- Privacy Policy

Home » Variables in Research – Definition, Types and Examples
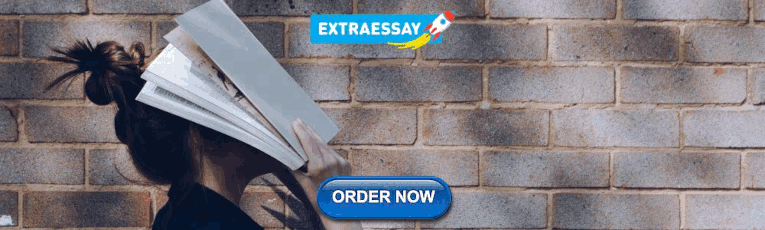
Variables in Research – Definition, Types and Examples
Table of Contents

Variables in Research
Definition:
In Research, Variables refer to characteristics or attributes that can be measured, manipulated, or controlled. They are the factors that researchers observe or manipulate to understand the relationship between them and the outcomes of interest.
Types of Variables in Research
Types of Variables in Research are as follows:
Independent Variable
This is the variable that is manipulated by the researcher. It is also known as the predictor variable, as it is used to predict changes in the dependent variable. Examples of independent variables include age, gender, dosage, and treatment type.
Dependent Variable
This is the variable that is measured or observed to determine the effects of the independent variable. It is also known as the outcome variable, as it is the variable that is affected by the independent variable. Examples of dependent variables include blood pressure, test scores, and reaction time.
Confounding Variable
This is a variable that can affect the relationship between the independent variable and the dependent variable. It is a variable that is not being studied but could impact the results of the study. For example, in a study on the effects of a new drug on a disease, a confounding variable could be the patient’s age, as older patients may have more severe symptoms.
Mediating Variable
This is a variable that explains the relationship between the independent variable and the dependent variable. It is a variable that comes in between the independent and dependent variables and is affected by the independent variable, which then affects the dependent variable. For example, in a study on the relationship between exercise and weight loss, the mediating variable could be metabolism, as exercise can increase metabolism, which can then lead to weight loss.
Moderator Variable
This is a variable that affects the strength or direction of the relationship between the independent variable and the dependent variable. It is a variable that influences the effect of the independent variable on the dependent variable. For example, in a study on the effects of caffeine on cognitive performance, the moderator variable could be age, as older adults may be more sensitive to the effects of caffeine than younger adults.
Control Variable
This is a variable that is held constant or controlled by the researcher to ensure that it does not affect the relationship between the independent variable and the dependent variable. Control variables are important to ensure that any observed effects are due to the independent variable and not to other factors. For example, in a study on the effects of a new teaching method on student performance, the control variables could include class size, teacher experience, and student demographics.
Continuous Variable
This is a variable that can take on any value within a certain range. Continuous variables can be measured on a scale and are often used in statistical analyses. Examples of continuous variables include height, weight, and temperature.
Categorical Variable
This is a variable that can take on a limited number of values or categories. Categorical variables can be nominal or ordinal. Nominal variables have no inherent order, while ordinal variables have a natural order. Examples of categorical variables include gender, race, and educational level.
Discrete Variable
This is a variable that can only take on specific values. Discrete variables are often used in counting or frequency analyses. Examples of discrete variables include the number of siblings a person has, the number of times a person exercises in a week, and the number of students in a classroom.
Dummy Variable
This is a variable that takes on only two values, typically 0 and 1, and is used to represent categorical variables in statistical analyses. Dummy variables are often used when a categorical variable cannot be used directly in an analysis. For example, in a study on the effects of gender on income, a dummy variable could be created, with 0 representing female and 1 representing male.
Extraneous Variable
This is a variable that has no relationship with the independent or dependent variable but can affect the outcome of the study. Extraneous variables can lead to erroneous conclusions and can be controlled through random assignment or statistical techniques.
Latent Variable
This is a variable that cannot be directly observed or measured, but is inferred from other variables. Latent variables are often used in psychological or social research to represent constructs such as personality traits, attitudes, or beliefs.
Moderator-mediator Variable
This is a variable that acts both as a moderator and a mediator. It can moderate the relationship between the independent and dependent variables and also mediate the relationship between the independent and dependent variables. Moderator-mediator variables are often used in complex statistical analyses.
Variables Analysis Methods
There are different methods to analyze variables in research, including:
- Descriptive statistics: This involves analyzing and summarizing data using measures such as mean, median, mode, range, standard deviation, and frequency distribution. Descriptive statistics are useful for understanding the basic characteristics of a data set.
- Inferential statistics : This involves making inferences about a population based on sample data. Inferential statistics use techniques such as hypothesis testing, confidence intervals, and regression analysis to draw conclusions from data.
- Correlation analysis: This involves examining the relationship between two or more variables. Correlation analysis can determine the strength and direction of the relationship between variables, and can be used to make predictions about future outcomes.
- Regression analysis: This involves examining the relationship between an independent variable and a dependent variable. Regression analysis can be used to predict the value of the dependent variable based on the value of the independent variable, and can also determine the significance of the relationship between the two variables.
- Factor analysis: This involves identifying patterns and relationships among a large number of variables. Factor analysis can be used to reduce the complexity of a data set and identify underlying factors or dimensions.
- Cluster analysis: This involves grouping data into clusters based on similarities between variables. Cluster analysis can be used to identify patterns or segments within a data set, and can be useful for market segmentation or customer profiling.
- Multivariate analysis : This involves analyzing multiple variables simultaneously. Multivariate analysis can be used to understand complex relationships between variables, and can be useful in fields such as social science, finance, and marketing.
Examples of Variables
- Age : This is a continuous variable that represents the age of an individual in years.
- Gender : This is a categorical variable that represents the biological sex of an individual and can take on values such as male and female.
- Education level: This is a categorical variable that represents the level of education completed by an individual and can take on values such as high school, college, and graduate school.
- Income : This is a continuous variable that represents the amount of money earned by an individual in a year.
- Weight : This is a continuous variable that represents the weight of an individual in kilograms or pounds.
- Ethnicity : This is a categorical variable that represents the ethnic background of an individual and can take on values such as Hispanic, African American, and Asian.
- Time spent on social media : This is a continuous variable that represents the amount of time an individual spends on social media in minutes or hours per day.
- Marital status: This is a categorical variable that represents the marital status of an individual and can take on values such as married, divorced, and single.
- Blood pressure : This is a continuous variable that represents the force of blood against the walls of arteries in millimeters of mercury.
- Job satisfaction : This is a continuous variable that represents an individual’s level of satisfaction with their job and can be measured using a Likert scale.
Applications of Variables
Variables are used in many different applications across various fields. Here are some examples:
- Scientific research: Variables are used in scientific research to understand the relationships between different factors and to make predictions about future outcomes. For example, scientists may study the effects of different variables on plant growth or the impact of environmental factors on animal behavior.
- Business and marketing: Variables are used in business and marketing to understand customer behavior and to make decisions about product development and marketing strategies. For example, businesses may study variables such as consumer preferences, spending habits, and market trends to identify opportunities for growth.
- Healthcare : Variables are used in healthcare to monitor patient health and to make treatment decisions. For example, doctors may use variables such as blood pressure, heart rate, and cholesterol levels to diagnose and treat cardiovascular disease.
- Education : Variables are used in education to measure student performance and to evaluate the effectiveness of teaching strategies. For example, teachers may use variables such as test scores, attendance, and class participation to assess student learning.
- Social sciences : Variables are used in social sciences to study human behavior and to understand the factors that influence social interactions. For example, sociologists may study variables such as income, education level, and family structure to examine patterns of social inequality.
Purpose of Variables
Variables serve several purposes in research, including:
- To provide a way of measuring and quantifying concepts: Variables help researchers measure and quantify abstract concepts such as attitudes, behaviors, and perceptions. By assigning numerical values to these concepts, researchers can analyze and compare data to draw meaningful conclusions.
- To help explain relationships between different factors: Variables help researchers identify and explain relationships between different factors. By analyzing how changes in one variable affect another variable, researchers can gain insight into the complex interplay between different factors.
- To make predictions about future outcomes : Variables help researchers make predictions about future outcomes based on past observations. By analyzing patterns and relationships between different variables, researchers can make informed predictions about how different factors may affect future outcomes.
- To test hypotheses: Variables help researchers test hypotheses and theories. By collecting and analyzing data on different variables, researchers can test whether their predictions are accurate and whether their hypotheses are supported by the evidence.
Characteristics of Variables
Characteristics of Variables are as follows:
- Measurement : Variables can be measured using different scales, such as nominal, ordinal, interval, or ratio scales. The scale used to measure a variable can affect the type of statistical analysis that can be applied.
- Range : Variables have a range of values that they can take on. The range can be finite, such as the number of students in a class, or infinite, such as the range of possible values for a continuous variable like temperature.
- Variability : Variables can have different levels of variability, which refers to the degree to which the values of the variable differ from each other. Highly variable variables have a wide range of values, while low variability variables have values that are more similar to each other.
- Validity and reliability : Variables should be both valid and reliable to ensure accurate and consistent measurement. Validity refers to the extent to which a variable measures what it is intended to measure, while reliability refers to the consistency of the measurement over time.
- Directionality: Some variables have directionality, meaning that the relationship between the variables is not symmetrical. For example, in a study of the relationship between smoking and lung cancer, smoking is the independent variable and lung cancer is the dependent variable.
Advantages of Variables
Here are some of the advantages of using variables in research:
- Control : Variables allow researchers to control the effects of external factors that could influence the outcome of the study. By manipulating and controlling variables, researchers can isolate the effects of specific factors and measure their impact on the outcome.
- Replicability : Variables make it possible for other researchers to replicate the study and test its findings. By defining and measuring variables consistently, other researchers can conduct similar studies to validate the original findings.
- Accuracy : Variables make it possible to measure phenomena accurately and objectively. By defining and measuring variables precisely, researchers can reduce bias and increase the accuracy of their findings.
- Generalizability : Variables allow researchers to generalize their findings to larger populations. By selecting variables that are representative of the population, researchers can draw conclusions that are applicable to a broader range of individuals.
- Clarity : Variables help researchers to communicate their findings more clearly and effectively. By defining and categorizing variables, researchers can organize and present their findings in a way that is easily understandable to others.
Disadvantages of Variables
Here are some of the main disadvantages of using variables in research:
- Simplification : Variables may oversimplify the complexity of real-world phenomena. By breaking down a phenomenon into variables, researchers may lose important information and context, which can affect the accuracy and generalizability of their findings.
- Measurement error : Variables rely on accurate and precise measurement, and measurement error can affect the reliability and validity of research findings. The use of subjective or poorly defined variables can also introduce measurement error into the study.
- Confounding variables : Confounding variables are factors that are not measured but that affect the relationship between the variables of interest. If confounding variables are not accounted for, they can distort or obscure the relationship between the variables of interest.
- Limited scope: Variables are defined by the researcher, and the scope of the study is therefore limited by the researcher’s choice of variables. This can lead to a narrow focus that overlooks important aspects of the phenomenon being studied.
- Ethical concerns: The selection and measurement of variables may raise ethical concerns, especially in studies involving human subjects. For example, using variables that are related to sensitive topics, such as race or sexuality, may raise concerns about privacy and discrimination.
About the author
Muhammad Hassan
Researcher, Academic Writer, Web developer
You may also like

Control Variable – Definition, Types and Examples

Moderating Variable – Definition, Analysis...

Categorical Variable – Definition, Types and...

Independent Variable – Definition, Types and...

Ratio Variable – Definition, Purpose and Examples

Ordinal Variable – Definition, Purpose and...
Have a language expert improve your writing
Run a free plagiarism check in 10 minutes, automatically generate references for free.
- Knowledge Base
- Methodology
- Types of Variables in Research | Definitions & Examples
Types of Variables in Research | Definitions & Examples
Published on 19 September 2022 by Rebecca Bevans . Revised on 28 November 2022.
In statistical research, a variable is defined as an attribute of an object of study. Choosing which variables to measure is central to good experimental design .
You need to know which types of variables you are working with in order to choose appropriate statistical tests and interpret the results of your study.
You can usually identify the type of variable by asking two questions:
- What type of data does the variable contain?
- What part of the experiment does the variable represent?
Table of contents
Types of data: quantitative vs categorical variables, parts of the experiment: independent vs dependent variables, other common types of variables, frequently asked questions about variables.
Data is a specific measurement of a variable – it is the value you record in your data sheet. Data is generally divided into two categories:
- Quantitative data represents amounts.
- Categorical data represents groupings.
A variable that contains quantitative data is a quantitative variable ; a variable that contains categorical data is a categorical variable . Each of these types of variable can be broken down into further types.
Quantitative variables
When you collect quantitative data, the numbers you record represent real amounts that can be added, subtracted, divided, etc. There are two types of quantitative variables: discrete and continuous .
Categorical variables
Categorical variables represent groupings of some kind. They are sometimes recorded as numbers, but the numbers represent categories rather than actual amounts of things.
There are three types of categorical variables: binary , nominal , and ordinal variables.
*Note that sometimes a variable can work as more than one type! An ordinal variable can also be used as a quantitative variable if the scale is numeric and doesn’t need to be kept as discrete integers. For example, star ratings on product reviews are ordinal (1 to 5 stars), but the average star rating is quantitative.
Example data sheet
To keep track of your salt-tolerance experiment, you make a data sheet where you record information about the variables in the experiment, like salt addition and plant health.
To gather information about plant responses over time, you can fill out the same data sheet every few days until the end of the experiment. This example sheet is colour-coded according to the type of variable: nominal , continuous , ordinal , and binary .
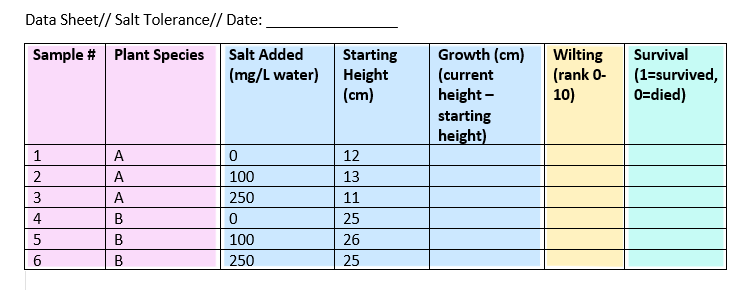
Prevent plagiarism, run a free check.
Experiments are usually designed to find out what effect one variable has on another – in our example, the effect of salt addition on plant growth.
You manipulate the independent variable (the one you think might be the cause ) and then measure the dependent variable (the one you think might be the effect ) to find out what this effect might be.
You will probably also have variables that you hold constant ( control variables ) in order to focus on your experimental treatment.
In this experiment, we have one independent and three dependent variables.
The other variables in the sheet can’t be classified as independent or dependent, but they do contain data that you will need in order to interpret your dependent and independent variables.
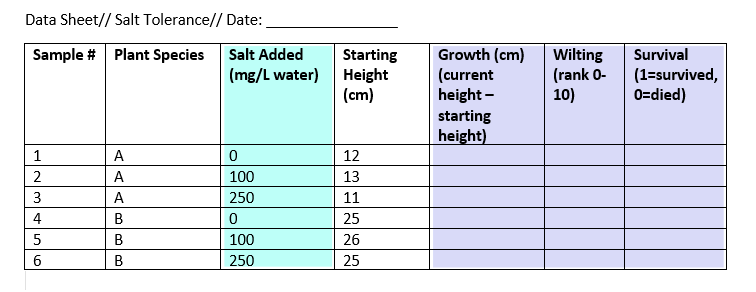
What about correlational research?
When you do correlational research , the terms ‘dependent’ and ‘independent’ don’t apply, because you are not trying to establish a cause-and-effect relationship.
However, there might be cases where one variable clearly precedes the other (for example, rainfall leads to mud, rather than the other way around). In these cases, you may call the preceding variable (i.e., the rainfall) the predictor variable and the following variable (i.e., the mud) the outcome variable .
Once you have defined your independent and dependent variables and determined whether they are categorical or quantitative, you will be able to choose the correct statistical test .
But there are many other ways of describing variables that help with interpreting your results. Some useful types of variable are listed below.
A confounding variable is closely related to both the independent and dependent variables in a study. An independent variable represents the supposed cause , while the dependent variable is the supposed effect . A confounding variable is a third variable that influences both the independent and dependent variables.
Failing to account for confounding variables can cause you to wrongly estimate the relationship between your independent and dependent variables.
Discrete and continuous variables are two types of quantitative variables :
- Discrete variables represent counts (e.g., the number of objects in a collection).
- Continuous variables represent measurable amounts (e.g., water volume or weight).
You can think of independent and dependent variables in terms of cause and effect: an independent variable is the variable you think is the cause , while a dependent variable is the effect .
In an experiment, you manipulate the independent variable and measure the outcome in the dependent variable. For example, in an experiment about the effect of nutrients on crop growth:
- The independent variable is the amount of nutrients added to the crop field.
- The dependent variable is the biomass of the crops at harvest time.
Defining your variables, and deciding how you will manipulate and measure them, is an important part of experimental design .
Cite this Scribbr article
If you want to cite this source, you can copy and paste the citation or click the ‘Cite this Scribbr article’ button to automatically add the citation to our free Reference Generator.
Bevans, R. (2022, November 28). Types of Variables in Research | Definitions & Examples. Scribbr. Retrieved 22 April 2024, from https://www.scribbr.co.uk/research-methods/variables-types/
Is this article helpful?
Rebecca Bevans
Other students also liked, a quick guide to experimental design | 5 steps & examples, quasi-experimental design | definition, types & examples, construct validity | definition, types, & examples.
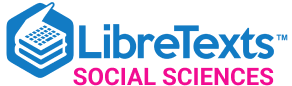
- school Campus Bookshelves
- menu_book Bookshelves
- perm_media Learning Objects
- login Login
- how_to_reg Request Instructor Account
- hub Instructor Commons
- Download Page (PDF)
- Download Full Book (PDF)
- Periodic Table
- Physics Constants
- Scientific Calculator
- Reference & Cite
- Tools expand_more
- Readability
selected template will load here
This action is not available.
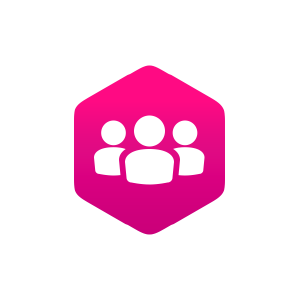
2.2: Concepts, Constructs, and Variables
- Last updated
- Save as PDF
- Page ID 26212
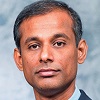
- Anol Bhattacherjee
- University of South Florida via Global Text Project
We discussed in Chapter 1 that although research can be exploratory, descriptive, or explanatory, most scientific research tend to be of the explanatory type in that they search for potential explanations of observed natural or social phenomena. Explanations require development of concepts or generalizable properties or characteristics associated with objects, events, or people. While objects such as a person, a firm, or a car are not concepts, their specific characteristics or behavior such as a person’s attitude toward immigrants, a firm’s capacity for innovation, and a car’s weight can be viewed as concepts.
Knowingly or unknowingly, we use different kinds of concepts in our everyday conversations. Some of these concepts have been developed over time through our shared language. Sometimes, we borrow concepts from other disciplines or languages to explain a phenomenon of interest. For instance, the idea of gravitation borrowed from physics can be used in business to describe why people tend to “gravitate” to their preferred shopping destinations. Likewise, the concept of distance can be used to explain the degree of social separation between two otherwise collocated individuals. Sometimes, we create our own concepts to describe a unique characteristic not described in prior research. For instance, technostress is a new concept referring to the mental stress one may face when asked to learn a new technology.
Concepts may also have progressive levels of abstraction. Some concepts such as a person’s weight are precise and objective, while other concepts such as a person’s personality may be more abstract and difficult to visualize. A construct is an abstract concept that is specifically chosen (or “created”) to explain a given phenomenon. A construct may be a simple concept, such as a person’s weight , or a combination of a set of related concepts such as a person’s communication skill , which may consist of several underlying concepts such as the person’s vocabulary , syntax , and spelling . The former instance (weight) is a unidimensional construct , while the latter (communication skill) is a multi-dimensional construct (i.e., it consists of multiple underlying concepts). The distinction between constructs and concepts are clearer in multi-dimensional constructs, where the higher order abstraction is called a construct and the lower order abstractions are called concepts. However, this distinction tends to blur in the case of unidimensional constructs.
Constructs used for scientific research must have precise and clear definitions that others can use to understand exactly what it means and what it does not mean. For instance, a seemingly simple construct such as income may refer to monthly or annual income, before-tax or after-tax income, and personal or family income, and is therefore neither precise nor clear. There are two types of definitions: dictionary definitions and operational definitions. In the more familiar dictionary definition, a construct is often defined in terms of a synonym. For instance, attitude may be defined as a disposition, a feeling, or an affect, and affect in turn is defined as an attitude. Such definitions of a circular nature are not particularly useful in scientific research for elaborating the meaning and content of that construct. Scientific research requires operational definitions that define constructs in terms of how they will be empirically measured. For instance, the operational definition of a construct such as temperature must specify whether we plan to measure temperature in Celsius, Fahrenheit, or Kelvin scale. A construct such as income should be defined in terms of whether we are interested in monthly or annual income, before-tax or after-tax income, and personal or family income. One can imagine that constructs such as learning , personality , and intelligence can be quite hard to define operationally.
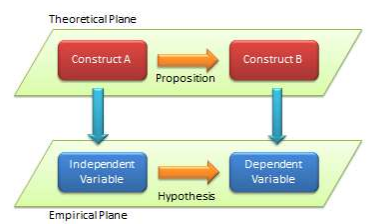
A term frequently associated with, and sometimes used interchangeably with, a construct is a variable. Etymologically speaking, a variable is a quantity that can vary (e.g., from low to high, negative to positive, etc.), in contrast to constants that do not vary (i.e., remain constant). However, in scientific research, a variable is a measurable representation of an abstract construct. As abstract entities, constructs are not directly measurable, and hence, we look for proxy measures called variables. For instance, a person’s intelligence is often measured as his or her IQ ( intelligence quotient ) score , which is an index generated from an analytical and pattern-matching test administered to people. In this case, intelligence is a construct, and IQ score is a variable that measures the intelligence construct. Whether IQ scores truly measures one’s intelligence is anyone’s guess (though many believe that they do), and depending on whether how well it measures intelligence, the IQ score may be a good or a poor measure of the intelligence construct. As shown in Figure 2.1, scientific research proceeds along two planes: a theoretical plane and an empirical plane. Constructs are conceptualized at the theoretical (abstract) plane, while variables are operationalized and measured at the empirical (observational) plane. Thinking like a researcher implies the ability to move back and forth between these two planes.
Depending on their intended use, variables may be classified as independent, dependent, moderating, mediating, or control variables. Variables that explain other variables are called independent variables , those that are explained by other variables are dependent variables , those that are explained by independent variables while also explaining dependent variables are mediating variables (or intermediate variables), and those that influence the relationship between independent and dependent variables are called moderating variables . As an example, if we state that higher intelligence causes improved learning among students, then intelligence is an independent variable and learning is a dependent variable. There may be other extraneous variables that are not pertinent to explaining a given dependent variable, but may have some impact on the dependent variable. These variables must be controlled for in a scientific study, and are therefore called control variables .
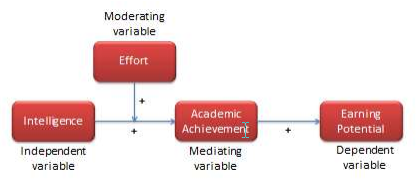
To understand the differences between these different variable types, consider the example shown in Figure 2.2. If we believe that intelligence influences (or explains) students’ academic achievement, then a measure of intelligence such as an IQ score is an independent variable, while a measure of academic success such as grade point average is a dependent variable. If we believe that the effect of intelligence on academic achievement also depends on the effort invested by the student in the learning process (i.e., between two equally intelligent students, the student who puts is more effort achieves higher academic achievement than one who puts in less effort), then effort becomes a moderating variable. Incidentally, one may also view effort as an independent variable and intelligence as a moderating variable. If academic achievement is viewed as an intermediate step to higher earning potential, then earning potential becomes the dependent variable for the independent variable academic achievement , and academic achievement becomes the mediating variable in the relationship between intelligence and earning potential. Hence, variable are defined as an independent, dependent, moderating, or mediating variable based on their nature of association with each other. The overall network of relationships between a set of related constructs is called a nomological network (see Figure 2.2). Thinking like a researcher requires not only being able to abstract constructs from observations, but also being able to mentally visualize a nomological network linking these abstract constructs.

Research Variables 101
Independent variables, dependent variables, control variables and more
By: Derek Jansen (MBA) | Expert Reviewed By: Kerryn Warren (PhD) | January 2023
If you’re new to the world of research, especially scientific research, you’re bound to run into the concept of variables , sooner or later. If you’re feeling a little confused, don’t worry – you’re not the only one! Independent variables, dependent variables, confounding variables – it’s a lot of jargon. In this post, we’ll unpack the terminology surrounding research variables using straightforward language and loads of examples .
Overview: Variables In Research
What (exactly) is a variable.
The simplest way to understand a variable is as any characteristic or attribute that can experience change or vary over time or context – hence the name “variable”. For example, the dosage of a particular medicine could be classified as a variable, as the amount can vary (i.e., a higher dose or a lower dose). Similarly, gender, age or ethnicity could be considered demographic variables, because each person varies in these respects.
Within research, especially scientific research, variables form the foundation of studies, as researchers are often interested in how one variable impacts another, and the relationships between different variables. For example:
- How someone’s age impacts their sleep quality
- How different teaching methods impact learning outcomes
- How diet impacts weight (gain or loss)
As you can see, variables are often used to explain relationships between different elements and phenomena. In scientific studies, especially experimental studies, the objective is often to understand the causal relationships between variables. In other words, the role of cause and effect between variables. This is achieved by manipulating certain variables while controlling others – and then observing the outcome. But, we’ll get into that a little later…
The “Big 3” Variables
Variables can be a little intimidating for new researchers because there are a wide variety of variables, and oftentimes, there are multiple labels for the same thing. To lay a firm foundation, we’ll first look at the three main types of variables, namely:
- Independent variables (IV)
- Dependant variables (DV)
- Control variables
What is an independent variable?
Simply put, the independent variable is the “ cause ” in the relationship between two (or more) variables. In other words, when the independent variable changes, it has an impact on another variable.
For example:
- Increasing the dosage of a medication (Variable A) could result in better (or worse) health outcomes for a patient (Variable B)
- Changing a teaching method (Variable A) could impact the test scores that students earn in a standardised test (Variable B)
- Varying one’s diet (Variable A) could result in weight loss or gain (Variable B).
It’s useful to know that independent variables can go by a few different names, including, explanatory variables (because they explain an event or outcome) and predictor variables (because they predict the value of another variable). Terminology aside though, the most important takeaway is that independent variables are assumed to be the “cause” in any cause-effect relationship. As you can imagine, these types of variables are of major interest to researchers, as many studies seek to understand the causal factors behind a phenomenon.
Need a helping hand?
What is a dependent variable?
While the independent variable is the “ cause ”, the dependent variable is the “ effect ” – or rather, the affected variable . In other words, the dependent variable is the variable that is assumed to change as a result of a change in the independent variable.
Keeping with the previous example, let’s look at some dependent variables in action:
- Health outcomes (DV) could be impacted by dosage changes of a medication (IV)
- Students’ scores (DV) could be impacted by teaching methods (IV)
- Weight gain or loss (DV) could be impacted by diet (IV)
In scientific studies, researchers will typically pay very close attention to the dependent variable (or variables), carefully measuring any changes in response to hypothesised independent variables. This can be tricky in practice, as it’s not always easy to reliably measure specific phenomena or outcomes – or to be certain that the actual cause of the change is in fact the independent variable.
As the adage goes, correlation is not causation . In other words, just because two variables have a relationship doesn’t mean that it’s a causal relationship – they may just happen to vary together. For example, you could find a correlation between the number of people who own a certain brand of car and the number of people who have a certain type of job. Just because the number of people who own that brand of car and the number of people who have that type of job is correlated, it doesn’t mean that owning that brand of car causes someone to have that type of job or vice versa. The correlation could, for example, be caused by another factor such as income level or age group, which would affect both car ownership and job type.
