Advertisement
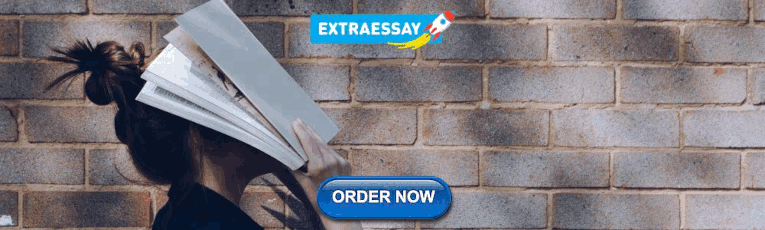
Major Depressive Disorder: Advances in Neuroscience Research and Translational Applications
- Open access
- Published: 13 February 2021
- Volume 37 , pages 863–880, ( 2021 )
Cite this article
You have full access to this open access article
- Zezhi Li 1 , 2 ,
- Meihua Ruan 3 ,
- Jun Chen 1 , 5 &
- Yiru Fang ORCID: orcid.org/0000-0002-8748-9085 1 , 4 , 5
112 Citations
16 Altmetric
Explore all metrics
A Correction to this article was published on 17 May 2021
This article has been updated
Major depressive disorder (MDD), also referred to as depression, is one of the most common psychiatric disorders with a high economic burden. The etiology of depression is still not clear, but it is generally believed that MDD is a multifactorial disease caused by the interaction of social, psychological, and biological aspects. Therefore, there is no exact pathological theory that can independently explain its pathogenesis, involving genetics, neurobiology, and neuroimaging. At present, there are many treatment measures for patients with depression, including drug therapy, psychotherapy, and neuromodulation technology. In recent years, great progress has been made in the development of new antidepressants, some of which have been applied in the clinic. This article mainly reviews the research progress, pathogenesis, and treatment of MDD.
Similar content being viewed by others
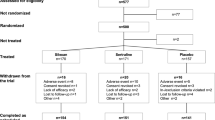
Lavender oil preparation Silexan is effective in mild-to-moderate major depression: a randomized, placebo- and reference-controlled trial
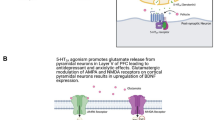
Molecular Mechanisms of Psilocybin and Implications for the Treatment of Depression
Psilocybin with psychological support for treatment-resistant depression: six-month follow-up.
Avoid common mistakes on your manuscript.
Major depressive disorder (MDD) also referred to as depression, is one of the most severe and common psychiatric disorders across the world. It is characterized by persistent sadness, loss of interest or pleasure, low energy, worse appetite and sleep, and even suicide, disrupting daily activities and psychosocial functions. Depression has an extreme global economic burden and has been listed as the third largest cause of disease burden by the World Health Organization since 2008, and is expected to rank the first by 2030 [ 1 , 2 ]. In 2016, the Global Burden of Diseases, Injuries, and Risk Factors Study demonstrated that depression caused 34.1 million of the total years lived with disability (YLDs), ranking as the fifth largest cause of YLD [ 3 ]. Therefore, the research progress and the clinical application of new discoveries or new technologies are imminent. In this review, we mainly discuss the current situation of research, developments in pathogenesis, and the management of depression.
Current Situation of Research on Depression
Analysis of published papers.
In the past decade, the total number of papers on depression published worldwide has increased year by year as shown in Fig. 1 A. Searching the Web of Science database, we found a total of 43,863 papers published in the field of depression from 2009 to 2019 (search strategy: TI = (depression$) or ts = ("major depressive disorder$")) and py = (2009 – 2019), Articles). The top 10 countries that published papers on the topic of depression are shown in Fig. 1 B. Among them, researchers in the USA published the most papers, followed by China. Compared with the USA, the gap in the total number of papers published in China is gradually narrowing (Fig. 1 C), but the quality gap reflected by the index (the total number of citations and the number of citations per paper) is still large, and is lower than the global average (Fig. 1 D). As shown in Fig. 1 E, the hot research topics in depression are as follows: depression management in primary care, interventions to prevent depression, the pathogenesis of depression, comorbidity of depression and other diseases, the risks of depression, neuroimaging studies of depression, and antidepressant treatment.
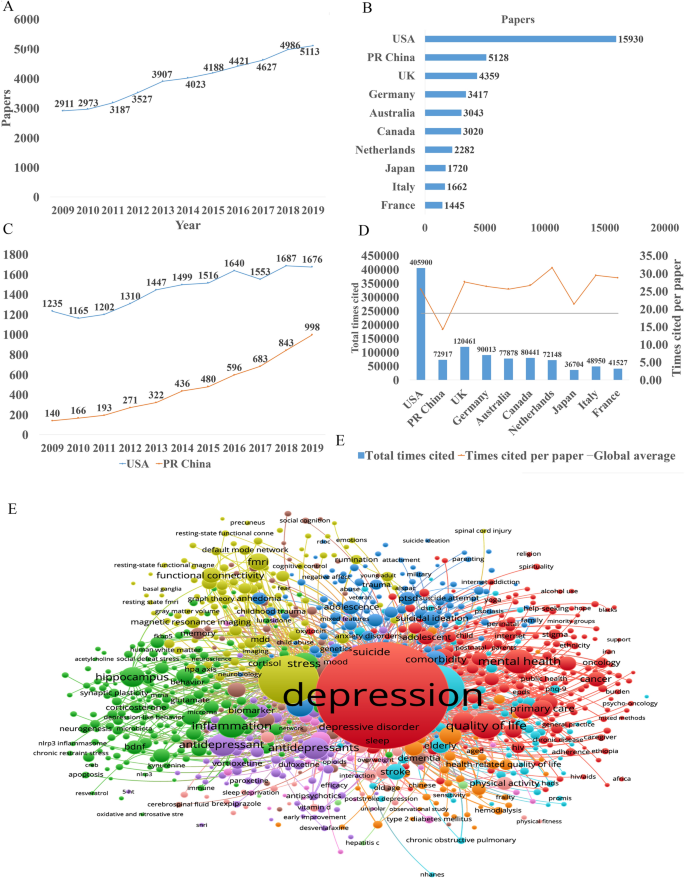
Analysis of published papers around the world from 2009 to 2019 in depressive disorder. A The total number of papers [from a search of the Web of Science database (search strategy: TI = (depression$) or ts = ("major depressive disorder$")) and py = (2009 – 2019), Articles)]. B The top 10 countries publishing on the topic. C Comparison of papers in China and the USA. D Citations for the top 10 countries and comparison with the global average. E Hot topics.
Analysis of Patented Technology Application
There were 16,228 patent applications in the field of depression between 2009 and 2019, according to the Derwent Innovation Patent database. The annual number and trend of these patents are shown in Fig. 2 A. The top 10 countries applying for patents related to depression are shown in Fig. 2 B. The USA ranks first in the number of depression-related patent applications, followed by China. The largest number of patents related to depression is the development of antidepressants, and drugs for neurodegenerative diseases such as dementia comorbid with depression. The top 10 technological areas of patents related to depression are shown in Fig. 2 C, and the trend in these areas have been stable over the past decade (Fig. 2 D).
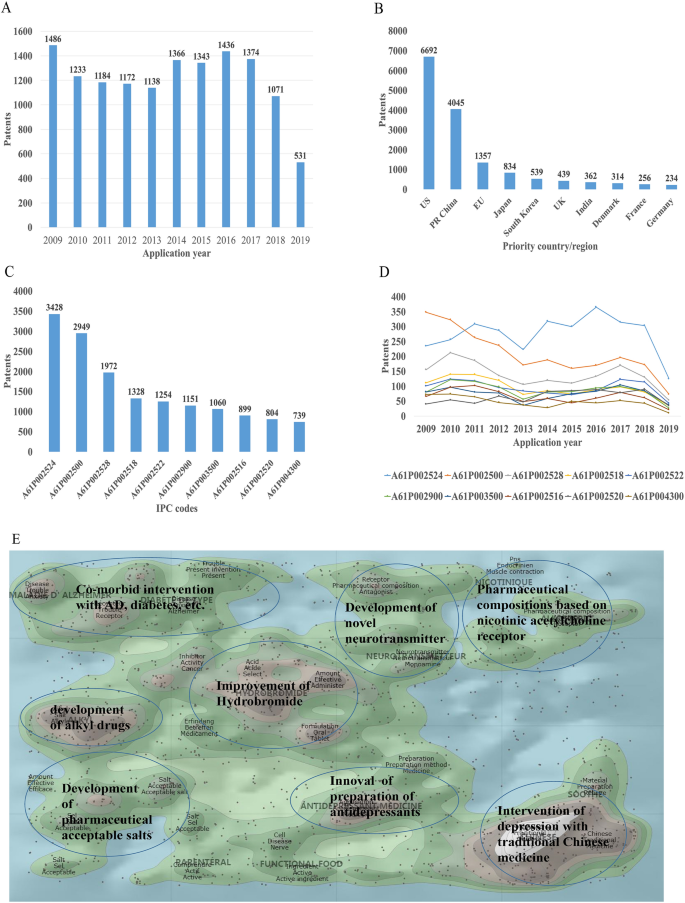
Analysis of patented technology applications from 2009 to 2019 in the field of depressive disorder. A Annual numbers and trends of patents (the Derwent Innovation patent database). B The top 10 countries/regions applying for patents. C The top 10 technological areas of patents. D The trend of patent assignees. E Global hot topic areas of patents.
Analysis of technical hotspots based on keyword clustering was conducted from the Derwent Innovation database using the "ThemeScape" tool. This demonstrated that the hot topic areas are as follows (Fig. 2 E): (1) improvement for formulation and the efficiency of hydrobromide, as well as optimization of the dosage; intervention for depression comorbid with AD, diabetes, and others; (3) development of alkyl drugs; (4) development of pharmaceutical acceptable salts as antidepressants; (5) innovation of the preparation of antidepressants; (6) development of novel antidepressants based on neurotransmitters; (7) development of compositions based on nicotinic acetylcholine receptors; and (8) intervention for depression with traditional Chinese medicine.
Analysis of Clinical Trial
There are 6,516 clinical trials in the field of depression in the ClinicalTrials.gov database, and among them, 1,737 valid trials include the ongoing recruitment of subjects, upcoming recruitment of subjects, and ongoing clinical trials. These clinical trials are mainly distributed in the USA (802 trials), Canada (155), China (114), France (93), Germany (66), UK (62), Spain (58), Denmark (41), Sweden (39), and Switzerland (23). The indications for clinical trials include various types of depression, such as minor depression, depression, severe depression, perinatal depression, postpartum depression, and depression comorbid with other psychiatric disorders or physical diseases, such as schizophrenia, epilepsy, stroke, cancer, diabetes, cardiovascular disease, and Parkinson's disease.
Based on the database of the Chinese Clinical Trial Registry website, a total of 143 clinical trials for depression have been carried out in China. According to the type of research, they are mainly interventional and observational studies, as well as a small number of related factor studies, epidemiological studies, and diagnostic trials. The research content involves postpartum, perinatal, senile, and other age groups with clinical diagnosis (imaging diagnosis) and intervention studies (drugs, acupuncture, electrical stimulation, transcranial magnetic stimulation). It also includes intervention studies on depression comorbid with coronary heart disease, diabetes, and heart failure.
New Medicine Development
According to the Cortellis database, 828 antidepressants were under development by the end of 2019, but only 292 of these are effective and active (Fig. 3 A). Large number of them have been discontinued or made no progress, indicating that the development of new drugs in the field of depression is extremely urgent.
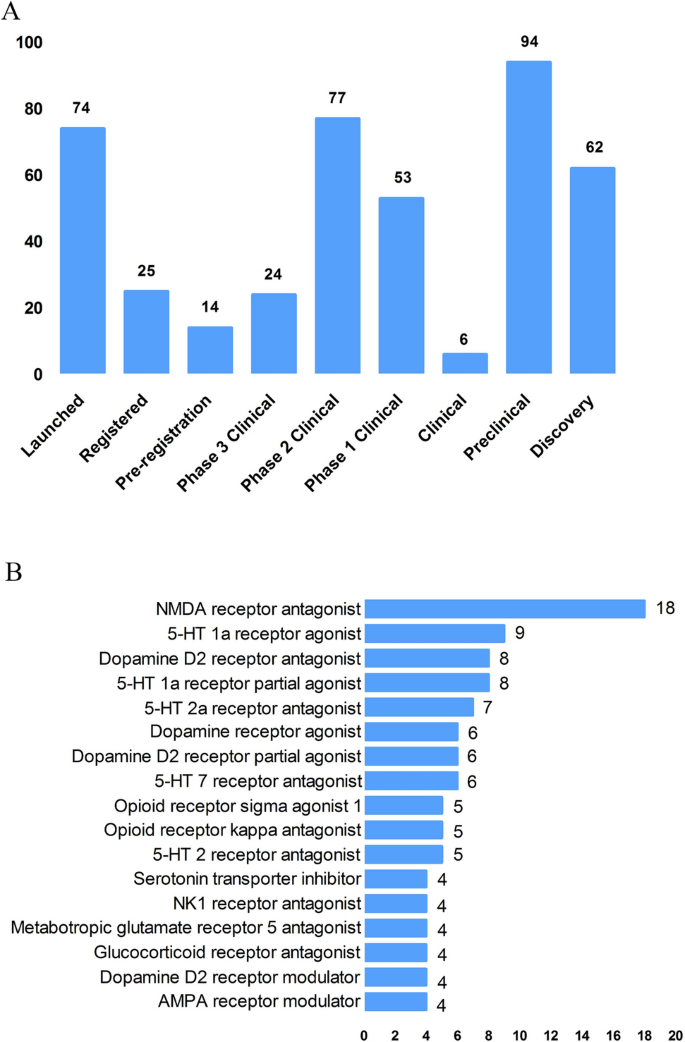
New medicine development from 2009 to 2019 in depressive disorder. A Development status of new candidate drugs. B Top target-based actions.
From the perspective of target-based actions, the most common new drugs are NMDA receptor antagonists, followed by 5-HT targets, as well as dopamine receptor agonists, opioid receptor antagonists and agonists, AMPA receptor modulators, glucocorticoid receptor antagonists, NK1 receptor antagonists, and serotonin transporter inhibitors (Fig. 3 B).
Epidemiology of Depression
The prevalence of depression varies greatly across cultures and countries. Previous surveys have demonstrated that the 12-month prevalence of depression was 0.3% in the Czech Republic, 10% in the USA, 4.5% in Mexico, and 5.2% in West Germany, and the lifetime prevalence of depression was 1.0% in the Czech Republic, 16.9% in the USA, 8.3% in Canada, and 9.0% in Chile [ 4 , 5 ]. A recent meta-analysis including 30 Countries showed that lifetime and 12-month prevalence depression were 10.8% and 7.2%, respectively [ 6 ]. In China, the lifetime prevalence of depression ranged from 1.6% to 5.5% [ 7 , 8 , 9 ]. An epidemiological study demonstrated that depression was the most common mood disorder with a life prevalence of 3.4% and a 12-month prevalence of 2.1% in China [ 10 ].
Some studies have also reported the prevalence in specific populations. The National Comorbidity Survey-Adolescent Supplement (NCS-A) survey in the USA showed that the lifetime and 12-month prevalence of depression in adolescents aged 13 to 18 were 11.0% and 7.5%, respectively [ 11 ]. A recent meta-analysis demonstrated that lifetime prevalence and 12-month prevalence were 2.8% and 2.3%, respectively, among the elderly population in China [ 12 ].
Neurobiological Pathogenesis of Depressive Disorder
The early hypothesis of monoamines in the pathophysiology of depression has been accepted by the scientific community. The evidence that monoamine oxidase inhibitors and tricyclic antidepressants promote monoamine neurotransmission supports this theory of depression [ 13 ]. So far, selective serotonin reuptake inhibitors and norepinephrine reuptake inhibitors are still the first-line antidepressants. However, there remain 1/3 to 2/3 of depressed patients who do not respond satisfactorily to initial antidepressant treatment, and even as many as 15%–40% do not respond to several pharmacological medicines [ 14 , 15 ]. Therefore, the underlying pathogenesis of depression is far beyond the simple monoamine mechanism.
Other hypotheses of depression have gradually received increasing attention because of biomarkers for depression and the effects pharmacological treatments, such as the stress-responsive hypothalamic pituitary adrenal (HPA) axis, neuroendocrine systems, the neurotrophic family of growth factors, and neuroinflammation.
Stress-Responsive HPA Axis
Stress is causative or a contributing factor to depression. Particularly, long-term or chronic stress can lead to dysfunction of the HPA axis and promote the secretion of hormones, including cortisol, adrenocorticotropic hormone, corticotropin-releasing hormone, arginine vasopressin, and vasopressin. About 40%–60% of patients with depression display a disturbed HPA axis, including hypercortisolemia, decreased rhythmicity, and elevated cortisol levels [ 16 , 17 ]. Mounting evidence has shown that stress-induced abnormality of the HPA axis is associated with depression and cognitive impairment, which is due to the increased secretion of cortisol and the insufficient inhibition of glucocorticoid receptor regulatory feedback [ 18 , 19 ]. In addition, it has been reported that the increase in cortisol levels is related to the severity of depression, especially in melancholic depression [ 20 , 21 ]. Further, patients with depression whose HPA axis was not normalized after treatment had a worse clinical response and prognosis [ 22 , 23 ]. Despite the above promising insights, unfortunately previous studies have shown that treatments regulating the HPA axis, such as glucocorticoid receptor antagonists, do not attenuate the symptoms of depressed patients [ 24 , 25 ].
Glutamate Signaling Pathway
Glutamate is the main excitatory neurotransmitter released by synapses in the brain; it is involved in synaptic plasticity, cognitive processes, and reward and emotional processes. Stress can induce presynaptic glutamate secretion by neurons and glutamate strongly binds to ionotropic glutamate receptors (iGluRs) including N-methyl-D-aspartate receptors (NMDARs) and α-amino-3-hydroxy-5-methyl-4-isoxazole-propionic acid receptors (AMPARs) [ 26 ] on the postsynaptic membrane to activate downstream signal pathways [ 27 ]. Accumulating evidence has suggested that the glutamate system is associated with the incidence of depression. Early studies have shown increased levels of glutamate in the peripheral blood, cerebrospinal fluid, and brain of depressed patients [ 28 , 29 ], as well as NMDAR subunit disturbance in the brain [ 30 , 31 ]. Blocking the function of NMDARs has an antidepressant effect and protects hippocampal neurons from morphological abnormalities induced by stress, while antidepressants reduce glutamate secretion and NMDARs [ 32 ]. Most importantly, NMDAR antagonists such as ketamine have been reported to have profound and rapid antidepressant effects on both animal models and the core symptoms of depressive patients [ 33 ]. On the other hand, ketamine can also increase the AMPAR pathway in hippocampal neurons by up-regulating the AMPA glutamate receptor 1 subunit [ 34 ]. Further, the AMPAR pathway may be involved in the mechanism of antidepressant effects. For example, preclinical studies have indicated that AMPAR antagonists might attenuate lithium-induced depressive behavior by increasing the levels of glutamate receptors 1 and 2 in the mouse hippocampus [ 35 ].
Gamma-Aminobutyric Acid (GABA)
Contrary to glutamate, GABA is the main inhibitory neurotransmitter. Although GABA neurons account for only a small proportion compared to glutamate, inhibitory neurotransmission is essential for brain function by balancing excitatory transmission [ 36 ]. Number of studies have shown that patients with depression have neurotransmission or functional defects of GABA [ 37 , 38 ]. Schür et al ., conducted a meta-analysis of magnetic resonance spectroscopy studies, which showed that the brain GABA level in depressive patients was lower than that in healthy controls, but no difference was found in depressive patients in remission [ 39 ]. Several postmortem studies have shown decreased levels of the GABA synthase glutamic acid decarboxylase in the prefrontal cortex of patients with depression [ 40 , 41 ]. It has been suggested that a functional imbalance of the GABA and glutamate systems contributes to the pathophysiology of depression, and activation of the GABA system might induce antidepressant activity, by which GABA A receptor mediators α2/α3 are considered potential antidepressant candidates [ 42 , 43 ]. Genetic mouse models, such as the GABA A receptor mutant mouse and conditional the Gad1-knockout mouse (GABA in hippocampus and cerebral cortex decreased by 50%) and optogenetic methods have verified that depression-like behavior is induced by changing the level of GABA [ 44 , 45 ].
Neurotrophin Family
The neurotrophin family plays a key role in neuroplasticity and neurogenesis. The neurotrophic hypothesis of depression postulates that a deficit of neurotrophic support leads to neuronal atrophy, the reduction of neurogenesis, and the destruction of glia support, while antidepressants attenuate or reverse these pathophysiological processes [ 46 ]. Among them, the most widely accepted hypothesis involves brain-derived neurotrophic factor (BDNF). This was initially triggered by evidence that stress reduces the BDNF levels in the animal brain, while antidepressants rescue or attenuate this reduction [ 47 , 48 ], and agents involved in the BDNF system have been reported to exert antidepressant-like effects [ 49 , 50 ]. In addition, mounting studies have reported that the BDNF level is decreased in the peripheral blood and at post-mortem in depressive patients, and some have reported that antidepressant treatment normalizes it [ 51 , 52 ]. Furthermore, some evidence also showed that the interaction of BDNF and its receptor gene is associated with treatment-resistant depression [ 15 ].
Recent studies reported that depressed patients have a lower level of the pro-domain of BDNF (BDNF pro-peptide) than controls. This is located presynaptically and promotes long-term depression in the hippocampus, suggesting that it is a promising synaptic regulator [ 53 ].
Neuroinflammation
The immune-inflammation hypothesis has attracted much attention, suggesting that the interactions between inflammatory pathways and neural circuits and neurotransmitters are involved in the pathogenesis and pathophysiological processes of depression. Early evidence found that patients with autoimmune or infectious diseases are more likely to develop depression than the general population [ 54 ]. In addition, individuals without depression may display depressive symptoms after treatment with cytokines or cytokine inducers, while antidepressants relieve these symptoms [ 55 , 56 ]. There is a complex interaction between the peripheral and central immune systems. Previous evidence suggested that peripheral inflammation/infection may spread to the central nervous system in some way and cause a neuroimmune response [ 55 , 57 ]: (1) Some cytokines produced in the peripheral immune response, such as IL-6 and IL-1 β, can leak into the brain through the blood-brain barrier (BBB). (2) Cytokines entering the central nervous system act directly on astrocytes, small stromal cells, and neurons. (3) Some peripheral immune cells can cross the BBB through specific transporters, such as monocytes. (4) Cytokines and chemokines in the circulation activate the central nervous system by regulating the surface receptors of astrocytes and endothelial cells at the BBB. (5) As an intermediary pathway, the immune inflammatory response transmits peripheral danger signals to the center, amplifies the signals, and shows the external phenotype of depressive behavior associated with stress/trauma/infection. (6) Cytokines and chemokines may act directly on neurons, change their plasticity and promote depression-like behavior.
Patients with depression show the core feature of the immune-inflammatory response, that is, increased concentrations of pro-inflammatory cytokines and their receptors, chemokines, and soluble adhesion molecules in peripheral blood and cerebrospinal fluid [ 58 , 59 , 60 ]. Peripheral immune-inflammatory response markers not only change the immune activation state in the brain that affects explicit behavior, but also can be used as an evaluation index or biological index of antidepressant therapy [ 61 , 62 ]. Li et al . showed that the level of TNF-α in patients with depression prior to treatment was higher than that in healthy controls. After treatment with venlafaxine, the level of TNF-α in patients with depression decreased significantly, and the level of TNF-α in the effective group decreased more [ 63 ]. A recent meta-analysis of 1,517 patients found that antidepressants significantly reduced peripheral IL-6, TNF-α, IL-10, and CCL-2, suggesting that antidepressants reduce markers of peripheral inflammatory factors [ 64 ]. Recently, Syed et al . also confirmed that untreated patients with depression had higher levels of inflammatory markers and increased levels of anti-inflammatory cytokines after antidepressant treatment, while increased levels of pro-inflammatory cytokines were found in non-responders [ 62 ]. Clinical studies have also found that anti-inflammatory cytokines, such as monoclonal antibodies and other cytokine inhibitors, may play an antidepressant role by blocking cytokines. The imbalance of pro-inflammatory and anti-inflammatory cytokines may be involved in the pathophysiological process of depression.
In addition, a recent study showed that microglia contribute to neuronal plasticity and neuroimmune interaction that are involved in the pathophysiology of depression [ 65 ]. When activated microglia promote inflammation, especially the excessive production of pro-inflammatory factors and cytotoxins in the central nervous system, depression-like behavior can gradually develop [ 65 , 66 ]. However, microglia change polarization as two types under different inflammatory states, regulating the balance of pro- and anti-inflammatory factors. These two types are M1 and M2 microglia; the former produces large number of pro-inflammatory cytokines after activation, and the latter produces anti-inflammatory cytokines. An imbalance of M1/M2 polarization of microglia may contribute to the pathophysiology of depression [ 67 ].
Microbiome-Gut-Brain Axis
The microbiota-gut-brain axis has recently gained more attention because of its ability to regulate brain activity. Many studies have shown that the microbiota-gut-brain axis plays an important role in regulating mood, behavior, and neuronal transmission in the brain [ 68 , 69 ]. It is well established that comorbidity of depression and gastrointestinal diseases is common [ 70 , 71 ]. Some antidepressants can attenuate the symptoms of patients with irritable bowel syndrome and eating disorders [ 72 ]. It has been reported that gut microbiome alterations are associated with depressive-like behaviors [ 73 , 74 ], and brain function [ 75 ]. Early animal studies have shown that stress can lead to long-term changes in the diversity and composition of intestinal microflora, and is accompanied by depressive behavior [ 76 , 77 ]. Interestingly, some evidence indicates that rodents exhibit depressive behavior after fecal transplants from patients with depression [ 74 ]. On the other hand, some probiotics attenuated depressive-like behavior in animal studies, [ 78 ] and had antidepressant effects on patients with depression in several double-blind, placebo-controlled clinical trials [ 79 , 80 ].
The potential mechanism may be that gut microbiota can interact with the brain through a variety of pathways or systems, including the HPA axis, and the neuroendocrine, autonomic, and neuroimmune systems [ 81 ]. For example, recent evidence demonstrated that gut microbiota can affect the levels of neurotransmitters in the gut and brain, including serotonin, dopamine, noradrenalin, glutamate, and GABA [ 82 ]. In addition, recent studies showed that changes in gut microbiota can also impair the gut barrier and promote higher levels of peripheral inflammatory cytokines [ 83 , 84 ]. Although recent research in this area has made significant progress, more clinical trials are needed to determine whether probiotics have any effect on the treatment of depression and what the potential underlying mechanisms are.
Other Systems and Pathways
There is no doubt that several other systems or pathways are also involved in the pathophysiology of depression, such as oxidant-antioxidant imbalance [ 85 ], mitochondrial dysfunction [ 86 , 87 ], and circadian rhythm-related genes [ 88 ], especially their critical interactions ( e.g. interaction between the HPA and mitochondrial metabolism [ 89 , 90 ], and the reciprocal interaction between oxidative stress and inflammation [ 2 , 85 ]). The pathogenesis of depression is complex and all the hypotheses should be integrated to consider the many interactions between various systems and pathways.
Advances in Various Kinds of Research on Depressive Disorder
Genetic, molecular, and neuroimaging studies continue to increase our understanding of the neurobiological basis of depression. However, it is still not clear to what extent the results of neurobiological studies can help improve the clinical and functional prognosis of patients. Therefore, over the past 10 years, the neurobiological study of depression has become an important measure to understand the pathophysiological mechanism and guide the treatment of depression.
Genetic Studies
Previous twin and adoption studies have indicated that depression has relatively low rate of heritability at 37% [ 91 ]. In addition, environmental factors such as stressful events are also involved in the pathogenesis of depression. Furthermore, complex psychiatric disorders, especially depression, are considered to be polygenic effects that interact with environmental factors [ 13 ]. Therefore, reliable identification of single causative genes for depression has proved to be challenging. The first genome-wide association studies (GWAS) for depression was published in 2009, and included 1,738 patients and 1,802 controls [ 92 , 93 ]. Although many subsequent GWASs have determined susceptible genes in the past decade, the impact of individual genes is so small that few results can be replicated [ 94 , 95 ]. So far, it is widely accepted that specific single genetic mutations may play minor and marginal roles in complex polygenic depression. Another major recognition in GWASs over the past decade is that prevalent candidate genes are usually not associated with depression. Further, the inconsistent results may also be due to the heterogeneity and polygenic nature of genetic and non-genetic risk factors for depression as well as the heterogeneity of depression subtypes [ 95 , 96 ]. Therefore, to date, the quality of research has been improved in two aspects: (1) the sample size has been maximized by combining the data of different evaluation models; and (2) more homogenous subtypes of depression have been selected to reduce phenotypic heterogeneity [ 97 ]. Levinson et al . pointed out that more than 75,000 to 100,000 cases should be considered to detect multiple depression associations [ 95 ]. Subsequently, several recent GWASs with larger sample sizes have been conducted. For example, Okbay et al . identified two loci associated with depression and replicated them in separate depression samples [ 98 ]. Wray et al . also found 44 risk loci associated with depression based on 135,458 cases and 344,901 controls [ 99 ]. A recent GWAS of 807,553 individuals with depression reported that 102 independent variants were associated with depression; these were involved in synaptic structure and neural transmission, and were verified in a further 1,507,153 individuals [ 100 ]. However, even with enough samples, GWASs still face severe challenges. A GWAS only marks the region of the genome and is not directly related to the potential biological function. In addition, a genetic association with the indicative phenotype of depression may only be part of many pathogenic pathways, or due to the indirect influence of intermediate traits in the causal pathway on the final result [ 101 ].
Given the diversity of findings, epigenetic factors are now being investigated. Recent studies indicated that epigenetic mechanisms may be the potential causes of "loss of heritability" in GWASs of depression. Over the past decade, a promising discovery has been that the effects of genetic information can be directly influenced by environment factors, and several specific genes are activated by environmental aspects. This process is described as interactions between genes and the environment, which is identified by the epigenetic mechanism. Environmental stressors cause alterations in gene expression in the brain, which may cause abnormal neuronal plasticity in areas related to the pathogenesis of the disease. Epigenetic events alter the structure of chromatin, thereby regulating gene expression involved in neuronal plasticity, stress behavior, depressive behavior, and antidepressant responses, including DNA methylation, histone acetylation, and the role of non-coding RNA. These new mechanisms of trans-generational transmission of epigenetic markers are considered a supplement to orthodox genetic heredity, providing the possibility for the discovery of new treatments for depression [ 102 , 103 ]. Recent studies imply that life experiences, including stress and enrichment, may affect cellular and molecular signaling pathways in sperm and influence the behavioral and physiological phenotypes of offspring in gender-specific patterns, which may also play an important role in the development of depression [ 103 ].
Brain Imaging and Neuroimaging Studies
Neuroimaging, including magnetic resonance imaging (MRI) and molecular imaging, provides a non-invasive technique for determining the underlying etiology and individualized treatment for depression. MRI can provide important data on brain structure, function, networks, and metabolism in patients with depression; it includes structural MRI (sMRI), functional MRI (fMRI), diffusion tensor imaging, and magnetic resonance spectroscopy.
Previous sMRI studies have found damaged gray matter in depression-associated brain areas, including the frontal lobe, anterior cingulate gyrus, hippocampus, putamen, thalamus, and amygdala. sMRI focuses on the thickness of gray matter and brain morphology [ 104 , 105 ]. A recent meta-analysis of 2,702 elderly patients with depression and 11,165 controls demonstrated that the volumes of the whole brain and hippocampus of patients with depression were lower than those of the control group [ 106 ]. Some evidence also showed that the hippocampal volume in depressive patients was lower than that of controls, and increased after treatment with antidepressants [ 107 ] and electroconvulsive therapy (ECT) [ 108 ], suggesting that the hippocampal volume plays a critical role in the development, treatment response, and clinical prognosis of depression. A recent study also reported that ECT increased the volume of the right hippocampus, amygdala, and putamen in patients with treatment-resistant depression [ 109 ]. In addition, postmortem research supported the MRI study showing that dentate gyrus volume was decreased in drug-naive patients with depression compared to healthy controls, and was potentially reversed by treatment with antidepressants [ 110 ].
Diffusion tensor imaging detects the microstructure of the white matter, which has been reported impaired in patients with depression [ 111 ]. A recent meta-analysis that included first-episode and drug-naïve depressive patients showed that the decrease in fractional anisotropy was negatively associated with illness duration and clinical severity [ 112 ].
fMRI, including resting-state and task-based fMRI, can divide the brain into self-related regions, such as the anterior cingulate cortex, posterior cingulate cortex, medial prefrontal cortex, precuneus, and dorsomedial thalamus. Many previous studies have shown the disturbance of several brain areas and intrinsic neural networks in patients with depression which could be rescued by antidepressants [ 113 , 114 , 115 , 116 ]. Further, some evidence also showed an association between brain network dysfunction and the clinical correlates of patients with depression, including clinical symptoms [ 117 ] and the response to antidepressants [ 118 , 119 ], ECT [ 120 , 121 ], and repetitive transcranial magnetic stimulation [ 122 ].
It is worth noting that brain imaging provides new insights into the large-scale brain circuits that underlie the pathophysiology of depressive disorder. In such studies, large-scale circuits are often referred to as “networks”. There is evidence that a variety of circuits are involved in the mechanisms of depressive disorder, including disruption of the default mode, salience, affective, reward, attention, and cognitive control circuits [ 123 ]. Over the past decade, the study of intra-circuit and inter-circuit connectivity dysfunctions in depression has escalated, in part due to advances in precision imaging and analysis techniques [ 124 ]. Circuit dysfunction is a potential biomarker to guide psychopharmacological treatment. For example, Williams et al . found that hyper-activation of the amygdala is associated with a negative phenotype that can predict the response to antidepressants [ 125 ]. Hou et al . showed that the baseline characteristics of the reward circuit predict early antidepressant responses [ 126 ].
Molecular imaging studies, including single photon emission computed tomography and positron emission tomography, focus on metabolic aspects such as amino-acids, neurotransmitters, glucose, and lipids at the cellular level in patients with depression. A recent meta-analysis examined glucose metabolism and found that glucose uptake dysfunction in different brain regions predicts the treatment response [ 127 ].
The most important and promising studies were conducted by the ENIGMA (Enhancing NeuroImaging Genetics through Meta Analysis) Consortium, which investigated the human brain across 43 countries. The ENIGMA-MDD Working Group was launched in 2012 to detect the structural and functional changes associated with MDD reliably and replicate them in various samples around the world [ 128 ]. So far, the ENIGMA-MDD Working Group has collected data from 4,372 MDD patients and 9,788 healthy controls across 14 countries, including 45 cohorts [ 128 ]. Their findings to date are shown in Table 1 [ 128 , 129 , 130 , 131 , 132 , 133 , 134 , 135 , 136 , 137 ].
Objective Index for Diagnosis of MDD
To date, the clinical diagnosis of depression is subjectively based on interviews according to diagnostic criteria ( e.g. International Classification of Diseases and Diagnostic and Statistical Manual diagnostic systems) and the severity of clinical symptoms are assessed by questionnaires, although patients may experience considerable differences in symptoms and subtypes [ 138 ]. Meanwhile, biomarkers including genetics, epigenetics, peripheral gene and protein expression, and neuroimaging markers may provide a promising supplement for the development of the objective diagnosis of MDD, [ 139 , 140 , 141 ]. However, the development of reliable diagnosis for MDD using biomarkers is still difficult and elusive, and all methods based on a single marker are insufficiently specific and sensitive for clinical use [ 142 ]. Papakostas et al . showed that a multi-assay, serum-based test including nine peripheral biomarkers (soluble tumor necrosis factor alpha receptor type II, resistin, prolactin, myeloperoxidase, epidermal growth factor, BDNF, alpha1 antitrypsin, apolipoprotein CIII, brain-derived neurotrophic factor, and cortisol) yielded a specificity of 81.3% and a sensitivity of 91.7% [ 142 ]. However, the sample size was relatively small and no other studies have yet validated their results. Therefore, further studies are needed to identify biomarker models that integrate all biological variables and clinical features to improve the specificity and sensitivity of diagnosis for MDD.
Management of Depression
The treatment strategies for depression consist of pharmacological treatment and non-pharmacological treatments including psychotherapy, ECT [ 98 ], and transcranial magnetic stimulation. As psychotherapy has been shown to have effects on depression including attenuating depressive symptoms and improving the quality of life [ 143 , 144 ]; several practice guidelines are increasingly recommending psychotherapy as a monotherapy or in combination with antidepressants [ 145 , 146 ].
Current Antidepressant Treatment
Antidepressants approved by the US Food and Drug Administration (FDA) are shown in Table 2 . Due to the relatively limited understanding of the etiology and pathophysiology of depression, almost all the previous antidepressants were discovered by accident a few decades ago. Although most antidepressants are usually safe and effective, there are still some limitations, including delayed efficacy (usually 2 weeks) and side-effects that affect the treatment compliance [ 147 ]. In addition, <50% of all patients with depression show complete remission through optimized treatment, including trials of multiple drugs with and without simultaneous psychotherapy. In the past few decades, most antidepressant discoveries focused on finding faster, safer, and more selective serotonin or norepinephrine receptor targets. In addition, there is an urgent need to develop new approaches to obtain more effective, safer, and faster antidepressants. In 2019, the FDA approved two new antidepressants: Esketamine for refractory depression and Bresanolone for postpartum depression. Esmolamine, a derivative of the anesthetic drug ketamine, was approved by the FDA for the treatment of refractory depression, based on a large number of preliminary clinical studies [ 148 ]. For example, several randomized controlled trials and meta-analysis studies showed the efficacy and safety of Esketamine in depression or treatment-resistant depression [ 26 , 149 , 150 ]. Although both are groundbreaking new interventions for these debilitating diseases and both are approved for use only under medical supervision, there are still concerns about potential misuse and problems in the evaluation of mental disorders [ 151 ].
To date, although several potential drugs have not yet been approved by the FDA, they are key milestones in the development of antidepressants that may be modified and used clinically in the future, such as compounds containing dextromethorphan (a non-selective NMDAR antago–nist), sarcosine (N-methylglycine, a glycine reuptake inhibitor), AMPAR modulators, and mGluR modulators [ 152 ].
Neuromodulation Therapy
Neuromodulation therapy acts through magnetic pulse, micro-current, or neural feedback technology within the treatment dose, acting on the central or peripheral nervous system to regulate the excitatory/inhibitory activity to reduce or attenuate the symptoms of the disease.
ECT is one of most effective treatments for depression, with the implementation of safer equipment and advancement of techniques such as modified ECT [ 153 ]. Mounting evidence from randomized controlled trial (RCT) and meta-analysis studies has shown that rTMS can treat depressive patients with safety [ 154 ]. Other promising treatments for depression have emerged, such as transcranial direct current stimulation (tDCS) [ 155 ], transcranial alternating current stimulation (tACS)[ 156 ], vagal nerve stimulation [ 157 ], deep brain stimulation [ 158 ] , and light therapy [ 159 ], but some of them are still experimental to some extent and have not been widely used. For example, compared to tDCS, tACS displays less sensory experience and adverse reactions with weak electrical current in a sine-wave pattern, but the evidence for the efficacy of tACS in the treatment of depression is still limited [ 160 ]. Alexander et al . recently demonstrated that there was no difference in efficacy among different treatments (sham, 10-Hz and 40-Hz tACS). However, only the 10-Hz tACS group had more responders than the sham and 40-Hz tACS groups at week 2 [ 156 ]. Further RCT studies are needed to verify the efficacy of tACS. In addition, the mechanism of the effect of neuromodulation therapy on depression needs to be further investigated.
Precision Medicine for Depression
Optimizing the treatment strategy is an effective way to improve the therapeutic effect on depression. However, each individual with depression may react very differently to different treatments. Therefore, this raises the question of personalized treatment, that is, which patients are suitable for which treatment. Over the past decade, psychiatrists and psychologists have focused on individual biomarkers and clinical characteristics to predict the efficiency of antidepressants and psychotherapies, including genetics, peripheral protein expression, electrophysiology, neuroimaging, neurocognitive performance, developmental trauma, and personality [ 161 ]. For example, Bradley et al . recently conducted a 12-week RCT, which demonstrated that the response rate and remission rates of the pharmacogenetic guidance group were significantly higher than those of the non-pharmacogenetic guidance group [ 162 ].
Subsequently, Greden et al . conducted an 8-week RCT of Genomics Used to Improve Depression Decisions (GUIDED) on 1,167 MDD patients and demonstrated that although there was no difference in symptom improvement between the pharmacogenomics-guided and non- pharmacogenomics-guided groups, the response rate and remission rate of the pharmacogenomics-guided group increased significantly [ 163 ].
A recent meta-analysis has shown that the baseline default mode network connectivity in patients with depression can predict the clinical responses to treatments including cognitive behavioral therapy, pharmacotherapy, ECT, rTMS, and transcutaneous vagus nerve stimulation [ 164 ]. However, so far, the biomarkers that predict treatment response at the individual level have not been well applied in the clinic, and there is still a lot of work to be conducted in the future.
Future Perspectives
Although considerable progress has been made in the study of depression during a past decade, the heterogeneity of the disease, the effectiveness of treatment, and the gap in translational medicine are critical challenges. The main dilemma is that our understanding of the etiology and pathophysiology of depression is inadequate, so our understanding of depression is not deep enough to develop more effective treatment. Animal models still cannot fully simulate this heterogeneous and complex mental disorder. Therefore, how to effectively match the indicators measured in animals with those measured in genetic research or the development of new antidepressants is another important challenge.
Change history
17 may 2021.
A Correction to this paper has been published: https://doi.org/10.1007/s12264-021-00694-9
Dadi AF, Miller ER, Bisetegn TA, Mwanri L. Global burden of antenatal depression and its association with adverse birth outcomes: an umbrella review. BMC Public Health 2020, 20: 173
Article PubMed PubMed Central Google Scholar
Zhu S, Zhao L, Fan Y, Lv Q, Wu K, Lang X. Interaction between TNF-alpha and oxidative stress status in first-episode drug-naive schizophrenia. Psychoneuroendocrinology 2020, 114: 104595
Article CAS PubMed Google Scholar
Disease GBD, Injury I, Prevalence C. Global, regional, and national incidence, prevalence, and years lived with disability for 328 diseases and injuries for 195 countries, 1990–2016: a systematic analysis for the Global Burden of Disease Study 2016. Lancet 2017, 390: 1211–1259
Article Google Scholar
Andrade L, Caraveo-Anduaga JJ, Berglund P, Bijl RV, De Graaf R, Vollebergh W, et al. The epidemiology of major depressive episodes: results from the International Consortium of Psychiatric Epidemiology (ICPE) Surveys. Int J Methods Psychiatr Res 2003, 12: 3–21
Article PubMed Google Scholar
Kessler RC, Bromet EJ. The epidemiology of depression across cultures. Annu Rev Public Health 2013, 34: 119–138
Lim GY, Tam WW, Lu Y, Ho CS, Zhang MW, Ho RC. Prevalence of depression in the community from 30 countries between 1994 and 2014. Sci Rep 2018, 8: 2861
Liu J, Yan F, Ma X, Guo HL, Tang YL, Rakofsky JJ, et al. Prevalence of major depressive disorder and socio-demographic correlates: Results of a representative household epidemiological survey in Beijing, China. J Affect Disord 2015, 179: 74–81
Zhang YS, Rao WW, Cui LJ, Li JF, Li L, Ng CH, et al. Prevalence of major depressive disorder and its socio-demographic correlates in the general adult population in Hebei province, China. J Affect Disord 2019, 252: 92–98
Ma X, Xiang YT, Cai ZJ, Li SR, Xiang YQ, Guo HL, et al. Prevalence and socio-demographic correlates of major depressive episode in rural and urban areas of Beijing, China. J Affect Disord 2009, 115: 323–330
Huang Y, Wang Y, Wang H, Liu Z, Yu X, Yan J, et al. Prevalence of mental disorders in China: a cross-sectional epidemiological study. Lancet Psychiatry 2019, 6: 211–224
Avenevoli S, Swendsen J, He JP, Burstein M, Merikangas KR. Major depression in the national comorbidity survey-adolescent supplement: prevalence, correlates, and treatment. J Am Acad Child Adolesc Psychiatry 2015, 54(37–44): e32
Google Scholar
Wang F, Zhang QE, Zhang L, Ng CH, Ungvari GS, Yuan Z, et al. Prevalence of major depressive disorder in older adults in China: A systematic review and meta-analysis. J Affect Disord 2018, 241: 297–304
Krishnan V, Nestler EJ. The molecular neurobiology of depression. Nature 2008, 455: 894–902
Article CAS PubMed PubMed Central Google Scholar
Rush AJ, Trivedi MH, Wisniewski SR, Nierenberg AA, Stewart JW, Warden D, et al. Acute and longer-term outcomes in depressed outpatients requiring one or several treatment steps: a STAR*D report. Am J Psychiatry 2006, 163: 1905–1917
Li Z, Zhang Y, Wang Z, Chen J, Fan J, Guan Y, et al. The role of BDNF, NTRK2 gene and their interaction in development of treatment-resistant depression: data from multicenter, prospective, longitudinal clinic practice. J Psychiatr Res 2013, 47: 8–14
Murphy BE. Steroids and depression. J Steroid Biochem Mol Biol 1991, 38: 537–559
Pariante CM, Lightman SL. The HPA axis in major depression: classical theories and new developments. Trends Neurosci 2008, 31: 464–468
Keller J, Gomez R, Williams G, Lembke A, Lazzeroni L, Murphy GM Jr, et al. HPA axis in major depression: cortisol, clinical symptomatology and genetic variation predict cognition. Mol Psychiatry 2017, 22: 527–536
Gomez RG, Fleming SH, Keller J, Flores B, Kenna H, DeBattista C, et al. The neuropsychological profile of psychotic major depression and its relation to cortisol. Biol Psychiatry 2006, 60: 472–478
Lamers F, Vogelzangs N, Merikangas KR, de Jonge P, Beekman AT, Penninx BW. Evidence for a differential role of HPA-axis function, inflammation and metabolic syndrome in melancholic versus atypical depression. Mol Psychiatry 2013, 18: 692–699
Nandam LS, Brazel M, Zhou M, Jhaveri DJ. Cortisol and major depressive disorder-translating findings from humans to animal models and back. Front Psychiatry 2019, 10: 974
Owashi T, Otsubo T, Oshima A, Nakagome K, Higuchi T, Kamijima K. Longitudinal neuroendocrine changes assessed by dexamethasone/CRH and growth hormone releasing hormone tests in psychotic depression. Psychoneuroendocrinology 2008, 33: 152–161
Mickey BJ, Ginsburg Y, Sitzmann AF, Grayhack C, Sen S, Kirschbaum C, et al. Cortisol trajectory, melancholia, and response to electroconvulsive therapy. J Psychiatr Res 2018, 103: 46–53
Stetler C, Miller GE. Depression and hypothalamic-pituitary-adrenal activation: a quantitative summary of four decades of research. Psychosom Med 2011, 73: 114–126
Aubry JM. CRF system and mood disorders. J Chem Neuroanat 2013, 54: 20–24
Correia-Melo FS, Leal GC, Vieira F, Jesus-Nunes AP, Mello RP, Magnavita G, et al. Efficacy and safety of adjunctive therapy using esketamine or racemic ketamine for adult treatment-resistant depression: A randomized, double-blind, non-inferiority study. J Affect Disord 2020, 264: 527–534
Duman RS, Voleti B. Signaling pathways underlying the pathophysiology and treatment of depression: novel mechanisms for rapid-acting agents. Trends Neurosci 2012, 35: 47–56
Sanacora G, Zarate CA, Krystal JH, Manji HK. Targeting the glutamatergic system to develop novel, improved therapeutics for mood disorders. Nat Rev Drug Discov 2008, 7: 426–437
Hashimoto K. The role of glutamate on the action of antidepressants. Prog Neuropsychopharmacol Biol Psychiatry 2011, 35: 1558–1568
Gray AL, Hyde TM, Deep-Soboslay A, Kleinman JE, Sodhi MS. Sex differences in glutamate receptor gene expression in major depression and suicide. Mol Psychiatry 2015, 20: 1057–1068
Chandley MJ, Szebeni A, Szebeni K, Crawford JD, Stockmeier CA, Turecki G, et al. Elevated gene expression of glutamate receptors in noradrenergic neurons from the locus coeruleus in major depression. Int J Neuropsychopharmacol 2014, 17: 1569–1578
Musazzi L, Treccani G, Mallei A, Popoli M. The action of antidepressants on the glutamate system: regulation of glutamate release and glutamate receptors. Biol Psychiatry 2013, 73: 1180–1188
Kadriu B, Musazzi L, Henter ID, Graves M, Popoli M, Zarate CA Jr. Glutamatergic Neurotransmission: Pathway to Developing Novel Rapid-Acting Antidepressant Treatments. Int J Neuropsychopharmacol 2019, 22: 119–135
Beurel E, Grieco SF, Amadei C, Downey K, Jope RS. Ketamine-induced inhibition of glycogen synthase kinase-3 contributes to the augmentation of alpha-amino-3-hydroxy-5-methylisoxazole-4-propionic acid (AMPA) receptor signaling. Bipolar Disord 2016, 18: 473–480
Gould TD, O’Donnell KC, Dow ER, Du J, Chen G, Manji HK. Involvement of AMPA receptors in the antidepressant-like effects of lithium in the mouse tail suspension test and forced swim test. Neuropharmacology 2008, 54: 577–587
Duman RS, Sanacora G, Krystal JH. Altered Connectivity in Depression: GABA and Glutamate Neurotransmitter Deficits and Reversal by Novel Treatments. Neuron 2019, 102: 75–90
Ghosal S, Hare B, Duman RS. Prefrontal Cortex GABAergic Deficits and Circuit Dysfunction in the Pathophysiology and Treatment of Chronic Stress and Depression. Curr Opin Behav Sci 2017, 14: 1–8
Fee C, Banasr M, Sibille E. Somatostatin-positive gamma-aminobutyric acid interneuron deficits in depression: Cortical microcircuit and therapeutic perspectives. Biol Psychiatry 2017, 82: 549–559
Schur RR, Draisma LW, Wijnen JP, Boks MP, Koevoets MG, Joels M, et al. Brain GABA levels across psychiatric disorders: A systematic literature review and meta-analysis of (1) H-MRS studies. Hum Brain Mapp 2016, 37: 3337–3352
Guilloux JP, Douillard-Guilloux G, Kota R, Wang X, Gardier AM, Martinowich K, et al. Molecular evidence for BDNF- and GABA-related dysfunctions in the amygdala of female subjects with major depression. Mol Psychiatry 2012, 17: 1130–1142
Karolewicz B, Maciag D, O’Dwyer G, Stockmeier CA, Feyissa AM, Rajkowska G. Reduced level of glutamic acid decarboxylase-67 kDa in the prefrontal cortex in major depression. Int J Neuropsychopharmacol 2010, 13: 411–420
Lener MS, Niciu MJ, Ballard ED, Park M, Park LT, Nugent AC, et al. Glutamate and gamma-aminobutyric acid systems in the pathophysiology of major depression and antidepressant response to ketamine. Biol Psychiatry 2017, 81: 886–897
Chen X, van Gerven J, Cohen A, Jacobs G. Human pharmacology of positive GABA-A subtype-selective receptor modulators for the treatment of anxiety. Acta Pharmacol Sin 2019, 40: 571–582
Ren Z, Pribiag H, Jefferson SJ, Shorey M, Fuchs T, Stellwagen D, et al. Bidirectional homeostatic regulation of a depression-related brain state by gamma-aminobutyric acidergic deficits and ketamine treatment. Biol Psychiatry 2016, 80: 457–468
Kolata SM, Nakao K, Jeevakumar V, Farmer-Alroth EL, Fujita Y, Bartley AF, et al. Neuropsychiatric phenotypes produced by GABA reduction in mouse cortex and hippocampus. Neuropsychopharmacology 2018, 43: 1445–1456
Duman RS, Li N. A neurotrophic hypothesis of depression: role of synaptogenesis in the actions of NMDA receptor antagonists. Philos Trans R Soc Lond B Biol Sci 2012, 367: 2475–2484
Albert PR, Benkelfat C, Descarries L. The neurobiology of depression—revisiting the serotonin hypothesis. I. Cellular and molecular mechanisms. Philos Trans R Soc Lond B Biol Sci 2012, 367: 2378–2381
Li K, Shen S, Ji YT, Li XY, Zhang LS, Wang XD. Melatonin augments the effects of fluoxetine on depression-like behavior and hippocampal BDNF-TrkB signaling. Neurosci Bull 2018, 34: 303–311
Zhang JJ, Gao TT, Wang Y, Wang JL, Guan W, Wang YJ, et al. Andrographolide exerts significant antidepressant-like effects involving the hippocampal BDNF system in mice. Int J Neuropsychopharmacol 2019, 22: 585–600
Wang JQ, Mao L. The ERK pathway: Molecular mechanisms and treatment of depression. Mol Neurobiol 2019, 56: 6197–6205
Chiou YJ, Huang TL. Serum brain-derived neurotrophic factors in taiwanese patients with drug-naive first-episode major depressive disorder: Effects of antidepressants. Int J Neuropsychopharmacol 2017, 20: 213–218
CAS PubMed Google Scholar
Youssef MM, Underwood MD, Huang YY, Hsiung SC, Liu Y, Simpson NR, et al. Association of BDNF Val66Met polymorphism and brain BDNF levels with major depression and suicide. Int J Neuropsychopharmacol 2018, 21: 528–538
Kojima M, Matsui K, Mizui T. BDNF pro-peptide: physiological mechanisms and implications for depression. Cell Tissue Res 2019, 377: 73–79
Jeon SW, Kim YK. Inflammation-induced depression: Its pathophysiology and therapeutic implications. J Neuroimmunol 2017, 313: 92–98
Miller AH, Raison CL. The role of inflammation in depression: from evolutionary imperative to modern treatment target. Nat Rev Immunol 2016, 16: 22–34
Zhao G, Liu X. Neuroimmune advance in depressive disorder. Adv Exp Med Biol 2019, 1180: 85–98
Li Z, Wang Z, Zhang C, Chen J, Su Y, Huang J, et al. Reduced ENA78 levels as novel biomarker for major depressive disorder and venlafaxine efficiency: Result from a prospective longitudinal study. Psychoneuroendocrinology 2017, 81: 113–121
Ambrosio G, Kaufmann FN, Manosso L, Platt N, Ghisleni G, Rodrigues ALS, et al. Depression and peripheral inflammatory profile of patients with obesity. Psychoneuroendocrinology 2018, 91: 132–141
Mao R, Zhang C, Chen J, Zhao G, Zhou R, Wang F, et al. Different levels of pro- and anti-inflammatory cytokines in patients with unipolar and bipolar depression. J Affect Disord 2018, 237: 65–72
Enache D, Pariante CM, Mondelli V. Markers of central inflammation in major depressive disorder: A systematic review and meta-analysis of studies examining cerebrospinal fluid, positron emission tomography and post-mortem brain tissue. Brain Behav Immun 2019, 81: 24–40
Haroon E, Daguanno AW, Woolwine BJ, Goldsmith DR, Baer WM, Wommack EC, et al. Antidepressant treatment resistance is associated with increased inflammatory markers in patients with major depressive disorder. Psychoneuroendocrinology 2018, 95: 43–49
Syed SA, Beurel E, Loewenstein DA, Lowell JA, Craighead WE, Dunlop BW, et al. Defective inflammatory pathways in never-treated depressed patients are associated with poor treatment response. Neuron 2018, 99(914–924): e913
Li Z, Qi D, Chen J, Zhang C, Yi Z, Yuan C, et al. Venlafaxine inhibits the upregulation of plasma tumor necrosis factor-alpha (TNF-alpha) in the Chinese patients with major depressive disorder: a prospective longitudinal study. Psychoneuroendocrinology 2013, 38: 107–114
Kohler CA, Freitas TH, Stubbs B, Maes M, Solmi M, Veronese N, et al. Peripheral alterations in cytokine and chemokine levels after antidepressant drug treatment for major depressive disorder: systematic review and meta-analysis. Mol Neurobiol 2018, 55: 4195–4206
Innes S, Pariante CM, Borsini A. Microglial-driven changes in synaptic plasticity: A possible role in major depressive disorder. Psychoneuroendocrinology 2019, 102: 236–247
Sochocka M, Diniz BS, Leszek J. Inflammatory response in the CNS: Friend or foe? Mol Neurobiol 2017, 54: 8071–8089
Zhang L, Zhang J, You Z. Switching of the microglial activation phenotype is a possible treatment for depression disorder. Front Cell Neurosci 2018, 12: 306
Sandhu KV, Sherwin E, Schellekens H, Stanton C, Dinan TG, Cryan JF. Feeding the microbiota-gut-brain axis: diet, microbiome, and neuropsychiatry. Transl Res 2017, 179: 223–244
Gonzalez-Arancibia C, Urrutia-Pinones J, Illanes-Gonzalez J, Martinez-Pinto J, Sotomayor-Zarate R, Julio-Pieper M, et al. Do your gut microbes affect your brain dopamine? Psychopharmacology (Berl) 2019, 236: 1611–1622
Article CAS Google Scholar
Kennedy PJ, Cryan JF, Dinan TG, Clarke G. Irritable bowel syndrome: a microbiome-gut-brain axis disorder? World J Gastroenterol 2014, 20: 14105–14125
Whitehead WE, Palsson O, Jones KR. Systematic review of the comorbidity of irritable bowel syndrome with other disorders: what are the causes and implications? Gastroenterology 2002, 122: 1140–1156
Ruepert L, Quartero AO, de Wit NJ, van der Heijden GJ, Rubin G, Muris JW. Bulking agents, antispasmodics and antidepressants for the treatment of irritable bowel syndrome. Cochrane Database Syst Rev 2011: CD003460.
