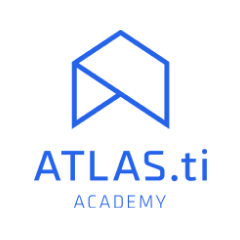
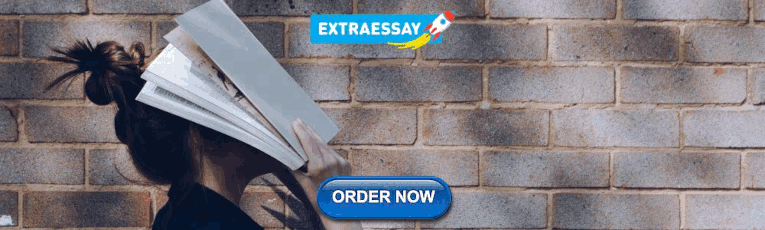
The Ultimate Guide to Qualitative Research - Part 1: The Basics
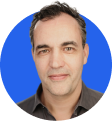
- Introduction and overview
- What is qualitative research?
- What is qualitative data?
- Examples of qualitative data
- Qualitative vs. quantitative research
- Mixed methods
- Qualitative research preparation
- Theoretical perspective
- Theoretical framework
- Literature reviews
- Research question
- Conceptual framework
- Conceptual vs. theoretical framework
- Data collection
- Qualitative research methods
- Focus groups
- Observational research
- Case studies
- Ethnographical research
- Ethical considerations
- Confidentiality and privacy
What is research bias?
Understanding unconscious bias, how to avoid bias in research, bias and subjectivity in research.
- Power dynamics
- Reflexivity
Bias in research
In a purely objective world, research bias would not exist because knowledge would be a fixed and unmovable resource; either one knows about a particular concept or phenomenon, or they don't. However, qualitative research and the social sciences both acknowledge that subjectivity and bias exist in every aspect of the social world, which naturally includes the research process too. This bias is manifest in the many different ways that knowledge is understood, constructed, and negotiated, both in and out of research.
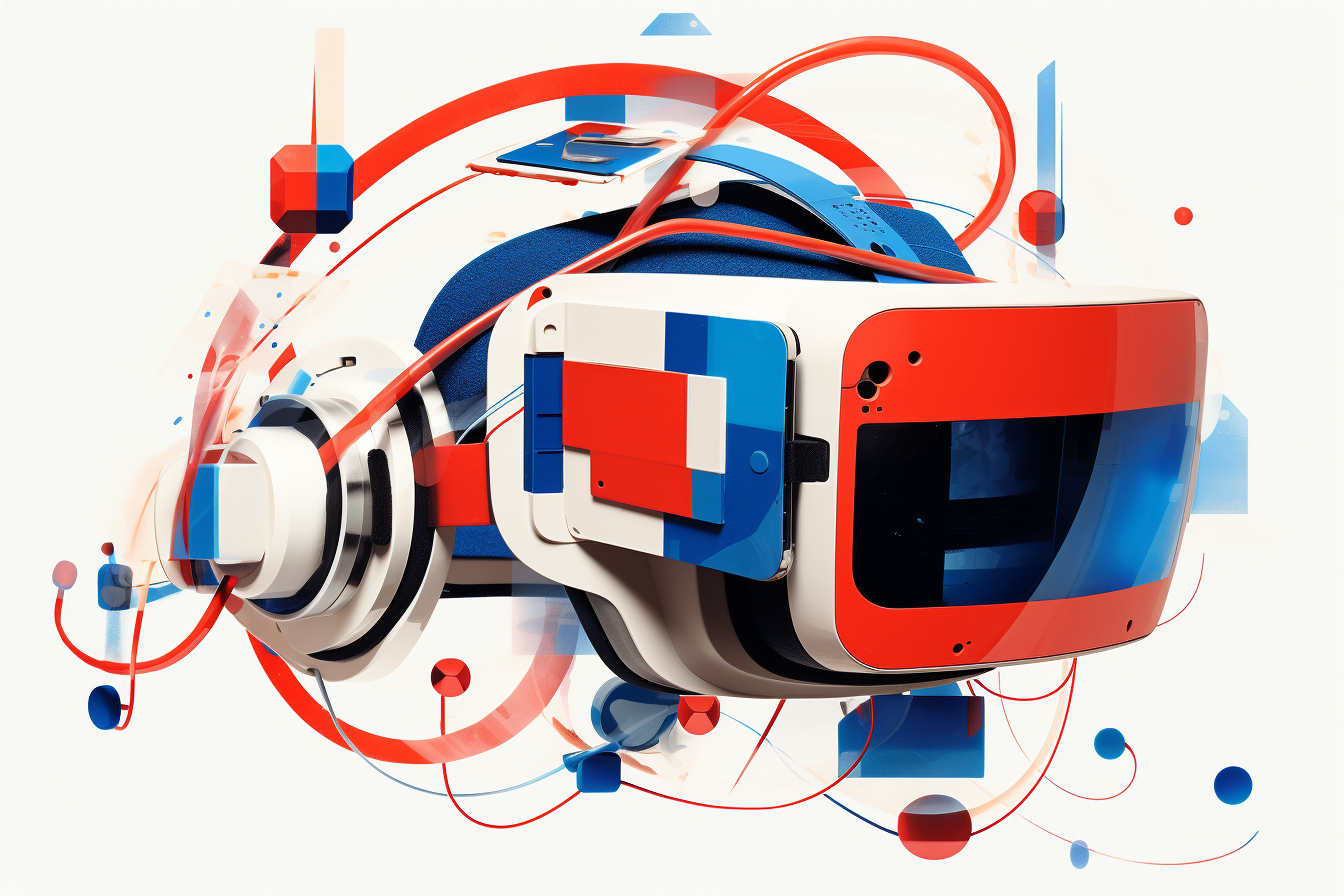
Understanding research bias has profound implications for data collection methods and data analysis , requiring researchers to take particular care of how to account for the insights generated from their data .
Research bias, often unavoidable, is a systematic error that can creep into any stage of the research process , skewing our understanding and interpretation of findings. From data collection to analysis, interpretation , and even publication , bias can distort the truth we seek to capture and communicate in our research.
It’s also important to distinguish between bias and subjectivity, especially when engaging in qualitative research . Most qualitative methodologies are based on epistemological and ontological assumptions that there is no such thing as a fixed or objective world that exists “out there” that can be empirically measured and understood through research. Rather, many qualitative researchers embrace the socially constructed nature of our reality and thus recognize that all data is produced within a particular context by participants with their own perspectives and interpretations. Moreover, the researcher’s own subjective experiences inevitably shape how they make sense of the data. These subjectivities are considered to be strengths, not limitations, of qualitative research approaches, because they open new avenues for knowledge generation. This is also why reflexivity is so important in qualitative research. When we refer to bias in this guide, on the other hand, we are referring to systematic errors that can negatively affect the research process but that can be mitigated through researchers’ careful efforts.
To fully grasp what research bias is, it's essential to understand the dual nature of bias. Bias is not inherently evil. It's simply a tendency, inclination, or prejudice for or against something. In our daily lives, we're subject to countless biases, many of which are unconscious. They help us navigate our world, make quick decisions, and understand complex situations. But when conducting research, these same biases can cause significant issues.
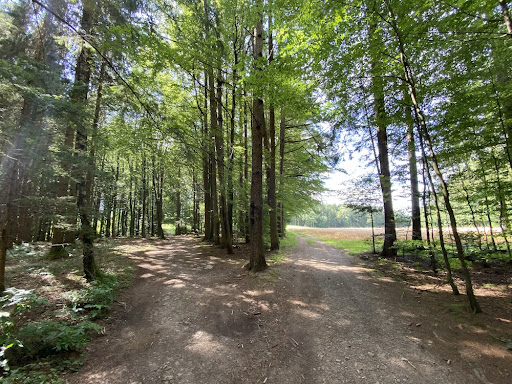
Research bias can affect the validity and credibility of research findings, leading to erroneous conclusions. It can emerge from the researcher's subconscious preferences or the methodological design of the study itself. For instance, if a researcher unconsciously favors a particular outcome of the study, this preference could affect how they interpret the results, leading to a type of bias known as confirmation bias.
Research bias can also arise due to the characteristics of study participants. If the researcher selectively recruits participants who are more likely to produce desired outcomes, this can result in selection bias.
Another form of bias can stem from data collection methods . If a survey question is phrased in a way that encourages a particular response, this can introduce response bias. Moreover, inappropriate survey questions can have a detrimental effect on future research if such studies are seen by the general population as biased toward particular outcomes depending on the preferences of the researcher.
Bias can also occur during data analysis . In qualitative research for instance, the researcher's preconceived notions and expectations can influence how they interpret and code qualitative data, a type of bias known as interpretation bias. It's also important to note that quantitative research is not free of bias either, as sampling bias and measurement bias can threaten the validity of any research findings.
Given these examples, it's clear that research bias is a complex issue that can take many forms and emerge at any stage in the research process. This section will delve deeper into specific types of research bias, provide examples, discuss why it's an issue, and provide strategies for identifying and mitigating bias in research.
What is an example of bias in research?
Bias can appear in numerous ways. One example is confirmation bias, where the researcher has a preconceived explanation for what is going on in their data, and any disconfirming evidence is (unconsciously) ignored. For instance, a researcher conducting a study on daily exercise habits might be inclined to conclude that meditation practices lead to greater engagement in exercise because that researcher has personally experienced these benefits. However, conducting rigorous research entails assessing all the data systematically and verifying one’s conclusions by checking for both supporting and refuting evidence.
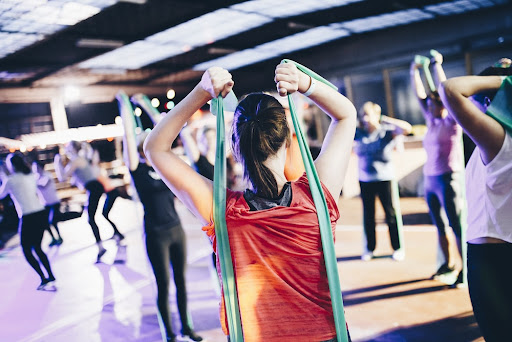
What is a common bias in research?
Confirmation bias is one of the most common forms of bias in research. It happens when researchers unconsciously focus on data that supports their ideas while ignoring or undervaluing data that contradicts their ideas. This bias can lead researchers to mistakenly confirm their theories, despite having insufficient or conflicting evidence.
What are the different types of bias?
There are several types of research bias, each presenting unique challenges. Some common types include:
Confirmation bias: As already mentioned, this happens when a researcher focuses on evidence supporting their theory while overlooking contradictory evidence.
Selection bias: This occurs when the researcher's method of choosing participants skews the sample in a particular direction.
Response bias: This happens when participants in a study respond inaccurately or falsely, often due to misleading or poorly worded questions.
Observer bias (or researcher bias): This occurs when the researcher unintentionally influences the results because of their expectations or preferences.
Publication bias: This type of bias arises when studies with positive results are more likely to get published, while studies with negative or null results are often ignored.
Analysis bias: This type of bias occurs when the data is manipulated or analyzed in a way that leads to a particular result, whether intentionally or unintentionally.
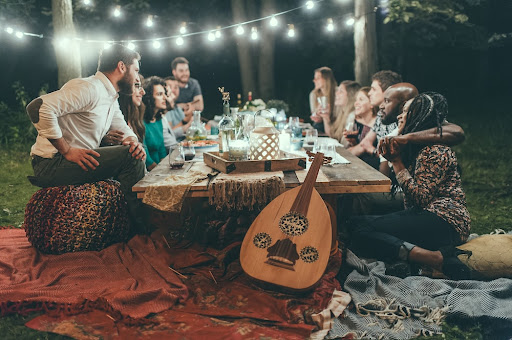
What is an example of researcher bias?
Researcher bias, also known as observer bias, can occur when a researcher's expectations or personal beliefs influence the results of a study. For instance, if a researcher believes that a particular therapy is effective, they might unconsciously interpret ambiguous results in a way that supports the efficacy of the therapy, even if the evidence is not strong enough.
Even quantitative research methodologies are not immune from bias from researchers. Market research surveys or clinical trial research, for example, may encounter bias when the researcher chooses a particular population or methodology to achieve a specific research outcome. Questions in customer feedback surveys whose data is employed in quantitative analysis can be structured in such a way as to bias survey respondents toward certain desired answers.
Turn your data into findings with ATLAS.ti
Key insights are at your fingertips with our powerful interface. See how with a free trial.
Identifying and avoiding bias in research
As we will remind you throughout this chapter, bias is not a phenomenon that can be removed altogether, nor should we think of it as something that should be eliminated. In a subjective world involving humans as researchers and research participants, bias is unavoidable and almost necessary for understanding social behavior. The section on reflexivity later in this guide will highlight how different perspectives among researchers and human subjects are addressed in qualitative research. That said, bias in excess can place the credibility of a study's findings into serious question. Scholars who read your research need to know what new knowledge you are generating, how it was generated, and why the knowledge you present should be considered persuasive. With that in mind, let's look at how bias can be identified and, where it interferes with research, minimized.
How do you identify bias in research?
Identifying bias involves a critical examination of your entire research study involving the formulation of the research question and hypothesis , the selection of study participants, the methods for data collection, and the analysis and interpretation of data. Researchers need to assess whether each stage has been influenced by bias that may have skewed the results. Tools such as bias checklists or guidelines, peer review , and reflexivity (reflecting on one's own biases) can be instrumental in identifying bias.
How do you identify research bias?
Identifying research bias often involves careful scrutiny of the research methodology and the researcher's interpretations. Was the sample of participants relevant to the research question ? Were the interview or survey questions leading? Were there any conflicts of interest that could have influenced the results? It also requires an understanding of the different types of bias and how they might manifest in a research context. Does the bias occur in the data collection process or when the researcher is analyzing data?
Research transparency requires a careful accounting of how the study was designed, conducted, and analyzed. In qualitative research involving human subjects, the researcher is responsible for documenting the characteristics of the research population and research context. With respect to research methods, the procedures and instruments used to collect and analyze data are described in as much detail as possible.
While describing study methodologies and research participants in painstaking detail may sound cumbersome, a clear and detailed description of the research design is necessary for good research. Without this level of detail, it is difficult for your research audience to identify whether bias exists, where bias occurs, and to what extent it may threaten the credibility of your findings.
How to recognize bias in a study?
Recognizing bias in a study requires a critical approach. The researcher should question every step of the research process: Was the sample of participants selected with care? Did the data collection methods encourage open and sincere responses? Did personal beliefs or expectations influence the interpretation of the results? External peer reviews can also be helpful in recognizing bias, as others might spot potential issues that the original researcher missed.
The subsequent sections of this chapter will delve into the impacts of research bias and strategies to avoid it. Through these discussions, researchers will be better equipped to handle bias in their work and contribute to building more credible knowledge.
Unconscious biases, also known as implicit biases, are attitudes or stereotypes that influence our understanding, actions, and decisions in an unconscious manner. These biases can inadvertently infiltrate the research process, skewing the results and conclusions. This section aims to delve deeper into understanding unconscious bias, its impact on research, and strategies to mitigate it.
What is unconscious bias?
Unconscious bias refers to prejudices or social stereotypes about certain groups that individuals form outside their conscious awareness. Everyone holds unconscious beliefs about various social and identity groups, and these biases stem from a tendency to organize social worlds into categories.
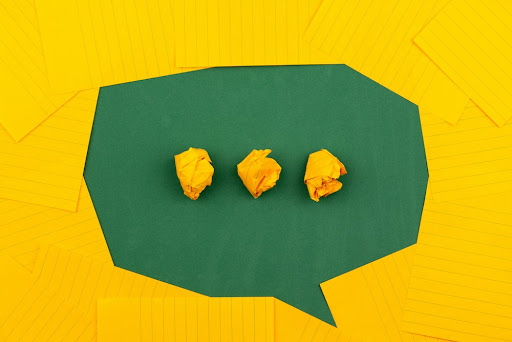
How does unconscious bias infiltrate research?
Unconscious bias can infiltrate research in several ways. It can affect how researchers formulate their research questions or hypotheses , how they interact with participants, their data collection methods, and how they interpret their data . For instance, a researcher might unknowingly favor participants who share similar characteristics with them, which could lead to biased results.
Implications of unconscious bias
The implications of unconscious research bias are far-reaching. It can compromise the validity of research findings , influence the choice of research topics, and affect peer review processes . Unconscious bias can also lead to a lack of diversity in research, which can severely limit the value and impact of the findings.
Strategies to mitigate unconscious research bias
While it's challenging to completely eliminate unconscious bias, several strategies can help mitigate its impact. These include being aware of potential unconscious biases, practicing reflexivity , seeking diverse perspectives for your study, and engaging in regular bias-checking activities, such as bias training and peer debriefing .
By understanding and acknowledging unconscious bias, researchers can take steps to limit its impact on their work, leading to more robust findings.
Why is researcher bias an issue?
Research bias is a pervasive issue that researchers must diligently consider and address. It can significantly impact the credibility of findings. Here, we break down the ramifications of bias into two key areas.
How bias affects validity
Research validity refers to the accuracy of the study findings, or the coherence between the researcher’s findings and the participants’ actual experiences. When bias sneaks into a study, it can distort findings and move them further away from the realities that were shared by the research participants. For example, if a researcher's personal beliefs influence their interpretation of data , the resulting conclusions may not reflect what the data show or what participants experienced.
The transferability problem
Transferability is the extent to which your study's findings can be applied beyond the specific context or sample studied. Applying knowledge from one context to a different context is how we can progress and make informed decisions. In quantitative research , the generalizability of a study is a key component that shapes the potential impact of the findings. In qualitative research , all data and knowledge that is produced is understood to be embedded within a particular context, so the notion of generalizability takes on a slightly different meaning. Rather than assuming that the study participants are statistically representative of the entire population, qualitative researchers can reflect on which aspects of their research context bear the most weight on their findings and how these findings may be transferable to other contexts that share key similarities.
How does bias affect research?
Research bias, if not identified and mitigated, can significantly impact research outcomes. The ripple effects of research bias extend beyond individual studies, impacting the body of knowledge in a field and influencing policy and practice. Here, we delve into three specific ways bias can affect research.
Distortion of research results
Bias can lead to a distortion of your study's findings. For instance, confirmation bias can cause a researcher to focus on data that supports their interpretation while disregarding data that contradicts it. This can skew the results and create a misleading picture of the phenomenon under study.
Undermining scientific progress
When research is influenced by bias, it not only misrepresents participants’ realities but can also impede scientific progress. Biased studies can lead researchers down the wrong path, resulting in wasted resources and efforts. Moreover, it could contribute to a body of literature that is skewed or inaccurate, misleading future research and theories.
Influencing policy and practice based on flawed findings
Research often informs policy and practice. If the research is biased, it can lead to the creation of policies or practices that are ineffective or even harmful. For example, a study with selection bias might conclude that a certain intervention is effective, leading to its broad implementation. However, suppose the transferability of the study's findings was not carefully considered. In that case, it may be risky to assume that the intervention will work as well in different populations, which could lead to ineffective or inequitable outcomes.
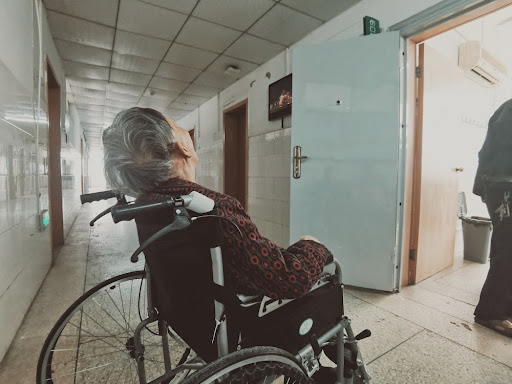
While it's almost impossible to eliminate bias in research entirely, it's crucial to mitigate its impact as much as possible. By employing thoughtful strategies at every stage of research, we can strive towards rigor and transparency , enhancing the quality of our findings. This section will delve into specific strategies for avoiding bias.
How do you know if your research is biased?
Determining whether your research is biased involves a careful review of your research design, data collection , analysis , and interpretation . It might require you to reflect critically on your own biases and expectations and how these might have influenced your research. External peer reviews can also be instrumental in spotting potential bias.
Strategies to mitigate bias
Minimizing bias involves careful planning and execution at all stages of a research study. These strategies could include formulating clear, unbiased research questions , ensuring that your sample meaningfully represents the research problem you are studying, crafting unbiased data collection instruments, and employing systematic data analysis techniques. Transparency and reflexivity throughout the process can also help minimize bias.
Mitigating bias in data collection
To mitigate bias in data collection, ensure your questions are clear, neutral, and not leading. Triangulation, or using multiple methods or data sources, can also help to reduce bias and increase the credibility of your findings.
Mitigating bias in data analysis
During data analysis , maintaining a high level of rigor is crucial. This might involve using systematic coding schemes in qualitative research or appropriate statistical tests in quantitative research . Regularly questioning your interpretations and considering alternative explanations can help reduce bias. Peer debriefing , where you discuss your analysis and interpretations with colleagues, can also be a valuable strategy.
By using these strategies, researchers can significantly reduce the impact of bias on their research, enhancing the quality and credibility of their findings and contributing to a more robust and meaningful body of knowledge.
Impact of cultural bias in research
Cultural bias is the tendency to interpret and judge phenomena by standards inherent to one's own culture. Given the increasingly multicultural and global nature of research, understanding and addressing cultural bias is paramount. This section will explore the concept of cultural bias, its impacts on research, and strategies to mitigate it.
What is cultural bias in research?
Cultural bias refers to the potential for a researcher's cultural background, experiences, and values to influence the research process and findings. This can occur consciously or unconsciously and can lead to misinterpretation of data, unfair representation of cultures, and biased conclusions.
How does cultural bias infiltrate research?
Cultural bias can infiltrate research at various stages. It can affect the framing of research questions , the design of the study, the methods of data collection , and the interpretation of results . For instance, a researcher might unintentionally design a study that does not consider the cultural context of the participants, leading to a biased understanding of the phenomenon being studied.
Implications of cultural bias
The implications of cultural bias are profound. Cultural bias can skew your findings, limit the transferability of results, and contribute to cultural misunderstandings and stereotypes. This can ultimately lead to inaccurate or ethnocentric conclusions, further perpetuating cultural bias and inequities.
As a result, many social science fields like sociology and anthropology have been critiqued for cultural biases in research. Some of the earliest research inquiries in anthropology, for example, have had the potential to reduce entire cultures to simplistic stereotypes when compared to mainstream norms. A contemporary researcher respecting ethical and cultural boundaries, on the other hand, should seek to properly place their understanding of social and cultural practices in sufficient context without inappropriately characterizing them.
Strategies to mitigate cultural bias
Mitigating cultural bias requires a concerted effort throughout the research study. These efforts could include educating oneself about other cultures, being aware of one's own cultural biases, incorporating culturally diverse perspectives into the research process, and being sensitive and respectful of cultural differences. It might also involve including team members with diverse cultural backgrounds or seeking external cultural consultants to challenge assumptions and provide alternative perspectives.
By acknowledging and addressing cultural bias, researchers can contribute to more culturally competent, equitable, and valid research. This not only enriches the scientific body of knowledge but also promotes cultural understanding and respect.
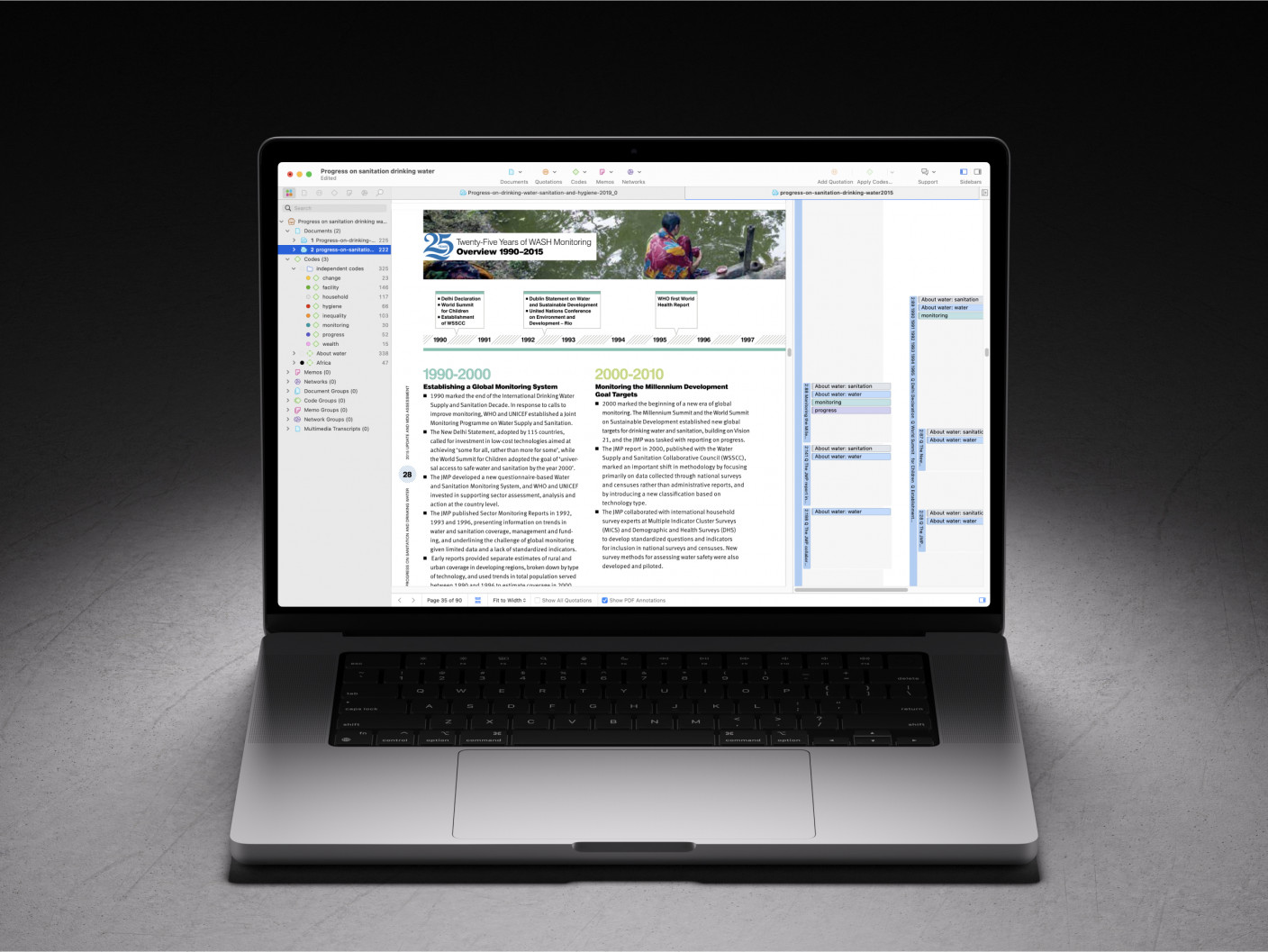
Ready to jumpstart your research with ATLAS.ti?
Conceptualize your research project with our intuitive data analysis interface. Download a free trial today.
Keep in mind that bias is a force to be mitigated, not a phenomenon that can be eliminated altogether, and the subjectivities of each person are what make our world so complex and interesting. As things are continuously changing and adapting, research knowledge is also continuously being updated as we further develop our understanding of the world around us.
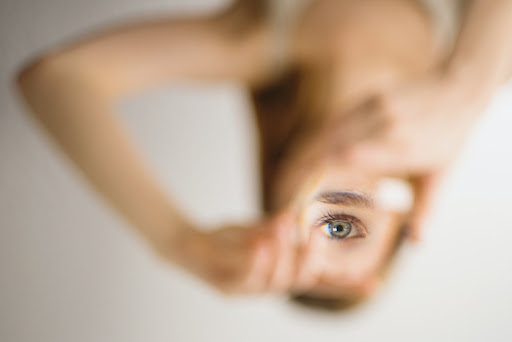
Ready to analyze your data with ATLAS.ti?
See how our intuitive software can draw key insights from your data with a free trial today.

