Systematic Literature Review of E-Learning Capabilities to Enhance Organizational Learning
- Open access
- Published: 01 February 2021
- Volume 24 , pages 619–635, ( 2022 )
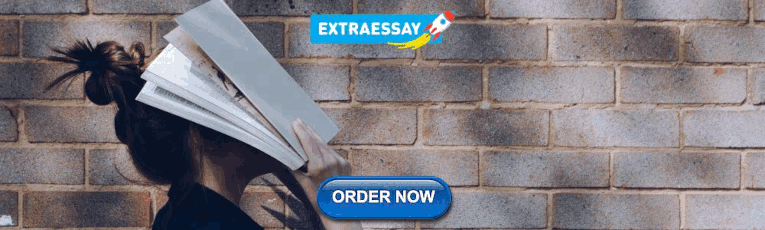
Cite this article
You have full access to this open access article
- Michail N. Giannakos 1 ,
- Patrick Mikalef 1 &
- Ilias O. Pappas ORCID: orcid.org/0000-0001-7528-3488 1 , 2
22k Accesses
33 Citations
Explore all metrics
E-learning systems are receiving ever increasing attention in academia, business and public administration. Major crises, like the pandemic, highlight the tremendous importance of the appropriate development of e-learning systems and its adoption and processes in organizations. Managers and employees who need efficient forms of training and learning flow within organizations do not have to gather in one place at the same time or to travel far away to attend courses. Contemporary affordances of e-learning systems allow users to perform different jobs or tasks for training courses according to their own scheduling, as well as to collaborate and share knowledge and experiences that result in rich learning flows within organizations. The purpose of this article is to provide a systematic review of empirical studies at the intersection of e-learning and organizational learning in order to summarize the current findings and guide future research. Forty-seven peer-reviewed articles were collected from a systematic literature search and analyzed based on a categorization of their main elements. This survey identifies five major directions of the research on the confluence of e-learning and organizational learning during the last decade. Future research should leverage big data produced from the platforms and investigate how the incorporation of advanced learning technologies (e.g., learning analytics, personalized learning) can help increase organizational value.
Similar content being viewed by others
Technology-Enhanced Organizational Learning: A Systematic Literature Review
Knowledge Transfer Through E-learning: Case of Tunisian Post
Organizational e-Learning Systems’ Success in Industry
Avoid common mistakes on your manuscript.
1 Introduction
E-learning covers the integration of information and communication technology (ICT) in environments with the main goal of fostering learning (Rosenberg and Foshay 2002 ). The term “e-learning” is often used as an umbrella term to portray several modes of digital learning environments (e.g., online, virtual learning environments, social learning technologies). Digitalization seems to challenge numerous business models in organizations and raises important questions about the meaning and practice of learning and development (Dignen and Burmeister 2020 ). Among other things, the digitalization of resources and processes enables flexible ways to foster learning across an organization’s different sections and personnel.
Learning has long been associated with formal or informal education and training. However organizational learning is much more than that. It can be defined as “a learning process within organizations that involves the interaction of individual and collective (group, organizational, and inter-organizational) levels of analysis and leads to achieving organizations’ goals” (Popova-Nowak and Cseh 2015 ) with a focus on the flow of knowledge across the different organizational levels (Oh 2019 ). Flow of knowledge or learning flow is the way in which new knowledge flows from the individual to the organizational level (i.e., feed forward) and vice versa (i.e., feedback) (Crossan et al. 1999 ; March 1991 ). Learning flow and the respective processes constitute the cornerstone of an organization’s learning activities (e.g., from physical training meetings to digital learning resources), they are directly connected to the psycho-social experiences of an organization’s members, and they eventually lead to organizational change (Crossan et al. 2011 ). The overall organizational learning is extremely important in an organization because it is associated with the process of creating value from an organizations’ intangible assets. Moreover, it combines notions from several different domains, such as organizational behavior, human resource management, artificial intelligence, and information technology (El Kadiri et al. 2016 ).
A growing body of literature lies at the intersection of e-learning and organizational learning. However, there is limited work on the qualities of e-learning and the potential of its qualities to enhance organizational learning (Popova-Nowak and Cseh 2015 ). Blockages and disruptions in the internal flow of knowledge is a major reason why organizational change initiatives often fail to produce their intended results (Dee and Leisyte 2017 ). In recent years, several models of organizational learning have been published (Berends and Lammers 2010 ; Oh 2019 ). However, detailed empirical studies indicate that learning does not always proceed smoothly in organizations; rather, the learning meets interruptions and breakdowns (Engeström et al. 2007 ).
Discontinuities and disruptions are common phenomena in organizational learning (Berends and Lammers 2010 ), and they stem from various causes. For example, organizational members’ low self-esteem, unsupportive technology and instructors (Garavan et al. 2019 ), and even crises like the Covid-19 pandemic can result in demotivated learners and overall unwanted consequences for their learning (Broadbent 2017 ). In a recent conceptual article, Popova-Nowak and Cseh ( 2015 ) emphasized that there is a limited use of multidisciplinary perspectives to investigate and explain the processes and importance of utilizing the available capabilities and resources and of creating contexts where learning is “attractive to individual agents so that they can be more engaged in exploring ways in which they can contribute through their learning to the ongoing renewal of organizational routines and practices” (Antonacopoulou and Chiva 2007 , p. 289).
Despite the importance of e-learning, the lack of systematic reviews in this area significantly hinders research on the highly promising value of e-learning capabilities for efficiently supporting organizational learning. This gap leaves practitioners and researchers in uncharted territories when faced with the task of implementing e-learning designs or deciding on their digital learning strategies to enhance the learning flow of their organizations. Hence, in order to derive meaningful theoretical and practical implications, as well as to identify important areas for future research, it is critical to understand how the core capabilities pertinent to e-learning possess the capacity to enhance organizational learning.
In this paper, we define e-learning enhanced organizational learning (eOL) as the utilization of digital technologies to enhance the process of improving actions through better knowledge and understanding in an organization. In recent years, a significant body of research has focused on the intersection of e-learning and organizational learning (e.g., Khandakar and Pangil 2019 ; Lin et al. 2019 ; Menolli et al. 2020 ; Turi et al. 2019 ; Xiang et al. 2020 ). However, there is a lack of systematic work that summarizes and conceptualizes the results in order to support organizations that want to move from being information-based enterprises to being knowledge-based ones (El Kadiri et al. 2016 ). In particular, recent technological advances have led to an increase in research that leverages e-learning capacities to support organizational learning, from virtual reality (VR) environments (Costello and McNaughton 2018 ; Muller Queiroz et al. 2018 ) to mobile computing applications (Renner et al. 2020 ) to adaptive learning and learning analytics (Zhang et al. 2019 ). These studies support different skills, consider different industries and organizations, and utilize various capacities while focusing on various learning objectives (Garavan et al. 2019 ). Our literature review aims to tease apart these particularities and to investigate how these elements have been utilized over the past decade in eOL research. Therefore, in this review we aim to answer the following research questions (RQs):
RQ1: What is the status of research at the intersection of e-learning and organizational learning, seen through the lens of areas of implementation (e.g., industries, public sector), technologies used, and methodologies (e.g., types of data and data analysis techniques employed)?
RQ2: How can e-learning be leveraged to enhance the process of improving actions through better knowledge and understanding in an organization?
Our motivation for this work is based on the emerging developments in the area of learning technologies that have created momentum for their adoption by organizations. This paper provides a review of research on e-learning capabilities to enhance organizational learning with the purpose of summarizing the findings and guiding future studies. This study can provide a springboard for other scholars and practitioners, especially in the area of knowledge-based enterprises, to examine e-learning approaches by taking into consideration the prior and ongoing research efforts. Therefore, in this paper we present a systematic literature review (SLR) (Kitchenham and Charters 2007 ) on the confluence of e-learning and organizational learning that uncovers initial findings on the value of e-learning to support organizational learning while also delineating several promising research streams.
The rest of this paper is organized as follows. In the next section, we present the related background work. The third section describes the methodology used for the literature review and how the studies were selected and analyzed. The fourth section presents the research findings derived from the data analysis based on the specific areas of focus. In the fifth section, we discuss the findings, the implications for practice and research, and the limitations of the selected methodological approach. In the final section, we summarize the conclusions from the study and make suggestions for future work.
2 Background and Related Work
2.1 e-learning systems.
E-learning systems provide solutions that deliver knowledge and information, facilitate learning, and increase performance by developing appropriate knowledge flow inside organizations (Menolli et al. 2020 ). Putting into practice and appropriately managing technological solutions, processes, and resources are necessary for the efficient utilization of e-learning in an organization (Alharthi et al. 2019 ). Examples of e-learning systems that have been widely adopted by various organizations are Canvas, Blackboard, and Moodle. Such systems provide innovative services for students, employees, managers, instructors, institutions, and other actors to support and enhance the learning processes and facilitate efficient knowledge flow (Garavan et al. 2019 ). Functionalities, such as creating modules to organize mini course information and learning materials or communication channels such as chat, forums, and video exchange, allow instructors and managers to develop appropriate training and knowledge exchange (Wang et al. 2011 ). Nowadays, the utilization of various e-learning capabilities is a commodity for supporting organizational and workplace learning. Such learning refers to training or knowledge development (also known in the literature as learning and development, HR development, and corporate training: Smith and Sadler-Smith 2006 ; Garavan et al. 2019 ) that takes place in the context of work.
Previous studies have focused on evaluating e-learning systems that utilize various models and frameworks. In particular, the development of maturity models, such as the e-learning capability maturity model (eLCMM), addresses technology-oriented concerns (Hammad et al. 2017 ) by overcoming the limitations of the domain-specific models (e.g., game-based learning: Serrano et al. 2012 ) or more generic lenses such as the e-learning maturity model (Marshall 2006 ). The aforementioned models are very relevant since they focus on assessing the organizational capabilities for sustainably developing, deploying, and maintaining e-learning. In particular, the eLCMM focuses on assessing the maturity of adopting e-learning systems and adds a feedback building block for improving learners’ experiences (Hammad et al. 2017 ). Our proposed literature review builds on the previously discussed models, lenses, and empirical studies, and it provides a review of research on e-learning capabilities with the aim of enhancing organizational learning in order to complement the findings of the established models and guide future studies.
E-learning systems can be categorized into different types, depending on their functionalities and affordances. One very popular e-learning type is the learning management system (LMS), which includes a virtual classroom and collaboration capabilities and allows the instructor to design and orchestrate a course or a module. An LMS can be either proprietary (e.g., Blackboard) or open source (e.g., Moodle). These two types differ in their features, costs, and the services they provide; for example, proprietary systems prioritize assessment tools for instructors, whereas open-source systems focus more on community development and engagement tools (Alharthi et al. 2019 ). In addition to LMS, e-learning systems can be categorized based on who controls the pace of learning; for example, an institutional learning environment (ILE) is provided by the organization and is usually used for instructor-led courses, while a personal learning environment (PLE) is proposed by the organization and is managed personally (i.e., learner-led courses). Many e-learning systems use a hybrid version of ILE and PLE that allows organizations to have either instructor-led or self-paced courses.
Besides the controlled e-learning systems, organizations have been using environments such as social media (Qi and Chau 2016 ), massive open online courses (MOOCs) (Weinhardt and Sitzmann 2018 ) and other web-based environments (Wang et al. 2011 ) to reinforce their organizational learning potential. These systems have been utilized through different types of technology (e.g., desktop applications, mobile) that leverage the various capabilities offered (e.g., social learning, VR, collaborative systems, smart and intelligent support) to reinforce the learning and knowledge flow potential of the organization. Although there is a growing body of research on e-learning systems for organizational learning due to the increasingly significant role of skills and expertise development in organizations, the role and alignment of the capabilities of the various e-learning systems with the expected competency development remains underexplored.
2.2 Organizational Learning
There is a large body of research on the utilization of technologies to improve the process and outcome dimensions of organizational learning (Crossan et al. 1999 ). Most studies have focused on the learning process and on the added value that new technologies can offer by replacing some of the face-to-face processes with virtual processes or by offering new, technology-mediated phases to the process (Menolli et al. 2020 ; Lau 2015 ) highlighted how VR capabilities can enhance organizational learning, describing the new challenges and frameworks needed in order to effectively utilize this potential. In the same vein, Zhang et al. ( 2017 ) described how VR influences reflective thinking and considered its indirect value to overall learning effectiveness. In general, contemporary research has investigated how novel technologies and approaches have been utilized to enhance organizational learning, and it has highlighted both the promises and the limitations of the use of different technologies within organizations.
In many organizations, alignment with the established infrastructure and routines, and adoption by employees are core elements for effective organizational learning (Wang et al. 2011 ). Strict policies, low digital competence, and operational challenges are some of the elements that hinder e-learning adoption by organizations (Garavan et al. 2019 ; Wang 2018 ) demonstrated the importance of organizational, managerial, and job support for utilizing individual and social learning in order to increase the adoption of organizational learning. Other studies have focused on the importance of communication through different social channels to develop understanding of new technology, to overcome the challenges employees face when engaging with new technology, and, thereby, to support organizational learning (Menolli et al. 2020 ). By considering the related work in the area of organizational learning, we identified a gap in aligning an organization’s learning needs with the capabilities offered by the various technologies. Thus, systematic work is needed to review e-learning capabilities and how these capabilities can efficiently support organizational learning.
2.3 E-learning Systems to Enhance Organizational Learning
When considering the interplay between e-learning systems and organizational learning, we observed that a major challenge for today’s organizations is to switch from being information-based enterprises to become knowledge-based enterprises (El Kadiri et al. 2016 ). Unidirectional learning flows, such as formal and informal training, are important but not sufficient to cover the needs that enterprises face (Manuti et al. 2015 ). To maintain enterprises’ competitiveness, enterprise staff have to operate in highly intense information and knowledge-oriented environments. Traditional learning approaches fail to substantiate learning flow on the basis of daily evidence and experience. Thus, novel, ubiquitous, and flexible learning mechanisms are needed, placing humans (e.g., employees, managers, civil servants) at the center of the information and learning flow and bridging traditional learning with experiential, social, and smart learning.
Organizations consider lack of skills and competences as being the major knowledge-related factors hampering innovation (El Kadiri et al. 2016 ). Thus, solutions need to be implemented that support informal, day-to-day, and work training (e.g., social learning, collaborative learning, VR/AR solutions) in order to develop individual staff competences and to upgrade the competence affordances at the organizational level. E-learning-enhanced organizational learning has been delivered primarily in the form of web-based learning (El Kadiri et al. 2016 ). More recently, the TEL tools portfolio has rapidly expanded to make more efficient joint use of novel learning concepts, methodologies, and technological enablers to achieve more direct, effective, and lasting learning impacts. Virtual learning environments, mobile-learning solutions, and AR/VR technologies and head-mounted displays have been employed so that trainees are empowered to follow their own training pace, learning topics, and assessment tests that fit their needs (Costello and McNaughton 2018 ; Mueller et al. 2011 ; Muller Queiroz et al. 2018 ). The expanding use of social networking tools has also brought attention to the contribution of social and collaborative learning (Hester et al. 2016 ; Wei and Ram 2016 ).
Contemporary learning systems supporting adaptive, personalized, and collaborative learning expand the tools available in eOL and contribute to the adoption, efficiency, and general prospects of the introduction of TEL in organizations (Cheng et al. 2011 ). In recent years, eOL has emphasized how enterprises share knowledge internally and externally, with particular attention being paid to systems that leverage collaborative learning and social learning functionalities (Qi and Chau 2016 ; Wang 2011 ). This is the essence of computer-supported collaborative learning (CSCL). The CSCL literature has developed a framework that combines individual learning, organizational learning, and collaborative learning, facilitated by establishing adequate learning flows and emerges effective learning in an enterprise learning (Goggins et al. 2013 ), in Fig. 1 .
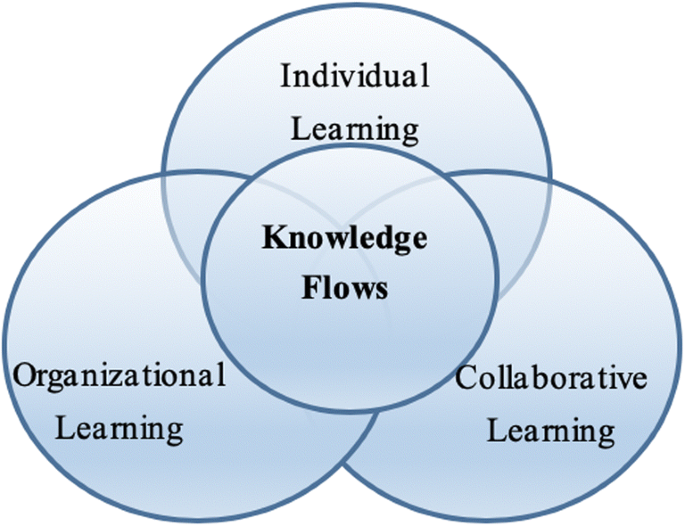
Representation of the combination of enterprise learning and knowledge flows. (adapted from Goggins et al. 2013 )
Establishing efficient knowledge and learning flows is a primary target for future data-driven enterprises (El Kadiri et al. 2016 ). Given the involved knowledge, the human resources, and the skills required by enterprises, there is a clear need for continuous, flexible, and efficient learning. This can be met by contemporary learning systems and practices that provide high adoption, smooth usage, high satisfaction, and close alignment with the current practices of an enterprise. Because the required competences of an enterprise evolve, the development of competence models needs to be agile and to leverage state-of-the art technologies that align with the organization’s processes and models. Therefore, in this paper we provide a review of the eOL research in order to summarize the findings, identify the various capabilities of eOL, and guide the development of organizational learning in future enterprises as well as in future studies.
3 Methodology
To answer our research questions, we conducted an SLR, which is a means of evaluating and interpreting all available research relevant to a particular research question, topic area, or phenomenon of interest. A SLR has the capacity to present a fair evaluation of a research topic by using a trustworthy, rigorous, and auditable methodology (Kitchenham and Charters 2007 ). The guidelines used (Kitchenham and Charters 2007 ) were derived from three existing guides adopted by medical researchers. Therefore, we adopted SLR guidelines that follow transparent and widely accepted procedures (especially in the area of software engineering and information systems, as well as in e-learning), minimize potential bias (researchers), and support reproducibility (Kitchenham and Charters 2007 ). Besides the minimization of bias and support for reproducibility, an SLR allows us to provide information about the impact of some phenomenon across a wide range of settings, contexts, and empirical methods. Another important advantage is that, if the selected studies give consistent results, SLRs can provide evidence that the phenomenon is robust and transferable (Kitchenham and Charters 2007 ).
3.1 Article Collection
Several procedures were followed to ensure a high-quality review of the literature of eOL. A comprehensive search of peer-reviewed articles was conducted in February 2019 (short papers, posters, dissertations, and reports were excluded), based on a relatively inclusive range of key terms: “organizational learning” & “elearning”, “organizational learning” & “e-learning”, “organisational learning” & “elearning”, and “organisational learning” & “e-learning”. Publications were selected from 2010 onwards, because we identified significant advances since 2010 (e.g., MOOCs, learning analytics, personalized learning) in the area of learning technologies. A wide variety of databases were searched, including SpringerLink, Wiley, ACM Digital Library, IEEE Xplore, Science Direct, SAGE, ERIC, AIS eLibrary, and Taylor & Francis. The selected databases were aligned with the SLR guidelines (Kitchenham and Charters 2007 ) and covered the major venues in IS and educational technology (e.g., a basket of eight IS journals, the top 20 journals in the Google Scholar IS subdiscipline, and the top 20 journals in the Google Scholar Educational Technology subdiscipline). The search process uncovered 2,347 peer-reviewed articles.
3.2 Inclusion and Exclusion Criteria
The selection phase determines the overall validity of the literature review, and thus it is important to define specific inclusion and exclusion criteria. As Dybå and Dingsøyr ( 2008 ) specified, the quality criteria should cover three main issues – namely, rigor, credibility, and relevance – that need to be considered when evaluating the quality of the selected studies. We applied eight quality criteria informed by the proposed Critical Appraisal Skills Programme (CASP) and related works (Dybå and Dingsøyr 2008 ). Table 1 presents these criteria.
Therefore, studies were eligible for inclusion if they were focused on eOL. The aforementioned criteria were applied in stages 2 and 3 of the selection process (see Fig. 2 ), when we assessed the papers based on their titles and abstracts, and read the full papers. From March 2020, we performed an additional search (stage 4) following the same process for papers published after the initial search period (i.e., 2010–February 2019). The additional search returned seven papers. Figure 2 summarizes the stages of the selection process.
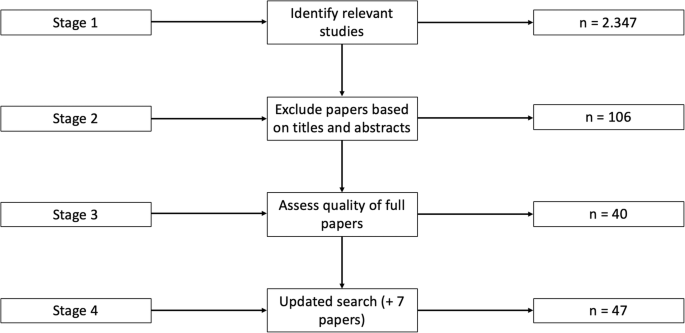
Stages of the selection process
3.3 Analysis
Each collected study was analyzed based on the following elements: study design (e.g., experiment, case study), area (e.g., IT, healthcare), technology (e.g., wiki, social media), population (e.g., managers, employees), sample size, unit of analysis (individual, firm), data collections (e.g., surveys, interviews), research method, data analysis, and the main research objective of the study. It is important to highlight that the articles were coded based on the reported information, that different authors reported information at different levels of granularity (e.g., an online system vs. the name of the system), and that in some cases the information was missing from the paper. Overall, we endeavored to code the articles as accurately and completely as possible.
The coding process was iterative with regular consensus meetings between the two researchers involved. The primary coder prepared the initial coding for a number of articles and both coders reviewed and agreed on the coding in order to reach the final codes presented in the Appendix . Disagreements between the coders and inexplicit aspects of the reviewed papers were discussed and resolved in regular consensus meetings. Although this process did not provide reliability indices (e.g., Cohen’s kappa), it did provide certain reliability in terms of consistency of the coding and what Krippendorff ( 2018 ) stated as the reliability of “the degree to which members of a designated community concur on the readings, interpretations, responses to, or uses of given texts or data”, which is considered acceptable research practice (McDonald et al. 2019 ).
In this section, we present the detailed results of the analysis of the 47 papers. Analysis of the studies was performed using non-statistical methods that considered the variables reported in the Appendix . This section is followed by an analysis and discussion of the categories.
4.1 Sample Size and Population Involved
The categories related to the sample of the articles and included the number of participants in each study (size), their position (e.g., managers, employees), and the area/topic covered by the study. The majority of the studies involved employees (29), with few studies involving managers (6), civil servants (2), learning specialists (2), clients, and researchers. Regarding the sample size, approximately half of the studies (20) were conducted with fewer than 100 participants; some (12) can be considered large-scale studies (more than 300 participants); and only a few (9) can be considered small scale (fewer than 20 participants). In relation to the area/topic of the study, most studies (11) were conducted in the context of the IT industry, but there was also good coverage of other important areas (i.e., healthcare, telecommunications, business, public sector). Interestingly, several studies either did not define the area or were implemented in a generic context (sector-agnostic studies, n = 10), and some studies were implemented in a multi-sector context (e.g., participants from different sections or companies, n = 4).
4.2 Research Methods
When assessing the status of research for an area, one of the most important aspects is the methodology used. By “method” in the Appendix , we refer to the distinction between quantitative, qualitative, and mixed methods research. In addition to the method, in our categorization protocol we also included “study design” to refer to the distinction between survey studies (i.e., those that gathered data by asking a group of participants), experiments (i.e., those that created situations to record beneficial data), and case studies (i.e., those that closely studied a group of individuals).
Based on this categorization, the Appendix shows that the majority of the papers were quantitative (34) and qualitative (7), with few studies (6) utilizing mixed methods. Regarding the study design, most of the studies were survey studies (26), 13 were case studies, and fewer were experiments (8). For most studies, the individual participant (40) was the unit of analysis, with few studies having the firm as the unit of analysis, and only one study using the training session as a unit of analysis. Regarding the measures used in the studies, most utilized surveys (39), with 11 using interviews, and only a few studies using field notes from focus groups (2) and log files from the systems (2). Only eight studies involved researchers using different measures to triangulate or extend their findings. Most articles used structural equation modeling (SEM) (17) to analyze their data, with 13 studies employing descriptive statistics, seven using content analysis, nine using regression analysis or analyses of variances/covariance, and one study using social network analysis (SNA).
4.3 Technologies
Concerning the technology used, most of the studies (17) did not study a specific system, referring instead in their investigation to a generic e-learning or technological solution. Several studies (9) named web-based learning environments, without describing the functionalities of the identified system. Other studies focused on online learning environments (4), collaborative learning systems (3), social learning systems (3), smart learning systems (2), podcasting (2), with the rest of the studies using a specific system (e.g., a wiki, mobile learning, e-portfolios, Second Life, web application).
4.4 Research Objectives
The research objectives of the studies could be separated into six main categories. The first category focuses on the intention of the employees to use the technology (9); the second focuses on the performance of the employees (8); the third focuses on the value/outcome for the organization (4); the fourth focuses on the actual usage of the system (7); the fifth focuses on employees’ satisfaction (4); and the sixth focuses on the ability of the proposed system to foster learning (9). In addition to these six categories, we also identified studies that focused on potential barriers for eOL in organizations (Stoffregen et al. 2016 ), the various benefits associated with the successful implementation of eOL (Liu et al. 2012 ), the feasibility of eOL (Kim et al. 2014 ; Mueller et al. 2011 ), and the alignment of the proposed innovation with the other processes and systems in the organization (Costello and McNaughton 2018 ).
4.5 E-learning Capabilities in Various Organizations and for Various Objectives
The technology used has an inherent role for both the organization and the expected eOL objective. E-learning systems are categorized based on their functionalities and affordances. Based on the information reported in the selected papers, we ranked them based on the different technologies and functionalities (e.g., collaborative, online, smart). To do so, we focused on the main elements described in the selected paper; for instance, a paper that described the system as wiki-based or indicated that the system was Second Life was ranked as such, rather than being added to collaborative systems or social learning respectively. We did this because we wanted to capture all the available information since it gave us additional insights (e.g., Second Life is both a social and a VR system).
To investigate the connection between the various technologies used to enhance organizational learning and their application in the various organizations, we utilized the coding (see Appendix ) and mapped the various e-learning technologies (or their affordances) with the research industries to which they applied (Fig. 3 ). There was occasionally a lack of detailed information about the capabilities of the e-learning systems applied (e.g., generic, or a web application, or an online system), which limited the insights. Figure 3 provides a useful mapping of the confluence of e-learning technologies and their application in the various industries.
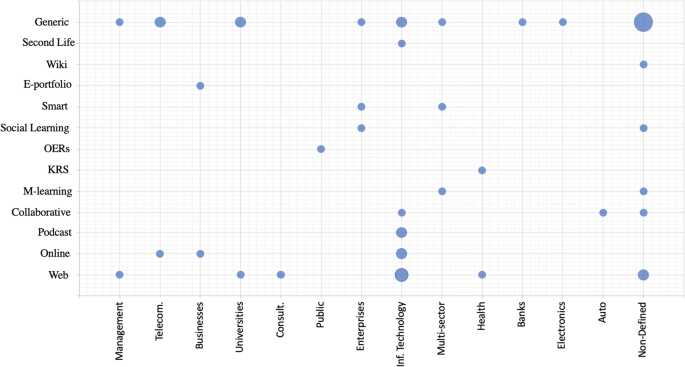
Association of the different e-learning technologies with the industries to which they are applied in the various studies. Note: The size of the circles depicts the frequency of studies, with the smallest circle representing one study and the largest representing six studies. The mapping is extracted from the data in the Appendix , which outlines the papers that belong in each of the circles
To investigate the connection between the various technologies used to enhance organizational learning and their intended objectives, we utilized the coding of the articles (see Appendix ) and mapped the various e-learning technologies (or their affordances) with the intended objectives, as reported in the various studies (Fig. 4 ). The results in Fig. 4 show the objectives that are central in eOL research (e.g., performance, fostering learning, adoption, and usage) as well as those objectives on which few studies have focused (e.g., alignment, feasibility, behavioral change). In addition, the results also indicate the limited utilization of the various e-learning capabilities (e.g., social, collaborative, smart) to achieve objectives connected with those capabilities (e.g., social learning and behavioral change, collaborative learning, and barriers).
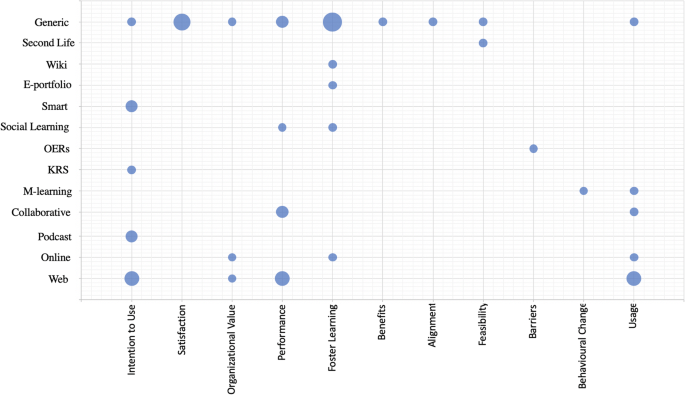
Association of the different e-learning technologies with the objectives investigated in the various studies. Note: The size of the circles depicts the frequency of studies, with the smallest circle representing one study and the largest representing five studies. The mapping is extracted from the data in the Appendix , which outlines the papers that belong in each of the circles
5 5. Discussion
After reviewing the 47 identified articles in the area of eOL, we can observe that all the works acknowledge the importance of the affordances offered by different e-learning technologies (e.g., remote collaboration, anytime anywhere), the importance of the relationship between eOL and employees’ satisfaction and performance, and the benefits associated with organizational value and outcome. Most of the studies agree that eOL provides employees, managers, and even clients with opportunities to learn in a more differentiated manner, compared to formal and face-to-face learning. However, how the organization adopts and puts into practice these capabilities to leverage them and achieve its goals are complex and challenging procedures that seem to be underexplored.
Several studies (Lee et al. 2015a ; Muller Queiroz et al. 2018 ; Tsai et al. 2010 ) focused on the positive effect of perceived managerial support, perceived usefulness, perceived ease of use, and other technology acceptance model (TAM) constructs of the e-learning system in supporting all three levels of learning (i.e., individual, collaborative, and organizational). Another interesting dimension highlighted by many studies (Choi and Ko 2012 ; Khalili et al. 2012 ; Yanson and Johnson 2016 ) is the role of socialization in the adoption and usage of the e-learning systems that offer these capabilities. Building connections and creating a shared learning space in the e-learning system is challenging but also critical for the learners (Yanson and Johnson 2016 ). This is consistent with the expectancy-theoretical explanation of how social context impacts on employees’ motivation to participate in learning (Lee et al. 2015a ; Muller Queiroz et al. 2018 ).
The organizational learning literature suggests that e-learning may be more appropriate for the acquisition of certain types of knowledge than others (e.g., procedural vs. declarative, or hard-skills vs. soft-skills); however, there is no empirical evidence for this (Yanson and Johnson 2016 ). To advance eOL research, there is a need for a significant move to address complex, strategic skills by including learning and development professionals (Garavan et al. 2019 ) and by developing strategic relationships. Another important element is to utilize e-learning technology that addresses and integrates organizational, individual, and social perspectives in eOL (Wang 2011 ). This is also identified in our literature review since we found only limited specialized e-learning systems in domain areas that have traditionally benefited from such technology. For instance, although there were studies that utilized VR environments (Costello and McNaughton 2018 ; Muller Queiroz et al. 2018 ) and video-based learning systems (Wei et al. 2013 ; Wei and Ram 2016 ), there was limited focus in contemporary eOL research on how specific affordances of the various environments that are used in organizations (e.g., Carnetsoft, Outotec HSC, and Simscale for simulations of working environments; or Raptivity, YouTube, and FStoppers to gain specific skills and how-to knowledge) can benefit the intended goals or be integrated with the unique qualities of the organization (e.g., IT, healthcare).
For the design and the development of the eOL approach, the organization needs to consider the alignment of individual learning needs, organizational objectives, and the necessary resources (Wang 2011 ). To achieve this, it is advisable for organizations to define the expected objectives, catalogue the individual needs, and select technologies that have the capacity to support and enrich learners with self-directed and socially constructed learning practices in the organization (Wang 2011 ). This needs to be done by taking into consideration that on-demand eOL is gradually replacing the classic static eOL curricula and processes (Dignen and Burmeister 2020 ).
Another important dimension of eOL research is the lenses used to approach effectiveness. The selected papers approached effectiveness with various objectives, such as fostering learning, usage of the e-learning system, employees’ performance, and the added organizational value (see Appendix ). To measure these indices, various metrics (quantitative, qualitative, and mixed) have been applied. The qualitative dimensions emphasize employees’ satisfaction and system usage (e.g., Menolli et al. 2020 ; Turi et al. 2019 ), as well as managers’ perceived gained value and benefits (e.g., Lee et al. 2015b ; Xiang et al. 2020 ) and firms’ perceived effective utilization of eOL resources (López-Nicolás and Meroño-Cerdán 2011 ). The quantitative dimensions focus on usage, feasibility, and experience at different levels within an organization, based on interviews, focus groups, and observations (Costello and McNaughton 2018 ; Michalski 2014 ; Stoffregen et al. 2016 ). However, it is not always clear the how eOL effectiveness has been measured, nor the extent to which eOL is well aligned with and is strategically impactful on delivering the strategic agenda of the organization (Garavan et al. 2019 ).
Research on digital technologies is developing rapidly, and big data and business analytics have the potential to pave the way for organizations’ digital transformation and sustainable development (Mikalef et al. 2018 ; Pappas et al. 2018 ); however, our review finds surprisingly limited use of big data and analytics in eOL. Despite contemporary e-learning systems adopting data-driven mechanisms, as well as advances in learning analytics (Siemens and Long 2011 ), the results of our analysis indicate that learner-generated data in the context of eOL are used in only a few studies to extract very limited insights with respect to the effectiveness of eOL and the intended objectives of the respective study (Hung et al. 2015 ; Renner et al. 2020 ; Rober and Cooper 2011 ). Therefore, eOL research needs to focus on data-driven qualities that will allow future researchers to gain deeper insights into which capabilities need to be developed to monitor the effectiveness of the various practices and technologies, their alignment with other functions of the organization, and how eOL can be a strategic and impactful vehicle for materializing the strategic agenda of the organization.
5.1 Status of eOL Research
The current review suggests that, while the efficient implementation of eOL entails certain challenges, there is also a great potential for improving employees’ performance as well as overall organizational outcome and value. There are also opportunities for improving organizations’ learning flow, which might not be feasible with formal learning and training. In order to construct the main research dimensions of eOL research and to look more deeply at the research objectives of the studies (the information we coded as objectives in the Appendix ), we performed a content analysis and grouped the research objectives. This enabled us to summarize the contemporary research on eOL according to five major categories, each of which is describes further below. As the research objectives of the published work shows, the research on eOL conducted during the last decade has particularly focused on the following five directions.
Investigating the capabilities of different technologies in different organizations.
Research has particularly focused on how easy the technology is to use, on how useful it is, or on how well aligned/integrated it is with other systems and processes within the organization. In addition, studies have used different learning technologies (e.g., smart, social, personalized) to enhance organizational learning in different contexts and according to different needs. However, most works have focused on affordances such as remote training and the development of static courses or modules to share information with learners. Although a few studies have utilized contemporary e-learning systems (see Appendix ), even in these studies there is a lack of alignment between the capabilities of those systems (e.g., open online course, adaptive support, social and collaborative learning) and the objectives and strategy of the organization (e.g., organizational value, fostering learning).
Enriching the learning flow and learning potential in different levels within an organization.
The reviewed work has emphasized how different factors contribute to different levels of organizational learning, and it has focused on practices that address individual, collaborative, and organizational learning within the structure of the organization. In particular, most of the reviewed studies recognize that organizational learning occurs at multiple levels: individual, team (or group), and organization. In other words, although each of the studies carried out an investigation within a given level (except for Garavan et al. 2019 ), there is a recognition and discussion of the different levels. Therefore, the results align with the 4I framework of organizational learning that recognizes how learning across the different levels is linked by social and psychological processes: intuiting, interpreting, integrating, and institutionalizing (the 4Is) (Crossan et al. 1999 ). However, most of the studies focused on the institutionalizing-intuiting link (i.e., top-down feedback); moreover, no studies focused on contemporary learning technologies and processes that strengthen the learning flow (e.g., self-regulated learning).
Identifying critical aspects for effective eOL.
There is a considerable amount of predominantly qualitative studies that focus on potential barriers to eOL implementation as well as on the risks and requirements associated with the feasibility and successful implementation of eOL. In the same vein, research has emphasized the importance of alignment of eOL (both in processes and in technologies) within the organization. These critical aspects for effective eOL are sometimes the main objectives of the studies (see Appendix ). However, most of the elements relating to the effectiveness of eOL were measured with questionnaires and interviews with employees and managers, and very little work was conducted on how to leverage the digital technologies employed in eOL, big data, and analytics in order to monitor the effectiveness of eOL.
Implementing employee-centric eOL.
In most of the studies, the main objective was to increase employees’ adoption, satisfaction, and usage of the e-learning system. In addition, several studies focused on the e-learning system’s ability to improve employees’ performance, increase the knowledge flow in the organization, and foster learning. Most of the approaches were employee-centric, with a small amount of studies focusing on managers and the firm in general. However, employees were seen as static entities within the organization, with limited work investigating how eOL-based training exposes employees to new knowledge, broadens their skills repertoire, and has tremendous potential for fostering innovation (Lin and Sanders 2017 ).
Achieving goals associated with the value creation of the organization.
A considerable number of studies utilized the firm (rather than the individual employee) as the unit of analysis. Such studies focused on how the implementation of eOL can increase employee performance, organizational value, and customer value. Although this is extremely helpful in furthering knowledge about eOL technologies and practices, a more granular investigation of the different e-learning systems and processes to address the various goals and strategies of the organization would enable researchers to extract practical insights on the design and implementation of eOL.
