECD Publishing), 19–31.
Csapó, B., and Funke, J. (eds) (2017b). The Nature of Problem Solving. Using Research to Inspire 21st Century Learning. Paris: OECD Publishing.
Danner, D., Hagemann, D., Holt, D. V., Hager, M., Schankin, A., Wüstenberg, S., et al. (2011a). Measuring performance in dynamic decision making. Reliability and validity of the Tailorshop simulation. J. Ind. Differ. 32, 225–233. doi: 10.1027/1614-0001/a000055
CrossRef Full Text
Danner, D., Hagemann, D., Schankin, A., Hager, M., and Funke, J. (2011b). Beyond IQ: a latent state-trait analysis of general intelligence, dynamic decision making, and implicit learning. Intelligence 39, 323–334. doi: 10.1016/j.intell.2011.06.004
Dew, N., Read, S., Sarasvathy, S. D., and Wiltbank, R. (2009). Effectual versus predictive logics in entrepreneurial decision-making: differences between experts and novices. J. Bus. Ventur. 24, 287–309. doi: 10.1016/j.jbusvent.2008.02.002
Dhami, M. K., Mandel, D. R., Mellers, B. A., and Tetlock, P. E. (2015). Improving intelligence analysis with decision science. Perspect. Psychol. Sci. 10, 753–757. doi: 10.1177/1745691615598511
Dillon, J. T. (1982). Problem finding and solving. J. Creat. Behav. 16, 97–111. doi: 10.1002/j.2162-6057.1982.tb00326.x
Dörner, D. (1975). Wie Menschen eine Welt verbessern wollten [How people wanted to improve a world]. Bild Der Wissenschaft 12, 48–53.
Dörner, D. (1980). On the difficulties people have in dealing with complexity. Simulat. Gam. 11, 87–106. doi: 10.1177/104687818001100108
Dörner, D. (1996). The Logic of Failure: Recognizing and Avoiding Error in Complex Situations. New York, NY: Basic Books.
Dörner, D., Drewes, U., and Reither, F. (1975). “Über das Problemlösen in sehr komplexen Realitätsbereichen,” in Bericht über den 29. Kongreß der DGfPs in Salzburg 1974, Band 1 , ed. W. H. Tack (Göttingen: Hogrefe), 339–340.
Dörner, D., and Güss, C. D. (2011). A psychological analysis of Adolf Hitler’s decision making as commander in chief: summa confidentia et nimius metus. Rev. Gen. Psychol. 15, 37–49. doi: 10.1037/a0022375
Dörner, D., and Güss, C. D. (2013). PSI: a computational architecture of cognition, motivation, and emotion. Rev. Gen. Psychol. 17, 297–317. doi: 10.1037/a0032947
Dörner, D., Kreuzig, H. W., Reither, F., and Stäudel, T. (1983). Lohhausen. Vom Umgang mit Unbestimmtheit und Komplexität. Bern: Huber.
Ederer, P., Patt, A., and Greiff, S. (2016). Complex problem-solving skills and innovativeness – evidence from occupational testing and regional data. Eur. J. Educ. 51, 244–256. doi: 10.1111/ejed.12176
Edwards, W. (1962). Dynamic decision theory and probabiIistic information processing. Hum. Factors 4, 59–73. doi: 10.1177/001872086200400201
Engelhart, M., Funke, J., and Sager, S. (2017). A web-based feedback study on optimization-based training and analysis of human decision making. J. Dynamic Dec. Mak. 3, 1–23.
Ericsson, K. A., and Simon, H. A. (1983). Protocol Analysis: Verbal Reports As Data. Cambridge, MA: Bradford.
Fischer, A., Greiff, S., and Funke, J. (2017). “The history of complex problem solving,” in The Nature of Problem Solving: Using Research to Inspire 21st Century Learning , eds B. Csapó and J. Funke (Paris: OECD Publishing), 107–121.
Fischer, A., Holt, D. V., and Funke, J. (2015). Promoting the growing field of dynamic decision making. J. Dynamic Decis. Mak. 1, 1–3. doi: 10.11588/jddm.2015.1.23807
Fischer, A., Holt, D. V., and Funke, J. (2016). The first year of the “journal of dynamic decision making.” J. Dynamic Decis. Mak. 2, 1–2. doi: 10.11588/jddm.2016.1.28995
Fischer, A., and Neubert, J. C. (2015). The multiple faces of complex problems: a model of problem solving competency and its implications for training and assessment. J. Dynamic Decis. Mak. 1, 1–14. doi: 10.11588/jddm.2015.1.23945
Frensch, P. A., and Funke, J. (eds) (1995a). Complex Problem Solving: The European Perspective. Hillsdale, NJ: Erlbaum.
Frensch, P. A., and Funke, J. (1995b). “Definitions, traditions, and a general framework for understanding complex problem solving,” in Complex Problem Solving: The European Perspective , eds P. A. Frensch and J. Funke (Hillsdale, NJ: Lawrence Erlbaum), 3–25.
Frischkorn, G. T., Greiff, S., and Wüstenberg, S. (2014). The development of complex problem solving in adolescence: a latent growth curve analysis. J. Educ. Psychol. 106, 1004–1020. doi: 10.1037/a0037114
Funke, J. (1985). Steuerung dynamischer Systeme durch Aufbau und Anwendung subjektiver Kausalmodelle. Z. Psychol. 193, 435–457.
Funke, J. (1986). Komplexes Problemlösen - Bestandsaufnahme und Perspektiven [Complex Problem Solving: Survey and Perspectives]. Heidelberg: Springer.
Funke, J. (1993). “Microworlds based on linear equation systems: a new approach to complex problem solving and experimental results,” in The Cognitive Psychology of Knowledge , eds G. Strube and K.-F. Wender (Amsterdam: Elsevier Science Publishers), 313–330.
Funke, J. (1995). “Experimental research on complex problem solving,” in Complex Problem Solving: The European Perspective , eds P. A. Frensch and J. Funke (Hillsdale, NJ: Erlbaum), 243–268.
Funke, J. (2010). Complex problem solving: a case for complex cognition? Cogn. Process. 11, 133–142. doi: 10.1007/s10339-009-0345-0
Funke, J. (2012). “Complex problem solving,” in Encyclopedia of the Sciences of Learning , Vol. 38, ed. N. M. Seel (Heidelberg: Springer), 682–685.
Funke, J. (2014a). Analysis of minimal complex systems and complex problem solving require different forms of causal cognition. Front. Psychol. 5:739. doi: 10.3389/fpsyg.2014.00739
Funke, J. (2014b). “Problem solving: what are the important questions?,” in Proceedings of the 36th Annual Conference of the Cognitive Science Society , eds P. Bello, M. Guarini, M. McShane, and B. Scassellati (Austin, TX: Cognitive Science Society), 493–498.
Funke, J., Fischer, A., and Holt, D. V. (2017). When less is less: solving multiple simple problems is not complex problem solving—A comment on Greiff et al. (2015). J. Intell. 5:5. doi: 10.3390/jintelligence5010005
Funke, J., Fischer, A., and Holt, D. V. (2018). “Competencies for complexity: problem solving in the 21st century,” in Assessment and Teaching of 21st Century Skills , eds E. Care, P. Griffin, and M. Wilson (Dordrecht: Springer), 3.
Funke, J., and Greiff, S. (2017). “Dynamic problem solving: multiple-item testing based on minimally complex systems,” in Competence Assessment in Education. Research, Models and Instruments , eds D. Leutner, J. Fleischer, J. Grünkorn, and E. Klieme (Heidelberg: Springer), 427–443.
Gobert, J. D., Kim, Y. J., Pedro, M. A. S., Kennedy, M., and Betts, C. G. (2015). Using educational data mining to assess students’ skills at designing and conducting experiments within a complex systems microworld. Think. Skills Creat. 18, 81–90. doi: 10.1016/j.tsc.2015.04.008
Goode, N., and Beckmann, J. F. (2010). You need to know: there is a causal relationship between structural knowledge and control performance in complex problem solving tasks. Intelligence 38, 345–352. doi: 10.1016/j.intell.2010.01.001
Gray, W. D. (2002). Simulated task environments: the role of high-fidelity simulations, scaled worlds, synthetic environments, and laboratory tasks in basic and applied cognitive research. Cogn. Sci. Q. 2, 205–227.
Greiff, S., and Fischer, A. (2013). Measuring complex problem solving: an educational application of psychological theories. J. Educ. Res. 5, 38–58.
Greiff, S., Fischer, A., Stadler, M., and Wüstenberg, S. (2015a). Assessing complex problem-solving skills with multiple complex systems. Think. Reason. 21, 356–382. doi: 10.1080/13546783.2014.989263
Greiff, S., Stadler, M., Sonnleitner, P., Wolff, C., and Martin, R. (2015b). Sometimes less is more: comparing the validity of complex problem solving measures. Intelligence 50, 100–113. doi: 10.1016/j.intell.2015.02.007
Greiff, S., Fischer, A., Wüstenberg, S., Sonnleitner, P., Brunner, M., and Martin, R. (2013a). A multitrait–multimethod study of assessment instruments for complex problem solving. Intelligence 41, 579–596. doi: 10.1016/j.intell.2013.07.012
Greiff, S., Holt, D. V., and Funke, J. (2013b). Perspectives on problem solving in educational assessment: analytical, interactive, and collaborative problem solving. J. Problem Solv. 5, 71–91. doi: 10.7771/1932-6246.1153
Greiff, S., Wüstenberg, S., Molnár, G., Fischer, A., Funke, J., and Csapó, B. (2013c). Complex problem solving in educational contexts—something beyond g: concept, assessment, measurement invariance, and construct validity. J. Educ. Psychol. 105, 364–379. doi: 10.1037/a0031856
Greiff, S., and Funke, J. (2009). “Measuring complex problem solving: the MicroDYN approach,” in The Transition to Computer-Based Assessment. New Approaches to Skills Assessment and Implications for Large-Scale Testing , eds F. Scheuermann and J. Björnsson (Luxembourg: Office for Official Publications of the European Communities), 157–163.
Greiff, S., and Funke, J. (2017). “Interactive problem solving: exploring the potential of minimal complex systems,” in The Nature of Problem Solving: Using Research to Inspire 21st Century Learning , eds B. Csapó and J. Funke (Paris: OECD Publishing), 93–105.
Greiff, S., and Martin, R. (2014). What you see is what you (don’t) get: a comment on Funke’s (2014) opinion paper. Front. Psychol. 5:1120. doi: 10.3389/fpsyg.2014.01120
Greiff, S., and Neubert, J. C. (2014). On the relation of complex problem solving, personality, fluid intelligence, and academic achievement. Learn. Ind. Diff. 36, 37–48. doi: 10.1016/j.lindif.2014.08.003
Greiff, S., Niepel, C., Scherer, R., and Martin, R. (2016). Understanding students’ performance in a computer-based assessment of complex problem solving: an analysis of behavioral data from computer-generated log files. Comput. Hum. Behav. 61, 36–46. doi: 10.1016/j.chb.2016.02.095
Greiff, S., Stadler, M., Sonnleitner, P., Wolff, C., and Martin, R. (2017). Sometimes more is too much: a rejoinder to the commentaries on Greiff et al. (2015). J. Intell. 5:6. doi: 10.3390/jintelligence5010006
Greiff, S., and Wüstenberg, S. (2014). Assessment with microworlds using MicroDYN: measurement invariance and latent mean comparisons. Eur. J. Psychol. Assess. 1, 1–11. doi: 10.1027/1015-5759/a000194
Greiff, S., and Wüstenberg, S. (2015). Komplexer Problemlösetest COMPRO [Complex Problem-Solving Test COMPRO]. Mödling: Schuhfried.
Greiff, S., Wüstenberg, S., and Funke, J. (2012). Dynamic problem solving: a new assessment perspective. Appl. Psychol. Measure. 36, 189–213. doi: 10.1177/0146621612439620
Griffin, P., and Care, E. (2015). “The ATC21S method,” in Assessment and Taching of 21st Century Skills , eds P. Griffin and E. Care (Dordrecht, NL: Springer), 3–33.
Güss, C. D., and Dörner, D. (2011). Cultural differences in dynamic decision-making strategies in a non-linear, time-delayed task. Cogn. Syst. Res. 12, 365–376. doi: 10.1016/j.cogsys.2010.12.003
Güss, C. D., Tuason, M. T., and Orduña, L. V. (2015). Strategies, tactics, and errors in dynamic decision making in an Asian sample. J. Dynamic Deci. Mak. 1, 1–14. doi: 10.11588/jddm.2015.1.13131
Güss, C. D., and Wiley, B. (2007). Metacognition of problem-solving strategies in Brazil, India, and the United States. J. Cogn. Cult. 7, 1–25. doi: 10.1163/156853707X171793
Herde, C. N., Wüstenberg, S., and Greiff, S. (2016). Assessment of complex problem solving: what we know and what we don’t know. Appl. Meas. Educ. 29, 265–277. doi: 10.1080/08957347.2016.1209208
Hermes, M., and Stelling, D. (2016). Context matters, but how much? Latent state – trait analysis of cognitive ability assessments. Int. J. Sel. Assess. 24, 285–295. doi: 10.1111/ijsa.12147
Hotaling, J. M., Fakhari, P., and Busemeyer, J. R. (2015). “Dynamic decision making,” in International Encyclopedia of the Social & Behavioral Sciences , 2nd Edn, eds N. J. Smelser and P. B. Batles (New York, NY: Elsevier), 709–714.
Hundertmark, J., Holt, D. V., Fischer, A., Said, N., and Fischer, H. (2015). System structure and cognitive ability as predictors of performance in dynamic system control tasks. J. Dynamic Deci. Mak. 1, 1–10. doi: 10.11588/jddm.2015.1.26416
Jäkel, F., and Schreiber, C. (2013). Introspection in problem solving. J. Problem Solv. 6, 20–33. doi: 10.7771/1932-6246.1131
Jansson, A. (1994). Pathologies in dynamic decision making: consequences or precursors of failure? Sprache Kogn. 13, 160–173.
Kaufman, J. C., and Beghetto, R. A. (2009). Beyond big and little: the four c model of creativity. Rev. Gen. Psychol. 13, 1–12. doi: 10.1037/a0013688
Knauff, M., and Wolf, A. G. (2010). Complex cognition: the science of human reasoning, problem-solving, and decision-making. Cogn. Process. 11, 99–102. doi: 10.1007/s10339-010-0362-z
Kretzschmar, A. (2017). Sometimes less is not enough: a commentary on Greiff et al. (2015). J. Intell. 5:4. doi: 10.3390/jintelligence5010004
Kretzschmar, A., Neubert, J. C., Wüstenberg, S., and Greiff, S. (2016). Construct validity of complex problem solving: a comprehensive view on different facets of intelligence and school grades. Intelligence 54, 55–69. doi: 10.1016/j.intell.2015.11.004
Kretzschmar, A., and Süß, H.-M. (2015). A study on the training of complex problem solving competence. J. Dynamic Deci. Mak. 1, 1–14. doi: 10.11588/jddm.2015.1.15455
Lee, H., and Cho, Y. (2007). Factors affecting problem finding depending on degree of structure of problem situation. J. Educ. Res. 101, 113–123. doi: 10.3200/JOER.101.2.113-125
Leutner, D., Fleischer, J., Wirth, J., Greiff, S., and Funke, J. (2012). Analytische und dynamische Problemlösekompetenz im Lichte internationaler Schulleistungsvergleichsstudien: Untersuchungen zur Dimensionalität. Psychol. Rundschau 63, 34–42. doi: 10.1026/0033-3042/a000108
Luchins, A. S. (1942). Mechanization in problem solving: the effect of einstellung. Psychol. Monogr. 54, 1–95. doi: 10.1037/h0093502
Mack, O., Khare, A., Krämer, A., and Burgartz, T. (eds) (2016). Managing in a VUCA world. Heidelberg: Springer.
Mainert, J., Kretzschmar, A., Neubert, J. C., and Greiff, S. (2015). Linking complex problem solving and general mental ability to career advancement: does a transversal skill reveal incremental predictive validity? Int. J. Lifelong Educ. 34, 393–411. doi: 10.1080/02601370.2015.1060024
Mainzer, K. (2009). Challenges of complexity in the 21st century. An interdisciplinary introduction. Eur. Rev. 17, 219–236. doi: 10.1017/S1062798709000714
Meadows, D. H., Meadows, D. L., and Randers, J. (1992). Beyond the Limits. Vermont, VA: Chelsea Green Publishing.
Meadows, D. H., Meadows, D. L., Randers, J., and Behrens, W. W. (1972). The Limits to Growth. New York, NY: Universe Books.
Meißner, A., Greiff, S., Frischkorn, G. T., and Steinmayr, R. (2016). Predicting complex problem solving and school grades with working memory and ability self-concept. Learn. Ind. Differ. 49, 323–331. doi: 10.1016/j.lindif.2016.04.006
Molnàr, G., Greiff, S., Wüstenberg, S., and Fischer, A. (2017). “Empirical study of computer-based assessment of domain-general complex problem-solving skills,” in The Nature of Problem Solving: Using research to Inspire 21st Century Learning , eds B. Csapó and J. Funke (Paris: OECD Publishing), 125–141.
National Research Council (2011). Assessing 21st Century Skills: Summary of a Workshop. Washington, DC: The National Academies Press.
Newell, A., Shaw, J. C., and Simon, H. A. (1959). A general problem-solving program for a computer. Comput. Automat. 8, 10–16.
Nisbett, R. E., and Wilson, T. D. (1977). Telling more than we can know: verbal reports on mental processes. Psychol. Rev. 84, 231–259. doi: 10.1037/0033-295X.84.3.231
OECD (2014). “PISA 2012 results,” in Creative Problem Solving: Students’ Skills in Tackling Real-Life problems , Vol. 5 (Paris: OECD Publishing).
Osman, M. (2010). Controlling uncertainty: a review of human behavior in complex dynamic environments. Psychol. Bull. 136, 65–86. doi: 10.1037/a0017815
Osman, M. (2012). The role of reward in dynamic decision making. Front. Neurosci. 6:35. doi: 10.3389/fnins.2012.00035
Qudrat-Ullah, H. (2015). Better Decision Making in Complex, Dynamic Tasks. Training with Human-Facilitated Interactive Learning Environments. Heidelberg: Springer.
Ramnarayan, S., Strohschneider, S., and Schaub, H. (1997). Trappings of expertise and the pursuit of failure. Simulat. Gam. 28, 28–43. doi: 10.1177/1046878197281004
Reuschenbach, B. (2008). Planen und Problemlösen im Komplexen Handlungsfeld Pflege. Berlin: Logos.
Rohe, M., Funke, J., Storch, M., and Weber, J. (2016). Can motto goals outperform learning and performance goals? Influence of goal setting on performance, intrinsic motivation, processing style, and affect in a complex problem solving task. J. Dynamic Deci. Mak. 2, 1–15. doi: 10.11588/jddm.2016.1.28510
Scherer, R., Greiff, S., and Hautamäki, J. (2015). Exploring the relation between time on task and ability in complex problem solving. Intelligence 48, 37–50. doi: 10.1016/j.intell.2014.10.003
Schoppek, W., and Fischer, A. (2015). Complex problem solving – single ability or complex phenomenon? Front. Psychol. 6:1669. doi: 10.3389/fpsyg.2015.01669
Schraw, G., Dunkle, M., and Bendixen, L. D. (1995). Cognitive processes in well-defined and ill-defined problem solving. Appl. Cogn. Psychol. 9, 523–538. doi: 10.1002/acp.2350090605
Schweizer, F., Wüstenberg, S., and Greiff, S. (2013). Validity of the MicroDYN approach: complex problem solving predicts school grades beyond working memory capacity. Learn. Ind. Differ. 24, 42–52. doi: 10.1016/j.lindif.2012.12.011
Schweizer, T. S., Schmalenberger, K. M., Eisenlohr-Moul, T. A., Mojzisch, A., Kaiser, S., and Funke, J. (2016). Cognitive and affective aspects of creative option generation in everyday life situations. Front. Psychol. 7:1132. doi: 10.3389/fpsyg.2016.01132
Selten, R., Pittnauer, S., and Hohnisch, M. (2012). Dealing with dynamic decision problems when knowledge of the environment is limited: an approach based on goal systems. J. Behav. Deci. Mak. 25, 443–457. doi: 10.1002/bdm.738
Simon, H. A. (1957). Administrative Behavior: A Study of Decision-Making Processes in Administrative Organizations , 2nd Edn. New York, NY: Macmillan.
Sonnleitner, P., Brunner, M., Keller, U., and Martin, R. (2014). Differential relations between facets of complex problem solving and students’ immigration background. J. Educ. Psychol. 106, 681–695. doi: 10.1037/a0035506
Spering, M., Wagener, D., and Funke, J. (2005). The role of emotions in complex problem solving. Cogn. Emot. 19, 1252–1261. doi: 10.1080/02699930500304886
Stadler, M., Becker, N., Gödker, M., Leutner, D., and Greiff, S. (2015). Complex problem solving and intelligence: a meta-analysis. Intelligence 53, 92–101. doi: 10.1016/j.intell.2015.09.005
Stadler, M., Niepel, C., and Greiff, S. (2016). Easily too difficult: estimating item difficulty in computer simulated microworlds. Comput. Hum. Behav. 65, 100–106. doi: 10.1016/j.chb.2016.08.025
Sternberg, R. J. (1995). “Expertise in complex problem solving: a comparison of alternative conceptions,” in Complex Problem Solving: The European Perspective , eds P. A. Frensch and J. Funke (Hillsdale, NJ: Erlbaum), 295–321.
Sternberg, R. J., and Frensch, P. A. (1991). Complex Problem Solving: Principles and Mechanisms. (eds) R. J. Sternberg and P. A. Frensch. Hillsdale, NJ: Erlbaum.
Strohschneider, S., and Güss, C. D. (1998). Planning and problem solving: differences between brazilian and german students. J. Cross-Cult. Psychol. 29, 695–716. doi: 10.1177/0022022198296002
Strohschneider, S., and Güss, C. D. (1999). The fate of the Moros: a cross-cultural exploration of strategies in complex and dynamic decision making. Int. J. Psychol. 34, 235–252. doi: 10.1080/002075999399873
Thimbleby, H. (2007). Press On. Principles of Interaction. Cambridge, MA: MIT Press.
Tobinski, D. A., and Fritz, A. (2017). “EcoSphere: a new paradigm for problem solving in complex systems,” in The Nature of Problem Solving: Using Research to Inspire 21st Century Learning , eds B. Csapó and J. Funke (Paris: OECD Publishing), 211–222.
Tremblay, S., Gagnon, J.-F., Lafond, D., Hodgetts, H. M., Doiron, M., and Jeuniaux, P. P. J. M. H. (2017). A cognitive prosthesis for complex decision-making. Appl. Ergon. 58, 349–360. doi: 10.1016/j.apergo.2016.07.009
Tschirgi, J. E. (1980). Sensible reasoning: a hypothesis about hypotheses. Child Dev. 51, 1–10. doi: 10.2307/1129583
Tuchman, B. W. (1984). The March of Folly. From Troy to Vietnam. New York, NY: Ballantine Books.
Verweij, M., and Thompson, M. (eds) (2006). Clumsy Solutions for A Complex World. Governance, Politics and Plural Perceptions. New York, NY: Palgrave Macmillan. doi: 10.1057/9780230624887
Viehrig, K., Siegmund, A., Funke, J., Wüstenberg, S., and Greiff, S. (2017). “The heidelberg inventory of geographic system competency model,” in Competence Assessment in Education. Research, Models and Instruments , eds D. Leutner, J. Fleischer, J. Grünkorn, and E. Klieme (Heidelberg: Springer), 31–53.
von Clausewitz, C. (1832). Vom Kriege [On war]. Berlin: Dämmler.
Wendt, A. N. (2017). The empirical potential of live streaming beyond cognitive psychology. J. Dynamic Deci. Mak. 3, 1–9. doi: 10.11588/jddm.2017.1.33724
Wiliam, D., and Black, P. (1996). Meanings and consequences: a basis for distinguishing formative and summative functions of assessment? Br. Educ. Res. J. 22, 537–548. doi: 10.1080/0141192960220502
World Economic Forum (2015). New Vsion for Education Unlocking the Potential of Technology. Geneva: World Economic Forum.
World Economic Forum (2016). Global Risks 2016: Insight Report , 11th Edn. Geneva: World Economic Forum.
Wüstenberg, S., Greiff, S., and Funke, J. (2012). Complex problem solving — more than reasoning? Intelligence 40, 1–14. doi: 10.1016/j.intell.2011.11.003
Wüstenberg, S., Greiff, S., Vainikainen, M.-P., and Murphy, K. (2016). Individual differences in students’ complex problem solving skills: how they evolve and what they imply. J. Educ. Psychol. 108, 1028–1044. doi: 10.1037/edu0000101
Wüstenberg, S., Stadler, M., Hautamäki, J., and Greiff, S. (2014). The role of strategy knowledge for the application of strategies in complex problem solving tasks. Technol. Knowl. Learn. 19, 127–146. doi: 10.1007/s10758-014-9222-8
Keywords : complex problem solving, validity, assessment, definition, MicroDYN
Citation: Dörner D and Funke J (2017) Complex Problem Solving: What It Is and What It Is Not. Front. Psychol. 8:1153. doi: 10.3389/fpsyg.2017.01153
Received: 14 March 2017; Accepted: 23 June 2017; Published: 11 July 2017.
Reviewed by:
Copyright © 2017 Dörner and Funke. This is an open-access article distributed under the terms of the Creative Commons Attribution License (CC BY) . The use, distribution or reproduction in other forums is permitted, provided the original author(s) or licensor are credited and that the original publication in this journal is cited, in accordance with accepted academic practice. No use, distribution or reproduction is permitted which does not comply with these terms.
*Correspondence: Joachim Funke, [email protected]
Disclaimer: All claims expressed in this article are solely those of the authors and do not necessarily represent those of their affiliated organizations, or those of the publisher, the editors and the reviewers. Any product that may be evaluated in this article or claim that may be made by its manufacturer is not guaranteed or endorsed by the publisher.

- SUGGESTED TOPICS
- The Magazine
- Newsletters
- Managing Yourself
- Managing Teams
- Work-life Balance
- The Big Idea
- Data & Visuals
- Reading Lists
- Case Selections
- HBR Learning
- Topic Feeds
- Account Settings
- Email Preferences
Share Podcast

The Right Way to Solve Complex Business Problems
Corey Phelps, a strategy professor at McGill University, says great problem solvers are hard to find. Even seasoned professionals at the highest levels of organizations regularly...
- Apple Podcasts
Corey Phelps, a strategy professor at McGill University, says great problem solvers are hard to find. Even seasoned professionals at the highest levels of organizations regularly fail to identify the real problem and instead jump to exploring solutions. Phelps identifies the common traps and outlines a research-proven method to solve problems effectively. He’s the coauthor of the book, Cracked it! How to solve big problems and sell solutions like top strategy consultants.
