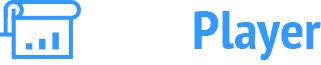
- My presentations
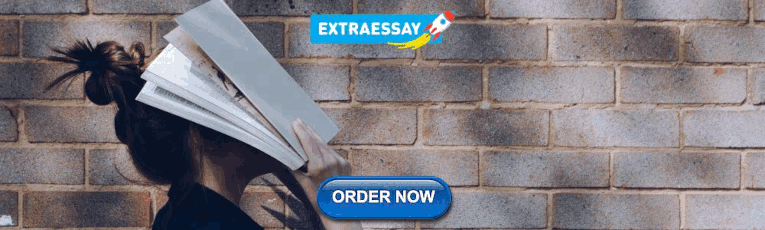
Auth with social network:
Download presentation
We think you have liked this presentation. If you wish to download it, please recommend it to your friends in any social system. Share buttons are a little bit lower. Thank you!
Presentation is loading. Please wait.
Inferential Statistics
Published by Juliet Fleming Modified over 5 years ago
Similar presentations
Presentation on theme: "Inferential Statistics"— Presentation transcript:
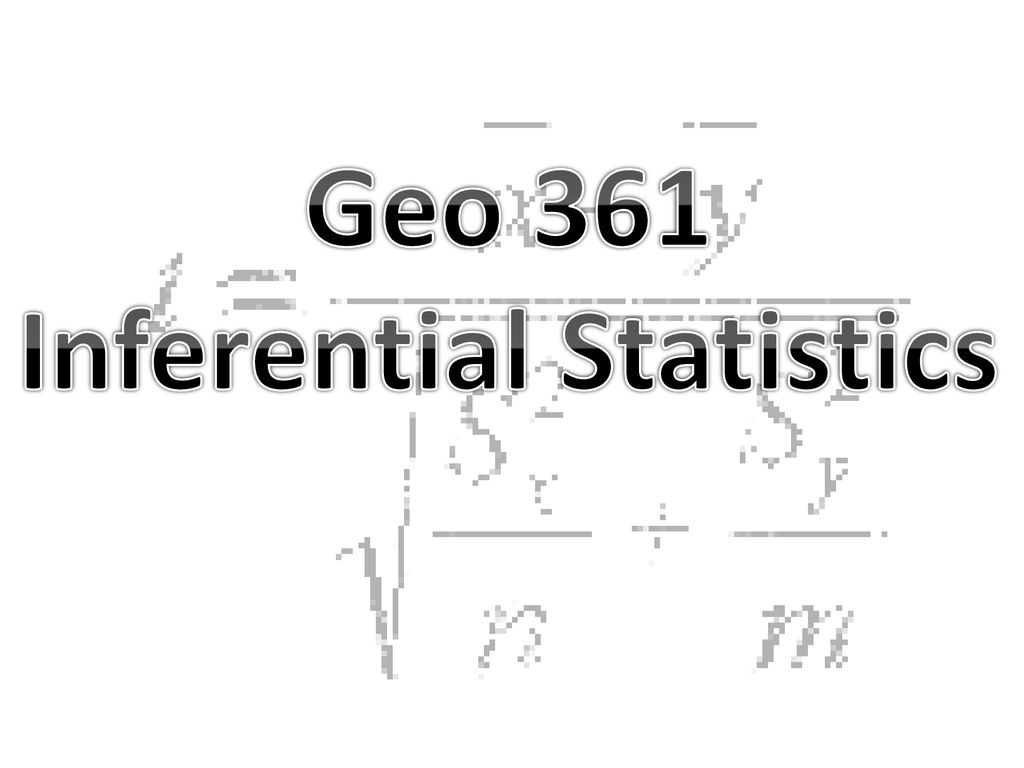
Chapter 9 Introduction to the t-statistic
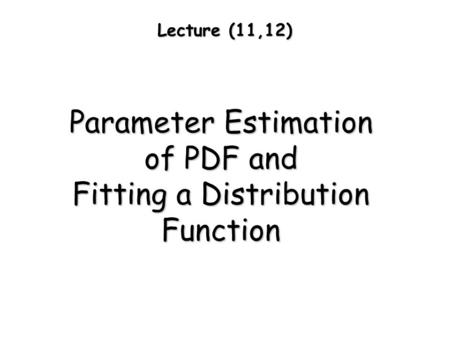
Lecture (11,12) Parameter Estimation of PDF and Fitting a Distribution Function.
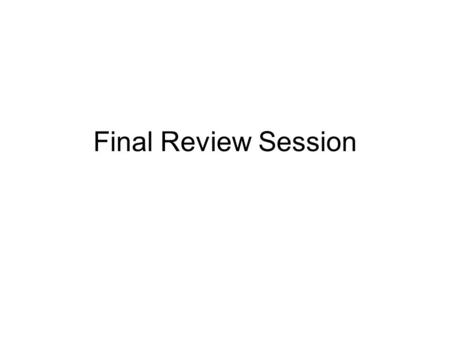
Final Review Session.
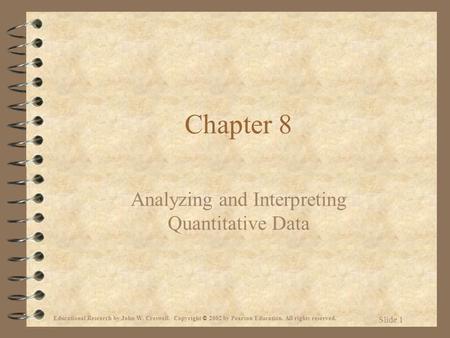
Educational Research by John W. Creswell. Copyright © 2002 by Pearson Education. All rights reserved. Slide 1 Chapter 8 Analyzing and Interpreting Quantitative.
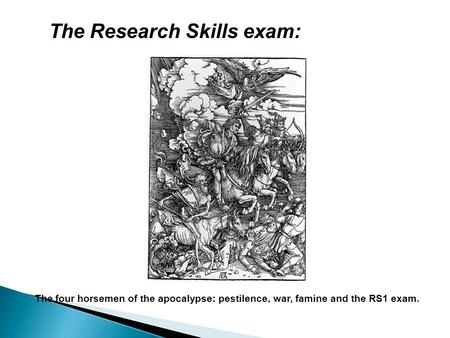
The Research Skills exam: The four horsemen of the apocalypse: pestilence, war, famine and the RS1 exam.
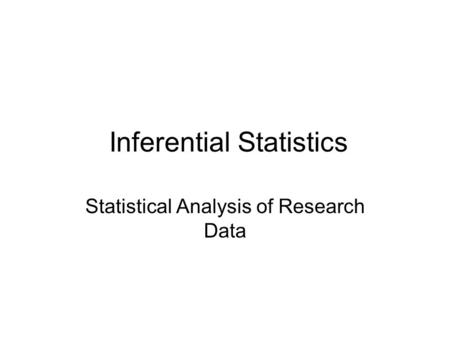
INFERENTIAL STATISTICS – Samples are only estimates of the population – Sample statistics will be slightly off from the true values of its population’s.
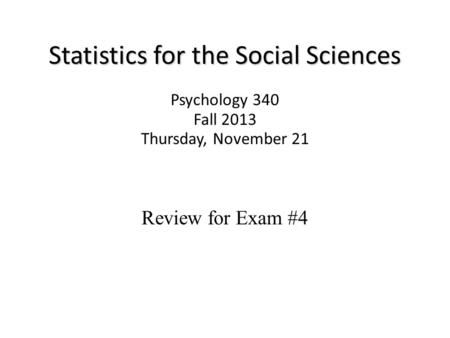
Statistics for the Social Sciences Psychology 340 Fall 2013 Thursday, November 21 Review for Exam #4.
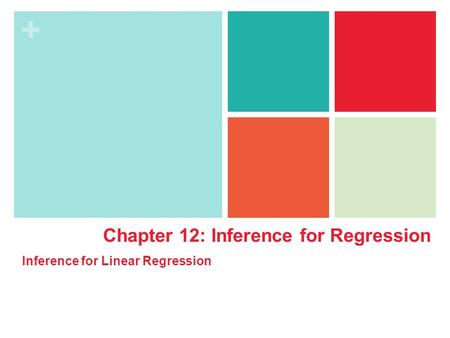
+ Chapter 12: Inference for Regression Inference for Linear Regression.
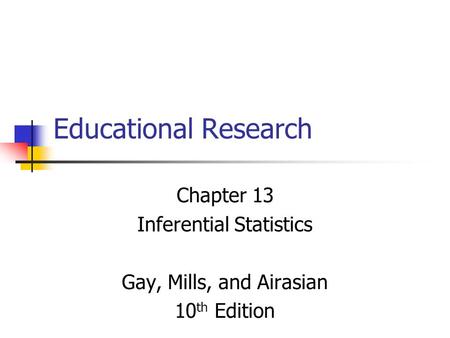
Educational Research Chapter 13 Inferential Statistics Gay, Mills, and Airasian 10 th Edition.
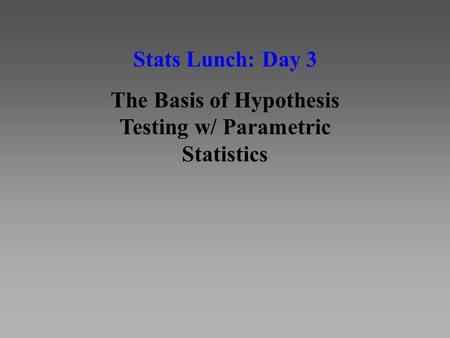
Stats Lunch: Day 3 The Basis of Hypothesis Testing w/ Parametric Statistics.
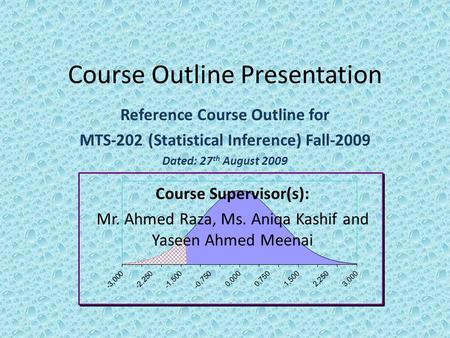
Course Outline Presentation Reference Course Outline for MTS-202 (Statistical Inference) Fall-2009 Dated: 27 th August 2009 Course Supervisor(s): Mr. Ahmed.