5.2 Research Agenda
By conducting an SLR and documenting the eOL research of the last decade, we have identified promising themes of research that have the potential to further eOL research and practice. To do so, we define a research agenda consisting of five thematic areas of research, as depicted in the research framework in Fig. 5 , and we provide some suggestions on how researchers could approach these challenges. In this visualization of the framework, on the left side we present the organizations as they were identified from our review (i.e., area/topic category in the Appendix ) and the multiple levels where organizational learning occurs (Costello and McNaughton 2018 ). On the right side, we summarize the objectives as they were identified from our review (i.e., the objectives category in the Appendix ). In the middle, we depict the orchestration that was conducted and how potential future research on eOL can improve the orchestration of the various elements and accelerate the achievement of the intended objectives. In particular, our proposed research agenda includes five research themes discussed in the following subsections.
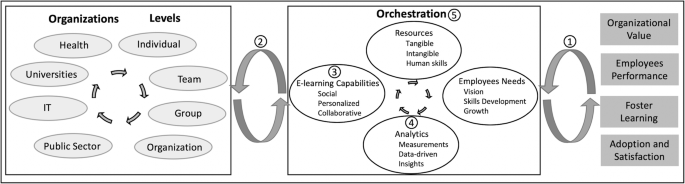
E-learning capabilities to enhance organizational research agenda
5.2.1 Theme 1: Couple E-learning Capabilities With the Intended Goals
The majority of the eOL studies either investigated a generic e-learning system using the umbrella term “e-learning” or did not provide enough details about the functionalities of the system (in most cases, it was simply defined as an online or web system). This indicates the very limited focus of the eOL research on the various capabilities of e-learning systems. In other words, the literature has been very detailed on the organizational value and employees’ acceptance of the technology, but less detailed on the capabilities of this technology that needs to be put into place to achieve the intended goals and strategic agenda. However, the capabilities of the e-learning systems and their use are not one-size-fits-all, and the intended goals (to obtain certain skills and competences) and employees’ needs and backgrounds play a determining role in the selection of the e-learning system (Al-Fraihat et al. 2020 ).
Only in a very few studies (Mueller et al. 2011 ; Renner et al. 2020 ) were the capabilities of the e-learning solutions (e.g., mobile learning, VR) utilized, and the results were found to significantly contribute to the intended goals. The intended knowledge can be procedural, declarative, general competence (e.g., presentation, communication, or leadership skills) or else, and its particularities and the pedagogical needs of the intended knowledge (e.g., a need for summative/formative feedback or for social learning support) should guide the selection of the e-learning system and the respective capabilities. Therefore, future research needs to investigate how the various capabilities offered by contemporary learning systems (e.g., assessment mechanisms, social learning, collaborative learning, personalized learning) can be utilized to adequately reinforce the intended goals (e.g., to train personnel to use a new tool, to improve presentation skills).
5.2.2 Theme 2: Embrace the Particularities of the Various Industries
Organizational learning entails sharing knowledge and enabling opportunities for growth at the individual, group, team, and organizational levels. Contemporary e-learning systems provide the medium to substantiate the necessary knowledge flow within organizations and to support employees’ overall learning. From the selected studies, we can infer that eOL research is either conducted in an industry-agnostic context (either generic or it was not properly reported) or there is a focus on the IT industry (see Appendix ). However, when looking at the few studies that provide results from different industries (Garavan et al. 2019 ; Lee et al. 2014 ), companies indicate that there are different practices, processes, and expectations, and that employees have different needs and perceptions with regards to e-learning systems and eOL in general. Such particularities influence the perceived dimensions of a learning organization. Some industries noted that eOL promoted the development of their learning organizations, whereas others reported that eOL did not seem to contribute to their development as a learning organization (Yoo and Huang 2016 ). Therefore, it is important that the implementation of organizational learning embraces the particularities of the various industries and future research needs to identify how the industry-specific characteristics can inform the design and development of organizational learning in promoting an organization’s goals and agenda.
5.2.3 Theme 3: Utilize E-learning Capabilities to Implement Employee-centric Approaches
For efficient organizational learning to be implemented, the processes and technologies need to recognize that learning is linked by social and psychological processes (Crossan et al. 1999 ). This allows employees to develop learning in various forms (e.g., social, emotional, personalized) and to develop elements such as self-awareness, self-control, and interpersonal skills that are vital for the organization. Looking at the contemporary eOL research, we notice that the exploration of e-learning capabilities to nurture the aforementioned elements and support employee-centric approaches is very limited (e.g., personalized technologies, adaptive assessment). Therefore, future research needs to collect data to understand how e-learning capabilities can be utilized in relation to employees’ needs and perceptions in order to provide solutions (e.g., collaborative, social, adaptive) that are employee-centric and focused on development, and that have the potential to move away from standard one-size-fits-all e-learning solutions to personalized and customized systems and processes.
5.2.4 Theme 4: Employ Analytics-enabled eOL
There is a lot of emphasis on measuring, via various qualitative and quantitative metrics, the effectiveness of eOL implemented at different levels in organizations. However, most of these metrics come from surveys and interviews that capture employees’ and managers’ perceptions of various aspects of eOL (e.g., fostering of learning, organizational value, employees’ performance), and very few studies utilize analytics (Hung et al. 2015 ; Renner et al. 2020 ; Rober and Cooper 2011 ). Given how digital technologies, big data, and business analytics pave the way towards organizations’ digital transformation and sustainable development (Mikalef et al. 2018 ; Pappas et al. 2018 ), and considering the learning analytics affordances of contemporary e-learning systems (Siemens and Long 2011 ), future work needs to investigate how learner/employee-generated data can be employed to inform practice and devise more accurate and temporal effectiveness metrics when measuring the importance and impact of eOL.
5.2.5 Theme 5: Orchestrate the Employees’ Needs, Resources, and Objectives in eOL Implementation
While considerable effort has been directed towards the various building blocks of eOL implementation, such as resources (intangible, tangible, and human skills) and employees’ needs (e.g., vision, growth, skills development), little is known so far about the processes and structures necessary for orchestrating those elements in order to achieve an organization’s intended goals and to materialize its overall agenda. In other words, eOL research has been very detailed on some of the elements that constitute efficient eOL, but less so on the interplay of those elements and how they need to be put into place. Prior literature on strategic resource planning has shown that competence in orchestrating such elements is a prerequisite to successfully increasing business value (Wang et al. 2012 ). Therefore, future research should not only investigate each of these elements in silos, but also consider their interplay, since it is likely that organizations with similar resources will exert highly varied levels in each of these elements (e.g., analytics-enabled, e-learning capabilities) to successfully materialize their goals (e.g., increase value, improve the competence base of their employees, modernize their organization).
5.3 Implications
Several implications for eOL have been revealed in this literature review. First, most studies agree that employees’ or trainees’ experience is extremely important for the successful implementation of eOL. Thus, keeping them in the design and implementation cycle of eOL will increase eOL adoption and satisfaction as well as reduce the risks and barriers. Another important implication addressed by some studies relates to the capabilities of the e-learning technologies, with easy-to-use, useful, and social technologies resulting in more efficient eOL (e.g., higher adoption and performance). Thus, it is important for organizations to incorporate these functionalities in the platform and reinforce them with appropriate content and support. This should not only benefit learning outcomes, but also provide the networking opportunities for employees to broaden their personal networks, which are often lost when companies move from face-to-face formal training to e-learning-enabled organizational learning.
5.4 Limitations
This review has some limitations. First, we had to make some methodological decisions (e.g., selection of databases, the search query) that might lead to certain biases in the results. However, tried to avoid such biases by considering all the major databases and following the steps indicated by Kitchenham and Charters ( 2007 ). Second, the selection of empirical studies and coding of the papers might pose another possible bias. However, the focus was clearly on the empirical evidence, the terminology employed (“e-learning”) is an umbrella term that covers the majority of the work in the area, and the coding of papers was checked by two researchers. Third, some elements of the papers were not described accurately, leading to some missing information in the coding of the papers. However, the amount of missing information was very small and could not affect the results significantly. Finally, we acknowledge that the selected methodology (Kitchenham and Charters 2007 ) includes potential biases (e.g., false negatives and false positives), and that different, equally valid methods (e.g., Okoli and Schabram 2010 ) might have been used and have resulted in slightly different outcomes. Nevertheless, despite the limitations of the selected methodology, it is a well-accepted and widely used literature review method in both software engineering and information systems (Boell and Cecez-Kecmanovic 2014 ), providing certain assurance of the results.
6 Conclusions and Future Work
We have presented an SLR of 47 contributions in the field of eOL over the last decade. With respect to RQ1, we analyzed the papers from different perspectives, such as research methodology, technology, industries, employees, and intended outcomes in terms of organizational value, employees’ performance, usage, and behavioral change. The detailed landscape is depicted in the Appendix and Figs. 3 and 4 ; with the results indicating the limited utilization of the various e-learning capabilities (e.g., social, collaborative) to achieve objectives connected with those capabilities (e.g., social learning and behavioral change, collaborative learning and overcoming barriers).
With respect to RQ2, we categorized the main findings of the selected papers into five areas that reflect the status of eOL research, and we have discussed the challenges and opportunities emerging from the current review. In addition, we have synthesized the extracted challenges and opportunities and proposed a research agenda consisting of five elements that provide suggestions on how researchers could approach these challenges and exploit the opportunities. Such an agenda will strengthen how e-learning can be leveraged to enhance the process of improving actions through better knowledge and understanding in an organization.
A number of suggestions for further research have emerged from reviewing prior and ongoing work on eOL. One recommendation for future researchers is to clearly describe the eOL approach by providing detailed information about the technologies and materials used, as well as the organizations. This will allow meta-analyses to be conducted and it will also identify the potential effects of a firm’s size or area on the performance and other aspects relating to organizational value. Future work should also focus on collecting and triangulating different types of data from different sources (e.g., systems’ logs). The reviewed studies were conducted mainly by using survey data, and they made limited use of data coming from the platforms; thus, the interpretations and triangulation between the different types of collected data were limited.
Al-Fraihat, D., Joy, M., & Sinclair, J. (2020). Evaluating E-learning systems success: An empirical study. Computers in Human Behavior, 102 , 67–86.
Article Google Scholar
Alharthi, A. D., Spichkova, M., & Hamilton, M. (2019). Sustainability requirements for eLearning systems: A systematic literature review and analysis. Requirements Engineering, 24 (4), 523–543.
Alsabawy, A. Y., Cater-Steel, A., & Soar, J. (2013). IT infrastructure services as a requirement for e-learning system success. Computers & Education, 69 , 431–451.
Antonacopoulou, E., & Chiva, R. (2007). The social complexity of organizational learning: The dynamics of learning and organizing. Management Learning, 38 , 277–295.
Berends, H., & Lammers, I. (2010). Explaining discontinuity in organizational learning: A process analysis. Organization Studies, 31 (8), 1045–1068.
Boell, S. K., & Cecez-Kecmanovic, D. (2014). A hermeneutic approach for conducting literature reviews and literature searches. Communications of the Association for Information Systems, 34 (1), 12.
Google Scholar
Bologa, R., & Lupu, A. R. (2014). Organizational learning networks that can increase the productivity of IT consulting companies. A case study for ERP consultants. Expert Systems with Applications, 41 (1), 126–136.
Broadbent, J. (2017). Comparing online and blended learner’s self-regulated learning strategies and academic performance. The Internet and Higher Education, 33 , 24–32.
Cheng, B., Wang, M., Moormann, J., Olaniran, B. A., & Chen, N. S. (2012). The effects of organizational learning environment factors on e-learning acceptance. Computers & Education, 58 (3), 885–899.
Cheng, B., Wang, M., Yang, S. J., & Peng, J. (2011). Acceptance of competency-based workplace e-learning systems: Effects of individual and peer learning support. Computers & Education, 57 (1), 1317–1333.
Choi, S., & Ko, I. (2012). Leveraging electronic collaboration to promote interorganizational learning. International Journal of Information Management, 32 (6), 550–559.
Costello, J. T., & McNaughton, R. B. (2018). Integrating a dynamic capabilities framework into workplace e-learning process evaluations. Knowledge and Process Management, 25 (2), 108–125.
Crossan, M. M., Lane, H. W., & White, R. E. (1999). An organizational learning framework: From intuition to institution. Academy of Management Review, 24 , 522–537.
Crossan, M. M., Maurer, C. C., & White, R. E. (2011). Reflections on the 2009 AMR decade award: Do we have a theory of organizational learning? Academy of Management Review, 36 (3), 446–460.
Dee, J., & Leisyte, L. (2017). Knowledge sharing and organizational change in higher education. The Learning Organization, 24 (5), 355–365. https://doi.org/10.1108/TLO-04-2017-0034
Dignen, B., & Burmeister, T. (2020). Learning and development in the organizations of the future. Three pillars of organization and leadership in disruptive times (pp. 207–232). Cham: Springer.
Chapter Google Scholar
Dybå, T., & Dingsøyr, T. (2008). Empirical studies of agile software development: A systematic review. Information and Software Technology, 50 (9–10), 833–859.
El Kadiri, S., Grabot, B., Thoben, K. D., Hribernik, K., Emmanouilidis, C., Von Cieminski, G., & Kiritsis, D. (2016). Current trends on ICT technologies for enterprise information systems. Computers in Industry, 79 , 14–33.
Engeström, Y., Kerosuo, H., & Kajamaa, A. (2007). Beyond discontinuity: Expansive organizational learning remembered. Management Learning, 38 (3), 319–336.
Gal, E., & Nachmias, R. (2011). Online learning and performance support in organizational environments using performance support platforms. Performance Improvement, 50 (8), 25–32.
Garavan, T. N., Heneghan, S., O’Brien, F., Gubbins, C., Lai, Y., Carbery, R., & Grant, K. (2019). L&D professionals in organisations: much ambition, unfilled promise. European Journal of Training and Development, 44 (1), 1–86.
Goggins, S. P., Jahnke, I., & Wulf, V. (2013). Computer-supported collaborative learning at the workplace . New York: Springer.
Book Google Scholar
Hammad, R., Odeh, M., & Khan, Z. (2017). ELCMM: An e-learning capability maturity model. In Proceedings of the 15th International Conference (e-Society 2017) (pp. 169–178).
Hester, A. J., Hutchins, H. M., & Burke-Smalley, L. A. (2016). Web 2.0 and transfer: Trainers’ use of technology to support employees’ learning transfer on the job. Performance Improvement Quarterly, 29 (3), 231–255.
Hung, Y. H., Lin, C. F., & Chang, R. I. (2015). Developing a dynamic inference expert system to support individual learning at work. British Journal of Educational Technology, 46 (6), 1378–1391.
Iris, R., & Vikas, A. (2011). E-Learning technologies: A key to dynamic capabilities. Computers in Human Behavior, 27 (5), 1868–1874.
Jia, H., Wang, M., Ran, W., Yang, S. J., Liao, J., & Chiu, D. K. (2011). Design of a performance-oriented workplace e-learning system using ontology. Expert Systems with Applications, 38 (4), 3372–3382.
Joo, Y. J., Lim, K. Y., & Park, S. Y. (2011). Investigating the structural relationships among organisational support, learning flow, learners’ satisfaction and learning transfer in corporate e-learning. British Journal of Educational Technology, 42 (6), 973–984.
Kaschig, A., Maier, R., Sandow, A., Lazoi, M., Barnes, S. A., Bimrose, J., … Schmidt, A. (2010). Knowledge maturing activities and practices fostering organisational learning: results of an empirical study. In European Conference on Technology Enhanced Learning (pp. 151–166). Berlin: Springer.
Khalili, A., Auer, S., Tarasowa, D., & Ermilov, I. (2012). SlideWiki: Elicitation and sharing of corporate knowledge using presentations. International Conference on Knowledge Engineering and Knowledge Management (pp. 302–316). Berlin: Springer.
Khandakar, M. S. A., & Pangil, F. (2019). Relationship between human resource management practices and informal workplace learning. Journal of Workplace Learning, 31 (8), 551–576.
Kim, M. K., Kim, S. M., & Bilir, M. K. (2014). Investigation of the dimensions of workplace learning environments (WLEs): Development of the WLE measure. Performance Improvement Quarterly, 27 (2), 35–57.
Kitchenham, B., & Charters, S. (2007). Guidelines for performing systematic literature reviews in software engineering. Technical Report EBSE-2007-01, 2007 . https://citeseerx.ist.psu.edu/viewdoc/download;jsessionid=35909B1B280E2032BF116BDC9DCB71EA? .
Krippendorff, K. (2018). Content analysis: an introduction to its methodology. Thousand Oaks: Sage Publications.
Lai, H. J. (2017). Examining civil servants’ decisions to use Web 2.0 tools for learning, based on the decomposed theory of planned behavior. Interactive Learning Environments, 25 (3), 295–305.
Lau, K. (2015). Organizational learning goes virtual? A study of employees’ learning achievement in stereoscopic 3D virtual reality. The Learning Organization, 22 (5), 289–303.
Lee, J., Choi, M., & Lee, H. (2015a). Factors affecting smart learning adoption in workplaces: Comparing large enterprises and SMEs. Information Technology and Management, 16 (4), 291–302.
Lee, J., Kim, D. W., & Zo, H. (2015b). Conjoint analysis on preferences of HRD managers and employees for effective implementation of m-learning: The case of South Korea. Telematics and Informatics, 32 (4), 940–948.
Lee, J., Zo, H., & Lee, H. (2014). Smart learning adoption in employees and HRD managers. British Journal of Educational Technology, 45 (6), 1082–1096.
Lin, C. H., & Sanders, K. (2017). HRM and innovation: A multi-level organizational learning perspective. Human Resource Management Journal, 27 (2), 300–317.
Lin, C. Y., Huang, C. K., & Zhang, H. (2019). Enhancing employee job satisfaction via e-learning: The mediating role of an organizational learning culture. International Journal of Human–Computer Interaction, 35 (7), 584–595.
Liu, Y. C., Huang, Y. A., & Lin, C. (2012). Organizational factors’ effects on the success of e-learning systems and organizational benefits: An empirical study in Taiwan. The International Review of Research in Open and Distributed Learning, 13 (4), 130–151.
López-Nicolás, C., & Meroño-Cerdán, ÁL. (2011). Strategic knowledge management, innovation and performance. International Journal of Information Management, 31 (6), 502–509.
Manuti, A., Pastore, S., Scardigno, A. F., Giancaspro, M. L., & Morciano, D. (2015). Formal and informal learning in the workplace: A research review. International Journal of Training and Development, 19 (1), 1–17.
March, J. G. (1991). Exploration and exploitation in organizational learning. Organization Science, 2 (1), 71–87.
Marshall, S. (2006). New Zealand Tertiary Institution E-learning Capability: Informing and Guiding eLearning Architectural Change and Development. Report to the ministry of education . NZ: Victoria University of Wellington.
McDonald, N., Schoenebeck, S., & Forte, A. (2019). Reliability and inter-rater reliability in qualitative research: Norms and guidelines for CSCW and HCI practice. In Proceedings of the ACM on Human–Computer Interaction, 3(CSCW) (pp. 1–23).
Menolli, A., Tirone, H., Reinehr, S., & Malucelli, A. (2020). Identifying organisational learning needs: An approach to the semi-automatic creation of course structures for software companies. Behaviour & Information Technology, 39 (11), 1140–1155.
Michalski, M. P. (2014). Symbolic meanings and e-learning in the workplace: The case of an intranet-based training tool. Management Learning, 45 (2), 145–166.
Mikalef, P., Pappas, I. O., Krogstie, J., & Giannakos, M. (2018). Big data analytics capabilities: A systematic literature review and research agenda. Information Systems and e-Business Management, 16 (3), 547–578.
Mitić, S., Nikolić, M., Jankov, J., Vukonjanski, J., & Terek, E. (2017). The impact of information technologies on communication satisfaction and organizational learning in companies in Serbia. Computers in Human Behavior, 76 , 87–101.
Mueller, J., Hutter, K., Fueller, J., & Matzler, K. (2011). Virtual worlds as knowledge management platform—A practice-perspective. Information Systems Journal, 21 (6), 479–501.
Muller Queiroz, A. C., Nascimento, M., Tori, A., Alejandro, R. Brashear, Veloso, T., de Melo, V., de Souza Meirelles, F., & da Silva Leme, M. I. (2018). Immersive virtual environments in corporate education and training. In AMCIS. https://aisel.aisnet.org/amcis2018/Education/Presentations/12/ .
Navimipour, N. J., & Zareie, B. (2015). A model for assessing the impact of e-learning systems on employees’ satisfaction. Computers in Human Behavior, 53 , 475–485.
Oh, S. Y. (2019). Effects of organizational learning on performance: The moderating roles of trust in leaders and organizational justice. Journal of Knowledge Management, 23, 313–331.
Okoli, C., & Schabram, K. (2010). A guide to conducting a systematic literature review of information systems research. Sprouts: Working Papers on Information Systems, 10 (26), 1–46.
Pappas, I. O., Mikalef, P., Giannakos, M. N., Krogstie, J., & Lekakos, G. (2018). Big data and business analytics ecosystems: paving the way towards digital transformation and sustainable societies. Information Systems and e-Business Management, 16, 479–491.
Popova-Nowak, I. V., & Cseh, M. (2015). The meaning of organizational learning: A meta-paradigm perspective. Human Resource Development Review, 14 (3), 299–331.
Qi, C., & Chau, P. Y. (2016). An empirical study of the effect of enterprise social media usage on organizational learning. In Pacific Asia Conference on Information Systems (PACIS'16). Proceedings , Paper 330. http://aisel.aisnet.org/pacis2016/330 .
Renner, B., Wesiak, G., Pammer-Schindler, V., Prilla, M., Müller, L., Morosini, D., … Cress, U. (2020). Computer-supported reflective learning: How apps can foster reflection at work. Behaviour & Information Technology, 39 (2), 167–187.
Rober, M. B., & Cooper, L. P. (2011, January). Capturing knowledge via an” Intrapedia”: A case study. In 2011 44th Hawaii International Conference on System Sciences (pp. 1–10). New York: IEEE.
Rosenberg, M. J., & Foshay, R. (2002). E-learning: Strategies for delivering knowledge in the digital age. Performance Improvement, 41 (5), 50–51.
Serrano, Á., Marchiori, E. J., del Blanco, Á., Torrente, J., & Fernández-Manjón, B. (2012). A framework to improve evaluation in educational games. The IEEE Global Engineering Education Conference (pp. 1–8). Marrakesh, Morocco.
Siadaty, M., Jovanović, J., Gašević, D., Jeremić, Z., & Holocher-Ertl, T. (2010). Leveraging semantic technologies for harmonization of individual and organizational learning. In European Conference on Technology Enhanced Learning (pp. 340–356). Berlin: Springer.
Siemens, G., & Long, P. (2011). Penetrating the fog: Analytics in learning and education. EDUCAUSE Review, 46 (5), 30.
Škerlavaj, M., Dimovski, V., Mrvar, A., & Pahor, M. (2010). Intra-organizational learning networks within knowledge-intensive learning environments. Interactive Learning Environments, 18 (1), 39–63.
Smith, P. J., & Sadler-Smith, E. (2006). Learning in organizations: Complexities and diversities . London: Routledge.
Stoffregen, J. D., Pawlowski, J. M., Ras, E., Tobias, E., Šćepanović, S., Fitzpatrick, D., … Friedrich, H. (2016). Barriers to open e-learning in public administrations: A comparative case study of the European countries Luxembourg, Germany, Montenegro and Ireland. Technological Forecasting and Social Change, 111 , 198–208.
Subramaniam, R., & Nakkeeran, S. (2019). Impact of corporate e-learning systems in enhancing the team performance in virtual software teams. In Smart Technologies and Innovation for a Sustainable Future (pp. 195–204). Berlin: Springer.
Tsai, C. H., Zhu, D. S., Ho, B. C. T., & Wu, D. D. (2010). The effect of reducing risk and improving personal motivation on the adoption of knowledge repository system. Technological Forecasting and Social Change, 77 (6), 840–856.
Turi, J. A., Sorooshian, S., & Javed, Y. (2019). Impact of the cognitive learning factors on sustainable organizational development. Heliyon, 5 (9), e02398.
Wang, M. (2011). Integrating organizational, social, and individual perspectives in Web 2.0-based workplace e-learning. Information Systems Frontiers, 13 (2), 191–205.
Wang, M. (2018). Effects of individual and social learning support on employees’ acceptance of performance-oriented e-learning. In E-Learning in the Workplace (pp. 141–159). Springer. https://doi.org/10.1007/978-3-319-64532-2_13 .
Wang, M., Ran, W., Liao, J., & Yang, S. J. (2010). A performance-oriented approach to e-learning in the workplace. Journal of Educational Technology & Society, 13 (4), 167–179.
Wang, M., Vogel, D., & Ran, W. (2011). Creating a performance-oriented e-learning environment: A design science approach. Information & Management, 48 (7), 260–269.
Wang, N., Liang, H., Zhong, W., Xue, Y., & Xiao, J. (2012). Resource structuring or capability building? An empirical study of the business value of information technology. Journal of Management Information Systems, 29 (2), 325–367.
Wang, S., & Wang, H. (2012). Organizational schemata of e-portfolios for fostering higher-order thinking. Information Systems Frontiers, 14 (2), 395–407.
Wei, K., & Ram, J. (2016). Perceived usefulness of podcasting in organizational learning: The role of information characteristics. Computers in Human Behavior, 64 , 859–870.
Wei, K., Sun, H., & Li, H. (2013). On the driving forces of diffusion of podcasting in organizational settings: A case study and propositions. In PACIS 2013. Proceedings , 217. http://aisel.aisnet.org/pacis2013/217 .
Weinhardt, J. M., & Sitzmann, T. (2018). Revolutionizing training and education? Three questions regarding massive open online courses (MOOCs). Human Resource Management Review, 29 (2), 218–225.
Xiang, Q., Zhang, J., & Liu, H. (2020). Organisational improvisation as a path to new opportunity identification for incumbent firms: An organisational learning view. Innovation, 22 (4), 422–446. https://doi.org/10.1080/14479338.2020.1713001 .
Yanson, R., & Johnson, R. D. (2016). An empirical examination of e-learning design: The role of trainee socialization and complexity in short term training. Computers & Education, 101 , 43–54.
Yoo, S. J., & Huang, W. D. (2016). Can e-learning system enhance learning culture in the workplace? A comparison among companies in South Korea. British Journal of Educational Technology, 47 (4), 575–591.
Zhang, X., Jiang, S., Ordóñez de Pablos, P., Lytras, M. D., & Sun, Y. (2017). How virtual reality affects perceived learning effectiveness: A task–technology fit perspective. Behaviour & Information Technology, 36 (5), 548–556.
Zhang, X., Meng, Y., de Pablos, P. O., & Sun, Y. (2019). Learning analytics in collaborative learning supported by Slack: From the perspective of engagement. Computers in Human Behavior, 92 , 625–633.
Download references
Open Access funding provided by NTNU Norwegian University of Science and Technology (incl St. Olavs Hospital - Trondheim University Hospital).
Author information
Authors and affiliations.
Norwegian University of Science and Technology, Trondheim, Norway
Michail N. Giannakos, Patrick Mikalef & Ilias O. Pappas
University of Agder, Kristiansand, Norway
Ilias O. Pappas
You can also search for this author in PubMed Google Scholar
Corresponding author
Correspondence to Ilias O. Pappas .
Additional information
Publisher’s note.
Springer Nature remains neutral with regard to jurisdictional claims in published maps and institutional affiliations.
Rights and permissions
Open Access This article is licensed under a Creative Commons Attribution 4.0 International License, which permits use, sharing, adaptation, distribution and reproduction in any medium or format, as long as you give appropriate credit to the original author(s) and the source, provide a link to the Creative Commons licence, and indicate if changes were made. The images or other third party material in this article are included in the article's Creative Commons licence, unless indicated otherwise in a credit line to the material. If material is not included in the article's Creative Commons licence and your intended use is not permitted by statutory regulation or exceeds the permitted use, you will need to obtain permission directly from the copyright holder. To view a copy of this licence, visit http://creativecommons.org/licenses/by/4.0/ .
Reprints and permissions
About this article
Giannakos, M.N., Mikalef, P. & Pappas, I.O. Systematic Literature Review of E-Learning Capabilities to Enhance Organizational Learning. Inf Syst Front 24 , 619–635 (2022). https://doi.org/10.1007/s10796-020-10097-2
Download citation
Accepted : 09 December 2020
Published : 01 February 2021
Issue Date : April 2022
DOI : https://doi.org/10.1007/s10796-020-10097-2
Share this article
Anyone you share the following link with will be able to read this content:
Sorry, a shareable link is not currently available for this article.
Provided by the Springer Nature SharedIt content-sharing initiative
- Organizational learning
- Literature review
- Learning environments
- Find a journal
- Publish with us
- Track your research
- Research article
- Open access
- Published: 02 December 2020
Integrating students’ perspectives about online learning: a hierarchy of factors
- Montgomery Van Wart 1 ,
- Anna Ni 1 ,
- Pamela Medina 1 ,
- Jesus Canelon 1 ,
- Melika Kordrostami 1 ,
- Jing Zhang 1 &
International Journal of Educational Technology in Higher Education volume 17 , Article number: 53 ( 2020 ) Cite this article
150k Accesses
54 Citations
24 Altmetric
Metrics details
This article reports on a large-scale ( n = 987), exploratory factor analysis study incorporating various concepts identified in the literature as critical success factors for online learning from the students’ perspective, and then determines their hierarchical significance. Seven factors--Basic Online Modality, Instructional Support, Teaching Presence, Cognitive Presence, Online Social Comfort, Online Interactive Modality, and Social Presence--were identified as significant and reliable. Regression analysis indicates the minimal factors for enrollment in future classes—when students consider convenience and scheduling—were Basic Online Modality, Cognitive Presence, and Online Social Comfort. Students who accepted or embraced online courses on their own merits wanted a minimum of Basic Online Modality, Teaching Presence, Cognitive Presence, Online Social Comfort, and Social Presence. Students, who preferred face-to-face classes and demanded a comparable experience, valued Online Interactive Modality and Instructional Support more highly. Recommendations for online course design, policy, and future research are provided.
Introduction
While there are different perspectives of the learning process such as learning achievement and faculty perspectives, students’ perspectives are especially critical since they are ultimately the raison d’être of the educational endeavor (Chickering & Gamson, 1987 ). More pragmatically, students’ perspectives provide invaluable, first-hand insights into their experiences and expectations (Dawson et al., 2019 ). The student perspective is especially important when new teaching approaches are used and when new technologies are being introduced (Arthur, 2009 ; Crews & Butterfield, 2014 ; Van Wart, Ni, Ready, Shayo, & Court, 2020 ). With the renewed interest in “active” education in general (Arruabarrena, Sánchez, Blanco, et al., 2019 ; Kay, MacDonald, & DiGiuseppe, 2019 ; Nouri, 2016 ; Vlachopoulos & Makri, 2017 ) and the flipped classroom approach in particular (Flores, del-Arco, & Silva, 2016 ; Gong, Yang, & Cai, 2020 ; Lundin, et al., 2018 ; Maycock, 2019 ; McGivney-Burelle, 2013 ; O’Flaherty & Phillips, 2015 ; Tucker , 2012 ) along with extraordinary shifts in the technology, the student perspective on online education is profoundly important. What shapes students’ perceptions of quality integrate are their own sense of learning achievement, satisfaction with the support they receive, technical proficiency of the process, intellectual and emotional stimulation, comfort with the process, and sense of learning community. The factors that students perceive as quality online teaching, however, has not been as clear as it might be for at least two reasons.
First, it is important to note that the overall online learning experience for students is also composed of non-teaching factors which we briefly mention. Three such factors are (1) convenience, (2) learner characteristics and readiness, and (3) antecedent conditions that may foster teaching quality but are not directly responsible for it. (1) Convenience is an enormous non-quality factor for students (Artino, 2010 ) which has driven up online demand around the world (Fidalgo, Thormann, Kulyk, et al., 2020 ; Inside Higher Education and Gallup, 2019 ; Legon & Garrett, 2019 ; Ortagus, 2017 ). This is important since satisfaction with online classes is frequently somewhat lower than face-to-face classes (Macon, 2011 ). However, the literature generally supports the relative equivalence of face-to-face and online modes regarding learning achievement criteria (Bernard et al., 2004 ; Nguyen, 2015 ; Ni, 2013 ; Sitzmann, Kraiger, Stewart, & Wisher, 2006 ; see Xu & Jaggars, 2014 for an alternate perspective). These contrasts are exemplified in a recent study of business students, in which online students using a flipped classroom approach outperformed their face-to-face peers, but ironically rated instructor performance lower (Harjoto, 2017 ). (2) Learner characteristics also affect the experience related to self-regulation in an active learning model, comfort with technology, and age, among others,which affect both receptiveness and readiness of online instruction. (Alqurashi, 2016 ; Cohen & Baruth, 2017 ; Kintu, Zhu, & Kagambe, 2017 ; Kuo, Walker, Schroder, & Belland, 2013 ; Ventura & Moscoloni, 2015 ) (3) Finally, numerous antecedent factors may lead to improved instruction, but are not themselves directly perceived by students such as instructor training (Brinkley-Etzkorn, 2018 ), and the sources of faculty motivation (e.g., incentives, recognition, social influence, and voluntariness) (Wingo, Ivankova, & Moss, 2017 ). Important as these factors are, mixing them with the perceptions of quality tends to obfuscate the quality factors directly perceived by students.
Second, while student perceptions of quality are used in innumerable studies, our overall understanding still needs to integrate them more holistically. Many studies use student perceptions of quality and overall effectiveness of individual tools and strategies in online contexts such as mobile devices (Drew & Mann, 2018 ), small groups (Choi, Land, & Turgeon, 2005 ), journals (Nair, Tay, & Koh, 2013 ), simulations (Vlachopoulos & Makri, 2017 ), video (Lange & Costley, 2020 ), etc. Such studies, however, cannot provide the overall context and comparative importance. Some studies have examined the overall learning experience of students with exploratory lists, but have mixed non-quality factors with quality of teaching factors making it difficult to discern the instructor’s versus contextual roles in quality (e.g., Asoodar, Vaezi, & Izanloo, 2016 ; Bollinger & Martindale, 2004 ; Farrell & Brunton, 2020 ; Hong, 2002 ; Song, Singleton, Hill, & Koh, 2004 ; Sun, Tsai, Finger, Chen, & Yeh, 2008 ). The application of technology adoption studies also fall into this category by essentially aggregating all teaching quality in the single category of performance ( Al-Gahtani, 2016 ; Artino, 2010 ). Some studies have used high-level teaching-oriented models, primarily the Community of Inquiry model (le Roux & Nagel, 2018 ), but empirical support has been mixed (Arbaugh et al., 2008 ); and its elegance (i.e., relying on only three factors) has not provided much insight to practitioners (Anderson, 2016 ; Cleveland-Innes & Campbell, 2012 ).
Research questions
Integration of studies and concepts explored continues to be fragmented and confusing despite the fact that the number of empirical studies related to student perceptions of quality factors has increased. It is important to have an empirical view of what students’ value in a single comprehensive study and, also, to know if there is a hierarchy of factors, ranging from students who are least to most critical of the online learning experience. This research study has two research questions.
The first research question is: What are the significant factors in creating a high-quality online learning experience from students’ perspectives? That is important to know because it should have a significant effect on the instructor’s design of online classes. The goal of this research question is identify a more articulated and empirically-supported set of factors capturing the full range of student expectations.
The second research question is: Is there a priority or hierarchy of factors related to students’ perceptions of online teaching quality that relate to their decisions to enroll in online classes? For example, is it possible to distinguish which factors are critical for enrollment decisions when students are primarily motivated by convenience and scheduling flexibility (minimum threshold)? Do these factors differ from students with a genuine acceptance of the general quality of online courses (a moderate threshold)? What are the factors that are important for the students who are the most critical of online course delivery (highest threshold)?
This article next reviews the literature on online education quality, focusing on the student perspective and reviews eight factors derived from it. The research methods section discusses the study structure and methods. Demographic data related to the sample are next, followed by the results, discussion, and conclusion.
Literature review
Online education is much discussed (Prinsloo, 2016 ; Van Wart et al., 2019 ; Zawacki-Richter & Naidu, 2016 ), but its perception is substantially influenced by where you stand and what you value (Otter et al., 2013 ; Tanner, Noser, & Totaro, 2009 ). Accrediting bodies care about meeting technical standards, proof of effectiveness, and consistency (Grandzol & Grandzol, 2006 ). Institutions care about reputation, rigor, student satisfaction, and institutional efficiency (Jung, 2011 ). Faculty care about subject coverage, student participation, faculty satisfaction, and faculty workload (Horvitz, Beach, Anderson, & Xia, 2015 ; Mansbach & Austin, 2018 ). For their part, students care about learning achievement (Marks, Sibley, & Arbaugh, 2005 ; O’Neill & Sai, 2014 ; Shen, Cho, Tsai, & Marra, 2013 ), but also view online education as a function of their enjoyment of classes, instructor capability and responsiveness, and comfort in the learning environment (e.g., Asoodar et al., 2016 ; Sebastianelli, Swift, & Tamimi, 2015 ). It is this last perspective, of students, upon which we focus.
It is important to note students do not sign up for online classes solely based on perceived quality. Perceptions of quality derive from notions of the capacity of online learning when ideal—relative to both learning achievement and satisfaction/enjoyment, and perceptions about the likelihood and experience of classes living up to expectations. Students also sign up because of convenience and flexibility, and personal notions of suitability about learning. Convenience and flexibility are enormous drivers of online registration (Lee, Stringer, & Du, 2017 ; Mann & Henneberry, 2012 ). Even when students say they prefer face-to-face classes to online, many enroll in online classes and re-enroll in the future if the experience meets minimum expectations. This study examines the threshold expectations of students when they are considering taking online classes.
