Phytoremediation for urban landscaping and air pollution control-a case study in Trivandrum city, Kerala, India
Affiliations.
- 1 Postgraduate Department and Research Centre of Botany, University College, University of Kerala, Trivandrum, Kerala, 695 034, India.
- 2 Postgraduate Department and Research Centre of Botany, University College, University of Kerala, Trivandrum, Kerala, 695 034, India. [email protected].
- PMID: 33164121
- DOI: 10.1007/s11356-020-11131-1
Air pollutant concentration of Trivandrum, the capital of Kerala, exceeded the limits of National Ambient Air Quality (NAAQ) standards, according to a study conducted in 2015 by NATPAC. These polluted corridors harbour vegetation on roadsides and traffic islands, planted solely for aesthetic appeal. Analysis of air pollution tolerance levels of existing plants can act as a scientific basis for efficient planning of the urban landscape. Sixty-seven species, including flowering, fruit-bearing, ornamental, shade-providing and timber-yielding species, were screened for their relative resistance to air pollution. Based on leaf pH, relative water content, chlorophyll and ascorbic acid levels, the Air Pollution Tolerance Indices (APTI) of each species were formulated and they were grouped into the following: tolerant, moderately tolerant, intermediate and sensitive groups. Agave americana (18.40), Cassia roxburghii (17.63), Anacardium occidentale (11.97), Cassia fistula (11.60), Mangifera indica (11.59) and Saraca asoca (10.88) may be considered for planting near green spaces like roundabouts and near pollution prone industrial areas, as they belong to tolerant category. Comparison of APTI during summer and monsoon also revealed the stability of Agave americana, Saraca asoca, Ficus benghalensis, Peltophorum pterocarpum, Ficus elastica, Ixora finlaysoniana, Mangifera indica, Canna indica and Delonix regia in maintaining pollution tolerance even during water disparity. Agave americana, Anacardium occidentale, Ficus elastica, Mangifera indica, Syzygium cumini, Ficus benghalensis, Nerium oleander and Ficus benjamina were found to be suited for mass planting, as was evident from their Anticipated Performance Indices (API).
Keywords: Air Pollution Tolerance Index; Air pollution; Anticipated Performance Index; Plant tolerance; Trivandrum; Urban landscaping.
- Air Pollutants* / analysis
- Air Pollution* / analysis
- Biodegradation, Environmental
- Environmental Monitoring
- Plant Leaves / chemistry
- Air Pollutants
The public interest litigation was instituted with a view to ensure healthy living and eradication of air pollution even though initially the provocation was the formation of fog in the city of Cochin. The scope of the writ petition was expanded by impleading industries and departments which were suspected to be the cause for the air pollution within the city and the adjoining areas of the city. An expert body like NEERI was entrusted with the work of study of the air pollution. The Government of Kerala had already notified under Sec.19(1) of the air (Prevention and Control of Pollution) Act, 1981 dated 31-7-1984 declaring the area coming under the Corporation of Cochin as an Air Pollution Control Area KEA No.1. The various sources of air pollution caused in Cochin are from industrial plants, motor vehicles, sewers and domestic drains emanating foul gases. Some of the major pollution are ammonia, sulphur dioxide, carbone monoxide, nitrogen dioxide etc..The Executive engineer, Air Pollution Control Cell of the Kerala State Pollution Control Board submitted a detailed report as per the directions of this Court. The report has given a background of the unusual phenomena called Smog in the city of Cochin, concluding that the presence of ammonia in humid winter conditions is one the principle cause of the often seen fog in Cochin city. The respondents replied that their factories have conformed to the standards prescribed and the emissions from their factories were within the norms prescribed by the Government. The Court, based on the reports of both these expert bodies, found that some of the major industries are yet to comply with the conditions of the consent orders. Similarly the Corporation of Cochin and the State Government are yet to make effective steps for the control of pollution, even with regard to the the emissions of gases from the open sewers,being one of the major air pollutant, and to pollution deriving from traffic congestion in the city. The Court held that the conclusion and recommendations of the NEERI are to be followed for maintaining the Cochin area clear of pollution,and are to be considered by the Pollution Control Board as to form part of the conditions for granting consent orders. Some of the recommendations of the NEERI are to be carried out by the State Government and the other departments. It direct The Executive Engineer, AIR Pollution Control Cell of the Kerala State Pollution Control Board to monitor and evaluate the performance of the industries and also the Corporation of Cochin and other bodies who are responsible in containing the air pollution in and around the city of Cochin and submit yearly reports on or before 31st December of the year, of the action taken in this regard to the Court.
Advertisement
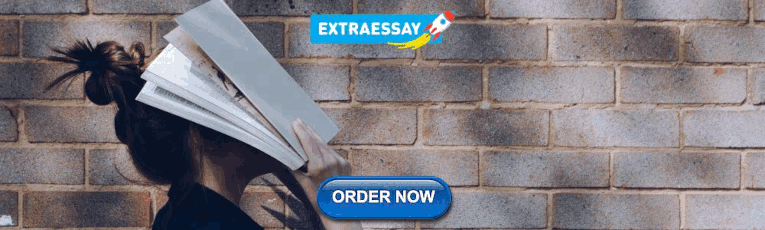
Impact of COVID-19 Lockdown on Ambient Air Quality in the Southwest Coastal Urban Regions of India
- Original Paper
- Published: 05 April 2023
- Volume 7 , pages 303–314, ( 2023 )
Cite this article
- George Thomas ORCID: orcid.org/0000-0002-7753-2350 1 ,
- Jobin Thomas 2 ,
- R. S. Devika 1 ,
- Anju Krishnan 1 ,
- Anju V. Mathew 1 &
- Amrutha J. Nair 1
1304 Accesses
Explore all metrics
The rapid growth of urban areas and population as well as associated development over recent decades have been a major factor controlling ambient air quality of the urban environment in Kerala (India). Being located at the southwestern fringe of the Indian peninsula, Kerala is one of the regions that has been significantly influenced by the activities in the Indian Ocean. The present study focuses on the effect of the COVID-19 lockdown (in 2021) on ambient air quality in the selected coastal metropolitan areas of Kerala. Although previous research studies reported improvement in ambient air quality in Kerala during the lockdown period, this study demonstrates the potential of onshore transport of air pollutants in controlling the air quality of coastal urban regions during the lockdown period. Data from the ambient air quality monitoring stations of the Kerala State Pollution Control Board in the urban areas of Thiruvananthapuram (TM), Kollam (KL), Kozhikode (KZ), and Kannur (KN) are used for the analysis. Temporal variation in the concentration of air pollutants during the pre-lockdown (PRLD), lockdown (LD), and post-lockdown (PTLD) periods (i.e., 1 March to 31 July) of 2021 is examined to assess the effect of lockdown measures on the National Air Quality Index (AQI). Results indicate a significant decline in the levels of air pollutants and subsequent improvement in air quality in the coastal urban areas. All the effect of lockdown measures has been evident in the AQI, an increase in the concentration of different pollutants including CO, SO 2 , and NH 3 during the LD period suggests contributions from multiple sources including onshore transport due to marine traffic and transboundary transport.
Similar content being viewed by others
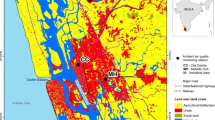
Non-uniform effect of COVID-19 lockdown on the air quality in different local climate zones of the urban region of Kochi, India
Impact of COVID-19 Lockdowns on Air Quality Trend in Trichy District of Tamil Nadu, India
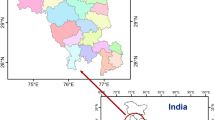
Temporal and spatial impact of lockdown during COVID-19 on air quality index in Haryana, India
Avoid common mistakes on your manuscript.
1 Introduction
The coronavirus disease (COVID-19) has been a recent global health crisis that impacted worldwide. The disaster has resulted in a tragically large number of fatalities and severe damage to people’s livelihoods and economic activities. Although the first COVID-19 case was reported in Wuhan (China), the disease affected practically every country in the world (Singh and Chauhan 2020 ; Tabarej and Minz 2022 ). Since the rapid transmission was facilitated by travel and mass gatherings, many countries adopted rigorous travel bans, physical distancing, and lockdowns forcing citizens to stay at home to slow down the rate of transmission. Since the lockdown was effective in controlling the transmission of the Severe Acute Respiratory Syndrome Coronavirus 2 (SARS-CoV-2) in India during the first wave (in 2020), the Government of India exerted another lockdown during the second surge of COVID-19 cases in 2021 through restrictions for travel as well as limiting industrial and commercial activities (Saharan et al. 2022 ; Kumar 2021 ). Similar to the effects of the lockdown in 2020, the restrictions imposed during the lockdown of 2021 also made noticeable impacts on the physical environment and air quality across India (Mahato and Pal 2022 ; Shukla et al. 2022 ; Ali et al. 2022 ).
Air pollution has been one of the most challenging problems in urbanized and industrialized areas worldwide and particularly in India (Khilnani and Tiwari 2018 ). Some of the urban areas in India are highly polluted (Lokhandwala and Gautam 2020 ) and about 90% of the population in India lives in areas where the air quality does not meet the WHO standards (Rodríguez 2020 ). Long-term exposure to air pollutants may result in a variety of health issues, mostly related to respiratory and cardiac systems, and even lead to premature death regions. Outdoor air pollution (in terms of particulate matter) in Kerala (a less-urbanized and less-industrialized State in India) has grown during recent years and the ambient air quality worsened at an alarming rate, specifically in the major urban centers, such as Thiruvananthapuram, Kochi and Kozhikode (Government of Kerala 2020). The number of automobiles in Kerala has increased more than 20 times since 1975, and Ernakulam, Thiruvananthapuram, and Kozhikode districts register the highest levels of automobile emissions (i.e., 40%). The major air pollutants in the region are particulate matter, sulphur dioxide (SO 2 ), nitrogen oxides (NO x ), carbon monoxide (CO), and ozone (O 3 ) (Jyothi et al. 2019 ). Numerous researchers addressed the ambient air quality issues of various parts of Kerala over the past few decades (e.g., Aggarwal 1999 ; Bency et al. 2003 ; Sarasamma and Narayanan 2014 ; Jyothi et al. 2019 ). Further, a few studies (Tobollik et al. 2015 ; Sumesh et al. 2017 ) highlighted the significance of the particulate matter in the ambient air quality and life expectancy of the regional population.
Although the lockdown measures during the COVID-19 pandemic wrecked the economy at global as well as regional scales, the lockdown period of 2020, as well as 2021, is characterized by improved air quality in urbanized and industrialized areas across India (Navinya et al. 2020 ; Mahato and Ghosh 2020 ; Das et al. 2021 ; Singh and Tyagi 2021 ). The reduction of air pollutant emissions was significant even in the less urbanized and less industrialized regions in India (e.g., Kerala) (e.g., Thomas et al. 2020 , 2021; Resmi et al. 2020 ). Even if anthropogenic activities were curtailed during the COVID-19 pandemic, it is reported that background air pollution (i.e., emissions from natural processes) predominated across South India, especially in the coastal regions (Madineni et al. 2021 ). The different studies carried out in Kerala to understand the effects of COVID-19 lockdowns in 2020 and 2021 observed unusually low emissions of air pollutants in the urbanized areas of the region. However, the impacts of the lockdowns on the ambient air quality of the coastal regions of Kerala, particularly in 2021, are less explored and discussed. Indeed, the lockdown period aids in understanding the natural sources of air pollution in such regions. Hence, the present study sought to address the impact of the COVID-19 lockdown (in 2021) on the ambient air quality in four coastal urban regions along the southwest coast of Kerala (India) during the lockdown associated with the second wave of COVID-19 in 2021.