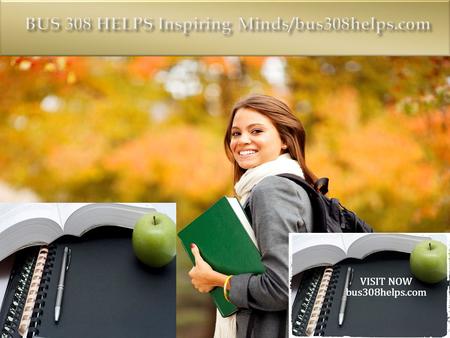
BUS 308 Entire Course (Ash Course) For more course tutorials visit BUS 308 Week 1 Assignment Problems 1.2, 1.17, 3.3 & 3.22 BUS 308.
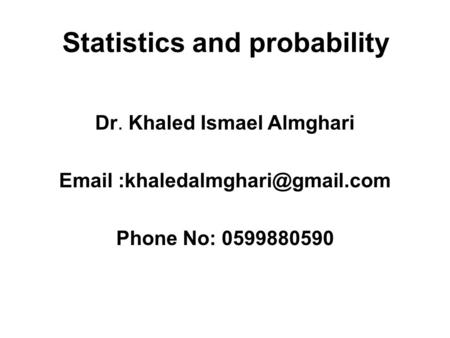
Statistics and probability Dr. Khaled Ismael Almghari Phone No:
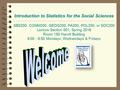
Introduction to Statistics for the Social Sciences SBS200, COMM200, GEOG200, PA200, POL200, or SOC200 Lecture Section 001, Spring 2016 Room 150 Harvill.
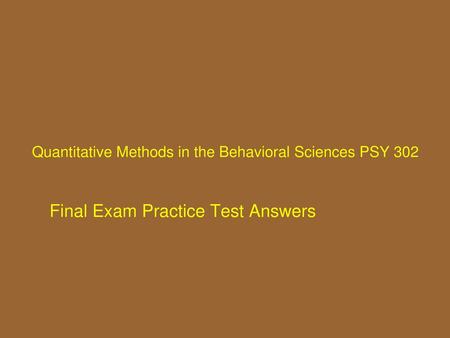
Quantitative Methods in the Behavioral Sciences PSY 302
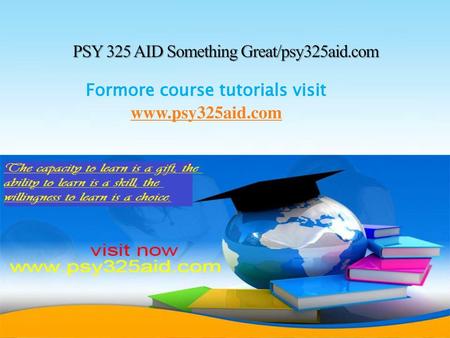
COM 295 STUDY Inspiring Minds/com295study.com
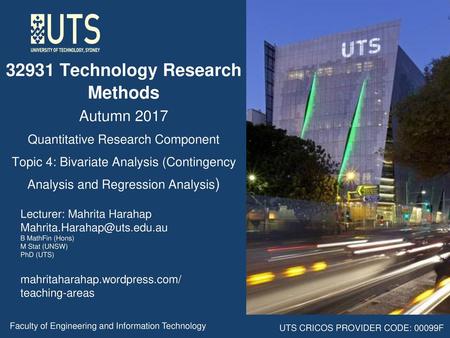
32931 Technology Research Methods Autumn 2017 Quantitative Research Component Topic 4: Bivariate Analysis (Contingency Analysis and Regression Analysis)
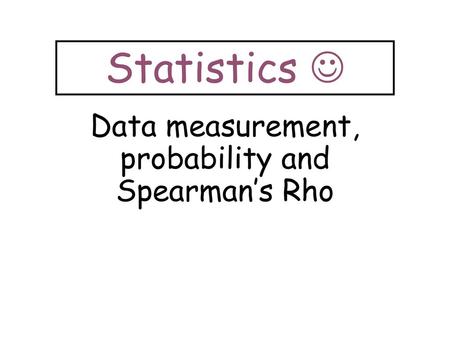
Data measurement, probability and Spearman’s Rho
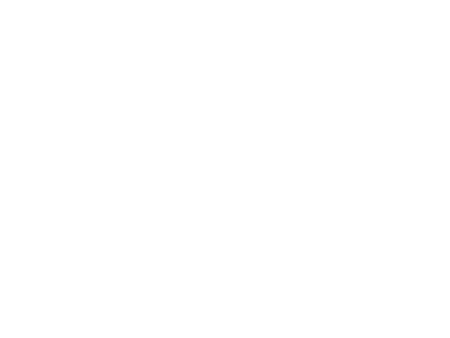
Practice As part of a program to reducing smoking, a national organization ran an advertising campaign to convince people to quit or reduce their smoking.
About project
© 2024 SlidePlayer.com Inc. All rights reserved.
Episode 12: Inferential Statistics and Hypothesis Testing Playbook
Using a case study on comparing salaries of police and social services department in new york for 2020 and 2021..
This post acts as a handy cheat sheet on how to draw important inferences from a given dataset using statistics and hypothesis testing.
Citywide Payroll Data (Fiscal Year) was taken from “Data.gov” . Each record represents the following statistics for every city employee:
Agency, Last Name, First Name, Middle Initial, Agency Start Date, Work Location Borough, Job Title Description, Leave Status as of the close of the FY (June 30th), Base Salary, Pay Basis, Regular Hours Paid, Regular Gross Paid, Overtime Hours worked, Total Overtime Paid, and Total Other Compensation.
For the purpose of simplicity, I have extracted the base salaries of only two departments i.e. Police department and Social Services for fiscal years 2020 and 2021 and have taken a subset of relevant columns.

- Step 0: Descriptive statistics and their interpretation.
We use the Data Analysis toolpack in Excel to quickly get descriptive stats for the two departments. Refer to sheet “descriptive_stats” in inferential_stats_case_study.xlsx [1].
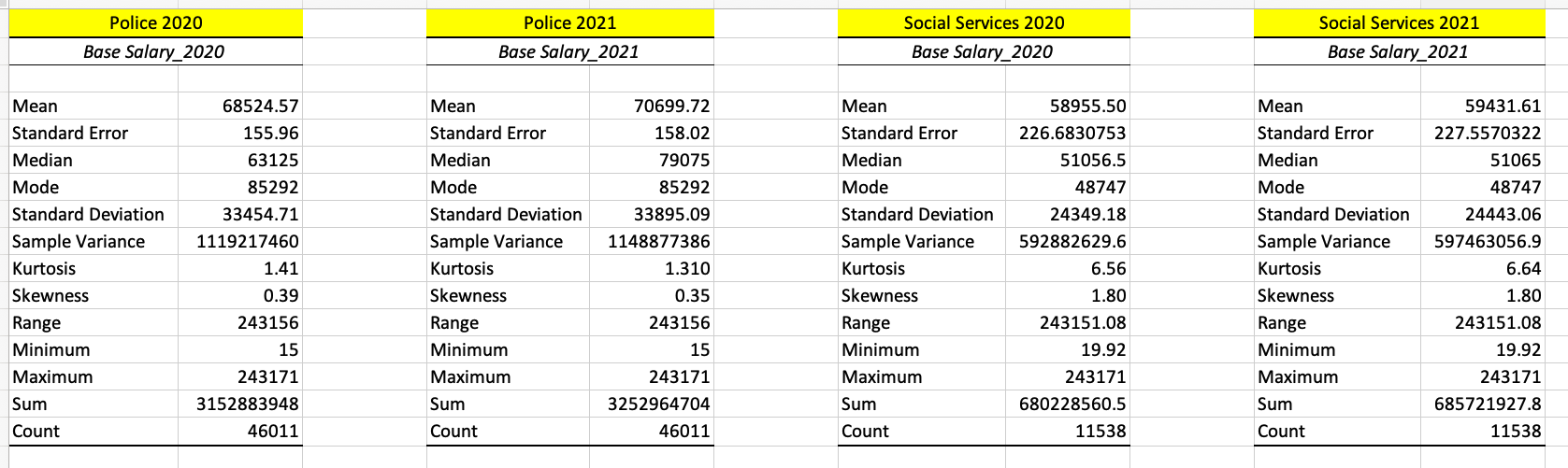
We make the following observations and come up with relevant questions related to these observations which we will later answer through various statistical tests:
- Is this difference statistically significant?
The police department’s salaries vary a bit more than the social services department as shown by the standard deviation.
- This implies a right/positive skew.
- But the small value for “Skew” for police shows that the tails don’t extend too far to the right.
- Kurtosis less than 3 also shows that the distribution for police’s salaries is thin-tailed and outlier frequency is low.
- Kurtosis is > 3 for Social Services which implies a higher outlier frequency and fat-tailed distribution.
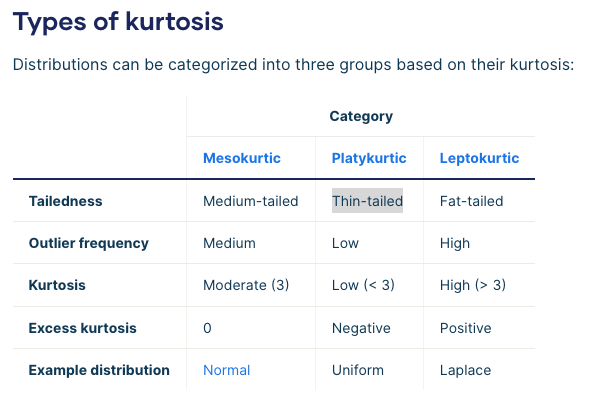
Inferential Statistics:
There are 3 main themes in inferential statistics and 2 subsequent hypothesis tests:
- Population Variance Known (z-test).