To confidently establish a causal relationship between an independent variable and a dependent variable (i.e., X causes Y), you’ll typically need an experimental design , where you have complete control over the environmen t and the variables of interest. But even so, this doesn’t always translate into the “real world”. Simply put, what happens in the lab sometimes stays in the lab!
As an alternative to pure experimental research, correlational or “ quasi-experimental ” research (where the researcher cannot manipulate or change variables) can be done on a much larger scale more easily, allowing one to understand specific relationships in the real world. These types of studies also assume some causality between independent and dependent variables, but it’s not always clear. So, if you go this route, you need to be cautious in terms of how you describe the impact and causality between variables and be sure to acknowledge any limitations in your own research.
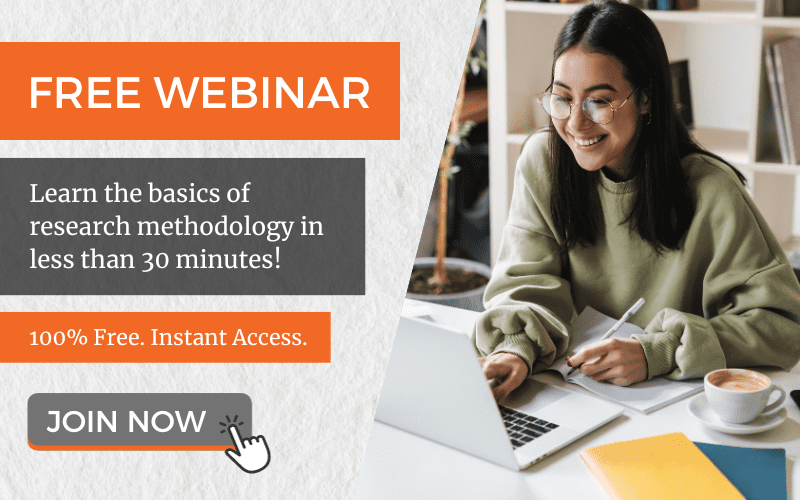
What is a control variable?
In an experimental design, a control variable (or controlled variable) is a variable that is intentionally held constant to ensure it doesn’t have an influence on any other variables. As a result, this variable remains unchanged throughout the course of the study. In other words, it’s a variable that’s not allowed to vary – tough life 🙂
As we mentioned earlier, one of the major challenges in identifying and measuring causal relationships is that it’s difficult to isolate the impact of variables other than the independent variable. Simply put, there’s always a risk that there are factors beyond the ones you’re specifically looking at that might be impacting the results of your study. So, to minimise the risk of this, researchers will attempt (as best possible) to hold other variables constant . These factors are then considered control variables.
Some examples of variables that you may need to control include:
- Temperature
- Time of day
- Noise or distractions
Which specific variables need to be controlled for will vary tremendously depending on the research project at hand, so there’s no generic list of control variables to consult. As a researcher, you’ll need to think carefully about all the factors that could vary within your research context and then consider how you’ll go about controlling them. A good starting point is to look at previous studies similar to yours and pay close attention to which variables they controlled for.
Of course, you won’t always be able to control every possible variable, and so, in many cases, you’ll just have to acknowledge their potential impact and account for them in the conclusions you draw. Every study has its limitations, so don’t get fixated or discouraged by troublesome variables. Nevertheless, always think carefully about the factors beyond what you’re focusing on – don’t make assumptions!
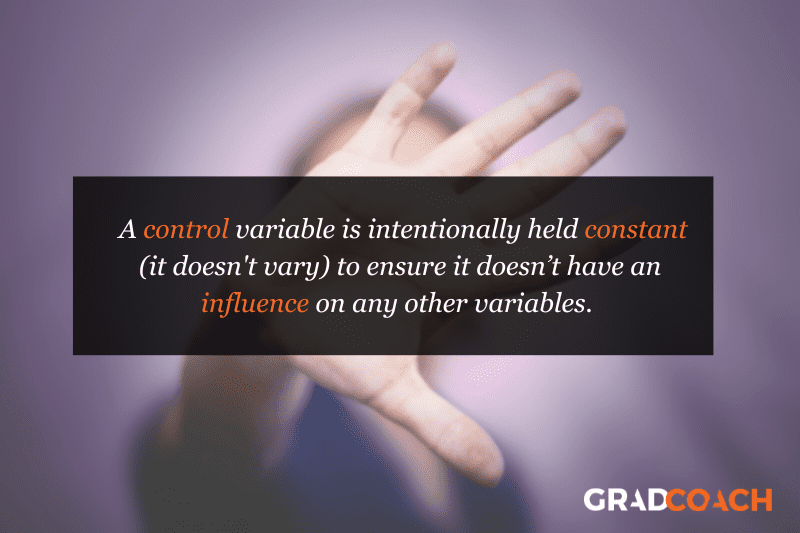
Other types of variables
As we mentioned, independent, dependent and control variables are the most common variables you’ll come across in your research, but they’re certainly not the only ones you need to be aware of. Next, we’ll look at a few “secondary” variables that you need to keep in mind as you design your research.
- Moderating variables
- Mediating variables
- Confounding variables
- Latent variables
Let’s jump into it…
What is a moderating variable?
A moderating variable is a variable that influences the strength or direction of the relationship between an independent variable and a dependent variable. In other words, moderating variables affect how much (or how little) the IV affects the DV, or whether the IV has a positive or negative relationship with the DV (i.e., moves in the same or opposite direction).
For example, in a study about the effects of sleep deprivation on academic performance, gender could be used as a moderating variable to see if there are any differences in how men and women respond to a lack of sleep. In such a case, one may find that gender has an influence on how much students’ scores suffer when they’re deprived of sleep.
It’s important to note that while moderators can have an influence on outcomes , they don’t necessarily cause them ; rather they modify or “moderate” existing relationships between other variables. This means that it’s possible for two different groups with similar characteristics, but different levels of moderation, to experience very different results from the same experiment or study design.
What is a mediating variable?
Mediating variables are often used to explain the relationship between the independent and dependent variable (s). For example, if you were researching the effects of age on job satisfaction, then education level could be considered a mediating variable, as it may explain why older people have higher job satisfaction than younger people – they may have more experience or better qualifications, which lead to greater job satisfaction.
Mediating variables also help researchers understand how different factors interact with each other to influence outcomes. For instance, if you wanted to study the effect of stress on academic performance, then coping strategies might act as a mediating factor by influencing both stress levels and academic performance simultaneously. For example, students who use effective coping strategies might be less stressed but also perform better academically due to their improved mental state.
In addition, mediating variables can provide insight into causal relationships between two variables by helping researchers determine whether changes in one factor directly cause changes in another – or whether there is an indirect relationship between them mediated by some third factor(s). For instance, if you wanted to investigate the impact of parental involvement on student achievement, you would need to consider family dynamics as a potential mediator, since it could influence both parental involvement and student achievement simultaneously.
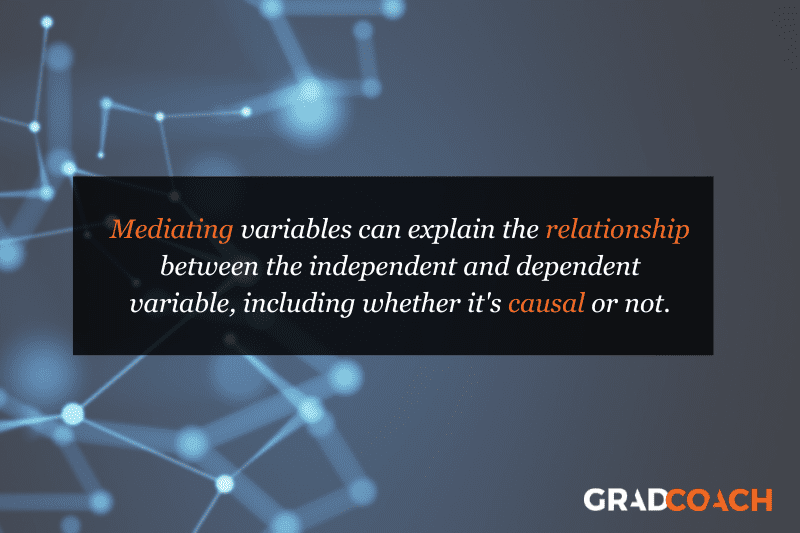
What is a confounding variable?
A confounding variable (also known as a third variable or lurking variable ) is an extraneous factor that can influence the relationship between two variables being studied. Specifically, for a variable to be considered a confounding variable, it needs to meet two criteria:
- It must be correlated with the independent variable (this can be causal or not)
- It must have a causal impact on the dependent variable (i.e., influence the DV)
Some common examples of confounding variables include demographic factors such as gender, ethnicity, socioeconomic status, age, education level, and health status. In addition to these, there are also environmental factors to consider. For example, air pollution could confound the impact of the variables of interest in a study investigating health outcomes.
Naturally, it’s important to identify as many confounding variables as possible when conducting your research, as they can heavily distort the results and lead you to draw incorrect conclusions . So, always think carefully about what factors may have a confounding effect on your variables of interest and try to manage these as best you can.
What is a latent variable?
Latent variables are unobservable factors that can influence the behaviour of individuals and explain certain outcomes within a study. They’re also known as hidden or underlying variables , and what makes them rather tricky is that they can’t be directly observed or measured . Instead, latent variables must be inferred from other observable data points such as responses to surveys or experiments.
For example, in a study of mental health, the variable “resilience” could be considered a latent variable. It can’t be directly measured , but it can be inferred from measures of mental health symptoms, stress, and coping mechanisms. The same applies to a lot of concepts we encounter every day – for example:
- Emotional intelligence
- Quality of life
- Business confidence
- Ease of use
One way in which we overcome the challenge of measuring the immeasurable is latent variable models (LVMs). An LVM is a type of statistical model that describes a relationship between observed variables and one or more unobserved (latent) variables. These models allow researchers to uncover patterns in their data which may not have been visible before, thanks to their complexity and interrelatedness with other variables. Those patterns can then inform hypotheses about cause-and-effect relationships among those same variables which were previously unknown prior to running the LVM. Powerful stuff, we say!
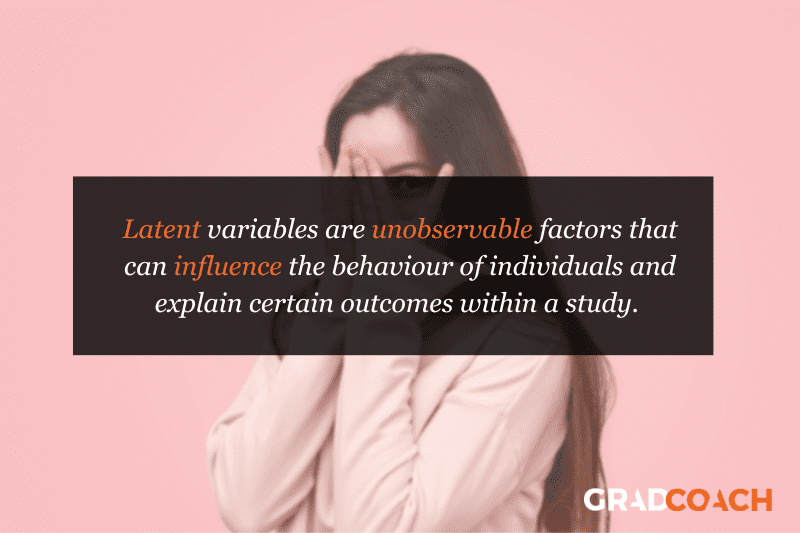
Let’s recap
In the world of scientific research, there’s no shortage of variable types, some of which have multiple names and some of which overlap with each other. In this post, we’ve covered some of the popular ones, but remember that this is not an exhaustive list .
To recap, we’ve explored:
- Independent variables (the “cause”)
- Dependent variables (the “effect”)
- Control variables (the variable that’s not allowed to vary)
If you’re still feeling a bit lost and need a helping hand with your research project, check out our 1-on-1 coaching service , where we guide you through each step of the research journey. Also, be sure to check out our free dissertation writing course and our collection of free, fully-editable chapter templates .
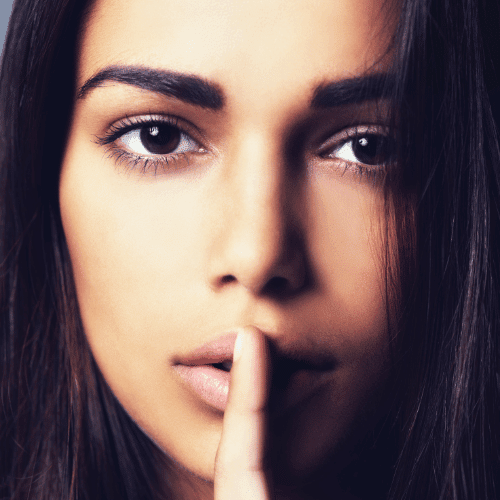
Psst... there’s more!
This post was based on one of our popular Research Bootcamps . If you're working on a research project, you'll definitely want to check this out ...
You Might Also Like:
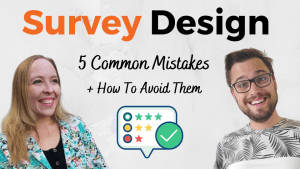
Very informative, concise and helpful. Thank you
Helping information.Thanks
practical and well-demonstrated
Very helpful and insightful
Submit a Comment Cancel reply
Your email address will not be published. Required fields are marked *
Save my name, email, and website in this browser for the next time I comment.
- Print Friendly
- How it works
Types of Variables – A Comprehensive Guide
Published by Carmen Troy at August 14th, 2021 , Revised On October 26, 2023
A variable is any qualitative or quantitative characteristic that can change and have more than one value, such as age, height, weight, gender, etc.
Before conducting research, it’s essential to know what needs to be measured or analysed and choose a suitable statistical test to present your study’s findings.
In most cases, you can do it by identifying the key issues/variables related to your research’s main topic.
Example: If you want to test whether the hybridisation of plants harms the health of people. You can use the key variables like agricultural techniques, type of soil, environmental factors, types of pesticides used, the process of hybridisation, type of yield obtained after hybridisation, type of yield without hybridisation, etc.
Variables are broadly categorised into:
- Independent variables
- Dependent variable
- Control variable
Independent Vs. Dependent Vs. Control Variable
The research includes finding ways:
- To change the independent variables.
- To prevent the controlled variables from changing.
- To measure the dependent variables.
Note: The term dependent and independent is not applicable in correlational research as this is not a controlled experiment. A researcher doesn’t have control over the variables. The association and between two or more variables are measured. If one variable affects another one, then it’s called the predictor variable and outcome variable.
Example: Correlation between investment (predictor variable) and profit (outcome variable)
What data collection best suits your research?
- Find out by hiring an expert from ResearchProspect today!
- Despite how challenging the subject may be, we are here to help you.
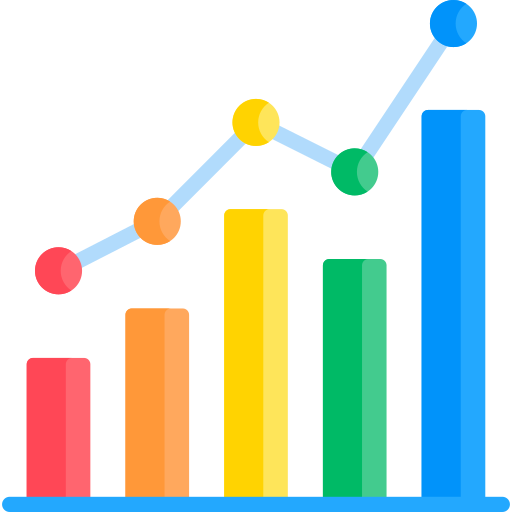
Types of Variables Based on the Types of Data
A data is referred to as the information and statistics gathered for analysis of a research topic. Data is broadly divided into two categories, such as:
Quantitative/Numerical data is associated with the aspects of measurement, quantity, and extent.
Categorial data is associated with groupings.
A qualitative variable consists of qualitative data, and a quantitative variable consists of a quantitative variable.
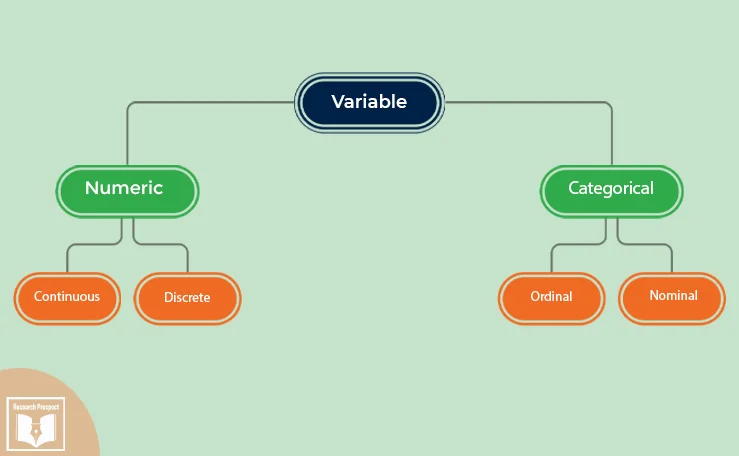
Quantitative Variable
The quantitative variable is associated with measurement, quantity, and extent, like how many . It follows the statistical, mathematical, and computational techniques in numerical data such as percentages and statistics. The research is conducted on a large group of population.
Example: Find out the weight of students of the fifth standard studying in government schools.
The quantitative variable can be further categorised into continuous and discrete.
Categorial Variable
The categorical variable includes measurements that vary in categories such as names but not in terms of rank or degree. It means one level of a categorical variable cannot be considered better or greater than another level.
Example: Gender, brands, colors, zip codes
The categorical variable is further categorised into three types:
Note: Sometimes, an ordinal variable also acts as a quantitative variable. Ordinal data has an order, but the intervals between scale points may be uneven.
Example: Numbers on a rating scale represent the reviews’ rank or range from below average to above average. However, it also represents a quantitative variable showing how many stars and how much rating is given.
Not sure which statistical tests to use for your data?
Let the experts at researchprospect do the daunting work for you..
Using our approach, we illustrate how to collect data, sample sizes, validity, reliability, credibility, and ethics, so you won’t have to do it all by yourself!
Other Types of Variables
It’s important to understand the difference between dependent and independent variables and know whether they are quantitative or categorical to choose the appropriate statistical test.
There are many other types of variables to help you differentiate and understand them.
Also, read a comprehensive guide written about inductive and deductive reasoning .
- Entertainment
- Online education
- Database management, storage, and retrieval
Frequently Asked Questions
What are the 10 types of variables in research.
The 10 types of variables in research are:
- Independent
- Confounding
- Categorical
- Extraneous.
What is an independent variable?
An independent variable, often termed the predictor or explanatory variable, is the variable manipulated or categorized in an experiment to observe its effect on another variable, called the dependent variable. It’s the presumed cause in a cause-and-effect relationship, determining if changes in it produce changes in the observed outcome.
What is a variable?
In research, a variable is any attribute, quantity, or characteristic that can be measured or counted. It can take on various values, making it “variable.” Variables can be classified as independent (manipulated), dependent (observed outcome), or control (kept constant). They form the foundation for hypotheses, observations, and data analysis in studies.
What is a dependent variable?
A dependent variable is the outcome or response being studied in an experiment or investigation. It’s what researchers measure to determine the effect of changes in the independent variable. In a cause-and-effect relationship, the dependent variable is presumed to be influenced or caused by the independent variable.
What is a variable in programming?
In programming, a variable is a symbolic name for a storage location that holds data or values. It allows data storage and retrieval for computational operations. Variables have types, like integer or string, determining the nature of data they can hold. They’re fundamental in manipulating and processing information in software.
What is a control variable?
A control variable in research is a factor that’s kept constant to ensure that it doesn’t influence the outcome. By controlling these variables, researchers can isolate the effects of the independent variable on the dependent variable, ensuring that other factors don’t skew the results or introduce bias into the experiment.
What is a controlled variable in science?
In science, a controlled variable is a factor that remains constant throughout an experiment. It ensures that any observed changes in the dependent variable are solely due to the independent variable, not other factors. By keeping controlled variables consistent, researchers can maintain experiment validity and accurately assess cause-and-effect relationships.
How many independent variables should an investigation have?
Ideally, an investigation should have one independent variable to clearly establish cause-and-effect relationships. Manipulating multiple independent variables simultaneously can complicate data interpretation.
However, in advanced research, experiments with multiple independent variables (factorial designs) are used, but they require careful planning to understand interactions between variables.
You May Also Like
A case study is a detailed analysis of a situation concerning organizations, industries, and markets. The case study generally aims at identifying the weak areas.
What are the different types of research you can use in your dissertation? Here are some guidelines to help you choose a research strategy that would make your research more credible.
Descriptive research is carried out to describe current issues, programs, and provides information about the issue through surveys and various fact-finding methods.
USEFUL LINKS
LEARNING RESOURCES
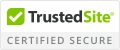
COMPANY DETAILS
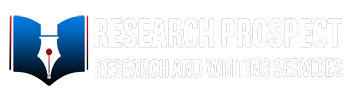
- How It Works
Independent and Dependent Variables
This guide discusses how to identify independent and dependent variables effectively and incorporate their description within the body of a research paper.
A variable can be anything you might aim to measure in your study, whether in the form of numerical data or reflecting complex phenomena such as feelings or reactions. Dependent variables change due to the other factors measured, especially if a study employs an experimental or semi-experimental design. Independent variables are stable: they are both presumed causes and conditions in the environment or milieu being manipulated.
Identifying Independent and Dependent Variables
Even though the definitions of the terms independent and dependent variables may appear to be clear, in the process of analyzing data resulting from actual research, identifying the variables properly might be challenging. Here is a simple rule that you can apply at all times: the independent variable is what a researcher changes, whereas the dependent variable is affected by these changes. To illustrate the difference, a number of examples are provided below.
- The purpose of Study 1 is to measure the impact of different plant fertilizers on how many fruits apple trees bear. Independent variable : plant fertilizers (chosen by researchers) Dependent variable : fruits that the trees bear (affected by choice of fertilizers)
- The purpose of Study 2 is to find an association between living in close vicinity to hydraulic fracturing sites and respiratory diseases. Independent variable: proximity to hydraulic fracturing sites (a presumed cause and a condition of the environment) Dependent variable: the percentage/ likelihood of suffering from respiratory diseases
Confusion is possible in identifying independent and dependent variables in the social sciences. When considering psychological phenomena and human behavior, it can be difficult to distinguish between cause and effect. For example, the purpose of Study 3 is to establish how tactics for coping with stress are linked to the level of stress-resilience in college students. Even though it is feasible to speculate that these variables are interdependent, the following factors should be taken into account in order to clearly define which variable is dependent and which is interdependent.
- The dependent variable is usually the objective of the research. In the study under examination, the levels of stress resilience are being investigated.
- The independent variable precedes the dependent variable. The chosen stress-related coping techniques help to build resilience; thus, they occur earlier.
Writing Style and Structure
Usually, the variables are first described in the introduction of a research paper and then in the method section. No strict guidelines for approaching the subject exist; however, academic writing demands that the researcher make clear and concise statements. It is only reasonable not to leave readers guessing which of the variables is dependent and which is independent. The description should reflect the literature review, where both types of variables are identified in the context of the previous research. For instance, in the case of Study 3, a researcher would have to provide an explanation as to the meaning of stress resilience and coping tactics.
In properly organizing a research paper, it is essential to outline and operationalize the appropriate independent and dependent variables. Moreover, the paper should differentiate clearly between independent and dependent variables. Finding the dependent variable is typically the objective of a study, whereas independent variables reflect influencing factors that can be manipulated. Distinguishing between the two types of variables in social sciences may be somewhat challenging as it can be easy to confuse cause with effect. Academic format calls for the author to mention the variables in the introduction and then provide a detailed description in the method section.
Unfortunately, your browser is too old to work on this site.
For full functionality of this site it is necessary to enable JavaScript.
- USC Libraries
- Research Guides
Organizing Your Social Sciences Research Paper
- Types of Research Designs
- Purpose of Guide
- Design Flaws to Avoid
- Independent and Dependent Variables
- Glossary of Research Terms
- Reading Research Effectively
- Narrowing a Topic Idea
- Broadening a Topic Idea
- Extending the Timeliness of a Topic Idea
- Academic Writing Style
- Applying Critical Thinking
- Choosing a Title
- Making an Outline
- Paragraph Development
- Research Process Video Series
- Executive Summary
- The C.A.R.S. Model
- Background Information
- The Research Problem/Question
- Theoretical Framework
- Citation Tracking
- Content Alert Services
- Evaluating Sources
- Primary Sources
- Secondary Sources
- Tiertiary Sources
- Scholarly vs. Popular Publications
- Qualitative Methods
- Quantitative Methods
- Insiderness
- Using Non-Textual Elements
- Limitations of the Study
- Common Grammar Mistakes
- Writing Concisely
- Avoiding Plagiarism
- Footnotes or Endnotes?
- Further Readings
- Generative AI and Writing
- USC Libraries Tutorials and Other Guides
- Bibliography
Introduction
Before beginning your paper, you need to decide how you plan to design the study .
The research design refers to the overall strategy and analytical approach that you have chosen in order to integrate, in a coherent and logical way, the different components of the study, thus ensuring that the research problem will be thoroughly investigated. It constitutes the blueprint for the collection, measurement, and interpretation of information and data. Note that the research problem determines the type of design you choose, not the other way around!
De Vaus, D. A. Research Design in Social Research . London: SAGE, 2001; Trochim, William M.K. Research Methods Knowledge Base. 2006.
General Structure and Writing Style
The function of a research design is to ensure that the evidence obtained enables you to effectively address the research problem logically and as unambiguously as possible . In social sciences research, obtaining information relevant to the research problem generally entails specifying the type of evidence needed to test the underlying assumptions of a theory, to evaluate a program, or to accurately describe and assess meaning related to an observable phenomenon.
With this in mind, a common mistake made by researchers is that they begin their investigations before they have thought critically about what information is required to address the research problem. Without attending to these design issues beforehand, the overall research problem will not be adequately addressed and any conclusions drawn will run the risk of being weak and unconvincing. As a consequence, the overall validity of the study will be undermined.
The length and complexity of describing the research design in your paper can vary considerably, but any well-developed description will achieve the following :
- Identify the research problem clearly and justify its selection, particularly in relation to any valid alternative designs that could have been used,
- Review and synthesize previously published literature associated with the research problem,
- Clearly and explicitly specify hypotheses [i.e., research questions] central to the problem,
- Effectively describe the information and/or data which will be necessary for an adequate testing of the hypotheses and explain how such information and/or data will be obtained, and
- Describe the methods of analysis to be applied to the data in determining whether or not the hypotheses are true or false.
The research design is usually incorporated into the introduction of your paper . You can obtain an overall sense of what to do by reviewing studies that have utilized the same research design [e.g., using a case study approach]. This can help you develop an outline to follow for your own paper.
NOTE : Use the SAGE Research Methods Online and Cases and the SAGE Research Methods Videos databases to search for scholarly resources on how to apply specific research designs and methods . The Research Methods Online database contains links to more than 175,000 pages of SAGE publisher's book, journal, and reference content on quantitative, qualitative, and mixed research methodologies. Also included is a collection of case studies of social research projects that can be used to help you better understand abstract or complex methodological concepts. The Research Methods Videos database contains hours of tutorials, interviews, video case studies, and mini-documentaries covering the entire research process.
Creswell, John W. and J. David Creswell. Research Design: Qualitative, Quantitative, and Mixed Methods Approaches . 5th edition. Thousand Oaks, CA: Sage, 2018; De Vaus, D. A. Research Design in Social Research . London: SAGE, 2001; Gorard, Stephen. Research Design: Creating Robust Approaches for the Social Sciences . Thousand Oaks, CA: Sage, 2013; Leedy, Paul D. and Jeanne Ellis Ormrod. Practical Research: Planning and Design . Tenth edition. Boston, MA: Pearson, 2013; Vogt, W. Paul, Dianna C. Gardner, and Lynne M. Haeffele. When to Use What Research Design . New York: Guilford, 2012.
