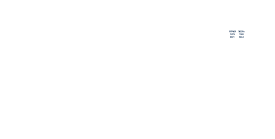
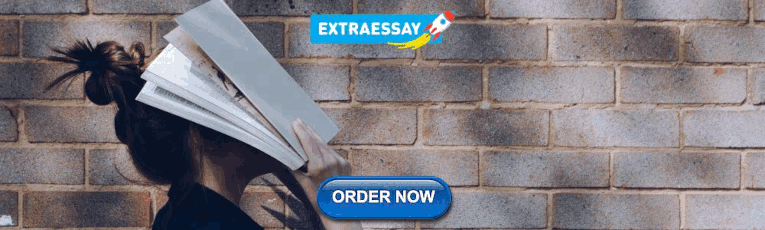
Sustainable Development Goal 1
End poverty in all its forms everywhere.
Sustainable Development Goal 1 is to “end poverty in all its forms everywhere”, according to the United Nations .
The visualizations and data below present the global perspective on where the world stands today and how it has changed.
Longer-term trends, research, and additional data on poverty can be found on Our World in Data, particularly our topic page on poverty .
The UN has defined 7 targets and 13 indicators for SDG 1. Targets specify the goals, and indicators represent the metrics by which the world tracks whether these targets are achieved. Below we quote the original text of all targets and show the data on the agreed indicators.
Target 1.1 Eradicate extreme poverty
Sdg indicator 1.1.1 share below the international poverty line.
Definition of the SDG indicator: Indicator 1.1.1 is the “proportion of the population living below the international poverty line by sex, age, employment status and geographic location (urban/rural)” in the UN SDG framework .
The international poverty line is defined as living on $2.15 per day ( updated from the previous poverty line of $1.90 in 2015).
This poverty line is measured in international dollars, a hypothetical currency that adjusts for price differences between countries (purchasing power parity). It is measured in prices of 2017 to adjust for price changes over time (inflation).
Data for this indicator on the proportion of the population below the international poverty line is shown in the interactive visualization. Breakdowns by sex, age, employment status, and geographical location are not available for all countries, but our topic page on poverty includes some relevant measures in this context.
Target: By 2030, “eradicate extreme poverty for all people, everywhere ” . This is defined by the UN based on the international poverty line. 1
More research: Further data and research can be found on the Our World in Data topic page on poverty .
Additional charts
- Share of population living in extreme poverty (historical estimates)
- Population living in extreme poverty by region
- Share of population living in multidimensional poverty
Target 1.2 Reduce poverty by at least 50%
Sdg indicator 1.2.1 share below the national poverty line.
Definition of the SDG indicator : Indicator 1.2.1 is the “proportion of the population living below the national poverty line” in the UN SDG framework .
National poverty lines differ by country depending on country circumstances, living standards, and cost of living.
Data for this indicator on the share of a country's population which lives below each country's specific national poverty line is shown in the interactive visualization.
Target: By 2030, “reduce at least by half the proportion of men, women, and children of all ages living in poverty in all its dimensions according to national definitions. ”
More research: Further data and research can be found at the Our World in Data topic page on poverty .
SDG Indicator 1.2.2 Share in multidimensional poverty according to national definitions
Definition of the SDG indicator : Indicator 1.2.2 is the “proportion of men, women and children of all ages living in poverty in all its dimensions according to national definitions” in the UN SDG framework .
This indicator is measured via related measures of multidimensional poverty, all of which are constructed according to national definitions. Multidimensional poverty refers to being deprived in a range of standard indicators related to health, education, and living standards.
Related data : Data on multidimensional poverty, measured as the Multidimensional Poverty Index (MPI), can be found in this chart . This data has much better coverage across countries and time, and is measured in a consistent way, making it comparable between countries.
Target 1.3 Implement social protection systems
Sdg indicator 1.3.1 population covered by social protection floors/systems.
Definition of the SDG indicator : Indicator 1.3.1 is the “proportion of population covered by social protection floors/systems, by sex, distinguishing children, unemployed persons, older persons, persons with disabilities, pregnant women, newborns, work-injury victims and the poor and the vulnerable” in the UN SDG framework .
This indicator is measured as the share of the population effectively covered by a social protection system. Such systems include child and maternity benefits, support for persons without a job, persons with disabilities, victims of work injuries, and older persons.
In our topic page on government spending , you can find additional data, including some of the breakdowns mentioned in the definition of indicator 1.3.1.
Target: The SDG target is to “implement nationally appropriate social protection systems and measures for all, including floors, and by 2030 achieve substantial coverage of the poor and the vulnerable.”
- Adequacy of social insurance systems
- Adequacy of unemployment benefits
- Adequacy of social safety net programs
Target 1.4 Equal rights to ownership, basic services, technology, and economic resources
Sdg indicator 1.4.1 access to basic services.
Definition of the SDG indicator : Indicator 1.4.1 is the “proportion of population living in households with access to basic services” in the UN SDG framework .
The UN defines basic services as “public service provision systems that meet human basic needs” and defines this indicator in terms of access to 9 components: drinking water, sanitation, hygiene facilities, clean fuels and technology, mobility, waste collection, health care, education, and information services. These components also appear elsewhere in the SDG framework as indicators.
Since internationally-comparable data on this indicator is currently unavailable, we show here the share of the world population with access to four basic services: improved drinking water, sanitation, electricity, and clean cooking fuels. You can view the data for different countries or regions using the “Change country” button at the top of the chart.
Target: By 2030, “ensure that all men and women, in particular the poor and the vulnerable, have access to basic services.” 2 This sets a target of universal access to basic services for all households.
More research: Further data and research can be found on the Our World in Data topic pages on clean water and sanitation , energy , and indoor air pollution .
- Access to electricity
- Access to clean cooking fuels
- Access to safe sanitation
- Access to safe drinking water
SDG Indicator 1.4.2 Secure tenure rights to land
Definition of the SDG indicator : Indicator 1.4.2 is the “proportion of total adult population with secure tenure rights to land, (a) with legally recognized documentation, and (b) who perceive their rights to land as secure, by sex and type of tenure” in the UN SDG framework .
Data for this indicator is shown in the interactive visualizations, with the first chart showing data on indicator 1.4.2(a) for the share of adults with legal documentation of their rights to land, and the second chart showing data on indicator 1.4.2(b) for the share of individuals who perceive their rights to land as secure.
Target: By 2030, “ensure that all men and women, in particular the poor and the vulnerable, have equal rights to economic resources, as well as access to basic services, ownership and control over land and other forms of property.” 2
Target 1.5 Build resilience to environmental, economic, and social disasters
Sdg indicator 1.5.1 deaths and affected persons from natural disasters.
Definition of the SDG indicators : Indicators 1.5.1 are the “number of deaths, missing persons and directly affected persons attributed to disasters per 100,000 population” in the UN SDG framework .
In the interactive visualizations, we show in the first chart a component of this indicator: the rate of deaths and missing persons from natural disasters, measured as the number of deaths and missing persons per 100,000 population per year. The other charts in the series include a range of metrics relevant to indicator 1.5.1.
Target: “By 2030, build the resilience of the poor and those in vulnerable situations and reduce their exposure and vulnerability to climate-related extreme events and other economic, social and environmental shocks and disasters”
More research: Further data and research can be found on the Our World in Data topic page on natural disasters .
SDG Indicator 1.5.2 Direct economic loss from natural disasters
Definition of the SDG indicator : Indicator 1.5.2 is the “direct economic loss attributed to disasters in relation to global gross domestic product (GDP)”in the UN SDG framework .
This indicator measures the ratio of direct economic loss from a disaster to gross domestic product, where direct economic loss is defined as the monetary value of totally or partially destroyed physical assets in the affected area. This includes losses in agriculture, all other productive assets, housing, critical infrastructure, and cultural heritage.
- Absolute economic losses from disasters by country
- Global weather-related disaster losses as a share of GDP
SDG Indicator 1.5.3 Disaster risk reduction strategies
Definition of the SDG indicator : Indicator 1.5.3 is the “number of countries that adopt and implement national disaster risk reduction strategies in line with the Sendai Framework for Disaster Risk Reduction 2015–2030” in the UN SDG framework .
The Sendai Framework for Disaster Risk Reduction 2015-2030 is an international agreement that aims to strengthen disaster preparedness to reduce risk and losses from disasters. Although the indicator definition is framed in terms of the number of countries adopting national disaster risk reduction strategies in line with the Sendai Framework, the United Nations tracks this measure in terms of country levels of implementation.
The interactive visualization shows data for this indicator in terms of levels of country implementation, on a scale from 0 to 1, based on an average score from 10 scored sub-indicators that collectively reflect progress towards implementation of the Sendai Framework.
- Disaster risk reduction score
SDG Indicator 1.5.4 Local disaster risk reduction
Definition of the SDG indicator : Indicator 1.5.4 is the “proportion of local governments that adopt and implement local disaster risk reduction strategies in line with national disaster risk reduction strategies” in the UN SDG framework .
In this context, “local governments” refers to sub-national administrative bodies responsible for developing disaster risk reduction strategies.
Data for this indicator is shown in the interactive visualization.
Target: By 2030, “build the resilience of the poor and those in vulnerable situations and reduce their exposure and vulnerability to climate-related extreme events and other disasters.” 3
Target 1.a Mobilization of resources to end poverty
Sdg indicator 1.a.1 development assistance for poverty reduction.
Definition of the SDG indicator : Indicator 1.a.1 is the “total official development assistance grants from all donors that focus on poverty reduction as a share of the recipient country’s gross national income” in the UN SDG framework .
Official development assistance refers to flows to countries and territories on the Organization for Economic Co-operation and Development’s Development Assistance Committee (DAC) and to multilateral institutions which meet a set of criteria related to the source of the funding, the purpose of the transaction, and the concessional nature of the funding.
This indicator is measured differently for donor and recipient countries, and data is accordingly shown separately for donor and recipient countries in the interactive visualizations. For recipient countries, this is shown as official development assistance grants focused on poverty reduction from all donors as a share of the recipient country’s gross national income. For donor countries, this is shown as bilateral official development assistance grants focused on poverty reduction as a share of a donor country’s gross national income.
Target: By 2030, “ensure significant mobilization of resources from a variety of sources to implement programmes and policies to end poverty in all its dimensions.” 4
SDG Indicator 1.a.2 Government spending on essential services
Definition of the SDG indicator: Indicator 1.a.2 is the “proportion of total government spending on essential services (education, health and social protection)” in the UN SDG framework .
Since internationally comparable data on all components of this indicator is not currently available, data on the percentage of government spending on health and education are shown in the two interactive visualizations.
Target: By 2030, “ensure significant mobilization of resources to implement programmes and policies to end poverty in all its dimensions.” 4
There is no defined target for this indicator.
More research: Further data and research can be found at the Our World in Data topic pages on financing healthcare , financing education , and public spending .
- Social spending
Target 1.b Policy frameworks for poverty eradication
Sdg indicator 1.b.1 pro-poor public spending.
Definition of the SDG indicator: Indicator 1.b.1 is “pro-poor public social spending” in the UN SDG framework .
This indicator measures spending by country governments to benefit the poor in terms of health, education, and direct transfers. Data is not currently available for most countries and is not reported here.
Target: “Create sound policy frameworks to support accelerated investment in poverty eradication actions.” 5
No data for this indicator
We are currently not aware of data for this indicator. You can notify us of available data for this indicator via our feedback form .
The full text of the target reads: “By 2030, eradicate extreme poverty for all people everywhere, currently measured as people living on less than $1.25 a day”. However, the poverty line has since been updated to $2.15 a day, and the UN tracks this measure accordingly.
Full text: ”By 2030, ensure that all men and women, in particular the poor and the vulnerable, have equal rights to economic resources, as well as access to basic services, ownership and control over land and other forms of property, inheritance, natural resources, appropriate new technology and financial services, including microfinance.”
Full text: “By 2030, build the resilience of the poor and those in vulnerable situations and reduce their exposure and vulnerability to climate-related extreme events and other economic, social, and environmental shocks and disasters.”
Full text: “Ensure significant mobilization of resources from a variety of sources, including through enhanced development cooperation, in order to provide adequate and predictable means for developing countries, in particular least developed countries, to implement programmes and policies to end poverty in all its dimensions”
Full text: ”Create sound policy frameworks at the national, regional and international levels, based on pro-poor and gender-sensitive development strategies, to support accelerated investment in poverty eradication actions”
Cite this work
Our articles and data visualizations rely on work from many different people and organizations. When citing this article, please also cite the underlying data sources. This article can be cited as:
BibTeX citation
Reuse this work freely
All visualizations, data, and code produced by Our World in Data are completely open access under the Creative Commons BY license . You have the permission to use, distribute, and reproduce these in any medium, provided the source and authors are credited.
The data produced by third parties and made available by Our World in Data is subject to the license terms from the original third-party authors. We will always indicate the original source of the data in our documentation, so you should always check the license of any such third-party data before use and redistribution.
All of our charts can be embedded in any site.
Our World in Data is free and accessible for everyone.
Help us do this work by making a donation.
- Human Development Report 2023-24
- The paths to equal
2023 Global Multidimensional Poverty Index (MPI)
- 2023 Gender Social Norms Index Publication
- Human Development Index
- Country Insights
- Human Climate Horizons data and insights platform
- Thematic Composite Indices
- Documentation and downloads
- What is Human development?
- NHDR/RHDR Report preparation toolkit
Unstacking global poverty: Data for high impact action
UNDP (United Nations Development Programme). 2023. 2023 Global Multidimensional Poverty Index (MPI): Unstacking global poverty: Data for high impact action. New York.
English , Spanish , French , Arabic
This report presents a compact update on the state of multidimensional poverty (henceforth referred to as “poverty”) in the world. It compiles data from 110 developing countries covering 6.1 billion people, accounting for 92 percent of the population in developing countries. It tells an important and persistent story about how prevalent poverty is in the world and provides insights into the lives of poor people, their deprivations and how intense their poverty is—to inform and accelerate efforts to end poverty in all its forms. As still only a few countries have data from after the COVID-19 pandemic, the report urgently calls for updated multidimensional poverty data. And while providing a sobering annual stock take of global poverty, the report also highlights examples of success in every region.
Learn more about MPI
Explore 2023 MPI data
Key findings (across 110 countries):, countries halved multidimensional poverty within 15 years., 534 million, 485 million, 566 million, mpi dimensions and indicators.
