Thank you for visiting nature.com. You are using a browser version with limited support for CSS. To obtain the best experience, we recommend you use a more up to date browser (or turn off compatibility mode in Internet Explorer). In the meantime, to ensure continued support, we are displaying the site without styles and JavaScript.
- View all journals
- My Account Login
- Explore content
- About the journal
- Publish with us
- Sign up for alerts
- Review Article
- Open access
- Published: 21 October 2019
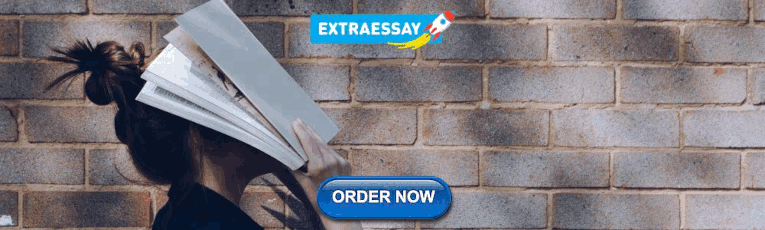
A systematic review of psychological, physical health factors, and quality of life in adult asthma
- Sabina Stanescu ORCID: orcid.org/0000-0003-0792-8939 1 ,
- Sarah E. Kirby 1 , 2 ,
- Mike Thomas ORCID: orcid.org/0000-0001-5939-1155 2 , 3 ,
- Lucy Yardley 1 &
- Ben Ainsworth 4
npj Primary Care Respiratory Medicine volume 29 , Article number: 37 ( 2019 ) Cite this article
11k Accesses
51 Citations
15 Altmetric
Metrics details
- Outcomes research
- Quality of life
Asthma is a common non-communicable disease, often characterized by activity limitation, negative effects on social life and relationships, problems with finding and keeping employment, and poor quality of life. The objective of the present study was to conduct a systematic review of the literature investigating the potential factors impacting quality of life (QoL) in asthma. Electronic searches were carried out on: MEDLINE, EMBASE, PsycINFO, the Cochrane Library, and Web of Science (initial search April 2017 and updated in January 2019). All primary research studies including asthma, psychological or physical health factors, and quality of life were included. Narrative synthesis was used to develop themes among findings in included studies in an attempt to identify variables impacting QoL in asthma. The search retrieved 43 eligible studies that were grouped in three themes: psychological factors (including anxiety and depression, other mental health conditions, illness representations, and emotion regulation), physical health factors (including BMI and chronic physical conditions), and multifactorial aspects, including the interplay of health and psychological factors and asthma. These were found to have a substantial impact on QoL in asthma, both directly and indirectly, by affecting self-management, activity levels and other outcomes. Findings suggest a complex and negative effect of health and psychological factors on QoL in asthma. The experience of living with asthma is multifaceted, and future research and intervention development studies should take this into account, as well as the variety of variables interacting and affecting the person.
Similar content being viewed by others
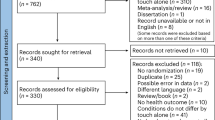
A systematic review and multivariate meta-analysis of the physical and mental health benefits of touch interventions
Julian Packheiser, Helena Hartmann, … Frédéric Michon
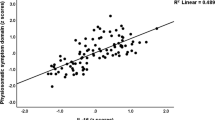
In major dysmood disorder, physiosomatic, chronic fatigue and fibromyalgia symptoms are driven by immune activation and increased immune-associated neurotoxicity
Michael Maes, Abbas F. Almulla, … Pimpayao Sodsai
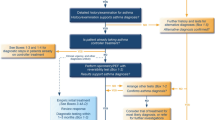
Key recommendations for primary care from the 2022 Global Initiative for Asthma (GINA) update
Mark L. Levy, Leonard B. Bacharier, … Helen K. Reddel
Introduction
Over 235 million people worldwide are living with asthma, which is one of the leading non-communicable diseases worldwide. 1 , 2 Symptoms, exacerbations, and triggers in asthma are associated with lower quality of life (QoL), tiredness, activity limitation, negative effects on social life and relationships, problems with finding and keeping employment, and reduced productivity. 3 , 4 , 5 , 6 , 7 People with asthma are up to six times more likely than the general population to have anxiety or depression, 8 and 16% of people with asthma in the UK have panic disorder, 9 compared to 1% in the general population. 10 People with brittle asthma (difficult-to-control asthma with severe, recurrent attacks) demonstrate even greater comorbidity and maladaptive coping styles. 11 Psychological dysfunction is often unrecognized in primary care, despite being significantly associated with poor asthma outcomes, including asthma control and QoL. 8 , 12 , 13 Indeed, the European Asthma Research and Innovation Partnership has identified understanding the role of psychological factors as an unmet need in improving asthma outcomes. 14 , 15 They propose that anxiety and depression are present at all three stages of the experience of asthma: onset, progression, and exacerbation. 14
A recent meta-analysis found that asthma diagnoses significantly increased the risk of psychological and health conditions (such as cardiovascular/cerebrovascular diseases, obesity, hypertension, diabetes, psychiatric and neurological comorbidities, gut and urinary conditions, cancer, and respiratory problems other than asthma). 16 In addition, studies have pointed towards an impact on QoL in people with asthma of additional health and psychological factors, such as comorbid anxiety or depression, higher body mass index(BMI), professional status, and feelings of lack of control over health (for example, refs 17 , 18 ). Such evidence reinforces the argument that the needs of people with asthma should be approached in conjunction with these additional factors, rather than using a single-illness approach, aiming to reduce the adversity of people’s experience. However, the extent to which psychological and physical health factors interact and impact asthma outcomes is yet to be systematically explored. This systematic review aims to provide a narrative synthesis of the literature exploring psychological and physical health factors that influence QoL in adults with asthma.
Study characteristics
The search and screening process identified 43 eligible papers, published between 2003 and 2019 (see Fig. 1 for PRISMA flowchart 19 ). The characteristics of each study are summarized below in Table 1 . Twelve studies were conducted in Europe, 20 , 21 , 22 , 23 , 24 , 25 , 26 , 27 , 28 , 29 , 30 , 31 17 in North America, 12 , 32 , 33 , 34 , 35 , 36 , 37 , 38 , 39 , 40 , 41 , 42 , 43 , 44 , 45 , 46 , 47 7 in Australia, 17 , 48 , 49 , 50 , 51 , 52 , 53 4 in Asia, 54 , 55 , 56 , 57 and 3 in Africa. 58 , 59 , 60 All papers employed a quantitative approach comprising 2 longitudinal studies 31 , 44 and 41 cross-sectional studies. Only 4 studies included a control group. 21 , 28 , 29 , 31 Overall, the majority of papers had a large sample size (ranging between 40 and 39,321 participants; 30 papers included a sample size of >100). The majority of studies recruited from primary care or the general population, using self-report to confirm a diagnosis of asthma. Only a few studies recruited from secondary and tertiary asthma clinics. 12 , 27 , 36 , 41 , 44 , 48 , 60 There was a high occurrence ( n = 14) of exclusion criteria relating to specific demographic or asthma characteristics, as well as mental health conditions and comorbidities, which restricted the study sample without a reason being given. Most studies used self-report measures, 17 , 20 , 21 , 22 , 23 , 24 , 25 , 26 , 27 , 28 , 29 , 30 , 32 , 33 , 34 , 35 , 36 , 37 , 39 , 41 , 42 , 43 , 44 , 45 , 46 , 48 , 49 , 51 , 52 , 53 , 54 , 55 , 56 , 57 , 58 , 59 , 60 with a small proportion using psychiatric interviews to screen for mental health conditions. 12 , 31 , 38 , 40 , 50 The majority of studies used asthma-specific QoL measures ( n = 29), 12 , 21 , 23 , 25 , 27 , 28 , 30 , 32 , 33 , 34 , 35 , 36 , 37 , 39 , 40 , 41 , 42 , 44 , 48 , 49 , 50 , 51 , 54 , 55 , 56 , 58 , 59 , 60 , 61 17 included an health-related QoL measure ( n = 18), 17 , 20 , 22 , 23 , 24 , 25 , 28 , 30 , 31 , 34 , 35 , 36 , 38 , 43 , 50 , 51 , 52 , 55 and 4 used general measures of QoL ( n = 7); 26 , 35 , 45 , 46 , 47 , 57 , 62 11 papers used >1 measure of QoL. 23 , 25 , 28 , 30 , 34 , 35 , 36 , 37 , 50 , 51 , 55 The average age across included studies was 42.1 years (and 61.57% were female). Papers report prevalence rates of between 16.8% and 48.9% for depression and between 13.3% and 44.4% for anxiety, 20 , 27 , 33 , 38 , 50 , 56 , 58 , 60 with an average of 28.31% for a diagnosis of anxiety or depression. Across several studies, the prevalence of other mental health conditions was 28.31% on average (ranging between 28% and 80%). 12 , 37 , 38 , 40 , 42 Between 72% and 86.9% of people with asthma had at least one additional physical condition and between 21% and 26.3% had ≥2; 25 , 34 , 56 26.36% had, on average, at least one other physical health condition. On average, people with asthma were significantly more likely to have a BMI of >30 (and between 61% and 75.1% had a BMI >25). 26 , 45 , 59 The quality assessment identified that most studies were of a reasonable quality; however, it should be noted that some measures used could be considered inappropriate for the research aim or the population under investigation. Examples include measuring general QoL with an asthma-specific measure or administering a geriatric depression questionnaire to a young adult population.
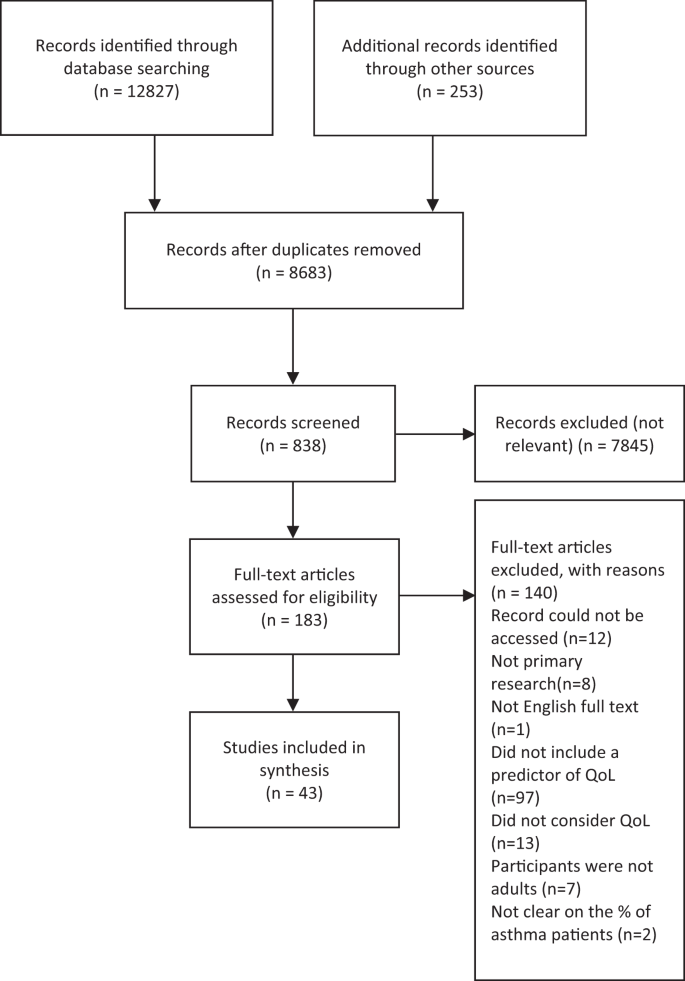
PRISMA statement of included and excluded papers
Narrative synthesis
Narrative synthesis generated three overarching themes: psychological factors, health factors, and multifactorial aspects (see Table 2 for themes and subtheme descriptions). Overall, patients with asthma demonstrated impaired QoL, which was further decreased by psychological factors (e.g. anxiety, depression, emotion regulation, illness perceptions), health risk factors (such as an increased BMI), and the presence of a co-existing mental health or physical condition (such as rhinitis, cardiovascular disease, diabetes, etc.). Having more than one co-existing condition or psychological factor impacted overall QoL even more substantially. Results for each of the aspects found are presented below.
Psychological factors
Within this first theme, four subthemes were generated. These comprised ‘anxiety and depression’, ‘other mental health conditions’, ‘emotional regulation’, and ‘illness representations’.
Anxiety and depression were notably the most commonly considered factors ( n = 30). A high prevalence of people with asthma showed symptoms of or clinical diagnoses of anxiety or depression, which appeared to play a key role in understanding the relationship between asthma and QoL. Overall, having a diagnosis of anxiety or depression was associated with poorer QoL across all dimensions (e.g. activity limitation, physical or mental wellbeing, social or role functioning, etc.), as well as health perceptions. 24 , 36 , 46 , 50 , 54 In particular, one study (of undergraduate students aged 18–25 years, with childhood-onset asthma) found that anxiety was significantly associated with asthma QoL, as was the interaction between anxiety and depression, 32 while others found that generally anxiety and depression both predicted worse QoL independently (refs 12 , 29 , 33 , 38 , 42 , 44 , 56 , 60 ). One study found that the average asthma-related QoL scores for people with asthma and depression were 1.4 times lower compared to people with asthma and no depression. 33 Having current depression or anxiety was associated with worse QoL than was having a lifetime diagnosis; this was in turn was greater than having no depression or anxiety. 45 Having a history of major depression was also significantly associated with worse physical and mental functioning, compared to those with asthma and no depression. 38 There was considerable variability across variance explained, with depression found to account for between 3% 40 and 56% 30 of the variance in QoL, whereas anxiety was found to account for between 2% 40 and 68%. 21
In contrast, one study found that having either a depressive or an anxiety disorder significantly impacted asthma QoL but having both was not significantly different than only having one, 40 which is dissonant with other studies. Another study of 90 people with difficult asthma found that having anxiety or depression had no significant effect on QoL. 48 In addition, although depression was associated with poorer QoL, it did not inflate the relationship between asthma severity and QoL. 29 All other studies were significant but showed only small-to-moderate effect sizes. Having a full clinical diagnosis of anxiety or depression was not significantly worse (in terms of QoL) than having only some symptoms of anxiety and depression.
Studies also considered the impact of anxiety and depression on specific subdomains of QoL and asthma-specific QoL. Having anxiety was not associated with physical functioning, mental health or health perception, 38 or the physical component of QoL. 20 Depression, however, was associated with significantly poorer QoL on physical dimensions and activity limitation, 20 , 21 , 23 , 30 , 38 , 45 , 53 , 55 , 58 although one study found significant results only for participants with uncontrolled asthma. 22 In relation to asthma-specific QoL, depression and anxiety were significantly associated with decreased asthma-specific QoL. 17 , 21 , 23 , 27 , 28 , 32 , 33 , 36 , 37 , 40 , 50 , 54 , 55 , 58 , 61
Nine studies looked at other mental health conditions, such as panic disorder with or without agoraphobia, 24 , 38 , 44 , 57 personality disorders, 31 alexithymia, 23 somatization, 38 mood disorders, 12 , 40 , 57 schizophrenia, eating disorders, substance use disorders, 38 and general occurrence of any psychiatric disorder. 12 , 17 The results in this subtheme were mixed, but overall they suggest that the presence of an additional mental health condition is significantly associated with a decrease in QoL in patients with asthma. 12 , 17 Panic disorder was also shown to be both significantly 24 and non-significantly 57 associated with poorer mental and physical components of QoL. Alexithymia in people with asthma was not associated with poorer QoL. 23 Having asthma and a personality disorder was associated with lower general QoL, 31 as well as lower scores for physical health, vitality, pain, general health, social function, mental health, and emotional role (physical function was not significant). This association was not found for people without asthma, suggesting that it is the combination of conditions (asthma and co-existing mental health conditions) that may lead to the negative impact on QoL. 31
The emotion regulation subtheme included studies that explored the relationship between emotional states, negative affect (not related to anxiety, depression, or other mental health conditions), or coping and QoL in people with asthma. QoL in asthma was found to be influenced by affect and a predisposition to negative states, as found by four studies. 28 , 39 , 41 , 51 For instance, a model of age, gender, negative affect, and medical problems accounted for 20% of symptoms and 23% of activity limitation. 39 This was supported by findings that negative mood is associated with poor scores on both the mental and physical components of the Asthma Quality of Life Questionnaires (AQLQ), 28 as well as a positive correlation between active coping and asthma QoL. 51 Despite heterogeneity, the impaired QoL was associated with impulsive-careless coping 41 and avoidant coping. 51 Overall, the presence of psychological distress seemed to affect people with asthma more than people without asthma in terms of QoL.
Illness-related cognitions are people’s patterns of beliefs about the characteristics of their conditions, which in turn influence their appraisal of severity and can determine future behaviours. 63 A number of illness-related cognitions and perceptions significantly predicted QoL in seven studies. 26 , 34 , 37 , 42 , 43 , 51 , 60 For instance, asthma self-efficacy 42 was positively associated with QoL. However, decreased QoL was significantly predicted by a series of varied illness perceptions: subjective illness severity, uncertainty in illness, illness intrusiveness, 43 perceived disability, 60 health beliefs and attitudes, 34 perceived severity, 34 level of confidence or self-efficacy in managing asthma, 51 satisfaction with illness, 51 anxiety sensitivity for physical concerns, 39 and satisfaction with life. 37 In addition, a model of subjective and objective illness severity accounted for 24% of the variance in QoL, further supporting the effect of illness perceptions on QoL. 34
Physical health factors
Two subthemes were generated in the physical health factors theme: additional physical conditions and BMI.
Ten papers examined additional physical conditions in relation to QoL in asthma; 25 , 27 , 34 , 39 , 46 , 47 , 48 , 49 , 52 , 53 most only referred to ‘comorbidity’ or ‘medical problems’ as a measure of frequency of additional conditions. 34 , 36 , 39 Some studies looked at both general and individual co-existing conditions 25 , 48 , 52 and others counted chronic conditions but did not include them in further analyses. 33 , 36 , 56 , 59 Of the ones that did explore individual conditions, the highest impact seemed to be provoked by musculoskeletal conditions. 25 Similarly, statistically and clinically significant decreases in activity levels were also found for people with asthma and multimorbid conditions. 52 Other conditions investigated included respiratory conditions, 47 diabetes, 25 , 48 obesity, 48 hypertension, 25 , 39 gastro-oesophageal reflux disorder, 48 rhinitis, 48 , 49 vocal cord dysfunction, 48 sleep apnoea, 48 musculoskeletal disorders, 25 , 39 arthritis, 39 , 52 heart disease, 25 stroke, 39 , 52 cancer, 39 , 52 osteoporosis, 52 dysfunctional breathing, 48 headaches, 39 and allergic status. 27 , 39 The consensus was that having an additional physical condition significantly decreased QoL in asthma, the effect being amplified with the addition of further conditions.
Eleven papers exploring BMI found that it consistently influenced QoL for people with asthma both directly as a multimorbid factor and indirectly by increasing the chance of additional conditions and activity limitation. 25 , 26 , 28 , 29 , 35 , 42 , 44 , 45 , 48 , 56 , 59 In particular, one study found that generic health status decreased for overweight and obese patients with asthma. People with asthma with obesity had on average 5.05 more restricted activity days than people without obesity or without asthma. 35 Other studies found that increased BMI was an independent factor in predicting poorer QoL 48 and that QoL was two times worse in overweight and three times worse in obese people with asthma. 59 In contrast, one study found that overweight BMI made no difference; however, being obese did. 27 Almost ½ of obese patients and 25% overweight patients had problems with mobility, pain, discomfort, self-care, and usual activities (compared to <15% people with asthma of normal weight). 26
Multifactorial aspects
Seven studies included statistical analyses to explore potential mechanisms for the relationship between asthma QoL and additional physical conditions, BMI, and psychological factors. 17 , 35 , 42 , 45 , 50 , 56 , 59 Results from studies in this group are complex, indicating that people with asthma are at a higher risk of adverse outcomes (such as exacerbated symptoms or decreased QoL) if they also have a high BMI and depression. 35 , 42 , 56 , 59 People with current depression and asthma are more likely to be obese and 3.9 times more likely to report fair or poor general health. 45 A few of these studies have explored the relationship between these factors further. For example, people with asthma and obesity were more likely to have additional physical comorbidities and poorer QoL. 59 Significant increases in major depression were associated with dyspnoea, 50 and depression and perceived control of asthma significantly mediated between BMI and QoL. 35 Higher BMI has also been associated with worse asthma-specific self-efficacy, which was in turn associated with decreased QoL. 42
The aim of the present review was to synthesise the literature exploring health and psychological factors that influence QoL in adults with asthma. Previous evidence shows that QoL is generally lower in people with asthma and compounded by poor asthma control and severity. 13 The narrative synthesis in the present study builds on this by identifying three themes, encompassing a number of factors that substantially explain further impairment in QoL for people with asthma. These were not limited to individual components but also combinations of co-existing conditions, risk factors, and health and psychological factors, which consistently showed a negative impact on QoL.
Anxiety and depression were the most commonly reported psychological factors associated with impaired QoL, but effects were also found for other mental health conditions, illness representations, and emotion regulation. These results are generally consistent with previous research showing not only that among people with asthma there are more people with depression than without 8 but also with an increase in depression, the risk of asthma increased. 64 Although the relationship between anxiety and depression and asthma-specific QoL were not further considered in the primary sources, they point towards either a link with activity limitation or a cumulative impact of the interaction between these psychological factors, which in turn affect the QoL of people with asthma. In addition, it is argued that people with asthma use more emotion-focused, and generally maladaptive, coping strategies, such as avoidance. 65 Despite this, psychotherapy, such as cognitive-behavioural therapy and counselling has had limited effectiveness in improving asthma outcomes. 66
Physical health factors, such as high BMI and co-occurring health conditions, were extremely common in people with asthma, consistent with existing literature. 16 This affects QoL both directly and indirectly, affecting self-management and illness perceptions. As such, non-pharmacological treatments such as lifestyle change and activity promotion could prove effective. For instance, a higher proportion of people with asthma seem to have overweight or obese BMI 67 and weight loss intervention studies have been associated with improvements in asthma symptoms. 68
One of the fundamental components of reduced QoL is activity limitation, which is especially relevant to people with asthma, with or without additional conditions or psychological risk factors. This has been widely acknowledged by previous research, to the extent that it has been included as one of the components of asthma-related QoL measures, such as the AQLQ. 69 Furthermore, it is not surprising that decreased QoL in adults with asthma is associated with depression or high BMI, both of which have been consistently associated with activity limitation (e.g. refs 70 , 71 ). In addition, depression was found to affect QoL on the physical components as well as the mental ones, which has interesting implications for future research and clinical practice.
It is important to note the high prevalence of anxiety, depression, and chronic conditions, despite frequent exclusion of comorbid psychiatric conditions. This was found throughout the included papers and is consistent with previous research (e.g. refs 8 , 16 ). This does not only mean that psychological and health factors significantly add to the burden of living with asthma but also that the occurrence of psychological dysfunction and health risk factors seem to be common in people with asthma. In addition, the complex nature of patients with chronic diseases such as asthma, with factors interacting, adds to the negative experience of living with asthma. Results are similar to previous meta-analyses and reviews, 8 , 72 pointing towards conclusive evidence that additional factors (physical or psychological) decrease QoL and functionality in asthma. Finally, these effects were consistent, regardless of the measure of QoL used (asthma specific, health related, or general). This suggests that the identified factors may affect people with asthma more than people without asthma or that the cumulative impact of comorbidities is greater than arithmetically assumed.
The quality of the present review needs to be discussed in relation to the methodology and robustness of the synthesis, determined by the quantity and quality of individual studies included. 73 The quality assessment identified that most studies were of a reasonable quality overall, although all papers had one or two elements that were of a slightly lower quality (this included aspects such as recruitment from only one hospital reducing generalizability or self-report vs objective measurement of weight for BMI calculations). However, this was not problematic for the purposes of this review as the focus was to identify potential factors considered in research rather than classify the methodological quality used to measure their impact on QoL. In addition, the search terms in this review could have limited the number and kind of studies included. For instance, not every potential comorbid condition was listed. This could be a focus for future research. Socio-demographic factors were not included, which can be considered a limitation; however, the breadth of the area was deemed too much for the scope of the present review and could also be the focus of future research. The majority of included studies were observational and as such could not be used to determine causal mechanisms. However, the aim of this review was only to identify potential factors involved in decreased QoL in asthma, rather than build a causal model. Similarly, the impact of individual factors was not measured and could be explored in future research.
A strength of the present review is that it uses a novel approach to QoL in asthma, by systematically taking into account additional aspects that influence the experience of living with asthma and impact QoL. Results suggest both a direct association of the identified aspects, as well as indirectly through interactions with other aspects of living with asthma, such as overarching illness perceptions and activity limitation. The present review emphasizes some interesting and novel findings for asthma and QoL research. Three main implications for future research and practice are proposed. First, for future research, the findings of this review should be used to further explore and understand the factors impacting QoL in people with asthma. It is crucial to explore the needs and experience of patients with complex medical problems, in order to unpick the different factors impacting on QoL. Second, the results are relevant for practitioners, particularly in primary care, as they draw attention to the prevalence of various physical and mental health factors that can interact and affect asthma outcomes. This could influence training or guidelines on potential factors to consider during appointments and consultations. Finally, most current non-pharmacological interventions for patients with chronic conditions tend to overlook the complex needs of patients in a multimorbidity context. As such, it is suggested that future intervention development should use a personalized, tailored approach that aims to address the needs of patients with complex medical problems in the wider context of their experience of living with asthma.
This review demonstrates that the themes and factors identified through inductive narrative synthesis illustrate that QoL in asthma cannot be determined in a simplistic way. The findings suggest a complex experience in living with asthma, one that has a stronger impact on QoL than the sum its of parts. People with asthma and their QoL cannot be viewed separately from the psychological and other health elements that they experience. Future research is encouraged to take a function-oriented approach to QoL in asthma, including management of multimorbid conditions when planning studies; clinical practice should also acknowledge the additional and complex needs of people with asthma by offering relevant, person-based tailored interventions.
Search strategy
The initial search was carried out in April 2017 and was updated in January 2019. Databases searched included MEDLINE, EMBASE, PsycINFO, the Cochrane Library, and Web of Science. Search terms used comprised a combination of the following key terms: asthma (MESH term), psychological/psychosocial and factor/determinant/predictor, comorbid, multimorbid, anxiety, depression, illness perception, illness cognition, illness representation, locus of control, self-efficacy, risk factor, quality of life, health-related quality of life, wellbeing, distress, health status, burden. In addition, a hand search of all the references of included papers was performed as well as a grey literature search on Google Scholar.
Study selection
Studies were included if they investigated psychological or physical health factors and included QoL in adults with asthma as primary or secondary outcome. Psychological factors were considered any modifiable factors, including thoughts, beliefs, attitudes, or emotions of people with asthma, as well as the presence of any co-occurring mental health condition. Physical health factors were defined as any physical comorbid or multimorbid condition or risk factor. These were chosen to allow as much inclusivity as possible and to reflect the exploratory nature of this review. Intervention studies were excluded, as they rarely considered the impact of health or psychological factors on QoL but rather investigated how interventions improved asthma outcomes. Studies were excluded if they were conference abstracts, reviews, or not primary research or the full text not in English, German, or Spanish language.
Data extraction and quality appraisal
Data extracted comprised authors, year of publication, study sample, predictors, QoL measurement (outcome), and findings. The AXIS tool 74 was used to assess the quality of included papers. This contains questions on study design, sample size justification, target population, sampling frame, sample selection, measurement validity and reliability, and overall methods and does not offer a numerical scale. No papers were excluded or weighted based on the quality assessment.
Data synthesis
Owing to heterogeneity of QoL measures and the range of variables used in the included studies, narrative synthesis was used to describe and group similar findings, explore patterns identified in the literature, and develop a narrative account of the results. 73 This is an approach to systematic reviews involving the synthesis of findings from multiple sources and relies primarily on word and text to summarise the findings.
All data generated or analysed during this study are included in this published article.
Reporting summary
Further information on research design is available in the Nature Research Reporting Summary linked to this article.
World Health Organization. Chronic Respiratory Diseases - Asthma http://www.who.int/respiratory/asthma/en/ (2018).
ASTHMA UK. Asthma Facts and Statistics https://www.asthma.org.uk/about/media/facts-and-statistics/ (2018).
Pickles, K. et al. “This illness diminishes me. What it does is like theft”: a qualitative meta‐synthesis of people’s experiences of living with asthma. Health Expectations 21 , 23–40 (2018).
Article PubMed Google Scholar
Goeman, D. P. & Douglass, J. A. Understanding asthma in older Australians: a qualitative approach. Med. J. Aust. 183 , S26–S27 (2005).
PubMed Google Scholar
Juniper, E. F. How important is quality of life in pediatric asthma? Pediatr. Pulmonol. 24 , 17–21 (1997).
Article Google Scholar
Accordini, S. et al. The socio‐economic burden of asthma is substantial in Europe. Allergy 63 , 116–124 (2008).
Article CAS PubMed Google Scholar
Goeman, D. P. et al. Patients’ views of the burden of asthma: a qualitative study. Med. J. Aust. 177 , 295–299 (2002).
Thomas, M., Bruton, A., Moffat, M. & Cleland, J. Asthma and psychological dysfunction. Prim. Care Respir. J. 20 , 250–256 (2011).
Article PubMed PubMed Central Google Scholar
Goodwin, R. D., Fergusson, D. M. & Horwood, L. J. Asthma and depressive and anxiety disorders among young persons in the community. Psychol. Med. 34 , 1465–1474 (2004).
Jenkins, R. et al. The national psychiatric morbidity surveys of Great Britain–strategy and methods. Psychol. Med. 27 , 765–774 (1997).
Miles, J., Garden, G., Tunnicliffe, W., Cayton, R. & Ayres, J. Psychological morbidity and coping skills in patients with brittle and non‐brittle asthma: a case‐control study. Clin. Exp. Allergy 27 , 1151–1159 (1997).
Lavoie, K. L. et al. Are psychiatric disorders associated with worse asthma control and quality of life in asthma patients? Respir. Med. 99 , 1249–1257 (2005).
Juniper, E. F., Guyatt, G. H., Ferrie, P. J. & Griffith, L. E. Measuring quality of life in asthma. Am. Rev. Respir. Dis. 147 , 832–832 (1993).
Edwards, M. R. et al. Addressing unmet needs in understanding asthma mechanisms. Eur. Respir. J. 49 , 1602448 (2017).
Masefield, S. et al. The future of asthma research and development: a roadmap from the European Asthma Research and Innovation Partnership (EARIP). Eur. Respir. J. 49 (2017).
Su, X. et al. Prevalence of comorbidities in asthma and nonasthma patients: a meta-analysis. Medicine 95 , e3459 (2016).
Adams, R. J. et al. Psychological factors and asthma quality of life: a population based study. Thorax 59 , 930–935 (2004).
Article CAS PubMed PubMed Central Google Scholar
Leynaert, B., Neukirch, C., Liard, R., Bousquet, J. & Neukirch, F. Quality of life in allergic rhinitis and asthma: a population-based study of young adults. Am. J. Respir. Crit. Care Med. 162 , 1391–1396 (2000).
Moher, D., Liberati, A., Tetzlaff, J., Altman, D. G. & Group, P. Preferred reporting items for systematic reviews and meta-analyses: the PRISMA statement. PLoS Med. 6 , e1000097 (2009).
Bohmer, M. M. et al. Factors associated with generic health-related quality of life in adult asthma patients in Germany: cross-sectional study. J. Asthma 54 , 325–334 (2017).
Oğuztürk, Ö. et al. Psychological status and quality of life in elderly patients with asthma. Psychosomatics 46 , 41–46 (2005).
Lomper, K., Chudiak, A., Uchmanowicz, I., Rosinczuk, J. & Jankowska-Polanska, B. Effects of depression and anxiety on asthma-related quality of life. Pneumonol. Alergol. Pol. 84 , 212–221 (2016).
Vazquez, I. et al. The role of alexithymia in quality of life and health care use in asthma. J. Asthma 47 , 797–804 (2010).
Sández, E. et al. Depression, panic-fear, and quality of life in near-fatal asthma patients. J. Clin. Psychol. Med. Settings 12 , 175–184 (2005).
Wijnhoven, H. A., Kriegsman, D. M., Hesselink, A. E., de Haan, M. & Schellevis, F. G. The influence of co-morbidity on health-related quality of life in asthma and COPD patients. Respir. Med. 97 , 468–475 (2003).
Gonzalez-Barcala, F.-J., de la Fuente-Cid, R., Tafalla, M., Nuevo, J. & Caamano-Isorna, F. Factors associated with health-related quality of life in adults with asthma. A cross-sectional study. Multidiscip. Respir. Med. 7 , 32 (2012).
Coban, H. & Aydemir, Y. The relationship between allergy and asthma control, quality of life, and emotional status in patients with asthma: a cross-sectional study. Allergy Asthma Clin. Immunol. 10 , 67 (2014).
Ekici, A., Ekici, M., Kara, T., Keles, H. & Kocyigit, P. Negative mood and quality of life in patients with asthma. Qual. Life Res. 15 , 49–56 (2006).
Al-Kalemji, A. et al. Factors influencing quality of life in asthmatics - a case-control study. Clin. Respir. J. 7 , 288–296 (2013).
Kullowatz, A., Kanniess, F., Dahme, B., Magnussen, H. & Ritz, T. Association of depression and anxiety with health care use and quality of life in asthma patients. Respir. Med. 101 , 638–644 (2007).
Yilmaz, A., Cumurcu, B. E., Etikan, I., Hasbek, E. & Doruk, S. The effect of personality disorders on asthma severity and quality of life. Iran. J. Allergy Asthma Immunol. 13 , 47–54 (2014).
Hommel, K. A., Chaney, J. M., Wagner, J. L. & McLaughlin, M. S. Asthma-specific quality of life in older adolescents and young adults with long-standing asthma: the role of anxiety and depression. J. Clin. Psychol. Med. Settings 9 , 185–192 (2002).
Krauskopf, K. A. et al. Depressive symptoms, low adherence, and poor asthma outcomes in the elderly. J. Asthma 50 , 260–266 (2013).
Erickson, S. R., Christian, R. D. Jr, Kirking, D. M. & Halman, L. J. Relationship between patient and disease characteristics, and health-related quality of life in adults with asthma. Respir. Med. 96 , 450–460 (2002).
Vortmann, M. & Eisner, M. D. BMI and health status among adults with asthma. Obesity (Silver Spring) 16 , 146–152 (2008).
Mancuso, C. A., Peterson, M. G. E. & Charlson, M. E. Effects of depressive symptoms on health-related quality of life in asthma patients. J. Gen. Intern. Med. 15 , 301–310 (2000).
Miedinger, D., Lavoie, K. L., L’Archeveque, J., Ghezzo, H. & Malo, J.-L. Identification of clinically significant psychological distress and psychiatric morbidity by examining quality of life in subjects with occupational asthma. Health Qua. Life Outcomes 9 , 76 (2011).
Afari, N., Schmaling, K. B., Barnhart, S. & Buchwald, D. Psychiatric comorbidity and functional status in adult patients with asthma. J. Clin. Psychol. Med. Settings 8 , 245–252 (2001).
Avallone, K. M., McLeish, A. C., Luberto, C. M. & Bernstein, J. A. Anxiety sensitivity, asthma control, and quality of life in adults with asthma. J. Asthma 49 , 57–62 (2012).
Lavoie, K. L. et al. What is worse for asthma control and quality of life - depressive disorders, anxiety disorders, or both? Chest 130 , 1039–1047 (2006).
McCormick, S. P. et al. Coping and social problem solving correlates of asthma control and quality of life. Chron. Respir. Dis. 11 , 15–21 (2014).
Lavoie, K. L. et al. Association of asthma self-efficacy to asthma control and quality of life. Ann. Behav. Med. 36 , 100–106 (2008).
Hullmann, S. E., Eddington, A. R., Molzon, E. S. & Mullins, L. L. Illness appraisals and health-related quality of life in adolescents and young adults with allergies and asthma. Int. J. Adolesc. Med. Health 25 , 31–38 (2013).
Favreau, H., Bacon, S. L., Labrecque, M. & Lavoie, K. L. Prospective impact of panic disorder and panic-anxiety on asthma control, health service use, and quality of life in adult patients with asthma over a 4-year follow-up. Psychosom. Med. 76 , 147–155 (2014).
Strine, T. W., Mokdad, A. H., Balluz, L. S., Berry, J. T. & Gonzalez, O. Impact of depression and anxiety on quality of life, health behaviors, and asthma control among adults in the United States with asthma, 2006. J. Asthma 45 , 123–133 (2008).
Pate, C. A., Zahran, H. S. & Bailey, C. M. Impaired health-related quality of life and related risk factors among US adults with asthma. J. Asthma 56, 431–439 (2018).
Urbstonaitis, R., Deshpande, M. & Arnoldi, J. Asthma and health related quality of life in late midlife adults. Res. Soc. Adm. Pharm. 15 , 61–69 (2019).
Tay, T. R. et al. Comorbidities in difficult asthma are independent risk factors for frequent exacerbations, poor control and diminished quality of life. Respirology 21 , 1384–1390 (2016).
Powell, H. et al. Rhinitis in pregnant women with asthma is associated with poorer asthma control and quality of life. J. Asthma 52 , 1023–1030 (2015).
Goldney, R. D., Ruffin, R., Fisher, L. J. & Wilson, D. H. Asthma symptoms associated with depression and lower quality of life: a population survey. Med. J. Aust. 178 , 437–441 (2003).
Adams, R. J., Wilson, D., Smith, B. J. & Ruffin, R. E. Impact of coping and socioeconomic factors on quality of life in adults with asthma. Respirology 9 , 87–95 (2004).
Adams, R. J. et al. Coexistent chronic conditions and asthma quality of life: a population-based study. Chest J. 129 , 285–291 (2006).
Deshmukh, V. M., Toelle, B. G., Usherwood, T., O’Grady, B. & Jenkins, C. R. The association of comorbid anxiety and depression with asthma-related quality of life and symptom perception in adults. Respirology 13 , 695–702 (2008).
Oga, T. et al. Analysis of longitudinal changes in the psychological status of patients with asthma. Respir. Med. 101 , 2133–2138 (2007).
Nishimura, K., Hajiro, T., Oga, T., Tsukino, M. & Ikeda, A. Health related quality of life in stable asthma: what are remaining quality of life problems in patients with well-controlled asthma? J. Asthma 41 , 57–65 (2004).
Choi, G.-S. et al. Prevalence and risk factors for depression in korean adult patients with asthma: is there a difference between elderly and non-elderly patients? J. Korean Med. Sci. 29 , 1626–1631 (2014).
Faye, A. D. et al. Do panic symptoms affect the quality of life and add to the disability in patients with bronchial asthma? Psychiatry J. 2015 , 608351–608351 (2015).
