
Home » Education » Difference Between Research Methods and Research Design
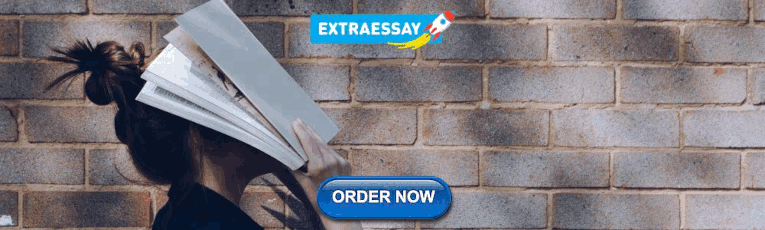
Difference Between Research Methods and Research Design
Main difference – research methods vs research design.
Research methods and research design are terms you must know before starting a research project. Both these elements are essential to the success of a research project. However, many new researchers assume research methods and research design to be the same. Research design is the overall structure of a research project. For example, if you are building a house, you need to have a good idea about what kind of house you are going to build; you cannot do anything without knowing this. A research design is the same – you cannot proceed with the research study without having a proper research design. Research methods are the procedures that are used to collect and analyze data. Thus, the main difference between research methods and research design is that research design is the overall structure of the research study whereas research methods are the various processes, procedures, and tools used to collect and analyze data.
1. What are Research Methods? – Definition, Features, Characteristics
2. What is Research Design? – Definition, Features, Characteristics

What are Research Methods
Research methods are concerned with the various research processes, procedures, and tools – techniques of gathering information, various ways of analyzing them. Research problems can be categorized into two basic sections: qualitative research and quantitative research . Researchers may use one or both of these methods (mixed method) in their research studies. The type of research method you choose would depend on your research questions or problem and research design.
The main aim of a research study is to produce new knowledge or deepen the existing understanding of a field. This can be done by three forms.
Exploratory research – identifies and outlines a problem or question
Constructive research – tests theories and suggests solutions to a problem or question
Empirical research – tests the viability of a solution using empirical evidence

What is a Research Design
Research design is the overall plan or structure of the research project. It indicates what type of study is planned and what kind of results are expected from this project. It specifically focuses on the final results of the research. It is almost impossible to proceed with a research project without a proper research design. The main function of a research design is to make sure that the information gathered throughout the research answers the initial question unambiguously. In other words, the final outcomes and conclusions of the research must correspond with the research problems chosen at the beginning of the research.
A research design can be,
Descriptive (case study, survey, naturalistic observation, etc.)
Correlational (case-control study, observational study, etc.)
Experimental (experiments)
Semi-experimental (field experiment, quasi-experiment, etc.)
Meta-analytic (meta-analysis)
Review ( literature review , systematic review)

Research Methods : Research methods are the procedures that will be used to collect and analyze data.
Research Design: Research design is the overall structure of the research.
Research Methods: Research methods focus on what type of methods are more suitable to collect and analyze the evidence we need.
Research Design: Research design focuses on what type of study is planned and what kind of results are expected from the research.
Research Methods: Research methods depend on the research design.
Research Design: Research design is based on the research question or problem.
De Vaus, D. A. 2001. Research design in social research. London: SAGE.
Image Courtesy:
“The Scientific Method” By CK-12 Foundation – File:High_School_Chemistry.pdf, page 23 (CC BY-SA 3.0) via Commons Wikimedia
“How to do ethnography” by Sam Ladner (CC BY 2.0) via Flickr
About the Author: Hasa
Hasanthi is a seasoned content writer and editor with over 8 years of experience. Armed with a BA degree in English and a knack for digital marketing, she explores her passions for literature, history, culture, and food through her engaging and informative writing.
You May Also Like These
Leave a reply cancel reply.
- University Libraries
- Research Guides
- Topic Guides
- Research Methods Guide
- Research Design & Method
Research Methods Guide: Research Design & Method
- Introduction
- Survey Research
- Interview Research
- Data Analysis
- Resources & Consultation
Tutorial Videos: Research Design & Method
Research Methods (sociology-focused)
Qualitative vs. Quantitative Methods (intro)
Qualitative vs. Quantitative Methods (advanced)
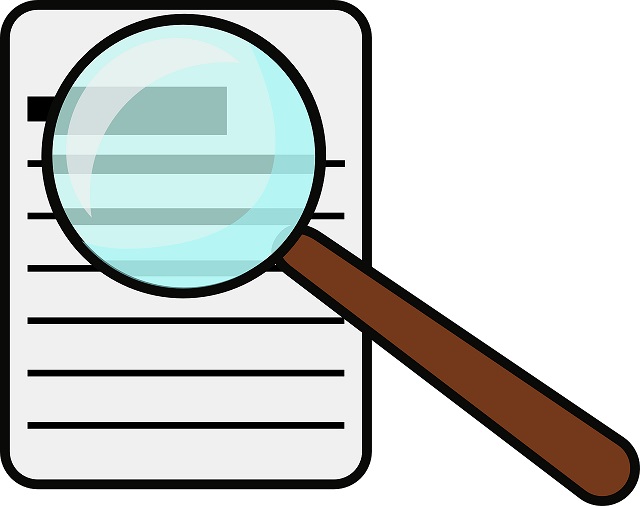
FAQ: Research Design & Method
What is the difference between Research Design and Research Method?
Research design is a plan to answer your research question. A research method is a strategy used to implement that plan. Research design and methods are different but closely related, because good research design ensures that the data you obtain will help you answer your research question more effectively.
Which research method should I choose ?
It depends on your research goal. It depends on what subjects (and who) you want to study. Let's say you are interested in studying what makes people happy, or why some students are more conscious about recycling on campus. To answer these questions, you need to make a decision about how to collect your data. Most frequently used methods include:
- Observation / Participant Observation
- Focus Groups
- Experiments
- Secondary Data Analysis / Archival Study
- Mixed Methods (combination of some of the above)
One particular method could be better suited to your research goal than others, because the data you collect from different methods will be different in quality and quantity. For instance, surveys are usually designed to produce relatively short answers, rather than the extensive responses expected in qualitative interviews.
What other factors should I consider when choosing one method over another?
Time for data collection and analysis is something you want to consider. An observation or interview method, so-called qualitative approach, helps you collect richer information, but it takes time. Using a survey helps you collect more data quickly, yet it may lack details. So, you will need to consider the time you have for research and the balance between strengths and weaknesses associated with each method (e.g., qualitative vs. quantitative).
- << Previous: Introduction
- Next: Survey Research >>
- Last Updated: Aug 21, 2023 10:42 AM
Research Design vs. Research Method
What's the difference.
Research design refers to the overall plan or structure of a research study, outlining the methods and procedures that will be used to collect and analyze data. It includes decisions about the research questions, sampling techniques, data collection methods, and data analysis procedures. On the other hand, research method refers to the specific techniques or tools that will be used to gather and analyze data within the research design. Research methods can include surveys, experiments, interviews, observations, and content analysis. In essence, research design provides the framework for the study, while research methods are the specific tools used to carry out the research.
Attribute | Research Design | Research Method |
---|---|---|
Definition | Plan or blueprint for conducting research | Techniques and procedures used to gather and analyze data |
Goal | To structure the research process | To collect and analyze data |
Types | Descriptive, exploratory, explanatory, etc. | Qualitative, quantitative, mixed methods, etc. |
Focus | Overall strategy for conducting research | Specific techniques used to gather data |
Flexibility | Can be flexible and adaptable | May be more rigid and structured |
Further Detail
Introduction.
Research design and research method are two crucial components of any research study. While they are often used interchangeably, they actually refer to different aspects of the research process. Understanding the distinctions between research design and research method is essential for conducting a successful research study.
Research Design
Research design refers to the overall plan or strategy that guides the research study. It outlines the structure of the study, including the research questions, hypotheses, variables, and data collection methods. Research design is crucial because it helps researchers to organize their study and ensure that they are addressing their research questions effectively.
There are several types of research designs, including experimental, correlational, descriptive, and exploratory. Each type of research design has its own strengths and weaknesses, and researchers must choose the most appropriate design based on their research questions and objectives.
Research design also includes decisions about the sampling strategy, data collection procedures, and data analysis techniques. These decisions are critical for ensuring the validity and reliability of the research findings.
In summary, research design is the blueprint for the research study, outlining the overall structure and strategy that will be used to address the research questions.
Research Method
Research method, on the other hand, refers to the specific techniques and procedures that researchers use to collect and analyze data. Research method is more focused on the practical aspects of conducting the research study, such as the tools and instruments that will be used to gather data.
There are various research methods that researchers can use, including surveys, experiments, interviews, observations, and case studies. Each research method has its own strengths and limitations, and researchers must choose the most appropriate method based on their research questions and objectives.
Research method also includes decisions about the data collection process, such as the sample size, data collection tools, and data analysis techniques. These decisions are crucial for ensuring the accuracy and reliability of the research findings.
In summary, research method is the practical implementation of the research design, involving the specific techniques and procedures that researchers use to collect and analyze data.
While research design and research method are distinct concepts, they are closely related and interdependent. Research design provides the overall framework for the research study, guiding the selection of research questions, hypotheses, variables, and data collection methods.
Research method, on the other hand, involves the practical implementation of the research design, determining the specific techniques and procedures that will be used to collect and analyze data. Research method is informed by the research design, as researchers must choose methods that are consistent with the overall structure and strategy of the study.
Both research design and research method are essential for conducting a successful research study. A well-designed research study with a clear research design and appropriate research methods is more likely to produce valid and reliable findings.
Ultimately, researchers must carefully consider both research design and research method when planning and conducting a research study, as they are both critical components of the research process.
Comparisons may contain inaccurate information about people, places, or facts. Please report any issues.

Research Design 101
Everything You Need To Get Started (With Examples)
By: Derek Jansen (MBA) | Reviewers: Eunice Rautenbach (DTech) & Kerryn Warren (PhD) | April 2023
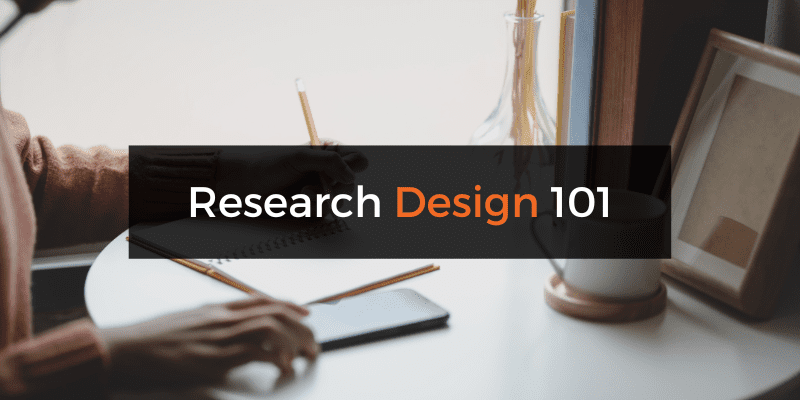
Navigating the world of research can be daunting, especially if you’re a first-time researcher. One concept you’re bound to run into fairly early in your research journey is that of “ research design ”. Here, we’ll guide you through the basics using practical examples , so that you can approach your research with confidence.
Overview: Research Design 101
What is research design.
- Research design types for quantitative studies
- Video explainer : quantitative research design
- Research design types for qualitative studies
- Video explainer : qualitative research design
- How to choose a research design
- Key takeaways
Research design refers to the overall plan, structure or strategy that guides a research project , from its conception to the final data analysis. A good research design serves as the blueprint for how you, as the researcher, will collect and analyse data while ensuring consistency, reliability and validity throughout your study.
Understanding different types of research designs is essential as helps ensure that your approach is suitable given your research aims, objectives and questions , as well as the resources you have available to you. Without a clear big-picture view of how you’ll design your research, you run the risk of potentially making misaligned choices in terms of your methodology – especially your sampling , data collection and data analysis decisions.
The problem with defining research design…
One of the reasons students struggle with a clear definition of research design is because the term is used very loosely across the internet, and even within academia.
Some sources claim that the three research design types are qualitative, quantitative and mixed methods , which isn’t quite accurate (these just refer to the type of data that you’ll collect and analyse). Other sources state that research design refers to the sum of all your design choices, suggesting it’s more like a research methodology . Others run off on other less common tangents. No wonder there’s confusion!
In this article, we’ll clear up the confusion. We’ll explain the most common research design types for both qualitative and quantitative research projects, whether that is for a full dissertation or thesis, or a smaller research paper or article.
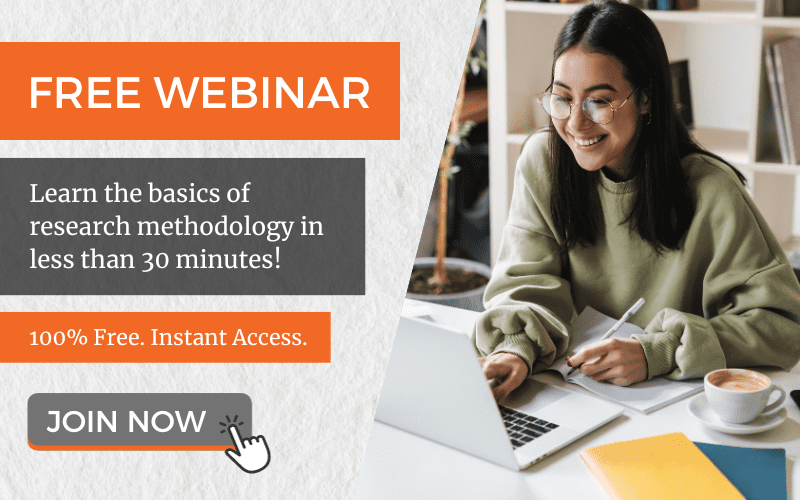
Research Design: Quantitative Studies
Quantitative research involves collecting and analysing data in a numerical form. Broadly speaking, there are four types of quantitative research designs: descriptive , correlational , experimental , and quasi-experimental .
Descriptive Research Design
As the name suggests, descriptive research design focuses on describing existing conditions, behaviours, or characteristics by systematically gathering information without manipulating any variables. In other words, there is no intervention on the researcher’s part – only data collection.
For example, if you’re studying smartphone addiction among adolescents in your community, you could deploy a survey to a sample of teens asking them to rate their agreement with certain statements that relate to smartphone addiction. The collected data would then provide insight regarding how widespread the issue may be – in other words, it would describe the situation.
The key defining attribute of this type of research design is that it purely describes the situation . In other words, descriptive research design does not explore potential relationships between different variables or the causes that may underlie those relationships. Therefore, descriptive research is useful for generating insight into a research problem by describing its characteristics . By doing so, it can provide valuable insights and is often used as a precursor to other research design types.
Correlational Research Design
Correlational design is a popular choice for researchers aiming to identify and measure the relationship between two or more variables without manipulating them . In other words, this type of research design is useful when you want to know whether a change in one thing tends to be accompanied by a change in another thing.
For example, if you wanted to explore the relationship between exercise frequency and overall health, you could use a correlational design to help you achieve this. In this case, you might gather data on participants’ exercise habits, as well as records of their health indicators like blood pressure, heart rate, or body mass index. Thereafter, you’d use a statistical test to assess whether there’s a relationship between the two variables (exercise frequency and health).
As you can see, correlational research design is useful when you want to explore potential relationships between variables that cannot be manipulated or controlled for ethical, practical, or logistical reasons. It is particularly helpful in terms of developing predictions , and given that it doesn’t involve the manipulation of variables, it can be implemented at a large scale more easily than experimental designs (which will look at next).
That said, it’s important to keep in mind that correlational research design has limitations – most notably that it cannot be used to establish causality . In other words, correlation does not equal causation . To establish causality, you’ll need to move into the realm of experimental design, coming up next…
Need a helping hand?
Experimental Research Design
Experimental research design is used to determine if there is a causal relationship between two or more variables . With this type of research design, you, as the researcher, manipulate one variable (the independent variable) while controlling others (dependent variables). Doing so allows you to observe the effect of the former on the latter and draw conclusions about potential causality.
For example, if you wanted to measure if/how different types of fertiliser affect plant growth, you could set up several groups of plants, with each group receiving a different type of fertiliser, as well as one with no fertiliser at all. You could then measure how much each plant group grew (on average) over time and compare the results from the different groups to see which fertiliser was most effective.
Overall, experimental research design provides researchers with a powerful way to identify and measure causal relationships (and the direction of causality) between variables. However, developing a rigorous experimental design can be challenging as it’s not always easy to control all the variables in a study. This often results in smaller sample sizes , which can reduce the statistical power and generalisability of the results.
Moreover, experimental research design requires random assignment . This means that the researcher needs to assign participants to different groups or conditions in a way that each participant has an equal chance of being assigned to any group (note that this is not the same as random sampling ). Doing so helps reduce the potential for bias and confounding variables . This need for random assignment can lead to ethics-related issues . For example, withholding a potentially beneficial medical treatment from a control group may be considered unethical in certain situations.
Quasi-Experimental Research Design
Quasi-experimental research design is used when the research aims involve identifying causal relations , but one cannot (or doesn’t want to) randomly assign participants to different groups (for practical or ethical reasons). Instead, with a quasi-experimental research design, the researcher relies on existing groups or pre-existing conditions to form groups for comparison.
For example, if you were studying the effects of a new teaching method on student achievement in a particular school district, you may be unable to randomly assign students to either group and instead have to choose classes or schools that already use different teaching methods. This way, you still achieve separate groups, without having to assign participants to specific groups yourself.
Naturally, quasi-experimental research designs have limitations when compared to experimental designs. Given that participant assignment is not random, it’s more difficult to confidently establish causality between variables, and, as a researcher, you have less control over other variables that may impact findings.
All that said, quasi-experimental designs can still be valuable in research contexts where random assignment is not possible and can often be undertaken on a much larger scale than experimental research, thus increasing the statistical power of the results. What’s important is that you, as the researcher, understand the limitations of the design and conduct your quasi-experiment as rigorously as possible, paying careful attention to any potential confounding variables .
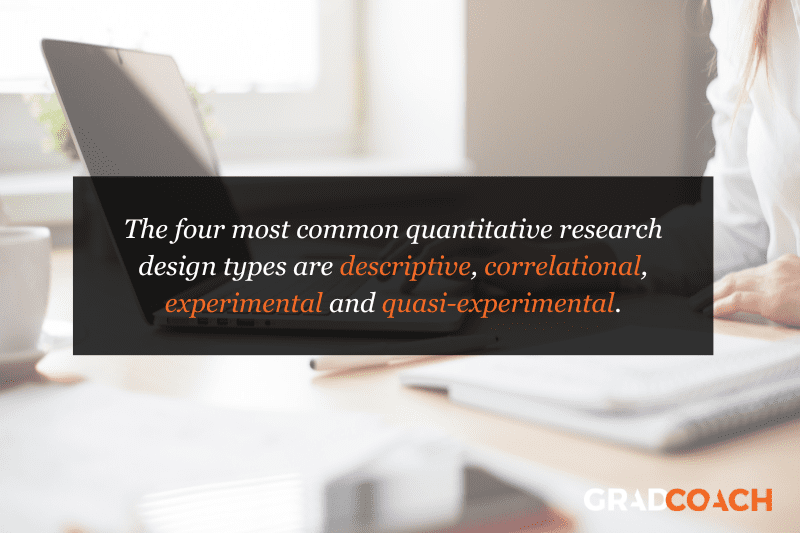
Research Design: Qualitative Studies
There are many different research design types when it comes to qualitative studies, but here we’ll narrow our focus to explore the “Big 4”. Specifically, we’ll look at phenomenological design, grounded theory design, ethnographic design, and case study design.
Phenomenological Research Design
Phenomenological design involves exploring the meaning of lived experiences and how they are perceived by individuals. This type of research design seeks to understand people’s perspectives , emotions, and behaviours in specific situations. Here, the aim for researchers is to uncover the essence of human experience without making any assumptions or imposing preconceived ideas on their subjects.
For example, you could adopt a phenomenological design to study why cancer survivors have such varied perceptions of their lives after overcoming their disease. This could be achieved by interviewing survivors and then analysing the data using a qualitative analysis method such as thematic analysis to identify commonalities and differences.
Phenomenological research design typically involves in-depth interviews or open-ended questionnaires to collect rich, detailed data about participants’ subjective experiences. This richness is one of the key strengths of phenomenological research design but, naturally, it also has limitations. These include potential biases in data collection and interpretation and the lack of generalisability of findings to broader populations.
Grounded Theory Research Design
Grounded theory (also referred to as “GT”) aims to develop theories by continuously and iteratively analysing and comparing data collected from a relatively large number of participants in a study. It takes an inductive (bottom-up) approach, with a focus on letting the data “speak for itself”, without being influenced by preexisting theories or the researcher’s preconceptions.
As an example, let’s assume your research aims involved understanding how people cope with chronic pain from a specific medical condition, with a view to developing a theory around this. In this case, grounded theory design would allow you to explore this concept thoroughly without preconceptions about what coping mechanisms might exist. You may find that some patients prefer cognitive-behavioural therapy (CBT) while others prefer to rely on herbal remedies. Based on multiple, iterative rounds of analysis, you could then develop a theory in this regard, derived directly from the data (as opposed to other preexisting theories and models).
Grounded theory typically involves collecting data through interviews or observations and then analysing it to identify patterns and themes that emerge from the data. These emerging ideas are then validated by collecting more data until a saturation point is reached (i.e., no new information can be squeezed from the data). From that base, a theory can then be developed .