Marin IA, Goertz JE, Ren T, Rich SS, Onengut-Gumuscu S, Farber E, et al. Microbiota alteration is associated with the development of stress-induced despair behavior. Sci Rep 2017, 7: 43859
Zheng P, Zeng B, Zhou C, Liu M, Fang Z, Xu X, et al. Gut microbiome remodeling induces depressive-like behaviors through a pathway mediated by the host’s metabolism. Mol Psychiatry 2016, 21: 786–796
Curtis K, Stewart CJ, Robinson M, Molfese DL, Gosnell SN, Kosten TR, et al. Insular resting state functional connectivity is associated with gut microbiota diversity. Eur J Neurosci 2019, 50: 2446–2452
O’Mahony SM, Marchesi JR, Scully P, Codling C, Ceolho AM, Quigley EM, et al. Early life stress alters behavior, immunity, and microbiota in rats: implications for irritable bowel syndrome and psychiatric illnesses. Biol Psychiatry 2009, 65: 263–267
Garcia-Rodenas CL, Bergonzelli GE, Nutten S, Schumann A, Cherbut C, Turini M, et al. Nutritional approach to restore impaired intestinal barrier function and growth after neonatal stress in rats. J Pediatr Gastroenterol Nutr 2006, 43: 16–24
Hao Z, Wang W, Guo R, Liu H. Faecalibacterium prausnitzii (ATCC 27766) has preventive and therapeutic effects on chronic unpredictable mild stress-induced depression-like and anxiety-like behavior in rats. Psychoneuroendocrinology 2019, 104: 132–142
Messaoudi M, Violle N, Bisson JF, Desor D, Javelot H, Rougeot C. Beneficial psychological effects of a probiotic formulation (Lactobacillus helveticus R0052 and Bifidobacterium longum R0175) in healthy human volunteers. Gut Microbes 2011, 2: 256–261
Rudzki L, Ostrowska L, Pawlak D, Malus A, Pawlak K, Waszkiewicz N, et al. Probiotic Lactobacillus Plantarum 299v decreases kynurenine concentration and improves cognitive functions in patients with major depression: A double-blind, randomized, placebo controlled study. Psychoneuroendocrinology 2019, 100: 213–222
Foster JA, McVey Neufeld KA. Gut-brain axis: how the microbiome influences anxiety and depression. Trends Neurosci 2013, 36: 305–312
Mittal R, Debs LH, Patel AP, Nguyen D, Patel K, O’Connor G, et al. Neurotransmitters: The critical modulators regulating gut-brain axis. J Cell Physiol 2017, 232: 2359–2372
Diviccaro S, Giatti S, Borgo F, Barcella M, Borghi E, Trejo JL, et al. Treatment of male rats with finasteride, an inhibitor of 5alpha-reductase enzyme, induces long-lasting effects on depressive-like behavior, hippocampal neurogenesis, neuroinflammation and gut microbiota composition. Psychoneuroendocrinology 2019, 99: 206–215
Kiecolt-Glaser JK, Wilson SJ, Bailey ML, Andridge R, Peng J, Jaremka LM, et al. Marital distress, depression, and a leaky gut: Translocation of bacterial endotoxin as a pathway to inflammation. Psychoneuroendocrinology 2018, 98: 52–60
Lindqvist D, Dhabhar FS, James SJ, Hough CM, Jain FA, Bersani FS, et al. Oxidative stress, inflammation and treatment response in major depression. Psychoneuroendocrinology 2017, 76: 197–205
Czarny P, Wigner P, Galecki P, Sliwinski T. The interplay between inflammation, oxidative stress, DNA damage, DNA repair and mitochondrial dysfunction in depression. Prog Neuropsychopharmacol Biol Psychiatry 2018, 80: 309–321
Xie X, Yu C, Zhou J, Xiao Q, Shen Q, Xiong Z, et al. Nicotinamide mononucleotide ameliorates the depression-like behaviors and is associated with attenuating the disruption of mitochondrial bioenergetics in depressed mice. J Affect Disord 2020, 263: 166–174
Wang XL, Yuan K, Zhang W, Li SX, Gao GF, Lu L. Regulation of circadian genes by the MAPK pathway: Implications for rapid antidepressant action. Neurosci Bull 2020, 36: 66–76
Xie X, Shen Q, Yu C, Xiao Q, Zhou J, Xiong Z, et al. Depression-like behaviors are accompanied by disrupted mitochondrial energy metabolism in chronic corticosterone-induced mice. J Steroid Biochem Mol Biol 2020, 200: 105607
Zhang LF, Shi L, Liu H, Meng FT, Liu YJ, Wu HM, et al. Increased hippocampal tau phosphorylation and axonal mitochondrial transport in a mouse model of chronic stress. Int J Neuropsychopharmacol 2012, 15: 337–348
Flint J, Kendler KS. The genetics of major depression. Neuron 2014, 81: 1214
Sullivan PF, de Geus EJ, Willemsen G, James MR, Smit JH, Zandbelt T, et al. Genome-wide association for major depressive disorder: a possible role for the presynaptic protein piccolo. Mol Psychiatry 2009, 14: 359–375
Dunn EC, Brown RC, Dai Y, Rosand J, Nugent NR, Amstadter AB, et al. Genetic determinants of depression: recent findings and future directions. Harv Rev Psychiatry 2015, 23: 1–18
Major Depressive Disorder Working Group of the Psychiatric GC, Ripke S, Wray NR, Lewis CM, Hamilton SP, Weissman MM, et al. A mega-analysis of genome-wide association studies for major depressive disorder. Mol Psychiatry 2013, 18: 497–511.
Levinson DF, Mostafavi S, Milaneschi Y, Rivera M, Ripke S, Wray NR, et al. Genetic studies of major depressive disorder: why are there no genome-wide association study findings and what can we do about it? Biol Psychiatry 2014, 76: 510–512
Sullivan PF, Agrawal A, Bulik CM, Andreassen OA, Borglum AD, Breen G, et al. Psychiatric genomics: An update and an agenda. Am J Psychiatry 2018, 175: 15–27
Schwabe I, Milaneschi Y, Gerring Z, Sullivan PF, Schulte E, Suppli NP, et al. Unraveling the genetic architecture of major depressive disorder: merits and pitfalls of the approaches used in genome-wide association studies. Psychol Med 2019, 49: 2646–2656
Okbay A, Baselmans BM, De Neve JE, Turley P, Nivard MG, Fontana MA, et al. Genetic variants associated with subjective well-being, depressive symptoms, and neuroticism identified through genome-wide analyses. Nat Genet 2016, 48: 624–633
Wray NR, Ripke S, Mattheisen M, Trzaskowski M, Byrne EM, Abdellaoui A, et al. Genome-wide association analyses identify 44 risk variants and refine the genetic architecture of major depression. Nat Genet 2018, 50: 668–681
Howard DM, Adams MJ, Clarke TK, Hafferty JD, Gibson J, Shirali M, et al. Genome-wide meta-analysis of depression identifies 102 independent variants and highlights the importance of the prefrontal brain regions. Nat Neurosci 2019, 22: 343–352
Ormel J, Hartman CA, Snieder H. The genetics of depression: successful genome-wide association studies introduce new challenges. Transl Psychiatry 2019, 9: 114
Uchida S, Yamagata H, Seki T, Watanabe Y. Epigenetic mechanisms of major depression: Targeting neuronal plasticity. Psychiatry Clin Neurosci 2018, 72: 212–227
Yeshurun S, Hannan AJ. Transgenerational epigenetic influences of paternal environmental exposures on brain function and predisposition to psychiatric disorders. Mol Psychiatry 2019, 24: 536–548
van Eijndhoven P, van Wingen G, Katzenbauer M, Groen W, Tepest R, Fernandez G, et al. Paralimbic cortical thickness in first-episode depression: evidence for trait-related differences in mood regulation. Am J Psychiatry 2013, 170: 1477–1486
Peng W, Chen Z, Yin L, Jia Z, Gong Q. Essential brain structural alterations in major depressive disorder: A voxel-wise meta-analysis on first episode, medication-naive patients. J Affect Disord 2016, 199: 114–123
Geerlings MI, Gerritsen L. Late-life depression, hippocampal volumes, and hypothalamic-pituitary-adrenal axis regulation: A systematic review and meta-analysis. Biol Psychiatry 2017, 82: 339–350
Maller JJ, Broadhouse K, Rush AJ, Gordon E, Koslow S, Grieve SM. Increased hippocampal tail volume predicts depression status and remission to anti-depressant medications in major depression. Mol Psychiatry 2018, 23: 1737–1744
Joshi SH, Espinoza RT, Pirnia T, Shi J, Wang Y, Ayers B, et al. Structural plasticity of the hippocampus and amygdala induced by electroconvulsive therapy in major depression. Biol Psychiatry 2016, 79: 282–292
Gryglewski G, Baldinger-Melich P, Seiger R, Godbersen GM, Michenthaler P, Klobl M, et al. Structural changes in amygdala nuclei, hippocampal subfields and cortical thickness following electroconvulsive therapy in treatment-resistant depression: longitudinal analysis. Br J Psychiatry 2019, 214: 159–167
Boldrini M, Santiago AN, Hen R, Dwork AJ, Rosoklija GB, Tamir H, et al. Hippocampal granule neuron number and dentate gyrus volume in antidepressant-treated and untreated major depression. Neuropsychopharmacology 2013, 38: 1068–1077
Liang S, Wang Q, Kong X, Deng W, Yang X, Li X, et al. White matter abnormalities in major depression biotypes identified by diffusion tensor imaging. Neurosci Bull 2019, 35: 867–876
Chen G, Guo Y, Zhu H, Kuang W, Bi F, Ai H, et al. Intrinsic disruption of white matter microarchitecture in first-episode, drug-naive major depressive disorder: A voxel-based meta-analysis of diffusion tensor imaging. Prog Neuropsychopharmacol Biol Psychiatry 2017, 76: 179–187
Brakowski J, Spinelli S, Dorig N, Bosch OG, Manoliu A, Holtforth MG, et al. Resting state brain network function in major depression - Depression symptomatology, antidepressant treatment effects, future research. J Psychiatr Res 2017, 92: 147–159
Dutta A, McKie S, Downey D, Thomas E, Juhasz G, Arnone D, et al. Regional default mode network connectivity in major depressive disorder: modulation by acute intravenous citalopram. Transl Psychiatry 2019, 9: 116
Meyer BM, Rabl U, Huemer J, Bartova L, Kalcher K, Provenzano J, et al. Prefrontal networks dynamically related to recovery from major depressive disorder: a longitudinal pharmacological fMRI study. Transl Psychiatry 2019, 9: 64
Muller VI, Cieslik EC, Serbanescu I, Laird AR, Fox PT, Eickhoff SB. Altered brain activity in unipolar depression revisited: Meta-analyses of neuroimaging studies. JAMA Psychiatry 2017, 74: 47–55
Connolly CG, Ho TC, Blom EH, LeWinn KZ, Sacchet MD, Tymofiyeva O, et al. Resting-state functional connectivity of the amygdala and longitudinal changes in depression severity in adolescent depression. J Affect Disord 2017, 207: 86–94
Godlewska BR, Browning M, Norbury R, Igoumenou A, Cowen PJ, Harmer CJ. Predicting treatment response in depression: The role of anterior cingulate cortex. Int J Neuropsychopharmacol 2018, 21: 988–996
Goldstein-Piekarski AN, Staveland BR, Ball TM, Yesavage J, Korgaonkar MS, Williams LM. Intrinsic functional connectivity predicts remission on antidepressants: a randomized controlled trial to identify clinically applicable imaging biomarkers. Transl Psychiatry 2018, 8: 57
Leaver AM, Vasavada M, Joshi SH, Wade B, Woods RP, Espinoza R, et al. Mechanisms of antidepressant response to electroconvulsive therapy studied with perfusion magnetic resonance imaging. Biol Psychiatry 2019, 85: 466–476
Miskowiak KW, Macoveanu J, Jorgensen MB, Stottrup MM, Ott CV, Jensen HM, et al. Neural response after a single ECT session during retrieval of emotional self-referent words in depression: A randomized, sham-controlled fMRI study. Int J Neuropsychopharmacol 2018, 21: 226–235
Du L, Liu H, Du W, Chao F, Zhang L, Wang K, et al. Stimulated left DLPFC-nucleus accumbens functional connectivity predicts the anti-depression and anti-anxiety effects of rTMS for depression. Transl Psychiatry 2018, 7: 3
Williams LM. Defining biotypes for depression and anxiety based on large-scale circuit dysfunction: a theoretical review of the evidence and future directions for clinical translation. Depress Anxiety 2017, 34: 9–24
Williams LM. Precision psychiatry: a neural circuit taxonomy for depression and anxiety. Lancet Psychiatry 2016, 3: 472–480
Williams LM, Korgaonkar MS, Song YC, Paton R, Eagles S, Goldstein-Piekarski A, et al. Amygdala reactivity to emotional faces in the prediction of general and medication-specific responses to antidepressant treatment in the randomized iSPOT-D trial. Neuropsychopharmacology 2015, 40: 2398–2408
Hou Z, Gong L, Zhi M, Yin Y, Zhang Y, Xie C, et al. Distinctive pretreatment features of bilateral nucleus accumbens networks predict early response to antidepressants in major depressive disorder. Brain Imaging Behav 2018, 12: 1042–1052
De Crescenzo F, Ciliberto M, Menghini D, Treglia G, Ebmeier KP, Janiri L. Is (18)F-FDG-PET suitable to predict clinical response to the treatment of geriatric depression? A systematic review of PET studies. Aging Ment Health 2017, 21: 889–894
Schmaal L, Pozzi E, T CH, van Velzen LS, Veer IM, Opel N, et al. ENIGMA MDD: seven years of global neuroimaging studies of major depression through worldwide data sharing. Transl Psychiatry 2020, 10: 172.
Schmaal L, Veltman DJ, van Erp TG, Samann PG, Frodl T, Jahanshad N, et al. Subcortical brain alterations in major depressive disorder: findings from the ENIGMA Major Depressive Disorder working group. Mol Psychiatry 2016, 21: 806–812
Schmaal L, Hibar DP, Samann PG, Hall GB, Baune BT, Jahanshad N, et al. Cortical abnormalities in adults and adolescents with major depression based on brain scans from 20 cohorts worldwide in the ENIGMA Major Depressive Disorder Working Group. Mol Psychiatry 2017, 22: 900–909
Frodl T, Janowitz D, Schmaal L, Tozzi L, Dobrowolny H, Stein DJ, et al. Childhood adversity impacts on brain subcortical structures relevant to depression. J Psychiatr Res 2017, 86: 58–65
Renteria ME, Schmaal L, Hibar DP, Couvy-Duchesne B, Strike LT, Mills NT, et al. Subcortical brain structure and suicidal behaviour in major depressive disorder: a meta-analysis from the ENIGMA-MDD working group. Transl Psychiatry 2017, 7: e1116
Tozzi L, Garczarek L, Janowitz D, Stein DJ, Wittfeld K, Dobrowolny H, et al. Interactive impact of childhood maltreatment, depression, and age on cortical brain structure: mega-analytic findings from a large multi-site cohort. Psychol Med 2020, 50: 1020–1031
de Kovel CGF, Aftanas L, Aleman A, Alexander-Bloch AF, Baune BT, Brack I, et al. No alterations of brain structural asymmetry in major depressive disorder: An ENIGMA consortium analysis. Am J Psychiatry 2019, 176: 1039–1049
Ho TC, Gutman B, Pozzi E, Grabe HJ, Hosten N, Wittfeld K, et al. Subcortical shape alterations in major depressive disorder: Findings from the ENIGMA major depressive disorder working group. Hum Brain Mapp 2020.
Han LKM, Dinga R, Hahn T, Ching CRK, Eyler LT, Aftanas L, et al. Brain aging in major depressive disorder: results from the ENIGMA major depressive disorder working group. Mol Psychiatry 2020.
van Velzen LS, Kelly S, Isaev D, Aleman A, Aftanas LI, Bauer J, et al. White matter disturbances in major depressive disorder: a coordinated analysis across 20 international cohorts in the ENIGMA MDD working group. Mol Psychiatry 2020, 25: 1511–1525
Lv X, Si T, Wang G, Wang H, Liu Q, Hu C, et al. The establishment of the objective diagnostic markers and personalized medical intervention in patients with major depressive disorder: rationale and protocol. BMC Psychiatry 2016, 16: 240
Fabbri C, Hosak L, Mossner R, Giegling I, Mandelli L, Bellivier F, et al. Consensus paper of the WFSBP Task Force on Genetics: Genetics, epigenetics and gene expression markers of major depressive disorder and antidepressant response. World J Biol Psychiatry 2017, 18: 5–28
Li Z, Zhang C, Fan J, Yuan C, Huang J, Chen J, et al. Brain-derived neurotrophic factor levels and bipolar disorder in patients in their first depressive episode: 3-year prospective longitudinal study. Br J Psychiatry 2014, 205: 29–35
Gong Q, He Y. Depression, neuroimaging and connectomics: a selective overview. Biol Psychiatry 2015, 77: 223–235
Papakostas GI, Shelton RC, Kinrys G, Henry ME, Bakow BR, Lipkin SH, et al. Assessment of a multi-assay, serum-based biological diagnostic test for major depressive disorder: a pilot and replication study. Mol Psychiatry 2013, 18: 332–339
Kolovos S, Kleiboer A, Cuijpers P. Effect of psychotherapy for depression on quality of life: meta-analysis. Br J Psychiatry 2016, 209: 460–468
Park LT, Zarate CA Jr. Depression in the primary care setting. N Engl J Med 2019, 380: 559–568
Health N C C F M . Depression: The treatment and management of depression in adults (updated edition) 2010.
Qaseem A, Barry MJ, Kansagara D. Clinical Guidelines Committee of the American College of P. Nonpharmacologic versus pharmacologic treatment of adult patients with major depressive disorder: A clinical practice guideline from the american college of physicians. Ann Intern Med 2016, 164: 350–359
Dodd S, Mitchell PB, Bauer M, Yatham L, Young AH, Kennedy SH, et al. Monitoring for antidepressant-associated adverse events in the treatment of patients with major depressive disorder: An international consensus statement. World J Biol Psychiatry 2018, 19: 330–348
Cristea IA, Naudet F. US Food and Drug Administration approval of esketamine and brexanolone. Lancet Psychiatry 2019, 6: 975–977
Zheng W, Cai DB, Xiang YQ, Zheng W, Jiang WL, Sim K, et al. Adjunctive intranasal esketamine for major depressive disorder: A systematic review of randomized double-blind controlled-placebo studies. J Affect Disord 2020, 265: 63–70
Popova V, Daly EJ, Trivedi M, Cooper K, Lane R, Lim P, et al. Efficacy and safety of flexibly dosed esketamine nasal spray combined with a newly initiated oral antidepressant in treatment-resistant depression: A Randomized Double-Blind Active-Controlled Study. Am J Psychiatry 2019, 176: 428–438
Turner EH. Esketamine for treatment-resistant depression: seven concerns about efficacy and FDA approval. Lancet Psychiatry 2019, 6: 977–979
Murrough JW, Abdallah CG, Mathew SJ. Targeting glutamate signalling in depression: progress and prospects. Nat Rev Drug Discov 2017, 16: 472–486
Gill SP, Kellner CH. Clinical practice recommendations for continuation and maintenance electroconvulsive therapy for depression: Outcomes from a review of the evidence and a consensus workshop held in Australia in May 2017. J ECT 2019, 35: 14–20
McClintock SM, Reti IM, Carpenter LL, McDonald WM, Dubin M, Taylor SF, et al. Consensus recommendations for the clinical application of repetitive transcranial magnetic stimulation (rTMS) in the treatment of depression. J Clin Psychiatry 2018, 79.
Chase HW, Boudewyn MA, Carter CS, Phillips ML. Transcranial direct current stimulation: a roadmap for research, from mechanism of action to clinical implementation. Mol Psychiatry 2020, 25: 397–407
Alexander ML, Alagapan S, Lugo CE, Mellin JM, Lustenberger C, Rubinow DR, et al. Double-blind, randomized pilot clinical trial targeting alpha oscillations with transcranial alternating current stimulation (tACS) for the treatment of major depressive disorder (MDD). Transl Psychiatry 2019, 9: 106
Akhtar H, Bukhari F, Nazir M, Anwar MN, Shahzad A. Therapeutic efficacy of neurostimulation for depression: Techniques, current modalities, and future challenges. Neurosci Bull 2016, 32: 115–126
Zhou C, Zhang H, Qin Y, Tian T, Xu B, Chen J, et al. A systematic review and meta-analysis of deep brain stimulation in treatment-resistant depression. Prog Neuropsychopharmacol Biol Psychiatry 2018, 82: 224–232
Li X, Li X. The antidepressant effect of light therapy from retinal projections. Neurosci Bull 2018, 34: 359–368
Shekelle PG, Cook IA, Miake-Lye IM, Booth MS, Beroes JM, Mak S. Benefits and harms of cranial electrical stimulation for chronic painful conditions, depression, anxiety, and insomnia: A systematic review. Ann Intern Med 2018, 168: 414–421
Kessler RC. The potential of predictive analytics to provide clinical decision support in depression treatment planning. Curr Opin Psychiatry 2018, 31: 32–39
Bradley P, Shiekh M, Mehra V, Vrbicky K, Layle S, Olson MC, et al. Improved efficacy with targeted pharmacogenetic-guided treatment of patients with depression and anxiety: A randomized clinical trial demonstrating clinical utility. J Psychiatr Res 2018, 96: 100–107
Greden JF, Parikh SV, Rothschild AJ, Thase ME, Dunlop BW, DeBattista C, et al. Impact of pharmacogenomics on clinical outcomes in major depressive disorder in the GUIDED trial: A large, patient- and rater-blinded, randomized, controlled study. J Psychiatr Res 2019, 111: 59–67
Long Z, Du L, Zhao J, Wu S, Zheng Q, Lei X. Prediction on treatment improvement in depression with resting state connectivity: A coordinate-based meta-analysis. J Affect Disord 2020, 276: 62–68
Download references
Acknowledgments
This review was supported by the National Basic Research Development Program of China (2016YFC1307100), the National Natural Science Foundation of China (81930033 and 81771465; 81401127), Shanghai Key Project of Science & Technology (2018SHZDZX05), Shanghai Jiao Tong University Medical Engineering Foundation (YG2016MS48), Shanghai Jiao Tong University School of Medicine (19XJ11006), the Sanming Project of Medicine in Shenzhen Municipality (SZSM201612006), the National Key Technologies R&D Program of China (2012BAI01B04), and the Innovative Research Team of High-level Local Universities in Shanghai.
Author information
Authors and affiliations.
Clinical Research Center and Division of Mood Disorders of Shanghai Mental Health Center, Shanghai Jiao Tong University School of Medicine, Shanghai, 200030, China
Zezhi Li, Jun Chen & Yiru Fang
Department of Neurology, Ren Ji Hospital, Shanghai Jiao Tong University School of Medicine, Shanghai, 200127, China
Shanghai Institute of Nutrition and Health, Shanghai Information Center for Life Sciences, Chinese Academy of Science, Shanghai, 200031, China
Meihua Ruan
Center for Excellence in Brain Science and Intelligence Technology, Chinese Academy of Science, Shanghai, 200031, China
Shanghai Key Laboratory of Psychotic Disorders, Shanghai, 201108, China
Jun Chen & Yiru Fang
You can also search for this author in PubMed Google Scholar
Corresponding author
Correspondence to Yiru Fang .
Ethics declarations
Conflicts of interest.
The authors declare no conflicts of interest.
Rights and permissions
Open Access This article is licensed under a Creative Commons Attribution 4.0 International License, which permits use, sharing, adaptation, distribution and reproduction in any medium or format, as long as you give appropriate credit to the original author(s) and the source, provide a link to the Creative Commons licence, and indicate if changes were made. The images or other third party material in this article are included in the article's Creative Commons licence, unless indicated otherwise in a credit line to the material. If material is not included in the article's Creative Commons licence and your intended use is not permitted by statutory regulation or exceeds the permitted use, you will need to obtain permission directly from the copyright holder. To view a copy of this licence, visit http://creativecommons.org/licenses/by/4.0/ .
Reprints and permissions
About this article
Li, Z., Ruan, M., Chen, J. et al. Major Depressive Disorder: Advances in Neuroscience Research and Translational Applications. Neurosci. Bull. 37 , 863–880 (2021). https://doi.org/10.1007/s12264-021-00638-3
Download citation
Received : 30 May 2020
Accepted : 30 September 2020
Published : 13 February 2021
Issue Date : June 2021
DOI : https://doi.org/10.1007/s12264-021-00638-3
Share this article
Anyone you share the following link with will be able to read this content:
Sorry, a shareable link is not currently available for this article.
Provided by the Springer Nature SharedIt content-sharing initiative
- Major depressive disorder
- Pathogenesis
- Find a journal
- Publish with us
- Track your research
- Search Menu
Sign in through your institution
- Browse content in Arts and Humanities
- Browse content in Archaeology
- Anglo-Saxon and Medieval Archaeology
- Archaeological Methodology and Techniques
- Archaeology by Region
- Archaeology of Religion
- Archaeology of Trade and Exchange
- Biblical Archaeology
- Contemporary and Public Archaeology
- Environmental Archaeology
- Historical Archaeology
- History and Theory of Archaeology
- Industrial Archaeology
- Landscape Archaeology
- Mortuary Archaeology
- Prehistoric Archaeology
- Underwater Archaeology
- Urban Archaeology
- Zooarchaeology
- Browse content in Architecture
- Architectural Structure and Design
- History of Architecture
- Residential and Domestic Buildings
- Theory of Architecture
- Browse content in Art
- Art Subjects and Themes
- History of Art
- Industrial and Commercial Art
- Theory of Art
- Biographical Studies
- Byzantine Studies
- Browse content in Classical Studies
- Classical History
- Classical Philosophy
- Classical Mythology
- Classical Literature
- Classical Reception
- Classical Art and Architecture
- Classical Oratory and Rhetoric
- Greek and Roman Epigraphy
- Greek and Roman Law
- Greek and Roman Papyrology
- Greek and Roman Archaeology
- Late Antiquity
- Religion in the Ancient World
- Digital Humanities
- Browse content in History
- Colonialism and Imperialism
- Diplomatic History
- Environmental History
- Genealogy, Heraldry, Names, and Honours
- Genocide and Ethnic Cleansing
- Historical Geography
- History by Period
- History of Emotions
- History of Agriculture
- History of Education
- History of Gender and Sexuality
- Industrial History
- Intellectual History
- International History
- Labour History
- Legal and Constitutional History
- Local and Family History
- Maritime History
- Military History
- National Liberation and Post-Colonialism
- Oral History
- Political History
- Public History
- Regional and National History
- Revolutions and Rebellions
- Slavery and Abolition of Slavery
- Social and Cultural History
- Theory, Methods, and Historiography
- Urban History
- World History
- Browse content in Language Teaching and Learning
- Language Learning (Specific Skills)
- Language Teaching Theory and Methods
- Browse content in Linguistics
- Applied Linguistics
- Cognitive Linguistics
- Computational Linguistics
- Forensic Linguistics
- Grammar, Syntax and Morphology
- Historical and Diachronic Linguistics
- History of English
- Language Acquisition
- Language Evolution
- Language Reference
- Language Variation
- Language Families
- Lexicography
- Linguistic Anthropology
- Linguistic Theories
- Linguistic Typology
- Phonetics and Phonology
- Psycholinguistics
- Sociolinguistics
- Translation and Interpretation
- Writing Systems
- Browse content in Literature
- Bibliography
- Children's Literature Studies
- Literary Studies (Asian)
- Literary Studies (European)
- Literary Studies (Eco-criticism)
- Literary Studies (Romanticism)
- Literary Studies (American)
- Literary Studies (Modernism)
- Literary Studies - World
- Literary Studies (1500 to 1800)
- Literary Studies (19th Century)
- Literary Studies (20th Century onwards)
- Literary Studies (African American Literature)
- Literary Studies (British and Irish)
- Literary Studies (Early and Medieval)
- Literary Studies (Fiction, Novelists, and Prose Writers)
- Literary Studies (Gender Studies)
- Literary Studies (Graphic Novels)
- Literary Studies (History of the Book)
- Literary Studies (Plays and Playwrights)
- Literary Studies (Poetry and Poets)
- Literary Studies (Postcolonial Literature)
- Literary Studies (Queer Studies)
- Literary Studies (Science Fiction)
- Literary Studies (Travel Literature)
- Literary Studies (War Literature)
- Literary Studies (Women's Writing)
- Literary Theory and Cultural Studies
- Mythology and Folklore
- Shakespeare Studies and Criticism
- Browse content in Media Studies
- Browse content in Music
- Applied Music
- Dance and Music
- Ethics in Music
- Ethnomusicology
- Gender and Sexuality in Music
- Medicine and Music
- Music Cultures
- Music and Religion
- Music and Media
- Music and Culture
- Music Education and Pedagogy
- Music Theory and Analysis
- Musical Scores, Lyrics, and Libretti
- Musical Structures, Styles, and Techniques
- Musicology and Music History
- Performance Practice and Studies
- Race and Ethnicity in Music
- Sound Studies
- Browse content in Performing Arts
- Browse content in Philosophy
- Aesthetics and Philosophy of Art
- Epistemology
- Feminist Philosophy
- History of Western Philosophy
- Metaphysics
- Moral Philosophy
- Non-Western Philosophy
- Philosophy of Science
- Philosophy of Language
- Philosophy of Mind
- Philosophy of Perception
- Philosophy of Action
- Philosophy of Law
- Philosophy of Religion
- Philosophy of Mathematics and Logic
- Practical Ethics
- Social and Political Philosophy
- Browse content in Religion
- Biblical Studies
- Christianity
- East Asian Religions
- History of Religion
- Judaism and Jewish Studies
- Qumran Studies
- Religion and Education
- Religion and Health
- Religion and Politics
- Religion and Science
- Religion and Law
- Religion and Art, Literature, and Music
- Religious Studies
- Browse content in Society and Culture
- Cookery, Food, and Drink
- Cultural Studies
- Customs and Traditions
- Ethical Issues and Debates
- Hobbies, Games, Arts and Crafts
- Natural world, Country Life, and Pets
- Popular Beliefs and Controversial Knowledge
- Sports and Outdoor Recreation
- Technology and Society
- Travel and Holiday
- Visual Culture
- Browse content in Law
- Arbitration
- Browse content in Company and Commercial Law
- Commercial Law
- Company Law
- Browse content in Comparative Law
- Systems of Law
- Competition Law
- Browse content in Constitutional and Administrative Law
- Government Powers
- Judicial Review
- Local Government Law
- Military and Defence Law
- Parliamentary and Legislative Practice
- Construction Law
- Contract Law
- Browse content in Criminal Law
- Criminal Procedure
- Criminal Evidence Law
- Sentencing and Punishment
- Employment and Labour Law
- Environment and Energy Law
- Browse content in Financial Law
- Banking Law
- Insolvency Law
- History of Law
- Human Rights and Immigration
- Intellectual Property Law
- Browse content in International Law
- Private International Law and Conflict of Laws
- Public International Law
- IT and Communications Law
- Jurisprudence and Philosophy of Law
- Law and Politics
- Law and Society
- Browse content in Legal System and Practice
- Courts and Procedure
- Legal Skills and Practice
- Primary Sources of Law
- Regulation of Legal Profession
- Medical and Healthcare Law
- Browse content in Policing
- Criminal Investigation and Detection
- Police and Security Services
- Police Procedure and Law
- Police Regional Planning
- Browse content in Property Law
- Personal Property Law
- Study and Revision
- Terrorism and National Security Law
- Browse content in Trusts Law
- Wills and Probate or Succession
- Browse content in Medicine and Health
- Browse content in Allied Health Professions
- Arts Therapies
- Clinical Science
- Dietetics and Nutrition
- Occupational Therapy
- Operating Department Practice
- Physiotherapy
- Radiography
- Speech and Language Therapy
- Browse content in Anaesthetics
- General Anaesthesia
- Neuroanaesthesia
- Browse content in Clinical Medicine
- Acute Medicine
- Cardiovascular Medicine
- Clinical Genetics
- Clinical Pharmacology and Therapeutics
- Dermatology
- Endocrinology and Diabetes
- Gastroenterology
- Genito-urinary Medicine
- Geriatric Medicine
- Infectious Diseases
- Medical Toxicology
- Medical Oncology
- Pain Medicine
- Palliative Medicine
- Rehabilitation Medicine
- Respiratory Medicine and Pulmonology
- Rheumatology
- Sleep Medicine
- Sports and Exercise Medicine
- Clinical Neuroscience
- Community Medical Services
- Critical Care
- Emergency Medicine
- Forensic Medicine
- Haematology
- History of Medicine
- Browse content in Medical Dentistry
- Oral and Maxillofacial Surgery
- Paediatric Dentistry
- Restorative Dentistry and Orthodontics
- Surgical Dentistry
- Browse content in Medical Skills
- Clinical Skills
- Communication Skills
- Nursing Skills
- Surgical Skills
- Medical Ethics
- Medical Statistics and Methodology
- Browse content in Neurology
- Clinical Neurophysiology
- Neuropathology
- Nursing Studies
- Browse content in Obstetrics and Gynaecology
- Gynaecology
- Occupational Medicine
- Ophthalmology
- Otolaryngology (ENT)
- Browse content in Paediatrics
- Neonatology
- Browse content in Pathology
- Chemical Pathology
- Clinical Cytogenetics and Molecular Genetics
- Histopathology
- Medical Microbiology and Virology
- Patient Education and Information
- Browse content in Pharmacology
- Psychopharmacology
- Browse content in Popular Health
- Caring for Others
- Complementary and Alternative Medicine
- Self-help and Personal Development
- Browse content in Preclinical Medicine
- Cell Biology
- Molecular Biology and Genetics
- Reproduction, Growth and Development
- Primary Care
- Professional Development in Medicine
- Browse content in Psychiatry
- Addiction Medicine
- Child and Adolescent Psychiatry
- Forensic Psychiatry
- Learning Disabilities
- Old Age Psychiatry
- Psychotherapy
- Browse content in Public Health and Epidemiology
- Epidemiology
- Public Health
- Browse content in Radiology
- Clinical Radiology
- Interventional Radiology
- Nuclear Medicine
- Radiation Oncology
- Reproductive Medicine
- Browse content in Surgery
- Cardiothoracic Surgery
- Gastro-intestinal and Colorectal Surgery
- General Surgery
- Neurosurgery
- Paediatric Surgery
- Peri-operative Care
- Plastic and Reconstructive Surgery
- Surgical Oncology
- Transplant Surgery
- Trauma and Orthopaedic Surgery
- Vascular Surgery
- Browse content in Science and Mathematics
- Browse content in Biological Sciences
- Aquatic Biology
- Biochemistry
- Bioinformatics and Computational Biology
- Developmental Biology
- Ecology and Conservation
- Evolutionary Biology
- Genetics and Genomics
- Microbiology
- Molecular and Cell Biology
- Natural History
- Plant Sciences and Forestry
- Research Methods in Life Sciences
- Structural Biology
- Systems Biology
- Zoology and Animal Sciences
- Browse content in Chemistry
- Analytical Chemistry
- Computational Chemistry
- Crystallography
- Environmental Chemistry
- Industrial Chemistry
- Inorganic Chemistry
- Materials Chemistry
- Medicinal Chemistry
- Mineralogy and Gems
- Organic Chemistry
- Physical Chemistry
- Polymer Chemistry
- Study and Communication Skills in Chemistry
- Theoretical Chemistry
- Browse content in Computer Science
- Artificial Intelligence
- Computer Architecture and Logic Design
- Game Studies
- Human-Computer Interaction
- Mathematical Theory of Computation
- Programming Languages
- Software Engineering
- Systems Analysis and Design
- Virtual Reality
- Browse content in Computing
- Business Applications
- Computer Security
- Computer Games
- Computer Networking and Communications
- Digital Lifestyle
- Graphical and Digital Media Applications
- Operating Systems
- Browse content in Earth Sciences and Geography
- Atmospheric Sciences
- Environmental Geography
- Geology and the Lithosphere
- Maps and Map-making
- Meteorology and Climatology
- Oceanography and Hydrology
- Palaeontology
- Physical Geography and Topography
- Regional Geography
- Soil Science
- Urban Geography
- Browse content in Engineering and Technology
- Agriculture and Farming
- Biological Engineering
- Civil Engineering, Surveying, and Building
- Electronics and Communications Engineering
- Energy Technology
- Engineering (General)
- Environmental Science, Engineering, and Technology
- History of Engineering and Technology
- Mechanical Engineering and Materials
- Technology of Industrial Chemistry
- Transport Technology and Trades
- Browse content in Environmental Science
- Applied Ecology (Environmental Science)
- Conservation of the Environment (Environmental Science)
- Environmental Sustainability
- Environmentalist Thought and Ideology (Environmental Science)
- Management of Land and Natural Resources (Environmental Science)
- Natural Disasters (Environmental Science)
- Nuclear Issues (Environmental Science)
- Pollution and Threats to the Environment (Environmental Science)
- Social Impact of Environmental Issues (Environmental Science)
- History of Science and Technology
- Browse content in Materials Science
- Ceramics and Glasses
- Composite Materials
- Metals, Alloying, and Corrosion
- Nanotechnology
- Browse content in Mathematics
- Applied Mathematics
- Biomathematics and Statistics
- History of Mathematics
- Mathematical Education
- Mathematical Finance
- Mathematical Analysis
- Numerical and Computational Mathematics
- Probability and Statistics
- Pure Mathematics
- Browse content in Neuroscience
- Cognition and Behavioural Neuroscience
- Development of the Nervous System
- Disorders of the Nervous System
- History of Neuroscience
- Invertebrate Neurobiology
- Molecular and Cellular Systems
- Neuroendocrinology and Autonomic Nervous System
- Neuroscientific Techniques
- Sensory and Motor Systems
- Browse content in Physics
- Astronomy and Astrophysics
- Atomic, Molecular, and Optical Physics
- Biological and Medical Physics
- Classical Mechanics
- Computational Physics
- Condensed Matter Physics
- Electromagnetism, Optics, and Acoustics
- History of Physics
- Mathematical and Statistical Physics
- Measurement Science
- Nuclear Physics
- Particles and Fields
- Plasma Physics
- Quantum Physics
- Relativity and Gravitation
- Semiconductor and Mesoscopic Physics
- Browse content in Psychology
- Affective Sciences
- Clinical Psychology
- Cognitive Psychology
- Cognitive Neuroscience
- Criminal and Forensic Psychology
- Developmental Psychology
- Educational Psychology
- Evolutionary Psychology
- Health Psychology
- History and Systems in Psychology
- Music Psychology
- Neuropsychology
- Organizational Psychology
- Psychological Assessment and Testing
- Psychology of Human-Technology Interaction
- Psychology Professional Development and Training
- Research Methods in Psychology
- Social Psychology
- Browse content in Social Sciences
- Browse content in Anthropology
- Anthropology of Religion
- Human Evolution
- Medical Anthropology
- Physical Anthropology
- Regional Anthropology
- Social and Cultural Anthropology
- Theory and Practice of Anthropology
- Browse content in Business and Management
- Business Strategy
- Business Ethics
- Business History
- Business and Government
- Business and Technology
- Business and the Environment
- Comparative Management
- Corporate Governance
- Corporate Social Responsibility
- Entrepreneurship
- Health Management
- Human Resource Management
- Industrial and Employment Relations
- Industry Studies
- Information and Communication Technologies
- International Business
- Knowledge Management
- Management and Management Techniques
- Operations Management
- Organizational Theory and Behaviour
- Pensions and Pension Management
- Public and Nonprofit Management
- Strategic Management
- Supply Chain Management
- Browse content in Criminology and Criminal Justice
- Criminal Justice
- Criminology
- Forms of Crime
- International and Comparative Criminology
- Youth Violence and Juvenile Justice
- Development Studies
- Browse content in Economics
- Agricultural, Environmental, and Natural Resource Economics
- Asian Economics
- Behavioural Finance
- Behavioural Economics and Neuroeconomics
- Econometrics and Mathematical Economics
- Economic Systems
- Economic History
- Economic Methodology
- Economic Development and Growth
- Financial Markets
- Financial Institutions and Services
- General Economics and Teaching
- Health, Education, and Welfare
- History of Economic Thought
- International Economics
- Labour and Demographic Economics
- Law and Economics
- Macroeconomics and Monetary Economics
- Microeconomics
- Public Economics
- Urban, Rural, and Regional Economics
- Welfare Economics
- Browse content in Education
- Adult Education and Continuous Learning
- Care and Counselling of Students
- Early Childhood and Elementary Education
- Educational Equipment and Technology
- Educational Strategies and Policy
- Higher and Further Education
- Organization and Management of Education
- Philosophy and Theory of Education
- Schools Studies
- Secondary Education
- Teaching of a Specific Subject
- Teaching of Specific Groups and Special Educational Needs
- Teaching Skills and Techniques
- Browse content in Environment
- Applied Ecology (Social Science)
- Climate Change
- Conservation of the Environment (Social Science)
- Environmentalist Thought and Ideology (Social Science)
- Natural Disasters (Environment)
- Social Impact of Environmental Issues (Social Science)
- Browse content in Human Geography
- Cultural Geography
- Economic Geography
- Political Geography
- Browse content in Interdisciplinary Studies
- Communication Studies
- Museums, Libraries, and Information Sciences
- Browse content in Politics
- African Politics
- Asian Politics
- Chinese Politics
- Comparative Politics
- Conflict Politics
- Elections and Electoral Studies
- Environmental Politics
- European Union
- Foreign Policy
- Gender and Politics
- Human Rights and Politics
- Indian Politics
- International Relations
- International Organization (Politics)
- International Political Economy
- Irish Politics
- Latin American Politics
- Middle Eastern Politics
- Political Methodology
- Political Communication
- Political Philosophy
- Political Sociology
- Political Behaviour
- Political Economy
- Political Institutions
- Political Theory
- Politics and Law
- Politics of Development
- Public Administration
- Public Policy
- Quantitative Political Methodology
- Regional Political Studies
- Russian Politics
- Security Studies
- State and Local Government
- UK Politics
- US Politics
- Browse content in Regional and Area Studies
- African Studies
- Asian Studies
- East Asian Studies
- Japanese Studies
- Latin American Studies
- Middle Eastern Studies
- Native American Studies
- Scottish Studies
- Browse content in Research and Information
- Research Methods
- Browse content in Social Work
- Addictions and Substance Misuse
- Adoption and Fostering
- Care of the Elderly
- Child and Adolescent Social Work
- Couple and Family Social Work
- Direct Practice and Clinical Social Work
- Emergency Services
- Human Behaviour and the Social Environment
- International and Global Issues in Social Work
- Mental and Behavioural Health
- Social Justice and Human Rights
- Social Policy and Advocacy
- Social Work and Crime and Justice
- Social Work Macro Practice
- Social Work Practice Settings
- Social Work Research and Evidence-based Practice
- Welfare and Benefit Systems
- Browse content in Sociology
- Childhood Studies
- Community Development
- Comparative and Historical Sociology
- Economic Sociology
- Gender and Sexuality
- Gerontology and Ageing
- Health, Illness, and Medicine
- Marriage and the Family
- Migration Studies
- Occupations, Professions, and Work
- Organizations
- Population and Demography
- Race and Ethnicity
- Social Theory
- Social Movements and Social Change
- Social Research and Statistics
- Social Stratification, Inequality, and Mobility
- Sociology of Religion
- Sociology of Education
- Sport and Leisure
- Urban and Rural Studies
- Browse content in Warfare and Defence
- Defence Strategy, Planning, and Research
- Land Forces and Warfare
- Military Administration
- Military Life and Institutions
- Naval Forces and Warfare
- Other Warfare and Defence Issues
- Peace Studies and Conflict Resolution
- Weapons and Equipment

- < Previous
- Next chapter >

1 Primer on Depression: Introduction and Overview
- Published: October 2019
- Cite Icon Cite
- Permissions Icon Permissions
Major depressive disorder (MDD) is a serious, debilitating, life-shortening illness that affects many persons of all ages and backgrounds. The point prevalence of MDD is high (2.3–3.2% in men; 4.5–9.3% in women) and the lifetime risk for MDD is 7% to 12% for men and 20% to 25% for women. MDD is a disabling disorder that costs the United States over $200 billion per year in direct and indirect costs. Depression also has detrimental effects on all aspects of social functioning (e.g., self-care, social role, and family life, including household, marital, kinship, and parental roles). While there have been several treatments that are efficacious, many individuals suffering from depression experience lifelong challenges due to the often chronic and episodic nature of the disease. Identifying strategies to find the right treatments for the right patients is critical.
Signed in as
Institutional accounts.
- GoogleCrawler [DO NOT DELETE]
- Google Scholar Indexing
Personal account
- Sign in with email/username & password
- Get email alerts
- Save searches
- Purchase content
- Activate your purchase/trial code
- Add your ORCID iD
Institutional access
Sign in with a library card.
- Sign in with username/password
- Recommend to your librarian
- Institutional account management
- Get help with access
Access to content on Oxford Academic is often provided through institutional subscriptions and purchases. If you are a member of an institution with an active account, you may be able to access content in one of the following ways:
IP based access
Typically, access is provided across an institutional network to a range of IP addresses. This authentication occurs automatically, and it is not possible to sign out of an IP authenticated account.
Choose this option to get remote access when outside your institution. Shibboleth/Open Athens technology is used to provide single sign-on between your institution’s website and Oxford Academic.
- Click Sign in through your institution.
- Select your institution from the list provided, which will take you to your institution's website to sign in.
- When on the institution site, please use the credentials provided by your institution. Do not use an Oxford Academic personal account.
- Following successful sign in, you will be returned to Oxford Academic.
If your institution is not listed or you cannot sign in to your institution’s website, please contact your librarian or administrator.
Enter your library card number to sign in. If you cannot sign in, please contact your librarian.
Society Members
Society member access to a journal is achieved in one of the following ways:
Sign in through society site
Many societies offer single sign-on between the society website and Oxford Academic. If you see ‘Sign in through society site’ in the sign in pane within a journal:
- Click Sign in through society site.
- When on the society site, please use the credentials provided by that society. Do not use an Oxford Academic personal account.
If you do not have a society account or have forgotten your username or password, please contact your society.
Sign in using a personal account
Some societies use Oxford Academic personal accounts to provide access to their members. See below.
A personal account can be used to get email alerts, save searches, purchase content, and activate subscriptions.
Some societies use Oxford Academic personal accounts to provide access to their members.
Viewing your signed in accounts
Click the account icon in the top right to:
- View your signed in personal account and access account management features.
- View the institutional accounts that are providing access.
Signed in but can't access content
Oxford Academic is home to a wide variety of products. The institutional subscription may not cover the content that you are trying to access. If you believe you should have access to that content, please contact your librarian.
For librarians and administrators, your personal account also provides access to institutional account management. Here you will find options to view and activate subscriptions, manage institutional settings and access options, access usage statistics, and more.
Our books are available by subscription or purchase to libraries and institutions.
- About Oxford Academic
- Publish journals with us
- University press partners
- What we publish
- New features
- Open access
- Rights and permissions
- Accessibility
- Advertising
- Media enquiries
- Oxford University Press
- Oxford Languages
- University of Oxford
Oxford University Press is a department of the University of Oxford. It furthers the University's objective of excellence in research, scholarship, and education by publishing worldwide
- Copyright © 2024 Oxford University Press
- Cookie settings
- Cookie policy
- Privacy policy
- Legal notice
This Feature Is Available To Subscribers Only
Sign In or Create an Account
This PDF is available to Subscribers Only
For full access to this pdf, sign in to an existing account, or purchase an annual subscription.
Thank you for visiting nature.com. You are using a browser version with limited support for CSS. To obtain the best experience, we recommend you use a more up to date browser (or turn off compatibility mode in Internet Explorer). In the meantime, to ensure continued support, we are displaying the site without styles and JavaScript.
- View all journals
- Explore content
- About the journal
- Publish with us
- Sign up for alerts
- Perspective
- Published: 14 April 2022
Revisiting the theoretical and methodological foundations of depression measurement
- Eiko I. Fried ORCID: orcid.org/0000-0001-7469-594X 1 ,
- Jessica K. Flake 2 &
- Donald J. Robinaugh 3 , 4
Nature Reviews Psychology volume 1 , pages 358–368 ( 2022 ) Cite this article
91 Citations
236 Altmetric
Metrics details
- Human behaviour
- Psychiatric disorders
Depressive disorders are among the leading causes of global disease burden, but there has been limited progress in understanding the causes of and treatments for these disorders. In this Perspective, we suggest that such progress depends crucially on our ability to measure depression. We review the many problems with depression measurement, including the limited evidence of validity and reliability. These issues raise grave concerns about common uses of depression measures, such as for diagnosis or tracking treatment progress. We argue that shortcomings arise because the measurement of depression rests on shaky methodological and theoretical foundations. Moving forward, we need to break with the field’s tradition, which has, for decades, divorced theories about depression from how we measure it. Instead, we suggest that epistemic iteration, an iterative exchange between theory and measurement, provides a crucial avenue for progressing how we measure depression.