Kolawole, M. S. et al. Health related quality of life and psychological variables among a sample of asthmatics in Ile-Ife South-Western Nigeria. Libyan J. Med. 6 , 1–5 (2011).
Maalej, S. et al. Association of obesity with asthma severity, control and quality of life. Tanaffos 11 , 38 (2012).
CAS PubMed PubMed Central Google Scholar
Adeyeye, O. O., Adewumi, T. A. & Adewuya, A. O. Effect of psychological and other factors on quality of life amongst asthma outpatients in Lagos, Nigeria. Respir. Med. 122 , 67–70 (2017).
Deshmukh, V. M., Toelle, B. G., Usherwood, T., O’grady, B. & Jenkins, C. R. The association of comorbid anxiety and depression with asthma‐related quality of life and symptom perception in adults. Respirology 13 , 695–702 (2008).
Al-kalemji, A. et al. Factors influencing quality of life in asthmatics–a case-control study. Clin. Respir. J. 7 , 288–296 (2013).
Petrie, K. & Weinman, J. Why illness perceptions matter. Clin. Med. 6 , 536–539 (2006).
Gerald, J. K. & Moreno, F. A. Asthma and depression: it’s complicated. J. Allergy Clin. Immunol. Pract. 4 , 74–75 (2016).
Barton, C., Clarke, D., Sulaiman, N. & Abramson, M. Coping as a mediator of psychosocial impediments to optimal management and control of asthma. Respir. Med. 97 , 747–761 (2003).
Yorke, J., Fleming, S., Shuldham, C., Rao, H. & Smith, H. Nonpharmacological interventions aimed at modifying health and behavioural outcomes for adults with asthma: a critical review. Clin. Exp. Allergy 45 , 1750–1764 (2015).
Lavoie, K. L., Bacon, S. L., Labrecque, M., Cartier, A. & Ditto, B. Higher BMI is associated with worse asthma control and quality of life but not asthma severity. Respir. Med. 100 , 648–657 (2006).
Pakhale, S., Baron, J., Dent, R., Vandemheen, K. & Aaron, S. D. Effects of weight loss on airway responsiveness in obese adults with asthma: does weight loss lead to reversibility of asthma? Chest 147 , 1582–1590 (2015).
Juniper, E., Guyatt, G., Cox, F., Ferrie, P. & King, D. Development and validation of the mini asthma quality of life questionnaire. Eur. Respir. J. 14 , 32–38 (1999).
Breslin, F. C., Gnam, W., Franche, R.-L., Mustard, C. & Lin, E. Depression and activity limitations: examining gender differences in the general population. Soc. Psychiatry Psychiatr. Epidemiol. 41 , 648–655 (2006).
Hassan, M., Joshi, A., Madhavan, S. & Amonkar, M. Obesity and health-related quality of life: a cross-sectional analysis of the US population. Int. J. Obes. 27 , 1227–1232 (2003).
Article CAS Google Scholar
Fortin, M. et al. Multimorbidity and quality of life in primary care: a systematic review. Health Qual. Life Outcomes 2 , 51 (2004).
Popay, J. et al. Guidance on the Conduct of Narrative Synthesis in Systematic Reviews. A Product from the ESRC Methods Program. Version 1 (Lancaster University, 2006).
Downes, M. J., Brennan, M. L., Williams, H. C. & Dean, R. S. Development of a critical appraisal tool to assess the quality of cross-sectional studies (AXIS). BMJ Open 6 , e011458 (2016).
Download references
Author information
Authors and affiliations.
Academic Unit of Psychology, University of Southampton, Southampton, UK
Sabina Stanescu, Sarah E. Kirby & Lucy Yardley
NIHR Southampton Respiratory Biomedical Research Unit, University of Southampton, Southampton, UK
Sarah E. Kirby & Mike Thomas
Primary Care and Population Sciences, University of Southampton, Southampton, UK
Mike Thomas
Department of Psychology, University of Bath, Bath, UK
Ben Ainsworth
You can also search for this author in PubMed Google Scholar
Contributions
S.S.—conception of the review, synthesis, wrote the first draft, commented on drafts. B.A. and S.K.—conception of the review and day-to-day conduct of the review, commented on drafts, updated the review, revised the paper. M.T.—conception of the review, commented on drafts. L.Y.—conception of the review, commented on drafts. All authors read and approved the final version of the manuscript.
Corresponding author
Correspondence to Sabina Stanescu .
Ethics declarations
Competing interests.
The authors declare no competing interests.
Additional information
Publisher’s note Springer Nature remains neutral with regard to jurisdictional claims in published maps and institutional affiliations.
Supplementary information
Reporting summary, rights and permissions.
Open Access This article is licensed under a Creative Commons Attribution 4.0 International License, which permits use, sharing, adaptation, distribution and reproduction in any medium or format, as long as you give appropriate credit to the original author(s) and the source, provide a link to the Creative Commons license, and indicate if changes were made. The images or other third party material in this article are included in the article’s Creative Commons license, unless indicated otherwise in a credit line to the material. If material is not included in the article’s Creative Commons license and your intended use is not permitted by statutory regulation or exceeds the permitted use, you will need to obtain permission directly from the copyright holder. To view a copy of this license, visit http://creativecommons.org/licenses/by/4.0/ .
Reprints and permissions
About this article
Cite this article.
Stanescu, S., Kirby, S.E., Thomas, M. et al. A systematic review of psychological, physical health factors, and quality of life in adult asthma. npj Prim. Care Respir. Med. 29 , 37 (2019). https://doi.org/10.1038/s41533-019-0149-3
Download citation
Received : 29 April 2019
Accepted : 24 September 2019
Published : 21 October 2019
DOI : https://doi.org/10.1038/s41533-019-0149-3
Share this article
Anyone you share the following link with will be able to read this content:
Sorry, a shareable link is not currently available for this article.
Provided by the Springer Nature SharedIt content-sharing initiative
This article is cited by
A systematic review of school transition interventions to improve mental health and wellbeing outcomes in children and young people.
- Caitlyn Donaldson
- Graham Moore
- Jemma Hawkins
School Mental Health (2023)
Two meta-analyses of the association between atopic diseases and core symptoms of attention deficit hyperactivity disorder
- Yu-Chieh Chuang
- Ching-Yun Wang
- Yu-Jui Huang
Scientific Reports (2022)
Asthma and early smoking associated with high risk of panic disorder in adolescents and young adults
- Meng-Huan Wu
- Wei-Er Wang
- Vincent Chin-Hung Chen
Social Psychiatry and Psychiatric Epidemiology (2022)
Health-Related Disparities in the Metropolitan Region Ruhr: Large-Scale Spatial Model of Local Asthma Prevalence, Accessibility of Health Facilities, and Socioeconomic and Environmental Factors
- Annette Ortwein
- Andreas P. Redecker
- Nicolai Moos
PFG – Journal of Photogrammetry, Remote Sensing and Geoinformation Science (2022)
Obesity, Inflammation, and Severe Asthma: an Update
- Varun Sharma
- Douglas C. Cowan
Current Allergy and Asthma Reports (2021)
Quick links
- Explore articles by subject
- Guide to authors
- Editorial policies
Sign up for the Nature Briefing newsletter — what matters in science, free to your inbox daily.

SYSTEMATIC REVIEW article
Exercise promotes brain health: a systematic review of fnirs studies.
- College of P. E. and Sports, Beijing Normal University, Beijing, China
Exercise can induce brain plasticity. Functional near-infrared spectroscopy (fNIRS) is a functional neuroimaging technique that exploits cerebral hemodynamics and has been widely used in the field of sports psychology to reveal the neural mechanisms underlying the effects of exercise. However, most existing fNIRS studies are cross-sectional and do not include exercise interventions. In addition, attributed to differences in experimental designs, the causal relationship between exercise and brain functions remains elusive. Hence, this systematic review aimed to determine the effects of exercise interventions on alterations in brain functional activity in healthy individuals using fNIRS and to determine the applicability of fNIRS in the research design of the effects of various exercise interventions on brain function. Scopus, Web of Science, PubMed, CNKI, Wanfang, and Weipu databases were searched for studies published up to June 15, 2021. This study was performed in accordance with the PRISMA guidelines. Two investigators independently selected articles and extracted relevant information. Disagreements were resolved by discussion with another author. Quality was assessed using the Cochrane risk-of-bias method. Data were pooled using random-effects models. A total of 29 studies were included in the analysis. Our results indicated that exercise interventions alter oxygenated hemoglobin levels in the prefrontal cortex and motor cortex, which are associated with improvements in higher cognitive functions (e.g., inhibitory control and working memory). The frontal cortex and motor cortex may be key regions for exercise-induced promotion of brain health. Future research is warranted on fluctuations in cerebral blood flow during exercise to elucidate the neural mechanism underlying the effects of exercise. Moreover, given that fNIRS is insensitive to motion, this technique is ideally suited for research during exercise interventions. Important factors include the study design, fNIRS device parameters, and exercise protocol. The examination of cerebral blood flow during exercise intervention is a future research direction that has the potential to identify cortical hemodynamic changes and elucidate the relationship between exercise and cognition. Future studies can combine multiple study designs to measure blood flow prior to and after exercise and during exercise in a more in-depth and comprehensive manner.
1 Introduction
Exercise intervention is a convenient and adaptive approach to effectively enhance the cognitive function and emotion of individuals ( Verburgh et al., 2014 ; Kawagoe et al., 2017 ). Indeed, an increasing number of studies have demonstrated its beneficial effects on the healthy development of brain function ( Mandolesi et al., 2018 ; Chen, 2020 ). Recent studies have predominantly focused on the variations in cognitive function and brain functional activity, such as cerebral blood flow, before and after exercise intervention ( Fujihara et al., 2021 ; Kim et al., 2021 ; Zhang et al., 2021 ). Exploring real-time alterations in cerebral blood flow during exercise interventions can reveal hemodynamic changes ( Endo et al., 2013 ; Eggenberger et al., 2016 ; Carius et al., 2020 ) and execution ( Chen et al., 2017 ; Coetsee and Terblanche, 2017 ; Yang et al., 2020 ) and enhance our understanding of the mechanism underlying the effects of exercise on the brain.
The development of functional near-infrared spectroscopy (fNIRS) has enabled the exploration of hemodynamic changes in cerebral blood flow during exercise interventions. Specifically, it allows non-invasive monitoring of brain tissue oxygenation and hemodynamics ( Hoshi, 2005 ) and possesses distinct advantages over other neuroimaging modalities, such as electroencephalography (EEG) and functional magnetic resonance imaging (fMRI). In addition, it balances both temporal resolution and spatial resolution and is comparatively less sensitive to motion ( Leff et al., 2011 ; Scarapicchia et al., 2017 ). Previous exercise intervention studies using fNIRS devices largely focused on exercise interventions such as walking ( Hamacher et al., 2015 ), posture, and walking ( Herold et al., 2017 ), which are practical within the laboratory setting. Given the diversity in experimental designs, the effects of exercise on the brain exhibit substantial variability.
The application of fNIRS in the field of sport and exercise psychology is heterogeneous due to variations in the utilization of fNIRS and experimental design. Therefore, to improve uniformity across different studies investigating the influence of exercise on brain functional activity, this review aimed to examine studies that employed near-infrared spectroscopy to detect changes in brain hemodynamics before, during, and after exercise. The purpose of this review was as follows: (1) offer recommendations regarding study designs and research related to fNIRS technology in exercise intervention studies; (2) analyze the designs of various exercise protocols and compare the results obtained after or during exercise; and (3) evaluate the characteristics of changes in cerebral blood flow after and during exercise. Overall, the objective of this review was to investigate the effects of various exercise interventions on alterations in brain functional activity from different perspectives (before and after exercise vs. during exercise).
This systematic review was performed and reported in accordance with the Preferred Reporting Items for Systematic Reviews and Meta-Analysis (PRISMA) guidelines ( Page et al., 2021 ) and the Cochrane Collaboration Handbook ( Higgins et al., 2019 ).
2.1 Search strategy
Two reviewers (J.M.H. and T.X.) conducted an independent literature search to screen related studies. The third reviewer, Q.Q.S., resolved disagreements by arbitration.
Scopus, Web of Science, PubMed, CNKI, Wanfang, and Weipu databases were searched from inception to June 15, 2021. The keywords were ( Verburgh et al., 2014 ) exercise (physical activity, exercise, fitness, and sport) and (2) fNIRS (functional near-infrared spectroscopy). These terms were consistently applied across each database, serving as the main topic and free-text words in the title.
2.2 Eligibility criteria
Studies were considered eligible if they fulfilled the following criteria: (1) the subjects were healthy; (2) the articles were published in the English language or Chinese language in peer-reviewed journals; (3) exercise-related intervention studies utilizing large muscle groups of the whole body; and (4) at least one cerebral cortical blood flow change was assessed using fNIRS.
Our review focused on the effect of exercise interventions on common healthy participants. The exclusion criteria were as follows: unclear exercise protocols, exercise protocols not designed to improve brain or cognitive health (e.g., exercise test to exhaustion), and studies involving combined interventions (e.g., nutrition and cognition). To ensure generalizability, research utilizing clinical samples (e.g., overweight/obese) and those examining special groups (athletes or people with long-term exercise habits) were excluded.
2.3 Data extraction
Duplicated studies screened from the database search and reference lists were initially excluded. Next, the titles and abstracts were individually evaluated by two authors (J.M.H. and T.X.) to further exclude articles based on the eligibility criteria. Afterward, the two authors independently evaluated the articles. Disagreements were resolved by discussion and consensus among the three authors (Q.Q.S., J.M.H., and T.X.).
The two authors independently extracted the following data from eligible studies: (1) basic information, including the year of publication, participant characteristics, and study design; (2) study design, including study group or condition design, fNIRS state (resting-state or task-design), physiological outcome index, and behavioral outcome index; (3) fNIRS device parameters, including types of fNIRS devices, fNIRS sampling frequency, number of light emitting diodes, laser diodes, channels, fNIRS instrument location and area of interest, and position/arrangement and placement of the light source and detector; (4) the exercise intervention design, covering exercise type, exercise intervention period, frequency of exercise, exercise intensity, and single intervention duration; and (5) the primary endpoints of the studies.
2.4 Risk of bias assessment
The risk of bias in selected studies was independently assessed by two authors (J.M.H. and T.X.) using the Cochrane Collaboration Risk-of-Bias tool ( Higgins et al., 2011 , 2019 ). Disagreements were resolved by discussion with another author (Q.Q.S.) to achieve consensus (see Table 1 and Figure 1 ).
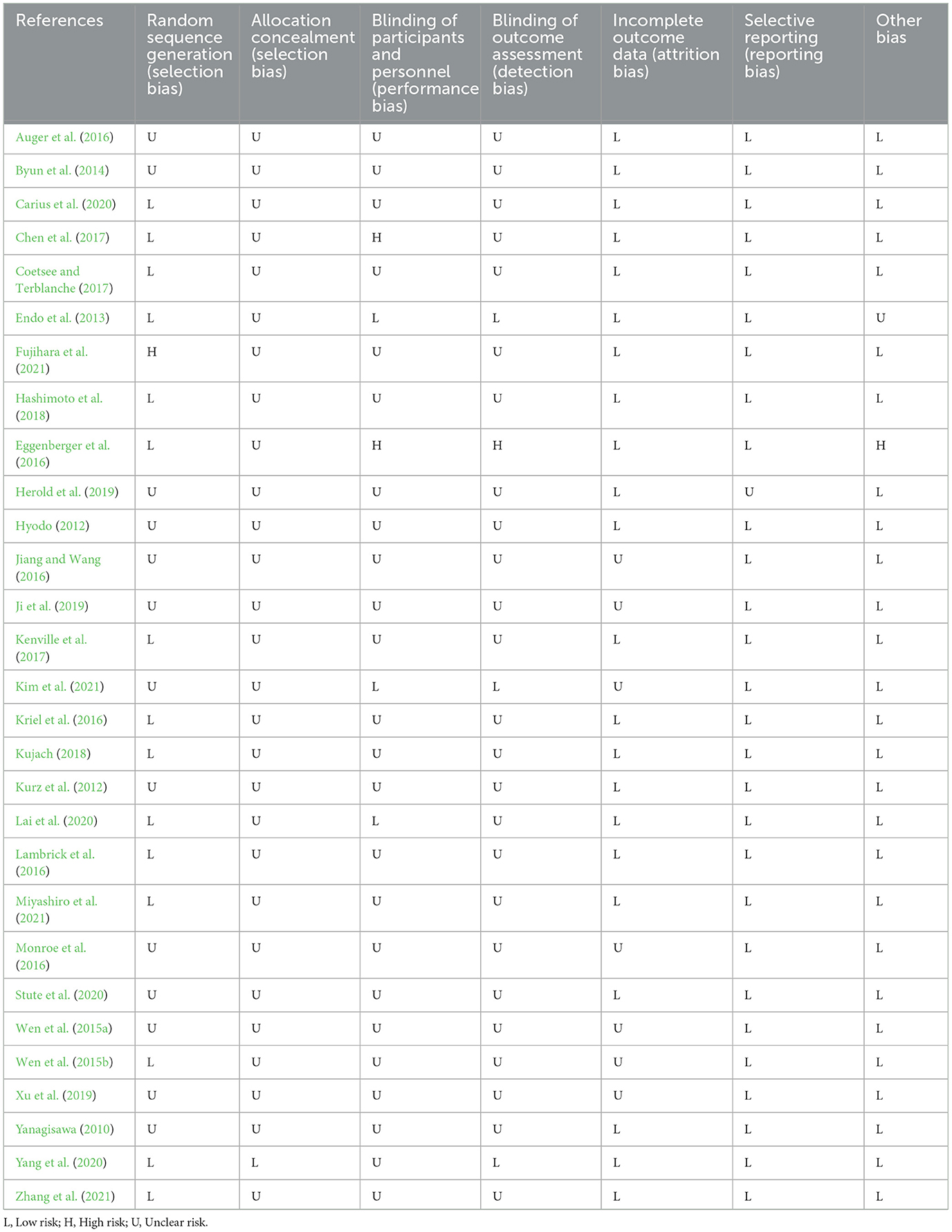
Table 1 . Quality of included studies.
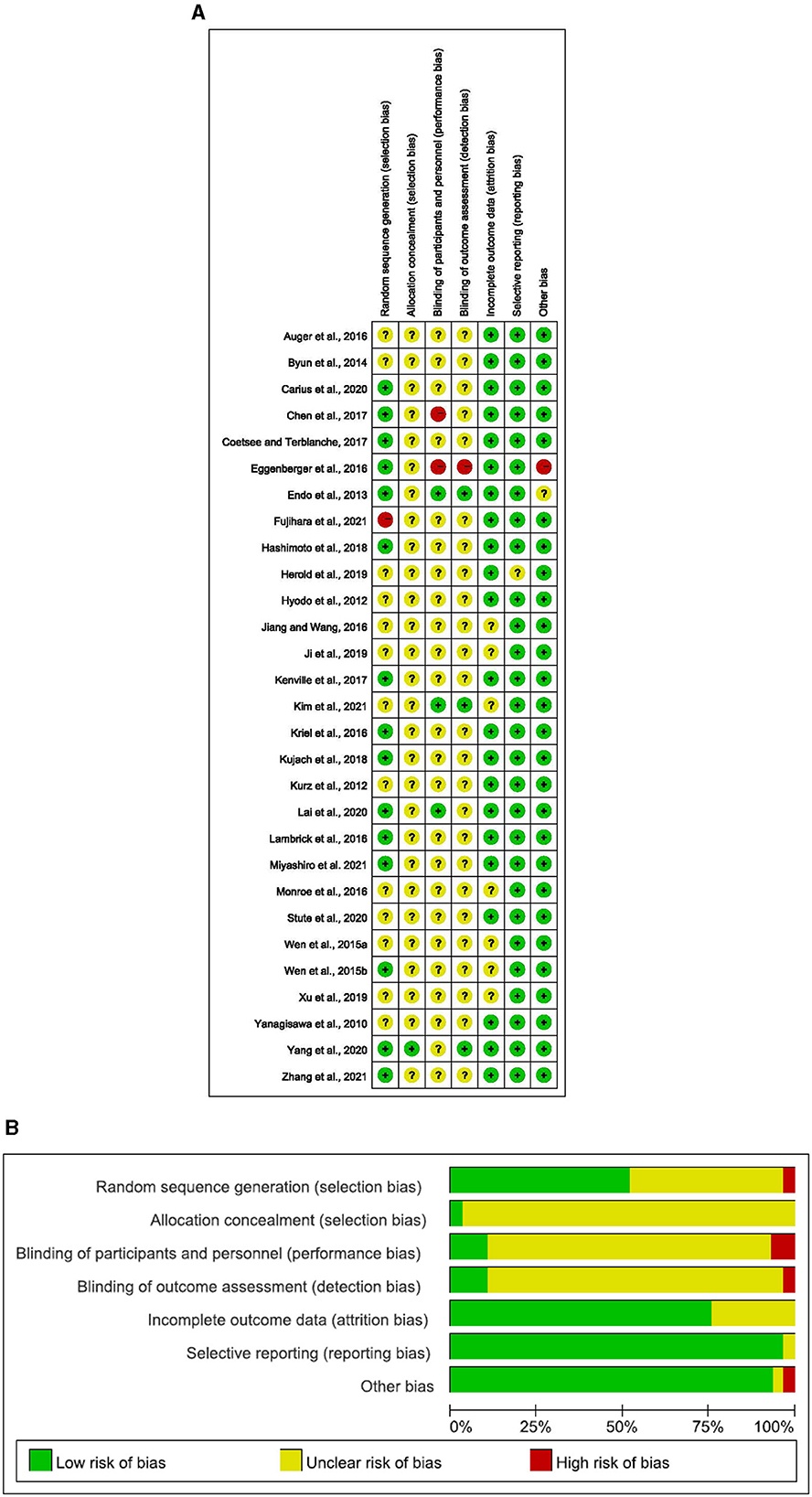
Figure 1 . (A) Risk of bias ratings. (B) Risk of bias graph: percentage of trials with low, unclear, or high risk of bias ratings for each domain (Yanagisawa, 2010 ; Hyodo, 2012 ; Kurz et al., 2012 ; Endo et al., 2013 ; Byun et al., 2014 ; Wen et al., 2015a , b ; Auger et al., 2016 ; Eggenberger et al., 2016 ; Jiang and Wang, 2016 ; Kriel et al., 2016 ; Lambrick et al., 2016 ; Monroe et al., 2016 ; Chen et al., 2017 ; Coetsee and Terblanche, 2017 ; Kenville et al., 2017 ; Hashimoto et al., 2018 ; Kujach, 2018 ; Herold et al., 2019 ; Ji et al., 2019 ; Xu et al., 2019 ; Carius et al., 2020 ; Lai et al., 2020 ; Stute et al., 2020 ; Yang et al., 2020 ; Kim et al., 2021 ; Miyashiro et al., 2021 ; Zhang et al., 2021 ).
3.1 Study selection and characteristics
The search process is detailed in a flow chart illustrated in Figure 2 . The search strategy yielded 6,220 studies from the pre-defined databases. After excluding duplicates and reviewing the full text, 69 studies met the criteria based on the consensus reached by the reviewers. From these, 22 eligible articles were included in the first category (cerebral hemodynamics were measured before and after exercise) and 8 in the second category (cerebral hemodynamics were measured during exercise). Among them, one study was simultaneously in both categories.
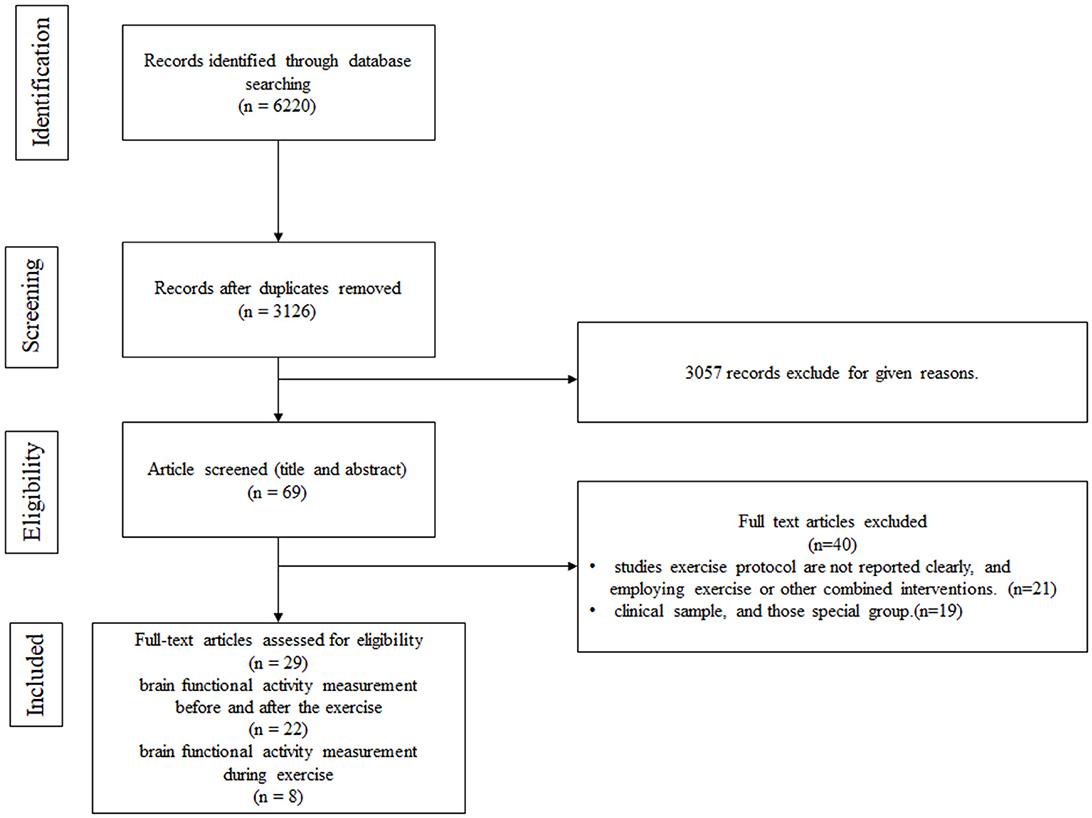
Figure 2 . Flow chart.
Overall, 29 studies were included in the systematic review. Regarding the study region, 18 studies were conducted in Asia (Yanagisawa, 2010 ; Hyodo, 2012 ; Endo et al., 2013 ; Byun et al., 2014 ; Wen et al., 2015a , b ; Jiang and Wang, 2016 ; Chen et al., 2017 ; Coetsee and Terblanche, 2017 ; Hashimoto et al., 2018 ; Kujach, 2018 ; Ji et al., 2019 ; Xu et al., 2019 ; Lai et al., 2020 ; Yang et al., 2020 ; Kim et al., 2021 ; Miyashiro et al., 2021 ; Zhang et al., 2021 ), 5 in Europe ( Eggenberger et al., 2016 ; Kenville et al., 2017 ; Herold et al., 2019 ; Carius et al., 2020 ; Stute et al., 2020 ), 2 in Oceania ( Kriel et al., 2016 ; Lambrick et al., 2016 ), 3 in North America ( Kurz et al., 2012 ; Auger et al., 2016 ; Monroe et al., 2016 ), and 1 in Africa ( Coetsee and Terblanche, 2017 ). A total of 664 participants were examined, with sample sizes ranging from 10 to 67. The age of patients across studies spanned from 72.3 months to 75 years.
3.2 Quality of included studies
The details on the quality of the included studies in bias risk assessment are summarized in the supporting material. Of note, 28 studies did not provide details on selective reporting, 27 studies reported no other biases, 23 studies reported complete outcome data, 15 studies reported random sequence generation, 1 study reported allocation concealment, 1 study reported blinding of participants and personnel, and 1 study reported blinding of outcome assessment.
3.3 Study design
Twenty-two studies measured cerebral hemodynamics before and after exercise interventions, eight studies (including only adults) documented cerebral hemodynamics during the exercise intervention, and one study recorded cerebral hemodynamics before, during, and after the exercise intervention.
3.3.1 Study design encompassing measurements before and after exercise intervention
In this category (see Table 2 ), 22 studies provided information on cerebral hemodynamics and activity before and after exercise in the exercise group compared to levels measured before exercise in this group (14 studies) (Yanagisawa, 2010 ; Hyodo, 2012 ; Endo et al., 2013 ; Kujach, 2018 ; Miyashiro et al., 2021 ; Byun et al., 2014 ; Wen et al., 2015a , b ; Jiang and Wang, 2016 ; Lambrick et al., 2016 ; Hashimoto et al., 2018 ; Ji et al., 2019 ; Xu et al., 2019 ; Kim et al., 2021 ) or cerebral hemodynamics levels measured before and after exercise in another group (8 studies) ( Eggenberger et al., 2016 ; Chen et al., 2017 ; Coetsee and Terblanche, 2017 ; Lai et al., 2020 ; Stute et al., 2020 ; Yang et al., 2020 ; Fujihara et al., 2021 ; Zhang et al., 2021 ).
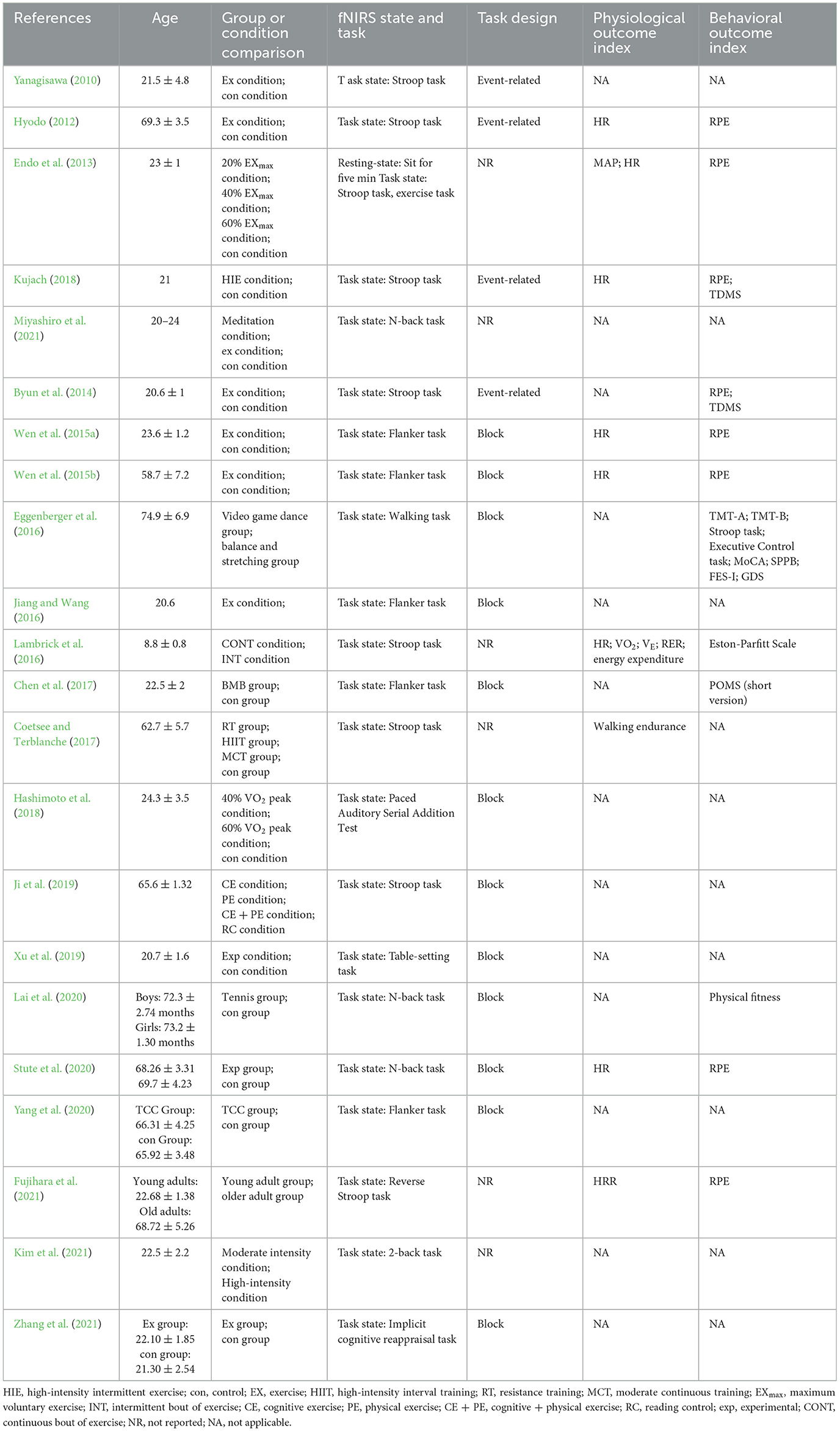
Table 2 . Study design of measurement before and after exercise intervention.
Only one study measured hemodynamic changes and activity in the resting state. In this particular study, baseline brain activity was assessed in the seated position for 5 min ( Endo et al., 2013 ).
All studies evaluated cortical hemodynamic activation using different task designs: 14 studies assessed inhibitory control (Flanker or Stroop task) (Yanagisawa, 2010 ; Hyodo, 2012 ; Endo et al., 2013 ; Byun et al., 2014 ; Wen et al., 2015a , b ; Jiang and Wang, 2016 ; Lambrick et al., 2016 ; Chen et al., 2017 ; Coetsee and Terblanche, 2017 ; Kujach, 2018 ; Ji et al., 2019 ; Yang et al., 2020 ; Fujihara et al., 2021 ), 3 studies examined working memory (N-back task) ( Lai et al., 2020 ; Stute et al., 2020 ; Kim et al., 2021 ), 1 study investigated attention (paced auditory serial addition test) ( Hashimoto et al., 2018 ), 1 study assessed cognitive reappraisal (implicit cognitive reappraisal task) ( Zhang et al., 2021 ), 1 study investigated the mirror neuron system (table-setting task) ( Xu et al., 2019 ), one study applied a concentration task (2-back task) ( Miyashiro et al., 2021 ), and one study assessed an exercise task (walking) ( Eggenberger et al., 2016 ). Interestingly, the majority of designs were block designs used in 12 studies ( Wen et al., 2015a , b ; Eggenberger et al., 2016 ; Jiang and Wang, 2016 ; Chen et al., 2017 ; Hashimoto et al., 2018 ; Ji et al., 2019 ; Xu et al., 2019 ; Lai et al., 2020 ; Yang et al., 2020 ; Zhang et al., 2021 ), whereas an event-related design was applied in four studies (Yanagisawa, 2010 ; Hyodo, 2012 ; Byun et al., 2014 ; Kujach, 2018 ). The remaining studies did not report the study design.
Nine studies carried out physiological measurements after exercise, among which seven studies measured heart rate (HR) (Hyodo, 2012 ; Endo et al., 2013 ; Wen et al., 2015a , b ; Lambrick et al., 2016 ; Kujach, 2018 ; Stute et al., 2020 ). Other physiological indicators, namely, heart rate reverse (HRR) ( Fujihara et al., 2021 ), mean arterial blood pressure (MAP) ( Endo et al., 2013 ), walking endurance ( Coetsee and Terblanche, 2017 ), oxygen intake (VO 2 ), minute ventilation (V E ), respiratory exchange ratio (RER), and energy expenditure, were exclusively analyzed in one particular study ( Lambrick et al., 2016 ).
Twelve studies investigated other behavioral indexes without examining cortical hemodynamic activation, among which eight studies measured Rating of Perceived Exertion (RPE) (Hyodo, 2012 ; Endo et al., 2013 ; Byun et al., 2014 ; Wen et al., 2015a , b ; Kujach, 2018 ; Stute et al., 2020 ; Fujihara et al., 2021 ), and two studies measured Two-Dimensional Mood Scale (TDMS) ( Byun et al., 2014 ; Kujach, 2018 ). Other behavioral indices, namely the Profile of Mood States (POMS) (short version) ( Chen et al., 2017 ), physical fitness ( Lai et al., 2020 ), Eston-Parfitt Scale ( Lambrick et al., 2016 ), Trail Making Test Part A (TMT-A), Trail Making Test Part B (TMT-B), Stroop Word-Color Interference task, Executive Control task, Montreal Cognitive Assessment (MoCA), Short Physical Performance Battery (SPPB), Falls Efficacy Scale International (FES-I), and geriatric depression scale (GDS), were solely analyzed in one study ( Eggenberger et al., 2016 ).
3.3.2 Study design involving measurements during exercise interventions
In this category (see Table 3 ), eight studies presented data on cerebral hemodynamic activation during the exercise intervention. Of note, all studies used exercise tasks to investigate the task design. Most studies either used block designs or did not specify the design, whilst few studies provided a detailed description of the design of exercise tasks. As anticipated, studies adopting a block design employed relatively short durations for each block, similar to the cognitive task, ranging from 20 to 40 seconds.
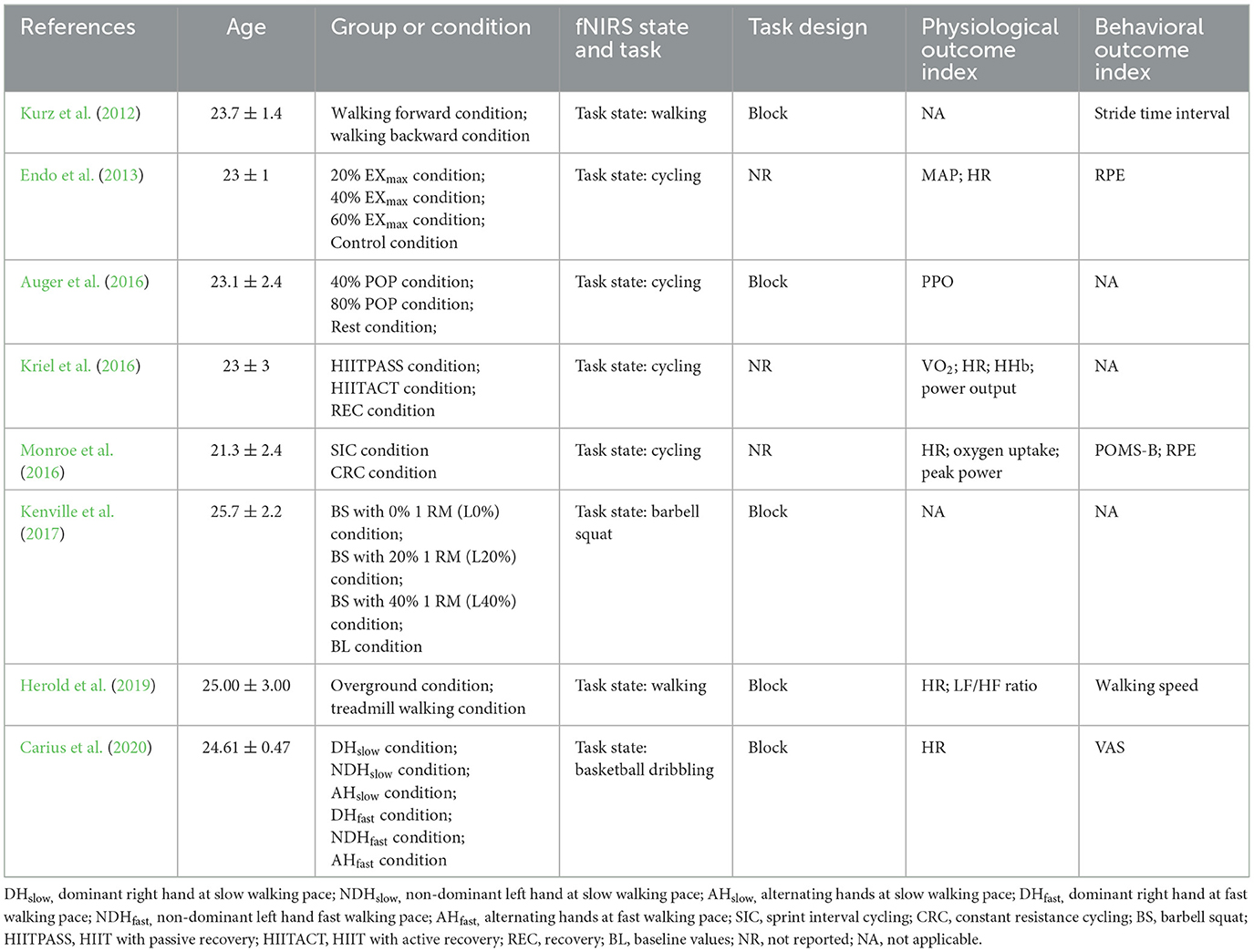
Table 3 . Study design of measurement during exercise interventions.