As you can see, grounded theory is ideally suited to studies where the research aims involve theory generation , especially in under-researched areas. Keep in mind though that this type of research design can be quite time-intensive , given the need for multiple rounds of data collection and analysis.
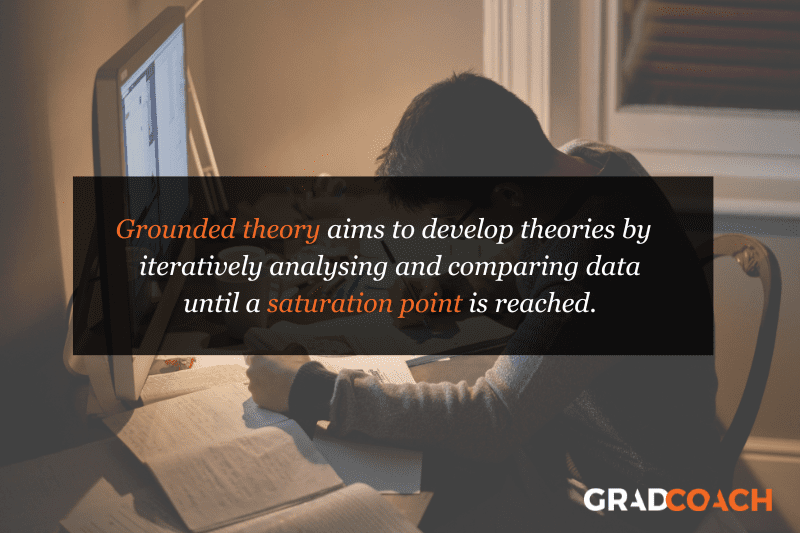
Ethnographic Research Design
Ethnographic design involves observing and studying a culture-sharing group of people in their natural setting to gain insight into their behaviours, beliefs, and values. The focus here is on observing participants in their natural environment (as opposed to a controlled environment). This typically involves the researcher spending an extended period of time with the participants in their environment, carefully observing and taking field notes .
All of this is not to say that ethnographic research design relies purely on observation. On the contrary, this design typically also involves in-depth interviews to explore participants’ views, beliefs, etc. However, unobtrusive observation is a core component of the ethnographic approach.
As an example, an ethnographer may study how different communities celebrate traditional festivals or how individuals from different generations interact with technology differently. This may involve a lengthy period of observation, combined with in-depth interviews to further explore specific areas of interest that emerge as a result of the observations that the researcher has made.
As you can probably imagine, ethnographic research design has the ability to provide rich, contextually embedded insights into the socio-cultural dynamics of human behaviour within a natural, uncontrived setting. Naturally, however, it does come with its own set of challenges, including researcher bias (since the researcher can become quite immersed in the group), participant confidentiality and, predictably, ethical complexities . All of these need to be carefully managed if you choose to adopt this type of research design.
Case Study Design
With case study research design, you, as the researcher, investigate a single individual (or a single group of individuals) to gain an in-depth understanding of their experiences, behaviours or outcomes. Unlike other research designs that are aimed at larger sample sizes, case studies offer a deep dive into the specific circumstances surrounding a person, group of people, event or phenomenon, generally within a bounded setting or context .
As an example, a case study design could be used to explore the factors influencing the success of a specific small business. This would involve diving deeply into the organisation to explore and understand what makes it tick – from marketing to HR to finance. In terms of data collection, this could include interviews with staff and management, review of policy documents and financial statements, surveying customers, etc.
While the above example is focused squarely on one organisation, it’s worth noting that case study research designs can have different variation s, including single-case, multiple-case and longitudinal designs. As you can see in the example, a single-case design involves intensely examining a single entity to understand its unique characteristics and complexities. Conversely, in a multiple-case design , multiple cases are compared and contrasted to identify patterns and commonalities. Lastly, in a longitudinal case design , a single case or multiple cases are studied over an extended period of time to understand how factors develop over time.
As you can see, a case study research design is particularly useful where a deep and contextualised understanding of a specific phenomenon or issue is desired. However, this strength is also its weakness. In other words, you can’t generalise the findings from a case study to the broader population. So, keep this in mind if you’re considering going the case study route.
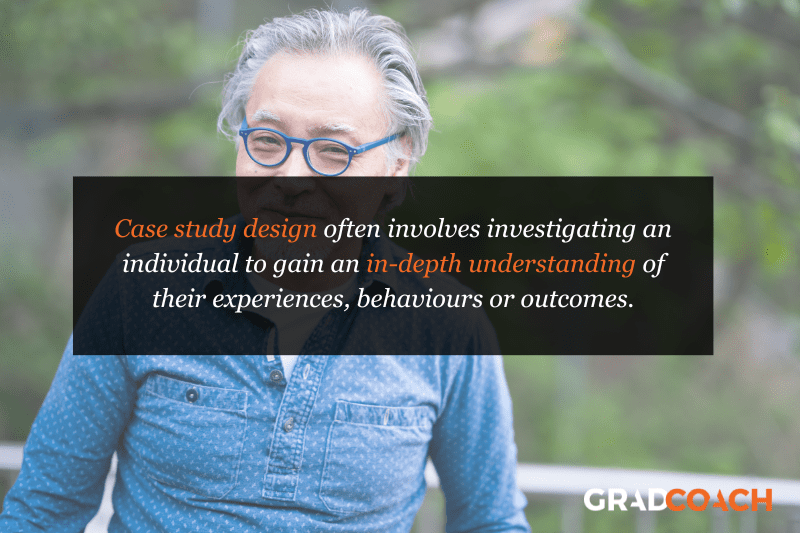
How To Choose A Research Design
Having worked through all of these potential research designs, you’d be forgiven for feeling a little overwhelmed and wondering, “ But how do I decide which research design to use? ”. While we could write an entire post covering that alone, here are a few factors to consider that will help you choose a suitable research design for your study.
Data type: The first determining factor is naturally the type of data you plan to be collecting – i.e., qualitative or quantitative. This may sound obvious, but we have to be clear about this – don’t try to use a quantitative research design on qualitative data (or vice versa)!
Research aim(s) and question(s): As with all methodological decisions, your research aim and research questions will heavily influence your research design. For example, if your research aims involve developing a theory from qualitative data, grounded theory would be a strong option. Similarly, if your research aims involve identifying and measuring relationships between variables, one of the experimental designs would likely be a better option.
Time: It’s essential that you consider any time constraints you have, as this will impact the type of research design you can choose. For example, if you’ve only got a month to complete your project, a lengthy design such as ethnography wouldn’t be a good fit.
Resources: Take into account the resources realistically available to you, as these need to factor into your research design choice. For example, if you require highly specialised lab equipment to execute an experimental design, you need to be sure that you’ll have access to that before you make a decision.
Keep in mind that when it comes to research, it’s important to manage your risks and play as conservatively as possible. If your entire project relies on you achieving a huge sample, having access to niche equipment or holding interviews with very difficult-to-reach participants, you’re creating risks that could kill your project. So, be sure to think through your choices carefully and make sure that you have backup plans for any existential risks. Remember that a relatively simple methodology executed well generally will typically earn better marks than a highly-complex methodology executed poorly.
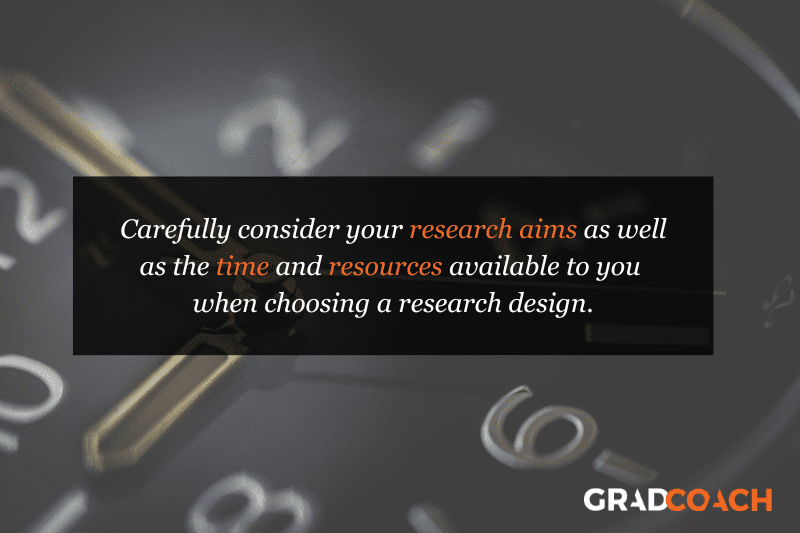
Recap: Key Takeaways
We’ve covered a lot of ground here. Let’s recap by looking at the key takeaways:
- Research design refers to the overall plan, structure or strategy that guides a research project, from its conception to the final analysis of data.
- Research designs for quantitative studies include descriptive , correlational , experimental and quasi-experimenta l designs.
- Research designs for qualitative studies include phenomenological , grounded theory , ethnographic and case study designs.
- When choosing a research design, you need to consider a variety of factors, including the type of data you’ll be working with, your research aims and questions, your time and the resources available to you.
If you need a helping hand with your research design (or any other aspect of your research), check out our private coaching services .
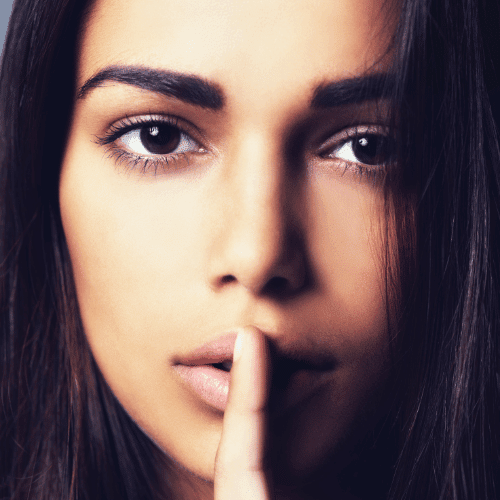
Psst... there’s more!
This post was based on one of our popular Research Bootcamps . If you're working on a research project, you'll definitely want to check this out ...
10 Comments
Is there any blog article explaining more on Case study research design? Is there a Case study write-up template? Thank you.
Thanks this was quite valuable to clarify such an important concept.
Thanks for this simplified explanations. it is quite very helpful.
This was really helpful. thanks
Thank you for your explanation. I think case study research design and the use of secondary data in researches needs to be talked about more in your videos and articles because there a lot of case studies research design tailored projects out there.
Please is there any template for a case study research design whose data type is a secondary data on your repository?
This post is very clear, comprehensive and has been very helpful to me. It has cleared the confusion I had in regard to research design and methodology.
This post is helpful, easy to understand, and deconstructs what a research design is. Thanks
how to cite this page
Thank you very much for the post. It is wonderful and has cleared many worries in my mind regarding research designs. I really appreciate .
how can I put this blog as my reference(APA style) in bibliography part?
Submit a Comment Cancel reply
Your email address will not be published. Required fields are marked *
Save my name, email, and website in this browser for the next time I comment.
- Print Friendly
Have a language expert improve your writing
Run a free plagiarism check in 10 minutes, automatically generate references for free.
- Knowledge Base
- Methodology
Types of Research Designs Compared | Examples
Published on 5 May 2022 by Shona McCombes . Revised on 10 October 2022.
When you start planning a research project, developing research questions and creating a research design , you will have to make various decisions about the type of research you want to do.
There are many ways to categorise different types of research. The words you use to describe your research depend on your discipline and field. In general, though, the form your research design takes will be shaped by:
- The type of knowledge you aim to produce
- The type of data you will collect and analyse
- The sampling methods , timescale, and location of the research
This article takes a look at some common distinctions made between different types of research and outlines the key differences between them.
Table of contents
Types of research aims, types of research data, types of sampling, timescale, and location.
The first thing to consider is what kind of knowledge your research aims to contribute.
Type of research | What’s the difference? | What to consider |
---|---|---|
Basic vs applied | Basic research aims to , while applied research aims to . | Do you want to expand scientific understanding or solve a practical problem? |
vs | Exploratory research aims to , while explanatory research aims to . | How much is already known about your research problem? Are you conducting initial research on a newly-identified issue, or seeking precise conclusions about an established issue? |
aims to , while aims to . | Is there already some theory on your research problem that you can use to develop , or do you want to propose new theories based on your findings? |
Prevent plagiarism, run a free check.
The next thing to consider is what type of data you will collect. Each kind of data is associated with a range of specific research methods and procedures.
Type of research | What’s the difference? | What to consider |
---|---|---|
Primary vs secondary | Primary data is (e.g., through interviews or experiments), while secondary data (e.g., in government surveys or scientific publications). | How much data is already available on your topic? Do you want to collect original data or analyse existing data (e.g., through a )? |
, while . | Is your research more concerned with measuring something or interpreting something? You can also create a research design that has elements of both. | |
vs | Descriptive research gathers data , while experimental research . | Do you want to identify characteristics, patterns, and or test causal relationships between ? |
Finally, you have to consider three closely related questions: How will you select the subjects or participants of the research? When and how often will you collect data from your subjects? And where will the research take place?
Type of research | What’s the difference? | What to consider |
---|---|---|
allows you to , while allows you to draw conclusions . | Do you want to produce knowledge that applies to many contexts or detailed knowledge about a specific context (e.g., in a )? | |
vs | Cross-sectional studies , while longitudinal studies . | Is your research question focused on understanding the current situation or tracking changes over time? |
Field vs laboratory | Field research takes place in , while laboratory research takes place in . | Do you want to find out how something occurs in the real world or draw firm conclusions about cause and effect? Laboratory experiments have higher but lower . |
Fixed vs flexible | In a fixed research design the subjects, timescale and location are begins, while in a flexible design these aspects may . | Do you want to test hypotheses and establish generalisable facts, or explore concepts and develop understanding? For measuring, testing, and making generalisations, a fixed research design has higher . |
Choosing among all these different research types is part of the process of creating your research design , which determines exactly how the research will be conducted. But the type of research is only the first step: next, you have to make more concrete decisions about your research methods and the details of the study.
Read more about creating a research design
Cite this Scribbr article
If you want to cite this source, you can copy and paste the citation or click the ‘Cite this Scribbr article’ button to automatically add the citation to our free Reference Generator.
McCombes, S. (2022, October 10). Types of Research Designs Compared | Examples. Scribbr. Retrieved 5 August 2024, from https://www.scribbr.co.uk/research-methods/types-of-research-designs/
Is this article helpful?
Shona McCombes
Other students also liked, sampling methods | types, techniques, & examples, qualitative vs quantitative research | examples & methods, between-subjects design | examples, pros & cons.
The Four Types of Research Design — Everything You Need to Know

Updated: July 23, 2024
Published: January 18, 2023
When you conduct research, you need to have a clear idea of what you want to achieve and how to accomplish it. A good research design enables you to collect accurate and reliable data to draw valid conclusions.
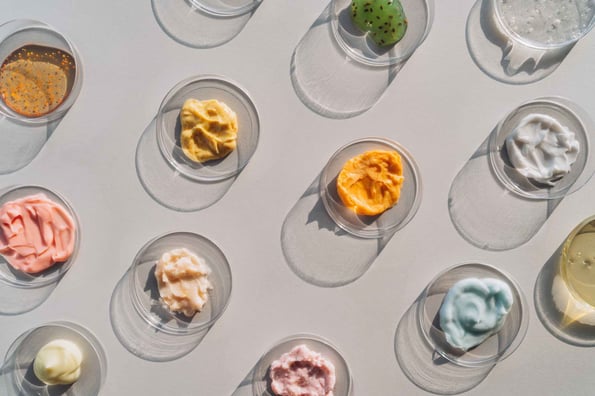
In this blog post, we'll outline the key features of the four common types of research design with real-life examples from UnderArmor, Carmex, and more. Then, you can easily choose the right approach for your project.
Table of Contents
What is research design?
The four types of research design, research design examples.
Research design is the process of planning and executing a study to answer specific questions. This process allows you to test hypotheses in the business or scientific fields.
Research design involves choosing the right methodology, selecting the most appropriate data collection methods, and devising a plan (or framework) for analyzing the data. In short, a good research design helps us to structure our research.
Marketers use different types of research design when conducting research .
There are four common types of research design — descriptive, correlational, experimental, and diagnostic designs. Let’s take a look at each in more detail.
Researchers use different designs to accomplish different research objectives. Here, we'll discuss how to choose the right type, the benefits of each, and use cases.
Research can also be classified as quantitative or qualitative at a higher level. Some experiments exhibit both qualitative and quantitative characteristics.
.png)
Free Market Research Kit
5 Research and Planning Templates + a Free Guide on How to Use Them in Your Market Research
- SWOT Analysis Template
- Survey Template
- Focus Group Template
Download Free
All fields are required.
You're all set!
Click this link to access this resource at any time.
Experimental
An experimental design is used when the researcher wants to examine how variables interact with each other. The researcher manipulates one variable (the independent variable) and observes the effect on another variable (the dependent variable).
In other words, the researcher wants to test a causal relationship between two or more variables.
In marketing, an example of experimental research would be comparing the effects of a television commercial versus an online advertisement conducted in a controlled environment (e.g. a lab). The objective of the research is to test which advertisement gets more attention among people of different age groups, gender, etc.
Another example is a study of the effect of music on productivity. A researcher assigns participants to one of two groups — those who listen to music while working and those who don't — and measure their productivity.
The main benefit of an experimental design is that it allows the researcher to draw causal relationships between variables.
One limitation: This research requires a great deal of control over the environment and participants, making it difficult to replicate in the real world. In addition, it’s quite costly.
Best for: Testing a cause-and-effect relationship (i.e., the effect of an independent variable on a dependent variable).
Correlational
A correlational design examines the relationship between two or more variables without intervening in the process.
Correlational design allows the analyst to observe natural relationships between variables. This results in data being more reflective of real-world situations.
For example, marketers can use correlational design to examine the relationship between brand loyalty and customer satisfaction. In particular, the researcher would look for patterns or trends in the data to see if there is a relationship between these two entities.
Similarly, you can study the relationship between physical activity and mental health. The analyst here would ask participants to complete surveys about their physical activity levels and mental health status. Data would show how the two variables are related.
Best for: Understanding the extent to which two or more variables are associated with each other in the real world.
Descriptive
Descriptive research refers to a systematic process of observing and describing what a subject does without influencing them.
Methods include surveys, interviews, case studies, and observations. Descriptive research aims to gather an in-depth understanding of a phenomenon and answers when/what/where.
SaaS companies use descriptive design to understand how customers interact with specific features. Findings can be used to spot patterns and roadblocks.
For instance, product managers can use screen recordings by Hotjar to observe in-app user behavior. This way, the team can precisely understand what is happening at a certain stage of the user journey and act accordingly.
Brand24, a social listening tool, tripled its sign-up conversion rate from 2.56% to 7.42%, thanks to locating friction points in the sign-up form through screen recordings.
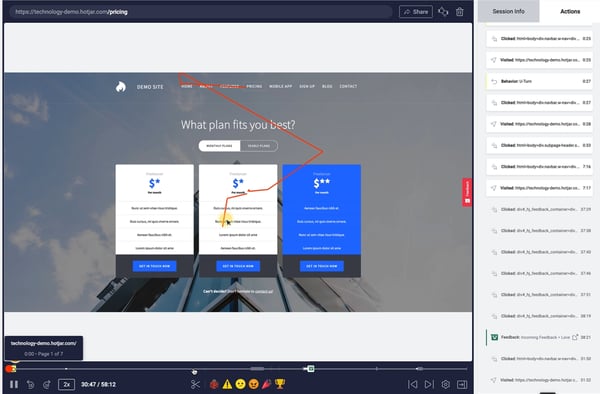
Carma Laboratories worked with research company MMR to measure customers’ reactions to the lip-care company’s packaging and product . The goal was to find the cause of low sales for a recently launched line extension in Europe.
The team moderated a live, online focus group. Participants were shown w product samples, while AI and NLP natural language processing identified key themes in customer feedback.
This helped uncover key reasons for poor performance and guided changes in packaging.
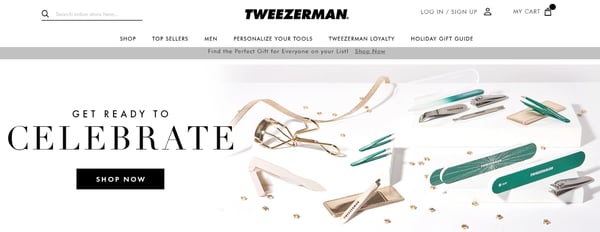
An official website of the United States government
The .gov means it’s official. Federal government websites often end in .gov or .mil. Before sharing sensitive information, make sure you’re on a federal government site.
The site is secure. The https:// ensures that you are connecting to the official website and that any information you provide is encrypted and transmitted securely.
- Publications
- Account settings
Preview improvements coming to the PMC website in October 2024. Learn More or Try it out now .
- Advanced Search
- Journal List
- J Grad Med Educ
- v.8(1); 2016 Feb
Research Design Considerations
Associated data.
Editor's Note: The online version of this article contains references and resources for further reading and the authors' professional information.
The Challenge
“I'd really like to do a survey” or “Let's conduct some interviews” might sound like reasonable starting points for a research project. However, it is crucial that researchers examine their philosophical assumptions and those underpinning their research questions before selecting data collection methods. Philosophical assumptions relate to ontology, or the nature of reality, and epistemology, the nature of knowledge. Alignment of the researcher's worldview (ie, ontology and epistemology) with methodology (research approach) and methods (specific data collection, analysis, and interpretation tools) is key to quality research design. This Rip Out will explain philosophical differences between quantitative and qualitative research designs and how they affect definitions of rigorous research.