How to Avoid Bias in Qualitative Research
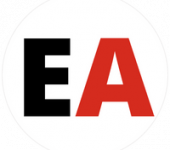
You can also listen to this article as an audio recording.
Research bias occurs when researchers try to influence the results of their work, in order to get the outcome they want. Often, researchers may not be aware they are doing this. Whether they are aware or not, such behavior clearly severely affects the impartiality of a study and greatly reduces the value of the results.
The Issues in Qualitative Research
Recently, I discussed the problem of bias with a researcher friend.
“I heard that research bias is a bigger problem for qualitative research than quantitative research.”
“Why is that?”
“Qualitative research relies more on the experience and judgment of the researcher. Also, the type of data collected is subjective and unique to the person or situation. So it is much harder to avoid bias than in quantitative research.”
“Are there ways to avoid bias ?”
“A good start is to recognize that bias exists in all research. We can then try to predict what type of bias we might have in our study, and try to avoid it as much as possible.”
Types of Bias in Research
“Are there different types of bias to watch out for?”
- There’s design bias , where the researcher does not consider bias in the design of the study. Factors like sample size , the range of participants, for example – all of these can cause bias.
- Next there’s also selection or sampling bias . For example, you might omit people of certain ages or ethnicities from your study. This is called omission bias. The other type, inclusive bias, is when you select a sample just because it is convenient. For example, if the people you select for your study are all college students, they are likely to share many characteristics.”
“Are there more?”
“Yes, there are lots of different types of bias.
- There’s procedural bias , where the way you carry out a study affects the results. For example, if you give people only a short time to answer questions, their responses will be rushed.
- There’s also measurement bias that can happen if the equipment you are using is faulty, or you are not using it correctly.”
“That’s a lot to think about.”
“I can think of three more.
- There’s interviewer bias , which is very hard to avoid. This is when an interviewer subconsciously influences the responses of the interviewee. Their body language might indicate their opinion, for example.
- Furthermore, there’s response bias , where someone tries to give the answers they think are “correct.”
- Finally, there’s reporting bias . This is often outside the researcher’s control. It means that research with positive, or exciting, results is far more likely to be reported, so can seem more critical.”
How to Avoid Bias in Research
“With so many types of bias, how can it be avoided?”
“There are a number of things the researcher can do to avoid bias.
- Read the guidelines : Check the guidelines of your institution or sponsor and make sure you follow them.
- Think about our objectives : Plan your study early. Be clear about what you want to achieve, and how. This will help to avoid bias when you start collecting data.”
“And next?”
- Maintain records : Keep detailed records. This reduces the chance of making mistakes.
- Be honest when reporting : Make sure you include all your results in your report. Even the results that don’t seem important. Finally, be honest about the limitations of your study in your report.”
Avoiding Participant Bias
“That explains what researchers can do. But what about participant bias?”
“Try asking indirect questions. People might change their answers to direct questions to make a good impression. But if you ask them what a friend or colleague might think, you might get a more honest response.”
“Are open-ended questions useful?”
“Yes. They allow information to flow more freely, by not forcing a limited set of answers. But even these should be used with caution . You should try to be impartial about all parts of the study, and avoid implying that there is a right answer. It might help to ask people to rate their responses on a scale of 1-5, for example, rather than agree/disagree.”
Reducing Researcher Bias
“All researchers should try to avoid confirmation bias. This is when you interpret your data in a way that supports your hypothesis. Secondly, you should make sure to analyze all your data, even if it doesn’t seem useful. Finally, always get an independent person to check your work, ideally several times during your study.”
Identifying and avoiding research bias in qualitative research is clearly tricky, with many different factors to consider. However, it is also vital. Biased research has little value; it is a waste of researchers’ valuable time and resources.
Learn even more about bias here . How did you overcome bias in your research? Share your experiences and thoughts in the comment section below.