When discussing students’ perceptions of quality, there is little clarity about the actual range of concepts because no integrated empirical studies exist comparing major factors found throughout the literature. Rather, there are practitioner-generated lists of micro-competencies such as the Quality Matters consortium for higher education (Quality Matters, 2018 ), or broad frameworks encompassing many aspects of quality beyond teaching (Open and Distant Learning Quality Council, 2012 ). While checklists are useful for practitioners and accreditation processes, they do not provide robust, theoretical bases for scholarly development. Overarching frameworks are heuristically useful, but not for pragmatic purposes or theory building arenas. The most prominent theoretical framework used in online literature is the Community of Inquiry (CoI) model (Arbaugh et al., 2008 ; Garrison, Anderson, & Archer, 2003 ), which divides instruction into teaching, cognitive, and social presence. Like deductive theories, however, the supportive evidence is mixed (Rourke & Kanuka, 2009 ), especially regarding the importance of social presence (Annand, 2011 ; Armellini and De Stefani, 2016 ). Conceptually, the problem is not so much with the narrow articulation of cognitive or social presence; cognitive presence is how the instructor provides opportunities for students to interact with material in robust, thought-provoking ways, and social presence refers to building a community of learning that incorporates student-to-student interactions. However, teaching presence includes everything else the instructor does—structuring the course, providing lectures, explaining assignments, creating rehearsal opportunities, supplying tests, grading, answering questions, and so on. These challenges become even more prominent in the online context. While the lecture as a single medium is paramount in face-to-face classes, it fades as the primary vehicle in online classes with increased use of detailed syllabi, electronic announcements, recorded and synchronous lectures, 24/7 communications related to student questions, etc. Amassing the pedagogical and technological elements related to teaching under a single concept provides little insight.
In addition to the CoI model, numerous concepts are suggested in single-factor empirical studies when focusing on quality from a student’s perspective, with overlapping conceptualizations and nonstandardized naming conventions. Seven distinct factors are derived here from the literature of student perceptions of online quality: Instructional Support, Teaching Presence, Basic Online Modality, Social Presence, Online Social Comfort, cognitive Presence, and Interactive Online Modality.
Instructional support
Instructional Support refers to students’ perceptions of techniques by the instructor used for input, rehearsal, feedback, and evaluation. Specifically, this entails providing detailed instructions, designed use of multimedia, and the balance between repetitive class features for ease of use, and techniques to prevent boredom. Instructional Support is often included as an element of Teaching Presence, but is also labeled “structure” (Lee & Rha, 2009 ; So & Brush, 2008 ) and instructor facilitation (Eom, Wen, & Ashill, 2006 ). A prime example of the difference between face-to-face and online education is the extensive use of the “flipped classroom” (Maycock, 2019 ; Wang, Huang, & Schunn, 2019 ) in which students move to rehearsal activities faster and more frequently than traditional classrooms, with less instructor lecture (Jung, 2011 ; Martin, Wang, & Sadaf, 2018 ). It has been consistently supported as an element of student perceptions of quality (Espasa & Meneses, 2010 ).
- Teaching presence
Teaching Presence refers to students’ perceptions about the quality of communication in lectures, directions, and individual feedback including encouragement (Jaggars & Xu, 2016 ; Marks et al., 2005 ). Specifically, instructor communication is clear, focused, and encouraging, and instructor feedback is customized and timely. If Instructional Support is what an instructor does before the course begins and in carrying out those plans, then Teaching Presence is what the instructor does while the class is conducted and in response to specific circumstances. For example, a course could be well designed but poorly delivered because the instructor is distracted; or a course could be poorly designed but an instructor might make up for the deficit by spending time and energy in elaborate communications and ad hoc teaching techniques. It is especially important in student satisfaction (Sebastianelli et al., 2015 ; Young, 2006 ) and also referred to as instructor presence (Asoodar et al., 2016 ), learner-instructor interaction (Marks et al., 2005 ), and staff support (Jung, 2011 ). As with Instructional Support, it has been consistently supported as an element of student perceptions of quality.
Basic online modality
Basic Online Modality refers to the competent use of basic online class tools—online grading, navigation methods, online grade book, and the announcements function. It is frequently clumped with instructional quality (Artino, 2010 ), service quality (Mohammadi, 2015 ), instructor expertise in e-teaching (Paechter, Maier, & Macher, 2010 ), and similar terms. As a narrowly defined concept, it is sometimes called technology (Asoodar et al., 2016 ; Bollinger & Martindale, 2004 ; Sun et al., 2008 ). The only empirical study that did not find Basic Online Modality significant, as technology, was Sun et al. ( 2008 ). Because Basic Online Modality is addressed with basic instructor training, some studies assert the importance of training (e.g., Asoodar et al., 2016 ).
Social presence
Social Presence refers to students’ perceptions of the quality of student-to-student interaction. Social Presence focuses on the quality of shared learning and collaboration among students, such as in threaded discussion responses (Garrison et al., 2003 ; Kehrwald, 2008 ). Much emphasized but challenged in the CoI literature (Rourke & Kanuka, 2009 ), it has mixed support in the online literature. While some studies found Social Presence or related concepts to be significant (e.g., Asoodar et al., 2016 ; Bollinger & Martindale, 2004 ; Eom et al., 2006 ; Richardson, Maeda, Lv, & Caskurlu, 2017 ), others found Social Presence insignificant (Joo, Lim, & Kim, 2011 ; So & Brush, 2008 ; Sun et al., 2008 ).
Online social comfort
Online Social Comfort refers to the instructor’s ability to provide an environment in which anxiety is low, and students feel comfortable interacting even when expressing opposing viewpoints. While numerous studies have examined anxiety (e.g., Liaw & Huang, 2013 ; Otter et al., 2013 ; Sun et al., 2008 ), only one found anxiety insignificant (Asoodar et al., 2016 ); many others have not examined the concept.
- Cognitive presence
Cognitive Presence refers to the engagement of students such that they perceive they are stimulated by the material and instructor to reflect deeply and critically, and seek to understand different perspectives (Garrison et al., 2003 ). The instructor provides instructional materials and facilitates an environment that piques interest, is reflective, and enhances inclusiveness of perspectives (Durabi, Arrastia, Nelson, Cornille, & Liang, 2011 ). Cognitive Presence includes enhancing the applicability of material for student’s potential or current careers. Cognitive Presence is supported as significant in many online studies (e.g., Artino, 2010 ; Asoodar et al., 2016 ; Joo et al., 2011 ; Marks et al., 2005 ; Sebastianelli et al., 2015 ; Sun et al., 2008 ). Further, while many instructors perceive that cognitive presence is diminished in online settings, neuroscientific studies indicate this need not be the case (Takamine, 2017 ). While numerous studies failed to examine Cognitive Presence, this review found no studies that lessened its significance for students.
Interactive online modality
Interactive Online Modality refers to the “high-end” usage of online functionality. That is, the instructor uses interactive online class tools—video lectures, videoconferencing, and small group discussions—well. It is often included in concepts such as instructional quality (Artino, 2010 ; Asoodar et al., 2016 ; Mohammadi, 2015 ; Otter et al., 2013 ; Paechter et al., 2010 ) or engagement (Clayton, Blumberg, & Anthony, 2018 ). While individual methods have been investigated (e.g. Durabi et al., 2011 ), high-end engagement methods have not.
Other independent variables affecting perceptions of quality include age, undergraduate versus graduate status, gender, ethnicity/race, discipline, educational motivation of students, and previous online experience. While age has been found to be small or insignificant, more notable effects have been reported at the level-of-study, with graduate students reporting higher “success” (Macon, 2011 ), and community college students having greater difficulty with online classes (Legon & Garrett, 2019 ; Xu & Jaggars, 2014 ). Ethnicity and race have also been small or insignificant. Some situational variations and student preferences can be captured by paying attention to disciplinary differences (Arbaugh, 2005 ; Macon, 2011 ). Motivation levels of students have been reported to be significant in completion and achievement, with better students doing as well across face-to-face and online modes, and weaker students having greater completion and achievement challenges (Clayton et al., 2018 ; Lu & Lemonde, 2013 ).
Research methods
To examine the various quality factors, we apply a critical success factor methodology, initially introduced to schools of business research in the 1970s. In 1981, Rockhart and Bullen codified an approach embodying principles of critical success factors (CSFs) as a way to identify the information needs of executives, detailing steps for the collection and analyzation of data to create a set of organizational CSFs (Rockhart & Bullen, 1981 ). CSFs describe the underlying or guiding principles which must be incorporated to ensure success.
Utilizing this methodology, CSFs in the context of this paper define key areas of instruction and design essential for an online class to be successful from a student’s perspective. Instructors implicitly know and consider these areas when setting up an online class and designing and directing activities and tasks important to achieving learning goals. CSFs make explicit those things good instructors may intuitively know and (should) do to enhance student learning. When made explicit, CSFs not only confirm the knowledge of successful instructors, but tap their intuition to guide and direct the accomplishment of quality instruction for entire programs. In addition, CSFs are linked with goals and objectives, helping generate a small number of truly important matters an instructor should focus attention on to achieve different thresholds of online success.
After a comprehensive literature review, an instrument was created to measure students’ perceptions about the importance of techniques and indicators leading to quality online classes. Items were designed to capture the major factors in the literature. The instrument was pilot studied during academic year 2017–18 with a 397 student sample, facilitating an exploratory factor analysis leading to important preliminary findings (reference withheld for review). Based on the pilot, survey items were added and refined to include seven groups of quality teaching factors and two groups of items related to students’ overall acceptance of online classes as well as a variable on their future online class enrollment. Demographic information was gathered to determine their effects on students’ levels of acceptance of online classes based on age, year in program, major, distance from university, number of online classes taken, high school experience with online classes, and communication preferences.
This paper draws evidence from a sample of students enrolled in educational programs at Jack H. Brown College of Business and Public Administration (JHBC), California State University San Bernardino (CSUSB). The JHBC offers a wide range of online courses for undergraduate and graduate programs. To ensure comparable learning outcomes, online classes and face-to-face classes of a certain subject are similar in size—undergraduate classes are generally capped at 60 and graduate classes at 30, and often taught by the same instructors. Students sometimes have the option to choose between both face-to-face and online modes of learning.
A Qualtrics survey link was sent out by 11 instructors to students who were unlikely to be cross-enrolled in classes during the 2018–19 academic year. 1 Approximately 2500 students were contacted, with some instructors providing class time to complete the anonymous survey. All students, whether they had taken an online class or not, were encouraged to respond. Nine hundred eighty-seven students responded, representing a 40% response rate. Although drawn from a single business school, it is a broad sample representing students from several disciplines—management, accounting and finance, marketing, information decision sciences, and public administration, as well as both graduate and undergraduate programs of study.
The sample age of students is young, with 78% being under 30. The sample has almost no lower division students (i.e., freshman and sophomore), 73% upper division students (i.e., junior and senior) and 24% graduate students (master’s level). Only 17% reported having taken a hybrid or online class in high school. There was a wide range of exposure to university level online courses, with 47% reporting having taken 1 to 4 classes, and 21% reporting no online class experience. As a Hispanic-serving institution, 54% self-identified as Latino, 18% White, and 13% Asian and Pacific Islander. The five largest majors were accounting & finance (25%), management (21%), master of public administration (16%), marketing (12%), and information decision sciences (10%). Seventy-four percent work full- or part-time. See Table 1 for demographic data.
Measures and procedure
To increase the reliability of evaluation scores, composite evaluation variables are formed after an exploratory factor analysis of individual evaluation items. A principle component method with Quartimin (oblique) rotation was applied to explore the factor construct of student perceptions of online teaching CSFs. The item correlations for student perceptions of importance coefficients greater than .30 were included, a commonly acceptable ratio in factor analysis. A simple least-squares regression analysis was applied to test the significance levels of factors on students’ impression of online classes.
Exploratory factor constructs
Using a threshold loading of 0.3 for items, 37 items loaded on seven factors. All factors were logically consistent. The first factor, with eight items, was labeled Teaching Presence. Items included providing clear instructions, staying on task, clear deadlines, and customized feedback on strengths and weaknesses. Teaching Presence items all related to instructor involvement during the course as a director, monitor, and learning facilitator. The second factor, with seven items, aligned with Cognitive Presence. Items included stimulating curiosity, opportunities for reflection, helping students construct explanations posed in online courses, and the applicability of material. The third factor, with six items, aligned with Social Presence defined as providing student-to-student learning opportunities. Items included getting to know course participants for sense of belonging, forming impressions of other students, and interacting with others. The fourth factor, with six new items as well as two (“interaction with other students” and “a sense of community in the class”) shared with the third factor, was Instructional Support which related to the instructor’s roles in providing students a cohesive learning experience. They included providing sufficient rehearsal, structured feedback, techniques for communication, navigation guide, detailed syllabus, and coordinating student interaction and creating a sense of online community. This factor also included enthusiasm which students generally interpreted as a robustly designed course, rather than animation in a traditional lecture. The fifth factor was labeled Basic Online Modality and focused on the basic technological requirements for a functional online course. Three items included allowing students to make online submissions, use of online gradebooks, and online grading. A fourth item is the use of online quizzes, viewed by students as mechanical practice opportunities rather than small tests and a fifth is navigation, a key component of Online Modality. The sixth factor, loaded on four items, was labeled Online Social Comfort. Items here included comfort discussing ideas online, comfort disagreeing, developing a sense of collaboration via discussion, and considering online communication as an excellent medium for social interaction. The final factor was called Interactive Online Modality because it included items for “richer” communications or interactions, no matter whether one- or two-way. Items included videoconferencing, instructor-generated videos, and small group discussions. Taken together, these seven explained 67% of the variance which is considered in the acceptable range in social science research for a robust model (Hair, Black, Babin, & Anderson, 2014 ). See Table 2 for the full list.
To test for factor reliability, the Cronbach alpha of variables were calculated. All produced values greater than 0.7, the standard threshold used for reliability, except for system trust which was therefore dropped. To gauge students’ sense of factor importance, all items were means averaged. Factor means (lower means indicating higher importance to students), ranged from 1.5 to 2.6 on a 5-point scale. Basic Online Modality was most important, followed by Instructional Support and Teaching Presence. Students deemed Cognitive Presence, Social Online Comfort, and Online Interactive Modality less important. The least important for this sample was Social Presence. Table 3 arrays the critical success factor means, standard deviations, and Cronbach alpha.
To determine whether particular subgroups of respondents viewed factors differently, a series of ANOVAs were conducted using factor means as dependent variables. Six demographic variables were used as independent variables: graduate vs. undergraduate, age, work status, ethnicity, discipline, and past online experience. To determine strength of association of the independent variables to each of the seven CSFs, eta squared was calculated for each ANOVA. Eta squared indicates the proportion of variance in the dependent variable explained by the independent variable. Eta squared values greater than .01, .06, and .14 are conventionally interpreted as small, medium, and large effect sizes, respectively (Green & Salkind, 2003 ). Table 4 summarizes the eta squared values for the ANOVA tests with Eta squared values less than .01 omitted.
While no significant differences in factor means among students in different disciplines in the College occur, all five other independent variables have some small effect on some or all CSFs. Graduate students tend to rate Online Interactive Modality, Instructional Support, Teaching Presence, and Cognitive Presence higher than undergraduates. Elder students value more Online Interactive Modality. Full-time working students rate all factors, except Social Online Comfort, slightly higher than part-timers and non-working students. Latino and White rate Basic Online Modality and Instructional Support higher; Asian and Pacific Islanders rate Social Presence higher. Students who have taken more online classes rate all factors higher.
In addition to factor scores, two variables are constructed to identify the resultant impressions labeled online experience. Both were logically consistent with a Cronbach’s α greater than 0.75. The first variable, with six items, labeled “online acceptance,” included items such as “I enjoy online learning,” “My overall impression of hybrid/online learning is very good,” and “the instructors of online/hybrid classes are generally responsive.” The second variable was labeled “face-to-face preference” and combines four items, including enjoying, learning, and communicating more in face-to-face classes, as well as perceiving greater fairness and equity. In addition to these two constructed variables, a one-item variable was also used subsequently in the regression analysis: “online enrollment.” That question asked: if hybrid/online classes are well taught and available, how much would online education make up your entire course selection going forward?
Regression results
As noted above, two constructed variables and one item were used as dependent variables for purposes of regression analysis. They were online acceptance, F2F preference, and the selection of online classes. In addition to seven quality-of-teaching factors identified by factor analysis, control variables included level of education (graduate versus undergraduate), age, ethnicity, work status, distance to university, and number of online/hybrid classes taken in the past. See Table 5 .
When the ETA squared values for ANOVA significance were measured for control factors, only one was close to a medium effect. Graduate versus undergraduate status had a .05 effect (considered medium) related to Online Interactive Modality, meaning graduate students were more sensitive to interactive modality than undergraduates. Multiple regression analysis of critical success factors and online impressions were conducted to compare under what conditions factors were significant. The only consistently significant control factor was number of online classes taken. The more classes students had taken online, the more inclined they were to take future classes. Level of program, age, ethnicity, and working status do not significantly affect students’ choice or overall acceptance of online classes.
The least restrictive condition was online enrollment (Table 6 ). That is, students might not feel online courses were ideal, but because of convenience and scheduling might enroll in them if minimum threshold expectations were met. When considering online enrollment three factors were significant and positive (at the 0.1 level): Basic Online Modality, Cognitive Presence, and Online Social Comfort. These least-demanding students expected classes to have basic technological functionality, provide good opportunities for knowledge acquisition, and provide comfortable interaction in small groups. Students who demand good Instructional Support (e.g., rehearsal opportunities, standardized feedback, clear syllabus) are less likely to enroll.
Online acceptance was more restrictive (see Table 7 ). This variable captured the idea that students not only enrolled in online classes out of necessity, but with an appreciation of the positive attributes of online instruction, which balanced the negative aspects. When this standard was applied, students expected not only Basic Online Modality, Cognitive Presence, and Online Social Comfort, but expected their instructors to be highly engaged virtually as the course progressed (Teaching Presence), and to create strong student-to-student dynamics (Social Presence). Students who rated Instructional Support higher are less accepting of online classes.
Another restrictive condition was catering to the needs of students who preferred face-to-face classes (see Table 8 ). That is, they preferred face-to-face classes even when online classes were well taught. Unlike students more accepting of, or more likely to enroll in, online classes, this group rates Instructional Support as critical to enrolling, rather than a negative factor when absent. Again different from the other two groups, these students demand appropriate interactive mechanisms (Online Interactive Modality) to enable richer communication (e.g., videoconferencing). Student-to-student collaboration (Social Presence) was also significant. This group also rated Cognitive Presence and Online Social Comfort as significant, but only in their absence. That is, these students were most attached to direct interaction with the instructor and other students rather than specific teaching methods. Interestingly, Basic Online Modality and Teaching Presence were not significant. Our interpretation here is this student group, most critical of online classes for its loss of physical interaction, are beyond being concerned with mechanical technical interaction and demand higher levels of interactivity and instructional sophistication.
Discussion and study limitations
Some past studies have used robust empirical methods to identify a single factor or a small number of factors related to quality from a student’s perspective, but have not sought to be relatively comprehensive. Others have used a longer series of itemized factors, but have less used less robust methods, and have not tied those factors back to the literature. This study has used the literature to develop a relatively comprehensive list of items focused on quality teaching in a single rigorous protocol. That is, while a Beta test had identified five coherent factors, substantial changes to the current survey that sharpened the focus on quality factors rather than antecedent factors, as well as better articulating the array of factors often lumped under the mantle of “teaching presence.” In addition, it has also examined them based on threshold expectations: from minimal, such as when flexibility is the driving consideration, to modest, such as when students want a “good” online class, to high, when students demand an interactive virtual experience equivalent to face-to-face.
Exploratory factor analysis identified seven factors that were reliable, coherent, and significant under different conditions. When considering students’ overall sense of importance, they are, in order: Basic Online Modality, Instructional Support, Teaching Presence, Cognitive Presence, Social Online Comfort, Interactive Online Modality, and Social Presence. Students are most concerned with the basics of a course first, that is the technological and instructor competence. Next they want engagement and virtual comfort. Social Presence, while valued, is the least critical from this overall perspective.
The factor analysis is quite consistent with the range of factors identified in the literature, pointing to the fact that students can differentiate among different aspects of what have been clumped as larger concepts, such as teaching presence. Essentially, the instructor’s role in quality can be divided into her/his command of basic online functionality, good design, and good presence during the class. The instructor’s command of basic functionality is paramount. Because so much of online classes must be built in advance of the class, quality of the class design is rated more highly than the instructor’s role in facilitating the class. Taken as a whole, the instructor’s role in traditional teaching elements is primary, as we would expect it to be. Cognitive presence, especially as pertinence of the instructional material and its applicability to student interests, has always been found significant when studied, and was highly rated as well in a single factor. Finally, the degree to which students feel comfortable with the online environment and enjoy the learner-learner aspect has been less supported in empirical studies, was found significant here, but rated the lowest among the factors of quality to students.
Regression analysis paints a more nuanced picture, depending on student focus. It also helps explain some of the heterogeneity of previous studies, depending on what the dependent variables were. If convenience and scheduling are critical and students are less demanding, minimum requirements are Basic Online Modality, Cognitive Presence, and Online Social Comfort. That is, students’ expect an instructor who knows how to use an online platform, delivers useful information, and who provides a comfortable learning environment. However, they do not expect to get poor design. They do not expect much in terms of the quality teaching presence, learner-to-learner interaction, or interactive teaching.
When students are signing up for critical classes, or they have both F2F and online options, they have a higher standard. That is, they not only expect the factors for decisions about enrolling in noncritical classes, but they also expect good Teaching and Social Presence. Students who simply need a class may be willing to teach themselves a bit more, but students who want a good class expect a highly present instructor in terms responsiveness and immediacy. “Good” classes must not only create a comfortable atmosphere, but in social science classes at least, must provide strong learner-to-learner interactions as well. At the time of the research, most students believe that you can have a good class without high interactivity via pre-recorded video and videoconference. That may, or may not, change over time as technology thresholds of various video media become easier to use, more reliable, and more commonplace.
The most demanding students are those who prefer F2F classes because of learning style preferences, poor past experiences, or both. Such students (seem to) assume that a worthwhile online class has basic functionality and that the instructor provides a strong presence. They are also critical of the absence of Cognitive Presence and Online Social Comfort. They want strong Instructional Support and Social Presence. But in addition, and uniquely, they expect Online Interactive Modality which provides the greatest verisimilitude to the traditional classroom as possible. More than the other two groups, these students crave human interaction in the learning process, both with the instructor and other students.
These findings shed light on the possible ramifications of the COVID-19 aftermath. Many universities around the world jumped from relatively low levels of online instruction in the beginning of spring 2020 to nearly 100% by mandate by the end of the spring term. The question becomes, what will happen after the mandate is removed? Will demand resume pre-crisis levels, will it increase modestly, or will it skyrocket? Time will be the best judge, but the findings here would suggest that the ability/interest of instructors and institutions to “rise to the occasion” with quality teaching will have as much effect on demand as students becoming more acclimated to online learning. If in the rush to get classes online many students experience shoddy basic functional competence, poor instructional design, sporadic teaching presence, and poorly implemented cognitive and social aspects, they may be quite willing to return to the traditional classroom. If faculty and institutions supporting them are able to increase the quality of classes despite time pressures, then most students may be interested in more hybrid and fully online classes. If instructors are able to introduce high quality interactive teaching, nearly the entire student population will be interested in more online classes. Of course students will have a variety of experiences, but this analysis suggests that those instructors, departments, and institutions that put greater effort into the temporary adjustment (and who resist less), will be substantially more likely to have increases in demand beyond what the modest national trajectory has been for the last decade or so.
There are several study limitations. First, the study does not include a sample of non-respondents. Non-responders may have a somewhat different profile. Second, the study draws from a single college and university. The profile derived here may vary significantly by type of student. Third, some survey statements may have led respondents to rate quality based upon experience rather than assess the general importance of online course elements. “I felt comfortable participating in the course discussions,” could be revised to “comfort in participating in course discussions.” The authors weighed differences among subgroups (e.g., among majors) as small and statistically insignificant. However, it is possible differences between biology and marketing students would be significant, leading factors to be differently ordered. Emphasis and ordering might vary at a community college versus research-oriented university (Gonzalez, 2009 ).
Availability of data and materials
We will make the data available.
Al-Gahtani, S. S. (2016). Empirical investigation of e-learning acceptance and assimilation: A structural equation model. Applied Comput Information , 12 , 27–50.
Google Scholar
Alqurashi, E. (2016). Self-efficacy in online learning environments: A literature review. Contemporary Issues Educ Res (CIER) , 9 (1), 45–52.
Anderson, T. (2016). A fourth presence for the Community of Inquiry model? Retrieved from https://virtualcanuck.ca/2016/01/04/a-fourth-presence-for-the-community-of-inquiry-model/ .
Annand, D. (2011). Social presence within the community of inquiry framework. The International Review of Research in Open and Distributed Learning , 12 (5), 40.
Arbaugh, J. B. (2005). How much does “subject matter” matter? A study of disciplinary effects in on-line MBA courses. Academy of Management Learning & Education , 4 (1), 57–73.
Arbaugh, J. B., Cleveland-Innes, M., Diaz, S. R., Garrison, D. R., Ice, P., Richardson, J. C., & Swan, K. P. (2008). Developing a community of inquiry instrument: Testing a measure of the Community of Inquiry framework using a multi-institutional sample. Internet and Higher Education , 11 , 133–136.
Armellini, A., & De Stefani, M. (2016). Social presence in the 21st century: An adjustment to the Community of Inquiry framework. British Journal of Educational Technology , 47 (6), 1202–1216.
Arruabarrena, R., Sánchez, A., Blanco, J. M., et al. (2019). Integration of good practices of active methodologies with the reuse of student-generated content. International Journal of Educational Technology in Higher Education , 16 , #10.
Arthur, L. (2009). From performativity to professionalism: Lecturers’ responses to student feedback. Teaching in Higher Education , 14 (4), 441–454.
Artino, A. R. (2010). Online or face-to-face learning? Exploring the personal factors that predict students’ choice of instructional format. Internet and Higher Education , 13 , 272–276.
Asoodar, M., Vaezi, S., & Izanloo, B. (2016). Framework to improve e-learner satisfaction and further strengthen e-learning implementation. Computers in Human Behavior , 63 , 704–716.
Bernard, R. M., et al. (2004). How does distance education compare with classroom instruction? A meta-analysis of the empirical literature. Review of Educational Research , 74 (3), 379–439.
Bollinger, D., & Martindale, T. (2004). Key factors for determining student satisfaction in online courses. Int J E-learning , 3 (1), 61–67.
Brinkley-Etzkorn, K. E. (2018). Learning to teach online: Measuring the influence of faculty development training on teaching effectiveness through a TPACK lens. The Internet and Higher Education , 38 , 28–35.
Chickering, A. W., & Gamson, Z. F. (1987). Seven principles for good practice in undergraduate education. AAHE Bulletin , 3 , 7.
Choi, I., Land, S. M., & Turgeon, A. J. (2005). Scaffolding peer-questioning strategies to facilitate metacognition during online small group discussion. Instructional Science , 33 , 483–511.
Clayton, K. E., Blumberg, F. C., & Anthony, J. A. (2018). Linkages between course status, perceived course value, and students’ preferences for traditional versus non-traditional learning environments. Computers & Education , 125 , 175–181.
Cleveland-Innes, M., & Campbell, P. (2012). Emotional presence, learning, and the online learning environment. The International Review of Research in Open and Distributed Learning , 13 (4), 269–292.
Cohen, A., & Baruth, O. (2017). Personality, learning, and satisfaction in fully online academic courses. Computers in Human Behavior , 72 , 1–12.
Crews, T., & Butterfield, J. (2014). Data for flipped classroom design: Using student feedback to identify the best components from online and face-to-face classes. Higher Education Studies , 4 (3), 38–47.
Dawson, P., Henderson, M., Mahoney, P., Phillips, M., Ryan, T., Boud, D., & Molloy, E. (2019). What makes for effective feedback: Staff and student perspectives. Assessment & Evaluation in Higher Education , 44 (1), 25–36.
Drew, C., & Mann, A. (2018). Unfitting, uncomfortable, unacademic: A sociological reading of an interactive mobile phone app in university lectures. International Journal of Educational Technology in Higher Education , 15 , #43.
Durabi, A., Arrastia, M., Nelson, D., Cornille, T., & Liang, X. (2011). Cognitive presence in asynchronous online learning: A comparison of four discussion strategies. Journal of Computer Assisted Learning , 27 (3), 216–227.
Eom, S. B., Wen, H. J., & Ashill, N. (2006). The determinants of students’ perceived learning outcomes and satisfaction in university online education: An empirical investigation. Decision Sciences Journal of Innovative Education , 4 (2), 215–235.
Espasa, A., & Meneses, J. (2010). Analysing feedback processes in an online teaching and learning environment: An exploratory study. Higher Education , 59 (3), 277–292.
Farrell, O., & Brunton, J. (2020). A balancing act: A window into online student engagement experiences. International Journal of Educational Technology in High Education , 17 , #25.
Fidalgo, P., Thormann, J., Kulyk, O., et al. (2020). Students’ perceptions on distance education: A multinational study. International Journal of Educational Technology in High Education , 17 , #18.
Flores, Ò., del-Arco, I., & Silva, P. (2016). The flipped classroom model at the university: Analysis based on professors’ and students’ assessment in the educational field. International Journal of Educational Technology in Higher Education , 13 , #21.
Garrison, D. R., Anderson, T., & Archer, W. (2003). A theory of critical inquiry in online distance education. Handbook of Distance Education , 1 , 113–127.
Gong, D., Yang, H. H., & Cai, J. (2020). Exploring the key influencing factors on college students’ computational thinking skills through flipped-classroom instruction. International Journal of Educational Technology in Higher Education , 17 , #19.
Gonzalez, C. (2009). Conceptions of, and approaches to, teaching online: A study of lecturers teaching postgraduate distance courses. Higher Education , 57 (3), 299–314.
Grandzol, J. R., & Grandzol, C. J. (2006). Best practices for online business Education. International Review of Research in Open and Distance Learning , 7 (1), 1–18.
Green, S. B., & Salkind, N. J. (2003). Using SPSS: Analyzing and understanding data , (3rd ed., ). Upper Saddle River: Prentice Hall.
Hair, J. F., Black, W. C., Babin, B. J., & Anderson, R. E. (2014). Multivariate data analysis: Pearson new international edition . Essex: Pearson Education Limited.
Harjoto, M. A. (2017). Blended versus face-to-face: Evidence from a graduate corporate finance class. Journal of Education for Business , 92 (3), 129–137.
Hong, K.-S. (2002). Relationships between students’ instructional variables with satisfaction and learning from a web-based course. The Internet and Higher Education , 5 , 267–281.
Horvitz, B. S., Beach, A. L., Anderson, M. L., & Xia, J. (2015). Examination of faculty self-efficacy related to online teaching. Innovation Higher Education , 40 , 305–316.
Inside Higher Education and Gallup. (2019). The 2019 survey of faculty attitudes on technology. Author .
Jaggars, S. S., & Xu, D. (2016). How do online course design features influence student performance? Computers and Education , 95 , 270–284.
Joo, Y. J., Lim, K. Y., & Kim, E. K. (2011). Online university students’ satisfaction and persistence: Examining perceived level of presence, usefulness and ease of use as predictor in a structural model. Computers & Education , 57 (2), 1654–1664.
Jung, I. (2011). The dimensions of e-learning quality: From the learner’s perspective. Educational Technology Research and Development , 59 (4), 445–464.
Kay, R., MacDonald, T., & DiGiuseppe, M. (2019). A comparison of lecture-based, active, and flipped classroom teaching approaches in higher education. Journal of Computing in Higher Education , 31 , 449–471.
Kehrwald, B. (2008). Understanding social presence in text-based online learning environments. Distance Education , 29 (1), 89–106.
Kintu, M. J., Zhu, C., & Kagambe, E. (2017). Blended learning effectiveness: The relationship between student characteristics, design features and outcomes. International Journal of Educational Technology in Higher Education , 14 , #7.
Kuo, Y.-C., Walker, A. E., Schroder, K. E., & Belland, B. R. (2013). Interaction, internet self-efficacy, and self-regulated learning as predictors of student satisfaction in online education courses. Internet and Education , 20 , 35–50.
Lange, C., & Costley, J. (2020). Improving online video lectures: Learning challenges created by media. International Journal of Educational Technology in Higher Education , 17 , #16.
le Roux, I., & Nagel, L. (2018). Seeking the best blend for deep learning in a flipped classroom – Viewing student perceptions through the Community of Inquiry lens. International Journal of Educational Technology in High Education , 15 , #16.
Lee, H.-J., & Rha, I. (2009). Influence of structure and interaction on student achievement and satisfaction in web-based distance learning. Educational Technology & Society , 12 (4), 372–382.
Lee, Y., Stringer, D., & Du, J. (2017). What determines students’ preference of online to F2F class? Business Education Innovation Journal , 9 (2), 97–102.
Legon, R., & Garrett, R. (2019). CHLOE 3: Behind the numbers . Published online by Quality Matters and Eduventures. https://www.qualitymatters.org/sites/default/files/research-docs-pdfs/CHLOE-3-Report-2019-Behind-the-Numbers.pdf
Liaw, S.-S., & Huang, H.-M. (2013). Perceived satisfaction, perceived usefulness and interactive learning environments as predictors of self-regulation in e-learning environments. Computers & Education , 60 (1), 14–24.
Lu, F., & Lemonde, M. (2013). A comparison of online versus face-to-face students teaching delivery in statistics instruction for undergraduate health science students. Advances in Health Science Education , 18 , 963–973.
Lundin, M., Bergviken Rensfeldt, A., Hillman, T., Lantz-Andersson, A., & Peterson, L. (2018). Higher education dominance and siloed knowledge: a systematic review of flipped classroom research. International Journal of Educational Technology in Higher Education , 15 (1).
Macon, D. K. (2011). Student satisfaction with online courses versus traditional courses: A meta-analysis . Disssertation: Northcentral University, CA.
Mann, J., & Henneberry, S. (2012). What characteristics of college students influence their decisions to select online courses? Online Journal of Distance Learning Administration , 15 (5), 1–14.
Mansbach, J., & Austin, A. E. (2018). Nuanced perspectives about online teaching: Mid-career senior faculty voices reflecting on academic work in the digital age. Innovative Higher Education , 43 (4), 257–272.
Marks, R. B., Sibley, S. D., & Arbaugh, J. B. (2005). A structural equation model of predictors for effective online learning. Journal of Management Education , 29 (4), 531–563.
Martin, F., Wang, C., & Sadaf, A. (2018). Student perception of facilitation strategies that enhance instructor presence, connectedness, engagement and learning in online courses. Internet and Higher Education , 37 , 52–65.
Maycock, K. W. (2019). Chalk and talk versus flipped learning: A case study. Journal of Computer Assisted Learning , 35 , 121–126.
McGivney-Burelle, J. (2013). Flipping Calculus. PRIMUS Problems, Resources, and Issues in Mathematics Undergraduate . Studies , 23 (5), 477–486.
Mohammadi, H. (2015). Investigating users’ perspectives on e-learning: An integration of TAM and IS success model. Computers in Human Behavior , 45 , 359–374.
Nair, S. S., Tay, L. Y., & Koh, J. H. L. (2013). Students’ motivation and teachers’ teaching practices towards the use of blogs for writing of online journals. Educational Media International , 50 (2), 108–119.
Nguyen, T. (2015). The effectiveness of online learning: Beyond no significant difference and future horizons. MERLOT Journal of Online Learning and Teaching , 11 (2), 309–319.
Ni, A. Y. (2013). Comparing the effectiveness of classroom and online learning: Teaching research methods. Journal of Public Affairs Education , 19 (2), 199–215.
Nouri, J. (2016). The flipped classroom: For active, effective and increased learning – Especially for low achievers. International Journal of Educational Technology in Higher Education , 13 , #33.
O’Neill, D. K., & Sai, T. H. (2014). Why not? Examining college students’ reasons for avoiding an online course. Higher Education , 68 (1), 1–14.
O'Flaherty, J., & Phillips, C. (2015). The use of flipped classrooms in higher education: A scoping review. The Internet and Higher Education , 25 , 85–95.
Open & Distant Learning Quality Council (2012). ODLQC standards . England: Author https://www.odlqc.org.uk/odlqc-standards .
Ortagus, J. C. (2017). From the periphery to prominence: An examination of the changing profile of online students in American higher education. Internet and Higher Education , 32 , 47–57.
Otter, R. R., Seipel, S., Graef, T., Alexander, B., Boraiko, C., Gray, J., … Sadler, K. (2013). Comparing student and faculty perceptions of online and traditional courses. Internet and Higher Education , 19 , 27–35.
Paechter, M., Maier, B., & Macher, D. (2010). Online or face-to-face? Students’ experiences and preferences in e-learning. Internet and Higher Education , 13 , 292–329.
Prinsloo, P. (2016). (re)considering distance education: Exploring its relevance, sustainability and value contribution. Distance Education , 37 (2), 139–145.
Quality Matters (2018). Specific review standards from the QM higher Education rubric , (6th ed., ). MD: MarylandOnline.
Richardson, J. C., Maeda, Y., Lv, J., & Caskurlu, S. (2017). Social presence in relation to students’ satisfaction and learning in the online environment: A meta-analysis. Computers in Human Behavior , 71 , 402–417.
Rockhart, J. F., & Bullen, C. V. (1981). A primer on critical success factors . Cambridge: Center for Information Systems Research, Massachusetts Institute of Technology.
Rourke, L., & Kanuka, H. (2009). Learning in Communities of Inquiry: A Review of the Literature. The Journal of Distance Education / Revue de l'ducation Distance , 23 (1), 19–48 Athabasca University Press. Retrieved August 2, 2020 from https://www.learntechlib.org/p/105542/ .
Sebastianelli, R., Swift, C., & Tamimi, N. (2015). Factors affecting perceived learning, satisfaction, and quality in the online MBA: A structural equation modeling approach. Journal of Education for Business , 90 (6), 296–305.
Shen, D., Cho, M.-H., Tsai, C.-L., & Marra, R. (2013). Unpacking online learning experiences: Online learning self-efficacy and learning satisfaction. Internet and Higher Education , 19 , 10–17.
Sitzmann, T., Kraiger, K., Stewart, D., & Wisher, R. (2006). The comparative effectiveness of web-based and classroom instruction: A meta-analysis. Personnel Psychology , 59 (3), 623–664.