Action Research Design
Definition and Purpose
The essentials of action research design follow a characteristic cycle whereby initially an exploratory stance is adopted, where an understanding of a problem is developed and plans are made for some form of interventionary strategy. Then the intervention is carried out [the "action" in action research] during which time, pertinent observations are collected in various forms. The new interventional strategies are carried out, and this cyclic process repeats, continuing until a sufficient understanding of [or a valid implementation solution for] the problem is achieved. The protocol is iterative or cyclical in nature and is intended to foster deeper understanding of a given situation, starting with conceptualizing and particularizing the problem and moving through several interventions and evaluations.
What do these studies tell you ?
- This is a collaborative and adaptive research design that lends itself to use in work or community situations.
- Design focuses on pragmatic and solution-driven research outcomes rather than testing theories.
- When practitioners use action research, it has the potential to increase the amount they learn consciously from their experience; the action research cycle can be regarded as a learning cycle.
- Action research studies often have direct and obvious relevance to improving practice and advocating for change.
- There are no hidden controls or preemption of direction by the researcher.
What these studies don't tell you ?
- It is harder to do than conducting conventional research because the researcher takes on responsibilities of advocating for change as well as for researching the topic.
- Action research is much harder to write up because it is less likely that you can use a standard format to report your findings effectively [i.e., data is often in the form of stories or observation].
- Personal over-involvement of the researcher may bias research results.
- The cyclic nature of action research to achieve its twin outcomes of action [e.g. change] and research [e.g. understanding] is time-consuming and complex to conduct.
- Advocating for change usually requires buy-in from study participants.
Coghlan, David and Mary Brydon-Miller. The Sage Encyclopedia of Action Research . Thousand Oaks, CA: Sage, 2014; Efron, Sara Efrat and Ruth Ravid. Action Research in Education: A Practical Guide . New York: Guilford, 2013; Gall, Meredith. Educational Research: An Introduction . Chapter 18, Action Research. 8th ed. Boston, MA: Pearson/Allyn and Bacon, 2007; Gorard, Stephen. Research Design: Creating Robust Approaches for the Social Sciences . Thousand Oaks, CA: Sage, 2013; Kemmis, Stephen and Robin McTaggart. “Participatory Action Research.” In Handbook of Qualitative Research . Norman Denzin and Yvonna S. Lincoln, eds. 2nd ed. (Thousand Oaks, CA: SAGE, 2000), pp. 567-605; McNiff, Jean. Writing and Doing Action Research . London: Sage, 2014; Reason, Peter and Hilary Bradbury. Handbook of Action Research: Participative Inquiry and Practice . Thousand Oaks, CA: SAGE, 2001.
Case Study Design
A case study is an in-depth study of a particular research problem rather than a sweeping statistical survey or comprehensive comparative inquiry. It is often used to narrow down a very broad field of research into one or a few easily researchable examples. The case study research design is also useful for testing whether a specific theory and model actually applies to phenomena in the real world. It is a useful design when not much is known about an issue or phenomenon.
- Approach excels at bringing us to an understanding of a complex issue through detailed contextual analysis of a limited number of events or conditions and their relationships.
- A researcher using a case study design can apply a variety of methodologies and rely on a variety of sources to investigate a research problem.
- Design can extend experience or add strength to what is already known through previous research.
- Social scientists, in particular, make wide use of this research design to examine contemporary real-life situations and provide the basis for the application of concepts and theories and the extension of methodologies.
- The design can provide detailed descriptions of specific and rare cases.
- A single or small number of cases offers little basis for establishing reliability or to generalize the findings to a wider population of people, places, or things.
- Intense exposure to the study of a case may bias a researcher's interpretation of the findings.
- Design does not facilitate assessment of cause and effect relationships.
- Vital information may be missing, making the case hard to interpret.
- The case may not be representative or typical of the larger problem being investigated.
- If the criteria for selecting a case is because it represents a very unusual or unique phenomenon or problem for study, then your interpretation of the findings can only apply to that particular case.
Case Studies. Writing@CSU. Colorado State University; Anastas, Jeane W. Research Design for Social Work and the Human Services . Chapter 4, Flexible Methods: Case Study Design. 2nd ed. New York: Columbia University Press, 1999; Gerring, John. “What Is a Case Study and What Is It Good for?” American Political Science Review 98 (May 2004): 341-354; Greenhalgh, Trisha, editor. Case Study Evaluation: Past, Present and Future Challenges . Bingley, UK: Emerald Group Publishing, 2015; Mills, Albert J. , Gabrielle Durepos, and Eiden Wiebe, editors. Encyclopedia of Case Study Research . Thousand Oaks, CA: SAGE Publications, 2010; Stake, Robert E. The Art of Case Study Research . Thousand Oaks, CA: SAGE, 1995; Yin, Robert K. Case Study Research: Design and Theory . Applied Social Research Methods Series, no. 5. 3rd ed. Thousand Oaks, CA: SAGE, 2003.
Causal Design
Causality studies may be thought of as understanding a phenomenon in terms of conditional statements in the form, “If X, then Y.” This type of research is used to measure what impact a specific change will have on existing norms and assumptions. Most social scientists seek causal explanations that reflect tests of hypotheses. Causal effect (nomothetic perspective) occurs when variation in one phenomenon, an independent variable, leads to or results, on average, in variation in another phenomenon, the dependent variable.
Conditions necessary for determining causality:
- Empirical association -- a valid conclusion is based on finding an association between the independent variable and the dependent variable.
- Appropriate time order -- to conclude that causation was involved, one must see that cases were exposed to variation in the independent variable before variation in the dependent variable.
- Nonspuriousness -- a relationship between two variables that is not due to variation in a third variable.
- Causality research designs assist researchers in understanding why the world works the way it does through the process of proving a causal link between variables and by the process of eliminating other possibilities.
- Replication is possible.
- There is greater confidence the study has internal validity due to the systematic subject selection and equity of groups being compared.
- Not all relationships are causal! The possibility always exists that, by sheer coincidence, two unrelated events appear to be related [e.g., Punxatawney Phil could accurately predict the duration of Winter for five consecutive years but, the fact remains, he's just a big, furry rodent].
- Conclusions about causal relationships are difficult to determine due to a variety of extraneous and confounding variables that exist in a social environment. This means causality can only be inferred, never proven.
- If two variables are correlated, the cause must come before the effect. However, even though two variables might be causally related, it can sometimes be difficult to determine which variable comes first and, therefore, to establish which variable is the actual cause and which is the actual effect.
Beach, Derek and Rasmus Brun Pedersen. Causal Case Study Methods: Foundations and Guidelines for Comparing, Matching, and Tracing . Ann Arbor, MI: University of Michigan Press, 2016; Bachman, Ronet. The Practice of Research in Criminology and Criminal Justice . Chapter 5, Causation and Research Designs. 3rd ed. Thousand Oaks, CA: Pine Forge Press, 2007; Brewer, Ernest W. and Jennifer Kubn. “Causal-Comparative Design.” In Encyclopedia of Research Design . Neil J. Salkind, editor. (Thousand Oaks, CA: Sage, 2010), pp. 125-132; Causal Research Design: Experimentation. Anonymous SlideShare Presentation; Gall, Meredith. Educational Research: An Introduction . Chapter 11, Nonexperimental Research: Correlational Designs. 8th ed. Boston, MA: Pearson/Allyn and Bacon, 2007; Trochim, William M.K. Research Methods Knowledge Base. 2006.
Cohort Design
Often used in the medical sciences, but also found in the applied social sciences, a cohort study generally refers to a study conducted over a period of time involving members of a population which the subject or representative member comes from, and who are united by some commonality or similarity. Using a quantitative framework, a cohort study makes note of statistical occurrence within a specialized subgroup, united by same or similar characteristics that are relevant to the research problem being investigated, rather than studying statistical occurrence within the general population. Using a qualitative framework, cohort studies generally gather data using methods of observation. Cohorts can be either "open" or "closed."
- Open Cohort Studies [dynamic populations, such as the population of Los Angeles] involve a population that is defined just by the state of being a part of the study in question (and being monitored for the outcome). Date of entry and exit from the study is individually defined, therefore, the size of the study population is not constant. In open cohort studies, researchers can only calculate rate based data, such as, incidence rates and variants thereof.
- Closed Cohort Studies [static populations, such as patients entered into a clinical trial] involve participants who enter into the study at one defining point in time and where it is presumed that no new participants can enter the cohort. Given this, the number of study participants remains constant (or can only decrease).
- The use of cohorts is often mandatory because a randomized control study may be unethical. For example, you cannot deliberately expose people to asbestos, you can only study its effects on those who have already been exposed. Research that measures risk factors often relies upon cohort designs.
- Because cohort studies measure potential causes before the outcome has occurred, they can demonstrate that these “causes” preceded the outcome, thereby avoiding the debate as to which is the cause and which is the effect.
- Cohort analysis is highly flexible and can provide insight into effects over time and related to a variety of different types of changes [e.g., social, cultural, political, economic, etc.].
- Either original data or secondary data can be used in this design.
- In cases where a comparative analysis of two cohorts is made [e.g., studying the effects of one group exposed to asbestos and one that has not], a researcher cannot control for all other factors that might differ between the two groups. These factors are known as confounding variables.
- Cohort studies can end up taking a long time to complete if the researcher must wait for the conditions of interest to develop within the group. This also increases the chance that key variables change during the course of the study, potentially impacting the validity of the findings.
- Due to the lack of randominization in the cohort design, its external validity is lower than that of study designs where the researcher randomly assigns participants.
Healy P, Devane D. “Methodological Considerations in Cohort Study Designs.” Nurse Researcher 18 (2011): 32-36; Glenn, Norval D, editor. Cohort Analysis . 2nd edition. Thousand Oaks, CA: Sage, 2005; Levin, Kate Ann. Study Design IV: Cohort Studies. Evidence-Based Dentistry 7 (2003): 51–52; Payne, Geoff. “Cohort Study.” In The SAGE Dictionary of Social Research Methods . Victor Jupp, editor. (Thousand Oaks, CA: Sage, 2006), pp. 31-33; Study Design 101. Himmelfarb Health Sciences Library. George Washington University, November 2011; Cohort Study. Wikipedia.
Cross-Sectional Design
Cross-sectional research designs have three distinctive features: no time dimension; a reliance on existing differences rather than change following intervention; and, groups are selected based on existing differences rather than random allocation. The cross-sectional design can only measure differences between or from among a variety of people, subjects, or phenomena rather than a process of change. As such, researchers using this design can only employ a relatively passive approach to making causal inferences based on findings.
- Cross-sectional studies provide a clear 'snapshot' of the outcome and the characteristics associated with it, at a specific point in time.
- Unlike an experimental design, where there is an active intervention by the researcher to produce and measure change or to create differences, cross-sectional designs focus on studying and drawing inferences from existing differences between people, subjects, or phenomena.
- Entails collecting data at and concerning one point in time. While longitudinal studies involve taking multiple measures over an extended period of time, cross-sectional research is focused on finding relationships between variables at one moment in time.
- Groups identified for study are purposely selected based upon existing differences in the sample rather than seeking random sampling.
- Cross-section studies are capable of using data from a large number of subjects and, unlike observational studies, is not geographically bound.
- Can estimate prevalence of an outcome of interest because the sample is usually taken from the whole population.
- Because cross-sectional designs generally use survey techniques to gather data, they are relatively inexpensive and take up little time to conduct.
- Finding people, subjects, or phenomena to study that are very similar except in one specific variable can be difficult.
- Results are static and time bound and, therefore, give no indication of a sequence of events or reveal historical or temporal contexts.
- Studies cannot be utilized to establish cause and effect relationships.
- This design only provides a snapshot of analysis so there is always the possibility that a study could have differing results if another time-frame had been chosen.
- There is no follow up to the findings.
Bethlehem, Jelke. "7: Cross-sectional Research." In Research Methodology in the Social, Behavioural and Life Sciences . Herman J Adèr and Gideon J Mellenbergh, editors. (London, England: Sage, 1999), pp. 110-43; Bourque, Linda B. “Cross-Sectional Design.” In The SAGE Encyclopedia of Social Science Research Methods . Michael S. Lewis-Beck, Alan Bryman, and Tim Futing Liao. (Thousand Oaks, CA: 2004), pp. 230-231; Hall, John. “Cross-Sectional Survey Design.” In Encyclopedia of Survey Research Methods . Paul J. Lavrakas, ed. (Thousand Oaks, CA: Sage, 2008), pp. 173-174; Helen Barratt, Maria Kirwan. Cross-Sectional Studies: Design Application, Strengths and Weaknesses of Cross-Sectional Studies. Healthknowledge, 2009. Cross-Sectional Study. Wikipedia.
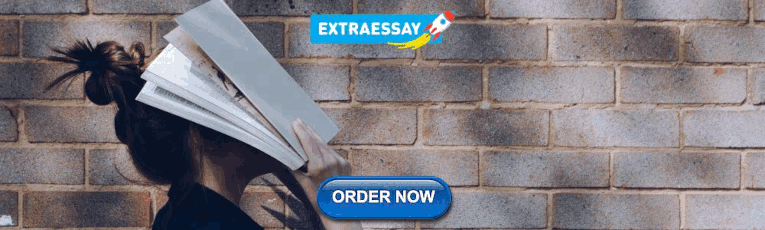
Descriptive Design
Descriptive research designs help provide answers to the questions of who, what, when, where, and how associated with a particular research problem; a descriptive study cannot conclusively ascertain answers to why. Descriptive research is used to obtain information concerning the current status of the phenomena and to describe "what exists" with respect to variables or conditions in a situation.
- The subject is being observed in a completely natural and unchanged natural environment. True experiments, whilst giving analyzable data, often adversely influence the normal behavior of the subject [a.k.a., the Heisenberg effect whereby measurements of certain systems cannot be made without affecting the systems].
- Descriptive research is often used as a pre-cursor to more quantitative research designs with the general overview giving some valuable pointers as to what variables are worth testing quantitatively.
- If the limitations are understood, they can be a useful tool in developing a more focused study.
- Descriptive studies can yield rich data that lead to important recommendations in practice.
- Appoach collects a large amount of data for detailed analysis.
- The results from a descriptive research cannot be used to discover a definitive answer or to disprove a hypothesis.
- Because descriptive designs often utilize observational methods [as opposed to quantitative methods], the results cannot be replicated.
- The descriptive function of research is heavily dependent on instrumentation for measurement and observation.
Anastas, Jeane W. Research Design for Social Work and the Human Services . Chapter 5, Flexible Methods: Descriptive Research. 2nd ed. New York: Columbia University Press, 1999; Given, Lisa M. "Descriptive Research." In Encyclopedia of Measurement and Statistics . Neil J. Salkind and Kristin Rasmussen, editors. (Thousand Oaks, CA: Sage, 2007), pp. 251-254; McNabb, Connie. Descriptive Research Methodologies. Powerpoint Presentation; Shuttleworth, Martyn. Descriptive Research Design, September 26, 2008; Erickson, G. Scott. "Descriptive Research Design." In New Methods of Market Research and Analysis . (Northampton, MA: Edward Elgar Publishing, 2017), pp. 51-77; Sahin, Sagufta, and Jayanta Mete. "A Brief Study on Descriptive Research: Its Nature and Application in Social Science." International Journal of Research and Analysis in Humanities 1 (2021): 11; K. Swatzell and P. Jennings. “Descriptive Research: The Nuts and Bolts.” Journal of the American Academy of Physician Assistants 20 (2007), pp. 55-56; Kane, E. Doing Your Own Research: Basic Descriptive Research in the Social Sciences and Humanities . London: Marion Boyars, 1985.
Experimental Design
A blueprint of the procedure that enables the researcher to maintain control over all factors that may affect the result of an experiment. In doing this, the researcher attempts to determine or predict what may occur. Experimental research is often used where there is time priority in a causal relationship (cause precedes effect), there is consistency in a causal relationship (a cause will always lead to the same effect), and the magnitude of the correlation is great. The classic experimental design specifies an experimental group and a control group. The independent variable is administered to the experimental group and not to the control group, and both groups are measured on the same dependent variable. Subsequent experimental designs have used more groups and more measurements over longer periods. True experiments must have control, randomization, and manipulation.
- Experimental research allows the researcher to control the situation. In so doing, it allows researchers to answer the question, “What causes something to occur?”
- Permits the researcher to identify cause and effect relationships between variables and to distinguish placebo effects from treatment effects.
- Experimental research designs support the ability to limit alternative explanations and to infer direct causal relationships in the study.
- Approach provides the highest level of evidence for single studies.
- The design is artificial, and results may not generalize well to the real world.
- The artificial settings of experiments may alter the behaviors or responses of participants.
- Experimental designs can be costly if special equipment or facilities are needed.
- Some research problems cannot be studied using an experiment because of ethical or technical reasons.
- Difficult to apply ethnographic and other qualitative methods to experimentally designed studies.
Anastas, Jeane W. Research Design for Social Work and the Human Services . Chapter 7, Flexible Methods: Experimental Research. 2nd ed. New York: Columbia University Press, 1999; Chapter 2: Research Design, Experimental Designs. School of Psychology, University of New England, 2000; Chow, Siu L. "Experimental Design." In Encyclopedia of Research Design . Neil J. Salkind, editor. (Thousand Oaks, CA: Sage, 2010), pp. 448-453; "Experimental Design." In Social Research Methods . Nicholas Walliman, editor. (London, England: Sage, 2006), pp, 101-110; Experimental Research. Research Methods by Dummies. Department of Psychology. California State University, Fresno, 2006; Kirk, Roger E. Experimental Design: Procedures for the Behavioral Sciences . 4th edition. Thousand Oaks, CA: Sage, 2013; Trochim, William M.K. Experimental Design. Research Methods Knowledge Base. 2006; Rasool, Shafqat. Experimental Research. Slideshare presentation.
Exploratory Design
An exploratory design is conducted about a research problem when there are few or no earlier studies to refer to or rely upon to predict an outcome . The focus is on gaining insights and familiarity for later investigation or undertaken when research problems are in a preliminary stage of investigation. Exploratory designs are often used to establish an understanding of how best to proceed in studying an issue or what methodology would effectively apply to gathering information about the issue.
The goals of exploratory research are intended to produce the following possible insights:
- Familiarity with basic details, settings, and concerns.
- Well grounded picture of the situation being developed.
- Generation of new ideas and assumptions.
- Development of tentative theories or hypotheses.
- Determination about whether a study is feasible in the future.
- Issues get refined for more systematic investigation and formulation of new research questions.
- Direction for future research and techniques get developed.
- Design is a useful approach for gaining background information on a particular topic.
- Exploratory research is flexible and can address research questions of all types (what, why, how).
- Provides an opportunity to define new terms and clarify existing concepts.
- Exploratory research is often used to generate formal hypotheses and develop more precise research problems.
- In the policy arena or applied to practice, exploratory studies help establish research priorities and where resources should be allocated.
- Exploratory research generally utilizes small sample sizes and, thus, findings are typically not generalizable to the population at large.
- The exploratory nature of the research inhibits an ability to make definitive conclusions about the findings. They provide insight but not definitive conclusions.
- The research process underpinning exploratory studies is flexible but often unstructured, leading to only tentative results that have limited value to decision-makers.
- Design lacks rigorous standards applied to methods of data gathering and analysis because one of the areas for exploration could be to determine what method or methodologies could best fit the research problem.
Cuthill, Michael. “Exploratory Research: Citizen Participation, Local Government, and Sustainable Development in Australia.” Sustainable Development 10 (2002): 79-89; Streb, Christoph K. "Exploratory Case Study." In Encyclopedia of Case Study Research . Albert J. Mills, Gabrielle Durepos and Eiden Wiebe, editors. (Thousand Oaks, CA: Sage, 2010), pp. 372-374; Taylor, P. J., G. Catalano, and D.R.F. Walker. “Exploratory Analysis of the World City Network.” Urban Studies 39 (December 2002): 2377-2394; Exploratory Research. Wikipedia.
Field Research Design
Sometimes referred to as ethnography or participant observation, designs around field research encompass a variety of interpretative procedures [e.g., observation and interviews] rooted in qualitative approaches to studying people individually or in groups while inhabiting their natural environment as opposed to using survey instruments or other forms of impersonal methods of data gathering. Information acquired from observational research takes the form of “ field notes ” that involves documenting what the researcher actually sees and hears while in the field. Findings do not consist of conclusive statements derived from numbers and statistics because field research involves analysis of words and observations of behavior. Conclusions, therefore, are developed from an interpretation of findings that reveal overriding themes, concepts, and ideas. More information can be found HERE .
- Field research is often necessary to fill gaps in understanding the research problem applied to local conditions or to specific groups of people that cannot be ascertained from existing data.
- The research helps contextualize already known information about a research problem, thereby facilitating ways to assess the origins, scope, and scale of a problem and to gage the causes, consequences, and means to resolve an issue based on deliberate interaction with people in their natural inhabited spaces.
- Enables the researcher to corroborate or confirm data by gathering additional information that supports or refutes findings reported in prior studies of the topic.
- Because the researcher in embedded in the field, they are better able to make observations or ask questions that reflect the specific cultural context of the setting being investigated.
- Observing the local reality offers the opportunity to gain new perspectives or obtain unique data that challenges existing theoretical propositions or long-standing assumptions found in the literature.
What these studies don't tell you
- A field research study requires extensive time and resources to carry out the multiple steps involved with preparing for the gathering of information, including for example, examining background information about the study site, obtaining permission to access the study site, and building trust and rapport with subjects.
- Requires a commitment to staying engaged in the field to ensure that you can adequately document events and behaviors as they unfold.
- The unpredictable nature of fieldwork means that researchers can never fully control the process of data gathering. They must maintain a flexible approach to studying the setting because events and circumstances can change quickly or unexpectedly.
- Findings can be difficult to interpret and verify without access to documents and other source materials that help to enhance the credibility of information obtained from the field [i.e., the act of triangulating the data].
- Linking the research problem to the selection of study participants inhabiting their natural environment is critical. However, this specificity limits the ability to generalize findings to different situations or in other contexts or to infer courses of action applied to other settings or groups of people.
- The reporting of findings must take into account how the researcher themselves may have inadvertently affected respondents and their behaviors.
Historical Design
The purpose of a historical research design is to collect, verify, and synthesize evidence from the past to establish facts that defend or refute a hypothesis. It uses secondary sources and a variety of primary documentary evidence, such as, diaries, official records, reports, archives, and non-textual information [maps, pictures, audio and visual recordings]. The limitation is that the sources must be both authentic and valid.
- The historical research design is unobtrusive; the act of research does not affect the results of the study.
- The historical approach is well suited for trend analysis.
- Historical records can add important contextual background required to more fully understand and interpret a research problem.
- There is often no possibility of researcher-subject interaction that could affect the findings.
- Historical sources can be used over and over to study different research problems or to replicate a previous study.
- The ability to fulfill the aims of your research are directly related to the amount and quality of documentation available to understand the research problem.
- Since historical research relies on data from the past, there is no way to manipulate it to control for contemporary contexts.
- Interpreting historical sources can be very time consuming.
- The sources of historical materials must be archived consistently to ensure access. This may especially challenging for digital or online-only sources.
- Original authors bring their own perspectives and biases to the interpretation of past events and these biases are more difficult to ascertain in historical resources.
- Due to the lack of control over external variables, historical research is very weak with regard to the demands of internal validity.
- It is rare that the entirety of historical documentation needed to fully address a research problem is available for interpretation, therefore, gaps need to be acknowledged.
Howell, Martha C. and Walter Prevenier. From Reliable Sources: An Introduction to Historical Methods . Ithaca, NY: Cornell University Press, 2001; Lundy, Karen Saucier. "Historical Research." In The Sage Encyclopedia of Qualitative Research Methods . Lisa M. Given, editor. (Thousand Oaks, CA: Sage, 2008), pp. 396-400; Marius, Richard. and Melvin E. Page. A Short Guide to Writing about History . 9th edition. Boston, MA: Pearson, 2015; Savitt, Ronald. “Historical Research in Marketing.” Journal of Marketing 44 (Autumn, 1980): 52-58; Gall, Meredith. Educational Research: An Introduction . Chapter 16, Historical Research. 8th ed. Boston, MA: Pearson/Allyn and Bacon, 2007.
Longitudinal Design
A longitudinal study follows the same sample over time and makes repeated observations. For example, with longitudinal surveys, the same group of people is interviewed at regular intervals, enabling researchers to track changes over time and to relate them to variables that might explain why the changes occur. Longitudinal research designs describe patterns of change and help establish the direction and magnitude of causal relationships. Measurements are taken on each variable over two or more distinct time periods. This allows the researcher to measure change in variables over time. It is a type of observational study sometimes referred to as a panel study.
- Longitudinal data facilitate the analysis of the duration of a particular phenomenon.
- Enables survey researchers to get close to the kinds of causal explanations usually attainable only with experiments.
- The design permits the measurement of differences or change in a variable from one period to another [i.e., the description of patterns of change over time].
- Longitudinal studies facilitate the prediction of future outcomes based upon earlier factors.
- The data collection method may change over time.
- Maintaining the integrity of the original sample can be difficult over an extended period of time.
- It can be difficult to show more than one variable at a time.
- This design often needs qualitative research data to explain fluctuations in the results.
- A longitudinal research design assumes present trends will continue unchanged.
- It can take a long period of time to gather results.
- There is a need to have a large sample size and accurate sampling to reach representativness.
Anastas, Jeane W. Research Design for Social Work and the Human Services . Chapter 6, Flexible Methods: Relational and Longitudinal Research. 2nd ed. New York: Columbia University Press, 1999; Forgues, Bernard, and Isabelle Vandangeon-Derumez. "Longitudinal Analyses." In Doing Management Research . Raymond-Alain Thiétart and Samantha Wauchope, editors. (London, England: Sage, 2001), pp. 332-351; Kalaian, Sema A. and Rafa M. Kasim. "Longitudinal Studies." In Encyclopedia of Survey Research Methods . Paul J. Lavrakas, ed. (Thousand Oaks, CA: Sage, 2008), pp. 440-441; Menard, Scott, editor. Longitudinal Research . Thousand Oaks, CA: Sage, 2002; Ployhart, Robert E. and Robert J. Vandenberg. "Longitudinal Research: The Theory, Design, and Analysis of Change.” Journal of Management 36 (January 2010): 94-120; Longitudinal Study. Wikipedia.
Meta-Analysis Design
Meta-analysis is an analytical methodology designed to systematically evaluate and summarize the results from a number of individual studies, thereby, increasing the overall sample size and the ability of the researcher to study effects of interest. The purpose is to not simply summarize existing knowledge, but to develop a new understanding of a research problem using synoptic reasoning. The main objectives of meta-analysis include analyzing differences in the results among studies and increasing the precision by which effects are estimated. A well-designed meta-analysis depends upon strict adherence to the criteria used for selecting studies and the availability of information in each study to properly analyze their findings. Lack of information can severely limit the type of analyzes and conclusions that can be reached. In addition, the more dissimilarity there is in the results among individual studies [heterogeneity], the more difficult it is to justify interpretations that govern a valid synopsis of results. A meta-analysis needs to fulfill the following requirements to ensure the validity of your findings:
- Clearly defined description of objectives, including precise definitions of the variables and outcomes that are being evaluated;
- A well-reasoned and well-documented justification for identification and selection of the studies;
- Assessment and explicit acknowledgment of any researcher bias in the identification and selection of those studies;
- Description and evaluation of the degree of heterogeneity among the sample size of studies reviewed; and,
- Justification of the techniques used to evaluate the studies.
- Can be an effective strategy for determining gaps in the literature.
- Provides a means of reviewing research published about a particular topic over an extended period of time and from a variety of sources.
- Is useful in clarifying what policy or programmatic actions can be justified on the basis of analyzing research results from multiple studies.
- Provides a method for overcoming small sample sizes in individual studies that previously may have had little relationship to each other.
- Can be used to generate new hypotheses or highlight research problems for future studies.
- Small violations in defining the criteria used for content analysis can lead to difficult to interpret and/or meaningless findings.
- A large sample size can yield reliable, but not necessarily valid, results.