Can you please tell me who is the author of this article: How to Avoid Bias in Qualitative Research Last updated May 3, 2019
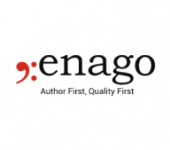
Hi Nuzhat, the author of this article is Enago Academy
Rate this article Cancel Reply
Your email address will not be published.
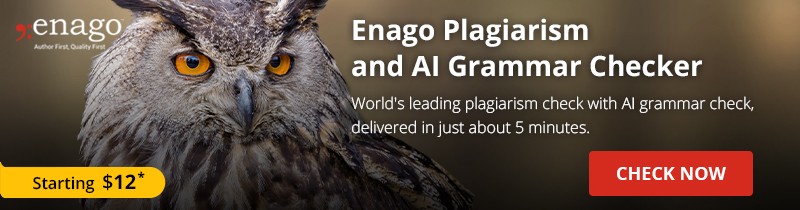
Enago Academy's Most Popular Articles
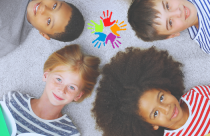
- Diversity and Inclusion
Need for Diversifying Academic Curricula: Embracing missing voices and marginalized perspectives
In classrooms worldwide, a single narrative often dominates, leaving many students feeling lost. These stories,…
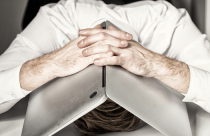
- Career Corner
- Trending Now
Recognizing the signs: A guide to overcoming academic burnout
As the sun set over the campus, casting long shadows through the library windows, Alex…
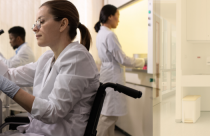
Reassessing the Lab Environment to Create an Equitable and Inclusive Space
The pursuit of scientific discovery has long been fueled by diverse minds and perspectives. Yet…
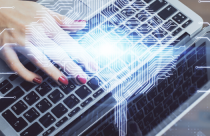
- AI in Academia
- Reporting Research
How to Improve Lab Report Writing: Best practices to follow with and without AI-assistance
Imagine you’re a scientist who just made a ground-breaking discovery! You want to share your…
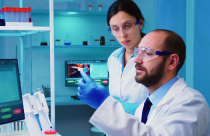
Achieving Research Excellence: Checklist for good research practices
Academia is built on the foundation of trustworthy and high-quality research, supported by the pillars…
7 Steps of Writing an Excellent Academic Book Chapter
When Your Thesis Advisor Asks You to Quit
Virtual Defense: Top 5 Online Thesis Defense Tips

Sign-up to read more
Subscribe for free to get unrestricted access to all our resources on research writing and academic publishing including:
- 2000+ blog articles
- 50+ Webinars
- 10+ Expert podcasts
- 50+ Infographics
- 10+ Checklists
- Research Guides
We hate spam too. We promise to protect your privacy and never spam you.
I am looking for Editing/ Proofreading services for my manuscript Tentative date of next journal submission:
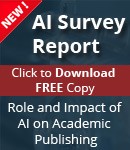
As a researcher, what do you consider most when choosing an image manipulation detector?