2 Materials and Methods
2.1 study area.
The present study focuses on the variations in the ambient air quality of four coastal urban centers in Kerala (India), namely, Thiruvananthapuram, Kollam, Kozhikode, and Kannur (Fig. 1 ). Physiographically, the region is classified into three major zones: highland (> 75.0 m above the mean sea level), midland (7.6–75.0 m above the mean sea level) and lowland and coastal plains (< 7.5 m above the mean sea level). The Western Ghats influences the physiographic variations and the orography determines the climate of Kerala. The windward slopes of the Western Ghats including the coastal plains enjoy a tropical humid climate (Am) with a mean annual rainfall of about 2800 mm (Thomas and Prasannakumar 2016 ) and a mean annual temperature of about 25 °C.
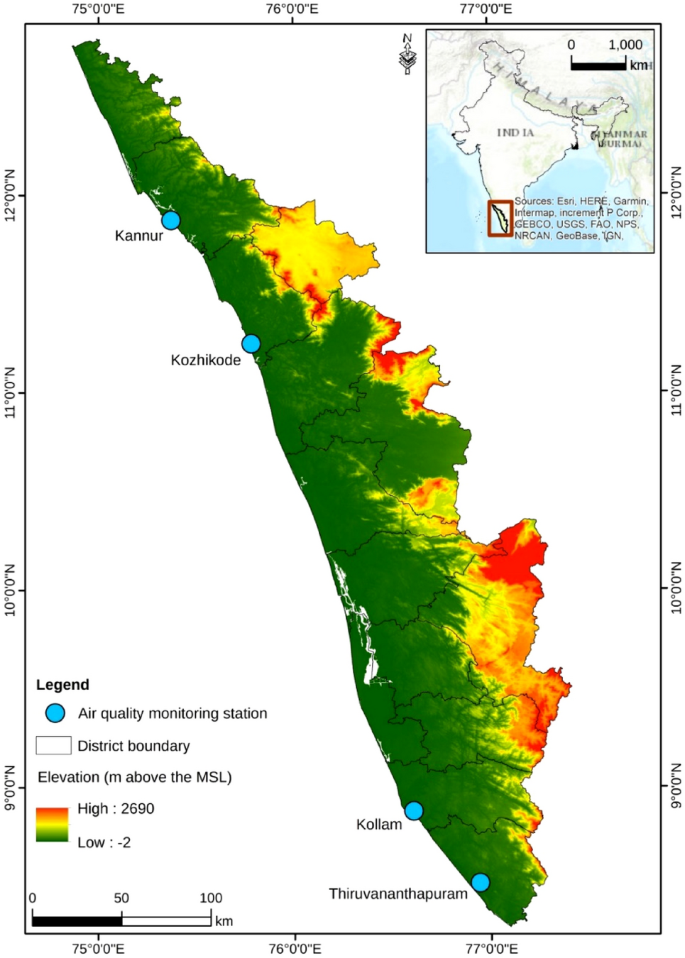
Ambient air quality monitoring stations (used in this study) of Kerala (India)
Major anthropogenic sources of air pollution in Kerala are industries and traffic. Although Kerala does not have large-scale industrial complexes, such as in many other states of India, the region supports several industrial clusters and most of them are located near the coastal urban regions. Based on the census 2011 report, Kerala has a population of 33,387,677 (i.e., 859 persons km −2 ), which is nearly 3% of the population of India ( https://censusindia.gov.in ). Further, the region witnessed rapid urbanization in the past decades, where the urban population of Kerala increased from 25.9% in 2001 to 47.7% in 2011 (Lal and Nair 2017 ). The region is well connected via road, railway, air, and water transport with a road density of 3.9 km −2 and recorded an increase in road traffic (12–14%) annually. Being located at the southwestern fringe of the Indian peninsula, marine traffic along the coastal routes of the Arabian Sea also serves as a critical source of air pollutants (Thangalakshmi and Sivasami 2022 ).
2.2 Ambient Air Quality Monitoring Stations
2.2.1 thiruvananthapuram (tm).
Thiruvananthapuram (TM) is the capital of Kerala and the headquarters of the Thiruvananthapuram district with a metropolitan population of about 1.7 million (Census of India 2011 ). The ambient air quality monitoring station is located at Plammoodu (Fig. 1 ; 76° 56′ 36" E and 8° 30′ 53" N), which is roughly 10 km from the shoreline. The region is accessible through road, railway, air, and water transportation modes. The city is a major hub of commercial and retail activities in Kerala and the urban area supports multiple industrial and chemical manufacturing units. Being the administrative capital of Kerala, the region hosts numerous government administrative offices. The region is also one of the major information technology hubs and academic nuclei in India. The mean annual rainfall of the region is about 1900 mm and the temperature ranges from 20 to 39 °C (Ansar et al. 2012 ; Nair et al. 2017 ).
2.2.2 Kollam (KL)
Kollam (KL) is another major urban area in the southern part of Kerala and is mainly developed along the coastal stretch of the Kollam district (Fig. 1 ). With a metropolitan population of 1.3 million (Census of India 2011 ), Kollam is one of the most densely populated areas in Kerala. The ambient air quality monitoring station is located at Kadapakkada (76° 36′ 26" E and 8° 52′ 43." N). A large number of industries (primarily chemical and food processing) exist in the region as the coastal belt is blessed with placer mineral deposits and due to the occurrence of one of the major fishing harbours in Kerala (Sarasamma and Narayanan 2014 ). The region receives an annual rainfall of 2400 mm with temperatures varying between 23 and 36 °C (Nair et al. 2017 ).
2.2.3 Kozhikode (KZ)
Kozhikode district is one of the developing urban centers with significant socioeconomic significance in Kerala. The ambient air quality monitoring station is located at Palayam (Fig. 1 ; 75° 47′ 3" E and 11° 14′ 56" N). It has a metropolitan population of over 2 million, making it one of the largest metropolitan areas in Kerala. The region is a major trade hub and commercial center in northern Kerala and supports a wide range of industries ranging from sawmills to chemical manufacturing. The annual average rainfall of the city is 3500 mm (Surendran et al. 2019 ) and the mean annual temperature is 26 °C.
2.2.4 Kannur (KN)
With 1.7 million population in the metropolitan region, Kannur is the sixth-most urbanized area in Kerala (Census of India 2011 ). The ambient air quality monitoring station at Kannur is geographically situated at Thavakkara (Fig. 1 ; 75° 21′ 19" E and 11° 52′ 8" N). The ambient air temperature ranges from 20 to 33 °C and the mean annual rainfall is 3400 mm (Whitaker and Whitaker 2006 ).
2.3 Data Collection
The air pollutant levels recorded in the four ambient air quality monitoring stations in Thiruvananthapuram, Kollam, Kozhikode, and Kannur districts (Fig. 1 ) were used for the present study. The daily mean concentrations of major pollutants, viz., particulate matter with a diameter of less than 2.5 µm and 10 µm (PM 2.5 and PM 10 ), carbon monoxide (CO), oxides of nitrogen (NO and NO 2 ), sulphur dioxide (SO 2 ), ammonia (NH 3 ) and ozone (O 3 ) from March 1 to July 31 in 2021 were used to calculate the air quality index (AQI) (CPCB 2014 ). Hourly data of eight air pollutants, including PM 2.5 , PM 10 , CO, NO, NO 2 , NH 3 , SO 2 , and O 3 are available in the online air quality data portal of the Central Pollution Control Board, Government of India. ( https://app.cpcbccr.com/ccr/#/caaqm-dashboard-all/caaqm-landing ). The entire study period was classified into three: the pre-lockdown (PRLD) period from 1 March to 7 May, the lockdown (LD) period from 8 to 31 May, and the post-lockdown (PTLD) period between 1 June and 31 July. The AQI was calculated from the concentrations of these eight pollutants (up to a 24 h averaging period), with a minimum of three pollutants (at least one of which is PM 2.5 or PM 10 ) (Eq. 1 ). The daily average value was calculated for all the stations starting at 12:00 am for a period of 24 h.
where B HI and B LO as breakpoint concentrations greater and smaller to Ci; Ci is the concentration of pollutant i; IN HI and IN LO are the corresponding AQI values. The AQI is classified as good (0–50), satisfactory (51–100), moderate (101–200), poor (201–300), very poor (301–400), and severe (401–500) (CPCB 2014 ).
3 Results and Discussion
3.1 aqi at thiruvananthapuram (tm).
The AQI calculated at the air quality monitoring station of TM showed significant temporal variability between the PRLD, LD, and PTLD periods (Table 1 ). The AQI at TM shows significant improvement during the LD period (median = 44; 24–64) compared to the PRLD (52; 34–105) and PTLD (55; 32–100) periods (Fig. 2 ). Majority of the days during the PRLD (56%) and PTLD (60%) periods in 2021 recorded ‘satisfactory’ air quality (50 < AQI ≤ 100), whereas about 75% of the total days had ‘good’ air quality (AQI ≤ 50) during the LD period. Moreover, 46% of the days in the PRLD and 40% of the PTLD periods also recorded ‘good’ air quality at TM. However, a few days in the PRLD period appeared to have moderate air quality also (Fig. 2 ). The AQI statistics at TM indicate a clear declining trend in the AQI values during the lockdown restrictions. However, the air quality was reinstated to its original level (i.e., PRLD period) after the lifting of the lockdown measures. The prominent pollutant for each day was determined for the entire study period (Fig. 2 ). The relative dominance of different air pollutants during the different periods (i.e., PRLD, LD, and PTLD) indicates that PM 10 was the dominant determining the AQI during the PRLD period. It may be noted that the AQI of about 68% of the total days during the PRLD period was influenced by PM 10 . However, CO was the prominent pollutant influencing the AQI during the LD (62%) and PTLD (70% days) periods, whereas PM 10 has only secondary significance.