Download this podcast
Welcome to the IdeaCast from Harvard Business Review. I’m Curt Nickisch.
Problem-solving is in demand. It’s considered the top skill for success at management consulting firms. And it’s increasingly desired for everyone, not just new MBA’s.
A report from the World Economic Forum predicts that more than one-third of all jobs across all industries will require complex problem-solving as one of their core skills by 2020.
The problem is, we’re often really bad at problem-solving. Our guest today says even the most educated and experienced of senior leaders go about it the wrong way.
COREY PHELPS: I think this is one of the misnomers about problem-solving. There’s this belief that because we do it so frequently – and especially for senior leaders, they have a lot of experience, they solve problems for a living – and as such we would expect them to be quite good at it. And I think what we find is that they’re not. They don’t solve problems well because they fall prey to basically the foibles of being a human being – they fall prey to the cognitive biases and the pitfalls of problem-solving.
CURT NICKISCH: That’s Corey Phelps. He says fixing these foibles is possible and almost straightforward. You can improve your problem-solving skills by following a disciplined method.
Corey Phelps is a strategy professor at McGill University. He’s also the co-author of the book “Cracked It: How to Solve Big Problems and Sell Solutions like Top Strategy C onsultants.” Corey thanks for coming on the show.
COREY PHELPS: Thank you for the opportunity to talk.
CURT NICKISCH: Another probably many, many biases that prevent people from solving big problems well.
COREY PHELPS: Absolutely.
CURT NICKISCH: What are some of the most common, or your favorite stumbling blocks?
COREY PHELPS: Well, one of my favorites is essentially the problem of jumping to solutions or the challenge of jumping to solutions.
CURT NICKISCH: Oh, come on Corey. That’s so much fun.
COREY PHELPS: It is, and it’s very much a result of how our brains have evolved to process information, but it’s my favorite because we all do it. And especially I would say it happens in organizations because in organizations when you layer on these time pressures and you layer on these concerns about efficiency and productivity, it creates enormous, I would say incentive to say “I don’t have time to carefully define and analyze the problem. I got to get a solution. I got to implement it as quick as possible.” And the fundamental bias I think is, is illustrated beautifully by Danny Kahneman in his book “Thinking, Fast and Slow,” is that our minds are essentially hardwired to think fast.
We are able to pay attention to a tiny little bit of information. We can then weave a very coherent story that makes sense to us. And then we can use that story to jump very quickly to a solution that we just know will work. And if we just were able to move from that approach of what Kahneman and cognitive psychologists called “System 1 thinking” to “System 2 thinking” – that is to slow down, be more deliberative, be more structured – we would be able to better understand the problem that we’re trying to solve and be more effective and exhaustive with the tools that we want to use to understand the problem before we actually go into solution-generation mode.
CURT NICKISCH: Complex problems demand different areas of expertise and often as individuals we’re coming to those problems with one of them. And I wonder if that’s often the problem of problem-solving, which is that a manager is approaching it from their own expertise and because of that, they see the problem through a certain way. Is that one of the cognitive biases that stop people from being effective problem solvers?
COREY PHELPS: Yeah. That’s often referred to as the expertise trap. It basically colors and influences what we pay attention to with respect to a particular problem. And it limits us with respect to the tools that we can bring to bear to solve that problem. In the world of psychology, there’s famous psychologist, Abraham Maslow, who is famous for the hierarchy of needs. He’s also famous for something that was a also known as MaSlow’s axiom, Maslow’s law. It’s also called the law of the instrument, and to paraphrase Maslow, he basically said, “Look, I suppose if the only tool that you have in your toolkit is a hammer, everything looks like a nail.”
His point is that if you’re, for example, a finance expert and your toolkit is the toolkit of let’s say, discounted cash flow analysis for valuation, then you’re going to see problems through that very narrow lens. Now, one of the ways out of this, I think to your point is collaboration becomes fundamentally important. And collaboration starts with the recognition that I don’t have all of the tools, all of the knowledge in me to effectively solve this. So I need to recruit people that can actually help me.
CURT NICKISCH: That’s really interesting. I wonder how much the fact that you have solved a problem before it makes you have a bias for that same solution for future problems?
COREY PHELPS: Yeah, that’s a great question. What you’re alluding to is analogical reasoning, and we know that human beings, one of the things that allows us to operate in novel settings is that we can draw on our past experience. And we do so when it comes to problem solving, often times without being conscious or mentally aware of it. We reach into our memory and we ask ourselves a very simple question: “Have I seen a problem like this before?”
And if it looks familiar to me, the tendency then is to say, “Okay, well what worked in solving that problem that I faced before?” And then to say, “Well, if it worked in that setting, then it should work in this setting.” So that’s reasoning by analogy.
Reasoning by analogy has a great upside. It allows human beings to not become overwhelmed by the tremendous novelty that they face in their daily lives. The downside is that if we don’t truly understand it at sort of a deep level, whether or not the two problems are similar or different, then we can make what cognitive psychologists called surface-level analogies.
And we can then say, “Oh, this looks a lot like the problem I faced before, that solution that worked there is going to easily work here.” And we try that solution and it fails and it fails largely because if we dug a little bit deeper, the two problems actually aren’t much alike at all in terms of their underlying causes.
CURT NICKISCH: The starkest example of this, I think, in your book is Ron Johnson who left Apple to become CEO of JC Penney. Can you talk about that a little bit and what that episode for the company says about this?
COREY PHELPS: So yes, its – Ron Johnson had been hired away from Target in the United States to, by Steve Jobs to help create Apple stores. Apple stores are as many people know the most successful physical retailer on the planet measured by, for example, sales per square foot or per square meter. He’s got the golden touch. He’s created this tremendously successful retail format for Apple.
So the day that it was announced that Ron Johnson was going to step into the CEO role at JC Penney, the stock price of JC Penney went up by almost 18 percent. So clearly he was viewed as the savior. Johnson moves very, very quickly. Within a few months, he announces that he has a strategic plan and it basically comes in three parts.
Part number one is he’s going to eliminate discount pricing. JC Penney had been a very aggressive sales promoter. The second piece of it is he’s going to completely change how they organize merchandise. It’s no longer going to be organized by function – so menswear, housewares, those sorts of things. It’s going to be organized by boutique, so there’s going to be a Levi’s boutique, a Martha Stewart Boutique, a Joe Fresh Boutique and so on.
And it would drop the JC P enney name, they would call it JCP. And he rolls this out over the course of about 12 months across the entire chain of over 1100 stores. What this tells us, he’s so confident in his solution, his strategic transformation, that he doesn’t think it’s worth it to test this out on one or two pilot stores.
CURT NICKISCH: Yeah, he was quoted as saying: “At Apple, we didn’t test anything.”
COREY PHELPS: We didn’t test. Yes. What worked at Apple, he assumed would work at JC Penney. And the critical thing that I think he missed is that JC Penney customers are very different from Apple store customers. In fact, JC Penney customers love the discount. They love the thrill of hunting for a deal.
CURT NICKISCH: Which seems so fundamental to business, right? Understanding your customer. It’s just kind of shocking, I guess, to hear the story.
COREY PHELPS: It is shocking and especially when you consider that Ron Johnson had spent his entire career in retail, so this is someone that had faced, had seen, problems in retailers for decades – for over three decades by the time that he got to JC Penney. So you would expect someone with that degree of experience in that industry wouldn’t make that leap of, well, what worked at Apple stores is going to work at JC Penney stores, but in fact that’s exactly what happened.
CURT NICKISCH: In your book, you essentially suggest four steps that you recommend people use. Tell us about the four steps then.
COREY PHELPS: So in the book we describe what we call the “Four S method,” so four stages, each of which starts with the letter “s”. So the first stage is “state the problem.” Stating the problem is fundamentally about defining what the problem is that you are attempting to solve.
CURT NICKISCH: And you probably would say don’t hurry over that first step or the other three are going to be kind of pointless.
COREY PHELPS: Yeah, that’s exactly the point of of laying out the four s’s. There’s a tremendous amount of desire even amongst senior executives to want to get in and fix the problem. In other words, what’s the trouble? What are the symptoms? What would define success? What are the constraints that we would be operating under? Who owns the problem? And then who are the key stakeholders?
Oftentimes that step is skipped over and we go right into, “I’ve got a hypothesis about what I think the solution is and I’m so obsessed with getting this thing fixed quickly, I’m not going to bother to analyze it particularly well or test the validity of my assumptions. I’m going to go right into implementation mode.”
The second step, what we call “structure the problem” is once you have defined the problem, you need to then start to identify what are the potential causes of that problem. So there are different tools that we talked about in the book that you can structure a problem for analysis. Once you’ve structured the problem for analysis and you’ve conducted the analysis that helps you identify what are the underlying causes that are contributing to it, which will then inform the third stage which is generating solutions for the problem and then testing and evaluating those solutions.
CURT NICKISCH: Is the danger that that third step – generating solutions – is the step that people spend the most time on or have the most fun with?
COREY PHELPS: Yeah. The danger is, is that what that’s naturally what people gravitate towards. So we want to skip over the first two, state and structure.
CURT NICKISCH: As soon as you said it, I was like, “let’s talk about that more.”
COREY PHELPS: Yeah. And we want to jump right into solutioning because people love to talk about their ideas that are going to fix the problem. And that’s actually a useful way to frame a discussion about solutions – we could, or we might do this – because it opens up possibilities for experimentation.
And the problem is that when we often talk about what we could do, we have very little understanding of what the problem is that we’re trying to solve and what are the underlying causes of that problem. Because as you said, solution generation is fun. Look, the classic example is brainstorming. Let’s get a bunch of people in a room and let’s talk about the ideas on how to fix this thing. And again, be deliberate, be disciplined. Do those first stages, the first two stages – state and structure – before you get into the solution generation phase.
CURT NICKISCH: Yeah. The other thing that often happens there is just the lack of awareness of just the cost of the different solutions – how much time, or what they would actually take to do.
COREY PHELPS: Yeah, and again, I’ll go back to that example I used of brainstorming where it’s fun to get a group of people together and talk about our ideas and how to fix the problem. There’s a couple challenges of that. One is what often happens when we do that is we tend to censor the solutions that we come up with. In other words, we ask ourselves, “if I say this idea, people are gonna, think I’m crazy, or people going to say: that’s stupid, that’ll never work, we can’t do that in our organization. It’s going to be too expensive, it’s going to take too much time. We don’t have the resources to do it.”
So brainstorming downside is we we self-sensor, so that’s where you need to have deep insight into your organization in terms of A. what’s going to be feasible, B. what’s going to be desirable on the part of the people that actually have the problem, who you’re trying to solve the problem for and C. from a business standpoint, is it going to be financially attractive for us?
So applying again a set of disciplined criteria that help you choose amongst those ideas for potential solutions. Then the last stage of the process which is selling – because it’s rare in any organization that someone or the group of people that come up with the solution actually have the power and the resources to implement it, so that means they’re going to have to persuade other people to buy into it and want to help.
CURT NICKISCH: Design thinking is another really different method essentially for solving problems or coming up with solutions that just aren’t arrived at through usual problem-solving or usual decision-making processes. I’m just wondering how design thinking comes to play when you’re also outlining these, you know, disciplined methods for stating and solving problems.
COREY PHELPS: For us it’s about choosing the right approach. You know what the potential causes of a problem are. You just don’t know which ones are operating in the particular problem you’re trying to solve. And what that means is that you’ve got a theory – and this is largely the world of strategy consultants – strategy consultants have theories. They have, if you hear them speak, deep understanding of different types of organizational problems, and what they bring is an analytic tool kit that says, “first we’re going to identify all the possible problems, all the possible causes I should say, of this problem. We’re going to figure out which ones are operating and we’re going to use that to come up with a solution.” Then you’ve got problems that you have no idea what the causes are. You’re in a world of unknown unknowns or unk-unks as the operations management people call them.
CURT NICKISCH: That’s terrible.
COREY PHELPS: In other words, you don’t have a theory. So the question is, how do you begin? Well, this is where design thinking can be quite valuable. Design thinking says: first off, let’s find out who are the human beings, the people that are actually experiencing this problem, and let’s go out and let’s talk to them. Let’s observe them. Let’s immerse ourselves in their experience and let’s start to develop an understanding of the causes of the problem from their perspective.
So rather than go into it and say, “I have a theory,” let’s go the design thinking route and let’s actually based upon interactions with users or customers, let’s actually develop a theory. And then we’ll use our new understanding or new insight into the causes of the problem to move into the solution generation phase.
CURT NICKISCH: Problem-solving – we know that that’s something that employers look for when they’re recruiting people. It is one of those phrases that, you know, I’m sure somebody out there has, has the title at a company Chief Problem Solver instead of CEO, right? So, it’s almost one of those phrases that so over used it can lose its meaning.
And if you are being hired or you’re trying to make a case for being on a team that’s tackling a problem, how do you make a compelling case that you are a good problem solver? How can you actually show it?
COREY PHELPS: It’s a great question and then I have two answers to this question. So one is, look at the end of the day, the proof is in the pudding. In other words, can you point to successful solutions that you’ve come up with – solutions that have actually been effective in solving a problem? So that’s one.
The second thing is can you actually articulate how you approach problem-solving? In other words, do you follow a method or are you reinventing the wheel every time you solve a problem? Is it an ad hoc approach? And I think this issue really comes to a head when it comes to the world of strategy consulting firms when they recruit. For example, Mckinsey, you’ve got the Mckinsey problem-solving test, which is again, a test that’s actually trying to elicit the extent to which people are good applicants are good at solving problems
And then you’ve got the case interview. And in the case interview, what they’re looking at is do you have a mastery over certain tools. But what they’re really looking at is, are you actually following a logical process to solve this problem? Because again, what they’re interested in is finding- to your point – people that are going to be good at solving complex organizational problems. So they’re trying to get some evidence that they can demonstrate that they’re good at it and some evidence that they follow a deliberate process.
CURT NICKISCH: So even if you’re not interviewing at a consulting firm, that’s a good approach, to show your thinking, show your process, show the questions you ask?
COREY PHELPS: Yeah, and to your point earlier, at least if we look at what recruiters of MBA students are saying these days, they’re saying, for example, according to the FT’s recent survey, they’re saying that we want people with really good problem solving skills, and by the same token, we find that that’s a skill that’s difficult for us to recruit for. And that reinforces our interest in this area because the fundamental idea for the book is to give people a method. We’re trying to equip not just MBA students but everybody that’s going to face complex problems with a toolkit to solve them better.
CURT NICKISCH: Corey, this has been really great. Thank you.
COREY PHELPS: Thanks for the opportunity. I appreciate it.
CURT NICKISCH: That’s Corey Phelps. He teaches strategy at McGill University, and he co-wrote the book “Cracked It: How to Solve Big Problems and Sell Solutions Like Top Strategy Consultants.”
This episode was produced by Mary Dooe. We got technical help from Rob Eckhardt. Adam Buchholz is our audio product manager.
Thanks for listening to the HBR IdeaCast. I’m Curt Nickisch.
- Subscribe On:
Latest in this series
This article is about decision making and problem solving, partner center.
- Bipolar Disorder
- Therapy Center
- When To See a Therapist
- Types of Therapy
- Best Online Therapy
- Best Couples Therapy
- Best Family Therapy
- Managing Stress
- Sleep and Dreaming
- Understanding Emotions
- Self-Improvement
- Healthy Relationships
- Student Resources
- Personality Types
- Guided Meditations
- Verywell Mind Insights
- 2023 Verywell Mind 25
- Mental Health in the Classroom
- Editorial Process
- Meet Our Review Board
- Crisis Support
Overview of the Problem-Solving Mental Process
Kendra Cherry, MS, is a psychosocial rehabilitation specialist, psychology educator, and author of the "Everything Psychology Book."
:max_bytes(150000):strip_icc():format(webp)/IMG_9791-89504ab694d54b66bbd72cb84ffb860e.jpg)
Rachel Goldman, PhD FTOS, is a licensed psychologist, clinical assistant professor, speaker, wellness expert specializing in eating behaviors, stress management, and health behavior change.
:max_bytes(150000):strip_icc():format(webp)/Rachel-Goldman-1000-a42451caacb6423abecbe6b74e628042.jpg)
- Identify the Problem
- Define the Problem
- Form a Strategy
- Organize Information
- Allocate Resources
- Monitor Progress
- Evaluate the Results
Frequently Asked Questions
Problem-solving is a mental process that involves discovering, analyzing, and solving problems. The ultimate goal of problem-solving is to overcome obstacles and find a solution that best resolves the issue.
The best strategy for solving a problem depends largely on the unique situation. In some cases, people are better off learning everything they can about the issue and then using factual knowledge to come up with a solution. In other instances, creativity and insight are the best options.
It is not necessary to follow problem-solving steps sequentially, It is common to skip steps or even go back through steps multiple times until the desired solution is reached.
In order to correctly solve a problem, it is often important to follow a series of steps. Researchers sometimes refer to this as the problem-solving cycle. While this cycle is portrayed sequentially, people rarely follow a rigid series of steps to find a solution.
The following steps include developing strategies and organizing knowledge.
1. Identifying the Problem
While it may seem like an obvious step, identifying the problem is not always as simple as it sounds. In some cases, people might mistakenly identify the wrong source of a problem, which will make attempts to solve it inefficient or even useless.
Some strategies that you might use to figure out the source of a problem include :
- Asking questions about the problem
- Breaking the problem down into smaller pieces
- Looking at the problem from different perspectives
- Conducting research to figure out what relationships exist between different variables
2. Defining the Problem
After the problem has been identified, it is important to fully define the problem so that it can be solved. You can define a problem by operationally defining each aspect of the problem and setting goals for what aspects of the problem you will address
At this point, you should focus on figuring out which aspects of the problems are facts and which are opinions. State the problem clearly and identify the scope of the solution.
3. Forming a Strategy
After the problem has been identified, it is time to start brainstorming potential solutions. This step usually involves generating as many ideas as possible without judging their quality. Once several possibilities have been generated, they can be evaluated and narrowed down.
The next step is to develop a strategy to solve the problem. The approach used will vary depending upon the situation and the individual's unique preferences. Common problem-solving strategies include heuristics and algorithms.
- Heuristics are mental shortcuts that are often based on solutions that have worked in the past. They can work well if the problem is similar to something you have encountered before and are often the best choice if you need a fast solution.
- Algorithms are step-by-step strategies that are guaranteed to produce a correct result. While this approach is great for accuracy, it can also consume time and resources.
Heuristics are often best used when time is of the essence, while algorithms are a better choice when a decision needs to be as accurate as possible.
4. Organizing Information
Before coming up with a solution, you need to first organize the available information. What do you know about the problem? What do you not know? The more information that is available the better prepared you will be to come up with an accurate solution.
When approaching a problem, it is important to make sure that you have all the data you need. Making a decision without adequate information can lead to biased or inaccurate results.
5. Allocating Resources
Of course, we don't always have unlimited money, time, and other resources to solve a problem. Before you begin to solve a problem, you need to determine how high priority it is.
If it is an important problem, it is probably worth allocating more resources to solving it. If, however, it is a fairly unimportant problem, then you do not want to spend too much of your available resources on coming up with a solution.
At this stage, it is important to consider all of the factors that might affect the problem at hand. This includes looking at the available resources, deadlines that need to be met, and any possible risks involved in each solution. After careful evaluation, a decision can be made about which solution to pursue.
6. Monitoring Progress
After selecting a problem-solving strategy, it is time to put the plan into action and see if it works. This step might involve trying out different solutions to see which one is the most effective.
It is also important to monitor the situation after implementing a solution to ensure that the problem has been solved and that no new problems have arisen as a result of the proposed solution.
Effective problem-solvers tend to monitor their progress as they work towards a solution. If they are not making good progress toward reaching their goal, they will reevaluate their approach or look for new strategies .
7. Evaluating the Results
After a solution has been reached, it is important to evaluate the results to determine if it is the best possible solution to the problem. This evaluation might be immediate, such as checking the results of a math problem to ensure the answer is correct, or it can be delayed, such as evaluating the success of a therapy program after several months of treatment.
Once a problem has been solved, it is important to take some time to reflect on the process that was used and evaluate the results. This will help you to improve your problem-solving skills and become more efficient at solving future problems.
A Word From Verywell
It is important to remember that there are many different problem-solving processes with different steps, and this is just one example. Problem-solving in real-world situations requires a great deal of resourcefulness, flexibility, resilience, and continuous interaction with the environment.
Get Advice From The Verywell Mind Podcast
Hosted by therapist Amy Morin, LCSW, this episode of The Verywell Mind Podcast shares how you can stop dwelling in a negative mindset.
Follow Now : Apple Podcasts / Spotify / Google Podcasts
You can become a better problem solving by:
- Practicing brainstorming and coming up with multiple potential solutions to problems
- Being open-minded and considering all possible options before making a decision
- Breaking down problems into smaller, more manageable pieces
- Asking for help when needed
- Researching different problem-solving techniques and trying out new ones
- Learning from mistakes and using them as opportunities to grow
It's important to communicate openly and honestly with your partner about what's going on. Try to see things from their perspective as well as your own. Work together to find a resolution that works for both of you. Be willing to compromise and accept that there may not be a perfect solution.
Take breaks if things are getting too heated, and come back to the problem when you feel calm and collected. Don't try to fix every problem on your own—consider asking a therapist or counselor for help and insight.
If you've tried everything and there doesn't seem to be a way to fix the problem, you may have to learn to accept it. This can be difficult, but try to focus on the positive aspects of your life and remember that every situation is temporary. Don't dwell on what's going wrong—instead, think about what's going right. Find support by talking to friends or family. Seek professional help if you're having trouble coping.
Davidson JE, Sternberg RJ, editors. The Psychology of Problem Solving . Cambridge University Press; 2003. doi:10.1017/CBO9780511615771
Sarathy V. Real world problem-solving . Front Hum Neurosci . 2018;12:261. Published 2018 Jun 26. doi:10.3389/fnhum.2018.00261
By Kendra Cherry, MSEd Kendra Cherry, MS, is a psychosocial rehabilitation specialist, psychology educator, and author of the "Everything Psychology Book."
- Courses for Individuals
Understanding and Solving Complex Business Problems
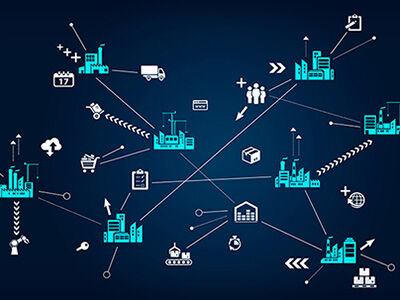
Management and Leadership
Certificate Credits
- Operations
- Systems Thinking
- Participants
Course Highlights
- Discover MIT's unique, powerful, and integrative System Dynamics approach to assess problems that will not go away
- Experience the Beer Game, which simulates the supply chain of the beer industry
- Learn a new way of thinking about and resolving complex, persistent problems that emerge from change
- Earn a certificate of course completion from the MIT Sloan School of Management
Why attend Understanding and Solving Complex Business Problems?
Systems thinking was designed to improve people's ability to manage organizations comprehensively in a volatile global environment. It offers managers a framework for understanding complex situations and the dynamics those situations produce. Systems thinking is a response to the rapid changes in technology, population, and economic activity that are transforming the world, and as a way to deal with the ever-increasing complexity of today's business.
Senior managers can use systems thinking to design policies that lead their organizations to high performance. The program is intended to give participants the tools and confidence to manage organizations with full understanding and solid strategy.
Course experience
This complex problem-solving course introduces participants to MIT's unique, powerful, and integrative System Dynamics approach to assess problems that will not go away and to produce the results they want. Through exercises and simulation models, participants experience the long-term side effects and impacts of decisions and understand the ways in which performance is tied to structures and policies.
Sample Schedule—Subject to Change
This program is designed for executives with decision-making responsibility who are looking for fresh ideas to resolve organizational problems.
Past participants have included
- VPs and EVPs
- Corporate planners and strategists
- Senior Project Managers
- Product Development Managers
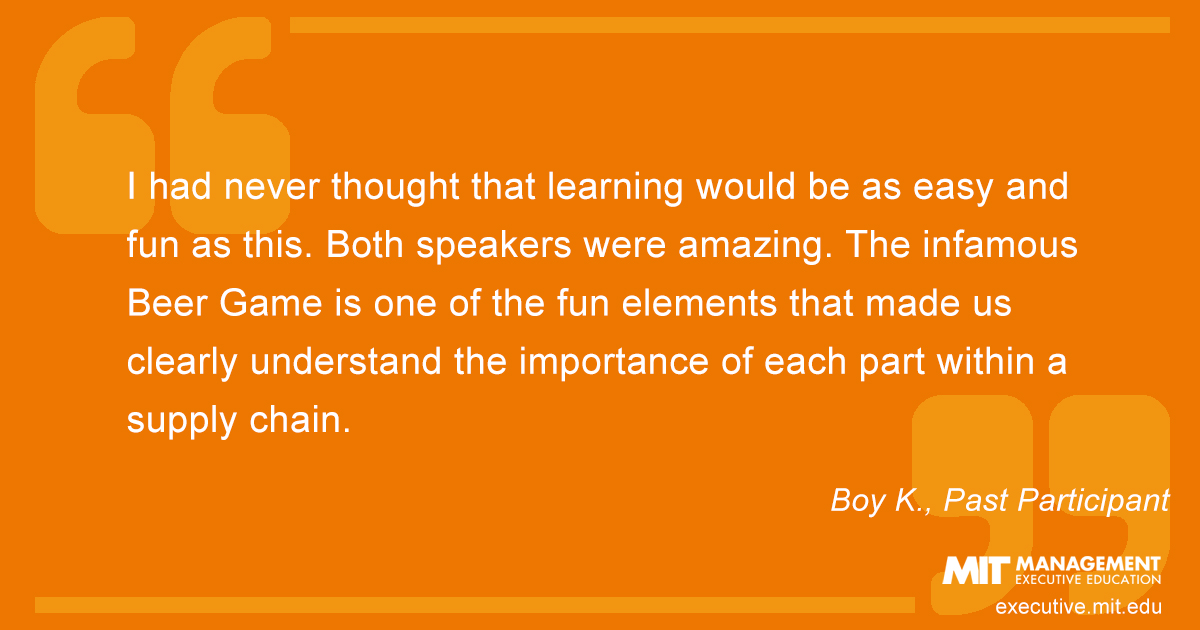
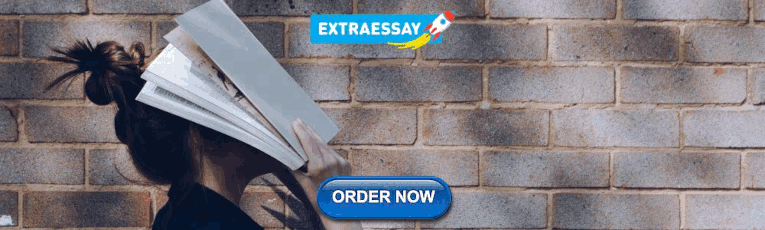
Request Course Information
Receive email updates related to this course, including faculty news and additional offering dates.