Research Bias 101: What You Need To Know
By: Derek Jansen (MBA) | Expert Reviewed By: Dr Eunice Rautenbach | September 2022
If you’re new to academic research, research bias (also sometimes called researcher bias) is one of the many things you need to understand to avoid compromising your study. If you’re not careful, research bias can ruin the credibility of your study.
In this post, we’ll unpack the thorny topic of research bias. We’ll explain what it is , look at some common types of research bias and share some tips to help you minimise the potential sources of bias in your research.
Overview: Research Bias 101
- What is research bias (or researcher bias)?
- Bias #1 – Selection bias
- Bias #2 – Analysis bias
- Bias #3 – Procedural (admin) bias
So, what is research bias?
Well, simply put, research bias is when the researcher – that’s you – intentionally or unintentionally skews the process of a systematic inquiry , which then of course skews the outcomes of the study . In other words, research bias is what happens when you affect the results of your research by influencing how you arrive at them.
For example, if you planned to research the effects of remote working arrangements across all levels of an organisation, but your sample consisted mostly of management-level respondents , you’d run into a form of research bias. In this case, excluding input from lower-level staff (in other words, not getting input from all levels of staff) means that the results of the study would be ‘biased’ in favour of a certain perspective – that of management.
Of course, if your research aims and research questions were only interested in the perspectives of managers, this sampling approach wouldn’t be a problem – but that’s not the case here, as there’s a misalignment between the research aims and the sample .
Now, it’s important to remember that research bias isn’t always deliberate or intended. Quite often, it’s just the result of a poorly designed study, or practical challenges in terms of getting a well-rounded, suitable sample. While perfect objectivity is the ideal, some level of bias is generally unavoidable when you’re undertaking a study. That said, as a savvy researcher, it’s your job to reduce potential sources of research bias as much as possible.
To minimize potential bias, you first need to know what to look for . So, next up, we’ll unpack three common types of research bias we see at Grad Coach when reviewing students’ projects . These include selection bias , analysis bias , and procedural bias . Keep in mind that there are many different forms of bias that can creep into your research, so don’t take this as a comprehensive list – it’s just a useful starting point.
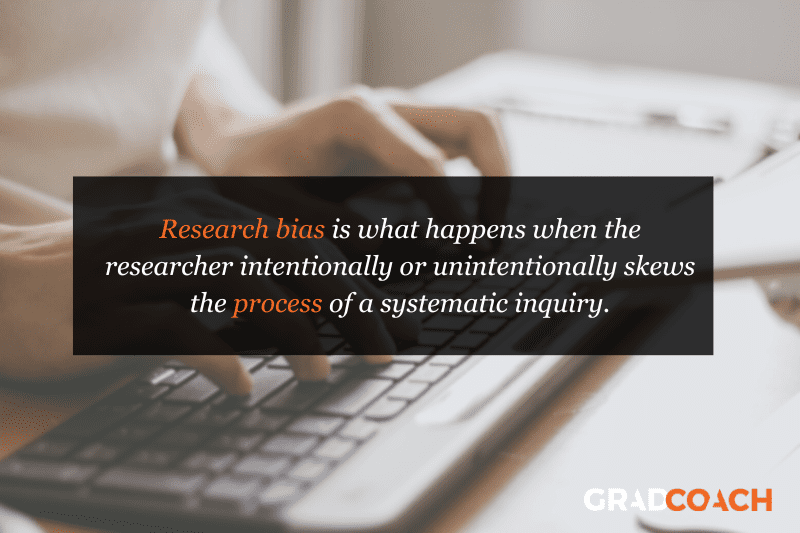
Bias #1 – Selection Bias
First up, we have selection bias . The example we looked at earlier (about only surveying management as opposed to all levels of employees) is a prime example of this type of research bias. In other words, selection bias occurs when your study’s design automatically excludes a relevant group from the research process and, therefore, negatively impacts the quality of the results.
With selection bias, the results of your study will be biased towards the group that it includes or favours, meaning that you’re likely to arrive at prejudiced results . For example, research into government policies that only includes participants who voted for a specific party is going to produce skewed results, as the views of those who voted for other parties will be excluded.
Selection bias commonly occurs in quantitative research , as the sampling strategy adopted can have a major impact on the statistical results . That said, selection bias does of course also come up in qualitative research as there’s still plenty room for skewed samples. So, it’s important to pay close attention to the makeup of your sample and make sure that you adopt a sampling strategy that aligns with your research aims. Of course, you’ll seldom achieve a perfect sample, and that okay. But, you need to be aware of how your sample may be skewed and factor this into your thinking when you analyse the resultant data.
Need a helping hand?
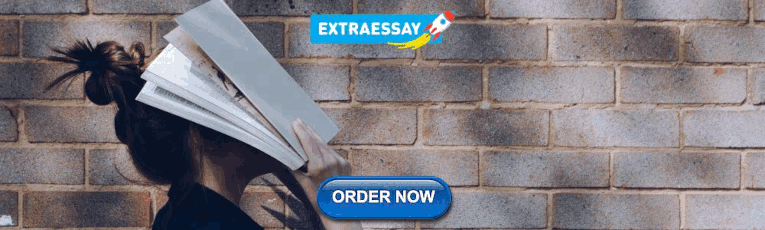
Bias #2 – Analysis Bias
Next up, we have analysis bias . Analysis bias occurs when the analysis itself emphasises or discounts certain data points , so as to favour a particular result (often the researcher’s own expected result or hypothesis). In other words, analysis bias happens when you prioritise the presentation of data that supports a certain idea or hypothesis , rather than presenting all the data indiscriminately .
For example, if your study was looking into consumer perceptions of a specific product, you might present more analysis of data that reflects positive sentiment toward the product, and give less real estate to the analysis that reflects negative sentiment. In other words, you’d cherry-pick the data that suits your desired outcomes and as a result, you’d create a bias in terms of the information conveyed by the study.
Although this kind of bias is common in quantitative research, it can just as easily occur in qualitative studies, given the amount of interpretive power the researcher has. This may not be intentional or even noticed by the researcher, given the inherent subjectivity in qualitative research. As humans, we naturally search for and interpret information in a way that confirms or supports our prior beliefs or values (in psychology, this is called “confirmation bias”). So, don’t make the mistake of thinking that analysis bias is always intentional and you don’t need to worry about it because you’re an honest researcher – it can creep up on anyone .
To reduce the risk of analysis bias, a good starting point is to determine your data analysis strategy in as much detail as possible, before you collect your data . In other words, decide, in advance, how you’ll prepare the data, which analysis method you’ll use, and be aware of how different analysis methods can favour different types of data. Also, take the time to reflect on your own pre-conceived notions and expectations regarding the analysis outcomes (in other words, what do you expect to find in the data), so that you’re fully aware of the potential influence you may have on the analysis – and therefore, hopefully, can minimize it.
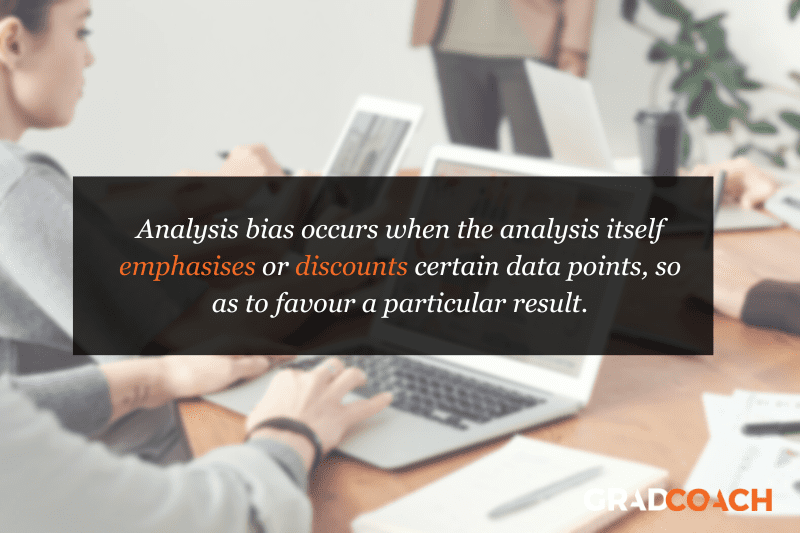
Bias #3 – Procedural Bias
Last but definitely not least, we have procedural bias , which is also sometimes referred to as administration bias . Procedural bias is easy to overlook, so it’s important to understand what it is and how to avoid it. This type of bias occurs when the administration of the study, especially the data collection aspect, has an impact on either who responds or how they respond.
A practical example of procedural bias would be when participants in a study are required to provide information under some form of constraint. For example, participants might be given insufficient time to complete a survey, resulting in incomplete or hastily-filled out forms that don’t necessarily reflect how they really feel. This can happen really easily, if, for example, you innocently ask your participants to fill out a survey during their lunch break.
Another form of procedural bias can happen when you improperly incentivise participation in a study. For example, offering a reward for completing a survey or interview might incline participants to provide false or inaccurate information just to get through the process as fast as possible and collect their reward. It could also potentially attract a particular type of respondent (a freebie seeker), resulting in a skewed sample that doesn’t really reflect your demographic of interest.
The format of your data collection method can also potentially contribute to procedural bias. If, for example, you decide to host your survey or interviews online, this could unintentionally exclude people who are not particularly tech-savvy, don’t have a suitable device or just don’t have a reliable internet connection. On the flip side, some people might find in-person interviews a bit intimidating (compared to online ones, at least), or they might find the physical environment in which they’re interviewed to be uncomfortable or awkward (maybe the boss is peering into the meeting room, for example). Either way, these factors all result in less useful data.
Although procedural bias is more common in qualitative research, it can come up in any form of fieldwork where you’re actively collecting data from study participants. So, it’s important to consider how your data is being collected and how this might impact respondents. Simply put, you need to take the respondent’s viewpoint and think about the challenges they might face, no matter how small or trivial these might seem. So, it’s always a good idea to have an informal discussion with a handful of potential respondents before you start collecting data and ask for their input regarding your proposed plan upfront.
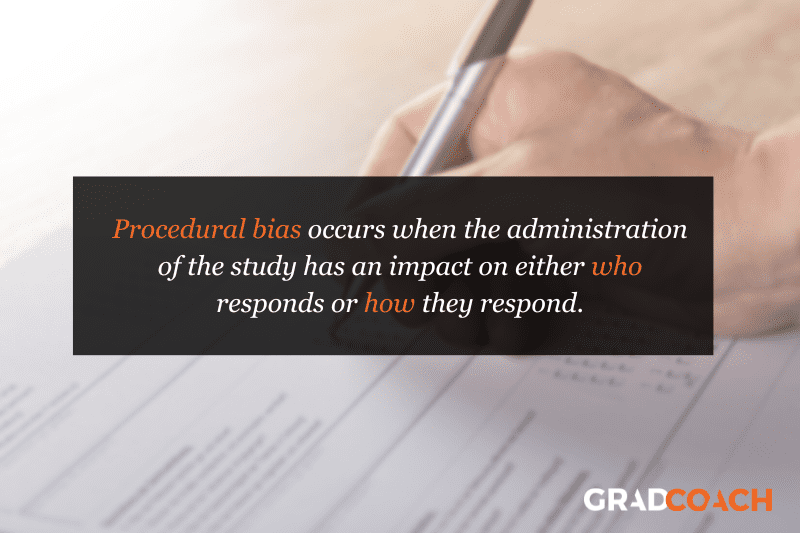
Let’s Recap
Ok, so let’s do a quick recap. Research bias refers to any instance where the researcher, or the research design , negatively influences the quality of a study’s results, whether intentionally or not.
The three common types of research bias we looked at are:
- Selection bias – where a skewed sample leads to skewed results
- Analysis bias – where the analysis method and/or approach leads to biased results – and,
- Procedural bias – where the administration of the study, especially the data collection aspect, has an impact on who responds and how they respond.
As I mentioned, there are many other forms of research bias, but we can only cover a handful here. So, be sure to familiarise yourself with as many potential sources of bias as possible to minimise the risk of research bias in your study.
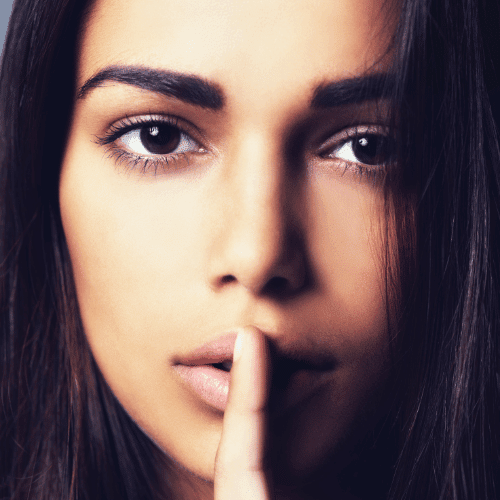
Psst... there’s more!
This post was based on one of our popular Research Bootcamps . If you're working on a research project, you'll definitely want to check this out ...
You Might Also Like:
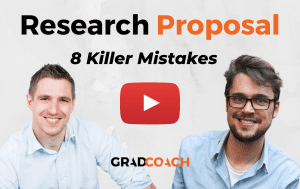
This is really educational and I really like the simplicity of the language in here, but i would like to know if there is also some guidance in regard to the problem statement and what it constitutes.
Do you have a blog or video that differentiates research assumptions, research propositions and research hypothesis?
Submit a Comment Cancel reply
Your email address will not be published. Required fields are marked *
Save my name, email, and website in this browser for the next time I comment.
- Print Friendly
The Impact of Bias on the Scholar-Practitioner's Doctoral Journey
Strategies to legitimize it.
This essay discusses the utilization of safeguard strategies, particularly Improvement Science principles, in the academic and professional writing of scholar-practitioners within EdD programs. These strategies bridge the gap between theory and practice, enabling graduate students to apply their scholarly insights meaningfully. The essay highlights the roles of bias, professional wisdom, positionality, and reflexivity in inquiry, empowering scholar-practitioners to develop authentic solutions to the problems of practice they encounter. Drawing on the recommendations of Perry and colleagues (2020), the essay emphasizes rigorous data collection, explicit theoretical frameworks, evidence of impact on practice, and transparent mitigation of biases. Strategies such as positionality and reflexivity statements, adoption of Improvement Science as a conceptual framework, critical questions as safeguards, and engagement with critical friend groups (CFG) enhance the integrity and rigor of scholar-practitioners' inquiries. By implementing these measures, scholar-practitioners foster a robust examination of problems of practice and contribute to the advancement of knowledge.
Blake, J., & Gibson, A. (2021). Critical friends group protocols deepen conversations in collaborative action research projects. Educational Action Research, 29(1), 133–148.
Bondi, L., Carr, D., Clark, C., Clegg, C. (Ed.). (2016). Towards professional wisdom: Practical deliberation in the people professions. Routledge.
Bourke, B. (2014). Positionality: Reflecting on the research process. Qualitative Report, 19(33), 1–9. https://doi.org/10.46743/2160-3715/2014.1026
Brown, B. (2010). The gifts of imperfection: Let go of who you think you’re supposed to be and embrace who you are. Simon and Schuster.
Creswell, J.W. (2014). Research design: Qualitative, quantitative, and mixed methods approaches (4th ed.). SAGE Publications, Inc.
Crow, R., Hinnant-Crawford, B.N., & Spaulding, D.T. (Eds). (2019). The educational leader’s guide to improvement science: Data, design and cases for reflection. Myers Education Press.
Dwyer, C. (2018). 12 common biases that affect how we make everyday decisions. Psychology Today. https://www.psychologytoday.com/us/blog/thoughts-on-thinking/201809/12-common-biases-that-affect-how-we-make-everyday-decisions
Grant, & Osanloo, A. (2014). Understanding, selecting, and integrating a theoretical framework in dissertation research: Creating the blueprint for your “house.” Administrative Issues Journal: Education, Practice, and Research, 4(2). https://doi.org/10.5929/2014.4.2.9
Holden Thorp, H. (2023). Put your whole self in. Science, 380(6643), 323. https://doi.org/10.1126/science.adi3753
Institute for Healthcare Improvement. (n.d.a). Science of improvement. https://www.ihi.org/about/Pages/ScienceofImprovement.aspx
Kaufman, J. C., & Beghetto, R. A. (2009). Beyond big and little: The four-c model of creativity. Review of General Psychology, 13(1), 1–12. https://doi.org/10.1037/a0013688
Kember, D., Ha, T. S., Lam, B. H., Lee, A., NG, S., Yan, L., & Yum, J. C. (1997). The diverse role of the critical friend in supporting educational action research projects. Educational Action Research, 5(3), 463–481.
Klein, W. C., & Bloom, M. (1995) Practice wisdom. Social Work, 40(6), 799–807. https://doi.org/10.1093/sw/40.6.799
Lambrev, V. (2023) Exploring the value of community-based learning in a professional doctorate: A practice theory perspective. Studies in Continuing Education, 45(1), 37–53.
Lambrev, V., & Cruz, B. C. (2021) Becoming scholarly practitioners: Creating community in online professional doctoral education. Distance Education, 42(4), 567–581. https://doi.org/10.1080/01587919.2021.1986374
Langley, G. J., Moen, R. D., Nolan, K. M., Nolan, T. W., Norman, C. L., & Provost, L. P. (2009). The improvement guide: A practical approach to enhancing organizational performance. John Wiley & Sons.
McNiff, J. (2008). The significance of “I” in educational research and the responsibility of intellectuals. South African Journal of Education, 28(3), 351–364. https://doi.org/10.15700/saje.v28n3a178
McNiff, J., & Whitehead, J. (2009). Doing and writing action research. SAGE Publications.
Mattoon, M. & McKean, E. (2020). Critical friends group® purpose & work. National school reform faculty. https://nsrfharmony.org/wp-content/uploads/2021/03/cfg_purpose_work_1-3.pdf
Noor, M. S. A. M., & Shafee, A. (2021). The role of critical friends in action research: A framework for design and implementation. Practitioner Research, 3, 1–33.
Pape, S. J., Bryant, C. L., JohnBull, R. M., Karp, K. S. (2022). Improvement science as a frame for the dissertation in practice: The John’s Hopkins experience. Impacting Education: Journal on Transforming Professional Practice, 7(1), 59–66. https://doi.org/10.5195/ie.2022.241
Perry, J. A. (Ed.). (2016). The EdD and the scholarly practitioner. IAP.