What Is Known
Worldviews offer different beliefs about what can be known and how it can be known, thereby shaping the types of research questions that are asked, the research approach taken, and ultimately, the data collection and analytic methods used. Ontology refers to the question of “What can we know?” Ontological viewpoints can be placed on a continuum: researchers at one end believe that an observable reality exists independent of our knowledge of it, while at the other end, researchers believe that reality is subjective and constructed, with no universal “truth” to be discovered. 1,2 Epistemology refers to the question of “How can we know?” 3 Epistemological positions also can be placed on a continuum, influenced by the researcher's ontological viewpoint. For example, the positivist worldview is based on belief in an objective reality and a truth to be discovered. Therefore, knowledge is produced through objective measurements and the quantitative relationships between variables. 4 This might include measuring the difference in examination scores between groups of learners who have been exposed to 2 different teaching formats, in order to determine whether a particular teaching format influenced the resulting examination scores.
In contrast, subjectivists (also referred to as constructionists or constructivists ) are at the opposite end of the continuum, and believe there are multiple or situated realities that are constructed in particular social, cultural, institutional, and historical contexts. According to this view, knowledge is created through the exploration of beliefs, perceptions, and experiences of the world, often captured and interpreted through observation, interviews, and focus groups. A researcher with this worldview might be interested in exploring the perceptions of students exposed to the 2 teaching formats, to better understand how learning is experienced in the 2 settings. It is crucial that there is alignment between ontology (what can we know?), epistemology (how can we know it?), methodology (what approach should be used?), and data collection and analysis methods (what specific tools should be used?). 5
Key Differences in Qualitative and Quantitative Approaches
Use of theory.
Quantitative approaches generally test theory, while qualitative approaches either use theory as a lens that shapes the research design or generate new theories inductively from their data. 4
Use of Logic
Quantitative approaches often involve deductive logic, starting off with general arguments of theories and concepts that result in data points. 4 Qualitative approaches often use inductive logic or both inductive and deductive logic, start with the data, and build up to a description, theory, or explanatory model. 4
Purpose of Results
Quantitative approaches attempt to generalize findings; qualitative approaches pay specific attention to particular individuals, groups, contexts, or cultures to provide a deep understanding of a phenomenon in local context. 4
Establishing Rigor
Quantitative researchers must collect evidence of validity and reliability. Some qualitative researchers also aim to establish validity and reliability. They seek to be as objective as possible through techniques, including cross-checking and cross-validating sources during observations. 6 Other qualitative researchers have developed specific frameworks, terminology, and criteria on which qualitative research should be evaluated. 6,7 For example, the use of credibility, transferability, dependability, and confirmability as criteria for rigor seek to establish the accuracy, trustworthiness, and believability of the research, rather than its validity and reliability. 8 Thus, the framework of rigor you choose will depend on your chosen methodology (see “Choosing a Qualitative Research Approach” Rip Out).
View of Objectivity
A goal of quantitative research is to maintain objectivity, in other words, to reduce the influence of the researcher on data collection as much as possible. Some qualitative researchers also attempt to reduce their own influence on the research. However, others suggest that these approaches subscribe to positivistic ideals, which are inappropriate for qualitative research, 6,9,10 as researchers should not seek to eliminate the effects of their influence on the study but to understand them through reflexivity . 11 Reflexivity is an acknowledgement that, to make sense of the social world, a researcher will inevitably draw on his or her own values, norms, and concepts, which prevent a totally objective view of the social world. 12
Sampling Strategies
Quantitative research favors using large, randomly generated samples, especially if the intent of the research is to generalize to other populations. 6 Instead, qualitative research often focuses on participants who are likely to provide rich information about the study questions, known as purposive sampling . 6
How You Can Start TODAY
- Consider how you can best address your research problem and what philosophical assumptions you are making.
- Consider your ontological and epistemological stance by asking yourself: What can I know about the phenomenon of interest? How can I know what I want to know? W hat approach should I use and why? Answers to these questions might be relatively fixed but should be flexible enough to guide methodological choices that best suit different research problems under study. 5
- Select an appropriate sampling strategy. Purposive sampling is often used in qualitative research, with a goal of finding information-rich cases, not to generalize. 6
- Be reflexive: Examine the ways in which your history, education, experiences, and worldviews have affected the research questions you have selected and your data collection methods, analyses, and writing. 13
How You Can Start TODAY—An Example
Let's assume that you want to know about resident learning on a particular clinical rotation. Your initial thought is to use end-of-rotation assessment scores as a way to measure learning. However, these assessments cannot tell you how or why residents are learning. While you cannot know for sure that residents are learning, consider what you can know—resident perceptions of their learning experiences on this rotation.
Next, you consider how to go about collecting this data—you could ask residents about their experiences in interviews or watch them in their natural settings. Since you would like to develop a theory of resident learning in clinical settings, you decide to use grounded theory as a methodology, as you believe asking residents about their experience using in-depth interviews is the best way for you to elicit the information you are seeking. You should also do more research on grounded theory by consulting related resources, and you will discover that grounded theory requires theoretical sampling. 14,15 You also decide to use the end-of-rotation assessment scores to help select your sample.
Since you want to know how and why students learn, you decide to sample extreme cases of students who have performed well and poorly on the end-of-rotation assessments. You think about how your background influences your standpoint about the research question: Were you ever a resident? How did you score on your end-of-rotation assessments? Did you feel this was an accurate representation of your learning? Are you a clinical faculty member now? Did your rotations prepare you well for this role? How does your history shape the way you view the problem? Seek to challenge, elaborate, and refine your assumptions throughout the research.
As you proceed with the interviews, they trigger further questions, and you then decide to conduct interviews with faculty members to get a more complete picture of the process of learning in this particular resident clinical rotation.
What You Can Do LONG TERM
- Familiarize yourself with published guides on conducting and evaluating qualitative research. 5,16–18 There is no one-size-fits-all formula for qualitative research. However, there are techniques for conducting your research in a way that stays true to the traditions of qualitative research.
- Consider the reporting style of your results. For some research approaches, it would be inappropriate to quantify results through frequency or numerical counts. 19 In this case, instead of saying “5 respondents reported X,” you might consider “respondents who reported X described Y.”
- Review the conventions and writing styles of articles published with a methodological approach similar to the one you are considering. If appropriate, consider using a reflexive writing style to demonstrate understanding of your own role in shaping the research. 6
Supplementary Material
Frequently asked questions
What are the main types of research design.
Quantitative research designs can be divided into two main categories:
- Correlational and descriptive designs are used to investigate characteristics, averages, trends, and associations between variables.
- Experimental and quasi-experimental designs are used to test causal relationships .
Qualitative research designs tend to be more flexible. Common types of qualitative design include case study , ethnography , and grounded theory designs.
Frequently asked questions: Methodology
Attrition refers to participants leaving a study. It always happens to some extent—for example, in randomized controlled trials for medical research.
Differential attrition occurs when attrition or dropout rates differ systematically between the intervention and the control group . As a result, the characteristics of the participants who drop out differ from the characteristics of those who stay in the study. Because of this, study results may be biased .
Action research is conducted in order to solve a particular issue immediately, while case studies are often conducted over a longer period of time and focus more on observing and analyzing a particular ongoing phenomenon.
Action research is focused on solving a problem or informing individual and community-based knowledge in a way that impacts teaching, learning, and other related processes. It is less focused on contributing theoretical input, instead producing actionable input.
Action research is particularly popular with educators as a form of systematic inquiry because it prioritizes reflection and bridges the gap between theory and practice. Educators are able to simultaneously investigate an issue as they solve it, and the method is very iterative and flexible.
A cycle of inquiry is another name for action research . It is usually visualized in a spiral shape following a series of steps, such as “planning → acting → observing → reflecting.”
To make quantitative observations , you need to use instruments that are capable of measuring the quantity you want to observe. For example, you might use a ruler to measure the length of an object or a thermometer to measure its temperature.
Criterion validity and construct validity are both types of measurement validity . In other words, they both show you how accurately a method measures something.
While construct validity is the degree to which a test or other measurement method measures what it claims to measure, criterion validity is the degree to which a test can predictively (in the future) or concurrently (in the present) measure something.
Construct validity is often considered the overarching type of measurement validity . You need to have face validity , content validity , and criterion validity in order to achieve construct validity.
Convergent validity and discriminant validity are both subtypes of construct validity . Together, they help you evaluate whether a test measures the concept it was designed to measure.
- Convergent validity indicates whether a test that is designed to measure a particular construct correlates with other tests that assess the same or similar construct.
- Discriminant validity indicates whether two tests that should not be highly related to each other are indeed not related. This type of validity is also called divergent validity .
You need to assess both in order to demonstrate construct validity. Neither one alone is sufficient for establishing construct validity.
- Discriminant validity indicates whether two tests that should not be highly related to each other are indeed not related
Content validity shows you how accurately a test or other measurement method taps into the various aspects of the specific construct you are researching.
In other words, it helps you answer the question: “does the test measure all aspects of the construct I want to measure?” If it does, then the test has high content validity.
The higher the content validity, the more accurate the measurement of the construct.
If the test fails to include parts of the construct, or irrelevant parts are included, the validity of the instrument is threatened, which brings your results into question.
Face validity and content validity are similar in that they both evaluate how suitable the content of a test is. The difference is that face validity is subjective, and assesses content at surface level.
When a test has strong face validity, anyone would agree that the test’s questions appear to measure what they are intended to measure.
For example, looking at a 4th grade math test consisting of problems in which students have to add and multiply, most people would agree that it has strong face validity (i.e., it looks like a math test).
On the other hand, content validity evaluates how well a test represents all the aspects of a topic. Assessing content validity is more systematic and relies on expert evaluation. of each question, analyzing whether each one covers the aspects that the test was designed to cover.
A 4th grade math test would have high content validity if it covered all the skills taught in that grade. Experts(in this case, math teachers), would have to evaluate the content validity by comparing the test to the learning objectives.
Snowball sampling is a non-probability sampling method . Unlike probability sampling (which involves some form of random selection ), the initial individuals selected to be studied are the ones who recruit new participants.
Because not every member of the target population has an equal chance of being recruited into the sample, selection in snowball sampling is non-random.
Snowball sampling is a non-probability sampling method , where there is not an equal chance for every member of the population to be included in the sample .
This means that you cannot use inferential statistics and make generalizations —often the goal of quantitative research . As such, a snowball sample is not representative of the target population and is usually a better fit for qualitative research .
Snowball sampling relies on the use of referrals. Here, the researcher recruits one or more initial participants, who then recruit the next ones.
Participants share similar characteristics and/or know each other. Because of this, not every member of the population has an equal chance of being included in the sample, giving rise to sampling bias .
Snowball sampling is best used in the following cases:
- If there is no sampling frame available (e.g., people with a rare disease)
- If the population of interest is hard to access or locate (e.g., people experiencing homelessness)
- If the research focuses on a sensitive topic (e.g., extramarital affairs)
The reproducibility and replicability of a study can be ensured by writing a transparent, detailed method section and using clear, unambiguous language.
Reproducibility and replicability are related terms.
- Reproducing research entails reanalyzing the existing data in the same manner.
- Replicating (or repeating ) the research entails reconducting the entire analysis, including the collection of new data .
- A successful reproduction shows that the data analyses were conducted in a fair and honest manner.
- A successful replication shows that the reliability of the results is high.
Stratified sampling and quota sampling both involve dividing the population into subgroups and selecting units from each subgroup. The purpose in both cases is to select a representative sample and/or to allow comparisons between subgroups.
The main difference is that in stratified sampling, you draw a random sample from each subgroup ( probability sampling ). In quota sampling you select a predetermined number or proportion of units, in a non-random manner ( non-probability sampling ).
Purposive and convenience sampling are both sampling methods that are typically used in qualitative data collection.
A convenience sample is drawn from a source that is conveniently accessible to the researcher. Convenience sampling does not distinguish characteristics among the participants. On the other hand, purposive sampling focuses on selecting participants possessing characteristics associated with the research study.
The findings of studies based on either convenience or purposive sampling can only be generalized to the (sub)population from which the sample is drawn, and not to the entire population.
Random sampling or probability sampling is based on random selection. This means that each unit has an equal chance (i.e., equal probability) of being included in the sample.
On the other hand, convenience sampling involves stopping people at random, which means that not everyone has an equal chance of being selected depending on the place, time, or day you are collecting your data.
Convenience sampling and quota sampling are both non-probability sampling methods. They both use non-random criteria like availability, geographical proximity, or expert knowledge to recruit study participants.
However, in convenience sampling, you continue to sample units or cases until you reach the required sample size.
In quota sampling, you first need to divide your population of interest into subgroups (strata) and estimate their proportions (quota) in the population. Then you can start your data collection, using convenience sampling to recruit participants, until the proportions in each subgroup coincide with the estimated proportions in the population.
A sampling frame is a list of every member in the entire population . It is important that the sampling frame is as complete as possible, so that your sample accurately reflects your population.
Stratified and cluster sampling may look similar, but bear in mind that groups created in cluster sampling are heterogeneous , so the individual characteristics in the cluster vary. In contrast, groups created in stratified sampling are homogeneous , as units share characteristics.
Relatedly, in cluster sampling you randomly select entire groups and include all units of each group in your sample. However, in stratified sampling, you select some units of all groups and include them in your sample. In this way, both methods can ensure that your sample is representative of the target population .
A systematic review is secondary research because it uses existing research. You don’t collect new data yourself.
The key difference between observational studies and experimental designs is that a well-done observational study does not influence the responses of participants, while experiments do have some sort of treatment condition applied to at least some participants by random assignment .
An observational study is a great choice for you if your research question is based purely on observations. If there are ethical, logistical, or practical concerns that prevent you from conducting a traditional experiment , an observational study may be a good choice. In an observational study, there is no interference or manipulation of the research subjects, as well as no control or treatment groups .
It’s often best to ask a variety of people to review your measurements. You can ask experts, such as other researchers, or laypeople, such as potential participants, to judge the face validity of tests.
While experts have a deep understanding of research methods , the people you’re studying can provide you with valuable insights you may have missed otherwise.
Face validity is important because it’s a simple first step to measuring the overall validity of a test or technique. It’s a relatively intuitive, quick, and easy way to start checking whether a new measure seems useful at first glance.
Good face validity means that anyone who reviews your measure says that it seems to be measuring what it’s supposed to. With poor face validity, someone reviewing your measure may be left confused about what you’re measuring and why you’re using this method.
Face validity is about whether a test appears to measure what it’s supposed to measure. This type of validity is concerned with whether a measure seems relevant and appropriate for what it’s assessing only on the surface.
Statistical analyses are often applied to test validity with data from your measures. You test convergent validity and discriminant validity with correlations to see if results from your test are positively or negatively related to those of other established tests.
You can also use regression analyses to assess whether your measure is actually predictive of outcomes that you expect it to predict theoretically. A regression analysis that supports your expectations strengthens your claim of construct validity .
When designing or evaluating a measure, construct validity helps you ensure you’re actually measuring the construct you’re interested in. If you don’t have construct validity, you may inadvertently measure unrelated or distinct constructs and lose precision in your research.
Construct validity is often considered the overarching type of measurement validity , because it covers all of the other types. You need to have face validity , content validity , and criterion validity to achieve construct validity.
Construct validity is about how well a test measures the concept it was designed to evaluate. It’s one of four types of measurement validity , which includes construct validity, face validity , and criterion validity.
There are two subtypes of construct validity.
- Convergent validity : The extent to which your measure corresponds to measures of related constructs
- Discriminant validity : The extent to which your measure is unrelated or negatively related to measures of distinct constructs
Naturalistic observation is a valuable tool because of its flexibility, external validity , and suitability for topics that can’t be studied in a lab setting.
The downsides of naturalistic observation include its lack of scientific control , ethical considerations , and potential for bias from observers and subjects.
Naturalistic observation is a qualitative research method where you record the behaviors of your research subjects in real world settings. You avoid interfering or influencing anything in a naturalistic observation.
You can think of naturalistic observation as “people watching” with a purpose.
A dependent variable is what changes as a result of the independent variable manipulation in experiments . It’s what you’re interested in measuring, and it “depends” on your independent variable.
In statistics, dependent variables are also called:
- Response variables (they respond to a change in another variable)
- Outcome variables (they represent the outcome you want to measure)
- Left-hand-side variables (they appear on the left-hand side of a regression equation)
An independent variable is the variable you manipulate, control, or vary in an experimental study to explore its effects. It’s called “independent” because it’s not influenced by any other variables in the study.
Independent variables are also called:
- Explanatory variables (they explain an event or outcome)
- Predictor variables (they can be used to predict the value of a dependent variable)
- Right-hand-side variables (they appear on the right-hand side of a regression equation).
As a rule of thumb, questions related to thoughts, beliefs, and feelings work well in focus groups. Take your time formulating strong questions, paying special attention to phrasing. Be careful to avoid leading questions , which can bias your responses.
Overall, your focus group questions should be:
- Open-ended and flexible
- Impossible to answer with “yes” or “no” (questions that start with “why” or “how” are often best)
- Unambiguous, getting straight to the point while still stimulating discussion
- Unbiased and neutral
A structured interview is a data collection method that relies on asking questions in a set order to collect data on a topic. They are often quantitative in nature. Structured interviews are best used when:
- You already have a very clear understanding of your topic. Perhaps significant research has already been conducted, or you have done some prior research yourself, but you already possess a baseline for designing strong structured questions.
- You are constrained in terms of time or resources and need to analyze your data quickly and efficiently.
- Your research question depends on strong parity between participants, with environmental conditions held constant.
More flexible interview options include semi-structured interviews , unstructured interviews , and focus groups .
Social desirability bias is the tendency for interview participants to give responses that will be viewed favorably by the interviewer or other participants. It occurs in all types of interviews and surveys , but is most common in semi-structured interviews , unstructured interviews , and focus groups .
Social desirability bias can be mitigated by ensuring participants feel at ease and comfortable sharing their views. Make sure to pay attention to your own body language and any physical or verbal cues, such as nodding or widening your eyes.
This type of bias can also occur in observations if the participants know they’re being observed. They might alter their behavior accordingly.
The interviewer effect is a type of bias that emerges when a characteristic of an interviewer (race, age, gender identity, etc.) influences the responses given by the interviewee.
There is a risk of an interviewer effect in all types of interviews , but it can be mitigated by writing really high-quality interview questions.
A semi-structured interview is a blend of structured and unstructured types of interviews. Semi-structured interviews are best used when:
- You have prior interview experience. Spontaneous questions are deceptively challenging, and it’s easy to accidentally ask a leading question or make a participant uncomfortable.
- Your research question is exploratory in nature. Participant answers can guide future research questions and help you develop a more robust knowledge base for future research.
An unstructured interview is the most flexible type of interview, but it is not always the best fit for your research topic.
Unstructured interviews are best used when:
- You are an experienced interviewer and have a very strong background in your research topic, since it is challenging to ask spontaneous, colloquial questions.
- Your research question is exploratory in nature. While you may have developed hypotheses, you are open to discovering new or shifting viewpoints through the interview process.
- You are seeking descriptive data, and are ready to ask questions that will deepen and contextualize your initial thoughts and hypotheses.
- Your research depends on forming connections with your participants and making them feel comfortable revealing deeper emotions, lived experiences, or thoughts.
The four most common types of interviews are:
- Structured interviews : The questions are predetermined in both topic and order.
- Semi-structured interviews : A few questions are predetermined, but other questions aren’t planned.
- Unstructured interviews : None of the questions are predetermined.
- Focus group interviews : The questions are presented to a group instead of one individual.
Deductive reasoning is commonly used in scientific research, and it’s especially associated with quantitative research .
In research, you might have come across something called the hypothetico-deductive method . It’s the scientific method of testing hypotheses to check whether your predictions are substantiated by real-world data.
Deductive reasoning is a logical approach where you progress from general ideas to specific conclusions. It’s often contrasted with inductive reasoning , where you start with specific observations and form general conclusions.
Deductive reasoning is also called deductive logic.
There are many different types of inductive reasoning that people use formally or informally.
Here are a few common types:
- Inductive generalization : You use observations about a sample to come to a conclusion about the population it came from.
- Statistical generalization: You use specific numbers about samples to make statements about populations.
- Causal reasoning: You make cause-and-effect links between different things.
- Sign reasoning: You make a conclusion about a correlational relationship between different things.
- Analogical reasoning: You make a conclusion about something based on its similarities to something else.
Inductive reasoning is a bottom-up approach, while deductive reasoning is top-down.
Inductive reasoning takes you from the specific to the general, while in deductive reasoning, you make inferences by going from general premises to specific conclusions.
In inductive research , you start by making observations or gathering data. Then, you take a broad scan of your data and search for patterns. Finally, you make general conclusions that you might incorporate into theories.
Inductive reasoning is a method of drawing conclusions by going from the specific to the general. It’s usually contrasted with deductive reasoning, where you proceed from general information to specific conclusions.
Inductive reasoning is also called inductive logic or bottom-up reasoning.
A hypothesis states your predictions about what your research will find. It is a tentative answer to your research question that has not yet been tested. For some research projects, you might have to write several hypotheses that address different aspects of your research question.
A hypothesis is not just a guess — it should be based on existing theories and knowledge. It also has to be testable, which means you can support or refute it through scientific research methods (such as experiments, observations and statistical analysis of data).