This is a preview of subscription content, access via your institution
Access options
Subscribe to this journal
Receive 12 digital issues and online access to articles
55,14 € per year
only 4,60 € per issue
Buy this article
- Purchase on Springer Link
- Instant access to full article PDF
Prices may be subject to local taxes which are calculated during checkout
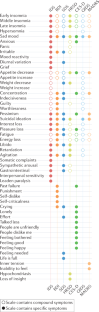
Similar content being viewed by others
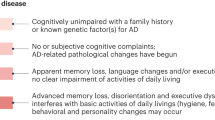
Alzheimer’s disease risk reduction in clinical practice: a priority in the emerging field of preventive neurology
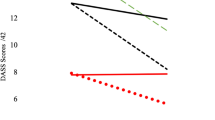
Psilocybin microdosers demonstrate greater observed improvements in mood and mental health at one month relative to non-microdosing controls
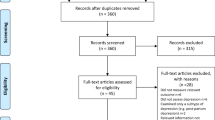
The serotonin theory of depression: a systematic umbrella review of the evidence
Data availability.
Data underlying Figs 1 , 2 and 3 can be found at https://osf.io/7dp5s/ .
Code availability
Code to reproduce Figs 1 and 2 (minus graphical edits performed by the journal art editor), and run the simulation underlying Fig. 3 , can be found at https://osf.io/7dp5s/ .
Santor, D. A., Gregus, M. & Welch, A. Eight decades of measurement in depression. Measurement 4 , 135–155 (2006).
Google Scholar
van Noorden, R., Maher, B. & Nuzzo, R. The top 100 papers. Nature 514 , 550–553 (2014).
Article PubMed Google Scholar
Hamilton, M. A rating scale for depression. J. Neurol. Neurosurg. Psychiatry 23 , 56–62 (1960).
Article PubMed PubMed Central Google Scholar
Beck, A. T., Ward, C. H., Mendelson, M., Mock, J. & Erbaugh, J. An inventory for measuring depression. Arch. Gen. Psychiatry 4 , 561–571 (1961).
Radloff, L. S. The CES-D scale: a self-report depression scale for research in the general population. Appl. Psychol. Meas. 1 , 385–401 (1977).
Article Google Scholar
Jorm, A. F., Patten, S. B., Brugha, T. S. & Mojtabi, R. Has increased provision of treatment reduced the prevalence of common mental disorders? Review of the evidence from four countries. World Psychiatry 16 , 90–99 (2017).
Kapur, S., Phillips, A. G. & Insel, T. Why has it taken so long for biological psychiatry to develop clinical tests and what to do about it? Mol. Psychiatry 17 , 1174–1179 (2012).
Scull, A. American psychiatry in the new millennium: a critical appraisal. Psychol. Med. https://doi.org/10.1017/S0033291721001975 (2021).
Cuijpers, P. et al. The effects of psychotherapies for depression on response, remission, reliable change, and deterioration: a meta-analysis. Acta Psychiatr. Scand. 144 , 288–299 (2021).
Khan, A. & Brown, W. A. Antidepressants versus placebo in major depression: an overview. World Psychiatry 14 , 294–300 (2015).
Kendler, K., Munõz, R. & Murphy, G. The development of the Feighner criteria: a historical perspective. Am. J. Psychiatry 167 , 134–142 (2010).
Spitzer, R. L. Psychiatric diagnosis: are clinicians still necessary? Compr. Psychiatry 24 , 399–411 (1983).
Horwitz, A. V. in The Encyclopedia of Clinical Psychology (eds Cautin, R. L. & Lilienfeld, S. O.) https://doi.org/10.1002/9781118625392.wbecp012 (Wiley, 2015).
Beck, A. Reliability of psychiatric diagnoses: 1. A critique of systematic studies. Am. J. Psychiatry 119 , 210–216 (1962).
Ash, P. The reliability of psychiatric diagnoses. J. Abnorm. Soc. Psychol. 44 , 272–276 (1949).
Feighner, J. P. et al. Diagnostic criteria for use in psychiatric research. Arch. Gen. Psychiatry 26 , 57–63 (1972).
APA. Diagnostic and Statistical Manual of Mental Disorders 3rd edn (American Psychiatric Association, 1980).
Fried, E. The 52 symptoms of major depression: lack of content overlap among seven common depression scales. J. Affect. Disord. 208 , 191–197 (2017).
Cipriani, A. et al. Comparative efficacy and acceptability of 21 antidepressant drugs for the acute treatment of adults with major depressive disorder: a systematic review and network meta-analysis. Lancet 391 , 1357–1366 (2018).
Cronbach, L. J. & Meehl, P. E. Construct validity in psychological tests. Psychol. Bull. 52 , 281–302 (1955).
Robins, E. & Guze, S. B. Establishment of diagnostic validity in psychiatric illness: its application to schizophrenia. Am. J. Psychiatry 126 , 983–987 (1970).
Bandalos, D. L. Measurement Theory and Applications for the Social Sciences (Guilford, 2018).
Kane, M. T. Validating the interpretations and uses of test scores. J. Educ. Meas. 50 , 1–73 (2013).
Mokkink, L. B. et al. The COSMIN study reached international consensus on taxonomy, terminology, and definitions of measurement properties for health-related patient-reported outcomes. J. Clin. Epidemiol. 63 , 737–745 (2010).
American Educational Research Association, American Psychological Association & National Council on Measurement in Education. Standards for Educational and Psychological Testing (Joint Committee on Standards for Educational and Psychological Testing, 2014).
Messick, S. Meaning and values in test validation: the science and ethics of assessment. Educ. Res. 18 , 5–11 (1989).
Fried, E. I. Corrigendum to “The 52 symptoms of major depression: lack of content overlap among seven common depression scales” [Journal of Affective Disorders, 208, 191–197]. J. Affect. Disord. 260 , 744 (2020).
Mew, E. J. et al. Systematic scoping review identifies heterogeneity in outcomes measured in adolescent depression clinical trials. J. Clin. Epidemiol. 126 , 71–79 (2020).
Chevance, A. M. et al. Identifying outcomes for depression that matter to patients, informal caregivers and healthcare professionals: qualitative content analysis of a large international online survey. Lancet Psychiatry 7 , 692–702 (2020).
Wittkampf, K. et al. The accuracy of Patient Health Questionnaire-9 in detecting depression and measuring depression severity in high-risk groups in primary care. Gen. Hosp. Psychiatry 31 , 451–459 (2009).
Sayer, N. N. A. et al. The relations between observer-rating and self-report of depressive symptomatology. Psychol. Assess. 5 , 350–360 (1993).
Furukawa, T. A. et al. Translating the BDI and BDI-II into the HAMD and vice versa with equipercentile linking. Epidemiol. Psychiatr. Sci. 29 , E24 (2019).
Fried, E. et al. Measuring depression over time … or not? Lack of unidimensionality and longitudinal measurement invariance in four common rating scales of depression. Psychol. Assess. 28 , 1354–1367 (2016).
Beck, A. T., Rush, A. J., Shaw, F. S. & Emery, G. Cognitive Therapy of Depression (Guilford, 1979).
Montgomery, S. A. & Asberg, M. A new depression scale designed to be sensitive to change. Br. J. Psychiatry 134 , 382–389 (1979).
von Glischinski, M., von Brachel, R., Thiele, C. & Hirschfeld, G. Not sad enough for a depression trial? A systematic review of depression measures and cut points in clinical trial registrations: systematic review of depression measures and cut points. J. Affect. Disord. 292 , 36–44 (2021).
Kroenke, K., Spitzer, R. L. & Williams, J. B. The PHQ-9: validity of a brief depression severity measure. J. Gen. Intern. Med. 16 , 606–613 (2001).
Levis, B. et al. Patient Health Questionnaire-9 scores do not accurately estimate depression prevalence: individual participant data meta-analysis. J. Clin. Epidemiol. 122 , 115–128.e1 (2020).
Whiston, S. Principles and Applications of Assessment in Counseling (Brooks/Cole, Cengage Learning, 2009).
Thombs, B. D., Kwakkenbos, L., Levis, A. W. & Benedetti, A. Addressing overestimation of the prevalence of depression based on self-report screening questionnaires. Can. Med. Assoc. J. 190 , 44–49 (2018).
Lavender, J. M. & Anderson, D. A. Effect of perceived anonymity in assessments of eating disordered behaviors and attitudes. Int. J. Eat. Disord. 42 , 546–551 (2009).
Keel, P. K., Crow, S., Davis, T. L. & Mitchell, J. E. Assessment of eating disorders: comparison of interview and questionnaire data from a long-term follow-up study of bulimia nervosa. J. Psychosom. Res. 53 , 1043–1047 (2002).
Croskerry, P. The importance of cognitive errors in diagnosis and strategies to minimize them. Acad. Med. 78 , 775–780 (2003).
Kim, N. S. & Ahn, W. Clinical psychologists’ theory-based representations of mental disorders predict their diagnostic reasoning and memory. J. Exp. Psychol. Gen. 131 , 451–476 (2002).
Aboraya, A. Clinicians’ opinions on the reliability of psychiatric diagnoses in clinical settings. Psychiatry 4 , 31–33 (2007).
PubMed PubMed Central Google Scholar
American Psychiatric Association. Diagnostic and Statistical Manual of Mental Disorders: DSM-IV-TR (American Psychiatric Association, 2000).
Ruscio, J., Zimmerman, M., McGlinchey, J. B., Chelminski, I. & Young, D. Diagnosing major depressive disorder XI: a taxometric investigation of the structure underlying DSM-IV symptoms. J. Nerv. Ment. Dis. 195 , 10–19 (2007).
Haslam, N. Categorical versus dimensional models of mental disorder: the taxometric evidence. Aust. N. Z. J. Psychiatry 37 , 696–704 (2003).
Haslam, N., Holland, E. & Kuppens, P. Categories versus dimensions in personality and psychopathology: a quantitative review of taxometric research. Psychol. Med. 42 , 903–920 (2012).
Nettle, D. in Maladapting Minds: Philosophy, Psychiatry, and Evolutionary Theory (eds Adriaens, P. R. & De Block, A.) 192–209 (Oxford Univ. Press, 2011).
Courtney, D. B. et al. Forks in the road: definitions of response, remission, recovery and other dichotomized outcomes in randomized controlled trials for adolescent depression. A scoping review. Depress. Anxiety 38 , 1152–1168 (2021).
Fried, E. & Nesse, R. M. Depression sum-scores don’t add up: why analyzing specific depression symptoms is essential. BMC Med. 13 , 1–11 (2015).
McNeish, D. & Wolf, M. G. Thinking twice about sum scores. Behav. Res. Methods 52 , 2287–2305 (2020).
Gullion, C. M. & Rush, A. J. Toward a generalizable model of symptoms in major depressive disorder. Biol. Psychiatry 44 , 959–972 (1998).
Helmes, E. & Nielson, W. R. An examination of the internal structure of the Center for Studies-Depression Scale in two medical samples. Pers. Individ. Dif. 25 , 735–743 (1998).
Shafer, A. B. Meta-analysis of the factor structures of four depression questionnaires: Beck, CES-D, Hamilton, and Zung. J. Clin. Psychol. 62 , 123–146 (2006).
van Loo, H. M., de Jonge, P., Romeijn, J.-W., Kessler, R. C. & Schoevers, R. A. Data-driven subtypes of major depressive disorder: a systematic review. BMC Med. 10 , 156 (2012).
Quilty, L. C. et al. The structure of the Montgomery–Åsberg Depression Rating Scale over the course of treatment for depression. Int. J. Methods Psychiatr. Res. 22 , 175–184 (2013).
Elhai, J. D. et al. The factor structure of major depression symptoms: a test of four competing models using the Patient Health Questionnaire-9. Psychiatry Res. 199 , 169–173 (2012).
Wardenaar, K. J. et al. The structure and dimensionality of the Inventory of Depressive Symptomatology Self Report (IDS-SR) in patients with depressive disorders and healthy controls. J. Affect. Disord. 125 , 146–154 (2010).
Wood, A. M., Taylor, P. J. & Joseph, S. Does the CES-D measure a continuum from depression to happiness? Comparing substantive and artifactual models. Psychiatry Res. 177 , 120–123 (2010).
Furukawa, T. et al. Cross-cultural equivalence in depression assessment: Japan–Europe–North American study. Acta Psychiatr. Scand. 112 , 279–285 (2005).
Lux, V. & Kendler, K. Deconstructing major depression: a validation study of the DSM-IV symptomatic criteria. Psychol. Med. 40 , 1679–1690 (2010).
Fried, E., Nesse, R. M., Zivin, K., Guille, C. & Sen, S. Depression is more than the sum score of its parts: individual DSM symptoms have different risk factors. Psychol. Med. 44 , 2067–2076 (2014).
Faravelli, C., Servi, P., Arends, J. & Strik, W. Number of symptoms, quantification, and qualification of depression. Compr. Psychiatry 37 , 307–315 (1996).
Tweed, D. L. Depression-related impairment: estimating concurrent and lingering effects. Psychol. Med. 23 , 373–386 (1993).
Fried, E. & Nesse, R. M. The impact of individual depressive symptoms on impairment of psychosocial functioning. PLoS ONE 9 , e90311 (2014).
Hasler, G., Drevets, W. C., Manji, H. K. & Charney, D. S. Discovering endophenotypes for major depression. Neuropsychopharmacology 29 , 1765–1781 (2004).
Myung, W. et al. Genetic association study of individual symptoms in depression. Psychiatry Res. 198 , 400–406 (2012).
Kendler, K., Aggen, S. H. & Neale, M. C. Evidence for multiple genetic factors underlying DSM-IV criteria for major depression. Am. J. Psychiatry 70 , 599–607 (2013).
Nagel, M., Watanabe, K., Stringer, S., Posthuma, D. & Van Der Sluis, S. Item-level analyses reveal genetic heterogeneity in neuroticism. Nat. Commun. 9 , 905 (2018).
Hilland, E. et al. Exploring the links between specific depression symptoms and brain structure: a network study. Psychiatry Clin. Neurosci. 74 , 220–221 (2020).
Fried, E. et al. Using network analysis to examine links between individual depressive symptoms, inflammatory markers, and covariates. Psychol. Med. 50 , 2682–2690 (2020).
Eeden, W. A. V. et al. Basal and LPS-stimulated inflammatory markers and the course of individual symptoms of depression. Transl. Psychiatry 10 , 235 (2020).
Keller, M. C. & Nesse, R. M. Is low mood an adaptation? Evidence for subtypes with symptoms that match precipitants. J. Affect. Disord. 86 , 27–35 (2005).
Keller, M. C. & Nesse, R. M. The evolutionary significance of depressive symptoms: different adverse situations lead to different depressive symptom patterns. J. Pers. Soc. Psychol. 91 , 316–330 (2006).
Keller, M. C., Neale, M. C. & Kendler, K. Association of different adverse life events with distinct patterns of depressive symptoms. Am. J. Psychiatry 164 , 1521–1529 (2007).
Cramer, A. O. J., Borsboom, D., Aggen, S. H. & Kendler, K. The pathoplasticity of dysphoric episodes: differential impact of stressful life events on the pattern of depressive symptom inter-correlations. Psychol. Med. 42 , 957–965 (2013).
Fried, E. et al. From loss to loneliness: the relationship between bereavement and depressive symptoms. J. Abnorm. Psychol. 124 , 256–265 (2015).
Rush, A. J., Gullion, C. M., Basco, M. R., Jarrett, R. B. & Trivedi, M. H. The Inventory of Depressive Symptomatology (IDS): psychometric properties. Psychol. Med. 26 , 477–486 (1996).
Rush, A. J. et al. The 16-Item Quick Inventory of Depressive Symptomatology (QIDS), Clinician Rating (QIDS-C), and Self-Report (QIDS-SR): a psychometric evaluation in patients with chronic major dDepression. Biol. Psychiatry 54 , 573–583 (2003).
Fried, E. & Nesse, R. M. Depression is not a consistent syndrome: an investigation of unique symptom patterns in the STAR*D study. J. Affect. Disord. 172 , 96–102 (2015).
Zimmerman, M., Ellison, W., Young, D., Chelminski, I. & Dalrymple, K. How many different ways do patients meet the diagnostic criteria for major depressive disorder? Compr. Psychiatry 56 , 29–34 (2014).
Lichtenberg, P. & Belmaker, R. H. Subtyping major depressive disorder. Psychother. Psychosom. 79 , 131–135 (2010).
Baumeister, H. & Parker, J. D. Meta-review of depressive subtyping models. J. Affect. Disord. 139 , 126–140 (2012).
Bech, P. Struggle for subtypes in primary and secondary depression and their mode-specific treatment or healing. Psychother. Psychosom. 79 , 331–338 (2010).
Lam, R. W. & Stewart, J. N. The validity of atypical depression in DSM-IV. Compr. Psychiatry 37 , 375–383 (1996).
Davidson, J. R. T. A history of the concept of atypical depression. J. Clin. Psychiatry 68 , 10–15 (2007).
Arnow, B. A. et al. Depression subtypes in predicting antidepressant response: a report from the iSPOT-D trial. Am. J. Psychiatry 172 , 743–750 (2015).
Paykel, E. S. Basic concepts of depression. Dialogues Clin. Neurosci. 10 , 279–289 (2008).
Rush, A. J. The varied clinical presentations of major depressive disorder. J. Clin. Psychiatry 68 , 4–10 (2007).
PubMed Google Scholar
Melartin, T. et al. Co-morbidity and stability of melancholic features in DSM-IV major depressive disorder. Psychol. Med. 34 , 1443 (2004).
Fried, E., Coomans, F. & Lorenzo-luaces, L. The 341 737 ways of qualifying for the melancholic specifier. Lancet Psychiatry 7 , 479–480 (2020).
Oquendo, M. A. et al. Instability of symptoms in recurrent major depression: a prospective study. Am. J. Psychiatry 161 , 255–261 (2004).
Coryell, W. et al. Recurrently situational (reactive) depression: a study of course, phenomenology and familial psychopathology. J. Affect. Disord. 31 , 203–210 (1994).
Pae, C. U., Tharwani, H., Marks, D. M., Masand, P. S. & Patkar, A. A. Atypical depression: a comprehensive review. CNS Drugs 23 , 1023–1037 (2009).
Magnusson, A. & Boivin, D. Seasonal affective disorder: an overview. Chronobiol. Int. 20 , 189–207 (2003).
Meyerhoff, J., Young, M. A. & Rohan, K. J. Patterns of depressive symptom remission during the treatment of seasonal affective disorder with cognitive-behavioral therapy or light therapy. Depress. Anxiety 35 , 457–467 (2018).
Lam, R. W. et al. Efficacy of bright light treatment, fluoxetine, and the combination in patients with nonseasonal major depressive disorder a randomized clinical trial. JAMA Psychiatry 73 , 56–63 (2016).
Meredith, W. Measurement invariance, factor analysis and factorial invariance. Psychometrika 58 , 525–543 (1993).
Kendler, K. et al. The similarity of the structure of DSM-IV criteria for major depression in depressed women from China, the United States and Europe. Psychol. Med. 45 , 1945–1954 (2015).
Yu, X., Tam, W. W. S., Wong, P. T. K., Lam, T. H. & Stewart, S. M. The Patient Health Questionnaire-9 for measuring depressive symptoms among the general population in Hong Kong. Compr. Psychiatry 53 , 95–102 (2012).
Nguyen, H. T., Kitner-Triolo, M., Evans, M. K. & Zonderman, A. B. Factorial invariance of the CES-D in low socioeconomic status African Americans compared with a nationally representative sample. Psychiatry Res. 126 , 177–187 (2004).
Crockett, L. J., Randall, B. A., Shen, Y.-L., Russell, S. T. & Driscoll, A. K. Measurement equivalence of the center for epidemiological studies depression scale for Latino and Anglo adolescents: a national study. J. Consult. Clin. Psychol. 73 , 47–58 (2005).
Baas, K. D. et al. Measurement invariance with respect to ethnicity of the Patient Health Questionnaire-9 (PHQ-9). J. Affect. Disord. 129 , 229–235 (2011).
Williams, C. D. et al. CES-D four-factor structure is confirmed, but not invariant, in a large cohort of African American women. Psychiatry Res. 150 , 173–180 (2007).
Stochl, J. et al. On dimensionality, measurement invariance, and suitability of sum scores for the PHQ-9 and the GAD-7. Assessment 29 , 355–366 (2022).
Fokkema, M., Smits, N., Kelderman, H. & Cuijpers, P. Response shifts in mental health interventions: an illustration of longitudinal measurement invariance. Psychol. Assess. 25 , 520–531 (2013).
Bagby, R. M., Ryder, A. G., Schuller, D. R. & Marshall, M. B. Reviews and overviews the hamilton depression rating scale: has the gold standard become a lead weight? Am. J. Psyc 161 , 2163–2177 (2004).
Trajković, G. et al. Reliability of the Hamilton Rating Scale for Depression: a meta-analysis over a period of 49 years. Psychiatry Res. 189 , 1–9 (2011).
Regier, D. A. et al. DSM-5 field trials in the United States and Canada, part II: test–retest reliability of selected categorical diagnoses. Am. J. Psychiatry 170 , 59–70 (2013).
Bruchmüller, K., Margraf, J., Suppiger, A. & Schneider, S. Popular or unpopular? Therapists’ use of structured interviews and their estimation of patient acceptance. Behav. Ther. 42 , 634–643 (2011).
Kupfer, D. J. & Kraemer, H. C. Field trial results guide DSM recommendations. Huffington Post http://www.huffingtonpost.com/david-j-kupfer-md/dsm-5_b_2083092.html (2013).
Clarke, D. E. et al. DSM-5 field trials in the United States and Canada, part I: study design, sampling strategy, implementation, and analytic approaches. Am. J. Psychiatry 170 , 43–58 (2012).
Fernández, A. et al. Is major depression adequately diagnosed and treated by general practitioners? Results from an epidemiological study. Gen. Hosp. Psychiatry 32 , 201–209 (2010).
Huxley, P. Mental illness in the community: the Goldberg–Huxley model of the pathway to psychiatric care. Nord. J. Psychiatry, Suppl. 50 , 47–53 (1996).
Flake, J. K., Pek, J. & Hehman, E. Construct validation in social and personality research: current practice and recommendations. Soc. Psychol. Personal. Sci. 8 , 370–378 (2017).
de Vet, H. C. W., Terwee, C. B., Mokkink, L. B. & Knol, D. L. Measurement in Medicine: A Practical Guide (Cambridge Univ. Press, 2011).
Beck, A. T., Steer, R. A., Ball, R. & Ranieri, W. Comparison of Beck Depression Inventories -IA and -II in psychiatric outpatients. J. Pers. Assess. 67 , 588–597 (1996).
McPherson, S. & Armstrong, D. Psychometric origins of depression. Hist. Hum. Sci. https://doi.org/10.1177/09526951211009085 (2021).
Lilienfeld, S. O. DSM-5: centripetal scientific and centrifugal. Clin. Psychol. Sci. Pract. 21 , 269–279 (2014).
Flake, J. K. & Fried, E. Measurement schmeasurement: questionable measurement practices and how to avoid them. Adv. Methods Pract. Psychol. Sci. 3 , 456–465 (2020).
Robinaugh, D. J., Haslbeck, J. M. B., Ryan, O., Fried, E. & Waldorp, L. J. Invisible hands and fine calipers: a call to use formal theory as a toolkit for theory construction. Perspect. Psychol. Sci. 16 , 725–743 (2021).
Robinaugh, D. J. et al. Advancing the network theory of mental disorders: a computational model of panic disorder. Preprint at PsyArXiv https://doi.org/10.31234/osf.io/km37w (2019).
Fried, E. Lack of theory building and testing impedes progress in the factor and network. Psychol. Inq. 31 , 271–288 (2020).
Van Bork, R., Wijsen, L. D. & Rhemtulla, M. Toward a causal interpretation of the common factor model. Disputatio 9 , 581–601 (2017).
Fried, E. Problematic assumptions have slowed down depression research: why symptoms, not syndromes are the way forward. Front. Psychol. 6 , 1–11 (2015).
Fried, E. & Cramer, A. O. J. Moving forward: challenges and directions for psychopathological network theory and methodology. Perspect. Psychol. Sci. 12 , 999–1020 (2017).
Fried, E. Moving forward: how depression heterogeneity hinders progress in treatment and research. Expert Rev. Neurother. 17 , 423–425 (2017).
Fried, E. & Robinaugh, D. J. Systems all the way down: embracing complexity in mental health research. BMC Med. 18 , 1–4 (2020).
Cicchetti, D. & Rogosch, F. A. Equifinality and multifinality in developmental psychopathology. Dev. Psychopathol. 8 , 597–600 (1996).
Borsboom, D. et al. Kinds versus continua: a review of psychometric approaches to uncover the structure of psychiatric constructs. Psychol. Med. 46 , 1567–1579 (2016).
Chang, H. Inventing Temperature: Measurement and Scientific Progress (Oxford Univ. Press, 2004).
Borsboom, D., van der Maas, H. L. J., Dalege, J., Kievit, R. & Haig, B. Theory construction methodology: a practical framework for theory formation in psychology. Perspect. Psychol. Sci. 16 , 756–766 (2020).
Borsboom, D. A network theory of mental disorders. World Psychiatry 16 , 5–13 (2017).
Kendler, K., Zachar, P. & Craver, C. What kinds of things are psychiatric disorders? Psychol. Med. 41 , 1143–1150 (2011).
Olthof, M., Hasselman, F., Maatman, F. O. & Bosman, A. M. T. Complexity theory of psychopathology. Preprint at PsyArXiv https://doi.org/10.31234/osf.io/f68ej (2021).
Robinaugh, D. J., Hoekstra, R. H. A., Toner, E. R. & Borsboom, D. The network approach to psychopathology: a review of the literature 2008–2018 and an agenda for future research. Psychol. Med. 50 , 353–366 (2020).
Hammen, C. Stress and depression. Annu. Rev. Clin. Psychol. 1 , 293–319 (2005).
Kendler, K., Karkowski, L. M. & Prescott, C. A. Causal relationship between stressful life events and the onset of major depression. Am. J. Psychiatry 156 , 837–841 (1999).
Mazure, C. M. Life stressors as risk factors in depression. Clin. Psychol. Sci. Pract. 5 , 291–313 (1998).
McKnight, P. E. & Kashdan, T. B. The importance of functional impairment to mental health outcomes: a case for reassessing our goals in depression treatment research. Clin. Psychol. Rev. 29 , 243–259 (2009).
Brouwer, M. E. et al. Psychological theories of depressive relapse and recurrence: a systematic review and meta-analysis of prospective studies. Clin. Psychol. Rev. 74 , 101773 (2019).
Myin-Germeys, I. & Kuppens, P. The Open Handbook of Experience Sampling Methodology: A Step-by-step Guide to Designing, Conducting, and Analyzing ESM Studies (Katholieke Universiteit Leuven, 2021).
Zung, W. W. K. A self-rating depression scale. Arch. Gen. Psychiatry 12 , 63–70 (1965).
Antony, M. M., Bieling, P. J., Cox, B. J., Enns, M. W. & Swinson, R. P. Psychometric properties of the 42-item and 21-item versions of the Depression Anxiety Stress Scales in clinical groups and a community sample. Psychol. Assess. 10 , 176–181 (1998).
Sijtsma, K. On the use, the misuse, and the very limited usefulness of cronbach. Psychometrika 74 , 107–120 (2009).
Smaldino, P. in Computational Social Psychology (eds Vallacher, R. B., Read, S. J. & Nowak, A.) (Taylor & Francis, 2017).
Presser, S. et al. Methods for testing and evaluating survey questions. Public Opin. Q. 68 , 109–130 (2004).
Gordon Wolf, M., Ihm, E., Maul, A. & Taves, A. Survey item validation. Preprint at PsyArXiv https://doi.org/10.31234/osf.io/k27w3 (2019).
Hawkes, N. & Brown, G. in Assessment in Cognitive Therapy (eds Brown, G. & Clark, D.) 243–267 (Guilford, 2015).
Willis, G. B. Cognitive Interviewing: A Tool for Improving Questionnaire Design (Sage, 2004).
Brown, G., Hawkes, N. & Tata, P. Construct validity and vulnerability to anxiety: a cognitive interviewing study of the revised Anxiety Sensitivity Index. J. Anxiety Disord. 23 , 942–949 (2009).
Patalay, P. & Fried, E. Editorial Perspective: Prescribing measures: unintended negative consequences of mandating standardized mental health measurement. J. Child. Psychol. Psychiatry 8 , 1032–1036 (2021).
Neumann, L. Transparency in Measurement: Reviewing 100 Empirical Papers Using the Hamilton Depression Rating Scale (Leiden Univ., 2020).
Williams, J. B. W. Standardizing the Hamilton Depression Rating Scale: past, present, and future. Eur. Arch. Psychiatry Clin. Neurosci. 251 , 6–12 (2001).
Cybulski, L., Mayo-Wilson, E., Grant, S., Corporation, R. & Monica, S. Improving transparency and reproducibility through registration: the status of intervention trials published in clinical psychology journals. J. Consult. Clin. Psychol. 84 , 753–767 (2016).
Ramagopalan, S. V. et al. Prevalence of primary outcome changes in clinical trials registered on ClinicalTrials.gov: a cross-sectional study. F1000Res. 3 , 77 (2018).
Monsour, A. et al. Primary outcome reporting in adolescent depression clinical trials needs standardization. BMC Med. Res. Methodol. 20 , 1–15 (2020).
Download references
Acknowledgements
The authors thank M.G. Wolf, N. Butcher and Z. Cohen for comments on earlier versions of this manuscript. E.I.F. is supported by funding from the European Research Council (ERC) under the European Union’s Horizon 2020 research and innovation programme (grant no. 949059). D.J.R. was supported by funding from the National Institute for Mental Health (K23 MH113805). The content is solely the responsibility of the authors and does not necessarily represent the views of any funding agency.
Author information
Authors and affiliations.
Department of Clinical Psychology, Leiden University, Leiden, The Netherlands
Eiko I. Fried
Department of Psychology, McGill University, Montreal, Québec, Canada
Jessica K. Flake
Department of Psychiatry, Massachusetts General Hospital, Boston, MA, USA
Donald J. Robinaugh
Department of Applied Psychology, Northeastern University, Boston, MA, USA
You can also search for this author in PubMed Google Scholar
Contributions
E.I.F and D.J.R. developed the idea and outline for the manuscript, E.I.F. and D.J.R. conducted background research for the manuscript and all authors contributed to writing and revision of the manuscript.
Corresponding author
Correspondence to Eiko I. Fried .
Ethics declarations
Competing interests.
The authors declare no competing interests.
Peer review
Peer review information.
Nature Reviews Psychology thanks Ioana Cristea, Kenneth Kendler and Suneeta Monga for their contribution to the peer review of this work.
Additional information
Publisher’s note.
Springer Nature remains neutral with regard to jurisdictional claims in published maps and institutional affiliations.
Supplementary information
Supplementary information, rights and permissions.
Reprints and permissions
About this article
Cite this article.
Fried, E.I., Flake, J.K. & Robinaugh, D.J. Revisiting the theoretical and methodological foundations of depression measurement. Nat Rev Psychol 1 , 358–368 (2022). https://doi.org/10.1038/s44159-022-00050-2
Download citation
Accepted : 22 March 2022
Published : 14 April 2022
Issue Date : June 2022
DOI : https://doi.org/10.1038/s44159-022-00050-2
Share this article
Anyone you share the following link with will be able to read this content:
Sorry, a shareable link is not currently available for this article.
Provided by the Springer Nature SharedIt content-sharing initiative
Quick links
- Explore articles by subject
- Guide to authors
- Editorial policies
Sign up for the Nature Briefing newsletter — what matters in science, free to your inbox daily.

Change Password
Your password must have 8 characters or more and contain 3 of the following:.
- a lower case character,
- an upper case character,
- a special character
Password Changed Successfully
Your password has been changed
- Sign in / Register
Request Username
Can't sign in? Forgot your username?
Enter your email address below and we will send you your username
If the address matches an existing account you will receive an email with instructions to retrieve your username
An Exploratory Study of Students with Depression in Undergraduate Research Experiences
- Katelyn M. Cooper
- Logan E. Gin
- M. Elizabeth Barnes
- Sara E. Brownell
*Address correspondence to: Katelyn M. Cooper ( E-mail Address: [email protected] ).
Department of Biology, University of Central Florida, Orlando, FL, 32816
Search for more papers by this author
Biology Education Research Lab, Research for Inclusive STEM Education Center, School of Life Sciences, Arizona State University, Tempe, AZ 85281
Depression is a top mental health concern among undergraduates and has been shown to disproportionately affect individuals who are underserved and underrepresented in science. As we aim to create a more inclusive scientific community, we argue that we need to examine the relationship between depression and scientific research. While studies have identified aspects of research that affect graduate student depression, we know of no studies that have explored the relationship between depression and undergraduate research. In this study, we sought to understand how undergraduates’ symptoms of depression affect their research experiences and how research affects undergraduates’ feelings of depression. We interviewed 35 undergraduate researchers majoring in the life sciences from 12 research-intensive public universities across the United States who identify with having depression. Using inductive and deductive coding, we identified that students’ depression affected their motivation and productivity, creativity and risk-taking, engagement and concentration, and self-perception and socializing in undergraduate research experiences. We found that students’ social connections, experiencing failure in research, getting help, receiving feedback, and the demands of research affected students’ depression. Based on this work, we articulate an initial set of evidence-based recommendations for research mentors to consider in promoting an inclusive research experience for students with depression.
INTRODUCTION
Depression is described as a common and serious mood disorder that results in persistent feelings of sadness and hopelessness, as well as a loss of interest in activities that one once enjoyed ( American Psychiatric Association [APA], 2013 ). Additional symptoms of depression include weight changes, difficulty sleeping, loss of energy, difficulty thinking or concentrating, feelings of worthlessness or excessive guilt, and suicidality ( APA, 2013 ). While depression results from a complex interaction of psychological, social, and biological factors ( World Health Organization, 2018 ), studies have shown that increased stress caused by college can be a significant contributor to student depression ( Dyson and Renk, 2006 ).
Depression is one of the top undergraduate mental health concerns, and the rate of depression among undergraduates continues to rise ( Center for Collegiate Mental Health, 2017 ). While we cannot discern whether these increasing rates of depression are due to increased awareness or increased incidence, it is clear that is a serious problem on college campuses. The percent of U.S. college students who self-reported a diagnosis with depression was recently estimated to be about 25% ( American College Health Association, 2019 ). However, higher rates have been reported, with one study estimating that up to 84% of undergraduates experience some level of depression ( Garlow et al. , 2008 ). Depression rates are typically higher among university students compared with the general population, despite being a more socially privileged group ( Ibrahim et al. , 2013 ). Prior studies have found that depression is negatively correlated with overall undergraduate academic performance ( Hysenbegasi et al. , 2005 ; Deroma et al. , 2009 ; American College Health Association, 2019 ). Specifically, diagnosed depression is associated with half a letter grade decrease in students’ grade point average ( Hysenbegasi et al. , 2005 ), and 21.6% of undergraduates reported that depression negatively affected their academic performance within the last year ( American College Health Association, 2019 ). Provided with a list of academic factors that may be affected by depression, students reported that depression contributed to lower exam grades, lower course grades, and not completing or dropping a course.
Students in the natural sciences may be particularly at risk for depression, given that such majors are noted to be particularly stressful due to their competitive nature and course work that is often perceived to “weed students out”( Everson et al. , 1993 ; Strenta et al. , 1994 ; American College Health Association, 2019 ; Seymour and Hunter, 2019 ). Science course instruction has also been described to be boring, repetitive, difficult, and math-intensive; these factors can create an environment that can trigger depression ( Seymour and Hewitt, 1997 ; Osborne and Collins, 2001 ; Armbruster et al ., 2009 ; Ceci and Williams, 2010 ). What also distinguishes science degree programs from other degree programs is that, increasingly, undergraduate research experiences are being proposed as an essential element of a science degree ( American Association for the Advancement of Science, 2011 ; President’s Council of Advisors on Science and Technology, 2012 ; National Academies of Sciences, Engineering, and Medicine [NASEM], 2017 ). However, there is some evidence that undergraduate research experiences can add to the stress of college for some students ( Cooper et al. , 2019c ). Students can garner multiple benefits from undergraduate research, including enhanced abilities to think critically ( Ishiyama, 2002 ; Bauer and Bennett, 2003 ; Brownell et al. , 2015 ), improved student learning ( Rauckhorst et al. , 2001 ; Brownell et al. , 2015 ), and increased student persistence in undergraduate science degree programs ( Jones et al. , 2010 ; Hernandez et al. , 2018 ). Notably, undergraduate research experiences are increasingly becoming a prerequisite for entry into medical and graduate programs in science, particularly elite programs ( Cooper et al. , 2019d ). Although some research experiences are embedded into formal lab courses as course-based undergraduate research experiences (CUREs; Auchincloss et al. , 2014 ; Brownell and Kloser, 2015 ), the majority likely entail working with faculty in their research labs. These undergraduate research experiences in faculty labs are often added on top of a student’s normal course work, so they essentially become an extracurricular activity that they have to juggle with course work, working, and/or personal obligations ( Cooper et al. , 2019c ). While the majority of the literature surrounding undergraduate research highlights undergraduate research as a positive experience ( NASEM, 2017 ), studies have demonstrated that undergraduate research experiences can be academically and emotionally challenging for students ( Mabrouk and Peters, 2000 ; Seymour et al. , 2004 ; Cooper et al. , 2019c ; Limeri et al. , 2019 ). In fact, 50% of students sampled nationally from public R1 institutions consider leaving their undergraduate research experience prematurely, and about half of those students, or 25% of all students, ultimately leave their undergraduate research experience ( Cooper et al. , 2019c ). Notably, 33.8% of these individuals cited a negative lab environment and 33.3% cited negative relationships with their mentors as factors that influenced their decision about whether to leave ( Cooper et al. , 2019c ). Therefore, students’ depression may be exacerbated in challenging undergraduate research experiences, because studies have shown that depression is positively correlated with student stress ( Hish et al. , 2019 ).
While depression has not been explored in the context of undergraduate research experiences, depression has become a prominent concern surrounding graduate students conducting scientific research. A recent study that examined the “graduate student mental health crisis” ( Flaherty, 2018 ) found that work–life balance and graduate students’ relationships with their research advisors may be contributing to their depression ( Evans et al. , 2018 ). Specifically, this survey of 2279 PhD and master’s students from diverse fields of study, including the biological/physical sciences, showed that 39% of graduate students have experienced moderate to severe depression. Fifty-five percent of the graduate students with depression who were surveyed disagreed with the statement “I have good work life balance,” compared to only 21% of students with depression who agreed. Additionally, the study highlighted that more students with depression disagreed than agreed with the following statements: their advisors provided “real” mentorship, their advisors provided ample support, their advisors positively impacted their emotional or mental well-being, their advisors were assets to their careers, and they felt valued by their mentors. Another recent study identified that depression severity in biomedical doctoral students was significantly associated with graduate program climate, a perceived lack of employment opportunities, and the quality of students’ research training environment ( Nagy et al. , 2019 ). Environmental stress, academic stress, and family and monetary stress have also been shown to be predictive of depression severity in biomedical doctoral students ( Hish et al. , 2019 ). Further, one study found that self-esteem is negatively correlated and stress is positively correlated with graduate student depression; presumably research environments that challenge students’ self-esteem and induce stress are likely contributing to depressive symptoms among graduate students ( Kreger, 1995 ). While these studies have focused on graduate students, and there are certainly notable distinctions between graduate and undergraduate research, the research-related factors that affect graduate student depression, including work–life balance, relationships with mentors, research environment, stress, and self-esteem, may also be relevant to depression among undergraduates conducting research. Importantly, undergraduates in the United States have reported identical levels of depression as graduate students but are often less likely to seek mental health care services ( Wyatt and Oswalt, 2013 ), which is concerning if undergraduate research experiences exacerbate depression.
Based on the literature on the stressors of undergraduate research experiences and the literature identifying some potential causes of graduate student depression, we identified three aspects of undergraduate research that may exacerbate undergraduates’ depression. Mentoring: Mentors can be an integral part of a students’ research experience, bolstering their connections with others in the science community, scholarly productivity, and science identity, as well as providing many other benefits ( Thiry and Laursen, 2011 ; Prunuske et al. , 2013 ; Byars-Winston et al. , 2015 ; Aikens et al. , 2016 , 2017 ; Thompson et al. , 2016 ; Estrada et al. , 2018 ). However, recent literature has highlighted that poor mentoring can negatively affect undergraduate researchers ( Cooper et al. , 2019c ; Limeri et al. , 2019 ). Specifically, one study of 33 undergraduate researchers who had conducted research at 10 institutions identified seven major ways that they experienced negative mentoring, which included absenteeism, abuse of power, interpersonal mismatch, lack of career support, lack of psychosocial support, misaligned expectations, and unequal treatment ( Limeri et al. , 2019 ). We hypothesize negative mentoring experiences may be particularly harmful for students with depression, because support, particularly social support, has been shown to be important for helping individuals with depression cope with difficult circumstances ( Aneshensel and Stone, 1982 ; Grav et al. , 2012 ). Failure: Experiencing failure has been hypothesized to be an important aspect of undergraduate research experiences that may help students develop some the most distinguishing abilities of outstanding scientists, such as coping with failure, navigating challenges, and persevering ( Laursen et al. , 2010 ; Gin et al. , 2018 ; Henry et al. , 2019 ). However, experiencing failure and the stress and fatigue that often accompany it may be particularly tough for students with depression ( Aldwin and Greenberger, 1987 ; Mongrain and Blackburn, 2005 ). Lab environment: Fairness, inclusion/exclusion, and social support within one’s organizational environment have been shown to be key factors that cause people to either want to remain in the work place and be productive or to want to leave ( Barak et al. , 2006 ; Cooper et al. , 2019c ). We hypothesize that dealing with exclusion or a lack of social support may exacerbate depression for some students; patients with clinical depression react to social exclusion with more pronounced negative emotions than do individuals without clinical depression ( Jobst et al. , 2015 ). While there are likely other aspects of undergraduate research that affect student depression, we hypothesize that these factors have the potential to exacerbate negative research experiences for students with depression.
Depression has been shown to disproportionately affect many populations that are underrepresented or underserved within the scientific community, including females ( American College Health Association, 2018 ; Evans et al. , 2018 ), first-generation college students ( Jenkins et al. , 2013 ), individuals from low socioeconomic backgrounds ( Eisenberg et al. , 2007 ), members of the LGBTQ+ community ( Eisenberg et al. , 2007 ; Evans et al. , 2018 ), and people with disabilities ( Turner and Noh, 1988 ). Therefore, as the science community strives to be more diverse and inclusive ( Intemann, 2009 ), it is important that we understand more about the relationship between depression and scientific research, because negative experiences with depression in scientific research may be contributing to the underrepresentation of these groups. Specifically, more information is needed about how the research process and environment of research experiences may affect depression.
Given the high rate of depression among undergraduates, the links between depression and graduate research, the potentially challenging environment of undergraduate research, and how depression could disproportionately impact students from underserved communities, it is imperative to begin to explore the relationship between scientific research and depression among undergraduates to create research experiences that could maximize student success. In this exploratory interview study, we aimed to 1) describe how undergraduates’ symptoms of depression affect their research experiences, 2) understand how undergraduate research affects students’ feelings of depression, and 3) identify recommendations based on the literature and undergraduates’ reported experiences to promote a positive research experience for students with depression.
This study was done with an approved Arizona State University Institutional Review Board protocol #7247.
In Fall 2018, we surveyed undergraduate researchers majoring in the life sciences across 25 research-intensive (R1) public institutions across the United States (specific details about the recruitment of the students who completed the survey can be found in Cooper et al. (2019c) ). The survey asked students for their opinions about their undergraduate research experiences and their demographic information and whether they would be interested in participating in a follow-up interview related to their research experiences. For the purpose of this study, we exclusively interviewed students about their undergraduate research experiences in faculty member labs; we did not consider students’ experiences in CUREs. Of the 768 undergraduate researchers who completed the survey, 65% ( n = 496) indicated that they would be interested in participating in a follow-up interview. In Spring 2019, we emailed the 496 students, explaining that we were interested in interviewing students with depression about their experiences in undergraduate research. Our specific prompt was: “If you identify as having depression, we would be interested in hearing about your experience in undergraduate research in a 30–60 minute online interview.” We did not define depression in our email recruitment because we conducted think-aloud interviews with four undergraduates who all correctly interpreted what we meant by depression ( APA, 2013 ). We had 35 students agree to participate in the interview study. The interview participants represented 12 of the 25 R1 public institutions that were represented in the initial survey.
Student Interviews
We developed an interview script to explore our research questions. Specifically, we were interested in how students’ symptoms of depression affect their research experiences, how undergraduate research negatively affects student depression, and how undergraduate research positively affects student depression.
We recognized that mental health, and specifically depression, can be a sensitive topic to discuss with undergraduates, and therefore we tried to minimize any discomfort that the interviewees might experience during the interview. Specifically, we conducted think-aloud interviews with three graduate students who self-identified with having depression at the time of the interview. We asked them to note whether any interview questions made them uncomfortable. We also sought their feedback on questions given their experiences as persons with depression who had once engaged in undergraduate research. We revised the interview protocol after each think-aloud interview. Next, we conducted four additional think-aloud interviews with undergraduates conducting basic science or biology education research who identified with having depression to establish cognitive validity of the questions and to elicit additional feedback about any questions that might make someone uncomfortable. The questions were revised after each think-aloud interview until no question was unclear or misinterpreted by the students and we were confident that the questions minimized students’ potential discomfort ( Trenor et al. , 2011 ). A copy of the final interview script can be found in the Supplemental Material.
All interviews were individually conducted by one of two researchers (K.M.C. and L.E.G.) who conducted the think-aloud interviews together to ensure that their interviewing practices were as similar as possible. The interviews were approximately an hour long, and students received a $15 gift card for their participation.
Personal, Research, and Depression Demographics
All student demographics and information about students’ research experiences were collected using the survey distributed to students in Fall 2018. We collected personal demographics, including the participants’ gender, race/ethnicity, college generation status, transfer status, financial stability, year in college, major, and age. We also collected information about the students’ research experiences, including the length of their first research experiences, the average number of hours they spend in research per week, how they were compensated for research, who their primary mentors were, and the focus areas of their research.
In the United States, mental healthcare is disproportionately unavailable to Black and Latinx individuals, as well as those who come from low socioeconomic backgrounds ( Kataoka et al. , 2002 ; Howell and McFeeters, 2008 ; Santiago et al. , 2013 ). Therefore, to minimize a biased sample, we invited anyone who identified with having depression to participate in our study; we did not require students to be diagnosed with depression or to be treated for depression in order to participate. However, we did collect information about whether students had been formally diagnosed with depression and whether they had been treated for depression. After the interview, all participants were sent a link to a short survey that asked them if they had ever been diagnosed with depression and how, if at all, they had ever been treated for depression. A copy of these survey questions can be found in the Supplemental Material. The combined demographic information of the participants is in Table 1 . The demographics for each individual student can be found in the Supplemental Material.
a Students reported the time they had spent in research 6 months before being interviewed and only reported on the length of time of their first research experiences.
b Students were invited to report multiple ways in which they were treated for their depression; other treatments included lifestyle changes and meditation.
c Students were invited to report multiple means of compensation for their research if they had been compensated for their time in different ways.
d Students were asked whether they felt financially stable, particularly during the undergraduate research experience.
e Students reported who they work/worked with most closely during their research experiences.
f Staff members included lab coordinators or lab managers.
g Other focus areas of research included sociology, linguistics, psychology, and public health.
Interview Analysis
The initial interview analysis aimed to explore each idea that a participant expressed ( Charmaz, 2006 ) and to identify reoccurring ideas throughout the interviews. First, three authors (K.M.C., L.E.G., and S.E.B.) individually reviewed a different set of 10 interviews and took detailed analytic notes ( Birks and Mills, 2015 ). Afterward, the authors compared their notes and identified reoccurring themes throughout the interviews using open coding methods ( Saldaña, 2015 ).
Once an initial set of themes was established, two researchers (K.M.C. and L.E.G.) individually reviewed the same set of 15 randomly selected interviews to validate the themes identified in the initial analysis and to screen for any additional themes that the initial analysis may have missed. Each researcher took detailed analytic notes throughout the review of an interview, which they discussed after reviewing each interview. The researchers compared what quotes from each interview they categorized into each theme. Using constant comparison methods, they assigned quotes to each theme and constantly compared the quotes to ensure that each quote fit within the description of the theme ( Glesne and Peshkin, 1992 ). In cases in which quotes were too different from other quotes, a new theme was created. This approach allowed for multiple revisions of the themes and allowed the authors to define a final set of codes; the researchers created a final codebook with refined definitions of emergent themes (the final coding rubric can be found in the Supplemental Material). Once the final codebook was established, the researchers (K.M.C. and L.E.G.) individually coded seven additional interviews (20% of all interviews) using the coding rubric. The researchers compared their codes, and their Cohen’s κ interrater score for these seven interviews was at an acceptable level (κ = 0.88; Landis and Koch, 1977 ). One researcher (L.E.G.) coded the remaining 28 out of 35 interviews. The researchers determined that data saturation had been reached with the current sample and no further recruitment was needed ( Guest et al. , 2006 ). We report on themes that were mentioned by at least 20% of students in the interview study. In the Supplemental Material, we provide the final coding rubric with the number of participants whose interview reflected each theme ( Hannah and Lautsch, 2011 ). Reporting the number of individuals who reported themes within qualitative data can lead to inaccurate conclusions about the generalizability of the results to a broader population. These qualitative data are meant to characterize a landscape of experiences that students with depression have in undergraduate research rather than to make claims about the prevalence of these experiences ( Glesne and Peshkin, 1992 ). Because inferences about the importance of these themes cannot be drawn from these counts, they are not included in the results of the paper ( Maxwell, 2010 ). Further, the limited number of interviewees made it not possible to examine whether there were trends based on students’ demographics or characteristics of their research experiences (e.g., their specific area of study). Quotes were lightly edited for clarity by inserting clarification brackets and using ellipses to indicate excluded text. Pseudonyms were given to all students to protect their privacy.
The Effect of Depressive Symptoms on Undergraduate Research
We asked students to describe the symptoms associated with their depression. Students described experiencing anxiety that is associated with their depression; this could be anxiety that precedes their depression or anxiety that results from a depressive episode or a period of time when an individual has depression symptoms. Further, students described difficulty getting out of bed or leaving the house, feeling tired, a lack of motivation, being overly self-critical, feeling apathetic, and having difficulty concentrating. We were particularly interested in how students’ symptoms of depression affected their experiences in undergraduate research. During the think-aloud interviews that were conducted before the interview study, graduate and undergraduate students consistently described that their depression affected their motivation in research, their creativity in research, and their productivity in research. Therefore, we explicitly asked undergraduate researchers how, if at all, their depression affected these three factors. We also asked students to describe any additional ways in which their depression affected their research experiences. Undergraduate researchers commonly described five additional ways in which their depression affected their research; for a detailed description of each way students’ research was affected and for example quotes, see Table 2 . Students described that their depression negatively affected their productivity in the lab. Commonly, students described that their productivity was directly affected by a lack of motivation or because they felt less creative, which hindered the research process. Additionally, students highlighted that they were sometimes less productive because their depression sometimes caused them to struggle to engage intellectually with their research or caused them to have difficulty remembering or concentrating; students described that they could do mundane or routine tasks when they felt depressed, but that they had difficulty with more complex and intellectually demanding tasks. However, students sometimes described that even mundane tasks could be difficult when they were required to remember specific steps; for example, some students struggled recalling a protocol from memory when their depression was particularly severe. Additionally, students noted that their depression made them more self-conscious, which sometimes held them back from sharing research ideas with their mentors or from taking risks such as applying to competitive programs. In addition to being self-conscious, students highlighted that their depression caused them to be overly self-critical, and some described experiencing imposter phenomenon ( Clance and Imes, 1978 ) or feeling like they were not talented enough to be in research and were accepted into a lab by a fluke or through luck. Finally, students described that depression often made them feel less social, and they struggled to socially engage with other members of the lab when they were feeling down.
The Effect of Undergraduate Research Experiences on Student Depression
We also wanted to explore how research impacted students’ feelings of depression. Undergraduates described how research both positively and negatively affected their depression. In the following sections, we present aspects of undergraduate research and examine how each positively and/or negatively affected students’ depression using embedded student quotes to highlight the relationships between related ideas.
Lab Environment: Relationships with Others in the Lab.
Some aspects of the lab environment, which we define as students’ physical, social, or psychological research space, could be particularly beneficial for students with depression.
Specifically, undergraduate researchers perceived that comfortable and positive social interactions with others in the lab helped their depression. Students acknowledged how beneficial their relationships with graduate students and postdocs could be.