So, H. J., & Brush, T. A. (2008). Student perceptions of collaborative learning, social presence and satisfaction in a blended learning environment: Relationships and critical factors. Computers & Education , 51 (1), 318–336.
Song, L., Singleton, E. S., Hill, J. R., & Koh, M. H. (2004). Improving online learning: Student perceptions of useful and challenging characteristics. The Internet and Higher Education , 7 (1), 59–70.
Sun, P. C., Tsai, R. J., Finger, G., Chen, Y. Y., & Yeh, D. (2008). What drives a successful e-learning? An empirical investigation of the critical factors influencing learner satisfaction. Computers & Education , 50 (4), 1183–1202.
Takamine, K. (2017). Michelle D. miller: Minds online: Teaching effectively with technology. Higher Education , 73 , 789–791.
Tanner, J. R., Noser, T. C., & Totaro, M. W. (2009). Business faculty and undergraduate students’ perceptions of online learning: A comparative study. Journal of Information Systems Education , 20 (1), 29.
Tucker, B. (2012). The flipped classroom. Education Next , 12 (1), 82–83.
Van Wart, M., Ni, A., Ready, D., Shayo, C., & Court, J. (2020). Factors leading to online learner satisfaction. Business Educational Innovation Journal , 12 (1), 15–24.
Van Wart, M., Ni, A., Rose, L., McWeeney, T., & Worrell, R. A. (2019). Literature review and model of online teaching effectiveness integrating concerns for learning achievement, student satisfaction, faculty satisfaction, and institutional results. Pan-Pacific . Journal of Business Research , 10 (1), 1–22.
Ventura, A. C., & Moscoloni, N. (2015). Learning styles and disciplinary differences: A cross-sectional study of undergraduate students. International Journal of Learning and Teaching , 1 (2), 88–93.
Vlachopoulos, D., & Makri, A. (2017). The effect of games and simulations on higher education: A systematic literature review. International Journal of Educational Technology in Higher Education , 14 , #22.
Wang, Y., Huang, X., & Schunn, C. D. (2019). Redesigning flipped classrooms: A learning model and its effects on student perceptions. Higher Education , 78 , 711–728.
Wingo, N. P., Ivankova, N. V., & Moss, J. A. (2017). Faculty perceptions about teaching online: Exploring the literature using the technology acceptance model as an organizing framework. Online Learning , 21 (1), 15–35.
Xu, D., & Jaggars, S. S. (2014). Performance gaps between online and face-to-face courses: Differences across types of students and academic subject areas. Journal of Higher Education , 85 (5), 633–659.
Young, S. (2006). Student views of effective online teaching in higher education. American Journal of Distance Education , 20 (2), 65–77.
Zawacki-Richter, O., & Naidu, S. (2016). Mapping research trends from 35 years of publications in distance Education. Distance Education , 37 (3), 245–269.
Download references
Acknowledgements
No external funding/ NA.
Author information
Authors and affiliations.
Development for the JHB College of Business and Public Administration, 5500 University Parkway, San Bernardino, California, 92407, USA
Montgomery Van Wart, Anna Ni, Pamela Medina, Jesus Canelon, Melika Kordrostami, Jing Zhang & Yu Liu
You can also search for this author in PubMed Google Scholar
Contributions
Equal. The author(s) read and approved the final manuscript.
Corresponding author
Correspondence to Montgomery Van Wart .
Ethics declarations
Competing interests.
We have no competing interests.
Additional information
Publisher’s note.
Springer Nature remains neutral with regard to jurisdictional claims in published maps and institutional affiliations.
Rights and permissions
Open Access This article is licensed under a Creative Commons Attribution 4.0 International License, which permits use, sharing, adaptation, distribution and reproduction in any medium or format, as long as you give appropriate credit to the original author(s) and the source, provide a link to the Creative Commons licence, and indicate if changes were made. The images or other third party material in this article are included in the article's Creative Commons licence, unless indicated otherwise in a credit line to the material. If material is not included in the article's Creative Commons licence and your intended use is not permitted by statutory regulation or exceeds the permitted use, you will need to obtain permission directly from the copyright holder. To view a copy of this licence, visit http://creativecommons.org/licenses/by/4.0/ .
Reprints and permissions
About this article
Cite this article.
Van Wart, M., Ni, A., Medina, P. et al. Integrating students’ perspectives about online learning: a hierarchy of factors. Int J Educ Technol High Educ 17 , 53 (2020). https://doi.org/10.1186/s41239-020-00229-8
Download citation
Received : 29 April 2020
Accepted : 30 July 2020
Published : 02 December 2020
DOI : https://doi.org/10.1186/s41239-020-00229-8
Share this article
Anyone you share the following link with will be able to read this content:
Sorry, a shareable link is not currently available for this article.
Provided by the Springer Nature SharedIt content-sharing initiative
- Online education
- Online teaching
- Student perceptions
- Online quality
- Student presence
Thank you for visiting nature.com. You are using a browser version with limited support for CSS. To obtain the best experience, we recommend you use a more up to date browser (or turn off compatibility mode in Internet Explorer). In the meantime, to ensure continued support, we are displaying the site without styles and JavaScript.
- View all journals
- Explore content
- About the journal
- Publish with us
- Sign up for alerts
- Open access
- Published: 13 July 2024
Exploring the impact of artificial intelligence on higher education: The dynamics of ethical, social, and educational implications
- Abdulrahman M. Al-Zahrani ORCID: orcid.org/0009-0007-9885-0730 1 &
- Talal M. Alasmari ORCID: orcid.org/0000-0002-3330-1980 1
Humanities and Social Sciences Communications volume 11 , Article number: 912 ( 2024 ) Cite this article
626 Accesses
7 Altmetric
Metrics details
- Science, technology and society
The increasing prevalence of Artificial Intelligence (AI) in higher education underscores the necessity to explore its implications on ethical, social, and educational dynamics within the sector. This study aims to comprehensively investigate the impact of AI on higher education in Saudi Arabia, delving into stakeholders’ attitudes, perceptions, and expectations regarding its implementation. The research hones in on key facets of AI in higher education, encompassing its influence on teaching and learning, ethical and social implications, and the anticipated role of AI in the future. Employing a quantitative approach through an online survey questionnaire ( N = 1113), this study reveals positive attitudes toward AI in higher education. Stakeholders recognize its potential to enhance teaching and learning, streamline administration, and foster innovation. Emphasis is placed on ethical considerations and guidelines for AI implementation, highlighting the imperative need to address issues such as privacy, security, and bias. Participants envision a future characterized by personalized learning experiences, ethically integrated AI, collaboration, and ongoing support for lifelong learning. Furthermore, the results illuminate the intricate interplay between AI usage, purposes, difficulties, and their impact on attitudes, perceptions, and future implications. Accordingly, the research underscores the necessity for a comprehensive understanding of AI integration, considering not only its technical aspects but also the ethical, social, and educational dimensions. By acknowledging the role of AI uses, AI usage purposes, and addressing associated difficulties, educational stakeholders can work towards harnessing the benefits of AI while ensuring responsible and effective implementation in teaching and learning contexts.
Similar content being viewed by others
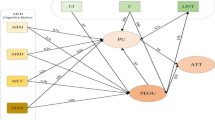
An explanatory study of factors influencing engagement in AI education at the K-12 Level: an extension of the classic TAM model
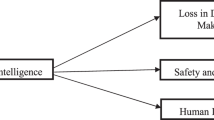
Impact of artificial intelligence on human loss in decision making, laziness and safety in education
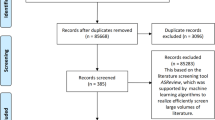
The dilemma and countermeasures of educational data ethics in the age of intelligence
Introduction.
Artificial Intelligence (AI) has become a transformative force, reshaping various industries such as communication systems, software applications, data storage, business operations, analytics, interactive platforms, cybersecurity, and social media (Al-Zahrani, 2023 ; Creely, 2022 ; Doncieux et al. 2022 ). Recent advancements in AI have profoundly impacted multiple aspects of life, including education and business. These advancements have fundamentally altered how we think, learn, operate, and thrive in an increasingly intelligent and interconnected world (Doncieux et al. 2022 ; Hassan et al. 2022 ; Jiang and Pang, 2023 ; Kuo et al. 2021 ; Vendraminelli et al. 2022 ; Zheng et al. 2023 ). Molenaar ( 2022 ) notes an ongoing fusion between humans and AI, resulting in the emergence of hybrid systems.
AI technologies have the potential to personalize learning experiences, automate administrative tasks, reduce workloads, offer instant feedback, tailor courses to individual progress, enhance student engagement, and optimize decision-making (Al-Zahrani, 2024a ; Chu et al. 2022 ; Dai and Ke, 2022 ; McKinsey and Company, 2022 ; UNESCO, 2021 ; Zheng et al. 2023 ). While the adoption of AI-powered tools in higher education initially progressed slowly, educators anticipate a future increase in their usage (McKinsey and Company, 2022 ).
In the realm of higher education, AI holds promise for addressing significant challenges and driving innovation in teaching and learning practices (Al-Zahrani, 2023 ; Al-Zahrani, 2024a ; Chu et al. 2022 ; Dai and Ke, 2022 ; McKinsey and Company, 2022 ; UNESCO, 2021 ). Educational settings, increasingly embracing AI technologies, include intelligent tutoring systems, adaptive learning platforms, chatbots, automated grading systems, and data analytics tools (Hassan et al. 2022 ; Jiang and Pang, 2023 ; Kuo et al. 2021 ; Vendraminelli et al. 2022 ; Zhao et al. 2022 ). To unlock these potential benefits, stakeholders in higher education and beyond must possess fundamental AI literacy to interact effectively with AI technology (Laupichler et al. 2022 ).
However, alongside these benefits, AI in higher education introduces several challenges and concerns, such as data privacy and security (Al-Zahrani, 2024b ; Carmody et al. 2021 ; Elliott and Soifer, 2022 ), algorithmic bias and discrimination (Bigman et al. 2022 ; Johnson, 2021 ; Kordzadeh and Ghasemaghaei, 2021 ; Wang et al. 2022 ), and various ethical considerations (Farisco et al. 2020 ; Kerr et al. 2020 ; Owe and Baum, 2021 ; Resseguier and Rodrigues, 2020 ; Ryan and Stahl, 2020 ; Stahl et al. 2021 ).
The research on AI in higher education is a dynamic and ever-evolving field. As AI gains prominence, it becomes essential to explore its impact on the educational, ethical, and social dynamics of the sector (e.g., Al-Zahrani, 2024b ; Chu et al. 2022 ; Dai and Ke, 2022 ; Zawacki-Richter et al. 2019 ). A significant knowledge gap exists regarding how various stakeholders in higher education, including students, faculty, and administrators, perceive the use of AI and envision its future role in the sector. Therefore, this study aims to thoroughly examine and understand the impact of AI on higher education, focusing on the following key aspects:
Attitudes and perceptions of students, faculty, and administrators: Investigating their attitudes, beliefs, and perspectives towards AI in higher education, exploring their concerns and expectations.
Impact on teaching and learning: Exploring how AI influences teaching and learning processes, assessing potential benefits and drawbacks in enhancing instructional approaches, personalizing learning experiences, and improving academic outcomes.
Ethical and social implications: Examining ethical considerations and social implications arising from AI implementation, including data privacy, algorithmic bias, fairness, transparency, and accountability.
Envisioned role of AI: Gaining insights into stakeholders’ visions for the future role of AI in higher education, exploring their expectations, aspirations, and concerns regarding integration and expansion.
By addressing these objectives, the study contributes to the understanding of AI’s impact on higher education and sheds light on the ethical, social, and educational dynamics that emerge. The research questions provide a framework to explore various aspects of AI in higher education, aligning with the study’s aim and scope:
RQ1: What are participants’ attitudes and perceptions towards the implementation of AI in higher education?
RQ2: What is the role of AI in teaching and learning in higher education?
RQ3: What ethical and social implications arise from the implementation of AI in higher education?
RQ4: How do participants envision the future role of AI in higher education?
RQ5: How do participants’ demographic characteristics impact their perspectives in terms of the ethical, social, and educational dynamics associated with AI implementation?
The remainder of this manuscript is organized as follows: Literature review presents a comprehensive review of the existing literature, covering key research areas related to AI in higher education, including pedagogical innovations, learning analytics and student support, assessment and grading, educators’ professional development, and ethical and social implications. Significance and novelty of the research describes the methodological approach employed in this study, detailing the research design, data collection procedures, and analytical methods. Methodology sheds light on the significance and novelty of this study, highlighting its holistic and comprehensive investigation of the multifaceted impact of AI on higher education. Results presents the study’s findings, organized according to the research questions, offering insights into participants’ attitudes and perceptions, the role of AI in teaching and learning, ethical and social implications, and envisioned future roles. Discussion provides a detailed discussion of the findings, situating them within the broader context of AI in higher education and drawing connections to existing literature. The final two sections (Conclusions and implications & Limitations and future research) conclude the manuscript by highlighting the study’s contributions, implications, limitations, and recommendations for future research.
Literature review
Research examining the impact of AI on higher education has witnessed substantial growth in recent years, as highlighted by notable studies (Al-Zahrani, 2023 ; Al-Zahrani, 2024a ; Bozkurt et al. 2021 ; Chu et al. 2022 ; Dai and Ke, 2022 ; Laupichler et al. 2022 ; Zawacki-Richter et al. 2019 ). Scholars from diverse fields, including education, computer science, psychology, and ethics, have explored various facets of AI implementation in higher education settings. Chu et al. ( 2022 ) scrutinized the top 50 AI studies in higher education from the Web of Science (WoS) database. Their analysis revealed a prevalent focus on predicting learners’ learning status, particularly dropout and retention rates, student models, and academic achievement. However, there is a noticeable lack of emphasis on higher-order thinking skills, collaboration, communication, self-efficacy, and AI skills in higher education studies (Chu et al. 2022 ). Laupichler et al. ( 2022 ) stress that research on AI in higher education is still in its early stages, necessitating refinement in defining AI literacy and determining appropriate content for non-experts to enhance their understanding of AI. This literature review provides an overview of key research areas and offers insights into existing knowledge.
Pedagogical innovations
One pivotal research domain explores the pedagogical implications of AI in higher education, recognizing its potential to revolutionize the educational process and enhance efficiency (Al-Zahrani, 2023 ; Al-Zahrani, 2024a ; Kuleto et al. 2021 ; Zheng et al. 2023 ). AI integration in transnational higher education, including distance and online education, holds the promise of improving efficiencies and transforming management, administration, student recruitment, and pedagogical processes, leading to enhanced sustainability and development (El-Ansari, 2021 ). Huang ( 2018 ) emphasizes AI’s role in innovating education, noting its ability to transform learning interactions from machine-focused to knowledge-centered approaches based on learner needs.
Numerous studies delve into how AI-powered technologies, such as intelligent tutoring systems and adaptive learning platforms, enhance personalized learning experiences, promote student engagement, and improve academic outcomes (Al-Zahrani, 2023 ; Al-Zahrani, 2024a ; Chu et al. 2022 ; Dai and Ke, 2022 ). Kuleto et al.‘s ( 2021 ) findings demonstrate the significance of AI in improving learning outcomes, particularly in enhancing students’ skills, promoting collaborative learning, and providing a more accessible research environment. Additionally, Seo et al. ( 2021 ) highlight the potential of incorporating AI systems in online learning to facilitate personalized learner-instructor interactions on a large scale. Kochmar et al. ( 2022 ) present experimental results showing that AI tutoring systems lead to significant overall improvements in student performance outcomes.
Furthermore, AI has the potential to transform higher education by enhancing teaching and learning, improving assessment and feedback, increasing access and retention, reducing costs and time, and supporting administration and management (Abdous, 2023 ; Al-Zahrani, 2024a ; Bates et al. 2020 ; Chu et al. 2022 ; Popenici and Kerr, 2017 ; UNESCO, 2021 ). Almaiah et al.‘s ( 2022 ) study suggests a positive inclination towards integrating AI into educational environments, attributing it to AI being recognized as an innovative teaching tool. Huang ( 2018 ) observes positive effects of AI teaching systems on environmental education for college students.
Moreover, AI can revolutionize social interactions within higher educational settings, impacting learners, teachers, and technological systems (Al-Zahrani, 2023 ; Al-Zahrani, 2024a ; Dai and Ke, 2022 ). Crown et al. ( 2011 ) demonstrate the positive impact of an interactive chatbot on engineering students’ engagement and motivation. Essel et al. ( 2022 ) find that students engaging with a virtual teaching assistant (chatbot) show improved academic performance. Kumar ( 2021 ) observes the positive impacts of chatbots on learning performance and teamwork.
Learning analytics and student support
AI’s potential for innovation in education is prominent in the realm of learning analytics and student support. There has been a shift towards utilizing student data and analytics to enhance the educational experience and improve learning outcomes (André Renz, 2021 ; Huang et al. 2021 ; Zheng et al. 2023 ). AI technologies enable real-time analysis of vast amounts of data not limited to students’ learning but about their emotions as well, offering advantages in identifying at-risk students, recommending personalized interventions, and facilitating timely feedback and assessment (Zhi Liu et al. 2024 ; X. Liu et al. 2023 ). Learning analytics and AI-driven student support systems can provide actionable insights to educators and enhance student success (André Renz, 2021 ; MET, 2022 ; Ouyang et al. 2023 ; Gallagher, 2020 ).
For example, Dong and Hu’s ( 2019 ) study successfully identified contextual factors differentiating high-achieving and low-achieving students in reading literacy using machine learning techniques. Li et al.‘s ( 2022 ) optimized AI-based genetic algorithm grouping method for collaborative groups in higher education outperformed traditional grouping methods. Ouyang et al. ( 2023 ) utilized AI algorithms and learning analytics to analyze groups’ collaboration patterns in online interaction settings.
Assessment and grading
AI’s role in automating assessment and grading processes is another significant area of interest. Scholars investigate the reliability and validity of AI-based grading systems, comparing them to traditional human grading methods, and explore the potential benefits and limitations of automated grading (Lockwood, 2014 ; CTL, 2023 ; CUPA, 2023 ; McNulty, 2023 ; Chen, 2023 ). AI in assessment, including Natural Language Processing (NLP) and plagiarism detection, can automate grading, reduce workload, and enable data-driven decision-making (Lockwood, 2014 ; CTL, 2023 ; CUPA, 2023 ; McNulty, 2023 ; Chen, 2023 ).
While the potential benefits of AI in assessment and grading are significant, it’s also important to consider its practical applications and impact on student outcomes. For instance, Susilawati et al.‘s ( 2022 ) study explored the positive influence of digital assessment and trust on student character and academic performance. Additionally, Hooda et al.‘s ( 2022 ) examination of AI-driven assessment and feedback practices revealed positive impacts on students’ learning outcomes. Learning analytics, in this context, enables higher education institutions to support the learning environment at multiple levels.
Educators’ professional development
AI contributes to pedagogical innovation in the domain of educators’ professional development. Research explores the implications of AI for educators’ professional development, focusing on how AI technologies can support instructors in developing positive perceptions and attitudes, adaptive teaching strategies, and personalized learning experiences (Al-Zahrani, 2023 ; Al-Zahrani, 2024a ; CTL, 2023 ; Chen, 2023 ; Seo et al. 2021 ).
As AI integration in education progresses, it becomes increasingly important to address the concerns and training needs of educators. Educational institutions, policymakers, and AI developers must collaboratively address concerns regarding AI integration and provide the necessary support and training for educators to effectively implement AI technologies in their teaching practices (Al-Zahrani, 2024a ).
One example of how AI can be applied to enhance educators’ professional development is through the use of machine learning to analyze student feedback. Esparza et al.‘s ( 2018 ) ‘SocialMining’ model utilizes machine learning algorithms to enhance teaching techniques based on student comments on teacher performance. Integrating AI into educators’ professional development holds promise for improving instructional practices and the overall quality of education, providing targeted support and personalized learning resources.
Ethical and social implications
The ethical and social dimensions of AI in higher education are critical considerations. AI’s advancement introduces ethical challenges and concerns, necessitating further research to explore the social implications of AI, including accountability in AI-mediated practices and its influence on teaching and learning relationships (Al-Zahrani, 2024b ; Bearman et al. 2022 ). Challenges related to privacy, ethics, and morality in AI-driven approaches require interdisciplinary collaborations for comprehensive research and development (Al-Zahrani, 2024b ; Hu et al. 2023 ; Zhang and Aslan, 2021 ).
Scholars delve into issues of algorithmic bias, discrimination, fairness, transparency, and accountability in AI-driven educational systems (Al-Zahrani, 2024b ; UNESCO, 2021 ; Abdous, 2023 ; Schiff, 2022 ). Ethical considerations in deploying AI technologies, ensuring equity and inclusivity, and balancing human instructors’ roles with AI tools are explored. The societal impact of AI, including changes in employment patterns and the transformation of the workforce, requires careful consideration (Bates et al. 2020 ; Popenici and Kerr, 2017 ; Lo Piano, 2020 ; Seo et al. 2021 ; Chen, 2023 ).
Ethical considerations in integrating AI into everyday environments should be thoroughly addressed (Al-Zahrani, 2024b ; Doncieux et al. 2022 ). This includes examining AI’s impact on human life and societies. Dignum ( 2017 ) emphasizes the importance of upholding societal values, considering moral and ethical implications, and ensuring transparency in AI reasoning processes. In Seo et al.‘s ( 2021 ) study, concerns arise regarding responsibility, agency, surveillance, and potential privacy violations by AI systems. Raising awareness about human-centered values and responsible, ethical AI development is crucial in addressing these concerns (Al-Zahrani, 2024b ; André Renz, 2021 ).
Table 1 summarizes the existing focus areas within each research domain related to AI in higher education and highlights the identified gaps that warrant further investigation. These gaps include aspects such as higher-order thinking skills, collaboration and communication, development of AI skills, large-scale implementation of learning analytics, ethical considerations in assessment and grading, addressing educators’ concerns and training needs, and a comprehensive exploration of ethical concerns and societal impacts.
Significance and novelty of the research
The novelty of this study lies in its holistic and comprehensive investigation of the multifaceted impact of AI on higher education in Saudi Arabia. It makes a unique contribution by capturing the perspectives of diverse stakeholders, including students, faculty, and administrators, through a robust quantitative approach with a large sample size ( N = 1113).
While previous research has explored specific aspects of AI in education, this study offers a comprehensive examination of stakeholders’ attitudes, perceptions, and expectations regarding AI implementation. Notably, it delves into the influence of AI on teaching and learning processes. Additionally, it explores the ethical and social implications arising from AI integration—an area that warrants further exploration.
Furthermore, the study sheds light on stakeholders’ visions for the future role of AI in higher education, providing valuable insights into their aspirations and concerns. By addressing these objectives within the Saudi Arabian context, the study contributes to the growing body of knowledge on AI in higher education and i.
Methodology
In this study, a quantitative approach was employed using a survey questionnaire to comprehensively explore the multifaceted impact of AI on higher education.
Research design
To gather insights into the attitudes, perceptions, and experiences of students and faculty members regarding AI in higher education, an online survey questionnaire was meticulously developed. The questionnaire comprised two main sections. The first section delved into participant demographics, encompassing age, gender, current occupation, education level, subjective AI expertise, utilized AI tools and services, frequency of usage, and purpose of usage.
The second section of the questionnaire consisted of 32 items designed to explore participants’ perspectives on AI in higher education, encompassing attitudes, perceptions, the role of AI in teaching and learning, ethical and social implications, and the envisioned future role of AI. These items were carefully developed based on an extensive review of the literature and aligned with the study’s research questions and objectives. The item development process involved identifying relevant constructs and themes, input from subject matter experts, and rigorous refinement to ensure clarity and relevance (see Appendix 1 ).
Validity and reliability
To ensure the questionnaire’s validity, three experts in the educational technology field meticulously reviewed it, providing suggestions and endorsing modifications.
The Cronbach’s alpha coefficient, a measure of internal consistency and reliability, was calculated to assess the questionnaire items. For the overall questionnaire, comprising all 32 items in the second section, the responses from the entire sample ( N = 1113) were coded and analyzed using SPSS (v. 22). This software computed the average inter-item correlation among the 32 items and used this to calculate the Cronbach’s alpha coefficient, yielding a robust value of α = 0.96. Furthermore, the Cronbach’s alpha was calculated separately for the sub-scales (attitudes and perceptions, role of AI in teaching and learning, ethical and social implications, and the future role of AI), following the same process of analyzing inter-item correlations within each subset of items. Table 2 shows detailed reliability statistics of the survey and its sub-scales.
The sampling strategy employed in this study aims to secure a representative sample that mirrors the diversity present in higher education institutions across Saudi Arabia. To achieve this objective, both public and private universities are included, thereby ensuring a comprehensive representation of various institutional contexts. The snowball technique, a method where existing participants recruit future participants from among their acquaintances, is employed to systematically identify participants for the current study.
By incorporating individuals from diverse disciplines, programs, and academic levels, our research endeavors to encompass a wide array of perspectives and experiences concerning the influence of AI in higher education. This inclusivity enhances the generalizability of our findings and contributes to a more thorough comprehension of the topic at hand. In total, a total of 1,113 participants were involved in the study.
Demographics
Table 3 presents an overview of the demographic characteristics of our study participants.
A significant majority (77.9%) falls within the age bracket of 24 years or younger, with comparatively smaller proportions in older age groups (Fig. 1 ).
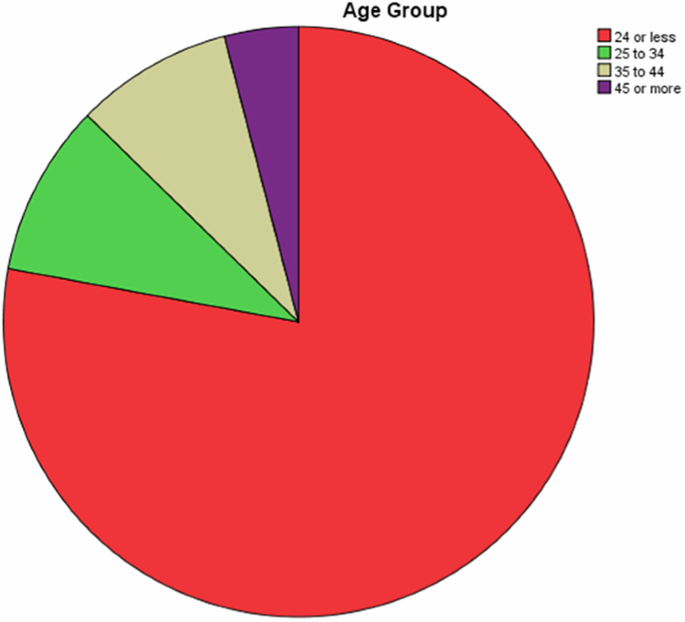
Sample Distribution based on Age.
Gender distribution reveals that females constitute a slightly higher percentage (55.3%) than males (44.7%). See Fig. 2 .

Sample Distribution based on Gender.
Examining the current occupations of participants, the predominant group (83.6%) identifies as students, followed by faculty members (10.1%) and administrators (6.3%) as shown in Fig. 3 .
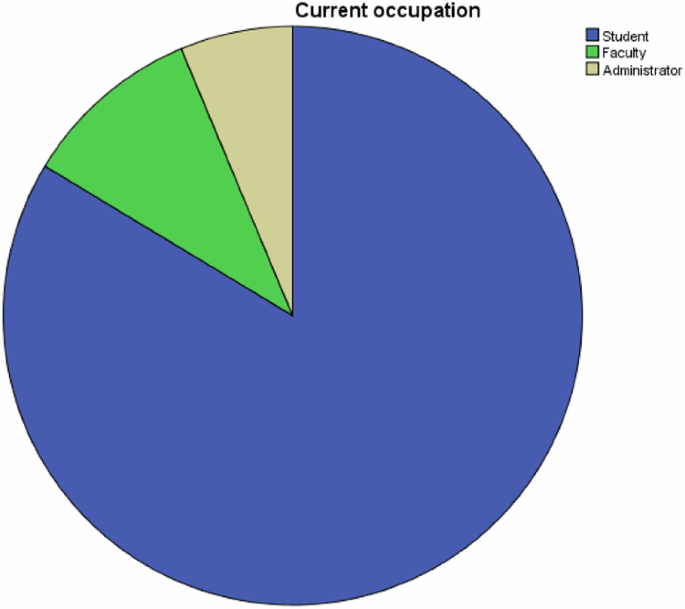
Sample Distribution based on Occupation.
Educational attainment varies (Fig. 4 ), with the majority (85.0%) holding a Bachelor’s degree, while a smaller percentage possess a Master’s degree (6.2%) or a PhD (8.8%).
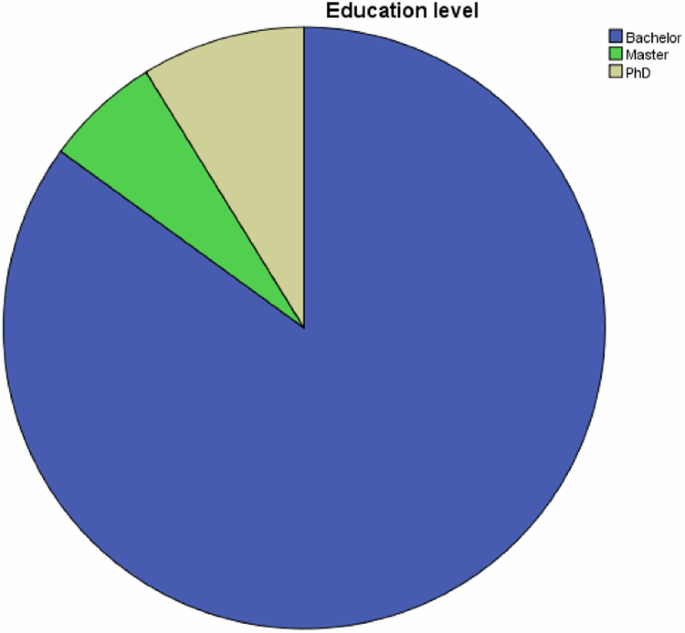
Sample Distribution based on Education Level.
Participants’ academic pursuits are diverse, with the most prevalent fields of study being Medicine, Engineering, or Computer Science (63.8%), followed by Literary, Humanities, or Education (21.7%), and Business, Commerce, or Law (14.5%). See Fig. 5 .
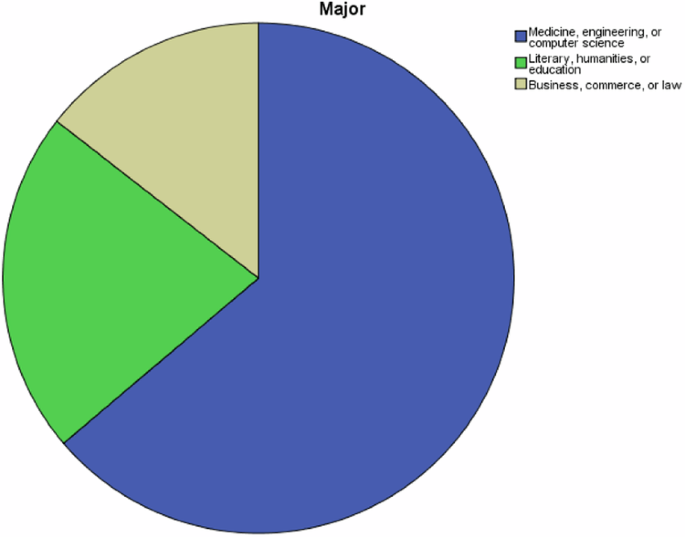
Sample Distribution based on Major.
In terms of self-perceived AI expertise, a significant portion (46.5%) rates their proficiency as low, while a slightly smaller percentage (43.9%) considers it to be medium, and a smaller fraction (9.6%) deems their AI expertise as high. See Fig. 6 .
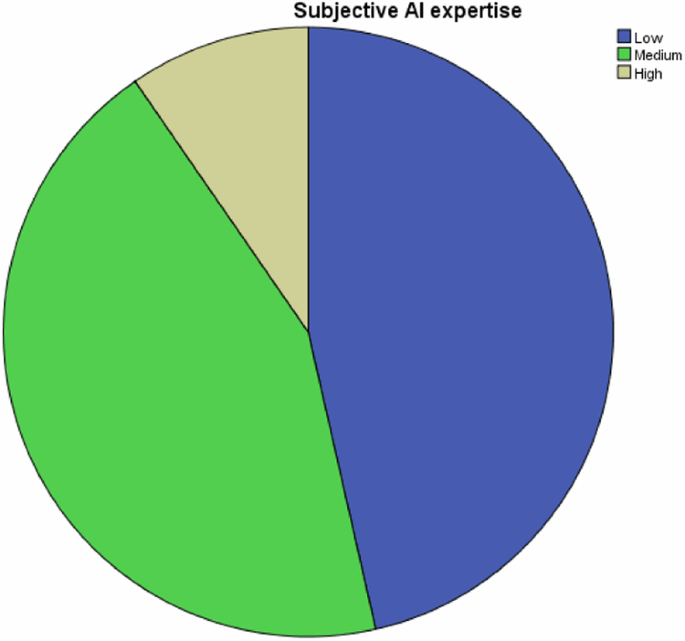
Sample Distribution based on Subjective AI Expertise.
Lastly, examining usage frequency, a noteworthy segment of participants (32.8%) engages with AI on a daily basis, while others utilize it on a weekly (21.2%), monthly (16.4%), or infrequent basis (29.6%). See Fig. 7 .
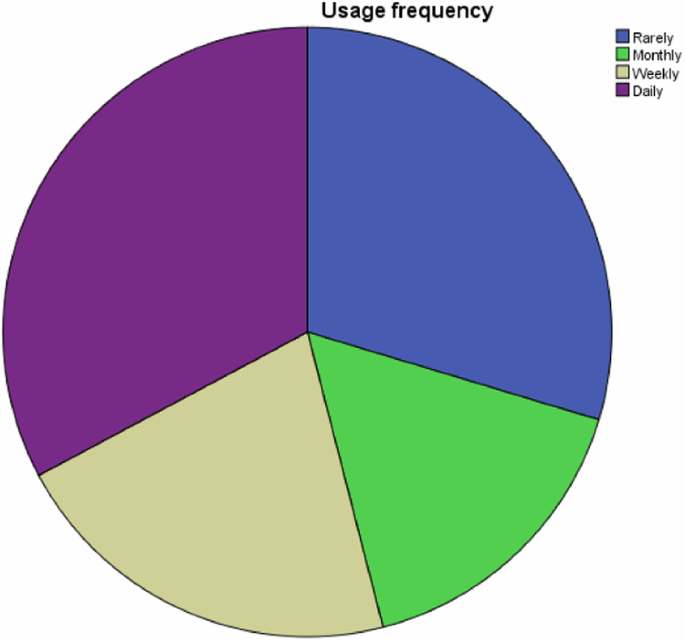
Sample Distribution based on Usage Frequency.
Table 4 presents a comprehensive overview of participant evaluations concerning AI tools, their purposes, and encountered negative experiences. When it comes to AI tools and services, face recognition services garnered the highest mean score of 4.32, signifying a positive evaluation among participants. Speech recognition services also received favorable ratings, boasting a mean score of 3.92. AI-Chatting tools obtained a commendable mean score of 3.85, reflecting a positive perception. On the other hand, AI-powered design, and creativity tools, along with Google AI services, received slightly lower mean scores of 3.60 each.
Examining the purposes of usage, general purposes received the highest mean score of 4.38, indicating strong positive evaluations. Educational purposes were also highly rated, achieving a mean score of 4.35. Research purposes garnered a positive evaluation with a mean score of 4.18, while entertainment purposes scored slightly lower at 3.96. e-Government and commercial purposes obtained lower mean scores of 3.49 and 3.34, respectively.
Delving into negative experiences, privacy and security concerns received a mean score of 3.57, indicating a moderate level of concern among participants. Technical issues during usage and installation both scored mean scores of 3.35, reflecting moderate challenges. Financial costs were rated with a mean score of 3.27, indicating a moderate level of cost-related concerns. Additionally, participants reported usage difficulties, which received a mean score of 3.02, suggesting a moderate level of difficulty encountered.
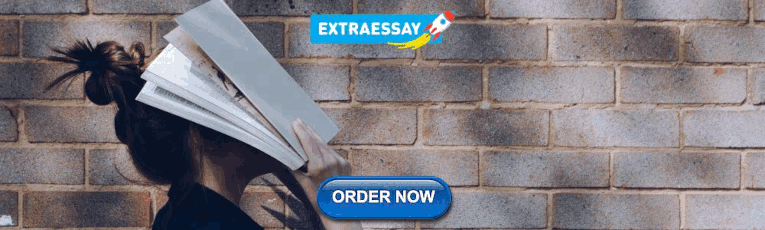
Attitudes and perceptions towards the use of AI in higher education (RQ1)
Table 5 reveals positive attitudes and perceptions towards the use of AI in higher education. Participants recognized AI’s potential to enhance the learning experience, improve access to resources, optimize administrative processes, and revolutionize higher education institutions. The mean scores ranged from 4.43 to 4.19, indicating strong agreement with these statements. These findings demonstrate participants’ openness and optimism towards integrating AI technologies in higher education.
Role of AI in Teaching and Learning in Higher Education (RQ2)
Table 6 highlights participants’ positive perceptions regarding the Role of AI in teaching and learning in higher education. They acknowledged AI’s potential to improve accessibility, facilitate personalized learning experiences, automate administrative tasks, create adaptive learning environments, provide real-time insights into student performance, enhance engagement and participation, influence teaching methods, and support the development of critical thinking and problem-solving skills. The mean scores ranged from 4.31 to 4.10, indicating strong agreement with these statements. Overall, participants recognized the positive effects of AI in higher education settings.
Ethical and social implications of AI in higher education (RQ3)
Table 7 reveals participants’ strong agreement regarding the ethical and social implications of using AI in higher education. They emphasized the need for ethical guidelines, respect for student autonomy, avoidance of societal inequalities, preservation of human interaction, addressing biases, prioritizing data ethics, ensuring transparency and accountability, and addressing privacy and security concerns. The mean scores ranged from 4.47 to 4.24, indicating a high level of agreement. Overall, participants recognized the importance of considering ethical and social aspects when integrating AI in higher education.