Perry, J. A., Zambo, D., & Crow, R. (2020). The improvement science dissertation in practice: A guide for faculty, committee members, and their students. Myers Education Press.
Popper, K. R. (1979). Objective knowledge: An evolutionary approach (Rev. ed.). Oxford University Press.
Popovic, A., & Huecker, M. R. (2022). Study bias. StatPearls Publishing. https://pubmed.ncbi.nlm.nih.gov/34662027
Schutte, N. S., & Malouff, J. M. (2020a). A meta‐analysis of the relationship between curiosity and creativity. The Journal of Creative Behavior, 54(4), 940–947.
Schutte, N. S., & Malouff, J. M. (2020b). Connections between curiosity, flow and creativity. Personality and Individual Differences, 152. https://doi.org/10.1016/j.paid.2019.109555
Smith, L. T. (2021). Decolonizing methodologies: Research and indigenous peoples (3rd edition). Zed Books. https://doi.org/10.5040/9781350225282
Son Hing, L. (2022). The myth of meritocracy in scientific institutions. Science, 377(6608), 824. https://www.science.org/doi/10.1126/science.add5909
Vassallo, P. (2004). Getting started with evaluation reports: Creating the structure. ETC: A Review of General Semantics, 61(3), 398–403.
How to Cite
- Endnote/Zotero/Mendeley (RIS)
Copyright (c) 2024 Laura M. Rodriguez López
This work is licensed under a Creative Commons Attribution 4.0 International License .
Authors who publish with this journal agree to the following terms:
- The Author retains copyright in the Work, where the term “Work” shall include all digital objects that may result in subsequent electronic publication or distribution.
- Upon acceptance of the Work, the author shall grant to the Publisher the right of first publication of the Work.
- Attribution—other users must attribute the Work in the manner specified by the author as indicated on the journal Web site;
- The Author is able to enter into separate, additional contractual arrangements for the nonexclusive distribution of the journal's published version of the Work (e.g., post it to an institutional repository or publish it in a book), as long as there is provided in the document an acknowledgement of its initial publication in this journal.
- Authors are permitted and encouraged to post online a prepublication manuscript (but not the Publisher’s final formatted PDF version of the Work) in institutional repositories or on their Websites prior to and during the submission process, as it can lead to productive exchanges, as well as earlier and greater citation of published work. Any such posting made before acceptance and publication of the Work shall be updated upon publication to include a reference to the Publisher-assigned DOI (Digital Object Identifier) and a link to the online abstract for the final published Work in the Journal.
- Upon Publisher’s request, the Author agrees to furnish promptly to Publisher, at the Author’s own expense, written evidence of the permissions, licenses, and consents for use of third-party material included within the Work, except as determined by Publisher to be covered by the principles of Fair Use.
- the Work is the Author’s original work;
- the Author has not transferred, and will not transfer, exclusive rights in the Work to any third party;
- the Work is not pending review or under consideration by another publisher;
- the Work has not previously been published;
- the Work contains no misrepresentation or infringement of the Work or property of other authors or third parties; and
- the Work contains no libel, invasion of privacy, or other unlawful matter.
- The Author agrees to indemnify and hold Publisher harmless from Author’s breach of the representations and warranties contained in Paragraph 6 above, as well as any claim or proceeding relating to Publisher’s use and publication of any content contained in the Work, including third-party content.
Revised 7/16/2018. Revision Description: Removed outdated link.
Make a Submission
ISSN 2472-5889 (online)
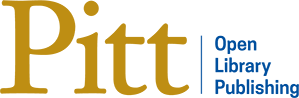
May 4, 2024
Implicit Bias Hurts Everyone. Here’s How to Overcome It
The environment shapes stereotypes and biases, but it is possible to recognize and change them
By Corey S. Powell & OpenMind Magazine
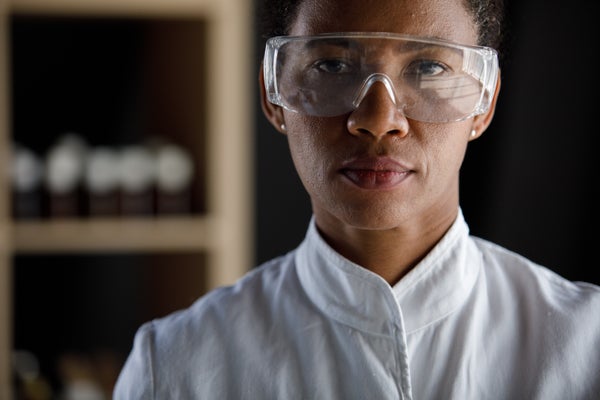
fotostorm/Getty Images
We all have a natural tendency to view the world in black and white—to the extent that it's hard not to hear "black" and immediately think "white." Fortunately, there are ways to activate the more subtle shadings in our minds. Kristin Pauker is a professor of psychology at the University of Hawaiʻi at Mānoa who studies stereotyping and prejudice, with a focus on how our environment shapes our biases. In this podcast and Q&A, she tells OpenMind co-editor Corey S. Powell how researchers measure and study bias, and how we can use their findings to make a more equitable world. (This conversation has been edited for length and clarity.)
When I hear “bias,” the first thing I think of is a conscious prejudice. But you study something a lot more subtle, which researchers call “implicit bias.” What is it, and how does it affect us?
Implicit bias is a form of bias that influences our decision-making, our interactions and our behaviors. It can be based on any social group membership, like race, gender, age, sexual orientation or even the color of your shirt. Often we’re not aware of the ways in which these biases are influencing us. Sometimes implicit bias gets called unconscious bias, which is a little bit of a misnomer. We can be aware of these biases, so it's not necessarily unconscious. But we often are not aware of the way in which they're influencing our behaviors and thoughts.
On supporting science journalism
If you're enjoying this article, consider supporting our award-winning journalism by subscribing . By purchasing a subscription you are helping to ensure the future of impactful stories about the discoveries and ideas shaping our world today.
You make it sound like almost anything can set us off. Why is bias so deeply ingrained in our heads?
Our brain likes to categorize things because it makes our world easier to process. We make categories as soon as we start learning about something. So we categorize fruits, we categorize vegetables, we categorize chairs, we categorize tables for their function—and we also categorize people. We know from research that categorization happens early in life, as early as 5 or 6, in some cases even 3 or 4. Categorization creates shortcuts that help us process information faster, but that also can lead us to make assumptions that may or may not hold in particular situations. What categories we use are directed by the environment that we're in. Our environment already has told us certain categories are really important, such as gender, age, race and ethnicity. We quickly form an association when we’re assigned to a particular group.
Listen to the Podcast
Kristin Pauker: We have to think about ways in which we can change the features of our environment—so that our weeds aren’t so prolific.
In your research, you use a diagnostic tool called an “ implicit association test .” How does it work, and what does it tell you?
Typically someone would show you examples of individuals who belong to categories, and then ask you to categorize those individuals. For example, you would see faces and you would categorize them as black and white. You’re asked to make a fast categorization, as fast as you can. Then you are presented with words that could be categorized as good or bad, like “hero” and “evil,” and again asked to categorize the words quickly. The complicated part happens when, say, good and white are paired together or bad and black are paired together. You're asked to categorize the faces and the words as you were before. Then it's flipped, so that bad and white are paired together, and good and black are paired together. You’re asked to make the categorizations once again with the new pairings.
The point of the test is, how quickly do you associate certain concepts together? Oftentimes if certain concepts are more closely paired in your mind, then it will be easier for you to make that association. Your response will be faster. When the pairing is less familiar to you or less closely associated, it takes you longer to respond. Additional processing needs to occur.
When you run this implicit association test on your test subjects or your students, are they often surprised by the results?
We’ve done it as a demonstration in the classroom, and I've had students come up and complain saying, “There’s something wrong with this test. I don't believe it.” They’ll try to poke all kinds of holes in the test because it gave them a score that wasn’t what they felt it should be according to what they think about themselves. This is the case, I think, for almost anyone. I've taken an implicit association test and found that I have a stronger association with men in science than women in science . And I'm a woman scientist! We can have and hold these biases because they’re prevalent in society, even if they’re biases that may not be beneficial to the group we belong to.
Studies show that even after you make people aware of their implicit biases, they can’t necessarily get rid of them. So are we stuck with our biases?
Those biases are hard to change and control, but that doesn't mean that they are un controllable and un changeable. It’s just that oftentimes there are many features in our environment that reinforce those biases. I was thinking about an analogy. Right now I’m struggling with weeds growing in my yard, invasive vines. It’s hard because there are so many things supporting the growth of these vines. I live in a place that has lots of sun and rain. Similarly, there’s so much in our environment that is supporting our biases. It’s hard to just cut them off and be like, OK, they're gone. We have to think about ways in which we can change the features of our environment—so that our weeds aren’t so prolific.
Common programs aimed at reducing bias, such as corporate diversity training workshops, often seem to stop at the stage of making people aware that bias exists. Is that why they haven’t worked very well ?
If people are told that they’re biased, the reaction that many of them have is, “Oh, that means I'm a racist? I'm not a racist!” Very defensive, because we associate this idea of being biased with a moral judgment that I'm a bad person. Because of that, awareness-raising can have the opposite of the intended effect. Being told that they're biased can make people worried and defensive, and they push back against that idea. They're not willing to accept it.
A lot of the diversity training models are based on the idea that you can just tell people about their biases and then get them to accept them and work on them. But, A, some people don't want to accept their biases. B, some people don't want to work on them. And C, the messaging around how we talk about these biases creates a misunderstanding that they can’t be changed. We talk about biases that are unconscious, biases that we all hold, that are formed early in life—it creates the idea, “Well, there’s nothing I can do, so why should I even try?”
How can we do better in talking about bias, so that people are more likely to embrace change instead of becoming defensive or defeated?
Some of it is about messaging. Biases are hard to change, but we should be discussing the ways in which these biases can change, even though it might take some time and work. You have to emphasize the idea that these things can change, or else why would we try? There is research showing that if you just give people their bias score, normally that doesn't result in them becoming more aware of their bias. But if you combine that score with a message that this is something controllable, people are less defensive and more willing to accept their biases.
What about concrete actions we can take to reduce the negative impact of implicit bias?
One thing is thinking about when we do interventions. A lot of times we’re trying to make changes in the workplace. We should be thinking more about how we're raising our children. The types of environments we're exposing them to, and the features that are in our schools , are good places to think about creating change. Prejudice is something that’s malleable.
Another thing is not always focusing on the person. So much of what we do in these interventions is try to change individual people's biases. But we can also think about our environment. What are the ways in which our environments are communicating these biases, and how can we make changes there? A clever idea people have been thinking about is trying to change consequences of biases. There's a researcher, Jason A. Okonofua , who talks about this and calls it “sidelining bias.” You're not targeting the person and trying to get rid of their biases. You're targeting the situations that support those biases. If you can change that situation and kind of cut it off, then the consequences of bias might not be as bad. It could lead to a judgment that is not so influenced by those biases.
There’s research showing that people make fairer hiring decisions when they work off tightly structured interviews and qualification checklists, which leave less room for subjective reactions. Is that the kind of “sidelining” strategy you’re talking about?
Yes, that’s been shown to be an effective way to sideline bias. If you set those criteria ahead of time, it's harder for you to shift a preference based on the person that you would like to hire. Another good example is finding ways to slow down the processes we're working on. Biases are more likely to influence our decision-making when we have to make really quick decisions or when we are stressed—which is the case for a lot of important decisions that we make.
Jennifer Eberhardt does research on these kinds of implicit biases. She worked with NextDoor (a neighborhood monitoring app) when they noticed a lot of racial profiling in the things people were reporting in their neighborhood. She worked with them to change the way that people report a suspicious person. Basically they added some extra steps to the checklist when you report something. Rather than just reporting that someone looks suspicious, a user had to indicate what about the behavior itself was suspicious. And then there was an explicit warning that they couldn't just say the reason for the suspicious behavior was someone's race. Including extra check steps slowed down the process and reduced the profiling.
It does feel like we’re making progress in addressing bias but, damn, it’s been a slow process. Where can we go from here?
A big part that’s missing in the research on implicit bias is creating tools that are useful for people. We still don’t know a lot about bias, but we know a lot more than we're willing to put into practice. For instance, creating resources for parents to be able to have conversations about bias , and to be aware that the everyday things we do are really important. This is something that many people want to tackle, but they don’t know how to do it. Just asking questions about what is usual and what is unusual has really interesting effects. We’ve done that with our son. He’d say something and I would ask, “Why is that something that only boys can do? You say girls can't do that, is that really the case? Can you think of examples where the opposite is true?”
This Q&A is part of a series of OpenMind essays, podcasts and videos supported by a generous grant from the Pulitzer Center 's Truth Decay initiative.
This story originally appeared on OpenMind , a digital magazine tackling science controversies and deceptions.