Marta: “I think always checking in on undergrads is important. It’s really easy [for us] to go a whole day without talking to anybody in the lab. But our grad students are like ‘Hey, what’s up? How’s school? What’s going on?’ (…) What helps me the most is having that strong support system. Sometimes just talking makes you feel better, but also having people that believe in you can really help you get out of that negative spiral. I think that can really help with depression.”
Kelley: “I know that anytime I need to talk to [my postdoc mentors] about something they’re always there for me. Over time we’ve developed a relationship where I know that outside of work and outside of the lab if I did want to talk to them about something I could talk to them. Even just talking to someone about hobbies and having that relationship alone is really helpful [for depression].”
In addition to highlighting the importance of developing relationships with graduate students or postdocs in the lab, students described that forming relationships with other undergraduates in the lab also helped their depression. Particularly, students described that other undergraduate researchers often validated their feelings about research, which in turn helped them realize that what they are thinking or feeling is normal, which tended to alleviate their negative thoughts. Interestingly, other undergraduates experiencing the same issues could sometimes help buffer them from perceiving that a mentor did not like them or that they were uniquely bad at research. In this article, we use the term “mentor” to refer to anyone who students referred to in the interviews as being their mentors or managing their research experiences; this includes graduate students, postdoctoral scholars, lab managers, and primary investigators (PIs).
Abby: “One of my best friends is in the lab with me. A lot of that friendship just comes from complaining about our stress with the lab and our annoyance with people in the lab. Like when we both agree like, ‘Yeah, the grad students were really off today, it wasn’t us,’ that helps. ‘It wasn’t me, it wasn’t my fault that we were having a rough day in lab; it was the grad students.’ Just being able to realize, ‘Hey, this isn’t all caused by us,’ you know? (…) We understand the stresses in the lab. We understand the details of what each other are doing in the lab, so when something doesn’t work out, we understand that it took them like eight hours to do that and it didn’t work. We provide empathy on a different level.”
Meleana: “It’s great to have solidarity in being confused about something, and it’s just that is a form of validation for me too. When we leave a lab meeting and I look at [another undergrad] I’m like, ‘Did you understand anything that they were just saying?’ And they’re like, ‘Oh, no.’ (…) It’s just really validating to hear from the other undergrads that we all seem to be struggling with the same things.”
Developing positive relationships with faculty mentors or PIs also helped alleviate some students’ depressive feelings, particularly when PIs shared their own struggles with students. This also seemed to normalize students’ concerns about their own experiences.
Alexandra: “[Talking with my PI] is helpful because he would talk about his struggles, and what he faced. A lot of it was very similar to my struggles. For example, he would say, ‘Oh, yeah, I failed this exam that I studied so hard for. I failed the GRE and I paid so much money to prepare for it.’ It just makes [my depression] better, like okay, this is normal for students to go through this. It’s not an out of this world thing where if you fail, you’re a failure and you can’t move on from it.”
Students’ relationships with others in the lab did not always positively impact their depression. Students described instances when the negative moods of the graduate students and PIs would often set the tone of the lab, which in turn worsened the mood of the undergraduate researchers.
Abby: “Sometimes [the grad students] are not in a good mood. The entire vibe of the lab is just off, and if you make a joke and it hits somebody wrong, they get all mad. It really depends on the grad students and the leadership and the mood that they’re in.”
Interviewer: “How does it affect your depression when the grad students are in a bad mood?”
Abby: “It definitely makes me feel worse. It feels like, again, that I really shouldn’t go ask them for help because they’re just not in the mood to help out. It makes me have more pressure on myself, and I have deadlines I need to meet, but I have a question for them, but they’re in a bad mood so I can’t ask. That’s another day wasted for me and it just puts more stress, which just adds to the depression.”
Additionally, some students described even more concerning behavior from research mentors, which negatively affected their depression.
Julie: “I had a primary investigator who is notorious in the department for screaming at people, being emotionally abusive, unreasonable, et cetera. (…) [He was] kind of harassing people, demeaning them, lying to them, et cetera, et cetera. (…) Being yelled at and constantly demeaned and harassed at all hours of the day and night, that was probably pretty bad for me.”
While the relationships between undergraduates and graduate, postdoc, and faculty mentors seemed to either alleviate or worsen students’ depressive symptoms, depending on the quality of the relationship, students in this study exclusively described their relationships with other undergraduates as positive for their depression. However, students did note that undergraduate research puts some of the best and brightest undergraduates in the same environment, which can result in students comparing themselves with their peers. Students described that this comparison would often lead them to feel badly about themselves, even though they would describe their personal relationship with a person to be good.
Meleana: “In just the research field in general, just feeling like I don’t really measure up to the people around me [can affect my depression]. A lot of the times it’s the beginning of a little spiral, mental spiral. There are some past undergrads that are talked about as they’re on this pedestal of being the ideal undergrads and that they were just so smart and contributed so much to the lab. I can never stop myself from wondering like, ‘Oh, I wonder if I’m having a contribution to the lab that’s similar or if I’m just another one of the undergrads that does the bare minimum and passes through and is just there.’”
Natasha: “But, on the other hand, [having another undergrad in the lab] also reminded me constantly that some people are invested in this and meant to do this and it’s not me. And that some people know a lot more than I do and will go further in this than I will.”
While students primarily expressed that their relationships with others in the lab affected their depression, some students explained that they struggled most with depression when the lab was empty; they described that they did not like being alone in the lab, because a lack of stimulation allowed their minds to be filled with negative thoughts.
Mia: “Those late nights definitely didn’t help [my depression]. I am alone, in the entire building. I’m left alone to think about my thoughts more, so not distracted by talking to people or interacting with people. I think more about how I’m feeling and the lack of progress I’m making, and the hopelessness I’m feeling. That kind of dragged things on, and I guess deepened my depression.”
Freddy: “Often times when I go to my office in the evening, that is when I would [ sic ] be prone to be more depressed. It’s being alone. I think about myself or mistakes or trying to correct mistakes or whatever’s going on in my life at the time. I become very introspective. I think I’m way too self-evaluating, way too self-deprecating and it’s when I’m alone when those things are really, really triggered. When I’m talking with somebody else, I forget about those things.”
In sum, students with depression highlighted that a lab environment full of positive and encouraging individuals was helpful for their depression, whereas isolating or competitive environments and negative interactions with others often resulted in more depressive feelings.
Doing Science: Experiencing Failure in Research, Getting Help, Receiving Feedback, Time Demands, and Important Contributions.
In addition to the lab environment, students also described that the process of doing science could affect their depression. Specifically, students explained that a large contributor to their depression was experiencing failure in research.
Interviewer: “Considering your experience in undergraduate research, what tends to trigger your feelings of depression?”
Heather: “Probably just not getting things right. Having to do an experiment over and over again. You don’t get the results you want. (…) The work is pretty meticulous and it’s frustrating when I do all this work, I do a whole experiment, and then I don’t get any results that I can use. That can be really frustrating. It adds to the stress. (…) It’s hard because you did all this other stuff before so you can plan for the research, and then something happens and all the stuff you did was worthless basically.”
Julie: “I felt very negatively about myself [when a project failed] and pretty panicked whenever something didn’t work because I felt like it was a direct reflection on my effort and/or intelligence, and then it was a big glaring personal failure.”
Students explained that their depression related to failing in research was exacerbated if they felt as though they could not seek help from their research mentors. Perceived insufficient mentor guidance has been shown to be a factor influencing student intention to leave undergraduate research ( Cooper et al. , 2019c ). Sometimes students talked about their research mentors being unavailable or unapproachable.
Michelle: “It just feels like [the graduate students] are not approachable. I feel like I can’t approach them to ask for their understanding in a certain situation. It makes [my depression] worse because I feel like I’m stuck, and that I’m being limited, and like there’s nothing I can do. So then I kind of feel like it’s my fault that I can’t do anything.”
Other times, students described that they did not seek help in fear that they would be negatively evaluated in research, which is a fear of being judged by others ( Watson and Friend, 1969 ; Weeks et al. , 2005 ; Cooper et al. , 2018 ). That is, students fear that their mentor would think negatively about them or judge them if they were to ask questions that their mentor thought they should know the answer to.
Meleana: “I would say [my depression] tends to come out more in being more reserved in asking questions because I think that comes more like a fear-based thing where I’m like, ‘Oh, I don’t feel like I’m good enough and so I don’t want to ask these questions because then my mentors will, I don’t know, think that I’m dumb or something.’”
Conversely, students described that mentors who were willing to help them alleviated their depressive feelings.
Crystal: “Yeah [my grad student] is always like, ‘Hey, I can check in on things in the lab because you’re allowed to ask me for that, you’re not totally alone in this,’ because he knows that I tend to take on all this responsibility and I don’t always know how to ask for help. He’s like, ‘You know, this is my lab too and I am here to help you as well,’ and just reminds me that I’m not shouldering this burden by myself.”
Ashlyn: “The graduate student who I work with is very kind and has a lot of patience and he really understands a lot of things and provides simple explanations. He does remind me about things and he will keep on me about certain tasks that I need to do in an understanding way, and it’s just because he’s patient and he listens.”
In addition to experiencing failure in science, students described that making mistakes when doing science also negatively affected their depression.
Abby: “I guess not making mistakes on experiments [is important in avoiding my depression]. Not necessarily that your experiment didn’t turn out to produce the data that you wanted, but just adding the wrong enzyme or messing something up like that. It’s like, ‘Oh, man,’ you know? You can get really down on yourself about that because it can be embarrassing.”
Commonly, students described that the potential for making mistakes increased their stress and anxiety regarding research; however, they explained that how other people responded to a potential mistake was what ultimately affected their depression.
Briana: “Sometimes if I made a mistake in correctly identifying an eye color [of a fly], [my PI] would just ridicule me in front of the other students. He corrected me but his method of correcting was very discouraging because it was a ridicule. It made the others laugh and I didn’t like that.”
Julie: “[My PI] explicitly [asked] if I had the dedication for science. A lot of times he said I had terrible judgment. A lot of times he said I couldn’t be trusted. Once I went to a conference with him, and, unfortunately, in front of another professor, he called me a klutz several times and there was another comment about how I never learn from my mistakes.”
When students did do things correctly, they described how important it could be for them to receive praise from their mentors. They explained that hearing praise and validation can be particularly helpful for students with depression, because their thoughts are often very negative and/or because they have low self-esteem.
Crystal: “[Something that helps my depression is] I have text messages from [my graduate student mentor] thanking me [and another undergraduate researcher] for all of the work that we’ve put in, that he would not be able to be as on track to finish as he is if he didn’t have our help.”
Interviewer: “Why is hearing praise from your mentor helpful?”
Crystal: “Because a lot of my depression focuses on everybody secretly hates you, nobody likes you, you’re going to die alone. So having that validation [from my graduate mentor] is important, because it flies in the face of what my depression tells me.”
Brian: “It reminds you that you exist outside of this negative world that you’ve created for yourself, and people don’t see you how you see yourself sometimes.”
Students also highlighted how research could be overwhelming, which negatively affected their depression. Particularly, students described that research demanded a lot of their time and that their mentors did not always seem to be aware that they were juggling school and other commitments in addition to their research. This stress exacerbated their depression.
Rose: “I feel like sometimes [my grad mentors] are not very understanding because grad students don’t take as many classes as [undergrads] do. I think sometimes they don’t understand when I say I can’t come in at all this week because I have finals and they’re like, ‘Why though?’”
Abby: “I just think being more understanding of student life would be great. We have classes as well as the lab, and classes are the priority. They forget what it’s like to be a student. You feel like they don’t understand and they could never understand when you say like, ‘I have three exams this week,’ and they’re like, ‘I don’t care. You need to finish this.’”
Conversely, some students reported that their research labs were very understanding of students’ schedules. Interestingly, these students talked most about how helpful it was to be able to take a mental health day and not do research on days when they felt down or depressed.
Marta: “My lab tech is very open, so she’ll tell us, ‘I can’t come in today. I have to take a mental health day.’ So she’s a really big advocate for that. And I think I won’t personally tell her that I’m taking a mental health day, but I’ll say, ‘I can’t come in today, but I’ll come in Friday and do those extra hours.’ And she’s like, ‘OK great, I’ll see you then.’ And it makes me feel good, because it helps me take care of myself first and then I can take care of everything else I need to do, which is amazing.”
Meleana: “Knowing that [my mentors] would be flexible if I told them that I’m crazy busy and can’t come into work nearly as much this week [helps my depression]. There is flexibility in allowing me to then care for myself.”
Interviewer: “Why is the flexibility helpful given the depression?”
Meleana: “Because sometimes for me things just take a little bit longer when I’m feeling down. I’m just less efficient to be honest, and so it’s helpful if I feel like I can only go into work for 10 hours in a week. It declutters my brain a little bit to not have to worry about all the things I have to do in work in addition the things that I need to do for school or clubs, or family or whatever.”
Despite the demanding nature of research, a subset of students highlighted that their research and research lab provided a sense of stability or familiarity that distracted them from their depression.
Freddy: “I’ll [do research] to run away from those [depressive] feelings or whatever. (…) I find sadly, I hate to admit it, but I do kind of run to [my lab]. I throw myself into work to distract myself from the feelings of depression and sadness.”
Rose: “When you’re sad or when you’re stressed you want to go to things you’re familiar with. So because lab has always been in my life, it’s this thing where it’s going to be there for me I guess. It’s like a good book that you always go back to and it’s familiar and it makes you feel good. So that’s how lab is. It’s not like the greatest thing in the world but it’s something that I’m used to, which is what I feel like a lot of people need when they’re sad and life is not going well.”
Many students also explained that research positively affects their depression because they perceive their research contribution to be important.
Ashlyn: “I feel like I’m dedicating myself to something that’s worthy and something that I believe in. It’s really important because it contextualizes those times when I am feeling depressed. It’s like, no, I do have these better things that I’m working on. Even when I don’t like myself and I don’t like who I am, which is again, depression brain, I can at least say, ‘Well, I have all these other people relying on me in research and in this area and that’s super important.’”
Jessica: “I mean, it just felt like the work that I was doing had meaning and when I feel like what I’m doing is actually going to contribute to the world, that usually really helps with [depression] because it’s like not every day you can feel like you’re doing something impactful.”
In sum, students highlighted that experiencing failure in research and making mistakes negatively contributed to depression, especially when help was unavailable or research mentors had a negative reaction. Additionally, students acknowledged that the research could be time-consuming, but that research mentors who were flexible helped assuage depressive feelings that were associated with feeling overwhelmed. Finally, research helped some students’ depression, because it felt familiar, provided a distraction from depression, and reminded students that they were contributing to a greater cause.
We believe that creating more inclusive research environments for students with depression is an important step toward broadening participation in science, not only to ensure that we are not discouraging students with depression from persisting in science, but also because depression has been shown to disproportionately affect underserved and underrepresented groups in science ( Turner and Noh, 1988 ; Eisenberg et al. , 2007 ; Jenkins et al. , 2013 ; American College Health Association, 2018 ). We initially hypothesized that three features of undergraduate research—research mentors, the lab environment, and failure—may have the potential to exacerbate student depression. We found this to be true; students highlighted that their relationships with their mentors as well as the overall lab environment could negatively affect their depression, but could also positively affect their research experiences. Students also noted that they struggled with failure, which is likely true of most students, but is known to be particularly difficult for students with depression ( Elliott et al. , 1997 ). We expand upon our findings by integrating literature on depression with the information that students provided in the interviews about how research mentors can best support students. We provide a set of evidence-based recommendations focused on mentoring, the lab environment, and failure for research mentors wanting to create more inclusive research environments for students with depression. Notably, only the first recommendation is specific to students with depression; the others reflect recommendations that have previously been described as “best practices” for research mentors ( NASEM, 2017 , 2019 ; Sorkness et al. , 2017 ) and likely would benefit most students. However, we examine how these recommendations may be particularly important for students with depression. As we hypothesized, these recommendations directly address three aspects of research: mentors, lab environment, and failure. A caveat of these recommendations is that more research needs to be done to explore the experiences of students with depression and how these practices actually impact students with depression, but our national sample of undergraduate researchers with depression can provide an initial starting point for a discussion about how to improve research experiences for these students.
Recommendations to Make Undergraduate Research Experiences More Inclusive for Students with Depression
Recognize student depression as a valid illness..
Allow students with depression to take time off of research by simply saying that they are sick and provide appropriate time for students to recover from depressive episodes. Also, make an effort to destigmatize mental health issues.
Undergraduate researchers described both psychological and physical symptoms that manifested as a result of their depression and highlighted how such symptoms prevented them from performing to their full potential in undergraduate research. For example, students described how their depression would cause them to feel unmotivated, which would often negatively affect their research productivity. In cases in which students were motivated enough to come in and do their research, they described having difficulty concentrating or engaging in the work. Further, when doing research, students felt less creative and less willing to take risks, which may alter the quality of their work. Students also sometimes struggled to socialize in the lab. They described feeling less social and feeling overly self-critical. In sum, students described that, when they experienced a depressive episode, they were not able to perform to the best of their ability, and it sometimes took a toll on them to try to act like nothing was wrong, when they were internally struggling with depression. We recommend that research mentors treat depression like any other physical illness; allowing students the chance to recover when they are experiencing a depressive episode can be extremely important to students and can allow them to maximize their productivity upon returning to research ( Judd et al. , 2000 ). Students explained that if they are not able to take the time to focus on recovering during a depressive episode, then they typically continue to struggle with depression, which negatively affects their research. This sentiment is echoed by researchers in psychiatry who have found that patients who do not fully recover from a depressive episode are more likely to relapse and to experience chronic depression ( Judd et al. , 2000 ). Students described not doing tasks or not showing up to research because of their depression but struggling with how to share that information with their research mentors. Often, students would not say anything, which caused them anxiety because they were worried about what others in the lab would say to them when they returned. Admittedly, many students understood why this behavior would cause their research mentors to be angry or frustrated, but they weighed the consequences of their research mentors’ displeasure against the consequences of revealing their depression and decided it was not worth admitting to being depressed. This aligns with literature that suggests that when individuals have concealable stigmatized identities, or identities that can be hidden and that carry negative stereotypes, such as depression, they will often keep them concealed to avoid negative judgment or criticism ( Link and Phelan, 2001 ; Quinn and Earnshaw, 2011 ; Jones and King, 2014 ; Cooper and Brownell, 2016 ; Cooper et al. , 2019b ; Cooper et al ., unpublished data ). Therefore, it is important for research mentors to be explicit with students that 1) they recognize mental illness as a valid sickness and 2) that students with mental illness can simply explain that they are sick if they need to take time off. This may be useful to overtly state on a research website or in a research syllabus, contract, or agreement if mentors use such documents when mentoring undergraduates in their lab. Further, research mentors can purposefully work to destigmatize mental health issues by explicitly stating that struggling with mental health issues, such as depression and anxiety, is common. While we do not recommend that mentors ask students directly about depression, because this can force students to share when they are not comfortable sharing, we do recommend providing opportunities for students to reveal their depression ( Chaudoir and Fisher, 2010 ). Mentors can regularly check in with students about how they’re doing, and talk openly about the importance of mental health, which may increase the chance that students may feel comfortable revealing their depression ( Chaudoir and Quinn, 2010 ; Cooper et al ., unpublished data ).
Foster a Positive Lab Environment.
Encourage positivity in the research lab, promote working in shared spaces to enhance social support among lab members, and alleviate competition among undergraduates.
Students in this study highlighted that the “leadership” of the lab, meaning graduate students, postdocs, lab managers, and PIs, were often responsible for establishing the tone of the lab; that is, if they were in a bad mood it would trickle down and negatively affect the moods of the undergraduates. Explicitly reminding lab leadership that their moods can both positively and negatively affect undergraduates may be important in establishing a positive lab environment. Further, students highlighted how they were most likely to experience negative thoughts when they were alone in the lab. Therefore, it may be helpful to encourage all lab members to work in a shared space to enhance social interactions among students and to maximize the likelihood that undergraduates have access to help when needed. A review of 51 studies in psychiatry supported our undergraduate researchers’ perceptions that social relationships positively impacted their depression; the study found that perceived emotional support (e.g., someone available to listen or give advice), perceived instrumental support (e.g., someone available to help with tasks), and large diverse social networks (e.g., being socially connected to a large number of people) were significantly protective against depression ( Santini et al. , 2015 ). Additionally, despite forming positive relationships with other undergraduates in the lab, many undergraduate researchers admitted to constantly comparing themselves with other undergraduates, which led them to feel inferior, negatively affecting their depression. Some students talked about mentors favoring current undergraduates or talking positively about past undergraduates, which further exacerbated their feelings of inferiority. A recent study of students in undergraduate research experiences highlighted that inequitable distribution of praise to undergraduates can create negative perceptions of lab environments for students (Cooper et al. , 2019). Further, the psychology literature has demonstrated that when people feel insecure in their social environments, it can cause them to focus on a hierarchical view of themselves and others, which can foster feelings of inferiority and increase their vulnerability to depression ( Gilbert et al. , 2009 ). Thus, we recommend that mentors be conscious of their behaviors so that they do not unintentionally promote competition among undergraduates or express favoritism toward current or past undergraduates. Praise is likely best used without comparison with others and not done in a public way, although more research on the impact of praise on undergraduate researchers needs to be done. While significant research has been done on mentoring and mentoring relationships in the context of undergraduate research ( Byars-Winston et al. , 2015 ; Aikens et al. , 2017 ; Estrada et al. , 2018 ; Limeri et al. , 2019 ; NASEM, 2019 ), much less has been done on the influence of the lab environment broadly and how people in nonmentoring roles can influence one another. Yet, this study indicates the potential influence of many different members of the lab, not only their mentors, on students with depression.
Develop More Personal Relationships with Undergraduate Researchers and Provide Sufficient Guidance.
Make an effort to establish more personal relationships with undergraduates and ensure that they perceive that they have access to sufficient help and guidance with regard to their research.
When we asked students explicitly how research mentors could help create more inclusive environments for undergraduate researchers with depression, students overwhelmingly said that building mentor–student relationships would be extremely helpful. Students suggested that mentors could get to know students on a more personal level by asking about their career interests or interests outside of academia. Students also remarked that establishing a more personal relationship could help build the trust needed in order for undergraduates to confide in their research mentors about their depression, which they perceived would strengthen their relationships further because they could be honest about when they were not feeling well or their mentors might even “check in” with them in times where they were acting differently than normal. This aligns with studies showing that undergraduates are most likely to reveal a stigmatized identity, such as depression, when they form a close relationship with someone ( Chaudoir and Quinn, 2010 ). Many were intimidated to ask for research-related help from their mentors and expressed that they wished they had established a better relationship so that they would feel more comfortable. Therefore, we recommend that research mentors try to establish relationships with their undergraduates and explicitly invite them to ask questions or seek help when needed. These recommendations are supported by national recommendations for mentoring ( NASEM, 2019 ) and by literature that demonstrates that both social support (listening and talking with students) and instrumental support (providing students with help) have been shown to be protective against depression ( Santini et al. , 2015 ).
Treat Undergraduates with Respect and Remember to Praise Them.
Avoid providing harsh criticism and remember to praise undergraduates. Students with depression often have low self-esteem and are especially self-critical. Therefore, praise can help calibrate their overly negative self-perceptions.
Students in this study described that receiving criticism from others, especially harsh criticism, was particularly difficult for them given their depression. Multiple studies have demonstrated that people with depression can have an abnormal or maladaptive response to negative feedback; scientists hypothesize that perceived failure on a particular task can trigger failure-related thoughts that interfere with subsequent performance ( Eshel and Roiser, 2010 ). Thus, it is important for research mentors to remember to make sure to avoid unnecessarily harsh criticisms that make students feel like they have failed (more about failure is described in the next recommendation). Further, students with depression often have low self-esteem or low “personal judgment of the worthiness that is expressed in the attitudes the individual holds towards oneself” ( Heatherton et al. , 2003 , p. 220; Sowislo and Orth, 2013 ). Specifically, a meta-analysis of longitudinal studies found that low self-esteem is predictive of depression ( Sowislo and Orth, 2013 ), and depression has also been shown to be highly related to self-criticism ( Luyten et al. , 2007 ). Indeed, nearly all of the students in our study described thinking that they are “not good enough,” “worthless,” or “inadequate,” which is consistent with literature showing that people with depression are self-critical ( Blatt et al. , 1982 ; Gilbert et al. , 2006 ) and can be less optimistic of their performance on future tasks and rate their overall performance on tasks less favorably than their peers without depression ( Cane and Gotlib, 1985 ). When we asked students what aspects of undergraduate research helped their depression, students described that praise from their mentors was especially impactful, because they thought so poorly of themselves and they needed to hear something positive from someone else in order to believe it could be true. Praise has been highlighted as an important aspect of mentoring in research for many years ( Ashford, 1996 ; Gelso and Lent, 2000 ; Brown et al. , 2009 ) and may be particularly important for students with depression. In fact, praise has been shown to enhance individuals’ motivation and subsequent productivity ( Hancock, 2002 ; Henderlong and Lepper, 2002 ), factors highlighted by students as negatively affecting their depression. However, something to keep in mind is that a student with depression and a student without depression may process praise differently. For a student with depression, a small comment that praises the student’s work may not be sufficient for the student to process that comment as praise. People with depression are hyposensitive to reward or have reward-processing deficits ( Eshel and Roiser, 2010 ); therefore, praise may affect students without depression more positively than it would affect students with depression. Research mentors should be mindful that students with depression often have a negative view of themselves, and while students report that praise is extremely important, they may have trouble processing such positive feedback.
Normalize Failure and Be Explicit about the Importance of Research Contributions.
Explicitly remind students that experiencing failure is expected in research. Also explain to students how their individual work relates to the overall project so that they can understand how their contributions are important. It can also be helpful to explain to students why the research project as a whole is important in the context of the greater scientific community.
Experiencing failure has been thought to be a potentially important aspect of undergraduate research, because it may provide students with the potential to develop integral scientific skills such as the ability to navigate challenges and persevere ( Laursen et al. , 2010 ; Gin et al. , 2018 ; Henry et al. , 2019 ). However, in the interviews, students described that when their science experiments failed, it was particularly tough for their depression. Students’ negative reaction to experiencing failure in research is unsurprising, given recent literature that has predicted that students may be inadequately prepared to approach failure in science ( Henry et al. , 2019 ). However, the literature suggests that students with depression may find experiencing failure in research to be especially difficult ( Elliott et al. , 1997 ; Mongrain and Blackburn, 2005 ; Jones et al. , 2009 ). One potential hypothesis is that students with depression may be more likely to have fixed mindsets or more likely to believe that their intelligence and capacity for specific abilities are unchangeable traits ( Schleider and Weisz, 2018 ); students with a fixed mindset have been hypothesized to have particularly negative responses to experiencing failure in research, because they are prone to quitting easily in the face of challenges and becoming defensive when criticized ( Forsythe and Johnson, 2017 ; Dweck, 2008 ). A study of life sciences undergraduates enrolled in CUREs identified three strategies of students who adopted adaptive coping mechanisms, or mechanisms that help an individual maintain well-being and/or move beyond the stressor when faced with failure in undergraduate research: 1) problem solving or engaging in strategic planning and decision making, 2) support seeking or finding comfort and help with research, and 3) cognitive restructuring or reframing a problem from negative to positive and engaging in self encouragement ( Gin et al. , 2018 ). We recommend that, when undergraduates experience failure in science, their mentors be proactive in helping them problem solve, providing help and support, and encouraging them. Students also explained that mentors sharing their own struggles as undergraduate and graduate students was helpful, because it normalized failure. Sharing personal failures in research has been recommended as an important way to provide students with psychosocial support during research ( NASEM, 2019 ). We also suggest that research mentors take time to explain to students why their tasks in the lab, no matter how small, contribute to the greater research project ( Cooper et al. , 2019a ). Additionally, it is important to make sure that students can explain how the research project as a whole is contributing to the scientific community ( Gin et al. , 2018 ). Students highlighted that contributing to something important was really helpful for their depression, which is unsurprising, given that studies have shown that meaning in life or people’s comprehension of their life experiences along with a sense of overarching purpose one is working toward has been shown to be inversely related to depression ( Steger, 2013 ).
Limitations and Future Directions
This work was a qualitative interview study intended to document a previously unstudied phenomenon: depression in the context of undergraduate research experiences. We chose to conduct semistructured interviews rather than a survey because of the need for initial exploration of this area, given the paucity of prior research. A strength of this study is the sampling approach. We recruited a national sample of 35 undergraduates engaged in undergraduate research at 12 different public R1 institutions. Despite our representative sample from R1 institutions, these findings may not be generalizable to students at other types of institutions; lab environments, mentoring structures, and interactions between faculty and undergraduate researchers may be different at other institution types (e.g., private R1 institutions, R2 institutions, master’s-granting institutions, primarily undergraduate institutions, and community colleges), so we caution against making generalizations about this work to all undergraduate research experiences. Future work could assess whether students with depression at other types of institutions have similar experiences to students at research-intensive institutions. Additionally, we intentionally did not explore the experiences of students with specific identities owing to our sample size and the small number of students in any particular group (e.g., students of a particular race, students with a graduate mentor as the primary mentor). We intend to conduct future quantitative studies to further explore how students’ identities and aspects of their research affect their experiences with depression in undergraduate research.
The students who participated in the study volunteered to be interviewed about their depression; therefore, it is possible that depression is a more salient part of these students’ identities and/or that they are more comfortable talking about their depression than the average population of students with depression. It is also important to acknowledge the personal nature of the topic and that some students may not have fully shared their experiences ( Krumpal, 2013 ), particularly those experiences that may be emotional or traumatizing ( Kahn and Garrison, 2009 ). Additionally, our sample was skewed toward females (77%). While females do make up approximately 60% of students in biology programs on average ( Eddy et al. , 2014 ), they are also more likely to report experiencing depression ( American College Health Association, 2018 ; Evans et al. , 2018 ). However, this could be because women have higher rates of depression or because males are less likely to report having depression; clinical bias, or practitioners’ subconscious tendencies to overlook male distress, may underestimate depression rates in men ( Smith et al. , 2018 ). Further, females are also more likely to volunteer to participate in studies ( Porter and Whitcomb, 2005 ); therefore, many interview studies have disproportionately more females in the data set (e.g., Cooper et al. , 2017 ). If we had been able to interview more male students, we might have identified different findings. Additionally, we limited our sample to life sciences students engaged in undergraduate research at public R1 institutions. It is possible that students in other majors may have different challenges and opportunities for students with depression, as well as different disciplinary stigmas associated with mental health.
In this exploratory interview study, we identified a variety of ways in which depression in undergraduates negatively affected their undergraduate research experiences. Specifically, we found that depression interfered with students’ motivation and productivity, creativity and risk-taking, engagement and concentration, and self-perception and socializing. We also identified that research can negatively affect depression in undergraduates. Experiencing failure in research can exacerbate student depression, especially when students do not have access to adequate guidance. Additionally, being alone or having negative interactions with others in the lab worsened students’ depression. However, we also found that undergraduate research can positively affect students’ depression. Research can provide a familiar space where students can feel as though they are contributing to something meaningful. Additionally, students reported that having access to adequate guidance and a social support network within the research lab also positively affected their depression. We hope that this work can spark conversations about how to make undergraduate research experiences more inclusive of students with depression and that it can stimulate additional research that more broadly explores the experiences of undergraduate researchers with depression.
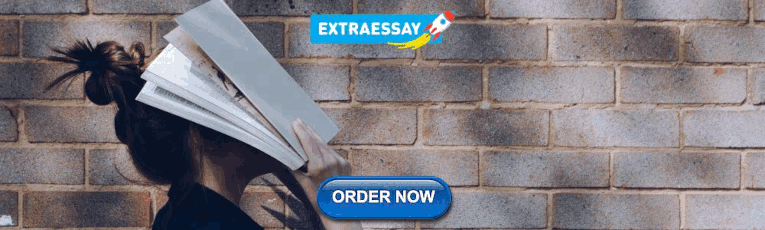
Important note
If you or a student experience symptoms of depression and want help, there are resources available to you. Many campuses provide counseling centers equipped to provide students, staff, and faculty with treatment for depression, as well as university-dedicated crisis hotlines. Additionally, there are free 24/7 services such as Crisis Text Line, which allows you to text a trained live crisis counselor (Text “CONNECT” to 741741; Text Depression Hotline , 2019 ), and phone hotlines such as the National Suicide Prevention Lifeline at 1-800-273-8255 (TALK). You can also learn more about depression and where to find help near you through the Anxiety and Depression Association of American website: https://adaa.org ( Anxiety and Depression Association of America, 2019 ) and the Depression and Biopolar Support Alliance: http://dbsalliance.org ( Depression and Biopolar Support Alliance, 2019 ).
ACKNOWLEDGMENTS
We are extremely grateful to the undergraduate researchers who shared their thoughts and experiences about depression with us. We acknowledge the ASU LEAP Scholars for helping us create the original survey and Rachel Scott for her helpful feedback on earlier drafts of this article. L.E.G. was supported by a National Science Foundation (NSF) Graduate Fellowship (DGE-1311230) and K.M.C. was partially supported by a Howard Hughes Medical Institute (HHMI) Inclusive Excellence grant (no. 11046) and an NSF grant (no. 1644236). Any opinions, findings, conclusions, or recommendations expressed in this material are those of the authors and do not necessarily reflect the views of the NSF or HHMI.
- Aikens, M. L., Robertson, M. M., Sadselia, S., Watkins, K., Evans, M., Runyon, C. R. , … & Dolan, E. L. ( 2017 ). Race and gender differences in undergraduate research mentoring structures and research outcomes . CBE—Life Sciences Education , 16 (2), ar34. Link , Google Scholar
- Aikens, M. L., Sadselia, S., Watkins, K., Evans, M., Eby, L. T., & Dolan, E. L. ( 2016 ). A social capital perspective on the mentoring of undergraduate life science researchers: An empirical study of undergraduate–postgraduate–faculty triads . CBE—Life Sciences Education , 15 (2), ar16. Link , Google Scholar
- Aldwin, C., & Greenberger, E. ( 1987 ). Cultural differences in the predictors of depression . American Journal of Community Psychology , 15 (6), 789–813. Medline , Google Scholar
- American Association for the Advancement of Science . ( 2011 ). Vision and change in undergraduate biology education: A call to action . Retrieved November 29, 2019, from http://visionandchange.org/files/2013/11/aaas-VISchange-web1113.pdf Google Scholar
- American College Health Association . ( 2018 ). Undergraduate reference group executive summary, Fall 2018 . Retrieved November 29, 2019, from www.acha.org/documents/ncha/NCHA-II_Fall_2018_Reference_Group_Executive_Summary.pdf Google Scholar
- American College Health Association . ( 2019 ). Retrieved November 29, 2019, from NCHA-II_SPRING_2019_UNDERGRADUATE_REFERENCE_GROUP_DATA_REPORT.pdf www.acha.org/documents/ncha/NCHA-II_SPRING_2019_UNDERGRADUATE_REFERENCE_GROUP_DATA_REPORT.pdf Google Scholar
- American Psychiatric Association . ( 2013 ). Diagnostic and statistical manual of mental disorders (5th ed.). Washington, DC: American Psychiatric Publishing. Google Scholar
- Aneshensel, C. S., & Stone, J. D. ( 1982 ). Stress and depression: A test of the buffering model of social support . Archives of General Psychiatry , 39 (12), 1392–1396. Medline , Google Scholar
- Anxiety and Depression Association of America . ( 2019 ). Home page . Retrieved November 29, 2019, from https://adaa.org Google Scholar
- Armbruster, P., Patel, M., Johnson, E., & Weiss, M. ( 2009 ). Active learning and student-centered pedagogy improve student attitudes and performance in introductory biology . CBE—Life Sciences Education , 8 (3), 203–213. Link , Google Scholar
- Ashford, S. J. ( 1996 ). Working with doctoral students: Rhythms of Academic Life: Personal Accounts of Careers in Academia . In Front, P. J.Taylor, M. S. (Eds.), Rhythms of Academic Life: Personal Accounts of Careers in Academia (pp. 153–158). Thousand Oaks, CA: Sage. Google Scholar
- Auchincloss, L. C., Laursen, S. L., Branchaw, J. L., Eagan, K., Graham, M., Hanauer, D. I. , … & Rowland, S. ( 2014 ). Assessment of course-based undergraduate research experiences: A meeting report . CBE—Life Sciences Education , 13 (1), 29–40. Link , Google Scholar
- Barak, M. E. M., Levin, A., Nissly, J. A., & Lane, C. J. ( 2006 ). Why do they leave? Modeling child welfare workers’ turnover intentions . Children and Youth Services Review , 28 (5), 548–577. Google Scholar
- Bauer, K. W., & Bennett, J. S. ( 2003 ). Alumni perceptions used to assess undergraduate research experience . Journal of Higher Education , 74 (2), 210–230. Google Scholar
- Birks, M., & Mills, J. ( 2015 ). Grounded theory: A practical guide . Thousand Oaks, CA: Sage. Google Scholar
- Blatt, S. J., Quinlan, D. M., Chevron, E. S., McDonald, C., & Zuroff, D. ( 1982 ). Dependency and self-criticism: Psychological dimensions of depression . Journal of Consulting and Clinical Psychology , 50 (1), 113. Medline , Google Scholar
- Brown, R. T., Daly, B. P., & Leong, F. T. ( 2009 ). Mentoring in research: A developmental approach . Professional Psychology: Research and Practice , 40 (3), 306. Google Scholar
- Brownell, S. E., Hekmat-Scafe, D. S., Singla, V., Seawell, P. C., Imam, J. F. C., Eddy, S. L. , … & Cyert, M. S. ( 2015 ). A high-enrollment course-based undergraduate research experience improves student conceptions of scientific thinking and ability to interpret data . CBE—Life Sciences Education , 14 (2), ar21. Link , Google Scholar
- Brownell, S. E., & Kloser, M. J. ( 2015 ). Toward a conceptual framework for measuring the effectiveness of course-based undergraduate research experiences in undergraduate biology . Studies in Higher Education , 40 (3), 525–544. Google Scholar
- Byars-Winston, A. M., Branchaw, J., Pfund, C., Leverett, P., & Newton, J. ( 2015 ). Culturally diverse undergraduate researchers’ academic outcomes and perceptions of their research mentoring relationships . International Journal of Science Education , 37 (15), 2533–2554. Medline , Google Scholar
- Cane, D. B., & Gotlib, I. H. ( 1985 ). Depression and the effects of positive and negative feedback on expectations, evaluations, and performance . Cognitive Therapy and Research , 9 (2), 145–160. Google Scholar
- Ceci, S. J., & Williams, W. M. ( 2010 ). Sex differences in math-intensive fields . Current Directions in Psychological Science , 19 (5), 275–279. Medline , Google Scholar
- Center for Collegiate Mental Health . ( 2017 ). Center for Collegiate Mental Health 2017 Annual Report . State College, PA: Penn State Universit. Google Scholar
- Charmaz, K. ( 2006 ). Constructing grounded theory: A practical guide through qualitative research . Thousand Oaks, CA: Sage. Google Scholar
- Chaudoir, S. R., & Fisher, J. D. ( 2010 ). The disclosure processes model: Understanding disclosure decision making and postdisclosure outcomes among people living with a concealable stigmatized identity . Psychological Bulletin , 136 (2), 236. Medline , Google Scholar
- Chaudoir, S. R., & Quinn, D. M. ( 2010 ). Revealing concealable stigmatized identities: The impact of disclosure motivations and positive first-disclosure experiences on fear of disclosure and well-being . Journal of Social Issues , 66 (3), 570–584. Medline , Google Scholar
- Clance, P. R., & Imes, S. A. ( 1978 ). The imposter phenomenon in high achieving women: Dynamics and therapeutic intervention . Psychotherapy: Theory, Research & Practice , 15 (3), 241. Google Scholar
- Cooper, K. M., Ashley, M., & Brownell, S. E. ( 2017 ). A bridge to active learning: A summer bridge program helps students maximize their active-learning experiences and the active-learning experiences of others . CBE—Life Sciences Education , 16 (1), ar17. Link , Google Scholar
- Cooper, K. M., Blattman, J. N., Hendrix, T., & Brownell, S. E. ( 2019a ). The impact of broadly relevant novel discoveries on student project ownership in a traditional lab course turned CURE . CBE—Life Sciences Education , 18 (4), ar57. Link , Google Scholar
- Cooper, K. M., & Brownell, S. E. ( 2016 ). Coming out in class: Challenges and benefits of active learning in a biology classroom for LGBTQIA students . CBE—Life Sciences Education , 15 (3), ar37. https://doi.org/10.1187/cbe.16-01-0074 Link , Google Scholar
- Cooper, K. M., Brownell, S. E., & Gormally, C. C. ( 2019b ). Coming out to the class: Identifying factors that influence college biology instructor decisions about whether to reveal their LGBQ identity in class . Journal of Women and Minorities in Science and Engineering , 25 (3). Google Scholar
- Cooper, K. M., Downing, V. R., & Brownell, S. E. ( 2018 ). The influence of active learning practices on student anxiety in large-enrollment college science classrooms . International Journal of STEM Education , 5 (1), 23. Medline , Google Scholar
- Cooper, K. M., Gin, L. E., Akeeh, B., Clark, C. E., Hunter, J. S., Roderick, T. B. , … & Brownell, S. E. ( 2019c ). Factors that predict life sciences student persistence in undergraduate research experiences . PLoS ONE , 14 (8). https://doi.org/10.1371/journal.pone.0220186 Google Scholar
- Cooper, K. M., Gin, L. E., & Brownell, S. E. ( 2019d ). Diagnosing differences in what introductory biology students in a fully online and an in-person biology degree program know and do regarding medical school admission . Advances in Physiology Education , 43 (2), 221–232. Medline , Google Scholar
- Cooper, K. M., Gin, L. E., & Brownell, S. E. ( In press ). Depression as a concealable stigmatized identity: What influences whether students conceal or reveal their depression in undergraduate research experiences? International Journal of STEM Education , ( in press ). Google Scholar
- Depression and Biopolar Support Alliance . ( 2019 ). Home page . Retrieved November 28, 2019, from www.dbsalliance.org Google Scholar
- Deroma, V. M., Leach, J. B., & Leverett, J. P. ( 2009 ). The relationship between depression and college academic performance . College Student Journal , 43 (2), 325–335. Google Scholar
- Dweck, C. S. ( 2008 ). Mindset: The new psychology of success . New York, NY: Random House Digital. Google Scholar
- Dyson, R., & Renk, K. ( 2006 ). Freshmen adaptation to university life: Depressive symptoms, stress, and coping . Journal of Clinical Psychology , 62 (10), 1231–1244. Medline , Google Scholar
- Eddy, S. L., Brownell, S. E., & Wenderoth, M. P. ( 2014 ). Gender gaps in achievement and participation in multiple introductory biology classrooms . CBE—Life Sciences Education , 13 (3), 478–492. https://doi.org/10.1187/cbe.13-10-0204 Link , Google Scholar
- Eisenberg, D., Gollust, S. E., Golberstein, E., & Hefner, J. L. ( 2007 ). Prevalence and correlates of depression, anxiety, and suicidality among university students . American Journal of Orthopsychiatry , 77 (4), 534–542. Medline , Google Scholar
- Elliott, R., Sahakian, B. J., Herrod, J. J., Robbins, T. W., & Paykel, E. S. ( 1997 ). Abnormal response to negative feedback in unipolar depression: Evidence for a diagnosis specific impairment . Journal of Neurology, Neurosurgery & Psychiatry , 63 (1), 74–82. Medline , Google Scholar
- Eshel, N., & Roiser, J. P. ( 2010 ). Reward and punishment processing in depression . Biological Psychiatry , 68 (2), 118–124. Medline , Google Scholar
- Estrada, M., Hernandez, P. R., & Schultz, P. W. ( 2018 ). A longitudinal study of how quality mentorship and research experience integrate underrepresented minorities into STEM careers . CBE—Life Sciences Education , 17 (1), ar9. Link , Google Scholar
- Evans, T. M., Bira, L., Gastelum, J. B., Weiss, L. T., & Vanderford, N. L. ( 2018 ). Evidence for a mental health crisis in graduate education . Nature Biotechnology , 36 (3), 282. Medline , Google Scholar
- Everson, H. T., Tobias, S., Hartman, H., & Gourgey, A. ( 1993 ). Test anxiety and the curriculum: The subject matters . Anxiety, Stress, and Coping , 6 (1), 1–8. Google Scholar
- Flaherty, C. ( 2018 ). New study says graduate students’ mental health is a “crisis.” Retrieved November 29, 2019, from www.insidehighered.com/news/2018/03/06/new-study-says-graduate-students-mental-health-crisis Google Scholar
- Forsythe, A., & Johnson, S. ( 2017 ). Thanks, but no-thanks for the feedback . Assessment & Evaluation in Higher Education , 42 (6), 850–859. Google Scholar
- Garlow, S. J., Rosenberg, J., Moore, J. D., Haas, A. P., Koestner, B., Hendin, H., & Nemeroff, C. B. ( 2008 ). Depression, desperation, and suicidal ideation in college students: Results from the American Foundation for Suicide Prevention College Screening Project at Emory University . Depression and Anxiety , 25 (6), 482–488. Medline , Google Scholar
- Gelso, C. J., & Lent, R. W. ( 2000 ). Scientific training and scholarly productivity: The person, the training environment, and their interaction . In Brown, S. D.Lent, R. W. (Eds.), Handbook of counseling psychology (pp. 109–139). Hoboken, NJ: John Wiley & Sons Inc. Google Scholar
- Gilbert, P., Baldwin, M. W., Irons, C., Baccus, J. R., & Palmer, M. ( 2006 ). Self-criticism and self-warmth: An imagery study exploring their relation to depression . Journal of Cognitive Psychotherapy , 20 (2), 183. Google Scholar
- Gilbert, P., McEwan, K., Bellew, R., Mills, A., & Gale, C. ( 2009 ). The dark side of competition: How competitive behaviour and striving to avoid inferiority are linked to depression, anxiety, stress and self-harm . Psychology and Psychotherapy: Theory, Research and Practice , 82 (2), 123–136. Medline , Google Scholar
- Gin, L. E., Rowland, A. A., Steinwand, B., Bruno, J., & Corwin, L. A. ( 2018 ). Students who fail to achieve predefined research goals may still experience many positive outcomes as a result of CURE participation . CBE—Life Sciences Education , 17 (4), ar57. Link , Google Scholar
- Glesne, C., & Peshkin, A. ( 1992 ). Becoming qualitative researchers: An introduction . London, England, UK: Longman. Google Scholar
- Grav, S., Hellzèn, O., Romild, U., & Stordal, E. ( 2012 ). Association between social support and depression in the general population: The HUNT study, a cross-sectional survey . Journal of Clinical Nursing , 21 (1–2), 111–120. Medline , Google Scholar
- Guest, G., Bunce, A., & Johnson, L. ( 2006 ). How many interviews are enough? An experiment with data saturation and variability . Field Methods , 18 (1), 59–82. Google Scholar
- Hancock, D. R. ( 2002 ). Influencing graduate students’ classroom achievement, homework habits and motivation to learn with verbal praise . Educational Research , 44 (1), 83–95. Google Scholar
- Hannah, D. R., & Lautsch, B. A. ( 2011 ). Counting in qualitative research: Why to conduct it, when to avoid it, and when to closet it . Journal of Management Inquiry , 20 (1), 14–22. Google Scholar
- Heatherton, T. F., & Wyland, C. L. ( 2003 ). Assessing self-esteem . In Lopez, S. J.Snyder, C. R. (Eds.), Positive psychological assessment: A handbook of models and measures (pp. 219–233). Washington, DC: American Psychological Association. https://doi.org/10.1037/10612-014 . Google Scholar
- Henderlong, J., & Lepper, M. R. ( 2002 ). The effects of praise on children’s intrinsic motivation: A review and synthesis . Psychological Bulletin , 128 (5), 774. Medline , Google Scholar
- Henry, M. A., Shorter, S., Charkoudian, L., Heemstra, J. M., & Corwin, L. A. ( 2019 ). FAIL is not a four-letter word: A theoretical framework for exploring undergraduate students’ approaches to academic challenge and responses to failure in STEM learning environments . CBE—Life Sciences Education , 18 (1), ar11. Link , Google Scholar
- Hernandez, P. R., Woodcock, A., Estrada, M., & Schultz, P. W. ( 2018 ). Undergraduate research experiences broaden diversity in the scientific workforce . BioScience , 68 (3), 204–211. Google Scholar
- Hish, A. J., Nagy, G. A., Fang, C. M., Kelley, L., Nicchitta, C. V., Dzirasa, K., & Rosenthal, M. Z. ( 2019 ). Applying the stress process model to stress–burnout and stress–depression relationships in biomedical doctoral students: A cross-sectional pilot study . CBE—Life Sciences Education , 18 (4), ar51. Link , Google Scholar
- Howell, E., & McFeeters, J. ( 2008 ). Children’s mental health care: Differences by race/ethnicity in urban/rural areas . Journal of Health Care for the Poor and Underserved , 19 (1), 237–247. Medline , Google Scholar
- Hysenbegasi, A., Hass, S. L., & Rowland, C. R. ( 2005 ). The impact of depression on the academic productivity of university students . Journal of Mental Health Policy and Economics , 8 (3), 145. Medline , Google Scholar
- Ibrahim, A. K., Kelly, S. J., Adams, C. E., & Glazebrook, C. ( 2013 ). A systematic review of studies of depression prevalence in university students . Journal of Psychiatric Research , 47 (3), 391–400. Medline , Google Scholar
- Intemann, K. ( 2009 ). Why diversity matters: Understanding and applying the diversity component of the National Science Foundation’s broader impacts criterion . Social Epistemology , 23 (3–4), 249–266. Google Scholar
- Ishiyama, J. ( 2002 ). Does early participation in undergraduate research benefit social science and humanities students? College Student Journal , 36 (3), 381–387. Google Scholar
- Jenkins, S. R., Belanger, A., Connally, M. L., Boals, A., & Durón, K. M. ( 2013 ). First-generation undergraduate students’ social support, depression, and life satisfaction . Journal of College Counseling , 16 (2), 129–142. Google Scholar
- Jobst, A., Sabass, L., Palagyi, A., Bauriedl-Schmidt, C., Mauer, M. C., Sarubin, N. , … & Zill, P. ( 2015 ). Effects of social exclusion on emotions and oxytocin and cortisol levels in patients with chronic depression . Journal of Psychiatric Research , 60 , 170–177. Medline , Google Scholar
- Jones, K. P., & King, E. B. ( 2014 ). Managing concealable stigmas at work: A review and multilevel model . Journal of Management , 40 (5), 1466–1494. Google Scholar
- Jones, M. T., Barlow, A. E., & Villarejo, M. ( 2010 ). Importance of undergraduate research for minority persistence and achievement in biology . Journal of Higher Education , 81 (1), 82–115. Google Scholar
- Jones, N. P., Papadakis, A. A., Hogan, C. M., & Strauman, T. J. ( 2009 ). Over and over again: Rumination, reflection, and promotion goal failure and their interactive effects on depressive symptoms . Behaviour Research and Therapy , 47 (3), 254–259. Medline , Google Scholar
- Judd, L. L., Paulus, M. J., Schettler, P. J., Akiskal, H. S., Endicott, J., Leon, A. C. , … & Keller, M. B. ( 2000 ). Does incomplete recovery from first lifetime major depressive episode herald a chronic course of illness? American Journal of Psychiatry , 157 (9), 1501–1504. Medline , Google Scholar
- Kahn, J. H., & Garrison, A. M. ( 2009 ). Emotional self-disclosure and emotional avoidance: Relations with symptoms of depression and anxiety . Journal of Counseling Psychology , 56 (4), 573. Google Scholar
- Kataoka, S. H., Zhang, L., & Wells, K. B. ( 2002 ). Unmet need for mental health care among US children: Variation by ethnicity and insurance status . American Journal of Psychiatry , 159 (9), 1548–1555. Medline , Google Scholar
- Kreger, D. W. ( 1995 ). Self-esteem, stress, and depression among graduate students . Psychological Reports , 76 (1), 345–346. Medline , Google Scholar
- Krumpal, I. ( 2013 ). Determinants of social desirability bias in sensitive surveys: A literature review . Quality & Quantity , 47 (4), 2025–2047. Google Scholar
- Landis, J. R., & Koch, G. G. ( 1977 ). An application of hierarchical kappa-type statistics in the assessment of majority agreement among multiple observers . Biometrics , 33 (2), 363–374. Medline , Google Scholar
- Laursen, S., Hunter, A.-B., Seymour, E., Thiry, H., & Melton, G. ( 2010 ). Undergraduate research in the sciences: Engaging students in real science . Hoboken, NJ: Wiley. Google Scholar
- Limeri, L. B., Asif, M. Z., Bridges, B. H., Esparza, D., Tuma, T. T., Sanders, D. , … & Maltese, A. V. ( 2019 ). “Where’s my mentor?” Characterizing negative mentoring experiences in undergraduate life science research . CBE—Life Sciences Education , 18 (4), ar61. Link , Google Scholar
- Link, B. G., & Phelan, J. C. ( 2001 ). Conceptualizing stigma . Annual Review of Sociology , 27 (1), 363–385. Google Scholar
- Luyten, P., Sabbe, B., Blatt, S. J., Meganck, S., Jansen, B., De Grave, C. , … & Corveleyn, J. ( 2007 ). Dependency and self-criticism: Relationship with major depressive disorder, severity of depression, and clinical presentation . Depression and Anxiety , 24 (8), 586–596. Medline , Google Scholar
- Mabrouk, P. A., & Peters, K. ( 2000 ). Student perspectives on undergraduate research (UR) experiences in chemistry and biology . CUR Quarterly , 21 (1), 25–33. Google Scholar
- Maxwell, J. A. ( 2010 ). Using numbers in qualitative research . Qualitative Inquiry , 16 (6), 475–482. Google Scholar
- Mongrain, M., & Blackburn, S. ( 2005 ). Cognitive vulnerability, lifetime risk, and the recurrence of major depression in graduate students . Cognitive Therapy and Research , 29 (6), 747–768. Google Scholar
- Nagy, G. A., Fang, C. M., Hish, A. J., Kelly, L., Nicchitta, C. V., Dzirasa, K., & Rosenthal, M. Z. ( 2019 ). Burnout and mental health problems in biomedical doctoral students . CBE—Life Sciences Education , 18 (2), ar27. Link , Google Scholar
- National Academies of Sciences, Engineering, and Medicine (NASEM) . ( 2017 ). Undergraduate research experiences for STEM students: Successes, challenges, and opportunities . Washington, DC: National Academies Press. https://doi.org/10.17226/24622 Google Scholar
- NASEM . ( 2019 ). The science of effective mentorship in STEMM . Washington, DC: National Academies Press. Retrieved November 29, 2019, from www.nap.edu/download/25568 Google Scholar
- Osborne, J., & Collins, S. ( 2001 ). Pupils’ views of the role and value of the science curriculum: A focus-group study . International Journal of Science Education , 23 (5), 441–467. https://doi.org/10.1080/09500690010006518 Google Scholar
- Porter, S. R., & Whitcomb, M. E. ( 2005 ). Non-response in student surveys: The role of demographics, engagement and personality . Research in Higher Education , 46 (2), 127–152. Google Scholar
- President’s Council of Advisors on Science and Technology . ( 2012 ). Engage to excel: Producing one million additional college graduates with degrees in science, Technology, Engineering, and mathematics . Washington, DC: U.S. Government Office of Science and Technology. Google Scholar
- Prunuske, A. J., Wilson, J., Walls, M., & Clarke, B. ( 2013 ). Experiences of mentors training underrepresented undergraduates in the research laboratory . CBE—Life Sciences Education , 12 (3), 403–409. Link , Google Scholar
- Quinn, D. M., & Earnshaw, V. A. ( 2011 ). Understanding concealable stigmatized identities: The role of identity in psychological, physical, and behavioral outcomes . Social Issues and Policy Review , 5 (1), 160–190. Google Scholar
- Rauckhorst, W. H., Czaja, J. A., & Baxter Magolda, M. ( 2001 ). Measuring the impact of the undergraduate research experience on student intellectual development . Snowbird, UT: Project Kaleidoscope Summer Institute. Google Scholar
- Saldaña, J. ( 2015 ). The coding manual for qualitative researchers . Thousand Oaks, CA: Sage. Google Scholar
- Santiago, C. D., Kaltman, S., & Miranda, J. ( 2013 ). Poverty and mental health: How do low-income adults and children fare in psychotherapy? Journal of Clinical Psychology , 69 (2), 115–126. Medline , Google Scholar
- Santini, Z. I., Koyanagi, A., Tyrovolas, S., Mason, C., & Haro, J. M. ( 2015 ). The association between social relationships and depression: A systematic review . Journal of Affective Disorders , 175 , 53–65. Medline , Google Scholar
- Schleider, J., & Weisz, J. ( 2018 ). A single-session growth mindset intervention for adolescent anxiety and depression: 9-month outcomes of a randomized trial . Journal of Child Psychology and Psychiatry , 59 (2), 160–170. Medline , Google Scholar
- Seymour, E., & Hewitt, N. M. ( 1997 ). Talking about leaving: Why undergraduates leave the sciences . Westview Press. Google Scholar
- Seymour, E., & Hunter, A.-B. ( 2019 ). Talking about leaving revisited . New York, NY: Springer. Google Scholar
- Seymour, E., Hunter, A.-B., Laursen, S. L., & DeAntoni, T. ( 2004 ). Establishing the benefits of research experiences for undergraduates in the sciences: First findings from a three-year study . Science Education , 88 (4), 493–534. Google Scholar
- Smith, D. T., Mouzon, D. M., & Elliott, M. ( 2018 ). Reviewing the assumptions about men’s mental health: An exploration of the gender binary . American Journal of Men’s Health , 12 (1), 78–89. Medline , Google Scholar
- Sorkness, C. A., Pfund, C., Ofili, E. O., Okuyemi, K. S., Vishwanatha, J. K., Zavala, M. E. , … & Deveci, A. ( 2017 ). A new approach to mentoring for research careers: The National Research Mentoring Network . BMC Proceedings , 11 , 22. Medline , Google Scholar
- Sowislo, J. F., & Orth, U. ( 2013 ). Does low self-esteem predict depression and anxiety? A meta-analysis of longitudinal studies . Psychological Bulletin , 139 (1), 213. Medline , Google Scholar
- Steger, M. F. ( 2013 ). Experiencing meaning in life: Optimal functioning at the nexus of well-being, psychopathology, and spirituality . In Wong, P. T. P. (Ed.), The human quest for meaning (pp. 211–230). England, UK: Routledge. Google Scholar
- Strenta, A. C., Elliott, R., Adair, R., Matier, M., & Scott, J. ( 1994 ). Choosing and leaving science in highly selective institutions . Research in Higher Education , 35 (5), 513–547. Google Scholar
- Text Depression Hotline . ( 2019 ). Crisis text line . Retrieved November 29, 2019, from www.crisistextline.org/depression Google Scholar
- Thiry, H., & Laursen, S. L. ( 2011 ). The role of student–advisor interactions in apprenticing undergraduate researchers into a scientific community of practice . Journal of Science Education and Technology , 20 (6), 771–784. Google Scholar
- Thompson, J. J., Conaway, E., & Dolan, E. L. ( 2016 ). Undergraduate students’ development of social, cultural, and human capital in a networked research experience . Cultural Studies of Science Education , 11 (4), 959–990. Google Scholar
- Trenor, J. M., Miller, M. K., & Gipson, K. G. ( 2011 ). Utilization of a think-aloud protocol to cognitively validate a survey instrument identifying social capital resources of engineering undergraduates . 118th American Society for Engineering Education Annual Conference and Exposition, Vancouver, BC, Canada . Google Scholar
- Turner, R. J., & Noh, S. ( 1988 ). Physical disability and depression: A longitudinal analysis . Journal of Health and Social Behavior , 29 (1), 23–37. Medline , Google Scholar
- Watson, D., & Friend, R. ( 1969 ). Measurement of social-evaluative anxiety . Journal of Consulting and Clinical Psychology , 33 (4), 448. Medline , Google Scholar
- Weeks, J. W., Heimberg, R. G., Fresco, D. M., Hart, T. A., Turk, C. L., Schneier, F. R., & Liebowitz, M. R. ( 2005 ). Empirical validation and psychometric evaluation of the Brief Fear of Negative Evaluation Scale in patients with social anxiety disorder . Psychological Assessment , 17 (2), 179. Medline , Google Scholar
- World Health Organization . ( 2018 ). Depression . Retrieved November 29, 2019, from www.who.int/news-room/fact-sheets/detail/depression Google Scholar
- Wyatt, T., & Oswalt, S. B. ( 2013 ). Comparing mental health issues among undergraduate and graduate students . American Journal of Health Education , 44 (2), 96–107. Google Scholar
- Carly A. Busch ,
- Tala Araghi ,
- Jingyi He ,
- Katelyn M. Cooper , and
- Colin Harrison, Monitoring Editor
- Emma C. Goodwin ,
- Danielle Pais ,
- Logan E. Gin , and
- Derek Braun, Monitoring Editor
- Baylee A. Edwards ,
- Chloe Bowen ,
- M. Elizabeth Barnes , and
- Tati Russo-Tait, Monitoring Editor
- Sara E. Grineski ,
- Danielle X. Morales , and
- Timothy W. Collins
- Carly A. Busch , and
- Tasneem F. Mohammed ,
- Erika M. Nadile ,
- Madison L. Witt ,
- Cindy Vargas ,
- Missy Tran ,
- Joseph Gazing Wolf ,
- Danielle Brister , and
- Sehoya Cotner, Monitoring Editor
- Katelyn M. Cooper ,
- Sarah L. Eddy , and
- Coping behavior versus coping style: characterizing a measure of coping in undergraduate STEM contexts 14 February 2022 | International Journal of STEM Education, Vol. 9, No. 1
- Lisa A. Corwin ,
- Michael E. Ramsey ,
- Eric A. Vance ,
- Elizabeth Woolner ,
- Stevie Maiden ,
- Nina Gustafson and
- Joseph A. Harsh
- Erin Shortlidge, Monitoring Editor
- K. Supriya ,
- Brian Sato, Monitoring Editor
- Logan E. Gin ,
- Clark Coffman, Monitoring Editor
- Nicholas J. Wiesenthal , and
- Maryrose Weatherton and
- Elisabeth E. Schussler
- Erika Offerdahl, Monitoring Editor
- Eight Recommendations to Promote Effective Study Habits for Biology Students Enrolled in Online Courses Journal of Microbiology & Biology Education, Vol. 23, No. 1
- Fostering professional development through undergraduate research: supporting faculty mentors and student researchers 30 March 2022 | Mentoring & Tutoring: Partnership in Learning, Vol. 30, No. 2
- Jeffrey Maloy ,
- Monika B. Kwapisz , and
- Bryce E. Hughes
- Terrell Morton, Monitoring Editor
- Anxiety and depression among US college students engaging in undergraduate research during the COVID-19 pandemic 14 December 2021 | Journal of American College Health, Vol. 9
- Danielle Brister ,
- Sara E. Brownell ,
- Chade T. Claiborne ,
- Curtis Lunt ,
- Kobe M. Walker ,
- Tamiru D. Warkina ,
- Yi Zheng , and
- Rebecca Price, Monitoring Editor
- Dominant Learning Styles of Interior Design Students in Generation Z 26 July 2021 | Journal of Interior Design, Vol. 46, No. 4
- Linking Emotional Intelligence, Physical Activity and Aggression among Undergraduates 26 November 2021 | International Journal of Environmental Research and Public Health, Vol. 18, No. 23
- Advancing undergraduate synthetic biology education: insights from a Canadian iGEM student perspective Canadian Journal of Microbiology, Vol. 67, No. 10
- Frank A. Guerrero ,
- Sara E. Brownell , and
- Jennifer Momsen, Monitoring Editor
- Nicholas J. Wiesenthal ,
- Isabella Ferreira , and
- Grant Ean Gardner, Monitoring Editor
- Carolyn E. Clark ,
- Deanna B. Elliott ,
- Travis B. Roderick ,
- Rachel A. Scott ,
- Denisse Arellano ,
- Diana Ramirez ,
- Kimberly Velarde ,
- Allyson Aeschliman ,
- Sarah T. Avalle ,
- Jessica Berkheimer ,
- Rachel Campos ,
- Michael Gerbasi ,
- Sophia Hughes ,
- Julie A. Roberts ,
- Quinn M. White ,
- Ehren Wittekind ,
- Rachelle Spell, Monitoring Editor
- Christine Pfund ,
- Janet L. Branchaw ,
- Melissa McDaniels ,
- Angela Byars-Winston ,
- Steven P. Lee ,, and
- Bruce Birren
- Vladimir Anokhin ,
- MacKenzie J. Gray ,
- Daniel E. Zajic ,
- Jason E. Podrabsky , and
- Erin E. Shortlidge
- Depression as a concealable stigmatized identity: what influences whether students conceal or reveal their depression in undergraduate research experiences? 4 June 2020 | International Journal of STEM Education, Vol. 7, No. 1
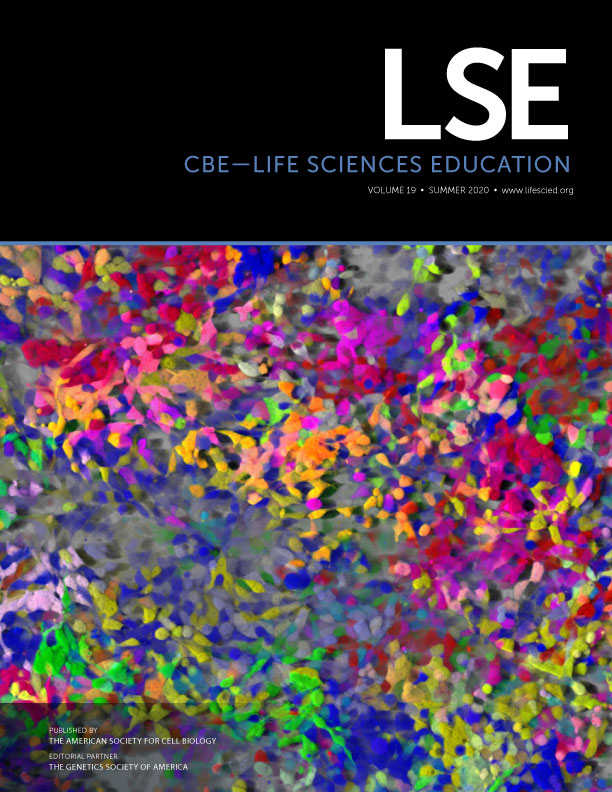
Submitted: 4 November 2019 Revised: 24 February 2020 Accepted: 6 March 2020
© 2020 K. M. Cooper, L. E. Gin, et al. CBE—Life Sciences Education © 2020 The American Society for Cell Biology. This article is distributed by The American Society for Cell Biology under license from the author(s). It is available to the public under an Attribution–Noncommercial–Share Alike 3.0 Unported Creative Commons License (http://creativecommons.org/licenses/by-nc-sa/3.0).