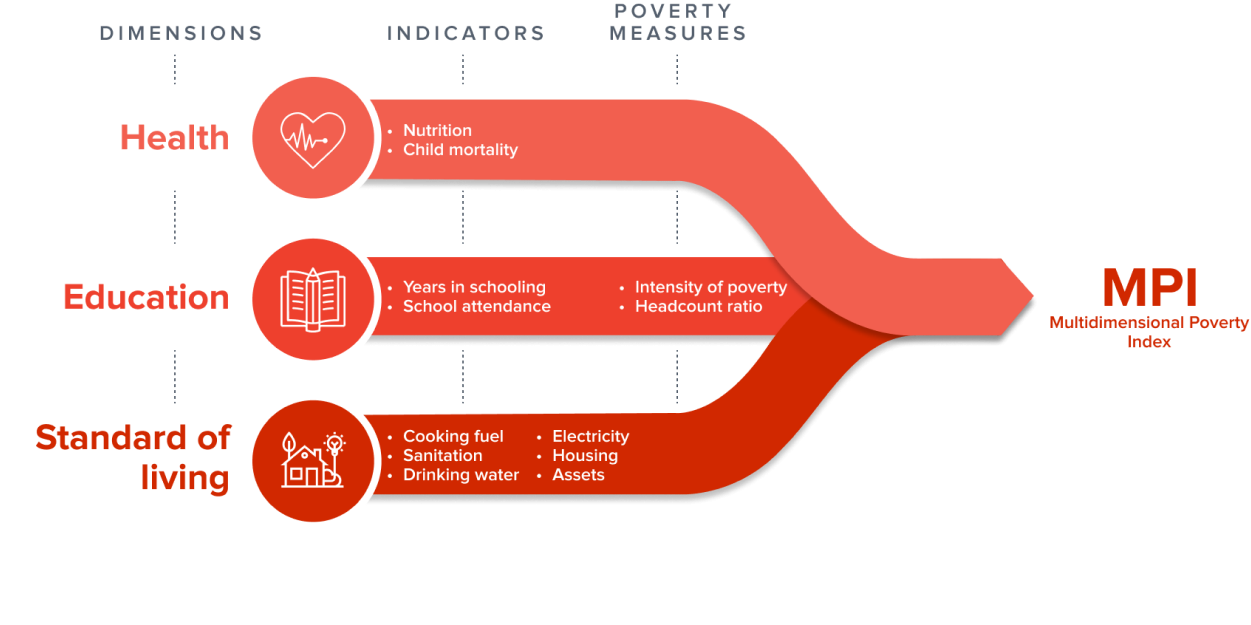
Annex: The dimensions, indicators, deprivation cutoffs, and weights of the global Multidimensional Poverty Index
1. Adults 19 to 70 years of age (229 to 840 months) are considered undernourished if their Body Mass Index (BMI) is below 18.5 kg/m2. Those 5 to 19 years (61 to 228 months) are identified as undernourished if their age-specific BMI values are below minus two standard deviations from the median of the reference population ( https://www.who.int/growthref/en/ ). In the majority of the countries, BMI-for-age covered people aged 15 to 19 years, as anthropometric data was only available for this age group; if other data were available, BMI-for-age was applied for all individuals 5 to 19 years. Children under 5 years (60 months and under) are considered undernourished if their z-score for either height-for-age (stunting) or weight-for-age (underweight) is below minus two standard deviations from the median of the reference population ( https://www.who.int/childgrowth/software/en/ ). Nutritional information is not provided for households without members eligible for measurement, these households are assumed to be not deprived in this indicator. 2. All reported deaths are used if the date of child’s death is not known. 3. Child mortality information is typically collected from women of reproductive ages 15-49 years. Households without women of such ages do not provide information about child’s deaths and are assumed to be not deprived in this indicator. 4. This country-specific age cutoff was introduced in 2020. Previously, the age cutoff was 10 years which did not recognize the fact that by age 10 children do not normally complete 6 years of schooling. 5. Source for official entrance age to primary school: United Nations Educational, Scientific and Cultural Organization, Institute for Statistics database. Education systems [UIS, http://data.uis.unesco.org/?ReportId=163 ]. 6. A household is considered to have access to improved sanitation if it has some type of flush toilet or latrine, or ventilated improved pit or composting toilet, provided that they are not shared. If the survey report uses other definitions of improved sanitation, we follow the survey report. 7. A household has access to improved drinking water if the water source is any of the following types: piped water, public tap, borehole or pump, protected well, protected spring or rainwater, and it is within 30 minutes’ walk (round trip). If the survey report uses other definitions of improved drinking water, we follow the survey report. 8. A few countries do not collect data on electricity because of 100% coverage. In such cases, we identify all households in the country as non-deprived in electricity. 9. A household is considered deprived if the dwelling’s floor is made of mud/clay/earth, sand or dung; or if the dwelling has no roof or walls or if either the roof or walls are constructed using natural materials such as cane, palm/trunks, sod/mud, dirt, grass/reeds, thatch, bamboo, sticks or rudimentary materials such as carton, plastic/ polythene sheeting, bamboo with mud/stone with mud, loosely packed stones, uncovered adobe, raw/reused wood, plywood, cardboard, unburnt brick or canvas/tent. 10. Television (TV) includes smart TV and black and white TV, telephone includes cell phones, computer includes tablets and laptops, and refrigerator includes freezers.
2019 MPI page
2020 MPI page
2021 MPI page
2022 MPI page
March 2023 global poverty update from the World Bank: the challenge of estimating poverty in the pandemic
Samuel kofi tetteh baah, r. andres castaneda aguilar, carolina diaz-bonilla, christoph lakner, minh cong nguyen, martha viveros.
Global poverty estimates were updated today on the Poverty and Inequality Platform (PIP) . This update includes new regional poverty aggregates in 2020 and 2021 for Latin America and the Caribbean, and in 2020 for Europe and Central Asia, and the group of advanced countries. These are the regions for which we now have sufficient survey data available during the COVID-19 pandemic. In total, 113 new country-years have been added, bringing the total number of surveys to more than 2,100.
This update also incorporates the usual changes to the input data, including revisions to existing welfare distributions, the inclusion of new welfare distributions, and revisions to price, national accounts, and population data used for global poverty monitoring (more details here ). Overall, these changes have resulted in minor revisions in global and regional poverty estimates.
Table 1 summarizes the revisions to the regional and global poverty estimates between the September 2022 data vintage and the March 2023 data vintage for the 2019 reference year at all three poverty lines. The global poverty headcount ratio at $2.15 is revised slightly up by 0.1 percentage points to 8.5 percent, resulting in a revision in the number of poor people from 648 to 659 million. This revision represents 11 million more people living in extreme poverty, largely driven by South Asia (5 million) and the Middle East and North Africa (4 million).
Table 1 Poverty estimates for reference year 2019, changes between September 2022 and March 2023 vintage by region and poverty lines
Similar limited changes in poverty estimates are observed at the higher lines of $3.65 and $6.85, which are typically used for measuring poverty in lower-middle- and upper-middle-income countries, respectively. At $3.65, the global poverty headcount ratio increases by 0.1 percentage points to 23.6 percent, representing 28 million more people living in poverty. At $6.85, the global poverty rate increases by 0.2 percentage points to 46.9 percent, representing 44 million people living in poverty. The upward revisions in poverty estimates at the higher lines are largely driven by South Asia and Sub-Saharan Africa.
This March 2023 global poverty update from the World Bank revises the previously published global and regional estimates from 1981 to 2019. Regional poverty estimates are now reported up to 2021, depending on sufficient data coverage over the period of the COVID-19 pandemic. Poverty data are reported for Europe and Central Asia until 2020, and Latin America and the Caribbean until 2021. For all other developing regions, poverty data are reported for pre-pandemic years (see Figure 1). More details are available here on how we have determined those regions for which to report post-2019 estimates.
Figure 1: Global and regional poverty estimates, 1990 - 2021
The data published in this PIP update, while incorporating more recent input data, do not change the overall perceptions about global poverty trends and the regional distribution of poverty. It is still the case that global poverty has been falling since the 1990s, and at a slower rate since 2014 ( World Bank 2022 ). Extreme poverty has been falling in all regions, except the Middle East and North Africa due to conflict and fragility ( World Bank 2020 ). Roughly 60% of the world’s extreme poor in 2019 lived in Sub-Saharan Africa alone, while 81% of the global poor at the poverty line of $3.65 lived in Sub-Saharan Africa or South Asia.
The authors gratefully acknowledge financial support from the UK Government through the Data and Evidence for Tackling Extreme Poverty (DEEP) Research Program.
- The World Region
Economist, Global Poverty and Inequality Data (GPID), Development Data Group, World Bank
Economist, Development Data Group, World Bank
Senior Economist, Poverty and Equity Global Practice, World Bank
Data Scientist
Program Manager, Development Data Group, World Bank
Senior Data Scientist, Poverty and Equity Global Practice, World Bank
Consultant, Development Data Group, World Bank
Join the Conversation
- Share on mail
- comments added

Search the United Nations
- Member States
Main Bodies
- Secretary-General
- Secretariat
- Emblem and Flag
- ICJ Statute
- Nobel Peace Prize
- Peace and Security
- Human Rights
- Humanitarian Aid
- Sustainable Development and Climate
- International Law
- Global Issues
- Official Languages
- Observances
- Events and News
- Get Involved
- Israel-Gaza
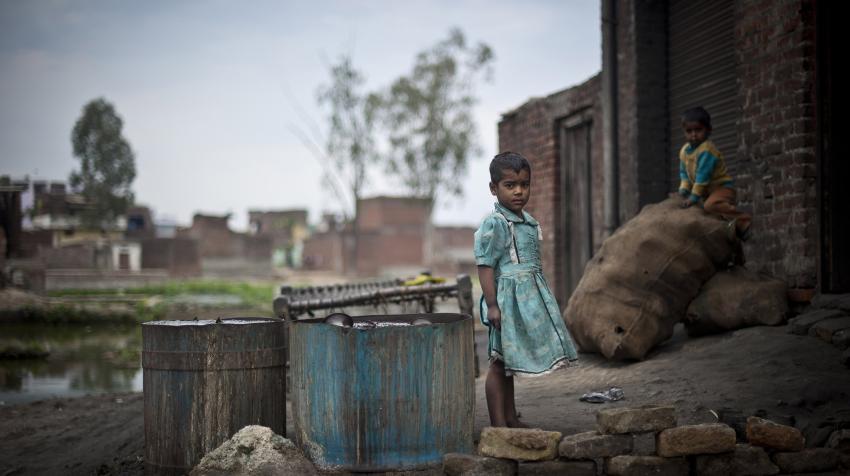
Ending Poverty
The COVID-19 pandemic has caused an increase in the number of people living in extreme poverty, for the first time in a generation. Progress in important areas, such as childhood vaccination and income equality between countries has been reversed, which has not happened in the past three decades. If the current trend continues, it is projected that by 2030, a shocking 575 million people will still be living in extreme poverty, and 84 million children will not be able to attend school. It is estimated that it will take almost 300 years to eliminate discriminatory laws, end child marriage and close gender gaps in legal protection. In 2020, with 71 million more people living in extreme poverty than the year before, the COVID-19 crisis caused the biggest setback in global poverty reduction in decades.
In 2020, with 71 million more people living in extreme poverty than the year before, the COVID-19 crisis caused the biggest setback in global poverty reduction in decades. In April 2020, the United Nations issued a framework for the immediate socio-economic response to COVID-19 and created the Secretary-General's UN COVID-19 Response and Recovery Fund .
From 1990 to 2014, the world made remarkable progress in reducing extreme poverty, with over one billion people moving out of that condition. The global poverty rate decreased by an average of 1.1 percentage points each year, from 37.8 percent to 11.2 percent in 2014. However, between 2014 and 2019, the pace of poverty reduction slowed to 0.6 percentage points per year, which is the slowest rate seen in the past three decades. Within the 24-year period, most of the poverty reduction was observed in East Asia and the Pacific, as well as South Asia.
What is Poverty?
Poverty entails more than the lack of income and productive resources to ensure sustainable livelihoods. Its manifestations include hunger and malnutrition, limited access to education and other basic services, social discrimination and exclusion, as well as the lack of participation in decision-making. In 2015, more than 736 million people lived below the international poverty line. Around 10 per cent of the world population (pre-pandemic) was living in extreme poverty and struggling to fulfil the most basic needs like health, education, and access to water and sanitation, to name a few. There were 122 women aged 25 to 34 living in poverty for every 100 men of the same age group, and more than 160 million children were at risk of continuing to live in extreme poverty by 2030.
Poverty facts and figures
- According to the most recent estimates, in 2023 almost 700 million people around the world were subsisting on less than $2.15.
- The share of the world’s workers living in extreme poverty fell by half over the last decade: from 14.3 per cent in 2010 to 7.1 per cent in 2019. However, in 2020 it rose for the first time in two decades after the COVID-19 pandemic.
- It is projected that the global goal of ending extreme poverty by 2030 will not be achieved , with almost 600 million people still living in extreme poverty.
- One out of six children lives in extreme poverty . Between 2013 and 2022, the number of children living on less than US$2.15 a day decreased from 383 million to 333 million, but the economic impact of COVID-19 led to three lost years of progress.
- In 2021, 53 per cent of the world’s population – 4.1 billion people – did not benefit from any form of social protection .
Poverty and the Sustainable Development Goals
Ending poverty in all its forms is the first of the 17 Sustainable Development Goals (SDGs) of the 2030 Agenda for Sustainable Development .
The SDGs’ main reference to combatting poverty is made in target 1.A : “Ensure significant mobilization of resources from a variety of sources, including through enhanced development cooperation, in order to provide adequate and predictable means for developing countries, in particular least developed countries, to implement programmes and policies to end poverty in all its dimensions.”
The SDGs also aim to create sound policy frameworks at national and regional levels, based on pro-poor and gender-sensitive development strategies to ensure that by 2030 all men and women have equal rights to economic resources, as well as access to basic services, ownership and control over land and other forms of property, inheritance, natural resources, appropriate new technology and financial services, including microfinance.
Measuring Poverty
There has been marked progress in reducing poverty over the past decades. In 2015, 10 per cent of the world’s population lived at or below $1.90 a day -down from 16 per cent in 2010 and 36 per cent in 1990- while in 2023 almost 700 million people around the world were subsisting on less than $2.15.
At current rates of progress, the world will likely not meet the global goal of ending extreme poverty by 2030 , with estimates indicating that nearly 600 million people will still be struggling with extreme poverty then.
Extreme poverty is concentrated in places where it will be hardest to eradicate— among the least developed countries, in conflict-affected areas, and in remote, rural areas. The outlook is also grim for the nearly 50 percent of the world’s population who live on less than $6.85 a day – the measure used for upper-middle-income countries.
Global Action
The 2030 Agenda for Sustainable Development promises to leave no one behind and to reach those furthest behind first. Meeting this ambitious development agenda requires visionary policies for sustainable, inclusive, sustained and equitable economic growth, supported by full employment and decent work for all, social integration, declining inequality, rising productivity and a favorable environment. In the 2030 Agenda, Goal 1 recognizes that ending poverty in all its forms everywhere is the greatest global challenge facing the world today and an indispensable requirement for sustainable development.
While progress in eradicating extreme poverty has been incremental and widespread, the persistence of poverty, including extreme poverty remains a major concern in Africa, the least developed countries, small island developing States, in some middle-income countries, and countries in situations of conflict and post-conflict countries. In light of these concerns, the General Assembly, at its seventy-second session, decided to proclaim the Third United Nations Decade for the Eradication of Poverty (2018–2027). The objective of the Third Decade is to maintain the momentum generated by the implementation of the Second United Nations Decade for the Eradication of Poverty (2008-2017) towards poverty eradication. Further, the 3rd Decade is also expected to support, in an efficient and coordinated manner, the internationally agreed development goals related to poverty eradication, including the Sustainable Development Goals.
Department of Economic and Social Affairs (DESA)
In 1995, the World Summit for Social Development held in Copenhagen, identified three core issues: poverty eradication, employment generation and social integration, in contributing to the creation of an international community that enables the building of secure, just, free and harmonious societies offering opportunities and higher standards of living for all.
Within the United Nations system , the Division for Social Policy and Development (DSPD) of the Department of Economic and Social Affairs (DESA) acts as Focal Point for the United Nations Decade for the Eradication of Poverty and undertakes activities that assist and facilitate governments in more effective implementation of the commitments and policies adopted in the Copenhagen Declaration on Social Development and the further initiatives on Social Development adopted at the 24th Special session of the General Assembly.