Review our Privacy Policy.
This program is designed to empower you to analyze complex problems in any area by using powerful yet very simple tools which are also very easy to use in real world, I enjoyed it a lot.
—Jia X.
Enroll Now!
What mit’s beer game teaches about panic hoarding, mit’s ‘beer game’ shows humans are weakest link in supply chains, the beer game, john sterman on system dynamics, how the ‘beer game’ helps retailers solve toilet paper crisis, course offerings.
Fostering complex problem solving for diverse learners: engaging an ethos of intentionality toward equitable access
- Published: 14 April 2020
- Volume 68 , pages 679–702, ( 2020 )
Cite this article
- Krista D. Glazewski 1 &
- Peggy A. Ertmer 2 , 3
1747 Accesses
6 Citations
2 Altmetric
Explore all metrics
Complex problem solving is an effective means to engage students in disciplinary content while also furnishing critical non-cognitive and life skills. Despite increased adoption of complex problem-solving methods in K-12 classrooms today (e.g., case-, project-, or problem-based learning), we know little about how to make these approaches accessible to linguistically and culturally diverse (LCD) students. In this paper, we promote a conceptual framework, based on an ethos of intentionality , that supports culturally responsive teaching (CRT). We provide specific questions to guide teachers’ implementation of an ethos of intentionality, through critical reflection and meaningful action, and discuss a framework for culturally relevant practice that operationalizes key central tenets (e.g., high expectations, cultural competence, and critical consciousness). Finally, we include strategies that can help teachers and designers translate the principles of the CRT framework into action with a specific focus on complex problem solving in classrooms.
This is a preview of subscription content, log in via an institution to check access.
Access this article
Price includes VAT (Russian Federation)
Instant access to the full article PDF.
Rent this article via DeepDyve
Institutional subscriptions
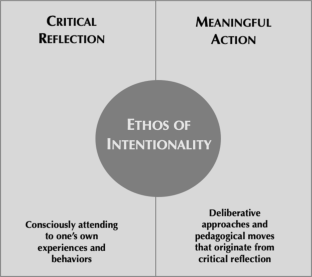
Similar content being viewed by others
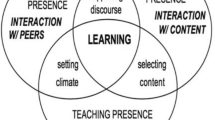
Investigating blended learning interactions in Philippine schools through the community of inquiry framework
Creating a Motivating Classroom Environment
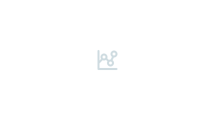
Distributed Scaffolding: Scaffolding Students in Classroom Environments
Aronson, B., & Laughter, J. (2015). The theory and practice of culturally relevant education: A synthesis of research across content areas. Review of Educational Research, 86 (1), 163–206. https://doi.org/10.3102/0034654315582066 .
Article Google Scholar
Amaral, O. M., Garrison, L., & Klentschy, M. (2002). Helping English learners increase achievement through inquiry-based science instruction. Bilingual Research Journal, 26 (2), 213–219. https://doi.org/10.1080/15235882.2002.10668709 .
Baker, B. D., & Corcoran, S. P. (2012). The stealth inequalities of school funding: How local tax systems and state aid formulas undermine equality . Washington, DC: Center for American Progress. Retrieved March 14, 2020 from http://www.americanprogress.org/wpcontent/uploads/2012/09/StealthInequities.pdf .
Barton, K. C., & McCully, A. W. (2012). Trying to see things differently: Northern Ireland students struggle to understand alternative historical perspectives. Theory and Research in Social Education, 40 (4), 371–408. https://doi.org/10.1080/00933104.2012.710928 .
Bell, P., Van Horne, K., & Cheng, B. H. (2017). Special issue: Designing learning environments for equitable disciplinary identification. Journal of the Learning Sciences, 26 , 367–375. https://doi.org/10.1080/10508406.2017.1336021 .
Belland, B. R. (2010). Portraits of middle school students constructing evidence-based arguments during problem-based learning: The impact of computer-based scaffolds. Educational Technology Research and Development, 58 (3), 285–309. https://doi.org/10.1007/s11423-009-9139-4 .
Belland, B. R., Ertmer, P. A., & Simons, K. D. (2006). Perceptions of the value of problem-based learning among students with special needs and their teachers. Interdisciplinary Journal of Problem-Based Learning, 1 (2), 1–18. https://doi.org/10.7771/1541-5015.1024 .
Belland, B. R., Glazewski, K. D., & Ertmer, P. A. (2009). Inclusion and problem-based learning: Roles of students in mixed-ability groups. Research on Middle Level Education , 32 (9), 1–19. https://doi.org/10.1080/19404476.2009.11462062 .
Bilgin, I., Karakuyu, Y., & Ay, Y. (2015). The effects of project-based learning on undergraduate students’ achievement and self-efficacy beliefs towards science teaching. Eurasia Journal of Mathematics, Science and Technology Education, 11 (3), 469–477. https://doi.org/10.12973/eurasia.2014.1015a .
Birr Moje, E., McIntosh Ciechanowski, K., Kramer, K., Ellis, L., Carrillo, R., & Collazo, T. (2004). Working toward third space in content area literacy: An examination of everyday funds of knowledge and discourse. Reading Research Quarterly, 39 (1), 38–70. https://doi.org/10.1598/rrq.39.1.4 .
Blasco, M. (2015). Making the tacit explicit: Rethinking culturally inclusive pedagogy in international student academic adaptation. Pedagogy, Culture and Society, 23 (1), 85–106.
Bogard, T., Liu, M., & Chiang, Y.-H. V. (2018). Thresholds of knowledge development in complex problem solving: A multiple case-study of advanced learners' cognitive processes. Educational Technology Research and Development, 61 , 465–503.
Bonner, E. P. (2014). Investigating practices of highly successful mathematics teachers of traditionally underserved students. Educational Studies in Mathematics, 86 (3), 377–399. https://doi.org/10.1007/s10649-014-9533-7 .
Brown, B. A. (2006). “It isn’t no slang that can be said about this stuff”: Language, identity, and appropriating science discourse. Journal of Research in Science Teaching, 43 (1), 96–126. https://doi.org/10.1002/tea.20096 .
Brown, B. A., Reveles, J. M., & Kelly, G. J. (2005). Scientific literacy and discursive identity: A theoretical framework for understanding science learning. Science Education, 89 (5), 779–802. https://doi.org/10.1002/sce.20069 .
Brown, J. C., Ring-Whalen, E. A., Roehrig, G. H., & Ellis, J. (2018). Advancing culturally responsive science education in secondary classrooms through an induction course. International Journal of Designs for Learning, 9 (1), 14–33.
Calabrese Barton, A., & Tan, E. (2010). We be Burnin’! Agency, identity, and science learning. Journal of the Learning Sciences, 19 (2), 187–229. https://doi.org/10.1080/10508400903530044 .
Danowitz, M. A., & Tuitt, F. (2011). Enacting inclusivity through engaged pedagogy: A higher education perspective. Equity and Excellence in Education, 44 (1), 40–56. https://doi.org/10.1080/10665684.2011.539474 .
Derry, S. J., Hmelo-Silver, C. E., Nagarajan, A., Chernobilsky, E., & Beitzel, B. (2006). Cognitive transfer revisited: Can we exploit new media to solve old problems on a large scale? Journal of Educational Computing Research , 35 , 145–162. https://doi.org/10.2190/0576-R724-T149-5432 .
Deslauriers, L., Schelew, E., & Wieman, C. (2011). Improved learning in a large-enrollment physics class. Science, 332 (6031), 862–864. https://doi.org/10.1126/science.1201783 .
Dolan, J. E. (2016). Splicing the divide: A review of research on the evolving digital divide among K-12 students. Journal of Research on Technology in Education, 48 (1), 16–37. https://doi.org/10.1080/15391523.2015.1103147 .
Eberlein, T., Kampmeier, J., Minderhout, V., Moog, R. S., Platt, T., Varma-Nelson, P., et al. (2008). Pedagogies of engagement in science: A comparison of PBL, POGIL, and PLTL. Biochemistry and Molecular Biology Education, 36 (4), 262–273. https://doi.org/10.1002/bmb.20204 .
Ertmer, P. A., & Glazewski, K. D. (2018). Problem-based learning: Essential design considerations. In R. A. Reiser & J. V. Dempsey (Eds.), Trends and issues in instructional design and technology (Vol. 4, pp. 286–295). New York: Pearson.
Google Scholar
Ertmer, P. A., & Glazewski, K. (2019). Scaffolding in PBL environments: Structuring and problematizing relevant task features. In N. Dabbah, W. Hung, & M. Moallem (Eds.), Handbook of problem-based learning (pp. 321–342). Hoboken, NY: Wiley Publishing.
Chapter Google Scholar
Ertmer, P. A., & Simons, K. D. (2006). Jumping the PBL implementation hurdle: Supporting the efforts of K-12 teachers. Interdisciplinary Journal of Problem-Based Learning . https://doi.org/10.7771/1541-5015.1005 .
Esteban-Guitart, M., & Moll, L. C. (2014). Funds of identity: A new concept based on the Funds of Knowledge approach. Culture & Psychology, 20 (1), 31–48.
Gallagher, S. A. (2005). Adapting problem-based learning for gifted students. In F. A. Karnes & S. M. Bean (Eds.), Methods and materials for teaching the gifted (pp. 285–311). Waco, TX: Prufrock Press.
Gallagher, S. A., & Gallagher, J. J. (2013). Using problem-based learning to explore unseen academic potential. Interdisciplinary Journal of Problem-Based Learning, 7 (1), 3–15. https://doi.org/10.7771/1541-5015.1322 .
Gay, G. (2001). Preparing for culturally responsive teaching. Journal of Teacher Education, 53 (2), 106–116. https://doi.org/10.1177/0022487104267587 .
Gay, G. (2010). Culturally responsive teaching: Theory, research, and practice . New York: Teachers College Press.
Gay, G. (2013). Teaching to and through cultural diversity. Curriculum Inquiry, 43 (1), 48–70. https://doi.org/10.1111/curi.12002 .
Glazewski, K. D., & Hmelo-Silver, C. E. (2019). Scaffolding and supporting use of information for ambitious learning practices. Information and Learning Sciences , 120 (1/2), 39–58. https://doi.org/10.1108/ILS-08-2018-0087 .
Goldberg, T. (2013). It’s in my veins: Identity and disciplinary practice in students’ discussions of a historical issue. Theory and Research in Social Education, 41 (1), 33–64. https://doi.org/10.1080/00933104.2012.757265 .
Goldman, S. R. (2003). Learning in complex domains: When and why do multiple representations help? Learning and Instruction, 13 (2), 239–244. https://doi.org/10.1016/s0959-4752(02)00023-3 .
González, N., Moll, L. C., & Amanti, C. (Eds.). (2005). Funds of knowledge: Theorizing practices in households, communities, and classrooms . Mahwah: Lawrence Erlbaum.
Gorski, P. C. (2008). Insisting on digital equity: Reframing the dominant discourse on multicultural education and technology. Urban Education, 44 , 348–364. https://doi.org/10.1177/0042085908318712 .
Gray, C. M., & Boling, E. (2016). Inscribing ethics and values in designs for learning: A problematic. Educational Technology Research and Development, 64 , 969–1001. https://doi.org/10.1007/s11423-016-9478-x .
Gutiérrez, K. D. (2008). Developing a sociocritical literacy in the third space. Reading Research Quarterly, 43 (2), 148–164. https://doi.org/10.1598/rrq.43.2.3 .
Gutierrez, K. D., Baquedano-Lopez, P., & Tegeda, C. (1999). Rethinking diversity: Hybridity and hybrid language practices in the third space. Mind, Culture, and Activity, 6 , 286–303. https://doi.org/10.1080/10749039909524733 .
Hanney, R., & Savin-Baden, M. (2013). The problem of projects: Understanding the theoretical underpinnings of project-led PBL. London Review of Education, 11 (1), 7–19. https://doi.org/10.1080/14748460.2012.761816 .
Hartman, P., Renguette, C., & Seig, M. T. (2018). Problem-based teacher-mentor education: Fostering literacy acquisition in multicultural classrooms. Interdisciplinary Journal of Problem-Based Learning . https://doi.org/10.7771/1541-5015.1659 .
Haruehansawasin, S., & Kiattikomol, P. (2018). Scaffolding in problem-based learning for low-achieving learners. The Journal of Educational Research, 111 (3), 363–370. https://doi.org/10.1080/00220671.2017.1287045 .
Hasni, A., Bousadra, F., Belletête, V., Benabdallah, A., Nicole, M. C., & Dumais, N. (2016). Trends in research on project-based science and technology teaching and learning at K–12 levels: A systematic review. Studies in Science Education, 52 (2), 199–231. https://doi.org/10.1080/03057267.2016.1226573 .
Hmelo-Silver, C. E. (2004). Problem-based learning: What and how do students learn? Educational Psychology Review, 16 (3), 235–266. https://doi.org/10.1023/B:EDPR.0000034022.16470.f3 .
Hmelo-Silver, C. E. (2013). Creating a learning space in problem-based learning. Interdisciplinary Journal of Problem-Based Learning . https://doi.org/10.7771/1541-5015.1334 .
Hmelo-Silver, C. E., & DeSimone, C. (2013). Problem-based learning: An instructional model of collaborative learning. In C. E. Hmelo-Silver, C. A. Chinn, C. K. K. Chan, & A. O’Donnell (Eds.), The international handbook of collaborative learning (pp. 382–398). New York: Routledge.
Hmelo-Silver, C. E., Duncan, R. G., & Chinn, C. A. (2007). Scaffolding and achievement in problem-based and inquiry learning: A response to Kirschner, Sweller, and Clark. Educational Psychologist, 42 (2), 99–107. https://doi.org/10.1080/00461520701263368 .
Hooks, B. (1994). Teaching to transgress: Education as the practice of freedom . New York: Routledge.
Howard, T. C. (2003). Culturally relevant pedagogy: Ingredients for critical teacher reflection. Theory into Practice, 42 (3), 195–202. https://doi.org/10.1207/s15430421tip4203_5 .
Hung, W. (2013). Problem-based learning: A learning environment for enhancing learning transfer. New Directions for Adult and Continuing Education, 137 , 28–38. https://doi.org/10.1002/ace .
Hwang, G. J., Chiu, L. Y., & Chen, C. H. (2015). A contextual game-based learning approach to improving students’ inquiry-based learning performance in social studies courses. Computers and Education, 81 , 13–25. https://doi.org/10.1016/j.compedu.2014.09.006 .
Jonassen, D. H. (2000). Toward a design theory of problem solving. Educational Technology Research and Development, 86 (2), 75–85. https://doi.org/10.1007/BF02300500 .
Jonassen, D., Strobel, J., & Lee, C. B. (2006). Everyday problem solving in engineering: Lessons for engineering educators. Journal of engineering education, 95 (2), 139–151. https://doi.org/10.1002/j.2168-9830.2006.tb00885.x .
Johnson, C. C. (2011). The road to culturally relevant science: Exploring how teachers navigate change in pedagogy. Journal of Research in Science Teaching, 48 (2), 170–198. https://doi.org/10.1002/tea.20405 .
Kim, H. (2016). Inquiry-based science and technology enrichment program for middle school-aged female students. Journal of Science Education and Technology, 25 , 174–186. https://doi.org/10.1007/s10956-015-9584-2 .
Kim, N. J., Belland, B. R., Lefler, M., Andreasen, L., Walker, A., & Axelrod, D. (2019). Computer-based scaffolding targeting individual versus groups in problem-centered instruction for STEM education: Meta-analysis. Educational Psychology Review . https://doi.org/10.1007/s10648-019-09502-3 .
Kolodner, J. L., Camp, P. J., Crismond, D., Fasse, J. G., Holbrook, J., Puntambekar, S., et al. (2003). Problem-based learning meets case-based reasoning in the middle school science classroom: Putting learning by design into practice. Journal of the Learning Sciences, 12 , 495–547. https://doi.org/10.1207/S15327809JLS1204_2 .
Komis, V., Romero, M., & Misirli, A. (2016). A scenario-based approach for designing educational robotics activities for co-creative problem solving. In International Conference EduRobotics 2016 (pp. 158–169). Cham: Springer.
Krapp, A., & Prenzel, M. (2011). Research on interest in science: Theories, methods, and findings. International Journal of Science Education, 33 (1), 27–50. https://doi.org/10.1080/09500693.2010.518645 .
Ladson-Billings, G. (1995). Toward a theory of culturally relevant pedagogy. American Educational Research Journal, 32 (3), 465–491.
Ladson-Billings, G. (2006). Liberatory consequences of literacy: A case of culturally relevant instruction for African American students. The Journal of Negro Education, 61 (3), 378–391. https://www.jstor.org/stable/2295255 .
Ladson-Billings, G. (2007). Pushing past the achievement gap: An essay on the language of deficit. Journal of Negro Education, 76 (3), 316–323.
Ladson-Billings, G. (2015). Culturally relevant pedagogy 2.0: a.k.a the Remix. Harvard Educational Review, 84 (1), 74–84. https://doi.org/10.17763/haer.84.1.p2rj131485484751 .
Lave, J., & Wenger, E. (1991). Situated learning: Legitimate peripheral participation . Cambridge: Cambridge University Press.
Book Google Scholar
Lazonder, A. W., & Harmsen, R. (2016). Meta-analysis of inquiry-based learning: Effects of guidance. Review of Educational Research, 86 (3), 681–718. https://doi.org/10.3102/0034654315627366 .
Lee, O. (2001). Culture and language in science education: What do we know and what do we need to know? Journal of Research in Science Teaching, 38 (5), 499–501. https://doi.org/10.1002/tea.1015 .
Lee, O. (2004). Teacher change in beliefs and practices in science and literacy instruction with English language learners. Journal of Research in Science Teaching, 41 (1), 65–93. https://doi.org/10.1002/tea.10125 .
Lee, O., & Buxton, C. A. (2013). Integrating science and English proficiency for English Language Learners. Theory into Practice, 52 , 36–42. https://doi.org/10.1007/s11423-016-9478-x .
Lee, O., Buxton, C., Lewis, S., & LeRoy, K. (2006). Science inquiry and student diversity: Enhanced abilities and continuing difficulties after an instructional intervention. Journal of Research in Science Teaching, 43 (7), 607–636. https://doi.org/10.1002/tea.20141 .
Lee, O., & Fradd, S. H. (1998). Science for all, including students from non-English language backgrounds. Educational Researcher, 27 (4), 12–21.
Lee, O., Quinn, H., & Valdés, G. (2013). Science and language for English language learners in relation to Next Generation Science Standards and with implications for Common Core State Standards for English language arts and mathematics. Educational Researcher, 42 (4), 223–233. https://doi.org/10.3102/0013189X13480524 .
Liu, M., Hsieh, P., Cho, Y., & Schallert, D. L. (2006). Middle school students’ self-efficacy, attitudes, and achievement in a computer-enhanced problem-based learning environment. Journal of Interactive Learning Research, 17 (3), 225–242.
Liu, M., Liu, S., Pan, Z., Zou, W., & Li, C. (2019). Examining science learning and attitude by at-risk students after they used a multimedia-enriched problem-based learning environment. Interdisciplinary Journal of Problem-Based Learning, 13 (1), 1–11. https://doi.org/10.7771/1541-5015.1752 .
Llosa, L., Lee, O., Jiang, F., Haas, A., O’Connor, C., Van Booven, C. D., et al. (2016). Impact of a large-scale science intervention focused on English Language Learners. American Educational Research Journal, 53 (2), 395–424. https://doi.org/10.3102/0002831216637348 .
Lopez, A. E. (2011). Culturally relevant pedagogy and critical literacy in diverse English classrooms: A case study of a secondary English teacher’s activism and agency. English Teaching: Practice and Critique, 10 (4), 75–93.
Lynch, S., Kuipers, J., Pyke, C., & Szesze, M. (2005). Examining the effects of a highly rated science curriculum unit on diverse students: Results from a planning grant. Journal of Research in Science Teaching, 42 (8), 912–946. https://doi.org/10.1002/tea.20080 .
Maker, C. J., & Zimmerman, R. (2008). Problem solving in a complex world: Integrating DISCOVER, TASC, and PBL in a teacher education project. Gifted Education International, 24 , 160–178. https://doi.org/10.1177/026142940802400305 .
Milner, H. R., IV. (2010). What does teacher education have to do with teaching? Implications for diversity studies. Journal of Teacher Education, 61 (1–2), 118–131. https://doi.org/10.1177/0022487109347670 .
Moje, E. B., Collazo, T., Carrillo, R., & Marx, R. W. (2001). “Maestro, what is ‘quality’?”: Language, literacy, and discourse in project-based science. Journal of Research in Science Teaching, 38 (4), 469–498. https://doi.org/10.1002/tea.1014 .
Morgan, K., & Brooks, D. W. (2012). Investigating a method of scaffolding student-designed experiments. Journal of Science Education and Technology, 21 (4), 513–522. https://doi.org/10.1007/s10956-011-9343-y .
Morrison, K. A., Robbins, H. H., & Rose, D. G. (2008). Operationalizing culturally relevant pedagogy: A synthesis of classroom-based research. Equity & Excellence in Education, 41 , 433–452. https://doi.org/10.1080/10665680802400006 .
National Center for Education Statistics. (2019a). Fast facts: Back to school statistics . https://nces.ed.gov/fastfacts/display.asp?id=372 .
National Center for Education Statistics. (2019b). Fast facts: English language learners . https://nces.ed.gov/FastFacts/display.asp?id=96 .
National Commission on Teaching and America’s Future. (2004). Fifty years after Brown v. Board of Education: A two-tiered education system . Washington, DC: Author.
National Council for the Social Studies (NCSS). (2013). The college, career, and civic life (C3) framework for Social Studies state standards: Guidance for enhancing the rigor of K- 12 civics, economics, geography, and history . https://www.socialstudies.org/system/files/c3/C3-Framework-for-Social-Studies.pdf .
NGSS Lead States. (2013). Next generation science standards: For states, by states . Washington, DC: National Academies Press. www.nextgenscience.org .
Paris, D. (2012). Culturally sustaining pedagogy: A needed change in stance, terminology, and practice. Educational Researcher, 41 (3), 93–97.
Pedersen, S., & Liu, M. (2002). The transfer of problem-solving skills from a problem-based learning environment: The effect of modeling an expert’s cognitive processes. Journal of Research on Technology in Education, 35 (2), 303–320. https://doi.org/10.1080/15391523.2002.10782388 .
Piaget, J. (1969). The psychology of the child . New York: Basic Books.
Ritzhaupt, A. D., Liu, F., Dawson, K., & Barron, A. E. (2014). Differences in student information and communication technology literacy based on socio-economic status, ethnicity, and gender. Journal of Research on Technology in Education, 45 , 291–307. https://doi.org/10.1080/15391523.2013.10782607 .
Saleh, A., Hmelo-Silver, C., Glazewski, K., Chen, Y., Mott, B., Rowe, J. P., & Lester, J. C. (2019). Collaborative inquiry play: A design case to frame integration of collaborative problem solving with story-centric games. Information and Learning Sciences , 120 (7/8), 547–566. https://doi.org/10.1108/ILS-03-2019-0024 .
Savin-Baden, M. (2016). The impact of transdisciplinary threshold concepts on student engagement in Problem-Based Learning: A conceptual synthesis. Interdisciplinary Journal of Problem-Based Learning, 10 (2), 9–13. https://doi.org/10.7771/1541-5015.1588 .
Schmidt, H. G., van der Molen, H. T., te Winkel, W. W. R., & Wijnen, W. H. F. W. (2009). Constructivist, problem-based learning does work: A meta-analysis of curricular comparisons involving a single medical school. Educational Psychologist, 44 (4), 227–249. https://doi.org/10.1080/00461520903213592 .
Simons, K. D., & Klein, J. D. (2007). The impact of scaffolding and student achievement levels in a problem-based learning environment. Instructional Science, 35 (1), 41–72. https://doi.org/10.1007/s11251-006-9002-5 .
Singh, K., Granville, M., & Dika, S. (2002). Mathematics and science achievement: Effects of motivation, interest, and academic engagement. Journal of Educational Research, 95 (6), 323–332. https://doi.org/10.1080/00220670209596607 .
Slavin, R. E. (1987). Ability grouping and student achievement in elementary schools: A best-evidence synthesis. Review of Educational Research, 57 (3), 293–336. https://doi.org/10.3102/00346543057003293 .
Sorge, C. (2007). What happens? Relationship of age and gender with science attitudes from elementary to middle school. Science Educator, 16 (2), 33–37.
Souto-Manning, M. (2009). Negotiating culturally responsive pedagogy through multicultural children’s literature: Towards critical democratic literacy practices in a first grade classroom. Journal of Early Childhood Literacy, 9 (1), 50–74. https://doi.org/10.1177/1468798408101105 .
Spector, J. M. (2008). A modeling methodology for assessing learning in complex domains. In P. Blumschein, W. Hung, & D. Jonassen (Eds.), Model-based approaches to learning: Using systems, models, and simulations to improve understanding and problem solving in complex domains (pp. 163–177). Rotterdam, NL: Sense Publishers.
Spratt, J., & Florian, L. (2015). Inclusive pedagogy: From learning to action. Supporting each individual in the context of “everybody”. Teaching and Teacher Education, 49 , 89–96. https://doi.org/10.1016/j.tate.2015.03.006 .
Tawfik, A., & Kolodner, J. L. (2016). Systematizing scaffolding for problem-based learning: A view from case-based reasoning. Interdisciplinary Journal of Problem-Based Learning, 10 (1), 5–6. https://doi.org/10.7771/1541-5015.1608 .
Ushomirsky, N., & Williams, D. (2015). Funding gaps 2015: Too many states still spend less on educating students who need the most . Washington, DC.
Van Horne, K., & Bell, P. (2017). Youth disciplinary identification during participation in contemporary project-based science investigations in school. Journal of the Learning Sciences, 26 (3), 437–476. https://doi.org/10.1080/10508406.2017.1330689 .
Wallace, S., Banks, T., Sedas, M., Glazewski, K., Brush, T. A., & McKay, C. (2017). What will keep the fish alive? Exploring the intersections of designing, making, and inquiry among middle school learners. International Journal of Designs for Learning, 8 (1), 11–21. https://doi.org/10.14434/ijdl.v8i1.22668 .
Warschauer, M. (2011). A literacy approach to the digital divide. Cadernos de Letras, 28 , 5–19.
Watson, W. E., Johnson, L., & Zgourides, G. D. (2002). The influence of ethnic diversity on leadership, group process, and performance: An examination of learning teams. International Journal of Intercultural Relations, 26 (1), 1–16. https://doi.org/10.1016/S0147-1767(01)00032-3 .
Wirkala, C., & Kuhn, D. (2011). Problem-based learning in K–12 education: Is it effective and how does it achieve its effects? American Educational Research Journal, 48 (5), 1157–1186. https://doi.org/10.3102/0002831211419491 .