These exercise tasks, such as walking ( Kurz et al., 2012 ; Herold et al., 2019 ), cycling ( Endo et al., 2013 ; Auger et al., 2016 ; Kriel et al., 2016 ; Monroe et al., 2016 ), basketball slalom dribbling ( Carius et al., 2020 ), and barbell squats ( Kenville et al., 2017 ) were easy to perform in laboratory settings.
Furthermore, six studies conducted physiological measurements, of which five studies measured HR ( Endo et al., 2013 ; Kriel et al., 2016 ; Monroe et al., 2016 ; Herold et al., 2019 ; Carius et al., 2020 ). Some physiological indicators, namely MAP ( Endo et al., 2013 ), VO 2 ( Kriel et al., 2016 ), peak power output (PPO) ( Auger et al., 2016 ), power output ( Kriel et al., 2016 ), peak power ( Monroe et al., 2016 ), oxygen uptake ( Monroe et al., 2016 ), and LF/HF ratio ( Herold et al., 2019 ), were only analyzed in one specific study.
Five studies investigated the effects of exercise on behavioral indices that only appeared in one particular study, namely stride time interval ( Kurz et al., 2012 ), PRE ( Endo et al., 2013 ; Monroe et al., 2016 ), Profile of Mood States-Brie (POMS-B) ( Monroe et al., 2016 ), walking speed ( Herold et al., 2019 ), and visual analog scale (VAS) ( Carius et al., 2020 ).
3.4 fNIRS devices
3.4.1 measurements before and after exercise interventions.
Most included studies conducted fNIRS tests before and after a single, long-term exercise intervention using eleven different fNIRS devices. The device sampling frequency ranged from 1 to 50 Hz, with the majority of devices utilizing 16 emitting diodes and 16 laser diodes. The number of channels ranged from 2 to 48.
Four studies focused on multiple cortical areas ( Hashimoto et al., 2018 ; Xu et al., 2019 ; Stute et al., 2020 ). Among them, one study focused on motor areas, such as the premotor cortex (PMC) ( Xu et al., 2019 ; Stute et al., 2020 ), whilst two studies reported findings on the parietal cortex ( Xu et al., 2019 ; Stute et al., 2020 ), such as the inferior parietal cortex (IPC) and superior parietal lobule (SPL). Besides, two studies reported data on the prefrontal cortex ( Stute et al., 2020 ; Yang et al., 2020 ), one study investigated temporal areas ( Hashimoto et al., 2018 ), and one study assessed the inferior frontal gyrus (IFG). Nineteen studies exclusively assessed activation of the PFC (Yanagisawa, 2010 ; Hyodo, 2012 ; Endo et al., 2013 ; Byun et al., 2014 ; Wen et al., 2015a , b ; Eggenberger et al., 2016 ; Jiang and Wang, 2016 ; Lambrick et al., 2016 ; Chen et al., 2017 ; Coetsee and Terblanche, 2017 ; Kujach, 2018 ; Ji et al., 2019 ; Lai et al., 2020 ; Yang et al., 2020 ; Fujihara et al., 2021 ; Kim et al., 2021 ; Miyashiro et al., 2021 ; Zhang et al., 2021 ). Details are listed in Table 4 .
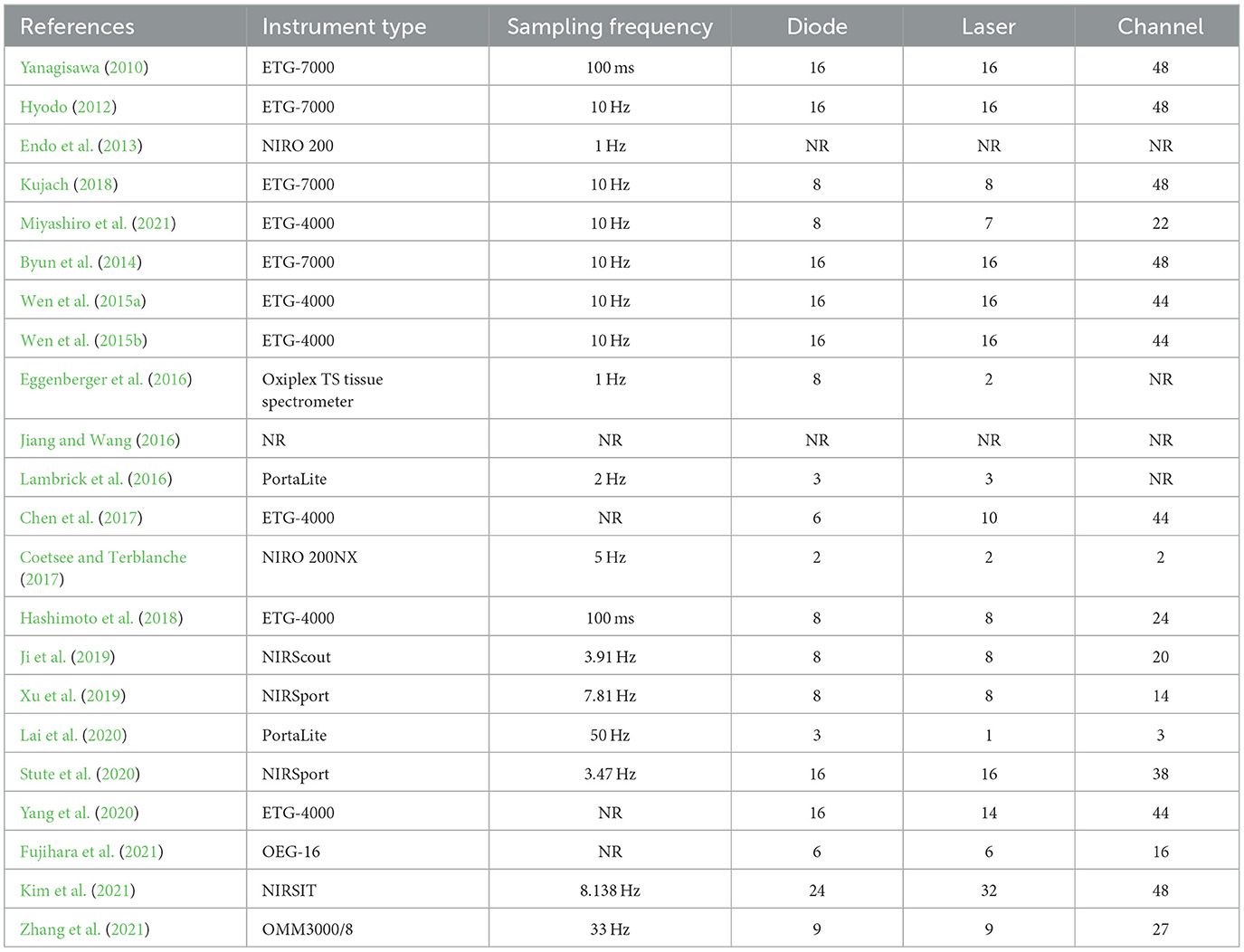
Table 4 . The fNIRS devices used in the study design for measurement before and after exercise intervention.
3.4.2 Measurements during exercise interventions
fNIRS was conducted during acute exercise interventions (see Table 5 ) using four distinct fNIRS devices were used. The device sampling frequency ranged from 1 to 1,000 Hz, and 8 emitting diodes and 8 laser diodes were employed in the majority of the studies. The number of channels ranged from 4 to 24.
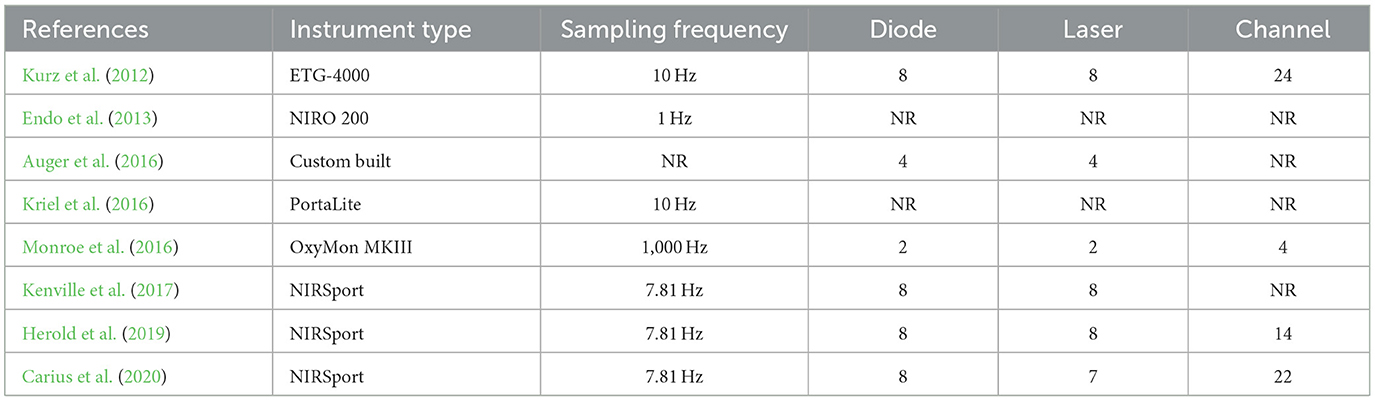
Table 5 . The fNIRS devices used in the study design for measurement during exercise intervention.
Four studies focused on multiple cortical areas ( Kurz et al., 2012 ; Kenville et al., 2017 ; Herold et al., 2019 ; Carius et al., 2020 ). All studies focused on motor areas, such as the PMC, primary motor cortex (M1), supplementary motor area (SMA), and precentral gyrus (PCG) ( Kurz et al., 2012 ; Kenville et al., 2017 ; Herold et al., 2019 ; Carius et al., 2020 ). Among them, three studies reported findings on the parietal cortex ( Kurz et al., 2012 ; Kenville et al., 2017 ; Carius et al., 2020 ), such as the IPC and SPL, one study reported data on the PFC ( Herold et al., 2019 ), one study assessed brain areas related to auditory, frontal and visual functions ( Kurz et al., 2012 ; Kenville et al., 2017 ), including the primary somatosensory cortex (SSC) and, postcentral gyrus (POCG). Lastly, four studies reported data on PFC activation ( Endo et al., 2013 ; Auger et al., 2016 ; Kriel et al., 2016 ; Monroe et al., 2016 ).
3.5 Exercise intervention
All exercise interventions were categorized into three types according to their frequency and duration, regardless of study design. In other words, they were measured before and after long-term exercise interventions ( n = 5), measured before and after one-time exercise interventions ( n = 17), and measured during one-time exercise interventions ( n = 8). Among them, merely one study presented data before, during, and after acute exercise interventions. Major confounding factors adjusted for across these studies included exercise type, duration, intensity, frequency, and duration of activity.
3.5.1 Design of measurements before and after exercise interventions
Five studies investigated hemodynamic changes before and after long-term exercise interventions. Since before-after tests were used, the influence of exercise on fNIRS imaging results was not considered. A broad range of exercise interventions was implemented in these studies, including walking ( Coetsee and Terblanche, 2017 ), Tai Chi Chuan (TCC) ( Yang et al., 2020 ), Baduanjin mind-body (BMB) ( Chen et al., 2017 ), tennis ( Lai et al., 2020 ) or interactive cognitive-motor video game dancing (DANCE), and balance and stretching training (BALANCE) ( Eggenberger et al., 2016 ). The exercise intervention period lasted 8 weeks in most studies ( Eggenberger et al., 2016 ; Chen et al., 2017 ; Lai et al., 2020 ; Yang et al., 2020 ), with only one study extending to 16 weeks ( Coetsee and Terblanche, 2017 ). The frequency of exercise ranged from 2 to 5 times a week. Exercise intensity was classified into three categories: low, moderate, and high. Most studies employed moderate exercise intensity, except for one study that did not report data on intensity ( Chen et al., 2017 ) and one that used moderate-vigorous ( Coetsee and Terblanche, 2017 ) intensity. The duration of a single intervention ranged from 30 min to 90 min. Details are listed in Table 6 .
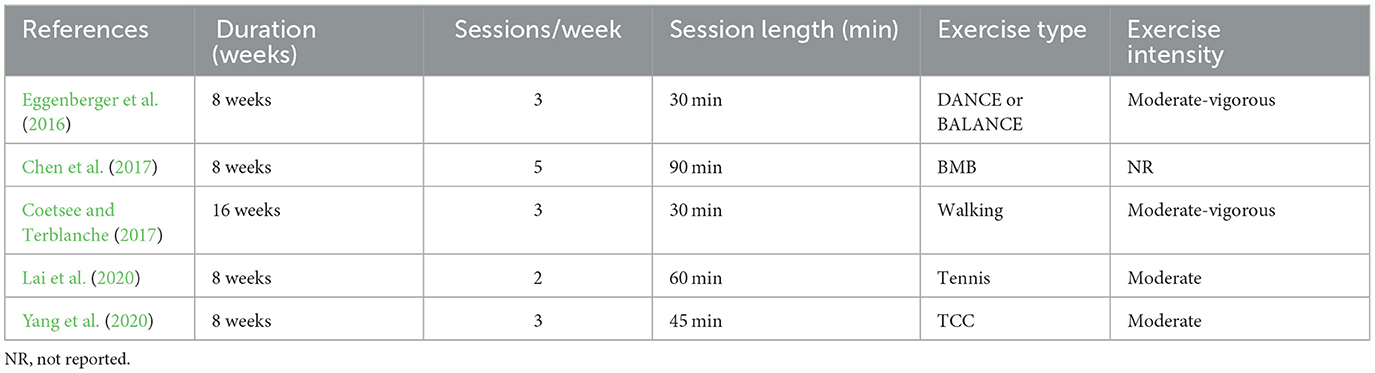
Table 6 . Exercise protocol used in the study design for measurement before and after long-term exercise intervention.
Seventeen studies measured cerebral blood flow before and after acute exercise interventions (see Table 7 ). Exercise types involved cycling and running in the majority of studies, with the exception of one study that incorporated push-ups ( Miyashiro et al., 2021 ). The duration of a single intervention varied from 10 min to 30 min, with seven studies employing a 10-min duration (Yanagisawa, 2010 ; Hyodo, 2012 ; Byun et al., 2014 ; Wen et al., 2015a , b ; Kujach, 2018 ; Kim et al., 2021 ), five studies opting for 15 min ( Endo et al., 2013 ; Hashimoto et al., 2018 ; Ji et al., 2019 ; Stute et al., 2020 ; Fujihara et al., 2021 ), two studies using a 20-min duration ( Jiang and Wang, 2016 ; Miyashiro et al., 2021 ), one study implementing a duration of 25 min ( Xu et al., 2019 ), and two studies extending to 30 min ( Lambrick et al., 2016 ; Zhang et al., 2021 ). Lastly, exercise intensity was mostly moderate.
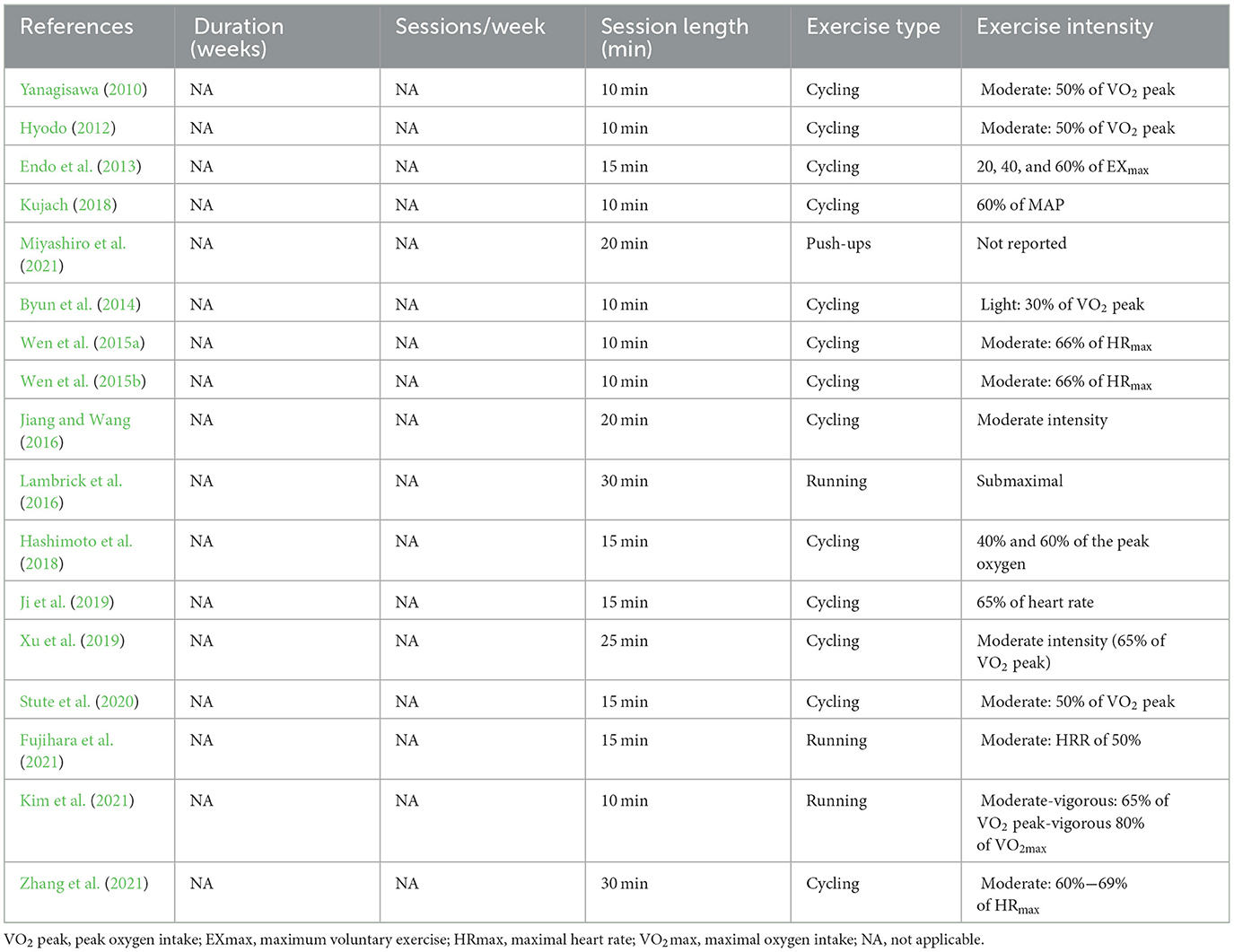
Table 7 . Exercise protocol used in the study design for measurement before and after acute exercise intervention.
3.5.2 Design of measurements during acute exercise intervention
In the one-time exercise interventions, eight studies measured fNIRS during the exercise intervention (see Table 8 ). These studies mainly selected exercise interventions involving minimal head movement, such as cycling ( Endo et al., 2013 ; Auger et al., 2016 ; Kriel et al., 2016 ; Monroe et al., 2016 ), basketball slalom dribbling ( Carius et al., 2020 ), barbell squats ( Kenville et al., 2017 ), and walking ( Kurz et al., 2012 ; Herold et al., 2019 ). Moreover, most studies implemented cycling and walking interventions, while four studies used cycling ( Endo et al., 2013 ; Auger et al., 2016 ; Kriel et al., 2016 ; Monroe et al., 2016 ), and two studies used walking ( Kurz et al., 2012 ; Herold et al., 2019 ). The duration of the intervention ranged from 10 min to 25 min. While moderate intensity was used in most of the eight studies, some studies did not report exercise intensity and instead reported data on the exercise load.
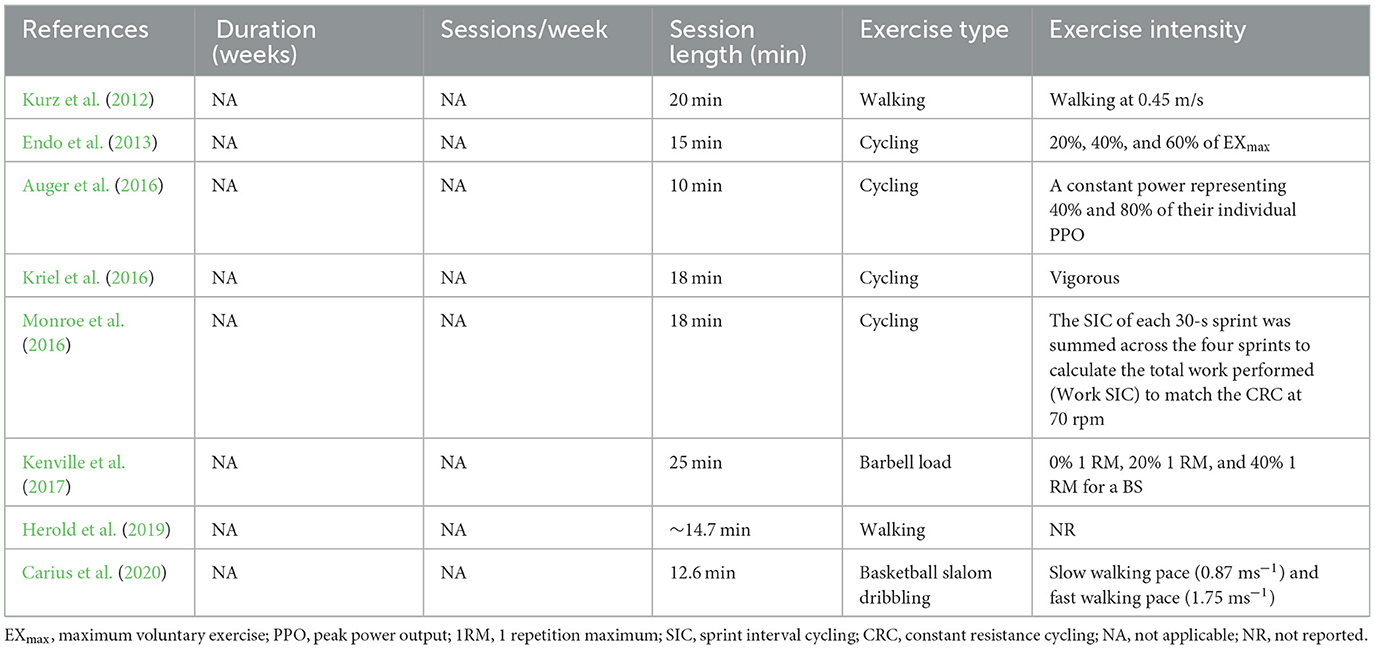
Table 8 . Exercise protocol used in the study design measurement during acute exercise intervention.
3.6 Main results
A total of 29 studies investigated oxyhemoglobin (oxy-Hb), deoxyhemoglobin (deoxy-Hb), and total hemoglobin (total Hb) levels following exercise interventions. Specifically, three studies measured oxy-Hb, deoxy-Hb, and total Hb levels ( Auger et al., 2016 ; Lambrick et al., 2016 ; Coetsee and Terblanche, 2017 ), eight studies measured oxy-Hb and deoxy-Hb levels (Hyodo, 2012 ; Kurz et al., 2012 ; Endo et al., 2013 ; Byun et al., 2014 ; Monroe et al., 2016 ; Kenville et al., 2017 ; Herold et al., 2019 ; Carius et al., 2020 ), 16 studies measured oxy-Hb levels (Yanagisawa, 2010 ; Wen et al., 2015a , b ; Eggenberger et al., 2016 ; Jiang and Wang, 2016 ; Chen et al., 2017 ; Hashimoto et al., 2018 ; Kujach, 2018 ; Ji et al., 2019 ; Xu et al., 2019 ; Lai et al., 2020 ; Yang et al., 2020 ; Fujihara et al., 2021 ; Kim et al., 2021 ; Miyashiro et al., 2021 ; Zhang et al., 2021 ), one study measured deoxy-Hb levels ( Kriel et al., 2016 ), and one study computed the HBdiff (oxy-Hb minus deoxy-Hb) ( Stute et al., 2020 ).
3.6.1 Changes in brain functional activity before and after exercise interventions
Five studies investigated cerebral blood flow after long-term exercise interventions (see Table 9 ). One study measured oxy-Hb, deoxy-Hb, and total Hb levels ( Coetsee and Terblanche, 2017 ), whilst the remaining four studies measured oxy-Hb levels ( Eggenberger et al., 2016 ; Chen et al., 2017 ; Lai et al., 2020 ; Yang et al., 2020 ). After the long-term intervention, oxy-Hb levels were increased in the left PFC during the flanker and N-back tasks. Likewise, deoxy-Hb levels were increased in the left PFC during the Stroop task across almost all studies. One study used a walking task and described that oxy-Hb levels were higher in the left PFC and right PFC during walking.
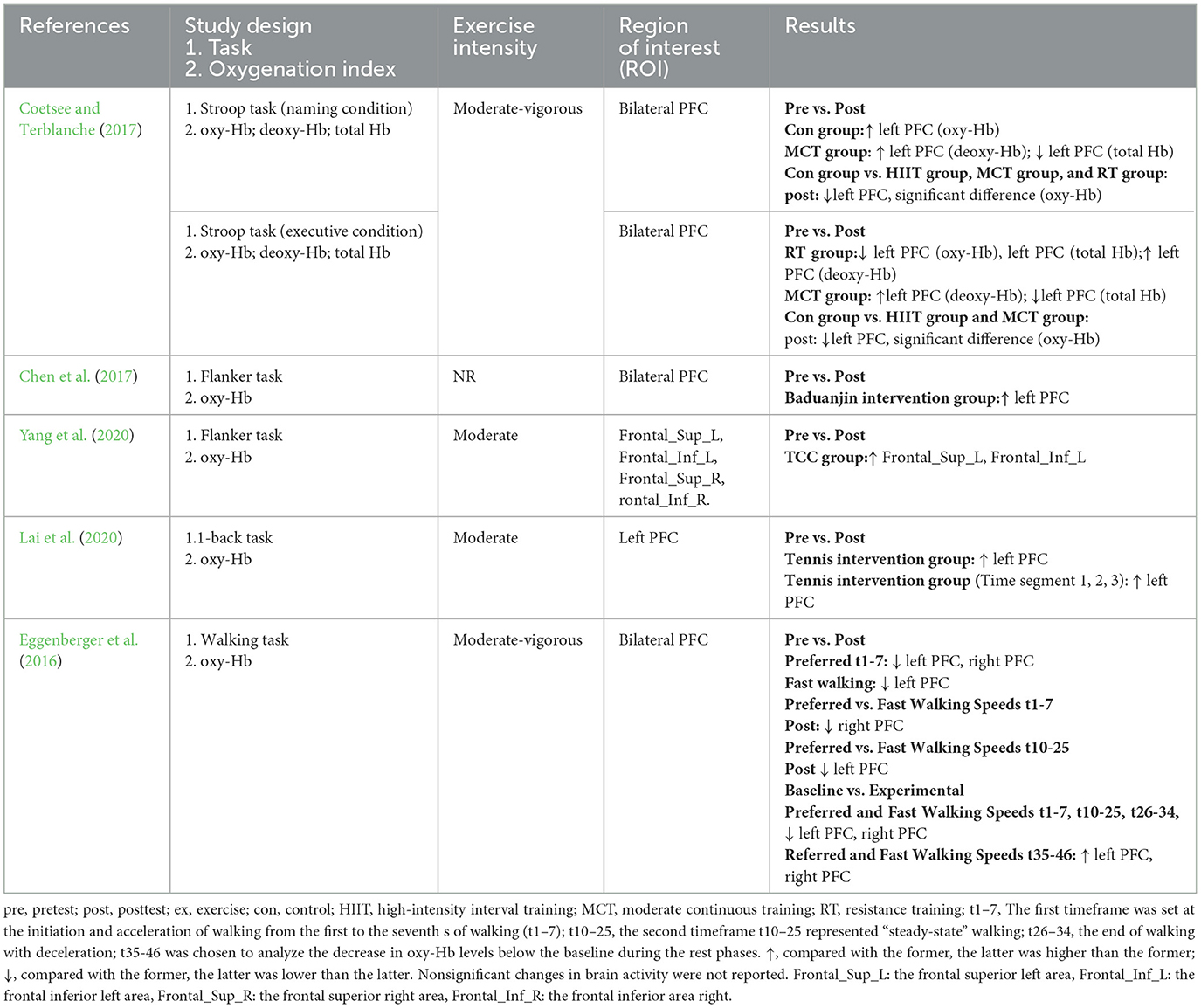
Table 9 . Changes of brain functional activity before and after long-term exercise interventions.
Seventeen studies investigated brain function before and after the acute exercise intervention (see Table 10 ). One study measured oxy-Hb, deoxy-Hb, and total Hb levels ( Lambrick et al., 2016 ), three studies analyzed oxy-Hb and deoxy-Hb levels (Yanagisawa, 2010 ; Endo et al., 2013 ; Miyashiro et al., 2021 ), 12 studies measured oxy-Hb levels (Yanagisawa, 2010 ; Wen et al., 2015a , b ; Jiang and Wang, 2016 ; Hashimoto et al., 2018 ; Kujach, 2018 ; Ji et al., 2019 ; Xu et al., 2019 ; Fujihara et al., 2021 ; Kim et al., 2021 ; Miyashiro et al., 2021 ; Zhang et al., 2021 ), and one study calculated the HBdiff (oxy-Hb minus deoxy-Hb) ( Herold et al., 2019 ). After the acute intervention, eight articles explored changes in the Stroop task (Yanagisawa, 2010 ; Hyodo, 2012 ; Endo et al., 2013 ; Byun et al., 2014 ; Lambrick et al., 2016 ; Kujach, 2018 ; Ji et al., 2019 ; Fujihara et al., 2021 ) and observed an increase in oxy-Hb levels in the left dorsolateral prefrontal cortex (DLPFC) (Yanagisawa, 2010 ; Hyodo, 2012 ; Byun et al., 2014 ; Kujach, 2018 ; Ji et al., 2019 ), bilateral PFC ( Endo et al., 2013 ), right frontopolar area (FPA) (Hyodo, 2012 ), left FPA ( Byun et al., 2014 ), middle PFC ( Fujihara et al., 2021 ), right ventrolateral prefrontal cortex (VLPFC) ( Ji et al., 2019 ), and supraorbital ridge of the dominant side ( Lambrick et al., 2016 ). Meanwhile, three articles explored changes in flanker task performance and observed that exercise resulted in an increase in oxy-Hb levels in the bilateral DLPFC ( Wen et al., 2015b ), right DLPFC, right FPA ( Wen et al., 2015a ), and left FPA ( Wen et al., 2015b ). Similarly, three articles explored fluctuations in performance on the n-back task and noted a rise in oxy-Hb levels in the bilateral orbitofrontal cortex (OFC) ( Miyashiro et al., 2021 ) and left DLPFC ( Kim et al., 2021 ) and a concomitant decrease in oxy-Hb levels in the right DLPFC during moderate-intensity exercise interventions ( Kim et al., 2021 ), whilst HBdiff was decreased in both regions (frontal and parietal) and hemispheres (left and right) at almost all time points ( Stute et al., 2020 ). One study applied the table-setting task ( Xu et al., 2019 ) and found elevated oxy-Hb levels in the PMC, SPL, inferior frontal gyrus (IFG), and rostral inferior parietal lobule (IPL). Another study used the Paced Auditory Serial Addition Test ( Hashimoto et al., 2018 ) and revealed that oxy-Hb levels in the left PFC increased with different exercise intensities. Finally, one study used the implicit cognitive reappraisal task but did not identify specific regions of interest (ROIs) with changes in activity after exercise and reported elevated oxy-Hb levels in channels 11, 16, 21, 23, and 27 ( Zhang et al., 2021 ).
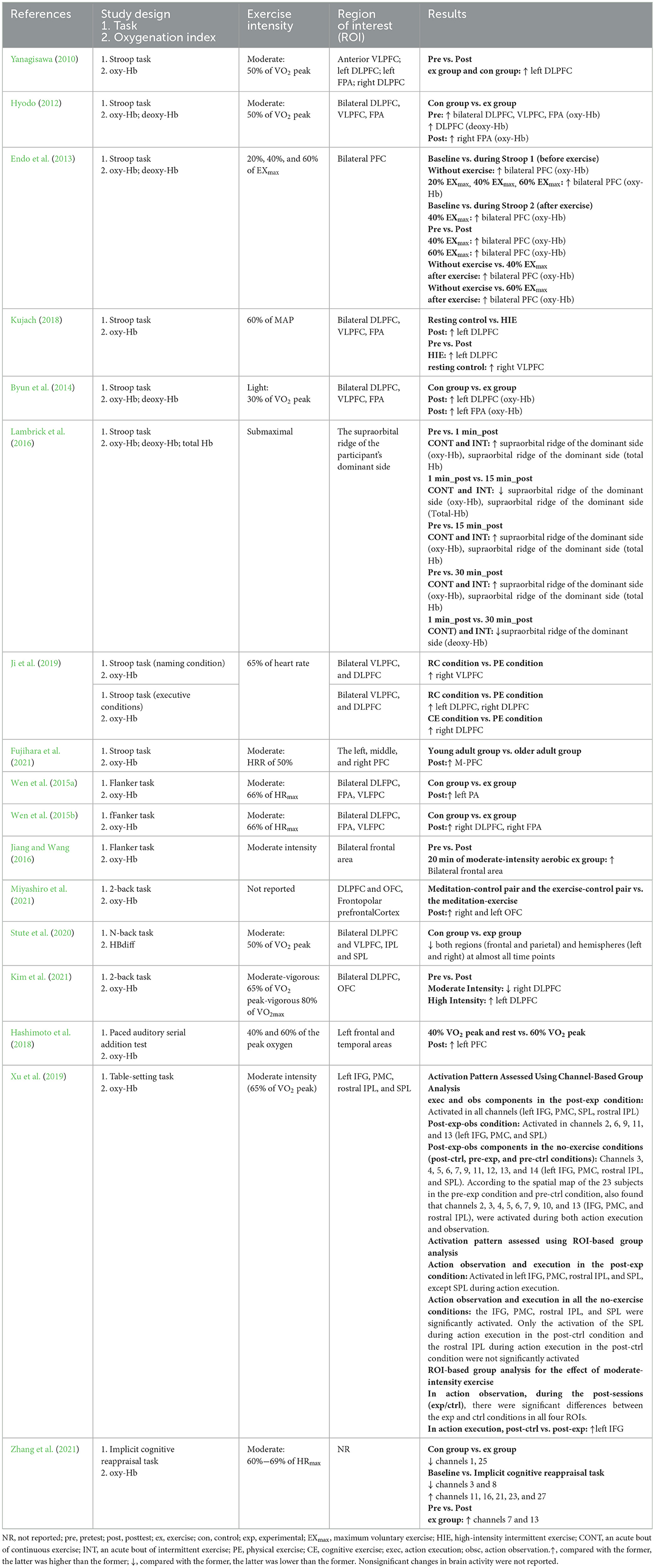
Table 10 . Changes of brain functional activity before and after acute exercise interventions.
3.6.2 Changes in brain functional activity during exercise interventions
Eight studies investigated hemodynamic changes during exercise interventions (see Table 11 ). Studies using a one-time exercise intervention measured cerebral blood flow during exercise ( Endo et al., 2013 ). Six studies analyzed both oxy-Hb and deoxy-Hb levels ( Kurz et al., 2012 ; Endo et al., 2013 ; Monroe et al., 2016 ; Kenville et al., 2017 ; Herold et al., 2019 ; Carius et al., 2020 ), one study exclusively analyzed oxy-Hb, deoxy-Hb, and total Hb levels ( Auger et al., 2016 ), and one study detected deoxy-Hb levels ( Kriel et al., 2016 ).