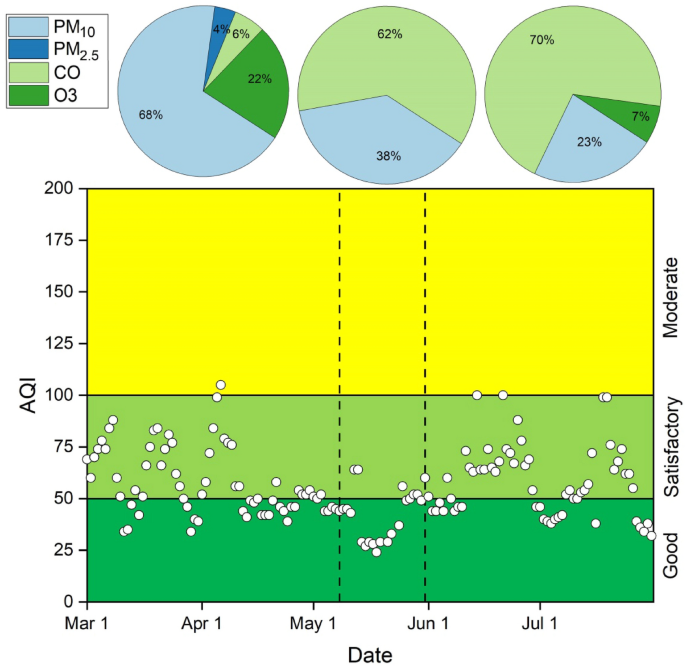
Daily time series of AQI recorded at TM. Relative dominance of different air pollutants influencing the AQI during the PRLD, LD, and PTLD periods is also shown in the pie charts
3.2 AQI at Kollam (KL)
A remarkable variation in air quality is noted at KL between the PRLD, LD, and PTLD periods (Table 1 ). Further, the temporal variability of the AQI at KL indicates an improvement in the air quality during the LD period (median = 49; 30–105) compared to the PRLD (89; 48–205) and PTLD (75; 47–124) periods (Fig. 3 ). About 58% of the days during the LD period recorded ‘good’ air quality, while 37% showed ‘satisfactory’ and 5% with moderate AQI. However, during the PRLD, 63% of the days had ‘satisfactory’ air quality, and 37% of the days were characterized by ‘moderate’ air quality. Although the AQI increased during the PTLD than the LD period, 83% of the days had satisfactory air quality while ‘moderate’ and ‘good’ air quality levels were noted during 12% and 5% of days, respectively. Similar to the temporal pattern of the AQI at TM, KL also showed an increasing trend in the AQI after lifting the lockdown restrictions. However, the AQI during the PTLD period did not rebound to the AQI levels of the PRLD period (Fig. 3 ). Among the different air pollutants recorded at KL, PM 10 (62% days) and PM 2.5 (38%days) dominated during the PRLD period (Fig. 3 ). Further, PM 10 was the prominent pollutant during the PRLD and LD periods, but CO was the prominent pollutant during the PTLD period.
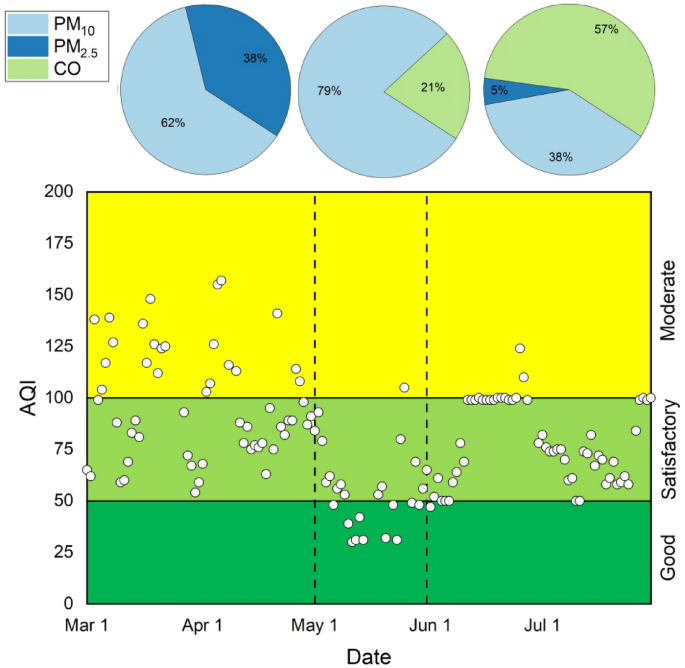
Daily time series of the AQI recorded at KL. Relative dominance of different air pollutants influencing the AQI during the PRLD, LD, and PTLD periods is also shown
3.3 AQI at Kozhikode (KZ)
The effect of the lockdown measures on air pollutant concentrations at KZ was evident in the improvement of air quality. The AQI showed a marked improvement at KZ during the LD period (median = 37; 49–76) compared to the PRLD (76; 51–86) and PTLD (49; 27–85) periods. About 95% of the days during the LD period recorded ‘good’ air quality while another 5% of the days had ‘satisfactory’ AQI levels (Fig. 4 ). However, ‘satisfactory’ air quality levels prevailed during the PRLD period. Although the withdrawal of the lockdown measures increased the AQI, 56% of the days in the PTLD period remained ‘good’, while 44% of the days had a ‘satisfactory’ air quality level. Although PM 10 was the prominent pollutant determining the AQI at KZ, CO was influential during the LD and PTLD periods (Fig. 4 ).
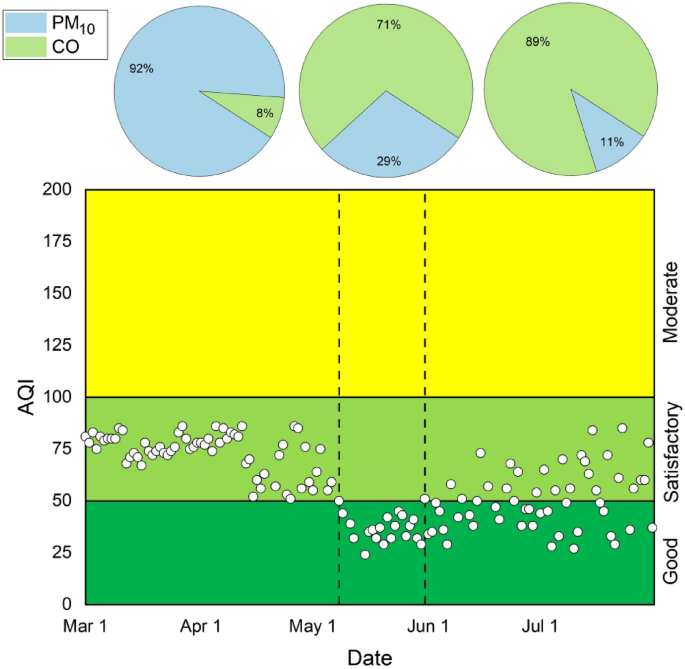
Daily time series of AQI recorded at KZ. Relative dominance of different air pollutants influencing the AQI during the PRLD, LD, and PTLD periods is also shown
3.4 AQI at Kannur (KN)
The temporal variability of the AQI at KN also indicated an improvement in air quality during the LD period (Median = 86; 47–127) compared to the PRLD (102; 66–258) and PTLD (54; 32–135) periods. About 77% of the days in the LD period recorded ‘satisfactory’ air quality levels and 23% of the days showed ‘moderate’ AQI values (Fig. 5 ). However, during the PRLD period, 47% of the days had ‘satisfactory’ level and 53% of the days appeared to have ‘moderate’ air quality. Interestingly, 41% of the days during the PTLD period were characterized by ‘good’ air quality, while 44% of the days registered ‘satisfactory’ and 15% of the days had ‘moderate’ air quality levels. A declining trend of the AQI values at KN is noted due to the lockdown restrictions. However, the trend continued in the PTLD period also. PM 10 was the dominant pollutant influencing the AQI at KN during all the periods. However, NO 2 was also significant during the PTLD period (Fig. 5 ).
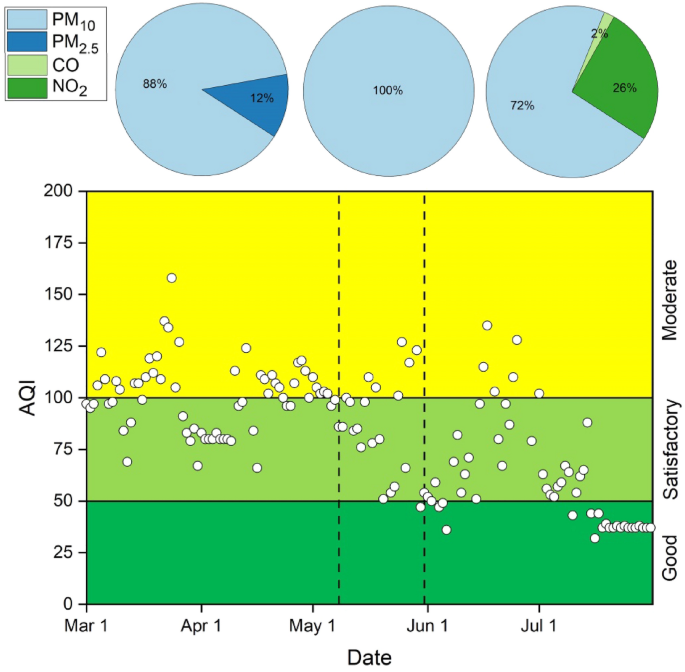
Daily time series of AQI recorded at KN. Relative dominance of different air pollutants influencing the AQI during the PRLD, LD, and PTLD periods is also shown
The reduction in the AQI values in the LD period compared to the PRLD period at all the monitoring stations indicates an improvement in the ambient air quality due to the lockdown measures. However, the effect of the lockdown was spatially varying where the largest changes in the AQI were noted at KZ (51%) and KL (45%), whereas the changes at TM and KN were 13% and 16%, respectively. Among the different prominent pollutants influencing the AQI, PM 10 was the determinant during the PRLD period at all the monitoring stations. Although PM 10 was the prominent pollutant at KL and KN in the LD period, CO was the major pollutant at TM and KZ. Further, CO was the prominent pollutant during the PTLD period at all the stations except KN, where PM 10 was the significant one controlling the AQI. In general, a gradual transition of the prominent air pollutant determining the AQI is noted from PRLD (PM 10 ) to PTLD (CO).
3.5 Variability of Air Pollutant Concentrations at Different Monitoring Stations
Figure 6 shows the daily time series of the concentration of different air pollutants at all the monitoring stations. The manifestations of the AQI pattern at the different stations in Kerala are indicative of improvement in air quality due to a decrease in air pollutant levels during the LD period. However, the rate of reduction of the pollutant concentration during the LD period differs among the stations (Fig. 6 ). For instance, the concentration of all the air pollutants (except SO 2 ) showed a significant reduction during the LD period at TM compared to the PRLD period. Significant reductions are noted in the particulate matter concentrations (i.e., 72 and 61% for PM 2.5 , and PM 10 , respectively), while NO (8%), NO 2 (29%), NH 3 (27%), CO (25%) and O 3 (32%) levels also decreased during the LD period (Table 2 ). However, the SO 2 levels in the LD period increased by about 7% compared to the PRLD period. The PTLD period at TM witnessed an increase in the concentration of most of the pollutants (i.e., PM 2.5 -11%, PM 10 -36%, NO 2 -24%, NH 3 -20%, SO 2 -6% and CO-17%) compared to the LD period. On the other hand, NO and O 3 levels were reduced by 24% and 21%, respectively. The concentration of pollutants in the PTLD period was also lesser than in the PRLD period, i.e., such as PM 2.5 (69%), PM 10 (47%), NO (30%), NO 2 (12%), NH 3 (12%), CO (13%) and O 3 (47%). But SO 2 levels of the PTLD period were about 13% higher than the PRLD period (Table 2 ).