The future role of AI in higher education (RQ4)
Table 8 indicates participants’ positive expectations regarding the future role of AI in higher education. They recognized AI’s potential to contribute to the development of intelligent tutoring systems, prioritize ethical considerations and human values, transform teaching and learning, foster collaboration and interdisciplinary research, develop personalized learning pathways, enhance assessment processes, address individual learning needs, and support the development of lifelong learning skills. The mean scores ranged from 4.36 to 4.24, indicating a high level of agreement. Overall, participants expressed optimism about the future integration of AI in higher education.
The Impact of Demographic Characteristics on the Participants’ Perspectives (RQ5)
Multivariate Analysis of Variance tests (MANOVA) were conducted on various factors indicate their significant effects on the variables under consideration. Table 9 provides information on the effect, F-value, Wilks’ Lambda, significance level (p-value), and partial eta squared (ηp²).
The Multivariate test yielded significant results for the variables total uses, total purposes, and total difficulties. For total uses, the test showed a significant effect (Wilks’ Lambda = 0.492, F = 2.845, p < 0.001, ηp² = 0.163), indicating that the independent variable(s) have a statistically significant impact on the uses of AI. Similarly, for total purposes, the test revealed a significant effect (Wilks’ Lambda = 0.547, F = 2.272, p < 0.001, ηp² = 0.140), suggesting that the independent variable(s) significantly influence the purposes of AI. In the case of total difficulties, the test demonstrated a significant effect (Wilks’ Lambda = 0.614, F = 1.898, p < 0.001, ηp² = 0.115), indicating that the independent variable(s) have a statistically significant impact on the difficulties associated with AI. These findings highlight the importance of the independent variable(s) in shaping attitudes, goals, and challenges related to AI use.
Table 10 presents the results of the tests of between-subjects effects for the variables total uses, total purposes, and total difficulties.
These tests examine the significance of the independent variables on the dependent variables as follows:
Uses have a significant impact (F = 4.570, p = 0.000, ηp² = 0.237) on attitudes and perceptions.
Uses have a significant impact (F = 4.803, p = 0.000, ηp² = 0.246) on role of AI in teaching and learning.
Uses show a significant impact (F = 2.035, p = 0.006, ηp² = 0.121) on ethical and social implications.
Uses have a significant impact (F = 3.713, p = 0.000, ηp² = 0.201) on future role of AI.
For purposes:
Purposes have a significant impact (F = 4.870, p = 0.000, ηp² = 0.257) on attitudes and perceptions.
Purposes have a significant impact (F = 5.177, p = 0.000, ηp² = 0.269) on role of AI in teaching and learning.
Purposes show a significant impact (F = 2.331, p = 0.001, ηp² = 0.142) on ethical and social implications.
Purposes have a significant impact (F = 4.086, p = 0.000, ηp² = 0.225) on future role of AI.
For difficulties:
Difficulties have a significant impact (F = 2.298, p = 0.002, ηp² = 0.135) on attitudes and perceptions.
Difficulties have a significant impact (F = 1.980, p = 0.008, ηp² = 0.118) on role of AI in teaching and learning.
Difficulties have a significant impact (F = 1.676, p = 0.036, ηp² = 0.102) on future role of AI.
Figure 8 provides a visual representation of these relationships, illustrating how the uses, purposes, and difficulties associated with AI correlate with attitudes, perceptions, and views on the role and future of AI in higher education.
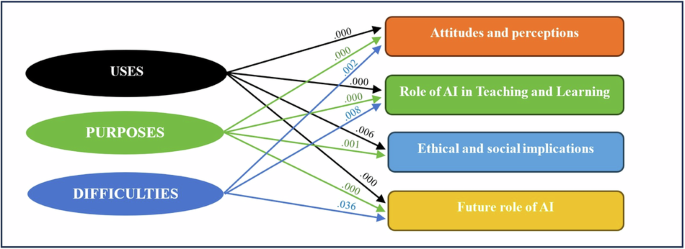
Summary of Relationships and p values.
This study delves into the repercussions of AI on higher education, scrutinizing its ethical, social, and educational ramifications. It investigates the viewpoints and attitudes of students, faculty, and administrators towards the implementation of AI, concentrating on AI’s role in teaching and learning, ethical concerns, and stakeholders’ expectations for its future integration in higher education. The findings contribute valuable insights for stakeholders in higher education, fostering a deeper comprehension of the impact of AI and emphasizing the ethical and educational dimensions of its application.
While a significant portion of participants reported low subjective expertise in AI, a noteworthy percentage indicated daily engagement with AI technologies. This suggests that despite perceiving limited expertise, individuals actively involve themselves with AI in their daily lives (Doncieux et al. 2022 ; Hassan et al. 2022 ; Jiang and Pang, 2023 ; Kuo et al. 2021 ; Vendraminelli et al. 2022 ). The increasing popularity of AI technologies among higher education stakeholders is a promising trend.
Results further unveiled positive evaluations of AI tools and services by participants. Face recognition services received the highest rating, followed by speech recognition services and AI-chatting tools like chatbots. This indicates a readiness within higher education to embrace these technologies, building on successful prior research findings, particularly regarding chatbot implementation (Crown et al. 2011 ; Dai and Ke, 2022 ; Essel et al. 2022 ; Kumar, 2021 ). Conversely, AI-powered design and creativity tools, along with Google AI services, received slightly lower ratings, likely due to the specialized knowledge and expertise required for operation. Participants acknowledge the value of AI for tasks like facial recognition, speech processing, and chatbots, yet there appears to be room for improvement in training and support for advanced AI-powered technologies.
Moreover, participants highly prioritize and value AI for general, educational, research, and entertainment purposes. However, there is slightly lower enthusiasm for AI in e-Government and commercial applications. This might stem from concerns about the perceived lack of impact, or ethical implications and potential risks associated with AI in these contexts, as established in prior research (Al-Zahrani, 2023 ; Al-Zahrani, 2024a ; Farisco et al. 2020 ; Kerr et al. 2020 ; Owe and Baum, 2021 ; Resseguier and Rodrigues, 2020 ; Ryan and Stahl, 2020 ; Stahl et al. 2021 ).
The study also delves into negative experiences associated with AI usage, revealing participants’ moderate concerns about privacy and security issues. This indicates an awareness of potential risks and implications, especially related to data privacy and security breaches. Robust privacy measures in AI applications are emphasized, aligning with previous research findings (André Renz, 2021 ; Crawford et al. 2023 ; Doncieux et al. 2022 ; Dignum, 2017 ; Seo et al. 2021 ; Zhang and Aslan, 2021 ). Additionally, challenges such as technical issues during usage, installation difficulties, usage problems, and financial costs were moderately reported. These results underscore the need for active administration, improved user interfaces, cost reduction, comprehensive training, and support to enhance the overall user experience of AI technologies.
Participants in the study exhibit positive attitudes and perceptions towards the use of AI in higher education. They recognize AI’s potential to enhance the learning experience, personalize instruction, improve student outcomes, and optimize administrative processes. Improved access to educational resources is also identified as a benefit of AI, enabling students to utilize digital libraries, online databases, and academic materials. This aligns with the broader discourse on technology’s transformative impact in education (Al-Zahrani, 2023 ; Al-Zahrani, 2024a ; Chu et al. 2022 ; Dai and Ke, 2022 ; El-Ansari, 2021 ; Kuleto et al. 2021 ; Kochmar et al. 2022 ; Huang, 2018 ; Seo et al. 2021 ; Z. Liu et al. 2023 ).
Furthermore, participants express positive perceptions regarding the role of AI in teaching and learning in higher education. They recognize its potential to improve accessibility, personalize learning experiences, automate administrative tasks, create adaptive learning environments, provide real-time insights into student performance, enhance engagement and participation, influence teaching methods, and support the development of critical thinking and problem-solving skills. These findings highlight the benefits and opportunities that AI brings to the educational process, including equal access to education, tailored instruction, efficient administrative operations, dynamic learning environments, data-driven decision-making, increased student engagement, and the cultivation of higher-order cognitive abilities.
Moreover, participants emphasize the importance of ethical and social implications of AI in higher education, underscoring the need for ethical guidelines to govern AI implementation. This includes respect for student autonomy, avoidance of societal inequalities, preservation of human interaction, addressing biases in AI systems, data ethics, transparency, accountability, and addressing privacy and security concerns. These results align with previous research (Al-Zahrani, 2024b ; André Renz, 2021 ; Crawford et al. 2023 ; Doncieux et al. 2022 ; Dignum, 2017 ; Seo et al. 2021 ; Zhang and Aslan, 2021 ).
Participants’ optimism regarding the future role of AI in higher education underscores its significant impact on various aspects of teaching, learning, and educational processes. This includes the development of intelligent tutoring systems for personalized and adaptive learning experiences, prioritizing ethical considerations and human values, transforming teaching and learning processes, fostering collaboration and interdisciplinary research, developing personalized learning pathways, enhancing assessment processes, addressing specific learning needs, and supporting the development of lifelong learning skills. These findings affirm the positive perceptions, attitudes, and the required ethical considerations identified in the current study, indicating the readiness of higher education to adopt recent advancements in AI technologies and their implications for educational contexts.
MANOVA tests emphasize the significance of total AI uses and total purposes in understanding attitudes, perceptions, and future implications of AI integration in teaching and learning. The results indicate that the extent and purposes of AI usage have notable impacts on various aspects of AI integration, influencing attitudes, perceptions, and perceived future implications. These findings underscore the importance of practical AI implementation in educational settings and its potential to shape individuals’ perceptions of AI’s role.
Furthermore, the significant effects of total purposes highlight the critical role of intentionality in AI implementation. The purposes for which AI is used in teaching and learning substantially impact attitudes, perceptions, and future implications. This underscores the need for clear goals and careful consideration of the ethical and social implications associated with AI integration.
Lastly, it is noteworthy that total difficulties had a significant influence on attitudes, perceptions, and the future role of AI, although to a lesser extent compared to total uses and total purposes. The difficulties encountered in AI usage are associated with individuals’ attitudes and perceptions towards AI, as well as their perspectives on its future role. These findings imply that addressing challenges and providing adequate support in AI implementation can contribute to more positive attitudes and perceptions, leading to effective integration of AI in higher education.
To sum up, these results illuminate the intricate interplay between AI usage, purposes, difficulties, and their impact on attitudes, perceptions, and future implications. They underscore the necessity for a comprehensive understanding of AI integration, considering not only its technical aspects but also the ethical, social, and educational dimensions. By acknowledging the role of AI uses, AI usage purposes, and addressing associated difficulties, educational stakeholders can work towards harnessing the benefits of AI while ensuring responsible and effective implementation in teaching and learning contexts.
Conclusions and implications
This study sheds light on the favorable attitudes and perceptions of stakeholders in higher education towards the adoption of AI. Participants not only acknowledged the value of AI in enriching teaching and learning experiences but also in improving resource accessibility, streamlining administrative processes, and fostering innovation within higher education institutions. Their enthusiasm extended particularly to AI tools and services, such as facial recognition, speech processing, and chatbots. However, they also recognized the imperative for advancements in more sophisticated AI technologies.
Ethical considerations took precedence among participants, underscoring the need for guidelines governing AI implementation. Privacy, security, and bias were identified as critical issues requiring attention. Despite these concerns, there was a prevailing optimism regarding AI’s future role in higher education, with participants envisioning personalized learning experiences, ethical AI integration, collaborative endeavors, and ongoing support for lifelong learning.
The study underscores how the utilization, purposes, and challenges of AI influence attitudes, perceptions, and future implications. Exposure and experience with AI emerged as key determinants shaping individuals’ perspectives. Aligning AI goals with educational objectives and effectively addressing associated challenges were identified as crucial factors in fostering positive attitudes and perceptions. These findings underscore the necessity for comprehensive implementation strategies, encompassing technical, ethical, social, and educational considerations.
In summary, this research offers insights for stakeholders in higher education, aiding their decision-making processes concerning the ethical and educational ramifications of AI. The study’s findings yield several implications for policy and practice in higher education:
Develop and implement ethical guidelines for AI integration.
Invest in professional development and training programs for faculty, administrators, and students.
Provide resources and infrastructure to support AI implementation.
Encourage collaboration and interdisciplinary research on AI in education.
Address data ethics and privacy concerns in AI implementation.
Establish evaluation frameworks to measure the impact of AI.
Foster collaborations with industry partners for AI development.
Continuously monitor and adapt AI implementations in response to challenges.
Limitations and future research
While this study offers valuable insights into AI’s impact on higher education, it is crucial to acknowledge its limitations. First, the study depends on participants’ self-reported data, which can be biased and prone to inaccuracies. Their responses may not always align with their actual behaviors or attitudes towards AI. Second, the study may not fully incorporate contextual factors that could influence participants’ attitudes and perceptions regarding AI in higher education. Factors like cultural, institutional, or regional differences could impact the findings. Finally, the study concentrates solely on AI’s impact on higher education and may not encompass the broader societal implications or perspectives from other sectors.
Future research can address the study’s limitations by conducting cross-cultural and comparative studies, conducting interdisciplinary research on AI in education, and performing comparative analysis with other educational contexts. This will enhance understanding of the influence of contextual factors, uncover broader societal implications, and identify unique considerations specific to higher education.
Data availability
Available as supplementary material.
Abdous M (2023) How AI Is Shaping the Future of Higher Ed. Inside Higher Ed | Higher Education News, Events and Jobs. https://www.insidehighered.com/views/2023/03/22/how-ai-shaping-future-higher-ed-opinion
Almaiah MA, Alfaisal R, Salloum SA, Hajjej F, Thabit S, El-Qirem FA, Al-Maroof RS (2022) Examining the Impact of Artificial Intelligence and Social and Computer Anxiety in E-Learning Settings: Students’ Perceptions at the University Level. Electronics 11(22):3662, https://www.mdpi.com/2079-9292/11/22/3662
Article Google Scholar
Al-Zahrani AM (2023) The impact of generative AI tools on researchers and research: Implications for academia in higher education. Innovations in Education and Teaching International, 1-15. https://doi.org/10.1080/14703297.2023.2271445
Al-Zahrani AM (2024a) From Traditionalism to Algorithms: Embracing Artificial Intelligence for Effective University Teaching and Learning. Educational Technology at IgMin 2(2):102–0112. https://doi.org/10.61927/igmin151
Article MathSciNet Google Scholar
Al-Zahrani AM (2024b) Unveiling the shadows: Beyond the hype of AI in education. Heliyon 10(9):e30696. https://doi.org/10.1016/j.heliyon.2024.e30696
Article PubMed PubMed Central Google Scholar
André Renz GV (2021) Reinvigorating the Discourse on Human-Centered Artificial Intelligence in Educational Technologies. Technology Innovation Management Review 11(5):5–16. https://doi.org/10.22215/timreview/1438
Bates T, Cobo C, Mariño O, Wheeler S (2020) Can artificial intelligence transform higher education? International Journal of Educational Technology in Higher Education 17(1):42. https://doi.org/10.1186/s41239-020-00218-x
Bearman M, Ryan J, Ajjawi R (2022) Discourses of artificial intelligence in higher education: a critical literature review. Higher Education. https://doi.org/10.1007/s10734-022-00937-2
Bigman YE, Wilson D, Arnestad MN, Waytz A, Gray K (2022) Algorithmic discrimination causes less moral outrage than human discrimination. Journal of Experimental Psychology 152(1):4–27. https://doi.org/10.1037/xge0001250
Article PubMed Google Scholar
Bozkurt A, Karadeniz A, Baneres D, Guerrero-Roldán AE, Rodríguez ME (2021) Artificial Intelligence and Reflections from Educational Landscape: A Review of AI Studies in Half a Century. Sustainability 13(2):800, https://www.mdpi.com/2071-1050/13/2/800
Cambridge University Press & Assessment (CUPA) (2023) The Cambridge approach to generative AI and assessment. https://www.cambridge.org/news-and-insights/news/The-Cambridge-approach-to-generative-AI-and-assessment
Carmody J, Shringarpure S, Van De Venter G (2021) AI and privacy concerns: a smart meter case study. Journal of Information, Communication and Ethics in Society 19(4):492–505. https://doi.org/10.1108/jices-04-2021-0042
Center for Teaching and Learning (CTL) (2023) AI Tools in Teaching and Learning: Guidance on understanding how AI tools can impact teaching and learning. https://teachingcommons.stanford.edu/news/ai-tools-teaching-and-learning
Chen C (2023) AI Will Transform Teaching and Learning. Let’s Get it Right. https://hai.stanford.edu/news/ai-will-transform-teaching-and-learning-lets-get-it-right
Chu HC, Hwang GH, Tu YF, Yang KH (2022) Roles and research trends of artificial intelligence in higher education: A systematic review of the top 50 most-cited articles. Australasian Journal of Educational Technology 38(3):22–42
Google Scholar
Crawford J, Cowling M, Allen K (2023) Leadership is needed for ethical ChatGPT: Character, assessment, and learning using artificial intelligence (AI). Journal of University Teaching & Learning Practice, 20(3). https://doi.org/10.53761/1.20.3.02
Creely E (2022) Conceiving Creativity and Learning in a World of Artificial Intelligence: A Thinking Model. In D Henriksen & P Mishra (Eds.), Creative Provocations: Speculations on the Future of Creativity, Technology & Learning (pp. 35-50). Springer International Publishing. https://doi.org/10.1007/978-3-031-14549-0_3
Crown S, Fuentes A, Jones R, Nambiar R, Crown D (2011) Anne G. Neering: Interactive chatbot to engage and motivate engineering students. 21 , 24-34
Dai C-P, Ke F (2022) Educational applications of artificial intelligence in simulation-based learning: A systematic mapping review. Computers and Education: Artificial Intelligence 3:100087. https://doi.org/10.1016/j.caeai.2022.100087
Dignum V (2017) Responsible Artificial Intelligence: Designing Ai for Human Values. ITU Journal: ICT Discoveries, 1 . https://dspace.daffodilvarsity.edu.bd:8080/handle/123456789/2181
Doncieux S, Chatila R, Straube S, Kirchner F (2022) Human-centered AI and robotics. AI Perspectives 4(1):1. https://doi.org/10.1186/s42467-021-00014-x
Dong X, Hu J (2019) An Exploration of Impact Factors Influencing Students’ Reading Literacy in Singapore with Machine Learning Approaches. International Journal of English Linguistics, 9 (5). https://doi.org/10.5539/ijel.v9n5p52
El-Ansari M (2021) Exploring the Applicability of Artificial Intelligence in Transnational Higher Education. International Journal of Management Cases 23-33(2):20, https://www.circleinternational.co.uk/wp-content/uploads/2021/07/IJMC-23.2.pdf
Elliott D, Soifer E (2022) AI Technologies, Privacy, and Security. Frontiers in Artificial Intelligence, 5 . https://doi.org/10.3389/frai.2022.826737
Esparza GG, Canul-Reich J, Zezzatti A, Margain L, Ponce J (2018) Mining: Students Comments about Teacher Performance Assessment using Machine Learning Algorithms. 9 (3), 26-40. http://cathi.uacj.mx/20.500.11961/5676
Essel HB, Vlachopoulos D, Tachie-Menson A, Johnson EE, Baah PK (2022) The impact of a virtual teaching assistant (chatbot) on students’ learning in Ghanaian higher education. International Journal of Educational Technology in Higher Education 19(1):57. https://doi.org/10.1186/s41239-022-00362-6
Farisco M, Evers K, Salles A (2020) Towards Establishing Criteria for the Ethical Analysis of Artificial Intelligence. Science and Engineering Ethics 26(5):2413–2425. https://doi.org/10.1007/s11948-020-00238-w
Gallagher S (2020) The Pandemic Pushed Universities Online. The Change Was Long Overdue. Harvard Business Review. https://hbr.org/2020/09/the-pandemic-pushed-universities-online-the-change-was-long-overdue
Hassan R, Ali A, Howe CW, Zin AM (2022) Constructive alignment by implementing design thinking approach in artificial intelligence course: Learners’ experience. AIP Conference Proceedings, 2433(1). https://doi.org/10.1063/5.0072986
Hooda M, Rana C, Dahiya O, Rizwan A, Hossain MS (2022) Artificial Intelligence for Assessment and Feedback to Enhance Student Success in Higher Education. Mathematical Problems in Engineering 2022:5215722. https://doi.org/10.1155/2022/5215722
Article CAS Google Scholar
Hu F, Qiu L, Wei S, Zhou H, Bathuure IA, Hu H (2023) The spatiotemporal evolution of global innovation networks and the changing position of China: a social network analysis based on cooperative patents. R and D Management. https://doi.org/10.1111/radm.12662
Huang C, Han Z, Li M, Wang X, Zhao W (2021) Sentiment evolution with interaction levels in blended learning environments: Using learning analytics and epistemic network analysis. Australasian Journal of Educational Technology 37(2):81–95. https://doi.org/10.14742/ajet.6749
Huang S-P (2018) Effects of Using Artificial Intelligence Teaching System for Environmental Education on Environmental Knowledge and Attitude. Eurasia Journal of Mathematics, Science and Technology Education 14(7):3277–3284. https://doi.org/10.29333/ejmste/91248
Jiang C, Pang Y (2023) Enhancing design thinking in engineering students with project-based learning. Computer Applications in Engineering Education, n/a(n/a). https://doi.org/10.1002/cae.22608
Johnson GM (2021) Algorithmic bias: on the implicit biases of social technology. Synthese 198(10):9941–9961. https://doi.org/10.1007/s11229-020-02696-y
Kerr A, Barry M, Kelleher JC (2020) Expectations of artificial intelligence and the performativity of ethics: Implications for communication governance. Big Data & Society 7(1):205395172091593. https://doi.org/10.1177/2053951720915939
Kochmar E, Vu DD, Belfer R, Gupta V, Serban IV, Pineau J (2022) Automated Data-Driven Generation of Personalized Pedagogical Interventions in Intelligent Tutoring Systems. International Journal of Artificial Intelligence in Education 32(2):323–349. https://doi.org/10.1007/s40593-021-00267-x
Kordzadeh N, Ghasemaghaei M (2021) Algorithmic bias: review, synthesis, and future research directions. European Journal of Information Systems 31(3):388–409. https://doi.org/10.1080/0960085x.2021.1927212
Kuleto V, Ilić M, Dumangiu M, Ranković M, Martins OMD, Păun D, Mihoreanu L (2021) Exploring Opportunities and Challenges of Artificial Intelligence and Machine Learning in Higher Education Institutions. Sustainability 13(18):10424, https://www.mdpi.com/2071-1050/13/18/10424
Kumar JA (2021) Educational chatbots for project-based learning: investigating learning outcomes for a team-based design course. International Journal of Educational Technology in Higher Education 18(1):65. https://doi.org/10.1186/s41239-021-00302-w
Kuo J, Song X, Chen C, Patel CD (2021) Fostering Design Thinking in Transdisciplinary Engineering Education. In Advances in transdisciplinary engineering. IOS Press. https://doi.org/10.3233/atde210083
Laupichler MC, Aster A, Schirch J, Raupach T (2022) Artificial intelligence literacy in higher and adult education: A scoping literature review. Computers and Education: Artificial Intelligence 3:100101. https://doi.org/10.1016/j.caeai.2022.100101
Li X, Ouyang F, Chen W (2022) Examining the effect of a genetic algorithm-enabled grouping method on collaborative performances, processes, and perceptions. Journal of Computing in Higher Education 34(3):790–819. https://doi.org/10.1007/s12528-022-09321-6
Liu X, Wang S, Lu S, Yin Z, Li X, Yin L, Tian J, Zheng W (2023) Adapting feature selection algorithms for the classification of Chinese texts. Systems 11(9):483. https://doi.org/10.3390/systems11090483
Liu Z, Kong X, Liu S, Yang Z (2023) Effects of computer-based mind mapping on students’ reflection, cognitive presence, and learning outcomes in an online course. Distance Education 44(3):544–562. https://doi.org/10.1080/01587919.2023.2226615
Liu Z, Wen C, Su Z, Liu S, Sun J, Kong W, Yang Z (2024) Emotion-semantic-aware dual contrastive learning for epistemic emotion identification of learner-generated reviews in MOOCs. IEEE Transactions on Neural Networks and Learning Systems, 1–14. https://doi.org/10.1109/tnnls.2023.3294636
Lo Piano S (2020) Ethical principles in machine learning and artificial intelligence: cases from the field and possible ways forward. Humanities and Social Sciences Communications 7(1):9. https://doi.org/10.1057/s41599-020-0501-9
Lockwood J (2014) Handbook of Automated Essay Evaluation Current Applications and New Directions Mark D. Shermis and Jill Burstein (eds.) (2013) New York: Routledge. pp. 194 ISBN: 9780415810968. Writing & Pedagogy 6(2):437–441. https://doi.org/10.1558/wap.v6i2.437
McKinsey & Company (2022). How technology is shaping learning in higher education. McKinsey & Company. https://www.mckinsey.com/industries/education/our-insights/how-technology-is-shaping-learning-in-higher-education#
McNulty N (2023) Using AI for Auto-Marking of Assessment: Revolutionising the Grading Process. https://www.niallmcnulty.com/2023/05/using-ai-for-auto-marking-of-assessment-revolutionising-the-grading-process/
Microsoft Education Team (MET) (2022) How data and AI are changing the world of education. https://educationblog.microsoft.com/en-us/2022/04/how-data-and-ai-are-changing-the-world-of-education
Molenaar I (2022) The concept of hybrid human-AI regulation: Exemplifying how to support young learners’ self-regulated learning. Computers and Education: Artificial Intelligence 3:100070. https://doi.org/10.1016/j.caeai.2022.100070
Ouyang F, Xu W, Cukurova M (2023) An artificial intelligence-driven learning analytics method to examine the collaborative problem-solving process from the complex adaptive systems perspective. International Journal of Computer-Supported Collaborative Learning 18(1):39–66. https://doi.org/10.1007/s11412-023-09387-z
Owe A, Baum SD (2021) Moral consideration of nonhumans in the ethics of artificial intelligence. AI And Ethics 1(4):517–528. https://doi.org/10.1007/s43681-021-00065-0
Popenici SAD, Kerr S (2017) Exploring the impact of artificial intelligence on teaching and learning in higher education. Research and Practice in Technology Enhanced Learning 12(1):22. https://doi.org/10.1186/s41039-017-0062-8
Resseguier A, Rodrigues R (2020) AI ethics should not remain toothless! A call to bring back the teeth of ethics. Big Data & Society 7(2):205395172094254. https://doi.org/10.1177/2053951720942541
Ryan M, Stahl BC (2020) Artificial intelligence ethics guidelines for developers and users: clarifying their content and normative implications. Journal of Information, Communication and Ethics in Society 19(1):61–86. https://doi.org/10.1108/jices-12-2019-0138
Schiff D (2022) Education for AI, not AI for Education: The Role of Education and Ethics in National AI Policy Strategies. International Journal of Artificial Intelligence in Education 32(3):527–563. https://doi.org/10.1007/s40593-021-00270-2
Seo K, Tang J, Roll I, Fels S, Yoon D (2021) The impact of artificial intelligence on learner–instructor interaction in online learning. International Journal of Educational Technology in Higher Education 18(1):54. https://doi.org/10.1186/s41239-021-00292-9
Stahl BC, Antoniou J, Ryan M, Macnish K, Jiya T (2021) Organisational responses to the ethical issues of artificial intelligence. AI & SOCIETY 37(1):23–37. https://doi.org/10.1007/s00146-021-01148-6
Susilawati E, Lubis H, Kesuma S, Pratama I (2022) Antecedents of Student Character in Higher Education: The role of the Automated Short Essay Scoring (ASES) digital technology-based assessment model. Eurasian Journal of Educational Research 98:203–220. https://doi.org/10.14689/ejer.2022.98.013
UNESCO (2021) Artificial Intelligence and Education. Guidance for Policy-makers. The United Nations Educational, Scientific and Cultural Organization, 1-50. https://doi.org/10.54675/PCSP7350
Vendraminelli L, Macchion L, Nosella A, Vinelli A (2022). Design thinking: strategy for digital transformation. Journal of Business Strategy, ahead-of-print(ahead-of-print). https://doi.org/10.1108/JBS-01-2022-0009
Wang C, Wang K, Bian AA, Islam MR, Keya KN, Foulds JR, Pan S (2022). Do Humans Prefer Debiased AI Algorithms? A Case Study in Career Recommendation. https://doi.org/10.1145/3490099.3511108
Zawacki-Richter O, Marín VI, Bond M, Gouverneur F (2019) Systematic review of research on artificial intelligence applications in higher education – where are the educators? International Journal of Educational Technology in Higher Education 16(1):39. https://doi.org/10.1186/s41239-019-0171-0
Zhang K, Aslan AB (2021) AI technologies for education: Recent research & future directions. Computers and Education: Artificial Intelligence 2:100025. https://doi.org/10.1016/j.caeai.2021.100025
Zhao X, Yang M, Qu Q, Xu R, Li J (2022) Exploring privileged features for relation extraction with contrastive student-teacher learning. IEEE Transactions on Knowledge and Data Engineering, 1–1. https://doi.org/10.1109/tkde.2022.3161584
Zheng W, Lu S, Cai Z, Wang R, Wang L, Yin L (2023) PAL-BERT: An Improved Question Answering Model. Computer Modeling in Engineering & Sciences, 1-17. https://doi.org/10.32604/cmes.2023.046692
Download references
Author information
Authors and affiliations.
University of Jeddah, Jeddah, Saudi Arabia
Abdulrahman M. Al-Zahrani & Talal M. Alasmari
You can also search for this author in PubMed Google Scholar
Contributions
Abdulrahman M. Al-Zahrani and Talal M. Al-Asmari contributed equally to this work.
Corresponding author
Correspondence to Abdulrahman M. Al-Zahrani .
Ethics declarations
Competing interests.
The authors declare no competing interests.
Ethical approval
The questionnaire and methodology for this study was approved by the Learning Design and Technology Department at University of Jeddah (#23-241 on Feb. 07, 2023).
Informed consent
Prior to data collection, informed consent was obtained from all participants. Each individual was provided with information regarding the purpose of the study, their rights as participants (including the right to withdraw at any point), and the measures taken to protect their personal data. Participants gave their consent to participate voluntarily.
Additional information
Publisher’s note Springer Nature remains neutral with regard to jurisdictional claims in published maps and institutional affiliations.
Supplementary information
Appendix 1: survey, research data, rights and permissions.
Open Access This article is licensed under a Creative Commons Attribution 4.0 International License, which permits use, sharing, adaptation, distribution and reproduction in any medium or format, as long as you give appropriate credit to the original author(s) and the source, provide a link to the Creative Commons licence, and indicate if changes were made. The images or other third party material in this article are included in the article’s Creative Commons licence, unless indicated otherwise in a credit line to the material. If material is not included in the article’s Creative Commons licence and your intended use is not permitted by statutory regulation or exceeds the permitted use, you will need to obtain permission directly from the copyright holder. To view a copy of this licence, visit http://creativecommons.org/licenses/by/4.0/ .
Reprints and permissions
About this article
Cite this article.
Al-Zahrani, A.M., Alasmari, T.M. Exploring the impact of artificial intelligence on higher education: The dynamics of ethical, social, and educational implications. Humanit Soc Sci Commun 11 , 912 (2024). https://doi.org/10.1057/s41599-024-03432-4
Download citation
Received : 04 December 2023
Accepted : 03 July 2024
Published : 13 July 2024
DOI : https://doi.org/10.1057/s41599-024-03432-4
Share this article
Anyone you share the following link with will be able to read this content:
Sorry, a shareable link is not currently available for this article.
Provided by the Springer Nature SharedIt content-sharing initiative
Quick links
- Explore articles by subject
- Guide to authors
- Editorial policies

Home News & Diary School Blog
Online Distance Learning: A Literature Review
29 Sep 2020
Uncategorised
This week’s blogpost is a guest post by Dr John L. Taylor , Director of Learning, Teaching and Innovation at Cranleigh School .
Dr Taylor is leading a free CIRL professional development webinar on project-based learning, on 17 November from 4-5pm GMT. The link will be available on CIRL’s Eventbrite page soon and the webinar recording will be added to CIRL’s Resources and Professional Development page .
What does the secondary research literature tell us about distance learning?
This blogpost offers a literature review on online distance learning, which is thematically divided into four sections. I first consider what the literature tells us about the efficacy of online distance learning (section 1) and the importance of building a learning community (section 2). I then discuss what the literature says in response to two questions: ‘Does online distance learning work better for some students?’ (section 3) and ‘Can online distance learning support the development of self-regulated learning?’ (section 4).
In this review, the following key terms are defined as follows:
- Distance learning: a ‘form of education in which the main elements include physical separation of teachers and students during instruction and the use of various technologies to facilitate student-teacher and student-student communication.’ [1]
- Online learning: ‘education that takes place over the internet’. [2] This can be subdivided into asynchronous online courses that do not take place in real-time and synchronous online courses in which teacher and student interact online simultaneously. [3]
- Blended learning: a hybrid mode of interaction which combines face-to-face in-person meetings with online interaction. [4] As blended learning is a hybrid model, either the face-to-face or the online elements may be dominant. So, for example, blended learning can occur when online instructional tools are used to support face-to-face learning in a classroom, or when some face-to-face instruction is interspersed with online learning as part of a longer course.
- A virtual school: ‘an entity approved by a state or governing body that offers courses through distance delivery – most commonly using the internet’. [5]
- Self-regulated learning: ‘the modulation of affective, cognitive and behavioural processes throughout a learning experience in order to reach a desired level of achievement’. [6] Self-regulating learning skills have been described as abilities such as planning, managing and controlling the learning process. [7] Processes that occur during self-regulated learning include goal setting, metacognition and self-assessment. [8]
1. The Efficacy of Online Distance Learning
That said, there is also evidence of equivalence across a number of outcome measures. A 2004 meta-analysis by Cathy Cavanaugh et al of 116 effect sizes measured across 14 K-12 web-delivered distance learning programmes between 1999 and 2004 found that there was no significant difference in outcomes between virtual and face-to-face schools. [10]
A 2015 study by Heather Kauffmann explored factors predictive of student success and satisfaction with online learning. [11] Kauffmann notes that several studies have found that online learning programmes lead to outcomes that are comparable to those of face-to-face programmes.
VanPortfliet and Anderson note that research into hybrid instruction indicates that students achieve outcomes that match, if not exceed, outcomes from other instructional modalities. In particular, academic achievement by students in hybrid programmes is consistently higher than that of students engaged in purely online programmes. [12]
The ongoing discussion in the literature suggests that it is difficult to draw general conclusions about the efficacy of online learning as such, not least because it constitutes in significant ways a distinctive mode of learning when compared with real-world instruction. It is perhaps better, then, to look more specifically at questions such as the comparative strengths and challenges of moving to virtual schooling, the conditions which need to be in place for it to function well and the manner in which this transition is experienced by learners with different capabilities.
2. The Importance of Building a Learning Community
A helpful summary of research about online learning by Jonathan Beale at CIRL contains an outline of principles concerning successful online distance learning programmes.The summary explores research-based recommendations for effective teaching and learning practices in online and blended environments made by Judith V. Boettcher and Rita-Marie Conrad in their 2016 work, The Online Teaching Survival Guide: Simple and Practical Pedagogical Tips . [13] A central emphasis of these recommendations is that successful online learning depends upon the formation of an online learning community, and this is only possible if there is regular online interaction between teachers and students:
Why is presence so important in the online environment? When faculty actively interact and engage students in a face-to-face classroom, the class evolves as a group and develops intellectual and personal bonds. The same type of community bonding happens in an online setting if the faculty presence is felt consistently. [14]
The significance of relationship building is noted in the Michigan Virtual Learning Research Institute’s Teacher Guide to Online Learning :
Creating a human-to-human bond with your online students, as well as with their parents/guardians and the student’s local online mentor, is critical in determining student success in your online course. This can be accomplished through effective individual and group communication, encouraging engagement in the course, productive and growth-focused feedback, and multiple opportunities for students to ask questions and learn in a way that is meaningful to them. [15]
Research into virtual learning emphasises the importance of the connection between students and their teachers. This can be lost if there is no ‘live’ contact element at all. As Beale notes, this does not necessarily mean that every lesson needs to include a video meeting, though there is a beneficial psychological impact of knowing that the teacher is still in contact and regular face-to-face online discussions can enable this. There are other forms – a discussion thread which begins during a lesson and is open throughout can perform the same role, though in cases where meeting functions are available, students may be directed to use these rather than email.
As well as the teacher-student relationship, student-student links are important. There is evidence of improved learning when students are asked to share their learning experiences with each other. [16]
Beale’s research summary also emphasizes the importance of a supportive and encouraging online environment. Distance learning is challenging for students and the experience can be frustrating and de-motivating if technology fails (e.g., if work gets lost or a live session cannot be joined due to a connection failure or time-zone difference). More than ever, teachers need to work at providing positive encouragement to their students, praising and rewarding success and acknowledging challenges when they exist. It is also valuable if teachers can identify new skills that students are acquiring – not least skills in problem-solving, using information technology and resilience – and encourage their classes when they see evidence of these.
3. Does online distance learning work better for some students?
Given that, more or less by definition, students participating in an online distance learning programme will be operating with a greater degree of autonomy, it may be expected that those who will be best suited to online learning will be those with the greatest propensity for self-regulated learning. This view is advanced in a review of the literature on virtual schools up until 2009, by Michael Barbour and Thomas Reeves:
The benefits associated with virtual schooling are expanding educational access, providing high-quality learning opportunities, improving student outcomes and skills, allowing for educational choice, and achieving administrative efficiency. However, the research to support these conjectures is limited at best. The challenges associated with virtual schooling include the conclusion that the only students typically successful in online learning environments are those who have independent orientations towards learning, highly motivated by intrinsic sources, and have strong time management, literacy, and technology skills. These characteristics are typically associated with adult learners. This stems from the fact that research into and practice of distance education has typically been targeted to adult learners. [17]
Given the lack of evidence noted by Barbour and Reeves, a more cautious conclusion would be that we may expect to find a relationship between outcomes from online distance learning programmes and the propensity of students for self-regulated learning, rather than the conclusion that this capacity is a precondition of success.