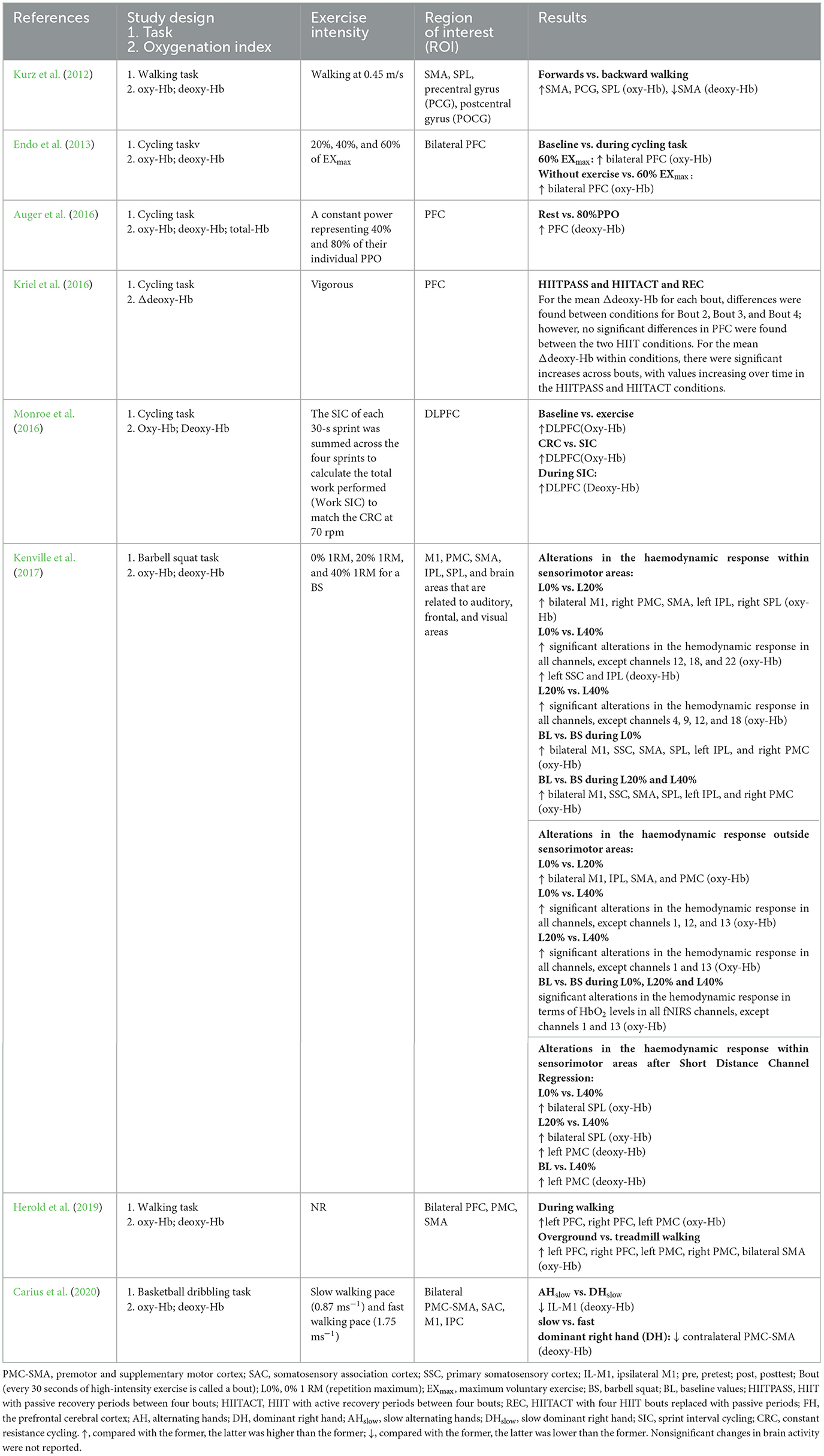
Table 11 . Changes of brain functional activity during acute exercise interventions.
During the acute intervention, eight studies explored changes in various exercise conditions. Three studies explored changes in different cycling intensities and noted that oxy-Hb levels were increased in bilateral PFC during exercise at the intensity of 60% EX max ( Endo et al., 2013 ) and that under all three conditions of rest, 40%, and 80% intensity levels. The subjects at rest exhibited significantly lower extracerebral and cerebral deoxy-Hb levels compared to values measured at the 80% intensity level of exercise. Furthermore, another study detected significant alterations in the hemodynamic response in almost all channels, with increased oxy-Hb in the bilateral SPL and left PMC after short-distance channel regression ( Kenville et al., 2017 ). At the same time, two studies explored the effects of different levels of exercise difficulties on cerebral hemodynamics. Four studies varied exercise intensity and found an increase in oxy-Hb levels in the bilateral PFC, bilateral M1, SSC, SMA, SPL, left IPL, and right PMC and a concurrent decrease in deoxy-Hb levels in the PFC. One study compared forward walking with backward walking and documented that oxy-Hb levels were higher in the SMA, PCG, and SPL, whereas deoxy-Hb levels were decreased in the SMA. Another study compared brain function in overground and treadmill conditions and observed an increase in oxy-Hb levels in the L-PFC, R-PFC, L-PMC, R-PMC, and B-SMA. One study explored the effect of active recovery on brain function and detected an increase in PFC activity. An earlier study employed BSDT to explore brain function under different basketball dribbling conditions and found that IL-M1 (deoxy-Hb levels) and contralateral PMC-SMA (deoxy-Hb levels) activities were decreased. One study investigated the effect of active recovery on brain function and evinced a significant interaction between condition and bout (every 30 seconds of high-intensity exercise is termed a bout) for mean changes in Δ[HHb] across bouts. Within conditions, significant increases in mean Δ[HHb] were observed across bouts, with values progressively increasing over time under HIITPASS and HIITACT conditions.
4 Discussion
4.1 study design.
Most task-related fNIRS studies aimed to detect activation before and after exercise. Notably, relatively few studies have been conducted on resting-state hemodynamics in this field; only one study examined changes in resting-state hemodynamics before and after exercise ( Endo et al., 2013 ) and did not identify significant changes. Studies performing measurements before and after exercise interventions provide evidence of the effects of exercise on an individual's neural mechanisms.
A minority of the included studies explored cerebral blood flow during exercise interventions ( Kurz et al., 2012 ; Endo et al., 2013 ; Auger et al., 2016 ; Kriel et al., 2016 ; Monroe et al., 2016 ; Kenville et al., 2017 ; Herold et al., 2019 ; Carius et al., 2020 ), all of which were task-designed and used exercise type as an exercise task. Nevertheless, it is worth mentioning that there are limited available exercise-type options, and they are relatively fixed. Most exercise tasks in those studies adopted the same design as cognitive tasks and controlled the duration of each trial to approximately 30 to 40 seconds ( Kurz et al., 2012 ; Kenville et al., 2017 ; Carius et al., 2020 ). However, from a practical perspective, exercise tasks lasting <30 seconds are challenging to implement. Thus, fNIRS should be implemented to develop an exercise task that combines the characteristics of the movement with the feasibility of fNIRS, and suitable methods should be selected to analyze the fNIRS data. Notably, although fNIRS is not as sensitive to motion artifacts as functional MRI and EEG, the rapid motion of any vibrating fiber may lead to substantial changes in the hemoglobin signal. Therefore, when designing exercise tasks, frequent and intense head movements should be avoided.
In addition, numerous studies have explored the effects of exercise interventions on behavioral and physiological indicators before, during, and after exercise. Most of the behavioral indicators are related to cognition (Yanagisawa, 2010 ; Hyodo, 2012 ; Endo et al., 2013 ; Byun et al., 2014 ; Wen et al., 2015a , b ; Jiang and Wang, 2016 ; Lambrick et al., 2016 ; Chen et al., 2017 ; Coetsee and Terblanche, 2017 ; Hashimoto et al., 2018 ; Kujach, 2018 ; Ji et al., 2019 ; Xu et al., 2019 ; Lai et al., 2020 ; Yang et al., 2020 ; Fujihara et al., 2021 ; Kim et al., 2021 ; Miyashiro et al., 2021 ) and emotion ( Zhang et al., 2021 ), while the primary physiological indicator is heart rate, which is closely related to exercise. However, studies examining the relationship between functional brain activity and the three behavioral and physiological domains were scarce.
Future studies can combine real-time changes in cerebral blood flow before, during, and after exercise in a more in-depth and comprehensive manner to establish the relationship between exercise and brain plasticity. In addition, the benefits and mechanisms of exercise interventions on the health of individuals should be explored from multiple perspectives, combining behavioral, physiological, and cerebral assessments.
4.2 fNIRS equipment and parameter settings
The included studies measured cerebral hemodynamics before and after exercise employing a relatively large number of channels. In studies designed to measure cerebral hemodynamics during exercise, the number of channels was relatively low, with the maximum number of channels in the included studies being 24 ( Kurz et al., 2012 ). Attributed to its task specificity, studies that measured cerebral hemodynamics during exercise intervention all used portable devices.
Besides, the selection of ROIs also varied with the study design. Studies designed to measure cerebral hemodynamic changes before and after exercise activity explored the effects of exercise on cognition, with the prefrontal lobe being a key region. In contrast, studies designed to measure cerebral hemodynamics during exercise intervention targeted more locations in the sensorimotor areas ( Endo et al., 2013 ; Auger et al., 2016 ; Kriel et al., 2016 ; Monroe et al., 2016 ; Kenville et al., 2017 ; Herold et al., 2019 ; Carius et al., 2020 ) or other brain areas.
Several exercise processes require a combination of physical activity and cognitive engagement. Hence, it is critical not only to place channels in the motor cortex but also to consider its impact on the prefrontal cortex. Advances in technology have led to an increase in the number of channels for portable devices, thus enabling the measurement of cerebral hemodynamics in multiple ROIs. Given the potential for signal quality issues for measurement during exercise, it is recommended to establish a short-distance channel and instruct participants to minimize head movement during the testing procedure.
4.3 Exercise intervention
Among studies designed to measure fluctuations in cerebral hemodynamics before and after exercise interventions, both long-term exercise interventions and short-term exercise interventions were available. Conversely, few long-term interventions were investigated, most likely due to challenges in conducting the assessment over extended periods. Exercise protocols for long-term interventions were flexible, featuring a diverse range of exercise protocols without overlap between studies and a uniform exercise frequency of 2 to 3 sessions per week ( Eggenberger et al., 2016 ; Coetsee and Terblanche, 2017 ; Lai et al., 2020 ; Yang et al., 2020 ). Only one study applied an exercise frequency of 5 times per week ( Chen et al., 2017 ). Similarly, exercise intensity and duration were relatively consistent, with all being of moderate intensity ( Eggenberger et al., 2016 ; Chen et al., 2017 ; Coetsee and Terblanche, 2017 ; Lai et al., 2020 ; Yang et al., 2020 ) and each session lasting over 30 min ( Chen et al., 2017 ; Lai et al., 2020 ; Yang et al., 2020 ).
At present, studies focusing on the effects of long-term exercise interventions on brain functional activity using fNIRS predominantly aim to detect changes in cognitive-task-related brain functional activity before and after exercise interventions but do not involve the detection of brain functional activity during the exercise task. In studies designed to measure changes in cerebral hemodynamics during acute exercise intervention, all exercise types were relatively easy to implement in the laboratory, requiring minimal activity space and offering flexibility, such as cycling and running. These factors may account for the fact that only three exercise programs were used. In the future, it might be possible to further explore changes in cerebral blood flow during other commonly practiced exercises that involve minimal head movement, such as Tai Chi Chuan and yoga.
The length of the single exercise session, on the other hand, did not exceed 30 min (Yanagisawa, 2010 ; Hyodo, 2012 ; Endo et al., 2013 ; Wen et al., 2015b ; Lambrick et al., 2016 ; Coetsee and Terblanche, 2017 ; Hashimoto et al., 2018 ; Kujach, 2018 ; Ji et al., 2019 ; Xu et al., 2019 ; Stute et al., 2020 ; Fujihara et al., 2021 ; Miyashiro et al., 2021 ; Zhang et al., 2021 ), with the majority lasting for only 10 min (Yanagisawa, 2010 ; Hyodo, 2012 ; Byun et al., 2014 ; Wen et al., 2015a , b ; Kujach, 2018 ; Kim et al., 2021 ). Consequently, changes in cerebral hemodynamics during prolonged exercise sessions remain enigmatic.
To explore hemodynamic changes during exercise, future studies may extend the duration of exercise sessions to 30 min to determine the effect of long exercise session durations on cerebral cortical blood flow.
4.4 Effect of exercise on cerebral cortical blood flow changes
In the exploration of task designs, the activation of hemoglobin was frequently discussed, with most studies using oxygenated hemoglobin as an indicator to evaluate brain activation (Yanagisawa, 2010 ; Wen et al., 2015a , b ; Eggenberger et al., 2016 ; Jiang and Wang, 2016 ; Chen et al., 2017 ; Hashimoto et al., 2018 ; Kujach, 2018 ; Ji et al., 2019 ; Xu et al., 2019 ; Lai et al., 2020 ; Yang et al., 2020 ; Fujihara et al., 2021 ; Kim et al., 2021 ; Miyashiro et al., 2021 ; Zhang et al., 2021 ).
Achieving consistent results with long-term exercise interventions was challenging due to the diversity of the tasks performed. Interestingly, although the adopted tasks were different, most studies identified changes in the left PFC during inhibitory control tasks ( Chen et al., 2017 ; Coetsee and Terblanche, 2017 ; Yang et al., 2020 ). In general, higher hemoglobin activity was observed in the cortical region after the cessation of one-time exercise compared with baseline cortical activity, similar to changes in cortical activation during cognitive tasks. Besides, alterations in the prefrontal cortex and motor cortex were observed during one-time exercise sessions.
Considering that fNIRS signals are significantly impacted by physiological artifacts of the system ( Caldwell et al., 2016 ), the influence of exercise on the cerebral cortex is assumed to mainly arise from physiological confounders after exercise. According to the findings of a methodological investigation, fNIRS signals are affected by systemic physiological artifacts for up to approximately 8 min after stopping cycling for 10 min ( Byun et al., 2014 ). Therefore, the results of studies that performed fNIRS tests after exercise (<~8 min) should consider the effect of physiological confounds. However, a reasonable hypothesis suggests that some fNIRS signals observed post-exercise originated from neuronal activity. The entire prefrontal cortex should be impacted by systemic physiological changes if the greater cortical activity observed after exercise is primarily a result of systemic physiological artifacts. The fact that greater cortical activity was observed only in specific regions of the prefrontal cortex rather than the entire prefrontal cortex is significant because it supports the hypothesis that the fNIRS signals were at least partially derived from neuronal activity. The positive neurobehavioural correlation between cognitive ability and cortical activity in various regions of the prefrontal cortex further supports this notion.
The review indicated that exercise interventions alter oxygenated hemoglobin levels in the prefrontal cortex and motor cortex, which are associated with improvements in higher cognitive functions such as inhibitory control and working memory. These findings further support the hypothesis that exercise promotes changes in the prefrontal and motor cortex, which may be key regions for exercise to promote brain health. To deepen our understanding of the fundamental processes by which exercise modifies the brain, future studies should concentrate on alterations in cerebral blood flow during physical exertion.
4.5 Limitations
Nevertheless, this review has limitations that merit acknowledgment. To begin, this review only explored studies involving exercise durations over 10 min, but future studies should expand their scope to explore durations of exercise that contribute to brain health. The primary purpose of this review was to conduct subgroup analysis based on different research designs (measuring fNIRS before, during, and after exercise intervention). Future analyses should aim to stratify group populations or exercise types into different subgroups. The systematic search, dual-author screening, eligibility assessment, and quality appraisal were employed to minimize biased selection of studies, whilst dual-author auditors ensured a thorough search. However, this review was not preregistered and not available for inspection by other researchers, and future reviews can be registered with PROSPERO in advance. A meta-analysis was not conducted due to the high degree of heterogeneity across studies and the low number of studies examining each outcome.
5 Conclusions and future perspectives
Overall, fNIRS is a promising neuroimaging tool that provides insights into changes in exercise-induced hemodynamics before, during, and after exercise interventions.
Studies using fNIRS to measure cerebral blood flow before and after exercise interventions have predominantly incorporated relatively few long-term interventions and predominantly featured short-term interventions. These studies have mainly focused on the effects of exercise on brain activity during cognitive tasks such as inhibitory control. However, the study equipment and study intervention protocols were less stringent and relatively more flexible. All studies on this topic have focused on changes in oxygenation in the prefrontal area of interest.
Few of the included studies measured hemodynamic changes during exercise, with all of them employing short-term interventions, relatively fixed exercise intervention protocols, minimal head motion, and short exercise durations. Of note, the exercises were easy to execute in the laboratory setting, and the fNIRS systems had a limited number of channels.
Exercise protocols for long-term interventions encompassed a wide range of exercise protocols and an exercise frequency ranging from 2 to 3 sessions per week. The exercise intensity was moderate, and the duration of a single session exceeded 30 min. Exercise types for long-term interventions were those that necessitated minimal space and were flexible, such as cycling and running. The duration of a single exercise session did not exceed 30 min, with most sessions lasting for 10 min. Finally, exercise intensities were maintained at a moderate level.
The results of this review signaled that exercise interventions alter oxygenated hemoglobin levels in the prefrontal cortex and motor cortex, which are associated with improvements in higher cognitive functions such as inhibitory control and working memory. The frontal cortex and motor cortex may be key regions for exercise to promote brain health. Future research could further focus on changes in cerebral blood flow during exercise to better understand the underlying mechanisms by which exercise influences brain function.
Moreover, due to its insensitivity to motion, the fNIRS is ideally suited for research during exercise interventions. It is paramount to thoroughly scrutinize the study design, fNIRS device parameters, and exercise protocols. Cerebral blood flow during exercise intervention represents a future research direction that has the potential both to identify cortical hemodynamic changes and elucidate the relationship between exercise and cognition.
Future studies can combine two study designs that measure blood flow before, during, and after exercise in a more in-depth and comprehensive manner. Additionally, they can utilize multiple indicators, such as functional connectivity, to accurately reflect the effects of exercise on brain functional networks.
Data availability statement
The original contributions presented in the study are included in the article/ Supplementary material , further inquiries can be directed to the corresponding authors.
Author contributions
Q-QS: Writing – original draft, Writing – review & editing. J-MH: Writing – review & editing. TX: Writing – review & editing. J-YZ: Writing – review & editing. D-LW: Writing – review & editing. YY: Writing – review & editing. RL: Writing – review & editing. Z-LX: Writing – review & editing. H-cY: Supervision, Writing – review & editing, Funding acquisition, Project administration. LC: Supervision, Writing – review & editing.
The author(s) declare financial support was received for the research, authorship, and/or publication of this article. This work was supported by the Key Program of the National Social Science Foundation (Grant No. 19ATY010).
Acknowledgments
We extend our gratitude to Professor Shaojun Luy from the College of P. E. and Sports, Beijing Normal University, China, for his invaluable assistance with the Tai Chi (Bafa Wubu) intervention.
Conflict of interest
The authors declare that the research was conducted in the absence of any commercial or financial relationships that could be construed as a potential conflict of interest.
Publisher's note
All claims expressed in this article are solely those of the authors and do not necessarily represent those of their affiliated organizations, or those of the publisher, the editors and the reviewers. Any product that may be evaluated in this article, or claim that may be made by its manufacturer, is not guaranteed or endorsed by the publisher.
Supplementary material
The Supplementary Material for this article can be found online at: https://www.frontiersin.org/articles/10.3389/fpsyg.2024.1327822/full#supplementary-material
Auger, H., Bherer, L., Boucher, É., Hoge, R., Lesage, F., Dehaes, M., et al. (2016). Quantification of extra-cerebral and cerebral hemoglobin concentrations during physical exercise using time-domain near infrared spectroscopy. Biomed. Opt. Expr . 7, 3826–3842. doi: 10.1364/BOE.7.003826
PubMed Abstract | Crossref Full Text | Google Scholar
Byun, K., Hyodo, K., Suwabe, K., Ochi, G., Sakairi, Y., Kato, M., et al. (2014). Positive effect of acute mild exercise on executive function via arousal-related prefrontal activations: an fNIRS study. Neuroimage 98, 336–345. doi: 10.1016/j.neuroimage.2014.04.067
Caldwell, M., Scholkmann, F., Wolf, U., Wolf, M., Elwell, C., Tachtsidis, I., et al. (2016). Modelling confounding effects from extracerebral contamination and systemic factors on functional near-infrared spectroscopy. Neuroimage 143, 91–105. doi: 10.1016/j.neuroimage.2016.08.058
Carius, D., Seidel-Marzi, O., Kaminski, E., Lisson, N., and Ragert, P. (2020). Characterizing hemodynamic response alterations during basketball dribbling. PLoS ONE 15:e0238318. doi: 10.1371/journal.pone.0238318
Chen, F-T, Hopman, R. J, Huang, C-J, Chu, C-H, Hillman, C. H, and Hung, T-M. (2020). The effect of exercise training on brain structure and function in older adults: a systematic review based on evidence from randomized control trials. J. Clin. Med . 9:914. doi: 10.3390/jcm9040914
Chen, T., Yue, G. H., Tian, Y., and Jiang, C. (2017). Baduanjin mind-body intervention improves the executive control function. Front. Psychol . 7:2015. doi: 10.3389/fpsyg.2016.02015
Coetsee, C., and Terblanche, E. (2017). Cerebral oxygenation during cortical activation: the differential influence of three exercise training modalities. A randomized controlled trial. Eur. J. Appl. Physiol . 117, 1617–1627. doi: 10.1007/s00421-017-3651-8
Eggenberger, P., Wolf, M., Schumann, M., and de Bruin, ED. (2016). Exergame and balance training modulate prefrontal brain activity during walking and enhance executive function in older adults. Front. Aging Neurosci . 8:66. doi: 10.3389/fnagi.2016.00066
Endo, K., Matsukawa, K., Liang, N., Nakatsuka, C., Tsuchimochi, H., Okamura, H., et al. (2013). Dynamic exercise improves cognitive function in association with increased prefrontal oxygenation. J. Physiol. Sci . 63, 287–298. doi: 10.1007/s12576-013-0267-6
Fujihara, H., Megumi, A., and Yasumura, A. (2021). The acute effect of moderate-intensity exercise on inhibitory control and activation of prefrontal cortex in younger and older adults. Exp. Brain Res . 239, 1765–1778. doi: 10.1007/s00221-021-06086-9
Hamacher, D., Herold, F., Wiegel, P., Hamacher, D., and Schega, L. (2015). Brain activity during walking: A systematic review. Neurosci. Biobehav. Rev . 57, 310–327. doi: 10.1016/j.neubiorev.2015.08.002
Crossref Full Text | Google Scholar
Hashimoto, N., Yokogawa, M., Kojima, H., Tanaka, S., and Nakagawa, T. (2018). Effect of moderate exercise intensities on the cortical activity in young adults. J. Phys. Ther. Sci . 30, 1257–1261. doi: 10.1589/jpts.30.1257
Herold, F., Aye, N., Hamacher, D., and Schega, L. (2019). Towards the neuromotor control processes of steady-state and speed-matched treadmill and overground walking. Brain Topogr . 32, 472–476. doi: 10.1007/s10548-019-00699-8
Herold, F., Wiegel, P., Scholkmann, F., Thiers, A., Hamacher, D., Schega, L., et al. (2017). Functional near-infrared spectroscopy in movement science: a systematic review on cortical activity in postural and walking tasks. Neurophotonics 4:041403. doi: 10.1117/1.NPh.4.4.041403
Higgins, J. P., Altman, D. G., Gøtzsche, P. C., Jüni, P., Moher, D., Oxman, A. D., et al. (2011). The Cochrane Collaboration's tool for assessing risk of bias in randomised trials. BMJ 343:d5928. doi: 10.1136/bmj.d5928
Higgins, J. P. T., Thomas, J., Chandler, J., Cumpston, M., Li, T., Page, M. J., et al. (2019). Cochrane Handbook for Systematic Reviews of Interventions. 2nd Edition. Chichester (UK): John Wiley and Sons. doi: 10.1002/9781119536604
Hoshi, Y. (2005). Functional near-infrared spectroscopy: potential and limitations in neuroimaging studies. Int. Rev. Neurobiol . 66, 237–266. doi: 10.1016/S0074-7742(05)66008-4
Hyodo, K, Dan, I, Suwabe, K, Kyutoku, Y, Yamada, Y, and Akahori, M. (2012). Acute moderate exercise enhances compensatory brain activation in older adults. Neurobiol. Aging . 33, 2621–2632. doi: 10.1016/j.neurobiolaging.2011.12.022
Ji, Z., Feng, T., Mei, L., Li, A., and Zhang, C. (2019). Influence of acute combined physical and cognitive exercise on cognitive function: an NIRS study. PeerJ . 7:e7418. doi: 10.7717/peerj.7418
Jiang, C., and Wang, Z. (2016). Effects of short-term aerobic exercise on cognitive control by near-infrared spectroscopy. J. Cap. Inst. Phys. Educ . 4, 356–359. doi: 10.14036/j.cnki.cn11-4513.2016.04.015
Kawagoe, T., Onoda, K., and Yamaguchi, S. (2017). Associations among executive function, cardiorespiratory fitness, and brain network properties in older adults. Sci. Rep . 7:40107. doi: 10.1038/srep40107
Kenville, R., Maudrich, T., Carius, D., and Ragert, P. (2017). Hemodynamic response alterations in sensorimotor areas as a function of barbell load levels during squatting: an fNIRS study. Front. Hum. Neurosci . 11:241. doi: 10.3389/fnhum.2017.00241
Kim, H. J., Bae, S., Huh, J. H., Lee, J. W., and Han, D. H. (2021). Hemodynamic changes in response to aerobic exercise: near-infrared spectroscopy study. Int. J. Sports Med . 42, 377–385. doi: 10.1055/a-1198-8465
Kriel, Y., Kerhervé, H. A., Askew, C. D., and Solomon, C. (2016). The effect of active versus passive recovery periods during high intensity intermittent exercise on local tissue oxygenation in 18 - 30 year old sedentary men. PLoS ONE 11:e0163733. doi: 10.1371/journal.pone.0163733
Kujach, S, Byun, K, Hyodo, K, Suwabe, K, Fukuie, T, and Laskowski, R. (2018). A transferable high-intensity intermittent exercise improves executive performance in association with dorsolateral prefrontal activation in young adults. Neuroimage 169, 117–125. doi: 10.1016/j.neuroimage.2017.12.003
Kurz, M. J., Wilson, T. W., and Arpin, D. J. (2012). Stride-time variability and sensorimotor cortical activation during walking. Neuroimage 59, 1602–1607. doi: 10.1016/j.neuroimage.2011.08.084
Lai, Y., Wang, Z., Yue, G. H., and Jiang, C. (2020). Determining whether tennis benefits the updating function in young children: a functional near-infrared spectroscopy study. Appl. Sci . 10:407. doi: 10.3390/app10010407
Lambrick, D., Stoner, L., Grigg, R., and Faulkner, J. (2016). Effects of continuous and intermittent exercise on executive function in children aged 8-10 years. Psychophysiology 53, 1335–1342. doi: 10.1111/psyp.12688
Leff, D. R., Orihuela-Espina, F., Elwell, C. E., Athanasiou, T., Delpy, D. T., Darzi, A. W., et al. (2011). Assessment of the cerebral cortex during motor task behaviours in adults: a systematic review of functional near infrared spectroscopy (fNIRS) studies. Neuroimage 54, 2922–2936. doi: 10.1016/j.neuroimage.2010.10.058
Mandolesi, L., Polverino, A., Montuori, S., Foti, F., Ferraioli, G., Sorrentino, P., et al. (2018). Effects of physical exercise on cognitive functioning and wellbeing: biological and psychological benefits. Front. Psychol . 9:509. doi: 10.3389/fpsyg.2018.00509
Miyashiro, S., Yamada, Y., Muta, T., Ishikawa, H., Abe, T., Hori, M., et al. (2021). Activation of the orbitofrontal cortex by both meditation and exercise: a near-infrared spectroscopy study. PLoS ONE 16:e0247685. doi: 10.1371/journal.pone.0247685
Monroe, D. C., Gist, N. H., Freese, E. C., O'Connor, P. J., McCully, K. K., Dishman, R. K., et al. (2016). Effects of sprint interval cycling on fatigue, energy, and cerebral oxygenation. Med. Sci. Sports Exerc . 48, 615–624. doi: 10.1249/MSS.0000000000000809
Page, M. J., McKenzie, J. E., Bossuyt, P. M., Boutron, I., Hoffmann, T. C., Mulrow, C. D., et al. (2021). The PRISMA 2020 statement: an updated guideline for reporting systematic reviews. BMJ 372:n71. doi: 10.1136/bmj.n71
Scarapicchia, V., Brown, C., Mayo, C., and Gawryluk, J. R. (2017). Functional magnetic resonance imaging and functional near-infrared spectroscopy: insights from combined recording studies. Front. Hum. Neurosci . 11:419. doi: 10.3389/fnhum.2017.00419
Stute, K., Hudl, N., Stojan, R., and Voelcker-Rehage, C. (2020). Shedding light on the effects of moderate acute exercise on working memory performance in healthy older adults: an fNIRS study. Brain Sci . 10:813. doi: 10.3390/brainsci10110813
Verburgh, L., Königs, M., Scherder, E. J., and Oosterlaan, J. (2014). Physical exercise and executive functions in preadolescent children, adolescents and young adults: a meta-analysis. Br. J. Sports Med . 48, 973–979. doi: 10.1136/bjsports-2012-091441
Wen, S., Xia, S., Li, L., Yang, Y., Tan, Z., Jiang, C., et al. (2015a). Effects of acute aerobic exercise on executive function in college students: evidence from fNIRS and behavioural experiments. J. Tianjin Inst. Phys. Educ. 30, 526–531. doi: 10.13297/j.cnki.issn1005-0000.2015.06.011
Wen, S., Xia, S., Li, S., and Jiang, C. (2015b). Effects of acute aerobic loading on executive function in older adults: evidence from FNIRS and behavioural experiments. Sports Sci . 35, 37–45. doi: 10.16469/j.css.201510006
Xu, Z., Hu, M., Wang, Z. R., Li, J., Hou, X. H., Xiang, M. Q., et al. (2019). The positive effect of moderate-intensity exercise on the mirror neuron system: an fNIRS study. Front. Psychol . 10:986. doi: 10.3389/fpsyg.2019.00986
Yanagisawa, H, Dan, I, Tsuzuki, D, Kato, M, Okamoto, M, and Kyutoku, Y. (2010). Acute moderate exercise elicits increased dorsolateral prefrontal activation and improves cognitive performance with Stroop test. Neuroimage 50, 1702–1710. doi: 10.1016/j.neuroimage.2009.12.023
Yang, Y., Chen, T., Shao, M., Yan, S., Yue, G. H., Jiang, C., et al. (2020). Effects of tai chi chuan on inhibitory control in elderly women: an fNIRS study. Front. Hum. Neurosci . 13:476. doi: 10.3389/fnhum.2019.00476
Zhang, Y., Shi, W., Wang, H., Liu, M., and Tang, D. (2021). The impact of acute exercise on implicit cognitive reappraisal in association with left dorsolateral prefronta activation: a fNIRS study. Behav. Brain Res . 406:113233. doi: 10.1016/j.bbr.2021.113233
Keywords: exercise, physical activity, brain plasticity, functional near-infrared spectroscopy (fNIRS), review
Citation: Shen Q-Q, Hou J-M, Xia T, Zhang J-Y, Wang D-L, Yang Y, Luo R, Xin Z-L, Yin H-c and Cui L (2024) Exercise promotes brain health: a systematic review of fNIRS studies. Front. Psychol. 15:1327822. doi: 10.3389/fpsyg.2024.1327822
Received: 25 October 2023; Accepted: 19 March 2024; Published: 10 April 2024.
Reviewed by:
Copyright © 2024 Shen, Hou, Xia, Zhang, Wang, Yang, Luo, Xin, Yin and Cui. This is an open-access article distributed under the terms of the Creative Commons Attribution License (CC BY) . The use, distribution or reproduction in other forums is permitted, provided the original author(s) and the copyright owner(s) are credited and that the original publication in this journal is cited, in accordance with accepted academic practice. No use, distribution or reproduction is permitted which does not comply with these terms.
*Correspondence: Heng-chan Yin, yinhengchan@bnu.edu.cn ; Lei Cui, cuilei@bnu.edu.cn
Disclaimer: All claims expressed in this article are solely those of the authors and do not necessarily represent those of their affiliated organizations, or those of the publisher, the editors and the reviewers. Any product that may be evaluated in this article or claim that may be made by its manufacturer is not guaranteed or endorsed by the publisher.
- Print this article
Improving Mental Health through Physical Activity: A Narrative Literature Review
- Victor M. Tringali
- Ryan C. Thoms
MBA, MS, CSCS
- Victor M. Tringali , Department of Human Resources, University of Virginia, Charlottesville, VA; School of Medicine, Department of Public Health Sciences, University of Virginia, Charlottlesville, VA, United States
- Ryan C. Thoms , Department of Kinesiology, School of Education and Human Development, Charlottesville, VA, United States
- Page/Article: 146–153
- DOI: 10.5334/paah.108
- Accepted on 23 Jun 2021
- Published on 5 Aug 2021
- Peer Reviewed
- Open access
- Published: 13 April 2024
Associations between transport modes and site-specific cancers: a systematic review and meta-analysis
- Win Thu 1 ,
- Alistair Woodward 1 ,
- Alana Cavadino 1 &
- Sandar Tin Tin 1 , 2
Environmental Health volume 23 , Article number: 39 ( 2024 ) Cite this article
Metrics details
Physical inactivity is a global public health problem. A practical solution would be to build physical activity into the daily routine by using active modes of transport. Choice of transport mode can influence cancer risk through their effects on levels of physical activity, sedentary time, and environmental pollution. This review synthesizes existing evidence on the associations of specific transport modes with risks of site-specific cancers.
Relevant literature was searched in PubMed, Embase, and Scopus from 1914 to 17th February 2023. For cancer sites with effect measures available for a specific transport mode from two or more studies, random effects meta-analyses were performed to pool relative risks (RR) comparing the highest vs. lowest activity group as well as per 10 Metabolic Equivalent of Task (MET) hour increment in transport-related physical activity per week ( ∼ 150 min of walking or 90 min of cycling).
27 eligible studies (11 cohort, 15 case-control, and 1 case-cohort) were identified, which reported the associations of transport modes with 10 site-specific cancers. In the meta-analysis, 10 MET hour increment in transport-related physical activity per week was associated with a reduction in risk for endometrial cancer (RR: 0.91, 95% CI: 0.83–0.997), colorectal cancer (RR: 0.95, 95% CI: 0.91–0.99) and breast cancer (RR: 0.99, 95% CI: 0.89–0.996). The highest level of walking only or walking and cycling combined modes, compared to the lowest level, were significantly associated with a 12% and 30% reduced risk of breast and endometrial cancers respectively. Cycling, compared to motorized modes, was associated with a lower risk of overall cancer incidence and mortality.
Active transport appears to reduce cancer risk, but evidence for cancer sites other than colorectum, breast, and endometrium is currently limited.
Peer Review reports
Introduction
Physical inactivity is a global public health problem, contributing to substantial disease and economic burden worldwide [ 1 , 2 ]. With rapid changes in technology, lifestyle, and habitual environment, people have been less active and more sedentary over the past few decades. Globally, about 1 in 4 adults were not active, i.e., did not meet the World Health Organization (WHO) recommendation of engaging at least 150–300 min of moderate-intensity or 75–150 min of vigorous-intensity aerobic physical activity per week [ 3 ], but the prevalence varied widely within and across countries [ 4 ]. If the current trends continue, it is unlikely that the WHO’s target to reduce physical inactivity by 10% in 2025 will be met.
One practical solution would be to build physical activity into the daily routine by using active modes of transport [ 5 ]. Walking and cycling have been shown to improve health (mainly all-cause mortality, cardiovascular disease, diabetes, and cancer) [ 6 ] and also provide social, economic and environmental benefits [ 7 , 8 ]. Car use, on the other hand, contributes to a significant proportion of daily sedentary time, and the situation is worsening with increasing traffic congestion/delays [ 9 ]; it has been associated with an increased risk of obesity and related outcomes [ 10 ]. Further, exposure to environmental pollutants such as nitrogen dioxide and/or particulate matter could differ across different road users [ 11 ], while it has been shown to increase the risk of certain cancers, particularly lung cancer [ 12 ].
While there is ample evidence linking leisure time physical activity or physical activity in general with a reduced risk for a number of cancer sites [ 13 , 14 ], and sedentary behavior in general with an increased risk [ 15 ], the findings may not be directly applicable to transport-related activity because the context and correlates of activity as well as its frequency, duration and intensity are likely to be different across different domains. We therefore reviewed the existing literature that reported the associations between transport modes and risks of site-specific cancers.
A systematic literature review and meta-analysis was conducted and reported according to the PRISMA guideline (Supplementary file S1 ). The review was not registered.
Search strategy and study selection
Relevant literature was searched from 1914 to 17th February 2023 in PubMed, Scopus, and Embase databases using the relevant search terms such as walking, cycling, car, public transport, commute and cancers. Site-specific cancers known to be associated with physical activity and body weight such as breast, colon, liver, esophageal adenocarcinoma and those associated with environmental factor such as lung and melanoma of skin were also searched (Supplementary file S2 ). The reference lists of systematic reviews on physical activity and cancers were also reviewed. Studies were included if they (1) used cohort, case-control, case-cohort or experimental design, (2) assessed transport modes such as walking, cycling, public transport or car use as the exposures of interest, (3) investigated one or more site-specific cancers, overall cancer incidence and/or mortality as the outcome(s), (4) reported effect measures associated with transport modes, and (5) published the full article in English. Studies that used cross-sectional design or mathematical modeling to estimate health impacts at the population level were excluded. Details of excluded studies after full text review, together with the reasons for exclusion, were provided in the Supplementary file S3 . WT conducted the search and selection, and STT oversaw the process.
Data extraction and study quality assessment
Information about title, first author, year of publication, study name (if available), country, study design, sample size, age range of the participants, follow-up duration (for cohort and case-cohort studies), data collection tool, measurement units for exposure(s), data sources for outcome(s), site-specific cancer assessed, effect measures, and confounders adjusted were extracted in a standardized data collection spreadsheet. The study quality was evaluated using the Newcastle-Ottawa Scale (NOS) [ 16 ], which scores the cohort and case-control studies based on three domains: selection of study groups, comparability of the groups and ascertainment of exposure (case-control studies) or outcome (cohort studies). For the second domain, a point was awarded for adjustment of Body Mass Index (BMI) - to evaluate the direct vs. indirect (through BMI) effect of physical activity on cancer risk, and another point for adjustment of physical activities from other domains - to isolate the effects of transport-related physical activity from other activities. A maximum of nine points were awarded, with a higher score indicating better quality [ 16 ]. For case-cohort studies, the NOS scale for cohort studies was used. WT conducted the data extraction and quality assessment, and STT oversaw the process.
Data analysis
For cancer sites with effect measures available for a specific transport mode from two or more studies, meta-analyses were performed using random effects models. The analyses compared the highest level of active transport such as walking, cycling or mixed mode with the lowest level as reported in the individual studies. Where necessary, the reference category for exposure was changed to the lowest group to facilitate pooling of the risks [ 17 ]. The pooled relative risks (RRs) and 95% CI were presented for breast, endometrial, colorectal and testicular cancers, and overall cancer mortality.
For studies that reported time or MET as measurement units, the dose-response effects were estimated using the trend estimation method proposed by Greenland and Longnecker [ 18 ]. The reported time spent for each mode/category was converted to MET hours (see Supplementary file S4 for conversion values and formulas used). For studies that only reported estimates for categorical exposures, study-specific slopes were calculated from the natural logs of the reported risk estimates across categories and risk estimates per unit change were then estimated. The pooled results were presented per 10 Metabolic Equivalent of Task (MET) hour increment in transport-related physical activity per week ( ∼ 150 min of walking or 90 min of cycling) to align with the WHO’s physical activity recommendation [ 3 ]. This approach enabled us to pool risk estimates from a large number of studies irrespective of how the exposures were assessed (e.g., walking and cycling separately or combined) or categorised. The results were presented for breast, endometrial, colorectal, prostate cancers, and overall cancer mortality.