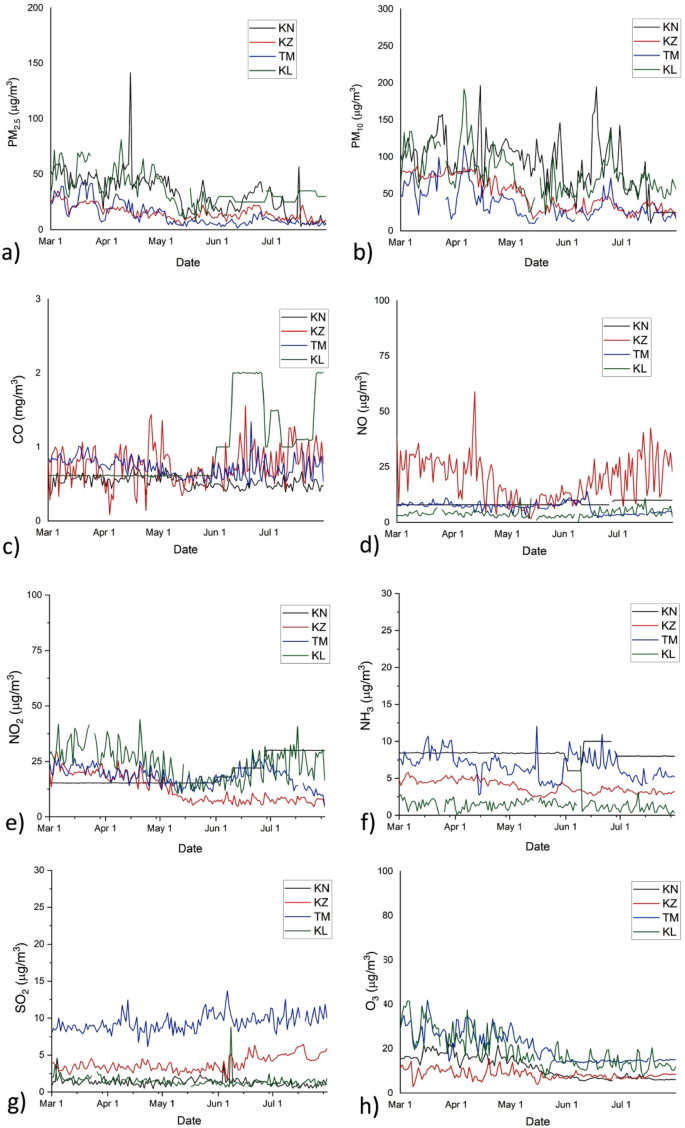
Daily time series of the concentration of different air pollutants recorded at TM, KL, KZ, KN: a PM 2.5 , b PM 10 , c CO, d NO, e NO 2 , f NH 3 , g SO 2 and h O 3
A remarkable reduction in the concentration of all the pollutants except NH 3 and CO was observed at KL during the LD period compared to the PRLD period (Fig. 6 ). The concentration of PM 2.5 , PM 10 , NO, NO 2 , SO 2, and O 3 was reduced by 57%, 47%,18%, 47%, 29%, and 44%, respectively (Table 2 ). However, the NH 3 level was increased by 18% and CO concentration remained unchanged during the LD period. However, during the PTLD period, all the air pollutants except NH 3 and O 3 showed a notable increase than the LD period. Among the different pollutants, the concentration of CO doubled in the PTLD period compared to the LD period. A comparison of the PRLD and PTLD periods at KL indicates that PM 2.5 , PM 10 , NO 2 , NH 3 , SO 2, and O 3 concentrations were reduced during the PTLD period by 40%, 32%, 22%, 20%, 18%, and 49%, respectively. However, NO and CO levels of the PTLD period were significantly higher (15% and 133%, respectively) than that of the PRLD period (Table 2 ).
The concentration of all the air pollutants at KZ showed a decrease during the LD period than the PRLD period (Fig. 6 ). The concentration of PM 2.5 , PM 10 , NO, NO 2 , NH 3 , SO 2 , CO, and O 3 were lowered by 42%, 57%, 61%, 59%, 34%, 9%, 25%, and 22%, respectively (Table 2 ). However, the PTLD period witnessed an increase in the concentration of PM 2.5 (12%), NO (136%), NH3 (6%), SO 2 (53%) CO (33%), and O 3 (4%) compared to the LD period. While the rate of increase in the PM 10 concentration in the PTLD period (compared to the PRLD period) was insignificant, NO 2 concentration was reduced by about 5%. Further, PM 2.5 , PM 10 , NO, NO 2 , NH 3 and O 3 levels in the PTLD period were lower than the PRLD period by 35%, 56%, 9%, 61%, 30%, and 19% respectively (Table 2 ). However, the concentration of SO 2 showed an increase (38%) during the PTLD compared to the PRLD period.
The concentration of PM 2.5 (49%), PM 10 (15%), CO (17%), and O 3 (40%) at KN decreased during the LD period compared to their concentration during the PRLD period (Fig. 6 ; Table 2 ). While the concentration of NO, NO 2, and NH 3 remained unchanged during the LD period and SO 2 increased by 21%. The particulate matter levels (PM 2.5 —19%, PM 10 —26%), SO 2 (29%), and O 3 (33%) showed a further decrease in the PTLD period than the LD period, whereas oxides of nitrogen increased (NO by 18% and NO 2 by 70%). The concentration of most of the air pollutants at KN showed a significant reduction in the PTLD period than the PRLD period, i.e., PM 2.5 (58%), PM 10 (37%), SO 2 (14%), CO (17%) and O 3 (60%). Contrastingly, the concentration of NO and NO 2 increased by 18% and 70% respectively (Table 2 ).
In general, all the ambient air quality stations in the coastal urban areas of Kerala witnessed a reduction in air pollutants during the LD period compared to the PRLD period. However, the concentration of various air pollutants returned to its original level (i.e., PRLD) after relaxing the lockdown measures during the PTLD period. Thomas et al. ( 2022 ) noted such behaviour of air pollutants in the Kochi urban area of Kerala. However, most of the pollutants recorded at KN show a continuation of the declining trend of the LD period in the PTLD period also.
4 Discussion
The results of this study indicate a reduction in the different air pollutants and an overall improvement in the ambient air quality in the coastal urban regions of Kerala in the LD period of 2021. Air pollution in Kerala is primarily contributed by vehicular traffic, industries, and construction works (Jyothi et al. 2019 ). Since the lockdown restricts most of such activities, the emission of pollutants from these sources would be lesser in the LD period compared to the PRLD period. The withdrawal of lockdown restrictions during the PTLD period resulted in an increase in the concentrations of prominent pollutants during PTLD period as compared to the LD period. Most of the pollutant species recorded in the monitoring stations follow such a trend, i.e., a drastic reduction in the LD period compared to the PRLD period and an increase in the PTLD period. Among the different pollutant species, particulate matter (PM 2.5 and PM 10 ) showed a significant reduction in the LD period. Since the particulate matter levels are mostly associated with construction activities and vehicular traffic and hence, cessation of such activities was reflected in the particulate matter levels. Since many of the gaseous pollutants, such as oxides of nitrogen (NO and NO 2 ) and CO are also primarily contributed by vehicular traffic, travel restrictions during the LD period could have reduced their concentration in the LD period compared to the PRLD period. Similarly, many of the industries of Kerala are located near the ambient air quality monitoring stations, e.g., Travancore Titanium Products (TM), and Kerala Minerals and Metals Limited (KL). Hence, restrictions in industrial activities are reflected in the reduced concentration of air pollutants. Although many of the pollutants in the PTLD period bounced back to their concentration to the level of the PRLD period, some of the pollutants (e.g., PM 2.5 and PM 10 ) did not return to their previous level, which is attributed to the effect of seasonal (monsoon) rainfall purging the particulate matter in the atmosphere.
It is interesting to note that the concentration of some of the pollutants got amplified during the LD period at many stations, even with the cessation of industrial and construction activities and travel restrictions. For instance, SO 2 recorded at TM and KN showed an increased concentration during the LD period (9.5 and 1.7 μg/m 3 , respectively) than the PRLD period (8.9 and 1.4 μg/m 3 ) (Table 2 ). However, the concentration of O 3 at most of the stations (TM, KL, and KN) showed a gradual reduction from the PRLD to PTLD period. Moreover, many of the pollutants (e.g., PM 2.5 , PM 10 , NH 3, and CO) at KN also exhibited a gradual reduction throughout the period (Table 2 ). Further, the temporal pattern of different pollutants also differs among the monitoring stations, which is an indication of the differences in the regional activities resulting in the emissions. Except for KN, CO levels at all the monitoring stations during the PTLD period either returned to the PRLD period or increased the concentration than the PRLD period. This is evident in the dominance of CO as the major factor determining the AQI in the PTLD period in most of the stations. Even during the LD period, CO was significant in controlling the AQI at many of the stations (TM, KL, and KZ) (Figs. 2 , 3 , and 4 ). Although CO is primarily contributed by automobile emissions and industries, emissions from other sources such as household activities, biomass burning, and agriculture activities could also be significant in the regional context. Although noted only at KL, the concentration of NH 3 increased by 18% during the LD compared to the PRLD period. Agricultural activities particularly before the onset of monsoon could be the main source of NH 3 emissions apart from other minor sources (Behera et al. 2013 ).
In the southwest coastal region, the Arabian Sea could be a substantial source of pollutants in the coastal region. The increase in SO 2 levels during the LD period particularly at TM could be attributed to the marine sources as Shanavas et al. ( 2020 ) observed that the emissions from marine traffic contribute to the concentration of SO 2 along the coastal regions of south India. According to Madineni et al. ( 2021 ), the relative increase in the concentration of some trace gases in the southern part of India suggests a contribution from other sources including emissions from natural sources (e.g., forest fires, biomass burning) and long-range transport. The results of the back-trajectory analysis by Thomas et al. ( 2020 ) also indicate that pollutant transport from nearby regions might have affected the concentration of various air pollutants in the region during the LD period of 2020. It is indisputable that the lockdown measures help reduce air pollutants thereby improving the air quality of the coastal regions of Kerala in 2021. However, the characteristic behaviour of many gaseous pollutants deviating from the generalized pattern is indicative of other sources of air pollution which are unaffected by the lockdown measures.
5 Summary and Conclusion
The impacts of the lockdown (due to the surge in COVID-19 cases in Kerala in 2021) on the ambient air quality of the coastal urban areas of Kerala (India) were investigated in this study. Temporal variability of different air pollutants (PM 2.5 , PM 10 , CO, NO, NO 2 , NH 3 , SO 2 , and O 3 ) and the AQI at different ambient air quality monitoring stations (TM, KL, KZ, and KN) was analyzed to understand the effect of lockdown measures on the pollutant concentration and air quality. We observed a notable reduction in the concentration of major pollutants and the enhancement of air quality at all the coastal air quality monitoring stations during the LD period compared to the PRLD period. However, the improvement in the ambient air quality was more significant at KZ (51%) and KL (45%), than at TM (13%) and KN (16%). Among the different pollutants, significant reductions in the LD period were noted in the particulate matter (PM 2.5 and PM 10 ) concentrations than the gaseous pollutants. The prominent pollutant determining the AQI at all the monitoring stations in the PRLD period was PM 10 which gradually changes to CO during the PTLD period. Although the lockdown restrictions imposed on anthropogenic activities were reflected in the reduction of air pollutant concentration and enhancement of ambient air quality across the region, the increase in the concentration of the pollutants, viz., CO, SO 2, and NH 3 during the LD period attributes to the contribution from the sources other than vehicular traffic, industries, and construction activities. Such sources include household emissions, burning of biomass, agriculture activities, onshore as well as long-range transport. Further, the relative influence of such sources strongly varies across the region, and extended research to understand the regional factors controlling the ambient air quality of Kerala (India).