A potential game-changer in accelerating SDG progress
At the 2023 SDG Summit held at the UN’s headquarters in New York, the General Assembly adopted a political declaration to accelerate action to achieve the 17 Sustainable Development Goals (SDG). The document aims to drive economic prosperity and well-being for all people while protecting the environment. In addition, it includes a commitment to financing for developing countries and supports the proposal of an SDG Stimulus of at least $500 billion annually, as well as an effective debt-relief mechanism.
- International Day for the Eradication of Poverty
Through resolution 47/196 adopted on 22 December 1992, the General Assembly declared 17 October as the International Day for the Eradication of Poverty .
The observance of the International Day for the Eradication of Poverty can be traced back to 17 October 1987. On that day, over a hundred thousand people gathered at the Trocadéro in Paris, where the Universal Declaration of Human Rights was signed in 1948, to honour the victims of extreme poverty, violence and hunger. They proclaimed that poverty is a violation of human rights and affirmed the need to come together to ensure that these rights are respected. These convictions are inscribed on a commemorative stone unveiled that day. Since then, people of all backgrounds, beliefs and social origins have gathered every year on October 17th to renew their commitment and show their solidarity with the poor.
- UN Famine Prevention and Response Coordinator
- What We Do: Promote Sustainable Development
- Sustainable Development Goal 1: No Poverty
- Department of Economic and Social Affairs
- The 2022 Global Multidimensional Poverty Index (MPI)
- World Bank: Understanding Poverty
- International Movement ATD Fourth World
- Special Rapporteur on extreme poverty and human rights
- Child and Youth Safety Online
- Disarmament
Related Stories from the UN System

Read more about ending poverty.
- General Assembly
- Security Council
- Economic and Social Council
- Trusteeship Council
- International Court of Justice
Departments / Offices
- UN System Directory
- UN System Chart
- Global Leadership
- UN Information Centres
Resources / Services
- Emergency information
- Reporting Wrongdoing
- Guidelines for gender-inclusive language
- UN iLibrary
- UN Chronicle
- UN Yearbook
- Publications for sale
- Media Accreditation
- NGO accreditation at ECOSOC
- NGO accreditation at DGC
- Visitors’ services
- Procurement
- Internships
- Academic Impact
- UN Archives
- UN Audiovisual Library
- How to donate to the UN system
- Information on COVID-19 (Coronavirus)
- Africa Renewal
- Ten ways the UN makes a difference
- High-level summits 2023
Key Documents
- Universal Declaration of Human Rights
- Convention on the Rights of the Child
- Statute of the International Court of Justice
- Annual Report of the Secretary-General on the Work of the Organization
News and Media
- Press Releases
- Spokesperson
- Social Media
- The Essential UN
- Awake at Night podcast
Issues / Campaigns
- Sustainable Development Goals
- Our Common Agenda
- Summit of the Future
- Climate Action
- UN and Sustainability
- Action for Peacekeeping (A4P)
- Global Ceasefire
- Global Crisis Response Group
- Call to Action for Human Rights
- Disability Inclusion Strategy
- Fight Racism
- Hate Speech
- Safety of Journalists
- Rule of Law
- Action to Counter Terrorism
- Victims of Terrorism
- Children and Armed Conflict
- Violence Against Children (SRSG)
- Sexual Violence in Conflict
- Refugees and Migrants
- Action Agenda on Internal Displacement
- Spotlight Initiative
- Preventing Sexual Exploitation and Abuse
- Prevention of Genocide and the Responsibility to Protect
- The Rwanda Genocide
- The Holocaust
- The Question of Palestine
- The Transatlantic Slave Trade
- Decolonization
- Messengers of Peace
- Roadmap for Digital Cooperation
- Digital Financing Task Force
- Data Strategy
- Countering Disinformation
- UN75: 2020 and Beyond
- Women Rise for All
- Stop the Red Sea Catastrophe
- Black Sea Grain Initiative Joint Coordination Centre
- Türkiye-Syria Earthquake Response (Donate)
- Israel-Gaza Crisis
Thank you for visiting nature.com. You are using a browser version with limited support for CSS. To obtain the best experience, we recommend you use a more up to date browser (or turn off compatibility mode in Internet Explorer). In the meantime, to ensure continued support, we are displaying the site without styles and JavaScript.
- View all journals
- My Account Login
- Explore content
- About the journal
- Publish with us
- Sign up for alerts
- Open access
- Published: 17 April 2024
How popularising higher education affects economic growth and poverty alleviation: empirical evidence from 38 countries
- Jian Li ORCID: orcid.org/0000-0002-3228-8163 1 na1 ,
- Eryong Xue ORCID: orcid.org/0000-0002-7079-5027 2 na1 ,
- Yukai Wei ORCID: orcid.org/0000-0002-5202-7307 2 &
- Yunshu He ORCID: orcid.org/0000-0003-4814-9835 2
Humanities and Social Sciences Communications volume 11 , Article number: 520 ( 2024 ) Cite this article
870 Accesses
3 Altmetric
Metrics details
The popularisation of higher education supports UNESCO’s aim of developing inclusive and equitable quality education to achieve the fourth Sustainable Development Goal. However, the effect of popularising higher education on economic growth and poverty alleviation remains unexplored. Therefore, this study investigated the effects of higher education and adult education within populations (popularisation of higher education) on economic growth (gross domestic product; GDP) and the poverty line using panel data from 38 countries. OLS and quantile regression were performed using data for the period 1995–2021 extracted from the OECD and World Bank databases. The results showed that the population segments with higher education had a significantly positive impact on GDP growth. Moreover, an increased proportion of the population with higher education, of working age, was found to be a contributing factor to GDP growth. Popularising higher education also played a positive role during the initial stage of social and economic development. This study also highlighted that popularising higher education play a key role to influence a country’s educational development and scientific and technological innovation drives the deepening of a country’s economy. It suggested that both national and local governments worldwide should pay much attention to the popularisation degree of higher education to greatly improve the innovative ability of talents and scientific and technological innovation in higher education for both the economic growth and poverty alleviation.
Similar content being viewed by others
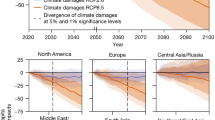
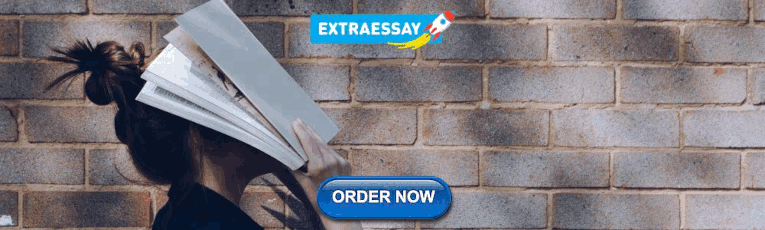
The economic commitment of climate change
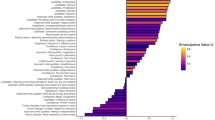
Worldwide divergence of values
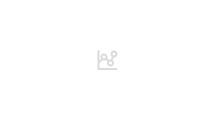
The impact of artificial intelligence on employment: the role of virtual agglomeration
Introduction.
The popularisation of higher education critically contributes to UNESCO’s efforts to realise the fourth Sustainable Development Goal of inclusive and equitable quality education (Ainscow, 2020 ; Bamberger and Kim, 2022 ).Popularisation of higher education expands the scale of higher education and its high growth rate introduces considerable challenges to the management structure of higher education, triggering a series of theoretical and practical concerns relating to the nature and function of higher education (Balestra and Ruiz, 2015 ; Brand, 2021 ). Given that education and social and economic development are mutually reinforcing, the expansion of higher education leads to an ascending spiral of development for individuals and/or economies. By contrast, a lack of education or early withdrawal from education leads to a downward spiral for them (Camilla, 2023 ). This relationship between education and development undergirds the model of poverty alleviation based on the return on education (Decancq, 2017 ). The previous studies emphasise the importance of the return on education as a multidimensional anti-poverty mechanism and thus a key factor in poverty alleviation (Fang et al., 2022 ; Chelli et al., 2022 ; Garritzmann, 2016 ). For example, return on education is the key factor enabling a transition from poverty alleviation through education to poverty alleviation through education (Gillian et al., 2021 ; Gong and Hong, 2021 ). Poverty alleviation is realised through an interlinking of these two processes and the promotion of the latter (Granata, 2022 ; Habibi and Zabardast, 2020 ). The educational resources can meet the needs of the poor mainly through the return on education at the levels of survival and life quality. In addition, the previous studies highlighted that, with a continuous expansion in the scale of higher education, its economic effect gradually appears to become marginal (Hoeller et al., 2014 ). The density of colleges and universities worldwide has increased considerably in recent years, but it is still inadequate to meet increasing demands resulting from the ongoing popularisation of higher education (Jericho, 2016 ). The increase in the number of colleges and universities has a positive effect in promoting economic development but with marginal benefits. (Julian, 2018 ).
Through reviewed the current relevant studies, it is found that there have limited studies that have simultaneously explored the effects of popularising higher education on economic growth and poverty alleviation. The previous research revealed that most studies have focused on the relations between popularisation of higher education and economic growth. However, a few empirical investigations have examined the effect of population segments with higher education and adult education (popularisation of higher education) on economic growth (GDP) and poverty reduction. Considering the scope and limitations of previous studies, it aimed to address the above research gap by investigating the effect of a population segment with high levels of higher education and adult education (popularisation of higher education) on economic growth (GDP) and the poverty line at a wide scale using panel data from 38 countries. The main research questions addressed in this study are as follows.
Q1: What is the effect of a population segment with higher education on GDP growth?
Q2: What is the effect of adult education on GDP growth?
Q3: What impact does a population segment with higher education have on reducing the proportion of those experiencing poverty?
Q4: What is the relation between an increased level of adult education and the proportion of the population experiencing poverty?
All these questions are relevant to an exploration of the effect of the population segment with higher education and adult education (popularisation of higher education) on economic growth (GDP) and the poverty line. This study is divided into several sections: the first section concentrates on examining the effect of popularising higher education on economic growth and the poverty line, the relationship between popularisation of higher education and poverty alleviation, and the relationship between popularisation of higher education and poverty alleviation. In the second section of method, to address this research gap, this study performed OLS and quantile regressions using data extracted from the OECD and World Bank databases for the period 1995–2021. An OLS regression model and a panel quantile model were used to analyse the effect of a population segment with higher education and adult education (popularisation of higher education) on economic growth (GDP) and the poverty line within 38 OECD countries. The impact of the proportion of people aged 24–64 years and 25–34 years who had completed higher education in relation to their peers on GDP and the proportion of people living in poverty in 38 OECD countries have been measured and analysed. The results and discussion have been provided at the last.
Literature review
The effect of popularising higher education on economic growth.
The population segment with higher education is regarded as an important contributor to economic growth, generating scientific knowledge and providing labour, which in turn increases human capital and productivity (Jungblut, 2017 ; Kalamova, 2020 ; Liu, 2017 ). As the scale of higher education expands, the emergence of knowledge power as a large-scale global phenomenon reflects the important role of an expanded educated labour force in the advancement of science and technology and the economy. For example, the relationship between higher education and economic development in European Union countries between 1997 and 2016 was analysed. Their findings revealed a statistically significant correlation between expanding higher education and economic growth in the selected countries. The one-way cause-and-effect relationship between education and economic development in these countries suggests that an increase in the proportion of the population enroled in higher education boosts economic performance. In addition, using a survey sample of 35 households, a retrospective study in Brazil, examined the role of educational expansion in reducing income inequality and poverty. Its findings suggest that it would take decades to reduce inequality and poverty in this country and that this outcome could only be achieved through a major expansion of the higher education sector. The growth needed to achieve this outcome would be considerable (Lamichhane et al., 2021 ). This reduction in inequality and poverty could only be achieved if optimistic assumptions about growth, matching job skills and the return on education do not fall short. In brief, education is not a panacea for reducing poverty and inequality. How three major stages of education contributed to the growth in labour productivity in 125 countries during the period 1999–2014 was also explored. They found that human capital is consistent with the educational returns of an average number of years of formal education at the levels of primary, secondary, and higher education. Their analysis showed that higher education had the greatest impact on labour productivity in the economies under study (Ledger et al., 2019 ). In addition, popularising higher education plays an important role in promoting economic growth, as the scale of higher education can guarantee the scale of human resources development by improving the quality of human resources and cultivating and distributing innovative scientific and technological talents. The scale of higher education guarantees the spread of science and technology and the popularisation of scientific and technological achievements (Mathias, 2023 ; Megyesiova and Lieskovska, 2018 ). The expanded scale of higher education worldwide has a spatial spillover effect on economic growth, which is strengthened through international cooperation in the fields of science and technology.
Popularising higher education also plays a direct role in cultivating and transporting scientific and technological talents to promote international scientific and technological cooperation (Mitic, 2018 ; Özdoğan Özbal, 2021 ; OECD, 2022 ; Pinheiro and Pillay, 2016 ). The scale of postgraduate education inhibited the total number of scientific and technological innovation achievements, indicating that there may be a trade-off between ‘quantity expansion’ and ‘quality upgrading’ of scientific and technological innovation achievements. Nevertheless, the positive effect on the number of high-tech innovation outcomes is significant, indicating that the supporting effect of graduate education on scientific and technological innovation is mainly concentrated in the high-tech fields (Pinheiro and Pillay, 2016 ; Rowe, 2019 ; Sahnoun and Abdennadher, 2022 ). The ‘talent increment’ of regional expansion and the ‘resource stock’ of graduate education have a combined promoting effect on high-tech innovation. There are differences in the effect of graduate education supporting high-tech innovation among provinces with different characteristics relating to the development of graduate education. The incremental expansion of high-quality talent is essential for enhancing the efficiency of material capital and stabilising the advantage of resource stocks. Using education statistics from OECD countries, Russia, and several other countries that participate in OECD education research, comparative and correlational analysis methods were applied to analyse how the scale of growth in young people’s participation in higher education is reflected in changes in their employment and economic activity. The results of their analysis showed that the growth in economic activity involving young graduates with a master’s degree exceeded that of college graduates after the 2009 financial crisis, and graduates fared better in the 2020 crisis, which was triggered by the COVID-19 pandemic.
The effect of popularisation of higher education on poverty alleviation
Popularisation of higher education is regarded as an essential factor contributing to poverty alleviation (Samo, 2022 ; Adams, 2013 ; Zapp, 2022 ). The higher education’s role in promoting economic growth can only be fully realised through the cultivation of talents suitable for the actual development situation of the country. Countries with food shortages, for example in Africa, also need to procure and train the right agricultural talent. Key drivers of sustainable agricultural production include access to improved technologies, sustainable growth of human, biological and natural resource capital, improvements in institutional performance and a favourable economic policy environment. Higher education graduates with the necessary ‘soft skills and business skills constitute an important pillar. Chakeredza ( 2008 ), who explored the effect of popularising higher education on poverty alleviation, suggested that the number of hungry people in Africa will continue to increase. Higher education in agriculture must be transformed, and efforts must focus on retaining faculty and on reviewing and redesigning institutional management systems, curriculum content and education delivery.