An official website of the United States government
The .gov means it’s official. Federal government websites often end in .gov or .mil. Before sharing sensitive information, make sure you’re on a federal government site.
The site is secure. The https:// ensures that you are connecting to the official website and that any information you provide is encrypted and transmitted securely.
- Publications
- Account settings
Preview improvements coming to the PMC website in October 2024. Learn More or Try it out now .
- Advanced Search
- Journal List

Protecting against researcher bias in secondary data analysis: challenges and potential solutions
Jessie r. baldwin.
1 Department of Clinical, Educational and Health Psychology, Division of Psychology and Language Sciences, University College London, London, WC1H 0AP UK
2 Social, Genetic and Developmental Psychiatry Centre, Institute of Psychiatry, Psychology and Neuroscience, King’s College London, London, UK
Jean-Baptiste Pingault
Tabea schoeler, hannah m. sallis.
3 MRC Integrative Epidemiology Unit at the University of Bristol, Bristol Medical School, University of Bristol, Bristol, UK
4 School of Psychological Science, University of Bristol, Bristol, UK
5 Centre for Academic Mental Health, Population Health Sciences, University of Bristol, Bristol, UK
Marcus R. Munafò
6 NIHR Biomedical Research Centre, University Hospitals Bristol NHS Foundation Trust and University of Bristol, Bristol, UK
Analysis of secondary data sources (such as cohort studies, survey data, and administrative records) has the potential to provide answers to science and society’s most pressing questions. However, researcher biases can lead to questionable research practices in secondary data analysis, which can distort the evidence base. While pre-registration can help to protect against researcher biases, it presents challenges for secondary data analysis. In this article, we describe these challenges and propose novel solutions and alternative approaches. Proposed solutions include approaches to (1) address bias linked to prior knowledge of the data, (2) enable pre-registration of non-hypothesis-driven research, (3) help ensure that pre-registered analyses will be appropriate for the data, and (4) address difficulties arising from reduced analytic flexibility in pre-registration. For each solution, we provide guidance on implementation for researchers and data guardians. The adoption of these practices can help to protect against researcher bias in secondary data analysis, to improve the robustness of research based on existing data.
Introduction
Secondary data analysis has the potential to provide answers to science and society’s most pressing questions. An abundance of secondary data exists—cohort studies, surveys, administrative data (e.g., health records, crime records, census data), financial data, and environmental data—that can be analysed by researchers in academia, industry, third-sector organisations, and the government. However, secondary data analysis is vulnerable to questionable research practices (QRPs) which can distort the evidence base. These QRPs include p-hacking (i.e., exploiting analytic flexibility to obtain statistically significant results), selective reporting of statistically significant, novel, or “clean” results, and hypothesising after the results are known (HARK-ing [i.e., presenting unexpected results as if they were predicted]; [ 1 ]. Indeed, findings obtained from secondary data analysis are not always replicable [ 2 , 3 ], reproducible [ 4 ], or robust to analytic choices [ 5 , 6 ]. Preventing QRPs in research based on secondary data is therefore critical for scientific and societal progress.
A primary cause of QRPs is common cognitive biases that affect the analysis, reporting, and interpretation of data [ 7 – 10 ]. For example, apophenia (the tendency to see patterns in random data) and confirmation bias (the tendency to focus on evidence that is consistent with one’s beliefs) can lead to particular analytical choices and selective reporting of “publishable” results [ 11 – 13 ]. In addition, hindsight bias (the tendency to view past events as predictable) can lead to HARK-ing, so that observed results appear more compelling.
The scope for these biases to distort research outputs from secondary data analysis is perhaps particularly acute, for two reasons. First, researchers now have increasing access to high-dimensional datasets that offer a multitude of ways to analyse the same data [ 6 ]. Such analytic flexibility can lead to different conclusions depending on the analytical choices made [ 5 , 14 , 15 ]. Second, current incentive structures in science reward researchers for publishing statistically significant, novel, and/or surprising findings [ 16 ]. This combination of opportunity and incentive may lead researchers—consciously or unconsciously—to run multiple analyses and only report the most “publishable” findings.
One way to help protect against the effects of researcher bias is to pre-register research plans [ 17 , 18 ]. This can be achieved by pre-specifying the rationale, hypotheses, methods, and analysis plans, and submitting these to either a third-party registry (e.g., the Open Science Framework [OSF]; https://osf.io/ ), or a journal in the form of a Registered Report [ 19 ]. Because research plans and hypotheses are specified before the results are known, pre-registration reduces the potential for cognitive biases to lead to p-hacking, selective reporting, and HARK-ing [ 20 ]. While pre-registration is not necessarily a panacea for preventing QRPs (Table (Table1), 1 ), meta-scientific evidence has found that pre-registered studies and Registered Reports are more likely to report null results [ 21 – 23 ], smaller effect sizes [ 24 ], and be replicated [ 25 ]. Pre-registration is increasingly being adopted in epidemiological research [ 26 , 27 ], and is even required for access to data from certain cohorts (e.g., the Twins Early Development Study [ 28 ]). However, pre-registration (and other open science practices; Table Table2) 2 ) can pose particular challenges to researchers conducting secondary data analysis [ 29 ], motivating the need for alternative approaches and solutions. Here we describe such challenges, before proposing potential solutions to protect against researcher bias in secondary data analysis (summarised in Fig. 1 ).
Limitations in the use of pre-registration to address QRPs
Challenges and potential solutions regarding sharing pre-existing data