Triangulation can help:
- Reduce research bias that comes from using a single method, theory, or investigator
- Enhance validity by approaching the same topic with different tools
- Establish credibility by giving you a complete picture of the research problem
But triangulation can also pose problems:
- It’s time-consuming and labor-intensive, often involving an interdisciplinary team.
- Your results may be inconsistent or even contradictory.
There are four main types of triangulation :
- Data triangulation : Using data from different times, spaces, and people
- Investigator triangulation : Involving multiple researchers in collecting or analyzing data
- Theory triangulation : Using varying theoretical perspectives in your research
- Methodological triangulation : Using different methodologies to approach the same topic
Many academic fields use peer review , largely to determine whether a manuscript is suitable for publication. Peer review enhances the credibility of the published manuscript.
However, peer review is also common in non-academic settings. The United Nations, the European Union, and many individual nations use peer review to evaluate grant applications. It is also widely used in medical and health-related fields as a teaching or quality-of-care measure.
Peer assessment is often used in the classroom as a pedagogical tool. Both receiving feedback and providing it are thought to enhance the learning process, helping students think critically and collaboratively.
Peer review can stop obviously problematic, falsified, or otherwise untrustworthy research from being published. It also represents an excellent opportunity to get feedback from renowned experts in your field. It acts as a first defense, helping you ensure your argument is clear and that there are no gaps, vague terms, or unanswered questions for readers who weren’t involved in the research process.
Peer-reviewed articles are considered a highly credible source due to this stringent process they go through before publication.
In general, the peer review process follows the following steps:
- First, the author submits the manuscript to the editor.
- Reject the manuscript and send it back to author, or
- Send it onward to the selected peer reviewer(s)
- Next, the peer review process occurs. The reviewer provides feedback, addressing any major or minor issues with the manuscript, and gives their advice regarding what edits should be made.
- Lastly, the edited manuscript is sent back to the author. They input the edits, and resubmit it to the editor for publication.
Exploratory research is often used when the issue you’re studying is new or when the data collection process is challenging for some reason.
You can use exploratory research if you have a general idea or a specific question that you want to study but there is no preexisting knowledge or paradigm with which to study it.
Exploratory research is a methodology approach that explores research questions that have not previously been studied in depth. It is often used when the issue you’re studying is new, or the data collection process is challenging in some way.
Explanatory research is used to investigate how or why a phenomenon occurs. Therefore, this type of research is often one of the first stages in the research process , serving as a jumping-off point for future research.
Exploratory research aims to explore the main aspects of an under-researched problem, while explanatory research aims to explain the causes and consequences of a well-defined problem.
Explanatory research is a research method used to investigate how or why something occurs when only a small amount of information is available pertaining to that topic. It can help you increase your understanding of a given topic.
Clean data are valid, accurate, complete, consistent, unique, and uniform. Dirty data include inconsistencies and errors.
Dirty data can come from any part of the research process, including poor research design , inappropriate measurement materials, or flawed data entry.
Data cleaning takes place between data collection and data analyses. But you can use some methods even before collecting data.
For clean data, you should start by designing measures that collect valid data. Data validation at the time of data entry or collection helps you minimize the amount of data cleaning you’ll need to do.
After data collection, you can use data standardization and data transformation to clean your data. You’ll also deal with any missing values, outliers, and duplicate values.
Every dataset requires different techniques to clean dirty data , but you need to address these issues in a systematic way. You focus on finding and resolving data points that don’t agree or fit with the rest of your dataset.
These data might be missing values, outliers, duplicate values, incorrectly formatted, or irrelevant. You’ll start with screening and diagnosing your data. Then, you’ll often standardize and accept or remove data to make your dataset consistent and valid.
Data cleaning is necessary for valid and appropriate analyses. Dirty data contain inconsistencies or errors , but cleaning your data helps you minimize or resolve these.
Without data cleaning, you could end up with a Type I or II error in your conclusion. These types of erroneous conclusions can be practically significant with important consequences, because they lead to misplaced investments or missed opportunities.
Data cleaning involves spotting and resolving potential data inconsistencies or errors to improve your data quality. An error is any value (e.g., recorded weight) that doesn’t reflect the true value (e.g., actual weight) of something that’s being measured.
In this process, you review, analyze, detect, modify, or remove “dirty” data to make your dataset “clean.” Data cleaning is also called data cleansing or data scrubbing.
Research misconduct means making up or falsifying data, manipulating data analyses, or misrepresenting results in research reports. It’s a form of academic fraud.
These actions are committed intentionally and can have serious consequences; research misconduct is not a simple mistake or a point of disagreement but a serious ethical failure.
Anonymity means you don’t know who the participants are, while confidentiality means you know who they are but remove identifying information from your research report. Both are important ethical considerations .
You can only guarantee anonymity by not collecting any personally identifying information—for example, names, phone numbers, email addresses, IP addresses, physical characteristics, photos, or videos.
You can keep data confidential by using aggregate information in your research report, so that you only refer to groups of participants rather than individuals.
Research ethics matter for scientific integrity, human rights and dignity, and collaboration between science and society. These principles make sure that participation in studies is voluntary, informed, and safe.
Ethical considerations in research are a set of principles that guide your research designs and practices. These principles include voluntary participation, informed consent, anonymity, confidentiality, potential for harm, and results communication.
Scientists and researchers must always adhere to a certain code of conduct when collecting data from others .
These considerations protect the rights of research participants, enhance research validity , and maintain scientific integrity.
In multistage sampling , you can use probability or non-probability sampling methods .
For a probability sample, you have to conduct probability sampling at every stage.
You can mix it up by using simple random sampling , systematic sampling , or stratified sampling to select units at different stages, depending on what is applicable and relevant to your study.
Multistage sampling can simplify data collection when you have large, geographically spread samples, and you can obtain a probability sample without a complete sampling frame.
But multistage sampling may not lead to a representative sample, and larger samples are needed for multistage samples to achieve the statistical properties of simple random samples .
These are four of the most common mixed methods designs :
- Convergent parallel: Quantitative and qualitative data are collected at the same time and analyzed separately. After both analyses are complete, compare your results to draw overall conclusions.
- Embedded: Quantitative and qualitative data are collected at the same time, but within a larger quantitative or qualitative design. One type of data is secondary to the other.
- Explanatory sequential: Quantitative data is collected and analyzed first, followed by qualitative data. You can use this design if you think your qualitative data will explain and contextualize your quantitative findings.
- Exploratory sequential: Qualitative data is collected and analyzed first, followed by quantitative data. You can use this design if you think the quantitative data will confirm or validate your qualitative findings.
Triangulation in research means using multiple datasets, methods, theories and/or investigators to address a research question. It’s a research strategy that can help you enhance the validity and credibility of your findings.
Triangulation is mainly used in qualitative research , but it’s also commonly applied in quantitative research . Mixed methods research always uses triangulation.
In multistage sampling , or multistage cluster sampling, you draw a sample from a population using smaller and smaller groups at each stage.
This method is often used to collect data from a large, geographically spread group of people in national surveys, for example. You take advantage of hierarchical groupings (e.g., from state to city to neighborhood) to create a sample that’s less expensive and time-consuming to collect data from.
No, the steepness or slope of the line isn’t related to the correlation coefficient value. The correlation coefficient only tells you how closely your data fit on a line, so two datasets with the same correlation coefficient can have very different slopes.
To find the slope of the line, you’ll need to perform a regression analysis .
Correlation coefficients always range between -1 and 1.
The sign of the coefficient tells you the direction of the relationship: a positive value means the variables change together in the same direction, while a negative value means they change together in opposite directions.
The absolute value of a number is equal to the number without its sign. The absolute value of a correlation coefficient tells you the magnitude of the correlation: the greater the absolute value, the stronger the correlation.
These are the assumptions your data must meet if you want to use Pearson’s r :
- Both variables are on an interval or ratio level of measurement
- Data from both variables follow normal distributions
- Your data have no outliers
- Your data is from a random or representative sample
- You expect a linear relationship between the two variables
A well-planned research design helps ensure that your methods match your research aims, that you collect high-quality data, and that you use the right kind of analysis to answer your questions, utilizing credible sources . This allows you to draw valid , trustworthy conclusions.
The priorities of a research design can vary depending on the field, but you usually have to specify:
- Your research questions and/or hypotheses
- Your overall approach (e.g., qualitative or quantitative )
- The type of design you’re using (e.g., a survey , experiment , or case study )
- Your sampling methods or criteria for selecting subjects
- Your data collection methods (e.g., questionnaires , observations)
- Your data collection procedures (e.g., operationalization , timing and data management)
- Your data analysis methods (e.g., statistical tests or thematic analysis )
A research design is a strategy for answering your research question . It defines your overall approach and determines how you will collect and analyze data.
Questionnaires can be self-administered or researcher-administered.
Self-administered questionnaires can be delivered online or in paper-and-pen formats, in person or through mail. All questions are standardized so that all respondents receive the same questions with identical wording.
Researcher-administered questionnaires are interviews that take place by phone, in-person, or online between researchers and respondents. You can gain deeper insights by clarifying questions for respondents or asking follow-up questions.
You can organize the questions logically, with a clear progression from simple to complex, or randomly between respondents. A logical flow helps respondents process the questionnaire easier and quicker, but it may lead to bias. Randomization can minimize the bias from order effects.
Closed-ended, or restricted-choice, questions offer respondents a fixed set of choices to select from. These questions are easier to answer quickly.
Open-ended or long-form questions allow respondents to answer in their own words. Because there are no restrictions on their choices, respondents can answer in ways that researchers may not have otherwise considered.
A questionnaire is a data collection tool or instrument, while a survey is an overarching research method that involves collecting and analyzing data from people using questionnaires.
The third variable and directionality problems are two main reasons why correlation isn’t causation .
The third variable problem means that a confounding variable affects both variables to make them seem causally related when they are not.
The directionality problem is when two variables correlate and might actually have a causal relationship, but it’s impossible to conclude which variable causes changes in the other.
Correlation describes an association between variables : when one variable changes, so does the other. A correlation is a statistical indicator of the relationship between variables.
Causation means that changes in one variable brings about changes in the other (i.e., there is a cause-and-effect relationship between variables). The two variables are correlated with each other, and there’s also a causal link between them.
While causation and correlation can exist simultaneously, correlation does not imply causation. In other words, correlation is simply a relationship where A relates to B—but A doesn’t necessarily cause B to happen (or vice versa). Mistaking correlation for causation is a common error and can lead to false cause fallacy .
Controlled experiments establish causality, whereas correlational studies only show associations between variables.
- In an experimental design , you manipulate an independent variable and measure its effect on a dependent variable. Other variables are controlled so they can’t impact the results.
- In a correlational design , you measure variables without manipulating any of them. You can test whether your variables change together, but you can’t be sure that one variable caused a change in another.
In general, correlational research is high in external validity while experimental research is high in internal validity .
A correlation is usually tested for two variables at a time, but you can test correlations between three or more variables.
A correlation coefficient is a single number that describes the strength and direction of the relationship between your variables.
Different types of correlation coefficients might be appropriate for your data based on their levels of measurement and distributions . The Pearson product-moment correlation coefficient (Pearson’s r ) is commonly used to assess a linear relationship between two quantitative variables.
A correlational research design investigates relationships between two variables (or more) without the researcher controlling or manipulating any of them. It’s a non-experimental type of quantitative research .
A correlation reflects the strength and/or direction of the association between two or more variables.
- A positive correlation means that both variables change in the same direction.
- A negative correlation means that the variables change in opposite directions.
- A zero correlation means there’s no relationship between the variables.
Random error is almost always present in scientific studies, even in highly controlled settings. While you can’t eradicate it completely, you can reduce random error by taking repeated measurements, using a large sample, and controlling extraneous variables .
You can avoid systematic error through careful design of your sampling , data collection , and analysis procedures. For example, use triangulation to measure your variables using multiple methods; regularly calibrate instruments or procedures; use random sampling and random assignment ; and apply masking (blinding) where possible.
Systematic error is generally a bigger problem in research.
With random error, multiple measurements will tend to cluster around the true value. When you’re collecting data from a large sample , the errors in different directions will cancel each other out.
Systematic errors are much more problematic because they can skew your data away from the true value. This can lead you to false conclusions ( Type I and II errors ) about the relationship between the variables you’re studying.
Random and systematic error are two types of measurement error.
Random error is a chance difference between the observed and true values of something (e.g., a researcher misreading a weighing scale records an incorrect measurement).
Systematic error is a consistent or proportional difference between the observed and true values of something (e.g., a miscalibrated scale consistently records weights as higher than they actually are).
On graphs, the explanatory variable is conventionally placed on the x-axis, while the response variable is placed on the y-axis.
- If you have quantitative variables , use a scatterplot or a line graph.
- If your response variable is categorical, use a scatterplot or a line graph.
- If your explanatory variable is categorical, use a bar graph.
The term “ explanatory variable ” is sometimes preferred over “ independent variable ” because, in real world contexts, independent variables are often influenced by other variables. This means they aren’t totally independent.
Multiple independent variables may also be correlated with each other, so “explanatory variables” is a more appropriate term.
The difference between explanatory and response variables is simple:
- An explanatory variable is the expected cause, and it explains the results.
- A response variable is the expected effect, and it responds to other variables.
In a controlled experiment , all extraneous variables are held constant so that they can’t influence the results. Controlled experiments require:
- A control group that receives a standard treatment, a fake treatment, or no treatment.
- Random assignment of participants to ensure the groups are equivalent.
Depending on your study topic, there are various other methods of controlling variables .
There are 4 main types of extraneous variables :
- Demand characteristics : environmental cues that encourage participants to conform to researchers’ expectations.
- Experimenter effects : unintentional actions by researchers that influence study outcomes.
- Situational variables : environmental variables that alter participants’ behaviors.
- Participant variables : any characteristic or aspect of a participant’s background that could affect study results.
An extraneous variable is any variable that you’re not investigating that can potentially affect the dependent variable of your research study.
A confounding variable is a type of extraneous variable that not only affects the dependent variable, but is also related to the independent variable.
In a factorial design, multiple independent variables are tested.
If you test two variables, each level of one independent variable is combined with each level of the other independent variable to create different conditions.
Within-subjects designs have many potential threats to internal validity , but they are also very statistically powerful .
Advantages:
- Only requires small samples
- Statistically powerful
- Removes the effects of individual differences on the outcomes
Disadvantages:
- Internal validity threats reduce the likelihood of establishing a direct relationship between variables
- Time-related effects, such as growth, can influence the outcomes
- Carryover effects mean that the specific order of different treatments affect the outcomes
While a between-subjects design has fewer threats to internal validity , it also requires more participants for high statistical power than a within-subjects design .
- Prevents carryover effects of learning and fatigue.
- Shorter study duration.
- Needs larger samples for high power.
- Uses more resources to recruit participants, administer sessions, cover costs, etc.
- Individual differences may be an alternative explanation for results.
Yes. Between-subjects and within-subjects designs can be combined in a single study when you have two or more independent variables (a factorial design). In a mixed factorial design, one variable is altered between subjects and another is altered within subjects.
In a between-subjects design , every participant experiences only one condition, and researchers assess group differences between participants in various conditions.
In a within-subjects design , each participant experiences all conditions, and researchers test the same participants repeatedly for differences between conditions.
The word “between” means that you’re comparing different conditions between groups, while the word “within” means you’re comparing different conditions within the same group.
Random assignment is used in experiments with a between-groups or independent measures design. In this research design, there’s usually a control group and one or more experimental groups. Random assignment helps ensure that the groups are comparable.
In general, you should always use random assignment in this type of experimental design when it is ethically possible and makes sense for your study topic.
To implement random assignment , assign a unique number to every member of your study’s sample .
Then, you can use a random number generator or a lottery method to randomly assign each number to a control or experimental group. You can also do so manually, by flipping a coin or rolling a dice to randomly assign participants to groups.
Random selection, or random sampling , is a way of selecting members of a population for your study’s sample.
In contrast, random assignment is a way of sorting the sample into control and experimental groups.
Random sampling enhances the external validity or generalizability of your results, while random assignment improves the internal validity of your study.
In experimental research, random assignment is a way of placing participants from your sample into different groups using randomization. With this method, every member of the sample has a known or equal chance of being placed in a control group or an experimental group.
“Controlling for a variable” means measuring extraneous variables and accounting for them statistically to remove their effects on other variables.
Researchers often model control variable data along with independent and dependent variable data in regression analyses and ANCOVAs . That way, you can isolate the control variable’s effects from the relationship between the variables of interest.
Control variables help you establish a correlational or causal relationship between variables by enhancing internal validity .
If you don’t control relevant extraneous variables , they may influence the outcomes of your study, and you may not be able to demonstrate that your results are really an effect of your independent variable .
A control variable is any variable that’s held constant in a research study. It’s not a variable of interest in the study, but it’s controlled because it could influence the outcomes.
Including mediators and moderators in your research helps you go beyond studying a simple relationship between two variables for a fuller picture of the real world. They are important to consider when studying complex correlational or causal relationships.
Mediators are part of the causal pathway of an effect, and they tell you how or why an effect takes place. Moderators usually help you judge the external validity of your study by identifying the limitations of when the relationship between variables holds.
If something is a mediating variable :
- It’s caused by the independent variable .
- It influences the dependent variable
- When it’s taken into account, the statistical correlation between the independent and dependent variables is higher than when it isn’t considered.
A confounder is a third variable that affects variables of interest and makes them seem related when they are not. In contrast, a mediator is the mechanism of a relationship between two variables: it explains the process by which they are related.
A mediator variable explains the process through which two variables are related, while a moderator variable affects the strength and direction of that relationship.
There are three key steps in systematic sampling :
- Define and list your population , ensuring that it is not ordered in a cyclical or periodic order.
- Decide on your sample size and calculate your interval, k , by dividing your population by your target sample size.
- Choose every k th member of the population as your sample.
Systematic sampling is a probability sampling method where researchers select members of the population at a regular interval – for example, by selecting every 15th person on a list of the population. If the population is in a random order, this can imitate the benefits of simple random sampling .
Yes, you can create a stratified sample using multiple characteristics, but you must ensure that every participant in your study belongs to one and only one subgroup. In this case, you multiply the numbers of subgroups for each characteristic to get the total number of groups.
For example, if you were stratifying by location with three subgroups (urban, rural, or suburban) and marital status with five subgroups (single, divorced, widowed, married, or partnered), you would have 3 x 5 = 15 subgroups.
You should use stratified sampling when your sample can be divided into mutually exclusive and exhaustive subgroups that you believe will take on different mean values for the variable that you’re studying.
Using stratified sampling will allow you to obtain more precise (with lower variance ) statistical estimates of whatever you are trying to measure.
For example, say you want to investigate how income differs based on educational attainment, but you know that this relationship can vary based on race. Using stratified sampling, you can ensure you obtain a large enough sample from each racial group, allowing you to draw more precise conclusions.
In stratified sampling , researchers divide subjects into subgroups called strata based on characteristics that they share (e.g., race, gender, educational attainment).
Once divided, each subgroup is randomly sampled using another probability sampling method.
Cluster sampling is more time- and cost-efficient than other probability sampling methods , particularly when it comes to large samples spread across a wide geographical area.
However, it provides less statistical certainty than other methods, such as simple random sampling , because it is difficult to ensure that your clusters properly represent the population as a whole.
There are three types of cluster sampling : single-stage, double-stage and multi-stage clustering. In all three types, you first divide the population into clusters, then randomly select clusters for use in your sample.
- In single-stage sampling , you collect data from every unit within the selected clusters.
- In double-stage sampling , you select a random sample of units from within the clusters.
- In multi-stage sampling , you repeat the procedure of randomly sampling elements from within the clusters until you have reached a manageable sample.
Cluster sampling is a probability sampling method in which you divide a population into clusters, such as districts or schools, and then randomly select some of these clusters as your sample.
The clusters should ideally each be mini-representations of the population as a whole.
If properly implemented, simple random sampling is usually the best sampling method for ensuring both internal and external validity . However, it can sometimes be impractical and expensive to implement, depending on the size of the population to be studied,
If you have a list of every member of the population and the ability to reach whichever members are selected, you can use simple random sampling.
The American Community Survey is an example of simple random sampling . In order to collect detailed data on the population of the US, the Census Bureau officials randomly select 3.5 million households per year and use a variety of methods to convince them to fill out the survey.
Simple random sampling is a type of probability sampling in which the researcher randomly selects a subset of participants from a population . Each member of the population has an equal chance of being selected. Data is then collected from as large a percentage as possible of this random subset.
Quasi-experimental design is most useful in situations where it would be unethical or impractical to run a true experiment .
Quasi-experiments have lower internal validity than true experiments, but they often have higher external validity as they can use real-world interventions instead of artificial laboratory settings.
A quasi-experiment is a type of research design that attempts to establish a cause-and-effect relationship. The main difference with a true experiment is that the groups are not randomly assigned.
Blinding is important to reduce research bias (e.g., observer bias , demand characteristics ) and ensure a study’s internal validity .
If participants know whether they are in a control or treatment group , they may adjust their behavior in ways that affect the outcome that researchers are trying to measure. If the people administering the treatment are aware of group assignment, they may treat participants differently and thus directly or indirectly influence the final results.
- In a single-blind study , only the participants are blinded.
- In a double-blind study , both participants and experimenters are blinded.
- In a triple-blind study , the assignment is hidden not only from participants and experimenters, but also from the researchers analyzing the data.
Blinding means hiding who is assigned to the treatment group and who is assigned to the control group in an experiment .