Woods, D. R. (2014). Problem-oriented learning, problem-based learning, problem-based synthesis, process oriented guided inquiry learning, peer-led team learning, model-eliciting activities, and project-based learning: What is best for you? Industrial and Engineering Chemistry Research, 53 (13), 5337–5354. https://doi.org/10.1021/ie401202k .
Zwiep, S. G., & Straits, W. J. (2013). Inquiry science: The gateway to English language proficiency. Journal of Science Teacher Education, 24 (8), 1315–1331. https://doi.org/10.1007/s10972-013-9357-9 .
Download references
There was no funding for this work.
Author information
Authors and affiliations.
Instructional Systems Technology, Indiana University, Bloomington, IN, 47405, USA
Krista D. Glazewski
Purdue University, West Lafayette, IN, 47907, USA
Peggy A. Ertmer
Littleton, CO, 80120, USA
You can also search for this author in PubMed Google Scholar
Corresponding author
Correspondence to Krista D. Glazewski .
Ethics declarations
Conflict of interests.
The authors declare that they have no conflict of interest.
Additional information
Publisher's note.
Springer Nature remains neutral with regard to jurisdictional claims in published maps and institutional affiliations.
Rights and permissions
Reprints and permissions
About this article
Glazewski, K.D., Ertmer, P.A. Fostering complex problem solving for diverse learners: engaging an ethos of intentionality toward equitable access. Education Tech Research Dev 68 , 679–702 (2020). https://doi.org/10.1007/s11423-020-09762-9
Download citation
Published : 14 April 2020
Issue Date : April 2020
DOI : https://doi.org/10.1007/s11423-020-09762-9
Share this article
Anyone you share the following link with will be able to read this content:
Sorry, a shareable link is not currently available for this article.
Provided by the Springer Nature SharedIt content-sharing initiative
- Complex problem solving
- Linguistic and culturally diverse learners
- Inquiry learning
- Culturally responsive teaching
- Problem-based learning
- Project-based learning
- Find a journal
- Publish with us
- Track your research

Serving technical professionals globally for over 75 years. Learn more about us.
MIT Professional Education 700 Technology Square Building NE48-200 Cambridge, MA 02139 USA
Accessibility
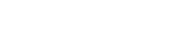
Solving Complex Problems: Structured Thinking, Design Principles, and AI
Sang-Gook Kim
Download the Course Schedule
How do you solve important, large-scale challenges with evolving and contradictory constraints? In this 5-day course, transform your approach to large-scale problem solving, from multi-stakeholder engineering projects to the online spread of misinformation. Alongside engineers and leaders from diverse industries, you’ll explore actionable innovative frameworks for assessing, communicating, and implementing complex systems—and significantly increase your likelihood of success.
THIS COURSE MAY BE TAKEN INDIVIDUALLY OR AS PART OF THE PROFESSIONAL CERTIFICATE PROGRAM IN INNOVATION & TECHNOLOGY OR THE PROFESSIONAL CERTIFICATE PROGRAM IN DESIGN & MANUFACTURING .
Engineering projects with shifting goals. Inefficient national healthcare systems. The online spread of misinformation. Every day, professionals are tasked with addressing major challenges that present opportunities for great triumph—or significant failure. How do you approach an important, large-scale challenge with evolving and contradictory constraints? Is the solution a new technology, a new policy, or something else altogether? In our new course Solving Complex Problems: Structured Thinking, Design Principles, and AI , you’ll acquire core principles that will change the way you approach and solve large-scale challenges—increasing your likelihood of success. Over the course of five days, you will explore proven design principles, heuristic-based insights, and problem-solving approaches, and learn how to persuasively present concepts and system architectures to stakeholders. Methods utilize recent developments in AI and Big Data, as well as innovative strategies from MIT Lincoln Laboratory that have been successfully applied to large and complex national defense systems. By taking part in interactive lectures and hands-on projects, you will learn to think through and leverage important steps, including problem abstraction, idea generation, concept development and refinement, system-level thinking, and proposal generation. Alongside an accomplished group of global peers, you will explore the strategies and frameworks you need to implement large-scale systems that can have a significant positive impact—and minimize the probability of failure.
Certificate of Completion from MIT Professional Education
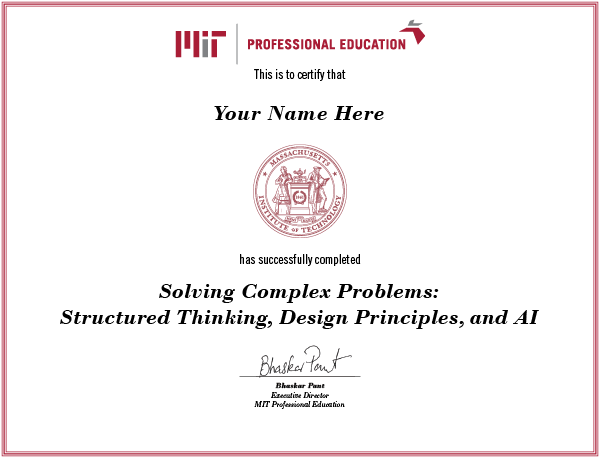
- Approach and solve large and complex problems.
- Assess end-to-end processes and associated challenges, in order to significantly increase the likelihood of success in developing more complex systems.
- Implement effective problem-solving techniques, including abstracting the problem, idea generation, concept development and refinement, system-level thinking, and proposal generation.
- Utilize system-level thinking skills to evaluate, refine, down select, and evaluate best ideas and concepts.
- Apply the Axiomatic Design methodology to a broad range of applications in manufacturing, product design, software, and architecture.
- Generate and present proposals that clearly articulate innovative ideas, clarify the limits of current strategies, define potential customers and impact, and outline a success-oriented system development and risk mitigation plan.
- Effectively communicate ideas and persuade others, and provide valuable feedback.
- Confidently develop and execute large-scale system concepts that will drive significant positive impact.
Edwin F. David Head of the Engineering Division, MIT Lincoln Laboratory
Jonathan E. Gans Group Leader of the Systems and Architectures Group, MIT Lincoln Laboratory
Robert T-I. Shin Principal Staff in the Intelligence, Surveillance, and Reconnaissance (ISR) and Tactical Systems Division, MIT Lincoln Laboratory Director, MIT Beaver Works
This course is appropriate for professionals who design or manage complex systems with shifting needs and goals. It is also well suited to those who want to improve the quality and performance of their operations and decision-making in a large-scale system environment. Potential participants include engineers, group leaders, and senior managers in government and industries including automotive, aerospace, semiconductors, engineering, manufacturing, healthcare, bio-medical, finance, architecture, public policy, education, and military.
Computer Requirements
A laptop with PowerPoint is required.
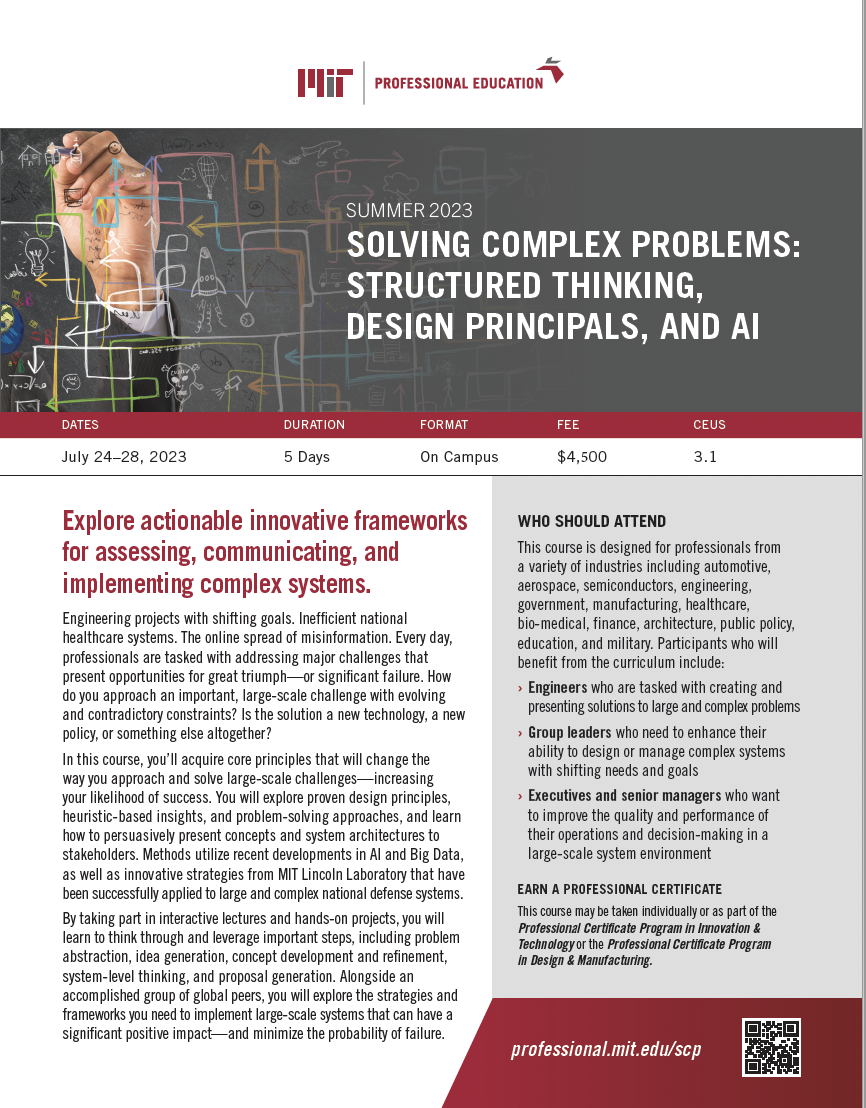
Thank you for visiting nature.com. You are using a browser version with limited support for CSS. To obtain the best experience, we recommend you use a more up to date browser (or turn off compatibility mode in Internet Explorer). In the meantime, to ensure continued support, we are displaying the site without styles and JavaScript.
- View all journals
- My Account Login
- Explore content
- About the journal
- Publish with us
- Sign up for alerts
- Data Descriptor
- Open access
- Published: 25 April 2024
Students’ performance, attitude, and classroom observation data to assess the effect of problem-based learning approach supplemented by YouTube videos in Ugandan classroom
- Nicholus Gumisirizah 1 ,
- Joseph Nzabahimana 1 &
- Charles M. Muwonge 2
Scientific Data volume 11 , Article number: 428 ( 2024 ) Cite this article
158 Accesses
Metrics details
- Applied physics
In response to global demands, Uganda’s Vision 2040 seeks to transform the country into a modern and prosperous nation by implementing Sustainable Development Goal (SDG) 4, focusing on equitable and quality education. The 21st-century workforce requires individuals who can effectively navigate complex workplace challenges. This dataset was gathered from Form-2 Ugandan secondary school students (aged 12 to 15) across 12 schools in the Sheema District. The dataset comprises three types of data: students’ performance in a physics topic (simple machines), their attitudes toward problem-solving and critical thinking when learning physics using Problem-Based Learning (PBL) supplemented by YouTube videos, and classroom observations documented with the reformed teaching observational protocol (RTOP). The intervention of teaching using PBL was executed in 2022, collecting data from 973 lower secondary school students. The intervention involved three approaches: one group (144 students) received PBL along with YouTube videos, another group of 482 students received PBL alone, and a third group (347 students) was taught using the traditional method. This data article explains the study’s data creation, collection, and analysis process. The dataset holds significance for secondary school teachers, policymakers, and researchers, offering insights into the impact of PBL with and without ICT resources on learning physics and students’ attitudes toward these learner-centered approaches.
Similar content being viewed by others
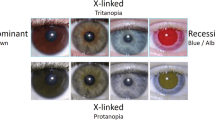
What colour are your eyes? Teaching the genetics of eye colour & colour vision. Edridge Green Lecture RCOphth Annual Congress Glasgow May 2019
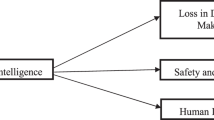
Impact of artificial intelligence on human loss in decision making, laziness and safety in education
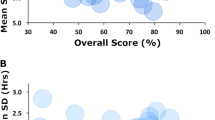
Sleep quality, duration, and consistency are associated with better academic performance in college students
Background & summary.
Physics education in secondary schools plays a vital role in developing students’ social, physical, leadership, and problem-solving skills. Understanding physics concepts equips learners to know how things work, enabling them to apply this understanding to real-life situations 1 . The physics teaching is structured around activity-based 2 chapters and topics, emphasizing hands-on experiences 3 and practical applicability in everyday life. However, many students find physics challenging, necessitating an active teaching approach. Teaching in physics remains dynamic and interactive, with teachers adopting various strategies to engage students actively. Reciprocal teaching involves dialogues between the teacher and small student groups, while peer collaboration fosters cooperative work on class activities. Problem-Based Learning (PBL) 4 , 5 , 6 is a student-centered approach that encourages group-based learning and teacher facilitation. It has been widely adopted in various educational fields, promoting problem-solving in learning environments. Implementing PBL follows a five-stage process:
Finding a problem
The teacher prepares a task for students to investigate, stimulating problem-solving abilities.
Organizing ideas on the problem
Learners investigate the problem, generate ideas, and receive probing questions from the facilitator to stimulate critical thinking.
The teacher facilitates the distribution of learners into groups, each focusing on solving a particular problem related to the main task. Responsibilities are assigned within each group, promoting cooperation.
Present findings
Learners present solutions to the problem and receive feedback from peers, consolidating their learning outcomes.
Generalizing
Problem-solving leads to the development of skills essential for solving complex, real-world situations. These skills, including problem-solving, creativity, communication, cooperation, and innovation, prepare students to adapt to change and overcome 21st-century challenges.
Integrating YouTube videos as Information and Communication Technology (ICT) tools within a PBL approach offers a multifaceted strategy to enhance physics education 7 , 8 . High-quality videos aligned with curriculum objectives introduce real-world problems and cater to diverse learning styles. Interactive features and accessibility allow continuous learning, and educators can curate playlists to align with curriculum goals. The flipped classroom model 9 combines videos with problem-solving discussions 10 , creating a dynamic learning environment that deepens students’ understanding of physics concepts and their practical applications.
Physics is a subject that holds a significant position in promoting scientific literacy, critical thinking, and essential life skills. However, conventional teaching methods often struggle to engage and empower students in the subject matter effectively. This inadequacy is a pressing concern, as it can hinder students from developing a strong foundation in physics, which is essential for their academic and practical pursuits. This study was critically important due to the existing challenges within physics education in Ugandan secondary schools. Incorporating innovative teaching approaches, such as PBL supplemented by YouTube videos, becomes pivotal in addressing these challenges. These methods can enhance students’ comprehension of physics and nurture vital skills like problem-solving, creativity, communication, cooperation, and innovation. These skills are indispensable for students to thrive in a rapidly evolving, knowledge-driven world.
Sharing the data generated through this study is equally significant. It is a valuable resource for educators, policymakers, curriculum designers, and researchers. By making this data accessible, the study contributes to the ongoing efforts to improve the quality and relevance of secondary education in Uganda. Educators can utilize this data to adopt innovative and effective teaching methods that align with the goals of the educational system, ultimately enhancing students’ performance and fostering lifelong learning. Policymakers and curriculum designers can use the insights derived from this data to conduct essential reviews and make informed decisions about teacher competence and the adoption of innovative teaching methodologies. Furthermore, researchers in similar fields can leverage this data to understand better the impact of PBL and the use of multimedia resources in education. This data identifies gaps and challenges and offers potential solutions and avenues for further research.
This data-sharing article presents insights into the effects of Problem-Based Learning (PBL) supplemented by YouTube videos on students’ comprehension of simple machines in physics within Ugandan lower secondary schools. The research collected data from 973 students, encompassing both public and private schools in the Sheema district of Uganda. Three primary types of data were collected: students’ performance data, attitude data, and classroom observation data.
Performance data was acquired through a Physics Learning Achievement Test (PLAT), involving students from various school types and teaching methods. Attitude data were collected via two surveys, one focusing on problem-solving ability (AAPS) and the other on critical thinking ability (CTMS) under PBL with YouTube videos. The Approaches to Problem-Solving Survey (AAPS) and the Critical Thinking Motivational Scale (CTMS) are measurement tools commonly used in the field of physics. The AAPS assesses various strategies individuals employ when solving problems, while the CTMS evaluates motivational factors influencing critical thinking abilities. The Reformed Teaching Observation Protocol (RTOP) assessed classroom practices and teaching methods.
The dataset, available in raw, filtered, and analyzed formats, offers valuable insights into the impact of innovative teaching methods on student performance, attitudes, and classroom practices. It addresses critical questions about the effectiveness of PBL approaches, with potential implications for science education in Uganda.
This dataset intends to assess the impact of PBL when supplemented with YouTube videos on Ugandan form-2 lower secondary schools in learning simple machines. The following are the research questions:
To what extent do PBL and PBL supplemented with YouTube videos enhance students’ conceptual understanding of simple machines in physics?
What are the problem-solving and critical thinking levels brought by learning with PBL supplemented by YouTube videos?
How is physics teaching reformed when learning simple machines in physics with PBL supplemented by YouTube videos?
Are there differences in students’ academic achievement for school type (government alongside private school)?
Ethics statements
The research project rigorously adhered to ethical standards established by the University of Rwanda College of Education’s (UR-CE) Research and Innovation Unit under the ethical protocol number Ref. 03/DRI-CE/078/EN/gi/2021, dated 30th November 2021. All necessary permissions were obtained systematically and ethically, as outlined in the research project description. Here is a summary of the ethical considerations and recruitment process:
Ethical protocol
The research project adhered to the ethical standards and principles of the UR-CE)‘s Research and Innovation Unit. The protocol number and approval date are explicitly mentioned, demonstrating a formal ethical review.
Permissions from authorities
The Ministry of Education and Sports obtained formal permission to access schools through the Permanent Secretary’s (PS) office. The PS communicated with the Chief Administrative Officer (CAO), District Education Officer (DEO), and Resident District Commissioner (RDC) to secure the necessary support for the study.
Engagement with schools
With the approval from the CAO, the DEO contacted school heads to inform them about the research study. The school heads responded positively and even provided physics teachers with three-day problem-based learning (PBL) training as part of the research. It is worth noting that all participating teachers held teaching qualifications, and as part of the research process, we provided them with a three-day training session specifically focused on implementing PBL interventions. This training aimed to ensure consistent delivery of PBL across treatment classrooms and schools, thereby mitigating variations attributable to individual teaching styles.
Informed consent
Teachers and students, with parental consent, willingly participated in the research study. Informed consent forms were signed, indicating they fully understood the study’s purpose, procedures, potential risks, and benefits. Anonymity was ensured for students by not including their names on the test papers.
The research employed purposive sampling to select 973 students from 12 schools. These schools were divided into three groups, each with a different teaching method: PBL with YouTube videos, PBL alone, and Traditional teaching.
Geographic considerations
Schools were selected from different town councils at extreme ends of the district, sharing similar characteristics suitable for the study. This approach helps ensure that the study’s findings are robust and generalizable.
Research design
The study utilized a non-equivalent comparison group pre/post-test design (Creswell, 2012). The study involves Form 2 students from six Sheema District, Western Uganda schools. Three selected schools were public, while the remaining three were private, offering a diverse representation of school types in the district. The selection of schools was purposeful, aiming to ensure diverse representation and maximize the study’s validity. This approach allowed for the strategic allocation of schools to treatment or control groups based on specific criteria pertinent to the research objectives. Notably, the selection criteria considered factors such as geographical location, school size, academic performance, and availability of resources to ensure a balanced representation of different educational contexts. The traditional method, characterized by conventional lectures supplemented with textbooks and teacher-centered content delivery, was employed in control group schools. Students in this group primarily learned through note-taking with minimal demonstrations. Conversely, four other secondary schools were designated as the first treatment group, where Problem-Based Learning (PBL) was implemented. Four additional schools comprised the second treatment group, which utilized PBL supplemented by educational YouTube videos. These groups collectively engaged in constructing knowledge and enhancing conceptual understanding. The participants in the study were form-2 students, ranging in age from 12 to 15 years, who were already enrolled in the schools.
We provide a performance (achievement) test to all 973 students before and after teaching interventions in all groups. We administered an attitude survey (motivation scale) and observed classes in the group that used PBL and YouTube videos. Table 1 presents the sample size under the teaching intervention of design groups implemented.
The objective of the performance test was to gauge students’ grasp of conceptual understanding acquired through the implementation of a problem-based learning approach following the completion of the topic on simple machines. The test, spanning 25 minutes, consisted of ten questions sourced from practice exercises on simple machines within form-two secondary learners’ physics textbooks. The National Curriculum Development Center and the Ministry of Education and Sports in Uganda approved these textbooks. The examination encompassed themes outlined in the approved lower secondary curriculum physics syllabus, covering concepts like the applications of simple machines, mechanical advantage, velocity ratio, and efficiency of machines. Specific topics included levers (covering classes and applications), pulley systems (encompassing types, applications, mechanical advantage, velocity ratio, and efficiency), inclined planes (including applications, mechanical advantage, velocity ratio, and efficiency), wheel and axle (exploring understanding, applications, and velocity ratio), gears (addressing simplification of work, applications, and velocity ratio), and methods of enhancing machine efficiency. The test was validated by four researchers from Mbarara University of Science and Technology (MUST) and the University of Rwanda College of Education (URCE). Test 1 was scored in MS Excel with “IF EXCACT” function, while Test 2 was manually marked, and results were entered in the same software.
Attitude surveys were all adopted from existing literature. Critical Thinking Motivational Scale (CTMS) was used as our Survey 1 and was adapted from Valenzuela et al . 11 , while Attitudes and Approaches to Problem-Solving Survey (AAPS) was used as our Survey 2 and was adapted from Singh and Mason 12 and available at Physport ( https://www.physport.org/assessments/assessment.cfm?A=AAPS ). Problem-solving and critical thinking are integral to effective physics education. They deepen students’ understanding by connecting theoretical concepts to real-world situations 13 , 14 . These skills encourage active engagement and foster analytical abilities, allowing students to break down complex problems. Additionally, they promote creativity, help apply theory to practice, and cultivate logical reasoning. Problem-solving and critical thinking prepare students for future challenges in scientific and engineering fields, encourage collaboration, boost confidence, and instill a mindset for lifelong learning. Incorporating these skills into physics teaching enhances academic performance and equips students with valuable personal and professional growth tools. We adopted all 19 items from CTMS and only 31 items from AAPS to meet our research aim. Thus, the last two items (32 and 33) in AAPS were removed as they were not related to the content delivered in our study. All these surveys were rated on a Likert scale (from strongly disagree to strongly agree). Items 1–4 are related to expectancy, items 5-8 to attainment, items 9-12 to utility, items 13-16 to interest, and items 17-19 to cost.
Classroom observation data was collected with the famous standardized reformed teaching observation protocol (RTOP) from Pibun and Sawada 15 and is available at Pysport ( https://www.physport.org/assessments/assessment.cfm?A=RTOP ). RTOP proved its validity and reliable results across the globe 16 , 17 , 18 , 19 with its potential to reveal reformed teaching while implementing a new teaching method. It comprises 25 statements where each item is evaluated on a 5-scale. It is scored 0 when such practice was not found in a lesson and 4 when a certain practice was very well described or observed in a delivered lesson. During classroom observation, an observer sits in the classroom and observes what the teacher and student do. He/she may take notes on what is happening but wait until the class is over to rate these 25 items.
Data Records
All data described in this descriptor are deposited in figshare ( https://figshare.com/articles/dataset/RTOP_Data_for_the_implementation_of_Problem-based_learning_in_a_Physics_classroom_Uganda/23974902 ) 20
To evaluate the impact of PBL teaching intervention on students’ performance and attitude toward learning physics, we gathered three data types (performance, attitude, and observation) presented in five datasets (two performance tests, two attitude surveys, and one classroom observation).
Students’ performance data
The student performance data comprises two datasets or MS Excel files. The first file contains data for test one titled “Performance data _ Test 1 (Multiple choice) _ 12102022 figshare.” This file contains data from ten multiple-choice questions. The file contains three sheets. The first sheet shows test items (all ten questions), the second presents pretest answer choices, and the third presents post-test answer choices or results. Each results sheet shows the school code (column B), student code (column C), school type (column D), and treatment group (column E) as variables. From column “F” to column “O” we see student answer choices under each test question. From column “Q” to column “Z” we marked the test (one score for each correct question). Column “AB” shows the percent score. Row “3” shows the expected correct answer, while row “4” shows variables and the number of test items.
The second file contains data for test two titled “Performance data _ Test 2 (Problem solving) _ 12102022 figshare.” This file contains data from ten-word problem kinds of questions. The file contains three sheets. The first sheet shows test items (all ten questions), the second presents pretest scores, and the third presents post-test scores or results. Each results sheet shows the school code (column C), student code (column D), school type (column E), and treatment group (column F) as variables. From column “G” to column “P” we see student scores under each test question. Column “R” shows the total score, while column “S” shows percent score. Row “3” shows the assigned score when each question’s expected correct answer was provided. Row “4” shows variables and several test items.
Students’ attitude data
The student attitude data comprises two datasets or MS Excel files. The first file contains data for the first survey titled “Motivation data _ Survey 1 (Critical thinking ability) _ 12102022 figshare.” This file contains data from 19 items of critical thinking ability survey. The file contains two sheets. The first sheet shows the pre-test results, while the second shows the post-test results. Each sheet shows the school code (column C), student code (column D), school type (column E), and treatment group (column F) as variables. From column “F” to column “O” we see student answer choices under each test question. From column “G” to column “Y” we see student answers or agreement (1: STRONGLY DISAGREE, 2: DISAGREE, 3,: NEUTRAL, 4: AGREE, AND 5: STRONGLY AGREE) to each item of the survey. Row “2” shows the survey title, while row “4” shows the variables and number of survey items.
The second file contains data for the second survey titled “Attitude data _ Survey 2 (Problem solving ability) _ 12102022 figshare.” This file contains data from 31 items related to problem-solving ability in learning physics. The file contains two sheets. The first sheet shows the pre-test results, while the second shows post-test results. Each sheet shows the school code (column C), student code (column D), school type (column E), and treatment group (column F) as variables. From column “G” to column “AK” we see student answers or agreement (1: STRONGLY DISAGREE, 2: DISAGREE, 3,: NEUTRAL, 4: AGREE, AND 5: STRONGLY AGREE) to each item of the survey. Row “2” shows the survey title, while row “4” shows the variables and number of survey items.
Classroom observation data
The file for classroom observation data is titled “Classroom observation data _ RTOP for video & pbl group _ 12102022 figshare” and contains only one sheet. From column “B” to column “C” we see RTOP while the following columns (D-AA) present data. Row “10” shows school codes, while row “5” shows several observations and frequencies under each school supplied with PBL and YouTube videos teaching intervention. The data range from 0 (never occurred) to 4 (very descriptive).