Kauffmann notes that students with the capacity for self-regulated learning tend to achieve better outcomes from online courses. This result is not surprising, given that in online learning more responsibility is placed on the learner. [18]
A 2019 review of 35 studies into online learning by Jacqueline Wong et al explores the connection between online learning and self-regulated learning. The study highlights the significance of supports for self-regulated learning such as the use of prompts or feedback in promoting the development and deployment of strategies for self-regulated learning, leading to better achievement in online learning:
In online learning environments where the instructor presence is low, learners have to make the decisions regarding when to study or how to approach the study materials. Therefore, learners’ ability to self-regulate their own learning becomes a crucial factor in their learning success … [S]upporting self-regulated learning strategies can help learners become better at regulating their learning, which in turn could enhance their learning performance. [19]
In a 2005 study of ‘Virtual High School’ (VHS), the oldest provider of distance learning courses to high school students in the United States, Susan Lowes notes that the VHS’s pedagogical approach ‘emphasizes student-centered teaching; collaborative, problem-based learning; small-group work; and authentic performance-based assessment’. [20] This approach, Lowes comments, is aligned with a growing body of literature on the characteristics of successful online courses.
Taking a more student-centred approach during online instruction fits with features of the online environment. It is natural to make more use of asynchronous assignments and to expect students to take more responsibility for their study, given that they are not subject to direct supervision in a classroom setting and may be accessing course materials outside of a conventional timetable.
4. Can online distance learning support the development of self-regulated learning?
It may be the case that, even if Barbour and Reeves are correct in claiming that only those students with an ‘independent orientation towards learning’typically achieve successful outcomes from online distance learning programmes, a countervailing relationship obtains insofar as participation in an online distance learning programme may foster the development of the propensity for self-regulated learning.
A controlled study in 2018 by Ruchan Uz and Adem Uzun of 167 undergraduate students on a programming language course compared blended learning with a traditional learning environment. The study found that, for the purpose of developing self-regulated learning skills, blended instruction was more effective than traditional instruction. [21]
In a 2011 review of 55 empirical studies, Matthew Bernacki, Anita Aguilar and James Byrnes noted that research suggests that:
[T]echnologically enhanced learning environments … represent an opportunity for students to build their ability to self-regulate, and for some, leverage their ability to apply self-regulated learning … to acquire knowledge. [22]
Their review suggests that the use of technologically enhanced learning environments can promote self-regulated learning and that such environments are best used by learners who can self-regulate their learning. [23]
However, an investigation by Peter Serdyukov and Robyn Hill into whether online students do learn independently argues that independent learning requires active promotion as well as a desire to promote autonomy on the part of the instructor and the necessary skills and motivation on the part of students. Where these conditions are not met, the aspiration to autonomy is frustrated, which can lead to negative outcomes from the online learning experience. [24]
Bernacki, Aguilar and Brynes employed an Opportunity-Propensity (O-P) framework. The O-P framework was introduced by Brynes and Miller in a 2007 paper exploring the relative importance of predictors of math and science achievement, where it was described as follows:
This framework assumes that high achievement is a function of three categories of factors: (a) opportunity factors (e.g., coursework), (b) propensity factors (e.g., prerequisite skills, motivation), and (c) distal factors (e.g., SES). [25]
It is plausible to suggest that the two-way relationship between self-regulated learning skills and successful participation in an online distance learning programme can be explained in terms of the opportunities online distance learning offers in three areas: first, to develop self-regulated learning skills afforded by the online distance learning environment; second, the prior propensity of learners to self-regulate their learning; and third, changes in distal factors (such as exclusive mediation of learning through online platforms to IT and parental involvement in learning).
Summary of Secondary Research Literature
The following points can be made about online distance learning based on the foregoing review:
- Successful online learning depends upon the formation of an online learning community. Regular online interaction between teachers and students is important in the development of an online community. Teacher-student and student-student links are part of this.
- Students with the capacity for self-regulated learning tend to achieve better outcomes from online courses.
- There is some evidence that online distance learning programmes can be used to help develop self-regulated learning skills. This is provided that both teacher and student are motivated by the goal of building autonomy .
- There is support in the research literature for using collaborative, problem-based learning and authentic performance-based assessment within online learning programmes.
Coda: review and revise
It is fair to say that the move to an entirely distance learning programme is the single biggest and most rapid change that many educators will ever have had to make. As with any large-scale rapid and fundamental innovation, it is hard to get everything right. We need to be willing to revise and refine. This may mean adapting to use a new software platform across the whole school if problems are found with existing provision, or it may be an adjustment to expectations about lesson length or frequency of feedback. Keeping distance learning programmes under review is also essential as we look towards a possible future in which it will co-exist with face-to-face teaching.
This literature review is an edited version of the literature review in my report, ‘An Investigation of Online Distance Learning at Cranleigh’ , September 2020, which can be downloaded here . In that report, the literature review is used to establish several conclusions about the implementation of online learning programmes. Those findings are compared to trends discernible in the responses to a questionnaire survey of three year groups at Cranleigh School (years 9, 10 and 12). The programme of study for these year groups was designed to provide continuity of delivery of the curriculum, in contrast to the programmes developed for years 11 and 13, where a customised programme of study was developed to bridge the gap created by the withdrawal of national public examinations during the summer term of 2020.
[1] ‘Distance learning | education | Britannica’ .
[2] Joshua Stern, ‘Introduction to Online Teaching and Learning’ .
[3] Fordham University, ‘Types of Online Learning’ .
[5] Michael K. Barbour and Thomas C. Reeves, ‘The reality of virtual schools: A review of the literature’, Computers & Education 52.2 (2009), pp. 402-416.
[6] Maaike A. van Houten‐Schat et al , ‘Self‐regulated learning in the clinical context: a systematic review’, Medical Education 52.10 (2018), pp. 1008-1015.
[7] René F. Kizilcec, Mar Pérez-Sanagustín & Jorge J. Maldonado, ‘Self-regulated learning strategies predict learner behavior and goal attainment in Massive Open Online Courses’, Computers & education 104 (2017), pp. 18-33.
[8] Sofie M. M. Loyens, Joshua Magda and Remy M. J. P. Rikers, ‘Self-directed learning in problem-based learning and its relationships with self-regulated learning’, Educational Psychology Review 20.4 (2008), pp. 411-427.
[9] Paul VanPortfliet and Michael Anderson, ‘Moving from online to hybrid course delivery: Increasing positive student outcomes’, Journal of Research in Innovative Teaching 6.1 (2013), pp. 80-87.
[10] Cathy Cavanaugh et al , ‘The effects of distance education on K-12 student outcomes: A meta-analysis’, Learning Point Associates/North Central Regional Educational Laboratory (NCREL), 2004.
[11] Heather Kauffman, ‘A review of predictive factors of student success in and satisfaction with online learning’, Research in Learning Technology 23 (2015).
[12] VanPortfliet & Anderson, op. cit., pp 82 – 83 .
[13] Judith V. Boettcher & Rita-Marie Conrad, The Online Teaching Survival Guide: Simple and Practical Pedagogical Tips (Second Edition; San Francisco, CA: Jossey-Bass, 2016).
[14] Ibid. Boettcher & Conrad’s chapter is reprinted with permission in this article , from which the quotation is taken.
[15] Michigan Virtual’s ‘Teacher Guide to Online Learning’ .
[16] Joan Van Tassel & Joseph Schmitz, ‘Enhancing learning in the virtual classroom’, Journal of Research in Innovative Teaching 6.1 (2013), pp. 37-53.
[17] Michael K. Barbour & Thomas C. Reeves, ‘The reality of virtual schools: A review of the literature’, Computers & Education 52.2 (2009), pp. 402-416.
[18] Heather Kauffman, ‘A review of predictive factors of student success in and satisfaction with online learning’, Research in Learning Technology 23 (2015).
[19] Jacqueline Wong et al , ‘Supporting self-regulated learning in online learning environments and MOOCs: A systematic review’, International Journal of Human–Computer Interaction 35.4-5 (2019), pp. 356-373.
[20] ‘Online Teaching and Classroom Change – CiteSeerX’ .
[21] Ruchan Uz & Adem Uzun, ‘The Influence of Blended Learning Environment on Self-Regulated and Self-Directed Learning Skills of Learners’, European Journal of Educational Research 7.4 (2018), pp. 877-886.
[22] Matthew L. Bernacki, Anita C. Aguilar & James P. Byrnes, ‘Self-regulated learning and technology-enhanced learning environments: An opportunity-propensity analysis’, Fostering self-regulated learning through ICT , IGI Global (2011), pp. 1-26.
[24] Peter Serdyukov & R. Hill, ‘Flying with clipped wings: Are students independent in online college classes’, Journal of Research in Innovative Teaching 6.1 (2013), pp. 52-65.
[25] James P. Byrnes & David C. Miller, ‘The relative importance of predictors of math and science achievement: An opportunity–propensity analysis’, Contemporary Educational Psychology 32.4 (2007), pp. 599-629.
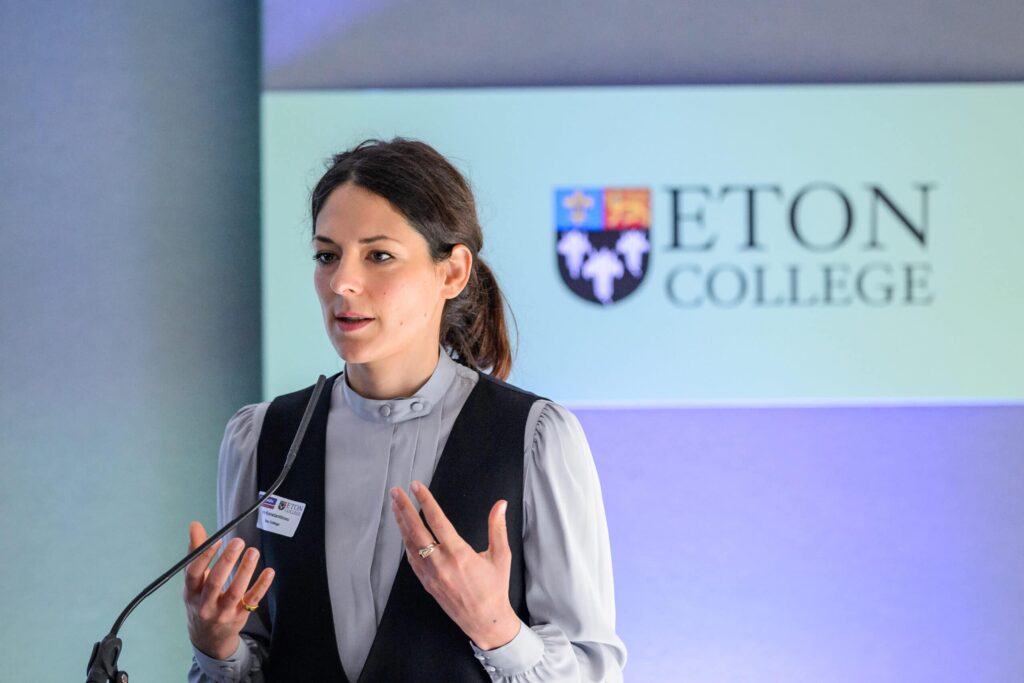
The Teaching and Learning Summit
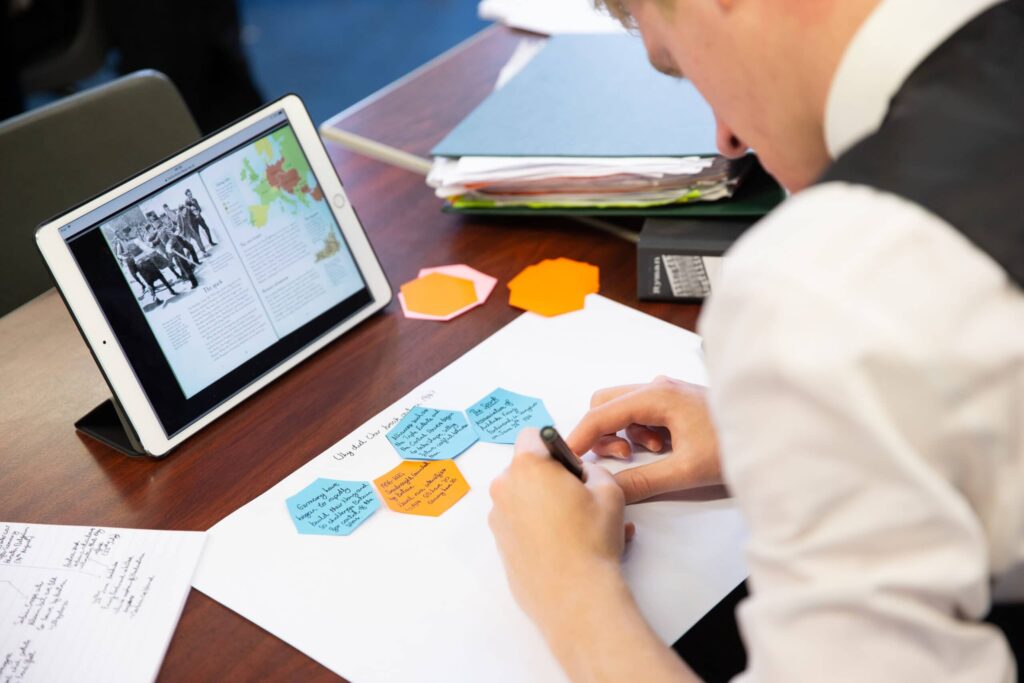
About the Centre
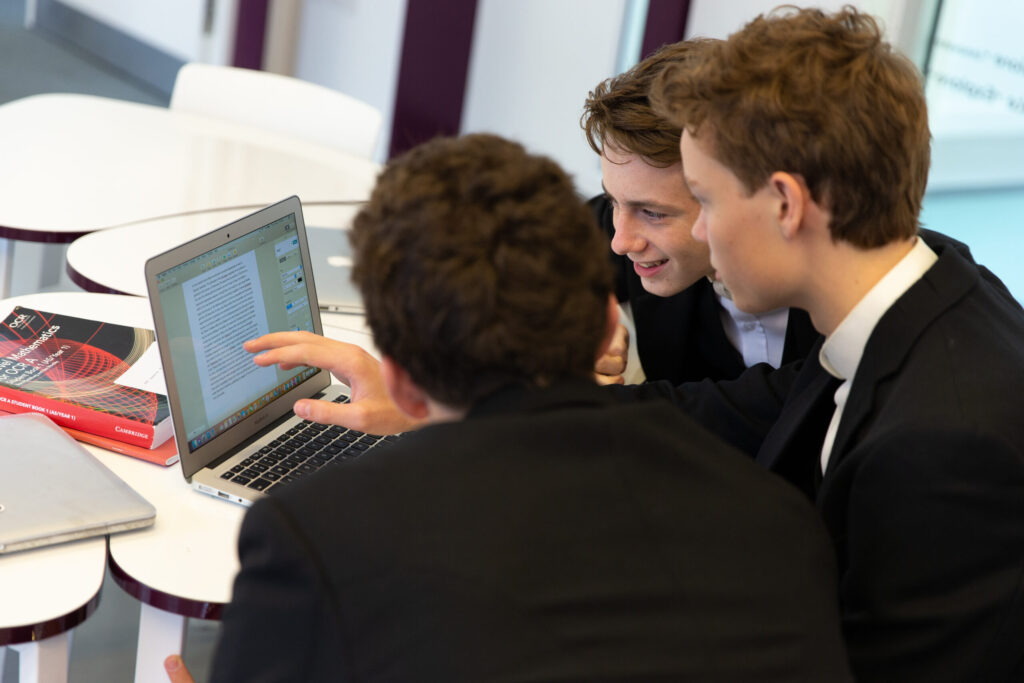
Our Research

Professional Development for Online Teaching: A Literature Review
- Heather Leary
- Chad Turley
- Matthew Cheney
- Zach Simmons
- Charles R. Graham
- Riley Hatch
The growth of online learning has created a need for instructors who can competently teach online. This literature review explores the research questions, program recommendations, and future research suggestions related to professional development for online instructors. Articles were selected and coded based on date of publication and the context of the professional development. Results indicate that most research questions focused on (a) professional development programs, (b) instructors, and (c) instructors’ online courses. Most program recommendations focused on (a) professional development programs, (b) context of professional development, and (c) instructors’ activity during professional development. Future recommendations for research topics focused on professional development programs and instructors, while future recommendations for research methods focused on research design and institutional settings. The findings suggest that while professional development for online instructors is important, consistency in both design and delivery is lacking. Future research is needed to provide guidance to programs, instructors, and institutions leading to satisfaction and success for more online students.
Alexiou-ray, J., & Bentley, C. C. (2016). Faculty professional development for quality online teaching. Online Journal of Distance Learning Administration, 19(2).
Archambault, L., Kennedy, K., Shelton, C., Dalal, M., McAllister, L. & Huyett, S. (2016). Incremental progress: Re-examining field experiences in K-12 online learning contexts in the United States. Journal of Online Learning Research, 2(3), 303-326.
Allen I.E., and Seaman, J. (2010). Class differences: Online education in the United States. Needham, MA: Babson Survey Research Group.
Allen, I. E., & Seaman, J. (2013). Changing course: Ten years of tracking online education in the United States. Needham, MA: Babson Survey Research Group.
Allen, I. E., Seaman, J., Poulin, R., & Straut, T. T. (2016). Online report card: Tracking online education in the United States. Needham, MA: Babson College Survey Research Group.
Attride-Stirling, J. (2001). Thematic networks: An analytic tool for qualitative research. Qualitative Research, 1(3), 385-405.
Barbour, M. K. (2012). Models and resources for online teacher preparation and mentoring. (K. Kennedy & L. Archambault, Eds.) Lessons learned in teacher mentoring: supporting educators in K-12 online learning environments. International Association for K-12 Online Learning.
Bigatel, P. M., Ragan, L. C., Kennan, S., May, J., & Redmond, B. F. (2012). The identification of competencies for online teaching success. Journal of Asynchronous Learning Network, 16(1), 59–78.
Bransford, J. D., Brown, A. L., & Cocking, R. R. (2000). How people learn: Brain, mind, experience, and school. Washington, D.C.: National Academies Press.
Carcioppolo, J. (2013). Designing a phase-based professional development program to improve andragogical effectiveness of faculty teaching online (Doctoral dissertation). Retrieved from ProQuest. (ED No. 553308)
Cobb, C., & Lowe, D. (2012). Influence of reduced seat time on satisfaction and perception of course development goals: A case study in faculty development. Journal of Asynchronous Learning Networks, 16(2), 85–98.
Davis, N. E., & Roblyer, M. D. (2005). Preparing teachers for the “schools that technology built”: Evaluation of a program to train teachers for virtual schooling. Journal of Research on Technology in Education, 37(4), 399–410.
Davis, N., Roblyer, M. D., Charania, A., Ferdig, R., Harms, C., Compton, L. K. L., & Cho, M. O. (2007). Illustrating the “virtual” in virtual schooling: Challenges and strategies for creating real tools to prepare virtual teachers. The Internet and Higher Education, 10(1), 27–39.
Davis, N., & Rose, R. (2007). Professional development for virtual schooling and online learning. Vienna, VA: North American Council for Online Learning. Retrieved from http://www.inacol.org/cms/wp-content/uploads/2012/11/NACOL_PDforVSandOlnLrng.pdf
Dawley, L., Rice, K., & Hinck, G. (2010). Going virtual! 2010: The status of professional development and unique needs of K-12 online teachers. Boise State University. Retrieved from https://edtech.boisestate.edu/goingvirtual/goingvirtual3.pdf
Easton, S. S. (2003). Clarifying the instructor’s role in online distance learning. Communication Education, 52(2), 87–105. https://doi.org/10.1080/03634520302470
Ferdig, R. E., Cavanaugh, C., DiPietro, M., Black, E., & Dawson, K. (2009). Virtual schooling standards and best practices for teacher education. Journal of Technology and Teacher Education, 17(4), 479–503.
Goodyear, P., Salmon, G., Spector, J. M., Steeples, C., & Tickner, S. (2001). Competences for online teaching: A special report. Educational Technology Research and Development, 49(1), 65–72.
Gregory, J., & Salmon, G. (2013). Professional development for online university teaching. Distance Education, 34(3), 256–270. https://doi.org/10.1080/01587919.2013.835771
Herman, J. H. (2012). Faculty development programs: The frequency and variety of professional development programs available to online instructors. Journal of Asynchronous Learning Networks, 16(5), 87–102.
International Association for K-12 Online Learning (iNACOL). (2011). National standards for quality online courses. Vienna, VA. Retrieved from http://www.inacol.org/cms/wp-content/uploads/2013/02/iNACOL_TeachingStandardsv2.pdf
Jaschik, S., & Lederman, D. (2016). The 2016 Inside Higher Ed survey of faculty attitudes on technology: A study by Gallup and Inside Higher Ed. Washington, DC. Gallup
Jaschik, S. & Lederman, D. (2018). The 2018 Inside Higher Ed survey of faculty attitudes on technology. Washington, DC: Gallup
Kebritchi, M., Lipschuetz, A., & Santiague, L. (2017). Issues and challenges for teaching successful online courses in higher education: A literature review. Journal of Educational Technology Systems, 46(1), 4–29.
Klein, J. M., Spector, J. M., Grabowski, B., & de la Teja, I. (2004). Instructor competencies: Standards for face-to-face, online, and blended settings. Greenwich, CT: Information Age Publishing.
Magda, A. J., Poulin, R., & Clinefelter, D. L. (2015). Recruiting, orienting, & supporting online adjunct faculty: A survey of practices. Louisville, KY: The Learning House, Inc.
Meyer, K. A, & Murrell, V. S. (2014a). A national study of theories and their importance for faculty development for online teaching. Online Journal of Distance Learning Administration, 17(2), 1–19.
Meyer, K. A, & Murrell, V. S. (2014b). A national study of training content and activities for faculty development for online teaching. Journal of Asynchronous Learning Networks, 18(1), 3–18.
Meyer, K. A. (2014). An analysis of the research on faculty development for online teaching and identification of new directions. Journal of Asynchronous Learning Networks, 17(4), 93–112.
Mishra, P., & Koehler, M. J. (2006). Technological pedagogical content knowledge: A new framework for teacher knowledge. Teachers College Record, 108(6), 1017–1054.
Muñoz Carril, P. C., Sanmamed, M. G., & Hernández Sellés, N. (2013). Pedagogical roles and competencies of university teachers practicing in the E-learning environment. International Review of Research in Open and Distance Learning, 14(3), 462–487.
National Education Association. (2006). Guide to teaching online courses. National Education Association, 1–21. Washington, D.C.: National Education Association.
Pearcy, M. (2014). Student, teacher, professor: Three perspectives on online education. History Teacher, 47(2), 169-185.
Pulham, E. B., & Graham, C. R. (2018). Comparing K-12 online and blended teaching competencies: A literature review. Distance Education, 39(3), 411-432.
Ragan, L., Bigatel, P. M., Kennan, S. S., & May, D. J. (2012). From research to practice: Towards the development of an integrated and comprehensive faculty development program. Journal of Asynchronous Learning Networks, 16(5), 71–86.
Rhode, J., Richter, S. & Miller, T. (2017). Designing personalized online teaching professional development through self-assessment. TechTrends, 61(5), 444-451.
Rice, K., & Dawley, L. (2009). The status of professional development for K-12 online teachers : Insights and implications. Journal of Technology and Teacher Education, 17(4), 523–545.
Seaman, J. (2009). Online learning as a strategic asset. Volume II: The paradox of faculty voices--Views and experiences with online learning. Retrieved from http://www.eric.ed.gov/contentdelivery/servlet/ERICServlet?accno=ED517311
Southern Regional Education Board. (2006). Standards for quality online teaching (Vol. 30318). Atlanta, GA: Southern Regional Education Board.
Taylor, A., & McQuiggan, C. A. (2008). Faculty development programming: If we build it, will they come? EDUCAUSE Quarterly, 31(3), 28–37.
Terosky, A. L., & Heasley, C. (2015). Supporting online faculty through a sense of community and collegiality. Online Learning, 19(3), 147–161.
Vaill, A. L., & Testori, P. A. (2012). Orientation, mentoring and ongoing support: A three-tiered approach to online faculty development. Journal of Asynchronous Learning Networks, 16(2), 111–119.
As a condition of publication, the author agrees to apply the Creative Commons – Attribution International 4.0 (CC-BY) License to OLJ articles. See: https://creativecommons.org/licenses/by/4.0/ .
This licence allows anyone to reproduce OLJ articles at no cost and without further permission as long as they attribute the author and the journal. This permission includes printing, sharing and other forms of distribution.
Author(s) hold copyright in their work, and retain publishing rights without restrictions
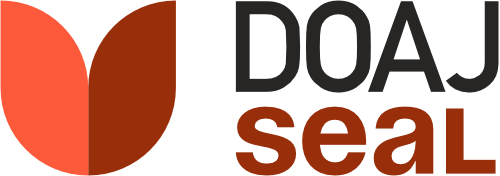
The DOAJ Seal is awarded to journals that demonstrate best practice in open access publishing
OLC Membership

OLC Research Center

Information
- For Readers
- For Authors
- For Librarians
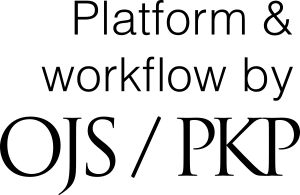
- Home
The Covid-19 pandemic had pressured institutions around the world to move to online learning. Students, teachers, and education administrators were not prepared for the sudden change in the mode of delivery. As a result, there have been issues with online learning. Many studies have been conducted in the last two years to explore the matter. In this paper, the author will attempt to provide a literature review of previous studies about barriers to online learning both before and after the pandemic. A comprehensive list of barriers is presented. As a result of the review, there is not much difference in the barrier to online learning before and after the pandemic. This paper aims to present a broad overview of the topic so educators and school administrators can develop a plan to enhance the quality of online education in the future.
Barriers to Online Education , E-Learning , Distance Education , Covid-19 Pandemic
Share and Cite:

1. Introduction
Online and distance education has been around for a couple of decades now. However, due to the Covid-19 pandemic in the last two years (2020 and 2021), we see a significant shift to online learning from the traditional mode of delivery. Many students and parents experienced online learning for the first time. Because of the lockdown in many places, online learning became the solution for kindergarten to doctoral level learners. Despite the many benefits that online learning brings to our education system, barriers to the effectiveness of this delivery mode are still present. This paper will summarize barriers to online learning and, thus, serve as a foundation for educators and education administrators to develop strategies to improve this learning mode in the future.
This paper will provide a broad literature review of current and past research on the topics of barriers to online learning. It will cover various education levels, including K-12 and Higher Education in many countries around the world. Several papers from the pre-pandemic time are also being included. The novelty of this paper is attempting to see if there is any major difference between the barrier to online learning before and after the pandemic.
2. Barriers to Online Learning Pre-Pandemic
E-learning or online learning refers to the usage of modern information and communication technologies to deliver educational content to students. This learning mode can overcome physical distance [1]. The phenomenon of e- learning became popular in the early 1990s due to the rapid evolution of the Internet. Despite the many benefits that eLearning brings to the educational landscape, some drawbacks yet need to be addressed. Muilenbug and Berge (2005) conducted a study to explore barriers to online learning; this is one of the earliest explorations of the topic. The context of the study was the United States. The study result identified eight barriers: a) administrative issues, b) social interaction, c) academic skills, d) technical skills, e) learner motivation, f) time and support for studies, g) cost and access to the Internet, and h) technical problems [2]. However, there might be changes to the barriers since the paper was first published because the eLearning industry has been evolving rapidly, according to Bezovski and Poorani [1].
In another study immediately before the pandemic, Aljaraideh and Bataineh (2019) researched the barrier to online learning for students in Jordan [3]. Four hundred students were asked to fill out questionnaires. The researchers conducted a pilot study on the first 50 respondents to ensure the reliability of the questionnaire. The authors then used quantitative methods to analyze the collected data. The findings showed that technological infrastructure was the primary barrier to online learning. Indeed, online learning was a new phenomenon in developing countries [3].
Aljaraideh and Bataineh (2019) also pointed out that first- and second-year students faced more significant barriers than third- and fourth-year students. This phenomenon can be explained as new students did not have much technical experience compared to their senior peers. Female students faced more barriers for the first two years than their male counterparts. However, it is the opposite in the last two years when male students faced more barriers. Online learning was new in Jordan, which explained the lack of technological infrastructure; especially, this study was conducted before the Covid-19 pandemic. Other similar studies below also identify an interaction between the student’s gender and the year variable.
Bates (2017) published a report on online education in Canada [4]. According to the author, Canada has an extensive online and distance education history. Even before the Covid-19 pandemic, online education enrolment had grown rapidly in North America. As of 2017, almost all Canadian post-secondary institutions (except Quebec) offer distance learning in various fields of study. Canadian institutions have been using the Internet, learning management systems, interactive lectures, social media, mobile devices, and synchronous sessions to deliver online courses. Many institutions already had comprehensive strategies for expanding online education before the pandemic as they recognized the importance of online education. Although the country has a long history of online learning, it still faces several issues. The main barriers pointed out by Canadian institutions were lack of resources and lack of specialists in learning technology. This barrier is related to online learning administrative support. As one sample solution to the problem, the provincial government of British Columbia has focused on developing Open Education Resources (OER), which can be valuable support for online education. With open resources, it is easier for students to have access to online resources [4].
3. Barriers to Online Learning during the Pandemic
Baticulon et al. (2021) studied the barrier to online learning in the context of medical students in the Philippines [5]. The authors collected data using the electronic survey in mid-2020 from 3670 medical students. Their survey includes various questions ranging from multiple choices on the Likert scale to open- ended questions. The majority of participants own smartphones and laptops or desktop computers. Less than half (41%) of the students were “physically and mentally capable of engaging in online learning” [5]. The barriers identified are adjustment to the online learning style, balance with family responsibilities, and communication issues between learners and instructors. First, since the pandemic came very suddenly, students, faculties, school administrators, and the curriculum were not yet ready to switch the delivery mode. Second, studying at home made it harder for students to balance family responsibilities [5].
Moreover, many students did not have a dedicated studying area at home. Thus, students got interfered from other family members. Third, communication issue between learners and teachers was raised. A possible explanation was that both the learners and teachers were unprepared for the transition [5].
Van and Thi (2021) conducted a mixed-method study to determine the barrier to online learning in Vietnam during the Covid-19 pandemic [6]. The sample size was 1165 students from various universities and high schools in Vietnam. The obstacles identified include lack of social interaction, cost and access to the Internet, learner motivation, and family distraction. Contradicting Aljaraideh and Bataineh’s study, technological skill is not a significant problem for the students. Students were somewhat prepared by having basic technical skills, prior IT training that they received in the previous years and reviewing instructional training videos for critical online learning platforms. The cost of accessing the Internet includes investment in hardware such as laptops or computers, or mobile devices. For students from rural areas, accessing the Internet can be more challenging compared to students from urban areas. The other factors, such as lack of interaction, student motivation, and family distraction, are consistent with other studies in this review [6].
In another case in Southeast Asia, the study conducted by Octaberlina and Muslimin (2020) explored the barrier to online learning for English as a Foreign Language (EFL) students in Indonesia [7]. The authors conducted a mixed- method survey of 25 EFL students. The identified barriers include unfamiliarity with e-learning, slow internet connection, and physical condition such as eye strain. The geographical nature of Indonesia can explain the issue with an internet connection. The country's population is spread out over a large number of islands [7].
Regarding the Learning Management System (LMS), many students were unfamiliar with Google Classroom, which was used at their institution. A proposed solution is to have training of the LMS before actual learning, reduce the file size for the learning materials to accommodate the slow internet connection, and ensure breaks during online learning sessions. Regarding reducing the file size of learning materials, a suggestion is to use audio instead of video since students can listen to the audio lecture while doing other things. Moreover, text and audio transmission will be better compared to videos with a slow internet connection. Participants in this research pointed out several distractions, such as online games and YouTube. Indeed, this might not be the case with students with a slow internet connection. The authors also discussed physical conditions such as eye constraints when looking at the computer screen for a prolonged period [7].
Anastasakis, Triantafyllou, and Petridis (2021) performed qualitative research to identify barriers to online learning during the pandemic in Greece [8]. The author used a qualitative survey to collect data from 2093 undergraduate students. The discovery is consistent with other studies on the same topic in different countries. The majority of the participants did not have any experience with online learning. Only half of the students were confident with online learning. Their finding confirms other research results regarding internet connection issues and lack of social interactions. The switch to online learning posed a significant challenge to universities worldwide. Other barriers related to the lecturers’ online teaching skills; are lecturers’ technical skills, not having synchronous sessions, not uploaded teaching materials, confusing timetables, and using various platforms [8]. As stated by Baticulon et al. (2021), both students and teachers had to switch to online learning in a short period [5]. Thus, the institution needs continuous training to help teachers adapt to the new environment. Other barriers are the administrative issue, the appropriateness of course content for online delivery, distractions in the environment, learners’ characteristics (i.e., time management, shyness, and disability), and engagement during online classes. According to the authors, such as Greece, these barriers exist in countries where distance or online learning is not well-established. Many universities did not have strategic plans to promote online or distance education before the pandemic. According to Moore (1989), as cited in Anastasakis, Triantafyllou, and Petridis (2021), effective education will require three types of interactions: learner-content (LC), learner-instructor (LI), and learner-learner (LL) [9]. However, due to the sudden switch to online learning, these interactions are either not met or merely met. According to the finding, several subjects that require lab work might not be appropriate to be fully online.
Another study was conducted to discover the barriers to online learning from students in Bangladesh by Islam and Habib (2021) [10]. The authors applied the quantitative method by surveying 394 university students with a semi-structured online questionnaire. The study includes 50.5% undergraduate students, 48% master students and 1.5% doctoral students. Moreover, 24.9% of students come from rural areas, 40.6% from suburban areas, and 34.5% from urban areas. About two-thirds of the respondents were male. The finding revealed a couple of themes 1) environment and situational barriers, 2) e-learning barriers, 3) psychological barriers, and 4) disruption of online learning adoption [10]. The barriers, according to Islam and Habib (2021), include:
Roslan and Halim (2021) performed a mixed-method study to explore the enablers and barriers to online learning in Malaysia [11]. The authors conducted a cross-sectional study of 178 participants and in-depth interviews with 10 participants from public medical schools in Malaysia. Several barriers emerged from the study, although all students own at least one learning device. First, 22.5% of students have no learning space at home. This issue can cause distraction during online learning. The second barrier is internet access: 21.9% of students have no wi-fi access, and 11.2% have no mobile broadband coverage. The study also found that using low bandwidth applications (such as Whats App and Telegram) and easily accessible platforms (such as YouTube) can help to ease the problem. The use of YouTube is slightly inconsistent with the other studies by Octaberlina and Muslimin (2020) in the neighbouring country of Indonesia [7]. However, both arguments are reasonable. On the one hand, Roslan and Halim (2021) pointed out that YouTube is easily accessible to all students compared to other platforms. On the other hand, Octaberlina and Muslimin (2020) argued that YouTube content could cause a distraction to students. Also, in some cases where students have a slow internet connection, loading video is a challenge [11].
Alshwiah studied the barrier to online learning faced by secondary students in Saudi Arabia [12]. Similar to the above study, mixed methods were used in this research. The first step is interviewing four parents and four students to explore the barriers. It is one of the rare studies that involved parents. Then, the author surveyed 518 respondents on the barriers. Private schools seem to perform better for online learning compared to public schools. Also, consistent with Aljaraideh and Bataineh (2019), female students faced more barriers than male students [3]. Several other barriers were identified, including a lack of computer equipment and high-speed internet connection. Another significant barrier is that the curriculum, which was traditionally used face-to-face, is now delivered online without proper review and redesign. In addition, teachers were not well trained to deliver online classes (online teaching skills). Poor online learning tools can also cause problems for the students: “lack of instructions, difficult navigation, an uninteresting interface, unresponsive website, and disorganized e-content” [12]. Indeed, these reasons are understandable due to a rapid switch to online learning from the traditional delivery mode. A confused grading policy also contributed to the problem, reducing the student’s motivation and productivity.
Kara (2021) conducted a qualitative case study on 44 university students to find out the barriers and enablers of online learning [13]. Data collection techniques include structured and semi-structured interviews with the research participants. Five main themes came out of the analysis: online content, online assignments, online assessments, instructor behaviour & practice, and psychological issues. Moreover, students also felt pressure from taking many online courses. The online content is hard to follow because of a lack of interaction with peers and teachers. The solutions include online video, teleconferencing software for synchronous learning mode, and how content is organized into modules. Students also pointed out that online assessment feedback are critical to their success in online learning. Thus, instructors should provide clear instructions and detailed feedback to students [13].
Regarding instructors’ behaviour, late replies and negative messages can hinder students’ success. This issue indicated that instructors are not prepared for online teaching. The last group on psychological issues is consistent with other studies, and it is about the distraction of learning at home. In general, the author also pointed out that as students take online courses, it positively impacts their mood during the lockdown period [13].
Li et al. (2021) conducted a similar study on postgraduate students in China. The authors pointed out that online learning developed significantly during the pandemic. Online learning platforms provide positive contributions to students learning process at the postgraduate level. The authors are optimistic that the challenge we see will create opportunities for new development in online learning. There are several suggestions from the study. First, teachers must be trained to become more familiar with online teaching. Second, each institution should
Table 1 . Summary of barriers to online learning.
develop and maintain unified or standardized online platforms to avoid confusion for students. Lastly, they also suggested that there should be other platforms to facilitate learning and research for postgraduate students, such as the Online Scientific Research Platform and Online Academic Exchange Platform. As the author stated, these platforms will benefit research activities for postgraduate students [14].
4. Conclusion
The paper has outlined many significant barriers to online learning in various studies from all over the world. It broadly covers cases from advanced nations (e.g., the United States & Canada) where online education has been well-established compared to other nations (e.g., Greece). Some barriers are rooted in online learning itself, such as physical issues like eye constraints when looking at the computer screen for an extended period. A sudden switch caused other barriers to online learning without any preparation due to the pandemic. Table 1 summarizes the barriers found in different studies. No significant difference was found for barriers before and after the pandemic. The limitation of this study is that it cannot cover all literature in the field. However, the literature review seems to be exhausted because the barriers are repeated and relatively consistent with each other. Future research could consider a case study to compare the barriers to online learning in developed countries with better technological infrastructure and underdeveloped countries with poor infrastructure. This paper can contribute positively to online education by listing barriers. It can guide educational administrators, educators, and researchers to understand the problems and develop solutions for the future. Thus, it will enhance the effectiveness of online education and benefit society at large.