A true experiment (a.k.a. a controlled experiment) always includes at least one control group that doesn’t receive the experimental treatment.
However, some experiments use a within-subjects design to test treatments without a control group. In these designs, you usually compare one group’s outcomes before and after a treatment (instead of comparing outcomes between different groups).
For strong internal validity , it’s usually best to include a control group if possible. Without a control group, it’s harder to be certain that the outcome was caused by the experimental treatment and not by other variables.
An experimental group, also known as a treatment group, receives the treatment whose effect researchers wish to study, whereas a control group does not. They should be identical in all other ways.
Individual Likert-type questions are generally considered ordinal data , because the items have clear rank order, but don’t have an even distribution.
Overall Likert scale scores are sometimes treated as interval data. These scores are considered to have directionality and even spacing between them.
The type of data determines what statistical tests you should use to analyze your data.
A Likert scale is a rating scale that quantitatively assesses opinions, attitudes, or behaviors. It is made up of 4 or more questions that measure a single attitude or trait when response scores are combined.
To use a Likert scale in a survey , you present participants with Likert-type questions or statements, and a continuum of items, usually with 5 or 7 possible responses, to capture their degree of agreement.
In scientific research, concepts are the abstract ideas or phenomena that are being studied (e.g., educational achievement). Variables are properties or characteristics of the concept (e.g., performance at school), while indicators are ways of measuring or quantifying variables (e.g., yearly grade reports).
The process of turning abstract concepts into measurable variables and indicators is called operationalization .
There are various approaches to qualitative data analysis , but they all share five steps in common:
- Prepare and organize your data.
- Review and explore your data.
- Develop a data coding system.
- Assign codes to the data.
- Identify recurring themes.
The specifics of each step depend on the focus of the analysis. Some common approaches include textual analysis , thematic analysis , and discourse analysis .
There are five common approaches to qualitative research :
- Grounded theory involves collecting data in order to develop new theories.
- Ethnography involves immersing yourself in a group or organization to understand its culture.
- Narrative research involves interpreting stories to understand how people make sense of their experiences and perceptions.
- Phenomenological research involves investigating phenomena through people’s lived experiences.
- Action research links theory and practice in several cycles to drive innovative changes.
Hypothesis testing is a formal procedure for investigating our ideas about the world using statistics. It is used by scientists to test specific predictions, called hypotheses , by calculating how likely it is that a pattern or relationship between variables could have arisen by chance.
Operationalization means turning abstract conceptual ideas into measurable observations.
For example, the concept of social anxiety isn’t directly observable, but it can be operationally defined in terms of self-rating scores, behavioral avoidance of crowded places, or physical anxiety symptoms in social situations.
Before collecting data , it’s important to consider how you will operationalize the variables that you want to measure.
When conducting research, collecting original data has significant advantages:
- You can tailor data collection to your specific research aims (e.g. understanding the needs of your consumers or user testing your website)
- You can control and standardize the process for high reliability and validity (e.g. choosing appropriate measurements and sampling methods )
However, there are also some drawbacks: data collection can be time-consuming, labor-intensive and expensive. In some cases, it’s more efficient to use secondary data that has already been collected by someone else, but the data might be less reliable.
Data collection is the systematic process by which observations or measurements are gathered in research. It is used in many different contexts by academics, governments, businesses, and other organizations.
There are several methods you can use to decrease the impact of confounding variables on your research: restriction, matching, statistical control and randomization.
In restriction , you restrict your sample by only including certain subjects that have the same values of potential confounding variables.
In matching , you match each of the subjects in your treatment group with a counterpart in the comparison group. The matched subjects have the same values on any potential confounding variables, and only differ in the independent variable .
In statistical control , you include potential confounders as variables in your regression .
In randomization , you randomly assign the treatment (or independent variable) in your study to a sufficiently large number of subjects, which allows you to control for all potential confounding variables.
A confounding variable is closely related to both the independent and dependent variables in a study. An independent variable represents the supposed cause , while the dependent variable is the supposed effect . A confounding variable is a third variable that influences both the independent and dependent variables.
Failing to account for confounding variables can cause you to wrongly estimate the relationship between your independent and dependent variables.
To ensure the internal validity of your research, you must consider the impact of confounding variables. If you fail to account for them, you might over- or underestimate the causal relationship between your independent and dependent variables , or even find a causal relationship where none exists.
Yes, but including more than one of either type requires multiple research questions .
For example, if you are interested in the effect of a diet on health, you can use multiple measures of health: blood sugar, blood pressure, weight, pulse, and many more. Each of these is its own dependent variable with its own research question.
You could also choose to look at the effect of exercise levels as well as diet, or even the additional effect of the two combined. Each of these is a separate independent variable .
To ensure the internal validity of an experiment , you should only change one independent variable at a time.
No. The value of a dependent variable depends on an independent variable, so a variable cannot be both independent and dependent at the same time. It must be either the cause or the effect, not both!
You want to find out how blood sugar levels are affected by drinking diet soda and regular soda, so you conduct an experiment .
- The type of soda – diet or regular – is the independent variable .
- The level of blood sugar that you measure is the dependent variable – it changes depending on the type of soda.
Determining cause and effect is one of the most important parts of scientific research. It’s essential to know which is the cause – the independent variable – and which is the effect – the dependent variable.
In non-probability sampling , the sample is selected based on non-random criteria, and not every member of the population has a chance of being included.
Common non-probability sampling methods include convenience sampling , voluntary response sampling, purposive sampling , snowball sampling, and quota sampling .
Probability sampling means that every member of the target population has a known chance of being included in the sample.
Probability sampling methods include simple random sampling , systematic sampling , stratified sampling , and cluster sampling .
Using careful research design and sampling procedures can help you avoid sampling bias . Oversampling can be used to correct undercoverage bias .
Some common types of sampling bias include self-selection bias , nonresponse bias , undercoverage bias , survivorship bias , pre-screening or advertising bias, and healthy user bias.
Sampling bias is a threat to external validity – it limits the generalizability of your findings to a broader group of people.
A sampling error is the difference between a population parameter and a sample statistic .
A statistic refers to measures about the sample , while a parameter refers to measures about the population .
Populations are used when a research question requires data from every member of the population. This is usually only feasible when the population is small and easily accessible.
Samples are used to make inferences about populations . Samples are easier to collect data from because they are practical, cost-effective, convenient, and manageable.
There are seven threats to external validity : selection bias , history, experimenter effect, Hawthorne effect , testing effect, aptitude-treatment and situation effect.
The two types of external validity are population validity (whether you can generalize to other groups of people) and ecological validity (whether you can generalize to other situations and settings).
The external validity of a study is the extent to which you can generalize your findings to different groups of people, situations, and measures.
Cross-sectional studies cannot establish a cause-and-effect relationship or analyze behavior over a period of time. To investigate cause and effect, you need to do a longitudinal study or an experimental study .
Cross-sectional studies are less expensive and time-consuming than many other types of study. They can provide useful insights into a population’s characteristics and identify correlations for further research.
Sometimes only cross-sectional data is available for analysis; other times your research question may only require a cross-sectional study to answer it.
Longitudinal studies can last anywhere from weeks to decades, although they tend to be at least a year long.
The 1970 British Cohort Study , which has collected data on the lives of 17,000 Brits since their births in 1970, is one well-known example of a longitudinal study .
Longitudinal studies are better to establish the correct sequence of events, identify changes over time, and provide insight into cause-and-effect relationships, but they also tend to be more expensive and time-consuming than other types of studies.
Longitudinal studies and cross-sectional studies are two different types of research design . In a cross-sectional study you collect data from a population at a specific point in time; in a longitudinal study you repeatedly collect data from the same sample over an extended period of time.
Longitudinal study | Cross-sectional study |
---|---|
observations | Observations at a in time |
Observes the multiple times | Observes (a “cross-section”) in the population |
Follows in participants over time | Provides of society at a given point |
There are eight threats to internal validity : history, maturation, instrumentation, testing, selection bias , regression to the mean, social interaction and attrition .
Internal validity is the extent to which you can be confident that a cause-and-effect relationship established in a study cannot be explained by other factors.
In mixed methods research , you use both qualitative and quantitative data collection and analysis methods to answer your research question .
The research methods you use depend on the type of data you need to answer your research question .
- If you want to measure something or test a hypothesis , use quantitative methods . If you want to explore ideas, thoughts and meanings, use qualitative methods .
- If you want to analyze a large amount of readily-available data, use secondary data. If you want data specific to your purposes with control over how it is generated, collect primary data.
- If you want to establish cause-and-effect relationships between variables , use experimental methods. If you want to understand the characteristics of a research subject, use descriptive methods.
A confounding variable , also called a confounder or confounding factor, is a third variable in a study examining a potential cause-and-effect relationship.
A confounding variable is related to both the supposed cause and the supposed effect of the study. It can be difficult to separate the true effect of the independent variable from the effect of the confounding variable.
In your research design , it’s important to identify potential confounding variables and plan how you will reduce their impact.
Discrete and continuous variables are two types of quantitative variables :
- Discrete variables represent counts (e.g. the number of objects in a collection).
- Continuous variables represent measurable amounts (e.g. water volume or weight).
Quantitative variables are any variables where the data represent amounts (e.g. height, weight, or age).
Categorical variables are any variables where the data represent groups. This includes rankings (e.g. finishing places in a race), classifications (e.g. brands of cereal), and binary outcomes (e.g. coin flips).
You need to know what type of variables you are working with to choose the right statistical test for your data and interpret your results .
You can think of independent and dependent variables in terms of cause and effect: an independent variable is the variable you think is the cause , while a dependent variable is the effect .
In an experiment, you manipulate the independent variable and measure the outcome in the dependent variable. For example, in an experiment about the effect of nutrients on crop growth:
- The independent variable is the amount of nutrients added to the crop field.
- The dependent variable is the biomass of the crops at harvest time.
Defining your variables, and deciding how you will manipulate and measure them, is an important part of experimental design .
Experimental design means planning a set of procedures to investigate a relationship between variables . To design a controlled experiment, you need:
- A testable hypothesis
- At least one independent variable that can be precisely manipulated
- At least one dependent variable that can be precisely measured
When designing the experiment, you decide:
- How you will manipulate the variable(s)
- How you will control for any potential confounding variables
- How many subjects or samples will be included in the study
- How subjects will be assigned to treatment levels
Experimental design is essential to the internal and external validity of your experiment.
I nternal validity is the degree of confidence that the causal relationship you are testing is not influenced by other factors or variables .
External validity is the extent to which your results can be generalized to other contexts.
The validity of your experiment depends on your experimental design .
Reliability and validity are both about how well a method measures something:
- Reliability refers to the consistency of a measure (whether the results can be reproduced under the same conditions).
- Validity refers to the accuracy of a measure (whether the results really do represent what they are supposed to measure).
If you are doing experimental research, you also have to consider the internal and external validity of your experiment.
A sample is a subset of individuals from a larger population . Sampling means selecting the group that you will actually collect data from in your research. For example, if you are researching the opinions of students in your university, you could survey a sample of 100 students.
In statistics, sampling allows you to test a hypothesis about the characteristics of a population.
Quantitative research deals with numbers and statistics, while qualitative research deals with words and meanings.
Quantitative methods allow you to systematically measure variables and test hypotheses . Qualitative methods allow you to explore concepts and experiences in more detail.
Methodology refers to the overarching strategy and rationale of your research project . It involves studying the methods used in your field and the theories or principles behind them, in order to develop an approach that matches your objectives.
Methods are the specific tools and procedures you use to collect and analyze data (for example, experiments, surveys , and statistical tests ).
In shorter scientific papers, where the aim is to report the findings of a specific study, you might simply describe what you did in a methods section .
In a longer or more complex research project, such as a thesis or dissertation , you will probably include a methodology section , where you explain your approach to answering the research questions and cite relevant sources to support your choice of methods.
Ask our team
Want to contact us directly? No problem. We are always here for you.
- Email [email protected]
- Start live chat
- Call +1 (510) 822-8066
- WhatsApp +31 20 261 6040
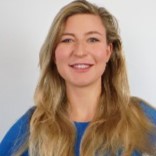
Our team helps students graduate by offering:
- A world-class citation generator
- Plagiarism Checker software powered by Turnitin
- Innovative Citation Checker software
- Professional proofreading services
- Over 300 helpful articles about academic writing, citing sources, plagiarism, and more
Scribbr specializes in editing study-related documents . We proofread:
- PhD dissertations
- Research proposals
- Personal statements
- Admission essays
- Motivation letters
- Reflection papers
- Journal articles
- Capstone projects
Scribbr’s Plagiarism Checker is powered by elements of Turnitin’s Similarity Checker , namely the plagiarism detection software and the Internet Archive and Premium Scholarly Publications content databases .
The add-on AI detector is powered by Scribbr’s proprietary software.
The Scribbr Citation Generator is developed using the open-source Citation Style Language (CSL) project and Frank Bennett’s citeproc-js . It’s the same technology used by dozens of other popular citation tools, including Mendeley and Zotero.
You can find all the citation styles and locales used in the Scribbr Citation Generator in our publicly accessible repository on Github .
Research Design: Types and Research Methods
Research design definition, research design types, and research design methods.
A Research Design is a methodical, well-organized procedure utilized by a researcher, or a scientist to carry out a scientific study. It is a comprehensive co-existence of already identified elements and any other information or data leading to a reasonable end result.
The research design is required to follow a pre-planned, well-thought-out methodology, in agreement with the pre-selected research type, in order to come up with an error-free, authentic conclusion.
What is the purpose of Research Design?
A Research Design provides a scientist, or a researcher with a well-structured, objective plan of study that enables him or her to efficiently assess causes and effect relationships between various dependent and independent variables such as the Classic Controlled Experiment.
What are the types of Research Design?
A Research Design is necessary since it enables the smooth sailing of the varying research components, thus making your research more reliable, efficient, and flexible.
There are four major types of research designs, which include:
- Descriptive Research
As the name implies, this is an in-depth sort of research design that answers what and how.
- Explanatory Research
This sort of research design explains the subject of the research and thereby answers what, why, and how.
- Exploratory Research
This sort of research explores the subject matter and answers what and how.
- Evaluation Research
This sort of research determines the productivity of the subject matter, or a program and is, therefore, quite extensive.
The Types of Evaluation Research are:
- Quantitative Research
- Qualitative Research
The Types of Quantitative Research are:
- Descriptive Design
- Correlational Design
- Quasi-Experimental Design
- Experimental Design
What is Quantitative Research Design?
Quantitative Research – A Quantitative Research analyzes variable relationships in terms of numbers and statistical strategies to review findings, and is generally divided into four main types, namely:
This sort of design describes the present status of a phenomenon, or a variable, and does not require any hypothesis for initiation: It is developed only after the data is collected. For instance; case-study , naturalistic observation , survey s, etc.
This sort of design utilizes statistical analysis in order to determine whether two variables are related or not e.g., case-control study , observational study .
This sort of design resembles a true experimental design, and is utilized when a standard research design is not applicable, however; it is not based upon randomized sample groups.
This sort of design determines cause and effect relationships among various variables in such a way that the independent variable is changed in order to observe its effect on the dependent variable e.g., experiment with random assignment.
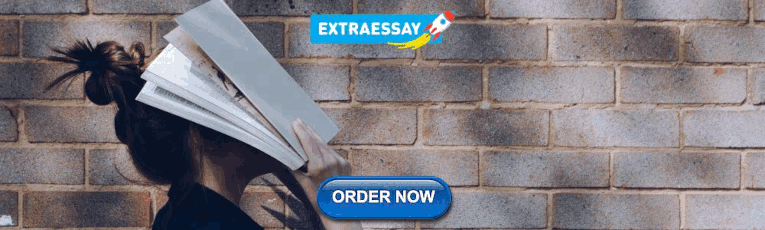
What is Qualitative Research Design?
Qualitative Research – A Quantitative Research is known as exploratory research since it is utilized to explore the answers to ‘what’ and ‘how’ rather than just predicting the results of research and it may share certain features with the research, such as:
- A central question that states the objective, or the problem;
- Has a pre-set procedure or sequence in order to answer research questions;
- Reviews the generated data;
- The collection and analysis of data are done in order to draw conclusions.
What is the difference between Fixed Design and Flexible Design?
A Fixed Design generally follows a pre-set or pre-determined design, or a sequence, before the collection of data, and is usually driven by theory. Additionally, the variables are usually measured via quantitative research.
A Flexible Design, on the other hand, offers freedom when it comes to the collection of data. This is usually because the variable, in this case, is not measured quantitatively e.g., culture. In a few cases, the theory may not even be available before the actual research begins.
How do you Create a Research Design?
- Pinpoint your learning outcomes, objectives, etc.;
- Pick out your research questions;
- Develop a research design;
- Choose a sampling framework;
- Pick suitable methods of data collection;
- Set up appropriate measurement instruments;
- Identify proper data analysis techniques, tools, etc.;
- Contemplate dissemination and publication of your findings.
What is the difference between Research Design and Approach?
A Research Design is the overall framework, or outline, or structure of a research proposal whereas a Research Approach incorporates various tools, techniques, procedures or processes utilized to collect or review data or information.
A Research Design demonstrates how your selected research design or method is applied to tackle a specific research question while a Research Approach utilizes different methods to answer different research questions.
What makes a good Research Design?
A good Research Design is usually characterized by the following features:
- A good research design ought to be flexible, effective, efficient, appropriate, reliable, economical, etc.
- A good research design ought to increase reliability and decrease bias of any data or information collected and reviewed.
- The good research design must be less prone to an error whenever it comes to experiments, surveys, etc.
Nevertheless, your research design is based upon your research problem, research objective, or any other research questions that you have. Thus, you may even be required to combine a variety of research designs and approaches in order to accomplish your research goal.
What is the Research Method?
A Research Method is a well-structured, logical, or a standard plan utilized by a researcher, or a scientist in order to conduct research.
For instance, Sociologists, during the course of their research, depending on both qualitative and quantitative research methods such as experiments, primary or secondary data collection, surveys, participant study or observation, etc.
What are the characteristics of Research Design?
A good research design ought to maximize reliability and minimize bigotries regarding the data collected and reviewed. Additionally, a research design ought to be error-free, thus a design that provides the least experimental error ought to be selected.
Yousaf Saeed
Related articles.
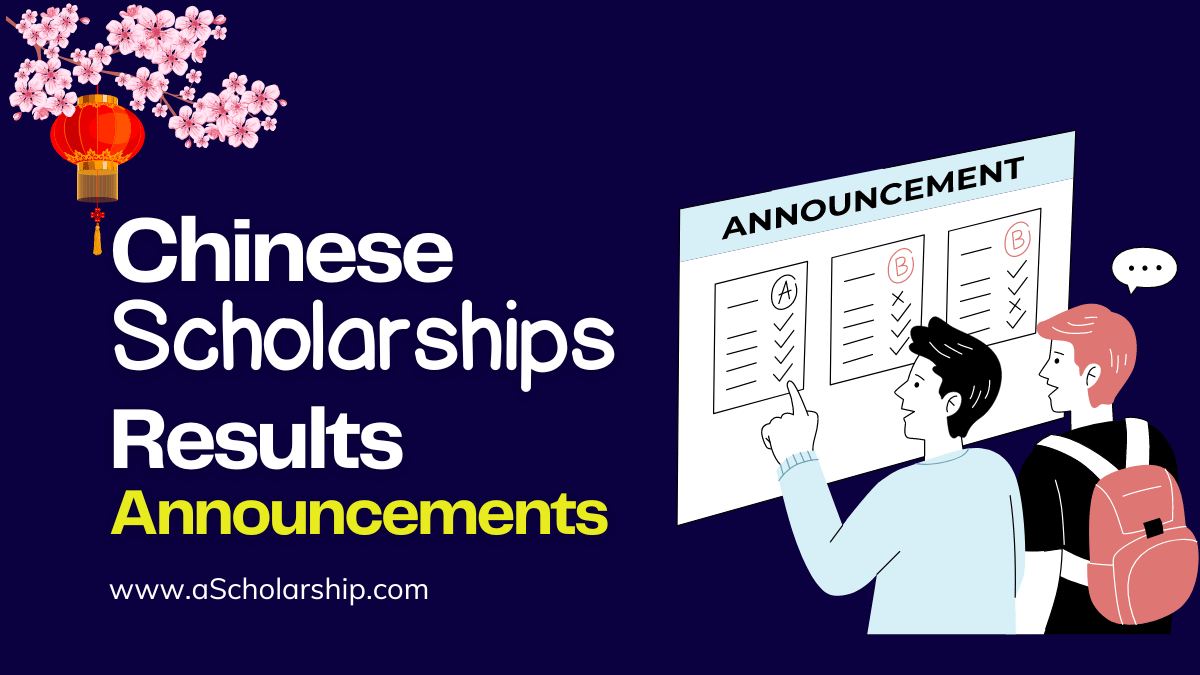
China Scholarships Result Announcement 2024 – When to Expect?