Data Availability
The Daily CPCB AQI data for more than 200 Indian stations is available open-source at https://app.cpcbccr.com/ccr/#/caaqm-dashboard-all/caaqm-landing .
Aggarwal AL (1999) Emission inventory for an industrial area of India. Environ Monit Assess 55(2):299–304. https://doi.org/10.1023/A:1005947026732
Article Google Scholar
Ali SY, Mukherjee P, Sunar S, Saha S, Saha P, Dutta S, Goswami S (2022) Significant effect of COVID-19 induced lockdown on air quality of the Indian Metropolitan City Kolkata using Air Quality Index and Health Air Quality Index. Hum Ecol Risk Assess 28(7):762–782. https://doi.org/10.1080/10807039.2022.2093155
Ansar S, Dhanya CR, Thomas G, Chandran A, John L, Prasanthi S, Vishnu R, Zachariah EJ (2012) A study of urban/rural cooling rates in Thiruvananthapuram. Kerala J Ind Geophys Union 16(1):29–36
Google Scholar
Behera SN, Sharma M, Aneja VP, Balasubramanian R (2013) Ammonia in the atmosphere: a review on emission sources, atmospheric chemistry and deposition on terrestrial bodies. Environ Sci Pollut Res 20(11):8092–8131. https://doi.org/10.1007/s11356-013-2051-9
Bency KT, Jansy J, Thakappan B, Kumar B, Sreelekha TT, Hareendran N, Nair P, Nair M. A (2003) Study on the air pollution related human diseases in Thiruvananthapuram City, Kerala. Proceedings of the Third International Conference on Environment and Health, Chennai, India p15–17.
Census of India (2011) Urban Agglomerations/Cities having population 1 million and above. Office of Registrar General of India, Government of India, New Delhi.
CPCB (2014) National Air Quality Index Report. Central Pollution Control Board, New Delhi.
Das M, Das A, Sarkar R, Saha S, Mandal P (2021) Regional scenario of air pollution in lockdown due to COVID-19 pandemic: Evidence from major urban agglomerations of India. Urban Clim 37:100821. https://doi.org/10.1016/j.uclim.2021.100821
Economic Review (2019) Kerala State Planning Board, Thiruvananthapuram, Kerala, India
Jyothi SN, Kartha K, Mohan A, Pai J, Prasad G (2019) Analysis of air pollution in three cities of kerala by using air quality index. J Phys Conf Ser 1362(1):012110. https://doi.org/10.1088/1742-6596/1362/1/012110
Khilnani GC, Tiwari P (2018) Air pollution in India and related adverse respiratory health effects: past, present, and future directions. Curr Opin Pulm Med 24(2):108–116. https://doi.org/10.1097/MCP.0000000000000463
Kumar S (2021) Second wave of COVID-19: emergency situation in India. J Travel Me 28(7):taab082. https://doi.org/10.1093/jtm/taab082
Lal P, Nair SB (2017) Urbanization in Kerala—What Does the Census Data Reveal? Indian J Human Develop 11(3):356–386. https://doi.org/10.1177/09737030187632
Lokhandwala S, Gautam P (2020) Indirect impact of COVID-19 on environment: A brief study in Indian context. Environ Res 188:109807. https://doi.org/10.1016/j.envres.2020.109807
Madineni VR, Dasari HP, Karumuri R, Viswanadhapalli Y, Perumal P, Hoteit I (2021) Natural processes dominate the pollution levels during COVID-19 lockdown over India. Sci Rep 11(1):1–4. https://doi.org/10.1038/s41598-021-94373-4
Mahato S, Ghosh KG (2020) Short-term exposure to ambient air quality of the most polluted Indian cities due to lockdown amid SARS-CoV-2. Environ Res 188:109835. https://doi.org/10.1016/j.envres.2020.109835
Mahato S, Pal S (2022) Revisiting air quality during lockdown persuaded by second surge of COVID-19 of megacity Delhi India. Urban Clim 41:101082. https://doi.org/10.1016/j.uclim.2021.101082
Nair A, Joseph KA, Nair KS (2014) Spatio-temporal analysis of rainfall trends over a maritime state (Kerala) of India during the last 100 years. Atmos Environ 88:123–132. https://doi.org/10.1016/j.atmosenv.2014.01.061
Navinya C, Patidar G, Phuleria HC (2020) Examining effects of the COVID-19 national lockdown on ambient air quality across urban India. Aerosol Air Qual Res 20(8):1759–1771. https://doi.org/10.4209/aaqr.2020.05.0256
Resmi CT, Nishanth T, Kumar MS, Manoj MG, Balachandramohan M, Valsaraj KT (2020) Air quality improvement during triple-lockdown in the coastal city of Kannur, Kerala to combat Covid-19 transmission. PeerJ 8:e9642. https://doi.org/10.7717/peerj.9642
Rodríguez-Urrego D, Rodríguez-Urrego L (2020) Air quality during the COVID-19: PM2.5 analysis in the 50 most polluted capital cities in the world. Environ Pollut 266:115042. https://doi.org/10.1016/j.envpol.2020.115042
Saharan US, Kumar R, Tripathy P, Sateesh M, Garg J, Sharma SK, Mandal TK (2022) Drivers of air pollution variability during second wave of COVID-19 in Delhi India. Urban Clim 41:101059. https://doi.org/10.1016/j.uclim.2021.101059
Sarasamma JD, Narayanan BK (2014) Air quality assessment in the surroundings of KMML industrial area, Chavara in Kerala. South India Aerosol Air Qual Res 14(6):1769–1778. https://doi.org/10.4209/aaqr.2013.10.0327
Shanavas AK, Zhou C, Menon R, Hopke PK (2020) PM10 source identification using the trajectory based potential source apportionment (TraPSA) toolkit at Kochi. India Atmos Pollut Res 11(9):1535–1542. https://doi.org/10.1016/j.apr.2020.06.019
Shukla S, Khan R, Saxena A, Sekar S, Ali EF, Shaheen SM (2022) Appraisal of COVID-19 lockdown and unlocking effects on the air quality of North India. Environ Res 204:112107. https://doi.org/10.1016/j.envres.2021.112107
Singh J, Tyagi B (2021) Transformation of air quality over a coastal tropical station Chennai during COVID-19 lockdown in India. Aerosol Air Qual Res 21(4):200490. https://doi.org/10.4209/aaqr.200490
Singh RP, Chauhan A (2020) Impact of lockdown on air quality in India during COVID-19 pandemic. Air Qual Atmos Health 13(8):921–928. https://doi.org/10.1007/s11869-020-00863-1
Sumesh RK, Rajeevan K, Resmi EA, Unnikrishnan CK (2017) Particulate matter concentrations in the southern tip of India: temporal variation, meteorological influences, and source identification. Earth Syst Environ 1(2):1–8. https://doi.org/10.1007/s41748-017-0015-9
Surendran U, Anagha B, Gopinath G, Joseph EJ (2019) Long-term rainfall analysis towards detection of meteorological drought over Kozhikode District of Kerala. J Clim Change 5(2):23–34. https://doi.org/10.3233/JCC190010
Tabarej MS, Minz S (2022) Spatio-temporal changes pattern in the hotspot’s footprint: a case study of confirmed, recovered and deceased cases of Covid-19 in India. Spat Inf Res 14:1–2. https://doi.org/10.1007/s41324-022-00443-8
Thangalakshmi S, Sivasami K (2022) Climate change over South Indian coast due to aerosol and air pollution. Int Adv Res J Sci Eng Technol 9(6):56–65. https://doi.org/10.17148/IARJSET.2022.9609
Thomas J, Prasannakumar V (2016) Temporal analysis of rainfall (1871–2012) and drought characteristics over a tropical monsoon-dominated State (Kerala) of India. J Hydrol 534:266–280. https://doi.org/10.1016/j.jhydrol.2016.01.013
Thomas J, Jainet PJ, Sudheer KP (2020) Ambient air quality of a less industrialized region of India (Kerala) during the COVID-19 lockdown. Anthropocene 32:100270. https://doi.org/10.1016/j.ancene.2020.100270
Thomas G, Thomas J, Mathew AV, Devika RS, Krishnan A, Nair AJ (2022) Non-uniform effect of COVID-19 lockdown on the air quality in different local climate zones of the urban region of Kochi India. Spat Inf Res. https://doi.org/10.1007/s41324-022-00481-2
Tobollik M, Razum O, Wintermeyer D, Plass D (2015) Burden of outdoor air pollution in Kerala, India—a first health risk assessment at state level. Int J Environ Res Public Health 12(9):10602–10619. https://doi.org/10.3390/ijerph120910602
Whitaker R, Whitaker S (2006) Analysis of Snakebite Data from Pappinisseri Vishachikilsa Society, Kannur, Kerala (India). Calicut Medical Journal 4(2):e2
Download references
This research did not receive any specific grant from funding agencies in the public, commercial, or not-for-profit sectors.
Author information
Authors and affiliations.
Department of Physics, Catholicate College, Pathanamthitta, Kerala, 689645, India
George Thomas, R. S. Devika, Anju Krishnan, Anju V. Mathew & Amrutha J. Nair
Department of Geological and Mining Engineering and Sciences, Michigan Technological University, Houghton, MI, 49931, USA
Jobin Thomas
You can also search for this author in PubMed Google Scholar
Contributions
GT: conceptualization, methodology, data curation, investigation, writing—original draft preparation; JT: data curation, writing—original draft preparation, reviewing and editing, visualization; RSD: data curation, writing—original draft preparation; AK: data curation, writing—original draft preparation; AVM: data curation, writing—original draft preparation; AJN: data curation, writing—original draft preparation.
Corresponding author
Correspondence to George Thomas .
Ethics declarations
Conflict of interest.
The authors declare that they have no known competing financial interests or personal relationships that could have appeared to influence the work reported in this paper.
Rights and permissions
Springer Nature or its licensor (e.g. a society or other partner) holds exclusive rights to this article under a publishing agreement with the author(s) or other rightsholder(s); author self-archiving of the accepted manuscript version of this article is solely governed by the terms of such publishing agreement and applicable law.
Reprints and permissions
About this article
Thomas, G., Thomas, J., Devika, R.S. et al. Impact of COVID-19 Lockdown on Ambient Air Quality in the Southwest Coastal Urban Regions of India. Aerosol Sci Eng 7 , 303–314 (2023). https://doi.org/10.1007/s41810-023-00180-x
Download citation
Received : 30 September 2022
Revised : 04 March 2023
Accepted : 22 March 2023
Published : 05 April 2023
Issue Date : September 2023
DOI : https://doi.org/10.1007/s41810-023-00180-x
Share this article
Anyone you share the following link with will be able to read this content:
Sorry, a shareable link is not currently available for this article.