Conflicts of Interest
The author declares no conflicts of interest.
[ ] | Bezovski, Z. and Poorani, S. (2016) The Evolution of E-Learning and New Trends. Information and Knowledge Management, 6, 50-57. |
[ ] | Muilenburg, L.Y. and Berge, Z.L. (2005) Student Barriers to Online Learning: A Factor Analytic Study. Distance Education, 26, 29-48. https://doi.org/10.1080/01587910500081269 |
[ ] | Aljaraideh, Y. and Al Bataineh, K. (2019) Jordanian Students' Barriers of Utilizing Online Learning: A Survey Study. International Education Studies, 12, 99-108. https://doi.org/10.5539/ies.v12n5p99 |
[ ] | Bates, T. (2017) The 2017 National Survey of Online Learning in Canadian Post-Secondary Education: Methodology and Results. International Journal of Educational Technology in Higher Education, 15, Article No. 29. https://doi.org/10.1186/s41239-018-0112-3 |
[ ] | Baticulon, R.E., Sy, J.J., Alberto, N.R.I., et al. (2021) Barriers to Online Learning in the Time of COVID-19: A National Survey of Medical Students in the Philippines. Medical Science Educator, 31, 615-626. https://doi.org/10.1007/s40670-021-01231-z |
[ ] | Van, D.T.H. and Thi, H.H.Q. (2021) Student Barriers to Prospects of Online Learning in Vietnam in the Context of Covid-19 Pandemic. Turkish Online Journal of Distance Education, 22, 110-123. https://doi.org/10.17718/tojde.961824 |
[ ] | Octaberlina, L.R. and Muslimin, A.I. (2020) EFL Students Perspective towards Online Learning Barriers and Alternatives Using Moodle/Google Classroom during COVID-19 Pandemic. International Journal of Higher Education, 9, 1-9. https://doi.org/10.5430/ijhe.v9n6p1 |
[ ] | Anastasakis, M., Triantafyllou, G. and Petridis, K. (2021) Undergraduates' Barriers to Online Learning during the Pandemic in Greece. Technology, Knowledge and Learning. https://link.springer.com/article/10.1007/s10758-021-09584-5#citeas |
[ ] | Moore, M.G. (1989) Three Types of Interaction. American Journal of Distance Education, 3, 1-7. https://doi.org/10.1080/08923648909526659 |
[ ] | Islam, M.T. and Habib, T.I. (2021) Barriers of Adopting Online Learning among the University Students in Bangladesh during Covid-19. Indonesian Journal on Learning and Advanced Education (IJOLAE), 4, 71-90. https://doi.org/10.23917/ijolae.v4i1.15215 |
[ ] | Roslan, N.S. and Halim, A.S. (2021) Enablers and Barriers to Online Learning among Medical Students during COVID-19 Pandemic: An Explanatory Mixed-Method Study. Sustainability (2071-1050), 13, Article 6086. https://doi.org/10.3390/su13116086 |
[ ] | Alshwiah, A.A. (2021) Barriers to Online Learning: Adjusting to the “New Normal” in the Time of COVID-19. Turkish Online Journal of Distance Education, 22, 212-228. https://doi.org/10.17718/tojde.1002858 |
[ ] | Kara, N. (2021) Enablers and Barriers of Online Learning during the COVID-19 Pandemic: A Case Study of an Online University Course. Journal of University Teaching and Learning Practice, 18, Article 11. https://doi.org/10.53761/1.18.4.11 |
[ ] | Li, Y., Wen, X., Li, L., Zhou, Y., Huang, L., Ling, B., Liao, X. and Tang, Q. (2021) Exploration of Online Education Mode for Postgraduate Education under the Background of COVID-19. Advances in Applied Sociology, 11, 223-230. https://doi.org/10.4236/aasoci.2021.115019 |
- Articles
- Archive
- Indexing
- Aims & Scope
- Editorial Board
- For Authors
- Publication Fees
Journals Menu
- Open Special Issues
- Published Special Issues
- Special Issues Guideline
- E-Mail Alert
- OALibJ Subscription
- Publication Ethics & OA Statement
- Frequently Asked Questions
- Recommend to Peers
- Recommend to Library
- History Issue
+1 323-425-8868 | |
+86 18163351462(WhatsApp) | |
Paper Publishing WeChat |
Copyright © 2024 by authors and Scientific Research Publishing Inc.

This work and the related PDF file are licensed under a Creative Commons Attribution 4.0 International License .
- Journals A-Z
About SCIRP
- Publication Fees
- For Authors
- Peer-Review Issues
- Special Issues
- Manuscript Tracking System
- Subscription
- Translation & Proofreading
- Volume & Issue
- Open Access
- Publication Ethics
- Preservation
- Privacy Policy
- Open access
- Published: 15 July 2024
Based case based learning and flipped classroom as a means to improve international students’ active learning and critical thinking ability
- Wanjing Yang 1 ,
- Xiaoyan Zhang 1 ,
- Xinhuan Chen 1 ,
- Jing Lu 1 &
- Fang Tian 1 , 2
BMC Medical Education volume 24 , Article number: 759 ( 2024 ) Cite this article
37 Accesses
Metrics details
International student education has become an important part of higher education and an important symbol to measure the level of higher education. To change the traditional teaching model, here we introduced a combination of Case-Based Learning (CBL)and Flipped Classroom (FC) into the pathophysiology course for international students. This study aimed to explore whether the active learning ability and critical thinking ability of international students can be improved, based on this new teaching model, improving the innovation ability of teachers’ team and students’ attitude to the reform.
The two chapters of Cardiac Insufficiency and Apoptosis in Pathophysiology are designed as a CBL + FC teaching method. Distribute the Self-assessment Scale on Active Learning and Critical Thinking (SSACT) and satisfaction questionnaire to international students to evaluate teaching reform based on CBL + FC.
Compared with the traditional classroom, the online flipped classroom based on CBL has significantly improved the learning enthusiasm, as these students are required to independently complete literature review, actively participate in classroom teaching, learn to use multiple learning strategies, and collaborate with other students to complete PowerPoint (PPT)production. At the same time, the students’ ability to raise problems and solve problems has been greatly improved by analyzing clinical cases; By consulting the literature, the theoretical knowledge learned can be better applied to clinical analysis. The results of the satisfaction survey also show that international students are more likely to accept the flipped classroom teaching mode.
Conclusions
This teaching mode will stimulate the learning motivation of international students, enhance teaching attraction and increase teaching interaction; At the same time, the CBL + FC teaching method can strengthen the evaluation of international students’ in and out of class and online learning, enhance students’ active learning ability and critical thinking ability, promote the development of personalized learning, and integrate with international medical education.
Peer Review reports
Introduction
At the beginning of the new-year in 2020, the epidemic caused by covid-19 is a major global public health event. Among the people affected by these restrictions, there is a special group of international students who come to China’s colleges and universities to study abroad [ 1 ]. Through the practice of teaching and management of international students for many years, research shows a clear understanding of the characteristics of the group of international students, such as: (1) most of the group of international students come from Asian countries, with great regional cultural differences; (2) The basic education level and development level of each country are different, and the acceptance ability also varies greatly; (3) The vast majority of international students like to participate, like to communicate with teachers, interactive learning and group discussion teaching [ 2 , 3 , 4 ]. Therefore, according to the characteristics of international students, how to change the traditional teaching mode of Pathophysiology for international medical students online with the single output of teachers, insufficient learning initiative of international students, paying attention to the deep integration of modern information technology and education and teaching, enhancing the interaction between teachers and students, students and students, has become an urgent problem to solve.
Active learning and continuous quality improvement are critical strategies when designing and refining medical school curricula [ 5 , 6 ]. Some studies showed that medical educators, both at our institution and nationwide, are deliberately re-evaluating their curricula to incorporate active learning instructional methodology [ 5 , 7 ]. In the study of Rose et al. showed that active learning, with regular incorporation of student feedback vis-àvis a PDSA cycle, was effective in achieving high student engagement in an Internal Medicine core clerkship session on antibiotic therapy. The study findings have potential implications for medical education and suggest that the application of the PDSA cycle can optimize active learning pedagogies and outcomes [ 8 ].
Critical thinking (CT) plays a central role in one’s learning and working, particularly in addition to medical education that transitions from knowledge-based curricula to competency-based curricula. It is crucial for students to learn and work further critically to evaluate existing knowledge and information. CT is vital to a health professional’s competence to assess, diagnose and care for patients correctly and effectively [ 9 ]. Studies by Ramlaul et al. indicated that as participants progressed from year one to year three, they recognized that critical thinking comprised not only of cognitive skills but affective skills too. They attributed their developing understanding of the meaning of critical thinking to clinical placement learning, understanding written feedback, and the expectations of professional practice [ 10 ]. Several other studies also revealed the relationship between critical thinking and academic success of medical professionals, such as a students’ PBL performance, health professions education and the motivation for learning [ 11 , 12 , 13 , 14 ].
Blended learning is a comprehensive and three-dimensional teaching mode that integrates various means such as offline teaching, online learning resources utilization, and mutual evaluation and feedback between students and teachers and students. Therefore, in the course of curriculum construction, it is necessary to promote and popularize this teaching method on a large scale, so that all teachers and students can actively participate in an efficient operation of “teaching” and “learning”. The teacher will change from the leading role in the traditional classroom to the supporting role in the blended learning mode, becoming the leader and supervisor, while the students will change from the traditional supporting role to the leading role, becoming the center and active element of the teaching link. Through the implementation of mixed teaching, students can be trained to learn actively, and the former “I want to learn” has become the current “I want to learn” situation. In the mixed teaching mode, teachers have changed the traditional way of teaching students “what is” into heuristic teaching of teaching students “why”, and gradually cultivate students to develop critical thinking methods such as raising questions, solving problems, and questioning, thus greatly improving the efficiency of teaching and learning. Many classes in higher education institutes now employ blended learning; whereby students learn in part at a supervised face-to-face location on campus, and in part through the Internet with some elements of student choice over place and pace [ 15 ]. Of the many different models of blended learning in practice, the use of flipped classroom approach has become increasingly widespread [ 16 , 17 , 18 ].
More and more studies show that compared with traditional teaching methods, the teaching effect of flipped classroom does have certain advantages [ 19 , 20 , 21 ]. In addition, with the progress of COVID-19, the research of online flipped classroom has also made some progress. Hew et al. showed that the participants in the fully online flipped classes performed as effectively as participants in the conventional flipped learning classes. Their qualitative analyses of student and staff reflection data identify seven good practices for videoconferencing - assisted online flipped classrooms [ 22 ]. Students in flipped courses exhibited gains in critical thinking, with the largest objective gains in intermediate and upper-level courses. Results from this study suggest that implementing active-learning strategies in the flipped classroom may benefit critical thinking and provide initial evidence suggesting that underrepresented and first-year students may experience a greater benefit [ 23 ].
CBL is an established approach used across disciplines where students apply their knowledge to real-world scenarios, promoting higher levels of cognition. Thus the objective of this study is to explore whether the active learning ability and critical thinking ability of international students can be improved by applying the innovation ability of teachers’ team, updating teaching ideas, and using the reform of CBL + FC teaching mode, so as to lay a foundation for building a first-class course of Pathophysiology for international students.
Materials and methods
Research object.
The study was conducted at the school of international education from 2021 to 2022, Zhengzhou University, which employs CBL + FC in the third grade international students (there are 296 students in total), majors include clinical medicine, pharmacy and stomatology. They were divided into two groups: 115 students majoring in clinical medicine participated in online flipped classrooms, while 181 students majoring in pharmacy and stomatology participated in traditional classrooms.
The same teaching faculty is responsible for the teaching process. Oral informed consent was obtained from each student and teacher. This study was approved by the school ethics committee (Zhengzhou University Life Sciences Ethics Review Committee).
Curriculum implementation plan and method
The two chapters of Cardiac Insufficiency and Apoptosis are designed as a CBL + flipped classroom teaching method, specific implementation process: A case is divided into multiple scenarios (parts) and send to students one week before class. Students summarize the symptoms and signs of the patient from the case, propose possible diagnosis, discuss the mechanism and raise questions. It is important to ask questions from the case. These questions can be solved by searching online in time during discussion, while the deeper questions can be recorded and assigned to each student. Later, students can acquire knowledge and independence through self-study methods, such as reading books, checking materials and MOOC study. The team leader will summarize and refine the questions raised by each group member, and 4–5 students make them into PPT (6–7 min), and reported in the class; the team leaders can communicate with each other to avoid solving the same problems, and each group should have its own focus. At last, teachers conduct in-depth analysis of the case, and make a general comment on the contents of each reporting group, especially to find out the highlights and innovations of students in the reporting process, and timely encourage and praise them.
Effect evaluation
the establishment and implementation of teaching information collection system, international students’ teaching evaluation system and information feedback system is not only an important teaching management measure, but also a way to mobilize students’ enthusiasm and give full play to their personality. At the same time, a questionnaire is designed to evaluate whether the teaching reform based on Case + flipped classroom can improve the autonomous learning ability and critical thinking ability of international students and whether they are satisfied with this teaching method.
Limitations and design
When conducting intervention studies on international students, it is crucial to ensure the consistency of the baseline conditions as much as possible to reduce bias. Of course, there are also some confounding factors that can affect the experimental results, such as:
1) International students from different countries may have different study habits or personalities;
2) The academic performance of international students upon admission varies;
3) International students may have a stronger sense of self-awareness.
In response to these factors, the following measures have been taken:
1) To reduce the impact of these differences on the results, researchers randomly assigned international students from different countries and majors into two groups, rather than grouping them by country or major;
2) The educational reform was placed in the sixth semester, at which point international students have generally adapted to the teaching methods in China and their academic performance is relatively stable, so this educational reform mainly focused on the students’ learning attitudes rather than solely on their grades;
3) Given the strong sense of autonomy among international students, their own choices were fully respected on the premise of random grouping. At the same time, the impact was reduced through the use of anonymous surveys and ensuring privacy protection.
Instruments for Self-assessment Scale on Active Learning and Critical Thinking (SSACT) and satisfaction questionnaire disposition assessment
The 14 item SSACT consisted of two domains “active learning” and “critical thinking” [ 24 , 25 ]. A five-point Likert scale questionnaire was used to evaluate student before and after class, ranging from 1 (strongly disagree) to 5 (strongly agree) (Table 1 ).
A five-point Likert scale Satisfaction questionnaire with 17 items was used to evaluate student perceptions of the effectiveness of CBL + Fipped classroom when we finished the class, ranging from 1 (strongly disagree) to 5 (strongly agree) [ 26 ].
Students were allowed to finish the questionnaire via an internet website,
where the data were collected from their submission. There are 296 students in total, 278 of them took part in the survey. In addition,106 students participated in the online flipped classroom and 172 students participated in the traditional classroom.
Statistical analysis
SPSS software is used to analyze the scores of students’ creative thinking evaluation, critical thinking evaluation and satisfaction evaluation of online flipped classroom. The measurement data are expressed by means and standard deviation, T-test was used to statistically infer students’ creative thinking and critical thinking ability.
1. In terms of students’ active learning, compared with the traditional classroom, the online flipped classroom has improved the basic learning enthusiasm (Fig. 1 ), especially in promoting the use of multiple learning strategies in autonomous learning (4.29 ± 0.74, p < 0.05), managed independent study effectively(4.23 ± 0.86, p < 0.05), encouraging other members to assist in learning (3.95 ± 1.01, p < 0.05), and reflected on the learning in each scenario based on the objectives (4.22 ± 0.86, p < 0.05). However, some students showed deficiencies in summarizing the key points of the outcome of the group discussion (Table 2 ).
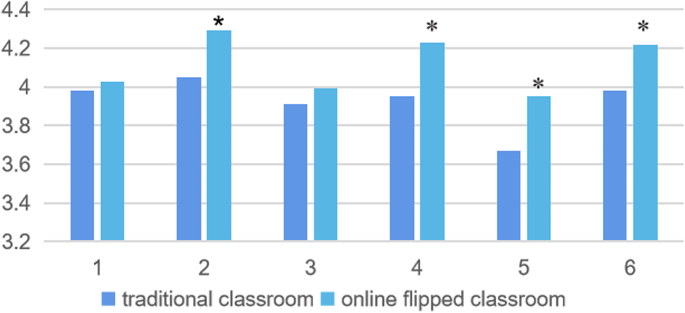
The comparison of active learning between online flipped classroom and traditional classroom. Notes * p < 0.05
2. In terms of students’ critical thinking, compared with the traditional classroom, the online flipped classroom has improved students’ critical thinking ability (Fig. 2 ), and several of them have improved significantly (Table 3 ): I analyzed information in the scenario using relevant theory and concepts (4.2 ± 0.74; p < 0.005), I could generate a discussion to explain the problem under discussion (4.05 ± 0.87; p < 0.005), I communicated my ideas clearly. (4.02 ± 0.94; p < 0.05), In the second meeting, I applied knowledge from my independent study to provide a solution to the problem being discussed(4.05 ± 0.84; p < 0.05).
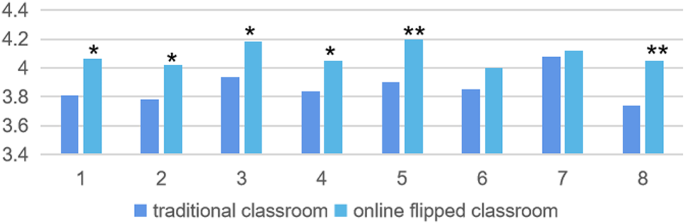
Comparison between online flipped classroom and traditional classroom in critical thinking. Notes * p < 0.05, ** p < 0.005
3. In terms of satisfaction survey, we sent out questionnaires to students to find out whether they are satisfied with the online flipping class. The overall questionnaire adopts a five-level scoring system, and the answer options are scored from low to high as 1, 2, 3, 4, and 5, which are very dissatisfied, dissatisfied, general, satisfied, and very satisfied (Table 4 ). 105 questionnaires were collected. Among them, the overall satisfaction of students is 3.96 on average, and the rate of very satisfied or satisfied with the online flipped classroom teaching is 75.6%, and the answer value of each item is also high. The score of online flipped classroom encouraging students to learn more about the conditions discussed through cases is 4.11, and the score of 4.13 in more chapters that hope this teaching method will be used is the highest, It shows that learners are relatively satisfied with the online flipped classroom teaching mode and affirm the possibility of continuous development. In addition, many students think that the online flipping class plays a great role in improving self-learning ability, analysis ability and learning interest. At the same time, students are also satisfied with the design of the case, the knowledge covered by the case and the organization of the course.
In the teaching process of international students, the vast majority of international students show the characteristics of publicity and participation. Many teachers find that international students don’t like traditional teaching methods. They like to communicate and interact with teachers in class. Group discussion and other forms of teaching are also preferred by students. They believe that this can show students’ own learning ability and level. In addition, they have a strong desire to complete their studies and get a degree. Therefore, in the teaching process, we should reasonably mobilize and give full play to the positive factors and personality publicity characteristics of international students in learning, promote teaching reform, and promote students’ participation in the teaching process.
At present, the education of international students has become an important part of higher education. Most international students choose to return to work after graduation and need to take the local medical practitioner examination. However, the curriculum and teaching management mode of our school mostly follow Chinese standards and characteristics, and there is a certain disconnect with international medical standards, which makes it difficult for international students to adapt to the domestic examination mode in a short time after graduation, and often requires a lot of energy and time to prepare for the examination. The growth and success of overseas students in China has become an important way for the world to know and understand China, and is of great positive significance in establishing China’s international image and enhancing the friendship between the Chinese people and the people of all countries in the world. Therefore, we must actively reform the curriculum construction of international medical students in China to realize the international integration of international student education.
Flipped classroom (FC) refers to a teaching mode that flips the traditional classroom teaching (TC), through the use of advanced information technology, students learn by themselves through online videos recorded by teachers and learning requirements before class, so that students can complete the learning of knowledge before class, solve problems through teachers’ guidance, discussion and communication in class, promote the internalization of knowledge, and complete the evaluation of learning results and knowledge improvement after class.
In Morton DA et al. research, they wanted to determine whether FC instruction is superior to TC instruction for learning gross anatomy. The results showed that students in an FC setting may perform better than those in a TC on assessments requiring higher cognition (e.g., analysis), but the same on those requiring lower cognition (e.g., memorization and recall) [ 27 ]. To compare the effects of Flipped Classroom vs. Traditional Classroom on students’ academic achievement, task value, and achievement emotions, O’Connor EE et al. proved that the positive emotional effects of FC on medical students’ motivational beliefs and achievement emotions can enhance academic performance. The FC approach provides medical students with the opportunity to develop self-directed learning skills while also providing opportunities to solidify already acquired knowledge and concepts through active learning strategies [ 28 ]. At last, in the research of Kraut et al. a total of 54 papers (33 quantitative, four qualitative, and 17 review) on FC met a priori criteria for inclusion and were critically appraised and reviewed. The top 10 highest scoring articles (five quantitative studies, two qualitative studies, and three review papers) are summarized in this article. Their results confirmed that (1) A Flipped Classroom or Blended Learning Approach is Effective for Procedural Learning; (2) Students in a Flipped Classroom Setting May Learn More Than Students in a Traditional Classroom Setting; (3) The Flipped Classroom Model is Beneficial for Learning Higher Cognition Tasks; (4) Learners Are More Engaged with Flipped Classroom, but Satisfaction Depends Largely on Teacher Prep Work [ 29 ].Therefore, flipped classroom can improve the purpose of teachers’ teaching, endow international students with autonomy in learning, and enhance the teaching effect. It has become a new concept and teaching mode of international students’ education and teaching in the stimulating the learning motivation and professional interest of international students, enrich teaching means, enhance teaching attraction, and increase teaching interaction; At the same time, by strengthening the evaluation of in class and online learning of international students, we can increase their active learning ability, promote personalized learning, improve their autonomous learning ability and critical thinking ability, promote the development of pathophysiology course, and integrate with international medical education.
Several studies showed that flipped classroom combined with case-based learning is an effective teaching modality in nephrology clerkship and other medical class [ 30 , 31 ]. Cai et al. also proved that CBL-based FC modality has promising effects on undergraduate pathology education and may be a better choice than traditional LBC. Further optimizations are needed to implement this novel approach in pathology and other medicine curricula [ 32 ]. Various evidence-based and student-centered strategies such as Team-Based Learning (TBL), Case-Based Learning (CBL), and Flipped Classroom (FC) have been recently applied to anatomy education and have shown to improve student engagement and interaction [ 33 , 34 ].
The advisability of early contact with clinical knowledge has been accepted by the world. There is no better teacher than “interest”. Let medical students contact clinical knowledge as early as possible, and closely combine book knowledge with clinical knowledge, which will improve students’ learning enthusiasm and cultivate good habits: active learning, autonomous learning, combined with clinical practice, so that students can give play to their subjective initiative.
Pathophysiology, as a bridge discipline between basic medicine and clinical medicine, plays a connecting role in medical education. Through the study of pathophysiology, students are trained to understand and deal with the scientific thinking methods of diseases, grasp the leading links and development trends of diseases, correctly use the knowledge and theory of pathophysiology, understand the essence of diseases from the outside to the inside, analyze and discuss the causes, mechanisms, functional metabolic changes and prevention and treatment principles of diseases, so as to cultivate students’ independent active learning ability, critical thinking ability and clinical thinking ability. Therefore, we adopt the teaching mode of “CBL + FC” in the teaching process. It is hoped that through the reform of this teaching mode, the autonomous learning ability and critical thinking ability of international students will be improved, and the learning of clinical cases will be used to realize the cultivation of early clinical thinking.
The results of this study show that compared with the traditional classroom, the online flipped classroom based on CBL has significantly improved the learning enthusiasm and enthusiasm of international students. Through independent literature review, PPT production, active participation in classroom teaching, learning to use a variety of learning strategies, and cooperation with other students. At the same time, the students’ ability to raise problems and solve problems has been greatly improved by analyzing clinical cases; By consulting the literature, the theoretical knowledge learned can be better applied to clinical analysis. The results of the satisfaction survey also show that international students are more likely to accept the flipped classroom teaching mode. Therefore, in view of the characteristics and needs of the international students in our university, the teaching reform of Pathophysiology adopts CBL + FC, focusing on stimulating the learning motivation and professional interests of the international students. By strengthening the evaluation of the internal and external and online learning of the international students, we can increase the independent learning ability, improve the critical thinking ability and promote personalized learning, which will be conducive to the development of the Pathophysiology course and integrate with international medical education.
This study had some limitations. Firstly, the international students’ sample size was limited, which might impact on the power of these results. Secondly, due to the impact of the epidemic, flipped classes cannot be carried out offline and face-to-face with students, which will also affect the effect of teaching reform. However, the overall effectiveness of CBL + FC can be enhanced by leveraging the unique characteristics of international students and promoting their enthusiasm for learning. Continuous evaluation and adaptation of teaching strategies are essential to ensure that all students benefit from the learning process and that the educational needs of international students are met effectively.
Through the training of this project, the students will not only have solid basic theoretical knowledge, but also have good communication ability and preliminary clinical thinking ability.
Data availability
Datasets generated or analyzed in this study are included in this published article. The Stata raw dataset can be provided on request. The corresponding author, Fang Tian, will provide additional data, if requested.
Liu Haitian D, Yichong. The current situation and development trend of studying abroad in China under the new situation. Economist. 2021; (7): 213–4.
Wang J. The characteristics of international students and the measures of teaching management. Med Edu Mgt. 2015;1(2):138–41.
Google Scholar
Dong Weijiang,Gong Huilin,Liu Wenbin,Zhou Jinsong,Si Kaiwei, Zhang Xu,Cheng Yanbin. Exploration and practice of online teachinging of basic medical courses for international students during the novel corona virus epidemic. China Med Educaion Technol. 2020;34(2):125–8.
Zhong Xi,Liu Yong. The Transformation and thoughts of Foreign Students Majoring Clinical Medicine Teaching Mode in the New Era. China Continuing Med Educ. 2021;13(15):98–103.
Graffam B. Active learning in medical education: strategies for beginning implementation. Med Teach. 2007;29(1):38–42.
Article Google Scholar
Blouin D, Tekian A. Accreditation of medical education programs: moving from student outcomes to continuous quality improvement measures. Acad Med. 2018;93(3):377–83.
McCoy L, Pettit RK, Kellar C, Morgan C. Tracking active learning in the medical school curriculum: a learning-centered approach. J Med Educ Curric Dev. 2018;5:2382120518765135.
Rose S, Hamill R, Caruso A, Appelbaum NP. Applying the Plan-Do-Study-Act cycle in medical education to refine an antibiotics therapy active learning session. BMC Med Educ. 2021;21(1):459.
Anonymous. Global minimum essential requirements in medical education. Med Teach. 2002;24(2):130–5.
Ramlaul A, Duncan D, Alltree J. The meaning of critical thinking in diagnostic radiography. Radiography. 2021;27:1166–71.
Ghazivakili Z, Nia RN, Panahi F, Karimi M, Gholsorkhi H. Zarrin Ahmadi. The role of critical thinking skills and learning styles of university students in their academic performance. J Adv Med Educ Prof. 2014;2(3):95–102.
Pu D, Ni J, Demao Song, Weiguang Zhang, Yuedan Wang,Liling Wu, Wang X, Wang Y. Influence of critical thinking disposition on the learning efficiency of problem-based learning in undergraduate medical students. BMC Medical Education. 2019;(19):1–8.
Reale,Daniel MC, Riche,Benjamin M, Witt,William A, Baker L. Development of critical thinking in health professions education: a meta-analysis of longitudinal studies. Currents Pharm Teach Learn.2018;(10):826–33.
Vanessa Arizo-Luque, Lucía Ramirez-Baena, María José Pujalte-Jesús, María Ángeles Rodríguez-Herrera, Ainhoa Lozano-Molina, Oscar Arrogante, José Luis Díaz-Agea. Does Self-Directed Learning with Simulation improve critical thinking and motivation of nursing students? A pre-post intervention study with the MAES© Methodology. Healthcare. 2022;(10):927–39.
Anja Garone B, Bruggeman B, Philipsen B, Pynoo J, Tondeur K, Struyven. Evaluating professional development for blended learning in higher education: a synthesis of qualitative evidence. Educ Inf Technol (Dordr). 2022;27(6):7599–628.
Giannakos MN, Krogstie J, Chrisochoides N. Reviewing the flipped classroom research: reflections for computer science education. In: Proceedings of the computer science education research conference.2014; (8):23–29.
Karabulut-Ilgu A, Jaramillo Cherrez N, Jahren CT. A systematic review of research on the flipped learning method in engineering education. Br J Educ Technol. 2017;(3):10–7.
O’Flaherty J, Phillips C. The use of flipped classrooms in higher education: a scoping review. Internet High Educ. 2015;(25):85–95.
Li S, Liao X, William Burdick and Kuang Tong. The effectiveness of flipped Classroom in Health professions Education in China: a systematic review. J Med Educ Curric Dev.2020;(7): 1–17.
Xiaoyu Wang,Junyi Li,Chengwei Wang. The effectiveness of flipped classroom on learning outcomes of medical statistics in a Chinese medical school. Biochem Mol Biol Educ. 2020;(48):344–9.
Ge L, Chen Y,Chunyi, Chen YZ, Liu J. Effectiveness of flflipped classroom vs traditional lectures in radiology education A meta-analysis.Medicine. 2020;(99):40–50.
Hew KF, Jia C. Gonda, and Shurui Bai.Transitioning to the new normal of learning in unpredictable times: pedagogical practices and learning performance in fully online flipped classrooms. Int J Educ Technol High Educ. 2020;17(1):57.
Melanie L, Styers, Peter A, Van Zandt, Katherine L, Hayden. Active learning in flipped Life Science Courses promotes development of critical thinking skills. CBE Life Sci Educ. 2018;17(3):39.
Umatul Khoiriyah C, Roberts C, Jorm CPM. Van Der Vleuten. Enhancing students’ learning in problem based learning: validation of a self-assessment scale for active learning and critical thinking. BMC Med Educ. 2015;(15):140.
Mumtaz MAS, Mumtaz R, Rizwan F, Nawaz Z. Nasir Javed Malik. Validation and effectiveness of Self-Assessment Scale on active learning and critical thinking (SSACT) using flipped Classroom and Journal Club Techniques. Res Square. 2023.
Li J, Li QL, Li J, Chen ML, Xie HF, Li YP, Chen X. Comparison of three problem-based learning conditions (real patients, digital and paper) with lecture-based learning in a dermatology course: a prospective randomized study from China. Med Teach. 2013;35(2):e963–70.
Morton DA, Colbert-Getz JM. Measuring the impact of the flipped anatomy classroom: the importance of categorizing an assessment by Bloom’s taxonomy. Anat Sci Educ. 2017;10(2):170–5.
O’Connor EE, Fried J, McNulty N, et al. Flipping Radiol Educ Right Side up Acad Radiol. 2016;23(7):810–22.
Aaron S, Kraut R, Omron HC-WJ, Jordan D, Manthey SJ, Wolf LM, Yarris. Stephen Johnson, Josh Kornegay. The flipped Classroom: a critical Appraisal. West J Emerg Med. 2019;20(3):527–36.
Yang F, Lin W, Wang Y. Flipped classroom combined with case-based learning is an effective teaching modality in nephrology clerkship. BMC Med Educ. 2021;(1):276.
Qian Q, Yan Y, Xue F, Lin J, Zhang F, Zhao JC. Disease 2019 (COVID-19) learning online: a flipped Classroom based on Micro-learning Combined with Case-based learning in Undergraduate Medical Students. Adv Med Educ Prac.2021;(12):835–42.
Cai L, Li YL, Hu XY, Li R. Implementation of flipped classroom combined with case-based learning: a promising and effective teaching modality in undergraduate pathology education.Medicine. (Baltimore). 2022;101(5):e28782.
Singh K, Bharatha A, Sa B, Adams OP. Majumder MAA.Teaching anatomy using an active and engaging learning strategy. BMC Med Educ. 2019;19(1):149.
Yang F, Lin W, Wang Y. Flipped classroom combined with case-based learning is an effective teaching modality in nephrology clerkship. BMC Med Educ. 2021;21(1):276.
Download references
Acknowledgements
We thank all colleagues and students who participated in this study.
Research and practice project of education and teaching reform of Zhengzhou University(special education for international students 2021ZZUJG XM-LXS004; 2022ZZUJGXM-LXS016; 2023ZZUJGXM-LXS023). Henan Medical Education Research Project (Wjlx2020355; Wjlx2021002)
Author information
Authors and affiliations.
Department of Pathophysiology, School of Basic Medical Sciences, Zhengzhou University, Zhengzhou, 450001, China
Wanjing Yang, Xiaoyan Zhang, Xinhuan Chen, Jing Lu & Fang Tian
Department of Pathology and Forensic Medicine, School of Basic Medical Sciences, Zhengzhou University, Zhengzhou, 450001, China
You can also search for this author in PubMed Google Scholar
Contributions
TF: Conceptualization; Writing original manuscript; Design of the study; Y-WJ and Z-XY: Data acquisition; Statistical analysis; Data interpretation; C-XH and LJ: Study Statistical analysis; Prepared the figures and tables; All the authors contributed to manuscript revision, read and approved the final submitted version.
Corresponding author
Correspondence to Fang Tian .
Ethics declarations
Ethics approval and consent to participate.
Oral informed consent was obtained from each student and teacher, and the study was approved by the Zhengzhou University Life Sciences Ethics Review Committee.
Consent for publication
Not applicable.
Competing interests
The authors declare no competing interests.
Additional information
Publisher’s note.
Springer Nature remains neutral with regard to jurisdictional claims in published maps and institutional affiliations.
Rights and permissions
Open Access This article is licensed under a Creative Commons Attribution 4.0 International License, which permits use, sharing, adaptation, distribution and reproduction in any medium or format, as long as you give appropriate credit to the original author(s) and the source, provide a link to the Creative Commons licence, and indicate if changes were made. The images or other third party material in this article are included in the article’s Creative Commons licence, unless indicated otherwise in a credit line to the material. If material is not included in the article’s Creative Commons licence and your intended use is not permitted by statutory regulation or exceeds the permitted use, you will need to obtain permission directly from the copyright holder. To view a copy of this licence, visit http://creativecommons.org/licenses/by/4.0/ . The Creative Commons Public Domain Dedication waiver ( http://creativecommons.org/publicdomain/zero/1.0/ ) applies to the data made available in this article, unless otherwise stated in a credit line to the data.
Reprints and permissions
About this article
Cite this article.
Yang, W., Zhang, X., Chen, X. et al. Based case based learning and flipped classroom as a means to improve international students’ active learning and critical thinking ability. BMC Med Educ 24 , 759 (2024). https://doi.org/10.1186/s12909-024-05758-8
Download citation
Received : 08 September 2023
Accepted : 09 July 2024
Published : 15 July 2024
DOI : https://doi.org/10.1186/s12909-024-05758-8
Share this article
Anyone you share the following link with will be able to read this content:
Sorry, a shareable link is not currently available for this article.
Provided by the Springer Nature SharedIt content-sharing initiative
- Case-based learning
- Flipped classroom
- Active learning
- Critical thinking
- International students
BMC Medical Education
ISSN: 1472-6920
- Submission enquiries: [email protected]
- General enquiries: [email protected]
- Open access
- Published: 11 July 2024
Machine learning to optimize literature screening in medical guideline development
- Wouter Harmsen 1 ,
- Janke de Groot 1 ,
- Albert Harkema 2 ,
- Ingeborg van Dusseldorp 1 ,
- Jonathan de Bruin 3 ,
- Sofie van den Brand 2 &
- Rens van de Schoot ORCID: orcid.org/0000-0001-7736-2091 2
Systematic Reviews volume 13 , Article number: 177 ( 2024 ) Cite this article
319 Accesses
1 Altmetric
Metrics details
In a time of exponential growth of new evidence supporting clinical decision-making, combined with a labor-intensive process of selecting this evidence, methods are needed to speed up current processes to keep medical guidelines up-to-date. This study evaluated the performance and feasibility of active learning to support the selection of relevant publications within medical guideline development and to study the role of noisy labels.
We used a mixed-methods design. Two independent clinicians’ manual process of literature selection was evaluated for 14 searches. This was followed by a series of simulations investigating the performance of random reading versus using screening prioritization based on active learning. We identified hard-to-find papers and checked the labels in a reflective dialogue.
Main outcome measures
Inter-rater reliability was assessed using Cohen’s Kappa ( ĸ ). To evaluate the performance of active learning, we used the Work Saved over Sampling at 95% recall (WSS@95) and percentage Relevant Records Found at reading only 10% of the total number of records (RRF@10). We used the average time to discovery (ATD) to detect records with potentially noisy labels. Finally, the accuracy of labeling was discussed in a reflective dialogue with guideline developers.
Mean ĸ for manual title-abstract selection by clinicians was 0.50 and varied between − 0.01 and 0.87 based on 5.021 abstracts. WSS@95 ranged from 50.15% (SD = 17.7) based on selection by clinicians to 69.24% (SD = 11.5) based on the selection by research methodologist up to 75.76% (SD = 12.2) based on the final full-text inclusion. A similar pattern was seen for RRF@10, ranging from 48.31% (SD = 23.3) to 62.8% (SD = 21.20) and 65.58% (SD = 23.25). The performance of active learning deteriorates with higher noise. Compared with the final full-text selection, the selection made by clinicians or research methodologists deteriorated WSS@95 by 25.61% and 6.25%, respectively.
While active machine learning tools can accelerate the process of literature screening within guideline development, they can only work as well as the input given by human raters. Noisy labels make noisy machine learning.
Peer Review reports
Introduction
Producing and updating trustworthy medical guidelines is a deliberative process that requires a substantial investment of time and resources [ 1 ]. In the Netherlands, medical guidelines in specialist medical care are being developed and revised in co-production between clinicians and guideline methodologists. There are over 650 medical specialists’ guidelines in the Netherlands, answering approximately 12,000 clinical questions. An essential element in guideline development is a systematic synthesis of the evidence. This systematic appraisal includes the formulation of clinical questions, selection of relevant sources, a systematic literature review, grading the certainty of the body of evidence using GRADE [ 2 ], and finally, translating the evidence into recommendations for clinical practice [ 3 ].