Technical Validation
Initially, we had 20 problem-solving questions, but evaluators rated 10 as valid, which were included in the final administration. We also initially had 15 multiple-choice questions, and evaluators rated 10 as appropriate and aligned with the study objectives. A pilot study was conducted with 90 students to evaluate the face validity and reliability of the questions. We assessed the reliability of these items using a split-half method and obtained a high reliability (r = 0.87) for multiple-choice items and a medium reliability (r = 0.68) measured by the Pearson product-moment correlation coefficient for problem-solving items. The split-half reliability assumes that the two halves of the test are equivalent in difficulty and content 21 .
CTMS and AAPS
During our pilot phase, the internal consistency of CTMS, assessed using Cronbach’s alpha, was found to be high (0.793) for all 19 items, medium (0.428) for expectancy, (0.411) for attainment, (0.686) for utility, (0.574) for interest, and (0.594) for cost. The AAPS exhibited an internal consistency reliability of Cronbach’s alpha = 0.685. It is important to note that the AAPS contains nine items formulated negatively. Therefore, for a positive attitude, students were required to respond with ‘Disagree’ or ‘Strongly disagree’ to these items (1, 3, 5, 8, 11, 12, 16, 23, and 30). Consequently, the reliability of the 22 positively formulated items was 0.601, while that of the negatively formulated items was 0.480.
Before observing actual classes, we underwent a 2-hour training session and watched and coded a YouTube classroom video on physics. The inter-rater agreement between the first author and the assistant exceeded 80% on two occasions, indicating the reliability of the data.
Scope and potential limitations
In our study, we recognized the significance of investigating potential bias in the results obtained from students in both private and public schools. To ensure the credibility and robustness of our findings, we conducted a comparative analysis to determine whether any notable disparities existed between these two groups. Our data collection process was comprehensive, encompassing a diverse range of schools, including both private and public institutions. This approach allowed us to capture a broad spectrum of socioeconomic backgrounds and educational settings. The study itself involved Form-2 students who were enrolled in schools situated in different town councils at opposite ends of the district. Despite their geographical diversity, these schools shared pertinent characteristics relevant to our research objectives. To facilitate our investigation, we categorized these schools into distinct treatment groups, comprising PBL alone and PBL with videos, along with a control group following traditional teaching methods. Importantly, we deliberately chose to maintain the existing class arrangements in these schools. Our commitment to preserving each school’s established class organization and cultural norms guided this decision.
However, it is essential to acknowledge the limitations inherent in the research design. One notable limitation is the observation of attitudes, which was limited to the student group exposed to the PBL with the video teaching method. This restriction may impact the generalizability of the findings, as attitudes toward learning may vary among students exposed to different instructional methods. Future research endeavors could consider incorporating measures to assess attitudes across all treatment groups to provide a more comprehensive understanding of the intervention’s effects.
The current data files do not contain information on individual teachers due to the scope and focus of the study. These variables could include educators’ teaching experience, pedagogical approach, content knowledge, and instructional effectiveness. Since we recognize the significance of teacher impact, we would consider incorporating such variables in future research projects to provide a more comprehensive analysis of instructional effectiveness and its associated factors.
Regarding the decision to maintain existing class arrangements in schools, particularly considering cultural norms, it is crucial to recognize its potential influence on the study outcomes. The intervention’s impact may have been influenced by preserving the existing class structures, including student composition and dynamics. For instance, certain class arrangements may foster greater collaboration and engagement, while others may present challenges in implementing collaborative learning approaches such as PBL. Therefore, future studies could explore the relationship between class arrangements and instructional effectiveness to provide insights into optimizing learning environments.
Usage Notes
Value of the data.
The data presented is valuable and beneficial to science education in Uganda as it elucidates the status of students’ content knowledge and their perceptions about learning simple machines with PBL approaches.
Policymakers and curriculum designers have the opportunity to conduct essential reviews that highlight the competence of teachers. This process can pave the way for advocating innovative and relevant teaching methodologies, subsequently informing the identification of professional development requirements for educators.
Researchers in similar fields can re-use these data to measure the effect of PBL intervention on student achievement, identify gaps, and predict possible remedies. Thus, data can be analyzed using various variables such as teaching intervention and school type.
Code availability
No custom code was used.
Putri, I. E. & Sinaga, P. Collaborative problem-solving: how to implement and measure it in science teaching and learning. in International conference on mathematics and science education (ICMSCE) 2020 vol 1806 (IOP PUBLISHING LTD, 2021).
Sokoloff, D. R. Active Learning of Introductory Optics: Interactive Lecture Demonstrations and Optics Magic Tricks. in ducation and Training in Optics and Photonics, OSA Technical Digest Series (Optical Society of America, 2007) . https://doi.org/10.1364/ETOP.2007.EWA2 (2007).
Dohn, N. B., Fago, A., Overgaard, J., Madsen, P. T. & Malte, H. Students’ motivation toward laboratory work in physiology teaching. Adv. Physiol. Educ. 40 , 313–318 (2016).
Article PubMed Google Scholar
Nguyen, D.-H., Gire, E. & Rebello, N. S. Facilitating Strategies for Solving Work-Energy Problems in Graphical and Equational Representations. in 2010 PHYSICS EDUCATION RESEARCH CONFERENCE (reds Singh, C., Sabella, M. & Rebello, S.) vol 1289 241–244 (AMER INST PHYSICS, 2010).
Seltpuk, G. S. & Tarak, M. Physics Teaching in Problem-Based Learning Physics Teaching in Problem-Based Learning. 844 , 1–2 (2017).
Bilgin, I. & Erdal, Ş. The Effects of Problem-Based Learning Instruction on University Students’ Performance of Conceptual and Quantitative Problems in Gas Concepts. EURASIA J. Math. Sci. Technol. Educ. 5 , 153–164 (2009).
Article Google Scholar
Susilawati, S., Rahmana, F. & Kosim, K. Practicality of problem-based physics learning tools with video assistance to improve problem-solving ability of students. J. Sci. Sci. Educ. 3 , 55–59 (2022).
Nasbey, H. & Raihanati, R. Developing a video education on the topic of Modern Physics based on problem-based learning (PBL) assisted PhET online learning. in Journal of Physics: Conference Series (reds F.C., W. et al .) vol 2377 (Institute of Physics, 2022).
Zhang, Y., Zhang, X., Zhang, K. & Shen, D. A Topic-based Computer Aided Instruction System for Programming Practice Courses. in Proceedings of the 2015 international conference on social science, education management and sports education (red Chen, L.) vol 39 1342–1345 (ATLANTIS PRESS, 2015).
Becerra-Labra, C., Gras-Martí, A. & Martínez Torregrosa, J. Effects of a problem-based structure of physics contents on conceptual learning and the ability to solve problems. Int. J. Sci. Educ. 34 , 1235–1253 (2012).
Valenzuela, J., Nieto, A. M. & Saiz, C. Critical Thinking Motivational Scale (CTMS): una aportación para el estudio de la relación entre el pensamiento crítico y la motivación. Electron. J. Res. Educ. Psychol. 9 , 823–848 (2011).
Singh, C. & Mason, A. Physics graduate students’ attitudes and approaches to problem solving. AIP Conf. Proc. 1179 , 273–276 (2009).
Article ADS Google Scholar
Ukobizaba, F., Ndihokubwayo, K., Mukuka, A. & Uwamahoro, J. Insights of teachers and students on mathematics teaching and learning in selected Rwandan secondary schools. African J. Educ. Stud. Math. Sci. 15 , 93–106 (2019).
Dorimana, A., Uworwabayeho, A. & Nizeyimana, G. Enhancing Upper Secondary Learners’ Problem - solving Abilities using Problem-based Learning in Mathematics. Int. J. Learn. Teach. Educ. Res. 21 , 235–252 (2022).
Piburn, M. et al . Reformed teaching observation protocol (RTOP) Training Guide . doi:ED419696 (Tempe, Arizona: Arizona Collaborative for Excellence in the Preparation of Teachers, 2000).
Ndihokubwayo, K., Uwamahoro, J. & Ndayambaje, I. Implementation of the Competence-Based Learning in Rwandan Physics Classrooms: First Assessment Based on the Reformed Teaching Observation Protocol. EURASIA J. Math. Sci. Technol. Educ. 16 , 1–8 (2020).
Ndihokubwayo, K., Uwamahoro, J. & Ndayambaje, I. Classroom observation data collected to document the implementation of physics competence-based curriculum in Rwanda. Data Br. 36 , 107055 (2021).
Article CAS Google Scholar
Park, S., Jang, J. Y., Chen, Y. C. & Jung, J. Is Pedagogical Content Knowledge (PCK) Necessary for Reformed Science Teaching?: Evidence from an Empirical Study. Res. Sci. Educ. 41 , 245–260 (2010).
MacIsaac, D., Sawada, D. & Falconer, K. Using the Reformed Teaching Observation Protocol (RTOP) as a Catalyst for Self- Reflective Change in Secondary Science Teaching. (2001).
Gumisirizah, N., Nzabahimana, J. & Muwonge, C. M. Students’ academic achievement test, Survey and RTOP data for the implementation of problem-based learning method supplemented by YouTube videos, Uganda. Figshare https://doi.org/10.6084/m9.figshare.23974902.v2 (2023).
Adalikwu, S. & Iorkpilgh, I. The Influence of Instructional Materials on Academic Performance of Senior Secondary School Students in Chemistry in Cross River State. Glob. J. Educ. Res. 12 , 39–45 (2013).
Google Scholar
Download references
Acknowledgements
We acknowledge the African Center for Excellence for Innovative Teaching and Learning Mathematics and Science (ACEITLMS) for funding this study and the authors of the research tools we used to free them to use. All study participants, teachers, and school headteachers are also well acknowledged.
Author information
Authors and affiliations.
African Center of Excellence for Innovative Teaching and Learning Mathematics and Science (ACEITLMS), University of Rwanda College of Education (URCE), Kayonza, P.O Box 55, Rwamagana, Rwanda
Nicholus Gumisirizah & Joseph Nzabahimana
Mbarara Science and Technology (MUST), Mbarara, Uganda
Charles M. Muwonge
You can also search for this author in PubMed Google Scholar
Contributions
Nicholus Gumisirizah: Conceptualization, Methodology, Visualization, Data curation, Software, Writing- Original draft preparation. Joseph Nzabahimana, Charles M. Muwonge: Conceptualization, Validation, Writing- Reviewing and Editing.
Corresponding author
Correspondence to Nicholus Gumisirizah .
Ethics declarations
Competing interests.
The authors declare that they have no known competing financial interests or personal relationships that could have appeared to influence the work reported in this paper.
Additional information
Publisher’s note Springer Nature remains neutral with regard to jurisdictional claims in published maps and institutional affiliations.
Rights and permissions
Open Access This article is licensed under a Creative Commons Attribution 4.0 International License, which permits use, sharing, adaptation, distribution and reproduction in any medium or format, as long as you give appropriate credit to the original author(s) and the source, provide a link to the Creative Commons licence, and indicate if changes were made. The images or other third party material in this article are included in the article’s Creative Commons licence, unless indicated otherwise in a credit line to the material. If material is not included in the article’s Creative Commons licence and your intended use is not permitted by statutory regulation or exceeds the permitted use, you will need to obtain permission directly from the copyright holder. To view a copy of this licence, visit http://creativecommons.org/licenses/by/4.0/ .
Reprints and permissions
About this article
Cite this article.
Gumisirizah, N., Nzabahimana, J. & Muwonge, C.M. Students’ performance, attitude, and classroom observation data to assess the effect of problem-based learning approach supplemented by YouTube videos in Ugandan classroom. Sci Data 11 , 428 (2024). https://doi.org/10.1038/s41597-024-03206-2
Download citation
Received : 04 November 2023
Accepted : 02 April 2024
Published : 25 April 2024
DOI : https://doi.org/10.1038/s41597-024-03206-2
Share this article
Anyone you share the following link with will be able to read this content:
Sorry, a shareable link is not currently available for this article.
Provided by the Springer Nature SharedIt content-sharing initiative
Quick links
- Explore articles by subject
- Guide to authors
- Editorial policies
Sign up for the Nature Briefing newsletter — what matters in science, free to your inbox daily.


An official website of the United States government
The .gov means it’s official. Federal government websites often end in .gov or .mil. Before sharing sensitive information, make sure you’re on a federal government site.
The site is secure. The https:// ensures that you are connecting to the official website and that any information you provide is encrypted and transmitted securely.
- Publications
- Account settings
Preview improvements coming to the PMC website in October 2024. Learn More or Try it out now .
- Advanced Search
- Journal List
- Front Psychol
Complex Problem Solving: What It Is and What It Is Not
Dietrich dörner.
1 Department of Psychology, University of Bamberg, Bamberg, Germany
Joachim Funke
2 Department of Psychology, Heidelberg University, Heidelberg, Germany
Computer-simulated scenarios have been part of psychological research on problem solving for more than 40 years. The shift in emphasis from simple toy problems to complex, more real-life oriented problems has been accompanied by discussions about the best ways to assess the process of solving complex problems. Psychometric issues such as reliable assessments and addressing correlations with other instruments have been in the foreground of these discussions and have left the content validity of complex problem solving in the background. In this paper, we return the focus to content issues and address the important features that define complex problems.
Succeeding in the 21st century requires many competencies, including creativity, life-long learning, and collaboration skills (e.g., National Research Council, 2011 ; Griffin and Care, 2015 ), to name only a few. One competence that seems to be of central importance is the ability to solve complex problems ( Mainzer, 2009 ). Mainzer quotes the Nobel prize winner Simon (1957) who wrote as early as 1957:
The capacity of the human mind for formulating and solving complex problems is very small compared with the size of the problem whose solution is required for objectively rational behavior in the real world or even for a reasonable approximation to such objective rationality. (p. 198)
The shift from well-defined to ill-defined problems came about as a result of a disillusion with the “general problem solver” ( Newell et al., 1959 ): The general problem solver was a computer software intended to solve all kind of problems that can be expressed through well-formed formulas. However, it soon became clear that this procedure was in fact a “special problem solver” that could only solve well-defined problems in a closed space. But real-world problems feature open boundaries and have no well-determined solution. In fact, the world is full of wicked problems and clumsy solutions ( Verweij and Thompson, 2006 ). As a result, solving well-defined problems and solving ill-defined problems requires different cognitive processes ( Schraw et al., 1995 ; but see Funke, 2010 ).
Well-defined problems have a clear set of means for reaching a precisely described goal state. For example: in a match-stick arithmetic problem, a person receives a false arithmetic expression constructed out of matchsticks (e.g., IV = III + III). According to the instructions, moving one of the matchsticks will make the equations true. Here, both the problem (find the appropriate stick to move) and the goal state (true arithmetic expression; solution is: VI = III + III) are defined clearly.
Ill-defined problems have no clear problem definition, their goal state is not defined clearly, and the means of moving towards the (diffusely described) goal state are not clear. For example: The goal state for solving the political conflict in the near-east conflict between Israel and Palestine is not clearly defined (living in peaceful harmony with each other?) and even if the conflict parties would agree on a two-state solution, this goal again leaves many issues unresolved. This type of problem is called a “complex problem” and is of central importance to this paper. All psychological processes that occur within individual persons and deal with the handling of such ill-defined complex problems will be subsumed under the umbrella term “complex problem solving” (CPS).
Systematic research on CPS started in the 1970s with observations of the behavior of participants who were confronted with computer simulated microworlds. For example, in one of those microworlds participants assumed the role of executives who were tasked to manage a company over a certain period of time (see Brehmer and Dörner, 1993 , for a discussion of this methodology). Today, CPS is an established concept and has even influenced large-scale assessments such as PISA (“Programme for International Student Assessment”), organized by the Organization for Economic Cooperation and Development ( OECD, 2014 ). According to the World Economic Forum, CPS is one of the most important competencies required in the future ( World Economic Forum, 2015 ). Numerous articles on the subject have been published in recent years, documenting the increasing research activity relating to this field. In the following collection of papers we list only those published in 2010 and later: theoretical papers ( Blech and Funke, 2010 ; Funke, 2010 ; Knauff and Wolf, 2010 ; Leutner et al., 2012 ; Selten et al., 2012 ; Wüstenberg et al., 2012 ; Greiff et al., 2013b ; Fischer and Neubert, 2015 ; Schoppek and Fischer, 2015 ), papers about measurement issues ( Danner et al., 2011a ; Greiff et al., 2012 , 2015a ; Alison et al., 2013 ; Gobert et al., 2015 ; Greiff and Fischer, 2013 ; Herde et al., 2016 ; Stadler et al., 2016 ), papers about applications ( Fischer and Neubert, 2015 ; Ederer et al., 2016 ; Tremblay et al., 2017 ), papers about differential effects ( Barth and Funke, 2010 ; Danner et al., 2011b ; Beckmann and Goode, 2014 ; Greiff and Neubert, 2014 ; Scherer et al., 2015 ; Meißner et al., 2016 ; Wüstenberg et al., 2016 ), one paper about developmental effects ( Frischkorn et al., 2014 ), one paper with a neuroscience background ( Osman, 2012 ) 1 , papers about cultural differences ( Güss and Dörner, 2011 ; Sonnleitner et al., 2014 ; Güss et al., 2015 ), papers about validity issues ( Goode and Beckmann, 2010 ; Greiff et al., 2013c ; Schweizer et al., 2013 ; Mainert et al., 2015 ; Funke et al., 2017 ; Greiff et al., 2017 , 2015b ; Kretzschmar et al., 2016 ; Kretzschmar, 2017 ), review papers and meta-analyses ( Osman, 2010 ; Stadler et al., 2015 ), and finally books ( Qudrat-Ullah, 2015 ; Csapó and Funke, 2017b ) and book chapters ( Funke, 2012 ; Hotaling et al., 2015 ; Funke and Greiff, 2017 ; Greiff and Funke, 2017 ; Csapó and Funke, 2017a ; Fischer et al., 2017 ; Molnàr et al., 2017 ; Tobinski and Fritz, 2017 ; Viehrig et al., 2017 ). In addition, a new “Journal of Dynamic Decision Making” (JDDM) has been launched ( Fischer et al., 2015 , 2016 ) to give the field an open-access outlet for research and discussion.
This paper aims to clarify aspects of validity: what should be meant by the term CPS and what not? This clarification seems necessary because misunderstandings in recent publications provide – from our point of view – a potentially misleading picture of the construct. We start this article with a historical review before attempting to systematize different positions. We conclude with a working definition.
Historical Review
The concept behind CPS goes back to the German phrase “komplexes Problemlösen” (CPS; the term “komplexes Problemlösen” was used as a book title by Funke, 1986 ). The concept was introduced in Germany by Dörner and colleagues in the mid-1970s (see Dörner et al., 1975 ; Dörner, 1975 ) for the first time. The German phrase was later translated to CPS in the titles of two edited volumes by Sternberg and Frensch (1991) and Frensch and Funke (1995a) that collected papers from different research traditions. Even though it looks as though the term was coined in the 1970s, Edwards (1962) used the term “dynamic decision making” to describe decisions that come in a sequence. He compared static with dynamic decision making, writing:
- simple In dynamic situations, a new complication not found in the static situations arises. The environment in which the decision is set may be changing, either as a function of the sequence of decisions, or independently of them, or both. It is this possibility of an environment which changes while you collect information about it which makes the task of dynamic decision theory so difficult and so much fun. (p. 60)
The ability to solve complex problems is typically measured via dynamic systems that contain several interrelated variables that participants need to alter. Early work (see, e.g., Dörner, 1980 ) used a simulation scenario called “Lohhausen” that contained more than 2000 variables that represented the activities of a small town: Participants had to take over the role of a mayor for a simulated period of 10 years. The simulation condensed these ten years to ten hours in real time. Later, researchers used smaller dynamic systems as scenarios either based on linear equations (see, e.g., Funke, 1993 ) or on finite state automata (see, e.g., Buchner and Funke, 1993 ). In these contexts, CPS consisted of the identification and control of dynamic task environments that were previously unknown to the participants. Different task environments came along with different degrees of fidelity ( Gray, 2002 ).
According to Funke (2012) , the typical attributes of complex systems are (a) complexity of the problem situation which is usually represented by the sheer number of involved variables; (b) connectivity and mutual dependencies between involved variables; (c) dynamics of the situation, which reflects the role of time and developments within a system; (d) intransparency (in part or full) about the involved variables and their current values; and (e) polytely (greek term for “many goals”), representing goal conflicts on different levels of analysis. This mixture of features is similar to what is called VUCA (volatility, uncertainty, complexity, ambiguity) in modern approaches to management (e.g., Mack et al., 2016 ).
In his evaluation of the CPS movement, Sternberg (1995) compared (young) European approaches to CPS with (older) American research on expertise. His analysis of the differences between the European and American traditions shows advantages but also potential drawbacks for each side. He states (p. 301): “I believe that although there are problems with the European approach, it deals with some fundamental questions that American research scarcely addresses.” So, even though the echo of the European approach did not enjoy strong resonance in the US at that time, it was valued by scholars like Sternberg and others. Before attending to validity issues, we will first present a short review of different streams.
Different Approaches to CPS
In the short history of CPS research, different approaches can be identified ( Buchner, 1995 ; Fischer et al., 2017 ). To systematize, we differentiate between the following five lines of research:
- simple (a) The search for individual differences comprises studies identifying interindividual differences that affect the ability to solve complex problems. This line of research is reflected, for example, in the early work by Dörner et al. (1983) and their “Lohhausen” study. Here, naïve student participants took over the role of the mayor of a small simulated town named Lohhausen for a simulation period of ten years. According to the results of the authors, it is not intelligence (as measured by conventional IQ tests) that predicts performance, but it is the ability to stay calm in the face of a challenging situation and the ability to switch easily between an analytic mode of processing and a more holistic one.
- simple (b) The search for cognitive processes deals with the processes behind understanding complex dynamic systems. Representative of this line of research is, for example, Berry and Broadbent’s (1984) work on implicit and explicit learning processes when people interact with a dynamic system called “Sugar Production”. They found that those who perform best in controlling a dynamic system can do so implicitly, without explicit knowledge of details regarding the systems’ relations.
- simple (c) The search for system factors seeks to identify the aspects of dynamic systems that determine the difficulty of complex problems and make some problems harder than others. Representative of this line of research is, for example, work by Funke (1985) , who systematically varied the number of causal effects within a dynamic system or the presence/absence of eigendynamics. He found, for example, that solution quality decreases as the number of systems relations increases.
- simple (d) The psychometric approach develops measurement instruments that can be used as an alternative to classical IQ tests, as something that goes “beyond IQ”. The MicroDYN approach ( Wüstenberg et al., 2012 ) is representative for this line of research that presents an alternative to reasoning tests (like Raven matrices). These authors demonstrated that a small improvement in predicting school grade point average beyond reasoning is possible with MicroDYN tests.
- simple (e) The experimental approach explores CPS under different experimental conditions. This approach uses CPS assessment instruments to test hypotheses derived from psychological theories and is sometimes used in research about cognitive processes (see above). Exemplary for this line of research is the work by Rohe et al. (2016) , who test the usefulness of “motto goals” in the context of complex problems compared to more traditional learning and performance goals. Motto goals differ from pure performance goals by activating positive affect and should lead to better goal attainment especially in complex situations (the mentioned study found no effect).
To be clear: these five approaches are not mutually exclusive and do overlap. But the differentiation helps to identify different research communities and different traditions. These communities had different opinions about scaling complexity.
The Race for Complexity: Use of More and More Complex Systems
In the early years of CPS research, microworlds started with systems containing about 20 variables (“Tailorshop”), soon reached 60 variables (“Moro”), and culminated in systems with about 2000 variables (“Lohhausen”). This race for complexity ended with the introduction of the concept of “minimal complex systems” (MCS; Greiff and Funke, 2009 ; Funke and Greiff, 2017 ), which ushered in a search for the lower bound of complexity instead of the higher bound, which could not be defined as easily. The idea behind this concept was that whereas the upper limits of complexity are unbound, the lower limits might be identifiable. Imagine starting with a simple system containing two variables with a simple linear connection between them; then, step by step, increase the number of variables and/or the type of connections. One soon reaches a point where the system can no longer be considered simple and has become a “complex system”. This point represents a minimal complex system. Despite some research having been conducted in this direction, the point of transition from simple to complex has not been identified clearly as of yet.
Some years later, the original “minimal complex systems” approach ( Greiff and Funke, 2009 ) shifted to the “multiple complex systems” approach ( Greiff et al., 2013a ). This shift is more than a slight change in wording: it is important because it taps into the issue of validity directly. Minimal complex systems have been introduced in the context of challenges from large-scale assessments like PISA 2012 that measure new aspects of problem solving, namely interactive problems besides static problem solving ( Greiff and Funke, 2017 ). PISA 2012 required test developers to remain within testing time constraints (given by the school class schedule). Also, test developers needed a large item pool for the construction of a broad class of problem solving items. It was clear from the beginning that MCS deal with simple dynamic situations that require controlled interaction: the exploration and control of simple ticket machines, simple mobile phones, or simple MP3 players (all of these example domains were developed within PISA 2012) – rather than really complex situations like managerial or political decision making.
As a consequence of this subtle but important shift in interpreting the letters MCS, the definition of CPS became a subject of debate recently ( Funke, 2014a ; Greiff and Martin, 2014 ; Funke et al., 2017 ). In the words of Funke (2014b , p. 495):
- simple It is funny that problems that nowadays come under the term ‘CPS’, are less complex (in terms of the previously described attributes of complex situations) than at the beginning of this new research tradition. The emphasis on psychometric qualities has led to a loss of variety. Systems thinking requires more than analyzing models with two or three linear equations – nonlinearity, cyclicity, rebound effects, etc. are inherent features of complex problems and should show up at least in some of the problems used for research and assessment purposes. Minimal complex systems run the danger of becoming minimal valid systems.
Searching for minimal complex systems is not the same as gaining insight into the way how humans deal with complexity and uncertainty. For psychometric purposes, it is appropriate to reduce complexity to a minimum; for understanding problem solving under conditions of overload, intransparency, and dynamics, it is necessary to realize those attributes with reasonable strength. This aspect is illustrated in the next section.
Importance of the Validity Issue
The most important reason for discussing the question of what complex problem solving is and what it is not stems from its phenomenology: if we lose sight of our phenomena, we are no longer doing good psychology. The relevant phenomena in the context of complex problems encompass many important aspects. In this section, we discuss four phenomena that are specific to complex problems. We consider these phenomena as critical for theory development and for the construction of assessment instruments (i.e., microworlds). These phenomena require theories for explaining them and they require assessment instruments eliciting them in a reliable way.