- Population Variance Unknown (t-test).
- Confidence Interval for Mean Difference, Dependent Samples: Example: Calculate the change in mean salary of NYPD from one year to the next.
- t-Test: Paired Two Sample for Means: Example: Testing whether change in police salary from one year to the next was statistically significant.
- t-Test: Two-Sample Assuming Equal Variances: Example: Testing whether the difference in police salary and social services salary is statistically significant.
Confidence Interval for Mean, Single Population:
- Step 1(a): If we know the population variance for the salary of each department, calculate the confidence interval for the mean salary of a police officer and a social worker in New York for the year 2020. Assume that the police department’s salary has a population standard deviation of $80k and social services has a population standard deviation of $60k.
Refer to sheet “1. Known variance, CI, z-table” in inferential_stats_case_study.xlsx [1].
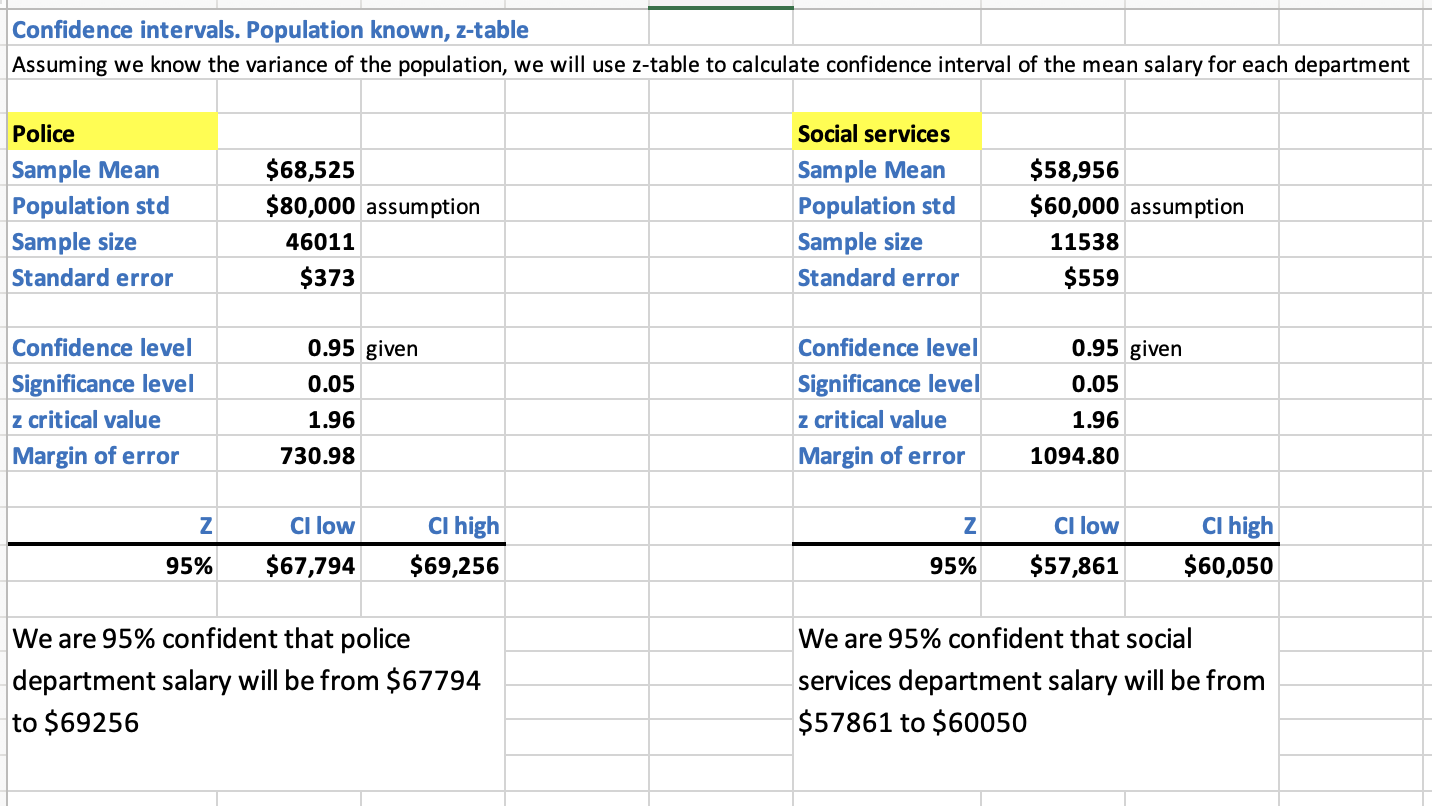
Interpretation: We are 95% confident that a police officer's salary was from $67,794 to $69,256 and a social worker's salary was from $57,861 to $60,050 in NYC in 2020.
- Step 1(b): If we do not know the population variance for the salary of each department, calculate the confidence interval for the mean salary of a police officer and a social worker in New York for the year 2020.
Refer to sheet “2. Unkown var, CI, t-table” in inferential_stats_case_study.xlsx [1].
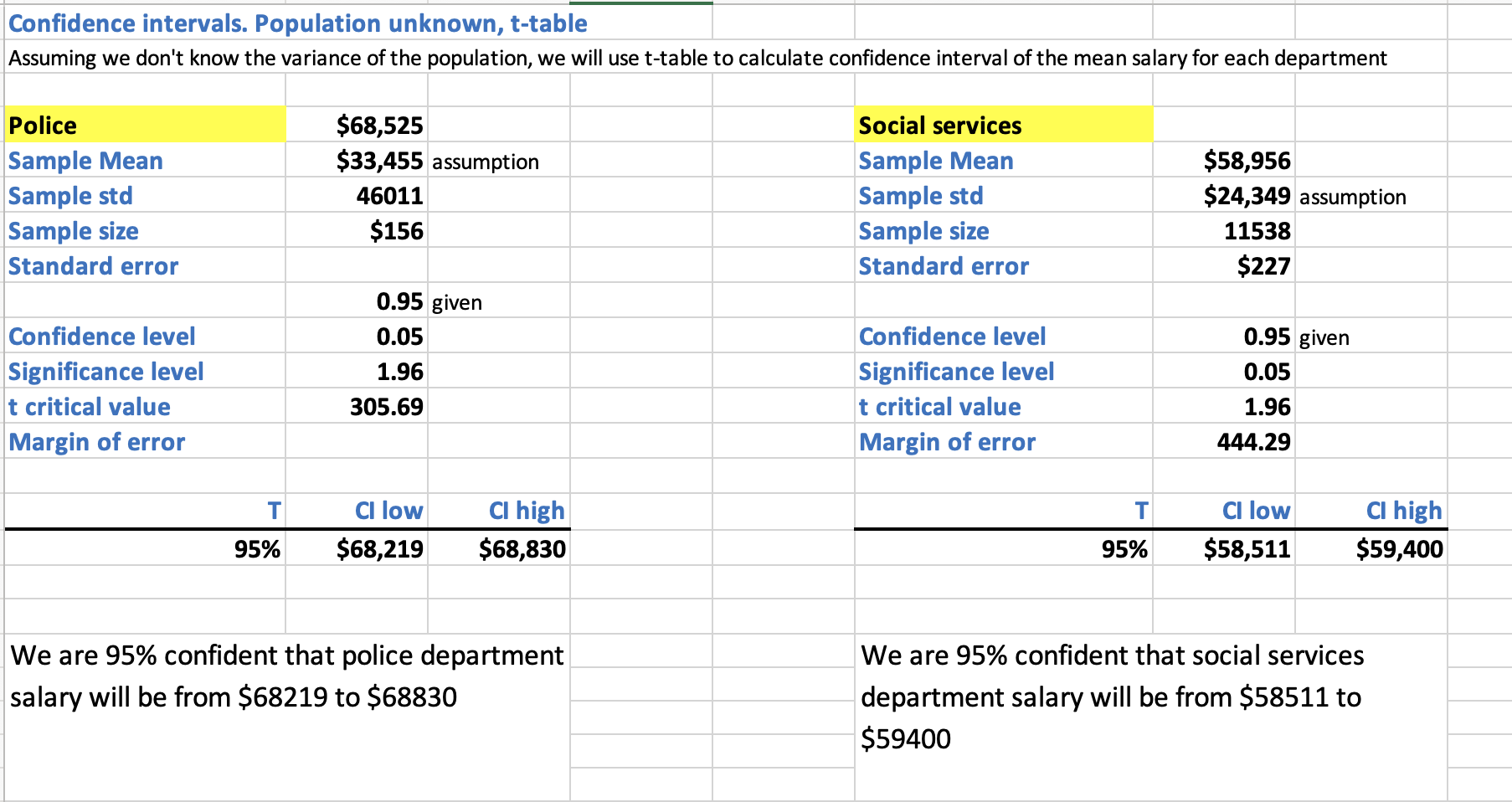
Interpretation: We are 95% confident that a police officer's salary was from $68,219 to $68,830 and a social worker's salary was from $58,511 to $59,400 in NYC in 2020.
Confidence Interval for Mean Difference, Dependent Samples:
- Step 2: We are interested in knowing the salary increase for each department from 2020 to 2021. Calculate the confidence interval for that increase with 95% confidence.
Refer to sheet “3. Two Means Dependent Samples” in inferential_stats_case_study.xlsx [1].