Challenges in pre-registering secondary data analysis and potential solutions (according to researcher motivations). Note : In the “Potential solution” column, blue boxes indicate solutions that are researcher-led; green boxes indicate solutions that should be facilitated by data guardians
Challenges of pre-registration for secondary data analysis
Prior knowledge of the data.
Researchers conducting secondary data analysis commonly analyse data from the same dataset multiple times throughout their careers. However, prior knowledge of the data increases risk of bias, as prior expectations about findings could motivate researchers to pursue certain analyses or questions. In the worst-case scenario, a researcher might perform multiple preliminary analyses, and only pursue those which lead to notable results (perhaps posting a pre-registration for these analyses, even though it is effectively post hoc). However, even if the researcher has not conducted specific analyses previously, they may be biased (either consciously or subconsciously) to pursue certain analyses after testing related questions with the same variables, or even by reading past studies on the dataset. As such, pre-registration cannot fully protect against researcher bias when researchers have previously accessed the data.
Research may not be hypothesis-driven
Pre-registration and Registered Reports are tailored towards hypothesis-driven, confirmatory research. For example, the OSF pre-registration template requires researchers to state “specific, concise, and testable hypotheses”, while Registered Reports do not permit purely exploratory research [ 30 ], although a new Exploratory Reports format now exists [ 31 ]. However, much research involving secondary data is not focused on hypothesis testing, but is exploratory, descriptive, or focused on estimation—in other words, examining the magnitude and robustness of an association as precisely as possible, rather than simply testing a point null. Furthermore, without a strong theoretical background, hypotheses will be arbitrary and could lead to unhelpful inferences [ 32 , 33 ], and so should be avoided in novel areas of research.
Pre-registered analyses are not appropriate for the data
With pre-registration, there is always a risk that the data will violate the assumptions of the pre-registered analyses [ 17 ]. For example, a researcher might pre-register a parametric test, only for the data to be non-normally distributed. However, in secondary data analysis, the extent to which the data shape the appropriate analysis can be considerable. First, longitudinal cohort studies are often subject to missing data and attrition. Approaches to deal with missing data (e.g., listwise deletion; multiple imputation) depend on the characteristics of missing data (e.g., the extent and patterns of missingness [ 34 ]), and so pre-specifying approaches to dealing with missingness may be difficult, or extremely complex. Second, certain analytical decisions depend on the nature of the observed data (e.g., the choice of covariates to include in a multiple regression might depend on the collinearity between the measures, or the degree of missingness of different measures that capture the same construct). Third, much secondary data (e.g., electronic health records and other administrative data) were never collected for research purposes, so can present several challenges that are impossible to predict in advance [ 35 ]. These issues can limit a researcher’s ability to pre-register a precise analytic plan prior to accessing secondary data.
Lack of flexibility in data analysis
Concerns have been raised that pre-registration limits flexibility in data analysis, including justifiable exploration [ 36 – 38 ]. For example, by requiring researchers to commit to a pre-registered analysis plan, pre-registration could prevent researchers from exploring novel questions (with a hypothesis-free approach), conducting follow-up analyses to investigate notable findings [ 39 ], or employing newly published methods with advantages over those pre-registered. While this concern is also likely to apply to primary data analysis, it is particularly relevant to certain fields involving secondary data analysis, such as genetic epidemiology, where new methods are rapidly being developed [ 40 ], and follow-up analyses are often required (e.g., in a genome-wide association study to further investigate the role of a genetic variant associated with a phenotype). However, this concern is perhaps over-stated – pre-registration does not preclude unplanned analyses; it simply makes it more transparent that these analyses are post hoc. Nevertheless, another understandable concern is that reduced analytic flexibility could lead to difficulties in publishing papers and accruing citations. For example, pre-registered studies are more likely to report null results [ 22 , 23 ], likely due to reduced analytic flexibility and selective reporting. While this is a positive outcome for research integrity, null results are less likely to be published [ 13 , 41 , 42 ] and cited [ 11 ], which could disadvantage researchers’ careers.
In this section, we describe potential solutions to address the challenges involved in pre-registering secondary data analysis, including approaches to (1) address bias linked to prior knowledge of the data, (2) enable pre-registration of non-hypothesis-driven research, (3) ensure that pre-planned analyses will be appropriate for the data, and (4) address potential difficulties arising from reduced analytic flexibility.
Challenge: Prior knowledge of the data
Declare prior access to data.
To increase transparency about potential biases arising from knowledge of the data, researchers could routinely report all prior data access in a pre-registration [ 29 ]. This would ideally include evidence from an independent gatekeeper (e.g., a data guardian of the study) stating whether data and relevant variables were accessed by each co-author. To facilitate this process, data guardians could set up a central “electronic checkout” system that records which researchers have accessed data, what data were accessed, and when [ 43 ]. The researcher or data guardian could then provide links to the checkout histories for all co-authors in the pre-registration, to verify their prior data access. If it is not feasible to provide such objective evidence, authors could self-certify their prior access to the dataset and where possible, relevant variables—preferably listing any publications and in-preparation studies based on the dataset [ 29 ]. Of course, self-certification relies on trust that researchers will accurately report prior data access, which could be challenging if the study involves a large number of authors, or authors who have been involved on many studies on the dataset. However, it is likely to be the most feasible option at present as many datasets do not have available electronic records of data access. For further guidance on self-certifying prior data access when pre-registering secondary data analysis studies on a third-party registry (e.g., the OSF), we recommend referring to the template by Van den Akker, Weston [ 29 ].
The extent to which prior access to data renders pre-registration invalid is debatable. On the one hand, even if data have been accessed previously, pre-registration is likely to reduce QRPs by encouraging researchers to commit to a pre-specified analytic strategy. On the other hand, pre-registration does not fully protect against researcher bias where data have already been accessed, and can lend added credibility to study claims, which may be unfounded. Reporting prior data access in a pre-registration is therefore important to make these potential biases transparent, so that readers and reviewers can judge the credibility of the findings accordingly. However, for a more rigorous solution which protects against researcher bias in the context of prior data access, researchers should consider adopting a multiverse approach.
Conduct a multiverse analysis
A multiverse analysis involves identifying all potential analytic choices that could justifiably be made to address a given research question (e.g., different ways to code a variable, combinations of covariates, and types of analytic model), implementing them all, and reporting the results [ 44 ]. Notably, this method differs from the traditional approach in which findings from only one analytic method are reported. It is conceptually similar to a sensitivity analysis, but it is far more comprehensive, as often hundreds or thousands of analytic choices are reported, rather than a handful. By showing the results from all defensible analytic approaches, multiverse analysis reduces scope for selective reporting and provides insight into the robustness of findings against analytical choices (for example, if there is a clear convergence of estimates, irrespective of most analytical choices). For causal questions in observational research, Directed Acyclic Graphs (DAGs) could be used to inform selection of covariates in multiverse approaches [ 45 ] (i.e., to ensure that confounders, rather than mediators or colliders, are controlled for).
Specification curve analysis [ 46 ] is a form of multiverse analysis that has been applied to examine the robustness of epidemiological findings to analytic choices [ 6 , 47 ]. Specification curve analysis involves three steps: (1) identifying all analytic choices – termed “specifications”, (2) displaying the results graphically with magnitude of effect size plotted against analytic choice, and (3) conducting joint inference across all results. When applied to the association between digital technology use and adolescent well-being [ 6 ], specification curve analysis showed that the (small, negative) association diminished after accounting for adequate control variables and recall bias – demonstrating the sensitivity of results to analytic choices.
Despite the benefits of the multiverse approach in addressing analytic flexibility, it is not without limitations. First, because each analytic choice is treated as equally valid, including less justifiable models could bias the results away from the truth. Second, the choice of specifications can be biased by prior knowledge (e.g., a researcher may choose to omit a covariate to obtain a particular result). Third, multiverse analysis may not entirely prevent selective reporting (e.g., if the full range of results are not reported), although pre-registering multiverse approaches (and specifying analytic choices) could mitigate this. Last, and perhaps most importantly, multiverse analysis is technically challenging (e.g., when there are hundreds or thousands of analytic choices) and can be impractical for complex analyses, very large datasets, or when computational resources are limited. However, this burden can be somewhat reduced by tutorials and packages which are being developed to standardise the procedure and reduce computational time [see 48 , 49 ].
Challenge: Research may not be hypothesis-driven
Pre-register research questions and conditions for interpreting findings.
Observational research arguably does not need to have a hypothesis to benefit from pre-registration. For studies that are descriptive or focused on estimation, we recommend pre-registering research questions, analysis plans, and criteria for interpretation. Analytic flexibility will be limited by pre-registering specific research questions and detailed analysis plans, while post hoc interpretation will be limited by pre-specifying criteria for interpretation [ 50 ]. The potential for HARK-ing will also be minimised because readers can compare the published study to the original pre-registration, where a-priori hypotheses were not specified.
Detailed guidance on how to pre-register research questions and analysis plans for secondary data is provided in Van den Akker’s [ 29 ] tutorial. To pre-specify conditions for interpretation, it is important to anticipate – as much as possible – all potential findings, and state how each would be interpreted. For example, suppose that a researcher aims to test a causal relationship between X and Y using a multivariate regression model with longitudinal data. Assuming that all potential confounders have been fully measured and controlled for (albeit a strong assumption) and statistical power is high, three broad sets of results and interpretations could be pre-specified. First, an association between X and Y that is similar in magnitude to the unadjusted association would be consistent with a causal relationship. Second, an association between X and Y that is attenuated after controlling for confounders would suggest that the relationship is partly causal and partly confounded. Third, a minimal, non-statistically significant adjusted association would suggest a lack of evidence for a causal effect of X on Y. Depending on the context of the study, criteria could also be provided on the threshold (or range of thresholds) at which the effect size would justify different interpretations [ 51 ], be considered practically meaningful, or the smallest effect size of interest for equivalence tests [ 52 ]. While researcher biases might still affect the pre-registered criteria for interpreting findings (e.g., toward over-interpreting a small effect size as meaningful), this bias will at least be transparent in the pre-registration.
Use a holdout sample to delineate exploratory and confirmatory research
Where researchers wish to integrate exploratory research into a pre-registered, confirmatory study, a holdout sample approach can be used [ 18 ]. Creating a holdout sample refers to the process of randomly splitting the dataset into two parts, often referred to as ‘training’ and ‘holdout’ datasets. To delineate exploratory and confirmatory research, researchers can first conduct exploratory data analysis on the training dataset (which should comprise a moderate fraction of the data, e.g., 35% [ 53 ]. Based on the results of the discovery process, researchers can pre-register hypotheses and analysis plans to formally test on the holdout dataset. This process has parallels with cross-validation in machine learning, in which the dataset is split and the model is developed on the training dataset, before being tested on the test dataset. The approach enables a flexible discovery process, before formally testing discoveries in a non-biased way.
When considering whether to use the holdout sample approach, three points should be noted. First, because the training dataset is not reusable, there will be a reduced sample size and loss of power relative to analysing the whole dataset. As such, the holdout sample approach will only be appropriate when the original dataset is large enough to provide sufficient power in the holdout dataset. Second, when the training dataset is used for exploration, subsequent confirmatory analyses on the holdout dataset may be overfitted (due to both datasets being drawn from the same sample), so replication in independent samples is recommended. Third, the holdout dataset should be created by an independent data manager or guardian, to ensure that the researcher does not have knowledge of the full dataset. However, it is straightforward to randomly split a dataset into a holdout and training sample and we provide example R code at: https://github.com/jr-baldwin/Researcher_Bias_Methods/blob/main/Holdout_script.md .
Challenge: Pre-registered analyses are not appropriate for the data
Use blinding to test proposed analyses.
One method to help ensure that pre-registered analyses will be appropriate for the data is to trial the analyses on a blinded dataset [ 54 ], before pre-registering. Data blinding involves obscuring the data values or labels prior to data analysis, so that the proposed analyses can be trialled on the data without observing the actual findings. Various types of blinding strategies exist [ 54 ], but one method that is appropriate for epidemiological data is “data scrambling” [ 55 ]. This involves randomly shuffling the data points so that any associations between variables are obscured, whilst the variable distributions (and amounts of missing data) remain the same. We provide a tutorial for how to implement this in R (see https://github.com/jr-baldwin/Researcher_Bias_Methods/blob/main/Data_scrambling_tutorial.md ). Ideally the data scrambling would be done by a data guardian who is independent of the research, to ensure that the main researcher does not access the data prior to pre-registering the analyses. Once the researcher is confident with the analyses, the study can be pre-registered, and the analyses conducted on the unscrambled dataset.
Blinded analysis offers several advantages for ensuring that pre-registered analyses are appropriate, with some limitations. First, blinded analysis allows researchers to directly check the distribution of variables and amounts of missingness, without having to make assumptions about the data that may not be met, or spend time planning contingencies for every possible scenario. Second, blinded analysis prevents researchers from gaining insight into the potential findings prior to pre-registration, because associations between variables are masked. However, because of this, blinded analysis does not enable researchers to check for collinearity, predictors of missing data, or other covariances that may be necessary for model specification. As such, blinded analysis will be most appropriate for researchers who wish to check the data distribution and amounts of missingness before pre-registering.
Trial analyses on a dataset excluding the outcome
Another method to help ensure that pre-registered analyses will be appropriate for the data is to trial analyses on a dataset excluding outcome data. For example, data managers could provide researchers with part of the dataset containing the exposure variable(s) plus any covariates and/or auxiliary variables. The researcher can then trial and refine the analyses ahead of pre-registering, without gaining insight into the main findings (which require the outcome data). This approach is used to mitigate bias in propensity score matching studies [ 26 , 56 ], as researchers use data on the exposure and covariates to create matched groups, prior to accessing any outcome data. Once the exposed and non-exposed groups have been matched effectively, researchers pre-register the protocol ahead of viewing the outcome data. Notably though, this approach could help researchers to identify and address other analytical challenges involving secondary data. For example, it could be used to check multivariable distributional characteristics, test for collinearity between multiple predictor variables, or identify predictors of missing data for multiple imputation.
This approach offers certain benefits for researchers keen to ensure that pre-registered analyses are appropriate for the observed data, with some limitations. Regarding benefits, researchers will be able to examine associations between variables (excluding the outcome), unlike the data scrambling approach described above. This would be helpful for checking certain assumptions (e.g., collinearity or characteristics of missing data such as whether it is missing at random). In addition, the approach is easy to implement, as the dataset can be initially created without the outcome variable, which can then be added after pre-registration, minimising burden on data guardians. Regarding limitations, it is possible that accessing variables in advance could provide some insight into the findings. For example, if a covariate is known to be highly correlated with the outcome, testing the association between the covariate and the exposure could give some indication of the relationship between the exposure and the outcome. To make this potential bias transparent, researchers should report the variables that they already accessed in the pre-registration. Another limitation is that researchers will not be able to identify analytical issues relating to the outcome data in advance of pre-registration. Therefore, this approach will be most appropriate where researchers wish to check various characteristics of the exposure variable(s) and covariates, rather than the outcome. However, a “mixed” approach could be applied in which outcome data is provided in scrambled format, to enable researchers to also assess distributional characteristics of the outcome. This would substantially reduce the number of potential challenges to be considered in pre-registered analytical pipelines.
Pre-register a decision tree
If it is not possible to access any of the data prior to pre-registering (e.g., to enable analyses to be trialled on a dataset that is blinded or missing outcome data), researchers could pre-register a decision tree. This defines the sequence of analyses and rules based on characteristics of the observed data [ 17 ]. For example, the decision tree could specify testing a normality assumption, and based on the results, whether to use a parametric or non-parametric test. Ideally, the decision tree should provide a contingency plan for each of the planned analyses, if assumptions are not fulfilled. Of course, it can be challenging and time consuming to anticipate every potential issue with the data and plan contingencies. However, investing time into pre-specifying a decision tree (or a set of contingency plans) could save time should issues arise during data analysis, and can reduce the likelihood of deviating from the pre-registration.
Challenge: Lack of flexibility in data analysis
Transparently report unplanned analyses.
Unplanned analyses (such as applying new methods or conducting follow-up tests to investigate an interesting or unexpected finding) are a natural and often important part of the scientific process. Despite common misconceptions, pre-registration does not permit such unplanned analyses from being included, as long as they are transparently reported as post-hoc. If there are methodological deviations, we recommend that researchers should (1) clearly state the reasons for using the new method, and (2) if possible, report results from both methods, to ideally show that the change in methods was not due to the results [ 57 ]. This information can either be provided in the manuscript or in an update to the original pre-registration (e.g., on the third-party registry such as the OSF), which can be useful when journal word limits are tight. Similarly, if researchers wish to include additional follow-up analyses to investigate an interesting or unexpected finding, this should be reported but labelled as “exploratory” or “post-hoc” in the manuscript.
Ensure a paper’s value does not depend on statistically significant results
Researchers may be concerned that reduced analytic flexibility from pre-registration could increase the likelihood of reporting null results [ 22 , 23 ], which are harder to publish [ 13 , 42 ]. To address this, we recommend taking steps to ensure that the value and success of a study does not depend on a significant p-value. First, methodologically strong research (e.g., with high statistical power, valid and reliable measures, robustness checks, and replication samples) will advance the field, whatever the findings. Second, methods can be applied to allow for the interpretation of statistically non-significant findings (e.g., Bayesian methods [ 58 ] or equivalence tests, which determine whether an observed effect is surprisingly small [ 52 , 59 , 60 ]. This means that the results will be informative whatever they show, in contrast to approaches relying solely on null hypothesis significance testing, where statistically non-significant findings cannot be interpreted as meaningful. Third, researchers can submit the proposed study as a Registered Report, where it will be evaluated before the results are available. This is arguably the strongest way to protect against publication bias, as in-principle study acceptance is granted without any knowledge of the results. In addition, Registered Reports can improve the methodology, as suggestions from expert reviewers can be incorporated into the pre-registered protocol.
Under a system that rewards novel and statistically significant findings, it is easy for subconscious human biases to lead to QRPs. However, researchers, along with data guardians, journals, funders, and institutions, have a responsibility to ensure that findings are reproducible and robust. While pre-registration can help to limit analytic flexibility and selective reporting, it involves several challenges for epidemiologists conducting secondary data analysis. The approaches described here aim to address these challenges (Fig. 1 ), to either improve the efficacy of pre-registration or provide an alternative approach to address analytic flexibility (e.g., a multiverse analysis). The responsibility in adopting these approaches should not only fall on researchers’ shoulders; data guardians also have an important role to play in recording and reporting access to data, providing blinded datasets and hold-out samples, and encouraging researchers to pre-register and adopt these solutions as part of their data request. Furthermore, wider stakeholders could incentivise these practices; for example, journals could provide a designated space for researchers to report deviations from the pre-registration, and funders could provide grants to establish best practice at the cohort level (e.g., data checkout systems, blinded datasets). Ease of adoption is key to ensure wide uptake, and we therefore encourage efforts to evaluate, simplify and improve these practices. Steps that could be taken to evaluate these practices are presented in Box 1.
More broadly, it is important to emphasise that researcher biases do not operate in isolation, but rather in the context of wider publication bias and a “publish or perish” culture. These incentive structures not only promote QRPs [ 61 ], but also discourage researchers from pre-registering and adopting other time-consuming reproducible methods. Therefore, in addition to targeting bias at the individual researcher level, wider initiatives from journals, funders, and institutions are required to address these institutional biases [ 7 ]. Systemic changes that reward rigorous and reproducible research will help researchers to provide unbiased answers to science and society’s most important questions.
Box 1. Evaluation of approaches
To evaluate, simplify and improve approaches to protect against researcher bias in secondary data analysis, the following steps could be taken.
Co-creation workshops to refine approaches
To obtain feedback on the approaches (including on any practical concerns or feasibility issues) co-creation workshops could be held with researchers, data managers, and wider stakeholders (e.g., journals, funders, and institutions).
Empirical research to evaluate efficacy of approaches
To evaluate the effectiveness of the approaches in preventing researcher bias and/or improving pre-registration, empirical research is needed. For example, to test the extent to which the multiverse analysis can reduce selective reporting, comparisons could be made between effect sizes from multiverse analyses versus effect sizes from meta-analyses (of non-pre-registered studies) addressing the same research question. If smaller effect sizes were found in multiverse analyses, it would suggest that the multiverse approach can reduce selective reporting. In addition, to test whether providing a blinded dataset or dataset missing outcome variables could help researchers develop an appropriate analytical protocol, researchers could be randomly assigned to receive such a dataset (or no dataset), prior to pre-registration. If researchers who received such a dataset had fewer eventual deviations from the pre-registered protocol (in the final study), it would suggest that this approach can help ensure that proposed analyses are appropriate for the data.
Pilot implementation of the measures
To assess the practical feasibility of the approaches, data managers could pilot measures for users of the dataset (e.g., required pre-registration for access to data, provision of datasets that are blinded or missing outcome variables). Feedback could then be collected from researchers and data managers via about the experience and ease of use.
Acknowledgements
The authors are grateful to Professor George Davey for his helpful comments on this article.
Author contributions
JRB and MRM developed the idea for the article. The first draft of the manuscript was written by JRB, with support from MRM and all authors commented on previous versions of the manuscript. All authors read and approved the final manuscript.
J.R.B is funded by a Wellcome Trust Sir Henry Wellcome fellowship (grant 215917/Z/19/Z). J.B.P is a supported by the Medical Research Foundation 2018 Emerging Leaders 1 st Prize in Adolescent Mental Health (MRF-160–0002-ELP-PINGA). M.R.M and H.M.S work in a unit that receives funding from the University of Bristol and the UK Medical Research Council (MC_UU_00011/5, MC_UU_00011/7), and M.R.M is also supported by the National Institute for Health Research (NIHR) Biomedical Research Centre at the University Hospitals Bristol National Health Service Foundation Trust and the University of Bristol.
Declarations
Author declares that they have no conflict of interest.
Publisher's Note
Springer Nature remains neutral with regard to jurisdictional claims in published maps and institutional affiliations.
A study found corporate recruiters have a bias against ex-entrepreneurs. They get stereotyped for not wanting to ‘be a small piece of the puzzle’