Meta-analysis was not conducted for the studies that compared active and non-active modes in relation to overall cancer incidence and mortality due to the potential overlap of the study samples.
For meta-analyses involving four or more studies, publication bias was assessed through the visual inspection of funnel plots, Begg’s rank correlation test, and Egger’s regression test for asymmetry. If significant associations were observed, sensitivity analyses were conducted by removing one study at a time from the initial meta-analysis to test the robustness of the results. Where possible, sub-group analyses were performed to assess variability of summary effects across population groups (Western vs. Asian), study design (cohort vs. case-control), measurement units (time vs. MET) and adjustment for BMI (yes vs. no). Metafor [ 19 ] and dosresmeta [ 20 ] R packages were used for meta-analysis and trend estimation. All authors have access to the data.
Of the 11,829 records identified, 27 unique studies (total 34 publications) were included, of which 22 studies (28 records) contributed to the meta-analyses (Fig. 1 ). There were four publications from the Netherlands Cohort Study which reported endometrial [ 21 ], ovarian [ 22 ], prostate [ 23 ], and colorectal [ 24 ] cancers, three publications from United Kingdom Biobank which reported lung [ 25 ], breast and colon [ 26 ], and overall cancer incidence and mortality [ 27 ], two publications from Shanghai Women’s Health Study which reported breast [ 28 ] and overall cancer mortality [ 29 ], and two publications from National Institutes of Health - American Association of Retired Persons Diet and Health Study which reported breast [ 30 ] and endometrial [ 31 ] cancers. Of the included studies, 20 compared the risks between the highest and lowest levels of active transport (e.g., walking, cycling, walking and cycling) and two compared the risk between active and non-active commuting modes. The majority used case-control design ( n = 15), followed by cohort ( n = 11) and case-cohort ( n = 1) designs. Most of the studies were conducted in North America, mainly in the United States (US) ( n = 7), followed by Europe ( n = 5), China ( n = 5), United Kingdom ( n = 4), Australia ( n = 2) and the remaining four studies were from India, Iran, Brazil and Nigeria. (Table 1 )
Almost half of the studies assessed walking and cycling combined, i.e., did not provide the risk estimates for each mode ( n = 13), while others assessed walking and cycling separately ( n = 8), or assessed only one mode (walking: n = 3 and cycling: n = 3). Most studies quantified active transport in terms of time spent (e.g., minutes per day, hours per week) ( n = 14) or MET ( n = 7), but others assessed it in terms of activity status (e.g., yes, no) ( n = 3), or in comparison to car or motorized mode ( n = 2), and distance ( n = 1) (Supplementary file S5 ). The studies reported the risks associated with ten site-specific cancers, most commonly breast ( n = 12), endometrial ( n = 5), and colorectal ( n = 4) cancers (Fig. 2 ). Cancer cases were identified through cancer registries, death registries, pathological reports, or hospital or medical records (Supplementary file S5 ). The NOS score for cohort studies ranged from 5 to 9, with an average score of 6.5, and the score for case-control studies ranged from 4 to 7, with an average score of 5.6 (Table 1 , detailed scoring in Supplementary file S6 , S7 ).
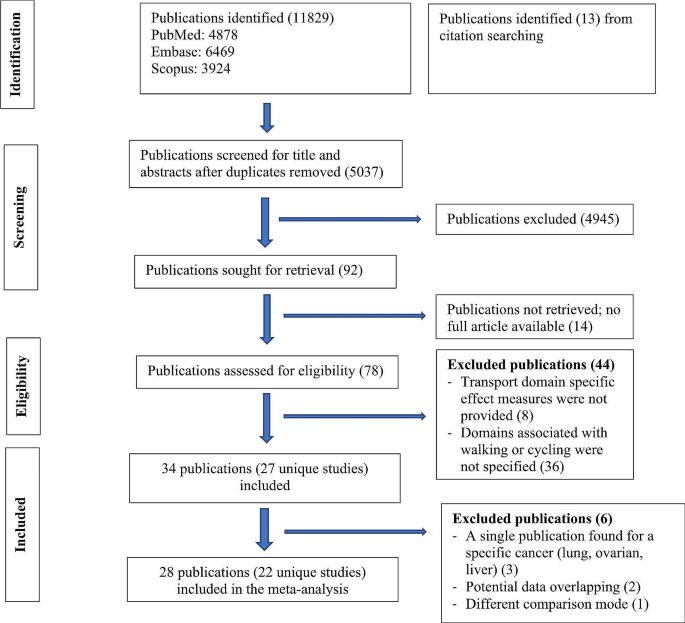
Flow diagram for study selection
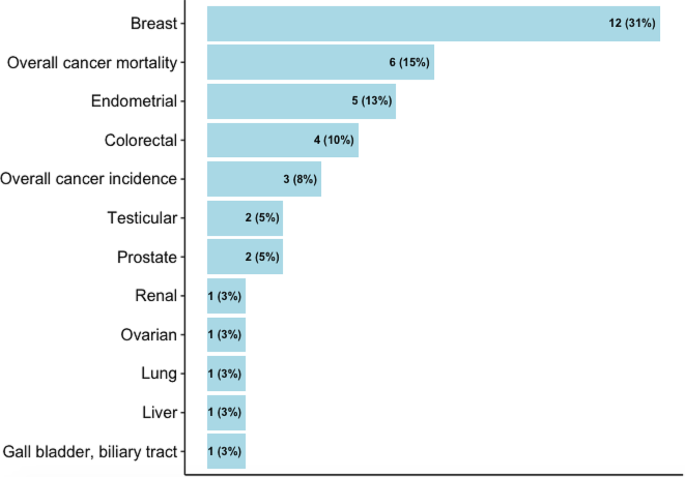
Cancers reported in the studies
Active transport studies
The pooled results were presented for breast, endometrial, colorectal, testicular and prostate cancers, and overall cancer mortality (Fig. 3 ). For other cancers where only one study was identified, the results from the individual study were presented.
Breast cancer
In the meta-analysis of six studies comparing the highest vs. lowest activity group, an inverse association was observed for walking (RR: 0.88, 95% CI: 0.78–0.98), a borderline inverse association for cycling (RR: 0.90, 95% CI: 0.77–1.05) and no significant association for walking and cycling combined (RR: 0.97, 95% CI: 0.84–1.12). 10 MET hour increment in transport-related physical activity per week ( ∼ 150 min of walking or 90 min of cycling) was associated with a marginally reduced risk (RR: 0.99, 95% CI: 0.97–0.996). (Fig. 3 , detailed forest plots in the supplementary file S9 )
Endometrial cancer
The meta-analysis of four studies indicated that walking and cycling combined was associated with a reduced risk of endometrial cancer (RR comparing highest vs. lowest: 0.70, 95% CI: 0.56–0.87; RR per 10 MET hour increment in activity per week: 0.91, 95% CI: 0.83–0.997). (Fig. 3 , detailed forest plots in S9 )
Colorectal cancer
In the meta-analysis of two studies, walking and cycling combined was associated with a reduced risk of colorectal cancer (RR comparing highest vs. lowest: 0.89, 95% CI: 0.78–1.01; RR per 10 MET hour increment in activity per week: 0.95, 95% CI: 0.91–0.99) (Fig. 3 , detailed forest plot in S9 ).
Testicular cancer
In the meta-analysis of two studies, there was no significant association between cycle commuting in adolescence and testicular germ cell cancer (RR comparing highest vs. lowest: 1.23, 95% CI: 0.71–2.13). (Fig. 3 , detailed forest plot in S9 )
Prostate cancer
10 MET hour increment per week for transport related physical activity was associated with a reduced risk of prostate cancer (RR: 0.96, 95% CI: 0.88–1.04) (Fig. 3 , detailed forest plot in S9 ).
Ovarian cancer
Only a case-cohort study assessed the relationship of walking and cycling combined mode with ovarian cancer risk, and reported no significant association (Supplementary file S5 ) [ 22 ].
Liver, gallbladder and biliary tract cancers
A cohort study reported a significant association of commuting physical activity with a reduced risk of gallbladder and biliary tract cancers in women (HR: 0.51, 95% CI: 0.28–0.94) but not in men (HR: 0.92, 95% CI: 0.61–1.37); there was no significant association with liver cancer in both sexes (supplementary file S5 ) [ 49 ].
Renal cancer
A case-control study assessed the association of walking and cycling with risk of renal cell carcinoma in white and black participants in the ages of 20s and 50s, and reported a significant association in the white participants in their 20s (OR comparing lowest vs. highest: 1.42, 95% CI: 1.10–1.83) but not in the black counterparts; the associations were also not significant in both groups in their 50s. (Supplementary file S5 ) [ 50 ].
Overall cancer mortality
In the meta-analyses of two studies, there was an inverse association for cycling only (RR comparing highest vs. lowest: 0.60, 95% CI: 0.34–1.04) and walking and cycling combined (RR: 0.98, 95% CI: 0.86–1.12), and also per 10 MET hour increment in activity per week (RR: 0.97, 95% CI: 0.92–1.01). (Fig. 3 , detailed forest plots in S9 )
In sub-group analyses, similar associations were observed between walking and breast cancer risk in terms of study design (cohort, case-control), population (western vs. Asian), measurement unit (time vs. MET), menopausal status (premenopausal and postmenopausal) and adjustment of BMI (yes vs. no); however, the associations were stronger in studies that adjusted for physical activity from other domains (Supplementary file S10 ). In the leave-one-out analyses assessing walking and breast cancer risk, the results were sensitive to effect sizes from some studies, but this was not the case for walking and cycling combined mode and endometrial cancer (Supplementary file S11 ). There was no evidence for funnel plot asymmetry; Egger’s regression tests and Begg’s ranks correlation tests were not significant (Supplementary file S12 ).
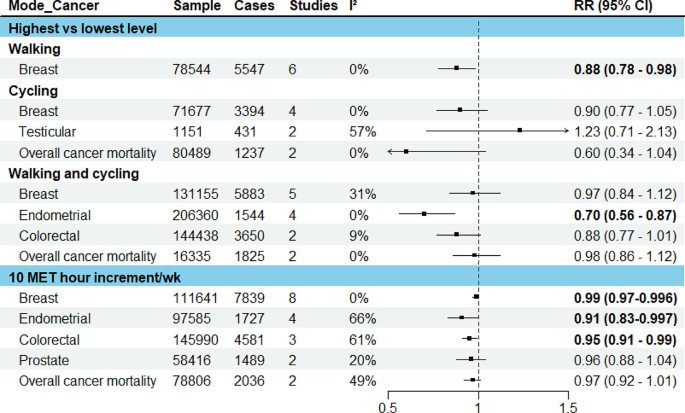
Results of meta-analysis for active transport studies. RE = a random-effects model, MET = Metabolic Equivalent of Task, I 2 = I 2 statistics for heterogeneity, RR = Summary relative risk
Studies comparing active vs. non-active modes for commuting
Four eligible publications were identified, of which three used the data from UK Biobank [ 25 , 26 , 27 ], one used the UK census data [ 51 ]. Three reported the associations for overall cancer incidence and mortality, and one reported the risk associated with lung cancer (Fig. 4 ). In the study that assessed lung cancer using the data from UK Biobank, when compared to automobile only mode, active modes did not show a significant association whereas frequent use of public transport (≥ 5 trips per week) was associated with an increased risk of lung cancer (HR: 1.58, 95% CI: 1.08–2.33) [ 25 ] (Fig. 4 ). In another UK Biobank study, no significant associations were observed for breast and colon cancers, and overall cancer incidence and mortality when more active patterns of commuting (walking, cycling, public transport, either alone or in combination with car) were compared to car only mode [ 26 ].
The results of two studies [ 27 , 51 ] that assessed overall cancer incidence and mortality were not combined as the outcome data was extracted from the same national cancer registry with an overlapped time frame (1991–2011 and 2007–2014), although the exposure information came from different sources (census and UK Biobank). In these studies, compared to private motorized mode or non-active mode, cycling was inversely associated with overall cancer incidence and mortality. Walking and public transport were also inversely associated with overall cancer incidence in the study that used the census data [ 51 ].
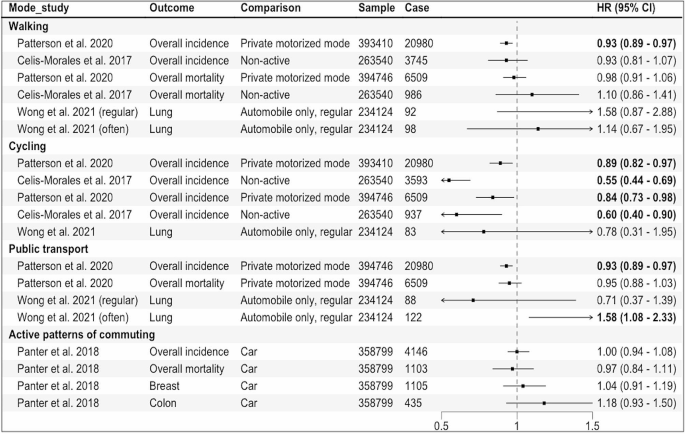
Results of the individual studies comparing active vs. non-active modes for commuting. Private motorized mode = car or motorcycle, Non-active = car or public transport, Active patterns of commuting = any other patterns including walking, cycling, public transport, either alone or in combination with car, HR = Hazard Ratio, regular:1–4, often: ≥5 work-bound trips/week
This review identified 27 studies (34 publications) that reported the associations of specific transport modes, mainly active transport modes, with risks of ten site-specific cancers along with overall cancer incidence and mortality. The most frequently studied cancer sites were breast, endometrium, and colorectum; our meta-analysis showed a reduction in risk of these cancers (1%, 9% and 5%, respectively) per 10 MET hour per week increment in transport-related physical activity ( ∼ 150 min of walking or 90 min of cycling).
We found an inverse association between active transport and risks of breast and endometrial cancers, with similar magnitude of risk reduction observed in previous systematic reviews on physical activity in general [ 55 , 56 ]. While obesity is known to increase post-menopausal but not pre-menopausal breast cancer risk [ 57 ], we found similar results by menopausal status. In contrast, an earlier review did not find any significant association between walking in general and risk of pre- or post-menopausal breast cancer [ 58 ], possibly because compared to walking for transport, walking for leisure or at home generally uses lower energy [ 59 ], and therefore may have less effect on body weight.
The inverse association of active transport with colorectal cancer risk observed in this review is also consistent with the findings from existing reviews on transport-related physical activity [ 60 ] as well as physical activity in general [ 61 ]. While physical activity in general or for leisure has also been associated with a reduced risk of many other cancer sites including liver, gastric, renal and lung [ 13 , 14 ], the evidence related to transport-related physical activity is currently limited.
Mechanisms linking physical activity with specific cancer sites have been proposed, including its effects on sex hormones (breast, endometrial and prostate cancers), insulin sensitivity, glucose metabolism and adipokines (obesity-related cancers), and inflammation and immune function (most cancers) [ 62 ]. For colorectal cancer, another potential mechanism is reduced contact time between carcinogens and bowel mucosa cells due to exercise-induced intestinal mobility [ 63 ].
The overall quality of the included studies, evaluated by NOS score, ranged from 4 to 9, and in general, cohort studies tend to have higher scores compared to case-control studies. The common criteria the studies did not meet include: inadequate exposure assessment, loss to follow-up (cohort studies) and low response rates (case-control studies). While we were not able to undertake subgroup analyses by NOS score due to the limited number of studies available, our subgroup analyses by study design showed similar associations between walking and breast cancer in cohort vs. case-control studies.
To our knowledge, this review represents the first systematic attempt to synthesize the existing evidence on specific transport modes and site-specific cancers. We provided mode-specific summary effects where possible and calculated the dose-response effects for transport-related physical activity, in line with WHO physical activity recommendation. When interpreting the findings, some limitations need to be considered. First, the review may not have included some eligible studies published in languages other than English. Second, due to the limited number of available studies, we were not able to pool the results separately for cohort and case-control studies; however, we conducted sub-group analyses by study design where possible. We were not able to evaluate the non-linear relationship between transport-related physical activity and the risks of site-specific cancers. While a recent systematic review on breast and colon cancers reported a linear relationship with physical activity [ 64 ], others suggested a non-linear relationship between physical activity and cancer risk [ 65 , 66 ]. Further, variations in measurement and categorization of the exposure across the studies make direct comparison of the results between different modes (e.g., walking vs. cycling) difficult. Finally, the majority of the studies included were conducted in high income countries in Europe, UK, and North America, limiting the generalizability of the findings to other populations and low and middle income countries where urbanization and motorization are mainly taking place [ 67 ].
Our findings suggest that transport choices may influence cancer risk, particularly of obesity-related cancers such as breast, colon and endometrial cancers. Breast cancer is the most common cancer in women globally, with an estimated over 2 million new cases (11.7% of all new cases) in 2020, while colon cancer stood at fourth place (over 1 million cases, 6% of total cases) [ 68 ]. The incidence of endometrial cancer also seems to be increasing in many countries particularly in younger women. Our findings indicate that the risks of these cancers can be reduced by meeting the WHO physical activity recommendation through active commuting ( ∼ 150 min of walking or 90 min of cycling per week). Yet, the current evidence is limited in relation to other cancer sites, underlying mechanisms, and potential environmental influences, requiring further exploration.
Given heterogeneity in exposure measurements in the existing studies, harmonizing choice of the assessment tool (e.g., using International Physical Activity Questionnaires that can capture information about all four physical activity domains including transport modes), and reporting the dose-response estimates for each transport mode such as walking and cycling separately rather than a combined mode would enhance comparability of results and provide mode-specific effects. Repeated or regular assessments of exposures/transport modes used throughout the study duration would capture changes and their potential impact on outcomes in cohort studies. Importantly, more research is needed in low and middle-income settings to generate context-specific evidence.
In conclusion, active transport modes appear to reduce cancer risk, but evidence for cancer sites other than colorectum, breast and endometrium is currently limited.
Data availability
No datasets were generated or analysed during the current study.
Lee IM, Shiroma EJ, Lobelo F, Puska P, Blair SN, Katzmarzyk PT. Effect of physical inactivity on major non-communicable diseases worldwide: an analysis of burden of disease and life expectancy. Lancet. 2012;380(9838):219–29. https://doi.org/10.1016/S0140-6736(12)61031-9 .
Article Google Scholar
Ding D, Lawson KD, Kolbe-Alexander TL, et al. The economic burden of physical inactivity: a global analysis of major non-communicable diseases. Lancet. 2016;388(10051):1311–24. https://doi.org/10.1016/S0140-6736(16)30383-X .
World Health Organization. Global status report on physical activity 2022.; 2022.
Guthold R, Stevens GA, Riley LM, Bull FC. Worldwide trends in insufficient physical activity from 2001 to 2016: a pooled analysis of 358 population-based surveys with 1·9 million participants. Lancet Global Health. 2018;6(10):e1077–86. https://doi.org/10.1016/S2214-109X(18)30357-7 .
Berrigan D, Troiano RP, McNeel T, DiSogra C, Ballard-Barbash R. Active transportation increases adherence to activity recommendations. Am J Prev Med. 2006;31(3):210–6. https://doi.org/10.1016/j.amepre.2006.04.007 .
Dinu M, Pagliai G, Macchi C, Sofi F. Active commuting and multiple Health outcomes: a systematic review and Meta-analysis. Sports Med. 2019;49(3):437–52. https://doi.org/10.1007/s40279-018-1023-0 .
Boniface S, Scantlebury R, Watkins SJ, Mindell JS. Health implications of transport: evidence of effects of transport on social interactions. J Transp Health. 2015;2(3):441–6. https://doi.org/10.1016/j.jth.2015.05.005 .
Higgins PAT. Exercise-based transportation reduces oil dependence, carbon emissions and obesity. Envir Conserv. 2005;32(3):197–202. https://doi.org/10.1017/S037689290500247X .
Article CAS Google Scholar
World Health Organization. Global Status Report on Road Safety 2018: Summary.; 2018.
Sugiyama T, Chandrabose M, Homer AR, Sugiyama M, Dunstan DW, Owen N. Car use and cardiovascular disease risk: systematic review and implications for transport research. J Transp Health. 2020;19:100930. https://doi.org/10.1016/j.jth.2020.100930 .
Panchal R, Panagi M, May HR, et al. Personal air pollution exposure during morning commute car and active transport journeys. J Transp Health. 2022;26:101365. https://doi.org/10.1016/j.jth.2022.101365 .
Turner MC, Andersen ZJ, Baccarelli A, et al. Outdoor air pollution and cancer: an overview of the current evidence and public health recommendations. CA Cancer J Clin. 2020;70(6):460–79. https://doi.org/10.3322/caac.21632 .
Moore SC, Lee IM, Weiderpass E, et al. Association of leisure-time physical activity with risk of 26 types of Cancer in 1.44 million adults. JAMA Intern Med. 2016;176(6):816. https://doi.org/10.1001/jamainternmed.2016.1548 .
Rezende LFMD, Sá THD, Markozannes G, et al. Physical activity and cancer: an umbrella review of the literature including 22 major anatomical sites and 770 000 cancer cases. Br J Sports Med. 2018;52(13):826–33. https://doi.org/10.1136/bjsports-2017-098391 .
Hermelink R, Leitzmann MF, Markozannes G, et al. Sedentary behavior and cancer–an umbrella review and meta-analysis. Eur J Epidemiol. 2022;37(5):447–60. https://doi.org/10.1007/s10654-022-00873-6 .
Wells G, Shea B, O’Connell D et al. The Newcastle-Ottawa Scale (NOS) for assessing the quality of nonrandomised studies in meta-analyses. The Newcastle-Ottawa Scale (NOS) for assessing the quality of nonrandomised studies in meta-analyses. https://www.ohri.ca/programs/clinical_epidemiology/oxford.asp .
Taylor K. Wanting a particular reference category in categorical risk data. Data extraction tips for meta-analysis. https://www.cebm.ox.ac.uk/resources/data-extraction-tips-meta-analysis/reference-category-risk-data .
Greenland S, Longnecker MP. Methods for Trend Estimation from Summarized Dose-Response Data, with applications to Meta-Analysis. Am J Epidemiol. 1992;135(11):1301–9. https://doi.org/10.1093/oxfordjournals.aje.a116237 .
Viechtbauer W. Conducting Meta-analyses in R with the metafor Package. J Stat Soft. 2010;36(3). https://doi.org/10.18637/jss.v036.i03 .
Crippa A, Orsini N. Multivariate Dose-Response Meta-Analysis: The dosresmeta R Package. J Stat Soft. 2016;72(Code Snippet 1). https://doi.org/10.18637/jss.v072.c01 .
Schouten LJ, Goldbohm RA, van den Brandt PA, Anthropometry. Physical activity, and Endometrial Cancer Risk: results from the Netherlands Cohort Study. JNCI J Natl Cancer Inst. 2004;96(21):1635–8. https://doi.org/10.1093/jnci/djh291 .
Biesma RG, Schouten LJ, Dirx MJM, Goldbohm RA, van den Brandt PA. Physical activity and risk of ovarian Cancer: results from the Netherlands Cohort Study (the Netherlands). Cancer Causes Control. 2006;17(1):109–15. https://doi.org/10.1007/s10552-005-0422-3 .
Zeegers MPA, Dirx MJM, van den Brandt PA. Physical activity and the risk of prostate Cancer in the Netherlands Cohort Study, results after 9.3 years of follow-up. Cancer Epidemiol Biomarkers Prev. 2005;14(6):1490–5. https://doi.org/10.1158/1055-9965.EPI-04-0771 .
Simons CCJM, Hughes LAE, van Engeland M, Goldbohm RA, van den Brandt PA, Weijenberg MP. Physical activity, occupational sitting time, and Colorectal Cancer Risk in the Netherlands Cohort Study. Am J Epidemiol. 2013;177(6):514–30. https://doi.org/10.1093/aje/kws280 .
Wong JYY, Jones RR, Breeze C, et al. Commute patterns, residential traffic-related air pollution, and lung cancer risk in the prospective UK Biobank cohort study. Environ Int. 2021;155:106698. https://doi.org/10.1016/j.envint.2021.106698 .
Panter J, Mytton O, Sharp S, et al. Using alternatives to the car and risk of all-cause, cardiovascular and cancer mortality. Heart. 2018;104(21):1749–55. https://doi.org/10.1136/heartjnl-2017-312699 .
Celis-Morales CA, Lyall DM, Welsh P, et al. Association between active commuting and incident cardiovascular disease, cancer, and mortality: prospective cohort study. BMJ Published Online April. 2017;19:j1456. https://doi.org/10.1136/bmj.j1456 .
Pronk A, Ji BT, Shu XO, et al. Physical activity and breast cancer risk in Chinese women. Br J Cancer. 2011;105(9):1443–50. https://doi.org/10.1038/bjc.2011.370 .
Matthews CE, Jurj AL, Shu Xo, et al. Influence of Exercise, walking, Cycling, and overall nonexercise physical activity on Mortality in Chinese women. Am J Epidemiol. 2007;165(12):1343–50. https://doi.org/10.1093/aje/kwm088 .
George SM, Irwin ML, Matthews CE, et al. Beyond recreational physical activity: examining Occupational and Household Activity, Transportation Activity, and sedentary behavior in relation to postmenopausal breast Cancer Risk. Am J Public Health. 2010;100(11):2288–95. https://doi.org/10.2105/AJPH.2009.180828 .
Gierach GL, Chang SC, Brinton LA, et al. Physical activity, sedentary behavior, and endometrial cancer risk in the NIH-AARP Diet and Health Study. Int J Cancer. 2009;124(9):2139–47. https://doi.org/10.1002/ijc.24059 .
Luoto R, Latikka P, Pukkala E, Hakulinen T, Vihko V. The effect of physical activity on breast cancer risk: a cohort study of 30,548 women. Eur J Epidemiol. 2000;16(10):973–80. https://doi.org/10.1023/A:1010847311422 .
Gomes MLB, Pinto SS, Domingues MR. Physical activity and breast Cancer: a case-control study in Southern Brazil. Nutr Cancer. 2022;74(1):149–57. https://doi.org/10.1080/01635581.2021.1880607 .
Azubuike SO, Hayes L, Sharp L, Alabi A, Oyesegun RA, McNally R. Physical activity and the risk of breast cancer among Nigerian women. Cancer Epidemiol. 2022;78:102163. https://doi.org/10.1016/j.canep.2022.102163 .
Si S, Boyle T, Heyworth J, Glass DC, Saunders C, Fritschi L. Lifetime physical activity and risk of breast cancer in pre-and post-menopausal women. Breast Cancer Res Treat. 2015;152(2):449–62. https://doi.org/10.1007/s10549-015-3489-x .
Mathew A, Gajalakshmi V, Rajan B, et al. Physical activity levels among urban and rural women in south India and the risk of breast cancer: a case–control study. Eur J Cancer Prev. 2009;18(5):368–76. https://doi.org/10.1097/CEJ.0b013e32832e1c46 .
Steindorf K. Case-control study of physical activity and breast Cancer risk among Premenopausal women in Germany. Am J Epidemiol. 2003;157(2):121–30. https://doi.org/10.1093/aje/kwf181 .
John EM, Horn-Ross PL, Koo J. Lifetime physical activity and breast cancer risk in a multiethnic population: the San Francisco Bay area breast cancer study. Cancer Epidemiol Biomarkers Prev. 2003;12(11 Pt 1):1143–52.
Google Scholar
Matthews CE, Shu XO, Jin F, et al. Lifetime physical activity and breast cancer risk in the Shanghai breast Cancer Study. Br J Cancer. 2001;84(7):994–1001. https://doi.org/10.1054/bjoc.2000.1671 .
Marcus PM, Newman B, Moorman PG, et al. Physical activity at age 12 and adult breast cancer risk (United States). Cancer Causes Control. 1999;10(4):293–302. https://doi.org/10.1023/A:1008971417282 .
Friberg E, Mantzoros CS, Wolk A. Physical activity and risk of Endometrial Cancer: a Population-based prospective cohort study. Cancer Epidemiol Biomarkers Prev. 2006;15(11):2136–40. https://doi.org/10.1158/1055-9965.EPI-06-0465 .
John EM, Koo J, Horn-Ross PL. Lifetime physical activity and risk of Endometrial Cancer. Cancer Epidemiol Biomarkers Prev. 2010;19(5):1276–83. https://doi.org/10.1158/1055-9965.EPI-09-1316 .
Matthews CE, Xu WH, Zheng W, et al. Physical activity and risk of Endometrial Cancer: a report from the Shanghai Endometrial Cancer Study. Cancer Epidemiol Biomarkers Prev. 2005;14(4):779–85. https://doi.org/10.1158/1055-9965.EPI-04-0665 .
Mahmood S, English DR, MacInnis RJ, et al. Domain-specific physical activity and the risk of colorectal cancer: results from the Melbourne Collaborative Cohort Study. BMC Cancer. 2018;18(1):1063. https://doi.org/10.1186/s12885-018-4961-x .
Hou L. Commuting physical activity and risk of Colon cancer in Shanghai, China. Am J Epidemiol. 2004;160(9):860–7. https://doi.org/10.1093/aje/kwh301 .
Littman AJ, Doody DR, Biggs ML, Weiss NS, Starr JR, Schwartz SM. Physical activity in adolescence and testicular germ cell cancer risk. Cancer Causes Control. 2009;20(8):1281–90. https://doi.org/10.1007/s10552-009-9347-6 .
Coldman AJ, Elwood JM, Gallagher RP. Sports activities and risk of testicular cancer. Br J Cancer. 1982;46(5):749–56. https://doi.org/10.1038/bjc.1982.267 .
Hosseini M, SeyedAlinaghi S, Mahmoudi M, McFarland W. A case-control study of risk factors for prostate cancer in Iran. Acta Med Iran. 2010;48(1):61–6.
Pang Y, Lv J, Kartsonaki C, et al. Association of physical activity with risk of hepatobiliary diseases in China: a prospective cohort study of 0.5 million people. Br J Sports Med. 2021;55(18):1024–33. https://doi.org/10.1136/bjsports-2020-102174 .
Xiao Q, Liao L, Matthews CE, et al. Physical activity and renal cell carcinoma among black and white americans: a case-control study. BMC Cancer. 2014;14(1):707. https://doi.org/10.1186/1471-2407-14-707 .
Patterson R, Panter J, Vamos EP, Cummins S, Millett C, Laverty AA. Associations between commute mode and cardiovascular disease, cancer, and all-cause mortality, and cancer incidence, using linked Census data over 25 years in England and Wales: a cohort study. Lancet Planet Health. 2020;4(5):e186–94. https://doi.org/10.1016/S2542-5196(20)30079-6 .
Sahlqvist S, Goodman A, Simmons RK, et al. The association of cycling with all-cause, cardiovascular and cancer mortality: findings from the population-based EPIC-Norfolk cohort. BMJ Open. 2013;3(11):e003797. https://doi.org/10.1136/bmjopen-2013-003797 .
Autenrieth CS, Baumert J, Baumeister SE, et al. Association between domains of physical activity and all-cause, cardiovascular and cancer mortality. Eur J Epidemiol. 2011;26(2):91–9. https://doi.org/10.1007/s10654-010-9517-6 .
Batty GD, Shipley MJ, Marmot M, Smith GD. Physical activity and cause-specific mortality in men: further evidence from the Whitehall study. Eur J Epidemiol. 2001;17(9):863–9. https://doi.org/10.1023/A:1015609909969 .
Wu Y, Zhang D, Kang S. Physical activity and risk of breast cancer: a meta-analysis of prospective studies. Breast Cancer Res Treat. 2013;137(3):869–82. https://doi.org/10.1007/s10549-012-2396-7 .
Moore SC, Gierach GL, Schatzkin A, Matthews CE. Physical activity, sedentary behaviours, and the prevention of endometrial cancer. Br J Cancer. 2010;103(7):933–8. https://doi.org/10.1038/sj.bjc.6605902 .
García-Estévez L, Cortés J, Pérez S, Calvo I, Gallegos I, Moreno-Bueno G. Obesity and breast Cancer: a paradoxical and controversial relationship influenced by Menopausal Status. Front Oncol. 2021;11:705911. https://doi.org/10.3389/fonc.2021.705911 .
Chan DSM, Abar L, Cariolou M, et al. World Cancer Research Fund International: continuous update project—systematic literature review and meta-analysis of observational cohort studies on physical activity, sedentary behavior, adiposity, and weight change and breast cancer risk. Cancer Causes Control. 2019;30(11):1183–200. https://doi.org/10.1007/s10552-019-01223-w .
Compedium of Physical Activities. 17-Walking. Accessed August 3. 2023. https://sites.google.com/site/compendiumofphysicalactivities/Activity-Categories/walking?authuser=0 .
Mahmood S, MacInnis RJ, English DR, Karahalios A, Lynch BM. Domain-specific physical activity and sedentary behaviour in relation to colon and rectal cancer risk: a systematic review and meta-analysis. Int J Epidemiol. 2017;46(6):1797–813. https://doi.org/10.1093/ije/dyx137 .
Samad AKA, Taylor RS, Marshall T, Chapman MAS. A meta-analysis of the association of physical activity with reduced risk of colorectal cancer. Colorect Dis. 2005;7(3):204–13. https://doi.org/10.1111/j.1463-1318.2005.00747.x .
McTiernan A. Mechanisms linking physical activity with cancer. Nat Rev Cancer. 2008;8(3):205–11. https://doi.org/10.1038/nrc2325 .
Peters HPF. Potential benefits and hazards of physical activity and exercise on the gastrointestinal tract. Gut. 2001;48(3):435–9. https://doi.org/10.1136/gut.48.3.435 .
Diao X, Ling Y, Zeng Y, et al. Physical activity and cancer risk: a dose-response analysis for the global burden of Disease Study 2019. Cancer Commun. 2023;43(11):1229–43. https://doi.org/10.1002/cac2.12488 .
Garcia L, Pearce M, Abbas A, et al. Non-occupational physical activity and risk of cardiovascular disease, cancer and mortality outcomes: a dose–response meta-analysis of large prospective studies. Br J Sports Med. 2023;57(15):979–89. https://doi.org/10.1136/bjsports-2022-105669 .
Li T, Wei S, Shi Y, et al. The dose–response effect of physical activity on cancer mortality: findings from 71 prospective cohort studies. Br J Sports Med. 2016;50(6):339–45. https://doi.org/10.1136/bjsports-2015-094927 .
Unite, Nations. Department of Economic and Social Affair. World urbanization prospects: the 2018 revision. United Nations; 2019.
Sung H, Ferlay J, Siegel RL, Cancer, et al. J Clin. 2021;71(3):209–49. https://doi.org/10.3322/caac.21660 .
Download references
Acknowledgements
STT is supported by Sir Charles Hercus Health Research Fellowship from the Health Research Council of New Zealand (Ref: 23/051).
STT is supported by Sir Charles Hercus Health Research Fellowship from the Health Research Council of New Zealand (Ref: 23/051). The funder had no role in the study design, data collection, data analysis, data interpretation, writing of the report, approval of the manuscript, or decision to submit the manuscript for publication.
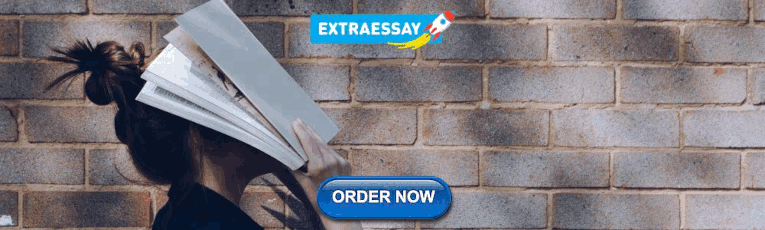
Author information
Authors and affiliations.
Epidemiology and Biostatistics, School of Population Health, University of Auckland, Auckland, New Zealand
Win Thu, Alistair Woodward, Alana Cavadino & Sandar Tin Tin
Cancer Epidemiology Unit, Oxford Population Health, University of Oxford, Oxford, UK
Sandar Tin Tin
You can also search for this author in PubMed Google Scholar
Contributions
STT, AW, WT designed the study. STT supervised the study. WT conducted literature search, data analysis and wrote the original draft with critical inputs from STT, AC, and AW. All authors had full access to all the data in the study and had final responsibility for the decision to submit for publication. More than one author has directly accessed and verified the underlying data reported in the manuscript.
Corresponding author
Correspondence to Sandar Tin Tin .
Ethics declarations
Ethics approval and consent to participate.
Not applicable.
Consent for publication
Competing interests.
The authors declare no competing interests.
Additional information
Publisher’s note.
Springer Nature remains neutral with regard to jurisdictional claims in published maps and institutional affiliations.
Electronic supplementary material
Below is the link to the electronic supplementary material.
Supplementary Material 1
: Supplementary file S1 PRISMA checklist. Supplementary file S2 Literature search strategy. Supplementary file S3 List of excluded full texts with reasons. Supplementary file S4 Metabolic Equivalent of Task (MET) values used and MET hour per week conversion formulas. Supplementary file S5 Measurement units, effect measures and covariates included in the studies. Supplementary file S6 Newcastle-Ottawa Score of the studies (cohort studies). Supplementary file S7 Newcastle-Ottawa Score of the studies (case control studies). Supplementary file S8 Risks estimates used in the meta-analyses (separate excel sheet). Supplementary file S9 Forest plots. Supplementary file S10 Sub-group and covariates adjustment analyses. Supplementary file S11 Sensitivity analysis. Supplementary file S12 Funnel plots
Rights and permissions
Open Access This article is licensed under a Creative Commons Attribution 4.0 International License, which permits use, sharing, adaptation, distribution and reproduction in any medium or format, as long as you give appropriate credit to the original author(s) and the source, provide a link to the Creative Commons licence, and indicate if changes were made. The images or other third party material in this article are included in the article’s Creative Commons licence, unless indicated otherwise in a credit line to the material. If material is not included in the article’s Creative Commons licence and your intended use is not permitted by statutory regulation or exceeds the permitted use, you will need to obtain permission directly from the copyright holder. To view a copy of this licence, visit http://creativecommons.org/licenses/by/4.0/ . The Creative Commons Public Domain Dedication waiver ( http://creativecommons.org/publicdomain/zero/1.0/ ) applies to the data made available in this article, unless otherwise stated in a credit line to the data.
Reprints and permissions
About this article
Cite this article.
Thu, W., Woodward, A., Cavadino, A. et al. Associations between transport modes and site-specific cancers: a systematic review and meta-analysis. Environ Health 23 , 39 (2024). https://doi.org/10.1186/s12940-024-01081-3
Download citation
Received : 20 November 2023
Accepted : 08 April 2024
Published : 13 April 2024
DOI : https://doi.org/10.1186/s12940-024-01081-3
Share this article
Anyone you share the following link with will be able to read this content:
Sorry, a shareable link is not currently available for this article.