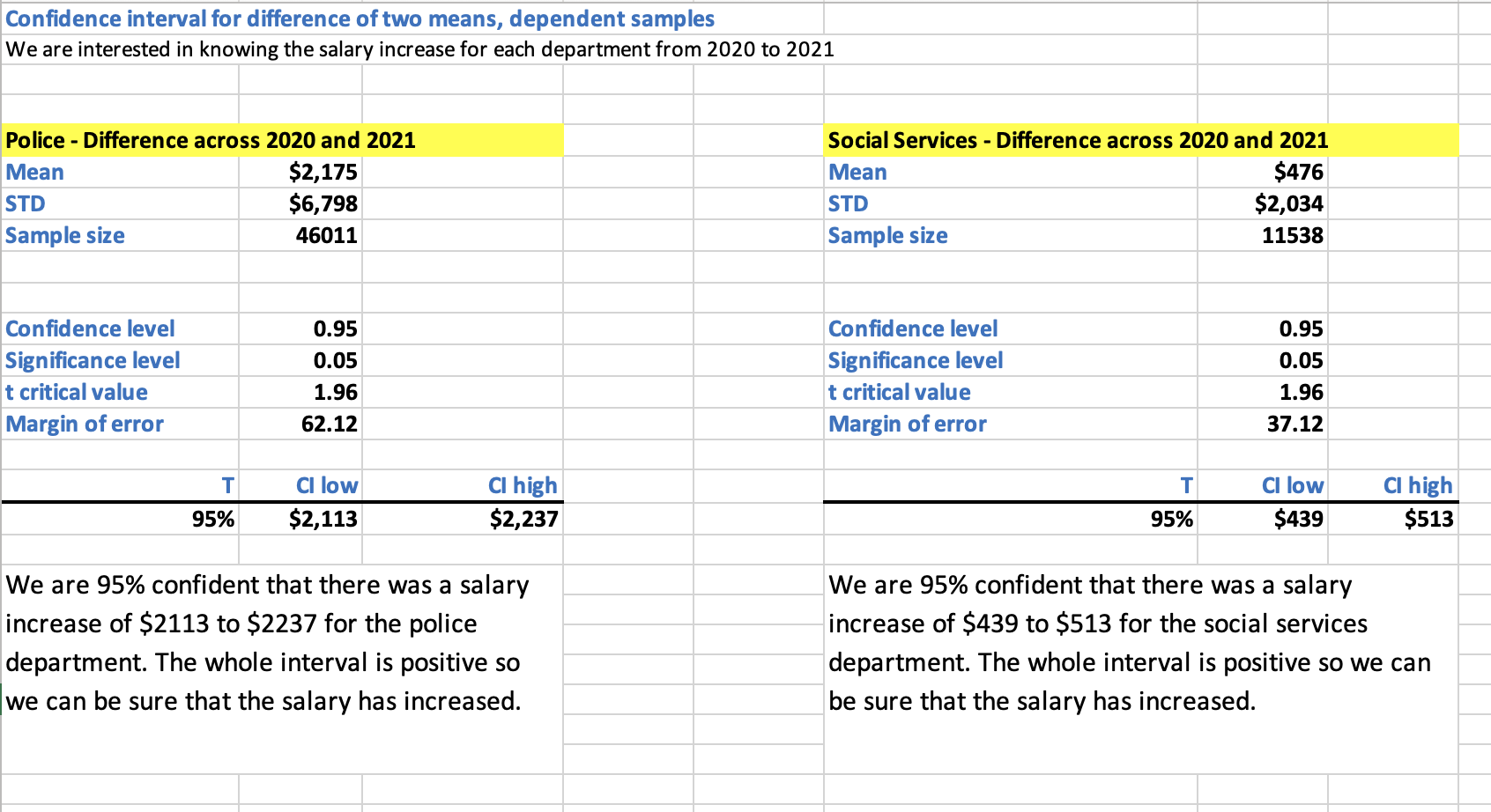
Interpretation: We are 95% confident that there was a salary increase of $2,113 to $2,237 for the police department. The whole interval is positive so we can be sure that the salary has increased. We are 95% confident that there was a salary increase of $439 to $513 for the social services department. The whole interval is positive so we can be sure that the salary has increased.
Confidence Interval for Mean Difference, Independent Samples
- Step 3(a): We are interested in knowing the salary difference between police and social services. Calculate the confidence interval for that difference with 95% confidence. Assume that the police department’s salary has a population standard deviation of $80k and social services has a population standard deviation of $60k.
Refer to sheet “4. Two Mean Ind Samp, known var” in inferential_stats_case_study.xlsx [1].
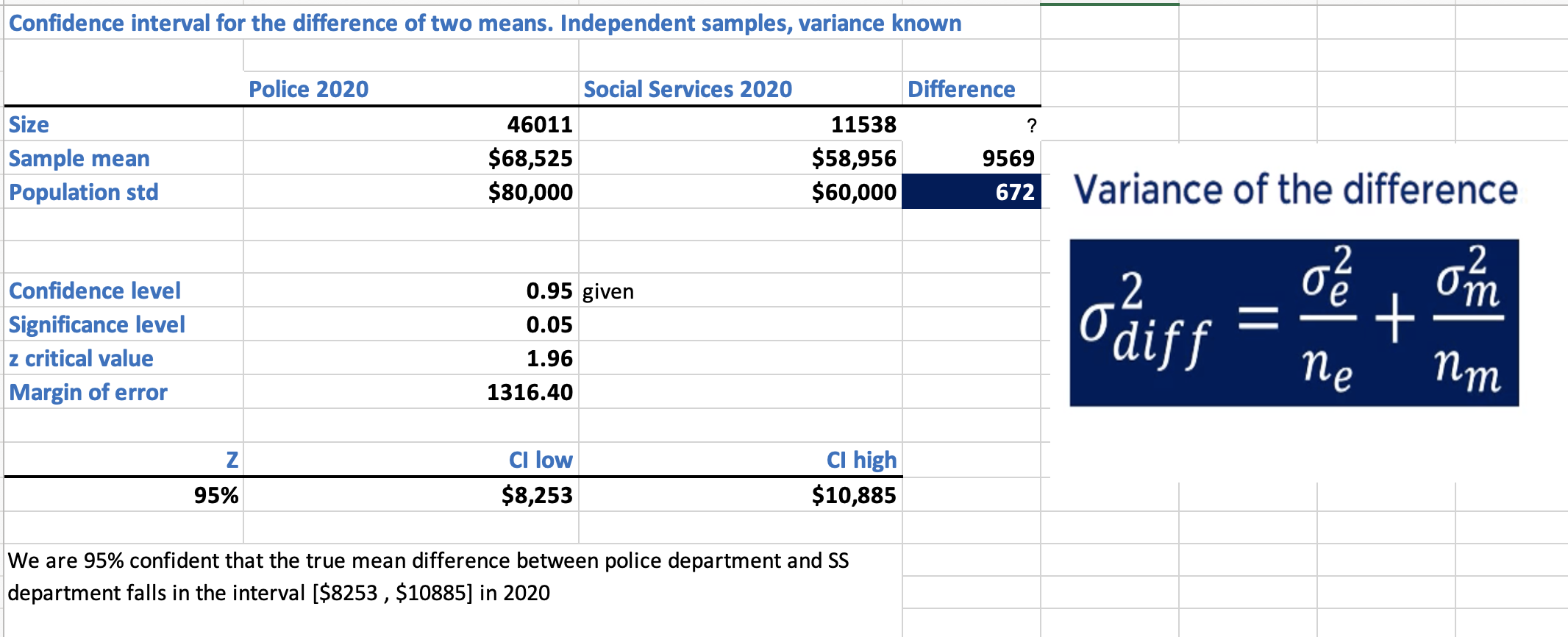
Interpretation: We are 95% confident that the true mean difference between police department and Social Services department falls in the interval [$8,253 , $10,885] in 2020.
- Step 3(b): We are interested in knowing the salary difference between police and social services. Calculate the confidence interval for that difference with 95% confidence. We do not known the population variance but we know it’s almost equal for both departments.
Refer to sheet “5. Two Mean Ind Samp, unkwn var” in inferential_stats_case_study.xlsx [1].
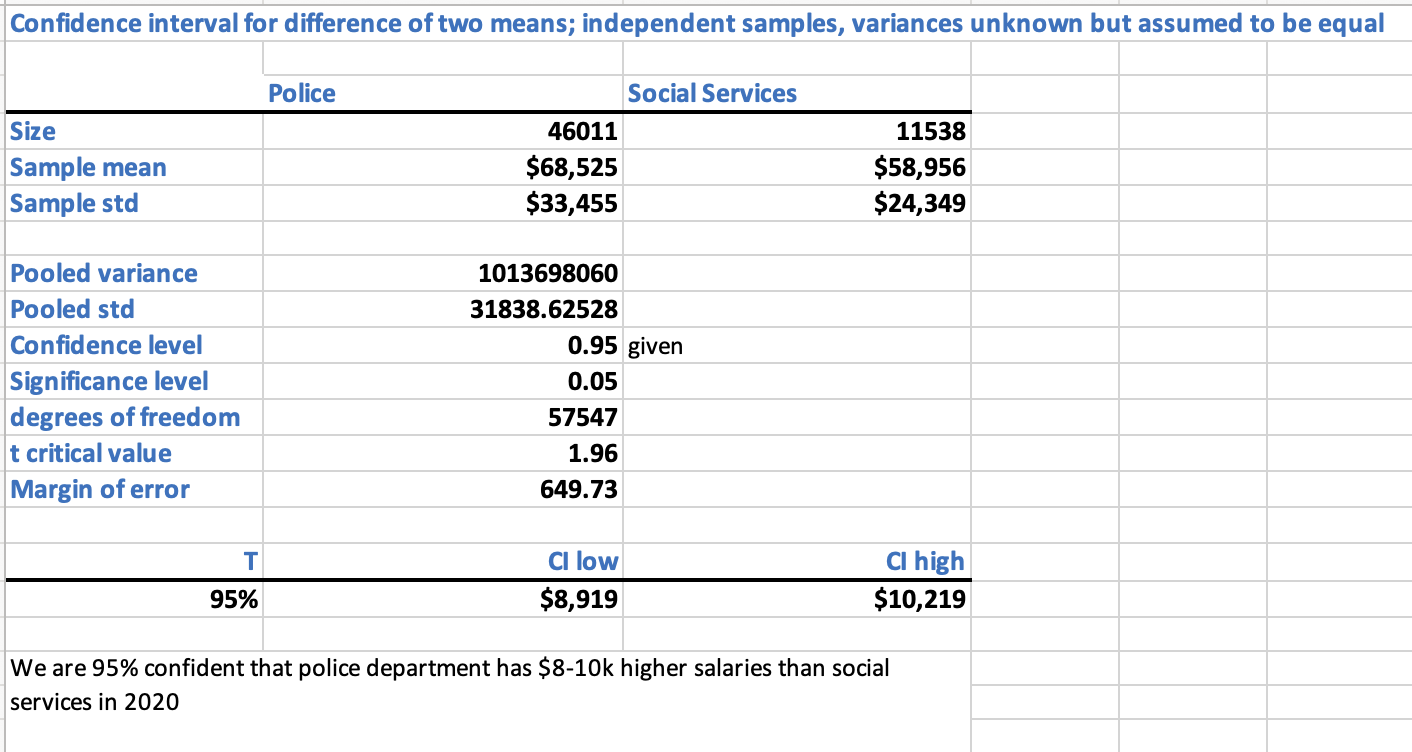
Interpretation: We are 95% confident that police department has $8-10k higher salaries than social services in 2020.