- A lack of uniformity regarding, for example, the type of literature reviewed, how methods are applied, and how findings are measured within the sample of studies you are analyzing, can make the process of synthesis difficult to perform.
- Depending on the sample size, the process of reviewing and synthesizing multiple studies can be very time consuming.
Beck, Lewis W. "The Synoptic Method." The Journal of Philosophy 36 (1939): 337-345; Cooper, Harris, Larry V. Hedges, and Jeffrey C. Valentine, eds. The Handbook of Research Synthesis and Meta-Analysis . 2nd edition. New York: Russell Sage Foundation, 2009; Guzzo, Richard A., Susan E. Jackson and Raymond A. Katzell. “Meta-Analysis Analysis.” In Research in Organizational Behavior , Volume 9. (Greenwich, CT: JAI Press, 1987), pp 407-442; Lipsey, Mark W. and David B. Wilson. Practical Meta-Analysis . Thousand Oaks, CA: Sage Publications, 2001; Study Design 101. Meta-Analysis. The Himmelfarb Health Sciences Library, George Washington University; Timulak, Ladislav. “Qualitative Meta-Analysis.” In The SAGE Handbook of Qualitative Data Analysis . Uwe Flick, editor. (Los Angeles, CA: Sage, 2013), pp. 481-495; Walker, Esteban, Adrian V. Hernandez, and Micheal W. Kattan. "Meta-Analysis: It's Strengths and Limitations." Cleveland Clinic Journal of Medicine 75 (June 2008): 431-439.
Mixed-Method Design
- Narrative and non-textual information can add meaning to numeric data, while numeric data can add precision to narrative and non-textual information.
- Can utilize existing data while at the same time generating and testing a grounded theory approach to describe and explain the phenomenon under study.
- A broader, more complex research problem can be investigated because the researcher is not constrained by using only one method.
- The strengths of one method can be used to overcome the inherent weaknesses of another method.
- Can provide stronger, more robust evidence to support a conclusion or set of recommendations.
- May generate new knowledge new insights or uncover hidden insights, patterns, or relationships that a single methodological approach might not reveal.
- Produces more complete knowledge and understanding of the research problem that can be used to increase the generalizability of findings applied to theory or practice.
- A researcher must be proficient in understanding how to apply multiple methods to investigating a research problem as well as be proficient in optimizing how to design a study that coherently melds them together.
- Can increase the likelihood of conflicting results or ambiguous findings that inhibit drawing a valid conclusion or setting forth a recommended course of action [e.g., sample interview responses do not support existing statistical data].
- Because the research design can be very complex, reporting the findings requires a well-organized narrative, clear writing style, and precise word choice.
- Design invites collaboration among experts. However, merging different investigative approaches and writing styles requires more attention to the overall research process than studies conducted using only one methodological paradigm.
- Concurrent merging of quantitative and qualitative research requires greater attention to having adequate sample sizes, using comparable samples, and applying a consistent unit of analysis. For sequential designs where one phase of qualitative research builds on the quantitative phase or vice versa, decisions about what results from the first phase to use in the next phase, the choice of samples and estimating reasonable sample sizes for both phases, and the interpretation of results from both phases can be difficult.
- Due to multiple forms of data being collected and analyzed, this design requires extensive time and resources to carry out the multiple steps involved in data gathering and interpretation.
Burch, Patricia and Carolyn J. Heinrich. Mixed Methods for Policy Research and Program Evaluation . Thousand Oaks, CA: Sage, 2016; Creswell, John w. et al. Best Practices for Mixed Methods Research in the Health Sciences . Bethesda, MD: Office of Behavioral and Social Sciences Research, National Institutes of Health, 2010Creswell, John W. Research Design: Qualitative, Quantitative, and Mixed Methods Approaches . 4th edition. Thousand Oaks, CA: Sage Publications, 2014; Domínguez, Silvia, editor. Mixed Methods Social Networks Research . Cambridge, UK: Cambridge University Press, 2014; Hesse-Biber, Sharlene Nagy. Mixed Methods Research: Merging Theory with Practice . New York: Guilford Press, 2010; Niglas, Katrin. “How the Novice Researcher Can Make Sense of Mixed Methods Designs.” International Journal of Multiple Research Approaches 3 (2009): 34-46; Onwuegbuzie, Anthony J. and Nancy L. Leech. “Linking Research Questions to Mixed Methods Data Analysis Procedures.” The Qualitative Report 11 (September 2006): 474-498; Tashakorri, Abbas and John W. Creswell. “The New Era of Mixed Methods.” Journal of Mixed Methods Research 1 (January 2007): 3-7; Zhanga, Wanqing. “Mixed Methods Application in Health Intervention Research: A Multiple Case Study.” International Journal of Multiple Research Approaches 8 (2014): 24-35 .
Observational Design
This type of research design draws a conclusion by comparing subjects against a control group, in cases where the researcher has no control over the experiment. There are two general types of observational designs. In direct observations, people know that you are watching them. Unobtrusive measures involve any method for studying behavior where individuals do not know they are being observed. An observational study allows a useful insight into a phenomenon and avoids the ethical and practical difficulties of setting up a large and cumbersome research project.
- Observational studies are usually flexible and do not necessarily need to be structured around a hypothesis about what you expect to observe [data is emergent rather than pre-existing].
- The researcher is able to collect in-depth information about a particular behavior.
- Can reveal interrelationships among multifaceted dimensions of group interactions.
- You can generalize your results to real life situations.
- Observational research is useful for discovering what variables may be important before applying other methods like experiments.
- Observation research designs account for the complexity of group behaviors.
- Reliability of data is low because seeing behaviors occur over and over again may be a time consuming task and are difficult to replicate.
- In observational research, findings may only reflect a unique sample population and, thus, cannot be generalized to other groups.
- There can be problems with bias as the researcher may only "see what they want to see."
- There is no possibility to determine "cause and effect" relationships since nothing is manipulated.
- Sources or subjects may not all be equally credible.
- Any group that is knowingly studied is altered to some degree by the presence of the researcher, therefore, potentially skewing any data collected.
Atkinson, Paul and Martyn Hammersley. “Ethnography and Participant Observation.” In Handbook of Qualitative Research . Norman K. Denzin and Yvonna S. Lincoln, eds. (Thousand Oaks, CA: Sage, 1994), pp. 248-261; Observational Research. Research Methods by Dummies. Department of Psychology. California State University, Fresno, 2006; Patton Michael Quinn. Qualitiative Research and Evaluation Methods . Chapter 6, Fieldwork Strategies and Observational Methods. 3rd ed. Thousand Oaks, CA: Sage, 2002; Payne, Geoff and Judy Payne. "Observation." In Key Concepts in Social Research . The SAGE Key Concepts series. (London, England: Sage, 2004), pp. 158-162; Rosenbaum, Paul R. Design of Observational Studies . New York: Springer, 2010;Williams, J. Patrick. "Nonparticipant Observation." In The Sage Encyclopedia of Qualitative Research Methods . Lisa M. Given, editor.(Thousand Oaks, CA: Sage, 2008), pp. 562-563.
Philosophical Design
Understood more as an broad approach to examining a research problem than a methodological design, philosophical analysis and argumentation is intended to challenge deeply embedded, often intractable, assumptions underpinning an area of study. This approach uses the tools of argumentation derived from philosophical traditions, concepts, models, and theories to critically explore and challenge, for example, the relevance of logic and evidence in academic debates, to analyze arguments about fundamental issues, or to discuss the root of existing discourse about a research problem. These overarching tools of analysis can be framed in three ways:
- Ontology -- the study that describes the nature of reality; for example, what is real and what is not, what is fundamental and what is derivative?
- Epistemology -- the study that explores the nature of knowledge; for example, by what means does knowledge and understanding depend upon and how can we be certain of what we know?
- Axiology -- the study of values; for example, what values does an individual or group hold and why? How are values related to interest, desire, will, experience, and means-to-end? And, what is the difference between a matter of fact and a matter of value?
- Can provide a basis for applying ethical decision-making to practice.
- Functions as a means of gaining greater self-understanding and self-knowledge about the purposes of research.
- Brings clarity to general guiding practices and principles of an individual or group.
- Philosophy informs methodology.
- Refine concepts and theories that are invoked in relatively unreflective modes of thought and discourse.
- Beyond methodology, philosophy also informs critical thinking about epistemology and the structure of reality (metaphysics).
- Offers clarity and definition to the practical and theoretical uses of terms, concepts, and ideas.
- Limited application to specific research problems [answering the "So What?" question in social science research].
- Analysis can be abstract, argumentative, and limited in its practical application to real-life issues.
- While a philosophical analysis may render problematic that which was once simple or taken-for-granted, the writing can be dense and subject to unnecessary jargon, overstatement, and/or excessive quotation and documentation.
- There are limitations in the use of metaphor as a vehicle of philosophical analysis.
- There can be analytical difficulties in moving from philosophy to advocacy and between abstract thought and application to the phenomenal world.
Burton, Dawn. "Part I, Philosophy of the Social Sciences." In Research Training for Social Scientists . (London, England: Sage, 2000), pp. 1-5; Chapter 4, Research Methodology and Design. Unisa Institutional Repository (UnisaIR), University of South Africa; Jarvie, Ian C., and Jesús Zamora-Bonilla, editors. The SAGE Handbook of the Philosophy of Social Sciences . London: Sage, 2011; Labaree, Robert V. and Ross Scimeca. “The Philosophical Problem of Truth in Librarianship.” The Library Quarterly 78 (January 2008): 43-70; Maykut, Pamela S. Beginning Qualitative Research: A Philosophic and Practical Guide . Washington, DC: Falmer Press, 1994; McLaughlin, Hugh. "The Philosophy of Social Research." In Understanding Social Work Research . 2nd edition. (London: SAGE Publications Ltd., 2012), pp. 24-47; Stanford Encyclopedia of Philosophy . Metaphysics Research Lab, CSLI, Stanford University, 2013.
Sequential Design
- The researcher has a limitless option when it comes to sample size and the sampling schedule.
- Due to the repetitive nature of this research design, minor changes and adjustments can be done during the initial parts of the study to correct and hone the research method.
- This is a useful design for exploratory studies.
- There is very little effort on the part of the researcher when performing this technique. It is generally not expensive, time consuming, or workforce intensive.
- Because the study is conducted serially, the results of one sample are known before the next sample is taken and analyzed. This provides opportunities for continuous improvement of sampling and methods of analysis.
- The sampling method is not representative of the entire population. The only possibility of approaching representativeness is when the researcher chooses to use a very large sample size significant enough to represent a significant portion of the entire population. In this case, moving on to study a second or more specific sample can be difficult.
- The design cannot be used to create conclusions and interpretations that pertain to an entire population because the sampling technique is not randomized. Generalizability from findings is, therefore, limited.
- Difficult to account for and interpret variation from one sample to another over time, particularly when using qualitative methods of data collection.
Betensky, Rebecca. Harvard University, Course Lecture Note slides; Bovaird, James A. and Kevin A. Kupzyk. "Sequential Design." In Encyclopedia of Research Design . Neil J. Salkind, editor. (Thousand Oaks, CA: Sage, 2010), pp. 1347-1352; Cresswell, John W. Et al. “Advanced Mixed-Methods Research Designs.” In Handbook of Mixed Methods in Social and Behavioral Research . Abbas Tashakkori and Charles Teddle, eds. (Thousand Oaks, CA: Sage, 2003), pp. 209-240; Henry, Gary T. "Sequential Sampling." In The SAGE Encyclopedia of Social Science Research Methods . Michael S. Lewis-Beck, Alan Bryman and Tim Futing Liao, editors. (Thousand Oaks, CA: Sage, 2004), pp. 1027-1028; Nataliya V. Ivankova. “Using Mixed-Methods Sequential Explanatory Design: From Theory to Practice.” Field Methods 18 (February 2006): 3-20; Bovaird, James A. and Kevin A. Kupzyk. “Sequential Design.” In Encyclopedia of Research Design . Neil J. Salkind, ed. Thousand Oaks, CA: Sage, 2010; Sequential Analysis. Wikipedia.
Systematic Review
- A systematic review synthesizes the findings of multiple studies related to each other by incorporating strategies of analysis and interpretation intended to reduce biases and random errors.
- The application of critical exploration, evaluation, and synthesis methods separates insignificant, unsound, or redundant research from the most salient and relevant studies worthy of reflection.
- They can be use to identify, justify, and refine hypotheses, recognize and avoid hidden problems in prior studies, and explain data inconsistencies and conflicts in data.
- Systematic reviews can be used to help policy makers formulate evidence-based guidelines and regulations.
- The use of strict, explicit, and pre-determined methods of synthesis, when applied appropriately, provide reliable estimates about the effects of interventions, evaluations, and effects related to the overarching research problem investigated by each study under review.
- Systematic reviews illuminate where knowledge or thorough understanding of a research problem is lacking and, therefore, can then be used to guide future research.
- The accepted inclusion of unpublished studies [i.e., grey literature] ensures the broadest possible way to analyze and interpret research on a topic.
- Results of the synthesis can be generalized and the findings extrapolated into the general population with more validity than most other types of studies .
- Systematic reviews do not create new knowledge per se; they are a method for synthesizing existing studies about a research problem in order to gain new insights and determine gaps in the literature.
- The way researchers have carried out their investigations [e.g., the period of time covered, number of participants, sources of data analyzed, etc.] can make it difficult to effectively synthesize studies.
- The inclusion of unpublished studies can introduce bias into the review because they may not have undergone a rigorous peer-review process prior to publication. Examples may include conference presentations or proceedings, publications from government agencies, white papers, working papers, and internal documents from organizations, and doctoral dissertations and Master's theses.
Denyer, David and David Tranfield. "Producing a Systematic Review." In The Sage Handbook of Organizational Research Methods . David A. Buchanan and Alan Bryman, editors. ( Thousand Oaks, CA: Sage Publications, 2009), pp. 671-689; Foster, Margaret J. and Sarah T. Jewell, editors. Assembling the Pieces of a Systematic Review: A Guide for Librarians . Lanham, MD: Rowman and Littlefield, 2017; Gough, David, Sandy Oliver, James Thomas, editors. Introduction to Systematic Reviews . 2nd edition. Los Angeles, CA: Sage Publications, 2017; Gopalakrishnan, S. and P. Ganeshkumar. “Systematic Reviews and Meta-analysis: Understanding the Best Evidence in Primary Healthcare.” Journal of Family Medicine and Primary Care 2 (2013): 9-14; Gough, David, James Thomas, and Sandy Oliver. "Clarifying Differences between Review Designs and Methods." Systematic Reviews 1 (2012): 1-9; Khan, Khalid S., Regina Kunz, Jos Kleijnen, and Gerd Antes. “Five Steps to Conducting a Systematic Review.” Journal of the Royal Society of Medicine 96 (2003): 118-121; Mulrow, C. D. “Systematic Reviews: Rationale for Systematic Reviews.” BMJ 309:597 (September 1994); O'Dwyer, Linda C., and Q. Eileen Wafford. "Addressing Challenges with Systematic Review Teams through Effective Communication: A Case Report." Journal of the Medical Library Association 109 (October 2021): 643-647; Okoli, Chitu, and Kira Schabram. "A Guide to Conducting a Systematic Literature Review of Information Systems Research." Sprouts: Working Papers on Information Systems 10 (2010); Siddaway, Andy P., Alex M. Wood, and Larry V. Hedges. "How to Do a Systematic Review: A Best Practice Guide for Conducting and Reporting Narrative Reviews, Meta-analyses, and Meta-syntheses." Annual Review of Psychology 70 (2019): 747-770; Torgerson, Carole J. “Publication Bias: The Achilles’ Heel of Systematic Reviews?” British Journal of Educational Studies 54 (March 2006): 89-102; Torgerson, Carole. Systematic Reviews . New York: Continuum, 2003.
- << Previous: Purpose of Guide
- Next: Design Flaws to Avoid >>
- Last Updated: Apr 24, 2024 10:51 AM
- URL: https://libguides.usc.edu/writingguide

What Are Variables in a Research Paper?
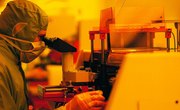
What Components Are Necessary for an Experiment to Be Valid?
Research papers will mention a variety of different variables, and, at first, these technical terms might seem difficult and confusing. But with a little practice, identifying these variables becomes second nature. Because they are sometimes not explicitly labeled in the research writeup, it is useful to have a real research paper on hand as you learn these terms, so you can get some hands-on practice at identifying them.
Independent Variable
The independent variable, also known as the IV, is the variable that the researchers are manipulating in an experiment or quasi-experiment. It is also the label given to the “criterion” variable in certain types of regression analysis. For example, if a researcher has two groups of people watch either a happy film or a sad film before giving an IQ test, the IV is the mood of the participants.
Dependent Variable
The dependent variable, or DV, is the one that is being measured by the researcher; it is the outcome variable. There is often confusion between the IV and the DV among new science students, but a good way to distinguish them is to remember that the outcome of measuring the DV is hypothesized to depend on the manipulation of the IV. In the above example, IQ was hypothesized to depend on the mood of the participants.
A covariate is a variable that the researchers include in an analysis to determine whether the IV is able to influence the DV over and above any effect the covariate might have. The classic example is when researchers take a baseline measurement, perform some manipulation, then take the measurement again. When they analyze this data, they will enter the baseline scores as a covariate, which will help cancel out any initial differences between the participants.
Extraneous Variables
An extraneous variable is a little different from the rest because it is not directly measured, or often even wanted, by the researchers. It is a variable that has an impact on the results of the experiment that the researchers didn't anticipate. For example, the heat of the room might be different between two groups of IQ tests, and the hot room might annoy people and affect their scores. Scientists try to control these variables, which means keeping them constant between groups.
Related Articles
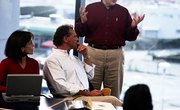
What Is Experimental Research Design?
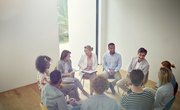
Why Do Experimental Procedures Include Control Samples?
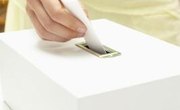
Independent Variables in Survey Methods
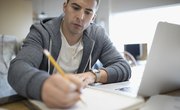
The Disadvantages of Logistic Regression
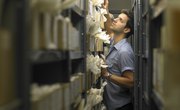
The Real Difference Between Reliability and Validity
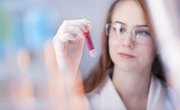
Qualitative and Quantitative Research Methods
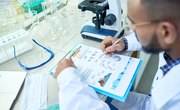
How to Evaluate Statistical Analysis
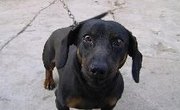
Ideas for Science Projects on Dog Behavior
- Laerd Statistics: Types of Variable
Warren Davies has been writing since 2007, focusing on bespoke projects for online clients such as PsyT and The Institute of Coaching. This has been alongside work in research, web design and blogging. A Linux user and gamer, warren trains in martial arts as a hobby. He has a Bachelor of Science and Master of Science in psychology, and further qualifications in statistics and business studies.
Click through the PLOS taxonomy to find articles in your field.
For more information about PLOS Subject Areas, click here .
Loading metrics
Open Access
Peer-reviewed
Research Article
Anxiety, Affect, Self-Esteem, and Stress: Mediation and Moderation Effects on Depression
Affiliations Department of Psychology, University of Gothenburg, Gothenburg, Sweden, Network for Empowerment and Well-Being, University of Gothenburg, Gothenburg, Sweden
Affiliation Network for Empowerment and Well-Being, University of Gothenburg, Gothenburg, Sweden
Affiliations Department of Psychology, University of Gothenburg, Gothenburg, Sweden, Network for Empowerment and Well-Being, University of Gothenburg, Gothenburg, Sweden, Department of Psychology, Education and Sport Science, Linneaus University, Kalmar, Sweden
* E-mail: [email protected]
Affiliations Network for Empowerment and Well-Being, University of Gothenburg, Gothenburg, Sweden, Center for Ethics, Law, and Mental Health (CELAM), University of Gothenburg, Gothenburg, Sweden, Institute of Neuroscience and Physiology, The Sahlgrenska Academy, University of Gothenburg, Gothenburg, Sweden
- Ali Al Nima,
- Patricia Rosenberg,
- Trevor Archer,
- Danilo Garcia
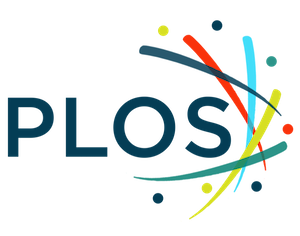
- Published: September 9, 2013
- https://doi.org/10.1371/journal.pone.0073265
- Reader Comments
23 Sep 2013: Nima AA, Rosenberg P, Archer T, Garcia D (2013) Correction: Anxiety, Affect, Self-Esteem, and Stress: Mediation and Moderation Effects on Depression. PLOS ONE 8(9): 10.1371/annotation/49e2c5c8-e8a8-4011-80fc-02c6724b2acc. https://doi.org/10.1371/annotation/49e2c5c8-e8a8-4011-80fc-02c6724b2acc View correction
Mediation analysis investigates whether a variable (i.e., mediator) changes in regard to an independent variable, in turn, affecting a dependent variable. Moderation analysis, on the other hand, investigates whether the statistical interaction between independent variables predict a dependent variable. Although this difference between these two types of analysis is explicit in current literature, there is still confusion with regard to the mediating and moderating effects of different variables on depression. The purpose of this study was to assess the mediating and moderating effects of anxiety, stress, positive affect, and negative affect on depression.
Two hundred and two university students (males = 93, females = 113) completed questionnaires assessing anxiety, stress, self-esteem, positive and negative affect, and depression. Mediation and moderation analyses were conducted using techniques based on standard multiple regression and hierarchical regression analyses.
Main Findings
The results indicated that (i) anxiety partially mediated the effects of both stress and self-esteem upon depression, (ii) that stress partially mediated the effects of anxiety and positive affect upon depression, (iii) that stress completely mediated the effects of self-esteem on depression, and (iv) that there was a significant interaction between stress and negative affect, and between positive affect and negative affect upon depression.
The study highlights different research questions that can be investigated depending on whether researchers decide to use the same variables as mediators and/or moderators.
Citation: Nima AA, Rosenberg P, Archer T, Garcia D (2013) Anxiety, Affect, Self-Esteem, and Stress: Mediation and Moderation Effects on Depression. PLoS ONE 8(9): e73265. https://doi.org/10.1371/journal.pone.0073265
Editor: Ben J. Harrison, The University of Melbourne, Australia
Received: February 21, 2013; Accepted: July 22, 2013; Published: September 9, 2013
Copyright: © 2013 Nima et al. This is an open-access article distributed under the terms of the Creative Commons Attribution License, which permits unrestricted use, distribution, and reproduction in any medium, provided the original author and source are credited.
Funding: The authors have no support or funding to report.
Competing interests: The authors have declared that no competing interests exist.
Introduction
Mediation refers to the covariance relationships among three variables: an independent variable (1), an assumed mediating variable (2), and a dependent variable (3). Mediation analysis investigates whether the mediating variable accounts for a significant amount of the shared variance between the independent and the dependent variables–the mediator changes in regard to the independent variable, in turn, affecting the dependent one [1] , [2] . On the other hand, moderation refers to the examination of the statistical interaction between independent variables in predicting a dependent variable [1] , [3] . In contrast to the mediator, the moderator is not expected to be correlated with both the independent and the dependent variable–Baron and Kenny [1] actually recommend that it is best if the moderator is not correlated with the independent variable and if the moderator is relatively stable, like a demographic variable (e.g., gender, socio-economic status) or a personality trait (e.g., affectivity).
Although both types of analysis lead to different conclusions [3] and the distinction between statistical procedures is part of the current literature [2] , there is still confusion about the use of moderation and mediation analyses using data pertaining to the prediction of depression. There are, for example, contradictions among studies that investigate mediating and moderating effects of anxiety, stress, self-esteem, and affect on depression. Depression, anxiety and stress are suggested to influence individuals' social relations and activities, work, and studies, as well as compromising decision-making and coping strategies [4] , [5] , [6] . Successfully coping with anxiety, depressiveness, and stressful situations may contribute to high levels of self-esteem and self-confidence, in addition increasing well-being, and psychological and physical health [6] . Thus, it is important to disentangle how these variables are related to each other. However, while some researchers perform mediation analysis with some of the variables mentioned here, other researchers conduct moderation analysis with the same variables. Seldom are both moderation and mediation performed on the same dataset. Before disentangling mediation and moderation effects on depression in the current literature, we briefly present the methodology behind the analysis performed in this study.
Mediation and moderation
Baron and Kenny [1] postulated several criteria for the analysis of a mediating effect: a significant correlation between the independent and the dependent variable, the independent variable must be significantly associated with the mediator, the mediator predicts the dependent variable even when the independent variable is controlled for, and the correlation between the independent and the dependent variable must be eliminated or reduced when the mediator is controlled for. All the criteria is then tested using the Sobel test which shows whether indirect effects are significant or not [1] , [7] . A complete mediating effect occurs when the correlation between the independent and the dependent variable are eliminated when the mediator is controlled for [8] . Analyses of mediation can, for example, help researchers to move beyond answering if high levels of stress lead to high levels of depression. With mediation analysis researchers might instead answer how stress is related to depression.
In contrast to mediation, moderation investigates the unique conditions under which two variables are related [3] . The third variable here, the moderator, is not an intermediate variable in the causal sequence from the independent to the dependent variable. For the analysis of moderation effects, the relation between the independent and dependent variable must be different at different levels of the moderator [3] . Moderators are included in the statistical analysis as an interaction term [1] . When analyzing moderating effects the variables should first be centered (i.e., calculating the mean to become 0 and the standard deviation to become 1) in order to avoid problems with multi-colinearity [8] . Moderating effects can be calculated using multiple hierarchical linear regressions whereby main effects are presented in the first step and interactions in the second step [1] . Analysis of moderation, for example, helps researchers to answer when or under which conditions stress is related to depression.
Mediation and moderation effects on depression
Cognitive vulnerability models suggest that maladaptive self-schema mirroring helplessness and low self-esteem explain the development and maintenance of depression (for a review see [9] ). These cognitive vulnerability factors become activated by negative life events or negative moods [10] and are suggested to interact with environmental stressors to increase risk for depression and other emotional disorders [11] , [10] . In this line of thinking, the experience of stress, low self-esteem, and negative emotions can cause depression, but also be used to explain how (i.e., mediation) and under which conditions (i.e., moderation) specific variables influence depression.
Using mediational analyses to investigate how cognitive therapy intervations reduced depression, researchers have showed that the intervention reduced anxiety, which in turn was responsible for 91% of the reduction in depression [12] . In the same study, reductions in depression, by the intervention, accounted only for 6% of the reduction in anxiety. Thus, anxiety seems to affect depression more than depression affects anxiety and, together with stress, is both a cause of and a powerful mediator influencing depression (See also [13] ). Indeed, there are positive relationships between depression, anxiety and stress in different cultures [14] . Moreover, while some studies show that stress (independent variable) increases anxiety (mediator), which in turn increased depression (dependent variable) [14] , other studies show that stress (moderator) interacts with maladaptive self-schemata (dependent variable) to increase depression (independent variable) [15] , [16] .
The present study
In order to illustrate how mediation and moderation can be used to address different research questions we first focus our attention to anxiety and stress as mediators of different variables that earlier have been shown to be related to depression. Secondly, we use all variables to find which of these variables moderate the effects on depression.
The specific aims of the present study were:
- To investigate if anxiety mediated the effect of stress, self-esteem, and affect on depression.
- To investigate if stress mediated the effects of anxiety, self-esteem, and affect on depression.
- To examine moderation effects between anxiety, stress, self-esteem, and affect on depression.
Ethics statement
This research protocol was approved by the Ethics Committee of the University of Gothenburg and written informed consent was obtained from all the study participants.
Participants
The present study was based upon a sample of 206 participants (males = 93, females = 113). All the participants were first year students in different disciplines at two universities in South Sweden. The mean age for the male students was 25.93 years ( SD = 6.66), and 25.30 years ( SD = 5.83) for the female students.