- Bipolar Disorder
- Therapy Center
- When To See a Therapist
- Types of Therapy
- Best Online Therapy
- Best Couples Therapy
- Best Family Therapy
- Managing Stress
- Sleep and Dreaming
- Understanding Emotions
- Self-Improvement
- Healthy Relationships
- Student Resources
- Personality Types
- Guided Meditations
- Verywell Mind Insights
- 2024 Verywell Mind 25
- Mental Health in the Classroom
- Editorial Process
- Meet Our Review Board
- Crisis Support
7 Depression Research Paper Topic Ideas
Nancy Schimelpfening, MS is the administrator for the non-profit depression support group Depression Sanctuary. Nancy has a lifetime of experience with depression, experiencing firsthand how devastating this illness can be.
Cara Lustik is a fact-checker and copywriter.
:max_bytes(150000):strip_icc():format(webp)/Cara-Lustik-1000-77abe13cf6c14a34a58c2a0ffb7297da.jpg)
In psychology classes, it's common for students to write a depression research paper. Researching depression may be beneficial if you have a personal interest in this topic and want to learn more, or if you're simply passionate about this mental health issue. However, since depression is a very complex subject, it offers many possible topics to focus on, which may leave you wondering where to begin.
If this is how you feel, here are a few research titles about depression to help inspire your topic choice. You can use these suggestions as actual research titles about depression, or you can use them to lead you to other more in-depth topics that you can look into further for your depression research paper.
What Is Depression?
Everyone experiences times when they feel a little bit blue or sad. This is a normal part of being human. Depression, however, is a medical condition that is quite different from everyday moodiness.
Your depression research paper may explore the basics, or it might delve deeper into the definition of clinical depression or the difference between clinical depression and sadness .
What Research Says About the Psychology of Depression
Studies suggest that there are biological, psychological, and social aspects to depression, giving you many different areas to consider for your research title about depression.
Types of Depression
There are several different types of depression that are dependent on how an individual's depression symptoms manifest themselves. Depression symptoms may vary in severity or in what is causing them. For instance, major depressive disorder (MDD) may have no identifiable cause, while postpartum depression is typically linked to pregnancy and childbirth.
Depressive symptoms may also be part of an illness called bipolar disorder. This includes fluctuations between depressive episodes and a state of extreme elation called mania. Bipolar disorder is a topic that offers many research opportunities, from its definition and its causes to associated risks, symptoms, and treatment.
Causes of Depression
The possible causes of depression are many and not yet well understood. However, it most likely results from an interplay of genetic vulnerability and environmental factors. Your depression research paper could explore one or more of these causes and reference the latest research on the topic.
For instance, how does an imbalance in brain chemistry or poor nutrition relate to depression? Is there a relationship between the stressful, busier lives of today's society and the rise of depression? How can grief or a major medical condition lead to overwhelming sadness and depression?
Who Is at Risk for Depression?
This is a good research question about depression as certain risk factors may make a person more prone to developing this mental health condition, such as a family history of depression, adverse childhood experiences, stress , illness, and gender . This is not a complete list of all risk factors, however, it's a good place to start.
The growing rate of depression in children, teenagers, and young adults is an interesting subtopic you can focus on as well. Whether you dive into the reasons behind the increase in rates of depression or discuss the treatment options that are safe for young people, there is a lot of research available in this area and many unanswered questions to consider.
Depression Signs and Symptoms
The signs of depression are those outward manifestations of the illness that a doctor can observe when they examine a patient. For example, a lack of emotional responsiveness is a visible sign. On the other hand, symptoms are subjective things about the illness that only the patient can observe, such as feelings of guilt or sadness.
An illness such as depression is often invisible to the outside observer. That is why it is very important for patients to make an accurate accounting of all of their symptoms so their doctor can diagnose them properly. In your depression research paper, you may explore these "invisible" symptoms of depression in adults or explore how depression symptoms can be different in children .
How Is Depression Diagnosed?
This is another good depression research topic because, in some ways, the diagnosis of depression is more of an art than a science. Doctors must generally rely upon the patient's set of symptoms and what they can observe about them during their examination to make a diagnosis.
While there are certain laboratory tests that can be performed to rule out other medical illnesses as a cause of depression, there is not yet a definitive test for depression itself.
If you'd like to pursue this topic, you may want to start with the Diagnostic and Statistical Manual of Mental Disorders (DSM). The fifth edition, known as DSM-5, offers a very detailed explanation that guides doctors to a diagnosis. You can also compare the current model of diagnosing depression to historical methods of diagnosis—how have these updates improved the way depression is treated?
Treatment Options for Depression
The first choice for depression treatment is generally an antidepressant medication. Selective serotonin reuptake inhibitors (SSRIs) are the most popular choice because they can be quite effective and tend to have fewer side effects than other types of antidepressants.
Psychotherapy, or talk therapy, is another effective and common choice. It is especially efficacious when combined with antidepressant therapy. Certain other treatments, such as electroconvulsive therapy (ECT) or vagus nerve stimulation (VNS), are most commonly used for patients who do not respond to more common forms of treatment.
Focusing on one of these treatments is an option for your depression research paper. Comparing and contrasting several different types of treatment can also make a good research title about depression.
A Word From Verywell
The topic of depression really can take you down many different roads. When making your final decision on which to pursue in your depression research paper, it's often helpful to start by listing a few areas that pique your interest.
From there, consider doing a little preliminary research. You may come across something that grabs your attention like a new study, a controversial topic you didn't know about, or something that hits a personal note. This will help you narrow your focus, giving you your final research title about depression.
Remes O, Mendes JF, Templeton P. Biological, psychological, and social determinants of depression: A review of recent literature . Brain Sci . 2021;11(12):1633. doi:10.3390/brainsci11121633
National Institute of Mental Health. Depression .
American Psychiatric Association. Diagnostic and Statistical Manual of Mental Disorders, Fifth Edition . American Psychiatric Association.
National Institute of Mental Health. Mental health medications .
Ferri, F. F. (2019). Ferri's Clinical Advisor 2020 E-Book: 5 Books in 1 . Netherlands: Elsevier Health Sciences.
By Nancy Schimelpfening Nancy Schimelpfening, MS is the administrator for the non-profit depression support group Depression Sanctuary. Nancy has a lifetime of experience with depression, experiencing firsthand how devastating this illness can be.
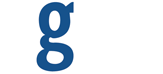
- Relationships of depression and …
- Editorial Board
- Information For Authors
- Advance Online Publications
- Current Issue
- Special Collections
- Scientific Integrity
- Publication Ethics and Publication Malpractice Statements
- Interviews with Outstanding Authors
Featured Nobel Articles
Elizabeth Blackburn , a member of the Editorial Board of Aging, won the Nobel Prize in Physiology or Medicine 2009, while being a member of the board. Elizabeth Blackburn co-authored a paper published in the first (inaugural) issue of Aging.
Andrew V. Schally , Nobel Prize Laureate, published his paper in Aging.
Shinya Yamanaka won the Nobel Prize in Physiology and Medicine 2012. Shinya Yamanaka co-authored a paper published in Aging.
Research Paper Advance Articles
Relationships of depression and antidepressant use with epigenetic age acceleration and all-cause mortality among postmenopausal women, may a. beydoun 1 , * , , hind a. beydoun 2 , 3 , * , , jason ashe 1 , , michael f. georgescu 1 , , steve horvath 4 , 5 , , ake lu 4 , , anthony s. zannas 6 , , aladdin h. shadyab 7 , , su yon jung 8 , , sylvia wassertheil-smoller 9 , , ramon casanova 10 , , alan b. zonderman 1 , , robert l. brunner 11 , ,.
- 1 Laboratory of Epidemiology and Population Sciences, National Institute on Aging, NIA/NIH/IRP, Baltimore, MD 21224, USA
- 2 VA National Center on Homelessness Among Veterans, U.S. Department of Veterans Affairs, Washington, DC 20420, USA
- 3 Department of Management, Policy, and Community Health, School of Public Health, University of Texas Health Science Center at Houston, Houston, TX 77030, USA
- 4 Department of Human Genetics, David Geffen School of Medicine, University of California Los Angeles, Los Angeles, CA 90095, USA
- 5 Department of Biostatistics, School of Public Health, University of California Los Angeles, Los Angeles, CA 90095, USA
- 6 Department of Psychiatry, School of Medicine, University of North Carolina at Chapel Hill, Chapel Hill, NC 27599, USA
- 7 Herbert Wertheim School of Public Health and Human Longevity Science and Division of Geriatrics, Gerontology, and Palliative Care, Department of Medicine, University of California, San Diego, CA 92093, USA
- 8 Department of Epidemiology, Fielding School of Public Health, Translational Sciences Section, School of Nursing, University of California, Los Angeles, CA 90095, USA
- 9 Department of Epidemiology and Population Health, Albert Einstein College of Medicine, Bronx, NY 10461, USA
- 10 Department of Biostatistics and Data Science, Wake Forest University School of Medicine, Winston-Salem, NC 27101, USA
- 11 Department of Family and Community Medicine (Emeritus), School of Medicine, University of Nevada, Reno, NV 89557, USA
Received: October 30, 2023 Accepted: May 3, 2024 Published: May 27, 2024
Cite this article, how to cite.
Beydoun MA , Beydoun HA , Ashe J , Georgescu MF , Horvath S , Lu A , Zannas AS , Shadyab AH , Jung SY , Wassertheil-Smoller S , Casanova R , Zonderman AB , Brunner RL , . Relationships of depression and antidepressant use with epigenetic age acceleration and all-cause mortality among postmenopausal women. Aging (Albany NY). 2024 May 27; . https://doi.org/10.18632/aging.205868 [Epub ahead of print]
Copy or Download citation:
Select the format you require from the list below
- Reference Manager
Click to copy this citation from the text box above or download the citation: Citation | Citation & Abstract
Copyright: © 2024 Beydoun et al. This is an open access article distributed under the terms of the Creative Commons Attribution License (CC BY 4.0), which permits unrestricted use, distribution, and reproduction in any medium, provided the original author and source are credited.
We investigated relations of depressive symptoms, antidepressant use, and epigenetic age acceleration with all-cause mortality risk among postmenopausal women. Data were analyzed from ≤1,900 participants in the Women's Health Initiative study testing four-way decomposition models. After a median 20.4y follow-up, 1,161 deaths occurred. Approximately 11% had elevated depressive symptoms (EDS + ), 7% were taking antidepressant medication at baseline (ANTIDEP + ), while 16.5% fell into either category (EDS_ANTIDEP + ). Baseline ANTIDEP + , longitudinal transition into ANTIDEP + and accelerated epigenetic aging directly predicted increased mortality risk. GrimAge DNA methylation age acceleration (AgeAccelGrim) partially mediated total effects of baseline ANTIDEP + and EDS_ANTIDEP + on all-cause mortality risk in socio-demographic factors-adjusted models (Pure Indirect Effect >0, P < 0.05; Total Effect >0, P < 0.05). Thus, higher AgeAccelGrim partially explained the relationship between antidepressant use and increased all-cause mortality risk, though only prior to controlling for lifestyle and health-related factors. Antidepressant use and epigenetic age acceleration independently predicted increased all-cause mortality risk. Further studies are needed in varying populations.
- Introduction
Frequently under-recognized [ 1 , 2 ], depression is a major contributor to the Global Burden of Diseases while being the most prevalent mental illness among geriatric populations [ 2 ]. Previous studies have established depression as a risk factor for age-related chronic conditions such as metabolic syndrome, diabetes mellitus, and cardiovascular disease [ 3 ]. By the same token, antidepressants are among the most widely prescribed medications in older adults [ 4 ]. Long-term health consequences of antidepressant use have not been adequately evaluated although a quarter of individuals prescribed antidepressants take them for ≥10 years [ 5 ]. Moreover, U.S. FDA guidance for long-term use of antidepressants relates mostly to Major Depressive Disorder (MDD), although a large percentage of antidepressant users do not meet the diagnostic criteria for MDD, and several classes of antidepressants are prescribed for other indications besides depression [ 6 ]. Although antidepressants can be effective at reducing depressive symptoms and potentially improving cognitive function and quality of life, they have been linked to side-effects such as weight gain, hyponatremia, reduced bone mineral density, tremor, sexual dysfunction, lessened general well-being, suicide, as well as increased risks of falls, fractures, and cardiovascular morbidities, with implications for compliance with prescribed treatments [ 4 , 7 ]. As such, it is important to examine the separate and joint contributions of depression and antidepressant use to age-related health outcomes and their underlying processes.
Postmenopausal women constitute a high-risk group for both depression and antidepressant use since mental illnesses – including depression – increase with age and predominantly affect females [ 2 ]. Evidence from the Women’s Health Initiative (WHI) suggests that depression or antidepressant use may increase the vulnerability of postmenopausal women to age-related health problems including weight gain [ 8 , 9 ], diabetes mellitus [ 10 , 11 ], pre-hypertension and hypertension [ 12 ], cardiovascular disease [ 8 , 13 ], cognitive dysfunction [ 4 , 14 ], colorectal cancer [ 6 ], bone loss and fracture [ 15 ], hip and knee osteoarthritis [ 16 ], Parkinson’s disease [ 17 ], as well as frailty [ 7 ], with detrimental impact on cancer survivorship [ 18 , 19 ], all-cause and cause-specific mortality [ 18 – 20 ] risks. Depression and antidepressants may be linked to health problems due to factors like inflammatory responses [ 21 – 24 ], neurotoxicity [ 25 , 26 ], and epigenetic changes [ 27 ], with certain antidepressants potentially possessing anti-inflammatory properties [ 6 ].
Previous studies focused on health disparities have explored epigenetic age acceleration as a potential mediator for the effect of demographic (e.g., race), socioeconomic (e.g., education) and psychosocial factors – including depression – on morbidity and mortality risks, in general, and among postmenopausal women, in particular [ 28 – 30 ]. A mediator is often defined as an intermediate variable on the pathway between an exposure and an outcome that explains part of the effect of the exposure on that outcome variable. Accounting for this third variable often alters the total effect (TE) between the exposure and the outcome leading to an attenuation of the TE towards the null, a phenomenon known as consistent mediation [ 31 – 33 ]. In other instances, the TE becomes biased away from the null value, a phenomenon known as inconsistent mediation [ 31 – 33 ]. More generally, a mediator is influenced by the exposure and is on the causal pathway between the exposure and the outcome [ 31 – 33 ]. A third variable can be both a mediator and a moderator and can also be only a moderator or neither of the two. A moderator interacts with the exposure of interest to alter the TE of the exposure on the outcome in a way that the effect of the exposure on the outcome differs across levels of that third variable [ 31 – 33 ]. Although several recent studies have examined the association between depression and epigenetic age acceleration [ 34 – 39 ], few also examined the use of antidepressants [ 38 , 39 ].
Epigenetic clocks are biomarkers that reflect biological aging based on DNA methylation (DNAm) of cytosine phosphate guanine (CpG) sites [ 28 ]. They are distinct from clinical and molecular markers that capture more limited aspects of aging [ 28 ]. Epigenetic clocks have been developed that predict longevity [ 29 ] and are strongly correlated with chronological age across distinct cell, tissue, and organ types [ 28 , 40 , 41 ]. Epigenetic clocks show promise in elucidating biological mechanisms pertaining to aging, chronic disease, and mortality risks [ 29 ]. Chronological age has been shown to increase levels of methylation in specific regions of the genome [ 29 ]. “DNAm age” – also known as “epigenetic age” – represents innate aging processes at the cellular level which have been linked to functional decline with age [ 30 ]. Epigenetic age can be estimated using multivariable regression models of DNAm profiles, and a discrepancy between DNAm age and chronological age, known as epigenetic age acceleration (EAA), has been associated with adverse health outcomes [ 28 – 30 , 41 ]. A higher “DNAm age” compared to chronological age suggests faster biological aging than expected [ 30 ]. Epigenetic age acceleration is linked to obesity, early menopause, Down syndrome, Werner syndrome, HIV infection, lung cancer, Alzheimer’s and Parkinson’s diseases, and is determined partly by genetic factors and partly by environmental, psychosocial, and behavioral factors [ 40 , 41 ]. Two epigenetic clocks, blood-based Hannum (71 CpGs) and pan-tissue Horvath (353 CpGs), can be used to derive extrinsic and intrinsic epigenetic age acceleration (EEAA and IEAA) by calculating the difference between DNAm and chronologic ages [ 28 , 42 ]. Age-related changes in methylation of 353 CpGs included in the Horvath epigenetic clock, are known to influence DNA replication and repair, lipid metabolism, oxidative stress, and other processes linked to chronic diseases [ 42 , 43 ], while epidemiologic evidence suggests that the Horvath estimator may predict cognitive function, lung function, physical strength, and premature mortality [ 42 ]. PhenoAge and GrimAge are next-generation epigenetic clocks from which EAA can also be estimated [ 29 , 30 ].
Taken together, depression is a prevalent mental disorder among older populations, is linked to various diseases, particularly in postmenopausal women, and as a result may be influenced by epigenetic clocks. Furthermore, postmenopausal women are more likely than other groups to be prescribed antidepressant medications. Thus, depression (or elevated depressive symptoms, EDS) and/or anti-depressant use’s potential association with mortality risk may be mediated or potentially moderated by epigenetic clocks. Moreover, epigenetic clocks have been associated with increased mortality risk [ 44 – 46 ]. This positive association may be mediated by or moderated through depressive symptoms and/or through anti-depressant use. The interplay between elevated depressive symptoms (and/or anti-depressant use), epigenetic age acceleration and mortality risk remains largely unknown, particularly among postmenopausal women.
The present cohort study performed longitudinal analyses of existing observational data from the Women’s Health Initiative (WHI) ancillary study, to examine epigenetic age acceleration as a mediator/moderator between EDS (and/or antidepressant use) as a primary exposure of interest and all-cause mortality as the outcome among postmenopausal women. A secondary analysis was also conducted with EAA as the main exposure, all-cause mortality the outcome of interest and potential mediators/moderators being elevated depressive symptoms (EDS) and/or antidepressant use.
- Materials and Methods
Data source
The WHI is a long-term study focused on strategies for preventing heart disease, breast, and colorectal cancers as well as osteoporosis in postmenopausal women. The WHI study design, eligibility criteria, recruitment methods and measurement protocols are described elsewhere [ 47 , 48 ]. Briefly, the WHI collected data on a multiethnic sample of postmenopausal women who were recruited and enrolled between 1993 and 1998 at 40 geographically diverse clinical centers (24 states and the District of Columbia) in the United States. The WHI study received institutional review board approval with informed consent from all participating clinical centers. WHI-Clinical Trials (CTs) ( n = 68,132) and WHI-Observational Study (OS) ( n = 93,676) are two components of the WHI ( n = 161,808). Whereas WHI-CTs evaluated outcomes of menopausal hormone therapy (Hormone Therapy (HT) Trials), calcium and vitamin D supplementation ((CaD) Trial), and a low-fat eating pattern (Dietary Modification Trial), the WHI-OS evaluated causes of morbidity and mortality in postmenopausal women. The main WHI studies occurred between 1993 and 2005, and of 150,076 participants who were actively followed-up at the end of these studies, 76.9% participated in Extension Study 1 (2005–2010) and 86.9% of those eligible participated in Extension Study 2 (2010–2020) [ 49 , 50 ]. At enrollment (1993–1998), WHI participants, 50–79 years of age, underwent a clinical examination and completed the same self-administered questionnaire covering demographics, general health, clinical and anthropometric characteristics, functional status, healthcare behaviors, reproductive, medical, and family history, personal habits, thoughts and feelings, therapeutic class of medication, hormones, supplements, and dietary intake, and several of these characteristics were assessed at later follow-up times.
Study participants
We restricted this analysis to WHI participants with available DNAm data at enrollment (1993–1998) who took part in an ancillary case-control study (BA23) focused on identifying novel genomic determinants of coronary heart disease, as previously reported by others [ 30 , 41 ]. In this integrative genomics study, cases and controls had already undergone genome-wide genotyping at baseline as well as profiling of seven cardiovascular biomarkers, with oversampling of African American and Hispanic participants [ 51 ]. Specifically, a stratified, racially/ethnically diverse sample of ≈ 2,200 WHI-CT participants with available stored serum were selected for analysis of DNA methylation at screening or annual visits [ 29 ]. At enrollment (1993–1998), blood samples were collected from participants, placed in EDTA tubes after an overnight fast, and stored at −80°C for processing by WHI core laboratories [ 29 ]. Patients with evidence of leukemia at enrollment (1993–1998) were excluded from these analysis [ 29 ]. Further details can be found under the following link: https://sp.whi.org/researchers/data/WHIStudies/StudySites/BA23/pages/home.aspx . In brief, this sub-study aimed at evaluating miRNA and methylation levels in coronary heart disease (CHD) events in 1070 patients and 1070 controls. Researchers used high-throughput genomic techniques to assess methylation status and miRNA levels in circulating white blood cells. They also used statistical techniques, machine learning, and sparse predictors to identify regulators and co-methylation modules linked to CHD. The study also reviewed genome-wide association studies to identify hundreds of molecular sub-phenotypes and CHD susceptibility polymorphisms.
Of the available 2,200 participants, 1,900 had complete data on baseline EDS and/or antidepressant use, and had known socio-demographics, particularly age, race, and ethnicity. All other demographic and socio-economic factors as well as lifestyle and health-related covariates were subjected to multiple imputation, as described later.
DNA methylation
Illumina Infinium Human-Methylation 450 Bead Chip at the HudsonAlpha Institute of Biotechnology was used to perform analyses of DNA methylation [ 29 ]. Epigenetic clocks were calculated using genome-wide DNA methylation data that can estimate epigenetic age (DNAm) using the proportion of modified signal at each CpG site [ 29 ].
Study variables
Elevated depressive symptoms, eds.
A depressive symptoms screening algorithm previously developed by Burnam et al. with scores ranging between 0 and 1 and higher scores consistent with greater burden of depressive symptoms were generated using 6 items from the 20-item CES-D scale and 2 items from the National Institute of Mental Health’s Diagnostic Interview Schedule. Furthermore, we dichotomized this variable based on a pre-established threshold of 0.06, whereby WHI participants with a score >0.06 have strong evidence of depressive symptoms whereas those with a score ≤0.06 do not [ 52 , 53 ]. Repeated measures of EDS at enrollment (1993–1998) and 3-year follow-up were examined to evaluate change over time. Specifically, women were classified as having no change (0) if their status did not change between enrollment (1993–1998) and 3-year follow-up time, an increase (or transition into EDS + ) (+) if they were non-depressed at enrollment (1993–1998) and depressed at 3-year follow-up time or a decrease (or transition out of EDS − ) (−) if they were depressed at enrollment (1993–1998) and non-depressed at 3-year follow-up.
Antidepressant use, ANTIDEP
WHI participants were instructed to bring prescription and non-prescription medication containers at enrollment (1993–1998). For medications used for >2 weeks, drug names and doses were entered into a medications database and assigned therapeutic class codes using the Master Drug Data Base (MDDB: Medi-Span, Indianapolis, IN; Medi-Span software: First DataBank, Inc., San Bruno, CA, USA). Antidepressant use at enrollment was defined as a dichotomous (‘yes’ or ‘no’) variable (form 44) based on the following therapeutic class codes used for WHI at baseline and at 3-years of follow-up: α-2 receptor antagonists (Tetracyclics) (580300), MAO inhibitors (581000), modified cyclics (581200), selective serotonin reuptake inhibitors (581600), serotonin-norepinephrine reuptake inhibitors (581800), tricyclic agents (582000), miscellaneous antidepressants (583000), antidepressant combinations (589900, 589980, 589985, 589987, 589990). Repeated measures of antidepressant use at enrollment (1993–1998) and 3-year follow-up were examined to evaluate change over time. Specifically, women were classified as having no change (0) if their status did not change between enrollment (1993–1998) and 3-year follow-up time, an increase (or transition into ANTIDEP + ) (+) if they were non-users of antidepressants at enrollment (1993–1998) and users of antidepressants at 3-year follow-up time or a decrease (i.e. transition out of ANITIDEP + ) (−) if they were users of antidepressants at enrollment (1993–1998) and non-users of antidepressants at 3-year follow-up. Due to sample size limitations, we combined all types of antidepressants for the main analyses.
EDS and/or antidepressant use
A categorical variable was defined by combining the dichotomous variables for EDS and antidepressant use (ANTIDEP) as follows: [ 1 ] no EDS and no antidepressant use; [ 2 ] no EDS and antidepressant use; [ 3 ] EDS and no antidepressant use; [ 4 ] EDS and antidepressant use. This variable was used to describe the interaction between depression and antidepressant use. Due to sample size limitations, a dichotomous version of depression and/or antidepressant use was defined for use in this analysis to compare women who had EDS or were antidepressant users to those who were neither depressed nor antidepressant users at enrollment (1993–1998) or 3 years of follow-up. Repeated measures of EDS and/or antidepressant use at enrollment (1993–1998) and 3-year follow-up was examined to evaluate change over time. Specifically, women were classified as having no change (0) if their status did not change between enrollment (1993–1998) and 3-year follow-up time, an increase or a transition into EDS + or ANTIDEP + (+) if they were either EDS − or non-users of antidepressants at enrollment (1993–1998) and either EDS + or users of antidepressants at 3-year follow-up time or a decrease or transition out of EDS + or ANTIDEP + (−) if they were EDS + or users of antidepressants at enrollment (1993–1998) and EDS − or non-users of antidepressants at 3-year follow-up.
Epigenetic age acceleration
We defined epigenetic age acceleration using residuals from regression of biological age on chronological age, with biological age defined according to four distinct epigenetic clocks, namely the blood-based Hannum (EEAA) and pan-tissue Horvath (IEAA) estimators described previously as well as next-generation estimators called PhenoAge and GrimAge yielding AgeAccelPheno and AgeAccelGrim, respectively. These four measures of epigenetic age acceleration have been previously calculated in the context of BA23 and are available for further analyses.
Extrinsic epigenetic age acceleration (EEAA)
The EEAA is based on 71 CpGs as well as counts of naïve and exhausted cytotoxic T cells, and plasma B cells, which are known to be associated with age in order to calculate Hannum’s estimator of “DNAm age” [ 29 , 30 ]. In sum, it is a weighted average of “DNAm age” and WBC cell composition that vary with age. In this study, we used residual values of EEAA from a regression model involving “weighted DNAm age” in relation to “chronological age” [ 29 , 30 ].
Intrinsic epigenetic age acceleration (IEAA)
IEAA is based on 353 CpGs used to calculate Horvath’s estimator for “DNAm age” [ 30 ]. In this study, we relied on an estimate of IEAA calculated as a residual from regressing “DNAm age” on “chronological age” and estimated measures of WBC cell composition (naïve and exhausted CD8+ T cells, CD4+ T cells, plasma B cells, natural killer cells, monocytes, and granulocytes) to adjust for confounding by WBC changes in composition occurring with aging [ 29 , 30 ].
AgeAccelPheno
This next-generation measure of epigenetic age acceleration is based on the “PhenoAge” estimator derived from an algorithm that comprises 513 CpGs and that can predict chronological age as well as a composite of physiological indicators such as albumin and glucose [ 29 , 30 ]. AgeAccelPheno was estimated using the residual method from a model with Age as the outcome.
AgeAccelGrim
This next-generation measure of epigenetic age acceleration is based on the “GrimAge” estimator derived in two stages. At the first stage, surrogates of pack-years of smoking and 12 plasma proteins were defined based on “DNAm age”. At the second stage, time-to-death was regressed on these surrogates. “GrimAge” epigenetic clock consists of 1,030 CpG sites that jointly predict mortality risk [ 29 , 30 ]. AgeAccelGrim was estimated using the residual method from a model with Age as the outcome.
Summary of the EAA measures
Epigenetic age acceleration is defined by utilizing residuals from regression of biological age on chronological age, using four distinct epigenetic clocks: blood-based Hannum (EEAA), pan-tissue Horvath (IEAA), PhenoAge, and GrimAge. These four measures of epigenetic age acceleration have been previously calculated in the context of BA23 and are available for further analyses. Extrinsic epigenetic age acceleration (EEAA) is based on 71 CpGs and counts of naïve and exhausted cytotoxic T cells and plasma B cells, which are known to be associated with age. Intrinsic epigenetic age acceleration (IEAA) is based on 353 CpGs and is used to calculate Horvath’s estimator for “DNAm age.” AgeAccelPheno is a next-generation measure of epigenetic age acceleration based on the “PhenoAge” estimator, which can predict chronological age and a composite of physiological indicators such as albumin and glucose. AgeAccelGrim is based on the “GrimAge” estimator, which consists of 1,030 CpG sites that jointly predict mortality risk.
All-cause mortality risk
Deaths were ascertained via semi-annual or annual follow-up with family, friends, and medical care providers of WHI participants, in addition to the National Death Index and obituaries [ 54 ]. In this study, participants were followed up through December 31st, 2021, to evaluate all-cause mortality risks. Censoring age for those who did not experience the event of interest was age at baseline plus the number of years for the longest follow-up time, given that follow-up ended in December 31st, 2021. Survival time was set up using baseline age for time of entry, age at event or censoring for time of exit (end of follow-up or loss to follow-up) and event (yes vs. no) for all-cause mortality, with the origin being baseline age.
The hypothesized relationships are potentially confounded by socio-demographic, lifestyle and health characteristics that are known to be associated with mortality risks and may be related to EDS and/or antidepressant use. Covariates collected at the enrollment visit included BA23 case-control status, socio-demographic characteristics (race (American Indian/Alaska Native, Asian, Native Hawaiian/Other Pacific Islanders, Black, White, More than one race, Unknown/Not reported), recoded into “White”, “Black” and “Others” (missing were excluded); ethnicity (Hispanic, non-Hispanic, Unknown/Not reported), education (less than high school, high school, some college, completed college or higher level), household income ( 55 ] at enrollment [ 56 ]. Weight was measured to the nearest 0.1 kg on a balance beam scale with the participant dressed in indoor clothing without shoes, while height was measured to the nearest 0.1 cm using a wall-mounted stadiometer. BMI was calculated as (weight (kg) ÷ (height 2 (m 2 )) and further categorized as 2 (underweight/normal weight); 25.0–29.9 kg/m 2 (overweight); and ≥30 kg/m 2 (obese). The history of cardiovascular disease was defined in terms of previous coronary heart disease, angina, aortic aneurysm, carotid endarterectomy or angioplasty, atrial fibrillation, congestive heart failure, cardiac arrest, stroke, or transient ischemic attack. The history of hypertension was defined as self-reported diagnosis or treatment for hypertension or evidence of high blood pressure based on systolic blood pressure (SBP) and diastolic blood pressure (DBP) measurements. The history of diabetes was defined as physician-diagnosed diabetes or use of diabetes medications. The history of hyperlipidemia was defined as using lipid-lowering medications or having been told of high cholesterol by a physician.
Statistical analysis
All statistical analyses were conducted using SAS version 9.4 (SAS Institute, Cary, NC, USA) for data management and STATA version 18 (StataCorp, College Station, TX, USA) for bivariate and multivariable analyses.
First, summary statistics included mean ± standard errors for continuous variables and frequencies with percentages for categorical variables. Kaplan-Meier estimates for survival probabilities were plotted against the 3 main baseline exposures of interest (baseline EDS, antidepressant use (ANTIDEP) and the combined baseline exposure EDS_ANTIDEP) across follow-up time (years) from baseline age to exit through censoring or event. Log-rank tests were conducted and other survival time descriptives (years) are reported along with exposure prevalence estimates.
Second, simple and multivariable linear, multinomial logistic or Cox PH regression models were constructed to estimate β±SE, Log e of the odds ratios (OR) or hazard ratios (HR) with their SE, respectively. We examined the bivariate association of baseline socio-demographic, lifestyle, and health characteristics with several alternative outcomes, namely epigenetic age acceleration, EDS and/or antidepressant use, as well as all-cause mortality risks. We further constructed binary and multinomial logistic regression models to examine the relationship of epigenetic age acceleration with EDS, antidepressant use as well as EDS and/or antidepressant use (defined as categorical and dichotomous variables) at enrollment (1993–1998) and 3-year follow-up.
Third, we constructed Cox regression models to examine the relationships of epigenetic age acceleration, EDS, antidepressant use as well as EDS and/or antidepressant use (defined as categorical and dichotomous variables) at enrollment (1993–1998) and 3-year follow-up with all-cause mortality risks, before and after adjustment of covariates.
Fourth, we applied causal mediation analyses to examine the mediating and/or moderating effects of epigenetic age acceleration on the relationship of EDS and antidepressant use at enrollment (1993–1998) (and the combination of the two), with mortality risk. Specifically, med4way STATA command was used to estimate the mediating and/or moderating effect of z-transformed epigenetic age acceleration scores on the TE of EDS and/or antidepressant use on mortality risk, controlling for baseline characteristics [ 57 – 59 ].
This causal mediation analysis is helpful in a counterfactual framework and in the context of observational data whereby two models are estimated, namely, a model for the mediator conditional on exposure and covariates and a model for the outcome conditional on the exposure, mediator, and covariates [ 57 – 59 ]. Assuming no unmeasured confounding and 4-way decomposition, the med4way command can also facilitate estimation of mediation but not interaction (pure indirect effect (PIE)), interaction but not mediation (reference interaction (INTREF)), both mediation and interaction (mediated interaction (INTMED)) and neither mediation nor interaction (controlled direct effect (CDE)), whereby the TE can be calculated as follows: TE = CDE+PIE+INTEF+INTMED [ 57 ]. Four-way decomposition parameters were estimated on the multiple-imputed data using Rubin’s rule to obtain averages of these estimates along with their Standard Errors and p -values. Percentages of TE accounted for by each of these four components were also estimated, though without SE or p -value, which cannot be directly estimated from multiple-imputed data. Thus, the statistical significance of the PIE in models where TE was statistically significant at a type I error of 0.05 and where PIE and TE have the same direction, determined consistent mediation. The degree to which TE was mediated with the third variable was determined by the percent PIE of TE.
Three alternative models were tested, by incrementally including exogenous covariates: Model 1 (unadjusted), Model 2 (adjusted only for socio-demographic variables, including measures of socio-economic status) and Model 3 (Model 2 further adjusted for lifestyle and health-related factors).
Fifth, to study bi-directional associations, another set of four-way decomposition models were conducted, whereby EDS and/or antidepressant use were considered as alternative mediators, while epigenetic age acceleration measures were the main exposures of interest assumed to have a positive TE on mortality risk. In this final set of models, the mediator equation was a logistic regression model given that all the alternative mediators were binary.
Given the limited sample size, we performed multiple imputations (5 datasets, 10 iterations) of covariates after selecting the sample of interest, based on inclusion and exclusion criteria, as described by Lee and Carlin [ 60 ]. Two-sided statistical tests were conducted at α = 0.05. Supplementary Figure 1 shows a graphical depiction of the study design, focusing on the time frame of measures for exposure, mediator and outcome as well as exclusion criteria.
After a mean time of follow-up of 20.4 years (range: 0.10–28.8 years), 1,161 deaths occurred in the largest selected sample of 1,900 postmenopausal women. Thus, median survival time was 21.9 years with an interquartile range of 15.7–27.48 years, and incidence rate was estimated at 3,247 per 100,000 P-Y (output and Stata script provided on GitHub at https://github.com/baydounm/WHI_EPIDGENETICCLOCK_DEP_MORT ). With proportions of 11–17% ( Figure 1D ), the associations of the 3 baseline exposures (EDS/ANTIDEP) with all-cause mortality risk is presented in Figure 1A – 1C , using Kaplan-Meier (K-M) survival probability estimates and log-rank tests. Overall, only baseline antidepressant use (ANTIDEP) and the combined exposure (EDS_ANTIDEP) were significantly associated with increased all-cause mortality risk in this sample (Log-rank test, 1 d.f. (ANTIDEP): 12.5, P = 0.0004; Figure 1B ; Log-rank test, 1 d.f. (EDS_ANTIDEP): 6.4, P = 0.012; Figure 1C ), while mortality risk was comparable between EDS + and EDS − groups.
Figure 1. ( A – D ) Elevated depressive symptoms and antidepressant use baseline exposures vs. all-cause mortality risk, Women’s Health Initiative Study. Abbreviations: EDS: Elevated depressive symptoms; ANTIDEP: Antidepressant use; EDS_ANTIDEP: Elevated depressive symptoms and/or antidepressant use.
Table 1 displays study sample characteristics, overall and their relationship with the four epigenetic age acceleration metrics. The mean ± SE of baseline age was 64.6 ± 0.2 y, 32% of the sample consisted of Black adults, while 65.7% were White. Hispanic ethnicity was self-reported by 14.8% of this sample. Over half (53%) were married/partnered, with 29.5% being college graduates and 30.0% with incomes below $20,000. In terms of lifestyle and health-related factors, 53.3% were never smokers, 15.4% were non-drinkers, Met-hour/week was on average 10.1, while body mass index was 29.8 kg.m −2 , with obesity screened for 43.9% of the sample. History of cardiovascular disease, hypertension, diabetes, and dyslipidemia was reported by 17.6%, 53.3%, 23.5% and 16.3%, respectively. Around 11% of the sample had EDS at baseline, while 12.5% reported their health as fair/poor. Bivariate differences in baseline characteristics were found in terms of epigenetic age acceleration metrics, with consistently faster acceleration with respect to hypertension and BMI, across all four metrics, and in the case of diabetes for 3 of 4 metrics. Most notably, and unlike all other epigenetic clocks under study, GrimAge age acceleration was increased by +0.78y when comparing those with EDS vs. not at baseline ( P p < 0.010). It is worth noting that EAA metrics differed by race, though without a consistent pattern that was discerned.
Table 1. Associations of sociodemographic, lifestyle and health characteristics with epigenetic age acceleration ( n = 1,900) – Women’s Health Initiative.
Table 2 presents the results of the bivariate associations between baseline characteristics and the key outcomes or mediators, namely all-cause mortality (using Cox PH models) and EDS/antidepressant use at baseline (using a series of bivariate logistic regression models). The odds of EDS or combination of EDS and antidepressant use was reduced with advancing age, with higher education and income, greater physical activity, and was increased among current smokers, with higher BMI, and among those reporting fair/poor health. The latter also predicted antidepressant use, which was less prevalent among Black participants compared to their White counterparts. All-cause mortality (1,161 deaths by end of follow-up), on the other hand, was increased with age, reduced among Black vs. White participants, as well as among Hispanic vs. non-Hispanic participants, increased among single and widowed participants compared to their married/partnered counterparts, reduced with educational attainment and income levels, as well as physical activity, increased among current smokers, and in the presence of cardiovascular disease, hypertension, diabetes, hyperlipidemia and with a self-rated health as fair/poor (vs. excellent/very good/good).
Table 2. Associations of sociodemographic, lifestyle and health characteristics with baseline elevated depressive symptoms (EDS), antidepression use and all-cause mortality risk ( n = 1,900) – Women’s Health Initiative.
The relationship between epigenetic age acceleration metrics and EDS/antidepressant use measures is presented in Table 3 , using a series of unadjusted and multivariable-adjusted logistic regression models. Among the four metrics, only AgeAccelGrim was associated with antidepressant use or the combination of EDS and antidepressant use, in both the unadjusted and the partially adjusted model for sociodemographic variables, including age, race, ethnicity, marital status, education and income. In contrast, antidepressant use or the combination of EDS and antidepressant use were no longer associated with AgeAccelGrim, upon further adjustment for lifestyle and health-related factors.
Table 3. Logistic regression models for elevated depressive symptoms (EDS) and/or antidepressant use at baseline, as predicted by estimates of epigenetic age acceleration ( n = 1,900) a .