There are many reasons for poverty, with a lack of education being an important one. Insufficient quality education leads to educational poverty. Using PISA data, Agasisti et al. ( 2021 ) investigated the extent of educational poverty in European countries, considering its incidence, breadth, depth and severity. For this study, they adopted an additive multidimensional poverty measure proposed by Alkirew and Foster. Their findings indicated that between 2006 and 2015, the depth and severity of poverty decreased in most of the countries under study. Moreover, the incidence of educational poverty in many European countries was related mainly to student characteristics and school factors. The expansion of higher education has a positive effect on economic development and poverty reduction by improving work skills within the labour force. Increased enrolment in higher education encourages individuals born in families with low education levels to avail of higher education opportunities. Evidently, the expanded scale of higher education in the process of promoting economic growth has enhanced the equity effect of intergenerational social mobility. The expansion of higher education improves total factor productivity, thus promoting economic transformation and advancement globally (Samo, 2022 ; Adams, 2013 ; Zapp, 2022 ). Furthermore, the previous studies have shown that the structure of higher education talent training has a significant impact on economic development. Therefore, government departments need to make constant efforts to improve relevant systems and promote the optimisation and upgrading of the structure of higher education talent training to meet the needs of future economic development.
Theoretical underpinnings
The relationship between education and economic growth is a classic issue in the study of educational economics. For example, in Solow’s view, the growth of per capita output comes from per capita capital stock and technological progress, but capital investment has the problem of diminishing marginal returns, and the long-term sustainable development of the economy depends on technological progress (Solow, 1957 ). The emphasis on technological progress is a very important point in Solow’s growth theory. It was Schultz who systematically analyzed the contribution of education to economic growth. Influenced by the progress of economic growth theory and national accounting methods, Schulz proposed human capital theory in the process of explaining Solow residuals (Schultz, 1961 ). believes that once human capital is included in economic growth, it will solve the paradoxes and puzzles faced in economic growth research. Starting with the difference in income of different types of workers in the labour market, he found that education and health factors are the main reasons for the income difference, and further clarified that the reason for the income difference is the difference in labor productivity (Schultz, 1961 ). Schultz ( 1961 ) believes that human resources include the quantity and quality of labor, and he mainly focuses on the skills and knowledge of people who can improve labor productivity. As for how to measure human capital investment, Schulz believes that the cost of human capital can be measured in the same way as physical capital. Lucas ( 1988 ) focuses on the mechanism of human capital accumulation and why human capital does not show diminishing marginal returns like physical capital. Lucas divides the effect of human capital into internal effect and external effect. Romer ( 1990 ) internalised technological progress, revealed the relationship between human capital and technological progress, and proposed that the stock of human capital determines the economic growth rate, and it is human capital rather than population that determines economic growth. Romer starts with three hypotheses: first, technological progress is central to long-term economic growth; Second, technological progress is formed by people’s response to market incentives, and market incentives determine technological progress. Third, technology is a special kind of product, and once the cost of the initial input is produced, the technology can be reproduced indefinitely at no cost or very low cost.
In other words, higher education is more about improving students’ ability and productivity, thereby increasing students’ income, and promoting economic growth. Higher education mainly affects economic growth through two aspects: one is the same as Schulz’s improvement of individual ability, and the internal effect of human capital, which directly affects the production process (Schultz, 1961 ). Second, Lucas emphasised the external effect of human capital, and the comprehensive effect of human capital on the whole society, which has the characteristics of increasing marginal benefit (Lucas, 1988 ). It emphasises that the human capital invested in technological innovation and the existing knowledge and technology stock of the whole society jointly determine technological innovation.
Research hypotheses and analytical model
In this study, an OLS regression model and a panel quantile model were used to analyse the effect of a population segment with higher education and adult education (popularisation of higher education) on economic growth (GDP) and the poverty line within 38 OECD countries. The study’s hypotheses were as follows:
Hypothesis 1: The effect of a population segment with higher education has a positive impact on GDP growth.
Hypothesis 2: Some level of adult education has a positive impact on GDP growth.
Hypothesis 3: A population segment with higher education has a positive impact by reducing the proportion of the population experiencing poverty.
Hypothesis 4: An increase in the level of adult education has a positive impact by reducing the proportion of the population experiencing poverty.
The widely used Mankiw-Romer-Weil model was applied in this study. The overall level of development of higher education and the popularisation of higher education were considered core elements that independently promote economic development and alleviate poverty. The following model was constructed by incorporating the variable of quality higher education into the Solow model:
where Y it refers to the output of i country in t year. The independent variables Qit and P it respectively represent the scale of development and the degree of popularisation of higher education in i country in t year. The following specific model was constructed:
The independent variables were the proportion of people aged 25–64 years with higher education (A) and the proportion of people aged 25–34 years with higher education within the same age group (B). The first variable reflects the population segment that has completed higher education and can work in the corresponding age group. The second reflects the degree of popularisation of higher education. The proportion of those who have completed higher education in relation to their peers is in the normal state, which can reflect the enrolment rate for the previous process of higher education, thus indicating the degree of popularisation of higher education.
The dependent variables were GDP and the poverty line (D). GDP is a measure the overall level of a country’s economic and social development. The poverty line refers to the proportion of people living on less than US$1.25 a day as a percentage of the country’s total population or the proportion of people living in poverty. Thus, it reflects the level of equity in social development. The figure of US$2.15 is used in the World Bank’s index and is based on the purchasing power parity in 2017 (see Table 1 ).
Data sources and selection of variables
This study measured the impact of the proportion of people aged 24–64 years and 25–34 years who had completed higher education in relation to their peers on GDP and the proportion of people living in poverty in 28 OECD countries. Specifically, this study assessed the impact of the overall level of development of higher education and the degree of its popularisation (the breadth of development of higher education) on GDP (the height of development of economic and social development) and the poverty line (the breadth of development of economic and social development). Data were sourced from the OECD database and the World Bank website covering the period 1995–2021. This study selected 38 OECD countries for this study: the United States, UK, France, Germany, Italy, Canada, Ireland, the Netherlands, Belgium, Luxembourg, Austria, Switzerland, Norway, Iceland, Denmark, Sweden, Spain, Portugal, Greece, Turkey, Japan, Finland, Australia, New Zealand, Mexico, the Czech Republic, Hungary, Poland, South Korea, Slovakia, Chile, Slovenia, Estonia, Israel, Latvia, Lithuania Colombia and Costa Rica. Figure 1 shows the distribution of the 38 OECD countries. Of these countries, 20 were founding members of the OECD when it was established in 1961, while the remaining 18 subsequently became members. After 1994, OECD membership expanded rapidly. Five new members were added within three years. OECD then entered a period of accelerated development, and its operations and advancement reached an optimal stage. Therefore, this study selected data from the OECD database and the World Bank website covering the period 1995–2021 to explore the relationship between higher education and economic and social development in OECD member countries.
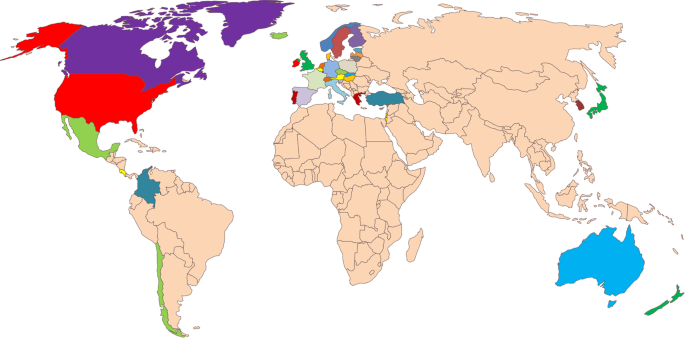
It expresses the geographical relations of the Atlantic region and simplifies the latitude and longitude lines and country symbols, highlighting the geographical distribution by highlighting OECD countries in color and other countries in apricot color.
The impact of the population segment with higher education on GDP growth
This study explored the impact of the population segment with higher education on GDP, taking the proportion of people aged 25–34 years who had completed higher education (B) and the proportion of people aged 25–64 years who had completed higher education (A) as the independent variables for the OLS regression. The square value of model R was 0.097, indicating that the two independent variables could explain 9.73% of the change in GDP. The model passed an F test ( F = 46.137, p = 0.000 < 0.05), indicating that at least one of the two independent variables impacted the GDP regression coefficient (C). The following formula was used:
The final analysis revealed that the regression coefficient value of A was 1.553 and the significance level was 0.01 ( t = 7.141, p = 0.000 < 0.01). Therefore, A had a significantly positive influence on C. Accordingly, the proportion of the population aged 25–64 years who had completed higher education, that is, the overall level of development of higher education was found to have a positive impact on GDP. The influence coefficient value was 1.533, indicating that an increase in the proportion of the population with completed higher education led to an increase in GDP.
The regression coefficient value of B was −0.813 at a 0.01 level of significance ( t = −4.300, p = 0.000 < 0.01), indicating that B had a significantly negative influence on C. The proportion of the population aged 25–34 years who had completed higher education, that is, the degree of popularisation of higher education had a negative effect on GDP, and the influence coefficient value was −0.813.
The negative impact on economic and social development caused by an increase in the popularity of higher education and the proportion of young people’s higher education experience may be attributed to the excess capacity of higher education. The development of higher education should be adapted to the national context. An excess of higher education and a lack of investment lead to a rise in the social cost of education and a decline in social outputs, which hinder social and economic development. At the same time, young people aged between 25 and 34 years occupy the position of’ export’ in the education process. With the increasing popularity of higher education, the supply of talents in the labour market generated through the recruitment of former higher education exceeds the demand for graduates with higher education within recruiting organisations. Consequently, issues such as wasted educational resources and knowledge, unemployment, excessive education, excess talents, an imbalance in the structure of higher education, excessive expansion and decreasing compatibility undermine economic operations and hinder GDP growth.
In this study, the variance decomposition and Pearson coefficient based on covariance calculation were analyzed. The variable of the number of 25–34-year-old who have completed higher education as a percentage of their peers explains 50.74% of the change in GDP. The variable of the proportion of 25–64-year-old who have completed higher education explains 49.26% of the change in GDP. The variable of 25- to 34-year-olds who completed higher education as a percentage of their peers explained 45.88% of the change in poverty line. The variable of the proportion of people aged 25–64 who have completed higher education explains 54.12% of the change in GDP (See Table 2 ).
The proportion of people aged 25–34 who have completed higher education in their peers and the proportion of people aged 25–64 who have completed higher education in their peers, GDP and poverty line showed significant correlation coefficients. The correlation between the proportion of people who have completed higher education at the age of 25–34 and the proportion of people who have completed higher education at the age of 25–64 is 0.931, and shows a significance of 0.01, which indicates that there is a significant positive correlation between the proportion of people who have completed higher education at the age of 25–34 and the proportion of people who have completed higher education at the age of 25–64. The correlation between the proportion of the number of people who have completed higher education at the age of 25–34 and the GDP is 0.209, and the significance is 0.01, which indicates that there is a significant positive correlation between the number of people who have completed higher education at the age of 25–34 and the GDP. The correlation between the number of people who have completed higher education and the poverty line at the age of 25–34 is −0.365, with a significance of 0.01, indicating a significant negative correlation between the number of people who have completed higher education and the poverty line at the age of 25–34 (See Table 2 ).
White test and BP test were used in this study. The test null hypothesis is that the model has no heteroscedasticity. The table above shows that both tests reject the null hypothesis ( p < 0.05), indicating that the model does have heteroscedasticity. When there is a heteroscedasticity problem, Robust and robust standard false regression is used (See Table 3 ).
The impact of a population segment with higher education on the poverty line
This study also explored the impact of a population segment with higher education on the poverty line. Specifically, this study performed an OLS regression in which the proportion of people aged 25–34 years who had completed higher education (B) and the proportion of those aged 25–64 years who had completed higher education (A) were the independent variables. As Table 2 shows, the R squared value was 0.134. This means that variables A and B could explain 13.37% of the change in the poverty line (D). The model passed the F test ( F = 48.771, p = 0.000 < 0.05), which means that at least one variable (A or B) had an impact on the poverty line. The formula for the change in the poverty line was expressed as follows:
The final analysis revealed that the regression coefficient value of the proportion of people aged 25–64 years who had completed higher education (A) was 0.005 but with no significance ( t = 0.428, p = 0.669 > 0.05), indicating that the population segment with higher education did not have an impact on the poverty line.
The regression coefficient value of the proportion of people aged 25–34 years who had completed higher education (B) was −0.048 at a significance level of 0.01 ( t = −4.305, p = 0.000 < 0.01), which means that in relation to their peers, the proportion of people aged 25–34 years who had completed higher education had a significantly negative impact on the proportion of poor people. A higher proportion of people aged 25–34-years who had completed higher education corresponded to a higher penetration rate of higher education and a lower proportion of those living in poverty. This phenomenon can be attributed to OECD’s support for the development of higher education in various countries. When the development of higher education reaches a certain level, the reduction of the proportion of the population segment experiencing poverty will no longer be affected by a simple expansion of the scale of extended higher education and the superposition of the total number of highly educated human resources. It will be influenced more by the reasonable distribution of educational resources and educational equity within higher education and its popularisation, that is, the increase in the proportion of the school-aged population aged 25–34 years based on the increase of the previous enrolment rate (see Table 4 ).
The effect of adult education on GDP growth
For quantile regression analysis, a total of nine models (with decimal points ranging from 0.10 to 0.90 and at intervals of 0.10) were estimated in this analysis, which aimed to explore the impact of the independent variables A and B on the dependent variable, GDP (C). When the quantile value was between 0.1 and 0.3, the proportion of the population aged 25–64 years who had completed higher education (A) had no significant positive impact on GDP growth, indicating that the development of higher education did not significantly affect economic and social development in poorer OECD countries. When the quantile value was between 0.4 and 0.6, the level of development of higher education had a significantly negative impact on economic and social development. Thus, for a country that had developed over a period, the advancement of higher education required multiple inputs, such as capital, material, and human resources.
During the early stage of the development of higher education, such inputs may, however, have a negative and weakening impact on social and economic development. The added cost of education and the lag between the output of educational achievements and the input of talents puts increased pressure on economic and social development during a certain period. When the quantile value was 0.7 or higher, the improvement of the overall level of higher education had a significantly positive impact on GDP growth, indicating the realisation of the talent training outcomes of higher education. Teaching and research outcomes were thus transformed into socially productive resources and power, with talents with higher education contributing to economic and social development.
When the quantile value was 0.1, the proportion of people aged 25–34 years who had completed higher education in relation to their peers (variable B), indicating the popularisation of higher education, had no significant impact on GDP growth. Thus, in extremely backward countries, the popularisation of higher education had little effect on economic and social development. When the quantile value ranged between 0.2 and 0.6, the popularisation of higher education had a significantly positive effect on GDP growth, indicating its contribution to economic growth.
When the quantile value was 0.7, the influence of variable B on variable C was no longer significant, indicating that social development would soon face the problem of overcapacity in higher education. When it exceeded 0.7, the ratio of eligible people aged 25–34 years who had completed higher education in relation to their peers had a significantly negative impact on GDP growth, revealing that with the development of the economy, society and education, higher education had become overexpanded. Thus, the cost of investing in education exceeded the social benefits, leading to overcapacity whereby the supply of higher education talents exceeded the demand. This situation led to wasted educational resources and excessive competition of talents, hindering economic growth (See Table 5 ).