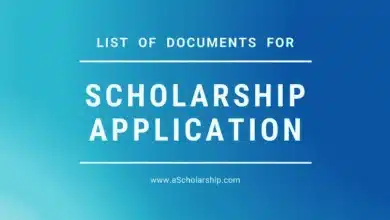
Documents Checklist for Scholarship Applications in 2024
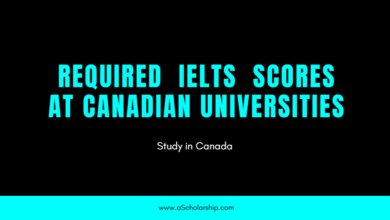
IELTS Score Required in Canadian Universities for Admissions in 2024
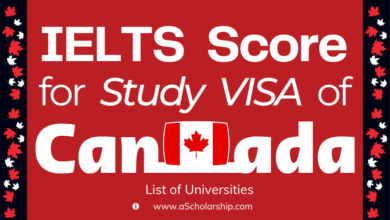
IELTS Scores for Canadian Study VISA and University Admissions in 2024
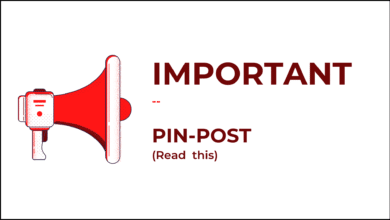
List of Fully Funded International Scholarships 2024-2025 for You
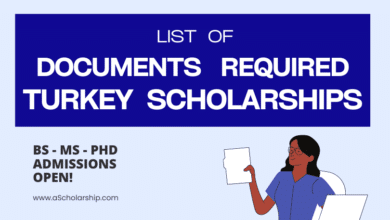
Documents to Apply for Turkey Government Scholarships 2023
Leave a reply cancel reply.
Your email address will not be published. Required fields are marked *
Save my name, email, and website in this browser for the next time I comment.
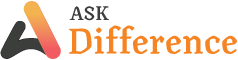
Research Methods vs. Research Design — What's the Difference?

Difference Between Research Methods and Research Design
Table of contents, key differences, comparison chart, interdependence, compare with definitions, research methods, research design, common curiosities, are surveys and interviews considered research methods, what's the primary difference between research methods and research design, what's an example of research design, which comes first: choosing research methods or formulating research design, is research design decided before selecting research methods, can a study have multiple research methods, why is research design important, can research design impact the study's outcomes, are research methods specific to disciplines, are case studies a research method or research design, how does one decide on research methods, what's the relationship between hypothesis and research design, can research design change during a study, can one research design utilize both qualitative and quantitative methods, are there standard research methods and designs for all studies, share your discovery.

Author Spotlight

Popular Comparisons
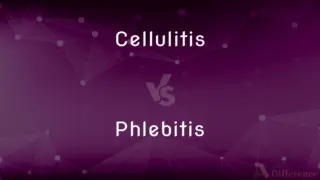
Trending Comparisons
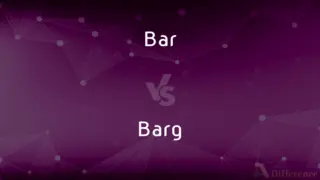
New Comparisons

Trending Terms
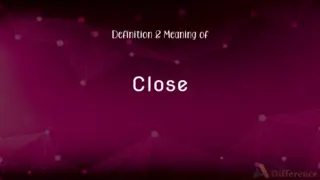
Exploring Differences in Automotive Logo Design Between Chinese and Western Cultures Based on Hofstedes Cultural Dimensions Theory
- Communications in Humanities Research 37(1):129-134
- 37(1):129-134
- This person is not on ResearchGate, or hasn't claimed this research yet.
Abstract and Figures

Discover the world's research
- 25+ million members
- 160+ million publication pages
- 2.3+ billion citations
- Pierre Bourdieu
- Geert Hofstede

- Recruit researchers
- Join for free
- Login Email Tip: Most researchers use their institutional email address as their ResearchGate login Password Forgot password? Keep me logged in Log in or Continue with Google Welcome back! Please log in. Email · Hint Tip: Most researchers use their institutional email address as their ResearchGate login Password Forgot password? Keep me logged in Log in or Continue with Google No account? Sign up
Which artificial sweetener is the safest choice?
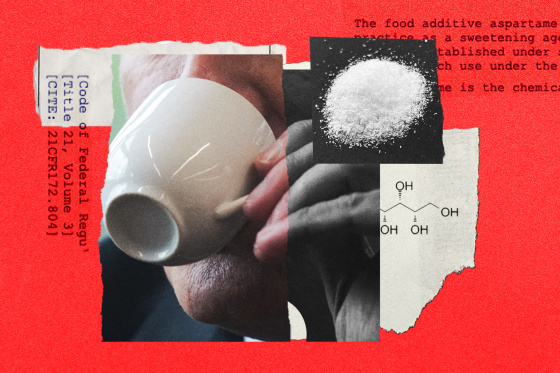
There’s mounting evidence that artificial sweeteners may be linked to heart disease and other possible health risks. Scientists say the findings are far from definitive, however, with some leading researchers calling for better-designed clinical trials investigating the long-term health effects of sugar substitutes.
That’s why, in separate trials, researchers are actively working to get a clearer understanding of how artificial sweeteners affect blood glucose levels, gut microbiome health and the cardiovascular system. Some studies are beginning to compare the alternatives against each other, while others hope to learn how they affect the body compared to sugar.
As it is, it’s difficult for consumers to determine which sugar alternative carries the fewest health risks. Most of the research is observational , meaning it doesn’t prove cause and effect. In some cases, researchers looked at people who ate nonsugar sweeteners, analyzed their incidence of certain health risks like heart attacks or diabetes, then noted associations between the two.
All the widely consumed alternatives such as saccharin, aspartame, sucralose, stevia, xylitol and erythritol are approved by the Food and Drug Administration. They’re found in countless products including sports drinks, energy bars, yogurts, cereals, beverages, candies, baked goods and syrups.
Even with FDA approval, Dr. Dariush Mozaffarian, a cardiologist and professor of nutrition science and policy at Tufts University, said “they’re all potentially worrisome and all understudied.”
In recent research, cardiologist Dr. Stanley Hazen at the Cleveland Clinic found that the high concentrations of the sugar alcohol sweeteners xylitol and erythritol may cause the platelets in the blood to become more sticky and prone to clotting, in turn raising the risk of heart attack and stroke. The phenomenon is similar to what happens with high cholesterol, Hazen said. If they get big enough, the clots can block blood flow through crucial veins and arteries.
Some experts say that instead of trying to pinpoint the safest nonsugar sweetener, better studies need to determine whether there’s a benefit to swapping out sugar in the first place.
After publishing research finding a connection between erythritol and increased risk of heart attack and stroke , Hazen and his colleagues conducted the first head-to-head human trial comparing the effects of consuming erythritol versus sugar on the blood platelets that control clotting. The results of that study are pending publication.
Vasanti Malik, an assistant professor of nutritional sciences at the University of Toronto, meanwhile, is conducting a study of more than 500 people directly comparing the health effects of drinking sugar-sweetened beverages, noncaloric sweeteners or water. Malik and her colleagues plan to measure obesity and heart health over time.
At Virginia Tech, registered dietitian Valisa Hedrick is working with the National Institutes of Health on another study comparing the effects of four different artificial sweeteners versus sugar on blood glucose levels and gut microbiome health. The study, which focuses on people with prediabetes, is a controlled feeding trial, meaning participants only eat the meals that NIH provides them, and nothing more.
This is important, Hedrick said, because one of the growing concerns with nonsugar sweeteners is that the products trick the brain in such a way that they increase sugar cravings. People may then end up eating more sugar throughout the day, spiking their blood glucose.
With a controlled study, the researchers can answer whether the sweeteners themselves raise blood glucose directly — not the sugar people could otherwise eat later.
The limits of sweetener studies
A research bias called reverse causation can make it difficult to draw decisive conclusions from prior studies, Malik said.
People often change their diets after they start developing diabetes or putting on weight, Malik said. These people, generally, are most likely to switch from sugar to nonsugar sweeteners. This is where the reverse causation comes into play.
“You get a spurious association between the intake of nonsugar sweeteners and the risk for diabetes,” she said. That is, the data ends up suggesting that these sweeteners are causing health problems that already existed.
Many studies also rely on people to report whether they’ve consumed nonsugar substitutes, which can be unreliable. Names like xylitol can be buried in a long list of ingredients.
Other studies, meanwhile — like Hazen’s erythritol and xylitol studies — may focus directly on what happens in the body after someone consumes one of these sweeteners, but they tend to enroll small numbers of people and track them only for a short time.
“A lot of these studies are really hard to interpret,” said Dr. Michelle Pearlman, a gastroenterologist and the CEO and co-founder of the Prime Institute in Miami. “And the problem is that there’s no head-to-head trials of people eating candy bars versus xylitol, so I can’t make any blanket statements recommending one or the other.”
Both Hedrick and Malik hope to share results from their respective studies in the next several years.
“We need experimental science alongside more rigorous observational research,” Malik said. “There are trials underway, and I think in the next five years we’ll have more clarity on the topic. We’re just not quite there.”
In a statement, the Calorie Control Council, an industry trade group representing more than two dozen sweetener manufacturers, said studies linking alternative sweeteners to health risks are based on flawed research and that the products are safe.
“It is irresponsible to amplify faulty research to those who look to alternative sweeteners to reduce overall sugar intake as well as the millions who use it as a tool to manage their health conditions, including obesity and diabetes,” Carla Saunders, the trade group’s president, said in the statement.
Why it’s important to know
Most low-calorie and sugar-free foods contain at least one sugar substitute, and many contain several. These products are growing more popular, especially in the U.S. By 2033, market research suggests sugar substitutes could be worth more than $28.57 billion.
“They’re ubiquitous,” Mozafarrian said. “And they’re proliferating because people have become so obsessed with avoiding sugar.”
Mozaffarian said these sweeteners soared in popularity following changes to U.S. nutrition labeling requirements in 2016 .
The change required manufacturers to list added sugars on a separate line beneath total sugars. The idea was to help consumers differentiate between foods with naturally occurring sugars, like fruit and plain Greek yogurt, and foods that had sugars mixed in.
“Now, the food industry has a big incentive to make that ‘added sugars’ number as small as possible,” he said. “So you’re seeing these compounds in everything, and we still don’t have enough information on them.”
Some products are labeled as “artificial sweeteners” or “natural sweeteners” based on whether they’re derived from natural sources or chemically engineered.
Even natural sweeteners go through heavy chemical processing, said Dr. Maria Carolina Delgado-Lelievre, a cardiologist at the University of Miami.
For example, stevia comes from processed stevia plant extract, monk fruit sweetener comes from processing a chemical in a gourdlike fruit grown in China, and sucralose is a chemically altered version of sugar about 600 times sweeter, according to the FDA .
Aspartame and saccharin are from human-made fusions of amino acids and chemicals.
Many of these sweeteners are so potent in tiny quantities that they’re mixed with xylitol or erythritol to bulk them up and fill a packet, said the Cleveland Clinic’s Hazen.
Given this label confusion, Hedrick said researchers are increasingly using the term “nonsugar sweeteners.”
Health risks of added sugars
Sugar, of course, is one of the country’s most pressing public health problems. Especially in soda and juice, excess sugar fuels the ongoing obesity epidemic , contributing to heart disease, liver disease, cancer and diabetes .
However, there’s a big difference between processed, concentrated sugars like high-fructose corn syrup and the natural sugars found in fruits, Pearlman, the Miami gastroenterologist, said. Processed sugars are highly addictive.
“Anything with high-fructose corn syrup stimulates the same reward centers in our brain as cocaine and heroin,” she said. “Natural sugars from fruit act differently in the body.”
Sugar’s bad rap has much more to do with the quantity people consume than any intrinsically bad property, experts agree.
“Added sugar is nuanced,” Mozaffarian said. “When you try to take that very real nuance and turn it into a simple message, you get the industry misleading consumers that foods are ‘not good.’”
A little bit of added sugar in otherwise healthy foods, he said — such as lightly sweetened whole-grain cereals — is usually OK.
“The harms of these different nonsugar sweeteners have been greatly underemphasized and the harms of small amounts of added sugar have been overemphasized,” he argued.
Sugar substitutes for children?
The U.S. government’s Dietary Guidelines for Americans recommend that anyone over the age of 2 consume less than 10% of their daily calories from added sugar, or the equivalent of roughly 12 teaspoons of added sugar. In reality, as of 2018, people in the U.S., including children, were consuming about 17 teaspoons of added sugar per day, on average.
Recently, the U.S. Department of Agriculture implemented a new rule limiting added sugars in public school lunches . Michael Goran, a professor of pediatrics at the University of Southern California’s Keck School of Medicine, said he worries that schools will replace sugary foods with artificially sweetened foods to comply with the new rules.
“There’s this general perception that these sweeteners are safe alternatives, but if they’re broadly applied to children, I unfortunately think that’s very risky,” he said.
Mozaffarian said that at their current levels of added sugar, most yogurts would no longer be allowed in school lunches once the new rule goes into effect.
“They’re just above the new limit, so it’s likely these yogurts are now going to be made with a series of sweeteners with uncertain health effects,” Mozaffarian said.
In the meantime, Pearlman said, it’s easy to see they haven’t helped the population become healthier on the whole.
“We have more chronic disease, more diabetes today than we’ve ever had before,” she said. “That shows that despite the diet industry being worth billions of dollars, we’re clearly missing the ball.”
A confusing body of limited research, coupled with the lack of clarity on food labels, puts consumers in a tough position when it comes to selecting the healthiest choices, the experts concluded.
All agreed on the best solution:
- Eat as many whole, unprocessed foods as you can.
- The less processed a food, the less likely it is to be loaded with added sugars or nonsugar sweeteners.
“If I had the choice of eating a store-bought cookie with a lot of sweeteners in it, a store-bought cookie with monk fruit, or a homemade cookie with sugar, I would choose the homemade cookie,” Goran said. “You can still enjoy the cookie, but maybe put a little less sugar in there.”
NBC News contributor Caroline Hopkins is a health and science journalist who covers cancer treatment for Precision Oncology News. She is a graduate of the Columbia University Graduate School of Journalism.
- Skip to main content
- Skip to search
- Skip to footer
Products and Services

Cisco Security
Master your goals. innovate. we'll tackle threats..
Get powerful security across all your networks, cloud, endpoints, and email to protect everything that matters, from anywhere.
If it's connected, you're protected

Cisco Security “The Hacker”
More connected users and devices creates more complexity. Cisco Security Cloud makes security easier for IT and safer for everyone anywhere security meets the network.
Deliver smarter, stronger security
Protect your organization across a multicloud environment, while simplifying security operations, improving scalability, and driving data-informed outcomes, powered by Cisco Talos.
Unlock better user experiences
Create a seamless experience that frustrates attackers, not users, by granting access from any device, anywhere, and adding more proactive security controls.
Deliver cost-effective defenses
Improve ROI by consolidating vendors, reducing complexity and integrating your security.
Strengthen security resilience
Unified, end-to-end protection maximizes value, minimizes risk, and closes security gaps everywhere to defend against evolving threats. Protect access, apps, and innovation across your network to secure your future.
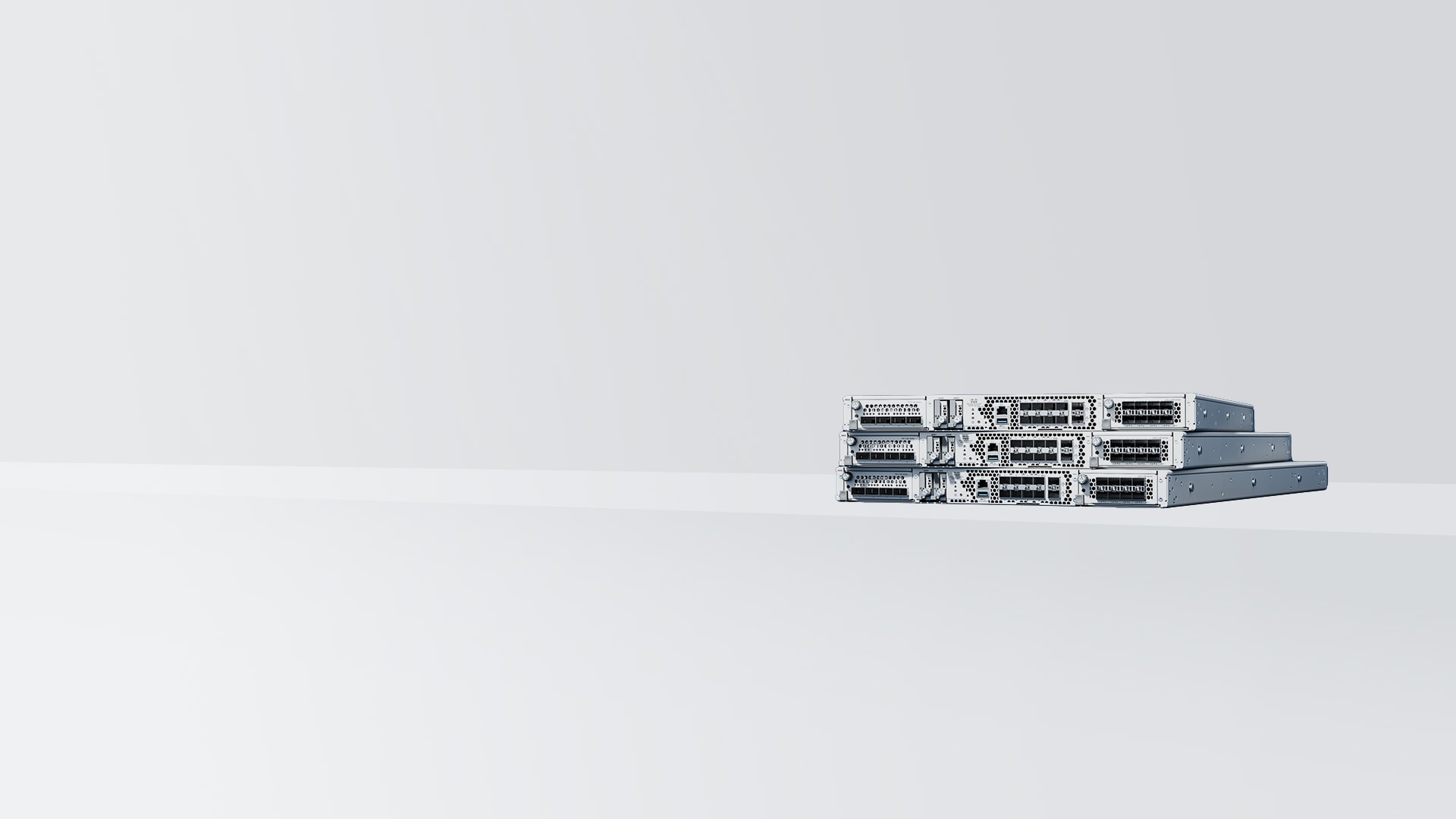
Cisco Secure Firewall
Better visibility and actionable insights across networks, clouds, endpoints, and email allows users to respond confidently to the most sophisticated threats at machine scale.
Featured security products
Cisco hypershield.
A new groundbreaking security architecture that makes hyperscaler technology accessible to enterprises of all sizes and delivers AI-native security for modern data centers and cloud.
Cisco Secure Access (SSE)
A converged cybersecurity solution, grounded in zero trust, that radically reduces risk and delights both end users and IT staff by safely connecting anything to anywhere.
Detect the most sophisticated threats sooner across all vectors and prioritize by impact for faster responses.
Cisco Multicloud Defense
Gain multidirectional protection across clouds to stop inbound attacks, data exfiltration, and lateral movement.
Secure applications and enable frictionless access with strong MFA and more. Establish user and device trust, gain visibility into devices, and enable secure access to all apps.
Cisco Identity Services Engine (ISE)
Simplify highly secure network access control with software-defined access and automation.
Security Suites delivered by Cisco Security Cloud
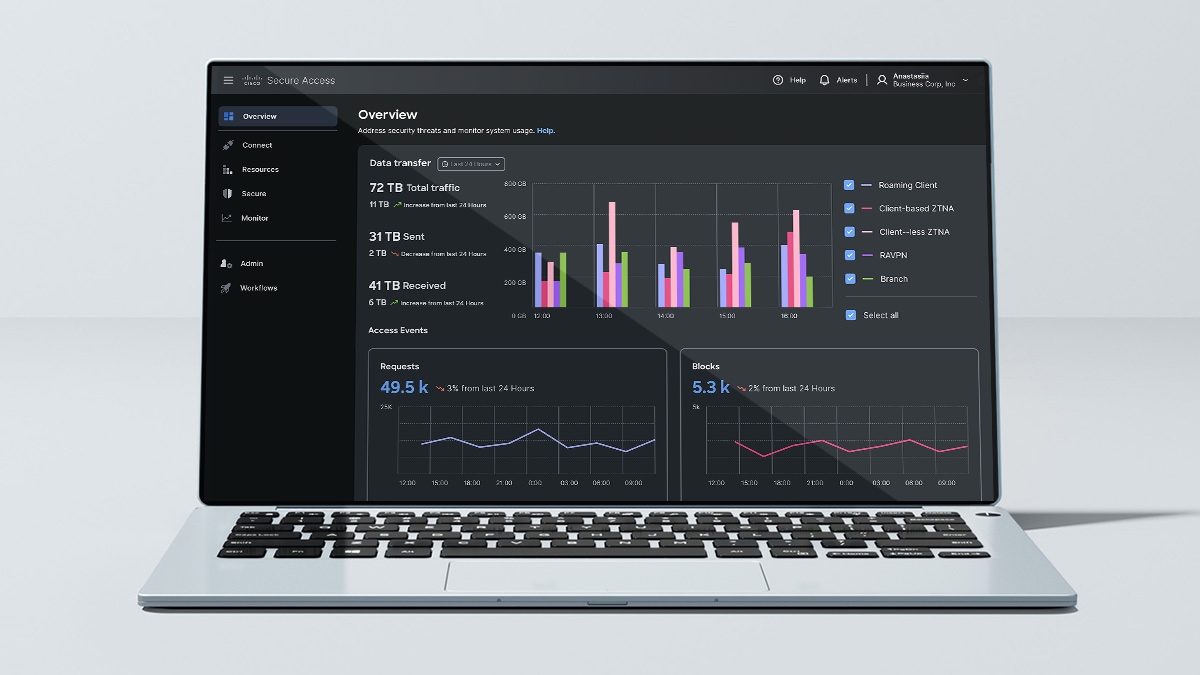
Cisco User Protection Suite
Get secure access to any application, on any device, from anywhere. Defend against threats targeting users and deliver seamless access for hybrid work.