Sometimes entrepreneurs do what many of them consider unthinkable: abandon their business to go become a salaried employee at a regular job.
Being a working stiff can be anathema to those with the founder gene, who find the uncertainty of entrepreneurship equal parts freeing and exhilarating. But sometimes the lack of stability can become too much to bear, either financially or emotionally. Life is a bit easier with a steady paycheck and without the constant fear your startup might go bust. But when ex-entrepreneurs want to return to the workforce, they often face undue stigmas . Recruiters balk at their unusual resumes, unsure how to evaluate a candidate with unorthodox work experiences. That’s to say nothing of the stereotype they face for being impetuous and egotistical .
“It’s really critical for them to be able to explain the elephant in the room,” says Debi Creasman, CEO of recruiting firm Raven Road Partners. “Because the vibe is that someone who’s done an entrepreneurial thing for a good long while is a bit of a maverick and doesn’t really want to fit into a confined structure or be a small piece of the puzzle.”
Former entrepreneurs are 35% less likely to get a job interview, according to research from the London Business School. This trend is usually referred to as the “entrepreneurship penalty.”
A separate study from Rutgers University’s School of Management and Labor Relations recently sought to understand if former entrepreneurs were less likely to get hired because founders are bad job candidates or because they face bias throughout the hiring process. The researchers asked recruiters to evaluate mock resumes with comparable levels of education and experience for candidates who had worked in traditional companies, startups, or both. The study found that 60% of recruiters responded less favorably to the mock resume of a former entrepreneur.
So while the stereotype of a mercurial, borderline antisocial founder in the mold of Steve Jobs or Elon Musk might exist (and perhaps be slightly true) the study points to the fact that companies tend to penalize entrepreneurs unfairly. One of the reasons why could be because they simply aren’t set up to evaluate candidates with unconventional backgrounds.
From a recruiter’s perspective, it’s hard to validate an entrepreneur’s experience, says Rutgers professor Jasmine Feng, one of the researchers.
“You are basically relying on information that is mostly self reported,” she adds. “So it’s really challenging for recruiters to understand whether their qualifications, experience, or job responsibilities are comparable to a conventional applicant’s.”
Nistha Dube, an aspiring content creator who left behind her entrepreneurial dreams in favor of a more traditional career path in education, says she regularly had to explain parts of her resume to skeptical recruiters. “I didn’t know which part of my experiences would be relevant and which wouldn’t,” she says. “I also had to explain why I’m leaving all that behind to get a job.”
Even having run a successful startup may not insulate candidates from the struggles of the entrepreneurship penalty. Research from the Harvard Business Review found that software engineering candidates with founder experience whose startups had been successful were 33% less likely to get offered a job interview than those whose companies had failed. Much of that could be because recruiters have concerns that formerly successful founders can be set in their ways because their methods already brought them to great success once.
“If their mindset is arrogant, potentially inflexible, or just sort of dogmatic in their approach, then that might not be as appealing to a recruiter in general,” Creasman says.
More often than not, the most successful founders are best suited for executive level roles, she adds. They can parlay those experiences into the C-suite, as former Everfi cofounder and CEO Jon Chapman did. There is “no doubt” that the success of Everfi, which he sold in 2022 for $750 million, played a role in him landing his current job as CEO of the esports company PlayVS, according to Chapman. “Had I not had that type of success it wouldn’t have played out that way for me necessarily,” he says.
Companies are eager for an ‘entrepreneurial’ culture
Meanwhile, entrepreneurs can still make valuable additions to traditional workplaces among the rank-and-file. In recent years, many companies have tried to reshape their cultures to foster more innovation . To do so, companies actively look to hire employees with some of the qualities founders tend to bring: out-of-the-box thinking, innovation, and an embrace of uncertainty. That’s especially relevant in the current business landscape where things are so volatile. The rise of AI, a murky interest rate environment, and a looming presidential election all make for particular turbulent times for companies—the exact sort of thing former entrepreneurs are suited to navigating.
Companies “are really struggling to grasp the concept of the new and the next,” Creasman says. “So when you hire somebody from an entrepreneurial background, they bring a sense of calm because they’ve lived in chaos for a long time.”
Chapman remembers his own experiences starting Everfi as being characterized by some seat-of-the-pants moments. “When you’re in a startup phase, you are constantly having to figure things out on the fly,” he said. “And make decisions quickly, without really knowing if you’re going to be right or wrong.”
To weed out the entrepreneurs that would be good hires from those who would ultimately chafe at being a cog in a bigger machine, Creasman suggests putting candidates through different scenarios during the interview. She suggests giving them an example of the sort of red tape they might encounter and asking them how they’d approach getting the proper approvals.
Creasman also advises recruiters to try and gauge whether someone is in it for the long haul. Research shows that ex-entrepreneurs do have a higher turnover rate compared to other employees. “Certainly skill sets are important, but mindset sometimes trumps that,” she said.
The Rutgers study also found that certain types of recruiters are less prone to a bias against entrepreneurs. Perhaps not very surprisingly, recruiters with their own entrepreneurial experiences are the most open to hiring former founders. The research also found that recruiters with a short tenure at the company and women responded more favorably to candidates who were ex-entrepreneurs. According to Feng, recruiters who had just joined the company didn’t have as much of its institutional thinking ingrained in them, while women were less likely to stereotype founders in general and instead just evaluated them using the qualifications on the mock resumes.
Addressing the reality that entrepreneurs may have a harder time than others landing a job is just another example of the adversity those who choose that career path face, Feng says. “Those who want to be entrepreneurs need to be aware this may not always be rosy,” she says. “This could be a very bumpy road. It may lead you back to the traditional workforce, and you need to be aware of some of the career risks related to this reality.”
For Dube, it was how bumpy that road turned out to be that ultimately led her to opt for a more stable, if traditional, career option. “Every part of that experience felt like I was scraping by and trying to make ends meet.”
Latest in Success

‘Judge Judy’ sues National Enquirer parent company over misattributed quote in Menendez Brothers story

McDonald’s may be willing to lose money on $5 meal deals if it means winning back disgruntled cash-strapped customers

Arthur Irving, one of Canada’s richest people and heir to a massive oil fortune, has died at 93

Meet America’s first female retail executive: By 25 years old she was Macy’s second in command and she created the iconic red star logo

After selling his startup for a life-changing $3.7 billion, Jyoti Bansal launched a VC firm and two high-value startups. Why?

Gen Z really do have it worse: Those in their early 20s are earning less and have more debt than millennials did at their age
Most popular.

The collapsed Baltimore bridge will be demolished soon, and the crew of the ship that’s trapped underneath will be onboard when the explosives go off

The housing crisis in the U.S. is flipped upside down in Japan, where each home that’s occupied could be next to an empty one by 2033

TV chef Gordon Ramsay spends an extra $7.6 million on staff as U.K. restaurant empire losses triple

Consumers were deprived of rare bourbons, including Pappy Van Winkle’s 23-year-old whiskey, by alcohol overseers

Meet the boomers who’d rather spend $100k to renovate their homes than risk the frozen housing market: ‘It would be too hard to purchase anything else’
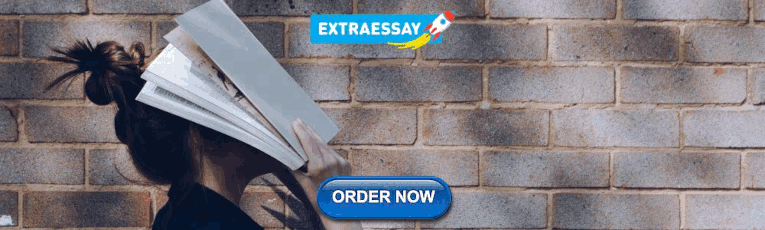
IMAGES
VIDEO
COMMENTS
Bias—commonly understood to be any influence that provides a distortion in the results of a study (Polit & Beck, 2014)—is a term drawn from the quantitative research paradigm.Most (though perhaps not all) of us would recognize the concept as being incompatible with the philosophical underpinnings of qualitative inquiry (Thorne, Stephens, & Truant, 2016).
Consider potential bias while constructing the interview and order the questions suitably. Ask general questions first, before moving to specific or sensitive questions. Leading questions and wording bias. Questions that lead or prompt the participants in the direction of probable outcomes may result in biased answers.
In qualitative research for instance, the researcher's preconceived notions and expectations can influence how they interpret and code qualitative data, a type of bias known as interpretation bias. It's also important to note that quantitative research is not free of bias either, as sampling bias and measurement bias can threaten the validity ...
Researcher reflexivity, essentially a researcher's insight into their own biases and rationale for decision-making as the study progresses, is critical to rigor. This article reviews common standards of rigor, quality scholarship criteria, and best practices for qualitative research from design through dissemination.
Introduction. Bias, perhaps best described as 'any process at any stage of inference which tends to produce results or conclusions that differ systematically from the truth,' can pollute the entire spectrum of research, including its design, analysis, interpretation and reporting. 1 It can taint entire bodies of research as much as it can individual studies. 2 3 Given this extensive ...
Even if bias is not a primary concern of qualitative research (Galdas, 2017), selective sampling can be an issue (Miles et al., 2014;Tellis, 2017). I chose two strategies to help limit this impact ...
In other words, their subjective perspective (or "bias") is fundamentally intertwined with qualitative research processes. And while the researcher's perspective has many positive impacts, failure to attend to reflexivity can negatively impact the knowledge built via qualitative research and those connected to it.
Abstract. This narrative review provides an overview on the topic of bias as part of Plastic and Reconstructive Surgery 's series of articles on evidence-based medicine. Bias can occur in the planning, data collection, analysis, and publication phases of research. Understanding research bias allows readers to critically and independently review ...
There's interviewer bias, which is very hard to avoid. This is when an interviewer subconsciously influences the responses of the interviewee. Their body language might indicate their opinion, for example. Furthermore, there's response bias, where someone tries to give the answers they think are "correct.".
His account of validity in qualitative research is, at least in part, an attempt to uncover 'theory-in-use'. He distinguishes five types of validity: descriptive validity, interpretive validity, theoretical validity, generalisability and evaluative validity.[1] Maxwell notes that in experimental research threats to validity are "addressed ...
Revisiting Bias in Qualitative Research: Reflections on Its Relationship With Funding and Impact. Recognizing and understanding research bias is crucial for determining the utility of study results and an essential aspect of evidence-based decision-making in the health professions. Research proposals and manuscripts that do not provide satis ...
Qualitative research can be very useful for starting an investigation into a new area of study, providing suggestions for concepts to be measured and hypotheses to be tested. ... Fortunately, there are ways to be aware of and perhaps reduce personal or political bias throughout the research and publication process, as we have discussed.
Research bias can occur in both qualitative and quantitative research. Understanding research bias is important for several reasons. Bias exists in all research, across research designs, and is difficult to eliminate. ... Researcher bias occurs when the researcher's beliefs or expectations influence the research design or data collection process.
Bias in research Joanna Smith,1 Helen Noble2 The aim of this article is to outline types of 'bias' across research designs, and consider strategies to minimise ... research cannot be applied to qualitative research. However, in the broadest context, these terms are applic-able, with validity referring to the integrity and applica- ...
Bias #2 - Analysis Bias. Next up, we have analysis bias.Analysis bias occurs when the analysis itself emphasises or discounts certain data points, so as to favour a particular result (often the researcher's own expected result or hypothesis).In other words, analysis bias happens when you prioritise the presentation of data that supports a certain idea or hypothesis, rather than presenting ...
Biases can exist in health research, in both quantitative and in qualitative research 1. Although it is not a new topic, the discussion about biases in qualitative research is still timid and demands greater attention and depth from researchers. According to Althubaiti 2, the problem of bias is still often ignored in practice.
The data collection phase is a critical juncture where researcher bias can significantly affect the credibility of qualitative studies. As you gather information through interviews, observations ...
This essay discusses the utilization of safeguard strategies, particularly Improvement Science principles, in the academic and professional writing of scholar-practitioners within EdD programs. These strategies bridge the gap between theory and practice, enabling graduate students to apply their scholarly insights meaningfully. The essay highlights the roles of bias, professional wisdom ...
There's a researcher, Jason A. Okonofua, who talks about this and calls it "sidelining bias." You're not targeting the person and trying to get rid of their biases. You're not targeting the ...
This study seeks to understand the challenges encountered by qualitative researchers while investigating sensitive topics. We make a valuable contribution to the existing literature on researcher well-being and the mitigation of potential adverse incidents during data collection in studies on sensitive topics. The researchers maintained a comprehensive diary while conducting a study on the ...
Revisiting Bias in Qualitative Research: Reflections on Its Relationship With Funding and Impact. Recognizing and understanding research bias is crucial for determining the utility of study results and an essential aspect of evidence-based decision-making in the health professions. Research proposals and manuscripts that do not provide satis ...
Both visual context and ICMS current amplitude were found to bias the qualitative experience of ICMS. In two tetraplegic participants, ICMS and visual stimuli were more likely to be experienced as occurring simultaneously when visual stimuli were more realistic, demonstrating an effect of visual context on the temporal binding window.
However, researcher biases can lead to questionable research practices in secondary data analysis, which can distort the evidence base. While pre-registration can help to protect against researcher biases, it presents challenges for secondary data analysis. In this article, we describe these challenges and propose novel solutions and ...
Revisiting Bias in Qualitative Research: Reflections on Its Relationship With Funding and Impact. Paul Galdas View all authors and affiliations. All Articles. ... The role of qualitative research within an evidence-based context: Can metasynthesis be the answer? International Journal of Nursing Studies, 46, 569-575. Crossref. PubMed. ISI ...
Former entrepreneurs are 35% less likely to get a job interview, according to research from the London Business School. This trend is usually referred to as the "entrepreneurship penalty."
Qualitative researchers can no longer dismiss the question of bias because the end result is not generalization of the findings since qualitative findings are being used in the care of patients. In the end, it becomes the best available evidence for quantitative questions and truth for the moment for quali-tative ones.