Hypothesis Testing
T-test: paired two sample for means:.
- Hari Singh is a pessimistic police officer and says there was no significant increase in NYPD salaries from 2020 to 2021. Run a hypothesis test to prove him right or wrong. Run a similar test for Social Services department.
We use t-Test: Paired Two Sample for Means from Data Anlysis Toolpack in Excel.
Refer to sheet “8. Test for mean. Dep samples” in inferential_stats_case_study.xlsx [1].
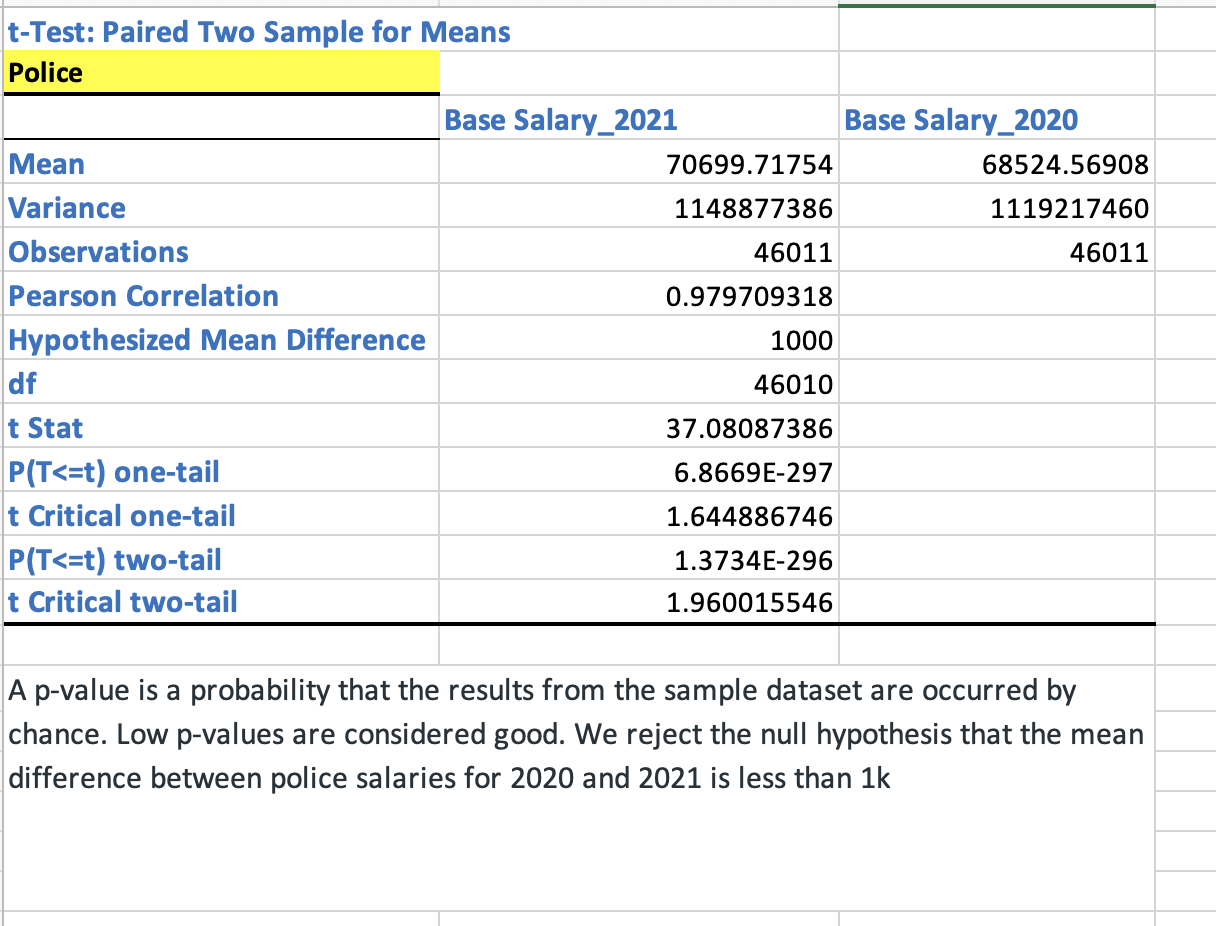
- We say that the null hypothesis is that the difference in salaries in 2020 and 2021 is less than or equal to $1000.
- A p-value is a probability that the results from the sample dataset are occurred by chance. Low p-values are considered good. Small p-value shows that we can reject the null hypothesis.
- We reject the null hypothesis because t Stat 37 > t critical one-tail of 1.64.
- Hari Singh is wrong. NYPD's salaries have increased from 2020 to 2021.
t-Test: Two-Sample Assuming Equal Variances:
- Christina works in NYC’s social services department and thinks that NYPD is being paid $10k or more on average than SS department. Run a hypothesis test to prove her right or wrong.
We use t-Test: Two-Sample Assuming Equal Variances from Data Anlysis Toolpack in Excel.
Refer to sheet “10. Test for mean, Ind samples” in inferential_stats_case_study.xlsx [1].
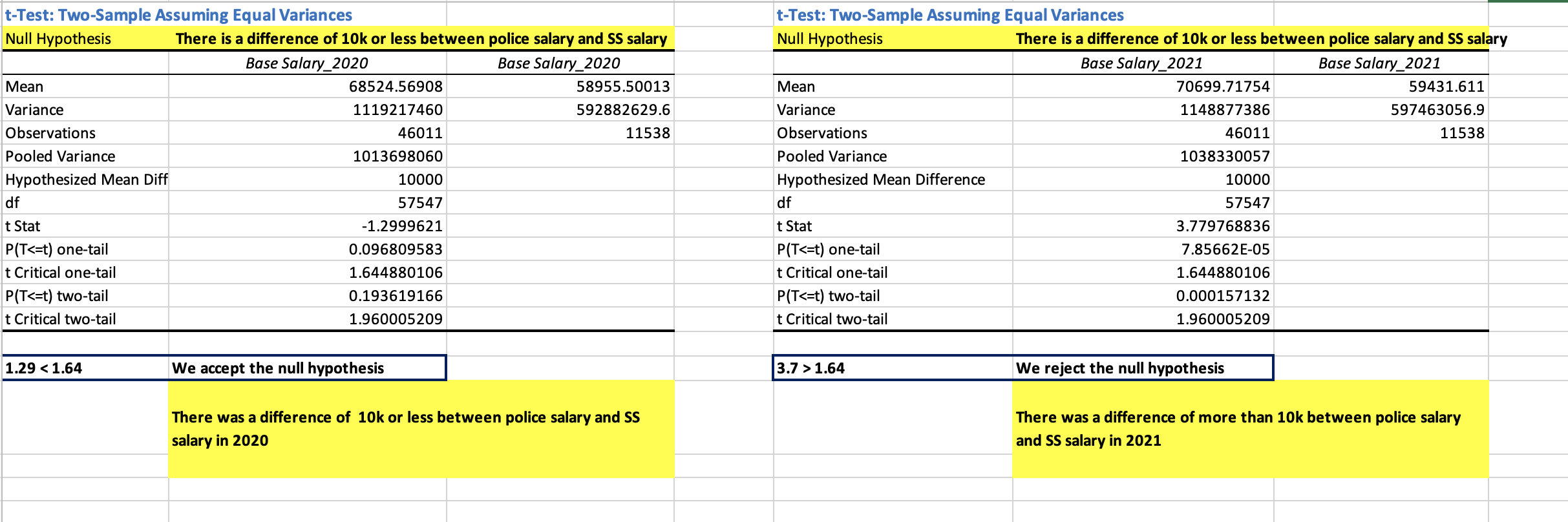
Christina is wrong for 2020 but spot for 2021! Social services was paid less than NYPD in 2021 by more than $10k.
Thought of the Week:
During my work as a data analyst, I generally enjoy presenting cool, new data visualizations to stakeholders across diverse departments and love walking them through complicated dashboards and wacthing them have their Aha! moment when they derive useful insights. However, sometimes I have struggled to push back on certain KPI requests that I find rather redundant and have a hard time explaining to executives that adding more metrics to dashboards won’t necessarily be useful.
A few days back, I came across an interesting article titled “Metrics-Focused Data Strategy with Model-First Data Products - Issue #48” by a group of analysts who write for Modern Data 101. They touched upon the concept of “The Metric Dependency Trees”. In their own words, “The objective of the metrics dependency tree is to understand what positively or negatively triggers the targeted metrics and then aid informed actions to pump the metrics as required. In other words, the metrics dependency tree is a brilliant way to instantly find the root causes (RCA) behind business fluctuations and solve them just as quickly. The metric tree also sheds light on the potential of new or enhanced metrics (metric evolution).”
This is something that a lot of us data analysts can keep in mind while engaging with stakeholders! Check out the complete article here [2].
Until next time!