Table 4 shows the main findings from a series of Cox PH models with incremental adjustment for covariates, examining the associations between epigenetic age acceleration metrics, EDS/antidepressant use exposures and the all-cause mortality outcome. In all models, EEAA, AgeAccelPheno, and AgeAccelGrim were associated with increased risk of mortality, suggesting that those metrics were predictive of mortality risk independently of sociodemographic, lifestyle, and health-related factors. In contrast, IEAA was only associated with all-cause mortality in the unadjusted and socio-demographic factor-adjusted models. While baseline EDS exposure was not associated with all-cause mortality, baseline antidepressant use and combined baseline EDS and antidepressant use were associated with this outcome in the unadjusted and socio-demographic factor-adjusted model, whereas antidepressant use was significantly associated with higher mortality risk after further adjustment for lifestyle and health-related factors. Furthermore, increased use of antidepressants between baseline and 3-year follow-up compared to no change in use was linked to increased mortality risk in all three models, with some attenuation between models 2 and 3 (Log e HR = +0.52 ± 0.15, P e HR = +0.42 ± 0.15 in Model 3, P n = 1,696; EDS status change: n = 473; combined EDS and anti-depressant use: n = 462).
Table 4. Cox proportional hazard models for baseline and change in elevated depressive symptom status (EDS status), antidepressant use (ANTIDEP), EDS and/or antidepressant use (EDS_ANTIDEP) as well as epigenetic age acceleration predictors of all-cause mortality risk ( n = 1,900).
Causal mediation using four-way decomposition models was carried out on the baseline exposures and potential mediators/moderators in relation to all-cause mortality risk. In Table 5 , the three exposures were baseline EDS and antidepressant exposures (EDS (yes vs. no); antidepressant use (yes vs. no); and EDS and/or antidepressant use (yes vs. no)), the mediators were the four epigenetic age acceleration metrics. The models were tested, unadjusted for exogenous variables (Model 1), adjusted for socio-demographic covariates (Model 2), and the fully adjusted model that added lifestyle and health-related covariates to Model 2 (Model 3). TEs were statistically significant for both antidepressant use and EDS and/or antidepressant use exposures. Those were largely controlled direct effects (CDE) in Models 1 and 2. Nevertheless, a small proportion of this TE (TE >0, P 0, P 0, P 0, P < 0.05) in Model 2 for the combined exposure at baseline (i.e., EDS_ANTIDEP). In contrast, when all exogenous variables were adjusted for in Model 3, only the TE of the antidepressant use variable remained statistically significant and was not mediated or moderated by any of the epigenetic age acceleration metrics, and therefore was mainly composed of a CDE.
Table 5. Causal mediation analysis (four-way decomposition models) of the total effects of baseline elevated depressive symptoms (EDS), antidepressant use (ANTIDEP) and combined exposure (EDS_ANTIDEP) on mortality risk with epigenetic age acceleration measures as alternative mediators and/or moderators ( n = 1,900).
In Supplementary Table 1 , a similar modeling strategy was carried out, though in this instance, epigenetic age acceleration metrics were the main exposures of interest while EDS/antidepressant use were the key potential mediators/moderators. In these models, TEs were generally indicative of a positive association between epigenetic age acceleration and mortality risk, even after adjustment for all potentially confounding variables. In all these models, TEs were mainly composed of controlled direct effects, suggesting that EDS, ANTIDEP and EDS_ANTIDEP at baseline did not mediate or moderate the association between epigenetic age acceleration and mortality risk.
Summary of findings
The study examined the impact of depressive symptoms, antidepressant use, and epigenetic age acceleration on all-cause mortality in postmenopausal women. Data from 1,900 participants were used to test associations between key exposures and outcomes. Bi-directional four-way decomposition models were conducted to examine the mediating and moderating roles of epigenetic age acceleration, EDS, and antidepressant use at baseline. After a median 20.4 years follow-up time, 1,161 deaths occurred. Around 11% had elevated depressive symptoms (EDS + ), 7% were taking antidepressant medication at baseline (ANTIDEP + ), while 16.5% fell into either category (EDS_ANTIDEP + ). Baseline ANTIDEP + , longitudinal increase in ANTIDEP + and accelerated epigenetic age acceleration directly predicted all-cause mortality risk. GrimAge age acceleration mediated part of the TEs of baseline ANTIDEP + and EDS_ANTIDEP + on all-cause mortality risk in sociodemographic factors-adjusted models, thus only prior to controlling for lifestyle and health-related factors (PIE >0, P 0, P < 0.05).
Previous studies
Epigenetic age acceleration has been connected to both MDD and depression symptoms. For instance, Han et al. used data from the Netherlands Study of Depression and Anxiety (NESDA) with a standard cut point (14) on the Inventory of Depressive Symptomology and a follow up of 4 years. They found significantly higher epigenetic age acceleration in patients with MDD ( n = 319 compared to controls n = 811) [ 61 ]. Although no additional associations between increased epigenetic age acceleration and cumulative clinical features were found, their data suggested that more advanced epigenetic age acceleration in MDD may be substantially explained by severity of depression [ 61 ]. In a recent analysis of the Healthy Aging of Neighborhoods of Diversity Across the Life Span (HANDLS) project, we found a cross-sectional relationship between two epigenetic age acceleration measures (the Horvath 1 and the Hannum clocks) and lower levels of positive affect only in White participants, which remained statistically significant after further adjusted for potential confounders, including in the case of the Hannum clock all socio-demographic and socio-economic factors in addition to health-related and/or dietary factors [ 34 ]. A case-control study with 60 age-matched controls and 49 MDD cases found a link between MDD and GrimAge acceleration [ 62 ]. GrimAge acceleration is a DNAm-based epigenetic clock which is an estimator of smoking pack-years and proxy DNAm biomarkers of seven different plasma proteins [ 62 ]. After adjusting for sex, current smoking status, and BMI, the link between GrimAge and MDD remained significant ( p = 0.015) [ 62 ]. Using data from the Health and Retirement Study, GrimAge DNAm age was linked to long-term, persistently higher depressive symptoms [ 63 ]. Nevertheless, when accounting for smoking and BMI this connection was considerably diminished, becoming marginally significant when comparing high vs. low depressive symptom levels in the fully adjusted model [ 63 ]. This is in line with the earlier study with HANDLS data [ 34 ], in which no link was detected between depressive symptoms and epigenetic aging using the Horvath and Hannum epigenetic clocks, and only a weak association with the reduced positive affect component of depressive symptom measurement. Our present results are consistent with these previous findings.
It is well established that depression is a major risk factor predictive of all-cause mortality for adults across the life span. However, additional consideration for older adults remains a public health concern, given their vulnerabilities to psychosocial difficulties, such as loneliness and social isolation that may have a role in the diagnosis and/or its severity. In several longitudinal studies, higher mortality rates were observed among older adults living with more depressive symptoms, clinically diagnosed minor and major depression, and history of mood disorders compared to nondepressed adults [ 64 , 65 ]. Prior studies have established these associations in populations outside of the U.S. [ 66 , 67 ] and in samples previously diagnosed with chronic health conditions such as cancer or diabetes [ 18 , 19 ]. Emerging work has shown modest or null associations between depression or depressive symptoms and all-cause mortality rates among older adult women [ 18 , 19 , 68 ]. Depression was related to all-cause mortality in postmenopausal women from China [ 68 ]. Other studies using U.S.-based female populations exclusively included women previously diagnosed with other comorbidities and terminal conditions such as breast and colorectal cancer and showed minimal effects [ 18 , 19 ]. In one study using participant data from the WHI, women who developed depression before a breast cancer diagnosis, unlike women with a prior history of depression, showed a modestly heightened risk for mortality [ 19 ]. This finding suggests that developing a mood disorder later in life along with other severe illnesses might increase risk, but the nuances of these mechanisms are still unclear. Prior depression or depressive symptoms appeared to be strong predictors of all-cause mortality or death from breast cancer in this sample. However, irrespective of how depression or EDS were conceptualized, neither depressive symptoms nor clinically significant depression influenced the risk of death among women with colorectal cancer [ 18 ]. Though depression often remains a robust predictor of death from any cause for older adults, chronic diseases and health behaviors may be meaningful contributors as well [ 64 , 65 ].
A primary surmised mechanism underlying mood disorder symptomatology and mortality risk proposes that for individuals living with minor, moderate, or major depression, the potential for hypothalamic-pituitary-adrenal (HPA) axis dysregulation is exacerbated, wherein neuroendocrine and inflammatory modulation can affect overall biological functioning and wellness [ 69 ]. Nevertheless, our study did not indicate that depression by itself was associated with all-cause mortality. In contrast, antidepressant use was an independent predictor of all-cause mortality, even after adjusting for key potential confounders including lifestyle and health-related factors. This coupled with persistent depressive symptoms or an increase in symptoms and medications over time simultaneously were also linked to all-cause mortality independently of measured risk factors. Evidence from the WHI study as a whole, including a much larger sample than the one used in our present study, suggests that depression or antidepressant use may increase the vulnerability of postmenopausal women to age-related health problems can increase risks for frailty [ 7 ], all-cause and cause-specific mortality [ 18 – 20 ]. Thus, our present sub-study may be underpowered to detect an association between EDS and mortality as compared to the larger study.
Several studies have investigated antidepressant use and mortality. The results are mixed with two showing lower and one showing higher mortality in treated patients. Acharya et al. found that anti-depressant medication treatment lowered all-cause mortality in a sample of veterans especially among those with increased cardiovascular risk [ 70 ]. Qian et al. examined how depression diagnosis and antidepressant use was associated with mortality in a sample of young Social Security Disability Insurance recipients [ 71 ]. They concluded that antidepressant treatment lowered mortality significantly [ 71 ]. Hansen et al. investigated second-generation antidepressants and concluded that in their fully adjusted models, antidepressant use could slightly increase all-cause mortality [ 72 ].
Finally, our study showed that GrimAge is a potential mediator explaining the association between antidepressant use and all-cause mortality, although only before we adjusted for lifestyle and health-related factors. Given that GrimAge was conceived of as an independent predictor for all-cause mortality risk, this finding is consistent with our hypothesis, and shows that this clock is specifically explaining some of the TE of antidepressant use on mortality. Furthermore, it has recently been argued that GrimAge was developed as a measure of healthspan/lifespan [ 73 ]. Therefore, it may be more sensitive to capturing the impacts of the environment than DNAm predictors of chronological age. As people age, their DNAm predictors (e.g. Horvath, Hannum clocks) become more tightly coded and less susceptible to environmental inputs [ 73 ]. Future studies should examine which parts of this clock may be contributing to this mediating effect.
Strengths and limitations
A strength of the study is that detailed data were collected at enrollment on all WHI participants, facilitating the evaluation of hypothesized relationships while taking key confounders into consideration. Second, it is possible to generalize study findings to postmenopausal women of diverse racial/ethnic backgrounds that reside in various geographical areas within the U.S.
However, there are several limitations of our study. First, ancillary study data were analyzed using a subsample of the original WHI participants, with missing data on exposure, mediator, moderator, outcome, and covariate variables potentially resulting in selection bias. In fact, the WHI includes participants who were recruited in a voluntary basis. It is not representative of all postmenopausal women in the U.S. Since there was no complex sampling design, and no weights in the original WHI study, there was no need to re-weight the analyses for this particular study which is based on a sub-sample of WHI. Third, measurement error is a notable issue when variables are assessed by self-report. Specifically, EDS was determined using a threshold on a screening instrument rather than a full diagnosis of depression and its severity. Therefore, it is still possible that use of antidepressants is a marker of severity of depression independently of EDS. Fourth, residual confounding due to unmeasured confounders as well as confounding by indication remain as concerns for observational study designs. Without repeated measurements of epigenetic age acceleration, depressive symptoms, and antidepressant use, reverse causality cannot be ruled out as an alternative explanation. Also, a causal relationship between epigenetic age acceleration, depressive symptoms, and antidepressant use, can only be definitively established in the context of an experimental design. Finally, the WHI is not population-based but involves volunteers at clinical centers, specifically targeting postmenopausal women. Therefore, its generalizability to men as well as younger and relatively less educated women is not possible.
- Conclusions
In summary, among postmenopausal women, higher GrimAge age acceleration partially explained the relationship between antidepressant use and increased all-cause mortality risk, though only prior to controlling for lifestyle and health-related factors. Antidepressant use and epigenetic age acceleration independently predicted increased all-cause mortality risk. Further studies are needed in comparable and diverse populations.
- Supplementary Materials
Supplementary Figure 1
Supplementary table 1.
- Author Contributions
MAB: Study concept, plan of analysis, data management, statistical analysis, literature search and review, write-up of the manuscript, revision of the manuscript. HAB: Study concept, plan of analysis, data management, statistical analysis, literature search and review, write-up of parts of the manuscript, revision of the manuscript. JA: Literature search and review, write-up of parts of the manuscript, revision of the manuscript. MFG: Data acquisition, data management, write-up of parts of the manuscript, revision of the manuscript. SH: Data acquisition, data management, write-up of parts of the manuscript, revision of the manuscript. AL: Data acquisition, data management, write-up of parts of the manuscript, revision of the manuscript. ASZ: Plan of analysis, literature search and review, write-up of parts of the manuscript, revision of the manuscript. AHS: Plan of analysis, literature search and review, write-up of parts of the manuscript, revision of the manuscript. SYJ: Plan of analysis, literature search and review, write-up of parts of the manuscript, revision of the manuscript. SWS: Plan of analysis, literature search and review, write-up of parts of the manuscript, revision of the manuscript. RC: Plan of analysis, literature search and review, write-up of parts of the manuscript, revision of the manuscript. ABZ: Plan of analysis, write-up of parts of the manuscript, revision of the manuscript. RLB: Study concept, data acquisition, plan of analysis, write-up of parts of the manuscript, revision of the manuscript.
- Conflicts of Interest
The authors declare no conflicts of interest related to this study.
- Ethical Statement and Consent
The WHI study received institutional review board approval with informed consent from all participating clinical centers.
The manuscript was supported in part by the Intramural Research Program of the National Institute on Aging in Baltimore, Maryland. The WHI program is funded by the National Heart, Lung, and Blood Institute, National Institutes of Health, U.S. Department of Health and Human Services through 75N92021D00001, 75N92021D00002, 75N92021D00003, 75N92021D00004, 75N92021D00005. A.H.S. was funded by grant RF1AG074345 from the National Institute on Aging. The authors thank the WHI investigators and staff for their dedication, and the study participants for making the program possible. A listing of WHI investigators can be found at: https://www-whi-org.s3.us-west-2.amazonaws.com/wp-content/uploads/WHI-Investigator-Short-List.pdf . The sponsors did not play a role in the study design, collection, analysis, and interpretation of data, in the writing of the report or in the decision to submit the article for publication. Dr. Hind A. Beydoun worked on this manuscript outside her tour of duty at the U.S. Department of Veterans Affairs.
- 1. Jaeschke K, Hanna F, Ali S, Chowdhary N, Dua T, Charlson F. Global estimates of service coverage for severe mental disorders: findings from the WHO Mental Health Atlas 2017. Glob Ment Health (Camb). 2021; 8:e27. https://doi.org/10.1017/gmh.2021.19 [ PubMed ]
- 2. Zenebe Y, Akele B, W/Selassie M, Necho M. Prevalence and determinants of depression among old age: a systematic review and meta-analysis. Ann Gen Psychiatry. 2021; 20:55. https://doi.org/10.1186/s12991-021-00375-x [ PubMed ]
- 3. Huang T, Balasubramanian R, Yao Y, Clish CB, Shadyab AH, Liu B, Tworoger SS, Rexrode KM, Manson JE, Kubzansky LD, Hankinson SE. Associations of depression status with plasma levels of candidate lipid and amino acid metabolites: a meta-analysis of individual data from three independent samples of US postmenopausal women. Mol Psychiatry. 2021; 26:3315–27. https://doi.org/10.1038/s41380-020-00870-9 [ PubMed ]
- 4. Goveas JS, Espeland MA, Woods NF, Wassertheil-Smoller S, Kotchen JM. Depressive symptoms and incidence of mild cognitive impairment and probable dementia in elderly women: the Women's Health Initiative Memory Study. J Am Geriatr Soc. 2011; 59:57–66. https://doi.org/10.1111/j.1532-5415.2010.03233.x [ PubMed ]
- 5. Pratt LA, Brody DJ, Gu Q. Antidepressant Use Among Persons Aged 12 and Over:United States,2011-2014. NCHS Data Brief. 2017; 1–8. [ PubMed ]
- 6. Kiridly-Calderbank JF, Sturgeon SR, Kroenke CH, Reeves KW. Antidepressant Use and Risk of Colorectal Cancer in the Women's Health Initiative. Cancer Epidemiol Biomarkers Prev. 2018; 27:892–8. https://doi.org/10.1158/1055-9965.EPI-17-1035 [ PubMed ]
- 7. Lakey SL, LaCroix AZ, Gray SL, Borson S, Williams CD, Calhoun D, Goveas JS, Smoller JW, Ockene JK, Masaki KH, Coday M, Rosal MC, Woods NF. Antidepressant use, depressive symptoms, and incident frailty in women aged 65 and older from the Women's Health Initiative Observational Study. J Am Geriatr Soc. 2012; 60:854–61. https://doi.org/10.1111/j.1532-5415.2012.03940.x [ PubMed ]
- 8. Ma Y, Balasubramanian R, Pagoto SL, Schneider KL, Hébert JR, Phillips LS, Goveas JS, Culver AL, Olendzki BC, Beck J, Smoller JW, Sepavich DM, Ockene JK, et al. Relations of depressive symptoms and antidepressant use to body mass index and selected biomarkers for diabetes and cardiovascular disease. Am J Public Health. 2013; 103:e34–43. https://doi.org/10.2105/AJPH.2013.301394 [ PubMed ]
- 9. Stanford FC, Cena H, Biino G, Umoren O, Jimenez M, Freeman MP, Shadyab AH, Wild RA, Womack CR, Banack HR, Manson JE. The association between weight-promoting medication use and weight gain in postmenopausal women: findings from the Women's Health Initiative. Menopause. 2020; 27:1117–25. https://doi.org/10.1097/GME.0000000000001589 [ PubMed ]
- 10. Frisard C, Gu X, Whitcomb B, Ma Y, Pekow P, Zorn M, Sepavich D, Balasubramanian R. Marginal structural models for the estimation of the risk of Diabetes Mellitus in the presence of elevated depressive symptoms and antidepressant medication use in the Women's Health Initiative observational and clinical trial cohorts. BMC Endocr Disord. 2015; 15:56. https://doi.org/10.1186/s12902-015-0049-7 [ PubMed ]
- 11. Ma Y, Balasubramanian R, Pagoto SL, Schneider KL, Culver AL, Olendzki B, Tinker L, Liu S, Safford M, Sepavich DM, Rosal MC, Ockene JK, Carnethon M, et al. Elevated depressive symptoms, antidepressant use, and diabetes in a large multiethnic national sample of postmenopausal women. Diabetes Care. 2011; 34:2390–2. https://doi.org/10.2337/dc11-1223 [ PubMed ]
- 12. Zambrana RE, López L, Dinwiddie GY, Ray RM, Eaton CB, Phillips LS, Wassertheil-Smoller S. Association of Baseline Depressive Symptoms with Prevalent and Incident Pre-Hypertension and Hypertension in Postmenopausal Hispanic Women: Results from the Women's Health Initiative. PLoS One. 2016; 11:e0152765. https://doi.org/10.1371/journal.pone.0152765 [ PubMed ]
- 13. Wassertheil-Smoller S, Shumaker S, Ockene J, Talavera GA, Greenland P, Cochrane B, Robbins J, Aragaki A, Dunbar-Jacob J. Depression and cardiovascular sequelae in postmenopausal women. The Women's Health Initiative (WHI). Arch Intern Med. 2004; 164:289–98. https://doi.org/10.1001/archinte.164.3.289 [ PubMed ]
- 14. Marano CM, Workman CI, Lyman CH, Munro CA, Kraut MA, Smith GS. Structural imaging in late-life depression: association with mood and cognitive responses to antidepressant treatment. Am J Geriatr Psychiatry. 2015; 23:4–12. https://doi.org/10.1016/j.jagp.2013.10.001 [ PubMed ]
- 15. Spangler L, Scholes D, Brunner RL, Robbins J, Reed SD, Newton KM, Melville JL, Lacroix AZ. Depressive symptoms, bone loss, and fractures in postmenopausal women. J Gen Intern Med. 2008; 23:567–74. https://doi.org/10.1007/s11606-008-0525-0 [ PubMed ]
- 16. Leaney AA, Lyttle JR, Segan J, Urquhart DM, Cicuttini FM, Chou L, Wluka AE. Antidepressants for hip and knee osteoarthritis. Cochrane Database Syst Rev. 2022; 10:CD012157. https://doi.org/10.1002/14651858.CD012157.pub2 [ PubMed ]
- 17. Beydoun HA, Saquib N, Wallace RB, Chen JC, Coday M, Naughton MJ, Beydoun MA, Shadyab AH, Zonderman AB, Brunner RL. Psychotropic medication use and Parkinson's disease risk amongst older women. Ann Clin Transl Neurol. 2022; 9:1163–76. https://doi.org/10.1002/acn3.51614 [ PubMed ]
- 18. Liang X, Hendryx M, Qi L, Lane D, Luo J. Association between prediagnosis depression and mortality among postmenopausal women with colorectal cancer. PLoS One. 2020; 15:e0244728. https://doi.org/10.1371/journal.pone.0244728 [ PubMed ]
- 19. Liang X, Margolis KL, Hendryx M, Reeves K, Wassertheil-Smoller S, Weitlauf J, Danhauer SC, Chlebowski RT, Caan B, Qi L, Lane D, Lavasani S, Luo J. Effect of depression before breast cancer diagnosis on mortality among postmenopausal women. Cancer. 2017; 123:3107–15. https://doi.org/10.1002/cncr.30688 [ PubMed ]
- 20. Smoller JW, Allison M, Cochrane BB, Curb JD, Perlis RH, Robinson JG, Rosal MC, Wenger NK, Wassertheil-Smoller S. Antidepressant use and risk of incident cardiovascular morbidity and mortality among postmenopausal women in the Women's Health Initiative study. Arch Intern Med. 2009; 169:2128–39. https://doi.org/10.1001/archinternmed.2009.436 [ PubMed ]
- 21. Ormel J, Spinhoven P, de Vries YA, Cramer AOJ, Siegle GJ, Bockting CLH, Hollon SD. The antidepressant standoff: why it continues and how to resolve it. Psychol Med. 2020; 50:177–86. https://doi.org/10.1017/S0033291719003295 [ PubMed ]
- 22. Lucassen PJ, Meerlo P, Naylor AS, van Dam AM, Dayer AG, Fuchs E, Oomen CA, Czéh B. Regulation of adult neurogenesis by stress, sleep disruption, exercise and inflammation: Implications for depression and antidepressant action. Eur Neuropsychopharmacol. 2010; 20:1–17. https://doi.org/10.1016/j.euroneuro.2009.08.003 [ PubMed ]
- 23. Fourrier C, Sampson E, Mills NT, Baune BT. Anti-inflammatory treatment of depression: study protocol for a randomised controlled trial of vortioxetine augmented with celecoxib or placebo. Trials. 2018; 19:447. https://doi.org/10.1186/s13063-018-2829-7 [ PubMed ]
- 24. Mease P, Kuritzky L, Wright WL, Mallick-Searle T, Fountaine R, Yang R, Sadrarhami M, Faison W, Johnston E, Viktrup L. Efficacy and safety of tanezumab, NSAIDs, and placebo in patients with moderate to severe hip or knee osteoarthritis and a history of depression, anxiety, or insomnia: post-hoc analysis of phase 3 trials. Curr Med Res Opin. 2022; 38:1909–22. https://doi.org/10.1080/03007995.2022.2113689 [ PubMed ]
- 25. Nogo D, Nazal H, Song Y, Teopiz KM, Ho R, McIntyre RS, Lui LMW, Rosenblat JD. A review of potential neuropathological changes associated with ketamine. Expert Opin Drug Saf. 2022; 21:813–31. https://doi.org/10.1080/14740338.2022.2071867 [ PubMed ]
- 26. Zhong X, Harris G, Smirnova L, Zufferey V, Sá RCD, Baldino Russo F, Baleeiro Beltrao Braga PC, Chesnut M, Zurich MG, Hogberg HT, Hartung T, Pamies D. Antidepressant Paroxetine Exerts Developmental Neurotoxicity in an iPSC-Derived 3D Human Brain Model. Front Cell Neurosci. 2020; 14:25. https://doi.org/10.3389/fncel.2020.00025 [ PubMed ]
- 27. Alnefeesi Y, Tamura JK, Lui LMW, Jawad MY, Ceban F, Ling S, Nasri F, Rosenblat JD, McIntyre RS. Trace amine-associated receptor 1 (TAAR1): Potential application in mood disorders: A systematic review. Neurosci Biobehav Rev. 2021; 131:192–210. https://doi.org/10.1016/j.neubiorev.2021.09.020 [ PubMed ]
- 28. Horvath S, Oshima J, Martin GM, Lu AT, Quach A, Cohen H, Felton S, Matsuyama M, Lowe D, Kabacik S, Wilson JG, Reiner AP, Maierhofer A, et al. Epigenetic clock for skin and blood cells applied to Hutchinson Gilford Progeria Syndrome and ex vivo studies. Aging (Albany NY). 2018; 10:1758–75. https://doi.org/10.18632/aging.101508 [ PubMed ]
- 29. Pottinger TD, Khan SS, Zheng Y, Zhang W, Tindle HA, Allison M, Wells G, Shadyab AH, Nassir R, Martin LW, Manson JE, Lloyd-Jones DM, Greenland P, et al. Association of cardiovascular health and epigenetic age acceleration. Clin Epigenetics. 2021; 13:42. https://doi.org/10.1186/s13148-021-01028-2 [ PubMed ]
- 30. Shadyab AH, McEvoy LK, Horvath S, Whitsel EA, Rapp SR, Espeland MA, Resnick SM, Manson JE, Chen JC, Chen BH, Li W, Hayden KM, Bao W, et al. Association of Epigenetic Age Acceleration With Incident Mild Cognitive Impairment and Dementia Among Older Women. J Gerontol A Biol Sci Med Sci. 2022; 77:1239–44. https://doi.org/10.1093/gerona/glab245 [ PubMed ]
- 31. Discacciati A, Bellavia A, Lee JJ, Mazumdar M, Valeri L. Med4way: a Stata command to investigate mediating and interactive mechanisms using the four-way effect decomposition. Int J Epidemiol. 2018. [Epub ahead of print]. https://doi.org/10.1093/ije/dyy236 [ PubMed ]
- 32. Pamplin Ii JR, Rudolph KE, Keyes KM, Susser ES, Bates LM. Investigating a Paradox: Toward a Better Understanding of the Relationships Between Racial Group Membership, Stress, and Major Depressive Disorder. Am J Epidemiol. 2023; 192:1845–53. https://doi.org/10.1093/aje/kwad128 [ PubMed ]
- 33. Karriker-Jaffe KJ, Foshee VA, Ennett ST. Examining how neighborhood disadvantage influences trajectories of adolescent violence: a look at social bonding and psychological distress. J Sch Health. 2011; 81:764–73. https://doi.org/10.1111/j.1746-1561.2011.00656.x [ PubMed ]
- 34. Beydoun MA, Hossain S, Chitrala KN, Tajuddin SM, Beydoun HA, Evans MK, Zonderman AB. Association between epigenetic age acceleration and depressive symptoms in a prospective cohort study of urban-dwelling adults. J Affect Disord. 2019; 257:64–73. https://doi.org/10.1016/j.jad.2019.06.032 [ PubMed ]
- 35. Cruz-Almeida Y, Johnson A, Meng L, Sinha P, Rani A, Yoder S, Huo Z, Foster TC, Fillingim RB. Epigenetic age predictors in community-dwelling adults with high impact knee pain. Mol Pain. 2022; 18:17448069221118004. https://doi.org/10.1177/17448069221118004 [ PubMed ]
- 36. Vetter VM, Drewelies J, Sommerer Y, Kalies CH, Regitz-Zagrosek V, Bertram L, Gerstorf D, Demuth I. Epigenetic aging and perceived psychological stress in old age. Transl Psychiatry. 2022; 12:410. https://doi.org/10.1038/s41398-022-02181-9 [ PubMed ]
- 37. Wolf EJ, Chen CD, Zhao X, Zhou Z, Morrison FG, Daskalakis NP, Stone A, Schichman S, Grenier JG, Fein-Schaffer D, Huber BR, Abraham CR, Miller MW, Logue MW, and Traumatic Stress Brain Research Group. Klotho, PTSD, and advanced epigenetic age in cortical tissue. Neuropsychopharmacology. 2021; 46:721–30. https://doi.org/10.1038/s41386-020-00884-5 [ PubMed ]
- 38. Appleton AA, Lin B, Kennedy EM, Holdsworth EA. Maternal depression and adverse neighbourhood conditions during pregnancy are associated with gestational epigenetic age deceleration. Epigenetics. 2022; 17:1905–19. https://doi.org/10.1080/15592294.2022.2090657 [ PubMed ]
- 39. Verhoeven JE, Yang R, Wolkowitz OM, Bersani FS, Lindqvist D, Mellon SH, Yehuda R, Flory JD, Lin J, Abu-Amara D, Makotkine I, Marmar C, Jett M, Hammamieh R. Epigenetic Age in Male Combat-Exposed War Veterans: Associations with Posttraumatic Stress Disorder Status. Mol Neuropsychiatry. 2018; 4:90–9. https://doi.org/10.1159/000491431 [ PubMed ]
- 40. Abdelwadoud M, Collen J, Edwards H, Mullins CD, Jobe SL, Labra C, Capaldi VF, Assefa SZ, Williams SG, Drake CL, Albrecht JS, Manber R, Mahoney A, et al. Engaging Stakeholders to Optimize Sleep Disorders' Management in the U.S. Military: A Qualitative Analysis. Mil Med. 2022; 187:e941–7. https://doi.org/10.1093/milmed/usab341 [ PubMed ]
- 41. Horvath S, Gurven M, Levine ME, Trumble BC, Kaplan H, Allayee H, Ritz BR, Chen B, Lu AT, Rickabaugh TM, Jamieson BD, Sun D, Li S, et al. An epigenetic clock analysis of race/ethnicity, sex, and coronary heart disease. Genome Biol. 2016; 17:171. https://doi.org/10.1186/s13059-016-1030-0 [ PubMed ]
- 42. Kim ES, Fong K, Lee L, Spiro A, Schwartz J, Whitsel E, Horvath S, Wang C, Hou L, Baccarelli AA, Li Y, Stewart J, Manson JE, et al. Optimism is not associated with two indicators of DNA methylation aging. Aging (Albany NY). 2019; 11:4970–89. https://doi.org/10.18632/aging.102090 [ PubMed ]
- 43. Lu AT, Xue L, Salfati EL, Chen BH, Ferrucci L, Levy D, Joehanes R, Murabito JM, Kiel DP, Tsai PC, Yet I, Bell JT, Mangino M, et al. GWAS of epigenetic aging rates in blood reveals a critical role for TERT. Nat Commun. 2018; 9:387. https://doi.org/10.1038/s41467-017-02697-5 [ PubMed ]
- 44. Marioni RE, Shah S, McRae AF, Chen BH, Colicino E, Harris SE, Gibson J, Henders AK, Redmond P, Cox SR, Pattie A, Corley J, Murphy L, et al. DNA methylation age of blood predicts all-cause mortality in later life. Genome Biol. 2015; 16:25. https://doi.org/10.1186/s13059-015-0584-6 [ PubMed ]
- 45. Chen BH, Marioni RE, Colicino E, Peters MJ, Ward-Caviness CK, Tsai PC, Roetker NS, Just AC, Demerath EW, Guan W, Bressler J, Fornage M, Studenski S, et al. DNA methylation-based measures of biological age: meta-analysis predicting time to death. Aging (Albany NY). 2016; 8:1844–65. https://doi.org/10.18632/aging.101020 [ PubMed ]
- 46. Horvath S, Raj K. DNA methylation-based biomarkers and the epigenetic clock theory of ageing. Nat Rev Genet. 2018; 19:371–84. https://doi.org/10.1038/s41576-018-0004-3 [ PubMed ]
- 47. Anderson GL, Manson J, Wallace R, Lund B, Hall D, Davis S, Shumaker S, Wang CY, Stein E, Prentice RL. Implementation of the Women's Health Initiative study design. Ann Epidemiol. 2003; 13:S5–17. https://doi.org/10.1016/s1047-2797(03)00043-7 [ PubMed ]
- 48. Hays J, Hunt JR, Hubbell FA, Anderson GL, Limacher M, Allen C, Rossouw JE. The Women's Health Initiative recruitment methods and results. Ann Epidemiol. 2003; 13:S18–77. https://doi.org/10.1016/s1047-2797(03)00042-5 [ PubMed ]
- 49. Cauley JA, Hovey KM, Stone KL, Andrews CA, Barbour KE, Hale L, Jackson RD, Johnson KC, LeBlanc ES, Li W, Zaslavsky O, Ochs-Balcom H, Wactawski-Wende J, Crandall CJ. Characteristics of Self-Reported Sleep and the Risk of Falls and Fractures: The Women's Health Initiative (WHI). J Bone Miner Res. 2019; 34:464–74. https://doi.org/10.1002/jbmr.3619 [ PubMed ]
- 50. Grieshober L, Wactawski-Wende J, Hageman Blair R, Mu L, Liu J, Nie J, Carty CL, Hale L, Kroenke CH, LaCroix AZ, Reiner AP, Ochs-Balcom HM. A Cross-Sectional Analysis of Telomere Length and Sleep in the Women's Health Initiative. Am J Epidemiol. 2019; 188:1616–26. https://doi.org/10.1093/aje/kwz134 [ PubMed ]
- 51. Levine ME, Hosgood HD, Chen B, Absher D, Assimes T, Horvath S. DNA methylation age of blood predicts future onset of lung cancer in the women's health initiative. Aging (Albany NY). 2015; 7:690–700. https://doi.org/10.18632/aging.100809 [ PubMed ]
- 52. Patel KV, Cochrane BB, Turk DC, Bastian LA, Haskell SG, Woods NF, Zaslavsky O, Wallace RB, Kerns RD. Association of Pain With Physical Function, Depressive Symptoms, Fatigue, and Sleep Quality Among Veteran and non-Veteran Postmenopausal Women. Gerontologist. 2016 (Suppl 1); 56:S91–101. https://doi.org/10.1093/geront/gnv670 [ PubMed ]
- 53. Danhauer SC, Brenes GA, Levine BJ, Young L, Tindle HA, Addington EL, Wallace RB, Naughton MJ, Garcia L, Safford M, Kim MM, LeBlanc ES, Snively BM, et al. Variability in sleep disturbance, physical activity and quality of life by level of depressive symptoms in women with Type 2 diabetes. Diabet Med. 2019; 36:1149–57. https://doi.org/10.1111/dme.13878 [ PubMed ]
- 54. Liu Z, Chen BH, Assimes TL, Ferrucci L, Horvath S, Levine ME. The role of epigenetic aging in education and racial/ethnic mortality disparities among older U.S. Women. Psychoneuroendocrinology. 2019; 104:18–24. https://doi.org/10.1016/j.psyneuen.2019.01.028 [ PubMed ]
- 55. Barma M, Khan F, Price RJG, Donnan PT, Messow CM, Ford I, McConnachie A, Struthers AD, McMurdo MET, Witham MD. Association between GDF-15 levels and changes in vascular and physical function in older patients with hypertension. Aging Clin Exp Res. 2017; 29:1055–9. https://doi.org/10.1007/s40520-016-0636-0 [ PubMed ]
- 56. Jung SY, Ho G, Rohan T, Strickler H, Bea J, Papp J, Sobel E, Zhang ZF, Crandall C. Interaction of insulin-like growth factor-I and insulin resistance-related genetic variants with lifestyle factors on postmenopausal breast cancer risk. Breast Cancer Res Treat. 2017; 164:475–95. https://doi.org/10.1007/s10549-017-4272-y [ PubMed ]
- 57. VanderWeele TJ. A unification of mediation and interaction: a 4-way decomposition. Epidemiology. 2014; 25:749–61. https://doi.org/10.1097/EDE.0000000000000121 [ PubMed ]
- 58. Valeri L, Vanderweele TJ. Mediation analysis allowing for exposure-mediator interactions and causal interpretation: theoretical assumptions and implementation with SAS and SPSS macros. Psychol Methods. 2013; 18:137–50. https://doi.org/10.1037/a0031034 [ PubMed ]
- 59. Robins JM, Greenland S. Identifiability and exchangeability for direct and indirect effects. Epidemiology. 1992; 3:143–55. https://doi.org/10.1097/00001648-199203000-00013 [ PubMed ]
- 60. Lee KJ, Carlin JB. Multiple imputation for missing data: fully conditional specification versus multivariate normal imputation. Am J Epidemiol. 2010; 171:624–32. https://doi.org/10.1093/aje/kwp425 [ PubMed ]
- 61. Han LKM, Aghajani M, Clark SL, Chan RF, Hattab MW, Shabalin AA, Zhao M, Kumar G, Xie LY, Jansen R, Milaneschi Y, Dean B, Aberg KA, et al. Epigenetic Aging in Major Depressive Disorder. Am J Psychiatry. 2018; 175:774–82. https://doi.org/10.1176/appi.ajp.2018.17060595 [ PubMed ]
- 62. Protsenko E, Yang R, Nier B, Reus V, Hammamieh R, Rampersaud R, Wu GWY, Hough CM, Epel E, Prather AA, Jett M, Gautam A, Mellon SH, Wolkowitz OM. "GrimAge," an epigenetic predictor of mortality, is accelerated in major depressive disorder. Transl Psychiatry. 2021; 11:193. https://doi.org/10.1038/s41398-021-01302-0 [ PubMed ]
- 63. Beydoun MA, Beydoun HA, Noren Hooten N, Maldonado AI, Weiss J, Evans MK, Zonderman AB. Epigenetic clocks and their association with trajectories in perceived discrimination and depressive symptoms among US middle-aged and older adults. Aging (Albany NY). 2022; 14:5311–44. https://doi.org/10.18632/aging.204150 [ PubMed ]
- 64. Pratt LA, Druss BG, Manderscheid RW, Walker ER. Excess mortality due to depression and anxiety in the United States: results from a nationally representative survey. Gen Hosp Psychiatry. 2016; 39:39–45. https://doi.org/10.1016/j.genhosppsych.2015.12.003 [ PubMed ]
- 65. Rethorst CD, Leonard D, Barlow CE, Willis BL, Trivedi MH, DeFina LF. Effects of depression, metabolic syndrome, and cardiorespiratory fitness on mortality: results from the Cooper Center Longitudinal Study. Psychol Med. 2017; 47:2414–20. https://doi.org/10.1017/S0033291717000897 [ PubMed ]
- 66. Paljärvi T, Tiihonen J, Lähteenvuo M, Tanskanen A, Fazel S, Taipale H. Mortality in psychotic depression: 18-year follow-up study. Br J Psychiatry. 2023; 222:37–43. https://doi.org/10.1192/bjp.2022.140 [ PubMed ]
- 67. Xia W, Jiang H, Di H, Feng J, Meng X, Xu M, Gan Y, Liu T, Lu Z. Association between self-reported depression and risk of all-cause mortality and cause-specific mortality. J Affect Disord. 2022; 299:353–8. https://doi.org/10.1016/j.jad.2021.12.018 [ PubMed ]
- 68. Guan S, Fang X, Gu X, Zhang Z, Tang Z, Wu X, Liu H, Wang C. The link of depression, untreated hypertension, and diabetes with mortality in postmenopausal women: A cohort study. Clin Exp Hypertens. 2021; 43:1–6. https://doi.org/10.1080/10641963.2020.1790584 [ PubMed ]
- 69. Zannas AS, Arloth J, Carrillo-Roa T, Iurato S, Röh S, Ressler KJ, Nemeroff CB, Smith AK, Bradley B, Heim C, Menke A, Lange JF, Brückl T, et al. Lifetime stress accelerates epigenetic aging in an urban, African American cohort: relevance of glucocorticoid signaling. Genome Biol. 2015; 16:266. https://doi.org/10.1186/s13059-015-0828-5 [ PubMed ]
- 70. Acharya T, Acharya S, Tringali S, Huang J. Association of antidepressant and atypical antipsychotic use with cardiovascular events and mortality in a veteran population. Pharmacotherapy. 2013; 33:1053–61. https://doi.org/10.1002/phar.1311 [ PubMed ]
- 71. Qian J, Simoni-Wastila L, Rattinger GB, Lehmann S, Langenberg P, Zuckerman IH, Terrin M. Associations of depression diagnosis and antidepressant treatment with mortality among young and disabled Medicare beneficiaries with COPD. Gen Hosp Psychiatry. 2013; 35:612–8. https://doi.org/10.1016/j.genhosppsych.2013.06.005 [ PubMed ]
- 72. Hansen RA, Khodneva Y, Glasser SP, Qian J, Redmond N, Safford MM. Antidepressant Medication Use and Its Association With Cardiovascular Disease and All-Cause Mortality in the Reasons for Geographic and Racial Differences in Stroke (REGARDS) Study. Ann Pharmacother. 2016; 50:253–61. https://doi.org/10.1177/1060028015625284 [ PubMed ]
- 73. Zannas AS, Linnstaedt SD, An X, Stevens JS, Harnett NG, Roeckner AR, Oliver KI, Rubinow DR, Binder EB, Koenen KC, Ressler KJ, McLean SA. Epigenetic aging and PTSD outcomes in the immediate aftermath of trauma. Psychol Med. 2023; 53:7170–9. https://doi.org/10.1017/S0033291723000636 [ PubMed ]
Corresponding Author
May A. Beydoun [email protected]
Paper Sections
Log in using your username and password
- Search More Search for this keyword Advanced search
- Latest content
- For authors
- Browse by collection
- BMJ Journals More You are viewing from: Google Indexer
You are here
- Volume 14, Issue 5
- Protocol for process evaluation of ARTEMIS cluster randomised controlled trial: an intervention for management of depression and suicide among adolescents living in slums in India
- Article Text
- Article info
- Citation Tools
- Rapid Responses
- Article metrics

- http://orcid.org/0000-0002-6236-1317 Ankita Mukherjee 1 ,
- http://orcid.org/0000-0002-0329-7271 Sandhya Kanaka Yatirajula 1 ,
- Sudha Kallakuri 2 ,
- http://orcid.org/0000-0002-9005-2810 Srilatha Paslawar 2 ,
- Heidi Lempp 3 ,
- Usha Raman 4 ,
- Ashok Kumar 5 ,
- Beverley M Essue 6 ,
- Rajesh Sagar 7 ,
- Renu Singh 8 ,
- http://orcid.org/0000-0002-6898-3870 David Peiris 9 , 10 ,
- Robyn Norton 9 , 11 ,
- Graham Thornicroft 12 ,
- http://orcid.org/0000-0001-6835-6175 Pallab Kumar Maulik 1 , 10
- 1 The George Institute for Global Health India , New Delhi , India
- 2 The George Institute for Global Health , Hyderabad , India
- 3 Inflammation Biology , King’s College London , London , UK
- 4 Department of Communication , University of Hyderabad , Hyderabad , India
- 5 Dr.A.V. Baliga Memorial Trust , New Delhi , India
- 6 Institute of Health Policy, Management and Evaluation , University of Toronto , Toronto , Ontario , Canada
- 7 Department of Psychiatry , All India Institute of Medical Sciences , New Delhi , India
- 8 Young Lives, India , New Delhi , India
- 9 The George Institute for Global Health , Sydney , New South Wales , Australia
- 10 University of New South Wales , Sydney , New South Wales , Australia
- 11 Imperial College London , London , UK
- 12 Centre for Global Mental Health and Centre for Implementation Science , Institute of Psychiatry, Psychology and Neuroscience, King’s College London , London , UK
- Correspondence to Dr Pallab Kumar Maulik; pmaulik{at}georgeinstitute.org.in
Introduction There are around 250 million adolescents (10–19 years) in India. The prevalence of mental health-related morbidity among adolescents in India is approximately 7.3%. Vulnerable subpopulations among adolescents such as those living in slum communities are particularly at risk due to poor living conditions, financial difficulty and limited access to support services. Adolescents’ Resilience and Treatment nEeds for Mental Health in Indian Slums (ARTEMIS) is a cluster randomised controlled trial of an intervention that intends to improve the mental health of adolescents living in slum communities in India. The aim of this paper is to describe the process evaluation protocol for ARTEMIS trial. The process evaluation will help to explain the intervention outcomes and understand how and why the intervention worked or did not work. It will identify contextual factors, intervention barriers and facilitators and the adaptations required for optimising implementation.
Methods Case study method will be used and the data will include a mix of quantitative metrics and qualitative data. The UK Medical Research Council’s guidance on evaluating complex interventions, the Reach, Efficacy, Adoption, Implementation and Maintenance Framework and the Affordability, Practicability, Effectiveness and cost-effectiveness, Acceptability, Safety/Side Effects and, Equity criteria will be used to develop a conceptual framework and a priori codes for qualitative data analysis. Quantitative data will be analysed using descriptive statistics. Implementation fidelity will also be measured.
Discussion The process evaluation will provide an understanding of outcomes and causal mechanisms that influenced any change in trial outcomes.
Ethics and dissemination Ethics Committee of the George Institute for Global Health India (project number 17/2020) and the Research Governance and Integrity Team, Imperial College, London (ICREC reference number: 22IC7718) have provided ethics approval. The Health Ministry’s Screening Committee has approved to the study (ID 2020-9770).
Trial registration number CTRI/2022/02/040307.
- Adolescents
- Protocols & guidelines
- Implementation Science
- MENTAL HEALTH
- Clinical Trial
- Depression & mood disorders
This is an open access article distributed in accordance with the Creative Commons Attribution 4.0 Unported (CC BY 4.0) license, which permits others to copy, redistribute, remix, transform and build upon this work for any purpose, provided the original work is properly cited, a link to the licence is given, and indication of whether changes were made. See: https://creativecommons.org/licenses/by/4.0/ .
https://doi.org/10.1136/bmjopen-2023-081844
Statistics from Altmetric.com
Request permissions.
If you wish to reuse any or all of this article please use the link below which will take you to the Copyright Clearance Center’s RightsLink service. You will be able to get a quick price and instant permission to reuse the content in many different ways.
STRENGTHS AND LIMITATIONS OF THIS STUDY
This study will use existing implementation science theories and frameworks and qualitative and quantitative data will be triangulated to arrive at a comprehensive understanding of the intervention.
This study will be guided by the Medical Research Council framework for developing and evaluating complex interventions and the case study method will also be used.
Cases will be purposively selected based on maximum variation approach. Both intervention and control sites will be selected as cases to the enable comparison, to understand contextual factors and to avoid the Hawthorne effect.
Introduction
India has about 250 million adolescents aged 10–19 years, comprising nearly one-fifth of India’s population. 1 The burden of mental health problems in adolescents is a growing concern globally. 2 In India, the prevalence of psychiatric disorders among adolescents is estimated to be about 7.3%. 3 Self-harm and depressive disorders are the leading cause of death and disability in the age group 15–19 years. 4
Mental health interventions for adolescents are important as 50% of adult mental disorders have an onset before the age of 14 years. 5 Marginalised populations, such as adolescents living in slum communities, are particularly vulnerable because of additional stressors related to poor living conditions, financial stress and poor access to support services. 6 7
There has been limited research to test community-based mental health interventions for adolescents living in slums in India. A scoping review of mental health interventions among adolescents in India found that of the 11 interventions included in the review, 9 were school based, 1 community based and 1 was digital. 8 Most of the school-based programmes used a life skills curriculum that resulted in improvements in depressive symptoms and overall mental well-being. The review recommended the need for more interventions for early and out-of-school adolescents to ensure that the most vulnerable adolescents were not missed out. An intervention to build mental health and resilience delivered by community-based peers among highly disadvantaged young women living in urban slums in Dehradun, a city in north India, showed sustained improvements in anxiety and depression and attitudes to gender equality among study participants. 9 A randomised controlled trial of a 5-month resilience-based programme among rural adolescent girls through government schools in the state of Bihar, India, delivered by local women showed that girls receiving the intervention (vs controls) had better emotional resilience, self-efficacy, social-emotional assets, psychological well-being and social well-being. 10 Another school-based pilot study of of POD (Problems, Options, Do it), titled Adventures (a smartphone-based blended problem-solving game-based intervention for adolescents with or at risk of anxiety, depression and conduct difficulties) was helpful in managing their problems and stress and improving the mental health of 13–19 years enrolled in secondary schools in the Indian state of Goa. 11 The Adolescents’ Resilience and Treatment nEeds for Mental Health in Indian Slums (ARTEMIS) cluster randomised control trial (cRCT) is testing a community-based intervention to improve the mental health outcomes for adolescents living in urban slum clusters in India.
This paper presents the protocol for process evaluation of the ARTEMIS cRCT. ARTEMIS is a community-based cluster randomised control trial that aims to reduce depression and the risk of suicide among adolescents living in slums. The intervention will use a mental health stigma reduction campaign with adolescents and parents of the study cohort to improve attitudes towards mental health and improve help seeking. A technology-enabled strategy will be employed for screening, clinical diagnosis and management of mental health problems (depression, other significant emotional or medically unexplained complaints and suicide risk) among adolescents by primary care doctors and community-based non-physician health workers (NPHWs) (described in detail below).
Process evaluations provide critical inputs in understanding how interventions work in particular contexts and thus, support implementation planning beyond the trial setting. 12
The aims of the process evaluation are to:
Assess implementation fidelity and understand how the intervention was implemented.
Identify key contextual factors that impact intervention delivery and outcomes.
Understand perceptions of different stakeholders about effectiveness, acceptability and scalability of intervention components.
Identify key facilitators and barriers in implementation of the intervention.
Explain any adaptations to the intervention or intervention refinement during the study and their possible impact on the outcomes.
Conceptual framework
The process evaluation will be guided by the Medical Research Council (MRC) framework for developing and evaluating complex interventions. 13 14 The framework highlights the importance of examining the implementation, the mechanism of impact and their interaction with contextual factors to better understand how and why an intervention does or does not work. A recent update to the framework recognises that complex interventions have several phases including intervention development, feasibility assessment, implementation and evaluation, which may not always be sequential. 15 It recommends six core areas of inquiry at each phase before moving on to the next phase. They include (1) the intervention and its interaction with context; (2) the programme theory; (3) ways of engaging with diverse stakeholders; (4) ways of intervention refinement; (5) identifying key uncertainties and (6) economic considerations. The process evaluation will focus on the first five areas of inquiry, and the overall objectives have been framed to address these key areas.
Study setting
The ARTEMIS cRCT will be implemented in 60 slum clusters across two cities New Delhi and Vijayawada in India. In each city, 30 slum clusters will be included. For this study, a slum cluster is defined as slums within wards or geographical areas identified as slums/resettlement colonies. A ward is a local authority area, typically used for electoral purposes. In certain cities of India, such as Mumbai and New Delhi, a ward is an administrative unit of the city region; a city area is divided into zones, which in turn contains numerous wards. New Delhi is a metropolis and one of the largest cities in India with a population of about 17 million and an estimated slum population of about 2 million. 16 17 Vijayawada is one of the largest cities in the state of Andhra Pradesh with an urban population of over 1.0 million 18 19 and an estimated slum population is estimated to be about 0.5 million. 17 The most widely spoken language in Delhi is Hindi while in Vijayawada it is Telugu.
Patient and public involvement
Adolescent Expert Advisory Groups (AEAGs) have been formed at each site. This group was involved throughout the intervention development phase in providing inputs and suggestions. Their contributions were critical for the cocreation of the anti-stigma content for adolescents. During the formative phase, 34 meetings were held where the AEAG provided valuable feedback on ways to engage adolescents in the anti-stigma campaign.
Study design
The study will use a mixed-method, multiple case study design. Each slum cluster will constitute a ‘case’ for the study. A total of six cases or clusters will be purposively selected taking a maximum variation approach. Slums will be purposively selected to represent different contexts, coverage and reach of intervention as well as the ease or difficulty of implementing the intervention.
Intervention description
The ARTEMIS cRCT will test an intervention to address depression, increased risk of self-harm/suicide or other significant emotional or medically unexplained complaints among adolescents living in urban slums in two cities in India. ARTEMIS has two components. The first is a campaign that aims to reduce stigma related to mental health and improve attitudes and behaviours towards adolescents with depression or at increased risk of self-harm/suicide. The second is a technology-enabled mHealth platform with an integrated electronic decision support system (EDSS), to help primary care doctors and NPHWs to diagnose and treat adolescents at high risk of depression, self-harm or suicide.
Before randomisation and the start of the intervention, a team of trained field investigators will screen adolescents at high risk of depression or suicide using Patient Health Questionnaire-9 (PHQ-9), which is a standardised psychometric tool for screening depression and suicide risk in the community. 20 21 Adolescents who obtain a PHQ 9 score of ≥10 and/or a score of ≥2 in the suicide risk question on the PHQ-9 will be deemed as ‘high risk’. Due to the time delay between screening and randomisation and potential natural remission, a second screening will be carried out for adolescents identified as ‘high risk’ before the baseline is administered. This process will help identify the final list of adolescents at ‘high risk’ in all the clusters. The following information will be collected from the study cohort by the trained field investigators: sociodemographic characteristics, history of mental illness, treatment history, comorbid conditions, stressful events experienced in the previous year, resilience, knowledge attitude and behaviours related to mental health and stigma associated with help seeking. A detailed protocol of the trial has been published. 22
A theory of change model for the intervention was developed with key stakeholders during the formative phase of the study in 2021. The logic model for intervention has been provided ( figure 1 ).