Provided by the Springer Nature SharedIt content-sharing initiative
- Transport modes
- Active transport
- Site-specific cancers
- Systematic review
- Meta-analysis
Environmental Health
ISSN: 1476-069X
- General enquiries: [email protected]
The role of pre-service physical education teachers in physical education - A bibliometric and systematic review
Affiliation.
- 1 Department of Physical Education, Korea University, Seoul, 02841, Republic of Korea.
- PMID: 38596074
- PMCID: PMC11002606
- DOI: 10.1016/j.heliyon.2024.e28702
Pre-service physical education teachers (PSPTs) have long been an important area of specific development in physical education and have become a significant force in the field of physical education and research over the past two decades. However, exploratory research on pre-service teachers remains relatively scarce, and lack a comprehensive scientific exploration of the scope of their role. Therefore, this study provides a comprehensive overview of pre-service physical education teacher education (PETE) from both a broad and specific perspective. Specifically, it includes the current state of PETE, the most influential authors, countries, journals, and literature, as well as specific research topics and future directions within PETE. Following the preferred reporting items for systematic reviews and meta-analyses (PRISMA) guidelines, a total of 340 articles were included, with 84 of them being empirical studies. The findings reveal that teacher training, diversity, equity, and inclusion in education, educational attitudes and beliefs, educational quality, educational methods and technology, career motivation, teaching models and strategies, and teacher assessment and reflection are major research themes. Visual analysis of the application of pre-service physical education teacher research highlights teacher training, diversity, equity, and inclusion in education, as well as instructional technology, as key areas of future focus. These insights contribute to the reasonable application of bibliometrics in the field of pre-service physical education teacher research.
Keywords: Bibliometrics; Instructional technology; Pre-service physical education teachers; Social career development; teacher inclusivity.
© 2024 The Author.
- Open access
- Published: 12 April 2024
The association between device-measured sitting time and cardiometabolic health risk factors in children
- Ana María Contardo Ayala ORCID: orcid.org/0000-0002-6192-0033 1 ,
- Nicola D. Ridgers 1 , 2 ,
- Anna Timperio 1 ,
- Lauren Arundell 1 ,
- David W. Dunstan 1 ,
- Kylie D. Hesketh 1 ,
- Robin M. Daly 1 &
- Jo Salmon 1
BMC Public Health volume 24 , Article number: 1015 ( 2024 ) Cite this article
Metrics details
There is limited evidence of the associations between postural-derived sitting time, waist-worn derived sedentary time and children’s health and the moderation effect of physical activity (PA). This study examined associations of children’s device-measured sitting time with cardiometabolic health risk factors, including moderation by physical activity.
Cross-sectional baseline data from children (mean-age 8.2 ± 0.5 years) in Melbourne, Australia (2010) participating in the TransformUs program were used. Children simultaneously wore an activ PAL to assess sitting time and an ActiGraph GT3X to assess sedentary time and physical activity intensity. Cardiometabolic health risk factors included: adiposity (body mass index [BMI], waist circumference [WC]), systolic and diastolic blood pressure (SBP, DBP), high-density lipoprotein (HDL), low-density lipoprotein (LDL), cholesterol, triglycerides, fasting plasma glucose (FPG), serum insulin, and 25-hydroxyvitaminD (25[OH]D). Linear regression models ( n = 71–113) assessed associations between sitting time with each health risk factor, adjusted for different PA intensities (i.e. light [LIPA], moderate-vigorous intensities [MVPA], separately on each model), age, sex, adiposity, and clustering by school. Interaction terms examined moderation. The analyses were repeated using device-measured sedentary time (i.e. ActiGraph GT3X) for comparison.
Sitting time was positively associated with SBP ( b = 0.015; 95%CI: 0.004, 0.026), DBP ( b = 0.012; 95%CI:0.004, 0.020), and FPG ( b = 0.001; 95%CI: 0.000, 0.000), after adjusting for higher PA intensities. The association between sitting time and insulin ( b = 0.003; 95%CI: 0.000, 0.006) was attenuated after adjusting for higher PA intensities. When the models were adjusted for LIPA and MVPA, there was a negative association with LDL ( b =-0.001; 95%CI: -0.002, -0.000 and b =-0.001; 95%CI: -0.003, -0.000, respectively). There was a negative association of sedentary time with WCz ( b =-0.003; 95%CI: -0.005, 0.000) and BMIz ( b =-0.003; 95%CI: -0.006, -0.000) when the models were adjusted by MVPA. Sedentary time was positively associated with triglycerides ( b = 0.001; 95%CI: 0.000, 0.001) but attenuated after adjusting for MVPA. No evidence of moderation effects was found.
Conclusions
Higher volumes of sitting and sedentary time were associated with some adverse associations on some cardiometabolic health risk factors in children. These associations were more evident when sitting time was the predictor. This suggests that reducing time spent sitting may benefit some cardiometabolic health outcomes, but future experimental research is needed to confirm causal relationships and identify the biological mechanisms that might be involved.
Trial registration
Australian New Zealand Clinical Trials Registry: ACTRN12609000715279.
Peer Review reports
Sedentary behaviours, characterised as waking behaviours with an energy expenditure ≤ 1.5 metabolic equivalents (METs) while in a sitting or reclining posture [ 1 ], have been associated with unhealthy cardiometabolic profiles in adults [ 2 ]. There has been significant interest in examining both cross-sectional and longitudinal associations between sedentary time volumes and patterns typically assessed using hip-worn devices, with a range of children’s health outcomes including adiposity markers [ 3 , 4 , 5 ], cardiorespiratory fitness [ 3 ], cardiometabolic risk [ 6 ] among children and adolescents. However, these associations have been mixed [ 4 , 7 , 8 , 9 , 10 , 11 , 12 ]. Some studies amongst youth have reported positive associations between sedentary time and cardiometabolic risk factors, which attenuate when adjusted for moderate- to vigorous-intensity physical activity (MVPA) [ 13 , 14 ]. In contrast, some studies have shown associations remain unchanged after adjustment for MVPA [ 15 , 16 ]. This may partly relate to the assessment used, which traditionally relies on accelerometer cut-points to estimate periods of ‘little too low movement’ and does not differentiate between postures when sitting or standing still [ 17 , 18 ].
While a novel computational method has been developed that classifies sitting posture from hip-mounted accelerometers among children [ 19 ], postural-detection devices (e.g. activ PAL) can differentiate between sitting and standing but have rarely been used to explore associations between sitting time and cardiometabolic risk factors among youth [ 20 , 21 ]. In the studies that have assessed sitting using postural devices, Contardo Ayala et al. [ 21 ] found no cross-sectional associations between activ PAL-measured sitting time and adiposity markers (body mass index [BMI] or waist circumference [WC] z-scores) among 219 adolescents (age = 14.9 ± 1.6 years). While some evidence of associations has been observed for components of sitting patterns (i.e., breaks, prolonged sitting) with adiposity and high-density lipoprotein cholesterol (HDL) among 118 children (aged 11–12 years) [ 20 ], total sitting volume was not reported due to collinearity in the model. Further research using postural devices is needed to better understand associations between sitting time and cardiometabolic risk factors in children.
Different analytical methods have been used to examine associations between movement behaviours and cardiometabolic health [ 14 ]. Historically, the focus has been on MVPA and sedentary time, often with adjustments for other intensities and assessed using one device [ 12 ]. However, little research has examined the potential health benefit of light-intensity physical activity (LIPA), and the use of different devices to capture sitting and physical activity of different intensities on these associations. It is important to understand the potential health benefits of LIPA, as it represents a substantial portion of daily physical activity in adolescents [ 22 ]. In addition, examining whether physical activity moderates associations between sitting and health, and which intensity of activity moderates the associations to a greater extent, has rarely been explored despite this potentially providing insights into how much physical activity is needed to counteract the detrimental effects of too much sitting. However, to our knowledge, the moderation effect of different physical activity intensities on the association between sitting time and health markers has only been reported in one study [ 21 ]. That study found that LIPA and not MVPA moderated the relationship between sitting and adiposity markers (i.e. BMI and WC) in adolescents [ 21 ], with negative associations between sitting and adiposity markers in those who engaged in greater amounts of LIPA. These findings suggest that increasing time spent in LIPA may attenuate the potential deleterious association of high levels of sitting on health. However, whether different PA intensities moderate relationships between sitting and other cardiometabolic risk factors among children has yet to be examined in this way.
The aim of this study was to examine the cross-sectional associations between children’s device-measured sitting time (i.e. activ PAL3) and cardiometabolic risk factors and to determine whether physical activity of varying intensities moderated associations between sitting time and cardiometabolic risk factors. We also repeated the analyses using device-measured sedentary time (i.e. ActiGraph GT3X) for comparison.
Study population
Baseline data from the TransformUs randomised controlled trial [ 23 ] were analysed. TransformUs was an 18-month, four-arm cluster-randomized controlled trial within primary schools in Melbourne, Australia (2010-12), aiming to increase children’s physical activity, decrease sedentary behaviour, and optimize health outcomes [ 23 , 24 ]. TransformUs was approved by the Deakin University Human Research Ethics Committee Group (141–2009), Victorian Department of Education and Early Childhood Development (2009_000344) and Catholic Education Office (1545).
Recruitment details have been published previously [ 24 ]. In brief, schools within a 50 km radius from the Melbourne Central Business District were identified. The school selection criteria included: enrolment greater than 300 students, having at least two grade three classes, being co-educational (i.e. no single-sex schools) and being located in the first, third or fifth quintile of the Socio-economic Index for Areas (SEIFA; for socio-economic diversity) [ 25 ]. In total, 20 schools were recruited. All grade three students were invited to consent to the opt-in evaluation assessments ( n = 1606). In total, 599 parents provided written informed consent for their child to participate in evaluation assessments (37%), and of these, 532 consented to wear an activ PAL. Due to equipment availability, a random sample of 216 children wore an activ PAL at baseline. Parental consent to collect children’s blood pressure and blood samples was obtained for 477 and 219 children, respectively. Baseline data collection occurred between February to June 2010.
Demographics
Children self-reported their sex and age in a survey completed during class time.
Physical activity, sitting and sedentary time
Participants were asked to concurrently wear two devices during waking hours (except for water-based activities) for eight days. Children wore an activ PAL3 inclinometer (PAL Technologies Ltd, Glasgow, UK) on the front of their right thigh (at the mid-point), attached via an elastic belt. The activ PAL3 is considered a valid measure of sitting [ 26 ]. Children also wore an ActiGraph GT3X (ActiGraph LLC, Pensacola, FL., USA) on an elastic belt on their right hip. The ActiGraph is commonly used to quantify physical activity in children [ 27 ]. All data were extracted using manufacturer software ( activ PAL Professional v7.2.29 and Actilife v6.11.18) and processed using a customised Microsoft Excel macro in 15-second periods. Consecutive zero counts for 20 min were used as non-wear time for both devices [ 28 , 29 ]. For each device, data were included in the analysis if participants had at least four valid days (including a weekend day), which was defined as ≥ 8 h/day of wear time for weekdays and ≥ 7 h/day for weekend days [ 30 , 31 ].
From the activ PAL data, the total volume of sitting was computed as the average duration per valid day (mins/day). From the ActiGraph data, Freedson age-adjusted cut-points [ 32 ] were used to obtain average time spent in sedentary time (< 100 counts/minutes), LIPA (i.e. 101 counts/minute to 3.99 METs) and MVPA (≥ 4 METS for those < 18years, based on age-specific thresholds [e.g. for a 8 year old is 802 counts/minute]) per valid day. LIPA was further dichotomised into low light-intensity physical activity (low-LIPA) (i.e. 101–799 counts/minute) and high light-intensity physical activity (high-LIPA) (i.e. 800 counts/minute to 3.99 METs). The cut-point of > 800 counts/min was based on previous research as it captures static LIPA, such as standing as high-LIPA [ 17 , 33 ]. Prior to the analysis, all activ PAL and ActiGraph-derived outcome variables were standardised (to account for variations in the amount of time individuals wear the device) according to total wear time during the period of interest as follows: (duration of ‘X’ within waking hours/wear time within waking hours) multiplied by 960 min, where ‘X’ is the activity (e.g., sitting, LIPA, low-LIPA, high-LIPA and MVPA) and waking hours are equivalent to 960 min–16 h.
Anthropometric measures
Measurements were taken at the schools by trained researchers using standardised protocols. Stature was measured to the nearest 0.1 cm using portable stadiometers (SECA 0123, SECA, Hamburg, Germany) and body mass to the nearest 0.1 kg using portable electronic scales (InnerScan 50; TANITA, Illinois, USA). WC was assessed using a flexible measuring tape at the narrowest, or middle, point between the bottom rib and the iliac crest. Two measurements were taken, with a third measure taken when a difference of over 0.5 cm (stature), 0.5 kg (body mass) and 1 cm (WC) was noted. BMI (kg/m 2 ) was calculated from body mass and stature, and participants were categorised according to the International Obesity Task Force definitions of healthy weight or overweight/obese [ 34 ]. BMI z-scores (BMIz) were calculated and converted to z-values, standardized for age and sex, using the World Health Organization Child Growth Standards [ 35 ]. WC z-scores (WCz) were calculated from raw anthropometric data using Stata function with UK growth chart (zanthro) [ 36 ]. Australian percentile curves for WC were utilised to determine age- and sex-specific waist circumference percentiles, where a WC ≥ 90th percentile was used to classify obesity and overweight was defined as a WC ≥ 75th percentile but less than the 90th percentile [ 37 ].
Blood pressure
Participants were asked to sit quietly for two minutes, then blood pressure (BP) was measured from the right arm using a paediatric cuff (OMRON HEM-907, Kyoto, Japan). Three measures were taken one-minute apart, on two different occasions one week apart. Average systolic and diastolic BP were calculated from four measures after removing the first measurement from weeks one and two [ 38 ].
Blood biomarkers
Following an overnight fast, a morning blood sample was collected from each child with parental consent by a trained phlebotomist at a local commercial pathology clinic (the same pathology company was used for all blood samples). All children were provided with a dermal anaesthetic topical cream (EMLA®) to apply 1 h before the blood collection. The following biomarkers were assessed by the pathology laboratory using standard laboratory techniques: HDL-cholesterol, LDL- cholesterol, total cholesterol, triglycerides, fasting plasma glucose (FPG), serum insulin, and serum 25-hydroxyvitamin D (25[OH]D). All assays were performed according to manufacturer’s instructions, and all samples were run in duplicate. Biomarker ranges considered as acceptable are presented in the Additional file 1 .
Statistical analysis
Statistical analyses were conducted using Stata 18.0 (Stata-Corp LPD46XX). Statistical significance was set at p < 0.05. Shapiro–Wilk test were used to check the normality of the data. Pearson’s correlations were used to test for multicollinearity, confirming the absence of collinearity between the variables studied (i.e. the correlation between sitting [from activ PAL] and each physical activity intensity variable [from ActiGraph] ranged between − 0.06 and 0.05). Collinearity was confirmed between sedentary time [from ActiGraph] and LIPA [from ActiGraph].
The main association of sitting (minutes/day) with cardiometabolic risk factors was examined using separate regression models (Model A) for each cardiometabolic health marker (11 models in total). The associations with cardiometabolic risk factors were further explored by adjusting for each PA intensity (LIPA and MVPA) separately, age, sex, adiposity (BMIz), season in which the assessment was conducted, and clustering by school (Models B to E). Moderation by LIPA, low-LIPA, high-LIPA, and MVPA were also examined by adding interaction terms to the respective main regression models and checking p values. The main association of sedentary time (minutes/day) with cardiometabolic risk factors was examined using separate regression models (Model A) for each cardiometabolic health marker (11 models in total). The associations with cardiometabolic risk factors were further explored by adjusting for MVPA, age, sex, adiposity (BMIz), season in which the assessment was conducted, and clustering by school (Model B). Moderation by MVPA was also examined. All moderator variables were centered around the individual variable mean.
activ PAL data: In total, 113 children provided valid activ PAL data, of whom 103 had valid ActiGraph data. For the analyses, 113 children had anthropometric measures, 111 had BP measures, and 71 had blood biomarkers. For the analysis of the ActiGraph data, 334-335 children had anthropometric measures, 323 had BP measures, and 159 had blood biomarkers.
Children (mean ± SD, age 8.1 ± 0.4 years, 58% girls) spent an average of 59% of their waking hours sitting, 41% in LIPA (33% and 8% in low and high LIPA, respectively), and 4% in MVPA (Table 1 ). All participants had their biomarkers in the acceptable range.
Associations of sitting time with health risk biomarkers
Table 2 reveals a positive association between sitting time and SBP, DBP, and FPG, persisting even after adjustments for age, sex, adiposity, season in which the assessment was conducted, and various physical activity intensity variables in the model. Similarly, sitting time was positively associated with insulin, but this association was attenuated after adjusting for the physical activity intensity variables. There was a negative association with LDL in the LIPA (i.e. total LIPA and low-LIPA) and MVPA adjusted models.
Associations of sedentary time with health risk biomarkers
There was a negative association with WCz and BMIz only when the models were adjusted by MVPA. Sedentary time was positively associated with triglycerides but attenuated after adjusting for MVPA (Table 3 ).
Moderation analysis
No significant interactions between sitting (i.e. SBP, p = 0.446; DBP, p = 0.958; LDL, p = 0.768; FPG, p = 0.160; and insulin, p = 0.117) and sedentary time (i.e. WCz, p = 0.569; BMIz, p = 0.599; and triglycerides, p = 0.388) and any of the physical activity intensity variables were found for the significant models (prior and after the adjustment for physical activity intensity).
This cross-sectional study aimed to examine associations between activ PAL-measured sitting time and cardiometabolic risk factors and to determine whether ActiGraph-measured LIPA and MVPA moderated the relationship between sitting and cardiometabolic risk factors among children. Sitting time was unfavourably associated with SBP, DBP, and FPG and these associations persisted after adjusting for each PA intensity separately. There was a trend for sitting time to be detrimentally associated with insulin, however, this association was attenuated after adjusting for the physical activity intensity variables. Also, there was a negative association with LDL, in the unexpected direction, in the LIPA (i.e. total LIPA and low-LIPA) and MVPA adjusted models. When sedentary time was the predictor, an unfavourably association was observed between WCz and BMIz, but only in models adjusted for MVPA. Sedentary time was positively associated with triglycerides; however, this association weakened after adjusting for MVPA. There was, however, no significant moderation effects of LIPA (total, low-LIPA, high-LIPA) nor MVPA on associations between children’s sitting and sedentary time and cardiometabolic risk factors, which was inconsistent with previous research in adolescents.
While the sample size was small, to our knowledge, this is the first study to show a significant, detrimental association between device-based measures of sitting time and BP and FPG, among children aged 7–10 years. A previous study that measured sitting time using activ PALs in older children (11–12 years) found no association with cardiometabolic risk outcomes after adjusting for MVPA [ 20 ]. Although demographically comparable, the total volume of MVPA by Stockwell (2019) was double that recorded in the current study (90 vs. 38.2 min/day). The amount of time spent on MVPA in the current study is consistent with the amount of MVPA that has previously been reported for this age group [ 39 ]. The total amount of MVPA that might be needed to attenuate the association of sitting on FPG (e.g. 60 min for adults [ 40 ]) is arguably unrealistic based on population prevalence data; therefore, it is necessary to further explore if engaging in more LIPA and/or MVPA could benefit health among young people. For example, studies using isotemporal substitution to explore the effects of replacing sedentary time with PA of different intensities have found that replacing sedentary time with MVPA, but not LIPA, was favourably associated with body fat percentage [ 41 ]. Longitudinal and experimental research with larger sample sizes and similar methods to measure sitting time ( activ PAL) are needed to determine if the findings of this study are spurious or reflect the actual associations of sitting time and cardiometabolic health makers among children.
The current study found an unfavourable association between sitting time and SBP and DBP, which also contrasts previous research [ 20 ]. The mechanisms responsible for the potential impact of excessive sitting and BP are unclear (e.g. sitting-induced reductions in blood flow and an increase in the accumulation of extravascular fluid in the legs [ 42 ] and reductions in conduit vessel flow and elevations in vasoactive mediators [ 42 ]), and there is limited evidence of this relationship among children. Previous studies, indicate a positive association between sedentary time [ 20 , 43 ], using hip-mounted devices, and higher levels of SBP/DBP in children and adolescents. However, contrasting findings exist, with some studies reporting null associations [ 44 , 45 , 46 ], highlighting the complex relationship between sitting/sedentary time and blood pressure in youth. Longer-term BP monitoring and experimental research are needed to explore the association between sitting time and BP. A weak unfavourable association between sitting time and insulin have been found in this study and similar to the finding among adults [ 47 ], however this association has not been found among adolescents [ 48 ]. The unexpected association with LDL observed in our study warrants careful consideration. While the explanation remains unclear, several factors could contribute to this unexpected finding. For example, the relationship between sitting time and LDL may be influenced by a complex interplay of factors, such as dietary habits, genetic predispositions, and overall lifestyle. In addition, the inclusion of LIPA in the model may have affected the results due to sitting time and LIPA often being highly inversely correlated. It may be that children who engage in higher levels of LIPA accumulate different patterns of sitting, which are known to have varied relationships with health outcomes. Further exploration of these factors may provide further insights.
This study found no associations between sitting and the remaining cardiometabolic risk factors (i.e. WCz, BMIz, Cholesterol, HDL, Triglycerides and serum 25[OH]D), which is consistent with previous literature [ 12 ]. Only one previous study showed that prolonged sitting (bouts ≥ 30 min) was negatively associated with adiposity markers and positively associated with HDL cholesterol [ 20 ]. However, participants in the study by Stockwell et al. were several years older (aged 11–12 years) than those participants in our study. Therefore, the younger children in the present study may have had less exposure to higher volumes of sitting to impact cardiometabolic risk factors, although studies have shown an impact at early ages [ 29 ]. Importantly, children in the current study were generally healthy (normal BP, blood biomarkers and only n = 28 overweight/obese), therefore, finding associations between total volume sitting and health can be more challenging, and sitting patterns might play an important role in these associations. The adverse relationship between total volume of sedentary time and WC and BMI aligns, which was non-significant when sitting time was the predictor, with findings from prior cross-sectional and longitudinal studies [ 49 ]. However, while evidence suggests a connection between sedentary time and adiposity markers, it remains inconclusive and lacks evidence of causation [ 7 ]. Nonetheless, the evidence supporting the relationship between sedentary time and adiposity markers appears to be increasing over time [ 49 ]. The adverse association with triglycerides aligns with previous research [ 43 ]. For instance, Strizich et al. [ 44 ] demonstrated an association between sedentary time and triglycerides, which aligns with the findings of this study. However, unlike our study, they did not observe a similar relationship with HDL.
In the current study, children spent 59% of their day sitting, which is consistent with previous studies that have reported between 57 and 78% of waking time spent sitting, measured with activ PALs [ 31 , 50 , 51 ]. Children in this study also spent 40% of their waking hours in LIPA and only 4% in MVPA; however, neither LIPA nor MVPA moderated the relationship between sitting and cardiometabolic risk factors. In a previous study, sitting time was not associated with adiposity markers (BMIz and WCz), but LIPA and low-LIPA showed to moderate the detrimental association between sitting and BMIz and WCz in adolescents [ 21 ]. The inconsistent findings between this study in children and adolescents could be partly because adolescents had a higher volume of sitting (i.e., 59% vs. 68% of the day, respectively) and less volume of LIPA (27% vs. 39%, respectively). It is also possible that the very low percentage of waking hours spent in MVPA ( ∼ 4%) is not enough to overcome the effects of two-thirds of the day spent sitting by the children. Experimental research is needed to understand if, for example, replacing sitting time with LIPA is beneficial for children and adolescents in the long term.
This study found no associations between sitting and sedentary time and serum 25[OH]D when the models were adjusted for the season in which the assessment was conducted. To our knowledge, no previous studies have examined the relationship between device-assessed sitting time and circulating vitamin D in children. However, a previous study suggested that higher self-reported screen time was related to reduced serum concentrations of 25[OH]D in 8–9 year olds ( n = 1464, not adjusted for MVPA) [ 52 ], but not 12–17 year olds ( n = 1152, adjusted for MVPA) [ 53 ]. It could be that the deleterious impacts of sitting, which might be attributed to lower sun exposure due to sitting indoors, is counterbalanced with higher intensity physical activities (e.g. outdoor play/MVPA), which is likely to be performed outdoors (Cleland et al., 2010). Furthermore, considering that 72% of the data was collected during summer and autumn, significant sun exposure likely occurred during this study. Participation in outdoor play and MVPA is typically greater amongst children than adolescents, which may also partly explain the findings in the current study. Further research focusing on associations between sitting and vitamin D is needed to clarify the role of PA (i.e. indoors v outdoors). In contrast to previous cross sectional and longitudinal research (e.g. increase in sedentary time was unfavourably associated with changes in cardiometabolic score, triglycerides and DBP) [ 6 ], this study did not find any associations with other cardiometabolic makers (i.e. BP, Cholesterol, HDL, LDL, FPG, serum 25[OH]D and insulin). This divergence underscores the complexity of the relationship between sedentary behaviour and cardiometabolic health, potentially influenced by various factors such as sample characteristics, measurement techniques, and confounding variables.
Strengths of this study included the use of device-based assessments of sitting time (i.e. postural detection device) and PA of different intensities (i.e. actigraphy), the inclusion of a range of cardiometabolic risk factors among children, as well as the use of WC as a measure of central adiposity, given that BMI, while widely used, does not distinguish lean and fat mass. The limitations of this study include the use of cross-sectional data collected in 2010 and the small sample size, which may limit statistical power and the generalisability of the findings to the wider population. Nonetheless, this study contributes uniquely to the literature by employing both activ PAL and ActiGraph devices in children to explore their associations with health markers and the moderation effects of physical activity. However, while the data were collected in 2010 and patterns of sitting (and activities undertaken while sitting) may have changed, the device used to capture sitting time has undergone minor changes and relationships between volumes of sitting time and health outcomes are likely to be constant [ 54 ]. The study included a generally healthy sample, which may limit the ability to find associations with health outcomes. A further limitation is that participants had to remove the monitors for water-based activities. It is possible that physical activity levels were underestimated as water activities were not included. Use of waterproof wearable monitors may overcome this limitation in future studies. Given the study’s limitations and the scarcity of literature with which to compare our findings, the results should be considered cautiously.
Our findings indicate that excessive sitting time is associated with some negative associations on cardiometabolic risk factors in children, suggesting that reducing the amount of time spent sitting could be a potential intervention to improve cardiometabolic health outcomes in children. However, further longitudinal and experimental studies are required to elucidate the relationship between sitting time and cardiometabolic health outcomes in children, and to inform targeted interventions to mitigate the adverse effects of sitting and sedentary time on health outcomes in children. Also, to examine the interplay between sitting time and different intensities of physical activity in relation to cardiometabolic risk factors also warranted.
Data availability
The dataset used for the current study could be made available on reasonable request, as the datasets are not publicly available due to ethical restrictions (participants have not consented to the use of their data for purposes other than those of which they originally consented)
Abbreviations
body mass index
blood pressure
Diabetes Australia Research Trust
diastolic blood pressure
fasting plasma glucose
high-density lipoprotein
high light-intensity physical activity
low-density lipoprotein
light-intensity physical activity
low light-intensity physical activity
metabolic equivalents
moderate- to vigorous-intensity physical activity
National Health and Medical Research Council
physical activity
systolic blood pressure
Socio-economic Index for Areas
waist circumference
25-hydroxyvitaminD
Tremblay MS, Aubert S, Barnes JD, Saunders TJ, Carson V, Latimer-Cheung AE, et al. Sedentary Behavior Research Network (SBRN) - terminology Consensus Project process and outcome. Int J Behav Nutr Phys Act. 2017;14(1):75.
Article PubMed PubMed Central Google Scholar
Young DR, Hivert MF, Alhassan S, Camhi SM, Ferguson JF, Katzmarzyk PT, et al. Sedentary Behavior and Cardiovascular Morbidity and Mortality: A Science Advisory from the American Heart Association. Circulation. 2016;134(13):e262–79.
Article PubMed Google Scholar
Jones MA, Skidmore PM, Stoner L, Harrex H, Saeedi P, Black K, et al. Associations of accelerometer-measured sedentary time, sedentary bouts, and physical activity with adiposity and fitness in children. J Sports Sci. 2020;38(1):114–20.
Marques A, Minderico C, Martins S, Palmeira A, Ekelund U, Sardinha LB. Cross-sectional and prospective associations between moderate to vigorous physical activity and sedentary time with adiposity in children. Int J Obes (Lond). 2016;40(1):28–33.
Article CAS PubMed Google Scholar
Santos DA, Magalhães JP, Júdice PB, Correia IR, Minderico CS, Ekelund U, et al. Fitness mediates activity and sedentary patterns associations with Adiposity in Youth. Med Sci Sports Exerc. 2019;51(2):323–9.
Júdice PB, Hetherington-Rauth M, Northstone K, Andersen LB, Wedderkopp N, Ekelund U et al. Changes in Physical Activity and Sedentary Patterns on Cardiometabolic Outcomes in the Transition to Adolescence: International Children’s Accelerometry Database 2.0. J Pediatr. 2020;225:166– 73.e1.
Biddle SJ, Garcia Bengoechea E, Wiesner G. Sedentary behaviour and adiposity in youth: a systematic review of reviews and analysis of causality. Int J Behav Nutr Phys Act. 2017;14(1):43.
Biddle SJH, Pearson N, Salmon J. Sedentary behaviors and adiposity in Young people: causality and conceptual model. Exerc Sport Sci Rev. 2018;46(1):18–25.
Cliff DP, Hesketh KD, Vella SA, Hinkley T, Tsiros MD, Ridgers ND, et al. Objectively measured sedentary behaviour and health and development in children and adolescents: systematic review and meta-analysis. Obes Rev. 2016;17(4):330–44.
Chaput J-P, Willumsen J, Bull F, Chou R, Ekelund U, Firth J, et al. 2020 WHO guidelines on physical activity and sedentary behaviour for children and adolescents aged 5–17 years: summary of the evidence. Int J Behav Nutr Phys Activity. 2020;17(1):141.
Article Google Scholar
Oliveira RGd, Guedes DP, Physical Activity S, Behavior. Cardiorespiratory Fitness and metabolic syndrome in adolescents: systematic review and Meta-analysis of Observational evidence. PLoS ONE. 2016;11(12):e0168503.
Skrede T, Steene-Johannessen J, Anderssen SA, Resaland GK, Ekelund U. The prospective association between objectively measured sedentary time, moderate-to-vigorous physical activity and cardiometabolic risk factors in youth: a systematic review and meta-analysis. Obes Rev. 2019;20(1):55–74.
Renninger M, Hansen BH, Steene-Johannessen J, Kriemler S, Froberg K, Northstone K, et al. Associations between accelerometry measured physical activity and sedentary time and the metabolic syndrome: a meta-analysis of more than 6000 children and adolescents. Pediatr Obes. 2020;15(1):e12578.
Froberg A, Raustorp A. Objectively measured sedentary behaviour and cardio-metabolic risk in youth: a review of evidence. Eur J Pediatr. 2014;173(7):845–60.
Mitchell JA, Pate RR, Beets MW, Nader PR. Time spent in sedentary behavior and changes in childhood BMI: a longitudinal study from ages 9 to 15 years. Int J Obes (Lond). 2013;37(1):54–60.
Cliff DP, Okely AD, Burrows TL, Jones RA, Morgan PJ, Collins CE, et al. Objectively measured sedentary behavior, physical activity, and plasma lipids in overweight and obese children. Obes (Silver Spring). 2013;21(2):382–5.
Article CAS Google Scholar
Ridgers ND, Salmon J, Ridley K, O’Connell E, Arundell L, Timperio A. Agreement between activPAL and ActiGraph for assessing children’s sedentary time. Int J Behav Nutr Phys Act. 2012;9(1):15.
Hardy LL, Hills AP, Timperio A, Cliff D, Lubans D, Morgan PJ, et al. A hitchhiker’s guide to assessing sedentary behaviour among young people: deciding what method to use. J Sci Med Sport. 2013;16(1):28–35.
Carlson JA, Ridgers ND, Nakandala S, Zablocki R, Tuz-Zahra F, Bellettiere J, et al. CHAP-child: an open source method for estimating sit-to-stand transitions and sedentary bout patterns from hip accelerometers among children. Int J Behav Nutr Phys Act. 2022;19(1):109.
Stockwell SL, Smith LR, Weaver HM, Hankins DJ, Bailey DP. Associations of sitting behavior patterns with cardiometabolic risk in children: the Sit Less for Health cross-sectional study. J Phys Act Health. 2019;12(10):856–42.
PubMed Google Scholar
Contardo Ayala AM, Salmon J, Dunstan DW, Arundell L, Timperio A. Does light-intensity physical activity moderate the relationship between sitting time and adiposity markers in adolescents? J Sport Health Sci. 2022;11(5):613–9.
Contardo Ayala AM, Salmon J, Dunstan DW, Arundell L, Parker K, Timperio A. Longitudinal changes in sitting patterns, physical activity, and Health outcomes in adolescents. Child [Internet]. 2019; 6(1).
Salmon J, Arundell L, Cerin E, Ridgers ND, Hesketh KD, Daly RM, et al. Transform-Us! Cluster RCT: 18-month and 30-month effects on children’s physical activity, sedentary time and cardiometabolic risk markers. Br J Sports Med. 2023;57(5):311–9.
Salmon J, Arundell L, Hume C, Brown H, Hesketh K, Dunstan DW, et al. A cluster-randomized controlled trial to reduce sedentary behavior and promote physical activity and health of 8–9 year olds: the Transform-Us! Study. BMC Public Health. 2011;11:759.
Australian Bureau of Statistics. Socio-Economic Indexes for Areas (SEIFA). 2016(27/03/2018).
Ridley K, Ridgers ND, Salmon J. Criterion validity of the activPAL and ActiGraph for assessing children’s sitting and standing time in a school classroom setting. Int J Behav Nutr Phys Act. 2016;13(1):75.
Cain KL, Sallis JF, Conway TL, Van Dyck D, Calhoon L. Using accelerometers in youth physical activity studies: a review of methods. J Phys Act Health. 2013;10(3):437–50.
Janssen X, Basterfield L, Parkinson KN, Pearce MS, Reilly JK, Adamson AJ, et al. Objective measurement of sedentary behavior: impact of non-wear time rules on changes in sedentary time. BMC Public Health. 2015;15:504.
Gabel L, Ridgers ND, Della Gatta PA, Arundell L, Cerin E, Robinson S, et al. Associations of sedentary time patterns and TV viewing time with inflammatory and endothelial function biomarkers in children. Pediatr Obes. 2016;11(3):194–201.
Mattocks C, Ness A, Leary S, Tilling K, Blair SN, Shield J, et al. Use of accelerometers in a large field-based study of children: protocols, design issues, and effects on precision. J Phys Act Health. 2008;5(Suppl 1):S98–111.
Ridgers ND, Timperio A, Cerin E, Salmon J. Within- and between-day associations between children’s sitting and physical activity time. BMC Public Health. 2015;15(1):950.
Freedson P, Pober D, Janz KF. Calibration of accelerometer output for children. Med Sci Sports Exerc. 2005;37(11 Suppl):S523–30.
Carson V, Ridgers ND, Howard BJ, Winkler EA, Healy GN, Owen N, et al. Light-intensity physical activity and cardiometabolic biomarkers in US adolescents. PLoS ONE. 2013;8(8):e71417.
Article CAS PubMed PubMed Central Google Scholar
Cole TJ, Flegal KM, Nicholls D, Jackson AA. Body mass index cut offs to define thinness in children and adolescents: international survey. BMJ. 2007;335(7612):194.
World Health Organisation. Growth, reference, study, Group. WHO Child Growth standards: Length/height-for-age, weight-for-age, weight-for-length, weight-for-height and body mass index-for-age: methods and development. Geneva: World Health Organization; 2006.
Google Scholar
Vidmar SI, Cole TJ, Pan H. Standardizing anthropometric measures in children and adolescents with functions for egen: update. Stata J. 2013;13(2):366–78.
Eisenmann JC. Waist circumference percentiles for 7- to 15-year-old Australian children. Acta Paediatr. 2005;94(9):1182–5.
National High Blood Pressure Education Program Working Group on High Blood Pressure in C, Adolescents. The fourth report on the diagnosis, evaluation, and treatment of high blood pressure in children and adolescents. Pediatrics. 2004;114(2 Suppl 4th Report):555– 76.
Cooper AR, Goodman A, Page AS, Sherar LB, Esliger DW, van Sluijs EM, et al. Objectively measured physical activity and sedentary time in youth: the international children’s accelerometry database (ICAD). Int J Behav Nutr Phys Act. 2015;12(1):113.
van Dijk JW, Venema M, van Mechelen W, Stehouwer CD, Hartgens F, van Loon LJ. Effect of moderate-intensity exercise versus activities of daily living on 24-hour blood glucose homeostasis in male patients with type 2 diabetes. Diabetes Care. 2013;36(11):3448–53.
Garcia-Hermoso A, Saavedra JM, Ramirez-Velez R, Ekelund U, Del Pozo-Cruz B. Reallocating sedentary time to moderate-to-vigorous physical activity but not to light-intensity physical activity is effective to reduce adiposity among youths: a systematic review and meta-analysis. Obes Rev. 2017;18(9):1088–95.
Dempsey PC, Larsen RN, Dunstan DW, Owen N, Kingwell BA. Sitting less and moving more: implications for hypertension. Hypertension. 2018;72(5):1037–46.
Ekelund U, Anderssen SA, Froberg K, Sardinha LB, Andersen LB, Brage S. Independent associations of physical activity and cardiorespiratory fitness with metabolic risk factors in children: the European youth heart study. Diabetologia. 2007;50(9):1832–40.
Strizich G, Kaplan RC, Sotres-Alvarez D, Diaz KM, Daigre AL, Carnethon MR, et al. Objectively measured sedentary behavior, physical activity, and Cardiometabolic Risk in Hispanic Youth: Hispanic Community Health Study/Study of latino youth. J Clin Endocrinol Metabolism. 2018;103(9):3289–98.
Carson V, Janssen I. Volume, patterns, and types of sedentary behavior and cardio-metabolic health in children and adolescents: a cross-sectional study. BMC Public Health. 2011;11(1):274.
Bell JA, Hamer M, Richmond RC, Timpson NJ, Carslake D, Davey Smith G. Associations of device-measured physical activity across adolescence with metabolic traits: prospective cohort study. PLoS Med. 2018;15(9):e1002649.
Barone Gibbs B, Pettee Gabriel K, Reis JP, Jakicic JM, Carnethon MR, Sternfeld B. Cross-sectional and longitudinal associations between objectively measured sedentary time and metabolic disease: the coronary artery Risk Development in Young adults (CARDIA) study. Diabetes Care. 2015;38(10):1835–43.