References:
[1] Excel Workbook [2] Metrics-Focused Data Strategy with Model-First Data Products
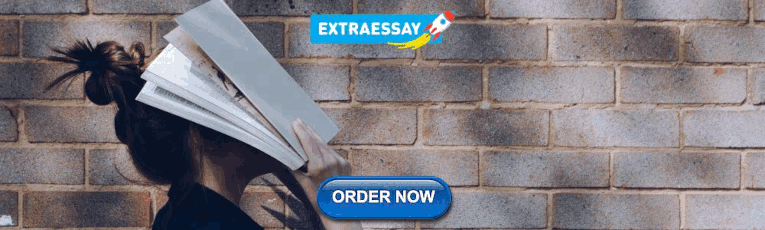
Share this:
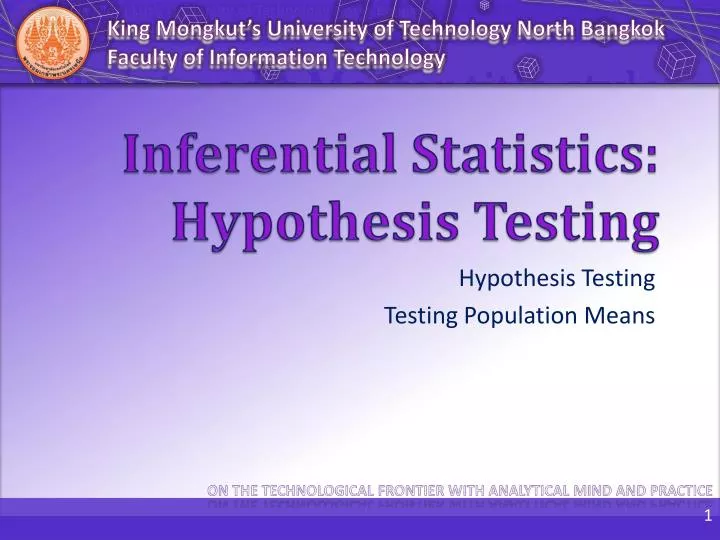
Inferential Statistics: Hypothesis Testing
Mar 30, 2019
860 likes | 1.08k Views
Inferential Statistics: Hypothesis Testing. Hypothesis Testing Testing Population Means. Inferential Statistics. Estimation Estimate population means Estimate population proportion Estimate population variance Hypothesis testing Testing population means
Share Presentation
- independent populations
- university reports
- data collection
- rational conclusion
- hypothesis testing process
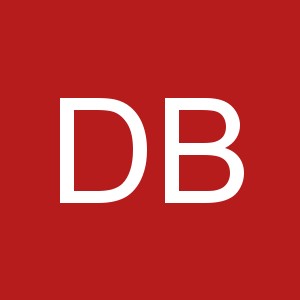
Presentation Transcript
Inferential Statistics: Hypothesis Testing Hypothesis Testing Testing Population Means
Inferential Statistics • Estimation • Estimate population means • Estimate population proportion • Estimate population variance • Hypothesis testing • Testing population means • Testing categorical data / proportion • Testing population variances • Hypothesis about many population means • One-way ANOVA • Two-way ANOVA
Hypothesis Testing • Hypothesis testing is a scientific method used for making decision, conclusion or prove of the finding of research • Perform on data collected from sample based on stated hypotheses • Hypothesis testing process ensure the reproducibility of result conclusion and the ability to infer the hypotheses on the population with certain confidence level
Hypothesis Testing Process • Forming statistical hypothesis / research hypothesis • Define statistical significant level (α) • Usually 0.05 (5%), or 0.01 for research needing higher accuracy • Select and calculate the appropriate statistic from data collected from sample • Accept/reject hypothesis based on corresponding score tables • Make conclusion / decision
Hypothesis • The expected rational conclusion of statistical analysis • Research Hypothesis • Written in text • Statistical Hypothesis • Written in mathematical form using parameter
Research Hypothesis • Text identifying the expected relation from the research • Based on the variable test • Relational hypothesis • Comparative hypothesis • Based on direction of relationship of variables • Directional hypothesis • Non-directional hypothesis
Relational and Comparative • Relational hypothesis focuses on the relationship between 2 or more variables • E.g. Hours of study is related to examination scores • Comparative hypothesis focuses on comparing the difference between 2 or more variables • E.g. Male students and female students have different exam scores
Directional and Non-directional Directional – able to identify the direction of the relationship between variables Non-directional – unable to clearly identify the direction of the relationship between variables
Research Hypothesis Examples • Handwriting and examination score are related • Relational, non-directional • Handwriting and examination score are positivelyrelated • Relational, directional • Female students get higher final exam score than male student • Comparative, directional • The scores of female and male students are different • Comparative, non-directional
Statistical Hypothesis Written in mathematical form Always use population parameter μ(Mu) = population mean σ (sigma) = Standard Deviation σ2 = variance p = population proportion
Statistical Hypothesis • H0: Null hypothesis • Always non-directional • Must have “=” (also “>=” and “<=”) • H1: Alternative hypothesis • Always directional, exclusively opposite to null hypothesis • Must not have “=” (only “>”, “<”, “!=”) • Be very careful when writing statistical hypothesis, some can be tricky
Statistical Hypothesis Examples Female students get higher final exam score than male student H0 : μf <= μm H1 : μf > μm Female students get final exam score higher than or equal to (no less than) male student H0 : ? H1 : ? The scores of female and male students are different H0 : μf = μm H1 : μf != μm
Error in Hypothesis Testing • Type I Error • Error caused by rejecting H0 when H0 is true • Probability of type I error is equal to α which is statistical significant level defined in the analysis • Type II Error • Error caused by accepting H0 when H0 is false • Probability of type I error is equal to β
Error in Hypothesis Testing
Power of Test • The probability to reject null hypothesis (H0) when it is false. • In other words, the sensitivity to accept alternate hypothesis (H1) when it is true • Power of Test depends on • Sufficient size of sample • The process of data collection • Appropriate selection of statistic according to population distribution and assumption of each statistic
Degree of Freedom • The value that indicates the degree of variability under certain criteria • Degree of Freedom (df) usually bind to the number of sample or groups within sample • E.g. in the estimation of mean, 1 of the sample loses its freedom. Therefore, the df is n-1 • If the mean of 5 data item is 10, and first four data item are 7, 9, 11, 13, the last item must be 10 • Thus, under the calculation of mean, the value of the last item cannot vary, losing its freedom
Hypothesis Testing • Directional Test / One-Tailed Test • Right-Tailed H0 : θ <= θk H1 : θ > θk • Left-Tailed H0 : θ >= θk H1 : θ < θk • Non-directional Test / Two-Tailed Test H0 : θ = θk H1 : θ != θk • θ: A population parameter
Testing Hypothesis of Population Mean
Testing Population Mean Single population Two independent populations Two dependent populations (paired samples)
Steps in Testing Mean • Forming statistical hypothesis from research hypothesis • Left-tailed, Right-tailed, Two-tailed • Define statistical significant level (α) • Usually 0.05 (5%), or 0.01 for research needing higher accuracy • Calculated test statistic • Compare the calculated value to critical value from table to determine if it falls into critical region Right-tailed Left-tailed Two-tailed • Finally, make decision/conclusion
Single Population Mean Known population variance σ2 Unknown population variance σ2, small sample (n < 30) Unknown population variance σ2, large sample (n ≥ 30)
Known Population Variance • Test mean of one sample against a test value • E.g. Test if average total score is more than 55 • Assumptions • Variable of interval or ratio scale • Sampling using probabilistic sampling • Each data item is independent of each other • Known population variance
Known Population Variance Hypotheses Critical Region H0 : μ < μ0 H1 : μ > μ0 σ2 known H0 : μ > μ0 H1: μ < μ0 σ2 known
Example 1 A sawmill cuts wood log into 30-inch lumbers. It is known that the variance of the lumber length is 0.5 inch2. To test the machine, 16 lumbers are sampled yielding the average of 30.25 inch. Assuming normal distribution of lumber population, test if this machine is accurate at significant level 0.05 Hypotheses
Example 1 Calculate test statistic Z-score from table The calculated z-score is -1.96 < 1.41 < 1.96, and does not fall in critical region. Thus cannot reject null hypothesis H0 Accept H0 and reject H1 The machine is accurate at significant level 0.05
Example 2 A research of student proficiency in a university reports that the average score is 100 with SD of 16. A researcher then use a proficiency test on 64 sample students from this university. The result is a mean score of 121. Test if the student proficiency increases at significant level 0.01 Hypotheses α = 0.01
Example 2 Calculate test statistic z-score from table The calculated z-score is 10.50 > 2.326, falling in critical region. Reject H0 and accept H1 The student proficiency increases at significant level 0.01
Unknown Population Variance • Assumptions • Variable of interval or ratio scale • Sampling using probabilistic sampling • Each data item is independent of each other • Unknown population variance
Unknown Population Variance, n<30 Hypotheses Critical Region df H0 : μ < μ0 H1 : μ > μ0 σ2 unknown H0 : μ > μ0 H1 : μ < μ0 σ2 unknown
Example 1 A company believe that its employees will work for the company for no less than 8 year. To test this, 16 sample of employee records are study and are found that they work for the company for 5, 9, 7, 11, 11, 8, 3, 7, 6, 8, 6, 4, 8, 2, 5, 6 years. Is the company’s belief true at significant level 0.05 assuming normal distribution of population. Hypothesis H0: ? H1: ? α = 0.05
Example 1 Calculate test statistic
Example 1 t-score from table The calculated t-score is -2.15 < -1.753, falling in left-tailed critical region. Reject H0 and accept H1 Conclusion?