The first phenomenon is the emergency reaction of the intellectual system ( Dörner, 1980 ): When dealing with complex systems, actors tend to (a) reduce their intellectual level by decreasing self-reflections, by decreasing their intentions, by stereotyping, and by reducing their realization of intentions, (b) they show a tendency for fast action with increased readiness for risk, with increased violations of rules, and with increased tendency to escape the situation, and (c) they degenerate their hypotheses formation by construction of more global hypotheses and reduced tests of hypotheses, by increasing entrenchment, and by decontextualizing their goals. This phenomenon illustrates the strong connection between cognition, emotion, and motivation that has been emphasized by Dörner (see, e.g., Dörner and Güss, 2013 ) from the beginning of his research tradition; the emergency reaction reveals a shift in the mode of information processing under the pressure of complexity.
The second phenomenon comprises cross-cultural differences with respect to strategy use ( Strohschneider and Güss, 1999 ; Güss and Wiley, 2007 ; Güss et al., 2015 ). Results from complex task environments illustrate the strong influence of context and background knowledge to an extent that cannot be found for knowledge-poor problems. For example, in a comparison between Brazilian and German participants, it turned out that Brazilians accept the given problem descriptions and are more optimistic about the results of their efforts, whereas Germans tend to inquire more about the background of the problems and take a more active approach but are less optimistic (according to Strohschneider and Güss, 1998 , p. 695).
The third phenomenon relates to failures that occur during the planning and acting stages ( Jansson, 1994 ; Ramnarayan et al., 1997 ), illustrating that rational procedures seem to be unlikely to be used in complex situations. The potential for failures ( Dörner, 1996 ) rises with the complexity of the problem. Jansson (1994) presents seven major areas for failures with complex situations: acting directly on current feedback; insufficient systematization; insufficient control of hypotheses and strategies; lack of self-reflection; selective information gathering; selective decision making; and thematic vagabonding.
The fourth phenomenon describes (a lack of) training and transfer effects ( Kretzschmar and Süß, 2015 ), which again illustrates the context dependency of strategies and knowledge (i.e., there is no strategy that is so universal that it can be used in many different problem situations). In their own experiment, the authors could show training effects only for knowledge acquisition, not for knowledge application. Only with specific feedback, performance in complex environments can be increased ( Engelhart et al., 2017 ).
These four phenomena illustrate why the type of complexity (or degree of simplicity) used in research really matters. Furthermore, they demonstrate effects that are specific for complex problems, but not for toy problems. These phenomena direct the attention to the important question: does the stimulus material used (i.e., the computer-simulated microworld) tap and elicit the manifold of phenomena described above?
Dealing with partly unknown complex systems requires courage, wisdom, knowledge, grit, and creativity. In creativity research, “little c” and “BIG C” are used to differentiate between everyday creativity and eminent creativity ( Beghetto and Kaufman, 2007 ; Kaufman and Beghetto, 2009 ). Everyday creativity is important for solving everyday problems (e.g., finding a clever fix for a broken spoke on my bicycle), eminent creativity changes the world (e.g., inventing solar cells for energy production). Maybe problem solving research should use a similar differentiation between “little p” and “BIG P” to mark toy problems on the one side and big societal challenges on the other. The question then remains: what can we learn about BIG P by studying little p? What phenomena are present in both types, and what phenomena are unique to each of the two extremes?
Discussing research on CPS requires reflecting on the field’s research methods. Even if the experimental approach has been successful for testing hypotheses (for an overview of older work, see Funke, 1995 ), other methods might provide additional and novel insights. Complex phenomena require complex approaches to understand them. The complex nature of complex systems imposes limitations on psychological experiments: The more complex the environments, the more difficult is it to keep conditions under experimental control. And if experiments have to be run in labs one should bring enough complexity into the lab to establish the phenomena mentioned, at least in part.
There are interesting options to be explored (again): think-aloud protocols , which have been discredited for many years ( Nisbett and Wilson, 1977 ) and yet are a valuable source for theory testing ( Ericsson and Simon, 1983 ); introspection ( Jäkel and Schreiber, 2013 ), which seems to be banned from psychological methods but nevertheless offers insights into thought processes; the use of life-streaming ( Wendt, 2017 ), a medium in which streamers generate a video stream of think-aloud data in computer-gaming; political decision-making ( Dhami et al., 2015 ) that demonstrates error-proneness in groups; historical case studies ( Dörner and Güss, 2011 ) that give insights into the thinking styles of political leaders; the use of the critical incident technique ( Reuschenbach, 2008 ) to construct complex scenarios; and simulations with different degrees of fidelity ( Gray, 2002 ).
The methods tool box is full of instruments that have to be explored more carefully before any individual instrument receives a ban or research narrows its focus to only one paradigm for data collection. Brehmer and Dörner (1993) discussed the tensions between “research in the laboratory and research in the field”, optimistically concluding “that the new methodology of computer-simulated microworlds will provide us with the means to bridge the gap between the laboratory and the field” (p. 183). The idea behind this optimism was that computer-simulated scenarios would bring more complexity from the outside world into the controlled lab environment. But this is not true for all simulated scenarios. In his paper on simulated environments, Gray (2002) differentiated computer-simulated environments with respect to three dimensions: (1) tractability (“the more training subjects require before they can use a simulated task environment, the less tractable it is”, p. 211), correspondence (“High correspondence simulated task environments simulate many aspects of one task environment. Low correspondence simulated task environments simulate one aspect of many task environments”, p. 214), and engagement (“A simulated task environment is engaging to the degree to which it involves and occupies the participants; that is, the degree to which they agree to take it seriously”, p. 217). But the mere fact that a task is called a “computer-simulated task environment” does not mean anything specific in terms of these three dimensions. This is one of several reasons why we should differentiate between those studies that do not address the core features of CPS and those that do.
What is not CPS?
Even though a growing number of references claiming to deal with complex problems exist (e.g., Greiff and Wüstenberg, 2015 ; Greiff et al., 2016 ), it would be better to label the requirements within these tasks “dynamic problem solving,” as it has been done adequately in earlier work ( Greiff et al., 2012 ). The dynamics behind on-off-switches ( Thimbleby, 2007 ) are remarkable but not really complex. Small nonlinear systems that exhibit stunningly complex and unstable behavior do exist – but they are not used in psychometric assessments of so-called CPS. There are other small systems (like MicroDYN scenarios: Greiff and Wüstenberg, 2014 ) that exhibit simple forms of system behavior that are completely predictable and stable. This type of simple systems is used frequently. It is even offered commercially as a complex problem-solving test called COMPRO ( Greiff and Wüstenberg, 2015 ) for business applications. But a closer look reveals that the label is not used correctly; within COMPRO, the used linear equations are far from being complex and the system can be handled properly by using only one strategy (see for more details Funke et al., 2017 ).
Why do simple linear systems not fall within CPS? At the surface, nonlinear and linear systems might appear similar because both only include 3–5 variables. But the difference is in terms of systems behavior as well as strategies and learning. If the behavior is simple (as in linear systems where more input is related to more output and vice versa), the system can be easily understood (participants in the MicroDYN world have 3 minutes to explore a complex system). If the behavior is complex (as in systems that contain strange attractors or negative feedback loops), things become more complicated and much more observation is needed to identify the hidden structure of the unknown system ( Berry and Broadbent, 1984 ; Hundertmark et al., 2015 ).
Another issue is learning. If tasks can be solved using a single (and not so complicated) strategy, steep learning curves are to be expected. The shift from problem solving to learned routine behavior occurs rapidly, as was demonstrated by Luchins (1942) . In his water jar experiments, participants quickly acquired a specific strategy (a mental set) for solving certain measurement problems that they later continued applying to problems that would have allowed for easier approaches. In the case of complex systems, learning can occur only on very general, abstract levels because it is difficult for human observers to make specific predictions. Routines dealing with complex systems are quite different from routines relating to linear systems.
What should not be studied under the label of CPS are pure learning effects, multiple-cue probability learning, or tasks that can be solved using a single strategy. This last issue is a problem for MicroDYN tasks that rely strongly on the VOTAT strategy (“vary one thing at a time”; see Tschirgi, 1980 ). In real-life, it is hard to imagine a business manager trying to solve her or his problems by means of VOTAT.
What is CPS?
In the early days of CPS research, planet Earth’s dynamics and complexities gained attention through such books as “The limits to growth” ( Meadows et al., 1972 ) and “Beyond the limits” ( Meadows et al., 1992 ). In the current decade, for example, the World Economic Forum (2016) attempts to identify the complexities and risks of our modern world. In order to understand the meaning of complexity and uncertainty, taking a look at the worlds’ most pressing issues is helpful. Searching for strategies to cope with these problems is a difficult task: surely there is no place for the simple principle of “vary-one-thing-at-a-time” (VOTAT) when it comes to global problems. The VOTAT strategy is helpful in the context of simple problems ( Wüstenberg et al., 2014 ); therefore, whether or not VOTAT is helpful in a given problem situation helps us distinguish simple from complex problems.
Because there exist no clear-cut strategies for complex problems, typical failures occur when dealing with uncertainty ( Dörner, 1996 ; Güss et al., 2015 ). Ramnarayan et al. (1997) put together a list of generic errors (e.g., not developing adequate action plans; lack of background control; learning from experience blocked by stereotype knowledge; reactive instead of proactive action) that are typical of knowledge-rich complex systems but cannot be found in simple problems.
Complex problem solving is not a one-dimensional, low-level construct. On the contrary, CPS is a multi-dimensional bundle of competencies existing at a high level of abstraction, similar to intelligence (but going beyond IQ). As Funke et al. (2018) state: “Assessment of transversal (in educational contexts: cross-curricular) competencies cannot be done with one or two types of assessment. The plurality of skills and competencies requires a plurality of assessment instruments.”
There are at least three different aspects of complex systems that are part of our understanding of a complex system: (1) a complex system can be described at different levels of abstraction; (2) a complex system develops over time, has a history, a current state, and a (potentially unpredictable) future; (3) a complex system is knowledge-rich and activates a large semantic network, together with a broad list of potential strategies (domain-specific as well as domain-general).
Complex problem solving is not only a cognitive process but is also an emotional one ( Spering et al., 2005 ; Barth and Funke, 2010 ) and strongly dependent on motivation (low-stakes versus high-stakes testing; see Hermes and Stelling, 2016 ).
Furthermore, CPS is a dynamic process unfolding over time, with different phases and with more differentiation than simply knowledge acquisition and knowledge application. Ideally, the process should entail identifying problems (see Dillon, 1982 ; Lee and Cho, 2007 ), even if in experimental settings, problems are provided to participants a priori . The more complex and open a given situation, the more options can be generated (T. S. Schweizer et al., 2016 ). In closed problems, these processes do not occur in the same way.
In analogy to the difference between formative (process-oriented) and summative (result-oriented) assessment ( Wiliam and Black, 1996 ; Bennett, 2011 ), CPS should not be reduced to the mere outcome of a solution process. The process leading up to the solution, including detours and errors made along the way, might provide a more differentiated impression of a person’s problem-solving abilities and competencies than the final result of such a process. This is one of the reasons why CPS environments are not, in fact, complex intelligence tests: research on CPS is not only about the outcome of the decision process, but it is also about the problem-solving process itself.
Complex problem solving is part of our daily life: finding the right person to share one’s life with, choosing a career that not only makes money, but that also makes us happy. Of course, CPS is not restricted to personal problems – life on Earth gives us many hard nuts to crack: climate change, population growth, the threat of war, the use and distribution of natural resources. In sum, many societal challenges can be seen as complex problems. To reduce that complexity to a one-hour lab activity on a random Friday afternoon puts it out of context and does not address CPS issues.
Theories about CPS should specify which populations they apply to. Across populations, one thing to consider is prior knowledge. CPS research with experts (e.g., Dew et al., 2009 ) is quite different from problem solving research using tasks that intentionally do not require any specific prior knowledge (see, e.g., Beckmann and Goode, 2014 ).
More than 20 years ago, Frensch and Funke (1995b) defined CPS as follows:
- simple CPS occurs to overcome barriers between a given state and a desired goal state by means of behavioral and/or cognitive, multi-step activities. The given state, goal state, and barriers between given state and goal state are complex, change dynamically during problem solving, and are intransparent. The exact properties of the given state, goal state, and barriers are unknown to the solver at the outset. CPS implies the efficient interaction between a solver and the situational requirements of the task, and involves a solver’s cognitive, emotional, personal, and social abilities and knowledge. (p. 18)
The above definition is rather formal and does not account for content or relations between the simulation and the real world. In a sense, we need a new definition of CPS that addresses these issues. Based on our previous arguments, we propose the following working definition:
- simple Complex problem solving is a collection of self-regulated psychological processes and activities necessary in dynamic environments to achieve ill-defined goals that cannot be reached by routine actions. Creative combinations of knowledge and a broad set of strategies are needed. Solutions are often more bricolage than perfect or optimal. The problem-solving process combines cognitive, emotional, and motivational aspects, particularly in high-stakes situations. Complex problems usually involve knowledge-rich requirements and collaboration among different persons.
The main differences to the older definition lie in the emphasis on (a) the self-regulation of processes, (b) creativity (as opposed to routine behavior), (c) the bricolage type of solution, and (d) the role of high-stakes challenges. Our new definition incorporates some aspects that have been discussed in this review but were not reflected in the 1995 definition, which focused on attributes of complex problems like dynamics or intransparency.
This leads us to the final reflection about the role of CPS for dealing with uncertainty and complexity in real life. We will distinguish thinking from reasoning and introduce the sense of possibility as an important aspect of validity.
CPS as Combining Reasoning and Thinking in an Uncertain Reality
Leading up to the Battle of Borodino in Leo Tolstoy’s novel “War and Peace”, Prince Andrei Bolkonsky explains the concept of war to his friend Pierre. Pierre expects war to resemble a game of chess: You position the troops and attempt to defeat your opponent by moving them in different directions.
“Far from it!”, Andrei responds. “In chess, you know the knight and his moves, you know the pawn and his combat strength. While in war, a battalion is sometimes stronger than a division and sometimes weaker than a company; it all depends on circumstances that can never be known. In war, you do not know the position of your enemy; some things you might be able to observe, some things you have to divine (but that depends on your ability to do so!) and many things cannot even be guessed at. In chess, you can see all of your opponent’s possible moves. In war, that is impossible. If you decide to attack, you cannot know whether the necessary conditions are met for you to succeed. Many a time, you cannot even know whether your troops will follow your orders…”
In essence, war is characterized by a high degree of uncertainty. A good commander (or politician) can add to that what he or she sees, tentatively fill in the blanks – and not just by means of logical deduction but also by intelligently bridging missing links. A bad commander extrapolates from what he sees and thus arrives at improper conclusions.
Many languages differentiate between two modes of mentalizing; for instance, the English language distinguishes between ‘thinking’ and ‘reasoning’. Reasoning denotes acute and exact mentalizing involving logical deductions. Such deductions are usually based on evidence and counterevidence. Thinking, however, is what is required to write novels. It is the construction of an initially unknown reality. But it is not a pipe dream, an unfounded process of fabrication. Rather, thinking asks us to imagine reality (“Wirklichkeitsfantasie”). In other words, a novelist has to possess a “sense of possibility” (“Möglichkeitssinn”, Robert Musil; in German, sense of possibility is often used synonymously with imagination even though imagination is not the same as sense of possibility, for imagination also encapsulates the impossible). This sense of possibility entails knowing the whole (or several wholes) or being able to construe an unknown whole that could accommodate a known part. The whole has to align with sociological and geographical givens, with the mentality of certain peoples or groups, and with the laws of physics and chemistry. Otherwise, the entire venture is ill-founded. A sense of possibility does not aim for the moon but imagines something that might be possible but has not been considered possible or even potentially possible so far.
Thinking is a means to eliminate uncertainty. This process requires both of the modes of thinking we have discussed thus far. Economic, political, or ecological decisions require us to first consider the situation at hand. Though certain situational aspects can be known, but many cannot. In fact, von Clausewitz (1832) posits that only about 25% of the necessary information is available when a military decision needs to be made. Even then, there is no way to guarantee that whatever information is available is also correct: Even if a piece of information was completely accurate yesterday, it might no longer apply today.
Once our sense of possibility has helped grasping a situation, problem solvers need to call on their reasoning skills. Not every situation requires the same action, and we may want to act this way or another to reach this or that goal. This appears logical, but it is a logic based on constantly shifting grounds: We cannot know whether necessary conditions are met, sometimes the assumptions we have made later turn out to be incorrect, and sometimes we have to revise our assumptions or make completely new ones. It is necessary to constantly switch between our sense of possibility and our sense of reality, that is, to switch between thinking and reasoning. It is an arduous process, and some people handle it well, while others do not.
If we are to believe Tuchman’s (1984) book, “The March of Folly”, most politicians and commanders are fools. According to Tuchman, not much has changed in the 3300 years that have elapsed since the misguided Trojans decided to welcome the left-behind wooden horse into their city that would end up dismantling Troy’s defensive walls. The Trojans, too, had been warned, but decided not to heed the warning. Although Laocoön had revealed the horse’s true nature to them by attacking it with a spear, making the weapons inside the horse ring, the Trojans refused to see the forest for the trees. They did not want to listen, they wanted the war to be over, and this desire ended up shaping their perception.
The objective of psychology is to predict and explain human actions and behavior as accurately as possible. However, thinking cannot be investigated by limiting its study to neatly confined fractions of reality such as the realms of propositional logic, chess, Go tasks, the Tower of Hanoi, and so forth. Within these systems, there is little need for a sense of possibility. But a sense of possibility – the ability to divine and construe an unknown reality – is at least as important as logical reasoning skills. Not researching the sense of possibility limits the validity of psychological research. All economic and political decision making draws upon this sense of possibility. By not exploring it, psychological research dedicated to the study of thinking cannot further the understanding of politicians’ competence and the reasons that underlie political mistakes. Christopher Clark identifies European diplomats’, politicians’, and commanders’ inability to form an accurate representation of reality as a reason for the outbreak of World War I. According to Clark’s (2012) book, “The Sleepwalkers”, the politicians of the time lived in their own make-believe world, wrongfully assuming that it was the same world everyone else inhabited. If CPS research wants to make significant contributions to the world, it has to acknowledge complexity and uncertainty as important aspects of it.
For more than 40 years, CPS has been a new subject of psychological research. During this time period, the initial emphasis on analyzing how humans deal with complex, dynamic, and uncertain situations has been lost. What is subsumed under the heading of CPS in modern research has lost the original complexities of real-life problems. From our point of view, the challenges of the 21st century require a return to the origins of this research tradition. We would encourage researchers in the field of problem solving to come back to the original ideas. There is enough complexity and uncertainty in the world to be studied. Improving our understanding of how humans deal with these global and pressing problems would be a worthwhile enterprise.
Author Contributions
JF drafted a first version of the manuscript, DD added further text and commented on the draft. JF finalized the manuscript.
Authors Note
After more than 40 years of controversial discussions between both authors, this is the first joint paper. We are happy to have done this now! We have found common ground!
Conflict of Interest Statement
The authors declare that the research was conducted in the absence of any commercial or financial relationships that could be construed as a potential conflict of interest.
Acknowledgments
The authors thank the Deutsche Forschungsgemeinschaft (DFG) for the continuous support of their research over many years. Thanks to Daniel Holt for his comments on validity issues, thanks to Julia Nolte who helped us by translating German text excerpts into readable English and helped us, together with Keri Hartman, to improve our style and grammar – thanks for that! We also thank the two reviewers for their helpful critical comments on earlier versions of this manuscript. Finally, we acknowledge financial support by Deutsche Forschungsgemeinschaft and Ruprecht-Karls-Universität Heidelberg within their funding programme Open Access Publishing .
1 The fMRI-paper from Anderson (2012) uses the term “complex problem solving” for tasks that do not fall in our understanding of CPS and is therefore excluded from this list.