Silva TO, Norde MM, Vasques AC, Zambom MP, Antonio MARGM, Rodrigues AMDB et al. Association of physical activity and sitting with metabolic syndrome and hyperglycemic clamp parameters in adolescents– BRAMS pediatric study. Front Endocrinol. 2023;14.
Lim J, Kim JS, Park S, Lee O, So WY. Relationship of physical activity and sedentary time with metabolic health in children and adolescents measured by accelerometer: a narrative review. Healthc (Basel). 2021;9(6).
Sherry AP, Pearson N, Ridgers ND, Barber SE, Bingham DD, Nagy LC, et al. activPAL-measured sitting levels and patterns in 9–10 years old children from a UK city. J Public Health (Oxf). 2019;41(4):757–64.
Hinckson EA, Hopkins WG, Aminian S, Ross K. Week-to-week differences of children’s habitual activity and postural allocation as measured by the ActivPAL monitor. Gait Posture. 2013;38(4):663–7.
Milagres LC, Rocha NP, Albuquerque FM, Castro APP, Filgueiras MS, Pessoa MC, et al. Sedentary behavior is associated with lower serum concentrations of vitamin D in Brazilian children. Public Health. 2017;152:75–8.
da Silva ACM, Cureau FV, de Oliveira CL, Giannini DT, Bloch KV, Kuschnir MCC, et al. Physical activity but not sedentary time is associated with vitamin D status in adolescents: study of cardiovascular risk in adolescents (ERICA). Eur J Clin Nutr. 2019;73(3):432–40.
Hoffmann B, Kobel S, Wartha O, Kettner S, Dreyhaupt J, Steinacker JM. High sedentary time in children is not only due to screen media use: a cross-sectional study. BMC Pediatr. 2019;19(1):154.
Download references
Acknowledgements
Not applicable.
TransformUs was supported by a National Health and Medical Research Council (NHMRC) Project Grant (2009–2013; ID533815) and a Diabetes Australia Research Trust (DART) grant. JS is supported by a Leadership Level 2 Fellowship, National Health and Medical Research Council (APP 1176885). NDR was supported by a National Heart Foundation of Australia Future Leader Fellowship (ID 101895). LA is supported by an Australian Research Council Discovery Early Career Researcher Award (DE220100847). DWD was supported Senior Research Fellowship (APP1078360) from the National Health and Medical Research Council and the Victorian Government’s Operational Infrastructure Support Program. KDH is supported by a Heart Foundation Future Leader Fellowship (105929). Aspects of this work were supported by a National Health and Medical Research Council, Centre of Research Excellence (APP1057608).
Author information
Authors and affiliations.
Institute for Physical Activity and Nutrition (IPAN), School of Exercise and Nutrition Sciences, Deakin University, 221 Burwood Highway, Geelong, Victoria, Australia
Ana María Contardo Ayala, Nicola D. Ridgers, Anna Timperio, Lauren Arundell, David W. Dunstan, Kylie D. Hesketh, Robin M. Daly & Jo Salmon
Alliance for Research in Exercise, Nutrition and Activity (ARENA), Allied Health and Human Performance, University of South Australia, Adelaide, South Australia, Australia
Nicola D. Ridgers
You can also search for this author in PubMed Google Scholar
Contributions
AMCA, AT, LA, JS conceived the study. AMCA analysed and interpreted the data; and wrote the first manuscript. NDR, AT, LA, DWD, KDH, RMD and JS assisted with data analysis and interpretation of the data. All authors commented on the conception of this manuscript and the data analysis plan, critically reviewed and revised the manuscript and approved the final manuscript as submitted.
Corresponding author
Correspondence to Ana María Contardo Ayala .
Ethics declarations
Ethics approval and consent to participate.
TransformUs obtained ethical approval from the Deakin University Human Research Ethics Committee (EC 141–2009), the Victorian Department of Education and Early Childhood Development (2009_000344) and the Catholic Education Office (Project Number 1545).
Consent for publication
Competing interests.
The authors declare no competing interests.
Additional information
Publisher’s note.
Springer Nature remains neutral with regard to jurisdictional claims in published maps and institutional affiliations.
Electronic supplementary material
Below is the link to the electronic supplementary material.
Supplementary Material 1
Rights and permissions.
Open Access This article is licensed under a Creative Commons Attribution 4.0 International License, which permits use, sharing, adaptation, distribution and reproduction in any medium or format, as long as you give appropriate credit to the original author(s) and the source, provide a link to the Creative Commons licence, and indicate if changes were made. The images or other third party material in this article are included in the article’s Creative Commons licence, unless indicated otherwise in a credit line to the material. If material is not included in the article’s Creative Commons licence and your intended use is not permitted by statutory regulation or exceeds the permitted use, you will need to obtain permission directly from the copyright holder. To view a copy of this licence, visit http://creativecommons.org/licenses/by/4.0/ . The Creative Commons Public Domain Dedication waiver ( http://creativecommons.org/publicdomain/zero/1.0/ ) applies to the data made available in this article, unless otherwise stated in a credit line to the data.
Reprints and permissions
About this article
Cite this article.
Contardo Ayala, A.M., Ridgers, N.D., Timperio, A. et al. The association between device-measured sitting time and cardiometabolic health risk factors in children. BMC Public Health 24 , 1015 (2024). https://doi.org/10.1186/s12889-024-18495-w
Download citation
Received : 14 December 2023
Accepted : 01 April 2024
Published : 12 April 2024
DOI : https://doi.org/10.1186/s12889-024-18495-w
Share this article
Anyone you share the following link with will be able to read this content:
Sorry, a shareable link is not currently available for this article.
Provided by the Springer Nature SharedIt content-sharing initiative
- Health outcomes
- Physical activity
- Sedentary behavior
BMC Public Health
ISSN: 1471-2458
- Submission enquiries: [email protected]
- General enquiries: [email protected]
- Share full article
Advertisement
Supported by
Large Scientific Review Confirms the Benefits of Physical Touch
Premature babies especially benefited from skin-to-skin contact, and women tended to respond more strongly than men did.

By Joanne Silberner
A hug, a handshake, a therapeutic massage. A newborn lying on a mother’s bare chest.
Physical touch can buoy well-being and lessen pain, depression and anxiety, according to a large new analysis of published research released on Monday in the journal Nature Human Behaviour.
Researchers from Germany and the Netherlands systematically reviewed years of research on touch, strokes, hugs and rubs. They also combined data from 137 studies, which included nearly 13,000 adults, children and infants. Each study compared individuals who had been physically touched in some way over the course of an experiment — or had touched an object like a fuzzy stuffed toy — to similar individuals who had not.
For example, one study showed that daily 20-minute gentle massages for six weeks in older people with dementia decreased aggressiveness and reduced the levels of a stress marker in the blood. Another found that massages boosted the mood of breast cancer patients. One study even showed that healthy young adults who caressed a robotic baby seal were happier, and felt less pain from a mild heat stimulus, than those who read an article about an astronomer.
Positive effects were particularly noticeable in premature babies, who “massively improve” with skin-to-skin contact, said Frédéric Michon, a researcher at the Netherlands Institute for Neuroscience and one of the study’s authors.
“There have been a lot of claims that touch is good, touch is healthy, touch is something that we all need,” said Rebecca Boehme, a neuroscientist at Linkoping University in Sweden, who reviewed the study for the journal. “But actually, nobody had looked at it from this broad, bird’s eye perspective.”
The analysis revealed some interesting and sometimes mysterious patterns. Among adults, sick people showed greater mental health benefits from touch than healthy people did. Who was doing the touching — a familiar person or a health care worker — didn’t matter. But the source of the touch did matter to newborns.
“One very intriguing finding that needs further support is that newborn babies benefit more from their parents’ touch than from a stranger’s touch,” said Ville Harjunen, a researcher at the University of Helsinki in Finland, who also reviewed the study for the journal. Babies’ preference for their parents could be related to smell, he speculated, or to the differences in the way parents hold them.
Women seem to benefit more from touch than men, which may be a cultural effect, Dr. Michon said. The frequency of the touch also mattered: A massage once every two years isn’t going to do much.
Several studies included in the review looked at what happened during the height of the Covid pandemic, when people were isolated and had less physical contact with others. “They found correlations during Covid times between touch deprivation and health aspects like depression and anxiety,” Dr. Michon said.
Touching the head appears to have more of a beneficial effect than touching the torso, some studies found. Dr. Michon couldn’t explain that finding, but thought it could have to do with the greater number of nerve endings on the face and scalp.
Another mystery: Studies of people in South America tended to show stronger health benefits of touch than did those studies that looked at people in North America or Europe. Dr. Michon said that culture may somehow play a role. But Dr. Boehme said the studies showing the differences between countries were too small to be definitive. “I think the mechanism behind this is biological,” she said. “I think that’s hard-wired and will be the same for all of us.”
In 2023, Jeeva Sankar, a pediatrics researcher at All India Institute of Medical Sciences, and a colleague published a rigorous review of skin-to-skin care for newborns. The analysis concluded that touch therapy for preterm or low-birth-weight infants should start as soon as possible and last eight hours or more, a recommendation that the World Health Organization adopted. Dr. Sankar said the new review was important because touch is often neglected in modern medical care, but it was too broad. He would have liked it to focus more on how various forms of touch could be integrated in medical care.
Dr. Michon stressed that the types of touch considered in these studies were positive experiences to which the volunteers agreed. “If someone doesn’t feel a touch as being pleasant, it’s likely going to stress them out,” he said.
An official website of the United States government
Here’s how you know
Official websites use .gov A .gov website belongs to an official government organization in the United States.
Secure .gov websites use HTTPS A lock ( Lock Locked padlock icon ) or https:// means you’ve safely connected to the .gov website. Share sensitive information only on official, secure websites.
- Entire Site
- Research & Funding
- Health Information
- About NIDDK
- Diabetes Overview
Healthy Living with Diabetes
- Español
On this page:
How can I plan what to eat or drink when I have diabetes?
How can physical activity help manage my diabetes, what can i do to reach or maintain a healthy weight, should i quit smoking, how can i take care of my mental health, clinical trials for healthy living with diabetes.
Healthy living is a way to manage diabetes . To have a healthy lifestyle, take steps now to plan healthy meals and snacks, do physical activities, get enough sleep, and quit smoking or using tobacco products.
Healthy living may help keep your body’s blood pressure , cholesterol , and blood glucose level, also called blood sugar level, in the range your primary health care professional recommends. Your primary health care professional may be a doctor, a physician assistant, or a nurse practitioner. Healthy living may also help prevent or delay health problems from diabetes that can affect your heart, kidneys, eyes, brain, and other parts of your body.
Making lifestyle changes can be hard, but starting with small changes and building from there may benefit your health. You may want to get help from family, loved ones, friends, and other trusted people in your community. You can also get information from your health care professionals.
What you choose to eat, how much you eat, and when you eat are parts of a meal plan. Having healthy foods and drinks can help keep your blood glucose, blood pressure, and cholesterol levels in the ranges your health care professional recommends. If you have overweight or obesity, a healthy meal plan—along with regular physical activity, getting enough sleep, and other healthy behaviors—may help you reach and maintain a healthy weight. In some cases, health care professionals may also recommend diabetes medicines that may help you lose weight, or weight-loss surgery, also called metabolic and bariatric surgery.
Choose healthy foods and drinks
There is no right or wrong way to choose healthy foods and drinks that may help manage your diabetes. Healthy meal plans for people who have diabetes may include
- dairy or plant-based dairy products
- nonstarchy vegetables
- protein foods
- whole grains
Try to choose foods that include nutrients such as vitamins, calcium , fiber , and healthy fats . Also try to choose drinks with little or no added sugar , such as tap or bottled water, low-fat or non-fat milk, and unsweetened tea, coffee, or sparkling water.
Try to plan meals and snacks that have fewer
- foods high in saturated fat
- foods high in sodium, a mineral found in salt
- sugary foods , such as cookies and cakes, and sweet drinks, such as soda, juice, flavored coffee, and sports drinks
Your body turns carbohydrates , or carbs, from food into glucose, which can raise your blood glucose level. Some fruits, beans, and starchy vegetables—such as potatoes and corn—have more carbs than other foods. Keep carbs in mind when planning your meals.
You should also limit how much alcohol you drink. If you take insulin or certain diabetes medicines , drinking alcohol can make your blood glucose level drop too low, which is called hypoglycemia . If you do drink alcohol, be sure to eat food when you drink and remember to check your blood glucose level after drinking. Talk with your health care team about your alcohol-drinking habits.
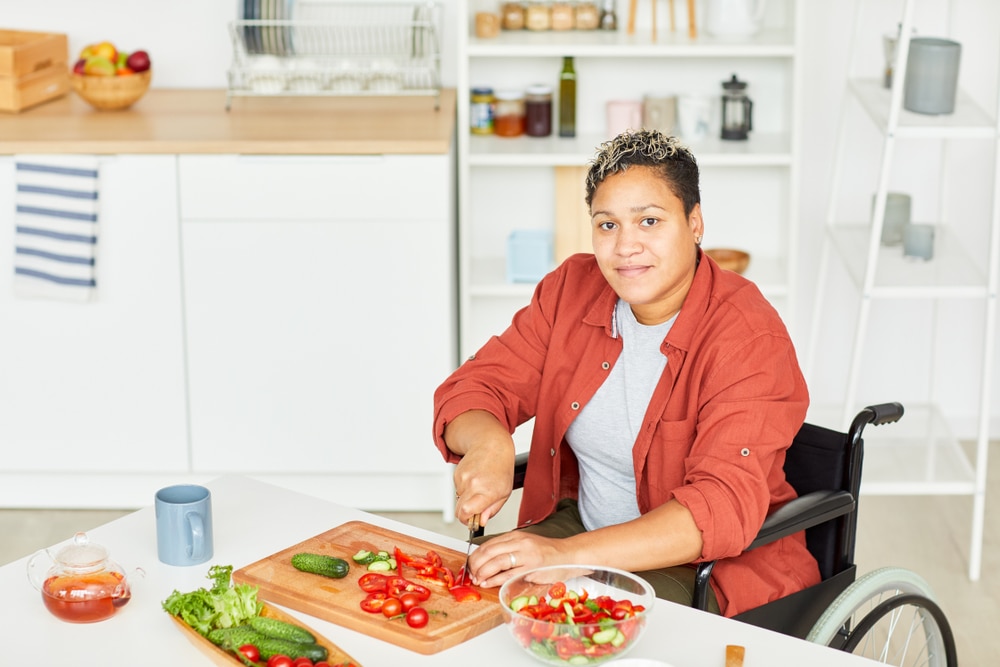
Find the best times to eat or drink
Talk with your health care professional or health care team about when you should eat or drink. The best time to have meals and snacks may depend on
- what medicines you take for diabetes
- what your level of physical activity or your work schedule is
- whether you have other health conditions or diseases
Ask your health care team if you should eat before, during, or after physical activity. Some diabetes medicines, such as sulfonylureas or insulin, may make your blood glucose level drop too low during exercise or if you skip or delay a meal.
Plan how much to eat or drink
You may worry that having diabetes means giving up foods and drinks you enjoy. The good news is you can still have your favorite foods and drinks, but you might need to have them in smaller portions or enjoy them less often.
For people who have diabetes, carb counting and the plate method are two common ways to plan how much to eat or drink. Talk with your health care professional or health care team to find a method that works for you.
Carb counting
Carbohydrate counting , or carb counting, means planning and keeping track of the amount of carbs you eat and drink in each meal or snack. Not all people with diabetes need to count carbs. However, if you take insulin, counting carbs can help you know how much insulin to take.
Plate method
The plate method helps you control portion sizes without counting and measuring. This method divides a 9-inch plate into the following three sections to help you choose the types and amounts of foods to eat for each meal.
- Nonstarchy vegetables—such as leafy greens, peppers, carrots, or green beans—should make up half of your plate.
- Carb foods that are high in fiber—such as brown rice, whole grains, beans, or fruits—should make up one-quarter of your plate.
- Protein foods—such as lean meats, fish, dairy, or tofu or other soy products—should make up one quarter of your plate.
If you are not taking insulin, you may not need to count carbs when using the plate method.
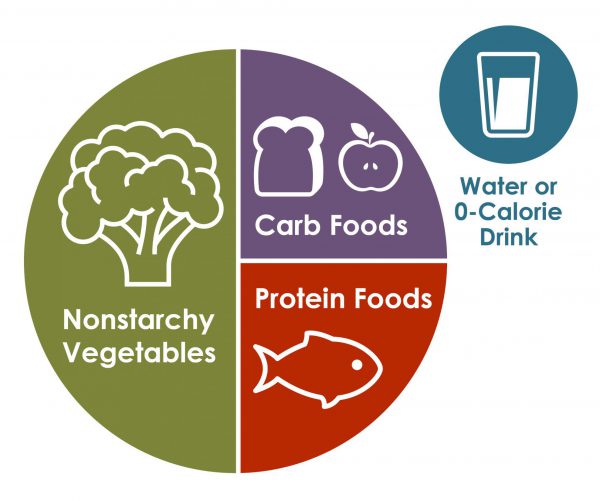
Work with your health care team to create a meal plan that works for you. You may want to have a diabetes educator or a registered dietitian on your team. A registered dietitian can provide medical nutrition therapy , which includes counseling to help you create and follow a meal plan. Your health care team may be able to recommend other resources, such as a healthy lifestyle coach, to help you with making changes. Ask your health care team or your insurance company if your benefits include medical nutrition therapy or other diabetes care resources.
Talk with your health care professional before taking dietary supplements
There is no clear proof that specific foods, herbs, spices, or dietary supplements —such as vitamins or minerals—can help manage diabetes. Your health care professional may ask you to take vitamins or minerals if you can’t get enough from foods. Talk with your health care professional before you take any supplements, because some may cause side effects or affect how well your diabetes medicines work.
Research shows that regular physical activity helps people manage their diabetes and stay healthy. Benefits of physical activity may include
- lower blood glucose, blood pressure, and cholesterol levels
- better heart health
- healthier weight
- better mood and sleep
- better balance and memory
Talk with your health care professional before starting a new physical activity or changing how much physical activity you do. They may suggest types of activities based on your ability, schedule, meal plan, interests, and diabetes medicines. Your health care professional may also tell you the best times of day to be active or what to do if your blood glucose level goes out of the range recommended for you.
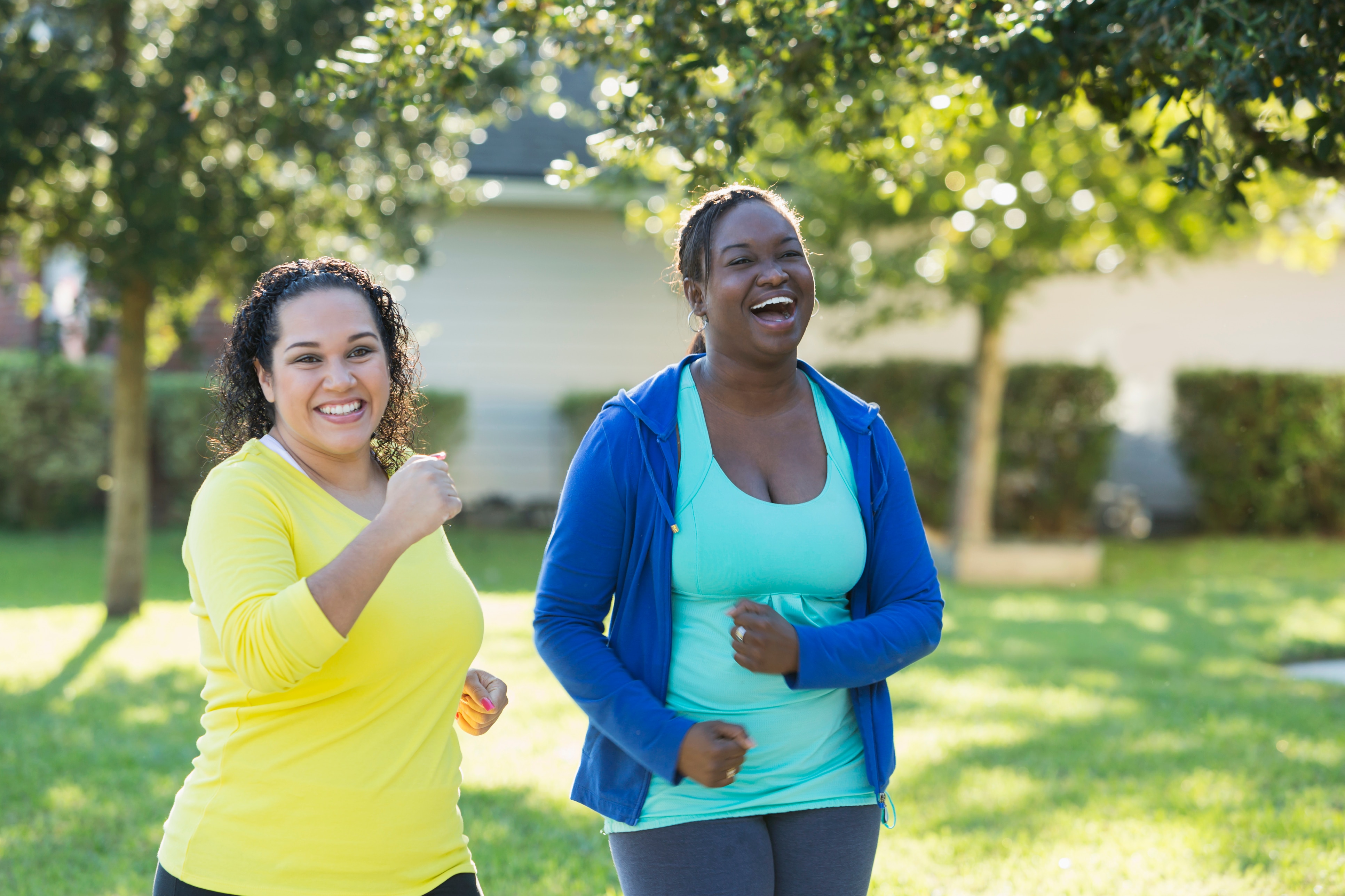
Do different types of physical activity
People with diabetes can be active, even if they take insulin or use technology such as insulin pumps .
Try to do different kinds of activities . While being more active may have more health benefits, any physical activity is better than none. Start slowly with activities you enjoy. You may be able to change your level of effort and try other activities over time. Having a friend or family member join you may help you stick to your routine.
The physical activities you do may need to be different if you are age 65 or older , are pregnant , or have a disability or health condition . Physical activities may also need to be different for children and teens . Ask your health care professional or health care team about activities that are safe for you.
Aerobic activities
Aerobic activities make you breathe harder and make your heart beat faster. You can try walking, dancing, wheelchair rolling, or swimming. Most adults should try to get at least 150 minutes of moderate-intensity physical activity each week. Aim to do 30 minutes a day on most days of the week. You don’t have to do all 30 minutes at one time. You can break up physical activity into small amounts during your day and still get the benefit. 1
Strength training or resistance training
Strength training or resistance training may make your muscles and bones stronger. You can try lifting weights or doing other exercises such as wall pushups or arm raises. Try to do this kind of training two times a week. 1
Balance and stretching activities
Balance and stretching activities may help you move better and have stronger muscles and bones. You may want to try standing on one leg or stretching your legs when sitting on the floor. Try to do these kinds of activities two or three times a week. 1
Some activities that need balance may be unsafe for people with nerve damage or vision problems caused by diabetes. Ask your health care professional or health care team about activities that are safe for you.
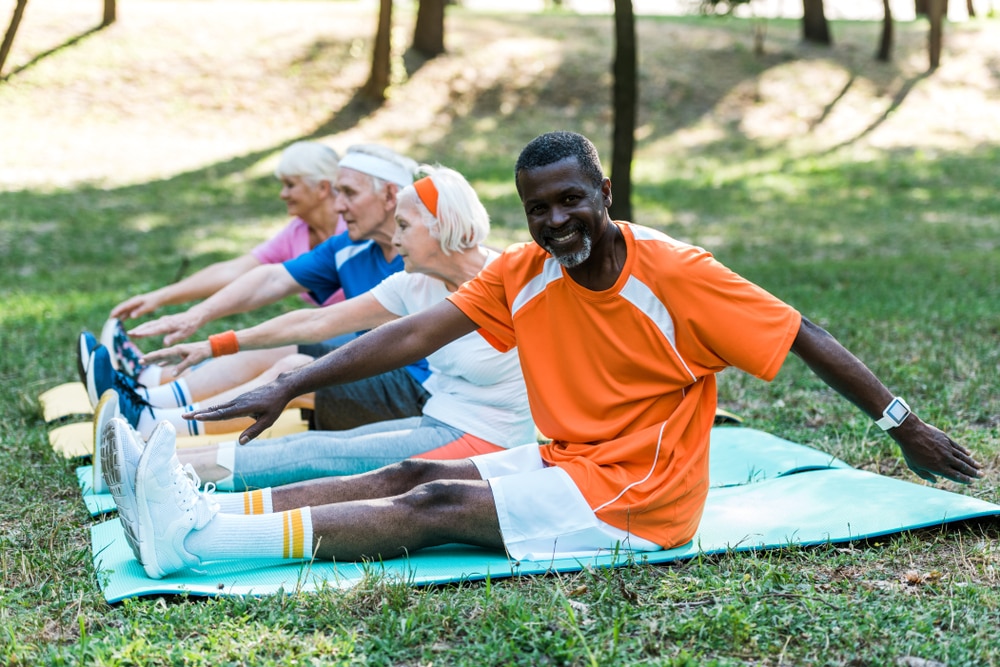
Stay safe during physical activity
Staying safe during physical activity is important. Here are some tips to keep in mind.
Drink liquids
Drinking liquids helps prevent dehydration , or the loss of too much water in your body. Drinking water is a way to stay hydrated. Sports drinks often have a lot of sugar and calories , and you don’t need them for most moderate physical activities.
Avoid low blood glucose
Check your blood glucose level before, during, and right after physical activity. Physical activity often lowers the level of glucose in your blood. Low blood glucose levels may last for hours or days after physical activity. You are most likely to have low blood glucose if you take insulin or some other diabetes medicines, such as sulfonylureas.
Ask your health care professional if you should take less insulin or eat carbs before, during, or after physical activity. Low blood glucose can be a serious medical emergency that must be treated right away. Take steps to protect yourself. You can learn how to treat low blood glucose , let other people know what to do if you need help, and use a medical alert bracelet.
Avoid high blood glucose and ketoacidosis
Taking less insulin before physical activity may help prevent low blood glucose, but it may also make you more likely to have high blood glucose. If your body does not have enough insulin, it can’t use glucose as a source of energy and will use fat instead. When your body uses fat for energy, your body makes chemicals called ketones .
High levels of ketones in your blood can lead to a condition called diabetic ketoacidosis (DKA) . DKA is a medical emergency that should be treated right away. DKA is most common in people with type 1 diabetes . Occasionally, DKA may affect people with type 2 diabetes who have lost their ability to produce insulin. Ask your health care professional how much insulin you should take before physical activity, whether you need to test your urine for ketones, and what level of ketones is dangerous for you.
Take care of your feet
People with diabetes may have problems with their feet because high blood glucose levels can damage blood vessels and nerves. To help prevent foot problems, wear comfortable and supportive shoes and take care of your feet before, during, and after physical activity.
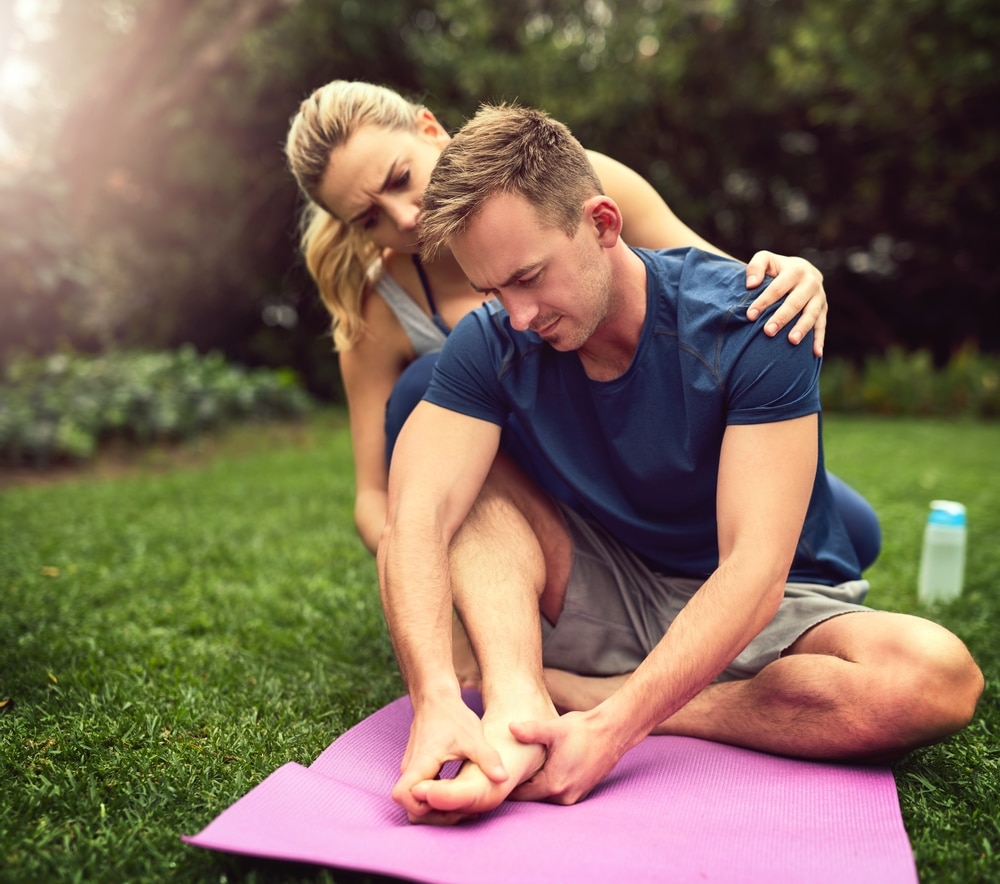
If you have diabetes, managing your weight may bring you several health benefits. Ask your health care professional or health care team if you are at a healthy weight or if you should try to lose weight.
If you are an adult with overweight or obesity, work with your health care team to create a weight-loss plan. Losing 5% to 7% of your current weight may help you prevent or improve some health problems and manage your blood glucose, cholesterol, and blood pressure levels. 2 If you are worried about your child’s weight and they have diabetes, talk with their health care professional before your child starts a new weight-loss plan.
You may be able to reach and maintain a healthy weight by
- following a healthy meal plan
- consuming fewer calories
- being physically active
- getting 7 to 8 hours of sleep each night 3
If you have type 2 diabetes, your health care professional may recommend diabetes medicines that may help you lose weight.
Online tools such as the Body Weight Planner may help you create eating and physical activity plans. You may want to talk with your health care professional about other options for managing your weight, including joining a weight-loss program that can provide helpful information, support, and behavioral or lifestyle counseling. These options may have a cost, so make sure to check the details of the programs.
Your health care professional may recommend weight-loss surgery if you aren’t able to reach a healthy weight with meal planning, physical activity, and taking diabetes medicines that help with weight loss.
If you are pregnant , trying to lose weight may not be healthy. However, you should ask your health care professional whether it makes sense to monitor or limit your weight gain during pregnancy.
Both diabetes and smoking —including using tobacco products and e-cigarettes—cause your blood vessels to narrow. Both diabetes and smoking increase your risk of having a heart attack or stroke , nerve damage , kidney disease , eye disease , or amputation . Secondhand smoke can also affect the health of your family or others who live with you.
If you smoke or use other tobacco products, stop. Ask for help . You don’t have to do it alone.
Feeling stressed, sad, or angry can be common for people with diabetes. Managing diabetes or learning to cope with new information about your health can be hard. People with chronic illnesses such as diabetes may develop anxiety or other mental health conditions .
Learn healthy ways to lower your stress , and ask for help from your health care team or a mental health professional. While it may be uncomfortable to talk about your feelings, finding a health care professional whom you trust and want to talk with may help you
- lower your feelings of stress, depression, or anxiety
- manage problems sleeping or remembering things
- see how diabetes affects your family, school, work, or financial situation
Ask your health care team for mental health resources for people with diabetes.
Sleeping too much or too little may raise your blood glucose levels. Your sleep habits may also affect your mental health and vice versa. People with diabetes and overweight or obesity can also have other health conditions that affect sleep, such as sleep apnea , which can raise your blood pressure and risk of heart disease.
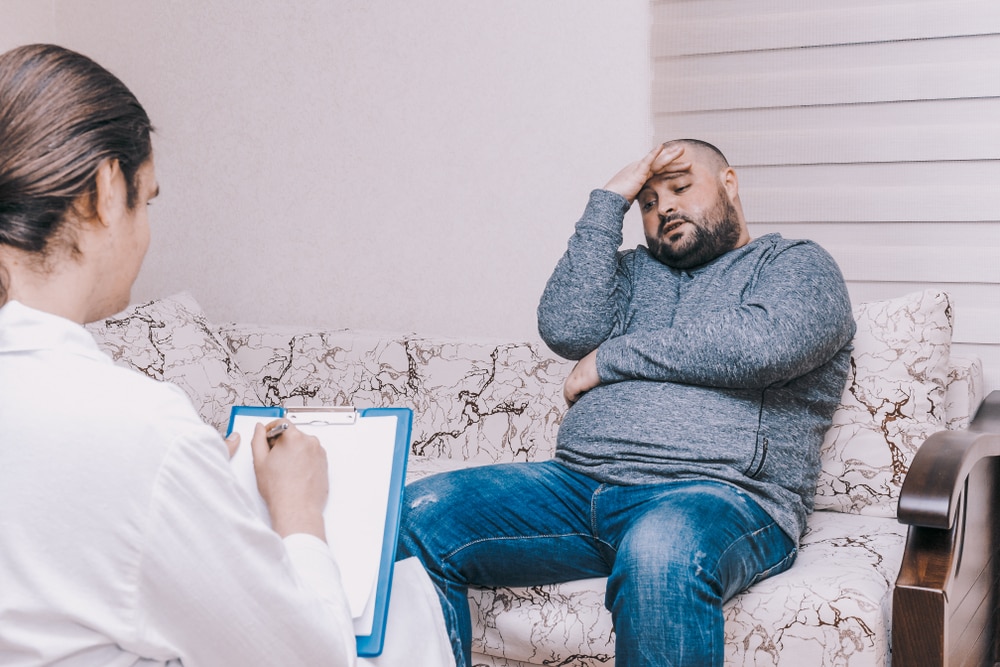
NIDDK conducts and supports clinical trials in many diseases and conditions, including diabetes. The trials look to find new ways to prevent, detect, or treat disease and improve quality of life.
What are clinical trials for healthy living with diabetes?
Clinical trials—and other types of clinical studies —are part of medical research and involve people like you. When you volunteer to take part in a clinical study, you help health care professionals and researchers learn more about disease and improve health care for people in the future.
Researchers are studying many aspects of healthy living for people with diabetes, such as
- how changing when you eat may affect body weight and metabolism
- how less access to healthy foods may affect diabetes management, other health problems, and risk of dying
- whether low-carbohydrate meal plans can help lower blood glucose levels
- which diabetes medicines are more likely to help people lose weight
Find out if clinical trials are right for you .
Watch a video of NIDDK Director Dr. Griffin P. Rodgers explaining the importance of participating in clinical trials.
What clinical trials for healthy living with diabetes are looking for participants?
You can view a filtered list of clinical studies on healthy living with diabetes that are federally funded, open, and recruiting at www.ClinicalTrials.gov . You can expand or narrow the list to include clinical studies from industry, universities, and individuals; however, the National Institutes of Health does not review these studies and cannot ensure they are safe for you. Always talk with your primary health care professional before you participate in a clinical study.
This content is provided as a service of the National Institute of Diabetes and Digestive and Kidney Diseases (NIDDK), part of the National Institutes of Health. NIDDK translates and disseminates research findings to increase knowledge and understanding about health and disease among patients, health professionals, and the public. Content produced by NIDDK is carefully reviewed by NIDDK scientists and other experts.
NIDDK would like to thank: Elizabeth M. Venditti, Ph.D., University of Pittsburgh School of Medicine.

An official website of the United States government
The .gov means it’s official. Federal government websites often end in .gov or .mil. Before sharing sensitive information, make sure you’re on a federal government site.
The site is secure. The https:// ensures that you are connecting to the official website and that any information you provide is encrypted and transmitted securely.
- Publications
- Account settings
Preview improvements coming to the PMC website in October 2024. Learn More or Try it out now .
- Advanced Search
- Journal List

Golf and Physical Health: A Systematic Review
Graeme g. sorbie.
1 Division of Sport and Exercise Sciences, School of Applied Sciences, Abertay University, Dundee, Scotland, UK
Alexander J. Beaumont
2 School of Science, Technology and Health, York St John University, York, UK
Ashley K. Williams
David lavallee, associated data.
There are no underlying data to share.
No previous systematic review has examined the physical health benefits of playing golf or caddying.
To establish the influence of golf participation and physical health in golfers and caddies. More specifically, the review intended to explore the domains of cardiovascular, metabolic and musculoskeletal health, in addition to body composition.
Systematic review.
Data Sources
Electronic literature searches were conducted using PubMed, SPORTDiscus and CINAHL databases in July 2021.
Eligibility Criteria
Experimental (randomised controlled trials, quasi-experiment, pre-post) and non-experimental (case–control, cross-sectional, cohort) articles relating to health and golf, written in English and published in peer-reviewed journals.
Of the 572 articles initially identified, 109 full-text articles were assessed for eligibility with 23 meeting the inclusion criteria. Sixteen articles were rated ‘good 'and seven ‘fair’. The influence of golf on physical health was mixed, although various articles displayed improvements in balance, systolic blood pressure (SBP) and diastolic blood pressure (DBP), high density lipoprotein-cholesterol (HDL-C) and the ratio of HDL to total cholesterol within golfers. Caddies observed improvements in bone mineral density (BMD), stiffness index and strength. Most of the findings indicate that playing golf or caddying does not influence body mass index (BMI); however, playing golf can positively change other body composition markers such as lean and fat mass.
This review demonstrated that golf participation may be an effective method for improving musculoskeletal and cardiovascular health, although mixed findings were observed. Moreover, limited longitudinal evidence suggests that playing golf can positively impact metabolic health and the influence on body composition may be parameter dependent. Additionally, the initial evidence suggests that caddying may improve musculoskeletal health. However, the studies included were limited by their methodological inconsistencies such as: study design, participant demographics and intervention prescription.
PROSPERO Registration
CRD42021267664.