The increased level of adult education and the proportion of the population experiencing poverty
Using the same model, this study explored the influence of the independent variables, A and B, on the poverty line (dependent variable D). The proportion of the population aged 25–64 years who had completed higher education (independent variable A) had no significant influence on the proportion of the population living in poverty, indicating that popularisation of education and economic and social development have been achieved to a certain extent in OECD countries, and improvements targeting the population experiencing poverty could no longer be achieved simply by increasing the volume and quantity of higher education. When the quantile value was 0.1, the proportion of people aged 25–34 years who had completed higher education in relation to their peers (independent variable B) had no significant effect on the proportion of the population experiencing poverty (dependent variable D). Therefore, the strategy of increasing higher education enrolment and the ratio of the eligible population through the fair allocation of educational resources, and thus the popularisation of education, would not be effective for a small population segment experiencing poverty. In other words, the population segment experiencing poverty in highly developed countries is less receptive to the popularisation of higher education. When the quantile value was 0.2, the independent variable, B, had a significantly positive impact on the dependent variable D, that is, an increase in the popularity of higher education led to an increase in the population segment experiencing poverty. This phenomenon can be interpreted as reflecting the inherent disadvantages of the welfare state in the field of education. A rise in the number of eligible young people aged 25–34 years who have completed higher education reflects the development trend of higher education towards fairness and popularisation following the redistribution of higher education resources.
The fair distribution of higher education resources leads to a lack of competition in the areas of teaching and career development. To a certain extent, reducing students’ willingness and enthusiasm to work may lead to poverty caused by the failure to achieve teaching results. When the quantile value was between 0.3 and 0.4, the independent variable, B, had no significant influence on the dependent variable D. In relatively poor countries, the popularisation of higher education contributes little to reducing the degree of poverty, so it may be necessary to explore ways of alleviating poverty from the perspective of improving the overall level and expanding the scale of basic higher education. When the quantile value was 0.5 or above, the independent variable B had a significantly negative impact on the dependent variable D, indicating that for countries with a relatively high proportion of their population experiencing poverty, the following strategy would be more effective.
Considering the quantile data, this study deemed that the degree of sensitivity of countries at different stages of economic development to the level of development and popularisation of higher education could be more intuitively evaluated using a radar map (see Fig. 2 ). Countries with sub-points 0.1–0.9 were defined along a spectrum as extremely backward, backward, moderately backward, slightly backward, moderate, preliminarily developed, moderately developed, developed, and highly developed. From the perspective of economic development, increasing the proportion of young people who complete higher education and popularising higher education had an obvious positive effect in backward and medium-developed countries, whereas the effect in highly developed countries was not obvious. Overall, the sensitivity of OECD countries to the high level of education penetration was found to be higher than the level of development of higher education. From the perspective of equitable economic development, the overall level of development of higher education had no significant impact on the poverty link in OECD countries, whereas OECD countries with differing economic development backgrounds and at varying stages of development evidenced relatively significant and stable sensitivity to the proportion of young and middle-aged people who completed higher education and the popularisation of higher education.
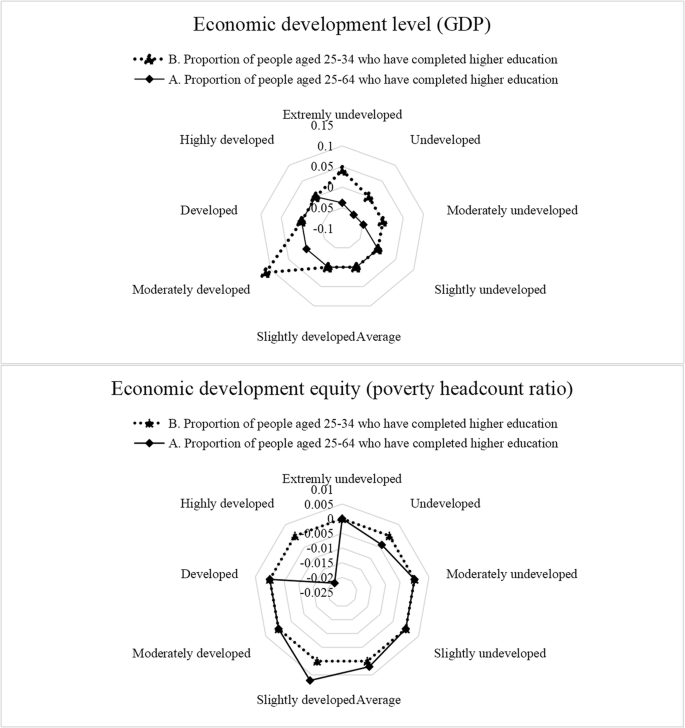
The dashed line represents the proportion of people aged 25–34 years who have completed higher education. The solid line represents the proportion of people aged 25–64 years who have completed higher education, the impact of the overall level of higher education.
Our findings indicated that population segments with higher education had a significantly positive impact on GDP growth in 38 OECD countries. An increase in the proportion of the population segment of working age who completed higher education was found to contribute to GDP growth. Moreover, an improvement in the popularity of higher education played a positive role during the initial stage of economic and social development.
At the same time, oversupply and overcapacity may result from a continuous improvement of higher education. A very large number of young people who have completed higher education can lead to excessive competition and wasted academic qualifications (Mathias, 2023 ; Megyesiova and Lieskovska, 2018 ). In turn, higher education knowledge unemployment, overinvestment, a structural imbalance, disorderly expansion and wasted resources can occur, which have detrimental impacts on economic operations.
Some studies have shown that strengthening the quality of higher education helps to improve cognitive abilities within the labour force, thereby enhancing the growth of the knowledge economy (Ainscow, 2020 ; Bamberger and Kim, 2022 ). Other studies have reported regional heterogeneity relating to the marginal effect of improving the quality of higher education on economic growth. Some scholars have analysed the influence of the quality of higher education on economic development from the perspective of human capital investment theory. Their findings indicate that the quality of higher education determines the composition and growth trend of social human capital. Because of differences in the degrees of development of different economies, the quality of higher education has a phased influence on economic growth (Balestra and Ruiz, 2015 ; Brand, 2021 ). Case studies of African developing countries by international scholars have revealed that quality factors are key to realising the economic development function of higher education. From the perspectives of both efficient financial investments by states in education poverty alleviation and the effects of economic, time and emotional investments of poor families and individuals in education poverty alleviation, it is necessary to take the return on education into consideration. Moreover, it is important to respond to reasonable concerns regarding the return on education for poor people and to strengthen their cognitive capacities to rationalise as well as their expectations regarding returns on education (Li et al., 2023 ). In this way, the intention to participate and behaviour of anti-poverty education will be generated, and the strategic upgrading of poverty alleviation combined with the promotion of aspirations and cognitive capacities will be emphasised.
Implications
Our use of panel data from 38 countries to deepen understanding of the effect of popularising higher education on economic growth and poverty reduction also has practical implications. The economic, social, and higher education undertakings in OECD countries evidence a certain level of development. The population segment with higher education has no significant impact on reducing the proportion of the population segment experiencing poverty. Simply increasing the proportion of people who complete higher education and expanding the scale of higher education will not effectively reduce poverty (Li and Xue, 2021 ). Providing more educational opportunities to poor people through the slanting of educational resources can help to reduce the proportion of poor people (Ainscow, 2020 ; Bamberger and Kim, 2022 ). For example, popularising higher education plays a key role to influence a country’s development level and scientific and technological innovation drives the deepening of a country’s economy (Bamberger and Kim, 2022 ). Technological progress is the core of economic growth, scientific and technological innovation brings technological change and development in all aspects, human capital promotes economic growth, and higher education trains talents and improves the capital attribute of human (Camilla, 2023 ). For endogenous economic growth theory, the economy does not rely on external forces to achieve sustained growth, and endogenous technological progress determines sustained economic growth. Popularising higher education worldwide brings the accumulation of human capital, improves the quality of workers, and scientific and technological innovation makes technological progress and high-quality economic development, practically. Human capital accumulation is also the process of continuous input of labour force, which covers the accumulation of human capital by labour force factors in formal education, training, and other learning processes. From the perspective of human capital, popularising higher education is the most direct and efficient way to promote the accumulation of human capital and improve the quality of labour force (Balestra and Ruiz, 2015 ; Brand, 2021 ). The popularisation degree of higher education is one of the important indicators to measure the development level of a country’s economic, and it is also the common trend of the development of higher education in all countries after World War II. In this transitional era, how to continue the achievements of higher education in the popular era and solve the existing problems as soon as possible is the heavy responsibility of our times. Therefore, at the initial stage of popularisation of higher education, it is necessary to re-examine the process of higher education popularisation globally and explore the internal logics between the popularisation of higher education and Sustainable Development Goal of inclusive and equitable quality education (Ainscow, 2020 ; Bamberger and Kim, 2022 ).
For policy suggestions, this study suggests that both national and local governments worldwide should pay much attention to the popularisation degree of higher education to greatly improve the innovative ability of talents and scientific and technological innovation in higher education. For example, they could promote scientific and technological innovation in an organised manner to serve national and regional economic and social development. Faced with the current situation in which global higher education has entered a stage of popularisation and new challenges and problems in serving regional economic and social development, national governments should continue to optimise the distribution and structure of higher education resources to support different regions, focusing on the major strategy of enhancing national competitiveness, serving economic and social development, and promoting common prosperity.
Contributions
This study novelty contributes on examining how popularising higher education affects economic growth and poverty alleviation, conceptually, methodologically, and practically. For instance, this study focuses on epitomising the conceptual and analytical model to explore the effects of higher education and adult education within populations (popularisation of higher education) on economic growth (gross domestic product; GDP) and the poverty line. In addition, this study novelty combines both Mankiw-Romer-Weil model Solow model to investigate the effects of higher education and adult education within populations on economic growth and the poverty through OLS regression model and quantile model. For the practical aspect, this study practically uncovers the implicit significance of the popularisation of higher education for advocating UNESCO’s aim of developing inclusive and equitable quality education to achieve the fourth Sustainable Development Goal.
Limitations
This study had some limitations. Data could have been collected from a larger sample of OECD countries to explore the effect of population segments with higher education and adult education (popularisation of higher education) on economic growth (GDP) and the poverty line. In addition, a qualitative component could be included in future studies to uncover the cultural and historical contexts of the effect of popularising higher education on economic growth and poverty reduction at the local level. Future studies should also investigate the causal relationship between the popularisation of higher education and economic growth. Additional empirical data and advanced research methods can be used to enable a shift from correlation to causality.
In conclusion, this study examined the effect of the population segment with higher education and adult education (popularisation of higher education) on economic growth (GDP) and the poverty line using panel data from 38 countries. The population segment with higher education was found to have a significant positive impact on promoting GDP growth. An increase in the proportion of the working-age population segment that had completed higher education was evidently conducive to GDP growth. Popularisation of higher education was also found to play a positive role in the initial stage of economic and social development.
Data availability
The data of OECD country GDP is retrieved from https://data.worldbank.org/indicator/NY.GDP.MKTP.CD?locations=1W , The data of OECD country poverty line is retrieved from https://data.worldbank.org/indicator/SI.POV.DDAY?locations=1W&start=1984&view=chart , The data of OECD country Population with tertiary education 25–34-year-old is retrieved from https://data.oecd.org/eduatt/population-with-tertiary-education.htm#indicator-chart , The data of OECD country Percentage of 25–64-year old’s who have completed higher education (%) is retrieved from https://data.oecd.org/eduatt/adult-education-level.htm#indicator-chart , The datasets generated during and/or analysed during the current study are available in Harvard Dataverse https://doi.org/10.7910/DVN/TP43QS .
Adams, R (2013). Education: poverty and inequality blamed as UK sinks in adult literacy league: OECD survey flags up big problems for England: Post-16 education blamed for failure to address issue. The Guardian . (2013, October 9). London, England
Agasisti T, Longobardi S, Prete V, Russo F (2021) The relevance of educational poverty in europe: determinants and remedies. J Policy Model 43(3):692–709. https://doi.org/10.1016/j.jpolmod.2020.03.015
Article Google Scholar
Ainscow M (2020) Promoting inclusion and equity in education: lessons from international experiences. Nord J Stud Educ Policy 6(1):7–16
Balestra C, Ruiz N (2015) Scale-invariant measurement of inequality and welfare in ordinal achievements: an application to subjective well-being and education in OECD Countries. Soc Indic Res 123(2):479–500. https://doi.org/10.1007/s11205-014-0751-2
Bamberger, A, & Kim, MJ (2022) The OECD’s influence on national higher education policies: internationalisation in Israel and South Korea. Comp Educ 18. https://doi.org/10.1080/03050068.2022.2147635
Brand M (2021) The OECD poverty rate: lessons from the russian case. Glob Soc Policy 21(1):144–147
Camilla S. (2023) The design of upper secondary education across OECD countries: Managing choice, coherence and specialisation. OECD Education Working Papers. https://doi.org/10.1787/158101f0-en
Chakeredza S, Temu A, Saka J, Munthali D, Muir-Leresche K, Akinnifesi F, Ajayi O, Sileshi G (2008) Tailoring Tertiary Agricultural Education for Sustainable Development in Sub-Saharan Africa: Opportunities and Challenges. J Sci Res Essay 3(8):326–332
Google Scholar
Chelli F, Ciommi M, Mariani F, Polinesi G, Recchioni MC, Lamonica GR, Salvati L (2022) A story of strengths and weaknesses in tertiary education: evaluating “Mobility” and “Opportunities” in OECD countries with composite indicators. Sustainability 14(24):16463. https://doi.org/10.3390/su142416463
Decancq, K (2017) Measuring multidimensional inequality in the OECD member countries with a distribution-sensitive better life index. Soc Indic Res 131(3):1057–1086. https://doi.org/10.1007/s11205-016-1289-2
Fang W, Farooq U, Liu Z, Lan J, Iram R (2022) Measuring energy efficiency financing: a way forward for reducing energy poverty through financial inclusion in OECD. Environ Sci Pollut Res 29(47):71923. https://doi.org/10.1007/s11356-022-20139-8
Garritzmann, JL (2016) The political economy of higher education finance: the politics of tuition fees and subsidies in OECD Countries, 1945–2015. Garritzmann. Germany, Berlin: Springer International Publishing
Gillian G, Lisa T, & Thomas W (2021) How are higher education systems in OECD countries resourced?: Evidence from an OECD Policy Survey.OCDE. https://doi.org/10.1787/0ac1fbad-en
Gong HJ, Hong JE (2021) Does postsecondary education attainment matter in community service engagement? Evidence from Across 18 OECD Countries. Educ Sci 11(3):1–17. https://doi.org/10.3390/educsci11030096
Article MathSciNet Google Scholar
Granata, M (2022) The OECD and technical education in post-war Mediterranean Europe. Labor History. https://doi.org/10.1080/0023656X.2022.2057459
Habibi, F & Zabardast, MA (2020) Digitalization, education and economic growth: a comparative analysis of Middle East and OECD countries. Technol Soc 63. https://doi.org/10.1016/j.techsoc.2020.101370
Hoeller P, Joumard I, Koske I (2014) Reducing income inequality while boosting economic growth: can it be done? Evidence from OECD Countries. Singap Econ Rev 59(1):1–22
Jericho, G (2016) Australia didn’t have a ‘great recession’? Tell that to young people; an OECD report has found a rise in youth not in employment, education or training (Neets) since 2008. While historically the rate isn’t high, benefits are so low that youth are now more likely to be living in poverty. Guardian. London, England. https://link.gale.com/apps/doc/A463552813/AONE?u=unimelb&sid=bookmark-AONE&xid=701996a9
Julian LG (2018) The political economy of higher education finance. the politics of tuition fees and subsidies in OECD Countries, 1945–2015. Czech Sociol Rev 54(3):479–482
Jungblut J (2017) The political economy of higher education finance. The politics of tuition fees and subsidies in OECD countries, 1945–2015. Eur J High Educ 7(1):96–99. https://doi.org/10.1080/21568235.2017.1265789
Kalamova, M (2020) Resourcing higher education: challenges, choices and consequences. Higher Education Policy Team. Paris: OECD Publishing
Lamichhane, S, Egilmez, G, Gedik, R, Bhutta, MKS, & Erenay, B (2021) Benchmarking OECD countries’ sustainable development performance: a goal-specific principal component analysis approach. J Clea Prod 287. https://doi.org/10.1016/j.jclepro.2020.125040
Ledger, S, Thier, M, Bailey, L, & Pitts, C (2019) OECD’s approach to measuring global competency: powerful voices shaping education. Teachers College Record, 121(8):1–40
Li, J & Xue. E (2021) Returnee faculty responses to internationalizing “Academic Ecology” for Creating World-class Universities in China’ Elite Universities. Higher Education. 81(5), 1063–1078
Li, J, & Xue. E, Liu, C, Li, X (2023) Integrated macro and micro analyses of student burden reduction policies in China: call for a collaborative “family–school–society” model. Humanities & Social Sciences Communications. 10, 184 (2023). https://doi.org/10.1057/s41599-023-01695-x
Liu, L (2017) Exploring the relationship between education economy and individual civic attitudes: a cross-national analysis in England, Spain, Sweden, Poland and Chinese Taipei
Lucas R (1988) On the mechanics of economic development. J. Monetary Econ 22(1):342
Mathias B (2023). The assessment of students’ creative and critical thinking skills in higher education across OECD countries: a review of policies and related practices. OECD Education Working Papers. https://doi.org/10.1787/35dbd439-en
Megyesiova, S, & Lieskovska, V (2018) Analysis of the sustainable development indicators in the OECD Countries. Sustainability 10(12). https://doi.org/10.3390/su10124554
Mitic RR (2018) The political economy of higher education finance: the politics of tuition fees and subsidies in OECD Countries, 1945-2015. Rev High Educ 41(3):484–487
OECD (2022) Education at a Glance 2022: OECD Indicators, OECD Publishing, Paris, https://doi.org/10.1787/3197152b-en
Özdoğan Özbal E (2021) Dynamic effects of higher education expenditures on human capital and economic growth: an evaluation of OECD countries. Policy Rev High Educ 5(2):174–196. https://doi.org/10.1080/23322969.2021.1893125
Pinheiro R, Pillay P (2016) Higher education and economic development in the OECD: policy lessons for other countries and regions. J High Educ Policy Manag 38(2):150
Romer M (1990) Endogenous technological change. J Political Econ 98(5):S71–S102
Rowe E (2019) Capitalism without capital: the intangible economy of education reform. Discourse 40(2):271
Sahnoun M, Abdennadher C (2022) Returns to investment in education in the OECD Countries: does governance quality matter? J Knowldege Econ 13(3):1819–1842. https://doi.org/10.1007/s13132-021-00783-0
Samo V. (2022). A snapshot of equity and inclusion in OECD education systems: findings from the Strength through Diversity Policy Survey. OECD Eduction Working Papers. https://doi.org/10.1787/801dd29b-en
Schultz (1961) Investment in human capital. Am Econ Rev 51(1):1–17
Solow RM (1957) Technical change and the aggregate production function. Rev Econ Stat 39(3):312–320
Zapp M (2022) Revisiting the global knowledge economy: the worldwide expansion of research and development personnel, 1980–2015. Minerva 60(2):181–208. https://doi.org/10.1007/s11024-021-09455-4
Article PubMed PubMed Central Google Scholar
Download references
Acknowledgements
This study is funded by 2021 National Social Science Foundation of Higher Education Ideological and Political Course research (Key project) Ideological and Political Education System Construction System Mechanism Research in New Era (No.: 21VSZ004).