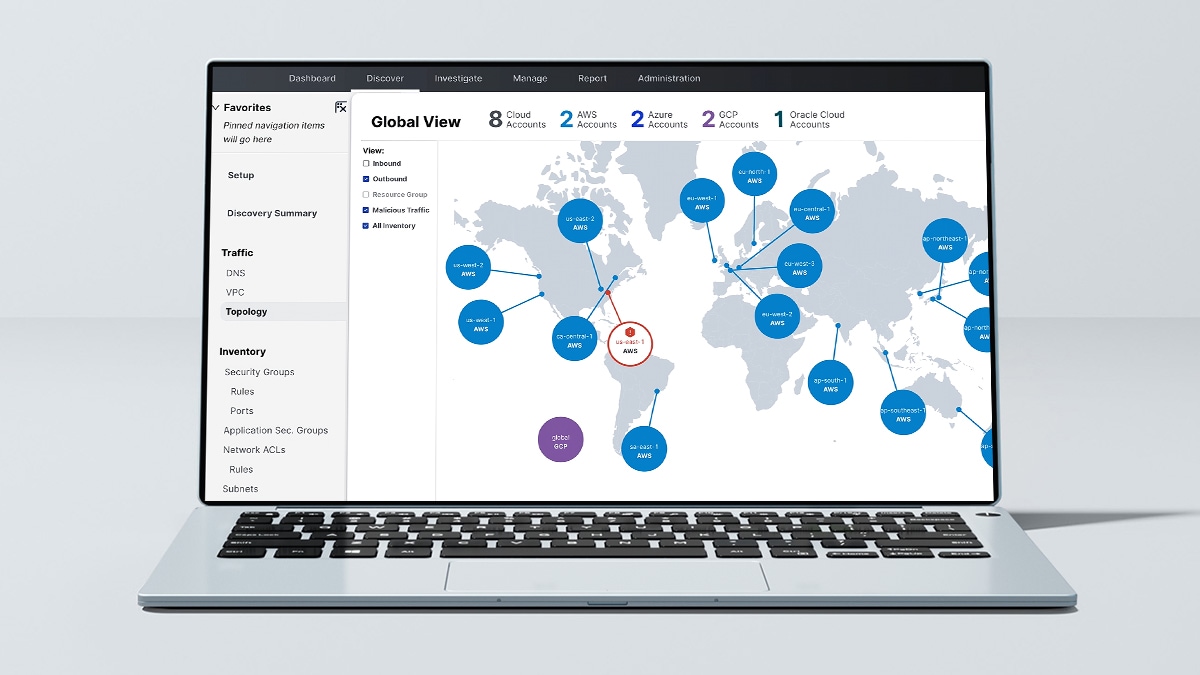
Cisco Cloud Protection Suite
Secure your apps and data with a powerful, flexible framework for a hybrid and multicloud world.
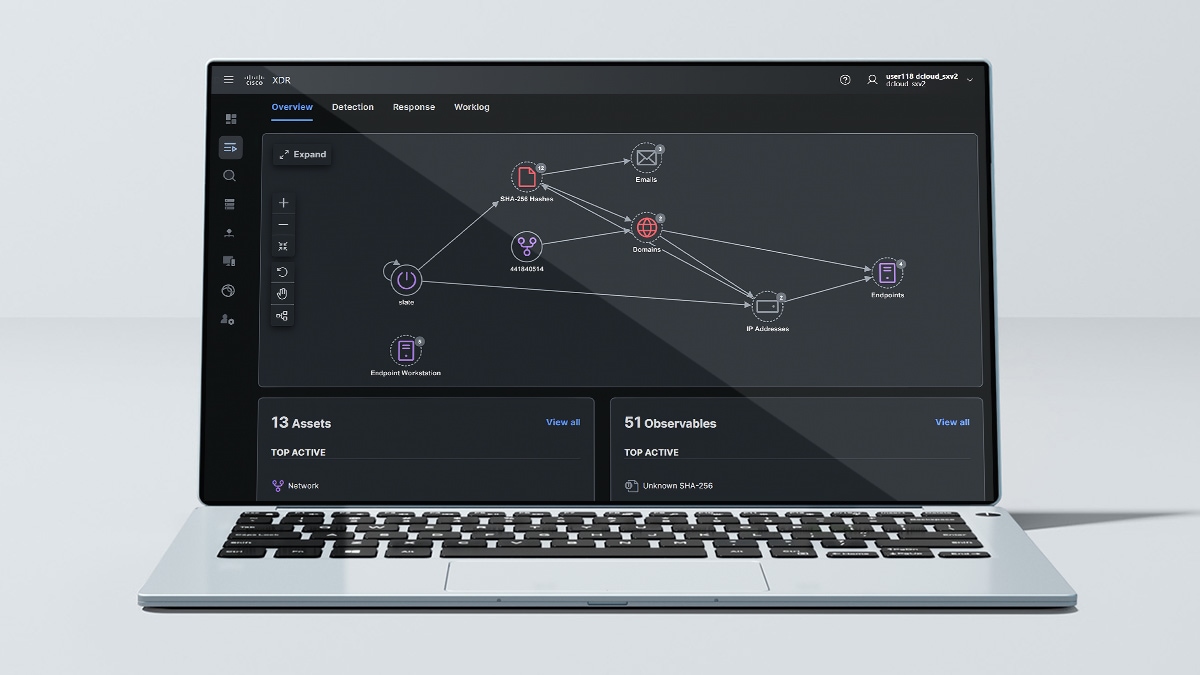
Cisco Breach Protection Suite
Secure your business by investigating, prioritizing, and resolving incidents through unified defense and contextual insights from data-backed, AI-powered security.
Customer stories and insights
Global partnerships fight to end child exploitation together.

"Marriott has long championed human rights and human trafficking awareness. Combating CSAM is an important extension of that work. The IWF provided the level of rigor we needed in a URL list, and Cisco's security technology provided the means to easily apply it."
Abbe Horswill, Director, Human Rights and Social Impact
Company: Marriott International
The NFL relies on Cisco
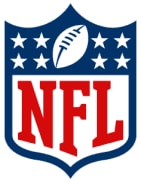
"From securing stadiums, broadcasts, and fans to protecting the largest live sporting event in America, the right tools and the right team are key in making sure things run smoothly, avoiding disruptions to the game, and safeguarding the data and devices that make mission-critical gameday operations possible."
Add value to security solutions
Cisco Security Enterprise Agreement
Instant savings
Experience security software buying flexibility with one easy-to-manage agreement.
Services for security
Let the experts secure your business
Get more from your investments and enable constant vigilance to protect your organization.
Sharpen your security insights
Cisco Cybersecurity Viewpoints
Set your vision to a more secure future with Cisco Cybersecurity Viewpoints. With specialized content from podcasts to industry news, you'll walk away with a deeper understanding of the trends, research, and topics in our rapidly changing world.
Amazon FBA vs FBM Comparison Guide: Which One is Better for Your Amazon Business?
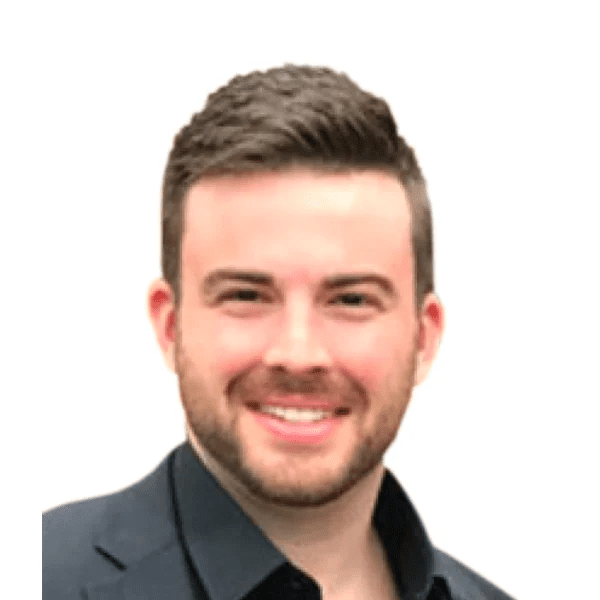
Brian Connolly
August 8, 2024
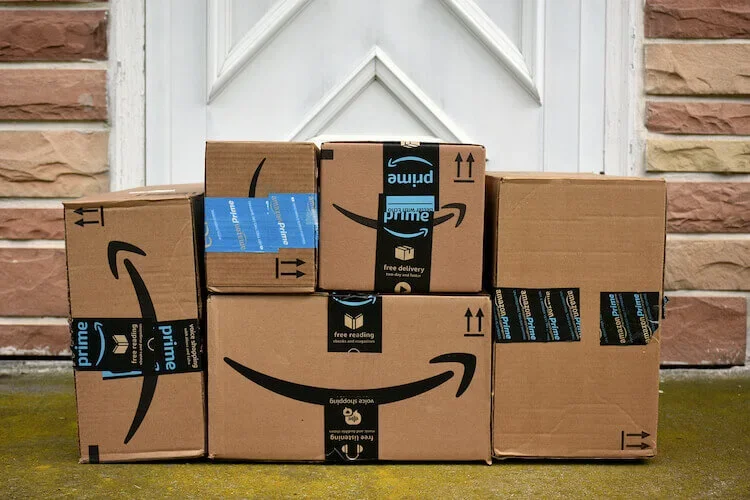
Table of Contents
What’s the difference between amazon fba and fbm, which amazon fulfillment method should sellers use, 7 factors to consider before choosing a fulfillment method on amazon, what are the key differences between fba and fbm sellers.
Last year, more than 60% of sales on Amazon came from third-party sellers — most of which are small to medium-sized businesses. Based on Jungle Scout’s 2024 State of the Seller Report , we know that nearly all Amazon sellers have different methods of finding success on Amazon.
A key differentiator among Amazon sellers is the fulfillment method they choose: Fulfillment by Amazon (FBA) and/or Fulfillment by Merchant (FBM).
Nearly all Amazon sellers (82%) use Amazon FBA, and over a third (34%) use Amazon FBM. Broken out, Amazon sellers sell:
- FBA only: 64%
- FBA and FBM: 14%
- FBM only: 22%
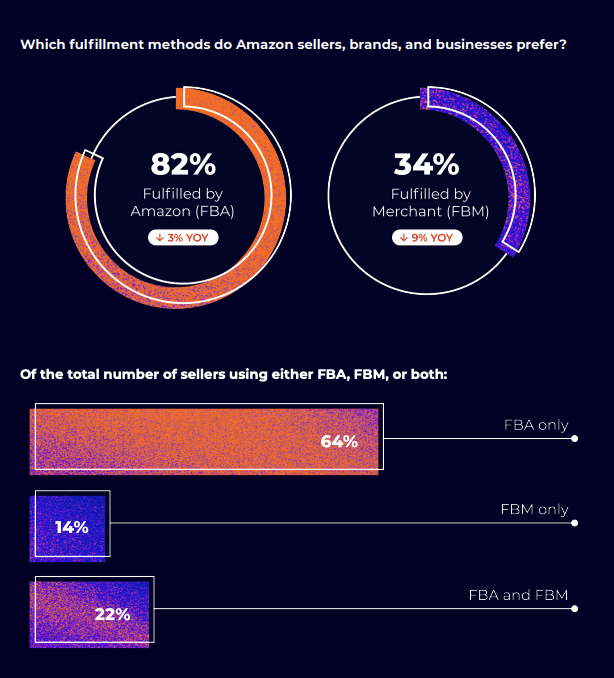
We’ll take a look at the primary differences between these two fulfillment methods — including which requires more experience and which is more profitable — as well as the factors you should consider when choosing whether to fulfill with Amazon FBA or FBM (or both!).
Here are the main differences between Amazon FBA and FBM:
- Fulfillment by Amazon (FBA): A fulfillment method on Amazon in which a seller (or a seller’s supplier) sends their products directly to Amazon’s fulfillment centers. Amazon then stores the inventory and handles the picking, packing, and shipping of all your orders — they’ll even handle customer service for orders fulfilled with FBA.
- Fulfillment by Merchant (FBM): A fulfillment method on Amazon in which a seller lists their products on Amazon, but manages all storage, shipping, and customer support themselves (or through a third-party fulfillment center ).
For both small businesses or at-home sellers to major brands with elaborate supply chains, all sorts of Amazon sellers can benefit from both Amazon FBA and FBM fulfillment methods. But how do you choose what’s right for your business?
There are seven major factors you should consider before you select a fulfillment method on Amazon.
- Size and weight of the product
- Control of customer experience
- Seller feedback
- Inventory turnover rate
- Expenses and fees
- Prime badge
Below, we’ll go into full detail on each of those factors, but first, is a quick overview of which fulfillment method you should use based on the 6 factors.
You should use FBM if…
- You want more control over your customers
- Excellent customer service practices are in place
- The products you sell are oversized or heavy
- You already have logistics in place
- You’re able to mitigate the expenses involved in fulfilling your own products
- Your inventory turns over slowly
You should use FBA if…
- The products you sell are small and lightweight
- You’re okay surrendering control to your customers to Amazon
- You want Amazon to handle your customer service
- Your inventory turns over quickly
- You do not have logistics in place
- Your expenses would be higher if you fulfilled your own products
Can Amazon sellers use both FBA and FBM fulfillment methods?
Yes. In fact, Jungle Scout’s data indicates that 9% of Amazon sellers sell both FBA and FBM.
If you have a large variety of products, you may consider using both to benefit from the two.
For example, imagine you sell two types of products. One product is oversized and turns over slowly. The second product is small and turns over quickly. To maximize your profits and minimize your expenses, you would use FBM to sell the large/slow-selling product and FBA to sell the small/fast-selling product.
You can also create an FBA and FBM offer for the same product under one ASIN . Some sellers do this in case their FBA inventory runs out but are still able to fulfill orders via FBM.
While FBA is an amazing service that most Amazon sellers take advantage of, it may not work for every product sold on the platform. Let’s go over the top 6 factors you should consider when choosing how to fulfill your products on Amazon.
1. Item size/weight
Amazon’s fee structure and logistics are beneficial to FBA sellers who have small, lightweight products that turnover (sell) quickly. Inversely, Amazon’s fees (specifically FBA fees and storage fees) are not conducive to selling oversized, heavy products.
To decide which fulfillment method works best for your product, you can use Amazon’s FBA revenue calculator to forecast fees and expenses.
Here’s an example of where FBM may make more sense for your business than FBA.Let’s say you are selling a non-inflatable kayak. Something of that size will take up a lot of space in Amazon’s fulfillment centers and be costly to ship. Assuming you have the space and capabilities to ship a kayak, it will likely be more profitable to ship an item of this size yourself.
Let’s use this product on Amazon as an example.
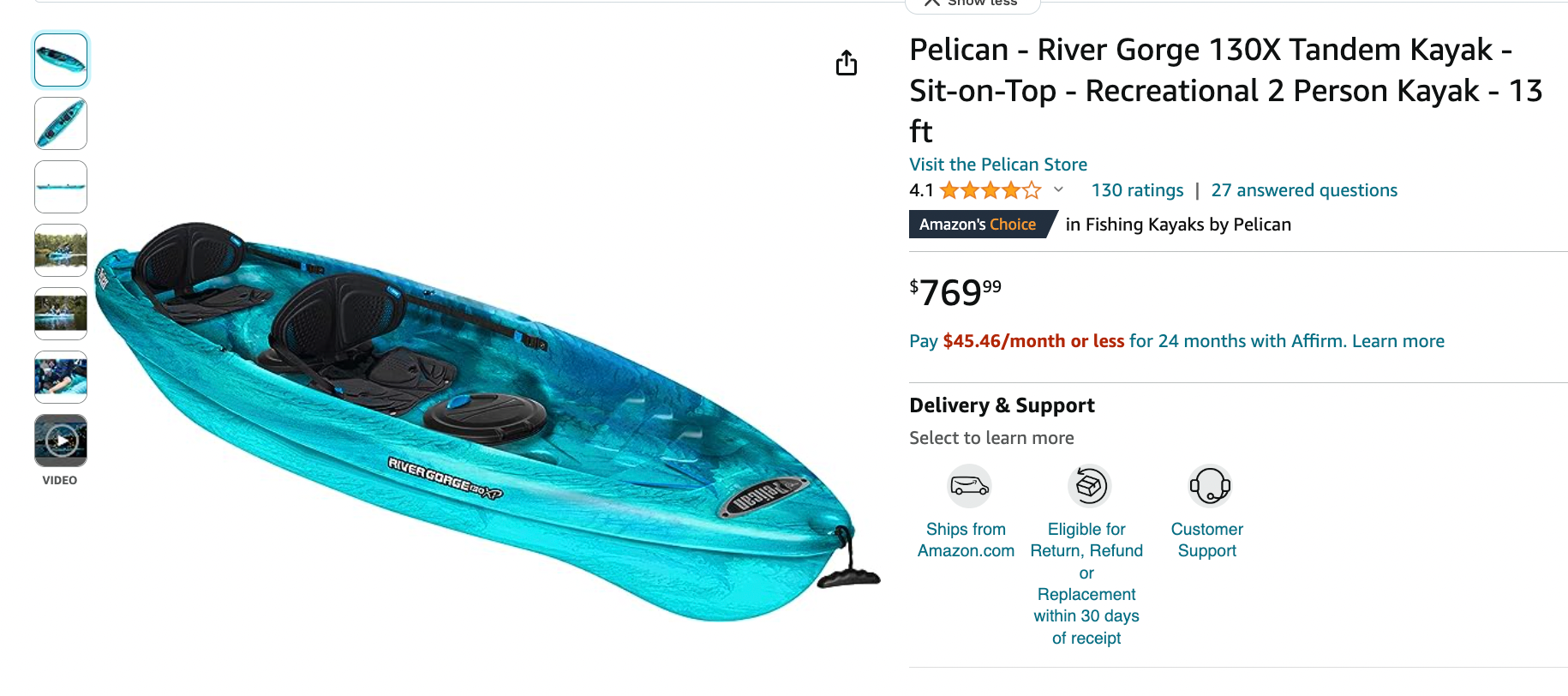
We’ll plug the ASIN of this product into the Amazon Revenue Calculator to get a breakdown of the fees for both FBA and FBM.
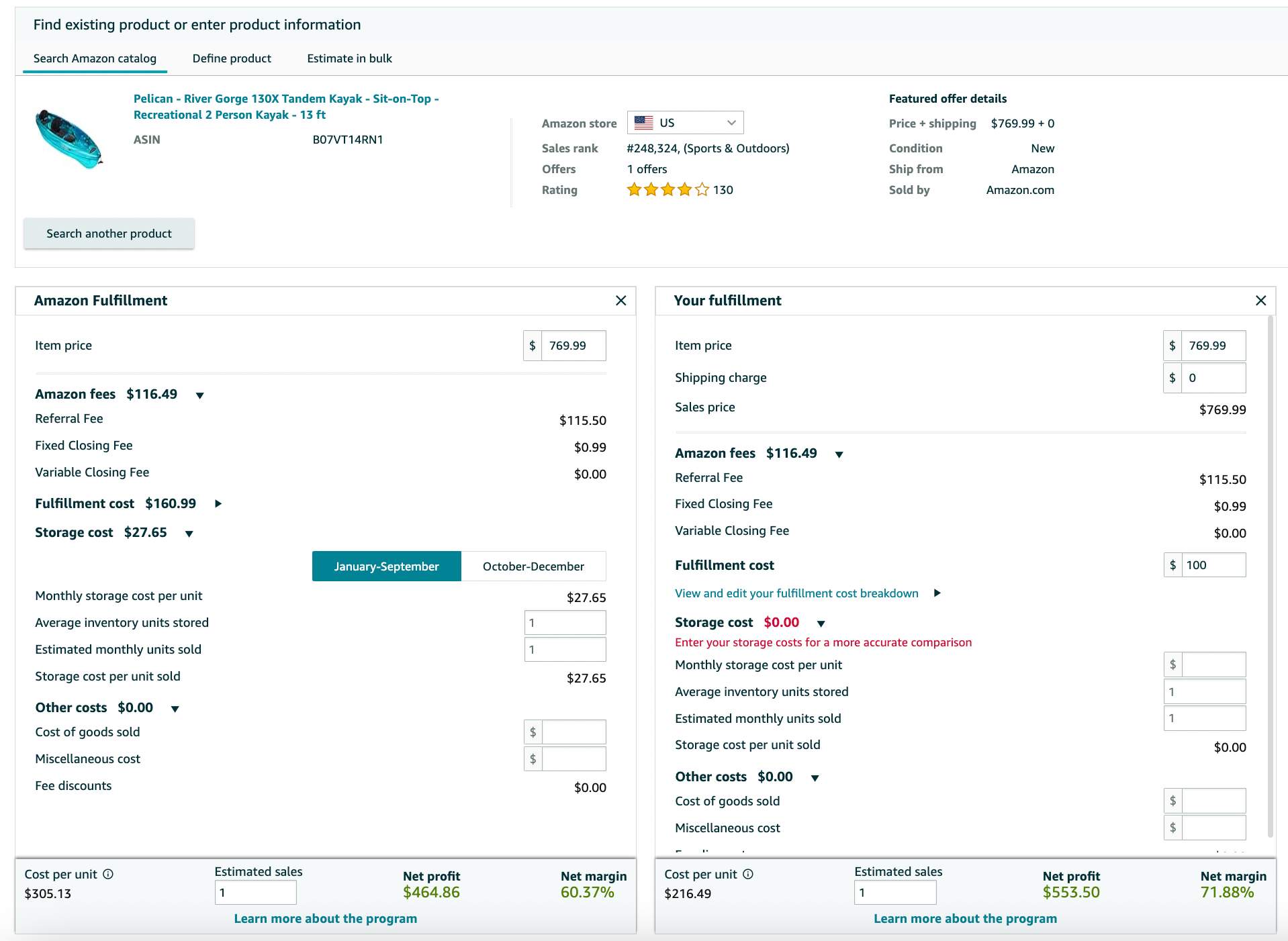
Before inputting the costs of goods, it will cost $305.13 in just Amazon fees and fulfillment fees if you use FBA. For the FBM example, I input the fulfillment cost as $100. If we can ship this product for that amount, we would profit roughly $100 more per unit — plus, save on monthly storage fees ($27.65 per unit) if a product this size was stored in FBA.