Introduction
The popularity of golf is ever-growing, with a 2.3 million increase in on-course participation in Great Britain and Ireland in 2020 [ 1 ], with 2.2% of the population reported to play golf bi-weekly [ 2 ]. More females are participating in golf [ 1 ], which is also a game accessible to people of all ages, from the young to the old. Beyond golf participation, caddies contribute to the sport at all levels of the game, from recreational to professional. Typically, the caddie’s roles include, but are not limited to, carrying golf bags, attending to the flag stick, and providing strategic support to the golfer [ 3 – 6 ].
The average age of registered golfers within the UK is 41 years [ 1 ], with a forecasted 42% of golfers within Europe being older than 60 years [ 7 ]. The World Health Organisation (WHO) [ 8 ] predicts that in less than a decade, one in six people will be older than 60 years. Whilst ageing is not a direct cause for cardiovascular diseases (CVD) [ 9 ], it is associated with biological degeneration and senescence, which heightens the risk for disease and health complications [ 10 ]. In addition, falls are more common with advancing age and with an increase in frailty [ 11 ]. Furthermore, developing musculoskeletal disorders within older adults are also rising and are amongst the most common health issues [ 12 , 13 ]. Within older adults, musculoskeletal disorders such as rheumatoid arthritis and osteoarthritis are associated with increased risk of falls [ 13 , 14 ]. While the population is living longer, these extra years may not be spent in good health [ 8 ], thus limiting the health span—the phase of life without disability and free from serious illness [ 15 ]. A loss of physiological function attenuates functional status and heightens the risk of mortality and morbidity [ 16 ]. In turn, preservation of physiological function is paramount in the attainment of optimal longevity, health span [ 15 ] and in delaying future age-related chronic diseases. Therefore, effective lifestyle-behavioural strategies, such as physical activity (PA), have been considered as primary approaches in the attempt to slow the declines in physical health and functional independence, in order to increase health span [ 16 ].
Physical activity is a cost-effective, non-pharmacological method for improving health, supported by a curvilinear dose–response relationship between PA level and health benefits [ 17 ]. Golf presents an opportunity to increase PA and provides improvements in risk factors for CVD and metabolic and musculoskeletal health [ 18 ]. The metabolic cost and heart-rate responses through playing golf are dependent on numerous factors. Examples include riding an electric cart compared to carrying clubs or using a pull cart [ 19 ], carrying a bag with one or two straps [ 20 , 21 ], and playing on a hilly course, although this is debated between studies [ 19 , 22 ]. Additionally, caddies can be expected to carry a bag of at least 12.5 kg [ 3 ], which presents an additional physical challenge to walking alone, during an on-course round of golf. General golf is considered to be a moderate-intensity activity with a metabolic doequivalent (METs) of 4.8 [ 18 , 23 ], with reports that a nine-hole golf round elicits 46% of peak MET for a healthy older (64 ± 8 years) population [ 24 ]. The relative intensity of activity varies depending on health status [ 24 ] and also increases progressively with age [ 25 ]. A systematic review identified that while a single round was sufficient to achieve the American College of Sports Medicine recommendations for energy expenditure, the mode of club transportation, age and skill level of golfers all contribute to variations in PA level [ 26 ].
A scoping review was recently conducted to establish the existing body of literature related to golf and health [ 18 ]. A variety of categories were explored concerning both physical and mental health, with a recommendation for a systematic review to be conducted to further the understanding between golf and health. Moreover, the focus has long been placed on golfers, while the effect of golf caddying on health has started to receive attention [ 3 ]. Given the absence of a focused systematic review on physical health and golf derived from peer-reviewed academic literature, the present systematic review aimed to establish the influence of golf participation and physical health in golfers and caddies. More specifically, we intended to explore the domains of cardiovascular, metabolic and musculoskeletal health, in addition to body composition.
This systematic literature review was conducted in accordance with the recommendations for the Preferred Reporting Items for Systematic Review and Meta-Analysis (PRISMA) statement [ 27 ]. The systematic review was registered using the PROSPERO International database of systematic review protocols (Registration Number: CRD42021267664).
Search Terms and Criteria for Inclusion
An electronic literature search was conducted using PubMed, SPORTDiscus and CINAHL databases in July 2021, to identify available research studies that were related to golf and health. In all databases, a title and abstract search was conducted using a string of search terms that included: (Golf* OR Caddy OR Caddie OR Caddies OR Caddying) AND (Health OR Physical OR Cardiovascular OR Musculoskeletal OR MSK OR MSD OR Metabolic). Additional filters were applied to include studies only published in English. Primary source, peer-reviewed articles were eligible to be included if they contained data relating to golf and health. Specific inclusion criteria were as follows:
- Peer-reviewed articles.
- Primary research articles with any study designs (i.e., observational, cross-sectional, experimental studies) that presented a non-golf control group (cross-sectional) or determined changes (cohort or experimental) with statistical analyses.
- Golfers of any age and skill level, including both competitive and recreational golfers.
- Caddies of any age and level.
- All forms of on-course and off-course golf (18 holes, nine holes, short-form golf, driving ranges, etc.).
- English language studies.
- Assessed markers of physical health pertaining to the domains of cardiovascular, metabolic, musculoskeletal health and body composition.
Conference proceedings, reviews, clinical commentaries, case reports, theses and dissertations were excluded. Titles and abstracts were reviewed independently by two authors (AJB and GGS) for relevance, followed by full-text screening to assess eligibility based on the inclusion and exclusion criteria. Reference lists of relevant reviews were screened for any additional articles that may have been missed from the electronic database search (AJB and GGS). During the review process, a third author (AKW) arbitrated any uncertainties in study inclusion.
Data Extraction
Included articles and relevant data were extracted into a custom Microsoft Excel sheet (Version 2016) by two authors independently (AJB and GGS). Extracted data included: (1) study details, such as authors, date of publication and study design; (2) participant characteristics, such as golf status (i.e., golfer or caddie), age, sex and skill level; (3) cardiovascular measurements including systolic (SBP) and diastolic blood pressure (DBP), cardiac function, inflammatory blood markers, maximum oxygen uptake ( V ˙ O 2 max ) and associations with CVD risk; (4) musculoskeletal variables including balance, flexibility, muscle mass, thickness, strength and endurance, bone mineral density (BMD), bone mineral content (BMC) and physical competency measures; (5) metabolic variables including blood lipid profiles, bone metabolic markers and bone resorption rates; (6) body composition measurements including body mass index (BMI), fat mass, abdominal skinfold thickness and waist circumference.
Data Analysis and Quality Assessment
Narrative data analysis was conducted in each of the four domains described previously. The National Heart, Lung, and Blood Institute (NHLBI) risk assessments tools were used to assess the quality of included articles as per the NHLBI guidelines [ 28 ]. The quality assessment was conducted by one author (AKW) and then confirmed by a second author (AJB). If there was any uncertainty regarding the grade of study quality, the assessors conferred to reach an agreement. This tool contains 14 items with questions returning a ‘Yes’, ‘No’ or ‘Not Reported answer’.
Study Selection
Of the 572 articles identified, 109 full-text articles were assessed for eligibility with 23 meeting criteria and included in the full-text review (Fig. 1 ). Sixteen articles were rated as good quality and seven fair, and no articles were considered to have any research fatal flaws. Most articles included musculoskeletal measures ( N = 16) along with body composition measures ( N = 15). Fewer studies included measures of a cardiovascular nature ( N = 5) and metabolic markers ( N = 6), whereby the focus was predominantly on blood lipid profile. There was a total of 14 non-experimental studies and nine intervention studies (Table (Table1). 1 ). See Table Table2 2 for summary details of the interventions employed.

PRISMA flow diagram of the exclusion process and study results
Demographics, quality of studies, outcome measures and a summary of main findings of articles investigating the influence of golf activity on physical health
24 h-HRmean mean heart rate by 24-h ECG, ApoA1 apolipoprotein A1, BBS Berg balance scale, BMC bone mineral content, BMD bone mineral density, BMI body mass index, CPK creatine phosphokinase, CVD cardiovascular disease, DBP diastolic blood pressure, E/e’ ratio of early mitral inflow velocity-to-early diastolic tissue velocity, FGF-21 fibroblast growth factor-21, HbA1c glycated haemoglobin, HDL high-density lipoprotein, HOMA-IR homeostatic model assessment for insulin resistance, HR heart rate, HR 100W heart rate at 100 watts, HR max maximum heart rate, hs CRP high-sensitivity C-reactive protein, hs TnT high-sensitivity troponin T, IL-6 interlukin-6, IQR interquartile range, IRR incidence rate ratios, LDL low-density lipoprotein, LV-Tei left ventricular Tei Index, N number of participants, NR not reported, NA not applicable, NT-proBNP N-terminal-pro B-type natriuretic peptide, PVCs/h premature ventricular contractions per hour, SBP systolic blood pressure, SBP 100W systolic blood pressure at 100 watts, SD standard deviation, SOT sensory organisation test, TUG timed up and go, V ˙ O 2 max maximum oxygen uptake, W max maximum power output
* Model adjusted for age, light-intensity sport score, moderate-intensity sport score, strenuous-intensity sport score, muscle strengthening score, walking score, and lifestyle physical activity score. Lifestyle physical activity score includes functional activities, such as light housework, heavy housework, lawn and yard care, caregiving, and paid or volunteer work
** Model adjusted for ‘*’ in addition to risk factors for falls
Summary of intervention study information relating to golf and health
Musculoskeletal Effects
An overview of results is shown in Table Table1. 1 . Sixteen studies included measures of a musculoskeletal nature, including strength and endurance measures [ 29 – 36 ], BMD or BMC [ 29 , 34 , 37 , 38 ], balance and stability measures [ 30 , 36 , 39 – 42 ], muscle mass and thickness [ 35 , 37 , 43 ], flexibility and mobility [ 36 ], or physical competency measures [ 30 , 32 , 33 , 44 ]. Musculoskeletal strength and endurance results were mixed, particularly amongst cross-sectional studies, where Stockdale et al. [ 33 ] observed no statistically significant differences in grip (golfers: 0.33 ± 0.06 N/kg; non-golfers: 0.29 ± 0.06 N/kg) or quadriceps strength between golfers and non-golfers (golfers: 2.78 ± 0.74 N/kg; non-golfers: 2.69 ± 0.83 N/kg). Chang et al. [ 29 ] also identified no significant difference in grip strength in golfers in comparison to control subjects. Mixed results were observed for intervention studies, whereby no significant changes were observed in grip strength in age-matched participants (golf mean difference = 0.85 kg, 95% confidence interval (CI): 0.04–1.67); control mean difference = 1.26 kg, 95% CI 0.40–2.13) [ 32 ]. Positive changes were, however, noted in one golf-training study where static back-extension time increased in the golf training group (from 93 to 101 s), whereas no change was observed for controls (91–89 s) [ 36 ].
BMD results were mostly non-significant; Chang et al. [ 29 ] noted 6.7% greater lumbar BMD in golfers than control—however, total body and hip BMD were similar. Other studies with golfers found no significant differences in BMD [ 37 , 38 ]. Stability and balance measures were largely positive for golfing groups, whereby improvements were shown after training (8.9% improvement on step test [ 30 ]) and when analysed cross-sectionally in comparison to a control population [ 39 , 41 , 42 ]. Despite a low number of papers with muscle mass and thickness measures, results were largely positive, with increased muscle mass noted in the arms [ 37 ] and increased muscle thickness in golfers in comparison to non-golfers [ 43 ]. Mixed results were shown for physical competency measures, where no change was observed in walking speed after a golf programme [ 32 ]; however, improvements in timed up-and-go (TUG) tests were observed [ 33 ] or were greater than in non-golfers (13.3%) [ 30 ].
Studies in caddies mostly showed positive results, with Achilles tendon stiffness index greater in caddies across three age groups in comparison to control participants [ 35 ]. Additionally, caddies demonstrated increased quadriceps strength [ 34 , 35 ], with improvements in BMD also observed [ 34 ].
Cardiovascular Effects
Five studies considered cardiovascular measures (Table (Table1), 1 ), including DBP and SBP [ 36 , 45 , 46 ], resting heart rate and aerobic performance [ 36 , 47 ], and risk of cardiovascular disease [ 48 , 49 ]. In the large cohort study conducted by Müller-Riemenschneider et al. [ 46 ], golf was associated with increased DBP in both models evaluated (Model 1: Effect size = 2.04, 95% CI 0.44–3.64; Model 2: Effect size = 1.85, 95% CI 0.28–3.42). In other studies where SBP and DBP reduced after a golf intervention [ 45 ], it should be noted that the quality of the study was only fair, or there was a trend towards reducing a high DBP only when subjects with a pre-intervention high DBP were considered (between group differences of – 3 mmHg in favour of golf intervention) [ 36 ]. Both studies that observed a cardiovascular disease found golf was not associated with disease incidence [ 48 , 49 ]. It is, however, to be noted that the cross-sectional study only made this comparison as a single time point, and thus cannot determine causality [ 49 ]. In addition, aerobic performance determined through V ˙ O 2 max [ 45 ] or 6-min walking test did not differ between golfers and controls [ 50 ], while submaximal exercise performance improved after golf training with lower SBP, HR at 100 W [ 45 ], and lower oxygen consumption, HR and lactate at 7 METs [ 36 ].
Metabolic Effects
Six studies included measures of a metabolic nature (Table (Table1). 1 ). This included measures of blood lipid profile (total, high-density lipoprotein cholesterol [HDL], low-density lipoprotein cholesterol [LDL] and triglycerides) [ 36 , 46 , 47 , 49 ] or within a caddy population, measures of bone metabolic markers [ 34 ] or bone resorption rates through urinary pyridinoline and deoxypyridinoline levels [ 35 ]. Mixed results were observed for blood lipid profiles, including no significant associations with golf [ 46 ], significantly higher odds of ever having high cholesterol – although not when controlled for age [ 49 ], positive results for HDL levels (training effect-adjusted mean difference between groups 0.05 mmol/L (95% CI 0.00–0.10); HDL-C improvement of 5 mg/dL) and the ratio of HDL to total cholesterol (training effect-adjusted mean difference between groups 1.2% (95% CI 0.2–2.2)) [ 36 , 47 ]. Urinary pyridinoline and deoxypyridinoline levels were found to not increase in caddies in comparison to controls pre- and post-menopause [ 35 ]. However, no significant trends were identified in bone metabolic markers [ 34 ].
Body Composition Effects
BMI data were included in 12 studies (Table (Table1). 1 ). Mixed results were observed with no differences in BMI when considered cross-sectionally [ 33 , 35 , 39 , 46 , 49 , 51 ], with only Herrick et al. [ 43 ] noting lower BMI in golfers (24.8 ± 2.5 kg·m −2 ) in comparison to controls (27.9 ± 3.5 kg·m −2 ). Longitudinal studies showed similar outcomes for BMI measurements, with significant reductions noted in fewer studies [ 36 ] than those that had no change or differences between groups [ 33 , 34 , 45 , 47 ]. Studies that measured lean or fat mass largely found positive results, including a − 8% change in abdominal skin fold measurement [ 36 , 37 ] rather than no change [ 29 ].
This systematic review investigated the effects that golf participation has on physical health, specifically musculoskeletal, cardiovascular and metabolic health and body composition. Although a previous scoping review has outlined the physical health benefits that golf can provide for an individual, further research was required to systematically describe the relationship between golf participation and physical health [ 18 ]. To our knowledge, this is the first systematic review addressing the impact that golf participation, including golf play and caddying, has on cardiovascular, metabolic and musculoskeletal health, as well as body composition. Overall, the review highlighted that golf play can, in some instances, positively impact measures of physical health; however, findings across domains were mixed. Furthermore, when considering the population of golf caddies, findings highlighted that caddying may positively affect musculoskeletal health, although research remains limited in this population.
Muscular Strength and Size
Golf may be beneficial for the preservation of muscle mass and thickness. However, it must be noted that findings conflicted regarding the lower limbs, with one article observing similar muscle mass in young male golfers (29 ± 1 years) and controls [ 37 ]. In contrast, a later study demonstrated that older female golfers (69 ± 4 years) had larger relative quadriceps muscle thickness than non-golfers [ 43 ]. The latter observation is of particular importance, since age is associated with sex-independent reductions in the muscle/body weight ratio [ 52 ]. Sarcopenia, an age-related loss of skeletal muscle mass and strength [ 53 ], has been demonstrated through meta-analytical study to be associated with increased falls and fractures in older adults [ 54 ].
While hormonal changes are in part responsible for sarcopenia, environmental declines in PA are also contributory [ 53 ]. Golf offers the possibility for increasing PA of a light-moderate intensity [ 26 ], which may contribute towards the PA guidelines published by the WHO [ 55 ]. Thus, golf participation can be encouraged in order to achieve PA recommendations [ 26 ]. It is possible that with increasing PA that is likely to occur with on-course golf activity [ 56 ], golf may provide a stimulus for lower limb muscular hypertrophy. While this proposal relates to muscle thickness from limited research, the potential benefits of golf did not extend to muscular strength. From the four studies that investigated muscular strength in golfers, the consensus was that there was similar grip strength between golfers and non-golfers. Similarly, specific to lower body muscular strength, only two studies have been conducted and both found that golf was not beneficial for either quadriceps strength [ 33 ] or peak hip abductor force [ 30 ]. Golfers were reported to have walked the course, with one study describing that clubs were transported using a pushcart [ 30 ], which was outlined within the original methodological protocol article [ 57 ]. Although the METs for walking and pulling clubs with a cart (5.3 METs) are greater than walking while carrying clubs (4.3 METs) [ 23 ], the latter mode may require an additional effort. Indeed, caddies can be expected to carry a bag of at least 12.5 kg [ 3 ], and this may, in part, explain contrasting observations in the golfing literature, since hand grip and quadriceps strengths were greater in caddies than in non-caddies [ 34 , 35 ]. Through cross-sectional observations, Hoshino et al. [ 35 ] reported in a small sample of long-term golf caddies who carry clubs that quadriceps strength was greater in caddies by 18.1 kg (difference in means) than in controls. The consequential benefits that may arise from carrying an additive load rather than solely walking are promising, and are likely to also contribute towards the PA guidelines, with the inclusion of strength-based exercise on at least 2 days per week [ 17 , 55 ].
Additionally, drawing comparisons between golfers and caddies is challenging as the conflicting findings may be due, but not limited to, the differences in the transportation of clubs, the volume of activity completed, and/or the participant demographics. Indeed, the exercise stimulus may not have been sufficient in the longitudinal study [ 30 ] and the older aged population used by Stockdale et al. [ 33 ] (see Tables Tables1 1 and and2 2 for descriptions). Moreover, previous research has indicated that the step count completed by golfers was 11,948 ± 1,781 per round [ 58 ], while caddies have been reported to complete 20,499 ± 812 per round [ 34 ]. While the variation in activity is noteworthy, it is of course important to note that the golf course and skill level would influence this.
Most studies that investigated balance demonstrated a positive influence from golf. Proactive balance tests, described as anticipation of predicted disturbances [ 59 ], including functional reach, were greater in golfers [ 39 ], and the TUG test was faster in golfers than in non-golfers [ 33 ], improving by 13.3% after 12 weeks of golf practice [ 30 ]. As noted by Stockdale et al. [ 33 ], physical performance from the TUG test indicated that non-golfers were below the threshold and consequently classed as sarcopenic, whereas the golfers exceeded this threshold for the prediction of sarcopenia. As a test of functional ability [ 60 ], the TUG test is an important predictor of falls in seniors [ 61 ], and the potential benefits from golf for superior functional ability are welcomed. Other performance tests, such as the Berg Balance Scale (BBS), considered the gold-standard for balance assessments [ 59 , 62 ], indicated further benefits of golf. Schachten and Jansen [ 40 ] found that 10 weeks of golf training improved BBS in middle-aged stroke survivors. Still, similar improvements were also noted for the parallel social communications group, although this study lacked randomisation into each treatment arm. In contrast, a two-group pre-test post-test golf intervention for 20 weeks suggested static and dynamic balance did not differ [ 36 ]. Thus, more intervention studies are required with adequate randomisation to determine the influence of golf on functional balance tests. One study reported a significantly lower incidence rate ratio (IRR) of falls for golfers compared with other leisure-time physical activities (LTPAs) when PA of multiple intensities was considered [ 44 ]. Although this association was dampened after controlling for LTPA and history of falls, this may suggest that a prominent element of golf (i.e., PA) is an important and mediating factor in the risk of falls. This information is of significant clinical relevance, since falls are the second leading cause of unintentional mortality [ 63 ]. The number of deaths from falls within England during 2019 increased with age and totalled 6,138 in those > 40 years of age [ 64 ]. The ramifications for golf to aid in the preservation of muscular performance and balance are promising, with the intention of maintaining functional ability and, by extension, healthy ageing [ 65 ].
Bone Mineral Density
Studies identified within the review highlight that playing golf has minimal effects on total body BMD or BMC [ 29 , 37 , 38 ]. The influence of regional BMD is contradictory, however, with Chang et al. [ 29 ] reporting 6.7% greater lumbar spine BMD in female elite golfers compared to controls. However, Jang et al. [ 38 ] and Dorado et al. [ 37 ] reported no differences in spine BMD when comparing male golfers participating in screen golf against control subjects. It should be noted that screen golf does not take into consideration walking on the golf course and the transportation of golf clubs; therefore, this may explain the conflicting findings. However, the reporting of transportation of clubs in other studies was lacking, thus it is not clear at present whether walking a course whilst carrying is influential. Sex differences may also be contributory, since the rate of production and loss in BMD differs between women and men [ 66 ]. This may help to provide some insight as to why Chang et al. [ 29 ] observed greater spine BMD in golfers, while male golfers [ 38 ] of a similar and younger age were comparable to controls.
In consideration of golf caddies, positive findings were observed in relation to BMD and Achilles stiffness index [ 34 , 35 ]. Specifically, Goto and colleagues [ 34 ] reported that female golf caddies, at a pre-menopausal stage, significantly increased lumbar spine BMD at 6 and 12 months and proximal femur BMD following 12 months of caddying. When taking into consideration the activity levels of these two groups, caddies walked over threefold the distance of the desk workers. Moreover, since oestrogen deficiency plays a key role in a net loss of bone [ 67 ], bone production in the pre-menopausal state may be important for the preservation of BMD, facilitated by caddying.
From the limited literature exploring the influence of golf on blood pressure, the findings may be conditional on both the training intervention and baseline blood pressure. Two studies observed reductions in SBP and DBP [ 45 , 47 ], which represent beneficial changes since high blood pressure is a modifiable risk factor for CVD [ 68 ]. However, the participants completed the golf training as a vacation, and thus stress may confound the association between golf training and markers of health, as suggested by the authors, concomitant with reduced fibroblast growth factor 21 (FGF-21) [ 47 ]. In contrast, a longer intervention of 20 weeks, which may better represent the influence of sustained golf play, suggested that blood pressure did not change based on group data [ 36 ]. However, subgroup analysis in those with the highest blood pressures observed -3 mmHg in DBP in the golf group compared to controls. While this observation is not supported by cross-sectional research having shown a positive association between golf and DBP [ 46 ], it may support the proposition through meta-analysis that those with hypertension may benefit the most from endurance training [ 69 ]. Moreover, the long-term impact of golf on CVD risk requires further substantiation, since one article identified no significant association with CVD incidence [ 48 ]. Additional work, whilst not included in the review due to a lack of a no-golf comparator/reference group, suggests that mortality is approximately 60% compared to that of the estimated general population [ 70 ]. Thus, future work is required to determine the long-term health implications and associated risk for CVD following golf participation.
Cardiorespiratory fitness ( V ˙ O 2 max ) is a strong predictor of mortality in men and women [ 71 ]. However, V ˙ O 2 max did not change after 1 week [ 45 ] or 20 weeks [ 36 ] of golf training, and nor did maximum power output ( W max ) [ 45 ]. It is possible that the intensity of golf was not sufficient to induce central adaptations in left ventricular function [ 45 ] and/or peripheral adaptation to elicit changes in aerobic fitness. However, low volume, high-intensity interval exercise in older men has yielded benefits in V ˙ O 2 max [ 72 ] and peak power output [ 73 ]. Nonetheless, studies in this review did observe improvements in submaximal exercise performance [ 36 , 45 ] and, thus, this suggests less cardiovascular demand with superior exercise economy after the golf training, despite no changes in V ˙ O 2 max .
Several metabolic variables were investigated (Table (Table1) 1 ) but with mixed findings, making it challenging to propose a consensus concerning metabolic health parameters. However, more frequently investigated was the blood lipid profile, with reports of no association with golf and triglycerides, HDL cholesterol and LDL cholesterol [ 46 ]. Intervention studies, however, reported increased HDL-C [ 47 ] and the ratio of HDL to total cholesterol [ 36 ] following golf training. This is encouraging since HDL is an important predictor of CVD risk and higher HDL is inversely associated with coronary heart disease [ 74 , 75 ]. However, although cross-sectional analysis golfers were at greater odds of being diagnosed with high cholesterol than non-golfers [ 49 ], this difference was abolished when controlling for both age and physical activity, which may be stronger determinants of total cholesterol than golf participation.
The disparity in results relating to blood lipid profile may be due in part to study designs. Both intervention studies, irrespective of duration, provided positive results after golf training, whereas the cross-sectional studies, which are likely influenced by confounding between-subject variables, were negligible or negative. Thus, additional confirmatory studies concerning long-term golf play and metabolic health are required.
Most cross-sectional [ 33 , 39 , 46 , 49 , 51 ] and intervention [ 45 , 47 ] studies indicated that playing golf or caddying [ 34 , 35 ] does not impact BMI. Some studies, however, have suggested the contrary, that BMI was lower in golfers than controls [ 43 ], reduced following a 20-week golf season [ 36 ], or increased by 0.7% following at least 15 × 90-min golf classes over a 5-month period [ 31 ]. Nevertheless, the true physiological significance of a 0.17 kg . m 2 increase as reported in the latter warrants mention. These studies observing changes in BMI were in the minority, but the contradiction may arise from the methodological inconsistencies of the golf interventions and participant demographics, making it difficult to draw sound conclusions in relation to the impact of within-subject long-term golf play and BMI. Indeed, BMI is associated with all-cause mortality in a J-shaped relationship [ 76 ], and an apparent lack of evidence to suggest golf produces a universal change in BMI, which could be inferred as both beneficial and deleterious depending on baseline BMI.
BMI may not be suitable for differentiating fat mass from lean mass, especially when assessing BMI change [ 77 ]. The influence of golf on body composition from cross-sectional studies was equally undecided as to whether golf is beneficial [ 29 , 37 , 38 , 46 ]. From intervention studies, fat mass did not differ between golfers and controls in two intervention studies; however, they were only 1 week in duration [ 45 , 47 ]. In contrast, a more substantial intervention period of 20 weeks yielded reductions in abdominal skinfold thickness and waist circumference [ 36 ]. These observations are of particular importance since central adiposity/waist circumference is a key component of the metabolic syndrome [ 78 ], which represents a constellation of risk factors associated with greater risk for developing CVD and type 2 diabetes mellitus [ 79 ]. Before a stronger conclusion can be elucidated, however, more intervention studies that are of sufficient duration are necessary.
Strengths and Limitations
The current review provides valuable insight into the health benefits of playing golf and caddying; however, there are some noteworthy limitations to consider. Whilst efforts were made to ensure a rigorous and thorough search process, it is possible that some articles may have been missed, which may include peer-reviewed journal articles not written in English. The reviewed studies provided a variety of study designs and wide heterogeneity. Additionally, the golfers and caddies differed in sex, age and sample size, and, as a result, a meta-analysis was not feasible at this moment in time. The review was limited to four outcome-measure categories in relation to health; therefore, there may be other outcome measures relevant to the health benefits of golf and caddying. However, the intention was to focus on physical health; other studies should continue to establish the influence of golf on mental health and wellbeing.
The NHLBI risk assessments tools demonstrated that the quality of included studies was ‘fair’ to ‘good’. In relation to intervention studies, approximately 70% were rated ‘good’, suggesting the current evidence is strong. While many longitudinal studies were rated ‘good’, these studies varied considerably with regard to: heterogeneity of participants, duration of the intervention (1 week to 12 months), golf activity performed, and frequency of activity. It is, therefore, difficult to draw sound recommendations regarding duration, activity and frequency of golf interventions to improve health. A limited number of studies ( N = 2) investigated the health benefits of caddying; nevertheless, the initial evidence suggests that golf caddying may have a positive impact on musculoskeletal health. To draw stronger conclusions within this area, however, additional longitudinal research is required, particularly within the male population. Furthermore, a limited number of studies ( N = 5) reported specifically that golfers or caddies walked the course [ 30 , 33 , 34 , 36 , 48 ], and only two studies indicated the golfers’ club transportation method [ 30 , 48 , 57 ]. As previously discussed, the metabolic demand of golf varies depending on the golfer’s club transportation choice and if they walk the course or use a motorized golf cart [ 23 , 26 ]. Therefore, future golf course participation-related studies should take into consideration golfers’ and caddies’ mode of transportation (e.g., walking or using a motorised cart) and club transportation (e.g., carrying clubs, pulling or pushing a cart) on the golf course, when initially recruiting their sample. In addition, this information should be reported within studies to improve clarity, which will enable appropriate between-study key findings. Moreover, whilst beyond the direct scope of this review, interest has increased relating to the adverse effects of golf participation pertaining to injuries and musculoskeletal and cardiovascular risks, in addition to skin-related issues associated with golf participation [ 3 , 18 , 80 , 81 ]. Accordingly, more studies are required to fully understand the depth and breadth of the impact that golf has on both players and caddies [ 3 ].
Beyond the limitations, this review is the first to systematically collate the literature in relation to the health benefits of playing golf and caddying. Thus, this review advances our understanding of the potential impact that playing golf and caddying can have on body composition and musculoskeletal, cardiovascular and metabolic health.
Conclusions
Golf may be an effective method for improving musculoskeletal, cardiovascular and metabolic health. Additionally, most of the evidence suggests that playing golf does not influence body composition (BMI). Yet, there was also evidence to suggest that golf was not beneficial for the domains studied, although this may be dependent on: the sample of golfers, study design, the length of the intervention, and the frequency of activity. Consequently, the influence of playing golf on physical health requires further study with consideration of such methodological factors. Furthermore, the initial evidence suggests that golf caddying may positively impact musculoskeletal health; however, it would be appropriate to conduct further investigations within this area due to the limited literature at present.
Declarations
The authors received no financial support for the research, authorship, and/or publication of this article.
Graeme G. Sorbie, Alexander J. Beaumont, Ashley K. Williams and David Lavallee declare that they have no conflicts of interest relevant to the content of this review.
Full ethics approval was granted from Abertay University School of Health Sciences Ethics Committee.
Not applicable.
GGS, AJB and AKW wrote the first draft of the manuscript. GGS and AJB conducted database searches and article identification. Data extraction was conducted by GGS, AJB and AKW, and methodological quality assessments were conducted by AKW and then confirmed by AJB. DL revised the original manuscript.
Graeme G. Sorbie and Alexander J. Beaumont share joint first authorship.
Articles included in the systematic review are denoted as *
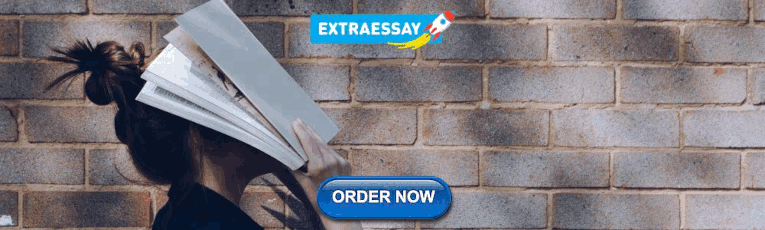
IMAGES
VIDEO
COMMENTS
The charge of the 2018 Physical Activity Guidelines Advisory Committee (PAGAC) was to review the scientific literature of the relationships between PA and QoL. The PAGAC Brain Health Subcommittee was to focus on the general population and not those with chronic diseases being covered by other PAGAC subcommittees.
In this systematic review of CSRs, we found a large body of evidence on the beneficial effects of physical activity/exercise on health outcomes in a wide range of heterogeneous populations. Our data shows a 13% reduction in mortality rates among 27,671 participants, and a small improvement in QOL and health-related QOL following various modes ...
2. Definitions of Physical Activity, Exercise, Training, Sport, and Health. Definitions and terms are based on "Physical activity in the prevention and treatment of disease" (FYSS, www.fyss.se [Swedish] []), World Health Organization (WHO) [] and the US Department of Human Services [].The definition of physical activity in FYSS is: "Physical activity is defined purely physiologically, as ...
Introduction and background. Physical activity has its origins in ancient history. It is thought that the Indus Valley civilization created the foundation of modern yoga in approximately 3000 B.C. during the early Bronze Age [].The beneficial role of physical activity in healthy living and preventing and managing health disorders is well documented in the literature.
Physical Fitness, Exercise Self-Efficacy, and Quality of Life in Adulthood: A Systematic Review August 2020 International Journal of Environmental Research and Public Health (IJERPH) 17(17):6343
Physical literacy is a multidimensional concept that describes a holistic foundation for physical activity engagement. Understanding the utilization and effectiveness of physical literacy in the context of health and the health care setting will support clinical and population health programming. The purpose of this rapid scoping review was to: 1) map the conceptualization of physical literacy ...
Receiving touch is of critical importance, as many studies have shown that touch promotes mental and physical well-being. We conducted a pre-registered (PROSPERO: CRD42022304281) systematic review ...
This review supports the encouragement of adolescent and young adult physical activity, noting the improvements seen across the physical, mental and social wellbeing outcomes. ... mental and social health through physical activity: A Systematic literature review Health Promot J Austr. 2022 Jul;33(3) :590 ...
Subjective Well-Being and Physical Health: A Narrative Literature Review with Suggestions for Future Research. Alex Zautra and Ann Hempel View all authors and affiliations. Volume 19, Issue 2. ... Stress and the Development of Physical Illness, in Health Psychology, Stane G. C., Cohen F., and Adler W. E. (eds.), Jossey-Bass, San Francisco, pp ...
Level of physical activity. Recommendations regarding the level of PA required for physical health benefits and the prevention of chronic disease, across the lifespan, are well established (U.S. Department of Health and Human Services, Citation 2018).It is recommended that children and adolescents aged 6-17 years engage in PA of moderate to vigorous intensity for a minimum of 60 min per day.
This systematic review aims to provide a narrative synthesis of the literature exploring psychological and physical health factors that influence QoL in adults with asthma. Results Study ...
The literature review summary has 4 main sections. This Introduction provides background information about the rationale for focusing on older adults and the process for reviewing the literature and developing the conclusions. The Methods utilized by the Literature Review Team are detailed in the following section.
As described earlier, negative psychological health, including depression, anxiety, and stress, is associated with smoking, lower levels of physical activity, poorer diet quality, and being overweight or obese. 25,95 These lifestyle factors are all causally linked to an increased risk of the development of CVD. 5 Although the question of ...
To begin, this review only explored studies involving exercise durations over 10 min, but future studies should expand their scope to explore durations of exercise that contribute to brain health. The primary purpose of this review was to conduct subgroup analysis based on different research designs (measuring fNIRS before, during, and after ...
Regular participation in physical activity, either recreational, or planned, structured, and repetitive is capable of yielding a multitude of health-improving benefits. Traditionally, physical activity has been recognized for its robust modulatory effects on cardiometabolic, neuromusculoskeletal, and immunological health and function. However, a sufficient quantity of emerging studies lends ...
Given the absence of a focused systematic review on physical health and golf derived from peer-reviewed academic literature, the present systematic review aimed to establish the influence of golf participation and physi-cal health in golfers and caddies. More specifically, we intended to explore the domains of cardiovascular, met-abolic and ...
DISCUSSION. This scoping review synthesised heterogeneous research documenting the impact of nature on health. Of the 39 included studies, nature-based interventions were found to have improved mental, physical/ physiological and cognitive health outcomes across 98%, 83%, and 75% of articles, respectively ( Figure 5 ).
Despite limitations in the literature, our review chronicles an evolution in the field of social capital and health in terms of size and sophistication. Overall, these studies suggest that social capital may be an important protective factor for some physical health outcomes, but further research is …
A large number of the studies have found that the most significant effects of physical exercise have been felt in the short and medium-term, while in the longer term the effects have been less significant. Empirical evidence has shown a positive relationship between physical training and selected mental health variables. In nonclinical studies the most significant effects of physical exercise ...
A literature review of evidence on physical activity ... showing graded health benefits of physical activity for reducing of a range of non-communicable diseases (US Surgeon General 1996). ... barriers to physical activity in New Zealand specific literature included health problems and concerns, lack of support and encouragement, and cultural ...
A literature review was completed to identify the evidence associated with the impact of exercise on mental health and well-being. Keywords: exercise, mental health, depression, anxiety, physical activity, mental health disorders, exercise therapy, mental health benefit . Benefits of Exercise on Mental Health: A Literature Review
Several mechanisms linking housing affordability and both physical and mental health have been proposed in a recent systematic review by Downing and expanded upon by Rodgers et al. ... Our review of the literature found generally adverse associations between housing instability and cardiometabolic health conditions of overweight/obesity ...
Physical inactivity is a global public health problem. A practical solution would be to build physical activity into the daily routine by using active modes of transport. Choice of transport mode can influence cancer risk through their effects on levels of physical activity, sedentary time, and environmental pollution. This review synthesizes existing evidence on the associations of specific ...
Objective: In this paper, we aimed to provide an overview of the antecedents and consequences of intelligent physical robot use in health care and to propose potential agendas for future research through a systematic literature review. Methods: We conducted a systematic literature review on intelligent physical robots in the health care field ...
Pre-service physical education teachers (PSPTs) have long been an important area of specific development in physical education and have become a significant force in the field of physical education and research over the past two decades. ... A bibliometric and systematic review Heliyon. 2024 Mar 28;10(7): e28702 ... countries, journals, and ...
In the literature, physical literacy itself is frequently seen as the outcome or endpoint, with a primary focus on fundamental movement skills in school-age children, ... Findings from this review suggest health care providers are not engaged with the construct of physical literacy. The absence of literature examining how health care providers ...
Background There is limited evidence of the associations between postural-derived sitting time, waist-worn derived sedentary time and children's health and the moderation effect of physical activity (PA). This study examined associations of children's device-measured sitting time with cardiometabolic health risk factors, including moderation by physical activity. Methods Cross-sectional ...
Scientists reviewed 212 studies involving 12,966 individuals, finding strong evidence of health benefits in adults that engaged in touch with other humans or objects such as weighted blankets.
Physical activity may help you manage your diabetes and stay healthy. Do different types of physical activity. People with diabetes can be active, even if they take insulin or use technology such as insulin pumps. Try to do different kinds of activities. While being more active may have more health benefits, any physical activity is better than ...
This is the first systematic review of literature having investigated the influence of golf and caddying on physical health. Golf may be beneficial for musculoskeletal, metabolic and cardiovascular health; however, findings were mixed and likely dependent on the parameters assessed, in addition to golfer's age, baseline health status and the golf activity performed.