Author information
These authors contributed equally: Jian Li, Eryong Xue.
Authors and Affiliations
Institute of International and Comparative Education, Beijing Normal University, Beijing, 100875, China
China Institute of Education Policy, Faculty of Education, Beijing Normal University, Beijing, 100875, China
Eryong Xue, Yukai Wei & Yunshu He
You can also search for this author in PubMed Google Scholar
Substantial contributions to the conception or design of the work: Jian Li and Eryong Xue; the acquisition, analysis, or interpretation of data for the work: Jian Li and Eryong Xue; drafting the work or revising it critically for important intellectual content: Eryong Xue, Jian Li, Yukai Wei, and Yunshu He; final approval of the version to be published: Eryong Xue and Jian Li.
Corresponding authors
Correspondence to Jian Li or Eryong Xue .
Ethics declarations
Competing interests.
The authors declare no competing interests.
Ethical approval
Ethical approval was not required as the study did not involve human participants. It was not required as this article used published data.
Informed consent
This article does not contain any studies with human participants performed by any of the authors.
Additional information
Publisher’s note Springer Nature remains neutral with regard to jurisdictional claims in published maps and institutional affiliations.
Rights and permissions
Open Access This article is licensed under a Creative Commons Attribution 4.0 International License, which permits use, sharing, adaptation, distribution and reproduction in any medium or format, as long as you give appropriate credit to the original author(s) and the source, provide a link to the Creative Commons licence, and indicate if changes were made. The images or other third party material in this article are included in the article’s Creative Commons licence, unless indicated otherwise in a credit line to the material. If material is not included in the article’s Creative Commons licence and your intended use is not permitted by statutory regulation or exceeds the permitted use, you will need to obtain permission directly from the copyright holder. To view a copy of this licence, visit http://creativecommons.org/licenses/by/4.0/ .
Reprints and permissions
About this article
Cite this article.
Li, J., Xue, E., Wei, Y. et al. How popularising higher education affects economic growth and poverty alleviation: empirical evidence from 38 countries. Humanit Soc Sci Commun 11 , 520 (2024). https://doi.org/10.1057/s41599-024-03013-5
Download citation
Received : 29 June 2023
Accepted : 02 April 2024
Published : 17 April 2024
DOI : https://doi.org/10.1057/s41599-024-03013-5
Share this article
Anyone you share the following link with will be able to read this content:
Sorry, a shareable link is not currently available for this article.
Provided by the Springer Nature SharedIt content-sharing initiative
Quick links
- Explore articles by subject
- Guide to authors
- Editorial policies

Persistent Gender Disparities Hinder Women's Safety and Productivity in Zimbabwe
Gender-based violence is a significant concern in Zimbabwe, with a substantial number of women experiencing physical and sexual violence. Photo: Adobe Stock
In Zimbabwe, gender-based violence (GBV) is a significant concern, with a substantial number of women experiencing physical and sexual violence. Approximately 39.4% of women have been subjected to physical violence, and an estimated 11.6% have faced sexual violence. Although there has been a decline in child marriage rates, 16.2% of women were married before the age of 18 as of 2022.
While Zimbabwe has taken several legislative steps against GBV by adopting various international and domestic laws, the Zimbabwe Gender-Based Violence Assessment underscores the necessity for more effective enforcement of GBV legislation and the establishment of legal frameworks that categorically criminalize GBV acts. Zimbabwe is a party to several global and regional legal instruments that promote gender equality and combat GBV. Additionally, the 2013 National Constitution of Zimbabwe is forward-thinking, prohibiting gender discrimination and all forms of GBV.
The GBV Assessment commends the policymakers' commitment to eliminating violence against women and children in Zimbabwe, as espoused in the recently launched Zimbabwe National Strategy to Prevent and Address Gender-based Violence 2023–2030 . However, the assessment notes that more work must be done on the legislative front. "The 2007 Domestic Violence Act needs to be amended to tackle harmful cultural practices and extend coverage to GBV incidents outside the domestic sphere," said Eneida Fernandes, World Bank Country Manager for Zimbabwe.
The assessment urges prompt law harmonization and recommends strengthening coordination platforms to avoid duplication of efforts and overlapping mandates. All sub-platforms should report to the National Gender Forum. It also calls for strengthening the Anti-Domestic Violence Council, which has been inefficient recently.
Recommendations from the report , to prevent GBV, suggest designing, implementing, and evaluating targeted, systematic, evidence-based awareness campaigns to alter social and gender norms towards non-violence and respectful relationships, particularly in GBV hotspots. For GBV response, it is recommended that the justice delivery system be improved by addressing the bottlenecks that lead to delays in GBV case resolutions and establishing “Fast Track” GBV courts.
Accompanying the GBV assessment is the Zimbabwe Gender Assessment , which notes that despite advancements in gender equality, such as securing women's reproductive rights, achieving gender parity in primary education, and increasing female enrollment in higher education, significant gender inequalities remain. These include the underrepresentation of women in wage employment, their overconcentration in the informal labor market, high youth unemployment among women, and high rates of teenage pregnancies and child marriages.
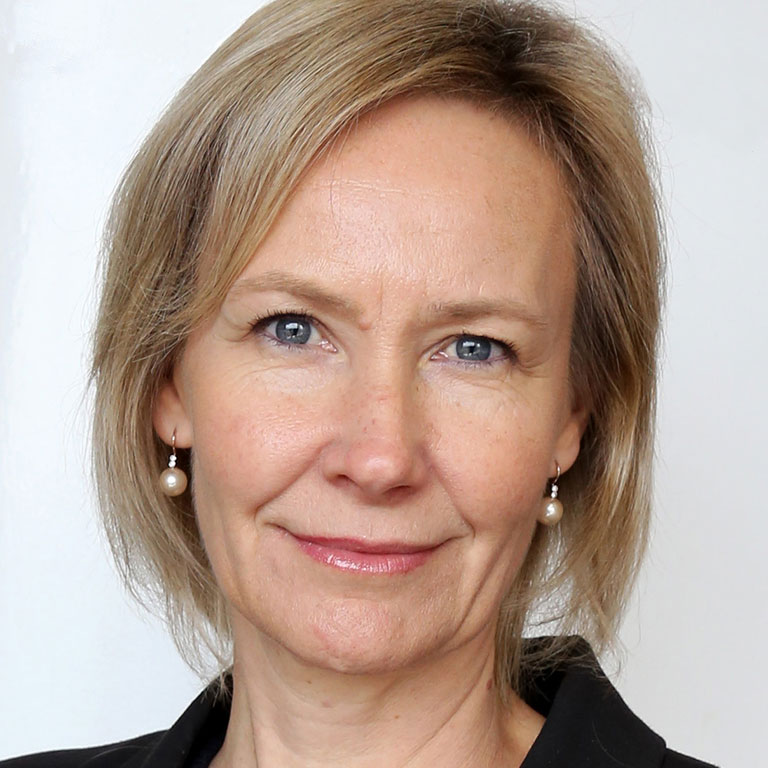
The Gender Assessment reveals that women are less likely to be employed in wage work and more likely to earn less than men. The labor force participation rate for me is 53% compared to 34% for women, and men outnumber women in most sectors. In the agriculture, forestry, and fishing sectors, men account for 58% of the industry labor market, while women make up 42%. Only 22% of working women are employed in wage or salaried positions, compared to 41% for men. Waged women employees earn, on average, about two-thirds of their male counterparts. This is driven by women’s concentration in less renumerated fields, limited work experience and skills, and unequal family and household care responsibilities.
To address gender disparities, recommendations from the report include , among other things, supporting socio-economic skills training for women, which has been shown to increase business outcomes among women microentrepreneurs. It also recommends supporting childcare services, such as offering preferential tax regimes to childcare centers and ensuring that women can engage across all areas of the economy. Addressing the gender divide in land ownership and assets and ring-fencing low-cost finance for women to purchase and own titled land and agricultural implements would also contribute to closing the gender gap.
World Bank in Zimbabwe
Zimbabwe Gender-Based Violence Assessment
Zimbabwe Gender Assessment
This site uses cookies to optimize functionality and give you the best possible experience. If you continue to navigate this website beyond this page, cookies will be placed on your browser. To learn more about cookies, click here .
Are There Too Many Farms in the World? New research on agricultural productivity in developing countries

Farm Scale and Productivity
Egc research summary, january 2022.
Several studies have shown that the relationship between farm size and productivity differs in developing and developed countries. In low-income countries, where small farms are prevalent, larger farms appear to be less productive than the smallest farms, while in advanced economies, larger farms become more productive with scale.
A new study uses data from India to connect the dots between these two phenomena, showing that farm productivity follows a U-shaped curve with farm size, and that substantial gains in output and income would result if farms in developing countries were significantly larger. The analysis suggests that agricultural consolidation – though a challenging policy prospect – could lead to significant economic growth and poverty reduction.
Results at a Glance
- New data analysis shows a U-shaped relationship between farm productivity and farm scale, observed globally.
- The smallest farms, which dominate low-income countries, are more productive than slightly larger farms because of the costs of hiring additional labor.
- Larger farms have increasing productivity with size because they can take advantage of machines that have higher capacity and lower cost at larger scales.
- In India, the optimal farm size is estimated to be 24.5 acres – over 7 times today’s average size of under three acres – using technology available in India today.
- Increasing all farms in India to the optimal size would increase output per acre by 42% and output per worker by 68%. The redistribution would result in an 87% decrease in the number of farms, but a reduction in the total labor force of only 16%.
Read the Publication
We show that labor market transaction costs explain why the smallest farms are more efficient than slightly larger farms in most low-income countries and that increases in machine capacity with operational scale result in the globally observed rising upper tail of productivity. We find evidence consistent with these mechanisms using Indian data, and we show that if all Indian farms were at the minimum scale required to maximize the return on land, the number of farms would be reduced by 82% and income per farm worker would rise by 68%.
Explaining the U-curve in low-income countries using unique data
In low-income countries like India, Indonesia, China, Tanzania, and Colombia, agriculture is dominated by farms that are, on average, much smaller than those in more developed countries, where farming is typically on a large scale. The relationship between farm productivity and farm size also shows a global pattern: the smallest farms in low-income countries are more productive than farms that are slightly bigger (decreasing returns to scale, in technical terms), while in the developed world, productivity increases with scale.
While the inverse relationship between farm size and productivity in low-income countries is well-established, a new study by Mark Rosenzweig , the Frank Altschul Professor of International Economics and an EGC affiliate, and coauthor Andrew D. Foster of Brown University, shows that the U-shape relationship between scale and productivity that is observed across richer and poorer countries occurs within a single low-income country – that is, productivity falls as farm size increases from its smallest unit, and then rises as farm size increases, after a threshold. What has remained a puzzle until now is what drives this pattern: why is the relationship between farm scale and productivity U-shaped? Why are small farms more productive than those which are slightly bigger but far less productive than the larger farms observed in high-income countries?