- Alison L., van den Heuvel C., Waring S., Power N., Long A., O’Hara T., et al. (2013). Immersive simulated learning environments for researching critical incidents: a knowledge synthesis of the literature and experiences of studying high-risk strategic decision making. J. Cogn. Eng. Deci. Mak. 7 255–272. 10.1177/1555343412468113 [ CrossRef ] [ Google Scholar ]
- Anderson J. R. (2012). Tracking problem solving by multivariate pattern analysis and hidden markov model algorithms. Neuropsychologia 50 487–498. 10.1016/j.neuropsychologia.2011.07.025 [ PMC free article ] [ PubMed ] [ CrossRef ] [ Google Scholar ]
- Barth C. M., Funke J. (2010). Negative affective environments improve complex solving performance. Cogn. Emot. 24 1259–1268. 10.1080/02699930903223766 [ CrossRef ] [ Google Scholar ]
- Beckmann J. F., Goode N. (2014). The benefit of being naïve and knowing it: the unfavourable impact of perceived context familiarity on learning in complex problem solving tasks. Instruct. Sci. 42 271–290. 10.1007/s11251-013-9280-7 [ CrossRef ] [ Google Scholar ]
- Beghetto R. A., Kaufman J. C. (2007). Toward a broader conception of creativity: a case for “mini-c” creativity. Psychol. Aesthetics Creat. Arts 1 73–79. 10.1037/1931-3896.1.2.73 [ CrossRef ] [ Google Scholar ]
- Bennett R. E. (2011). Formative assessment: a critical review. Assess. Educ. Princ. Policy Pract. 18 5–25. 10.1080/0969594X.2010.513678 [ CrossRef ] [ Google Scholar ]
- Berry D. C., Broadbent D. E. (1984). On the relationship between task performance and associated verbalizable knowledge. Q. J. Exp. Psychol. 36 209–231. 10.1080/14640748408402156 [ CrossRef ] [ Google Scholar ]
- Blech C., Funke J. (2010). You cannot have your cake and eat it, too: how induced goal conflicts affect complex problem solving. Open Psychol. J. 3 42–53. 10.2174/1874350101003010042 [ CrossRef ] [ Google Scholar ]
- Brehmer B., Dörner D. (1993). Experiments with computer-simulated microworlds: escaping both the narrow straits of the laboratory and the deep blue sea of the field study. Comput. Hum. Behav. 9 171–184. 10.1016/0747-5632(93)90005-D [ CrossRef ] [ Google Scholar ]
- Buchner A. (1995). “Basic topics and approaches to the study of complex problem solving,” in Complex Problem Solving: The European Perspective , eds Frensch P. A., Funke J. (Hillsdale, NJ: Erlbaum; ), 27–63. [ Google Scholar ]
- Buchner A., Funke J. (1993). Finite state automata: dynamic task environments in problem solving research. Q. J. Exp. Psychol. 46A , 83–118. 10.1080/14640749308401068 [ CrossRef ] [ Google Scholar ]
- Clark C. (2012). The Sleepwalkers: How Europe Went to War in 1914 . London: Allen Lane. [ Google Scholar ]
- Csapó B., Funke J. (2017a). “The development and assessment of problem solving in 21st-century schools,” in The Nature of Problem Solving: Using Research to Inspire 21st Century Learning , eds Csapó B., Funke J. (Paris: OECD Publishing; ), 19–31. [ Google Scholar ]
- Csapó B., Funke J. (eds) (2017b). The Nature of Problem Solving. Using Research to Inspire 21st Century Learning. Paris: OECD Publishing. [ Google Scholar ]
- Danner D., Hagemann D., Holt D. V., Hager M., Schankin A., Wüstenberg S., et al. (2011a). Measuring performance in dynamic decision making. Reliability and validity of the Tailorshop simulation. J. Ind. Differ. 32 225–233. 10.1027/1614-0001/a000055 [ CrossRef ] [ Google Scholar ]
- Danner D., Hagemann D., Schankin A., Hager M., Funke J. (2011b). Beyond IQ: a latent state-trait analysis of general intelligence, dynamic decision making, and implicit learning. Intelligence 39 323–334. 10.1016/j.intell.2011.06.004 [ CrossRef ] [ Google Scholar ]
- Dew N., Read S., Sarasvathy S. D., Wiltbank R. (2009). Effectual versus predictive logics in entrepreneurial decision-making: differences between experts and novices. J. Bus. Ventur. 24 287–309. 10.1016/j.jbusvent.2008.02.002 [ CrossRef ] [ Google Scholar ]
- Dhami M. K., Mandel D. R., Mellers B. A., Tetlock P. E. (2015). Improving intelligence analysis with decision science. Perspect. Psychol. Sci. 10 753–757. 10.1177/1745691615598511 [ PubMed ] [ CrossRef ] [ Google Scholar ]
- Dillon J. T. (1982). Problem finding and solving. J. Creat. Behav. 16 97–111. 10.1002/j.2162-6057.1982.tb00326.x [ CrossRef ] [ Google Scholar ]
- Dörner D. (1975). Wie Menschen eine Welt verbessern wollten [How people wanted to improve a world]. Bild Der Wissenschaft 12 48–53. [ Google Scholar ]
- Dörner D. (1980). On the difficulties people have in dealing with complexity. Simulat. Gam. 11 87–106. 10.1177/104687818001100108 [ CrossRef ] [ Google Scholar ]
- Dörner D. (1996). The Logic of Failure: Recognizing and Avoiding Error in Complex Situations. New York, NY: Basic Books. [ Google Scholar ]
- Dörner D., Drewes U., Reither F. (1975). “Über das Problemlösen in sehr komplexen Realitätsbereichen,” in Bericht über den 29. Kongreß der DGfPs in Salzburg 1974 Band 1 , ed. Tack W. H. (Göttingen: Hogrefe; ), 339–340. [ Google Scholar ]
- Dörner D., Güss C. D. (2011). A psychological analysis of Adolf Hitler’s decision making as commander in chief: summa confidentia et nimius metus. Rev. Gen. Psychol. 15 37–49. 10.1037/a0022375 [ CrossRef ] [ Google Scholar ]
- Dörner D., Güss C. D. (2013). PSI: a computational architecture of cognition, motivation, and emotion. Rev. Gen. Psychol. 17 297–317. 10.1037/a0032947 [ CrossRef ] [ Google Scholar ]
- Dörner D., Kreuzig H. W., Reither F., Stäudel T. (1983). Lohhausen. Vom Umgang mit Unbestimmtheit und Komplexität. Bern: Huber. [ Google Scholar ]
- Ederer P., Patt A., Greiff S. (2016). Complex problem-solving skills and innovativeness – evidence from occupational testing and regional data. Eur. J. Educ. 51 244–256. 10.1111/ejed.12176 [ CrossRef ] [ Google Scholar ]
- Edwards W. (1962). Dynamic decision theory and probabiIistic information processing. Hum. Factors 4 59–73. 10.1177/001872086200400201 [ CrossRef ] [ Google Scholar ]
- Engelhart M., Funke J., Sager S. (2017). A web-based feedback study on optimization-based training and analysis of human decision making. J. Dynamic Dec. Mak. 3 1–23. [ Google Scholar ]
- Ericsson K. A., Simon H. A. (1983). Protocol Analysis: Verbal Reports As Data. Cambridge, MA: Bradford. [ Google Scholar ]
- Fischer A., Greiff S., Funke J. (2017). “The history of complex problem solving,” in The Nature of Problem Solving: Using Research to Inspire 21st Century Learning , eds Csapó B., Funke J. (Paris: OECD Publishing; ), 107–121. [ Google Scholar ]
- Fischer A., Holt D. V., Funke J. (2015). Promoting the growing field of dynamic decision making. J. Dynamic Decis. Mak. 1 1–3. 10.11588/jddm.2015.1.23807 [ CrossRef ] [ Google Scholar ]
- Fischer A., Holt D. V., Funke J. (2016). The first year of the “journal of dynamic decision making.” J. Dynamic Decis. Mak. 2 1–2. 10.11588/jddm.2016.1.28995 [ CrossRef ] [ Google Scholar ]
- Fischer A., Neubert J. C. (2015). The multiple faces of complex problems: a model of problem solving competency and its implications for training and assessment. J. Dynamic Decis. Mak. 1 1–14. 10.11588/jddm.2015.1.23945 [ CrossRef ] [ Google Scholar ]
- Frensch P. A., Funke J. (eds) (1995a). Complex Problem Solving: The European Perspective. Hillsdale, NJ: Erlbaum. [ Google Scholar ]
- Frensch P. A., Funke J. (1995b). “Definitions, traditions, and a general framework for understanding complex problem solving,” in Complex Problem Solving: The European Perspective , eds Frensch P. A., Funke J. (Hillsdale, NJ: Lawrence Erlbaum; ), 3–25. [ Google Scholar ]
- Frischkorn G. T., Greiff S., Wüstenberg S. (2014). The development of complex problem solving in adolescence: a latent growth curve analysis. J. Educ. Psychol. 106 1004–1020. 10.1037/a0037114 [ CrossRef ] [ Google Scholar ]
- Funke J. (1985). Steuerung dynamischer Systeme durch Aufbau und Anwendung subjektiver Kausalmodelle. Z. Psychol. 193 435–457. [ Google Scholar ]
- Funke J. (1986). Komplexes Problemlösen - Bestandsaufnahme und Perspektiven [Complex Problem Solving: Survey and Perspectives]. Heidelberg: Springer. [ Google Scholar ]
- Funke J. (1993). “Microworlds based on linear equation systems: a new approach to complex problem solving and experimental results,” in The Cognitive Psychology of Knowledge , eds Strube G., Wender K.-F. (Amsterdam: Elsevier Science Publishers; ), 313–330. [ Google Scholar ]
- Funke J. (1995). “Experimental research on complex problem solving,” in Complex Problem Solving: The European Perspective , eds Frensch P. A., Funke J. (Hillsdale, NJ: Erlbaum; ), 243–268. [ Google Scholar ]
- Funke J. (2010). Complex problem solving: a case for complex cognition? Cogn. Process. 11 133–142. 10.1007/s10339-009-0345-0 [ PubMed ] [ CrossRef ] [ Google Scholar ]
- Funke J. (2012). “Complex problem solving,” in Encyclopedia of the Sciences of Learning Vol. 38 ed. Seel N. M. (Heidelberg: Springer; ), 682–685. [ Google Scholar ]
- Funke J. (2014a). Analysis of minimal complex systems and complex problem solving require different forms of causal cognition. Front. Psychol. 5 : 739 10.3389/fpsyg.2014.00739 [ PMC free article ] [ PubMed ] [ CrossRef ] [ Google Scholar ]
- Funke J. (2014b). “Problem solving: what are the important questions?,” in Proceedings of the 36th Annual Conference of the Cognitive Science Society , eds Bello P., Guarini M., McShane M., Scassellati B. (Austin, TX: Cognitive Science Society; ), 493–498. [ Google Scholar ]
- Funke J., Fischer A., Holt D. V. (2017). When less is less: solving multiple simple problems is not complex problem solving—A comment on Greiff et al. (2015). J. Intell. 5 : 5 10.3390/jintelligence5010005 [ PMC free article ] [ PubMed ] [ CrossRef ] [ Google Scholar ]
- Funke J., Fischer A., Holt D. V. (2018). “Competencies for complexity: problem solving in the 21st century,” in Assessment and Teaching of 21st Century Skills , eds Care E., Griffin P., Wilson M. (Dordrecht: Springer; ), 3. [ Google Scholar ]
- Funke J., Greiff S. (2017). “Dynamic problem solving: multiple-item testing based on minimally complex systems,” in Competence Assessment in Education. Research, Models and Instruments , eds Leutner D., Fleischer J., Grünkorn J., Klieme E. (Heidelberg: Springer; ), 427–443. [ Google Scholar ]
- Gobert J. D., Kim Y. J., Pedro M. A. S., Kennedy M., Betts C. G. (2015). Using educational data mining to assess students’ skills at designing and conducting experiments within a complex systems microworld. Think. Skills Creat. 18 81–90. 10.1016/j.tsc.2015.04.008 [ CrossRef ] [ Google Scholar ]
- Goode N., Beckmann J. F. (2010). You need to know: there is a causal relationship between structural knowledge and control performance in complex problem solving tasks. Intelligence 38 345–352. 10.1016/j.intell.2010.01.001 [ CrossRef ] [ Google Scholar ]
- Gray W. D. (2002). Simulated task environments: the role of high-fidelity simulations, scaled worlds, synthetic environments, and laboratory tasks in basic and applied cognitive research. Cogn. Sci. Q. 2 205–227. [ Google Scholar ]
- Greiff S., Fischer A. (2013). Measuring complex problem solving: an educational application of psychological theories. J. Educ. Res. 5 38–58. [ Google Scholar ]
- Greiff S., Fischer A., Stadler M., Wüstenberg S. (2015a). Assessing complex problem-solving skills with multiple complex systems. Think. Reason. 21 356–382. 10.1080/13546783.2014.989263 [ CrossRef ] [ Google Scholar ]
- Greiff S., Stadler M., Sonnleitner P., Wolff C., Martin R. (2015b). Sometimes less is more: comparing the validity of complex problem solving measures. Intelligence 50 100–113. 10.1016/j.intell.2015.02.007 [ CrossRef ] [ Google Scholar ]
- Greiff S., Fischer A., Wüstenberg S., Sonnleitner P., Brunner M., Martin R. (2013a). A multitrait–multimethod study of assessment instruments for complex problem solving. Intelligence 41 579–596. 10.1016/j.intell.2013.07.012 [ CrossRef ] [ Google Scholar ]
- Greiff S., Holt D. V., Funke J. (2013b). Perspectives on problem solving in educational assessment: analytical, interactive, and collaborative problem solving. J. Problem Solv. 5 71–91. 10.7771/1932-6246.1153 [ CrossRef ] [ Google Scholar ]
- Greiff S., Wüstenberg S., Molnár G., Fischer A., Funke J., Csapó B. (2013c). Complex problem solving in educational contexts—something beyond g: concept, assessment, measurement invariance, and construct validity. J. Educ. Psychol. 105 364–379. 10.1037/a0031856 [ CrossRef ] [ Google Scholar ]
- Greiff S., Funke J. (2009). “Measuring complex problem solving: the MicroDYN approach,” in The Transition to Computer-Based Assessment. New Approaches to Skills Assessment and Implications for Large-Scale Testing , eds Scheuermann F., Björnsson J. (Luxembourg: Office for Official Publications of the European Communities; ), 157–163. [ Google Scholar ]
- Greiff S., Funke J. (2017). “Interactive problem solving: exploring the potential of minimal complex systems,” in The Nature of Problem Solving: Using Research to Inspire 21st Century Learning , eds Csapó B., Funke J. (Paris: OECD Publishing; ), 93–105. [ Google Scholar ]
- Greiff S., Martin R. (2014). What you see is what you (don’t) get: a comment on Funke’s (2014) opinion paper. Front. Psychol. 5 : 1120 10.3389/fpsyg.2014.01120 [ PMC free article ] [ PubMed ] [ CrossRef ] [ Google Scholar ]
- Greiff S., Neubert J. C. (2014). On the relation of complex problem solving, personality, fluid intelligence, and academic achievement. Learn. Ind. Diff. 36 37–48. 10.1016/j.lindif.2014.08.003 [ CrossRef ] [ Google Scholar ]
- Greiff S., Niepel C., Scherer R., Martin R. (2016). Understanding students’ performance in a computer-based assessment of complex problem solving: an analysis of behavioral data from computer-generated log files. Comput. Hum. Behav. 61 36–46. 10.1016/j.chb.2016.02.095 [ CrossRef ] [ Google Scholar ]
- Greiff S., Stadler M., Sonnleitner P., Wolff C., Martin R. (2017). Sometimes more is too much: a rejoinder to the commentaries on Greif et al. (2015). J. Intell. 5 : 6 10.3390/jintelligence5010006 [ PMC free article ] [ PubMed ] [ CrossRef ] [ Google Scholar ]
- Greiff S., Wüstenberg S. (2014). Assessment with microworlds using MicroDYN: measurement invariance and latent mean comparisons. Eur. J. Psychol. Assess. 1 1–11. 10.1027/1015-5759/a000194 [ CrossRef ] [ Google Scholar ]
- Greiff S., Wüstenberg S. (2015). Komplexer Problemlösetest COMPRO [Complex Problem-Solving Test COMPRO]. Mödling: Schuhfried. [ Google Scholar ]
- Greiff S., Wüstenberg S., Funke J. (2012). Dynamic problem solving: a new assessment perspective. Appl. Psychol. Measure. 36 189–213. 10.1177/0146621612439620 [ CrossRef ] [ Google Scholar ]
- Griffin P., Care E. (2015). “The ATC21S method,” in Assessment and Taching of 21st Century Skills , eds Griffin P., Care E. (Dordrecht, NL: Springer; ), 3–33. [ Google Scholar ]
- Güss C. D., Dörner D. (2011). Cultural differences in dynamic decision-making strategies in a non-linear, time-delayed task. Cogn. Syst. Res. 12 365–376. 10.1016/j.cogsys.2010.12.003 [ CrossRef ] [ Google Scholar ]
- Güss C. D., Tuason M. T., Orduña L. V. (2015). Strategies, tactics, and errors in dynamic decision making in an Asian sample. J. Dynamic Deci. Mak. 1 1–14. 10.11588/jddm.2015.1.13131 [ CrossRef ] [ Google Scholar ]
- Güss C. D., Wiley B. (2007). Metacognition of problem-solving strategies in Brazil, India, and the United States. J. Cogn. Cult. 7 1–25. 10.1163/156853707X171793 [ CrossRef ] [ Google Scholar ]
- Herde C. N., Wüstenberg S., Greiff S. (2016). Assessment of complex problem solving: what we know and what we don’t know. Appl. Meas. Educ. 29 265–277. 10.1080/08957347.2016.1209208 [ CrossRef ] [ Google Scholar ]
- Hermes M., Stelling D. (2016). Context matters, but how much? Latent state – trait analysis of cognitive ability assessments. Int. J. Sel. Assess. 24 285–295. 10.1111/ijsa.12147 [ CrossRef ] [ Google Scholar ]
- Hotaling J. M., Fakhari P., Busemeyer J. R. (2015). “Dynamic decision making,” in International Encyclopedia of the Social & Behavioral Sciences , 2nd Edn, eds Smelser N. J., Batles P. B. (New York, NY: Elsevier; ), 709–714. [ Google Scholar ]
- Hundertmark J., Holt D. V., Fischer A., Said N., Fischer H. (2015). System structure and cognitive ability as predictors of performance in dynamic system control tasks. J. Dynamic Deci. Mak. 1 1–10. 10.11588/jddm.2015.1.26416 [ CrossRef ] [ Google Scholar ]
- Jäkel F., Schreiber C. (2013). Introspection in problem solving. J. Problem Solv. 6 20–33. 10.7771/1932-6246.1131 [ CrossRef ] [ Google Scholar ]
- Jansson A. (1994). Pathologies in dynamic decision making: consequences or precursors of failure? Sprache Kogn. 13 160–173. [ Google Scholar ]
- Kaufman J. C., Beghetto R. A. (2009). Beyond big and little: the four c model of creativity. Rev. Gen. Psychol. 13 1–12. 10.1037/a0013688 [ CrossRef ] [ Google Scholar ]
- Knauff M., Wolf A. G. (2010). Complex cognition: the science of human reasoning, problem-solving, and decision-making. Cogn. Process. 11 99–102. 10.1007/s10339-010-0362-z [ PubMed ] [ CrossRef ] [ Google Scholar ]
- Kretzschmar A. (2017). Sometimes less is not enough: a commentary on Greiff et al. (2015). J. Intell. 5 : 4 10.3390/jintelligence5010004 [ PMC free article ] [ PubMed ] [ CrossRef ] [ Google Scholar ]
- Kretzschmar A., Neubert J. C., Wüstenberg S., Greiff S. (2016). Construct validity of complex problem solving: a comprehensive view on different facets of intelligence and school grades. Intelligence 54 55–69. 10.1016/j.intell.2015.11.004 [ CrossRef ] [ Google Scholar ]
- Kretzschmar A., Süß H.-M. (2015). A study on the training of complex problem solving competence. J. Dynamic Deci. Mak. 1 1–14. 10.11588/jddm.2015.1.15455 [ CrossRef ] [ Google Scholar ]
- Lee H., Cho Y. (2007). Factors affecting problem finding depending on degree of structure of problem situation. J. Educ. Res. 101 113–123. 10.3200/JOER.101.2.113-125 [ CrossRef ] [ Google Scholar ]
- Leutner D., Fleischer J., Wirth J., Greiff S., Funke J. (2012). Analytische und dynamische Problemlösekompetenz im Lichte internationaler Schulleistungsvergleichsstudien: Untersuchungen zur Dimensionalität. Psychol. Rundschau 63 34–42. 10.1026/0033-3042/a000108 [ CrossRef ] [ Google Scholar ]
- Luchins A. S. (1942). Mechanization in problem solving: the effect of einstellung. Psychol. Monogr. 54 1–95. 10.1037/h0093502 [ CrossRef ] [ Google Scholar ]
- Mack O., Khare A., Krämer A., Burgartz T. (eds) (2016). Managing in a VUCA world. Heidelberg: Springer. [ Google Scholar ]
- Mainert J., Kretzschmar A., Neubert J. C., Greiff S. (2015). Linking complex problem solving and general mental ability to career advancement: does a transversal skill reveal incremental predictive validity? Int. J. Lifelong Educ. 34 393–411. 10.1080/02601370.2015.1060024 [ CrossRef ] [ Google Scholar ]
- Mainzer K. (2009). Challenges of complexity in the 21st century. An interdisciplinary introduction. Eur. Rev. 17 219–236. 10.1017/S1062798709000714 [ CrossRef ] [ Google Scholar ]
- Meadows D. H., Meadows D. L., Randers J. (1992). Beyond the Limits. Vermont, VA: Chelsea Green Publishing. [ Google Scholar ]
- Meadows D. H., Meadows D. L., Randers J., Behrens W. W. (1972). The Limits to Growth. New York, NY: Universe Books. [ Google Scholar ]
- Meißner A., Greiff S., Frischkorn G. T., Steinmayr R. (2016). Predicting complex problem solving and school grades with working memory and ability self-concept. Learn. Ind. Differ. 49 323–331. 10.1016/j.lindif.2016.04.006 [ CrossRef ] [ Google Scholar ]
- Molnàr G., Greiff S., Wüstenberg S., Fischer A. (2017). “Empirical study of computer-based assessment of domain-general complex problem-solving skills,” in The Nature of Problem Solving: Using research to Inspire 21st Century Learning , eds Csapó B., Funke J. (Paris: OECD Publishing; ), 125–141. [ Google Scholar ]
- National Research Council (2011). Assessing 21st Century Skills: Summary of a Workshop. Washington, DC: The National Academies Press. [ PubMed ] [ Google Scholar ]
- Newell A., Shaw J. C., Simon H. A. (1959). A general problem-solving program for a computer. Comput. Automat. 8 10–16. [ Google Scholar ]
- Nisbett R. E., Wilson T. D. (1977). Telling more than we can know: verbal reports on mental processes. Psychol. Rev. 84 231–259. 10.1037/0033-295X.84.3.231 [ CrossRef ] [ Google Scholar ]
- OECD (2014). “PISA 2012 results,” in Creative Problem Solving: Students’ Skills in Tackling Real-Life problems , Vol. 5 (Paris: OECD Publishing; ). [ Google Scholar ]
- Osman M. (2010). Controlling uncertainty: a review of human behavior in complex dynamic environments. Psychol. Bull. 136 65–86. 10.1037/a0017815 [ PubMed ] [ CrossRef ] [ Google Scholar ]
- Osman M. (2012). The role of reward in dynamic decision making. Front. Neurosci. 6 : 35 10.3389/fnins.2012.00035 [ PMC free article ] [ PubMed ] [ CrossRef ] [ Google Scholar ]
- Qudrat-Ullah H. (2015). Better Decision Making in Complex, Dynamic Tasks. Training with Human-Facilitated Interactive Learning Environments. Heidelberg: Springer. [ Google Scholar ]
- Ramnarayan S., Strohschneider S., Schaub H. (1997). Trappings of expertise and the pursuit of failure. Simulat. Gam. 28 28–43. 10.1177/1046878197281004 [ CrossRef ] [ Google Scholar ]
- Reuschenbach B. (2008). Planen und Problemlösen im Komplexen Handlungsfeld Pflege. Berlin: Logos. [ Google Scholar ]
- Rohe M., Funke J., Storch M., Weber J. (2016). Can motto goals outperform learning and performance goals? Influence of goal setting on performance, intrinsic motivation, processing style, and affect in a complex problem solving task. J. Dynamic Deci. Mak. 2 1–15. 10.11588/jddm.2016.1.28510 [ CrossRef ] [ Google Scholar ]
- Scherer R., Greiff S., Hautamäki J. (2015). Exploring the relation between time on task and ability in complex problem solving. Intelligence 48 37–50. 10.1016/j.intell.2014.10.003 [ CrossRef ] [ Google Scholar ]
- Schoppek W., Fischer A. (2015). Complex problem solving – single ability or complex phenomenon? Front. Psychol. 6 : 1669 10.3389/fpsyg.2015.01669 [ PMC free article ] [ PubMed ] [ CrossRef ] [ Google Scholar ]
- Schraw G., Dunkle M., Bendixen L. D. (1995). Cognitive processes in well-defined and ill-defined problem solving. Appl. Cogn. Psychol. 9 523–538. 10.1002/acp.2350090605 [ CrossRef ] [ Google Scholar ]
- Schweizer F., Wüstenberg S., Greiff S. (2013). Validity of the MicroDYN approach: complex problem solving predicts school grades beyond working memory capacity. Learn. Ind. Differ. 24 42–52. 10.1016/j.lindif.2012.12.011 [ CrossRef ] [ Google Scholar ]
- Schweizer T. S., Schmalenberger K. M., Eisenlohr-Moul T. A., Mojzisch A., Kaiser S., Funke J. (2016). Cognitive and affective aspects of creative option generation in everyday life situations. Front. Psychol. 7 : 1132 10.3389/fpsyg.2016.01132 [ PMC free article ] [ PubMed ] [ CrossRef ] [ Google Scholar ]
- Selten R., Pittnauer S., Hohnisch M. (2012). Dealing with dynamic decision problems when knowledge of the environment is limited: an approach based on goal systems. J. Behav. Deci. Mak. 25 443–457. 10.1002/bdm.738 [ CrossRef ] [ Google Scholar ]
- Simon H. A. (1957). Administrative Behavior: A Study of Decision-Making Processes in Administrative Organizations , 2nd Edn New York, NY: Macmillan. [ Google Scholar ]
- Sonnleitner P., Brunner M., Keller U., Martin R. (2014). Differential relations between facets of complex problem solving and students’ immigration background. J. Educ. Psychol. 106 681–695. 10.1037/a0035506 [ CrossRef ] [ Google Scholar ]
- Spering M., Wagener D., Funke J. (2005). The role of emotions in complex problem solving. Cogn. Emot. 19 1252–1261. 10.1080/02699930500304886 [ CrossRef ] [ Google Scholar ]
- Stadler M., Becker N., Gödker M., Leutner D., Greiff S. (2015). Complex problem solving and intelligence: a meta-analysis. Intelligence 53 92–101. 10.1016/j.intell.2015.09.005 [ CrossRef ] [ Google Scholar ]
- Stadler M., Niepel C., Greiff S. (2016). Easily too difficult: estimating item difficulty in computer simulated microworlds. Comput. Hum. Behav. 65 100–106. 10.1016/j.chb.2016.08.025 [ CrossRef ] [ Google Scholar ]
- Sternberg R. J. (1995). “Expertise in complex problem solving: a comparison of alternative conceptions,” in Complex Problem Solving: The European Perspective , eds Frensch P. A., Funke J. (Hillsdale, NJ: Erlbaum; ), 295–321. [ Google Scholar ]
- Sternberg R. J., Frensch P. A. (1991). Complex Problem Solving: Principles and Mechanisms. (eds) Sternberg R. J., Frensch P. A. Hillsdale, NJ: Erlbaum. [ Google Scholar ]
- Strohschneider S., Güss C. D. (1998). Planning and problem solving: differences between brazilian and german students. J. Cross-Cult. Psychol. 29 695–716. 10.1177/0022022198296002 [ CrossRef ] [ Google Scholar ]
- Strohschneider S., Güss C. D. (1999). The fate of the Moros: a cross-cultural exploration of strategies in complex and dynamic decision making. Int. J. Psychol. 34 235–252. 10.1080/002075999399873 [ CrossRef ] [ Google Scholar ]
- Thimbleby H. (2007). Press On. Principles of Interaction. Cambridge, MA: MIT Press. [ Google Scholar ]
- Tobinski D. A., Fritz A. (2017). “EcoSphere: a new paradigm for problem solving in complex systems,” in The Nature of Problem Solving: Using Research to Inspire 21st Century Learning , eds Csapó B., Funke J. (Paris: OECD Publishing; ), 211–222. [ Google Scholar ]
- Tremblay S., Gagnon J.-F., Lafond D., Hodgetts H. M., Doiron M., Jeuniaux P. P. J. M. H. (2017). A cognitive prosthesis for complex decision-making. Appl. Ergon. 58 349–360. 10.1016/j.apergo.2016.07.009 [ PubMed ] [ CrossRef ] [ Google Scholar ]
- Tschirgi J. E. (1980). Sensible reasoning: a hypothesis about hypotheses. Child Dev. 51 1–10. 10.2307/1129583 [ CrossRef ] [ Google Scholar ]
- Tuchman B. W. (1984). The March of Folly. From Troy to Vietnam. New York, NY: Ballantine Books. [ Google Scholar ]
- Verweij M., Thompson M. (eds) (2006). Clumsy Solutions for A Complex World. Governance, Politics and Plural Perceptions. New York, NY: Palgrave Macmillan; 10.1057/9780230624887 [ CrossRef ] [ Google Scholar ]
- Viehrig K., Siegmund A., Funke J., Wüstenberg S., Greiff S. (2017). “The heidelberg inventory of geographic system competency model,” in Competence Assessment in Education. Research, Models and Instruments , eds Leutner D., Fleischer J., Grünkorn J., Klieme E. (Heidelberg: Springer; ), 31–53. [ Google Scholar ]
- von Clausewitz C. (1832). Vom Kriege [On war]. Berlin: Dämmler. [ Google Scholar ]
- Wendt A. N. (2017). The empirical potential of live streaming beyond cognitive psychology. J. Dynamic Deci. Mak. 3 1–9. 10.11588/jddm.2017.1.33724 [ CrossRef ] [ Google Scholar ]
- Wiliam D., Black P. (1996). Meanings and consequences: a basis for distinguishing formative and summative functions of assessment? Br. Educ. Res. J. 22 537–548. 10.1080/0141192960220502 [ CrossRef ] [ Google Scholar ]
- World Economic Forum (2015). New Vsion for Education Unlocking the Potential of Technology. Geneva: World Economic Forum. [ Google Scholar ]
- World Economic Forum (2016). Global Risks 2016: Insight Report , 11th Edn Geneva: World Economic Forum. [ Google Scholar ]
- Wüstenberg S., Greiff S., Funke J. (2012). Complex problem solving — more than reasoning? Intelligence 40 1–14. 10.1016/j.intell.2011.11.003 [ CrossRef ] [ Google Scholar ]
- Wüstenberg S., Greiff S., Vainikainen M.-P., Murphy K. (2016). Individual differences in students’ complex problem solving skills: how they evolve and what they imply. J. Educ. Psychol. 108 1028–1044. 10.1037/edu0000101 [ CrossRef ] [ Google Scholar ]
- Wüstenberg S., Stadler M., Hautamäki J., Greiff S. (2014). The role of strategy knowledge for the application of strategies in complex problem solving tasks. Technol. Knowl. Learn. 19 127–146. 10.1007/s10758-014-9222-8 [ CrossRef ] [ Google Scholar ]
- Computer Vision
- Federated Learning
- Reinforcement Learning
- Natural Language Processing
- New Releases
- AI Dev Tools
- Advisory Board Members
- 🐝 Partnership and Promotion
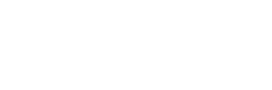
A detailed analysis reveals that the incorporation of filler tokens allows transformers to solve complex algorithmic problems, such as the 3SUM problem, with high accuracy. For instance, in experiments where transformers were provided with filler tokens, the models achieved perfect accuracy on the 3SUM problem with input lengths up to 12, demonstrating a significant computational advantage over models operating without such tokens.
The research quantitatively illustrates the performance improvement with filler tokens. Models trained with these tokens surpassed the baseline immediate-answer models and exhibited enhanced problem-solving abilities on more complex tasks. Specifically, filler tokens consistently improved model performance in setups where the input sequence involved higher-dimensional data, achieving accuracies near 100% on tasks that would otherwise stump models without this augmentation.

In conclusion, the study demonstrates that traditional transformer model limitations can be overcome by integrating nonsensical filler tokens into their input sequences. This innovative method bypasses the constraints of standard token utilization and significantly enhances the models’ computational capabilities. By employing filler tokens, researchers were able to improve the performance of transformers on complex tasks such as the 3SUM problem, where they achieved near-perfect accuracy. These results highlight a promising new direction for enhancing AI problem-solving abilities and suggest a potential paradigm shift in how computational resources are managed within language models.
Check out the Paper. All credit for this research goes to the researchers of this project. Also, don’t forget to follow us on Twitter . Join our Telegram Channel , Discord Channel , and LinkedIn Gr oup .
If you like our work, you will love our newsletter..
Don’t Forget to join our 40k+ ML SubReddit
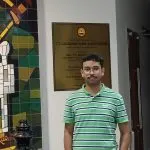
Sana Hassan
Sana Hassan, a consulting intern at Marktechpost and dual-degree student at IIT Madras, is passionate about applying technology and AI to address real-world challenges. With a keen interest in solving practical problems, he brings a fresh perspective to the intersection of AI and real-life solutions.