In total, 206 questionnaires were distributed to the students. Together 202 questionnaires were responded to leaving a total dropout of 1.94%. This dropout concerned three sections that the participants chose not to respond to at all, and one section that was completed incorrectly. None of these four questionnaires was included in the analyses.
Instruments
Hospital anxiety and depression scale [17] ..
The Swedish translation of this instrument [18] was used to measure anxiety and depression. The instrument consists of 14 statements (7 of which measure depression and 7 measure anxiety) to which participants are asked to respond grade of agreement on a Likert scale (0 to 3). The utility, reliability and validity of the instrument has been shown in multiple studies (e.g., [19] ).
Perceived Stress Scale [20] .
The Swedish version [21] of this instrument was used to measures individuals' experience of stress. The instrument consist of 14 statements to which participants rate on a Likert scale (0 = never , 4 = very often ). High values indicate that the individual expresses a high degree of stress.
Rosenberg's Self-Esteem Scale [22] .
The Rosenberg's Self-Esteem Scale (Swedish version by Lindwall [23] ) consists of 10 statements focusing on general feelings toward the self. Participants are asked to report grade of agreement in a four-point Likert scale (1 = agree not at all, 4 = agree completely ). This is the most widely used instrument for estimation of self-esteem with high levels of reliability and validity (e.g., [24] , [25] ).
Positive Affect and Negative Affect Schedule [26] .
This is a widely applied instrument for measuring individuals' self-reported mood and feelings. The Swedish version has been used among participants of different ages and occupations (e.g., [27] , [28] , [29] ). The instrument consists of 20 adjectives, 10 positive affect (e.g., proud, strong) and 10 negative affect (e.g., afraid, irritable). The adjectives are rated on a five-point Likert scale (1 = not at all , 5 = very much ). The instrument is a reliable, valid, and effective self-report instrument for estimating these two important and independent aspects of mood [26] .
Questionnaires were distributed to the participants on several different locations within the university, including the library and lecture halls. Participants were asked to complete the questionnaire after being informed about the purpose and duration (10–15 minutes) of the study. Participants were also ensured complete anonymity and informed that they could end their participation whenever they liked.
Correlational analysis
Depression showed positive, significant relationships with anxiety, stress and negative affect. Table 1 presents the correlation coefficients, mean values and standard deviations ( sd ), as well as Cronbach ' s α for all the variables in the study.
- PPT PowerPoint slide
- PNG larger image
- TIFF original image
https://doi.org/10.1371/journal.pone.0073265.t001
Mediation analysis
Regression analyses were performed in order to investigate if anxiety mediated the effect of stress, self-esteem, and affect on depression (aim 1). The first regression showed that stress ( B = .03, 95% CI [.02,.05], β = .36, t = 4.32, p <.001), self-esteem ( B = −.03, 95% CI [−.05, −.01], β = −.24, t = −3.20, p <.001), and positive affect ( B = −.02, 95% CI [−.05, −.01], β = −.19, t = −2.93, p = .004) had each an unique effect on depression. Surprisingly, negative affect did not predict depression ( p = 0.77) and was therefore removed from the mediation model, thus not included in further analysis.
The second regression tested whether stress, self-esteem and positive affect uniquely predicted the mediator (i.e., anxiety). Stress was found to be positively associated ( B = .21, 95% CI [.15,.27], β = .47, t = 7.35, p <.001), whereas self-esteem was negatively associated ( B = −.29, 95% CI [−.38, −.21], β = −.42, t = −6.48, p <.001) to anxiety. Positive affect, however, was not associated to anxiety ( p = .50) and was therefore removed from further analysis.
A hierarchical regression analysis using depression as the outcome variable was performed using stress and self-esteem as predictors in the first step, and anxiety as predictor in the second step. This analysis allows the examination of whether stress and self-esteem predict depression and if this relation is weaken in the presence of anxiety as the mediator. The result indicated that, in the first step, both stress ( B = .04, 95% CI [.03,.05], β = .45, t = 6.43, p <.001) and self-esteem ( B = .04, 95% CI [.03,.05], β = .45, t = 6.43, p <.001) predicted depression. When anxiety (i.e., the mediator) was controlled for predictability was reduced somewhat but was still significant for stress ( B = .03, 95% CI [.02,.04], β = .33, t = 4.29, p <.001) and for self-esteem ( B = −.03, 95% CI [−.05, −.01], β = −.20, t = −2.62, p = .009). Anxiety, as a mediator, predicted depression even when both stress and self-esteem were controlled for ( B = .05, 95% CI [.02,.08], β = .26, t = 3.17, p = .002). Anxiety improved the prediction of depression over-and-above the independent variables (i.e., stress and self-esteem) (Δ R 2 = .03, F (1, 198) = 10.06, p = .002). See Table 2 for the details.
https://doi.org/10.1371/journal.pone.0073265.t002
A Sobel test was conducted to test the mediating criteria and to assess whether indirect effects were significant or not. The result showed that the complete pathway from stress (independent variable) to anxiety (mediator) to depression (dependent variable) was significant ( z = 2.89, p = .003). The complete pathway from self-esteem (independent variable) to anxiety (mediator) to depression (dependent variable) was also significant ( z = 2.82, p = .004). Thus, indicating that anxiety partially mediates the effects of both stress and self-esteem on depression. This result may indicate also that both stress and self-esteem contribute directly to explain the variation in depression and indirectly via experienced level of anxiety (see Figure 1 ).
Changes in Beta weights when the mediator is present are highlighted in red.
https://doi.org/10.1371/journal.pone.0073265.g001
For the second aim, regression analyses were performed in order to test if stress mediated the effect of anxiety, self-esteem, and affect on depression. The first regression showed that anxiety ( B = .07, 95% CI [.04,.10], β = .37, t = 4.57, p <.001), self-esteem ( B = −.02, 95% CI [−.05, −.01], β = −.18, t = −2.23, p = .03), and positive affect ( B = −.03, 95% CI [−.04, −.02], β = −.27, t = −4.35, p <.001) predicted depression independently of each other. Negative affect did not predict depression ( p = 0.74) and was therefore removed from further analysis.
The second regression investigated if anxiety, self-esteem and positive affect uniquely predicted the mediator (i.e., stress). Stress was positively associated to anxiety ( B = 1.01, 95% CI [.75, 1.30], β = .46, t = 7.35, p <.001), negatively associated to self-esteem ( B = −.30, 95% CI [−.50, −.01], β = −.19, t = −2.90, p = .004), and a negatively associated to positive affect ( B = −.33, 95% CI [−.46, −.20], β = −.27, t = −5.02, p <.001).
A hierarchical regression analysis using depression as the outcome and anxiety, self-esteem, and positive affect as the predictors in the first step, and stress as the predictor in the second step, allowed the examination of whether anxiety, self-esteem and positive affect predicted depression and if this association would weaken when stress (i.e., the mediator) was present. In the first step of the regression anxiety ( B = .07, 95% CI [.05,.10], β = .38, t = 5.31, p = .02), self-esteem ( B = −.03, 95% CI [−.05, −.01], β = −.18, t = −2.41, p = .02), and positive affect ( B = −.03, 95% CI [−.04, −.02], β = −.27, t = −4.36, p <.001) significantly explained depression. When stress (i.e., the mediator) was controlled for, predictability was reduced somewhat but was still significant for anxiety ( B = .05, 95% CI [.02,.08], β = .05, t = 4.29, p <.001) and for positive affect ( B = −.02, 95% CI [−.04, −.01], β = −.20, t = −3.16, p = .002), whereas self-esteem did not reach significance ( p < = .08). In the second step, the mediator (i.e., stress) predicted depression even when anxiety, self-esteem, and positive affect were controlled for ( B = .02, 95% CI [.08,.04], β = .25, t = 3.07, p = .002). Stress improved the prediction of depression over-and-above the independent variables (i.e., anxiety, self-esteem and positive affect) (Δ R 2 = .02, F (1, 197) = 9.40, p = .002). See Table 3 for the details.
https://doi.org/10.1371/journal.pone.0073265.t003
Furthermore, the Sobel test indicated that the complete pathways from the independent variables (anxiety: z = 2.81, p = .004; self-esteem: z = 2.05, p = .04; positive affect: z = 2.58, p <.01) to the mediator (i.e., stress), to the outcome (i.e., depression) were significant. These specific results might be explained on the basis that stress partially mediated the effects of both anxiety and positive affect on depression while stress completely mediated the effects of self-esteem on depression. In other words, anxiety and positive affect contributed directly to explain the variation in depression and indirectly via the experienced level of stress. Self-esteem contributed only indirectly via the experienced level of stress to explain the variation in depression. In other words, stress effects on depression originate from “its own power” and explained more of the variation in depression than self-esteem (see Figure 2 ).
https://doi.org/10.1371/journal.pone.0073265.g002
Moderation analysis
Multiple linear regression analyses were used in order to examine moderation effects between anxiety, stress, self-esteem and affect on depression. The analysis indicated that about 52% of the variation in the dependent variable (i.e., depression) could be explained by the main effects and the interaction effects ( R 2 = .55, adjusted R 2 = .51, F (55, 186) = 14.87, p <.001). When the variables (dependent and independent) were standardized, both the standardized regression coefficients beta (β) and the unstandardized regression coefficients beta (B) became the same value with regard to the main effects. Three of the main effects were significant and contributed uniquely to high levels of depression: anxiety ( B = .26, t = 3.12, p = .002), stress ( B = .25, t = 2.86, p = .005), and self-esteem ( B = −.17, t = −2.17, p = .03). The main effect of positive affect was also significant and contributed to low levels of depression ( B = −.16, t = −2.027, p = .02) (see Figure 3 ). Furthermore, the results indicated that two moderator effects were significant. These were the interaction between stress and negative affect ( B = −.28, β = −.39, t = −2.36, p = .02) (see Figure 4 ) and the interaction between positive affect and negative affect ( B = −.21, β = −.29, t = −2.30, p = .02) ( Figure 5 ).
https://doi.org/10.1371/journal.pone.0073265.g003
Low stress and low negative affect leads to lower levels of depression compared to high stress and high negative affect.
https://doi.org/10.1371/journal.pone.0073265.g004
High positive affect and low negative affect lead to lower levels of depression compared to low positive affect and high negative affect.
https://doi.org/10.1371/journal.pone.0073265.g005
The results in the present study show that (i) anxiety partially mediated the effects of both stress and self-esteem on depression, (ii) that stress partially mediated the effects of anxiety and positive affect on depression, (iii) that stress completely mediated the effects of self-esteem on depression, and (iv) that there was a significant interaction between stress and negative affect, and positive affect and negative affect on depression.
Mediating effects
The study suggests that anxiety contributes directly to explaining the variance in depression while stress and self-esteem might contribute directly to explaining the variance in depression and indirectly by increasing feelings of anxiety. Indeed, individuals who experience stress over a long period of time are susceptible to increased anxiety and depression [30] , [31] and previous research shows that high self-esteem seems to buffer against anxiety and depression [32] , [33] . The study also showed that stress partially mediated the effects of both anxiety and positive affect on depression and that stress completely mediated the effects of self-esteem on depression. Anxiety and positive affect contributed directly to explain the variation in depression and indirectly to the experienced level of stress. Self-esteem contributed only indirectly via the experienced level of stress to explain the variation in depression, i.e. stress affects depression on the basis of ‘its own power’ and explains much more of the variation in depressive experiences than self-esteem. In general, individuals who experience low anxiety and frequently experience positive affect seem to experience low stress, which might reduce their levels of depression. Academic stress, for instance, may increase the risk for experiencing depression among students [34] . Although self-esteem did not emerged as an important variable here, under circumstances in which difficulties in life become chronic, some researchers suggest that low self-esteem facilitates the experience of stress [35] .
Moderator effects/interaction effects
The present study showed that the interaction between stress and negative affect and between positive and negative affect influenced self-reported depression symptoms. Moderation effects between stress and negative affect imply that the students experiencing low levels of stress and low negative affect reported lower levels of depression than those who experience high levels of stress and high negative affect. This result confirms earlier findings that underline the strong positive association between negative affect and both stress and depression [36] , [37] . Nevertheless, negative affect by itself did not predicted depression. In this regard, it is important to point out that the absence of positive emotions is a better predictor of morbidity than the presence of negative emotions [38] , [39] . A modification to this statement, as illustrated by the results discussed next, could be that the presence of negative emotions in conjunction with the absence of positive emotions increases morbidity.
The moderating effects between positive and negative affect on the experience of depression imply that the students experiencing high levels of positive affect and low levels of negative affect reported lower levels of depression than those who experience low levels of positive affect and high levels of negative affect. This result fits previous observations indicating that different combinations of these affect dimensions are related to different measures of physical and mental health and well-being, such as, blood pressure, depression, quality of sleep, anxiety, life satisfaction, psychological well-being, and self-regulation [40] – [51] .
Limitations
The result indicated a relatively low mean value for depression ( M = 3.69), perhaps because the studied population was university students. These might limit the generalization power of the results and might also explain why negative affect, commonly associated to depression, was not related to depression in the present study. Moreover, there is a potential influence of single source/single method variance on the findings, especially given the high correlation between all the variables under examination.
Conclusions
The present study highlights different results that could be arrived depending on whether researchers decide to use variables as mediators or moderators. For example, when using meditational analyses, anxiety and stress seem to be important factors that explain how the different variables used here influence depression–increases in anxiety and stress by any other factor seem to lead to increases in depression. In contrast, when moderation analyses were used, the interaction of stress and affect predicted depression and the interaction of both affectivity dimensions (i.e., positive and negative affect) also predicted depression–stress might increase depression under the condition that the individual is high in negative affectivity, in turn, negative affectivity might increase depression under the condition that the individual experiences low positive affectivity.
Acknowledgments
The authors would like to thank the reviewers for their openness and suggestions, which significantly improved the article.
Author Contributions
Conceived and designed the experiments: AAN TA. Performed the experiments: AAN. Analyzed the data: AAN DG. Contributed reagents/materials/analysis tools: AAN TA DG. Wrote the paper: AAN PR TA DG.
- View Article
- Google Scholar
- 3. MacKinnon DP, Luecken LJ (2008) How and for Whom? Mediation and Moderation in Health Psychology. Health Psychol 27 (2 Suppl.): s99–s102.
- 4. Aaroe R (2006) Vinn över din depression [Defeat depression]. Stockholm: Liber.
- 5. Agerberg M (1998) Ut ur mörkret [Out from the Darkness]. Stockholm: Nordstedt.
- 6. Gilbert P (2005) Hantera din depression [Cope with your Depression]. Stockholm: Bokförlaget Prisma.
- 8. Tabachnick BG, Fidell LS (2007) Using Multivariate Statistics, Fifth Edition. Boston: Pearson Education, Inc.
- 10. Beck AT (1967) Depression: Causes and treatment. Philadelphia: University of Pennsylvania Press.
- 21. Eskin M, Parr D (1996) Introducing a Swedish version of an instrument measuring mental stress. Stockholm: Psykologiska institutionen Stockholms Universitet.
- 22. Rosenberg M (1965) Society and the Adolescent Self-Image. Princeton, NJ: Princeton University Press.
- 23. Lindwall M (2011) Självkänsla – Bortom populärpsykologi & enkla sanningar [Self-Esteem – Beyond Popular Psychology and Simple Truths]. Lund:Studentlitteratur.
- 25. Blascovich J, Tomaka J (1991) Measures of self-esteem. In: Robinson JP, Shaver PR, Wrightsman LS (Red.) Measures of personality and social psychological attitudes San Diego: Academic Press. 161–194.
- 30. Eysenck M (Ed.) (2000) Psychology: an integrated approach. New York: Oxford University Press.
- 31. Lazarus RS, Folkman S (1984) Stress, Appraisal, and Coping. New York: Springer.
- 32. Johnson M (2003) Självkänsla och anpassning [Self-esteem and Adaptation]. Lund: Studentlitteratur.
- 33. Cullberg Weston M (2005) Ditt inre centrum – Om självkänsla, självbild och konturen av ditt själv [Your Inner Centre – About Self-esteem, Self-image and the Contours of Yourself]. Stockholm: Natur och Kultur.
- 34. Lindén M (1997) Studentens livssituation. Frihet, sårbarhet, kris och utveckling [Students' Life Situation. Freedom, Vulnerability, Crisis and Development]. Uppsala: Studenthälsan.
- 35. Williams S (1995) Press utan stress ger maximal prestation [Pressure without Stress gives Maximal Performance]. Malmö: Richters förlag.
- 37. Garcia D, Kerekes N, Andersson-Arntén A–C, Archer T (2012) Temperament, Character, and Adolescents' Depressive Symptoms: Focusing on Affect. Depress Res Treat. DOI:10.1155/2012/925372.
- 40. Garcia D, Ghiabi B, Moradi S, Siddiqui A, Archer T (2013) The Happy Personality: A Tale of Two Philosophies. In Morris EF, Jackson M-A editors. Psychology of Personality. New York: Nova Science Publishers. 41–59.
- 41. Schütz E, Nima AA, Sailer U, Andersson-Arntén A–C, Archer T, Garcia D (2013) The affective profiles in the USA: Happiness, depression, life satisfaction, and happiness-increasing strategies. In press.
- 43. Garcia D, Nima AA, Archer T (2013) Temperament and Character's Relationship to Subjective Well- Being in Salvadorian Adolescents and Young Adults. In press.
- 44. Garcia D (2013) La vie en Rose: High Levels of Well-Being and Events Inside and Outside Autobiographical Memory. J Happiness Stud. DOI: 10.1007/s10902-013-9443-x.
- 48. Adrianson L, Djumaludin A, Neila R, Archer T (2013) Cultural influences upon health, affect, self-esteem and impulsiveness: An Indonesian-Swedish comparison. Int J Res Stud Psychol. DOI: 10.5861/ijrsp.2013.228.
Regions & Countries
- Publications
- Our Methods
- Short Reads
- Tools & Resources
Read Our Research On:
How Pew Research Center will report on generations moving forward
Journalists, researchers and the public often look at society through the lens of generation, using terms like Millennial or Gen Z to describe groups of similarly aged people. This approach can help readers see themselves in the data and assess where we are and where we’re headed as a country.
Pew Research Center has been at the forefront of generational research over the years, telling the story of Millennials as they came of age politically and as they moved more firmly into adult life . In recent years, we’ve also been eager to learn about Gen Z as the leading edge of this generation moves into adulthood.
But generational research has become a crowded arena. The field has been flooded with content that’s often sold as research but is more like clickbait or marketing mythology. There’s also been a growing chorus of criticism about generational research and generational labels in particular.
Recently, as we were preparing to embark on a major research project related to Gen Z, we decided to take a step back and consider how we can study generations in a way that aligns with our values of accuracy, rigor and providing a foundation of facts that enriches the public dialogue.
A typical generation spans 15 to 18 years. As many critics of generational research point out, there is great diversity of thought, experience and behavior within generations.
We set out on a yearlong process of assessing the landscape of generational research. We spoke with experts from outside Pew Research Center, including those who have been publicly critical of our generational analysis, to get their take on the pros and cons of this type of work. We invested in methodological testing to determine whether we could compare findings from our earlier telephone surveys to the online ones we’re conducting now. And we experimented with higher-level statistical analyses that would allow us to isolate the effect of generation.
What emerged from this process was a set of clear guidelines that will help frame our approach going forward. Many of these are principles we’ve always adhered to , but others will require us to change the way we’ve been doing things in recent years.
Here’s a short overview of how we’ll approach generational research in the future:
We’ll only do generational analysis when we have historical data that allows us to compare generations at similar stages of life. When comparing generations, it’s crucial to control for age. In other words, researchers need to look at each generation or age cohort at a similar point in the life cycle. (“Age cohort” is a fancy way of referring to a group of people who were born around the same time.)
When doing this kind of research, the question isn’t whether young adults today are different from middle-aged or older adults today. The question is whether young adults today are different from young adults at some specific point in the past.
To answer this question, it’s necessary to have data that’s been collected over a considerable amount of time – think decades. Standard surveys don’t allow for this type of analysis. We can look at differences across age groups, but we can’t compare age groups over time.
Another complication is that the surveys we conducted 20 or 30 years ago aren’t usually comparable enough to the surveys we’re doing today. Our earlier surveys were done over the phone, and we’ve since transitioned to our nationally representative online survey panel , the American Trends Panel . Our internal testing showed that on many topics, respondents answer questions differently depending on the way they’re being interviewed. So we can’t use most of our surveys from the late 1980s and early 2000s to compare Gen Z with Millennials and Gen Xers at a similar stage of life.
This means that most generational analysis we do will use datasets that have employed similar methodologies over a long period of time, such as surveys from the U.S. Census Bureau. A good example is our 2020 report on Millennial families , which used census data going back to the late 1960s. The report showed that Millennials are marrying and forming families at a much different pace than the generations that came before them.
Even when we have historical data, we will attempt to control for other factors beyond age in making generational comparisons. If we accept that there are real differences across generations, we’re basically saying that people who were born around the same time share certain attitudes or beliefs – and that their views have been influenced by external forces that uniquely shaped them during their formative years. Those forces may have been social changes, economic circumstances, technological advances or political movements.
When we see that younger adults have different views than their older counterparts, it may be driven by their demographic traits rather than the fact that they belong to a particular generation.
The tricky part is isolating those forces from events or circumstances that have affected all age groups, not just one generation. These are often called “period effects.” An example of a period effect is the Watergate scandal, which drove down trust in government among all age groups. Differences in trust across age groups in the wake of Watergate shouldn’t be attributed to the outsize impact that event had on one age group or another, because the change occurred across the board.
Changing demographics also may play a role in patterns that might at first seem like generational differences. We know that the United States has become more racially and ethnically diverse in recent decades, and that race and ethnicity are linked with certain key social and political views. When we see that younger adults have different views than their older counterparts, it may be driven by their demographic traits rather than the fact that they belong to a particular generation.
Controlling for these factors can involve complicated statistical analysis that helps determine whether the differences we see across age groups are indeed due to generation or not. This additional step adds rigor to the process. Unfortunately, it’s often absent from current discussions about Gen Z, Millennials and other generations.
When we can’t do generational analysis, we still see value in looking at differences by age and will do so where it makes sense. Age is one of the most common predictors of differences in attitudes and behaviors. And even if age gaps aren’t rooted in generational differences, they can still be illuminating. They help us understand how people across the age spectrum are responding to key trends, technological breakthroughs and historical events.
Each stage of life comes with a unique set of experiences. Young adults are often at the leading edge of changing attitudes on emerging social trends. Take views on same-sex marriage , for example, or attitudes about gender identity .
Many middle-aged adults, in turn, face the challenge of raising children while also providing care and support to their aging parents. And older adults have their own obstacles and opportunities. All of these stories – rooted in the life cycle, not in generations – are important and compelling, and we can tell them by analyzing our surveys at any given point in time.
When we do have the data to study groups of similarly aged people over time, we won’t always default to using the standard generational definitions and labels. While generational labels are simple and catchy, there are other ways to analyze age cohorts. For example, some observers have suggested grouping people by the decade in which they were born. This would create narrower cohorts in which the members may share more in common. People could also be grouped relative to their age during key historical events (such as the Great Recession or the COVID-19 pandemic) or technological innovations (like the invention of the iPhone).
By choosing not to use the standard generational labels when they’re not appropriate, we can avoid reinforcing harmful stereotypes or oversimplifying people’s complex lived experiences.
Existing generational definitions also may be too broad and arbitrary to capture differences that exist among narrower cohorts. A typical generation spans 15 to 18 years. As many critics of generational research point out, there is great diversity of thought, experience and behavior within generations. The key is to pick a lens that’s most appropriate for the research question that’s being studied. If we’re looking at political views and how they’ve shifted over time, for example, we might group people together according to the first presidential election in which they were eligible to vote.
With these considerations in mind, our audiences should not expect to see a lot of new research coming out of Pew Research Center that uses the generational lens. We’ll only talk about generations when it adds value, advances important national debates and highlights meaningful societal trends.
- Age & Generations
- Demographic Research
- Generation X
- Generation Z
- Generations
- Greatest Generation
- Methodological Research
- Millennials
- Silent Generation
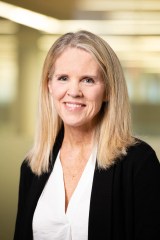
Kim Parker is director of social trends research at Pew Research Center
How Teens and Parents Approach Screen Time
Who are you the art and science of measuring identity, u.s. centenarian population is projected to quadruple over the next 30 years, older workers are growing in number and earning higher wages, teens, social media and technology 2023, most popular.
1615 L St. NW, Suite 800 Washington, DC 20036 USA (+1) 202-419-4300 | Main (+1) 202-857-8562 | Fax (+1) 202-419-4372 | Media Inquiries
Research Topics
- Coronavirus (COVID-19)
- Economy & Work
- Family & Relationships
- Gender & LGBTQ
- Immigration & Migration
- International Affairs
- Internet & Technology
- News Habits & Media
- Non-U.S. Governments
- Other Topics
- Politics & Policy
- Race & Ethnicity
- Email Newsletters
ABOUT PEW RESEARCH CENTER Pew Research Center is a nonpartisan fact tank that informs the public about the issues, attitudes and trends shaping the world. It conducts public opinion polling, demographic research, media content analysis and other empirical social science research. Pew Research Center does not take policy positions. It is a subsidiary of The Pew Charitable Trusts .
Copyright 2024 Pew Research Center
Terms & Conditions
Privacy Policy
Cookie Settings
Reprints, Permissions & Use Policy
Suggestions or feedback?
MIT News | Massachusetts Institute of Technology
- Machine learning
- Social justice
- Black holes
- Classes and programs
Departments
- Aeronautics and Astronautics
- Brain and Cognitive Sciences
- Architecture
- Political Science
- Mechanical Engineering
Centers, Labs, & Programs
- Abdul Latif Jameel Poverty Action Lab (J-PAL)
- Picower Institute for Learning and Memory
- Lincoln Laboratory
- School of Architecture + Planning
- School of Engineering
- School of Humanities, Arts, and Social Sciences
- Sloan School of Management
- School of Science
- MIT Schwarzman College of Computing
Researchers detect a new molecule in space
Press contact :.
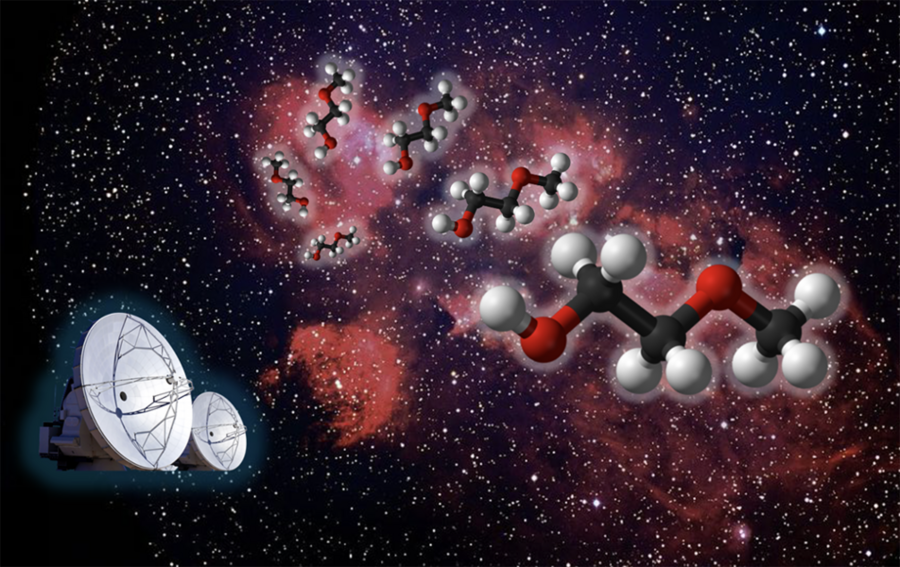
Previous image Next image
New research from the group of MIT Professor Brett McGuire has revealed the presence of a previously unknown molecule in space. The team's open-access paper, “ Rotational Spectrum and First Interstellar Detection of 2-Methoxyethanol Using ALMA Observations of NGC 6334I ,” appears in April 12 issue of The Astrophysical Journal Letters .