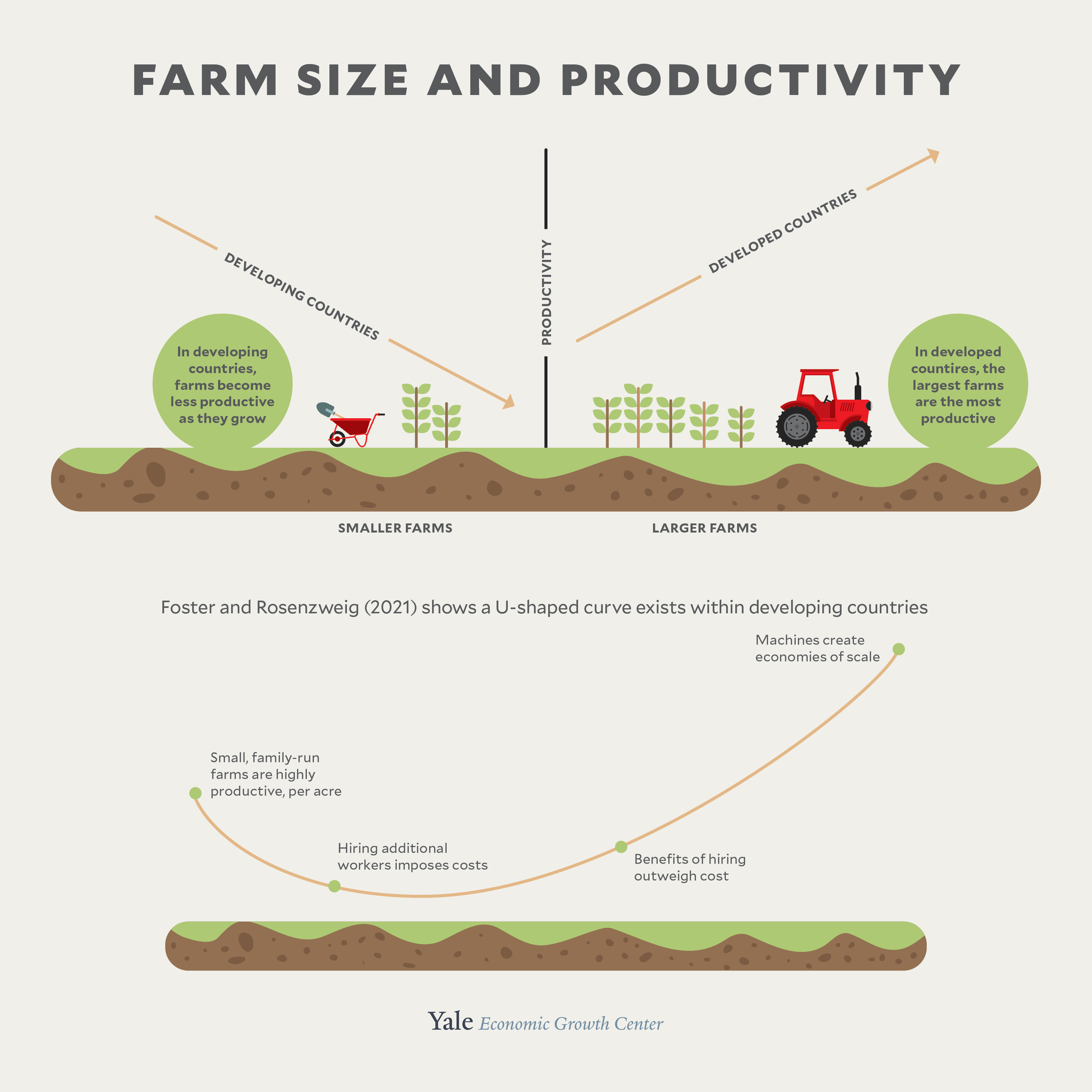
The authors take advantage of unique data from an Indian panel survey, which uniquely contained a substantial fraction of larger farms, to show that this U-curve relationship is driven by two factors: the transaction costs of labor (i.e. the cost of hiring additional farm workers) and the scale-economies of machine capacities.
Explained in simple terms: on a very small farm, family members work the land, participate in wage work on other farms, and operate their farm efficiently. As farm size increases, the family members increase their level of on-farm work until they are unable to spend any more time working (this point of complete self-sufficiency is known as autarky). Because hiring additional labor comes with additional transaction costs (finding workers and travel costs) and thus lowers net income, the family continues to work the land as farm size increases (decreasing labor to land ratio, or productivity) until such point that the benefit of hiring additional workers outweighs the cost, and productivity starts to increase – which is where we observe the bottom of the curve. After this point, productivity rises with farm size, as larger farms can take advantage of machines that have higher capacity at larger scales and lower labor use – mirroring the economies of scale that are well-observed in developed countries.
Increasing farm size could expand agricultural output and reduce poverty
The discovery of this U-shaped pattern within a single context means that what researchers had previously understood about farming in low-income countries was wrong: that is, the smallest farms are not necessarily the most productive. In short, there are too many small farms – especially in low-income countries. The research implies that increasing farm size through consolidation would ultimately increase agricultural output, while substantially reducing poverty in agriculture. The authors illustrate this point using their model to show that the minimum farm size in India that would maximize return on land using only locally- available machinery is 24.5 acres – over 7 times the mean farm size in India today. If all farms were this size, the number of farms in India would decrease by 82%, total output would increase by 42% and income per farm worker would increase by 68%. However, there would only be a 16% decline in the total agricultural workforce, owing to the under-utilization of labor in most small-scale farms.
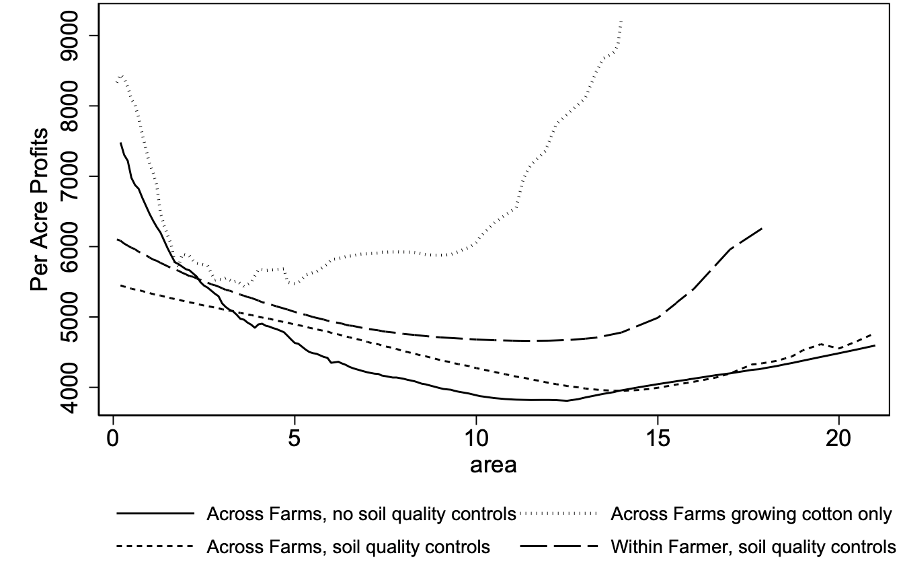
A graph from Foster and Rosenzweig (2021) showing real profits per acre by owned area in India, with the different lines taking into account differences in plot quality and farmer characteristics. Data come from the International Crops Research Institute for the Semi-Arid Tropics (ICRISAT) survey of India, 2009-14.
The authors call attention to the fact that their findings are consistent with a study the government of India made over 70 years ago. In 1947, the United Provinces Zamindari Commission concluded that there were too many small farms in India and that this had a negative effect on the country’s output and poverty levels. Today, however, India’s government is in support of the large number of small land-holdings, and as recently as last year, the Minister of Agriculture stated that while Indian farms are smaller than they have ever been, output is increasing so that there is no evidence that small scale farming is not a cause of the low level of agricultural productivity. Until now.
Rosenzweig argues the government’s conclusion in 1947 is still true today. He stresses that part of the reason behind the consistent defence of small agriculture is that, historically, all the evidence from small farm countries suggested that increasing farm size would decrease productivity. “When you look at existing data, they show that the smallest farms are the most productive . There has been no evidence to refute this view – until now, when we get to see the U-shape because we have more data than before on the few larger farms that exist in India today.”
A challenge for global development
The research suggests that having too many small farms is a development bottleneck for the world and for large producers like India. Agriculture is the dominant economic activity in low-income countries, accounting for, on average, more than 25% of GDP and employing at least 40% of the total workforce . This new evidence thus presents a big idea for growth: it implies that increasing farm size through consolidation could substantially increase agricultural output while reducing poverty and agricultural employment . “If we want to understand barriers to growth, this shows that small farming is both a symptom and a cause of under-development,” Rosenzweig says.
While these findings are compelling for low-income countries, the authors argue that the likelihood that small-scale farmers would increase their landholdings to reach the optimal farm size, on their own, is remote. This is because of the initial decreasing returns to increasing farm size previously described, and the enormous level of coordination required by farmers to “leap the chasm” from a small to a much a larger farm when all neighboring farms are also small.
When asked for historical examples of how countries made this shift, Rosenzweig points to France. Following the Second World War, France enacted several major policy changes concerning agricultural land use. Starting with the establishment of INRA (the French Institute for Agricultural Research) in 1946 and extending through the establishment of Common Agricultural Policy by the EU in 1962, compulsory land consolidation in France was used to increase land productivity by lowering the costs of production. Initially enacted in response to the food shortages of the war, land consolidation offered an opportunity to modernize farming practices and to contribute affordable food for the nation and for trade across Europe. Land consolidation is ongoing in Europe and encompassed 38 million agricultural hectares (one quarter of all cultivated land) as of 2004.
Rosenzweig suggests that his study provides evidence that economists and governments have been thinking incorrectly about small-scale farming in recent decades, and that technologies and processes that augment output on a small scale, emphasized by many NGO’s, are unlikely to make big shifts in agricultural productivity. Small farms are likely to remain the dominant form of production in low-income countries for the foreseeable future, but a policy shift favoring agricultural consolidation, though a challenging prospect, could lead to significant poverty reduction.
Research Summary by Emma Lambert-Porter. Infographic designed by Goodness Okoro.
Numbers, Facts and Trends Shaping Your World
Read our research on:
Full Topic List
Regions & Countries
- Publications
- Our Methods
- Short Reads
- Tools & Resources
Read Our Research On:
- Wealth Surged in the Pandemic, but Debt Endures for Poorer Black and Hispanic Families
2. Wealth gaps across racial and ethnic groups
Table of contents.
- The range of wealth within racial and ethnic groups
- The share of racial or ethnic groups’ wealth held by richer households, not counting the top 1%
- Households with no wealth or in debt
- How wealth varies by race and ethnicity
- The wealth of White households relative to the wealth of other households
- Households in the lower, middle or upper tiers of wealth
- The wealth of U.S. households by income tier
- Racial and ethnic wealth gaps by income tier
- Types of assets owned by U.S. households
- The value of assets owned by households
- The importance of home equity
- The debt level of households
- How high is household debt?
- Acknowledgments
- Data source
- Race and ethnicity
- Household head
- Adjusting for inflation
- Defining income tiers
- Statistical testing
Household wealth or net worth is the value of assets owned by every member of the household minus their debt. The terms are used interchangeably in this report. Assets include owned homes, vehicles, financial accounts, retirement accounts, stocks, bonds and mutual funds, and more. Debt refers to home mortgage loans, education loans, credit card balances, and any other loan or credit extended to the household. Net worth is negative when debt levels are greater than asset values. ( Refer to the methodology for more details.)
“Poorer,” “more solvent,” “wealthier” and “richer” refer to a household’s wealth status relative to other households of the same race and ethnicity . Poorer households rank in the bottom 25% when compared with other households of the same race and ethnicity; more solvent households place from the 25th up to and including the 50th percentile; wealthier households from the 50th up to and including the 75th percentile; and the richer are in the top 25%. The bottom 1% and the top 1% are excluded prior to the ranking so extreme values do not affect estimates for poorer and richer households.
Lower, middle and upper wealth tiers refer to a household’s wealth status relative to all other households in the U.S. The middle wealth tier consists of households whose wealth lies between one-quarter to four times as much as the median wealth of U.S. households – between $41,700 and $667,500 in 2021. Households in the lower wealth tier had wealth less than $41,700, and households in the upper wealth tier had wealth more than $667,500. The wealth tier boundaries vary across years. (Dollar amounts are expressed in December 2021 prices.)
Middle-income households are those with an income that is two-thirds to double that of the U.S. median household income, after incomes have been adjusted for household size and difference in the cost of living across areas. This was about $4,800 to $14,300 monthly in December 2021, for a household of three. Lower-income households had incomes less than $4,800, and upper-income households had incomes greater than $14,300. The income tier boundaries vary across years. (Dollar amounts are expressed in December 2021 prices.)
Households are grouped by the race and ethnicity of the survey reference person, or the household head. White, Black and Asian include those who report being only one race and are not Hispanic. Multiracial includes non-Hispanics of two or more races. Hispanics are of any race. American Indian or Pacific Islander households are not covered in our analysis because of small sample sizes.
The increase in the wealth of U.S. households from 2019 to 2021 was spread widely across racial and ethnic groups. Despite improved fortunes, Black, Hispanic and multiracial households trailed White and Asian households by large margins from the following perspectives, both before and after the pandemic:
- Their wealth relative to the wealth of White and Asian households.
- The shares of households that are in the middle or upper tiers of wealth.
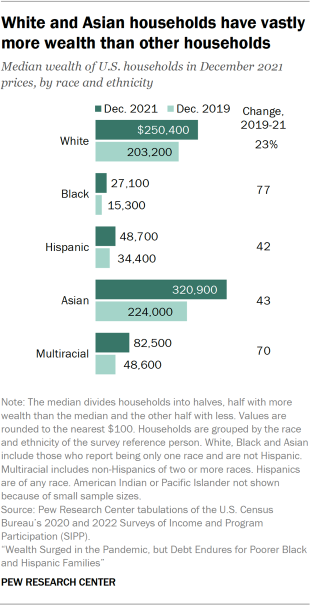
Asian households overall had more wealth than other households two years since the start of the pandemic. In 2021, Asian households had a median net worth of $320,900, compared with $250,400 for White households. The median net worth of Hispanic households ($48,700) and Black households ($27,100) was much less.
In dollar amounts, the wealth gap between White households and Black and Hispanic households increased from 2019 to 2021. For example, in 2019, the typical White household had $168,800 more in wealth than the typical Hispanic household. This gap increased to $201,700 in 2021.
In percentage terms, Black (77%), Hispanic (42%), Asian (43%) and White (23%) households all experienced strong gains in median wealth from 2019 to 2021.
What factors might explain the large wealth gaps across racial and ethnic groups that have endured for decades, if not longer ?
With respect to Black households, evidence points to the role of slavery and post-slavery practices, such as segregation, that created disparate opportunities for wealth accumulation . For Hispanic households, the relative youth of the population and immigration status play a role. In turn, these factors likely contribute to the lower rates and sizes of inheritances received by Black and Hispanic households in comparison with White households.
Asian households overall have more wealth than other households partly because of the higher level of education among Asian adults . In 2021, 61% of Asians ages 25 and older had a bachelor’s degree or higher level of education, compared with 42% of White adults. As a result, the typical Asian household has a much higher income than other households, with more potential for building wealth. But the Asian American population is very diverse, and income varies widely across Asian origin groups . As shown in the following chapter , lower-income Asian households had less wealth than lower-income White households in 2021.
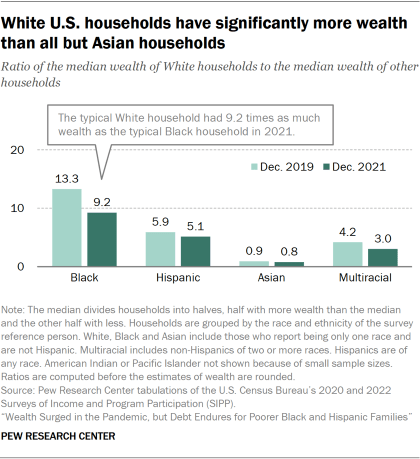
In 2021, the typical White household had 9.2 times as much wealth as the typical Black household – $250,400 vs. $27,100. This ratio stood at 13.3 in 2019, prior to the onset of the pandemic.