Provided by the Springer Nature SharedIt content-sharing initiative
- Air quality
- Find a journal
- Publish with us
- Track your research

An official website of the United States government
The .gov means it’s official. Federal government websites often end in .gov or .mil. Before sharing sensitive information, make sure you’re on a federal government site.
The site is secure. The https:// ensures that you are connecting to the official website and that any information you provide is encrypted and transmitted securely.
- Publications
- Account settings
Preview improvements coming to the PMC website in October 2024. Learn More or Try it out now .
- Advanced Search
- Journal List
- Springer Nature - PMC COVID-19 Collection

Air pollution prediction with machine learning: a case study of Indian cities
1 Sikh National College, Qadian, Guru Nanak Dev University, Amritsar, Punjab India
B. P. Pande
2 Department of Computer Applications, LSM, Government PG College, Pithoragarh, Uttarakhand India
The survival of mankind cannot be imagined without air. Consistent developments in almost all realms of modern human society affected the health of the air adversely. Daily industrial, transport, and domestic activities are stirring hazardous pollutants in our environment. Monitoring and predicting air quality have become essentially important in this era, especially in developing countries like India. In contrast to the traditional methods, the prediction technologies based on machine learning techniques are proved to be the most efficient tools to study such modern hazards. The present work investigates six years of air pollution data from 23 Indian cities for air quality analysis and prediction. The dataset is well preprocessed and key features are selected through the correlation analysis. An exploratory data analysis is exercised to develop insights into various hidden patterns in the dataset and pollutants directly affecting the air quality index are identified. A significant fall in almost all pollutants is observed in the pandemic year, 2020. The data imbalance problem is solved with a resampling technique and five machine learning models are employed to predict air quality. The results of these models are compared with the standard metrics. The Gaussian Naive Bayes model achieves the highest accuracy while the Support Vector Machine model exhibits the lowest accuracy. The performances of these models are evaluated and compared through established performance parameters. The XGBoost model performed the best among the other models and gets the highest linearity between the predicted and actual data.
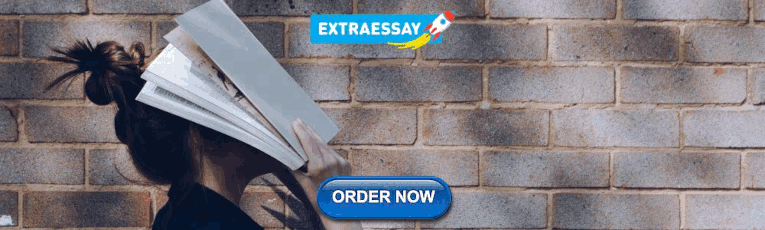
Introduction
Energy consumption and its consequences are inevitable in modern age human activities. The anthropogenic sources of air pollution include emissions from industrial plants; automobiles; planes; burning of straw, coal, and kerosene; aerosol cans, etc. Various dangerous pollutants like CO, CO 2 , Particulate Matter (PM), NO 2 , SO 2 , O 3 , NH 3 , Pb, etc. are being released into our environment every day. Chemicals and particles constituting air pollution affect the health of humans, animals, and even plants. Air pollution can cause a multitude of serious diseases in humans, from bronchitis to heart disease, from pneumonia to lung cancer, etc. Poor air conditions lead to other contemporary environmental issues like global warming, acid rain, reduced visibility, smog, aerosol formation, climate change, and premature deaths. Scientists have realized that air pollution bears the potential to affect historical monuments adversely (Rogers 2019 ). Vehicle emissions, atmospheric releases of power plants and factories, agriculture exhausts, etc. are responsible for increased greenhouse gases. The greenhouse gases adversely affect climate conditions and consequently, the growth of plants (Fahad et al. 2021a ). Emissions of inorganic carbons and greenhouse gases also affect plant-soil interactions (Fahad et al. 2021b ). Climatic fluctuations not only affect humans and animals but agricultural factors and productivity are also greatly influenced (Sönmez et al. 2021 ). Economic losses are the allied consequences too. The Air Quality Index (AQI) , an assessment parameter is related to public health directly. A higher level of AQI indicates more dangerous exposure for the human population. Therefore, the urge to predict the AQI in advance motivated the scientists to monitor and model air quality. Monitoring and predicting AQI, especially in urban areas has become a vital and challenging task with increasing motor and industrial developments. Mostly, the air quality-based studies and research works target the developing countries, although the concentration of the most deadly pollutant like PM 2.5 is found to be in multiple folds in developing countries (Rybarczyk and Zalakeviciute 2021 ). A few researchers endeavored to undertake the study of air quality prediction for Indian cities. After going through the available literature, a strong need had been felt to fill this gap by attempting analysis and prediction of AQI for India.
Various models have been exercised in the literature to predict AQI, like statistical, deterministic, physical, and Machine Learning (ML) models. The traditional techniques based on probability, and statistics are very complex and less efficient. The ML-based AQI prediction models have been proved to be more reliable and consistent. Advanced technologies and sensors made data collection easy and precise. The accurate and reliable predictions through such huge environmental data require rigorous analysis which only ML algorithms can deal with efficiently. Al-Jamimi et al. ( 2018 ) thoroughly discussed the importance of supervised ML algorithms for applied environment protection issues. The present work investigates six years of air pollution data of the Indian cities and analyzes twelve air pollutants and AQI. The dataset is preprocessed and cleaned first, then methods of data visualization are applied to develop better insights and to investigate hidden patterns and trends. This work exploits the essence of correlation coefficient with ML models which has been exercised by very few scholars in the literature (Alade et al. 2019a ). The data imbalance is identified and addressed with a resampling technique. Five popular ML models are exercised in context with this resampling technique. Their performances are then compared through standard metrics. These metrics are utilized by many scholars of the realm (see Table Table1) 1 ) and some other authors of ML applications like Ayturan et al. ( 2020 ), Alade et al. ( 2019b ), Al-Jamimi et al ( 2019 ), and Al-Jamimi and Saleh ( 2019 ), etc.
Research works on AQI prediction through ML technology
Section 2 presents the literature survey with a comparative analysis of the literary works in the realm of air quality prediction with ML. Section 3 describes the dataset being studied, preprocessing, and feature selection techniques applied. Section 4 deals with observing hidden patterns in the dataset through data visualisation. Section 5 is dedicated to the experimental design, analysis of seasonal trends, empirical results, and discussions. The final section concludes the present work.
Date: 17 February 2022.
Place: Qadian, Punjab and Pithoragarh, Uttarakhand, India.
A brief literature review
Gopalakrishnan ( 2021 ) combined Google’s Street view data and ML to predict air quality at different places in Oakland city, California. He targeted the places where the data were unavailable. The author developed a web application to predict air quality for any location in the city neighborhoods. Sanjeev ( 2021 ) studied a dataset that included the concentration of pollutants and meteorological factors. The author analyzed and predicted the air quality and claimed that the Random Forest (RF) classifier performed the best as it is less prone to over-fitting.
Castelli et al. ( 2020 ) endeavored to forecast air quality in California in terms of pollutants and particulate levels through the Support Vector Regression (SVR) ML algorithm. The authors claimed to develop a novel method to model hourly atmospheric pollution. Doreswamy et al. ( 2020 ) investigated ML predictive models for forecasting PM concentration in the air. The authors studied six years of air quality monitoring data in Taiwan and applied existing models. They claimed that predicted values and actual values were very close to each other. Liang et al. ( 2020 ) studied the performances of six ML classifiers to predict the AQI of Taiwan based on 11 years of data. The authors reported that Adaptive Boosting (AdaBoost) and Stacking Ensemble are most suitable for air quality prediction but the forecasting performance varies over different geographical regions. Madan et al. ( 2020 ) compared twenty different literary works over pollutants studied, ML algorithms applied, and their respective performances. The authors found that many works incorporated meteorological data such as humidity, wind speed, and temperature to predict pollution levels more accurately. They found that the Neural Network (NN) and boosting models outperformed the other eminent ML algorithms. Madhuri et al. ( 2020 ) mentioned that wind speed, wind direction, humidity, and temperature played a significant role in the concentration of air pollutants. The authors employed supervised ML techniques to predict the AQI and found that the RF algorithm exhibited the least classification errors. Monisri et al. ( 2020 ) collected air pollution data from various sources and endeavored to develop a mixed model for predicting air quality. The authors claimed that the proposed model aims to help people in small towns to analyze and predict air quality. Nahar et al. ( 2020 ) developed a model to predict AQI based on ML classifiers. Their authors studied the data collected over the tenure of 28 months by the ministry of environment, Jordan, and identified the concentrations of pollutants. Their proposed model detected the most contaminated areas with satisfying accuracy. Patil et al. ( 2020 ) presented some literary works on various ML techniques for AQI modeling and forecasting. The authors found that Artificial Neural Network (ANN) , Linear Regression (LR), and Logistic Regression (LogR) models were exploited by most of the scholars for AQI prediction.
Bhalgat et al. ( 2019 ) applied the ML technique to predict the concentration of SO 2 in the environment of Maharashtra, India. The authors concluded that being highly polluted, some cities of this Indian province require grave attention. The authors mentioned that their model was not capable of exhibiting expected outputs. Mahalingam et al. ( 2019 ) developed a model to predict the AQI of smart cities and tested it in Delhi, India. The authors reported that the medium Gaussian Support Vector Machine (SVM) exhibited maximum accuracy. The authors claim that their model can be used in other smart cities too. Soundari et al. ( 2019 ) developed a model based on NNs to predict the AQI of India. The authors claimed that their proposed model could predict the AQI of the whole county, of any province, or of any geographical region when the past data on concentration of pollutants were available.
Sweileh et al. ( 2018 ) came up with a very interesting study about the analysis of global peer-reviewed literature about air pollution and respiratory health. The authors extracted 3635 documents from the Scopus database published between 1990 and 2017. They observed that there was a substantial increase in publications from 2007 to 2017. The authors reported active countries, institutions, journals, authors, international collaborations in the realm and concluded that research works on air pollution and respiratory health had been receiving a lot of attention. They suggested securing public opinions about mitigation of outdoor air pollution and investment in green technologies. Zhu et al. ( 2018 ) refined the problem of AQI prediction as a multi-task learning problem. The authors utilized large-scale optimization techniques and endeavored to reduce the number of parameters. Based on their empirical results, they claimed that the proposed model exhibited better results than existing regression models.
Bellinger et al. ( 2017 ) carried out a detailed literature analysis on the application of ML and data mining methods toward air pollution epidemiology. The authors found that the researchers from Europe, China, and the USA were very active in this realm and the following classifiers had been widely applied: Decision Tree (DT) , SVMs , K-means clustering, and the APRIORI algorithm. Rybarczyk and Zalakeviciute ( 2017 ) endeavored to develop a model that correlated traffic density with air pollution. The author mentioned that such traffic data collection was economical, and integrating it with meteorological features boosted accuracy. The authors found that the hybrid model performed the best and accuracy based on morning time data was the highest.
Table Table1 1 shown below presents a concise and comparative analysis of the literary works in the realm of AQI prediction.