- Sana Hassan https://www.marktechpost.com/author/sana-hassan/ OpenVoice V2: Evolving Multilingual Voice Cloning with Enhanced Style Control and Cross-Lingual Capabilities
- Sana Hassan https://www.marktechpost.com/author/sana-hassan/ SEED-Bench-2-Plus: An Extensive Benchmark Specifically Designed for Evaluating Multimodal Large Language Models (MLLMs) in Text-Rich Scenarios
- Sana Hassan https://www.marktechpost.com/author/sana-hassan/ This Machine Learning Paper from ICMC-USP, NYU, and Capital-One Introduces T-Explainer: A Novel AI Framework for Consistent and Reliable Machine Learning Model Explanations
- Sana Hassan https://www.marktechpost.com/author/sana-hassan/ Microsoft's GeckOpt Optimizes Large Language Models: Enhancing Computational Efficiency with Intent-Based Tool Selection in Machine Learning Systems
RELATED ARTICLES MORE FROM AUTHOR
Llama-3-based openbiollm-llama3-70b and 8b: outperforming gpt-4, gemini, meditron-70b, med-palm-1 and med-palm-2 in medical-domain, openvoice v2: evolving multilingual voice cloning with enhanced style control and cross-lingual capabilities, physics-based deep learning: insights into physics-informed neural networks (pinns), researchers at uc san diego propose drs: a novel machine learning approach for learning reusable dense rewards for multi-stage tasks in a data-driven manner, seed-bench-2-plus: an extensive benchmark specifically designed for evaluating multimodal large language models (mllms) in text-rich scenarios, syntheval: a novel open-source machine learning framework for detailed utility and privacy evaluation of tabular synthetic data, researchers at uc san diego propose drs: a novel machine learning approach for learning..., seed-bench-2-plus: an extensive benchmark specifically designed for evaluating multimodal large language models (mllms) in..., syntheval: a novel open-source machine learning framework for detailed utility and privacy evaluation of..., this ai paper introduces a novel artificial intelligence approach in precision text retrieval using..., top data science courses in 2024, drbenchmark: the first-ever publicly available french biomedical large language understanding benchmark, this ai paper from apple introduces a weakly-supervised pre-training method for vision models using....
- AI Magazine
- Privacy & TC
- Cookie Policy
🐝 FREE AI Webinar: AI/ML-Driven Forecasting for Power Demand, Supply & Pricing [May 3, 2024]
Thank You 🙌
Privacy Overview
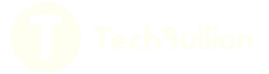
TechBullion
A comprehensive guide to director interview questions.

The Art of Interviewing: A Comprehensive Guide to Director Interview Questions
Interviewing candidates for a director-level role requires thoughtful preparation and strategic questioning to assess critical leadership qualities. Well-crafted director interview questions reveal how potential hires approach complex problem-solving, communicate vision, manage teams, oversee budgets, and drive results.
Understanding the Director Role
Before drafting targeted questions, it’s essential to understand a director’s multifaceted responsibilities. As strategic leaders, directors are accountable for:
- Strategic Planning and Goal Setting: Directors develop long-term strategic plans that align with the company’s mission and vision. They also set specific goals and benchmarks for measuring success.
- Team Leadership and Management: Directors build, lead, and manage teams of managers, providing oversight for junior staff productivity, development, and culture.
- Performance Management: Directors track departmental and employee performance versus goals, initiating improvement plans as needed.
- Financial Management and Budgeting: Directors oversee budgets, allocate resources efficiently, control costs, and ensure financial accountability across their division.
- Risk Management: Directors assess potential risks related to their operations and implement appropriate risk mitigation strategies and contingency plans.
- Communication and Collaboration: Directors collaborate cross-functionally to achieve organizational objectives, communicating frequently with stakeholders.
- Problem-Solving and Decision-Making: Directors analyze issues, weigh solutions, and make timely data-driven decisions, even in uncertainty.
- Talent Development: Directors nurture talent, building benches of future leaders to sustain growth.
Crafting Powerful Director Interview Questions
Here are key strategies to craft effective director interview questions:
- Specificity: Avoid vague, open-ended questions that allow for general, theoretical responses. Frame questions around specific leadership scenarios relevant to the role.
- Open-mindedness: Encourage detailed responses by primarily using “what,” “why,” and “how” questions stems rather than yes/no or short answer questions.
- Clarity and Conciseness: Be clear and concise, so candidates understand exactly what knowledge or experience you’re exploring with each question.
- Consistency Across Candidates: Ask each candidate the same baseline script of questions to allow easier comparisons between responses.
- Include Follow-Up Questions: Draft 2-3 follow-ups for each scripted question to dive deeper into the initial response when warranted.
- Match Questions to Skills: Ensure questions directly correlate with required leadership capabilities for success in this role.
- Review with Stakeholders: Allow key hiring decision-makers to review and provide input on draft questions.
- Adapt Questions to the Role: Tailor questions to specialty areas like Operations, IT, Finance, Marketing, etc.
Thorough preparation dramatically improves the interview’s effectiveness for evaluating director-caliber candidates.
Director Interview Questions by Category
Let’s delve into specific questions for each interview category, providing examples tailored for different director roles:
Leadership Philosophy
These big-picture questions reveal leadership style and values:
- What does effective leadership mean to you? How have you demonstrated those qualities recently?
- How would you describe your leadership style and approach?
- What are some leadership principles or philosophies that guide you?
Follow-up questions could probe what factors shaped their leadership style, how they adapted their approach across different roles or the challenges they’ve faced implementing their style.
Strategy and Vision
Uncover strategic thinking capabilities with questions like:
- What is your approach to developing a comprehensive strategic plan for your division?
- How do you distill an organization’s overarching strategy into an actionable plan for your department?
- Describe how you have aligned your team’s goals to corporate objectives in past roles.
Dig deeper into their strategic planning process, factors they consider, how they drive alignment, and how they course correct strategy.
Financial Oversight
Assess budgeting experience and financial acumen with questions that include:
- Discuss your experience creating and managing large budgets. What have been your biggest challenges and successes?
- How have you optimized financial performance in past leadership roles? What are some strategies and tools you’ve used?
- Describe a time you needed to reduce expenses without sacrificing operational performance.
Follow up on how they allocate resources, approach cost control, identify inefficiencies, and balance budgets amid complex demands.
Team Leadership
Build a picture of their team leadership skills . Sample questions:
- Discuss your approach to leading and managing a large team to drive results. Describe strategies for effectively delegating and empowering staff.
- How do you foster professional growth and development on your teams?
- What are some best practices you employ to cultivate team culture and morale?
Ask candidates to share examples demonstrating their leadership capabilities and team development effectiveness.
Talent Development
Explore their commitment to mentoring emerging leaders by asking:
- How have you supported talent development and succession planning in your past roles?
- What strategies and opportunities do you utilize to nurture high potential future leaders on your team?
- Describe your mentoring style. What core advice do you find yourself giving developing leaders?
Dig deeper into formal programs they’ve implemented, lessons learned from mentees, and their philosophies on nurturing talent.
Agility and Change Management
Assess adaptability by inquiring:
- How do you drive buy-in and adoption for major initiatives or changes?
- Describe a time you needed to pivot strategy quickly in response to sudden changes in the market or industry. How did you approach and communicate this to your team?
- Tell me about a time you implemented a substantial change or improvement within your department. How did you get staff aligned with the vision??
In their responses, look for demonstrations of clear communication, empathy, flexibility, and change leadership skills.
Problem-Solving
Understand their problem-solving process by asking:
- Walk me through how you recently solved a complex business challenge or issue your department was facing.
- Describe a time when you needed to be creative in developing solutions under a tight budget, time, or resource constraints.
- What is your process for making difficult strategic decisions with long-term consequences?
Listen for a sound, logical decision-making approach rooted in data analysis, stakeholder input, and analytical thinking.
Conflict Management
Assess people skills important for directors:
- Describe a time you needed to resolve a tense conflict between colleagues or business partners. How did you approach this?
- Tell me about a time you coached an employee through a behavior issue affecting their performance. How did you approach this sensitive topic?
- How do you gain alignment when stakeholders have sharply opposing views on strategic priorities or plans?
Probe into their communication skills, empathy, mediation strategies, and composure during conflict.
Learn how they handle and rebound from failure:
- Tell me about a time one of your initiatives or projects failed. What factors contributed to this outcome? Most importantly, how did you respond?
- Describe a mistake you made early in your leadership career and what you learned from that experience.
- How do you handle criticism or disagreement from your team on business decisions?
Listen for accountability, reflection, and commitment to continuous improvement.
Evaluating Responses and Identifying Red Flags
When evaluating responses, watch for these red flags:
- Lack of Specifics: Vague responses lacking real examples may indicate embellished experience.
- No Follow-Through Examples: The inability to cite tangible outcomes their actions achieved suggests ineffectiveness.
- Blames Others: Consistently faulting subordinates, resources, or management for failures points to poor accountability.
- Unclear Communication: Answers that meander or lack concision raise concerns about directing teams.
- No Strategic Vision: Candidates who get lost in tactics tying back to objectives may need a more strategic perspective.
- Flawed Decision-Making Process: Illogical or emotion-driven choices versus data-based analysis are worrisome.
- People Problems: Directors must collaborate effectively at all levels. Red flags include authoritarian tendencies, lack of empathy, conflict avoidance, or conformity over conviction.
- Cultural Misfit: Look for philosophies, motivations, and values aligned with your workplace culture for optimal retention and performance.
Hiring a new director is a consequential decision for any organization. This leadership role demands both a strategic perspective and the ability to execute tactically. An incisive interview process is essential for evaluating candidates’ qualifications. The director should exemplify skills like financial oversight, talent development, change management, analytical thinking, and team leadership. Carefully crafted questions that conversationally probe these capabilities reveal who will best fulfill this vital position. Selecting the right director ensures your organization has the leadership in place to achieve its mission and drive long-term success.
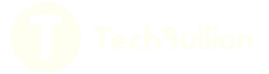
Recommended for you
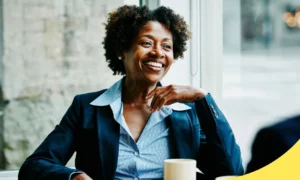
Trending Stories
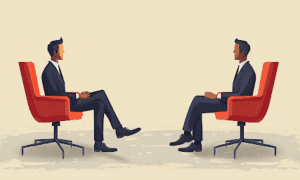
The Art of Interviewing: A Comprehensive Guide to Director Interview Questions Interviewing candidates for...
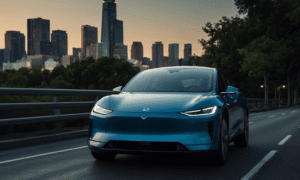
Former Tesla SVP Drew Baglino To Sell $181.5 Million Worth Of Stock
The SEC filed a document on Thursday revealing that former Tesla executive Drew Baglino,...
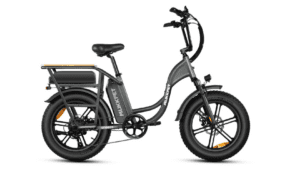
Discover the Mukkpet BREEZE+: Redefining Home Commuting and Cargo Transport
Ready to make your daily commute and family outings a whole lot more exciting?...
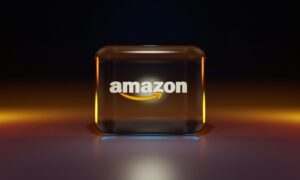
AWS Plans to Invest $11 Billion in Indiana to Create 1,000 New Jobs
AWS plans to invest $11 billion in Indiana to create at least 1,000 new...
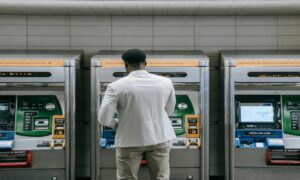
From Traditional to Tech-Savvy: How Corporate Banking is Evolving with Technology
As the world becomes increasingly digital, the corporate banking sector is also experiencing a...
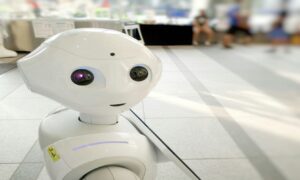
Alibaba’s Dingtalk Upgrades AI and Launched a Marketplace of 200 AI-Powered Agents
Alibaba’s Dingtalk has upgraded its AI and launched a marketplace of 200 AI-powered agents....
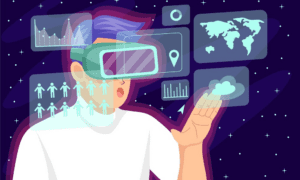
Immersive media: The Revolution of Digital Experiences
Discover the impact of the digital revolution in today’s world Immersive media is a...
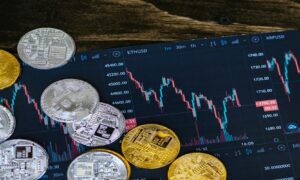
The Future of Money: How Cryptocurrency is Transforming the Financial Industry
Welcome to the future of money, where digital currencies are revolutionizing the financial industry...
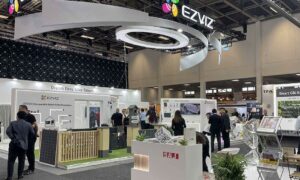
Major Technology and Innovation Exhibitions in Germany in 2024 and 2025
Technology and innovation exhibitions play an important role in today’s world. At no time...
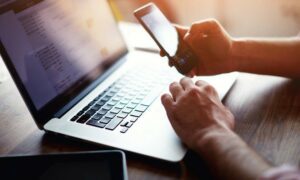
How Small Business Owners Can Boost Their Online Presence
If 2022 taught us anything about small businesses, it is that nothing is certain....
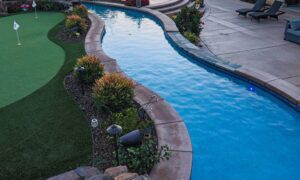
Essential Tips for Choosing the Right Pool Builder in Central Texas
Choosing the right pool builder is a crucial decision for any homeowner in Central...
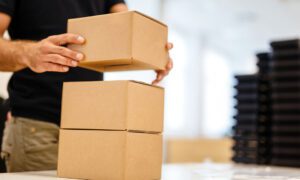
Mastering the Art of Packaging: Best Practices for Using Ship Boxes in E-commerce
In the e-commerce world, the saying “first impressions count” is more relevant than ever....
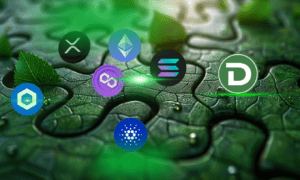
Ark Invest Buys $40M Worth Of Its Spot Bitcoin ETF; DTX Presale Records Exciting Whale Activity
TLDR Bitcoin (BTC) dips 0.10% post-Ark Invest’s Bitcoin ETF purchase. Stage 2 of DTX...
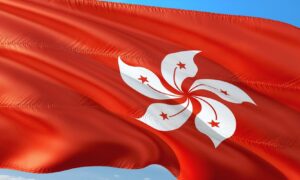
Alibaba Cloud Celebrates its 10th Anniversary in Hong Kong.
Alibaba Cloud has celebrated its 10th anniversary in Hong Kong with different events. Takeaway...
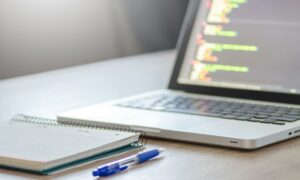
How Does AI Contribute to Data-Driven Decision-Making in Startups?
How Does AI Contribute to Data-Driven Decision-Making in Startups? In the fast-paced world of...
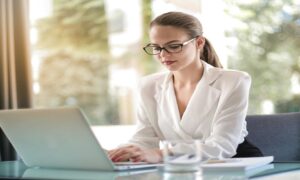
8 Ways Corporate Banking Technology is Changing the Financial Ecosystem
Corporate banking technology is revolutionizing the way businesses and institutions handle their financial transactions,...
The Future of Banking: How Corporate Banking Technology is Changing the Game
Gone are the days of waiting in long lines at the bank or having...
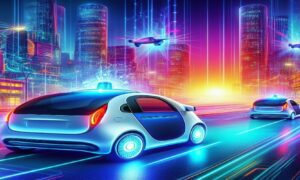
Steering Towards Safety: How AI is Revolutionizing Public Transport
Today, we’re diving deep into a topic that’s as fascinating as it is vital...
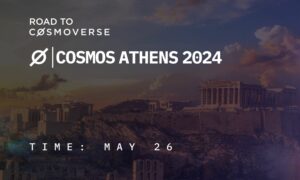
Cosmos Athens 2024: Uniting Devs & Blockchain Visionaries in Greece
Mark your calendars for Cosmos Athens 2024, a premier blockchain technology summit powered by...
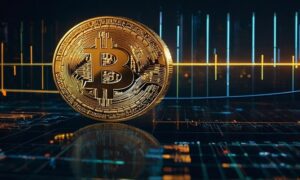
The Evolution of Cryptocurrency Exchanges
Exchanges play a pivotal role in facilitating the buying, selling, and trading of...
Like Us On Facebook
Latest interview.
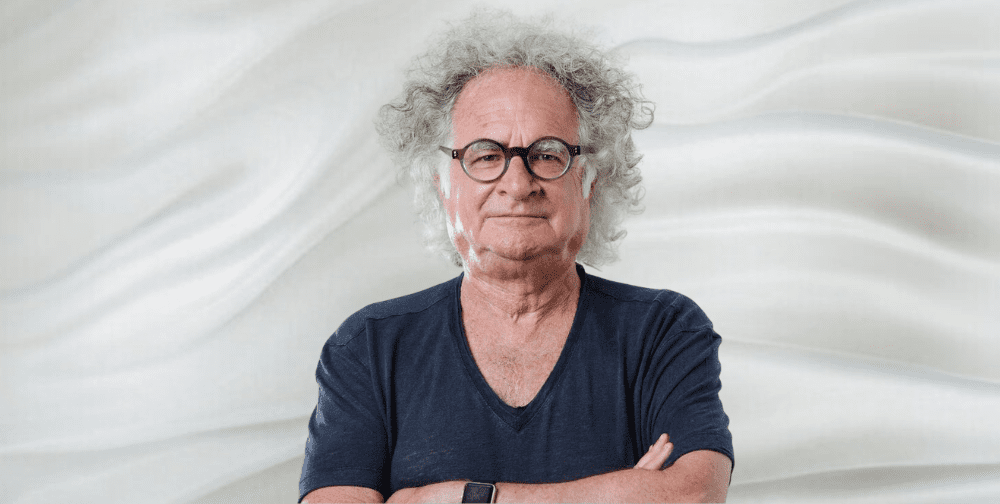
Avram Miller Talks About Renovaro And Its Impact In Diagnosing Cancers and Infectious Diseases With AI
The potential impact of AI on healthcare is vast and transformative. With the use of AI platforms for diagnosing diseases like cancer,...
Latest Press Release
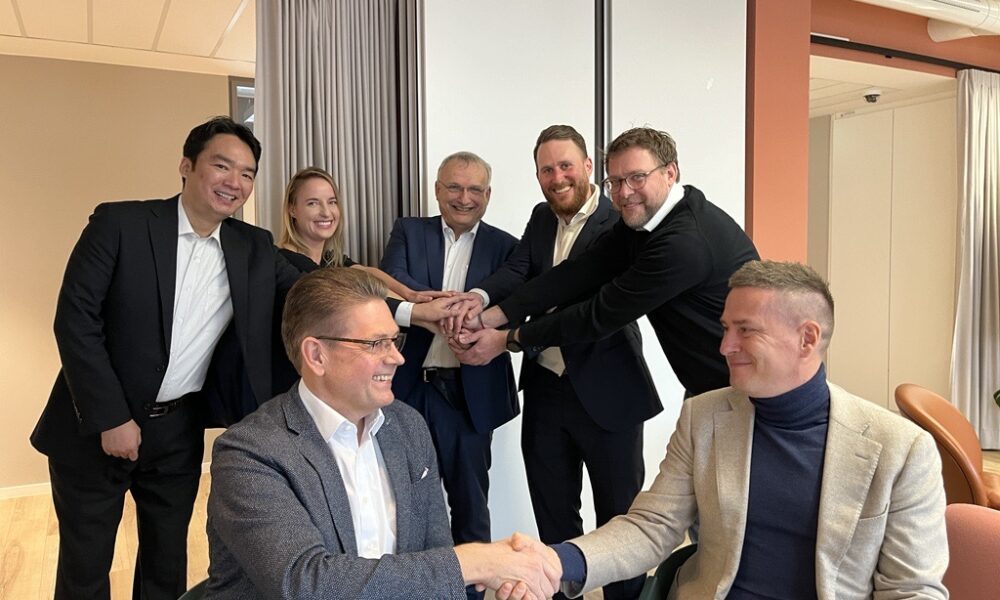
NTT DATA and Reiz Tech Announce New Venture to Target DACH
The joint venture – LITIT — built on previous collaborative successes of its parent companies, aims to transform the IT landscape of...
Pin It on Pinterest
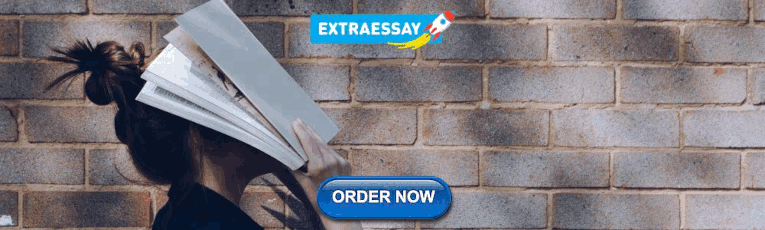
IMAGES
VIDEO
COMMENTS
Complex problem solving is a series of observations and informed decisions used to find and implement a solution to a problem. Beyond finding and implementing a solution, complex problem solving also involves considering future changes to circumstance, resources and capabilities that may affect the trajectory of the process and success of the ...
Collaborating with others can help you develop your complex problem-solving skills. Working in a team environment can expose you to new ideas and approaches, help you identify blind spots, and provide opportunities for feedback and support. 3.2.5 Seek Out Challenging Problems. Developing complex problem-solving skills requires practice.
Not every problem-solving approach is right for every challenge, and deciding on the right method for the challenge at hand is a key part of being an effective team. ... A process of iterating and making small changes quickly can help generate momentum and an appetite for solving complex problems. Problem-solving strategies can live and die on ...
And third, pay attention to how you're feeling. Embracing complexity means learning to better manage tough emotions like fear and anger. The problems we're facing often seem as complex as they ...
1. Overview. In The 7 Timeless Steps to Guide You Through Complex Problem Solving, we discussed a generic approach that could be systematically applied to solving complex problems.Since not all problems are complex, and many gradations of complexity exist, it is probably a good idea to start by defining what complex problem-solving involves and what categories of problems are most suitable to ...
Complex problem solving is not only a cognitive process but is also an emotional one (Spering et al., 2005; Barth and ... "Microworlds based on linear equation systems: a new approach to complex problem solving and experimental results," in The Cognitive Psychology of Knowledge, eds G. Strube and K.-F. Wender (Amsterdam: Elsevier Science ...
In insight problem-solving, the cognitive processes that help you solve a problem happen outside your conscious awareness. 4. Working backward. Working backward is a problem-solving approach often ...
The McKinsey guide to problem solving. Become a better problem solver with insights and advice from leaders around the world on topics including developing a problem-solving mindset, solving problems in uncertain times, problem solving with AI, and much more.
Complex problem solving takes place for reducing the barrier between a given start state and an intended goal state with the help of cognitive activities and behavior. Start state, intended goal state, and barriers prove complexity, change dynamically over time, and can be partially intransparent. In contrast to solving simple problems, with ...
The Complex Problem Solving program provides a sound but flexible framework for a structured approach to business or personal dilemmas. It enables a situation to be better understood and a clear, detailed reasoning of the possibilities for resolution.
All episodes. Details. Transcript. December 04, 2018. Corey Phelps, a strategy professor at McGill University, says great problem solvers are hard to find. Even seasoned professionals at the ...
Finding a suitable solution for issues can be accomplished by following the basic four-step problem-solving process and methodology outlined below. Step. Characteristics. 1. Define the problem. Differentiate fact from opinion. Specify underlying causes. Consult each faction involved for information. State the problem specifically.
To effectively tackle complex challenges, a strategic approach is essential. This approach involves a systematic and comprehensive analysis of the problem, identification of root causes, and ...
Problem-solving is a mental process that involves discovering, analyzing, and solving problems. The ultimate goal of problem-solving is to overcome obstacles and find a solution that best resolves the issue. The best strategy for solving a problem depends largely on the unique situation. In some cases, people are better off learning everything ...
This complex problem-solving course introduces participants to MIT's unique, powerful, and integrative System Dynamics approach to assess problems that will not go away and to produce the results they want. Through exercises and simulation models, participants experience the long-term side effects and impacts of decisions and understand the ...
Specialization - 4 course series. SOLVING COMPLEX PROBLEMS will teach you revolutionary new problem-solving skills. Involving lectures from over 50 experts from all faculties at Macquarie University, we look at solving complex problems in a way that has never been done before. Please note that this specialisation will be discontinued on Monday ...
Complex problem solving is an effective means to engage students in disciplinary content while also furnishing critical non-cognitive and life skills. Despite increased adoption of complex problem-solving methods in K-12 classrooms today (e.g., case-, project-, or problem-based learning), we know little about how to make these approaches accessible to linguistically and culturally diverse (LCD ...
In this 5-day course, transform your approach to large-scale problem solving, from multi-stakeholder engineering projects to the online spread of misinformation. Alongside engineers and leaders from diverse industries, you'll explore actionable innovative frameworks for assessing, communicating, and implementing complex systems—and ...
When you reach a good-enough-for-now idea, end the meeting and try it. Often teams reach this point in 30 minutes and then spend the next couple of hours trying to perfect it, because they're ...
Complex problem solving (CPS) is considered to be one of the most important skills for successful learning. In an effort to explore the nature of CPS, this study aims to investigate the role of inductive reasoning (IR) and combinatorial reasoning (CR) in the problem-solving process of students using statistically distinguishable exploration strategies in the CPS environment.
Here are some things you can do to improve your ability to solve complex problems: 1. Always be learning. Prepare your mind to be a bit faster and deal with things in a better way by constantly ...
Creative problem solving is a valuable skill that can transform the way you approach and resolve complex issues. It involves thinking outside the box and applying innovative methods to find solutions.
Problem-solving leads to the development of skills essential for solving complex, real-world situations. These skills, including problem-solving, creativity, communication, cooperation, and ...
Go to: Computer-simulated scenarios have been part of psychological research on problem solving for more than 40 years. The shift in emphasis from simple toy problems to complex, more real-life oriented problems has been accompanied by discussions about the best ways to assess the process of solving complex problems.
Models trained with these tokens surpassed the baseline immediate-answer models and exhibited enhanced problem-solving abilities on more complex tasks. Specifically, filler tokens consistently improved model performance in setups where the input sequence involved higher-dimensional data, achieving accuracies near 100% on tasks that would ...
The Art of Interviewing: A Comprehensive Guide to Director Interview Questions Interviewing candidates for a director-level role requires thoughtful preparation and strategic questioning to assess critical leadership qualities. Well-crafted director interview questions reveal how potential hires approach complex problem-solving, communicate vision, manage teams, oversee budgets, and drive results.