- Download figure
- Open in new tab
- Download powerpoint
Logic model for ARTEMIS. IEC, Information Education,Communication; IT, Information Technology; ASHAs, Accredited Social Health Activists.
Intervention arm
In the intervention arm, the two components (1) the anti-stigma campaign and (2) the technology-enabled mHealth-based EDSS for screening, diagnosis and management of depression and self-harm will be delivered. ( figure 1 ).
Anti-stigma campaign
The anti-stigma campaign will include several Information Education and Communication (IEC) materials containing key messages around addressing mental health stigma, myths related to mental health, highlighting major stressors and their impact on mental health of adolescents and the importance of help seeking for mental health problems. The IEC materials will include posters, pamphlets, brochures, videos of persons with lived experience, animation videos, audio dramas, games, magic shows, rallies and street plays. The materials will be cocreated with adolescents from the study sites. AEAGs will be created in both locations consisting of adolescents from the community, with the purpose of involving them in working with the implementation team to cocreate the anti-stigma campaign. Details of the process of forming AEAGs and their engagement in shaping the anti-stigma campaign have been published elsewhere. 23
Peer-educators
Based on findings from the formative phase of the study, peer groups were established comprising adolescents from the community in the age group 13–19 years, some of whom were from the study cohort. Their role will be to provide support to adolescents who approach them to discuss problems and to promote mental health awareness in the community. All the members of the peer groups will be provided basic training by the research team on mental health, safe practices while using social media, addiction and substance use and promoting mental well-being through engaging in pleasurable activities such as listening to music, sharing problems with trusted individuals, having friends, exercising and eating healthily.
Technology-enabled EDSS
The EDSS platform has been developed to assist primary care doctors and NPHWs to screen, diagnose and manage depression, other significant emotional or medically unexplained complaints and suicide risk among adolescents. High-risk adolescents will be referred to the doctor electronically via the mHealth platform by the NPHWs. In each cluster, one doctor and several NPHWs will be trained. The NPHWs will be trained to use the EDSS to provide basic supportive advice to adolescents at high risk of depression or self-harm, refer them to the primary care doctors, and track treatment advice given by the doctors and will follow-up on treatment adherence. The NPHWs will make regular home visits to encourage adolescents at high risk and their families to seek help from the doctor. The NPHWs will also use the EDSS to clinically monitor the severity of high-risk individuals at 4 months and at 10 months after the start of the intervention. High-risk individuals will be provided referral cards to take to the primary care doctors. The primary care doctors will be trained to use an EDSS to clinically diagnose and manage adolescents with depression, other significant emotional or medically unexplained complaints and self-harm/suicide ideation based on WHO’s mhGAP (Mental Health Gap Action Programme)-Intervention Guide algorithm. 24 Adolescents needing specialist care will be referred to a psychiatrist in a government facility. The EDSS will also capture and store (on a cloud server) information regarding adolescents’ visits and the type of care provided by the primary care doctor—counselling/pharmacotherapy/combination of both/referral/follow-up. All data will be encrypted and protected using user-defined passwords. The information will be visible to the NPHWs, the project supervisors and research team only. A colour-coded traffic light system integrated in the application will assist the NPHWs to identify high-risk adolescents who need to be prioritised for follow-up. The EDSS will also provide them with simple questions tailored to the priority level as indicated by the traffic-light coding system, that they can ask the adolescents during follow-up to ensure appropriate treatment adherence or follow-up with doctors or mental health specialists.
Control arm
The control arm will receive enhanced usual care that will include general psychoeducation through pamphlets to raise awareness of common mental disorders in adolescents among the wider community. Parents/guardians of adolescents identified as high risk will be advised to consult a primary care doctor or mental health professional. Those with high scores on PHQ-9 (a score of ≥15 on PHQ9) and/or at high risk of suicide (suicide score ≥2), indicating severe depression and/or high risk of suicide will be asked to seek care immediately and will be given a list of health facilities (with contact details) where such treatment is available. All NPHWs will be asked to follow up with the adolescents and their families more intensively and will recommend that they visit a doctor/psychiatrist immediately.
Data collection
Quantitative data for the process evaluation will draw on metrics collected and sourced from the open-source medical record system or OpenMRS; ( OpenMRS.org ). The OpenMRS is a standardised community-driven open-source software for storing and processing medical record information. Quantitative data will be collected throughout the intervention via the mHealth platform. This will include data on screening outcome, treatment seeking, follow-ups and treatment provided for the high-risk cohort. Data will also be used to understand usage patterns of the EDSS by primary care doctors and NPHWs. The mHealth platform will capture this data in tablets used by NPHWs and primary care doctors for screening, treatment and follow-up of the high-risk cohort. These data will be used to assess reach, effectiveness and service utilisation. Data on competency and fidelity measures will also be captured throughout the intervention.
Qualitative data collection will include key informant interviews (KIIs) and focus group discussions (FGDs) with various stakeholders including high-risk and non-high-risk cohorts in both arms, parents, primary care doctors, NPHWs, members of the AEAG and peer leaders. A total of 33 FGDs (each with 6–12 participants) and 28 interviews are planned across the 6 purposively selected slum clusters equally divided between both sites. This will include four intervention clusters (two each in Delhi and Vijayawada) and two control clusters (one each in Delhi Vijayawada) ( table 1 ). The KIIs and FGDs with stakeholders will help capture information related to fidelity of the intervention and identify any gaps that could be addressed. Written informed consent will be sought from all participants selected for KIIs and FGDs.
- View inline
Qualitative data collection plan
Data analysis
The conceptual framework for the study and how it integrates with the key parameters from the MRC frameworks is provided in table 2 .
Conceptual Framework for Process Evaluation
The case study methodology will also be used as it is recognised to be suitable to study complex interventions in different contexts and helps to capture ‘the complexity of the case, the relationship between the intervention and the context and how the intervention worked (or did not)’. 25 Following Pfadenhauer et al , we define context as ‘a set of characteristics and circumstances that consist of active and unique factors that surround the implementation. As such it is not a backdrop for implementation but interacts, influences, modifies and facilitates or constrains the intervention and its implementation’. 26 We will be selecting both intervention and control sites as cases to avoid the Hawthorne effect. Two slum cluster in the control arm will also be selected for the case study to enable comparison, to understand contextual factors and any changes to usual care in the absence of an intervention. The importance of studying changes in control arms has been recognised as being useful in understanding intervention impact. 27 A multiple case study design will be used where each case will be analysed at the case level, but we will also pull together all the cases from the intervention and control arms for a more in-depth analysis and comparison. Basic descriptive analysis will be conducted with the quantitative data. Qualitative data will be transcribed by a professional vendor and the transcripts will be read by at least two members of the research team and coded. Coding will be carried out using a priori codes based on the conceptual framework ( table 3 ) with the help of NVivo 12 software.
Data Sources and Areas of Inquiry for Qualitative Data Collection
Any additional codes emerging during the analysis will be added to the coding framework. Members of the research team will read transcripts separately and come up with codes. The research team will then compare and combine their codes to evaluate their fit and usefulness and will examine the differences in code to see if any new insights can be generated. The code list will be finalised after a discussion between the researchers to establish agreement among coders. Qualitative and quantitative data will be triangulated to arrive at a comprehensive understanding of the intervention. Triangulation helps in confirming the findings from quantitative and qualitative data thereby increasing validity as well as leading to a better understanding of phenomena being studied. 28
The quantitative data will be used understand implementation outcomes. Indicators to study implementation outcomes will be informed by the conceptual framework and relevant implementation science theories and frameworks ( table 2 ). These include the RE-AIM 29 (Reach, Efficacy, Adoption, Implementation and Maintenance) framework and the APEASE (Affordability, Practicability, Effectiveness and cost-effectiveness, Acceptability, Safety/Side Effects and, Equity) criteria for evaluation of behaviour change interventions. 30–32 The APEASE criteria have some similarities with RE-AIM but includes two additional parameters, which are- practicability and safety. An intervention is practicable if ‘it can be delivered as designed through the means intended to the target population’. 30 Data will be extracted from OpenMRS and descriptive statistics will be used to get totals, proportions and changes over time.
Assessment of implementation fidelity
Implementation fidelity refers to the extent to which an intervention was implemented as intended. We will assess fidelity in three components, such as (a) delivery of anti-stigma campaign, (b) implementation of the mHealth component and (3) trainings. Indicators have been developed for all these components to measure the frequency of exposure and coverage. The quality of training provided to primary doctors on the mhGAP, NPHWs on the priority listing app and peer educators on promoting mental well-being and competency will be assessed through various tools including a post-training satisfaction survey, pre–post test self-assessment checklists as well as though rating by trainers and staff on competency checklists.
This paper describes the design of a mixed-method process evaluation for the ARTEMIS cRCT, which involves an anti-stigma campaign and a mobile device-based decision support system for primary care doctors and NPHWs, to improve treatment of adolescents at high risk of depression, other significant emotional or medically unexplained complaints and suicide risk.
The process evaluation will help us understand and explain key causal mechanisms that led to change and will, therefore, strengthen the understanding of the implementation process by highlighting various barriers and facilitators. Furthermore, it will provide an understanding of how the local context played a role in the way the intervention was implemented and help identify the need and impact of any adaptations made to the intervention. The process evaluation will provide stakeholder perspectives on aspects of the intervention that worked and those that need further adaptation.
Strengths and limitations
The protocol was developed using existing implementation science theories and frameworks and combines qualitative and quantitative measures to understand key aspects of how the intervention was implemented and which aspects worked or need further improvement. The MRC framework, which is a comprehensive framework, widely used in the field of implementation science will be used. The case study method using a maximum variation approach will be used to make suitable comparisons among different contexts and avoid Hawthorne. The ARTEMIS intervention does not directly address social determinants which impact mental health outcomes. In this study, we will collect additional qualitative and quantitative data on social support, socioeconomic conditions, education and the local context of participants which will help to better understand and explain linkages between mental health outcomes and social determinants.
Ethics and dissemination
The study received formal ethics approval from the Ethics Committee of the George Institute for Global Health India on 4 September 2020 (project number 17/2020). The study also received formal ethics approval from the Research Governance and Integrity Team, Imperial College, London on 8 June 2022 (ICREC reference number: 22IC7718). The Health Ministry’s Screening Committee and Indian Council for Medical Research have also provided approval to the project (ID 2020-9770). Findings will be disseminated to study participants and other stakeholders at a policy symposium. All identifiable personal data will be stored in password-protected secured servers located at The George Institute for Global, India office in Hyderabad. Only deidentified data will be disseminated. Data will be available with the Principal Investigator (PI) on an accessible data repository, which can be accessed by other researchers, subject to a formal request to the PI to access the data for research purposes.
Trial status
At the time of writing the paper, the intervention had started in both sites. Randomisation was executed in Vijayawada and in Delhi on 12 December 2022. Intervention components were implemented in both the sites.
Ethics statements
Patient consent for publication.
Not applicable.
Acknowledgments
We acknowledge the contribution of all members of the Adolescent Expert Advisory Group in Delhi and Vijayawada who have generously contributed their time and expertise to the development of ARTEMIS. GT is supported by the National Institute for Health and Care Research (NIHR) Applied Research Collaboration South London (NIHR ARC South London) at King’s College Hospital NHS Foundation Trust.
- Najman J , et al
- Gururaj G ,
- Varghese M ,
- Benegal V , et al
- Maulik PK , et al
- Patton GC ,
- Sawyer SM ,
- Santelli JS , et al
- Culbreth R ,
- Ndetei D , et al
- Subbaraman R ,
- Shitole T , et al
- Lakiang T ,
- Kathuria N , et al
- Mathias K ,
- Armstrong G , et al
- Leventhal KS ,
- Gillham J ,
- DeMaria L , et al
- Gonsalves PP ,
- Hodgson ES ,
- Bhat B , et al
- Lockwood I ,
- Walker RM ,
- Latimer S , et al
- Barker M , et al
- Macintyre S , et al
- Skivington K ,
- Matthews L ,
- Simpson SA , et al
- Office of the Registrar General & Census Commissioner
- Ministry of Housing and Urban Poverty Alleviation Government of India
- Government of Andhra Pradesh
- Kroenke K ,
- Spitzer RL ,
- Williams JBW
- Yatirajula SK ,
- Kallakuri S ,
- Paslawar S , et al
- ↵ WHO: mhGAP Intervention Guide for mental, neurological and substance use disorders in non- specialized health settings (Version 1.0) , Available : www.who.int/mental_health/mhgap
- Pfadenhauer L ,
- Burns J , et al
- Chirwa E , et al
- Bekhet AK ,
- Zauszniewski JA
- Gainforth HL
- Rogers MB , et al
- Brierley ML ,
- Bailey DP , et al
X @DrSrilatha_p, @No
Contributors The paper was conceptualised by PKM. AM wrote the original draft with contributions from SKY. PKM commented on multiple drafts before sending a prefinal version to everyone listed as authors. SK, SP, HL, UR, BME, RaS, ReS, DP, RN and GT reviewed the draft and provided critical intellectual inputs and comments to the draft. PKM led the implementation of the trial in India along with SKY, SK, SP and AM. SKY, SK and SP played a key role in implementing research activities in the sites. All authors read and approved the final manuscript.
Funding Adolescents’ Resilience and Treatment nEeds for Mental health in Indian Slums (ARTEMIS) is funded by UK Research and Innovation/Medical Research Council (UKRI/MRC), Grant no: MR/S023224/1. SKY, SK and SP are all partially or fully supported by ARTEMIS. PKM and AM are partially supported through NHMRC/GACD grant (SMART MentalHealth-APP1143911) and ARTEMIS. PKM is the PI for ARTEMIS and Co-PI for SMART Mental Health. HL and GT are partly support by ARTEMIS. GT is also supported by the National Institute for Health Research (NIHR) Applied Research Collaboration South London at King’s College London. GT is also supported by the MRC-UKRI in relation to the Indigo Partnership (MR/R023697/1) awards.
Disclaimer The views expressed are those of the author(s) and not necessarily those of the NHS, the NIHR or the Department of Health and Social Care. The funding agency had no role in the writing of the manuscript or the decision to submit it for publication.
Competing interests We declare that the George Institute for Global Health has a part-owned social enterprise, George Health Enterprises, which has commercial relationships involving digital health innovations.
Patient and public involvement Patients and/or the public were involved in the design, or conduct, or reporting, or dissemination plans of this research. Refer to the Methods section for further details.
Provenance and peer review Not commissioned; externally peer reviewed.
Read the full text or download the PDF:
- Call to +1 844 889-9952
Depression: Development, Treatment, and Recovery
Introduction, condition development, signs and symptoms, treatment and therapeutic options, recovery and long-term prognosis, supporting research for treatment protocols.
A widespread and dangerous condition that has a detrimental impact on a person’s feelings, thoughts, and behavior is depression, often known as major depressive disorder. This results in depressed sentiments or a loss of interest in past hobbies for the person (Holford, 2007). This can impair his or her capacity to perform at work and at home and cause a variety of mental and physical issues. Self-hatred and feelings of worthlessness commonly accompany depression, which is one of the most common problems in the world.
Depression is frequently brought on by a combination of factors. These could be chronic stress, unresolved grief, or substance abuse, and in some people, depression may be brought on by a variety of different circumstances. The chances of having depression may rise if a person is feeling sick. Moreover, several research has revealed that depression is more prevalent in older persons and in those who experience challenging social and economic circumstances (Holford, 2007). Moreover, the likelihood of getting depression rises if the person avoids seeing relatives and close friends and tries to resolve their issues on their own when these stressful situations occur.
The complex interplay between social, psychological, and biological elements is the root cause of depression. Childhood hardship, loss, and unemployment are all factors that can contribute to the onset of depression as well as act as its trigger (Holford, 2007). Moreover, the signs can be presented in fatigue, irritability or restlessness, and changes in appetite. In addition to a depressed mood, a diagnosis of severe depression includes a few persistent symptoms, such as loss of interest in most or all regular activities and a sense of pessimism (Holford, 2007). Depending on the individual and his or her unique situation, the symptoms can change. They may also change with the disease’s stage or depend on personal experience.
For depression, there are several therapeutic options that can be prescribed in healthcare facilities. The typical course of treatment for psychological issues is in-depth discussions and behavioral training. Cognitive behavioral therapy (CBT) is the sort of psychological treatment for depression that is most often utilized. CBT and other outpatient therapies like depth psychology and systemic therapy are covered by statutory health insurance companies in the world (Alexopoulos, 2019). The patient can subsequently apply for a whole program of outpatient psychological care.
It is important to consider other variables that can aid in the process of recovery. A diet with a high concentration of fruits, vegetables, whole grains, and seafood appears to be linked to a lower incidence of depression (Coryell, 2021). At the same time, avoiding fatty foods and high-carbonated drinks is essential. More vitamin D, zinc, and protein should be consumed, according to dietary supplement and nutritional guidelines (Alexopoulos, 2019). The requirement for lifestyle modifications is taken into consideration by doctors. This might involve practicing meditation, getting more sleep, and managing stress. Within these considerations, a sample diet for a day can look like this – a breakfast with salad that includes carrots and nuts in order to facilitate the saturation of vitamins B and C, a lunch of whole grain meal with beans or chicken to provide organism with vitamin D and protein, and dinner of fish (salmon or tuna) and rice, to saturate zinc and calcium.
The results suggest that risk variables that may be changed have an impact on the long-term prognosis of depression. In comparison to characteristics that might be altered by receiving earlier and more effective therapy, factors that are mostly fixed at the beginning of treatment, such as the history of recurrent depression and baseline severity, are usually less significant (Alexopoulos, 2019). Early identification can shorten the time before therapy, and more intense acute care and organized follow-up can lessen the severity of depression. For instance, lingering depression symptoms after three months of therapy very definitely reflect both the effectiveness of the therapy and genuine therapy resistance (Holford, 2007). Thus, different variables are observed within the course of recovery.
Observational studies cannot, however, possibly show how these numerous components interact. The number of patients recovering from a depressed episode has increased as a consequence of recent randomized trials that have shown the value of comprehensive depression treatment programs in primary healthcare (Alexopoulos, 2019). More organized and successful depression treatment programs might have a significant impact on their long-term trajectory. If therapy is stopped, relapse should be closely monitored, and if it does, treatment should be begun at once (Ross, 2002). This indicates the need for further research in depression studies.
There are several depression treatment plans. For instance, the continuation phase’s objective is to preserve the benefits obtained during the acute phase of treatment and avoid the return of symptoms. The ideal number of sessions per week for psychotherapy may depend on the particular type and goals of the therapy and the number of sessions required (Alexopoulos, 2019). The severity of the condition, the patient’s preparedness for treatment, the availability of social support, and the existence of medical issues are other variables that may influence how frequently a patient sees a psychotherapist (Holford, 2007). Thus, depression treatment protocols can influence the development of the patient.
To obtain the help they need to recover, people with depression or other mood disorders should engage closely with a mental health practitioner. His or her performance at work and at home may suffer, and depression itself may result in a number of mental and physical issues. Regardless of individual factors, it may have an impact on everyone. As a result, it is critical to be aware of the causes of overeating and to know how to manage it.
Alexopoulos, G. S. (2019). Mechanisms and treatment of late-life depression . Translational Psychiatry, 9 (1), 188. Web.
Coryell, W. (2021). Depressive Disorders . Merck Manual . Web.
Holford, P. (2007). Optimum nutrition for the mind . Hachette UK.
Ross, J. (2002). The mood cure: The 4-step program to take charge of your emotions today . Penguin.
Cite this paper
Select style
- Chicago (A-D)
- Chicago (N-B)
PsychologyWriting. (2024, May 24). Depression: Development, Treatment, and Recovery. https://psychologywriting.com/depression-development-treatment-and-recovery/
"Depression: Development, Treatment, and Recovery." PsychologyWriting , 24 May 2024, psychologywriting.com/depression-development-treatment-and-recovery/.
PsychologyWriting . (2024) 'Depression: Development, Treatment, and Recovery'. 24 May.
PsychologyWriting . 2024. "Depression: Development, Treatment, and Recovery." May 24, 2024. https://psychologywriting.com/depression-development-treatment-and-recovery/.
1. PsychologyWriting . "Depression: Development, Treatment, and Recovery." May 24, 2024. https://psychologywriting.com/depression-development-treatment-and-recovery/.
Bibliography
PsychologyWriting . "Depression: Development, Treatment, and Recovery." May 24, 2024. https://psychologywriting.com/depression-development-treatment-and-recovery/.

An official website of the United States government
The .gov means it’s official. Federal government websites often end in .gov or .mil. Before sharing sensitive information, make sure you’re on a federal government site.
The site is secure. The https:// ensures that you are connecting to the official website and that any information you provide is encrypted and transmitted securely.
- Publications
- Account settings
Preview improvements coming to the PMC website in October 2024. Learn More or Try it out now .
- Advanced Search
- Journal List
- v.31(1); Spring 2008
The Nature of Clinical Depression: Symptoms, Syndromes, and Behavior Analysis
In this article we discuss the traditional behavioral models of depression and some of the challenges analyzing a phenomenon with such complex and varied features. We present the traditional model and suggest that it does not capture the complexity of the phenomenon, nor do syndromal models of depression that dominate the mainstream conceptualization of depression. Instead, we emphasize ideographic analysis and present depression as a maladaptive dysregulation of an ultimately adaptive elicited emotional response. We emphasize environmental factors, specifically aversive control and private verbal events, in terms of relational frame theory, that may transform an adaptive response into a maladaptive disorder. We consider the role of negative thought processes and rumination, common and debilitating aspects of depression that have traditionally been neglected by behavior analysts.
As the field of clinical behavior analysis grows, it will benefit from analyses of increasingly complex and common clinical phenomena, especially those with significant public health implications. One such phenomenon is clinical depression, considered to be the “common cold” of outpatient populations. Up to 25 million people in the United States alone meet criteria for some type of depressive disorder in a given year (M. B. Keller, 1994 ). Depressive disorders also result in considerable financial expenditure including time spent away from the workplace and an increase in health care costs. Based on broad measures that include work absenteeism, treatment costs, and other factors, the annual economic cost of depressive disorders in the United States may be over $40 billion ( Antonouccio, Thomas, & Danton, 1997 ). Suicide is the ultimate cost.
Perhaps nowhere in clinical psychology is the medicalization of behavioral problems more complete than with depression. Depression is largely seen by the general public and mainstream media as a neuropsychiatric illness (e.g., Wingert & Kantrowitz, 2002 ) with a fluctuating course that is best described in disease-state terms such as disorder, episodes, remission, recovery, relapse , and recurrence ( Frank et al., 1991 ). An additional assumption is that this disorder may be diagnosed and labeled using the symptom checklists of the standard diagnostic system, the Diagnostic and Statistical Manual of Mental Disorders ( DSM-IV-TR ; American Psychiatric Association, 2000 ). The basic ontological assumption is that depression is an illness that occurs episodically and can be described adequately in medical terms. Thus, more depression is treated in primary care than in any other mental health or health care setting ( Kessler, McGonagle, Swartz, Blazer, & Nelson, 2003 ; Shapiro, 1984 ), and guidelines for treatment in these settings recommend antidepressant treatment without specialty referral unless the patient has complicating factors such as comorbid substance use or suicide risk ( Schulberg, Katon, Simon, & Rush, 1998 ). Even in these cases, specialty referral is first to psychiatry for medication management, and only a small number of individuals diagnosed with depression will be seen by a clinical psychologist, much less a behaviorally oriented practitioner.
A hallmark of behavior analysis has been its condemnation of the misappropriation of lay terms as scientific, technical terms (e.g., Skinner, 1945 ). The first task is precise specification of the behavioral phenomena invoked by the term. There are several obstacles to achieving this precision with the term depression, which have been excellently presented for the term anxiety by Friman, Hayes, and Wilson (1998) . The case for depression is quite similar. First, the term depression was never meant as a technical term and actually has a metaphorical, idiomatic basis. Second, our psychiatric nomenclature and mainstream usage of the term suggest that depression is an empirical phenomenon with an essential composition. To a behavior analyst, the term depression is not a technical term, does not precisely map onto any empirical or behavioral phenomena, and has no essential composition. Thus, given the exhaustive medicalization of the phenomenon of depression, there exists an immense gap between a behavioral analysis of depression and mainstream usage of it as a medical term with its various associations and meanings.
Behavior-analytic writings on clinical depression (e.g., Dougher & Hackbert, 1994 , 2000 ; Ferster, 1973 ; Lewinsohn, 1974 ; see Eifert, Beach, & Wilson, 1998 , for an alternative, paradigmatic behavioral model) have been illuminative but sparse. Although research on depression has outpaced research on virtually every other disorder by psychiatric and cognitive-behavioral researchers, behavior analysts have been alarmingly silent. There are undoubtedly many reasons for this silence (e.g., a lack of training programs that focus on behavior analysis and traditional psychopathology and more reinforcement for studying familiar topics). More relevant to the current paper is the possibility that the exhaustive medicalization of the term; the wealth of non-behavior-analytic research data on biology and genetics, personality, and cognitive factors; and the emphasis on private events in depression—on how depression feels and on changing that feeling—may function to evoke avoidance in behavior analysts.
This is unfortunate, because behavior analysis can not only provide an integrative view of depression, taking into consideration genetics, biology, enduring patterns of responding labeled personality , verbal (“cognitive”) behavior, and private events, but it can do so with a theoretical consistency and pragmatic utility unmatched by other theoretical systems. In this paper we attempt to start at the beginning, with a discussion of what depression is to a behavior analyst and how this contrasts with mainstream usage of the term as a medical syndrome. We review the traditional operant model of depression that emphasized reductions in behavior as a response to environmental events. We then tackle several areas of inquiry important to an understanding of depression that have traditionally been neglected by behavior analysts, including private events and the role of verbal behavior in depression. We see this not as completing a behavioral analysis but as a reminder of the importance of idiographic, functional analyses of specific individuals for this complex phenomenon.
What Is Depression? Tacting Depression and Its Symptoms
We describe depression in radical behavioral terms, emphasizing the occasions on which the term is used and deemphasizing any underlying unitary disease, physiological, or emotional state to which the term refers. Depression comes from the late Latin word depressare and the classical Latin word deprimere . Deprimere literally means “press down”; de translates into “down” and premere translates into “to press.” In essence, the term appears to denote a feeling of heaviness, of being “pressed down,” that is also referred to as “sad,” “blue,” or simply “down.” Depression also refers to a depressed topography or the fact of being pressed down. Depression as a referent to mood or emotional state appeared as early as 1665 and merely meant a lowering of mood or spirits ( Simpson & Weiner, 1989 ). Thus, the core experience of depression appears to be a private event tacted as depressed or in psychiatric terms as dysphoric . However, a minority of individuals will meet criteria for depression and deny depressed mood or present with irritable mood instead. These individuals may have deficits in accurate tacting of private experience, or they may represent diagnostic Type II errors and should not be classified as depressed.
It is important not to associate what is tacted as depression with a specific pattern of physiological responding or reify it as a particular emotional state. The antecedent conditions and underlying physiologies associated with the experience of depression may vary widely, and no core composition can be assumed. Emotional states such as sadness are simply co-occurring behavioral responses (elicited unconditioned reflexes, conditioned reflexes, operant predispositions) that appear to be integrated because the behaviors are occasioned by common discriminanda and are controlled by common consequences ( Skinner, 1953 ). For example, a child with overbearing parents experiences an emotional state of sadness and a co-occurring behavioral response of crying when her parents criticize her. The crying is negatively reinforced when her parents comfort her and stop criticizing her, which may also result in a change of her emotional state.
The particular quality of an emotional state labeled depression should vary with the characteristics of the environmental triggers. For example, private events labeled as depressed may be associated with overworking and receiving little reinforcement for long stretches of time or with grieving the death of a loved partner. In each case the underlying physiology is presumably different, but the experienced phenomena may be sufficiently similar to prompt the tact. More specific discrimination training may be useful (e.g., the first situation may be better labeled as burned out and the second as grieving ) but given the problems associated with training the tacting of private events ( Moore, 1980 ) it is not clear that an individual will be able to make these discriminations reliably.
The psychiatric nomenclature emphasizes this core experience and several additional symptoms. Depressed mood or dysphoria is the primary feature of major depressive disorder (MDD), the most common depressive diagnosis. In addition to this core experience, there are several other symptoms of MDD, including loss of interest in activities, sleep and appetite changes, guilt and hopelessness, fatigue, restlessness, concentration problems, and suicidal ideation. As discussed in detail below, the medical model holds that this constellation of symptoms represents a syndrome, but complexity is immediately introduced because the presence and nature of these symptoms vary considerably across clients ( Líndal & Stefánsson, 1991 ). For example, some clients experience vegetative symptoms of depression (decreased appetite and insomnia) whereas, less commonly, others experience reversed vegetative symptoms of increased appetite and hypersomnia. Similarly, psychomotor retardation is more common and agitation is less common, and both may be demonstrated by the same individual at different times.
To account for this complexity, DSM-IV-TR has parsed depression into various additional categories, each with similar and overlapping characteristics, and there are an increasing number of diagnostic categories of depressive disorders or problems involving sad or irritable affect. In fact, Appendix B of DSM-IV-TR lists 17 proposed disorders for further study, six of which deal with disorders of mood. Although detailed review of these subcategories is outside the purview of this paper, it should be noted that although there may indeed be different syndromes with different etiologies and treatment implications, a behavior-analytic view holds that the current proliferation of depressive disorders is largely unnecessary. We see not several distinct disorders but a phenomenon of depression with great variability in time course, symptom severity, and correlated conditions. All the disorders share a depressed mood symptom that parallels the core experience of the problem, and all share several additional criteria with MDD, often differing only in duration or number of symptoms. From an idiographic behavior-analytic perspective, there exists not one or three or several depressive disorders—there are as many depressive disorders as there are depressed individuals.
The constellation of depressive disorders with shared characteristics suggests that the tact depression involves a variety of public and private antecedent stimulating events that vary from occasion to occasion but have sufficient overlapping properties to occasion consistent usage of the term. We view the diversity of additional symptoms represented by these disorders as consistent with the diversity of environmental causes of depression, physiological states labeled depression , and psychological responses to the environmental causes and physiological states. Thus, no overarching depressive syndromes are posited or assumed at this point. Nonetheless, commonalities in history, environmental antecedents, and symptom presentation exist and may guide treatment decisions.
Thus, our understanding of depression must allow for the great variety of stimulus conditions that occasion use of the term. We can discard several classes of use that we can simply label as incorrect. For example, an individual learning a foreign language may simply state the wrong word. Likewise, a person may be diagnosed with depression but later it is determined that the person has a large cancerous tumor that is causing the symptoms. Although a complete behavioral analysis must account for these usages, they are not interesting from a clinical standpoint. More important are instances in which the term is not used but could be. For example, a person visits a psychologist and complains of several symptoms of depression but not depressed mood. Another person would label the private experience as “depressed,” but the current client did not develop adequate private stimulus control over the experience. The psychologist performs a diagnostic interview, and the client falls one symptom short of the diagnosis of MDD. In this case it is advantageous to consider the person depressed even though it is possible that neither the psychologist nor the client will use the term.
Traditional Behavioral Models of Depression
Skinner wrote very little on depression; when he did, he emphasized overt behavior rather than the core affective experience, in line with an operant rather than respondent model. For example, in 1953 he wrote,
If we remove a man from his characteristic surroundings, a large part of his social behavior cannot be emitted and may therefore become more and more probable: he will return to his old surroundings whenever possible and will be particularly “sociable” when he does so. Other parts of his behavior become strong because they are automatically reinforced under the prevailing deprivation; he will talk to anyone who will listen about his old surroundings, his old friends, and what he used to do. This is all a result of deprivation. But nostalgia is also an emotional condition in which there is a general weakening of other forms of behavior—a “depression,” which may be quite profound. We cannot classify this as the result of deprivation because the behavior which is thus affected has not been specifically restrained. (p. 165)
Three aspects of this passage are noteworthy. First, as Skinner typically did, by placing the term depression in quotes he was careful to avoid giving it any special status other than that of a verbal description. As discussed above, this practice of placing such terms in quotes may be awkward and tiresome but serves as a reminder that certain assumptions are not to be made when using them. The quotes also serve as a reminder of an important verbal quality to the term, discussed below. Second, Skinner described the core experience as an “emotional condition,” suggesting an elicited component. Consistent with an operant model, he did not elaborate on this point and instead focused on overt behavioral reductions. Third, Skinner highlighted the centrality of reduced positive reinforcement in depression. Simply put, social behavior depends on a reinforcing environment; change the environment so that responses do not yield reinforcement and one reduces the behavior.
This notion became the foundation of Lewinsohn's (1974) theory and dominated the behavioral literature for several decades. Lewinsohn described depression as characterized primarily by a low rate of response-contingent positive reinforcement (RCPR). In a nutshell, Lewinsohn emphasized environmental events that produce losses of major sources of RCPR, such as a divorce or the loss of a job, and social skills deficits that limit an individual's ability to reobtain RCPR once it has been lost. Thus, his model focused on the behavioral reductions often seen in depression. Lewinsohn assumed the core experience to be an elicited by-product of these situations, but he did not detail this process. Other symptoms of depression (e.g., fatigue, somatic symptoms, and cognitive symptoms) were assumed to be evoked or to be secondary elaborations of other symptoms.
We hold that Lewinsohn's (1974) characterization of the core affective experience of depression as an elicited by-product of losses of or reductions in positive reinforcement is fundamental to understanding depression. Some cases of depression clearly are described best by Lewinsohn's model, such as single, discrete episodes of depression with clear environmental precipitants and with symptom profiles that emphasize behavioral reductions that resolve when the environments are reinstated. For example, a person may become depressed after a divorce or loss of job, and the depression resolves when the person finds a new relationship partner or a new job. With cases of chronic depression, Lewinsohn's model emphasizes persistently insufficient levels of reinforcement and social skills deficits that prevent the individual from changing the situation, and this model also seems to be adequate for some cases. For example, a person who becomes depressed after a divorce, resulting in a net reduction in positive reinforcement, and does not have adequate social skills for initiating new romantic relationships will likely become chronically depressed until the necessary social skills are learned.
All of this is nothing new. However, Lewinsohn's (1974) model vastly underestimated the variety and complexity of factors that can reduce behavior. Indeed, the field of behavior analysis, if nothing else, has demonstrated functional processes that can increase or decrease behavior. All functional processes that decrease behavior are potentially relevant, if the behavioral reductions produced are large and generalized and a dysphoric reaction occurs concomitant with the behavioral reductions. For example, extreme persistent and uncontrollable punishment may lead to substantial behavioral reductions, elicited negative affect, and depression as per Seligman's early learned helplessness model ( Overmier & Seligman, 1967 ).
Further consideration of this complexity is provided by Hopko, Lejuez, Ruggiero, and Eifert (2003) and Lejuez, Hopko, and Hopko (2001) , who analyzed depression in terms of the matching law ( Herrnstein, 1970 ). Briefly, this suggests that the behavioral reductions seen in depression are not accurately seen as the simple product of reductions in positive reinforcement but rather as the product of ratios of reinforcement for depressed relative to nondepressed (or healthy) behavior. In other words, the sum total of reinforcement available in a person's environment must be taken into consideration, not just reinforcement for target behaviors. As a simple example, a depressed person may not get out of bed due to loss of a job (loss of positive reinforcer for getting out of bed), but positive reinforcers for staying in bed (e.g., spouse who now takes care of the person or makes the person breakfast) must also be considered. The bottom line is that the situation is complicated, and nothing less than a complete functional analysis of the individual's environment is required if one is to attempt a full functional analysis of depression.
Aversive Control in Depression
Skinner also suggested that depression may be an emotional response to aversive controlling practices, especially aversive social control (1953, pp. 360–363). Similarly, Ferster (1973) suggested that depression is characterized as much by increased escape and avoidance repertoires as by reduced positive repertoires. In fact, research indicates that more cases of depression are characterized by the accrual of multiple chronic mild stressors, such as work-related stress, homemaking demands, and financial trouble than by major losses such as divorce or the loss of a job ( Billings & Moos, 1984 ; Kessler, 1997 ; Mazure, 1998 ; Monroe & Depue, 1991 ; Paykel, 1982 ). In these cases we suggest that the core elicited affective experience of depression is as much a product of increased aversive control as it is reduced appetitive control.
It is important to recognize, however, that the two sources of control are often intimately related. Ferster (1973) suggested that the depressed escape and avoidance repertoire is largely passive, which also leads to a decrease in positive reinforcement relative to what an active repertoire would provide. For example, consider a client who stayed in bed all day and did not go to work, thereby avoiding a stressful meeting with his boss where he believed he was going to be reprimanded. Staying in bed successfully avoids this outcome, but it also prevents contact with other contingencies that might function to ameliorate depression—for example, if the client was wrong and no reprimand was forthcoming. Similarly, an individual with social phobia, which is highly comorbid with depression ( Mineka, Watson, & Clark, 1998 ), may be negatively reinforced by successfully avoiding situations that may result in social humiliation or embarrassment, but avoidance of such situations also reduces opportunities for contact with positive social reinforcement. In other words, an increase in aversive social control here almost guarantees a decrease in appetitive social control. These aversive environments evoke and maintain behavior that is immediately effective as a response to these contingencies but maladaptive over the long term in that access to positive reinforcers is diminished.
Such aversive situations may elicit anxiety rather than depression per se, but the point is that a repertoire characterized by excessive escape and avoidance behavior (and elicited affect labeled anxiety ) will undoubtedly result in decreased contact with positive reinforcement (and elicited affect labeled depression ) over time. Thus, anxiety should precede and then become comorbid with depression, and this pattern appears to characterize many comorbid cases ( Mineka et al., 1998 ). In fact, the well-established comorbidity of anxiety and depressive disorders should be a function of the degree to which anxious avoidance also results in a loss of positive reinforcement. Hayes, Wilson, Gifford, Follette, and Strosahl (1996) have provided a convincing review showing that avoidance may underlie a host of psychological problems, including depression, and the specific relation between avoidance and depression has received empirical support as well (reviewed by Ottenbreit & Dobson, 2004 ). Finally, research indicates that over the course of treatment for social phobia, change in anxiety predicts change in depression, but change in depression does not predict change in anxiety ( Moscovitch, Hofmann, Suvak, & In-Albon, 2005 ), suggesting that symptoms of depression are at least partially maintained by a social environment that has aversive functions.
To summarize our analysis to this point, there are many pathways to depression. Depression is not a precise, technical term and has no essential composition. It is not a syndrome. The term refers to a chronic experience of feeling sad or down and to associated symptoms that vary widely. This symptomatic heterogeneity is due to the heterogeneity of historical and environmental controlling variables. That said, some processes may be more common in depression, and awareness of these processes would help to limit what could be a vast assessment of many potentially irrelevant variables ( Hayes & Follette, 1992 ). Two broad processes have been highlighted here: (a) losses of, reductions in, or persistently insufficient levels of positive reinforcement as per Lewinsohn (1974) , and (b) increases in environmental aversive control (negatively reinforcing and punishment contingencies). When chronic, both processes may be seen as functioning as enduring motivating operations for depression ( Dougher & Hackbert, 2000 ). Of course, multiple sources of control are probable.
An Adaptive Syndrome or Maladaptive Response? Genetics and Evolutionary Theories
In contrast to an idiographic functional analysis of depression, the medical disease model posits that depression is a syndrome or multiple syndromes and one inherits risk for this syndromal response. The model relies to a considerable degree on research indicating at least some genetic involvement in depression ( Wallace, Schneider, & McGuffin, 2002 ). However, the family, twin, and adoption studies on which this conclusion is based point to a larger environmental contribution than genetic contribution in all but the most severe cases of depression ( Wallace et al., 2002 ). Furthermore, researchers and theorists from a variety of perspectives have highlighted methodological flaws and unsubstantiated assumptions of this research ( Ceci & Williams, 1999 ; Hayes, 1998 ; Turkheimer, 1998 ) that have the collective effect of lowering heritability estimates even further as well as questioning their very basis. Nonetheless, it seems likely that some inherited vulnerability to depression exists in some cases, and a full behavior-analytic account can include this possibility.
A typical behavioral argument against the medical disease model of depression is to accept that depression is a syndrome but posit that it is adaptive, the product of contingencies of survival ( Skinner, 1953 ). In fact, many evolutionary explanations for depression have been offered (e.g., Bowlby, 1980 ; Gilbert, 1992 ; Leahy, 1997 ; Price, Sloman, Gardner, Gilbert, & Rohde, 1994 ; P. J. Watson & Andrews, 2002 ; see McGuire & Troisi, 1998 , for a review), and such evolutionary accounts are important to consider and are consistent with behavioral theory ( Corwin & O'Donohue, 1995 ). There are three broad themes under which these theories fall: resource conservation, social competition, and attachment ( Allen & Badcock, 2003 ).
Theories of resource conservation posit that depression permits the conservation of resources and disengagement from unsuccessful goal-directed activity by decreasing appetite, energy levels, and motivation ( Leahy, 1997 ; Nesse, 2000 ). For instance, when in a new environment with unknown contingencies, such as traveling to a foreign country, one is more likely to be functioning in a way to avoid negative reinforcement or punishment while trying to learn the rules of the new environment. If one were to engage in a goal-directed activity, such as trying to obtain a job, one would likely not be successful. Social-competition theories view depression as a deescalation or yielding reaction to a defeat. This is said to be adaptive because it signals submission to the victor and allows acceptance of social subordination and the avoidance of unnecessary conflict ( Price, 1967 , 1998 ; Price et al., 1994 ). An example of this can be seen in a boxing match, when one fighter is knocked down for a full 10 counts. The loser typically displays behaviors including sloped posture, decreased eye contact, and avoidance (all depressed behaviors) as opposed to getting back up and continuing to fight. Finally, attachment theories of the adaptive nature of depression claim that a depressive reaction is an adaptive response to the loss of interpersonal relationships that helps to maintain the proximity of caregivers or reestablish an attachment by signaling a need for assistance from others and eliciting that assistance ( Averill, 1968 ; Bowlby, 1980 ; Frijda, 1994 ). This can easily be seen by a lost boy in a busy mall. When the child begins to cry, passersby typically attend to him, try to find the boy's parents, and comfort him during the search.
We suggest that depression is neither a syndrome nor adaptive. Any theory of depression as an adaptive syndrome has to overcome two primary hurdles inherent in the phenomenon. First, given the variability in symptom profiles in depression, one has to pick which set of symptoms of depression comprises the syndrome, or alternately posit multiple syndromes with different symptom sets (M. C. Keller & Nesse, 2006 ). For example, are both melancholic and atypical depression adaptive syndromes? Given that some of symptoms associated with melancholic depression (insomnia and loss of appetite) are the opposite of those associated with atypical depression (hypersomnia and increased appetite), it is impossible for the same theory to account for both presentations.
Second, the nature and chronicity of depressive symptoms seem to be maladaptive. For example, a transient sad mood in response to a loss certainly seems adaptive in that it elicits empathy and evokes helping behaviors in others. If this is true, then such an affective respondent reaction may have evolved due to contingencies of survival. It would be expected to have certain losses as antecedents and to resolve when support is acquired. However, in clinical depression the sad mood is often chronic and unresponsive to helping behaviors. In fact, although the evolutionary account suggests that the response should garner social support, research is clear that depressive behaviors result in decreased social support ( Coyne, 1976 ; Gotlib & Lee, 1989 ; Joiner & Metalsky, 2001 ) and worse psychosocial functioning in general ( Barnett & Gotlib, 1988 ). Suicide is another example. Although suicidal gestures may be seen as operant attempts to garner support ( Linehan, 1993 ), completed suicide is difficult to conceive of as an operant (i.e., learned) behavior ( Hayes, Strosahl, & Wilson, 1999 ) and is clearly not adaptive in terms of survival.
A more likely scenario is that depression itself is not adaptive, but the core experience represents a variation of an adaptive affective response ( Nettle, 2004 ; also see Nesse, 2000 ). In other words, the capability to experience moderate low mood or sadness in appropriate situations (but not become clinically depressed) may have many of the same short-term benefits that have been used to support the claim that depression is adaptive. Support for this view comes from personality researchers, who have posited the temperamental trait of negative affectivity as a trait that is selected for and normally distributed ( Nettle, 2004 ; D. Watson & Clark, 1984 ), and considerable research suggests that this trait may be a vulnerability factor for both depression and anxiety (L. A. Clark & Watson, 1991 ; L. A. Clark, Watson, & Mineka, 1994 ).
Although the notions of temperament or traits are unnecessary, it is reasonable to suggest that there may be a range in the duration and magnitude of affective reactions that are adaptive. A depressed individual could represent a deviation from that range in that he or she experiences negative affect longer and to a greater extent in response to an environmental event. In other words, the propensity to experience mild and appropriate levels of negative affect may be adaptive and thus appear on a continuum; those at one of the extreme ends of this continuum may be quite sensitive to fluctuations in reinforcement contingencies, suffer from chronic negative affect, and be at risk for clinical depression.
It is important to remember that we are proposing a scenario in which there is a genetic contribution to the likelihood of the core affective experience in depression but the remaining symptoms are potentially free to vary and should be described in terms of antecedents and consequences. Of course, there may be an adaptive, normally distributed range in the sensitivity of these additional behaviors (e.g., sleep) to environmental stimuli that represent separate inherited vulnerabilities. This view of depression is consistent with recent biological findings that suggest that depression is likely a product of multiple genes and a complex gene–environment interaction ( Wallace et al., 2002 ), as well as neuroscientific findings of mixed and variable structural and functional abnormalities in several brain regions, with few depressed individuals displaying the complete package of deficits, leading researchers to conclude that depression refers to a heterogeneous group of disorders as well ( Davidson, Pizzagalli, Nitschke, & Putnam, 2002 ). Thus, other scientific fields are taking tentative steps away from a syndromal view of depression and toward an idiographic analysis.
The Shift From Adaptive to Maladaptive Behavior
As discussed above, elicited affective experiences are normal, adaptive, and not disordered. Depression appears to be a maladaptive dysregulation or extension of this adaptive experience. Genetic vulnerabilities aside, it is important to identify the historical and environmental processes responsible for this shift from a normal, adaptive experience of elicited affect to a disordered experience of depression.
Obviously, chronically maladaptive environments may produce chronically maladaptive behavior. Perhaps the simplest and ultimate example of this is a concentration camp ( Frankel, 1984 ). Such an environment, almost completely lacking in positive reinforcers and abundant in stable and salient aversive stimuli, may result in rather consistent depressed behavior and negative affect. However, it is safe to say that most depressed people do not live in such environments. Processes through which environments characterized by variable positive and negative reinforcers and punishers result in relatively stable experiences of depression need to be identified. For example, consider a person who has a handful of close friends with whom she interacts with on a regular basis, men who are showing interest in her romantically, good career prospects including an upcoming promotion (all opportunities for positive reinforcement), but still cannot sleep at night and considers herself to be depressed. The question remains, why do so many people engage in repertoires that are more consistent with impoverished environments than with those environments in which they live? Below we consider two processes: avoidance of aversive private events and the role of verbal behavior.
Avoidance of Private Events
Two similar processes by which an adaptive elicited response can lead to chronic and maladaptive depression in the absence of chronically maladaptive environments recently have been proposed and linked to treatment techniques: Martell, Addis, and Jacobson's (2001) theory behind behavioral activation (BA) and Hayes et al.'s (1999) model of experiential avoidance for acceptance and commitment therapy (ACT). The two models differ in several respects (see Kanter, Baruch, & Gaynor, 2006 , for a full comparison).
Both models argue that problematic avoidance in depression is not always a response to the environment per se, but is a response to the core aversive experience of depression (which is in turn a response to the environment). Both models suggest that the core affective experience, once elicited, may play a functional role in maintaining, exacerbating, and creating the additional symptoms of depression. Specifically, if we allow that the initial elicited private response is functionally aversive, it may evoke behavior designed to avoid and escape the private response. For example, after a difficult breakup, a man may experience an increase in feelings of anxiety and negative self-referential thoughts. Although this individual now may avoid public stimuli based on formal stimulus properties (e.g., romantic relationships), he also may avoid the newly elicited thoughts and feelings in a variety of ways (e.g., heavy drinking). The key to understanding how this applies to depression is the notion that avoidance of private events, even when it works in the short term, produces additional long-term problems. In the example above, the man feels better in the short run after drinking, but the long-term consequences would likely include an even more impoverished environment. Through this process, flexible repertoires of problem solving and repertoires based on stable positive reinforcement are either extinguished, depotentiated, or never developed.
BA interventions have focused on disrupting how aversive private events can function as discriminative stimuli or motivating operations for avoidance behavior. For example, consider a client who stayed in bed all day because she felt depressed and thereby was able to avoid the additional stress and fatigue associated with her unpleasant work situation. Although she may experience her work situation as aversive to some extent at all times, the heaviness and fatigue experienced upon awakening in the morning and tacted as “feeling depressed” may signal that working would be experienced as especially aversive on that particular day. According to BA, staying in bed in this situation is negatively reinforced through avoidance of an especially aversive work day. However, it creates more long-term problems and solves none in that it does nothing to address the aversive work situation proactively ( Kanter et al., 2006 ).
ACT offers additional theoretical elaborations that suggest a more prominent role for verbal behavior in avoidance processes. First, ACT suggests that experiential avoidance repertoires are maintained over long periods of time because they are rule governed or verbally controlled ( Hayes & Ju, 1998 ). In other words, individuals develop rules that dictate experiential avoidance, and these rule-governed avoidance repertoires may persist in the face of histories of reinforcement to the contrary. For example, a depressed man may tell himself, “If bad things happen in my life, I will take it like a man.” Such self-talk may lead to denial of certain private events such as sadness or grief (e.g., after his father died) despite an environment that would shape more effective behavior (e.g., a loving wife who wants to discuss his feelings), were it not for verbal control.
There may in fact be no way to distinguish a rule-governed avoidance repertoire (i.e., ACT) from a directly conditioned avoidance repertoire (i.e., BA) in a clinical setting, in that the topographies may look similar, the relevant reinforcement histories are distal, and reporting on them accurately will be unreliable. At issue is the degree to which a depressed individual's avoidance is rule governed. In fact, Rehm (1979 , 1989) has argued that depressed individuals demonstrate deficits in the ability to generate and follow rules, and his self-management therapy program attempts to improve self-monitoring, self-evaluation, and self-reinforcement skills. In accord with these views are findings that depressed individuals demonstrate increased self-reported preferences for immediate over delayed reinforcement compared to nondepressed individuals, suggesting less rule following in depressed individuals ( Rehm & Plakosh, 1975 ). Two additional studies have shown that dysphoric individuals demonstrate greater schedule sensitivity and less rule-governed behavior compared to nondepressed individuals ( Baruch, Kanter, Busch, Richardson, & Barnes-Holmes, 2007 ; Rosenfarb, Burker, Morris, & Cush, 1993 ). However, these studies demonstrate significant variability in schedule sensitivity, and McAuliffe (2003) found the opposite (increased rule-governed behavior in depressed adolescents), again highlighting the need for idiographic analysis and acknowledging both increased rule-governed behavior and decreased rule-governed behavior to be problematic in depression.
To reiterate the important themes at this point, it bears repeating that an idiographic analysis is required. Some cases of depression may be adequately conceptualized in terms of Lewinsohn's (1974) traditional model of reductions in response-contingent positive reinforcement, whereas others may be more accurately conceptualized in terms of ACT's or BA's models of avoidance. In both the traditional model and the new conceptualizations, the core experience is seen as an elicited response to environmental events that produce reductions in positive reinforcement. However, the new conceptualizations speculate how one's reaction to that experience may in fact perpetuate and exacerbate it, and in some cases this may be the case.
The Functions of Private Events in Behavior Analysis
Allowing that a private response is functionally aversive creates some problems for behavior analysis. Simply put, the classic exhortation to focus functional analyses on manipulable environmental variables may lead some to conclude that private events are simple respondent by-products and have no functional value. This stance on the nonfunctional value of private events is one of the great perplexities of behavior analysis. It is a perplexity because, to most humans, thoughts and emotions—as we have come to label them—are not only felt quite strongly at times but it feels as if they control our behavior ( Schnaitter, 1978 ). This is especially true regarding avoidance behavior, which is often described as negatively reinforced by a reduction in aversive emotional experience (e.g., Barlow, 2002 ). In other words, it seems as if we avoid not only the conditions that occasion depression but also feeling depression itself. It may have been behavior analysts' rigid adherence to this simple view of private events, which runs counter to common sense for many researchers, therapists, and clients, that bolstered the cognitive revolution and the subsequent obsolescence of behavioral approaches to treatment of depression, as well as adult outpatient psychotherapy in general, which is dominated by “feeling” talk.
Skinner presented a much more nuanced and complex view. On the one hand, he consistently defined reinforcers and discriminative stimuli as environmental stimuli on pragmatic rather than ontological grounds ( Skinner, 1945 , 1953 ). Simply put, reinforcers are labeled as such only if functional analysis has determined, or at least in principle could determine, that a manipulable event evidences such a function. Private events in general are not manipulable in this sense and thus have been typically defined as dependent rather than independent variables. Put differently, Skinner consistently argued that emotions are not causes.