Evidence synthesis starts with translating a clinical question into a research question. Hereafter, a medical information specialist systematically searches literature in different databases. Then, literature screening is performed independently by two clinicians who label relevant publications based on inclusion and exclusion criteria in the title or abstract. Once the relevant publications have been selected, a guideline methodologist with more experience in systematically selecting relevant publications from large datasets supports further title-abstract selection, assessing the methodological quality of the selected papers. Literature screening is time-consuming, with an estimated 0.9 and 7 min per reference per reviewer for abstract and full-text screening, respectively. Since a single literature search can easily result in hundreds to thousands of publications, this can add up to 100–1000 min of selection based on title and abstract and even more so for full-text selection [ 4 ]. In an era of exponential growth of new evidence, combined with a labor-intensive process, there is a need for methods to speed up current processes to keep medical guidelines up-to-date.
The rapidly evolving field of artificial intelligence (AI) has allowed the development of tools that assist in finding relevant texts for search tasks [ 5 , 6 , 7 , 8 , 9 , 10 , 11 , 12 , 13 , 14 , 15 , 16 ]. A well-established approach to increasing the efficiency of title and abstract screening is screening prioritization [ 17 ] via active learning [ 18 ]. With machine learning models, relevance scores for each publication can be computed. Then, assessors label titles and abstracts (relevant versus irrelevant) for each most relevant record, and the model iteratively updates its predictions based on the given labels and prioritizes articles that are most likely to be relevant. Active learning is found to be extremely effective for systematic reviewing (see for a systematic review [ 19 ]).
Implementing active learning could save a tremendous amount of work and time and may open a new window of opportunity in the context of evidence-based guideline development. However, active learning works under the strong assumption that given labels are correct [ 20 ]. While research with experienced reviewers may be straightforward, working with clinical questions and clinicians in the daily practice of guideline development may be more complex. Most clinicians are not experienced with title-abstract selection and often perform this task in addition to their daily work in the clinic. With large numbers of abstracts and limited time, clinicians can become distracted or fatigued, introducing variability in the quality of their annotations. This variability in human performance may hinder the applicability of active learning in guideline development. Given the potential of active learning and the more complex context of guideline development, this practice-based study aimed to evaluate the performance and feasibility of active learning to support literature screening within the context of guideline development.
We aim to evaluate the added value of using active learning and the impact of noisy labels during three stages of the review process: (1) title abstract selection by clinicians, (2) additional title abstract selection by experienced research methodologists, and (3) final full-text inclusions after expert consensus. In what follows, we present the 14 datasets used and the workflow for manual literature screening in guideline development and introduce the setup of active learning. This is followed by a simulation study mimicking the screening process for the 14 clinical questions, comparing the performance of literature screening using active learning versus manual selection in terms of work saved over sampling and the average time to discovery for identifying hard-to-find papers potentially having a noisy label [ 21 , 22 ]. We then present the results of the discussion of the hard-to-find papers in a reflective dialogue with the research methodologists and evaluate reasons that facilitate or hamper the performance of active learning.
We selected 14 clinical questions from recently published clinical guidelines containing manually labeled datasets, providing a wide range of types and complexity of clinical questions; see Table 1 . The datasets were derived from different guidelines published between 2019 and 2021, covering different types of questions, e.g., diagnostic, prognostic, and intervention types of questions. In order to be sure that the guidelines had been authorized and thus finished, we selected those that are openly published on the Dutch Medical Guideline Database [Richtlijnendatabase.nl]. Per clinical question, two clinicians independently labeled title-abstracts using prespecified inclusion and exclusion criteria. The datasets contained (at least) the papers’ title and abstract plus the labels relevant/irrelevant for each annotator (clinician and research methodologist) and the column with the final inclusion. Duplicates and papers with missing abstracts were removed from the dataset. All datasets can be found on the Open Science Framework page of the project: https://osf.io/vt3n4/ .
Manual screening
To evaluate inter-rater reliability for the manual literature screening, we used Cohen’s Kappa index measure [ 23 ]. Cohen’s Kappa gives relevant information on the amount of consensus among different raters with higher scores indicating better interrater agreement.
Utilizing the labeled datasets, we conducted various simulation studies to explore the intricacies of model performance. Each simulation emulates the screening process using a specific model, guiding the algorithm through the dataset according to predefined strategies using a specific active learning model. The performance is typically evaluated by randomly screening a labeled dataset. This setup allows the simulation to replicate the screening process, akin to a researcher conducting AI-assisted screening, thereby providing a realistic representation of how the model would perform in practical applications. These simulations are distinct from traditional statistical simulation studies in several key aspects. Firstly, the primary objective of our simulations is to evaluate the efficacy of AI algorithms in literature screening. This is in contrast to typical statistical simulations, which often focus on assessing theoretical statistical properties such as power, bias, or variance under various hypothetical scenarios. Also, we make use of real-world, labeled datasets, diverging from the standard practice in statistical simulations that frequently rely on hypothetical or synthetically generated data. This use of actual data from literature ensures a more practical and application-oriented assessment of the model’s performance.
The simulations were conducted with the command line interface of ASReview version v0.16 [ 24 ]. ASReview has been proven to be a valid tool for the selection of literature in numerous studies [ 15 , 21 , 25 , 26 , 27 , 28 , 29 , 30 , 31 , 32 , 33 ]. We used Naïve Bayes as the classifier for the simulation study with TF-IDF as the feature extraction technique.
In our study, each dataset underwent simulations targeting different sets of relevant records as defined by three groups: (1) clinicians, (2) a combination of clinicians and research methodologists, and (3) the final inclusion decisions. We included one relevant record as a prior inclusion for each simulation’s training data, along with ten randomly selected irrelevant records. We conducted multiple runs for each dataset to mitigate the potential bias introduced by the starting paper in the model’s first iteration, varying the relevant paper used in the initial training. The outcomes are averaged over these runs to ensure a balanced assessment. Consistency was maintained within each run of a dataset by using the same ten irrelevant records.
We analyzed the model performance of active learning by calculating the following three outcome measures: the Work Saved over Sampling (WSS), which indicates the reduction in publications needed to be screened at a given level of recall [ 17 ]. The WSS is typically measured at a recall level of 95%; WSS@95 reflects the amount of work saved by using active learning at the cost of failing to identify 5% of relevant publications. Note that humans typically misclassify about 10% [ 34 ]. Secondly, we computed the metric Relevant Records Found (RRF), which represents the proportion of relevant publications that are found after screening a prespecified percentage of all publications. Here, we calculated RRF@10, which represents the percentage of relevant publications found after screening only 10% of all publications. Thirdly, we calculate the average time to discovery (ATD) [ 21 ] and the fraction of non-reviewed relevant publications during the review (except the relevant publications in the initial dataset). The ATD is an indicator of the performance throughout the entire screening process instead of performance at some arbitrary cutoff value. The ATD is computed by taking the average of the time to discovery (TD) of all relevant publications. The TD for a given relevant publication i is computed as the fraction of publications needed to screen to detect i . We used this metric to identify hard-to-find papers potentially having a noisy label.
We also plotted recall curves to visualize model performance throughout the entire simulation. Recall curves give information in two directions; they display the number of publications that need to be screened and the number of relevant publications.
All scripts to reproduce the simulations are available at https://doi.org/10.5281/zenodo.5031390
Reflective dialogue
In order to better understand the differences in performance across the different datasets, we organized a reflective dialogue. In a two 3.5-h session, seven research methodologists who initially labeled the datasets critically appraised the quality of the labeled datasets in light of the performance. Specifically, we wanted to know why some publications were found very easy and others more difficult to zoom in on the hard-to-find papers to identify possible noisy labels.
The selected datasets encompass seven different medical fields, addressing intervention, diagnostic, and prognostic types of questions. Table 1 details the datasets by guideline topic, medical specialty, type of question, number of abstracts screened, minute screening time, and Cohen’s Kappa ( ĸ ) for interrater agreement.
In our study, twenty-four clinicians independently screened a total of 5021 abstracts across all datasets. From these, they identified 339 potentially relevant publications, which required 3766 min of screening time. The mean ĸ for interrater agreement across all datasets was 0.50, with individual values ranging from − 0.01 to 0.87, as detailed in Table 1 for each specific dataset.
Out of the 339 publications initially identified as relevant by clinicians, the research methodologists excluded 166 (49%) due to methodological concerns. A further 45 (13.3%) were excluded after full-text review, leaving 128 publications for final full-text inclusion. Table 1 also reflects these figures, presenting a breakdown of the initial abstract screening results for each of the 14 purposefully selected datasets, including the specific medical specialty and question type they pertain to.
The simulation study results are summarized in Table 2 , presenting a comprehensive analysis of datasets labeled by clinicians and research methodologists following full-text selection.
It showed that the Work Saved over Sampling (WSS@95) was lowest for clinicians and ranged from 32.31 to 97.99%, with a mean of 50.15% (SD = 17.74); followed by the research methodologist, it ranged from 45.34 to 95.7%, with a mean of 69.24% (SD = 11.51); and simulating the full-text inclusions resulted in the highest WSS@95 that ranged from 61.41 to 96.68% (0.92), with a mean of 75.76% (SD = 12.16).
A similar pattern emerged for RRF@10 which, for clinicians, ranged from 28.10 to 100%, with a mean of 48.31% (SD = 23.32); for the research methodologist, it ranged from 25.00 to 100%, with a mean of 62.78% (SD = 21.20); and simulating full-text inclusions gave an RRF@10 that ranged from 20.00 to 100% (0.92), with a mean of 65.58% (SD = 23.25). ATD Ranged from screening 20 to 62 abstracts.
Figure 1 presents recall curves for all simulations, and as can observed, the recall curves differ across datasets but always outperform randomly reading the records, which is the standard approach.
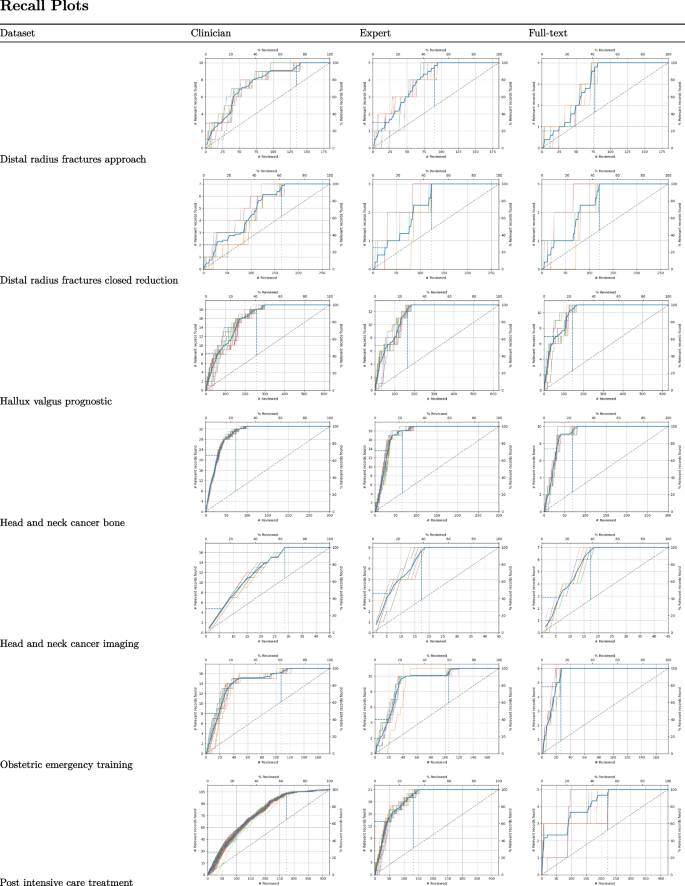
Recall plots of the simulated datasets. The first row indicates the number of relevant records found for each simulation run as displayed as a function of the number of records screened for each of the three levels (clinician, guideline methodologist, final decision). The vertical line indicates when 95% of the relevant records have been found. The Y -axis presents the number of relevant papers minus one paper selected for training data. Note that the simulation for the dataset Shoulder_replacement_diagnostic shows no recall lines because only one relevant paper was included, and at least two relevant records are needed for a simulation study
To illustrate these results in a more tangible context, we discuss one dataset in detail: Distal_radius_fractures_approach . Out of the 195 records identified in the search, 11 (5.64%) were indicated as relevant by the clinicians, 6 (3.08%) by the guideline methodologist, and, ultimately, only 5 (2.56%) were included in the final protocol. Zooming in on WSS@95 for full-text inclusions, on average, after screening 43% of the records ( n = 83), all records (5 out of 5) would have been found. If one screened records in a random order, at this point, one would have found 3 of the relevant records, and finding 5 of the relevant records would take, on average, 186 records. In other words, the time that can be saved using active learning expressed as the percentage of records that do not have to be screened is 61% (sd = 5.43), while still identifying 95% of the relevant records. The RRF@10 is 20% (sd = 11.18), meaning that after screening 10% of records, 20% of the relevant records have been identified.
During these sessions, we reflected on the current progress of selecting relevant publications and how this affected some of the difficulties in active learning. The discussion during the reflective dialogue revealed that almost half (= 49%) of the selected publications by the clinicians did not meet the predefined inclusion criteria, e.g., PICO criteria or study design, and were, therefore, later re-labeled as irrelevant by the research methodologists.
In this reflective dialogue, we also discussed the performance of active learning in specific datasets. While for some active learning seemed to be hindered by incorrect inclusion by the clinicians, in other samples, active learning had difficulty due to the structure of the abstracts from studies other than RCTs. For example, the recall plots for the dataset Distal_radius_fractures_approach showed that the clinicians identified five papers as relevant, which were later deemed irrelevant by the guideline methodologists. Methodologists mentioned how clinicians would often include studies for other reasons (interesting to read, not daring to exclude, or not knowing the exact inclusion criteria). This led to the mention of the “noisy labels” for inclusions that should not have been included in the first place. In the current manual process, these are excluded by the methodologist, which takes extra time. For other datasets (i.e., Shoulder_replacement_surgery , Total_knee_replacement , and Shoulder_dystocia_positioning ), active learning seems to have difficulty in finding systematic reviews and observational studies compared to randomized control trials. As discussed, this may be inherent to the way the abstracts are structured, e.g., RCTs often describe a strict comparison, while this may be less evident for systematic reviews and observational studies.
The purpose of this practice-based study was to evaluate the performance and feasibility of active learning to support the selection of relevant publications within the context of guideline development. Although ASReview has been proven to be a valid tool for the selection of literature in numerous studies [ 15 , 21 , 25 , 26 , 27 , 28 , 29 , 30 , 31 , 32 , 33 ], none tested the performance on medical guidelines. We evaluated the performance of active learning on labeled datasets from 14 clinical questions across the three different stages of the review process. The simulations show a considerable variation in the reduction of papers needed to be screened (13–98%). The variation is caused by the clearness and coherence of the abstracts, the specificity of the inclusion and exclusion criteria, and whether the information for full-text inclusion is actually present in the abstract. On average, however, when active learning models were used, the WSS@95 was 50% for the screening done by clinicians. After additional assessment by an experienced research methodologist, the average WSS@95 increased to 69%, with a further increase to 75% after final full-text inclusion. This means that the performance of active learning increases with more accurate title-abstract labeling, which underlines the importance of strict inclusion and exclusion criteria.
The results of the reflective dialogue emphasize that in the current way of selection, inclusion and exclusion by clinicians in guideline development is not always as straightforward as in systematic reviews by researchers. Our results align with the hypothesis that active learning works under the strong assumption that given labels are correct [ 20 ]. During our reflective dialogue session, the notion of “noisy labels” was introduced for the initial screening process. This notion was confirmed in the low to moderate interrater reliability of the manual title-abstract screening, with an average kappa of 0.5 in line with other recent findings [ 35 ]. Therefore, our main conclusion is that active learning models can speed up the process of literature screening within guideline development but, at the same time, assume correct labels of inclusion and exclusion, as our data showed that performance was dependent on the quality of the annotations.
Our next question, therefore, was to find the “noise” in the manual screening process. Some interesting themes emerged when looking at the differences between the selections made by the clinicians and the professional guideline developers. Guideline methodologists realized that clinicians often include publications based on the PICO criteria and out of personal interest or fear of leaving out important data. Indeed, when re-examined, many articles did not fall within the PICO criteria or the predefined criteria regarding methodological concerns (e.g., RCT vs. case–control studies or cohort studies). On average, there was a 49% drop in inclusion when the guideline methodologist re-evaluated the original inclusion made by the clinicians.
A question of interest for future study is when to trust that all relevant literature on the topic has been retrieved based on our results and others. In this study, we plotted recall curves to visualize active learning performance and organized discussion meetings to reason why some publications were more difficult to find. Looking at the examples, this often happened when the search had followed a slightly different process. In the current workflow, due to limited resources, pragmatic choices are being made not to include all individual studies when a recent systematic review is available. For active learning models, it takes time to “learn” this adapted (non-logical) strategy. For instance, plateaus occur in some of the recall plots, and after a series of irrelevant records have been identified, a new relevant record was found. Interestingly, when time is saved by working with active learning tools, these pragmatic choices might not be necessary anymore and may lead to a much larger and more complete set of inclusions than the manual workflow.
Strengths and weaknesses
While an obvious weakness concerns the number of datasets included, in this study, we did not cover all types of clinical questions, and our findings are mainly based on intervention types of questions. On the other hand, a strength of this study is that we evaluated the daily practice of guideline development using real-world data from previously developed guidelines. While studies are reporting on tools implementing active learning in systematic reviews, there is little evidence of implementing such tools in daily practice [ 36 , 37 , 38 ]. Our “real world” data provided us with new challenges not seen before because it is frequently tested in research settings without going back to the initial screeners, leaving out more pragmatic and human-interest choices that influence literature screening.
This type of practice-based study has shown potential ways to use and improve current practice. In our sample, active learning detected the most relevant studies with a significant reduction in the number of abstracts that needed to be screened. The system performed better when the inclusion and exclusion criteria were adhered to more strictly. The findings brought us to look at the workflow needing more attention to guide the clinicians in the systematic selection of papers. This is beneficial not only when using software like ASReview, where the principle of “quality in, quality out” seems to apply, but also when using the manual selection of papers. After abstract screening, almost half of the inclusions were incorrect, which is higher than the error rates reported in systematic reviews, with a mean error rate of nearly 11% over 25 systematic reviews [ 34 ]. Methods to improve literature selection have been described [ 39 , 40 ] and include recommendations to include reflection and group discussion, resulting in a more iterative process, practical tips like taking regular breaks and coding in small batches at a time to prevent fatigue, but also setting up unambiguous inclusion criteria and adjusting the codebook during the process if needed. While the inclusion of two independent reviewers is often assumed to be the best way to reduce bias, these authors also advise regularly assessing interrater reliability as part of reflective and learning practice.
We also defined some remaining questions for future research. As described above, in guideline development, research questions do not always yield prior inclusion papers, while the performance of active learning partially depends on at least one relevant starting paper to learn from. A possible solution that needs to be explored might be to start with a dummy abstract containing all relevant elements from the PICO. At the same time, we need more samples of research questions in clinical guidelines to further evaluate the use of AI tools in different questions and contexts. In this study, we evaluated a limited set of retrospective data using one active learning algorithm, and future studies could explore more datasets, different active learning algorithms, or different tools in different phases of the process of guideline development to evaluate further the human–machine interaction and how this affects the process of guideline development.
Conclusions
This study shows a 50–75% reduction in abstracts that needed to be screened to find and select all relevant literature for inclusion in medical guidelines when using active learning models. At the same time, this study also shows the importance of the quality of the human input as it directly relates to the performance of active machine learning. The next step would be to evaluate how to apply active learning in the workflow of guideline development, how to improve human input, and what it means for both the timeframe to develop new recommendations and the transparency and quality of these evidence-based recommendations.
AI statement
During the research and the creation of this research paper, AI-based tools like Grammarly, for grammar and language mechanics and OpenAI’s ChatGPT, for code creation and content review, were utilized. These were supplementary aids, and all final interpretations and content decisions were the sole responsibility of the authors.
Availability of data and materials
All scripts that were used during this study, including preprocessing, analyzing, and simulation scripts for results, figures, and tables published in this paper, can be found on the GitHub page of the project: https://doi.org/10.5281/zenodo.5031390 . The 14 systematic review datasets are openly available on the Open Science Framework https://osf.io/vt3n4/
Graham R, Mancher M, Wolman DM, Greenfield S, Steinberg E. Committee on standards for developing trustworthy clinical practice guidelines; institute of medicine. Clinical Practice Guidelines We Can Trust | The National Academies Press. 2011. https://doi.org/10.17226/13058 .
Guyatt GH, Oxman AD, Vist GE, Kunz R, Falck-Ytter Y, Alonso-Coello P, et al. GRADE: an emerging consensus on rating quality of evidence and strength of recommendations. BMJ. 2008;336(7650):924–6.
Article PubMed PubMed Central Google Scholar
Guyatt GH, Oxman AD, Kunz R, Falck-Ytter Y, Vist GE, Liberati A, et al. Going from evidence to recommendations. BMJ. 2008;336(7652):1049–51.
Wang Z, Asi N, Elraiyah TA. others Dual computer monitors to increase efficiency of conducting systematic reviews. J Clin Epidemiol. 2014;67:1353–7.
Adam GP, Wallace BC, Trikalinos TA. Semi-automated tools for systematic searches. In: Evangelou E, Veroniki AA, editors. Meta-Research [Internet]. New York, NY: Springer US; 2022 [cited 2024 Jan 10]. p. 17–40. (Methods in Molecular Biology; vol. 2345). Available from: https://link.springer.com/ https://doi.org/10.1007/978-1-0716-1566-9_2
Cierco Jimenez R, Lee T, Rosillo N, Cordova R, Cree IA, Gonzalez A, et al. Machine learning computational tools to assist the performance of systematic reviews: a mapping review. BMC Med Res Methodol. 2022;22(1):322.
Cowie K, Rahmatullah A, Hardy N, Holub K, Kallmes K. Web-based software tools for systematic literature review in medicine: systematic search and feature analysis. JMIR Med Inform. 2022;10(5):e33219.
Harrison H, Griffin SJ, Kuhn I, Usher-Smith JA. Software tools to support title and abstract screening for systematic reviews in healthcare: an evaluation. BMC Med Res Methodol. 2020;20(1):7.
Khalil H, Ameen D, Zarnegar A. Tools to support the automation of systematic reviews: a scoping review. J Clin Epidemiol. 2022;144:22–42.
Article PubMed Google Scholar
Nieto González DM, Bustacara Medina CJ. Optimización de estrategias de búsquedas científicas médicas utilizando técnicas de inteligencia artificial [Internet]. Pontificia Universidad Javeriana; 2022 [cited 2024 Jan 10]. Available from: https://repository.javeriana.edu.co/handle/10554/58492
O’Mara-Eves A, Thomas J, McNaught J, Miwa M, Ananiadou S. Using text mining for study identification in systematic reviews: a systematic review of current approaches. Syst Rev. 2015;4(1):5.
Pellegrini M, Marsili F. Evaluating software tools to conduct systematic reviews: a feature analysis and user survey. Form@re. 2021;21(2):124–40.
Article Google Scholar
Robledo S, Grisales Aguirre AM, Hughes M, Eggers F. “Hasta la vista, baby” – will machine learning terminate human literature reviews in entrepreneurship? J Small Bus Manage. 2023;61(3):1314–43.
Scott AM, Forbes C, Clark J, Carter M, Glasziou P, Munn Z. Systematic review automation tools improve efficiency but lack of knowledge impedes their adoption: a survey. J Clin Epidemiol. 2021;138:80–94.
van de Schoot R, de Bruin J, Schram R, Zahedi P, de Boer J, Weijdema F, et al. An open source machine learning framework for efficient and transparent systematic reviews. Nat Mach Intell. 2021;3(2):125–33.
Wagner G, Lukyanenko R, Paré G. Artificial intelligence and the conduct of literature reviews. J Inf Technol. 2022;37(2):209–26.
Cohen AM, Ambert K, McDonagh M. Cross-topic learning for work prioritization in systematic review creation and update. J AmMed Inform Assoc. 2009;16:690–704.
Settles B. Active Learning. Vol. 6. Synthesis lectures on artificial intelligence and machine learning; 2012. https://doi.org/10.1007/978-3-031-01560-1 .
Teijema JJ, Seuren S, Anadria D, Bagheri A, van de Schoot R. Simulation-based active learning for systematic reviews: a systematic review of the literature. 2023. https://doi.org/10.31234/osf.io/67zmt .
Ipeirotis PG, Provost F, Sheng VS, Wang J. Repeated labeling using multiple noisy labelers. Data Min Knowl Disc. 2014;28(2):402–41.
Ferdinands G, Schram R, de Bruin J, Bagheri A, Oberski DL, Tummers L, et al. Performance of active learning models for screening prioritization in systematic reviews: a simulation study into the Average Time to Discover relevant records. Syst Rev. 2023;12(1):100.
Byrne F, et al. Impact of Active learning model and prior knowledge on discovery time of elusive relevant papers: a simulation study. Syst Rev. 2024. https://doi.org/10.1186/s13643-024-02587-0 .
Cohen J. A coefficient of agreement for nominal scales. Educ Psychol Measur. 1960;20(1):37–46.
ASReview LAB developers. ASReview LAB - a tool for AI-assisted systematic reviews [Internet]. Zenodo; 2024 [cited 2024 Jan 12]. Available from: https://zenodo.org/doi/10.5281/zenodo.3345592
Campos DG, Fütterer T, Gfrörer T, Lavelle-Hill RE, Murayama K, König L, et al. Screening smarter, not harder: a comparative analysis of machine learning screening algorithms and heuristic stopping criteria for systematic reviews in educational research. 2023;36. https://doi.org/10.1007/s10648-024-09862-5 .
Ferdinands G. AI-assisted systematic reviewing: selecting studies to compare Bayesian versus Frequentist SEM for small sample sizes. Multivariate Behav Res. 2021;56:153–4.
Nedelcu A, Oerther B, Engel H, Sigle A, Schmucker C, Schoots IG, et al. A machine learning framework reduces the manual workload for systematic reviews of the diagnostic performance of prostate magnetic resonance imaging. Eur Urol Open Sci. 2023;56:11–4.
Oude Wolcherink MJ, Pouwels X, van Dijk SHB, Doggen CJM, Koffijberg H. Can artificial intelligence separate the wheat from the chaff in systematic reviews of health economic articles? Expert Rev Pharmacoecon Outcomes Res. 2023;23(9):1049–56.
Article CAS PubMed Google Scholar
Pijls BG. Machine learning assisted systematic reviewing in orthopaedics. J Orthop. 2023;48:103-106. Published 2023. https://doi.org/10.1016/j.jor.2023.11.051 .
Romanov S. Optimising ASReview simulations: a generic multiprocessing solution for ‘light-data’ and ‘heavy-data’ users. 2023. Data Intell. 2024. https://doi.org/10.1162/dint_a_00244 .
Scherhag J, Burgard T. Performance of semi-automated screening using Rayyan and ASReview: a retrospective analysis of potential work reduction and different stopping rules. Big Data & Research Syntheses 2023, Frankfurt, Germany. 2023. In: ZPID (Leibniz Institute for Psychology); 2023. https://doi.org/10.23668/psycharchives.12843 .
Teijema JJ, de Bruin J, Bagheri A, van de Schoot R. Large-scale simulation study of active learning models for systematic reviews. 2023. https://doi.org/10.31234/osf.io/2w3rm
Teijema JJ, Hofstee L, Brouwer M, de Bruin J, Ferdinands G, de Boer J, et al. Active learning-based systematic reviewing using switching classification models: the case of the onset, maintenance, and relapse of depressive disorders. Front Res Metrics Analytics. 2023;8:1178181.
Wang Z, Nayfeh T, Tetzlaff J, O’Blenis P, Murad MH. Error rates of human reviewers during abstract screening in systematic reviews. Bencharit S, editor. PLoS ONE. 2020;15(1):e0227742.
Pérez J, Díaz J, Garcia-Martin J, Tabuenca B. Systematic literature reviews in software engineering—enhancement of the study selection process using Cohen’s Kappa statistic. J Syst Softw. 2020;168:110657.
O’Connor AM, Tsafnat G, Gilbert SB, Thayer KA, Wolfe MS. Moving toward the automation of the systematic review process: a summary of discussions at the second meeting of International Collaboration for the Automation of Systematic Reviews (ICASR). Syst Rev. 2018;7(1):3.
O’Connor AM, Tsafnat G, Thomas J, Glasziou P, Gilbert SB, Hutton B. A question of trust: can we build an evidence base to gain trust in systematic review automation technologies? Syst Rev. 2019;8(1):143.
van Altena AJ, Spijker R, Olabarriaga SD. Usage of automation tools in systematic reviews. Res Synth Methods. 2019;10(1):72–82.
Ali NB, Petersen K. Evaluating strategies for study selection in systematic literature studies. In: Proceedings of the 8th ACM/IEEE International Symposium on Empirical Software Engineering and Measurement [Internet]. Torino Italy: ACM; 2014 [cited 2024 Jan 12]. p. 1–4. Available from: https://dl.acm.org/doi/ https://doi.org/10.1145/2652524.2652557
Belur J, Tompson L, Thornton A, Simon M. Interrater reliability in systematic review methodology: exploring variation in coder decision-making. Sociol Methods Res. 2021;50(2):837–65.
Download references
This project was funded by the Dutch Research Council in the “Corona: Fast-track data” (2020/SGW/00909334) and by ZonMw (project 516022528).
Author information
Authors and affiliations.
Knowlegde Institute for the Federation of Medical Specialists, Utrecht, The Netherlands
Wouter Harmsen, Janke de Groot & Ingeborg van Dusseldorp
Department of Methodology and Statistics, Faculty of Social and Behavioral Sciences, Utrecht University, Utrecht, The Netherlands
Albert Harkema, Sofie van den Brand & Rens van de Schoot
Department of Research and Data Management Services, Information Technology Services, Utrecht University, Utrecht, the Netherlands
Jonathan de Bruin
You can also search for this author in PubMed Google Scholar
Contributions
Conceptualization: WH, JdG, IvD, RvdS.
Methodology: WH, RvdS, JdB.
Software: AH, SvdB, JdB, RvdS.
Validation: AH, SvdB.
Formal analysis: WH, AH, SvdB.
Resources: JB.
Data curation: WH, JdG, IvD, SvdB.
Writing—original draft: WH.
Writing—review and editing: JdG, IvD, RvdS.
Visualization: AH, SvdB.
Supervision: JdG, RvdS.
Funding acquisition: RvdS, JdG.
Corresponding author
Correspondence to Rens van de Schoot .
Ethics declarations
Competing interests.
The authors declare that they have no competing interests.
Additional information
Publisher’s note.
Springer Nature remains neutral with regard to jurisdictional claims in published maps and institutional affiliations.
Rights and permissions
Open Access This article is licensed under a Creative Commons Attribution 4.0 International License, which permits use, sharing, adaptation, distribution and reproduction in any medium or format, as long as you give appropriate credit to the original author(s) and the source, provide a link to the Creative Commons licence, and indicate if changes were made. The images or other third party material in this article are included in the article's Creative Commons licence, unless indicated otherwise in a credit line to the material. If material is not included in the article's Creative Commons licence and your intended use is not permitted by statutory regulation or exceeds the permitted use, you will need to obtain permission directly from the copyright holder. To view a copy of this licence, visit http://creativecommons.org/licenses/by/4.0/ . The Creative Commons Public Domain Dedication waiver ( http://creativecommons.org/publicdomain/zero/1.0/ ) applies to the data made available in this article, unless otherwise stated in a credit line to the data.
Reprints and permissions
About this article
Cite this article.
Harmsen, W., de Groot, J., Harkema, A. et al. Machine learning to optimize literature screening in medical guideline development. Syst Rev 13 , 177 (2024). https://doi.org/10.1186/s13643-024-02590-5
Download citation
Received : 21 June 2022
Accepted : 20 June 2024
Published : 11 July 2024
DOI : https://doi.org/10.1186/s13643-024-02590-5
Share this article
Anyone you share the following link with will be able to read this content:
Sorry, a shareable link is not currently available for this article.
Provided by the Springer Nature SharedIt content-sharing initiative
- Guideline development
- Active learning
- Machine learning
- Systematic reviewing
Systematic Reviews
ISSN: 2046-4053
- Submission enquiries: Access here and click Contact Us
- General enquiries: [email protected]
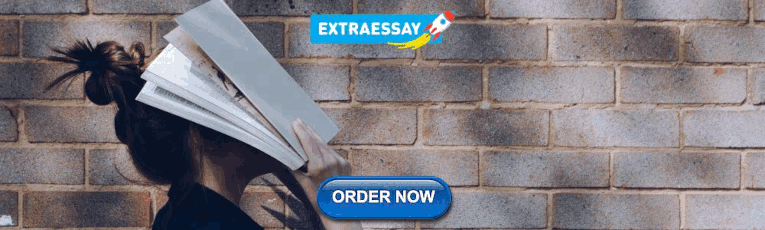
IMAGES
VIDEO
COMMENTS
This article reviews the effectiveness of online learning courses for higher education using meta-analysis, and discusses the factors and challenges that influence online learning outcomes.
Research highlights certain dearth such as the weakness of online teaching infrastructure, the limited exposure of teachers to online teaching, the information gap, non-conducive environment for learning at home, equity and academic excellence in terms of higher education.
Online learning research was categorized into twelve themes and a framework across learner, course and instructor, and organizational levels was developed. Online learner characteristics and online engagement were examined in a high number of studies and were consistent with three of the prior systematic reviews.
Implications for policymakers are that many teachers need intensive professional development about digital technology. INTRODUCTION This systematic review of the research literature on online and blended learning from schools starts by outlining recent perspectives on emergency remote learning, as occurred during the Covid-19 pandemic.
Through a literature review of the factors affecting adoption, the continuation of technology use, and learning outcomes, this paper discusses an integration of online learning with virtual communities to foster student engagement for obtaining better learning outcomes.
Abstract Since most schools and learners had no choice but to learn online during the pandemic, online learning became the mainstream learning mode rather than a substitute for traditional face-to-face learning. Given this enormous change in online learning, we conducted a systematic review of 191 of the most recent online learning studies published during the COVID-19 era. The systematic ...
Online learning as a concept and as a keyword has consistently been a focus of education research for over two decades. In this paper, we present results from a systematic literature review for the...
Abstract and Figures In this introduction to the special issue on systematic reviews of research on online learning, we introduce the need for systematic reviews on online learning.
Research highlights certain dearth such as the weakness of online teaching infrastructure, the limited exposure of teachers to online teaching, the information gap, non-conducive environment for learning at home, equity and academic excellence in terms of higher education. This article evaluates the impact of the COVID-19 pandemic on teaching and learning process across the world. The ...
This literature review has focused on the factors that affect students' learning experiences in e-learning, online learning and blended learning in higher education, with particular emphasis on professional education and teacher training.
This paper first presents a review of the existing literature, focusing on the impact of the pandemic on online learning and discussing the nine significant factors influencing online learning ...
Abstract The COVID-19 pandemic has forced online learning to be a "new normal" during the past three years, which highly emphasizes students' improved digital literacy. This study aims to present a literature review of students' digital literacy.
Therefore, in this paper we present a systematic literature review (SLR) (Kitchenham and Charters 2007) on the confluence of e-learning and organizational learning that uncovers initial findings on the value of e-learning to support organizational learning while also delineating several promising research streams.
A Systematic Literature Review of Definitions of Online Learning (1988-2018) | Online learning as a concept and as a keyword has consistently been a focus of education research for over two decades.
This article reports on a large-scale (n = 987), exploratory factor analysis study incorporating various concepts identified in the literature as critical success factors for online learning from the students' perspective, and then determines their hierarchical significance. Seven factors--Basic Online Modality, Instructional Support, Teaching Presence, Cognitive Presence, Online Social ...
Abstract This study compares university students' performance in traditional learning to that of online learning during the pandemic, and analyses the implications of the shift to online learning from a faculty's perspective.
They also had to create learning environments for student teachers doing their preparation in the light of the requirements of teacher education programmes and the conditions in which both universities and schools had to operate. This paper provides a review of the literature on online teaching and learning practices in teacher education.
Abstract. Online learning refers to instruction that is delivered electronically through various multimedia and Internet platforms and applications. It is used interchangeably with other terms such as web-based learning, e-learning, computer-assisted instruction, and Internet-based learning. This chapter includes a review of the literature ...
Given this enormous change in online learning, we conducted a systematic review of 191 of the most recent online learning studies published during the COVID-19 era.
This literature review explores the research questions, program recommendations, and future research suggestions related to professional development for online instructors. Articles were selected and coded based on date of publication and the context of the professional development. Results indicate that most research questions focused on (a ...
This literature review provides an overview of key research areas and offers insights into existing knowledge. ... highlight the potential of incorporating AI systems in online learning to ...
What does the secondary research literature tell us about distance learning? This blogpost offers a literature review on online distance learning, which is thematically divided into four sections.
The growth of online learning has created a need for instructors who can competently teach online. This literature review explores the research questions, program recommendations, and future research suggestions related to professional development for online instructors. Articles were selected and coded based on date of publication and the ...
Online interdisciplinary learning platform construction for teachers. ... This study adopts a systematic literature review method to systematically sort out 20 international studies on the interdisciplinary competence of primary and secondary school teachers from 2019 to 2023, including basic information of the literature, research design ...
This paper will provide a broad literature review of current and past research on the topics of barriers to online learning. It will cover various education levels, including K-12 and Higher Education in many countries around the world. Several papers from the pre-pandemic time are also being included.
Compared with the traditional classroom, the online flipped classroom based on CBL has significantly improved the learning enthusiasm, as these students are required to independently complete literature review, actively participate in classroom teaching, learn to use multiple learning strategies, and collaborate with other students to complete ...
This study evaluated the performance and feasibility of active learning to support the selection of relevant publications within medical guideline development and to study the role of noisy labels. We used a mixed-methods design. Two independent clinicians' manual process of literature selection was evaluated for 14 searches.
The Literature Study Module provides immediate access to peer-selected, contemporary articles that are relevant to specialty practice. After learners review the content, they must successfully complete an online assessment to earn recertification credit.