It has been observed that research works in air quality analysis and prediction for Indian cities acquired lesser attention from scholars. In spite of the fact that out of the ten most polluted cities in the world, nine cities are Indian (Deshpande 2021 ), very few researchers investigated AQI prediction from the Indian perspective. The present work endeavors to fill this gap by studying 5 years of substantial air pollution data from twenty-three Indian cities. The current study is an earnest attempt to contribute to the literature with novel ideas of data visualizations, exploiting correlation coefficient-based statistical outliers for analytics, and comparison of five key ML models over standard performance metrics.
Material and methods
Some Indian cities fall in the array of the most polluted cities in the world, and the threat of air pollution is being raised day by day. Poor air quality in India is now considered a significant health challenge and a major obstacle to economic growth. According to a new study released jointly by a UK-based non-profit management firm, Dalberg Advisors and Industrial Development Corporation , air pollution in India caused annual losses of up to Rs 7 lakh crore ($95 billion) (Dalberg 2019 ). The main pollutant emissions in India are due to the energy production industry, vehicle traffic on roads, soil and road dust, waste incineration, power plants, open waste burning, etc. The present research investigates air pollution data extracted from the Central Pollution Control Board (CPCB) , India. 1 This dataset possesses observations from January 2015 to July 2020 and it is comprised of 12 features with 29,531 instances from 23 different Indian cities. Table Table2 2 presented below provides brief descriptive statistics of the pollutants/particles and AQI from this dataset.
Statistics of various pollutants and AQI in the CPCB dataset
Analysis of some major air pollutants such as PM 2.5 , PM 10 , NO 2 , CO, SO 2 , O 3, etc. and prediction of AQI are the essence of the current work. The methodological steps of the adopted process are presented in the following figure (Fig. (Fig.1 1 ).

Flowchart of the proposed model
Data preprocessing
Quality of data is the first and most important prerequisite for effective visualization and creation of efficient ML models. The preprocessing steps help in reducing the noise present in the data which eventually increases the processing speed and generalization capability of ML algorithms. Outliers and missing data are the two most common errors in data extraction and monitoring applications. The data preprocessing step performs various operations on data such as filling out not-a-number (NAN) data, removing or changing outlier data, etc. Figure 2 shown below presents a view of the missing values in each feature of the dataset. Observe that among all other features, Xylene has the most missing values and CO has the least missing values. A large number of missing values may be existing due to a variety of factors, such as a station that can sense data but does not possess a device to record it.

Missing values of the features and their percentages
All the missing values are filled with the median values against each feature to solve the missing data problem. Next, a normalisation process has been applied to standardize the data, ensuring that the significance of variables is unaffected by their ranges or units. The data normalisation process helps to bring different data attributes into a similar scale of measurement. This process plays a vital role in the stable training of ML models and boosts performance. The datatypes of all the variables are also examined during normalisation. For example, the dataset is collected from different monitoring stations which deal with different representations of dates. Thus, the date ‘Monday, May 17, 2021’ may be represented as ‘17/5/2021’ or as ‘17–05-2021’ etc. Such date feature has been normalised through the datetime Python library.
Feature selection
The CPCB dataset under study involves a specific parameter viz, AQI and government agencies use this parameter to alert people about the quality of the air and also practice forecasting it. According to the National Ambient Air Quality Standards , there are six AQI categories: good (0–50), satisfactory (51–100), moderate (101–200), poor (201–300), very poor (301–400), and severe (401–500). Scholars in the realm suggest that reducing input variables lowers the computational cost of modeling and enhances prediction performance. A correlation-based feature selection method has been exploited in the present work to determine the optimal number of input variables (pollutants) when developing a predictive model. Statistical correlation-based feature selection algorithms compute correlations between every pair of the input variable and the target variable. The variables possessing the strongest correlation with the target variable are then filtered for further study. Since many ML algorithms are sensitive to outliers, any feature in the input dataset which does not follow the general trend of that data must be found. For the present dataset, a correlation-based statistical outliers detection method has been applied to identify the outliers. To select significant features, the correlation analysis of the AQI feature has been exercised with features of other pollutants. Figure 3 , shown below clearly reveals that pollutants PM 10 , PM 2.5 , CO, NO 2 , SO 2 , NO X, and NO are generally responsible for the AQI to attain higher values. These pollutants are correlated with AQI based on the correlation values above the threshold of 0.4.

Correlation heatmap of AQI with other pollutants (Threshold: 0.4)
Table Table3 3 given below shows the exact correlation values of each pollutant of the dataset with AQI.
Correlation between AQI and pollutants
Many ML models function better when data have a normal distribution and underperform when data have a skewed distribution. Therefore, it is necessary to identify the skewness being present in the features and to perform some transformations and mappings which convert the skewed distribution into a normal distribution. Figure 4 , given below shows that the features of Benzene , Toluene , CO, and Xylene are highly skewed. To make these skewed features more normal, the logarithmic transformations have been used to reduce the impact of outliers by normalising magnitude differences.

Skewness present in dataset features
Exploratory data analysis
This section of the present study deals with data exploration and analysis for finding various hidden patterns present in the dataset. Exploratory data analysis is the first step in data analytics which is performed before applying any ML model. Under this, the following important things are being analyzed: (a) exploring statuses and trends of air pollutants over the past six years i.e. from 2015 to 2020; (b) exploring the distribution of pollutants in the air along with top-six polluted cities with their average AQI values; and (c) estimating top four pollutants which are directly involved in increasing the AQI values.
Exploring the trends of air pollutants over the last six years
India has become one of the few countries having the most severe air pollution resulting from rapid industrialization and booming urbanization over the last several years. Air pollution is among grave public health and environmental issues, and the Health Effects Institute (HEI) ranks it among the top five global risk factors for mortality (IHME 2019 ). According to the HEI research, the emission of PM was the third leading cause of death in 2017, and this rate was highest in India. Based on the emissions of PM 2.5 and other pollutants, the World Health Organization (WHO) ranked India as the fifth most polluted country (Gurjar, 2021 ). The trends of various pollutants from 2015 to 2020 are observed and shown in the figure below (Fig. 5 ). Observe that except for O 3 and Benzene , all other pollutants exhibited a significant fall in 2020. The year 2020 witnessed the most strict lockdown in the history of mankind and ceased industrial, automobile, and aviation activities in India and the world served as some ambrosia for the ailing environment and air.

Intensities of various pollutants from 2015 to 2020
Figure 6 shown below depicts the average AQI values over the aforementioned tenure for the six most polluted cities in India.

The six most polluted Indian cities with their average AQI values from 2015 to 2020
Pollutants that are directly involved in increasing AQI values
The correlation values between different pollutants and AQI have been exercised and the pollutants for which this correlation value is greater than the threshold of 0.5, i.e. the correlation is strongly positive have been identified. Figure 7 shown below depicts the concentration of four such pollutants in various cities in India.

Pollutants governing AQI directly
Results and discussion
This section deals with the experimental design and empirical analysis for predicting AQI values through the pollutants present in the air. The air pollution dataset is split into training (75%) and testing (25%) subsets before evaluating ML models. The Google Colab Pro cloud platform with Intel(R) Xeon(R) CPU @ 2.30 GHz, Tesla P100-PCIE-16 GB, 12.8 GB RAM, and 180 GB of disc space has been utilized for executing Python scripts. The Python libraries like Scikit-learn , NumPy , Pandas , Seaborn , etc. are exploited for various data processing tasks. Next, the dataset is explored with the motive to find the overall value of the AQI with respect to those pollutants which have a significant role in raising the AQI value. In Fig. 8 shown below, a timeline graph of AQI is depicted over some particular pollutants which are directly responsible for higher values of AQI. From Fig. 8 , it is clear that each pollutant grows and drops year after year, and their values do not remain constant every year. PM 2.5 and PM 10 have seasonal effects, with higher pollution levels in the winter than in the summer. After 2018, the level of SO 2 began to rise, but the level of O 3 stayed unchanged from 2018 to 2020. The same trend can be seen in BTX 2 levels as well. Except for CO, practically every pollutant has exhibited seasonal variations.

Timeline graph of AQI with respect to specific pollutants
To examine the seasonality of the data thoroughly, Box plot visualizations are employed. Box plots categorise data into different periods by grouping the entire information in years and months. Figure Figure9 9 presents the Box plots of various pollutants over time, both annually and monthly. Notice that pollution levels in India decrease between June and August. It may be the consequence of the inception of the Monsoon in the Indian subcontinent during this tenure. BTX levels exhibit a significant drop between March and April, a modest rise from May to September, and a sharp surge from October to December. The median values for 2020 are lower than those for previous years, indicating that pollution may have decreased substantially in 2020. Strict lockdown ceased human and industrial activities in India during the COVID-19 pandemic are the obvious reasons for this observed phenomenon.

Variation analysis of pollutants through Box plots
Next, the detailed development of ML-based AQI prediction models is discussed. Finally, the performance of the AQI forecasting models is evaluated. The target attribute, AQI_Bucket has some missing values which result in the unequal splitting of the classes. Many ML models ignore this imbalanced datasets problem which may lead to poor classification and prediction performances. To overcome this data imbalance problem, the SMOTE (Synthetic Minority Oversampling Technique) has been applied. In this technique, the algorithm synthesizes new elements for minority classes rather than creating copies of already existing elements. It functions by randomly choosing a point from the minority class and computing the k-nearest neighbor distances for the selected point. The newly created synthetic points are added between the chosen point and its neighbors. To implement SMOTE for class imbalance, we have used an imbalanced-learn Python library in the SMOTE class. Now, five popular ML models, KNN , Gaussian Naive Bayes (GNB) , SVM , RF , and XGBoost have been employed to predict the AQI level with SMOTE and without SMOTE resampling technique. Table Table4 4 shown below presents the results of used ML models in terms of accuracy, precision, recall, and F1-score during the training phase. Precision tells the fraction of relevant instances present in the retrieved instances, while recall is the fraction of relevant instances that have been retrieved. Accuracy is the ratio of the correctly labeled attributes to the whole pool of variables. F1-score is a weighted average of precision and recall. Note that the XGBoost model achieved the highest accuracy, while the SVM model exhibited the lowest accuracy.
Comparison of model results in the training set
The performances of the ML models for the training set are evaluated against the standard performance parameters, viz MAE , RMSE , Root Mean Squared Logarithmic Error (RMSLE) , and coefficient of determination, i.e. R 2 (Table (Table5). 5 ). These performance measures have been exploited extensively in the literature. Table Table5 5 given below provides error statistics of the ML models applied with and without SMOTE resampling technique on the training set. The XGBoost model outperformed other models in terms of error statistics when exercised without the SMOTE technique. On the other hand, the RF model performed relatively good among others in terms of error statistics when exercised with the SMOTE technique. The XGBoost model performed equally good in this area in terms of MAE and RMSLE. These observations are marked bold in Table Table5 5 .
Results of ML algorithms for AQI Prediction with and without SMOTE (training set)
Table Table6 6 shown below presents the results of employed ML models obtained during the testing phase. It is evident from Table Table6 6 that the XGBoost model surpassed the other models again, whereas the SVM model attained the lowest accuracy in the testing phase too.
Comparison of model results in the testing set
The performances of the ML models for the testing set are evaluated against the standard performance parameters as above (Table (Table7 7 ).