However, in other places Skinner allowed private events to participate, partially, in the control of behavior. For example, he wrote,
Emotional responses may be interpreted as in part an escape from the emotional components of anxiety. Thus we avoid the dentist's office, not only because it precedes painful stimulation and is therefore a negative reinforcer, but because, having preceded such stimulation, it arouses a complex emotional condition which is also aversive. The total effect may be extremely powerful. (1953, p. 179)
In this example, the emotional components of anxiety clearly have taken on functional stimulus properties. Likewise, Skinner's analysis of self-knowledge (1957, 1974) depended heavily on the supposition that private events exert discriminative control over tacting. In this case, the use of the term private event rather than private behavior may have been Skinner's acknowledgment of the complexity, but the complexity is not resolved simply by changing the term. In these cases, although the private events in question are assumed to have acquired at least partial control over other behavior, environmental variables are important for the historical development of the control (see also Hayes & Brownstein, 1986). For example, in the case of the tact of “sad,” the private stimulation involved is seen as the discriminative stimulus for the resulting tact, and that stimulation has obtained functional significance through social mediation ( Moore, 1980 ). Thus, it is consistent with behavior analysis (or at least, with behavior analysis's inconsistency) to allow functionally salient private events to evoke avoidance behavior.
The Role of Verbal Behavior in Depression
Perhaps the biggest obstacle for traditional behavioral theorists to overcome when discussing depression is the role of language. In general, an extremely large and unquestionable body of research establishes the presence of negative cognitive content during depressive episodes, leading cognitive researchers to assume a causal role for cognition in depression (D. A. Clark, Beck, & Alford, 1999 ). Although longitudinal research has failed to establish negative cognitive biases as independent predictors of depression ( Ingram, Miranda, & Segal, 1998 ), it is clear that thinking influences feeling on a moment-to-moment basis. Cognitive researchers see this influence as sufficiently causal, but behavior analysts instead search for environmental conditions responsible for such behavior–behavior relations ( Hayes & Brownstein, 1986 ). Regardless, it is clear that negative thinking predominates in many depressions, and such thinking may elicit aversive affect.
Research on stimulus equivalence (e.g., Sidman, 1994 ) readily accounts for the relation between cognition and mood. Simply put, through participation in equivalence relations with nonverbal stimuli, verbal stimuli may obtain eliciting functions. Although there are many examples of this effect, perhaps the clearest example is work by Dougher and colleagues on the transfer of aversive elicitation and avoidance functions through equivalence classes. Using match-to-sample procedures, Dougher, Augustson, Markham, Greenway, and Wulfert (1994) taught 8 subjects two four-member equivalence classes, paired one member of one class with electric shock, and then demonstrated transfer of elicited arousal to other members of the class that had not been directly paired with the shock. Augustson and Dougher (1997) subsequently demonstrated that avoidance responding similarly transfers through equivalence classes. After pairing one member of one class with shock, subjects were taught that they could avoid this member by repeatedly pressing a key on the keyboard. Subjects then demonstrated transfer of avoidance response functions to other class members.
Growing research on relational frame theory (RFT; Hayes, Barnes-Holmes, & Roche, 2001 ) extends these findings. According to RFT, verbal behavior (including thinking) is technically seen as the behavior of framing events relationally: responding to one stimulus in terms of its given or inferred relation to other stimuli. For example, a woman caught speeding receives a ticket. If that person thinks that people who get speeding tickets are bad drivers, she may then consider herself a bad driver. RFT views equivalence as just one type of relation (i.e., sameness ) and views deriving relations among stimuli in the absence of direct conditioning as a generalized operant ( Barnes-Holmes & Barnes-Holmes, 2000 ).
Responding in accordance with other derived relations has also been demonstrated, including relations of sameness, opposition , and difference ( Roche & Barnes, 1996 , 1997 ; Steele & Hayes, 1991 ; Whelan & Barnes-Holmes, 2004 ), more than and less than ( Dymond & Barnes, 1995 ; O'Hora, Roche, Barnes-Holmes, & Smeets, 2002 ; Whelan, Barnes-Holmes, & Dymond, 2006 ), and before and after ( O'Hora, Barnes-Holmes, Roche, & Smeets, 2004 ; see also Barnes & Roche, 1996 ; Hayes & Barnes, 1997 ). Evidence is mounting that these relations may result in the transformation of functions in accordance with the relations trained, akin to the transfer of function seen with equivalence relations (for a review, see Dymond & Rehfeldt, 2001 ). Thus if that same woman who received a speeding ticket has a history of avoiding authority figures who reprimanded her in the past (e.g., teachers and supervisors), she may then begin to avoid police officers as well. These stimulus functions may be quite arbitrary and unrelated to current environmental features. Thus, the behavior of relational framing has a potentially transformative effect on the environment; environmental stimuli that would otherwise control behavior may not do so and new stimuli, idiosyncratic to the individual's verbal learning history, may exert control.
The importance of these findings to depression, and other psychological disorders, cannot be overstated. To the extent that stimulus equivalence and RFT present a behavior-analytic model of language and cognition, these theories provide behavior analysts with a vocabulary and theory with which cognitive variables can be conceptualized and understood. Negative self-statements so often seen in depression acquire their meanings and functions through transformations of function that occur in relational framing. For verbal stimuli to obtain these specific functions, previous specific-exemplar training involving the specific stimuli participating in relational frames is not necessary. All that is necessary is a history that establishes relational framing as a generalized operant and a history in which the specific stimuli at issue are related in a relational network.
There appear to be two uses of the term relational network , and a brief diversion on this issue is necessary because one of the usages may be potentially confusing to behavior analysts. First, a relational network may refer to a sentence or another unit of speech that sets the context for relational activity ( Barnes-Holmes, Hayes, Dymond, & O'Hora, 2001 )—there is no issue with this usage. Second, a relational network may be used to graphically depict the full set of relations between specific stimuli and the transformations of function that are relevant to a particular stimulus. Such networks are often displayed in RFT or stimulus equivalence experiments to depict the specific relations trained, but a network may also be employed more loosely when the history can only be assumed. For example, Blackledge (2003) displayed a network to account for a person taking a walk in the woods that elicits fear due to a verbal history in which it was learned that snakes are to be found in woods. This usage bears considerable resemblance to nonbehavioral entities such as schemas and requires clarification. One has to be careful to maintain that the network, unlike a schema, is the not the cause of behavior. The network is a description of a history, and it is this history, along with the current environmental and verbal events, that functions as the cause. The history is described in terms of a network to emphasize how the functions of any term in the network may be transformed in accordance with the network, but such transformations are a product of a history of verbal behavior described as a network, not the network per se. It is easy to lose sight of behavior analysis at this point; thus, it is important to remember that these functions of relational framing were obtained through a history of interaction with the social and verbal community. Historical environmental factors result in the transformation and reduction of control by the current environment. These effects are easily described in terms of relational networks and stimulus functions that are transformed across members of the network.
The important point is that verbal behavior can dysregulate and extend normal adaptive experiences of aversive elicitation into disordered experiences. Consider an individual who has received a poor work evaluation. This naturally elicits aversive affect that, if transient, can be considered normal and adaptive. However, this individual may begin to think about the event, and the content of thinking will be a complex product of multiple historical and current antecedents. Given a history that has established high-strength relational networks of “loss,” “failure,” “helplessness,” or similar networks, a transient setback such as a poor job evaluation can become functionally overwhelming. The key point is that the core experience of depression, the elicited affect, was normal and adaptive without verbal elaboration. With verbal elaboration, however, the experience is magnified and extended, and may become disordered.
Examples of verbal elaborations of potentially normal experiences abound in the clinical literature on depression. In fact, cognitive therapy for depression ( Beck, Rush, Shaw, & Emery, 1979 ) assumes challenging these unrealistic verbal elaborations to be the primary task of therapy. Discussion of whether such cognitive interventions are successful, for the reasons cognitive therapists say they are, is beyond the scope of this paper. Rather, we simply highlight the finding that many depressed individuals appear to have become depressed in the absence of environmental histories that would indicate such a response to be adaptive, and point to verbal behavior to account for the elaboration of such histories into a disorder. Language vastly expands the range of situations that can function as depression-eliciting and depression-maintaining stimuli, because the functions of the stimuli largely may be determined by one's idiosyncratic verbal learning history.
As an example, a depressed individual may respond to all social events as participating in a verbal relation with a host of other aversive stimuli (e.g., the words fake, small-talk, embarrassment, boring, stressful, idiot, foolish, exposed and the words for a range of aversive private sensations germane to escape, panic, etc.). Although another individual may respond to the relation between the stimuli party and stressful on occasion, there is flexibility in responding based on other historical and contextual features. For the depressed individual, however, this verbal class may be so well formed through a fairly idiosyncratic history of verbal and nonverbal pairings of these stimuli, and so negatively reinforced through past derived escape and avoidance experiences, that there may be few or no contexts in which the term party does not elicit the functions of other aversive stimuli or function as a derived discriminative stimulus for escape or avoidance. This rigid avoidance repertoire vastly narrows the range of behavioral options available and most likely will lead to rather stable reductions in response-contingent social reinforcement.
The Function of Rumination
In addition to negative cognitive content in depression, research clearly identifies a particular ruminative cognitive style in depression. In fact, a ruminative cognitive style predicts the onset ( Just & Alloy, 1997 ; Nolen-Hoeksema, 2000 ), length ( Umberson, Wortman, & Kessler, 1992 ), and severity ( Nolen-Hoeksema, Parker, & Larson, 1994 ) of depressive episodes. Depressed individuals may spend long periods of time “lost in thought,” rehashing events of the day and stewing over problems; this leads to increased negatively biased thoughts, poor problem solving, inhibition of operant behavior, impaired concentration, increased stress, and increased problems ( Lyubomirsky & Tkach, 2004 ). A complete behavioral analysis of depression needs to account for the relation between negative cognitive content and depressed mood as well as the function of rumination.
An appreciation of the somewhat unique features of verbal behavior in terms of antecedent and consequential control provides some insight into the function of rumination in depression. First, it bears repeating that thinking, like any behavior, is under the control of multiple and complex historical and situational stimuli. Consider a depressed student attending a lecture in a class in which she is doing poorly. She is having a hard time keeping up with the professor, and thoughts about her poor performance on the last test occur. The initial stimuli for such thoughts are obvious. As this continues, it is common that this student may end up thinking about a completely different topic, with unexpected twists and turns in thought, arriving at thoughts that she will never get her degree, that there must be something wrong with her brain, and that she is a complete failure. She now begins to think about a negative interaction with a friend the previous day, and thinks that her friendship was never genuine, that she is a failure as a friend as well as in school, and so forth. These twists and turns may increasingly be under the stimulus control of previous thoughts and decreasingly under the control of the current external environment. As described by RFT, the contextual cues that occasion relational framing and its content may themselves be relational and arbitrary in nature; thus, other than previous verbal behavior (which may be private), little environmental support is necessary to occasion verbal behavior and control its content. This in fact is consistent with Skinner's (1953) account. As Skinner put it, “The speaker's own verbal behavior automatically supplies stimuli for echoic, textual, or intraverbal behavior, and these in turn generate stimuli for further responses” (p. 439). He referred to such a thinking process as a “simple soliloquy” and noted, “Regardless of the respectability of the connections, such a ‘train of thought’ … is scarcely to be distinguished from a ‘flight of ideas’” (p. 439). We may refer to such thinking instead as rumination.
The question of reinforcing variables for such behavior remains. RFT suggests that verbal behavior occurs so frequently and relentlessly because of a history of reinforcement provided by the wider community for coherence or sense making in one's verbal behavior (see Hayes et al., 1999 ). It is argued that during early language-training experiences, the verbal behavior of the speaker is evaluated for coherence by the verbal community and is differentially reinforced. Over time, these processes (the verbal behavior, its evaluation in terms of coherence, and its reinforcement) become covert and automatic so that the derivation and rehearsal of coherent verbal relations becomes self-reinforcing (see Barnes-Holmes et al., 2001 ). Skinner (1953) highlighted that thinking is productive, in that it has an effect on the thinker and is reinforcing because it does so. Both processes are undoubtedly applicable. We would like the depressed student to think about her class performance and the poor friend interaction in such a way that it leads to improved performances and interactions in the future, but in many cases it does not. More likely is an avoidance function—the rumination may function to reduce the anxiety about the class performance and the interaction without increasing the anxiety of dealing with the problems in the moments they occur. As long as the cognitive solutions make sense and reduce anxiety, the rumination may continue, even if it is ultimately unproductive.
Accepting that sense making is reinforcing, one may still argue that the content of rumination often does not make sense and should not be reinforcing. In the current example, one poor performance in a class does not make one a failure as a student, and one poor friend interaction does not make one a failure as a friend. Indeed, pointing out that such content is not logical and is not evidence based is the hallmark of cognitive therapy ( Beck et al., 1979 ). However, to a behavior analyst sense making is idiographic and occurs in the context of the individual's unique history and experiences. For this individual, we would expect a history in which other negative experiences were interpreted as evidence of complete failure by caregivers and important others, or something similar ( Bolling, Kohlenberg, & Parker, 2000 ). The current context also plays a role: The negative affect of depression provides a context in which interpretations of failure make sense, and the interpretation of friendship failure makes sense given the previous interpretation of school failure.
An important point is that as this individual continues to ruminate, the class lecture is continuing and the student is now largely divorced from contact with any potentially external controlling variables. Thus, verbal behavior, when it occurs, may be quite relentless in overpowering, transforming, and reducing environmental control. As seen in the example of rumination, if the aforementioned student continues ruminative thought throughout her class period she would not be engaged in class discussions and would therefore miss any opportunities of praise or encouragement from the professor. She may also miss necessary information for her next assignment, thereby not only reducing her rate of receiving response-contingent reinforcement but also increasing the likelihood of punishment through a lowered grade. After leaving class and realizing this mistake, she may continue to ruminate now about her difficulty in lecture as well as her previous interaction, which results in further attentional distancing from her immediate environment and additional negative affect.
Summary and Conclusions
There are many pathways to depression. Depression is not a precise, technical term, has no essential composition, and is not a syndrome. The term refers to a core experience of feeling sad or down and to associated symptoms that vary widely. This symptomatic heterogeneity is due to the heterogeneity of historical antecedents and consequences. The core experience may be seen as an elicited by-product of losses of, reductions in, or persistently insufficient levels of positive reinforcement. However, Lewinsohn (1974) , with his focus on environments characterized directly by losses of response-contingent positive reinforcement, presented a fairly unitary operant model that obscured the heterogeneity of depressive symptom profiles. Given the ubiquity of depression and the diversity of its symptom presentations, both reductions in positive reinforcement and increases in aversive control may function as enduring motivating operations ( Dougher & Hackbert, 2000 ). Of course, multiple sources of control are probable. Thus, idiographic assessment is required to determine both the relative importance of positive and aversive control and to determine specific target variables for any given individual. Clinical behavior analysts treating depression would be well served to engage in detailed, idiographic, and historical functional assessments that inform treatment course and technique.
We argue that a modern behavioral account of depression must incorporate controlling variables at both environmental and interoceptive levels, with a recognition of the role of avoidance and verbal behavioral processes. We have emphasized that the core experience of depression is a private event—elicited negative affect that is felt and tacted in a variety of ways. This affect itself is not problematic and is in fact adaptive, but it becomes chronic, maladaptive, or dysregulated through environmental and learned behavioral processes. A key process may be avoidance: Responses to the original private events that function to avoid or escape the event may be negatively reinforced and establish a cycle of increasing negative affect and avoidance, a vicious spiral into depression.
Verbal processes may be particularly important as well, in that research on stimulus equivalence and RFT clearly demonstrates how private stimulus events can be transformed and elaborated when related verbally to other events. Thus, stimulus events that would not otherwise function to elicit depressive affect may come to do so through verbal processes, already aversive stimuli may become more so through verbal processes, and verbal processes may establish avoidance and other dysfunctional responses to such stimuli that otherwise would not be established. Rumination—a hallmark feature of depression—is an example of how verbal behavioral processes may result in negative reinforcement through reducing contact with the current physical environment but exacerbate depressive functioning. Behavior-analytic research on the role of verbal processes in depression is in its infancy, but as research in these areas continues to accumulate, analyses of complex phenomena such as depression may benefit greatly.
- Allen N.B, Badcock P.B.T. The social risk hypothesis of depressed mood: Evolutionary, psychosocial, and neurobiological perspectives. Psychological Bulletin. 2003; 129 :887–913. [ PubMed ] [ Google Scholar ]
- American Psychiatric Association. Diagnostic and statistical manual of mental disorders , (text rev.) Washington, DC: Author; 2000. [ Google Scholar ]
- Antonouccio D.O, Thomas M, Danton W.G. A cost-effectiveness analysis of cognitive behavior therapy and fluoxetine (Prozac) in the treatment of depression. Behavior Therapy. 1997; 28 :187–210. [ Google Scholar ]
- Augustson E.M, Dougher M.J. The transfer of avoidance evoking functions through stimulus equivalence classes. Journal of Behavior Therapy & Experimental Psychiatry. 1997; 28 :181–191. [ PubMed ] [ Google Scholar ]
- Averill J. Grief: Its nature and significance. Psychological Bulletin. 1968; 70 :721–748. [ PubMed ] [ Google Scholar ]
- Barlow D.H. Anxiety and its disorders: The nature and treatment of anxiety and panic (2nd ed.) New York: Guilford; 2002. [ Google Scholar ]
- Barnes D, Roche B. Relational frame theory and stimulus equivalence are fundamentally different: A reply to Saunders' commentary. The Psychological Record. 1996; 46 :489–507. [ Google Scholar ]
- Barnes-Holmes D, Barnes-Holmes Y. Explaining complex behavior: Two perspectives on the concept of generalized operant classes? The Psychological Record. 2000; 50 :251–265. [ Google Scholar ]
- Barnes-Holmes D, Hayes S.C, Dymond S, O'Hora D. Multiple stimulus relations and the transformation of stimulus functions. In: Hayes S.C, Barnes-Holmes D, Roche B, editors. Relational frame theory: A post-Skinnerian account of human language and cognition. New York: Plenum; 2001. pp. 51–71. [ Google Scholar ]
- Barnett P.A, Gotlib I.H. Psychosocial functioning and depression: Distinguishing among antecedents, concomitants, and consequences. Psychological Bulletin. 1988; 104 :97–126. [ PubMed ] [ Google Scholar ]
- Baruch D.E, Kanter J.W, Busch A.M, Richardson J.V, Barnes-Holmes D. The differential effect of instructions on dysphoric and non-dysphoric individuals. The Psychological Record. 2007; 57 :543–554. [ Google Scholar ]
- Beck A.T, Rush A.J, Shaw B.F, Emery G. Cognitive therapy of depression. New York: Guilford; 1979. [ Google Scholar ]
- Billings A.G, Moos R.H. Chronic and nonchronic unipolar depression: The differential role of environmental stressors and resources. Journal of Nervous & Mental Disease. 1984; 172 :65–75. [ PubMed ] [ Google Scholar ]
- Blackledge J.T. An introduction to relational frame theory: Basics and applications. The Behavior Analyst Today. 2003; 3 :421–433. [ Google Scholar ]
- Bolling M, Kohlenberg R, Parker C. Behavior analysis and depression. In: Dougher M, editor. Clinical behavior: Analytic approaches to treatment. Reno: Context Press; 2000. pp. 127–152. [ Google Scholar ]
- Bowlby J. Attachment and loss: Vol. 3. Loss, sadness and depression. New York: Basic Books; 1980. [ Google Scholar ]
- Ceci S.J, Williams W.M. The nature–nurture debate: The essential readings. Malden, MA: Blackwell; 1999. [ Google Scholar ]
- Clark D.A, Beck A.T, Alford B.A. Scientific foundations of cognitive theory and therapy of depression. New York: Wiley; 1999. [ Google Scholar ]
- Clark L.A, Watson D. Tripartite model of anxiety and depression: Psychometric evidence and taxonomic implications. Journal of Abnormal Psychology. 1991; 100 :316–336. [ PubMed ] [ Google Scholar ]
- Clark L.A, Watson D, Mineka S. Temperament, personality, and the mood and anxiety disorders. Journal of Abnormal Psychology. 1994; 103 :103–116. [ PubMed ] [ Google Scholar ]
- Corwin J.V, O'Donohue W.T. Evolutionary theory and behavior therapy. In: O'Donohue W.T, Krasner L, editors. Theories of behavior therapy: Exploring behavior change. Washington, DC: American Psychological Association; 1995. pp. 475–494. [ Google Scholar ]
- Coyne J.C. Toward an interactional description of depression. Psychiatry: Journal for the Study of Interpersonal Processes. 1976; 39 :28–40. [ PubMed ] [ Google Scholar ]
- Davidson R.J, Pizzagalli D, Nitschke J.B, Putnam K. Depression: Perspectives from affective neuroscience. Annual Review of Psychology. 2002; 53 :545–574. [ PubMed ] [ Google Scholar ]
- Dougher M.J, Augustson E, Markham M.R, Greenway D.E, Wulfert E. The transfer of respondent eliciting and extinction functions through stimulus equivalence. Journal of the Experimental Analysis of Behavior. 1994; 62 :331–351. [ PMC free article ] [ PubMed ] [ Google Scholar ]
- Dougher M.J, Hackbert L. A behavior-analytic account of depression and a case report using acceptance-based procedures. The Behavior Analyst. 1994; 17 :321–334. [ PMC free article ] [ PubMed ] [ Google Scholar ]
- Dougher M.J, Hackbert L. Establishing operations, cognition, and emotion. The Behavior Analyst. 2000; 23 :11–24. [ PMC free article ] [ PubMed ] [ Google Scholar ]
- Dymond S, Barnes D. A transformation of self-discrimination response functions in accordance with the arbitrarily applicable relations of sameness, more than, and less than. Journal of the Experimental Analysis of Behavior. 1995; 64 :163–184. [ PMC free article ] [ PubMed ] [ Google Scholar ]
- Dymond S, Rehfeldt R.A. Supplemental measures of derived stimulus relations. Experimental Analysis of Human Behavior Bulletin. 2001; 19 :8–12. [ Google Scholar ]
- Eifert G.H, Beach B.K, Wilson P.H. Depression: Behavioral principles and implications for treatment and relapse prevention. In: Plaud J.J, Eifert G.H, editors. From behavior theory to behavior therapy. Needham Heights, MA: Allyn & Bacon; 1998. pp. 68–97. [ Google Scholar ]
- Ferster C.B. A functional analysis of depression. American Psychologist. 1973; 28 :857–870. [ PubMed ] [ Google Scholar ]
- Frank E, Prien R.F, Jarrett R.B, Keller M.B, Kupfer D.J, Lavori P.W, et al. Conceptualization and rationale for consensus definitions of terms in major depressive disorder: Remission, recovery, relapse, and recurrence. Archives of General Psychiatry. 1991; 48 :851–855. [ PubMed ] [ Google Scholar ]
- Frankel V. Man's search for meaning. New York: Simon and Schuster; 1984. [ Google Scholar ]
- Frijda N.H. Varieties of affect: Emotions and episodes, moods, and sentiments. In: Ekman P, Davidson R.J, editors. The nature of emotion: Fundamental questions. New York: Oxford University Press; 1994. pp. 59–67. [ Google Scholar ]
- Friman P.C, Hayes S.C, Wilson K.G. Why behavior analysts should study emotion: The example of anxiety. Journal of Applied Behavior Analysis. 1998; 30 :137–156. [ PMC free article ] [ PubMed ] [ Google Scholar ]
- Gilbert P. Depression: The evolution of powerlessness. New York: Guilford; 1992. [ Google Scholar ]
- Gotlib I.H, Lee C.M. The social functioning of depressed patients: A longitudinal assessment. Journal of Social and Clinical Psychology. 1989; 8 :223–237. [ Google Scholar ]
- Hayes S.C. Resisting biologism. The Behavior Therapist. 1998; 21 :95–97. [ Google Scholar ]
- Hayes S.C, Barnes D. Analyzing derived stimulus relations requires more than the concept of stimulus class. Journal of the Experimental Analysis of Behavior. 1997; 68 :225–233. [ PMC free article ] [ PubMed ] [ Google Scholar ]
- Hayes S.C, Barnes-Holmes D, Roche B, editors. Relational frame theory: A post-Skinnerian account of human language and cognition. New York: Kluwer/Plenum; 2001. [ PubMed ] [ Google Scholar ]
- Hayes S.C, Brownstein A.J. Mentalism, private events, and scientific explanation: A defense of B. F. Skinner's view. In: Modgil S, Modgil C, editors. B. F. Skinner: Consensus and controversy. Sussex, England: Falmer; 1986. pp. 207–218. [ Google Scholar ]
- Hayes S.C, Follette W.C. Can functional analysis provide a substitute for syndromal classification? Behavioral Assessment. 1992; 14 :345–365. [ Google Scholar ]
- Hayes S.C, Ju W. The applied implications of rule-governed behavior. In: O'Donohue W.T, editor. Learning and behavior therapy. Needham Heights, MA: Allyn & Bacon; 1998. pp. 374–391. [ Google Scholar ]
- Hayes S.C, Strosahl K.D, Wilson K.G. Acceptance and commitment therapy: An experiential approach to behavior change. New York: Guilford; 1999. [ Google Scholar ]
- Hayes S.C, Wilson K.G, Gifford E.V, Follette V.M, Strosahl K. Emotional avoidance and behavioral disorders: A functional dimensional approach to diagnosis and treatment. Journal of Consulting and Clinical Psychology. 1996; 64 :1152–1168. [ PubMed ] [ Google Scholar ]
- Herrnstein R.J. On the law of effect. Journal of the Experimental Analysis of Behavior. 1970; 13 :243–266. [ PMC free article ] [ PubMed ] [ Google Scholar ]
- Hopko D, Lejuez C, Ruggiero K, Eifert G. Contemporary behavioral activation treatments for depression: Procedures, principles and progress. Clinical Psychology Review. 2003; 23 :699–717. [ PubMed ] [ Google Scholar ]
- Ingram R.E, Miranda J, Segal Z.V. Cognitive vulnerability to depression. New York: Guilford; 1998. [ Google Scholar ]
- Joiner T.E, Metalsky G.I. Excessive reassurance seeking: Delineating a risk factor involved in the development of depressive symptoms. Psychological Science. 2001; 12 :371–378. [ PubMed ] [ Google Scholar ]
- Just N, Alloy L.A. The response styles theory of depression: Tests and an extension of the theory. Journal of Abnormal Psychology. 1997; 106 :221–229. [ PubMed ] [ Google Scholar ]
- Kanter J.W, Baruch D.E, Gaynor S.T. Acceptance and commitment therapy and behavioral activation for the treatment of depression: Description and comparison. The Behavior Analyst. 2006; 29 :161–185. [ PMC free article ] [ PubMed ] [ Google Scholar ]
- Keller M.B. Depression: A long-term illness. British Journal of Psychiatry. 1994; 165 :9–15. [ PubMed ] [ Google Scholar ]
- Keller M.C, Nesse R.M. The evolutionary significance of depressive symptoms: Different adverse situations lead to different depressive symptom patterns. Journal of Personality and Social Psychology. 2006; 91 :316–330. [ PubMed ] [ Google Scholar ]
- Kessler R.C. The effects of stressful life events on depression. Annual Review of Psychology. 1997; 48 :191–214. [ PubMed ] [ Google Scholar ]
- Kessler R.C, McGonagle K.A, Swartz M, Blazer D.G, Nelson C.B. Sex and depression in the National Comorbidity Survey: I. Lifetime prevalence, chronicity and recurrence. Journal of Affective Disorders. 2003; 29 :85–96. [ PubMed ] [ Google Scholar ]
- Leahy M. Alterations in individuals' behaviour in contexts of high-risk environments. Journal of Personality. 1997; 22 :339–406. [ Google Scholar ]
- Lejuez C.W, Hopko D.R, Hopko S.D. A brief behavioral activation treatment for depression: Treatment manual. Behavior Modification. 2001; 25 :255–286. [ PubMed ] [ Google Scholar ]
- Lewinsohn P.M. A behavioral approach to depression. In: Friedman R.J, Katz M.M, editors. Psychology of depression: Contemporary theory and research. Oxford, England: Wiley; 1974. pp. 157–178. [ Google Scholar ]
- Líndal E, Stefánsson J.G. The frequency of depressive symptoms in a general population with reference to DSM-III . International Journal of Social Psychiatry. 1991; 37 :233–241. [ PubMed ] [ Google Scholar ]
- Linehan M.M. Cognitive-behavioral treatment of borderline personality disorder. New York: Guilford; 1993. [ Google Scholar ]
- Lyubomirsky S, Tkach C. The consequences of dysphoric rumination. In: Papageorgiou C, Wells A, editors. Depressive rumination: Nature, theory and treatment. Oxford, England: Wiley; 2004. pp. 21–42. [ Google Scholar ]
- Martell C.R, Addis M.E, Jacobson N.S. Depression in context: Strategies for guided action. New York: Norton; 2001. [ Google Scholar ]
- Mazure C.M. Life stressors as risk factors in depression. Clinical Psychology: Science & Practice. 1998; 5 :291–313. [ Google Scholar ]
- McAuliffe D. Rule-following and depressive symtomology in an adolescent population: An experimental analysis. 2003 Unpublished doctoral dissertation, National University of Ireland, Maynooth. [ PubMed ] [ Google Scholar ]
- McGuire M.T, Troisi A. Prevalence differences in depression among males and females: Are there evolutionary explanations? British Journal of Medical Psychology. 1998; 71 :479–491. [ PubMed ] [ Google Scholar ]
- Mineka S, Watson D, Clark L.A. Comorbidity of anxiety and unipolar mood disorders. Annual Review of Psychology. 1998; 49 :377–412. [ PubMed ] [ Google Scholar ]
- Monroe S.M, Depue R.A. Life stress and depression. In: Becker J, Kleinman A, editors. Psychosocial aspects of depression. Hillsdale, NJ: Erlbaum; 1991. pp. 1101–1130. [ Google Scholar ]
- Moore J. On behaviorism and private events. The Psychological Record. 1980; 30 :459–475. [ Google Scholar ]
- Moscovitch D.A, Hofmann S.G, Suvak M.K, In-Albon T. Mediation of changes in anxiety and depression during treatment of social phobia. Journal of Consulting and Clinical Psychology. 2005; 73 :945–952. [ PubMed ] [ Google Scholar ]
- Nesse R.M. Is depression an adaptation? Archives of General Psychiatry. 2000; 57 :14–20. [ PubMed ] [ Google Scholar ]
- Nettle D. Evolutionary origins of depression a review and reformulation. Journal of Affective Disorders. 2004; 81 :91–102. [ PubMed ] [ Google Scholar ]
- Nolen-Hoeksema S. The role of rumination in depressive disorders and mixed anxiety/depressive symptoms. Journal of Abnormal Psychology. 2000; 109 :504–511. [ PubMed ] [ Google Scholar ]
- Nolen-Hoeksema S, Parker L.E, Larson J. Ruminative coping with depressed mood following loss. Journal of Personality and Social Psychology. 1994; 67 :92–104. [ PubMed ] [ Google Scholar ]
- O'Hora D, Barnes-Holmes D, Roche B, Smeets P.M. Derived relational networks and control by novel instructions: A possible model of generative verbal responding. The Psychological Record. 2004; 54 :437–460. [ Google Scholar ]
- O'Hora D, Roche B, Barnes-Holmes D, Smeets P. Response latencies to multiple derived stimulus relations: Testing two predictions of relational frame theory. The Psychological Record. 2002; 52 :51–75. [ Google Scholar ]
- Ottenbreit N.D, Dobson K.S. Avoidance and depression: The construction of the cognitive-behavioral avoidance scale. Behaviour Research & Therapy. 2004; 42 :293–313. [ PubMed ] [ Google Scholar ]
- Overmier J.B, Seligman M.E.P. Effects of inescapable shock upon subsequent escape and avoidance responding. Journal of Comparative and Physiological Psychology. 1967; 63 ((1)):28–33. [ PubMed ] [ Google Scholar ]
- Paykel E.S. Psychopharmacology of suicide. Journal of Affective Disorders. 1982; 4 :271–273. [ PubMed ] [ Google Scholar ]
- Price J.S. The dominance hierarchy and the evolution of mental illness. Lancet. 1967; 7502 :243–246. [ Google Scholar ]
- Price J. The adaptive function of mood change. British Journal of Medical Psychology. 1998; 71 :465–477. [ PubMed ] [ Google Scholar ]
- Price J, Sloman L, Gardner R, Gilbert P, Rohde P. The social competition hypothesis of depression. British Journal of Psychiatry. 1994; 164 :309–315. [ PubMed ] [ Google Scholar ]
- Rehm L.P. A comparison of self-control and assertion skills treatments of depression. Behavior Therapy. 1979; 10 :429–442. [ Google Scholar ]
- Rehm L.P. Behavioral models of anxiety and depression. In: Kendall P.C, Watson D, editors. Anxiety and depression: Distinctive and overlapping features. San Diego: Academic Press; 1989. pp. 55–79. [ Google Scholar ]
- Rehm L.P, Plakosh P. Preference for immediate reinforcement in depression. Journal of Behavior Therapy and Experimental Psychiatry. 1975; 6 :101–103. [ Google Scholar ]
- Roche B, Barnes D. Arbitrarily applicable relational responding and sexual categorization: A critical test of the derived difference relation. The Psychological Record. 1996; 46 :451–475. [ Google Scholar ]
- Roche B, Barnes D. A transformation of respondently conditioned stimulus function in accordance with arbitrarily applicable relations. Journal of the Experimental Analysis of Behavior. 1997; 67 :275–301. [ PMC free article ] [ PubMed ] [ Google Scholar ]
- Rosenfarb I.S, Burker E.J, Morris S.A, Cush D.T. Effects of changing contingencies on depressed and nondepressed individuals. Journal of Abnormal Psychology. 1993; 102 :642–646. [ PubMed ] [ Google Scholar ]
- Schnaitter R. Private causes. Behaviorism. 1978; 6 :1–12. [ Google Scholar ]
- Schulberg H.C, Katon W, Simon G.E, Rush A.J. Treating major depression in primary care practice: An update of the Agency for Health Care Policy and Research Practice guidelines. Archives of General Psychiatry. 1998; 55 :1121–1127. [ PubMed ] [ Google Scholar ]
- Shapiro S. Utilization of health and mental health services: Three epidemiologic catchment area sites. Archives of General Psychiatry. 1984; 41 :971–978. [ PubMed ] [ Google Scholar ]
- Sidman M. Equivalence relations and behavior: A research story. Boston: Authors Cooperative; 1994. [ Google Scholar ]
- Simpson J, Weiner E, editors. Oxford English dictionary. Cary, NC: Oxford University Press; 1989. [ Google Scholar ]
- Skinner B.F. The operational analysis of psychological terms. Psychological Review. 1945; 52 :270–277. [ Google Scholar ]
- Skinner B.F. Science and human behavior. Oxford, England: Macmillan; 1953. [ Google Scholar ]
- Skinner B.F. About behaviorism. New York: Knopf; 1974. [ Google Scholar ]
- Steele D, Hayes S.C. Stimulus equivalence and arbitrarily applicable relational responding. Journal of the Experimental Analysis of Behavior. 1991; 56 :519–555. [ PMC free article ] [ PubMed ] [ Google Scholar ]
- Turkheimer E. Heritability and biological explanation. Psychological Review. 1998; 105 :782–791. [ PubMed ] [ Google Scholar ]
- Umberson D, Wortman C.B, Kessler R.C. Widowhood and depression: Explaining long-term gender differences in vulnerability. Journal of Health and Social Behavior. 1992; 33 :10–24. [ PubMed ] [ Google Scholar ]
- Wallace J, Schneider T, McGuffin P. Genetics of depression. In: Gotlib I.H, Hammen C.L, editors. Handbook of depression. New York: Guilford; 2002. pp. 169–191. [ Google Scholar ]
- Watson D, Clark L.A. Negative affectivity: The disposition to experience aversive emotional states. Psychological Bulletin. 1984; 96 :465–490. [ PubMed ] [ Google Scholar ]
- Watson P.J, Andrews P.W. Toward a revised evolutionary adaptationist analysis of depression: The social navigation hypothesis. Journal of Affective Disorders. 2002; 72 :1–14. [ PubMed ] [ Google Scholar ]
- Whelan R, Barnes-Holmes D. A derived transformation of consequential functions in accordance with the relational frames of same and opposite. Journal of the Experimental Analysis of Behavior. 2004; 82 :177–195. [ PMC free article ] [ PubMed ] [ Google Scholar ]
- Whelan R, Barnes-Holmes D, Dymond S. The transformation of consequential functions in accordance with the relational frames of more than and less than. Journal of the Experimental Analysis of Behavior. 2006; 86 :317–335. [ PMC free article ] [ PubMed ] [ Google Scholar ]
- Wingert P, Kantrowitz B. Young and depressed. Newsweek. 2002 Oct 7;:53–61. [ PubMed ] [ Google Scholar ]
What’s New This Week and Prior
No featured items available.
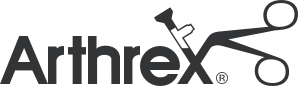

A .gov website belongs to an official government organization in the United States.
A lock ( ) or https:// means you've safely connected to the .gov website. Share sensitive information only on official, secure websites.
- About Violence Prevention
- Resources for Action
- Cardiff Model
Violence Prevention
About The Public Health Approach to Violence Prevention
Violence Topics
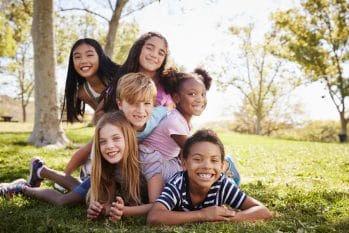
About Adverse Childhood Experiences
About Child Abuse and Neglect
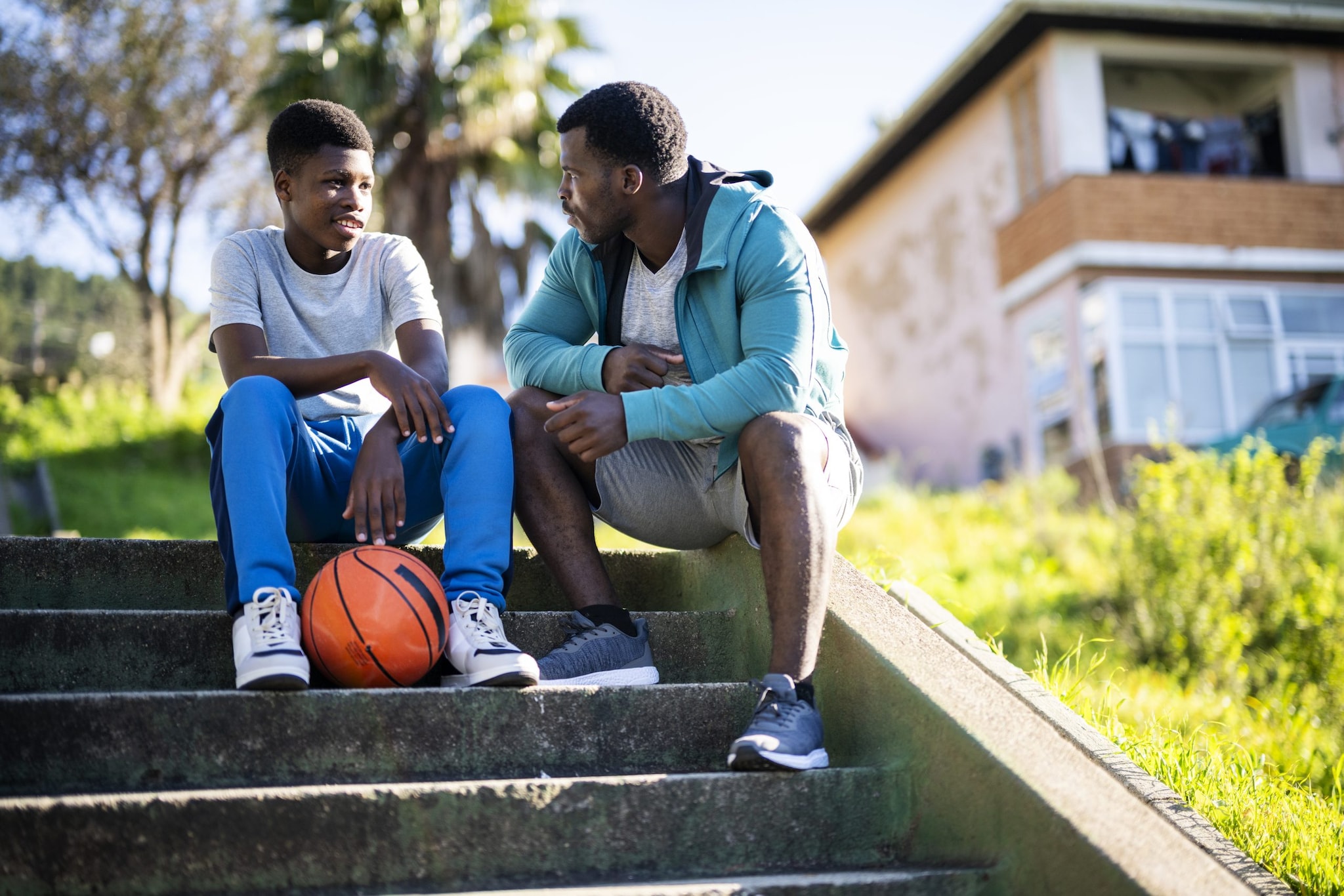
About Community Violence
About Firearm Injury and Death
About Intimate Partner Violence
About Sexual Violence
About Youth Violence
About Abuse of Older Persons
About Violence Against Children and Youth Surveys
About The National Intimate Partner and Sexual Violence Survey (NISVS)
About The National Violent Death Reporting System (NVDRS)
Featured Resources
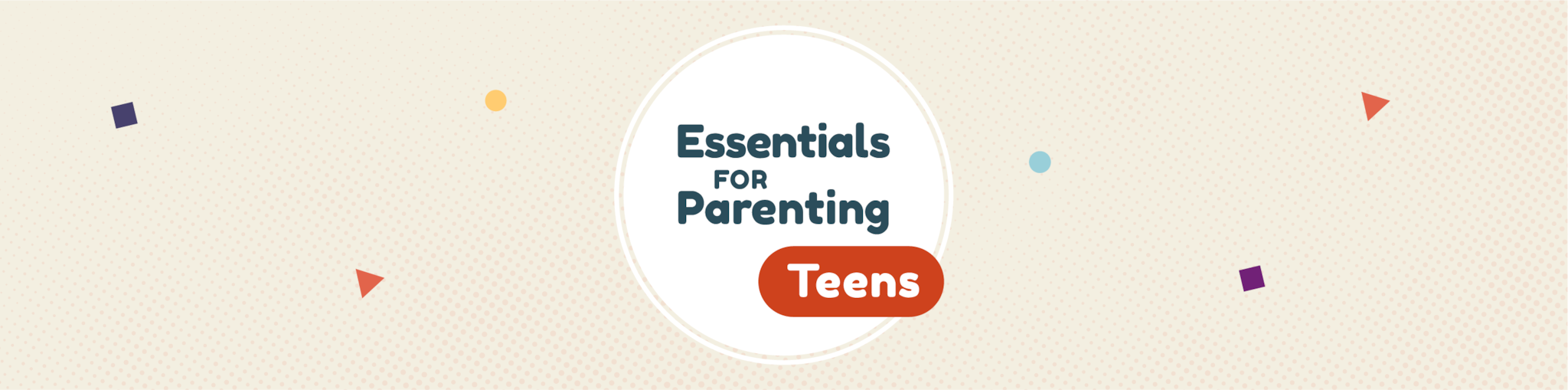
Essentials for Parenting Teens
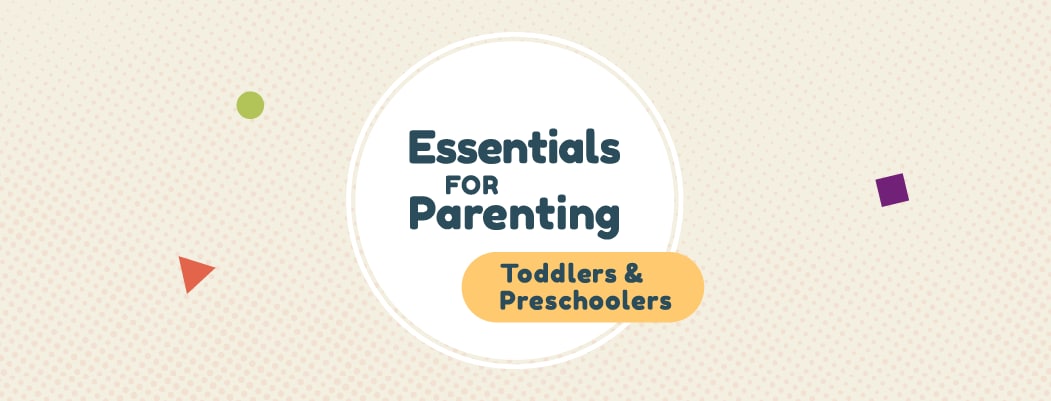
Essentials for Parenting Toddlers and Preschoolers
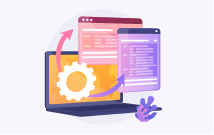
Cardiff Violence Prevention Model Toolkit
Violence is an urgent public health problem. CDC is committed to preventing violence so that everyone can be safe and healthy.
For Everyone
Public health.
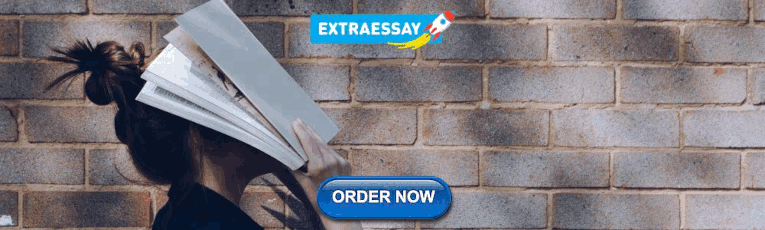
IMAGES
VIDEO
COMMENTS
Introduction. Depression is one of the most common mental health issues, ... This review may be used as an evidence base by those in public health, clinical practice, and research. This paper discusses key areas in depression research; however, an exhaustive discussion of all the risk factors and determinants linked to depression and their ...
Abstract. Major depression is a mood disorder characterized by a sense of inadequacy, despondency, decreased activity, pessimism, anhedonia and sadness where these symptoms severely disrupt and ...
INTRODUCTION. Depression is a common psychiatric disorder and a major contributor to the global burden of diseases. According to the World Health Organization, depression is the second-leading cause of disability in the world and is projected to rank first by 2030[].Depression is also associated with high rates of suicidal behavior and mortality[].
In line with the Research Domain Criteria Project launched by the National Institute of Mental Health (Insel, 2014; Insel et al., 2010), a distinguished aim in developing an integrated neuroscientific model of depression therefore has to be the separation of distinct aetiological and pathophysiological trajectories which, although eventually ...
Depression is a mood disorder that causes a persistent feeling of sadness and loss of interest. The American Psychiatric Association's Diagnostic Statistical Manual of Mental Disorders, Fifth Edition (DSM-5) classifies the depressive disorders into Disruptive mood dysregulation disorder; Major depressive disorder; Persistent depressive ...
Analysis of Published Papers. In the past decade, the total number of papers on depression published worldwide has increased year by year as shown in Fig. 1A. Searching the Web of Science database, we found a total of 43,863 papers published in the field of depression from 2009 to 2019 (search strategy: TI = (depression$) or ts = ("major depressive disorder$")) and py = (2009-2019), Articles).
The paper gives a complete overview of what is known about therapies for depression. ... we have developed a 'Meta-analytic Research Domain' (MARD) of all randomized trials of psychological treatments of depression. ... Introduction. About 280 million people worldwide suffer from a depressive disorder (WHO, 2022).
The idea that depression is the result of abnormalities in brain chemicals, particularly serotonin (5-hydroxytryptamine or 5-HT), has been influential for decades, and provides an important ...
Abstract. One of the most consistent findings in the depression literature is that stressful life events predict the onset and course of depressive episodes. Cognitive and biological responses to life stressors have both been identified, albeit largely independently, as central to understanding the association between stress and depression.
Abstract. Major depressive disorder (MDD) is a serious, debilitating, life-shortening illness that affects many persons of all ages and backgrounds. The point prevalence of MDD is high (2.3-3.2% in men; 4.5-9.3% in women) and the lifetime risk for MDD is 7% to 12% for men and 20% to 25% for women. MDD is a disabling disorder that costs the ...
Among 16 studies reporting the prevalence of clinically significant symptoms of depression across 23,469 Ph.D. students, the pooled estimate of the proportion of students with depression was 0.24 ...
According to the fall 2007 American College Health Association-National College Health Assessment ( 1), a national survey of approximately 20,500 college students on 39 campuses, 43.2% of the students reported "feeling so depressed it was difficult to function" at least once in the past 12 months.More than 3,200 university students reported being diagnosed as having depression, with 39.2% of ...
These include three scales which are among the 100 most cited papers across all fields of science 2: the Hamilton Rating Scale for Depression (HRSD) 3, the Beck Depression Inventory (BDI) 4 and ...
INTRODUCTION. Depression is described as a common and serious mood disorder that results in persistent feelings of sadness and hopelessness, as well as a loss of interest in activities that one once enjoyed (American Psychiatric Association [APA], 2013).Additional symptoms of depression include weight changes, difficulty sleeping, loss of energy, difficulty thinking or concentrating, feelings ...
At the same time, studies of trait anxiety suggest that moderate (vs. low) ELS is associated with greater self-reported anxiety. This study tested the hypothesis that stress inoculation effects are evident for implicit (nonconscious) but not explicit (conscious) aspects of anxiety. Methods: Ninety-seven healthy women were assessed for ELS and ...
Irrespective of other social experiences, higher loneliness scores at baseline were associated with higher depression symptom severity scores during 12 years of follow-up among adults aged 50 years and older. Interventions that reduce loneliness could prevent or reduce depression in older adults, which presents a growing public health problem worldwide.
The possible causes of depression are many and not yet well understood. However, it most likely results from an interplay of genetic vulnerability and environmental factors. Your depression research paper could explore one or more of these causes and reference the latest research on the topic. For instance, how does an imbalance in brain ...
Depression (also called major depressive disorder or clinical depression) is different. It can cause severe symptoms that affect how you feel, think, and handle daily activities, such as sleeping, eating, or working. It is an illness that can affect anyone— regardless of age, race, income, culture, or education. Research suggests that
The research is done on both gender, male and female. 29 students are female and. male students are 112. This portion of research is to check whether female students can have more chances. of ...
Introduction. Frequently under-recognized [1, 2], depression is a major contributor to the Global Burden of Diseases while being the most prevalent mental illness among geriatric populations [].Previous studies have established depression as a risk factor for age-related chronic conditions such as metabolic syndrome, diabetes mellitus, and cardiovascular disease [].
Introduction. India has about 250 million adolescents aged 10-19 years, comprising nearly one-fifth of India's population.1 The burden of mental health problems in adolescents is a growing concern globally.2 In India, the prevalence of psychiatric disorders among adolescents is estimated to be about 7.3%.3 Self-harm and depressive disorders are the leading cause of death and disability in ...
Introduction. A widespread and dangerous condition that has a detrimental impact on a person's feelings, thoughts, and behavior is depression, often known as major depressive disorder. This results in depressed sentiments or a loss of interest in past hobbies for the person (Holford, 2007). This can impair his or her capacity to perform at ...
More relevant to the current paper is the possibility that the exhaustive medicalization of the term; the wealth of non-behavior-analytic research data on biology and genetics, personality, and cognitive factors; and the emphasis on private events in depression—on how depression feels and on changing that feeling—may function to evoke ...
Semantic Scholar extracted view of "Introduction to IJRSM 35(2): Celebration of independent health research targeted at fostering safety of health interventions." by L. Ziganshina ... The true proportion of patients on depression drugs who can stop safely without relapse is likely considerably higher than the 50% found, and the length of taper ...
Accordingly, mental self-renewal is defined in this paper as the 'mind's ability to cultivate inner transformation, adapt continuously to change, and consequently produce novel and applicable outcomes in interaction with its environment' (Mononen & Kallio, 2024).In this research, the construct was built and elaborated on based on the previous research and analysed as follows:
WHO reported that 685 000 women died from BC in 2020, about 15% of cancer deaths among women. 1 On the other hand, the World Cancer Research Fund (WCRF) reported over 2.26 million new cases worldwide, contributing 15.5% of the new cases diagnosed in 2020. 2 It is estimated that about 268600 and 2670 new cases of invasive BC will be diagnosed in ...
The National Academies of Sciences, Engineering, and Medicine ( NASEM ), also known as the National Academies, is a congressionally chartered organization that serves as the collective scientific national academy of the United States. The name is used interchangeably in two senses: (1) as an umbrella term or parent organization for its three ...
Background: The ketogenic diet (KD) has been highly developed in the past for the treatment of epileptic pathological states in children and adults. Recently, the current re-emergence in its popularity mainly focuses on the therapy of cardiometabolic diseases. The KD can also have anti-inflammatory and neuroprotective activities which may be applied to the prevention and/or co-treatment of a ...
Trusted by surgeons for more than 40 years, Arthrex is a global leader in minimally invasive orthopedic products and procedures, rigorous clinical research, and world-class medical education.
A free online resource for parents and caregivers of 11 to 17-year-olds. Resources for Action can help communities use the best available evidence to prevent violence.