Zachary T.P. Fried , a graduate student in the McGuire group and the lead author of the publication, worked to assemble a puzzle comprised of pieces collected from across the globe, extending beyond MIT to France, Florida, Virginia, and Copenhagen, to achieve this exciting discovery.
“Our group tries to understand what molecules are present in regions of space where stars and solar systems will eventually take shape,” explains Fried. “This allows us to piece together how chemistry evolves alongside the process of star and planet formation. We do this by looking at the rotational spectra of molecules, the unique patterns of light they give off as they tumble end-over-end in space. These patterns are fingerprints (barcodes) for molecules. To detect new molecules in space, we first must have an idea of what molecule we want to look for, then we can record its spectrum in the lab here on Earth, and then finally we look for that spectrum in space using telescopes.”
Searching for molecules in space
The McGuire Group has recently begun to utilize machine learning to suggest good target molecules to search for. In 2023, one of these machine learning models suggested the researchers target a molecule known as 2-methoxyethanol.
“There are a number of 'methoxy' molecules in space, like dimethyl ether, methoxymethanol, ethyl methyl ether, and methyl formate, but 2-methoxyethanol would be the largest and most complex ever seen,” says Fried. To detect this molecule using radiotelescope observations, the group first needed to measure and analyze its rotational spectrum on Earth. The researchers combined experiments from the University of Lille (Lille, France), the New College of Florida (Sarasota, Florida), and the McGuire lab at MIT to measure this spectrum over a broadband region of frequencies ranging from the microwave to sub-millimeter wave regimes (approximately 8 to 500 gigahertz).
The data gleaned from these measurements permitted a search for the molecule using Atacama Large Millimeter/submillimeter Array (ALMA) observations toward two separate star-forming regions: NGC 6334I and IRAS 16293-2422B. Members of the McGuire group analyzed these telescope observations alongside researchers at the National Radio Astronomy Observatory (Charlottesville, Virginia) and the University of Copenhagen, Denmark.
“Ultimately, we observed 25 rotational lines of 2-methoxyethanol that lined up with the molecular signal observed toward NGC 6334I (the barcode matched!), thus resulting in a secure detection of 2-methoxyethanol in this source,” says Fried. “This allowed us to then derive physical parameters of the molecule toward NGC 6334I, such as its abundance and excitation temperature. It also enabled an investigation of the possible chemical formation pathways from known interstellar precursors.”
Looking forward
Molecular discoveries like this one help the researchers to better understand the development of molecular complexity in space during the star formation process. 2-methoxyethanol, which contains 13 atoms, is quite large for interstellar standards — as of 2021, only six species larger than 13 atoms were detected outside the solar system , many by McGuire’s group, and all of them existing as ringed structures.
“Continued observations of large molecules and subsequent derivations of their abundances allows us to advance our knowledge of how efficiently large molecules can form and by which specific reactions they may be produced,” says Fried. “Additionally, since we detected this molecule in NGC 6334I but not in IRAS 16293-2422B, we were presented with a unique opportunity to look into how the differing physical conditions of these two sources may be affecting the chemistry that can occur.”
Share this news article on:
Related links.
- McGuire Lab
- Department of Chemistry
Related Topics
- Space, astronomy and planetary science
- Astrophysics
Related Articles
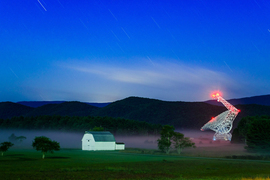
Found in space: Complex carbon-based molecules
Previous item Next item
More MIT News
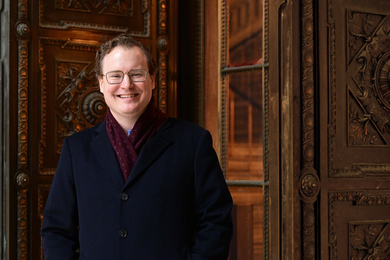
Exploring the history of data-driven arguments in public life
Read full story →
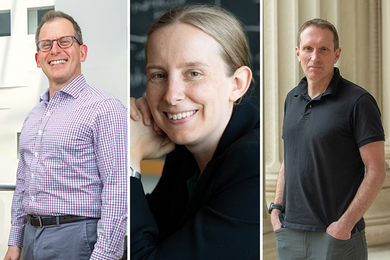
Three from MIT awarded 2024 Guggenheim Fellowships
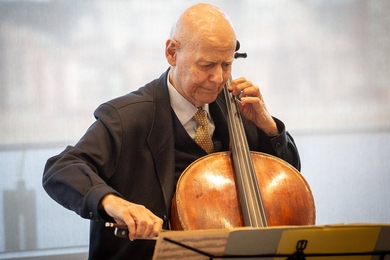
A musical life: Carlos Prieto ’59 in conversation and concert
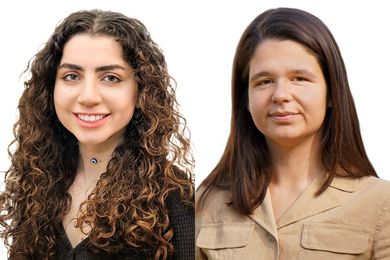
Two from MIT awarded 2024 Paul and Daisy Soros Fellowships for New Americans
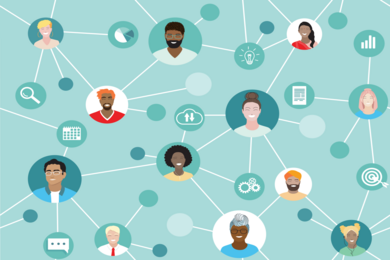
MIT Emerging Talent opens pathways for underserved global learners
The MIT Edgerton Center’s third annual showcase dazzles onlookers
- More news on MIT News homepage →
Massachusetts Institute of Technology 77 Massachusetts Avenue, Cambridge, MA, USA
- Map (opens in new window)
- Events (opens in new window)
- People (opens in new window)
- Careers (opens in new window)
- Accessibility
- Social Media Hub
- MIT on Facebook
- MIT on YouTube
- MIT on Instagram

An official website of the United States government
The .gov means it’s official. Federal government websites often end in .gov or .mil. Before sharing sensitive information, make sure you’re on a federal government site.
The site is secure. The https:// ensures that you are connecting to the official website and that any information you provide is encrypted and transmitted securely.
- Publications
- Account settings
Preview improvements coming to the PMC website in October 2024. Learn More or Try it out now .
- Advanced Search
- Journal List
- Wiley-Blackwell Online Open

Variable selection – A review and recommendations for the practicing statistician
Georg heinze.
1 Section for Clinical Biometrics, Center for Medical Statistics, Informatics and Intelligent Systems, Medical University of Vienna, Vienna, 1090, Austria
Christine Wallisch
Daniela dunkler, associated data.
Statistical models support medical research by facilitating individualized outcome prognostication conditional on independent variables or by estimating effects of risk factors adjusted for covariates. Theory of statistical models is well‐established if the set of independent variables to consider is fixed and small. Hence, we can assume that effect estimates are unbiased and the usual methods for confidence interval estimation are valid. In routine work, however, it is not known a priori which covariates should be included in a model, and often we are confronted with the number of candidate variables in the range 10–30. This number is often too large to be considered in a statistical model. We provide an overview of various available variable selection methods that are based on significance or information criteria, penalized likelihood, the change‐in‐estimate criterion, background knowledge, or combinations thereof. These methods were usually developed in the context of a linear regression model and then transferred to more generalized linear models or models for censored survival data. Variable selection, in particular if used in explanatory modeling where effect estimates are of central interest, can compromise stability of a final model, unbiasedness of regression coefficients, and validity of p ‐values or confidence intervals. Therefore, we give pragmatic recommendations for the practicing statistician on application of variable selection methods in general (low‐dimensional) modeling problems and on performing stability investigations and inference. We also propose some quantities based on resampling the entire variable selection process to be routinely reported by software packages offering automated variable selection algorithms.
1. INTRODUCTION
Statistical models are useful tools applied in many research fields dealing with empirical data. They connect an outcome variable to one or several so‐called independent variables (IVs; a list of abbreviations can be found in the Supporting Information Table S1) and quantify the strength of association between IVs and outcome variable. By expressing these associations in simple, interpretable quantities, statistical models can provide insight to the way how multiple drivers cooperate to cause a specific outcome. In life sciences, many such mechanisms are still not well understood but it is often plausible to assume that they are multifactorial. Therefore, empirical data collection and multivariable analysis are important contributors to knowledge generation.
Statistical models have been defined and described in various ways, ranging from pure mathematical notation over philosophical aspects to emphasis on their usefulness. While many scientists agree that the formal definition of a statistical model is “ A set of probability distributions on the sample space 𝒮” (Cox & Hinkley, 1979 ), this does not satisfactorily describe its relevance in science. More self‐explanatory descriptions are “ Statistical models summarize patterns of the data available for analysis ” (Steyerberg, 2009 ), “ A powerful tool for developing and testing theories by way of causal explanation, prediction, and description ” (Shmueli, 2010 ), “ A simplification or approximation of reality” (Burnham & Anderson, 2002 ) or “ A statistical model represents, often in considerably idealized form, the data‐generating process ” (Wikipedia, 2017 ).
The question whether empirical observations are realizations of an ascertainable data‐generating mechanism, which possibly can be broken down to simple, comprehensible rules, is as old as science itself. For example, ancient Greek philosopher Aristotle has often been quoted with “ Nature operates in the shortest ways possible ” (AZquotes.com, 2017a ), or physicist Isaac Newton with “ We are to admit no more causes of natural things than such as are both true and sufficient to explain their appearance ” (Newton, Motte, & Machin, 1729 ). By contrast, many modern life scientists cast doubts on the existence of a single “true model” that could be detected by empirical data collection. Examples are Burnham and Anderson ( 2002 ) (“ We do not accept the notion that there is a simple “true model” in the biological sciences .”), Steyerberg ( 2009 ) (“ We recognize that true models do not exist. … A model will only reflect underlying patterns, and hence should not be confused with reality .”), Box and Draper ( 1987 ) (Essentially, “ all models are wrong, but some are useful ”), or Breiman ( 2001a ) (“ I started reading Annals of Statistics, and was bemused: Every article started with “Assume that the data are generated by the following model: … “ followed by mathematics exploring inference, hypothesis testing and asymptotics .”).
Following this skepticism, in our view “ Statistical models are simple mathematical rules derived from empirical data describing the association between an outcome and several explanatory variables ” (Dunkler, Plischke, Leffondré, & Heinze, 2014 ). In order to be of use, statistical models should be valid, that is providing predictions with acceptable accuracy, and practically useful, that is allowing for conclusions such as “how large is the expected change in the outcome if one of the explanatory variables changes by one unit”. If interpretability of a statistical model is of relevance, simplicity must also be kept in mind. Seconders of this proposal include universal scientist and artist Leonardo da Vinci (to whom the quote “ Simplicity is the ultimate sophistication ” has often been attributed), possibly physicist Albert Einstein (“ Make everything as simple as possible, but not simpler ”), and businessman Sir Richard Branson, founder of the Virgin Group (“ Complexity is our enemy. Any fool can make something complicated ”). (AZquotes.com, 2017b – d ) Practical usefulness, for example concerning the costs of collecting variables needed to apply a model, is a further important aspect of statistical modeling, otherwise a model is likely to be "quickly forgotten" (Wyatt and Altman, 1995). Finally, as we recognize that no statistical model comes without any assumptions, we conclude that robustness to mild or moderate violations of those assumptions is also a key requirement.
The quote by Sir Richard Branson ends with the apodosis “ It is hard to keep things simple ”. In the context of data‐driven variable selection, many statisticians agree with Branson, as variable selection can induce problems such as biased regression coefficients and p ‐values and invalidity of nominal confidence levels. According to the famous problem‐solving principle “Occam's razor,” simpler solutions are preferable to more complex ones as they are easier to validate. This principle is often used to justify ”simpler models”. However, in search of simpler models, statistical analysis gets actually more complex, as then additional problems such as model instability, the possibility of several equally likely competing models, the problem of postselection inference, etc. has to be tackled. Therefore, various authors of textbooks on statistical modeling have raised differentially graded concerns about the use of variable selection methods in practice, depending on their personal experience and research areas where weak or strong theories may dominate (Burnham & Anderson, 2002 ; Harrell Jr., 2015 ; Royston & Sauerbrei, 2008 ; Steyerberg, 2009 ), while in other fields of empirical research, for example in machine learning, variable (or feature) selection seems to be the standard. Therefore, there is an urgent need for guidance through these partly controversial positions on the relevance of variable selection methods in real‐life data analysis in life sciences.
The types of statistical models that we will consider here are those that use a linear combination of IVs as their core ingredient. Furthermore, we require that regression coefficients β or simple transformations thereof, for example exp(β), have a practical interpretation for scientists. However, we do not impose any restrictions on the type of outcome variable, whether continuous, binary, count, or time‐to‐event, or on its distribution. This is because most of the methods that are described and discussed here were developed for the linear model with Gaussian errors, but were then transferred to other models for noncontinuous outcome variables.
This paper is based on a lecture held in the series “Education for Statistics in Practice” by Georg Heinze and Daniela Dunkler at the conference of the “Deutsche Arbeitsgemeinschaft Statistik” in Göttingen, Germany, March 2016. The slides used in the lecture are available at http://www.biometrische-gesellschaft.de/arbeitsgruppen/weiterbildung/education-for-statistics-in-practice.html . The paper is intended for the practicing statistician, whose routine work includes the development, estimation, and interpretation of statistical models for observational data to help answering research questions in life sciences. Based on our own experience, we assume that the statistician is responsible for statistical study design and analysis, but is working in an interdisciplinary environment with the possibility to discuss questions on the meaning of variables with applied life scientists, who often act as the principal investigators (PI). We will first review various statistical prerequisites for variable selection, and will subsequently use this toolbox to describe the most important variable selection methods that are applied in life sciences. We will discuss the impact of variable selection methods on properties of regression coefficients, confidence intervals, and p ‐values. Recommendations for practitioners and software developers are then given, and their application exemplified in analysis of a real study.
2. VARIABLE SELECTION TOOLBOX
2.1. statistical prerequisites, 2.1.1. main purposes of models.
Shmueli ( 2010 ) identified three main purposes of statistical models. Within predictive research questions, predictive (or prognostic) models have the aim to accurately predict an outcome value from a set of predictors. Explanatory models are used in etiological research and should explain differences in outcome values by differences in explanatory variables. Finally, descriptive models should “ capture the association between dependent and independent variables ” (Shmueli, 2010 ). In the life sciences, models of all three types are needed. Still, they differ in the way they are used and interpreted. While prognostic models focus on predictions, explanatory models are used to estimate (causal) effects of risk factors or exposures by means of adjusted effect estimation, and descriptive models can have elements of both.
The purpose of statistical modeling in a particular analysis will have impact on the selection of IVs for a model. This becomes apparent when considering (Hastie, Tibshirani, & Friedman, 2009 , p. 223)
While the expected prediction error is the quantity of main concern in predictive modeling, explanatory modeling primarily aims at minimizing bias, in particular in the regression coefficients. Descriptive models also focus on the regression coefficients, but do not consider causality in a formal manner. Here, we mainly deal with the latter type of models, which are probably the most often used in life sciences, but we always keep in mind interpretability of effect estimates and accuracy of predictions.
2.1.2. Linear predictor models and interpretation of regression coefficients
Depending on the type of outcome variable, models that are often used in the life sciences are the linear model, the logistic model and the semiparametric Cox ( 1972 ) model. The linear model is defined by Y = β 0 + β 1 X 1 + … + β k X k + ε , with Y a continuous outcome variable and ε ∼ N (0, σ 2 ) a normally distributed error. The logistic model is expressed by Pr( Y = 1) = expit ( β 0 + β 1 X 1 + … + β K X k ), with expit ( z ) = exp( z )/[1 + exp( z )], and Y a binary outcome variable. For time‐to‐event data, the semiparametric Cox proportional hazards regression model is often used and has the form h ( X , t ) = h 0 ( t )exp( β 1 X 1 + … + β k X k ), where h ( x , t ) denotes the hazard of an individual with covariate row vector x at time t , and h 0 ( t ) is an unspecified baseline hazard. All three models became popular in life sciences because regression coefficients (or transforms thereof such as exp( · )) are readily interpretable. The IVs X 1 , … , X k have the role of “explanatory variables” in explanatory models and of “predictors” in predictive models.
Common assumptions of these models are linearity , that is the expected outcome value is thought to be modeled by a linear combination of IVs, and additivity , that is the effects of the IVs can be added. Various extensions of the basic “linear predictor” models exist that can relax the linearity assumption, such as polynomial models, splines, fractional polynomials, or generalized additive models, but will not be considered here. Still, all these modifications assume additivity of effects, even if a particular IV is no longer included in the model as a single, untransformed model term. Relaxation of the additivity assumption would require consideration of interaction (product) terms between IVs, or the use of more complex functional relationship such as power functions. In the following, we will not consider these modifications, and will denote the linear, logistic, and Cox model as “linear predictor models”.
In a setting with several IVs, the fundamental interpretation of a regression coefficient β j in a linear predictor model is that of the expected change in outcome (or log odds or log hazard) if X j changes by one unit and all other variables X 1 , … X j −1 , X j +1 , … , X k are held constant . Consequently, β j measures the conditional effect of X j . However, as outlined above, the existence of a single true model and hence of correct model specification can rarely be assumed. This implies that the interpretation of β j changes if the set of IVs in the model changes and X j is correlated with other IVs. (In logistic and Cox regression models, this may even happen for uncorrelated variables (Robinson & Jewell, 1991 ).) As an example, consider a model explaining percentage of body fat by weight, height, and abdomen circumference, three highly correlated IVs. The study is explained in more detail in Section 3 . Table 1 shows regression coefficients resulting from four potential models. We see that the coefficient of weight changes considerably in magnitude and even in its sign if different IVs are used for adjustment; this is because the meaning of the weight coefficient is fundamentally different in the four models. Also the effect of height can be deemed irrelevant or highly predictive, depending on whether abdomen circumference was adjusted for or not. Comparison of adjusted R 2 of the models underlines the dominance of abdomen circumference. Therefore, any variable selection applied in a linear predictor model with correlated IVs will always change the interpretation of effects . This is of high relevance in explanatory or descriptive models, where there is an interest in interpretability of regression coefficients.
Four potential models to estimate body fat in %
R adj 2 , adjusted R 2 ; SE, standard error
2.1.3. Events‐per‐variable
The ratio between sample size (or the number of events in Cox models or the number of the less frequent outcome in logistic regression) and the number of IVs is often expressed in a simple ratio, termed “events‐per‐variable” (EPV). EPV quantifies the balance between the amount of information provided by the data and the number of unknown parameters that should be estimated. It is intuitive to assume that with limited sample size it cannot be possible to accurately estimate many regression coefficients. Therefore, simple rules like “EPV should at least be greater than ten” became popular in the literature (Harrell, Lee, Califf, Pryor, & Rosati, 1984 ). Recently, Harrell ( 2015 , p. 72) recommended a more stringent rule of 1:15. However, expressing a possible disparity between sample size and number of parameters to be estimated in a simple ratio is often oversimplifying the problem because beyond the number of IVs, many other quantities such as the strength of effects or the correlation structure of IVs may influence accuracy (Courvoisier, Combescure, Agoritsas, Gayet‐Ageron, & Perneger, 2011 ). Nevertheless, EPV is easy to assess and can roughly inform about the maximum size of a model that could in principle be fitted to a data set. Some further discussion can be found in Schumacher, Holländer, Schwarzer, Binder, and Sauerbrei ( 2012 ).
If a model may also include extensions such as non‐linear transformations of IVs or interaction (product) terms requiring additional degrees of freedom (DF), then this must be accounted for in the denominator of EPV. When considering variable selection, it is the total number of candidate IVs (and of their considered transformations) that counts; this has often been misconceived (Heinze & Dunkler, 2017 ).
2.2. Criteria for selecting variables
2.2.1. significance criteria.
Hypothesis tests are the most popular criteria used for selecting variables in practical modeling problems. Without loss of generality, consider that we compare two models M 1 : β 0 + β 1 X 1 + β 2 X 2 and M 2 : γ 0 + γ 1 X 1 . The null hypothesis that β 2 = 0 implies that β 0 = γ 0 as well as β 1 = γ 1 . Such a hypothesis could be tested by comparing the log likelihoods of M 1 and M 2 (using a likelihood ratio test), requiring fitting of the two models. Logistic and Cox models need iterative fitting, such that this test is often approximated by step‐up (score test) or step‐down (Wald test) tests. The score test only needs the fitted M 2 model, assumes β 0 = γ 0 and β 1 = γ 1 and evaluates the derivative of the log likelihood at β 2 = 0. It is typically used in forward steps to screen IVs currently not included in a model for their ability to improve model fit. By contrast, the Wald test starts at M 1 and evaluates the significance of β 2 by comparing the ratio of its estimate and its standard error with an appropriate t distribution (for linear models) or standard normal distribution (for logistic or Cox regression). It is routinely contained in the standard output of many software packages, and lends itself for a step‐down procedure. Likelihood ratio tests provide the best control over nuisance parameters by maximizing the likelihood over them both in M 1 and M 2 . In particular, if several coefficients are being tested simultaneously, likelihood ratio tests for model comparison are preferred over Wald or score tests.
Iterated testing between models yields the forward selection (FS) or backward elimination (BE) variable selection algorithms, depending on whether one starts with an empty model or with a model with all IVs that were considered upfront. Iterative FS or BE is done, using prespecified significance levels α F or α B , until no further variables are included or excluded. A FS procedure that includes BE steps is often denoted as a stepwise (forward) selection procedure, and correspondingly, a BE procedure with FS steps is denoted as stepwise backward selection. Most statisticians prefer BE over FS, especially when collinearity is present (Mantel, 1970 ). However, when models can become complex, for example in the context of high‐dimensional data, then FS is still possible.
As it is always required in statistical inference, tests between models are reliable only if these comparisons are prespecified, that is if a small number of prespecified models are compared. However, tests performed during the procedure of model‐building are not pre‐specified, as the models to be compared are often already the result from (several) previous variable inclusion or exclusion steps. This causes two problems in the interpretability of p ‐values for coefficient tests from a model derived by variable selection. First, unaccounted multiple testing will generally lead to underestimated p ‐values. Second, p ‐values for coefficient tests from a model do not test whether a variable is relevant per se, but rather whether it is relevant given the particular set of adjustment variables in that specific model.
2.2.2. Information criteria
While significance criteria are usually applied to include or exclude IVs from a model, the focus of information criteria is on selecting a model from a set of plausible models. Since including more IVs in a model will slightly increase the apparent model fit (as expressed by means of model likelihood), information criteria were developed to penalize the apparent model fit for model complexity. In his seminal work, Akaike ( 1973 ) proposed to approximate the expectation of the cross‐validated log likelihood, E t e s t E t r a i n [ log L ( x t e s t | β ^ t r a i n ) ] , by log L ( x t r a i n | β ^ t r a i n ) − k , where log L ( x t e s t | β ^ t r a i n ) and log L ( x t r a i n | β ^ t r a i n ) are the apparent log likelihood from applying a model to an independent test data set, and the log likelihood from applying it to the data with which it was developed, respectively. The Akaike information criterion (AIC) is formulated equivalently as − 2 log L ( x t r a i n | β ^ t r a i n ) + 2 k (“smaller is better” formulation).
AIC can be used to compare two models even if they are not hierarchically nested. It can also be employed to select one out of several models. For example, it is often used as selection criterion in a “best subset” selection procedure evaluating all (2 k for k variables) models resulting from all possible combinations of IVs.
If several models were fitted to a data set, let denote Δ i = A I C i − A I C m i n the AIC of model M i minus the minimum AIC across all models. Burnham and Anderson ( 2002 , p. 70) denoted models with Δ i ≤ 2 as having “substantial empirical support,” models with 4 ≤ Δ i ≤ 7 as having “considerably less empirical support,” and models with Δ i > 10 as having essentially no support. Akaike weights can be derived from the Δ i ’s computed for a set of R competing models as
These weights transform the AIC values back to scale of relative likelihood of a model. They describe the probability that a model M i is the actual best model in terms of Kullback–Leibler information conditional on the assumption that one of the R models must be the Kullback–Leibler best model.
While at first sight selection based on information criteria seems different to significance‐based selection, there is a connection between these two concepts. Consider two competing hierarchically nested models differing by one DF. Here, AIC optimization corresponds to significance‐based selection at a significance level of 0.157. More generally, the significance level corresponding to AIC selection in hierarchical model comparisons is α A I C ( D F ) = 1 − F χ 2 , D F (2 · D F ), with F χ 2 , D F ( x ) denoting the cumulative distribution function of the χ DF 2 distribution evaluated at x . Therefore, AIC‐selected models will generally contain effects with p ‐values (in the final model) lower than approximately 0.157. Moreover, as sample size gets larger, they will include a larger number of irrelevant IVs, in line with the belief that there exists no true data generating model. The Bayesian information criterion (BIC) was developed for situations where one assumes existence of a true model that is in the scope of all models that are considered in model selection. With selection guided by BIC, the selected model converges to the “true” data generating model (in a pointwise manner) (Schwarz, 1978 ). BIC is defined as B I C = −2log L + log( n ) · k , where n is the sample size (or, in Cox or logistic models, the number of events or number of less frequent outcomes, respectively). It also has a significance correspondence, α B I C ( D F , n ) = 1 − F χ 2 , D F (log( n ) · D F ); for example α B I C ( D F = 1, n = 100) = 0.032. Consequently, for any suitable sample size the penalty factor of BIC is larger than that of AIC and BIC will select smaller models.
2.2.3. Penalized likelihood
Model selection can also be achieved by applying least angle selection and shrinkage operator (LASSO) penalties, which are based on subtracting a multiple (λ) of the absolute sum of regression coefficients from the log likelihood and thus setting some regression coefficients to zero (Tibshirani, 1996 ). Availability of efficient software (e.g. PROC GLMSELECT in SAS software or the R package glmnet ) (Friedman, Hastie, & Tibshirani, 2010 ; SAS Institute Inc., 2016 ) allows to fit linear, logistic, and Cox models with such penalties, and to optimize the tuning parameter λ, which controls the penalization strength via cross‐validation or information criteria.
LASSO models have been used extensively in high‐dimensional model selection problems, that is when the number of IVs k by far exceeds the sample size n . In low‐dimensional problems ( k < n ) researchers are usually interested in interpretable regression coefficients, and for that purpose LASSO models are far less understood than for their predictive performance. Regression coefficients estimated by the LASSO are biased by intention, but can have smaller mean squared error (MSE) than conventional estimates. Because of the bias, their interpretation in explanatory or descriptive models is difficult, and confidence intervals based on resampling procedures such as the percentile bootstrap do not reach their claimed nominal level. Recently the problem of performing valid inference on the regression coefficients after model selection by the LASSO was investigated (Taylor & Tibshirani, 2015 ) and a solution was proposed. However, there is still not enough evidence on the performance of this method. Valid inference requires that both the bias and the variance components in the sampling distribution of the regression coefficients are adequately captured. However, to determine the bias component, one needs an estimate of the bias, essentially requiring comparison with an unbiased regression coefficient, which in turn cannot be obtained within the variance‐reducing penalization framework. Therefore, postselection confidence intervals can be even wider than their maximum likelihood counterparts computed from a model that includes all considered IVs, which is also demonstrated in one of the examples of Taylor and Tibshirani ( 2015 ).
Another problem with LASSO estimation is its dependence on the scale of the covariates. Therefore, LASSO implementations in standard software perform an internal standardization to unit variance which in some of its software implementations is invisible to the user as regression coefficients are transformed back and reported on the original scale. Still, it is not clear if this type of “one size fits all” standardization is optimal for all modeling purposes; consider, for example the typical case of a mixture of continuous and binary covariates, where continuous covariates can have different skewness and binary covariates can have substantially different degrees of balance. This case has also been addressed, for example by Porzelius, Schumacher, and Binder ( 2010 ).