White households also had significantly more wealth than Hispanic households (5.1 times) and multiracial households (3.0 times) in 2021. But the median wealth of White households was about 20% less than that of Asian households.
The wealth gaps between White households and Black and Hispanic households in 2019 and 2021 are in line with recent history . But the apparent shrinking of racial and ethnic wealth gaps during the pandemic stands in contrast with what happened during the Great Recession of 2007-09 .
In the Great Recession, the median wealth of White households jumped to nearly 20 times the wealth of Black households and 15 times that of Hispanic households. The key factors then were a sharp decline in home prices and a steep rise in home foreclosures , non-events during the pandemic. Black and Hispanic households saw more harm from these developments during the Great Recession because housing is a more valuable part of their collection of assets .
How does the wealth of a household compare with all other U.S. households? What shares of households are in the middle tier of wealth, and what shares are in the lower or upper tiers?
To address these questions, we grouped U.S. households into three tiers of wealth. The middle tier consists of households whose wealth lies between one-quarter and four times the median wealth of U.S. households – between $41,700 and $667,500 in 2021. Households in the lower tier had wealth less than $41,700 in 2021, and households in the upper tier had wealth more than $667,500.
Fewer than half of U.S. households (44%, or 59 million) were in the middle tier in 2021. This was so despite the wide range of the middle tier – those near the top had 16 times as much wealth as those near the bottom. At the same time, about a third of households (32%, or 43 million) were in the lower wealth tier, and about a quarter (23%, or 31 million) were in the upper tier.
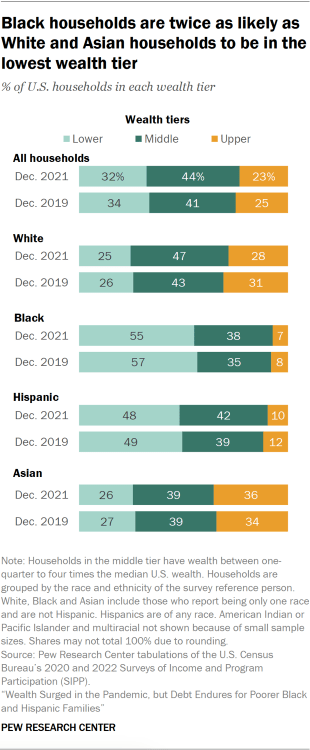
Only 45% of Black households were in either the middle or upper wealth tiers in 2021, the lowest share among the groups examined. The majority of Black households (55%) were in the lower wealth tier in 2021 – that is, they had less than $41,700 in wealth. A somewhat greater share of Hispanic households (52%) were in the middle or upper wealth tier, and 48% were in the lower wealth tier.
In contrast, more than 70% of White and Asian households were in either the middle or upper wealth tier. Roughly one-third of Asian households (36%) were in the upper tier alone, with a net worth of more than $667,500 in 2021. Slightly more than a quarter (28%) of White households were in the upper tier.
Increases in household wealth during the pandemic did lead to a more equal distribution of wealth. The share of households in the middle tier increased from 41% in 2019 to 44% in 2021. Meanwhile, the shares of households in the lower and upper wealth tiers edged down by 2 percentage points each.
A similar shift to the middle was seen in Hispanic and White households, whose shares in the middle wealth tier increased by 3 to 4 points from 2019 to 2021. However, no such change was experienced by Asian households, with their share in the middle wealth tier holding at 39%.
Sign up for our weekly newsletter
Fresh data delivery Saturday mornings
Sign up for The Briefing
Weekly updates on the world of news & information
- Asian Americans
- Black Americans
- COVID-19 & the Economy
- Economic Inequality
- Hispanics/Latinos
- Income & Wages
- Income, Wealth & Poverty
- Racial & Ethnic Groups
Key facts about Asian Americans living in poverty
Methodology: 2023 focus groups of asian americans, 1 in 10: redefining the asian american dream (short film), the hardships and dreams of asian americans living in poverty, key facts about asian american eligible voters in 2024, most popular, report materials.
1615 L St. NW, Suite 800 Washington, DC 20036 USA (+1) 202-419-4300 | Main (+1) 202-857-8562 | Fax (+1) 202-419-4372 | Media Inquiries
Research Topics
- Age & Generations
- Coronavirus (COVID-19)
- Economy & Work
- Family & Relationships
- Gender & LGBTQ
- Immigration & Migration
- International Affairs
- Internet & Technology
- Methodological Research
- News Habits & Media
- Non-U.S. Governments
- Other Topics
- Politics & Policy
- Race & Ethnicity
- Email Newsletters
ABOUT PEW RESEARCH CENTER Pew Research Center is a nonpartisan fact tank that informs the public about the issues, attitudes and trends shaping the world. It conducts public opinion polling, demographic research, media content analysis and other empirical social science research. Pew Research Center does not take policy positions. It is a subsidiary of The Pew Charitable Trusts .
Copyright 2024 Pew Research Center
Terms & Conditions
Privacy Policy
Cookie Settings
Reprints, Permissions & Use Policy
Advancing social justice, promoting decent work ILO is a specialized agency of the United Nations

Main Figures on Forced Labour
27.6 million
people are in forced labour.
US$ 236 billion
generated in illegal profits every year.
3.9 million
of them are in State-imposed form of forced labour.
of them are women and girls (4.9 million in forced commercial sexual exploitation, and 6 million in other economic sectors).
of them are children (3.3 million). More than half of these children are in commercial sexual exploitation.
3 times more
risk of forced labour for migrant workers.
- Victims of forced labour include 17.3 million exploited in the private sector; 6.3 million in forced commercial sexual exploitation, and 3.9 million in forced labour imposed by State.
- The Asia and the Pacific region has the highest number of people in forced labour (15.1 million) and the Arab States the highest prevalence (5.3 per thousand people).
- Addressing decent work deficits in the informal economy, as part of broader efforts towards economic formalization, is a priority for progress against forced labour.
Source: 2022 Global Estimates
Forced Labour Observatory
The Forced Labour Observatory (FLO) is a database that provides comprehensive global and country information on forced labour, including on international and national legal and institutional frameworks; enforcement, prevention and protection measures, as well as information related to access to justice; remedies, and cooperation.
Global Reports

2021 Global Estimates of Modern Slavery: Forced Labour and Forced Marriage
The latest estimates show that forced labour and forced marriage have increased significantly in the last five years, according to the International Labour Organization, Walk Free and the International Organisation for Migration.
- Full Report
- Executive Summary
- Press Release
- Third estimates: Modern Slavery (2017)
- Second estimates: Forced Labour (2012)
- First estimates: Forced Labour (2005)

Profits and Poverty: The Economics of Forced Labour (2024)
The study investigates the underlying factors that drive forced labour, of which a major one is illegal profits.
- Press release
- First edition of the report and second estimates (2014)
- First estimates of illegal profits from forced labour (2005)
Main Statistical Tools on Forced Labour
- Hard to See, Harder to Count: Guidelines for Forced Labour Surveys
- Ethical Guidelines for Research on Forced Labour
- Evidence Gap Map on Forced Labour
- Global Research Agenda (child labour, forced labour and human trafficking)
ICLS and forced labour

The International Conference of Labour Statisticians (ICLS) is the authoritative body to set global standards in labour statistics. During its 20th meeting, in October 2018, the ICLS adopted the "Guidelines concerning the measurement of forced labour". The intent of the guidelines is to facilitate the process of testing the measurement of forced labour in different national circumstances and/or measurement objectives.
- Guidelines concerning the measurement of forced labour (ICLS 2018)
- Measurement of forced labour: stocktaking and way forward (ICLS 2023 Room document 22)
- All ICLS documents
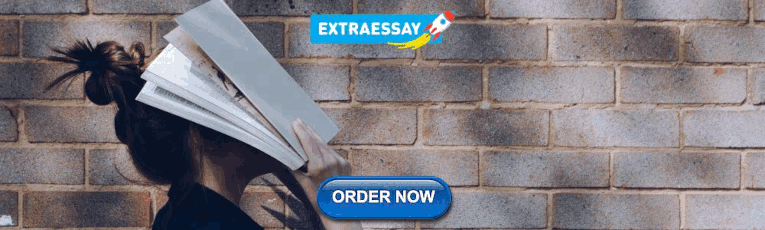
IMAGES
COMMENTS
Overview. Around 700 million people live on less than $2.15 per day, the extreme poverty line. Extreme poverty remains concentrated in parts of Sub-Saharan Africa, fragile and conflict-affected areas, and rural areas. After decades of progress, the pace of global poverty reduction began to slow by 2015, in tandem with subdued economic growth.
The International Poverty Line of $2.15 per day (in 2017 international-$) is the best known absolute poverty line and is used by the World Bank and the UN to measure extreme poverty around the world. The value of relative poverty lines instead rises and falls as average incomes change within a given country.
Global poverty estimates were updated today on the World Bank's Poverty and Inequality Platform (PIP). More than 100 new surveys were added to the PIP database, bringing the total number of surveys to more than 2,300. With more recent survey data, this March 2024 PIP update is the first to report a global poverty number for 2020-2022, the period of the COVID-19 pandemic.
We can apply this $30-a-day-poverty-line to the global income distribution to see the share in poverty as judged by the definition of poverty in high-income countries. 5. The latest global data tells us that the huge majority - 84% of the world population - live on less than $30 per day. That means 6.7 billion people.
The World Development Report of 2013 measures, perhaps for the first time, inequality of opportunity to labor market outcomes in a discrete setting. It focuses on Europe and Central Asia. Latest research from the World Bank on Poverty, including reports, studies, publications, working papers and articles.
Sustainable Development Goal 1 is to "end poverty in all its forms everywhere", according to the United Nations. The visualizations and data below present the global perspective on where the world stands today and how it has changed. Longer-term trends, research, and additional data on poverty can be found on Our World in Data, particularly ...
The World Bank's Poverty and Shared Prosperity series provides the latest estimates and trends in global poverty and shared prosperity. The 2022 edition provides the first comprehensive look at the landscape of poverty in the aftermath of an extraordinary series of shocks to the global economy. Global progress in reducing extreme poverty has ...
Posted on: July 11, 2023. This report presents a compact update on the state of multidimensional poverty (henceforth referred to as "poverty") in the world. It compiles data from 110 developing countries covering 6.1 billion people, accounting for 92 percent of the population in developing countries. It tells an important and persistent ...
The World Bank's Poverty and Inequality Research Program has two main objectives: (1) improve current data as well as methods and tools for poverty and inequality analysis and (2) use the improved data and existing data sources to better understand the economic and social processes determining the extent of poverty and inequality and to assess the effectiveness of specific policies in reducing ...
Understanding Poverty. UNDERSTANDING POVERTY. We face big challenges to help the world's poorest people and ensure that everyone sees benefits from economic growth. Data and research help us understand these challenges and set priorities, share knowledge of what works, and measure progress.
Regional poverty estimates are now reported up to 2021, depending on sufficient data coverage over the period of the COVID-19 pandemic. Poverty data are reported for Europe and Central Asia until 2020, and Latin America and the Caribbean until 2021. For all other developing regions, poverty data are reported for pre-pandemic years (see Figure 1).
Measuring Poverty. There has been marked progress in reducing poverty over the past decades. In 2015, 10 per cent of the world's population lived at or below $1.90 a day -down from 16 per cent ...
Similar concerns have been raised about global development: research into poverty interventions is often absent in the poorest countries, owing to conflict and political instability.
Measuring Poverty. The World Bank's mission is to end extreme poverty and boost shared prosperity on a livable planet. To monitor progress and understand better ways to reduce poverty, it is important to measure poverty regularly. The international poverty line is set at $2.15 per person per day using 2017 prices.
The United Nations has a Sustainable Development Goal (SDG) to eradicate extreme poverty by 2030; this was always ambitious, even when policymakers and researchers set the SDGs in 2015. It is now ...
Highlights. When key uncertainty sources are introduced the dollar-a-day method identifies a 5.19% global poverty reduction instead of the 50% of the MDG1 target (1990-2015). In light of the identified uncertainties, the profile of the global poor and the distribution of poverty around the world may be substantially misleading.
Senior Research Analyst (former), Center for Sustainable Development . ... World Poverty Clock. In 1990, 1.9 billion people, or 36% of the world, lived below the $1.90 per day extreme
The poverty line refers to the proportion of people living on less than US$1.25 a day as a percentage of the country's total population or the proportion of people living in poverty.
Poverty At-A-Glance. Around 700 million people live today in extreme poverty - they subsist on less than $2.15 per day, the extreme poverty line. After several decades of continuous global poverty reduction, a period of significant crises and shocks resulted in three years of lost progress between 2020-2022.
1. Introduction. Poverty "is one of the defining challenges of the 21st Century facing the world" (Gweshengwe et al., Citation 2020, p. 1).In 2019, about 1.3 billion people in 101 countries were living in poverty (United Nations Development Programme and Oxford Poverty and Human Development Initiative, Citation 2019).For this reason, the 2030 Global Agenda for Sustainable Development Goals ...
The new WHO/World Bank reports also warn that financial hardship is likely to become more intense as poverty grows, incomes fall, and governments face tighter fiscal constraints. "Even before the COVID-19 pandemic struck, almost 1 billion people were spending more than 10 per cent of their household budget on health," said Juan Pablo Uribe ...
The Hardships and Dreams of Asian Americans Living in Poverty. About one-in-ten Asian Americans live in poverty. Pew Research Center conducted 18 focus groups in 12 languages to explore their stories and experiences. reportDec 4, 2023.
Target 1.1: Eradicate extreme poverty for all people everywhere. Achievement: In 2015, 10.8% of people (800 million) were living in extreme poverty. By the end of 2022, people living in poverty were reduced by only 2.4% as 8.4% of people (670 million) were still living in extreme poverty. Target 1.2: Reduce at least by half the proportion of ...
particularly for case study research. Poverty is also associated with insufficient outcomes with respect to health, nutrition and literacy, to deficient social relations, to insecurity, and to low self-confidence and powerlessness. ... fiOur dream is a world free of poverty,fl writes the World Bank, and its first mission statement is
South Africa is one of the countries that has high poverty and unemployment rates and low education levels, which trigger high social inequality. ... Discover the world's research. 25+ million ...
With 189 member countries, staff from more than 170 countries, and offices in over 130 locations, the World Bank Group is a unique global partnership: five institutions working for sustainable solutions that reduce poverty and build shared prosperity in developing countries.
The research suggests that having too many small farms is a development bottleneck for the world and for large producers like India. Agriculture is the dominant economic activity in low-income countries, accounting for, on average, more than 25% of GDP and employing at least 40% of the total workforce.
This study aims to contribute to the empirical research on social and economic conditions of people with disabilities in developing countries. Using comparable data and methods across countries, this study presents a snapshot of economic and poverty situation of working-age persons with disabilities and their households in 15 developing countries.
The Hardships and Dreams of Asian Americans Living in Poverty. short read Jan 10, 2024. ... ABOUT PEW RESEARCH CENTER Pew Research Center is a nonpartisan fact tank that informs the public about the issues, attitudes and trends shaping the world. It conducts public opinion polling, demographic research, media content analysis and other ...
Profits and Poverty: The Economics of Forced Labour (2024) 3.9 million. of them are in State-imposed form of forced labour. 2022 Global Estimates. 39.4%. ... Global Research Agenda (child labour, forced labour and human trafficking) ICLS and forced labour.