Example 2 H0 : μ < 17 H1 : μ > 17 A study of complementary class with a sample of 25 students. After the class, the average exam score of the sample is 22 with SD of 5.6. Test if this complementary class can increase the score above the criterion of 17 at significant level 0.05. Hypothesis α = 0.05
Example 2 Calculate test statistic t-score from table: t0.05(24)= 1.711 The calculated t-score is 2.50 > 1.711, falling in right-tailed critical region. Reject H0 and accept H1 The complementary class can increase the exam score above the criterion at significant level 0.05
Unknown Population Variance, n ≥ 30 Hypotheses Critical Region H0 : μ < μ0 H1 : μ > μ0 σ2 unknown H0 : μ > μ0 H1 : μ < μ0 σ2 unknown
Example 1 By sampling 100 female deceased in the previous year, it is found that the average lifespan is 71.8 year with SD of 8.9 year. Does this data support the assumption that the average lifespan of current female citizen is more than 70 years at significant level 0.05? Hypothesis α = 0.05
Example 1 Calculate test statistic z-score from table: The calculated z-score is 2.02 > 1.645, falling in right-tailed critical region. Reject H0 and accept H1 The average lifespan of current female citizen is more than 70 years at significant level 0.05
Example 2 According to a company, the food expense of its employees is 550 THB. In order to adjust salaries, the company want to know if this expense increases. From a sample of 30 employee, the average food expense is 590 THB with SD of 90. (Significant level 0.01) Hypothesis α = 0.01
Example 2 Calculate test statistic z-score from table: Z0.01= 2.326 The calculated z-score is 2.434 > 2.326, falling in right-tailed critical region. Reject H0 and accept H1 The food expense of employees increases at significant level 0.01
Two Independent Populations • Known variances of the two populations • Known • Unknown population variances, small sample (n < 30) • Unknown • Unknown population variances, large sample (n ≥ 30) • Unknown • Unknown population variances but known to be equal
Known Variances of Two Populations • Test mean of one sample against another • Assumptions • Variables (samples) are independent of each other • Variables of interval or ratio scale • Sampling using probabilistic sampling from population of normal distribution • Each data item is independent of each other • Known population variances
Known Variances of Two Populations Hypotheses Critical Region H0 : μ1 – μ2< d H1 : μ1 – μ2 > d σ12 ,σ22 known H0 : μ1 – μ2> d H1 : μ1 – μ2 < d σ12 ,σ22 known
Example H0 : μ1 = μ2OR μ1 -μ2 = 0 H1 : μ1 ≠μ2OR μ1 -μ2 ≠ 0 The quality comparison between golf balls of type A and B is based on drive distance. From the sample of 25 golf balls from each group, the average drive distances of type A and B is 275 yards and 290 yards respectively. If both types have the same population variance of 225, assuming normal distribution of drive distance, test if both types are of the same quality. Hypothesis α= 0.05
- More by User
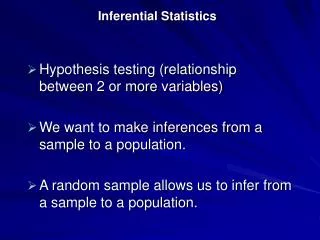
Inferential Statistics
Inferential Statistics. Hypothesis testing (relationship between 2 or more variables) We want to make inferences from a sample to a population. A random sample allows us to infer from a sample to a population. Inferential Statistics. Significance Tests Z scores (one sample case)
601 views • 22 slides
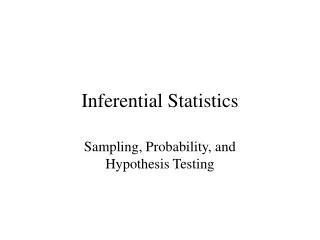
Inferential Statistics. Sampling, Probability, and Hypothesis Testing. Review of Sampling. Population – group of people, communities, or organizations studied. Includes all possible objects of study.
591 views • 26 slides
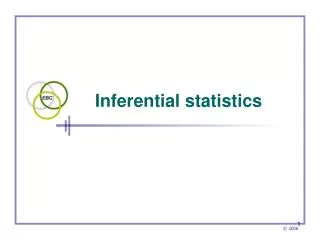
Inferential statistics
Inferential statistics. In inferential statistics. Data from samples are used to make inferences about populations Researchers can make generalizations about an entire population based on a smaller number of observations
1.66k views • 101 slides
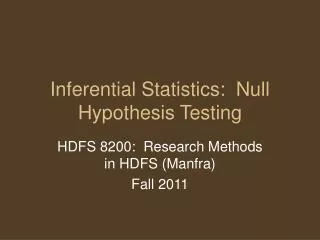
Inferential Statistics: Null Hypothesis Testing
Inferential Statistics: Null Hypothesis Testing. HDFS 8200: Research Methods in HDFS (Manfra) Fall 2011. Review. Generate a substantive hypothesis from the following observation made by a child life specialist who teaches infant massage:
500 views • 27 slides
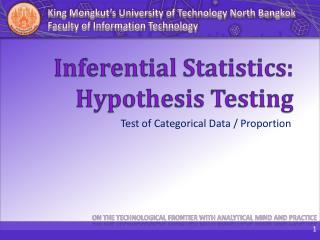
Inferential Statistics: Hypothesis Testing. Test of Categorical Data / Proportion. Inferential Statistics. Estimation Estimate population means Estimate population proportion Estimate population variance Hypothesis testing Testing population means Testing categorical data / proportion
128 views • 0 slides
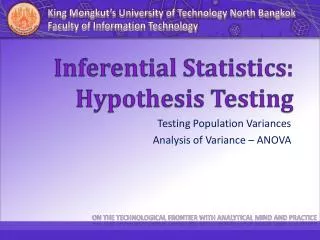
Inferential Statistics: Hypothesis Testing. Testing Population Variances Analysis of Variance – ANOVA. Content. Estimation Estimate population means Estimate population proportion Estimate population variance Hypothesis testing Testing population means
1.16k views • 67 slides
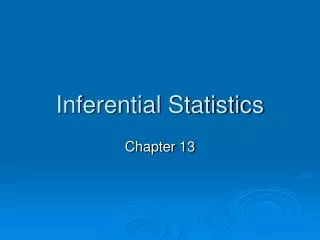
Inferential Statistics. Chapter 13. Inferential Statistics. Inferential stats are used to determine whether we can make statements that the results found in the present experiment reflect a true difference in the entire population of interest and not just the sample used in the experiment.
532 views • 18 slides
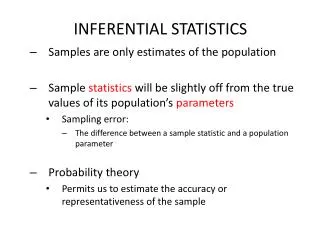
INFERENTIAL STATISTICS
INFERENTIAL STATISTICS. Samples are only estimates of the population Sample statistics will be slightly off from the true values of its population’s parameters Sampling error: The difference between a sample statistic and a population parameter Probability theory
580 views • 17 slides
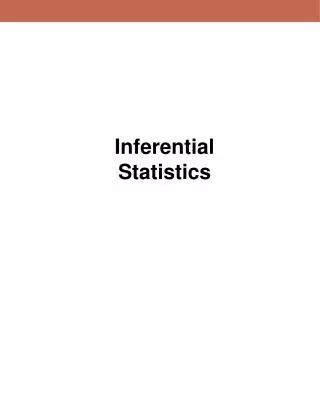
Inferential Statistics. Inferential Statistics. With inferential statistics you can do the following:
558 views • 41 slides
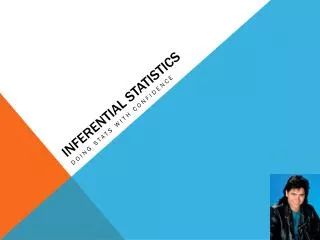
Inferential Statistics. Doing stats with confidence . Greek symbols everywhere . Greek symbols everywhere . Population: the total set of items that we are concerned about Parameter: a measure used to summarize a population (could include mean, median, standard deviation)
487 views • 36 slides
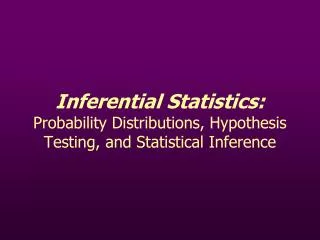
Inferential Statistics: Probability Distributions, Hypothesis Testing, and Statistical Inference
Inferential Statistics: Probability Distributions, Hypothesis Testing, and Statistical Inference. Key Lessons from the Shark/Spider/ Candybug /Fiddler Labs.
440 views • 19 slides
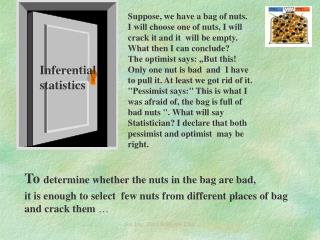
Suppose, we have a bag of nuts. I will choose one of nut s , I will crack it and it will be empty. What then I can conclude? The optimist says: „ But this! O nly one nut is bad and I have to pull it. At least we got rid of it.
505 views • 30 slides
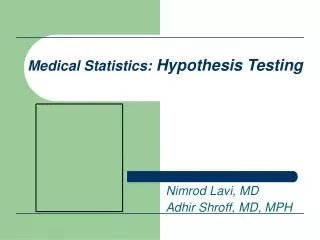
Medical Statistics: Hypothesis Testing
Medical Statistics: Hypothesis Testing. Nimrod Lavi, MD Adhir Shroff, MD, MPH. Agenda. Types of variables Descriptive statistics What is a hypothesis Definition of a p-value Sample vs. universe Comparative statistics T-tests Chi-square. Agenda. Types of variables
798 views • 56 slides
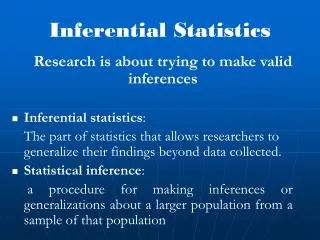
Inferential Statistics. Research is about trying to make valid inferences. Inferential statistics : T he part of statistics that allows researchers to generalize their findings beyond data collected. Statistical inference :
2.84k views • 104 slides
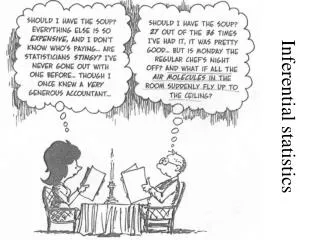
Inferential statistics. Why statistics are important. Statistics are concerned with difference – how much does one feature of an environment differ from another Magnitude : The comparative strength of two variables.