2.2.4. Change‐in‐estimate criterion
In particular in epidemiologic research the change‐in‐estimate criterion is often applied to select adjustment variables for explanatory models (Bursac, Gauss, Williams, & Hosmer, 2008 ; Lee, 2014 ; Maldonado & Greenland, 1993 ; Mickey & Greenland, 1989 ; Vansteelandt, Bekaert, & Claeskens, 2012 ). Reconsidering models M 1 and M 2 of Section 2.2 Significance Criteria , the change‐in‐estimate δ X 1 ( − X 2 ) = γ 1 − β 1 is the change in the regression coefficient of X 1 (a “passive” IV, M 1 : β 0 + β 1 X 1 + β 2 X 2 ) by removing X 2 (an “active” IV; M 2 : γ 0 + γ 1 X 1 ) from a model. The change‐in‐estimate criterion is often also expressed as a “relative” change δ X 1 ( − X 2 ) / β 1 × 100 % . These criteria were also incorporated in the “purposeful selection algorithm” proposed by Hosmer, Lemeshow et al. ( 2011 ; 2013 ). Recently, the criterion was investigated by Dunkler et al. ( 2014 ). These authors approximated the change‐in‐estimate by δ ^ X 1 ( − X 2 ) = − β ^ 2 σ ^ 12 / σ ^ 2 2 , where σ ^ 2 2 and σ ^ 12 are the variance of β ^ 2 and the covariance of β ^ 1 and β ^ 2 , respectively. Using this approximation, it could be shown that eliminating a “significant” IV from a model will lead to a “significant” change‐in‐estimate, and eliminating a “nonsignificant” IV to a “nonsignificant” change‐in‐estimate. Moreover, the authors standardized the change‐in‐estimate criterion for use in linear, logistic, and Cox regression models, such that the change‐in‐estimate can be compared to a single, common threshold value τ in order to decide whether an IV X j should be dropped from a model or not. Dunkler et al. ( 2014 ) suggested to combine the standardized change‐in‐estimate criterion with significance‐based BE, yielding the “augmented backward elimination” (ABE) procedure. Simulation results showed that in general, ABE leads to larger models and less biased regression coefficients than BE, and to MSE of regression coefficients that often fall between those achieved by BE and by models including all considered IVs. Therefore, ABE may be useful to eliminate IVs from a model that are irrelevant both for model fit and for interpretation of βs of other IVs, for example for confounder selection. However, experience with this approach is still limited.
2.2.5. Background knowledge
Many authors have repeatedly highlighted the importance of using background knowledge to guide variable selection. Background knowledge can be incorporated at least at two stages, and it requires an intensive interplay between the PI of the research project (usually a nonstatistician) and the statistician in charge of designing and performing statistical analysis. At the first stage, the PI will use subject‐specific knowledge to derive a list of IVs which in principle are relevant as predictors or adjustment variables for the study in question. This list will mostly be based on the availability of variables, and must not take into account the empirical association of IVs with the outcome variable in the data set. The number of IVs to include in the list may also be guided by the EPV (see our discussion in Section 2.1 Events‐per‐variable ).
Together with the PI, the statistician will go through the list and critically question the role and further properties of each of the variables, such as chronology of measurement collection, costs of collection, quality of measurement, or availability also to the “user” of the model. Having appropriately pruned this working set of IVs, a first multivariable model could be estimated (the “ global model” ).
It is often helpful to draft a sketch of a graph visualizing assumed causal dependencies between the IVs, where the level of formalization of those dependencies may sometimes reach that of a directed acyclic graph (DAG) (Greenland, Pearl, & Robins, 1999 ). In developing explanatory models, such a DAG is a necessary prerequisite to identify the sets of variables necessary to adjust for in order to avoid bias (Evans, Chaix, Lobbedez, Verger, & Flahault, 2012 ; Greenland et al., 1999 ; VanderWeele & Shpitser, 2011 ). Developing DAGs can also be of help in predictive model building, for example to identify redundant or alternative predictors.
Given that one of the IVs is of primary interest, for example expressing an exposure the effect of which should be inferred by a study, DAGs allow to classify the other IVs into confounders, mediators, and colliders (Andersen & Skovgaard, 2010 ). (A short explanation of differences between confounders, mediators, and colliders can be found in the Supporting Information Figure S1). This is important because in order to obtain an unbiased estimate of the causal effect of the exposure on the outcome, all confounders should be adjusted for, but colliders or mediators must not be included in a model. Simple and more involved DAGs are exemplified by Greenland et al. ( 1999 ). These authors point out that counter to intuition, adjusting for an IV in a multivariable model may not only eliminate association between marginally associated IVs, but may also induce an association between marginally unassociated IVs. They provide extended rules to be applied to DAGs in order to determine the set of IVs necessary for adjustment. DAGs always heavily rely on prior belief of the investigators on the roles of the explanatory variables, which will not be always available in research projects. Therefore, robust confounder selection procedures based on DAGs were proposed such as the disjunctive cause criterion (VanderWeele & Shpitser, 2011 ).
Often one is willing to trade in a little bias in return for considerably reduced variance. This means, that even in explanatory models where the set of adjustment variables necessary to control confounding is assumed to be known, some of the confounders’ association with the outcome may be so weak that adjustment may increase variance in the effect estimate of main interest more than reducing its bias. Consequently, depending on whether the true association of a potential confounder with the outcome is weak or strong, variable selection may be beneficial or harmful, even if performed with the same significance criterion. This is exemplified by means of a simple simulation study in Figure 1 . From this simplified simulation study we conclude, that (i) if background knowledge on the strength of a confounder exists, it should be used to decide whether variable selection should be applied or not, and (ii) one cannot recommend a universally applicable significance criterion for variable selection that fits all needs.

Simulation study to illustrate possible differential effects of variable selection. Graphs show scatterplots of estimated regression coefficients β ^ 1 and β ^ 2 in 50 simulated datasets of size N = 50 with two standard normal IVs with correlation ρ = 0.5. Circles and dots indicate simulated datasets where a test of the null hypothesis β 2 = 0 yields p ‐values greater or lower than 0.157, respectively. The dashed lines are regression lines of β 1 on β 2 ; thus they indicate how β 1 would change if β 2 is set to 0
2.3. Variable selection algorithms
Table 2 lists some of the most popular variable selection methods for explanatory or descriptive models. Each variable selection algorithm has one or several tuning parameters that can be fixed to a prespecified value or estimated, for example by cross‐validation or AIC optimization. Note that tenfold cross‐validation and selection by AIC are asymptotically equivalent (Stone, 1977 ). Moreover, comparison of two hierarchically nested models with a difference of one DF by AIC is equivalent to performing a likelihood ratio test at a significance level of 0.157.
Some popular variable selection algorithms
2.4. Consequences of variable selection and possible remedies
2.4.1. consequences of variable selection.
The basis of unbiased and accurate regression coefficients and predictions is the identification of the true data generating mechanism. In Section 1 , we already engaged in a philosophical discussion on whether the existence of such a mechanism can be assumed at all, and if such a mechanism would be “simple enough” to be detectable by regression models with linear predictors. These important aspects held aside, falsely including or excluding IVs will have direct consequences on the variance and the bias of the regression coefficients (Figure 2 ). However, as Figure 2 shows, these dependencies can be complex and their direction and magnitude depend on the correlation of IVs and are hard to predict in a particular data set.

A schematic network of dependencies arising from variable selection. β, regression coefficient; IV, independent variable; RMSE, root mean squared error
In general, while variable selection has often been described as inducing a bias away from zero, nonselection of a variable could also be interpreted as shrinkage toward zero. Consequently, variable selection methods often reduce the RMSE of regression coefficients, in particular for weak or noise predictors. From our experience, this shrinkage effect is quite extreme for LASSO at small sample sizes (EPV < 10), which can result in considerably reduced RMSEs of true regression coefficients. This comes at the cost of predictions that are considerably biased toward the mean and may even have larger RMSEs than competing methods. In particular, this may be observed at the tails of the distribution of the true outcome variable.
While univariable variable selection, that is including those IVs in a multivariable model that show significant association with the outcome in univariable models, is one of the most popular approaches in many fields of research, it should be generally avoided (Heinze & Dunkler, 2017 ; Sun, Shook, & Kay, 1996 ). Among the significance‐based selection methods, we prefer BE over FS (Mantel, 1970 ). From our experience, use of the AIC, which corresponds to α B ≈ 0.157, but no smaller α B is recommendable if less than 25 EPV are available. For samples with more EPV, researchers believing that a true simple model exists and is identifiable may prefer a more stringent threshold ( α B = 0.05). They may also prefer BIC as it has the potential to identify the correct model asymptotically.
LASSO selection tends to select more IVs than BE, underestimating the effects of IVs with an effect while assigning nonzero values to the regression coefficients of some IVs without an effect. Generally, it results in more homogenous shrinkage of all regression coefficients, while BE shrinks effects of IVs without an effect more heavily than effects of IVs with an effect.
2.4.2. Model stability
A very important, but often ignored problem of data‐driven variable selection is model stability, that is the robustness of the selected model to small perturbations of the data set (Sauerbrei, Buchholz, Boulesteix, & Binder, 2015 ). Bootstrap resampling with replacement or subsampling without replacement are valuable tools to investigate and quantify model stability of selected models (De Bin, Janitza, Sauerbrei, & Boulesteix, 2016 ; Sauerbrei & Schumacher, 1992 ). The basic idea is to draw B resamples from the original data set and to repeat variable selection in each of the resamples. Important types of quantities that this approach can provide are (i) bootstrap inclusion frequencies to quantify how likely an IV is selected, (ii) sampling distributions of regression coefficients, (iii) model selection frequencies to quantify how likely a particular set of IVs is selected, and (iv) pairwise inclusion frequencies, evaluating whether pairs of (correlated) IVs are competing for selection.
Inclusion frequencies of any type will always depend on the chosen selection criteria, for example the significance level α B for including effects in a model, or the criterion τ for evaluating changes‐in‐estimate. The dependence of these quantities on the selection criterion can be visualized by model stability paths, as exemplified by Dunkler et al. ( 2014 ), Sauerbrei et al. ( 2015 ), or Meinshausen and Bühlmann ( 2010 ). The use of such resampling methods often leads to simpler final models (Sauerbrei, 1999 ).
Moreover, if setting a resampled regression coefficient of an IV not selected in a particular resample to 0, the resampled distribution of regression coefficients can give insight in the sampling distribution caused by variable selection. For example, the median regression coefficient for each IV computed over the resamples can yield a sparse model that is less prone to overestimation than if variable selection is applied only to the original data set. The 2.5th and 97.5th percentiles of the resampled regression coefficients can serve as resampling‐based confidence intervals, taking into account model uncertainty without making assumptions on the shape of the sampling distribution. However, they may severely underestimate the true variability if bootstrap inclusion frequencies are low, for example below 50%.
To derive a predictor that incorporates model uncertainty, Augustin, Sauerbrei, and Schumacher ( 2005 ) and Buchholz, Holländer, and Sauerbrei ( 2008 ), proposed a two‐stage bootstrap procedure. In the first step, IVs are screened based on their inclusion frequencies, and IVs with negligible effects eliminated. In the second step, bootstrap model averaging is used to obtain an aggregated model. The regression coefficients are simply averaged over the bootstrap resamples. Variances for the regression coefficients can be obtained by making use of Buckland, Burnham, and Augustin ( 1997 )’s variance formula taking into account both within‐model variance and model selection uncertainty. This approach has two control parameters, the significance level in the variable selection procedure and the minimum bootstrap inclusion frequency required to include an IV in the second step.
Pairwise inclusion frequencies can be easily compared against their expected values given independent selection of the pair of IVs. Values below the expectation would give rise for assuming selection competition between the two IVs, while values above the expectation indicate joint selection of a “rope team” of IVs; an IV's effect is then amplified by adjustment for a particular other IV.
2.4.3. Shrinkage and bias‐variance trade‐off
Shrinkage has two meanings in statistics: as a phenomenon , shrinkage describes the situation where predictions from a model are too optimistic, that is if observed outcomes are closer to the overall mean outcome than the predictions. As a technique it prepares estimates such that the phenomenon of shrinkage should not occur, that is a shrinkage estimator anticipates shrinkage and adjusts estimators accordingly. Even unbiased estimators can yield shrinkage (see Harrell Jr. ( 2015 , p. 75) for an intuitive explanation). Shrinkage estimators are generally biased, usually toward zero. Shrinkage estimators can be obtained by postestimation procedures (Dunkler, Sauerbrei, & Heinze, 2016 ) or by penalized likelihood; for example the LASSO is a shrinkage estimator.
Shrinking estimates, that is taking a certain amount of bias toward zero into account, can have desirable effects on the variance of those estimates. Intuitively, this decrease in variance results naturally from restricting the sampling space of regression coefficients when applying shrinkage methods (Greenland, 2000 ; Hastie et al., 2009 , p. 225). Therefore, shrinkage methods are useful in prediction modeling tasks where the focus is on obtaining accurate predictions, that is predictions with a low MSE.
Shrinkage methods were also proposed to reduce overestimation bias of regression coefficients and MSE in models obtained by variable selection (Dunkler et al., 2016 ; Sauerbrei, 1999 ; van Houwelingen & Sauerbrei, 2013 ). In particular, parameterwise shrinkage assigns different shrinkage factors to each regression coefficient depending on the strength of association that it expresses (Sauerbrei, 1999 ). Regression coefficients for which selection is less stable are shrunken more strongly than coefficients for which selection is stable. The shrinkage factors are obtained by leave‐one‐out resampling. Dunkler et al. ( 2016 ) suggest an extension of these shrinkage factors for semantically related regression coefficients (e.g. dummy variables coding a categorical IV), propose a computational approximation to their estimation and provide an R package shrink to facilitate their application in practice.
3. TOWARD RECOMMENDATIONS
3.1. recommendations for practicing statisticians.
Many researchers seek advice for the typical situation where there are many IVs to be potentially considered in a model but where sample size is limited (EPV ≈10 or lower). In applied research, variable selection methods have too often been misused, giving such data‐driven methods the exclusive control over model building. This has often resulted from common misconceptions about the capabilities of variable selection methods (Heinze & Dunkler, 2017 ).
3.1.1. Generate an initial working set of variables
Modeling should start with defendable assumptions on the roles of IVs that can be based on background knowledge (that a computer program typically does not possess), that is from previous studies in the same field of research, from expert knowledge or from common sense. Following this golden rule, an initial working set of IVs, the “ global model ,” can often be compiled without yet using the data set at hand to uncover the relationships of IVs with the outcome (Harrell Jr., 2015 ). The assumed relationships between the variables of this working set may be summarized, for example by drafting a DAG (Andersen & Skovgaard, 2010 ). Often it may be only possible to draw a rudimentary DAG or apply a simple criterion like the disjunctive cause criterion (VanderWeele & Shpitser, 2011 ). It may then well turn out that some IVs are not needed in a multivariable model because of redundancy or because their effects, adjusted for others, are not of much interest per se.
Using background knowledge only it should be aimed to determine whether the association of each variable with the outcome, given the other IVs, is assumed to be relatively strong, or rather unclear. The EPV ratio should be computed for the global model ( E P V g l o b a l ).
3.1.2. Decide whether variable selection should be applied, which IVs are considered and which variable selection method is employed
We advise not to consider variable selection on “strong” IVs, and to subject IVs with unclear role to variable selection only with a sufficient sample size. If variable selection is applied, it should be accompanied by stability investigations. Some further rules based on the E P V g l o b a l can be found in Table 3 . Of course, these limits are subject to the aforementioned limitations of E P V . They are rough rules of thumb based on our experience, the assumption that there exist some nonpredictive IVs, our simulations done for this and our previous paper (Dunkler et al., 2014 ), and on recommendations of other authors. The recommended E P V g l o b a l limits should be adapted to the situation, for example raised if correlations between candidate IVs are particularly strong, or lowered if the candidate variables are all independent of each other. As we have mentioned above, some authors even suggest an E P V g l o b a l of at least 15 as a meaningful number needed for interpretability of a global model (Harrell, 2015 ). Among the variable selection procedures BE is preferred as it starts with the assumed unbiased global model. AIC ( α B = 0.157) could be used as a default stopping criterion, but in some situations larger values of α B may be needed to increase stability. Only in very large data sets, E P V g l o b a l > 100, one could consider the BIC or α B ≤ 0.05. If it should be guaranteed that important predictors or confounders should not be missed, then ABE may be a useful extension to BE.
Some recommendations on variable selection, shrinkage, and stability investigations based on events‐per‐variable ratios
3.1.3. Perform stability investigations and sensitivity analyses
Variable selection generally introduces additional uncertainty. In the following subsection, we propose a list of quantities useful to assess selection stability and model uncertainty, which should be routinely reported whenever variable selection is employed. In some software such as SAS /PROC GLMSELECT, most of those quantities are already available. In other packages such as Stata or R, they can be easily obtained using few lines of additional code, following our example R codes provided as Supporting Information on the journal's web page. Unfortunately, this possibility is missing in some popular software packages, for example in IBM SPSS Statistics .
Stability investigations should at least comprise the assessment of the impact of variable selection on bias and variance of regression coefficient and the computation of bootstrap inclusion frequencies. Optionally, model selection frequencies, and pairwise inclusion frequencies could be added. An extended stability investigation as that performed by Royston and Sauerbrei ( 2003 ), who performed a re‐analysis of the bootstrap inclusion fractions of each IV using log‐linear analysis, can be very informative but may go beyond the usual requirements.
Overestimation bias results if a variable has been selected only because its regression coefficient appeared to be extreme in the particular sample. This conditional bias can be expressed relative to the (assumed unbiased) regression coefficient in the global model estimated on the original data, and computed as the difference of the mean of resampled regression coefficients computed from those resamples where the variable was selected and the global model regression coefficient, divided by the global model regression coefficient.
For assessing variance, we propose to compute the root mean squared difference (RMSD) of the bootstrap estimates of a regression coefficient and its corresponding (assumed unbiased) value in the global model estimated on the original data. The “RMSD ratio,” that is RMSD divided by the standard error of that coefficient in the global model, intuitively expresses the variance inflation or deflation caused by variable selection.
Furthermore, we advise to perform sensitivity analyses by changing decisions made in the various analysis steps. For example, there may be competing sets of assumptions on the roles and assumed strengths of variables in the first step that might lead to different working sets. Or, selection or nonselection of IVs and estimated regression coefficients are sensitive to the p ‐value thresholds used. This sensitivity can be visualized using stability paths as exemplified in Dunkler et al. ( 2014 ). Sensitivity analyses should be prespecified in the analysis protocol.
The most unpleasant side effect of variable selection is its impact on inference about true values of regression coefficients by means of tests and confidence intervals. Only with large sample sizes, corresponding to EPV greater than 50 or 100, we can trust in the asymptotic ability of some variable selection methods to identify the true model, thus making inference conditional on the selected model approximately valid. With more unfavorable EPVs, model instability adds a nonnegligible source of uncertainty, which would be simply ignored by performing inference only conditional on the selected model. It has been pointed out that valid postselection inference cannot be achieved (Leeb & Pötscher, 2005 ).
Depending on a specific situation, we propose some pragmatic solutions:
Solution : Perform inference in the global model.
In Section 3.1 , we discuss how to use background knowledge to build a global model without uncovering the relationship of the IVs with the outcome variable. Thus, this model can be used to provide a valid p ‐value for an IV that is adjusted for all other IVs that were in principle considered in model‐building. The purpose of variable selection would then be restricted to reducing this global model to a prediction model of higher practical usability, but not to draw conclusions about effects.
Solution : Perform multimodel inference with AIC.
Burnham and Anderson ( 2002 ) have proposed to use a few prespecified, approximately equally plausible models for drawing inference about regression coefficients. In order to compute total variances, the average within‐model variance and the between‐model variance should be added, where within‐model variances are weighted by model importance measured, for example by Akaike weights or by bootstrap model frequencies (Buckland et al., 1997 ). This type of inference may be indicated for example for explanatory models, where there are often strong arguments against the plausibility of a global model, and if there are only a few competing models.
Solution : Perform multi‐model inference with the resampled distribution of regression coefficients.
In absence of a strong theory model selection is often purely based on the evidence provided by the data, for example by applying a variable selection algorithm. The sampling distribution of a regression coefficient can be obtained by bootstrap resampling, repeating variable selection in each bootstrap resample. If this sampling distribution is approximately centered at the estimate from the global model, then there is no indication of bias induced by variable selection, and multimodel inference can be performed by evaluating quantiles of the sampling distribution to define confidence intervals or to derive p ‐values. In other cases such intervals may give at least a realistic impression of variability. The median of the bootstrap distribution could be used as multimodel point estimate, having the advantage to be zero in case a variable was selected in less than 50% of the resamples. Alternative proposals to yield a parsimonious aggregated model were made by Augustin et al. ( 2005 ) and Buchholz et al. ( 2008 ). Finally, we would like to point at the shrinkage methods that were developed to reduce the MSE of estimates and predictions, and which can also serve to reduce a possible overestimation bias induced by variable selection (Dunkler et al., 2016 ; Sauerbrei, 1999 ; van Houwelingen, 2001 ; van Houwelingen & Sauerbrei, 2013 ).
3.2. Recommendations for developers of standard software packages
BE, FS, or stepwise selection algorithms are available in many statistical software packages, see Table 4 for an overview. However, in many cases these implementations leave the user alone with an uncritically reported “finally selected model” with coefficients, standard errors, confidence intervals, and p ‐values that do not differ from those that would be computed for a predefined model. Usually, implementations do not make any account of the modeling decisions made to arrive at that final model. The reported quantities often overstate the true relationship of the selected variables with the outcome, which often results in conditional bias away from zero, and underestimated standard errors, widths of confidence intervals, and p ‐values. Reporting a single final model generates the impression that selection or nonselection of an IV is a safe bet. This impression arises partly because no variance is reported for the nonselected variables, and partly because the reported standard errors of selected variables do not account for the selection uncertainty.
Implementations of variable selection methods and resampling‐based stability analysis in selected statistical softwares
With the recent advances in computing speed, there is no excuse for still refraining from including stability investigations using resampling methods in the default output of standard software implementations of variable selection algorithms. As a minimum, we would call for reporting the following quantities, cf. also Altman, McShane, Sauerbrei, & Taube ( 2012 ):
- The EPV ratio, computed from the number of candidate variables, accompanied by a warning note if a user attempts to invoke variable selection if EPV is lower than 25.
- The global model including all candidate variables with regression coefficients and standard errors. (See also REMARK guidelines, item 17, Altman et al., 2012 ).
- Bootstrap inclusion frequencies for each candidate variable (not only the selected ones).
- The RMSD of the bootstrapped regression coefficients compared to the regression coefficients of the global model is given by R M S D ( β j ) = ∑ b ( β ^ b o o t , j ( b ) − β ^ g l o b a l , j ) 2 / n b o o t , j = 1 , … , k . We propose that software output should at least contain an “RMSD ratio,” which is the RMSD divided by the standard error of β ^ g l o b a l , j .
- Relative bias conditional on selection, computed as [ ( β ^ ¯ b o o t ) / ( β ^ g l o b a l · B I F ) − 1 ] × 100 % with β ^ ¯ b o o t , β ^ g l o b a l and BIF denoting the mean bootstrapped estimate, the global model estimate, and the bootstrap inclusion frequency of an IV, respectively.
- The bootstrap model selection frequencies for the finally selected model and the most often selected models, for example the list of models with cumulative frequency of at least 80%, or the list of 20 top‐ranked models, whichever list is shorter.
- A matrix with pairwise inclusion frequencies, which are suitably summarized, for example as odds ratios obtained by log‐linear analysis (see Royston & Sauerbrei, 2003 ) or as “significant” pairwise over‐ or underselection.
In the next section, we exemplify how these quantities may help the analyst in getting more insight in the variable selection mechanism and in interpreting the results of variable selection.
3.3. Application: Approximation of proportion body fat by simple anthropometry
Research question: “ We would like to approximate the proportion of body fat by simple anthropometric measures ”
Johnson's ( 1996 ) body fat study was intended as an educational data set to teach multivariable linear regression in the classroom. Nevertheless, the example has a relevant scientific question, namely the approximation of a costly measurement of body density (from which proportion of body fat can be derived with Siri's ( 1956 ) formula) by a combination of age, height, weight, and ten simple anthropometric circumference measures through multivariable linear regression. The data set, consisting of measurements on 252 men, appears at several places in the statistical model‐building literature, for example also in the books of Burnham and Anderson ( 2002 ) and of Royston and Sauerbrei ( 2008 ), and in several journal articles. The data set is available at https://ww2.amstat.org/publications/jse/v4n1/datasets.johnson.html . (In line with literature we removed one observation with implausible values, thus, the analysis set consists of 251 subjects). The interesting feature of the data set is that many of the anthropometric measures are intrinsically correlated: out of 13 IVs, there are two pairs of IVs (hip with weight, abdomen with chest) having Pearson correlation coefficients greater than 0.9, and a group of ten IVs with all pairwise correlation coefficients greater than 0.5 (forearm, biceps, wrist, neck, knee, hip, weight, thigh, abdomen, chest). These high correlations impose some challenges in model development and interpretation. In particular, interpretation of regression coefficients as adjusted effects in the global model, or, if variable selection is applied, interpretation of non‐selected variables as “nonpredictive” seems problematic.
We have analyzed this data set following the recommendations given above. E P V g l o b a l amounts to 251 / 13 = 19.3. We consider abdomen and height as two central IVs for estimating body fat proportion, and will not subject these two to variable selection. In our prior assessment, we further believe that all other IVs may be strongly interrelated and exchangeable when used for body fat estimation. Therefore, we will subject them to BE with AIC as stopping criterion. This gives a model consisting of the IVs abdomen, height, wrist, age, neck, forearm, and chest (Table 5 ). The adjusted R 2 only slightly increases from 73.99% in the global model to 74.16% in the selected model. The estimated shrinkage factor of the latter model is 0.983 and therefore additional post‐estimation shrinkage is not necessary. (We do not recommend parameterwise shrinkage factors for this data set because of the high correlations between the variables, see Dunkler et al., 2016 ). Bootstrap resampling indicates the instability of this model, as it was selected in only 1.9% of the resamples, and if models are ranked by their selection frequencies, it ranks only fourth (Table 6 ). However, a low bootstrap model frequency like this is not untypical in a biomedical data set. Variable selection adds to uncertainty about the regression coefficients, which is evidenced by RMSD ratios all above 1, except for knee (0.78) and for weight (0.95). By contrast, the model‐based standard errors in the selected model, which ignore the selection uncertainty, would wrongly suggest more precise estimates. Relative conditional bias quantifies how much variable‐selection‐induced bias one would have to expect if an IV is selected. This bias is negligible for height, abdomen, wrist, or age, all of which with bootstrap inclusion frequencies greater than 80%, but becomes more relevant in IVs for which selection is less sure. A sparse aggregate over the different models estimated in the bootstrap procedure could be obtained by the bootstrap medians, in this analysis it resembles the selected model. The coefficients of the selected IVs are very similar to the global model estimates, indicating no selection bias in the aggregated model. The 2.5th and 97.5th percentiles can be interpreted as limits of 95% confidence intervals obtained by resampling‐based multi‐model inference.
Body fat study: global model, model selected by backward elimination with a significance level of 0.157 (AIC selection), and some bootstrap‐derived quantities useful for assessing model uncertainty
RMSD, root mean squared difference, see Section 3.2(iv).
Body fat study: model selection frequencies. Selected model is model 4
Table 6 shows model selection frequencies. The highest selection frequency is only 3.2% and is obtained for the model including height, abdomen, wrist, age, chest, and biceps. Notably, this is not the final model from the original data set and there are many competing models with similar selection frequencies. The model suggested by the bootstrap medians is the same as the selected model; however, for neck and forearm the bootstrap medians are clearly closer to zero than in the selected model.
Pairwise inclusion frequencies inform about “rope teams” and “competitors” among the IVs (Supporting Information Table S2). For example, thigh and biceps were both selected in only 14.3% of the resamples, while one would expect a frequency of 19.8% ( = 47.9% × 43.1%) given independent selection. Therefore, the pair is flagged with “‐” in the lower triangle of Supporting Information Table S1. Thigh and hip are flagged with “+” because they are simultaneously selected in 28.7% of the resamples, while the expectation under independency is only 19.8%. In this table, we used significance of a χ 2 test at the 0.01 level as the formal criterion for the flags. Interestingly, age forms a “rope team” with neck, forearm, chest, and thigh, but weight is a competitor to age.