Make sure you calculate your products costs before deciding which fulfillment method works best.
2. Control of customer experience
FBM sellers store their own products, ship their own products, and handle all of the customer service for their Amazon sales.
On the other hand, sellers who use FBA use Amazon to store and ship their products and handle customer service on their behalf. FBA sellers rarely, if ever, speak with their customers. As such, FBM sellers have greater control over their customer’s experience.
If you want a more hands-off approach, especially when dealing with issues such as customer returns, FBA is the option for you.
3. Seller feedback
Amazon’s seller feedback system is a way for customers to communicate to Amazon their satisfaction with third party sellers and the resulting transactions.
Seller feedback has two components:
- Star rating: Like a product review, the shoppers can rate the seller’s performance on a scale of 1-5.
- Comments: In addition to the star rating, shoppers can leave comments on why they granted a certain rating to the seller.
Note that the seller rating is different from a product rating/review. The seller rating only covers the elements of the actual transaction such as shipping, whether or not the product matched its description on Amazon, and seller communication.
Because Amazon handles the majority of the steps involved in fulfilling FBA products, FBA sellers have less to worry about when it comes to seller feedback. In fact, if Amazon handles an FBA seller’s transaction and the seller receives negative feedback, the seller can request for the feedback to be removed.
Because FBM sellers have more control over their transactions, they are more susceptible to receiving negative seller feedback on Amazon. As such, FBM sellers must focus more on the condition of the products they send, the speed at which they send them, and how they handle all communications with sellers.
4. Turnover rates
A seller’s turnover rate is the speed in which they sell and restock inventory. An FBA seller’s turnover rate is important because Amazon tracks the length of time that inventory remains in these fulfillment centers.
The longer a product stays in a fulfillment center, the more storage fees the product accrues. Then, if products are stored in an Amazon fulfillment center for 180 days or longer, your inventory will start incurring an aged inventory surcharge (previously known as a long-term storage fee) and it will negatively affect your IPI score and ability to send more inventory to FBA .
READ MORE | How to Improve Your Amazon Inventory Performance Index Score
Therefore, sellers with products that have slower turnover rates should consider fulfilling their own products to avoid Amazon’s expensive storage fees.
5. Logistics
Picking, packing, and shipping one’s own products can be incredibly time consuming. Therefore, it’s recommended that a seller just starting with Amazon who does not already have their own fulfillment logistics in place start with Amazon FBA. That way, they can focus on the other important elements of building a business using Amazon.
For sellers who are adding Amazon as a new sales channel and already have logistics in place, they may still want to review whether or not Amazon FBA can save them money on fulfillment, especially if they sell and ship high-turnover products.
Amazon FBA sellers must pay FBA fees to ship goods via Amazon’s fulfillment network. FBA fees cover the costs to pick, pack, and ship the goods to the consumer. In a way, they are “shipping and handling expenses” that Amazon charges.
While Amazon FBM sellers do not have to pay FBA fees, they must still consider the expenses involved in handling and shipping their own products. This includes storage costs, labor costs, product packaging costs, and the actual cost to ship the product.
If these costs are greater than what the fees would be to let Amazon handle the fulfillment, the seller should consider using Amazon FBA.
7. Prime badge
Products that are fulfilled by Amazon will automatically be eligible for Prime shipping and will feature the famous Prime badge on your listing. There are over 200 million Amazon Prime members worldwide, and they’re looking for Prime products that will arrive to their house in 1-2 days.
Prime shipping eligibility is a huge advantage of using FBA over FBM. Though, there is a way to offer Prime shipping as an FBM seller with Seller-Fulfilled Prime.
READ MORE | Amazon Seller-Fulfilled Prime 2023 Guide
Beyond the methods they use to fulfill their products, we discovered a few key differences between sellers who use FBA and those who use FBM — according to data from our 2023 State of the Seller Report.
Which is more profitable: Amazon FBA vs FBM?
FBM sellers have more sales and larger profit margins.
- 28% of FBM sellers earn more than $25,000 per month in revenue versus 12% of FBA sellers
- 35% of FBA sellers have profit margins over 20% versus 38% of FBM sellers
Which sellers get started selling faster: Amazon FBA vs FBM?
FBM sellers launched their Amazon businesses faster than FBA sellers.
- 42% of FBM sellers took less than two months to get started on Amazon versus 41% of FBA sellers
- 15% of FBM sellers realized a profit in fewer than three months versus 14% of FBA sellers
Which method requires less time to manage: Amazon FBA vs FBM?
FBA sellers spend a similar amount of time on their business as FBM sellers.
- 22% of FBM sellers spend 11-20 hours per week in the Amazon business versus 22% of FBA sellers
- Both FBM and FBA sellers attribute their success on Amazon to having time to commit to their businesses (53% and 52%, respectively)
What are the top product categories for FBA and FBM?
Fulfillment by amazon.
Home & Kitchen | 30% |
Toys & Games | 15% |
Sports & Outdoors | 14% |
Health, Household & Baby Care | 16% |
Beauty & Personal Care | 24% |
Kitchen & Dining | 30% |
Office Products | 12% |
Tools & Home Improvement | 10% |
11% | |
Garden & Outdoor | 11% |
Fulfillment by Merchant
Home & Kitchen | 25% |
Health, Household & Baby Care | 18% |
Toys & Games | 17% |
Sports & Outdoors | 16% |
Beauty & Personal Care | 26% |
Tools & Home Improvement | 13% |
Books | 16% |
Office Products | 14% |
Garden & Outdoor | 14% |
Kitchen & Dining | 25% |
Other findings: Selling Amazon FBA vs FBM
FBA sellers are heavily focused on private label sales, while FBM sellers engage in other sales models at much higher rates.
- 55% of FBA sellers use the private label business model versus 44% of FBM sellers
- 35% of FBM sellers use the wholesale business model versus 26% of FBA sellers
FBM sellers tend to start with less start-up capital
- 17% of FBM sellers started with $2,501-$5,000 versus 18% of FBA sellers
- 46% of FBA sellers started with more than $5,000 versus 43% of FBM sellers
What fulfillment method do you use for your Amazon business?
We hope this article gives you a better understanding on the differences between FBA and FBM. Which fulfillment method do you use for your business? Let us know in the comments.
Take your Amazon business to the next level.
Leverage Jungle Scout’s industry-leading Amazon intelligence to start and scale your brand.
Ecommerce Expert & Writer at Jungle Scout
Brian Connolly is an Amazon seller, ecommerce expert, and writer for Jungle Scout. He lives in the New Jersey Shore area with his wife and cat. When he isn’t writing advice online for aspiring and experienced Amazon sellers for Jungle Scout, he spends his free time boating, fishing, and selling boating-themed items on his Amazon business.
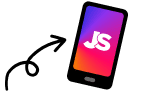
Stay in the Amazon seller loop
Thank you for subscribing!
Recommended Posts
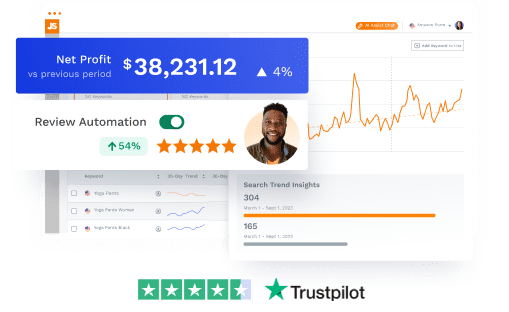
Market intelligence for sellers
The industry-leading product and keyword data to help you sell smarter on Amazon.
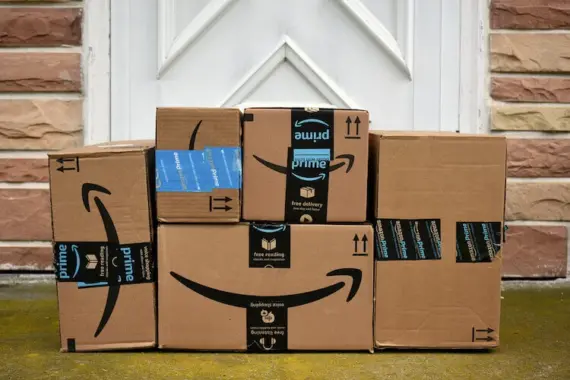
How to Grow Your Market Share on Amazon with Jungle Scout Competitive Intelligence
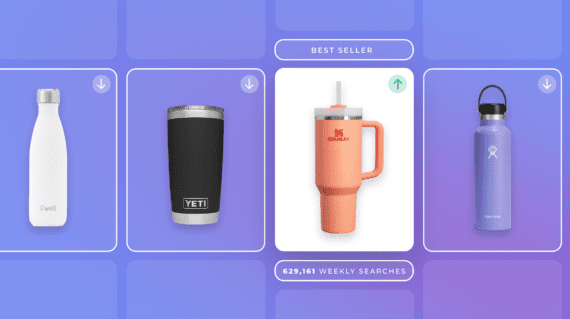
An Inside Look at the Billion-Dollar Water Bottle Market on Amazon: Stanley, YETI, Hydro Flask & More
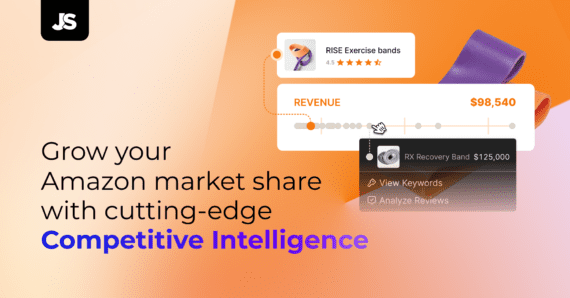
Introducing Jungle Scout Competitive Intelligence for Amazon Brands and Sellers
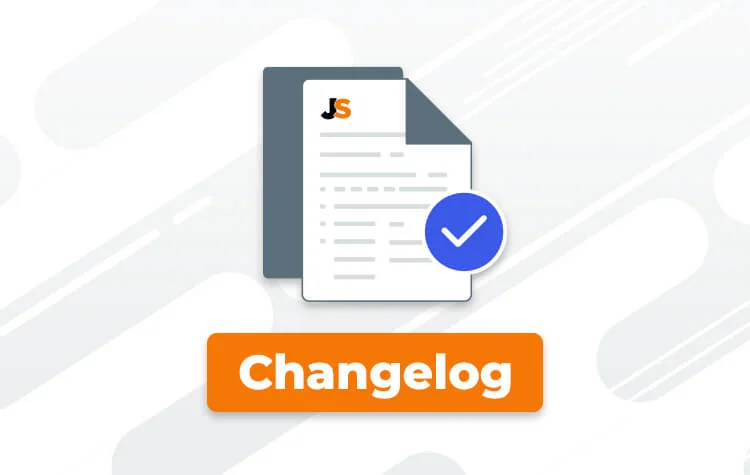
Jungle Scout Changelog: The Latest Product Updates from Jungle Scout
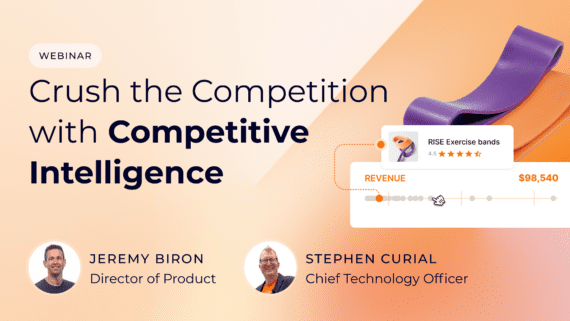
Crush the Competition with Jungle Scout’s NEW Competitive Intelligence
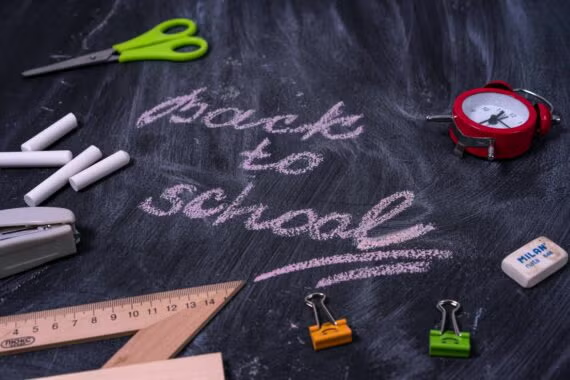
What to Sell on Amazon FBA: The Best 5 Products for August 2024
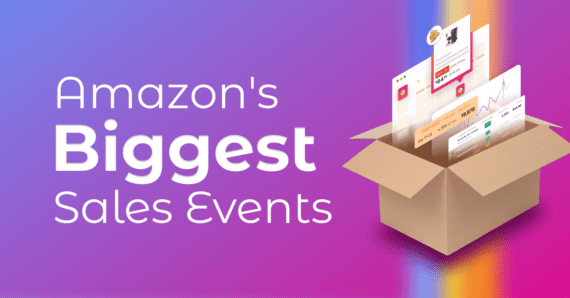
Amazon’s Biggest Sales Events: Stats & Strategies for Prime Day, Black Friday, & Cyber Monday
Stay in the loop.
Get the industry's best e-commerce articles, videos, reports, and more — delivered to your inbox weekly.
Thank you for signing up!
Leave a comment
Your email address will not be published. Required fields are marked with *
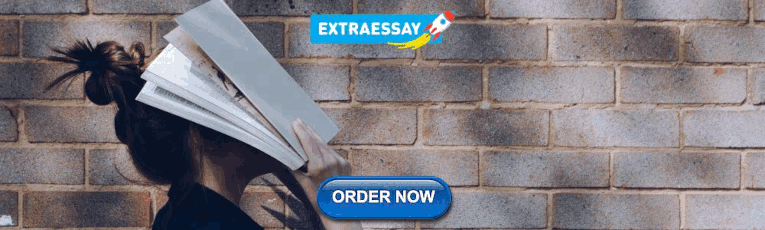
IMAGES
COMMENTS
Research design and research methods are two essential components of any research study. While research design provides the overall plan and structure, research methods are the practical tools used to collect and analyze data. Both have distinct attributes that contribute to the reliability, validity, and generalizability of research findings.
A research design is a strategy for answering your research question using empirical data. Creating a research design means making decisions about: Your overall research objectives and approach. Whether you'll rely on primary research or secondary research. Your sampling methods or criteria for selecting subjects. Your data collection methods.
Research methods are the procedures that are used to collect and analyze data. Thus, the main difference between research methods and research design is that research design is the overall structure of the research study whereas research methods are the various processes, procedures, and tools used to collect and analyze data. 1.
What is the difference between Research Design and Research Method? Research design is a plan to answer your research question. A research method is a strategy used to implement that plan. Research design and methods are different but closely related, because good research design ensures that the data you obtain will help you answer your ...
Types of Research Designs Compared | Guide & Examples. Published on June 20, 2019 by Shona McCombes.Revised on June 22, 2023. When you start planning a research project, developing research questions and creating a research design, you will have to make various decisions about the type of research you want to do.. There are many ways to categorize different types of research.
Types of study design. Medical research is classified into primary and secondary research. Clinical/experimental studies are performed in primary research, whereas secondary research consolidates available studies as reviews, systematic reviews and meta-analyses. Three main areas in primary research are basic medical research, clinical research ...
Research design provides the overall framework for the research study, guiding the selection of research questions, hypotheses, variables, and data collection methods. Research method, on the other hand, involves the practical implementation of the research design, determining the specific techniques and procedures that will be used to collect ...
Research design methods refer to the systematic approaches and techniques used to plan, structure, and conduct a research study. The choice of research design method depends on the research questions, objectives, and the nature of the study. Here are some key research design methods commonly used in various fields: 1.
Experimental Research Design. Experimental research design is used to determine if there is a causal relationship between two or more variables.With this type of research design, you, as the researcher, manipulate one variable (the independent variable) while controlling others (dependent variables). Doing so allows you to observe the effect of the former on the latter and draw conclusions ...
Step 2: Choose a type of research design. Within both qualitative and quantitative approaches, there are several types of research design to choose from. Each type provides a framework for the overall shape of your research. Types of quantitative research designs. Quantitative designs can be split into four main types.
Types of Research Designs Compared | Examples. Published on 5 May 2022 by Shona McCombes.Revised on 10 October 2022. When you start planning a research project, developing research questions and creating a research design, you will have to make various decisions about the type of research you want to do.. There are many ways to categorise different types of research.
This will guide your research design and help you select appropriate methods. Select a research design: There are many different research designs to choose from, including experimental, survey, case study, and qualitative designs. Choose a design that best fits your research question and objectives.
In short, a good research design helps us to structure our research. Marketers use different types of research design when conducting research. There are four common types of research design — descriptive, correlational, experimental, and diagnostic designs. Let's take a look at each in more detail.
The cross-sectional design can only measure differences between or from among a variety of people, subjects, or phenomena rather than a process of change. As such, researchers using this design can only employ a relatively passive approach to making causal inferences based on findings. ... This type of research design draws a conclusion by ...
The study design used to answer a particular research question depends on the nature of the question and the availability of resources. In this article, which is the first part of a series on "study designs," we provide an overview of research study designs and their classification. The subsequent articles will focus on individual designs.
To achieve the research purpose, a comparison analysis was conducted to study the differences in research design perspectives and approaches. Three dominant works related to research design, by Earl R. Babbie, Elizabethann O'Sullivan et al., and John W. Creswell, were selected as representative of the wide array of approaches in the PA ...
A research design is a framework that has been created to find answers to research questions. [citation needed] Design types and sub-types. There are many ways to classify research designs. Nonetheless, the list below offers a number of useful distinctions between possible research designs. ... This type of research is involved with a group ...
Purposive sampling is often used in qualitative research, with a goal of finding information-rich cases, not to generalize. 6. Be reflexive: Examine the ways in which your history, education, experiences, and worldviews have affected the research questions you have selected and your data collection methods, analyses, and writing. 13. Go to:
Quantitative research designs can be divided into two main categories: Correlational and descriptive designs are used to investigate characteristics, averages, trends, and associations between variables. Experimental and quasi-experimental designs are used to test causal relationships. Qualitative research designs tend to be more flexible.
A Research Design is a methodical, well-organized procedure utilized by a researcher, or a scientist to carry out a scientific study. It is a comprehensive co-existence of already identified elements and any other information or data leading to a reasonable end result. The research design is required to follow a pre-planned, well-thought-out ...
It is a causal research design type where the researcher tries to observe the impact of a variable on a dependent one. In doing so, the researcher attempts to determine or predict what may occur based on experimental models (Anastas, 1999). ... What is the difference between descriptive and experimental research design? Descriptive research ...
Research Methods and Research Design are foundational concepts in any scientific study. While Research Methods refer to the specific techniques and procedures used to collect and analyze data, Research Design provides the overall structure or blueprint that dictates how the study will be conducted. When a researcher selects specific Research ...
of ans wering the research ques tion or testing from hypothesis. This type of research d esign. includes descriptive design, exploratory design, experimental design, longitudinal design, cross ...
Quasi-experimental research design is used to investigate cause-and-effect relationships between variables. This research method is similar to experimental research design, but it lacks full control over the independent variable. Researchers use quasi-experimental research designs when it is not feasible or ethical to manipulate the independent ...
Exploring Differences in Automotive Logo Design Between Chinese and Western Cultures Based on Hofstedes Cultural Dimensions Theory. ... Art and Design Research, 12(04), 67-71.
After publishing research finding a connection between erythritol and increased risk of heart attack and stroke, Hazen and his colleagues conducted the first head-to-head human trial comparing the ...
"From securing stadiums, broadcasts, and fans to protecting the largest live sporting event in America, the right tools and the right team are key in making sure things run smoothly, avoiding disruptions to the game, and safeguarding the data and devices that make mission-critical gameday operations possible."
Streamline market research and make informed e-commerce decisions. Competitive ... We'll take a look at the primary differences between these two fulfillment methods — including which requires more experience and which is more profitable — as well as the factors you should consider when choosing whether to fulfill with Amazon FBA or FBM ...