722 views • 42 slides
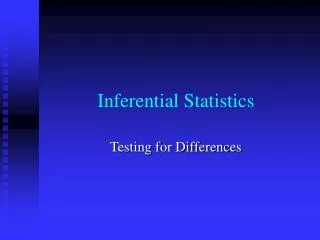
Inferential Statistics. Testing for Differences. Introduction. Whether the research design is experimental, quasi-experimental, or non-experimental, many researchers develop their studies to look for differences
334 views • 16 slides
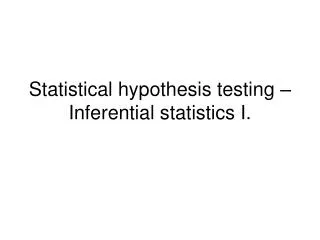
Statistical hypothesis testing – Inferential statistics I.
Statistical hypothesis testing – Inferential statistics I. What is hypothesis testing?. Hypothesis : a theoretical statement concerning a certain feature of the studied statistical population . We want to know if our hypotheses are true or not by doing research.
390 views • 10 slides
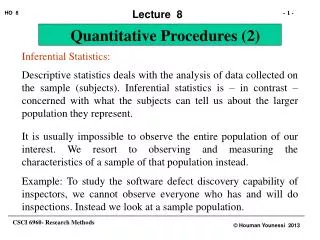
Inferential Statistics:
Inferential Statistics:. Descriptive statistics deals with the analysis of data collected on the sample (subjects). Inferential statistics is – in contrast – concerned with what the subjects can tell us about the larger population they represent.
728 views • 23 slides
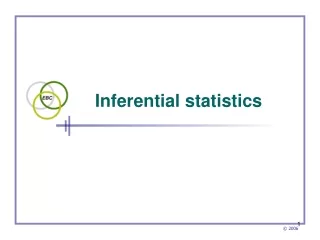
1.06k views • 101 slides
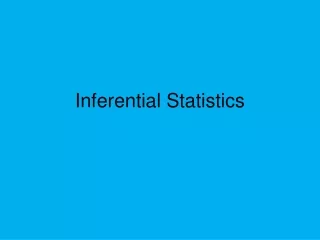
Inferential Statistics. Which Statistic Do I Use?. Dependent Variable Type Continuous Categorical Number of Factors (Independent Variables) One Two or More (Factorial Analysis) Number of Levels (of the Independent Variable) Two Three or More (Between or Repeated Measures).
369 views • 28 slides
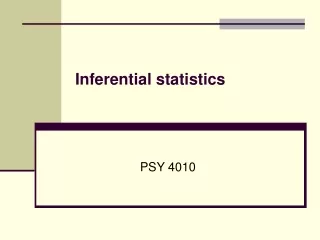
Inferential statistics. PSY 4010. Central concepts in inferential statistics:. Sampling error Sampling distribution Standard error Null hypothesis and alternative hypothesis Level of significance Type I and Type II error One-tailed and two-tailed tests Degrees of freedom
435 views • 37 slides
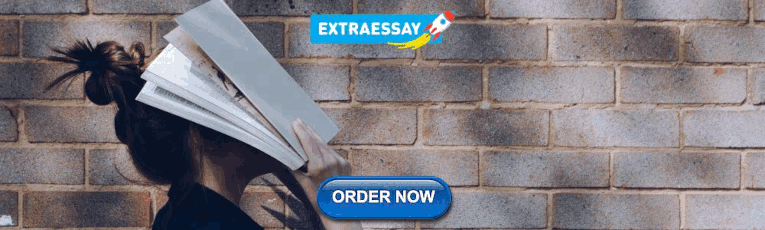
IMAGES
VIDEO
COMMENTS
Refers to a contrast between groups of participants who were assigned to groups through matched pairs, natural pairs , or repeated measures. We are essentially comparing scores within the same participants (subjects). Test‐Retest, Time1‐Time2, Trial Types.
Inferential Statistics - Download as a PDF or view online for free ... Inferential statistics.ppt. ... • Summarize • Simplify • Presentation of data Inferential Statistics • Generalize from samples to pops • Hypothesis testing • Relationships among variables Describing data Make predictions 3.
Hypothesis testing ppt final. ... Inferential statistics/ Hypothesis testing 8 9. Outline Concept Motivation Example: Quality Control Problem Null & Alternative Hypothesis One & Two - tailed tests Type I & Type II errors Courtroom Analogy Fallacies in Statistical Hypothesis Testing Example: ...
Inferences drawn from statistics. Test hypothesis with "test statistic". z-scores (for now...) Examine if obtained difference is different than what is expected by chance. When you reject the null hypothesis: "The findings are statistically significant.". When you fail to reject the null hypothesis: "There was no evidence found that
Inferential Statistics pt. 2. A hypothesis test is a statistical test that is used to determine whether there is enough evidence in a sample of data to infer that a certain condition is true for the entire population. A hypothesis test examines two opposing hypotheses about a population: the null hypothesis and the alternative hypothesis.
Basic Concepts Underlying Inferential Statistics. Level of Significance. For every test, the researcher must select a minimum value that the statistical test must exceed to be regarded as significant. Generally, the larger the sample size the smaller the test score must be to reach statistical significance.
Hypothesis Testing in the Real World. Hypothesis Testing in the Real World. Thus far, Hypothesis testing has been presented for situations in which the population variances are known. T-tests allow you to compare means for which the population variances are unknown T-test for a single sample. Hypothesis Testing in the Real World. 2k views ...
The confusing thing is that we are not directly testing whether or not there is a treatment effect, or relationship ! We are testing how consistent the data is with the hypothesis that there is no treatment effect, relationship, etc. ! Thus, a treatment effect is demonstrated indirectly if the data is inconsistent with the null hypothesis
Inferential statistics is not used to prove hypotheses. It is used to demonstrate that null hypotheses are not true. Think about this as steps: You want to know that something is true but you can't absolutely know because you can't test all cases. Instead you focus on the opposite of what you want to know is true (the null hypothesis).
9 Basic Logic of Hypothesis Testing (1) State a hypothesis about the population Hypothesis: prediction about the relationship between variables; how IV affects DV e.g. People who prefer Hagen Daaz ice cream will have a mean IQ that is higher than average at 130 (2) Use the hypothesis to predict the characteristics the sample should have (3) Obtain a random sample random sampling: when all ...
Example: Inferential statistics. You randomly select a sample of 11th graders in your state and collect data on their SAT scores and other characteristics. You can use inferential statistics to make estimates and test hypotheses about the whole population of 11th graders in the state based on your sample data.
This document discusses inferential statistics and hypothesis testing. It introduces inferential statistics, which is used to draw inferences from samples, and covers topics like t-tests, correlation coefficients, and chi-square tests. Hypothesis testing is defined as a statistical method used to make decisions using experimental data. The basics of hypothesis testing, parameters like the null ...
Mini Lecture Inferential Statistics & Hypothesis Testing. Probability and POPULATIONs. Inferential statistics. based on the laws of probability. able to draw conclusions about population parameters based on statistics from a representative sample. estimate population parameters from sample statistics. To estimate population parameters best to ...
Download ppt "Inferential Statistics". Aren't you the lucky ones. This lecture is the last one for this course and is about the formal statistical testing of research hypotheses. We will get to defining formal research hypotheses suitable for statistical inference testing below, but before we do a brief reprise on research questions is in order.
4 PART III: PROBABILITY AND THE FOUNDATIONS OF INFERENTIAL STATISTICS 8.2 FOUR STEPS TO HYPOTHESIS TESTING The goal of hypothesis testing is to determine the likelihood that a population parameter, such as the mean, is likely to be true. In this section, we describe the four steps of hypothesis testing that were briefly introduced in Section 8.1:
From this data, test if the proportion of defect is more than 2% at significant level 0.05. Hypotheses α = 0.05. Example 1 Calculate test statistic z-score from table: z0.05 = 1.645 The calculated z-score is 1.429 < 1.645, not falling in right-tailed critical region.
Inferential Statistics: An Introduction. 16 1. Statistics as a subject area has a vast scope and application. It finds its application in fields like policy planning, management, education, marketing, agriculture, medicine and so on, though, one of its major application is in research. 2.
Hypothesis Testing. Overview. This is the other part of inferential statistics, hypothesis testing Hypothesis testing and estimation are two different approaches to two similar problems Estimation is the process of using sample data to estimate the value of a population parameter. 1.72k views • 126 slides
Inferential statistics provides us with a way to test this hypothesis, i.e., make a decision about the relationship between exercise and quality of life in depressed adolescent patients. To test our hypothesis, we need to reformulate it as two statements or hypotheses, the null hypothesis and the alternative hypothesis.
Episode 12: Inferential Statistics and Hypothesis Testing Playbook Thursday, 04/25/2024. This post acts as a handy cheat sheet on how to draw important inferences from a given dataset using statistics and hypothesis testing. Dataset: Citywide Payroll Data (Fiscal Year) was taken from "Data.gov". Each record represents the following ...
From the sample of 25 golf balls from each group, the average drive distances of type A and B is 275 yards and 290 yards respectively. If both types have the same population variance of 225, assuming normal distribution of drive distance, test if both types are of the same quality. Hypothesis α= 0.05.
Statistical Modeling. (6 Editable Slides) Details. Reviews. Download our Inferential Statistics PPT template to describe the process, which helps make predictions and generalizations from the data sample taken from a population. Statisticians and data analysts can use this fully customizable deck to discuss how this branch of statistics ...
14. Step 5: Critical value Demystifying statistics! - Lecture 4 SBCM, Joint Program - RiyadhSBCM, Joint Program - Riyadh • Critical value - a line on a graph that splits the graph into sections. One or two of the sections is the "rejection region"; if your test value falls into that region, then you reject the null hypothesis • These values are obtained from statistical tables ...