Analyses of two further case studies can be found in the Supporting Information on the journal's web page.
We have explained underlying concepts and important consequences of variable selection methods that may still not be clear to many practitioners and software developers. Variable selection algorithms are available in any statistical software package, but stability investigations are still not part of those implementations (Table 4 ). Therefore, we have proposed some quantities to be routinely computed in standard software packages whenever a user requests to apply a variable selection algorithm. We have also exemplified how these quantities may help the analyst. We strongly believe that the practice of conducting and reporting variable selection will improve greatly if this request finds its way into routine.
We have focused on models in which effect estimates should be interpretable (in addition to pure prediction models where this is not the case), but we did not consider methods that were exclusively designed for confounder selection. Our “augmented backward elimination” algorithm that we introduced in Dunkler et al. ( 2014 ) may be particularly interesting for studies with that purpose. It gives the researcher more control on the role of the IVs in model building and often results in models that include more IVs than by BE, increasing the stability of those models. Augmented backward elimination is implemented in a SAS macro (Dunkler et al., 2014 ) and an R package (Blagus, 2017 ). We refer the interested reader to the former paper for further details on that method. De Luna, Waernbaum, and Richardson ( 2011 ), VanderWeele and Shpitser ( 2011 ) and Greenland and colleagues (Greenland et al., 1999 ; Greenland, 2008; Greenland and Pearce, 2015 ) suggested some other approaches for confounder selection.
Our review was written under the assumption that nonlinear relationships of continuous IVs with the outcome and interactions between IVs do not play a role. Both are important but also difficult topics requiring separate papers.
We have not covered more refined versions of penalized estimation such as the adaptive LASSO (Zou, 2006 ) or smoothly clipped absolute deviation (SCAD) (Fan & Li, 2001 ). Those methods were intended to reduce the false inclusion rates that were observed for the LASSO and improve its performances in situations where existence of a true data generating mechanism can be assumed. We have also not considered boosting (Bühlmann & Yu, 2003 ), random forests (Breiman, 2001b ) or other machine learning techniques that may provide variable selection, mainly in the context of high‐dimensional data, that is when the number of IVs exceeds the effective sample size. In such cases these methods may still find a sparse set of IVs, for example genetic markers, predicting the outcome accurately, but the resulting models will hardly serve an explanatory purpose in the sense of Shmueli ( 2010 ). Incorporating biological background knowledge about genetic networks and pathways (Binder & Schumacher, 2009 ) and dimension reduction techniques may often greatly reduce the number of IVs to an order of magnitude comparable to the sample size (cf. Sokolov, Carlin, Paull, Baertsch, & Stuart, 2016 , pp. 2–4). Regularization methods may then still be needed because of the failure of classical methods to deal with the high dimensionality, but resulting models will be more stable and better interpretable than those purely developed by data mining.
Variable selection methods have always been seen controversially. Users of statistical modeling software appreciate the built‐in automation to select the “relevant” effects, and often apply a reverse argument to conclude that nonselection of effects means that they are not relevant. By contrast, many experienced statisticians have warned for the instability issues and invalidated inference implied by datadependent model building. Obviously, evidence supported guidance is urgently needed. Topic group 2 “Selection of variables and functional forms in multivariable analysis” of the recently launched initiative “Strengthening Analytical Thinking for Observational Studies (STRATOS)” has started to work on it (Sauerbrei, Abrahamowicz, Altman, le Cessie, and Carpenter ( 2014 ); https://www.stratos-initiative.org , assessed November 10, 2017).
We have compiled the above recommendations believing that it needs both, a basic understanding of the possible model instabilities incurred by variable selection methods and the availability of software tools for routine use to assess and eventually correct this instability. If samples are not too small and if applied with care, variable selection methods may reduce the MSE of regression coefficients and predictions by separating irrelevant information from a model. As we have outlined above, statistical models can serve different purposes. In explanatory research, where effect estimation for one or a few IVs plays a central role, the starting point will usually be a set of IVs with assumed relationship with the outcome and those IVs of main interest. Variable selection methods may then be used to sort out irrelevant IVs in order to improve accuracy of the effect estimates of main interest (VanderWeele & Shpitser, 2011 ). In predictive research, variable selection may improve the accuracy of the predictions, but background knowledge can also be incorporated, going as far as updating the coefficients of an existing model with new data, and employing variable selection methods to assess that coefficients to update (Moons et al., 2012 ; Su, Jaki, Hickey, Buchan, & Sperrin, 2016 ). Descriptive models are perhaps the most frequent type of models estimated in life sciences, and here variable selection may help to obtain interpretable and practically applicable models. In all three cases, the exemplified stability investigations, readily available in the R package abe (Blagus, 2017 ), can be used to assess possible additional uncertainties induced.
CONFLICT OF INTEREST
The authors have declared no conflict of interest.
Supporting information
Supporting Material
Heinze G, Wallisch C, Dunkler D. Variable selection – A review and recommendations for the practicing statistician . Biometrical Journal . 2018; 60 :431–449. https://doi.org/10.1002/bimj.201700067 [ PMC free article ] [ PubMed ] [ Google Scholar ]
- Akaike, H. (1973). Formation theory and an extension of the maximum likelihood principle In Petrov B. N. & Csaki F. (Eds.), Second international symposium on information theory (pp. 267–281). Budapest, HU: Akadémiai Kiado. [ Google Scholar ]
- Altman, D. , McShane, L. , Sauerbrei, W. , & Taube, S. E. (2012). Reporting recommendations for tumor marker prognostic studies (REMARK): Explanation and elaboration . PLoS Medicine , 9 ( 5 ), e1001216. [ PMC free article ] [ PubMed ] [ Google Scholar ]
- Andersen, P. K. , & Skovgaard, L. T. (2010). Regression with linear predictors . New York, NY: Springer. [ Google Scholar ]
- AZQuotes.com . (2017a). Retrieved from https://www.azquotes.com/quote/1458996 [accessed 06 February 2017].
- AZQuotes.com . (2017b). Retrieved from https://www.azquotes.com/quote/303076 [accessed 11 April 2017].
- AZQuotes.com . (2017c). Retrieved from https://www.azquotes.com/quote/87334 [accessed 07 February 2017].
- AZQuotes.com . (2017d). Retrieved from https://www.azquotes.com/quote/705691 [accessed 07 February 2017].
- Augustin, N. , Sauerbrei, W. , & Schumacher, M. (2005). The practical utility of incorporating model selection uncertainty into prognostic models for survival data . Statistical Modelling , 5 , 95–118. [ Google Scholar ]
- Binder, H. , & Schumacher, M. (2009). Incorporating pathway information into boosting estimation of high‐dimensional risk prediction models . BMC Bioinformatics , 10 , 18. [ PMC free article ] [ PubMed ] [ Google Scholar ]
- Blagus, R. (2017). abe: Augmented Backward Elimination . R package version 3.0.1. URL Retrieved from https://CRAN.R-project.org/package=abe [accessed 13 November 2017]
- Box, G. E. P. , & Draper, N. R. (1987). Empirical model‐building and response surfaces . New York, NY: Wiley. [ Google Scholar ]
- Breiman, L. (2001a). Statistical modeling: The two cultures . Statistical Science , 16 , 199–231. [ Google Scholar ]
- Breiman, L. (2001b). Random forests . Machine Learning , 45 ( 1 ), 5–32. [ Google Scholar ]
- Buchholz, A. , Holländer, N. , & Sauerbrei, W. (2008). On properties of predictors derived with a two‐step bootstrap model averaging approach—A simulation study in the linear regression model . Computational Statistics & Data Analysis , 52 , 2778–2793. [ Google Scholar ]
- Buckland, S. T. , Burnham, K. P. , & Augustin, N. H. (1997). Model selection: An integral part of inference . Biometrics , 53 , 603–618. [ Google Scholar ]
- Bühlmann, P. , & Yu, B. (2003). Boosting with the L2 loss: Regression and classification . Journal of the American Statistical Association , 98 ( 462 ), 324–339. [ Google Scholar ]
- Burnham, K. P. , & Anderson, D. R. (2002). Model selection and multimodel inference: A practical information‐theoretic approach . New York, NY: Springer. [ Google Scholar ]
- Bursac, Z. , Gauss, C. H. , Williams, D. K. , & Hosmer, D. W. (2008). Purposeful selection of variables in logistic regression . Source Code for Biology and Medicine , 3 , 17. [ PMC free article ] [ PubMed ] [ Google Scholar ]
- Courvoisier, D. S. , Combescure, C. , Agoritsas, T. , Gayet‐Ageron, A. , & Perneger, T. V. (2011). Performance of logistic regression modeling: Beyond the number of events per variable, the role of data structure . Journal of Clinical Epidemiology , 64 , 993–1000. [ PubMed ] [ Google Scholar ]
- Cox, D. R. (1972). Regression models and life‐tables . Journal of the Royal Statistical Society, Series B , 34 ( 2 ), 187–220. [ Google Scholar ]
- Cox, D. R. , & Hinkley, D. V. (1979). Theoretical statistics (1st ed.). Boca Raton, FL: Chapman and Hall/CRC. [ Google Scholar ]
- De Bin, R. , Janitza, S. , Sauerbrei, W. , & Boulesteix, A. L. (2016). Subsampling versus bootstrapping in resampling‐based model selection for multivariable regression . Biometrics , 72 , 272–280. [ PubMed ] [ Google Scholar ]
- De Luna, X. , Waernbaum, I. , & Richardson, T. S. (2011). Covariate selection for the nonparametric estimation of an average treatment effect . Biometrika , 98 , 861–875. [ Google Scholar ]
- Dunkler, D. , Plischke, M. , Leffondré, K. , & Heinze, G. (2014). Augmented backward elimination: A pragmatic and purposeful way to develop statistical models . PLoS One , 9 , https://doi.org/10.1371/journal.pone.0113677 . [ PMC free article ] [ PubMed ] [ Google Scholar ]
- Dunkler, D. , Sauerbrei, W. , & Heinze, G. (2016). Global, parameterwise and joint shrinkage factor estimation . Journal of Statistical Software , 69 , 1–19. [ Google Scholar ]
- Evans, D. , Chaix, B. , Lobbedez, T. , Verger, C. , & Flahault, A. (2012). Combining directed acyclic graphs and the change‐in‐estimate procedure as a novel approach to adjustment‐variable selection in epidemiology . BMC Medical Research Methodology , 12 , 156. [ PMC free article ] [ PubMed ] [ Google Scholar ]
- Fan, J. , & Li, R. (2001). Variable selection via nonconcave penalized likelihood and its oracle properties . Journal of the American Statistical Association , 96 , 1348–1360. [ Google Scholar ]
- Friedman, J. , Hastie, T. , & Tibshirani, R. (2010). Regularization paths for generalized linear models via coordinate descent . Journal of Statistical Software , 33 , 1–22. [ PMC free article ] [ PubMed ] [ Google Scholar ]
- Greenland, S. (2000). Principles of multilevel modelling . International Journal of Epidemiology , 29 , 158–167. [ PubMed ] [ Google Scholar ]
- Greenland, S. (2008). Invited commentary: Variable selection versus shrinkage in the control of multiple confounders . American Journal of Epidemiology , 167 , 523–529. [ PubMed ] [ Google Scholar ]
- Greenland, S. , & Pearce, N. (2015). Statistical foundations for model‐based adjustments . Annual Review of Public Health , 36 , 89–108. [ PubMed ] [ Google Scholar ]
- Greenland, S. , Pearl, J. , & Robins, J. M. (1999). Causal diagrams for epidemiologic research . Epidemiology , 10 , 37–48. [ PubMed ] [ Google Scholar ]
- Harrell, F. E. (2015). Regression modeling strategies. With applications to linear models, logistic regression, and survival analysis . New York, Berlin, Heidelberg: Springer. [ Google Scholar ]
- Harrell, F. E. , Lee, K. L. , Califf, R. M. , Pryor, D. B. , & Rosati, R. A. (1984). Regression modelling strategies for improved prognostic prediction . Statistics in Medicine , 3 , 143–152. [ PubMed ] [ Google Scholar ]
- Hastie, T. , Tibshirani, R. , & Friedman, J. H. (2009). The elements of statistical learning: Data mining, inference, and prediction (2nd ed.). New York, NY: Springer. [ Google Scholar ]
- Heinze, G. , & Dunkler, D. (2017). Five myths about variable selection . Transplant International , 30 , 6–10. [ PubMed ] [ Google Scholar ]
- Hosmer, D. W. , Lemeshow, S. , & May, S. (2011). Applied survival analysis: Regression modeling of time to event data (2nd ed.). Hoboken, NJ: Wiley. [ Google Scholar ]
- Hosmer, D. W. , Lemeshow, S. , & Sturdivant, R. X. (2013). Applied logistic regression (3rd ed.). Hoboken, NJ: Wiley. [ Google Scholar ]
- Johnson, R. W. (1996). Fitting percentage of body fat to simple body measurements . Journal of Statistics Education , 4 ( 1 ), 265–266. [ Google Scholar ]
- Lee, P. H. (2014). Is a cutoff of 10% appropriate for the change‐in‐estimate criterion of confounder identification ? Journal of Epidemiology , 24 , 161–167. [ PMC free article ] [ PubMed ] [ Google Scholar ]
- Leeb, H. , & Pötscher, B. M. (2005). Model selection and inference: Facts and fiction . Econometric Theory , 21 , 21–59. [ Google Scholar ]
- Maldonado, G. , & Greenland, S. (1993). Simulation study of confounder‐selection strategies . American Journal of Epidemiology , 138 , 923–936. [ PubMed ] [ Google Scholar ]
- Mantel, N. (1970). Why stepdown procedures in variable selection . Technometrics , 12 , 621–625. [ Google Scholar ]
- Meinshausen, N. , & Bühlmann, P. (2010). Stability selection . Journal of the Royal Statistical Society Series B Statistical Methodology , 72 , 417–473. [ Google Scholar ]
- Mickey, R. M. , & Greenland, S. (1989). The impact of confounder selection criteria on effect estimation . American Journal of Epidemiology , 129 , 125–137. [ PubMed ] [ Google Scholar ]
- Moons, K. G. M. , Kengne, A. P. , Grobbee, D. E. , Royston, P. , Vergouwe, Y. , Altman, D. G. , & Woodward, M. (2012). Risk prediction models: II. External validation, model updating, and impact assessment . Heart , 98 , 691–698. [ PubMed ] [ Google Scholar ]
- Newton, I. , Motte, A. , & Machin, J. (1729). The mathematical principles of natural philosophy . London, UK: B. Motte. [ Google Scholar ]
- Porzelius, C. , Schumacher, M. , & Binder, H. (2010). Sparse regression techniques in low‐dimensional survival data settings . Statistical Computing , 20 , 151–163. [ Google Scholar ]
- Robinson, L. D. , & Jewell, N. P. (1991). Some surprising results about covariate adjustment in logistic regression models . International Statistical Review/Revue Internationale de Statistique , 59 , 227–240. [ Google Scholar ]
- Royston, P. , & Sauerbrei, W. (2008). Multivariable model‐building. A pragmatic approach to regression analysis based on fractional polynomials for modelling continuous variables . Chichester, UK: John Wiley & Sons, Ltd. [ Google Scholar ]
- Royston, P. , & Sauerbrei, W. (2003). Stability of multivariable fractional polynomial models with selection of variables and transformations: A bootstrap investigation . Statistics in Medicine , 22 , 639–659. [ PubMed ] [ Google Scholar ]
- SAS Institute Inc. (2016). SAS/STAT®14.2 User's Guide . Cary, NC: SAS Institute Inc.. [ Google Scholar ]
- Sauerbrei, W. (1999). The use of resampling methods to simplify regression models in medical statistics . Journal of the Royal Statistical Society Series C Applied Statistics , 48 , 313–329. [ Google Scholar ]
- Sauerbrei, W. , Buchholz, A. , Boulesteix, A.‐L. , & Binder, H. (2015). On stability issues in deriving multivariable regression models . Biometrical Journal , 57 , 531–555. [ PubMed ] [ Google Scholar ]
- Sauerbrei, W. , Abrahamowicz, M. , Altman, D. G. , le Cessie, S. , & on behalf of the, S. i. (2014). Strengthening analytical thinking for observational studies: The STRATOS initiative . Statistics in Medicine , 33 , 5413–5432. [ PMC free article ] [ PubMed ] [ Google Scholar ]
- Sauerbrei, W. , & Schumacher, M. (1992). A bootstrap resampling procedure for model building: Application to the Cox regression model . Statistics in Medicine , 11 , 2093–2109. [ PubMed ] [ Google Scholar ]
- Schumacher, M. , Holländer, N. , Schwarzer, G. , Binder, H. , & Sauerbrei, W. (2012). Prognostic factor studies In Rowley C. J. & Hoering A. (Eds.), Handbook of statistics in clinical oncology (3rd ed.). Boca Raton, FL: CRC Press. [ Google Scholar ]
- Schwarz, G. (1978). Estimating the dimension of a model . The Annals of Statistics , 6 , 461–464. [ Google Scholar ]
- Shmueli, G. (2010). To explain or to predict? Statistical Science , 25 , 289–310. [ Google Scholar ]
- Siri, W. E. (1956). The gross composition of the body . Advances in Biological and Medical Physics , 4 , 239–280. [ PubMed ] [ Google Scholar ]
- Sokolov, A. , Carlin, D. E. , Paull, E. O. , Baertsch, R. , & Stuart, J. M. (2016). Pathway‐based genomics prediction using generalized elastic net . PLoS Computational Biology , 12 ( 3 ), e1004790. [ PMC free article ] [ PubMed ] [ Google Scholar ]
- Su, T.‐L. , Jaki, T. , Hickey, G. L. , Buchan, I. , & Sperrin, M. (2016). A review of statistical updating methods for clinical prediction models . Statistical Methods in Medical Research , https://doi.org/10.1177/0962280215626466 . [ PubMed ] [ Google Scholar ]
- Steyerberg, E. (2009). Clinical prediction models: A practical approach to development, validation, and updating . New York, NY: Springer. [ Google Scholar ]
- Stone, M. (1977). An asymptotic equivalence of choice of model by cross‐validation and Akaike's criterion . Journal of the Royal Statistical Society. Series B (Methodological) , 39 , 44–47. [ Google Scholar ]
- Sun, G.‐W. , Shook, T. L. , & Kay, G. L. (1996). Inappropriate use of bivariable analysis to screen risk factors for use in multivariable analysis . Journal of Clinical Epidemiology , 49 , 907–916. [ PubMed ] [ Google Scholar ]
- Taylor, J. , & Tibshirani, R. J. (2015). Statistical learning and selective inference . Proceedings of the National Academy of Sciences of the United States of America , 112 , 7629–7634. [ PMC free article ] [ PubMed ] [ Google Scholar ]
- Tibshirani, R. (1996). Regression shrinkage and selection via the Lasso . Journal of the Royal Statistical Society Series B‐Methodological , 58 , 267–288. [ Google Scholar ]
- van Houwelingen, H. C. (2001). Shrinkage and penalized likelihood as methods to improve predictive accuaracy . Statistica Neerlandica , 55 , 17–34. [ Google Scholar ]
- van Houwelingen, H. C. , & Sauerbrei, W. (2013). Cross‐validation, shrinkage and variable selection in linear regression revisited . Open Journal of Statistics , 3 , 79–102. [ Google Scholar ]
- VanderWeele, T. J. , & Shpitser, I. (2011). A new criterion for confounder selection . Biometrics , 67 , 1406–1413. [ PMC free article ] [ PubMed ] [ Google Scholar ]
- Vansteelandt, S. , Bekaert, M. , & Claeskens, G. (2012). On model selection and model misspecification in causal inference . Statistical Methods in Medical Research , 21 , 7–30. [ PubMed ] [ Google Scholar ]
- Wikimedia Foundation, Inc. (2017). Statistical model . URL Retrieved from https://en.wikipedia.org/wiki/Statistical_model [accessed 06 February 2017].
- Wyatt, J. C. , & Altman, D. G. (1995). Commentary: Prognostic models: clinically useful or quickly forgotten ? BMJ , 311 , https://doi.org/10.1136/bmj.311.7019.1539 . [ Google Scholar ]
- Zou, H. (2006). The adaptive LASSO and its oracle properties . Journal of the American Statistical Association , 101 , 1418–1429. [ Google Scholar ]
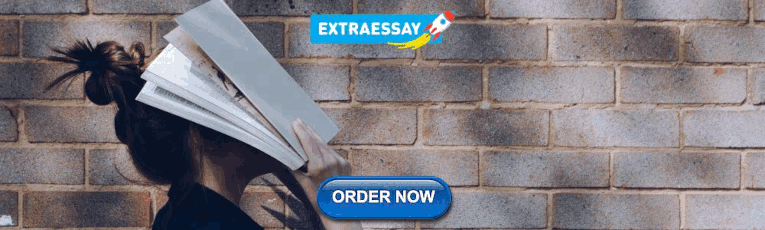
IMAGES
VIDEO
COMMENTS
Examples. Discrete variables (aka integer variables) Counts of individual items or values. Number of students in a class. Number of different tree species in a forest. Continuous variables (aka ratio variables) Measurements of continuous or non-finite values. Distance.
Categorical Variable. This is a variable that can take on a limited number of values or categories. Categorical variables can be nominal or ordinal. Nominal variables have no inherent order, while ordinal variables have a natural order. Examples of categorical variables include gender, race, and educational level.
Suitable statistical design represents a critical factor in permitting inferences from any research or scientific study.[1] Numerous statistical designs are implementable due to the advancement of software available for extensive data analysis.[1] Healthcare providers must possess some statistical knowledge to interpret new studies and provide up-to-date patient care. We present an overview of ...
Designation of the dependent and independent variable involves unpacking the research problem in a way that identifies a general cause and effect and classifying these variables as either independent or dependent. The variables should be outlined in the introduction of your paper and explained in more detail in the methods section. There are no ...
Compare your paper to billions of pages and articles with Scribbr's Turnitin-powered plagiarism checker. Run a free check. ... Types of Variables in Research | Definitions & Examples. Published on 19 September 2022 by Rebecca Bevans. Revised on 28 November 2022. In statistical research, a variable is defined as an attribute of an object of ...
As shown in Figure 2.1, scientific research proceeds along two planes: a theoretical plane and an empirical plane. Constructs are conceptualized at the theoretical (abstract) plane, while variables are operationalized and measured at the empirical (observational) plane. Thinking like a researcher implies the ability to move back and forth ...
Abstract. This short "snippet" covers three important aspects related to statistics - the concept of variables, the importance, and practical aspects related to descriptive statistics and issues related to sampling - types of sampling and sample size estimation. Keywords: Biostatistics, descriptive statistics, sample size, variables.
Distinguishing between independent and dependent variables can be tricky when designing a complex study or reading an academic research paper. A dependent variable from one study can be the independent variable in another study, so it's important to pay attention to research design. Here are some tips for identifying each variable type.
While the independent variable is the " cause ", the dependent variable is the " effect " - or rather, the affected variable. In other words, the dependent variable is the variable that is assumed to change as a result of a change in the independent variable. Keeping with the previous example, let's look at some dependent variables ...
The answer is written in length in the discussion section of the paper. Thus, the research question gives a preview of the different parts and variables of the study meant to address the problem posed in the research question.1 An excellent research question clarifies the research writing while facilitating understanding of the research topic ...
The purpose of research is to describe and explain variance in the world, that is, variance that. occurs naturally in the world or chang e that we create due to manipulation. Variables are ...
You can use the key variables like agricultural techniques, type of soil, environmental factors, types of pesticides used, the process of hybridisation, type of yield obtained after hybridisation, type of yield without hybridisation, etc. Variables are broadly categorised into: Independent Vs. Dependent Vs.
The independent variable precedes the dependent variable. The chosen stress-related coping techniques help to build resilience; thus, they occur earlier. Writing Style and Structure. Usually, the variables are first described in the introduction of a research paper and then in the method section.
A variable is the characteristic or attribute of an individual, group, educational system, or the environment that is of interest in a research study. Variables can be straightforward and easy to ...
Before beginning your paper, you need to decide how you plan to design the study.. The research design refers to the overall strategy and analytical approach that you have chosen in order to integrate, in a coherent and logical way, the different components of the study, thus ensuring that the research problem will be thoroughly investigated. It constitutes the blueprint for the collection ...
A research design is a strategy for answering your research question using empirical data. Creating a research design means making decisions about: Your overall research objectives and approach. Whether you'll rely on primary research or secondary research. Your sampling methods or criteria for selecting subjects. Your data collection methods.
The independent variable, also known as the IV, is the variable that the researchers are manipulating in an experiment or quasi-experiment. It is also the label given to the "criterion" variable in certain types of regression analysis. For example, if a researcher has two groups of people watch either a happy film or a sad film before ...
Background Mediation analysis investigates whether a variable (i.e., mediator) changes in regard to an independent variable, in turn, affecting a dependent variable. Moderation analysis, on the other hand, investigates whether the statistical interaction between independent variables predict a dependent variable. Although this difference between these two types of analysis is explicit in ...
Google Scholar provides a simple way to broadly search for scholarly literature. Search across a wide variety of disciplines and sources: articles, theses, books, abstracts and court opinions.
Experimental research serves as a fundamental scientific method aimed at unraveling cause-and-effect relationships between variables across various disciplines. This paper delineates the key features of experimental research, including the manipulation of variables, controlled conditions, random assignment, and meticulous measurement techniques ...
The simulation is produced by the Terrestrial Systems Modeling Platform (TSMP) and performed in a free evolution mode over Europe. TSMP simulations incorporate variables from underground to the top of the atmosphere (ground-to-atmosphere; G2A) and are widely used for research studies related to water cycle and climate change.
Common quantitative variables in research include age and weight. An important note is that there can often be a choice for whether to treat a variable as quantitative or categorical. For example, in a study looking at body mass index (BMI), BMI could be defined as a quantitative variable or as a categorical variable, with each patient's BMI ...
We find that GDP growth shocks and the corresponding comovements of macroeconomic variables are the key drivers of debt to GDP, accounting for 40% of the observed yearly variation in 17 advanced economies since the 1980s. ... Disclaimer: IMF Working Papers describe research in progress by the author(s) and are published to elicit comments and ...
For example, Republicans and Republican-leaning independents are much more likely than Democrats and Democratic leaners to say reducing crime should be a top priority for the president and Congress this year (68% vs. 47%), according to a recent Pew Research Center survey. How does crime in the U.S. differ by demographic characteristics?
ABOUT PEW RESEARCH CENTER Pew Research Center is a nonpartisan fact tank that informs the public about the issues, attitudes and trends shaping the world. It conducts public opinion polling, demographic research, media content analysis and other empirical social science research. Pew Research Center does not take policy positions.
New research from the group of MIT Professor Brett McGuire has revealed the presence of a previously unknown molecule in space. The team's open-access paper, "Rotational Spectrum and First Interstellar Detection of 2-Methoxyethanol Using ALMA Observations of NGC 6334I," appears in April 12 issue of The Astrophysical Journal Letters. Zachary T.P. Fried, a graduate student in the McGuire ...
Gene editing has the potential to solve fundamental challenges in agriculture, biotechnology, and human health. CRISPR-based gene editors derived from microbes, while powerful, often show significant functional tradeoffs when ported into non-native environments, such as human cells. Artificial intelligence (AI) enabled design provides a powerful alternative with potential to bypass ...
Research methods are specific procedures for collecting and analyzing data. Developing your research methods is an integral part of your research design. When planning your methods, there are two key decisions you will make. First, decide how you will collect data. Your methods depend on what type of data you need to answer your research question:
This paper explored the use of machine learning techniques to differentiate between two different musical eras of the same rock band, including the technique of Logistic Regression. Logistic regression (LR) is a widely used statistical modeling method for binary classification in supervised machine learning. It is often used to predict whether a given event belongs to one of two categories.
The paper is intended for the practicing statistician, whose routine work includes the development, estimation, and interpretation of statistical models for observational data to help answering research questions in life sciences. ... In applied research, variable selection methods have too often been misused, giving such data‐driven methods ...