Results of ML algorithms for AQI prediction with and without SMOTE (testing set)
The above table summarizes the performances of various ML models applied with and without SMOTE resampling technique on the testing set. It is observed that all ML models exhibited improvement in almost all assessment metrics when applied with SMOTE resampling technique. The GNB model attained the best values of R 2 in both cases. The XGBoost model performed the best in terms of error statistics and attained the most optimum values in both experimental genres. These observations are marked bold in Table Table7 7 .
Prediction of air quality is a challenging task because of the dynamic environment, unpredictability, and variability in space and time of pollutants. The grave consequences of air pollution on humans, animals, plants, monuments, climate, and environment call for consistent air quality monitoring and analysis, especially in developing countries. However, lesser attention for researchers has been observed for AQI prediction for India. In the present work, air pollution data of 23 Indian cities for a tenure of six years are investigated. The dataset is cleaned and preprocessed first by filling NAN values, addressing outliers, and normalising data values. Then correlation-based feature selection technique is exercised to filter AQI affecting pollutants for further study and logarithmic transformations are applied to the skewed features. The exploratory data analysis methods are exercised to find various hidden patterns present in the dataset. It was found that almost all pollutants exhibited a significant fall in 2020. The data imbalance problem is addressed by the SMOTE analysis. The dataset is split into train-test subsets by the ratio of 75–25% respectively. ML-based AQI prediction is carried out with and without SMOTE resampling technique and a comparative analysis is presented. The results of ML models for both the train-test subsets are presented in terms of standard metrics like accuracy, precision, recall, and F1-Score. For both the train-test sets, the XGBoost model attained the highest accuracy and the SVM model exhibited the lowest accuracy. The classical statistical error metrics, namely MAE, RMSE, RMSLE, and R 2 are then evaluated to assess and compare the performances of ML models. The XGBoost model comes out to be the overall best performer by attaining the optimum values in both training and testing phases. For the training phase, the RF model performed relatively good when exercised with SMOTE. On the other hand, almost all ML models exhibited improvements in the testing phase. In this phase, the GNB model attained the best results for R 2 in target predictions. The present research endeavors to contribute to the literature by addressing air quality analysis and prediction for India which might have not been properly studied. This work can be extended by employing deep learning techniques for AQI prediction.
Acknowledgements
No organization funded the present research.
Declarations
The authors declare that they have no conflict of interest.
1 The dataset can be downloaded from: https://app.cpcbccr.com/ccr/#/caaqm-dashboard-all/caaqm-landing/data .
2 BTX is the combined name given to Benzene , Toluene , and Xylene.
- Alade IO, Rahman MAA, Saleh TA. Predicting the specific heat capacity of alumina/ethylene glycol nanofluids using support vector regression model optimized with Bayesian algorithm. Sol Energy. 2019; 183 :74–82. doi: 10.1016/j.solener.2019.02.060. [ CrossRef ] [ Google Scholar ]
- Alade IO, Rahman MAA, Saleh TA. Modeling and prediction of the specific heat capacity of Al 2 O 3 /water nanofluids using hybrid genetic algorithm/support vector regression model. Nano-Struct Nano-Objects. 2019; 17 :103–111. doi: 10.1016/j.nanoso.2018.12.001. [ CrossRef ] [ Google Scholar ]
- Al-Jamimi HA, Saleh TA. Transparent predictive modelling of catalytic hydrodesulfurization using an interval type-2 fuzzy logic. J Clean Prod. 2019; 231 :1079–1088. doi: 10.1016/j.jclepro.2019.05.224. [ CrossRef ] [ Google Scholar ]
- Al-Jamimi HA, Al-Azani S, Saleh TA. Supervised machine learning techniques in the desulfurization of oil products for environmental protection: a review. Process Saf Environ Prot. 2018; 120 :57–71. doi: 10.1016/j.psep.2018.08.021. [ CrossRef ] [ Google Scholar ]
- Al-Jamimi HA, Bagudu A, Saleh TA. An intelligent approach for the modeling and experimental optimization of molecular hydrodesulfurization over AlMoCoBi catalyst. J Mol Liq. 2019; 278 :376–384. doi: 10.1016/j.molliq.2018.12.144. [ CrossRef ] [ Google Scholar ]
- Ayturan YA, Ayturan ZC, Altun HO, Kongoli C, Tuncez FD, Dursun S, Ozturk A. Short-term prediction of PM2.5 pollution with deep learning methods. Global NEST J. 2020; 22 (1):126–131. [ Google Scholar ]
- Bellinger C, Jabbar MSM, Zaïane O, Osornio-Vargas A. A systematic review of data mining and machine learning for air pollution epidemiology. BMC Public Health. 2017 doi: 10.1186/s12889-017-4914-3. [ PMC free article ] [ PubMed ] [ CrossRef ] [ Google Scholar ]
- Bhalgat P, Bhoite S, Pitare S. Air Quality Prediction using Machine Learning Algorithms. Int J Comput Appl Technol Res. 2019; 8 (9):367–370. doi: 10.7753/IJCATR0809.1006. [ CrossRef ] [ Google Scholar ]
- Castelli M, Clemente FM, Popoviˇc A, Silva S, Vanneschi L. A machine learning approach to predict air quality in California. Complexity. 2020; 2020 (8049504):1–23. doi: 10.1155/2020/8049504. [ CrossRef ] [ Google Scholar ]
- Dalberg (2019) Air pollution and its impact on business: the silent pandemic. https://www.cleanairfund.org/wp-content/uploads/2021/04/01042021_Business-Cost-of-Air-Pollution_Long-Form-Report.pdf
- Deshpande T (2021) India Has 9 Of World's 10 most-polluted cities, but few air quality monitors. indiaspend. https://www.indiaspend.com/pollution/india-has-9-of-worlds-10-most-polluted-cities-but-few-air-quality-monitors-792521
- Doreswamy HKS, Yogesh KM, Gad I. Forecasting Air pollution particulate matter (PM2.5) using machine learning regression models. Procedia Comput Sci. 2020; 171 :2057–2066. doi: 10.1016/j.procs.2020.04.221. [ CrossRef ] [ Google Scholar ]
- Fahad S, Sönmez O, Saud S, Wang D, Wu C, Adnan M, Turan, V (2021a) Plant growth regulators for climate-smart agriculture (1st ed.). CRC Press. 10.1201/9781003109013
- Fahad, S, Sönmez O, Saud S, Wang D, Wu C, Adnan M, Turan V (2021b) Sustainable soil and land management and climate change (1st ed.). CRC Press. 10.1201/9781003108894
- Gopalakrishnan V (2021) Hyperlocal air quality prediction using machine learning. Towards data science. https://towardsdatascience.com/hyperlocal-air-quality-prediction-using-machine-learning-ed3a661b9a71
- Gurjar BR (2021) Air pollution in india: major issues and challenges. energy future 9(2):12–27. https://www.magzter.com/stories/Education/Energy-Future/AIR-POLLUTION-IN-INDIA-MAJOR-ISSUES-AND-CHALLENGES
- IHME (2019) State of global air 2019 report. http://www.healthdata.org/news-release/state-global-air-2019-report
- Liang Y, Maimury Y, Chen AH, Josue RCJ. Machine learning-based prediction of air quality. Appl Sci. 2020; 10 (9151):1–17. doi: 10.3390/app10249151. [ CrossRef ] [ Google Scholar ]
- Madan T, Sagar S, Virmani D (2020) Air quality prediction using machine learning algorithms–a review. In: 2nd international conference on advances in computing, communication control and networking (ICACCCN) pp 140–145. 10.1109/ICACCCN51052.2020.9362912
- Madhuri VM, Samyama GGH, Kamalapurkar S. Air pollution prediction using machine learning supervised learning approach. Int J Sci Technol Res. 2020; 9 (4):118–123. [ Google Scholar ]
- Mahalingam U, Elangovan K, Dobhal H, Valliappa C, Shrestha S, Kedam G (2019) A machine learning model for air quality prediction for smart cities. In: 2019 international conference on wireless communications signal processing and networking (WiSPNET). IEEE 452–457. 10.1109/WiSPNET45539.2019.9032734
- Monisri PR, Vikas RK, Rohit NK, Varma MC, Chaithanya BN. Prediction and analysis of air quality using machine learning. Int J Adv Sci Technol. 2020; 29 (5):6934–6943. [ Google Scholar ]
- Nahar K, Ottom MA, Alshibli F, Shquier MA. Air quality index using machine learning—a jordan case study. COMPUSOFT, Int J Adv Comput Technol. 2020; 9 (9):3831–3840. [ Google Scholar ]
- Patil RM, Dinde HT, Powar SK (2020) A literature review on prediction of air quality index and forecasting ambient air pollutants using machine learning algorithms 5(8):1148–1152
- Rogers CD (2019) Pollution's impact on historical monuments pollution's impact on historical monuments. SCIENCING. https://sciencing.com/about-6372037-pollution-s-impact-historical-monuments.html
- Rybarczyk Y, Zalakeviciute R (2017) Regression models to predict air pollution from affordable data collections. In: H. Farhadi (Ed.), Machine learning advanced techniques and emerging applications pp 15–48. IntechOpen. 10.5772/intechopen.71848
- Rybarczyk Y, Zalakeviciute R. Assessing the COVID-19 impact on air quality: a machine learning approach. Geophys Res Lett. 2021 doi: 10.1029/2020GL091202. [ PMC free article ] [ PubMed ] [ CrossRef ] [ Google Scholar ]
- Sanjeev D. Implementation of machine learning algorithms for analysis and prediction of air quality. Int. J. Eng. Res. Technol. 2021; 10 (3):533–538. [ Google Scholar ]
- Sönmez O, Saud S, Wang D, Wu C, Adnan M, Turan V (2021) Climate change and plants: biodiversity, growth and interactions (S. Fahad, Ed.) (1st ed.). CRC Press. 10.1201/9781003108931
- Soundari AG, Jeslin JG, Akshaya AC. Indian air quality prediction and analysis using machine learning. Int J Appl Eng Res. 2019; 14 (11):181–186. [ Google Scholar ]
- Sweileh WM, Al-Jabi SW, Zyoud SH, Sawalha AF. Outdoor air pollution and respiratory health: a bibliometric analysis of publications in peer-reviewed journals (1900–2017) Multidiscip Respiratory Med. 2018 doi: 10.1186/s40248-018-0128-5. [ PMC free article ] [ PubMed ] [ CrossRef ] [ Google Scholar ]
- Zhu D, Cai C, Yang T, Zhou X. A machine learning approach for air quality prediction: model regularization and optimization. Big Data and Cognitive Comput. 2018 doi: 10.3390/bdcc2010005. [ CrossRef ] [ Google Scholar ]
Air pollution on the rise in Kerala: Report
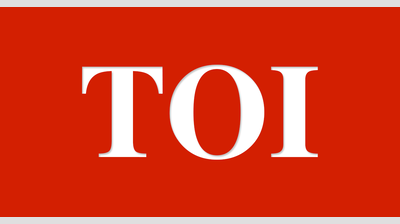
About the Author
Sudha Nambudiri reports from the southern state of Kerala. She writes on climate change, science and technology, social issues, and culture.
Visual Stories
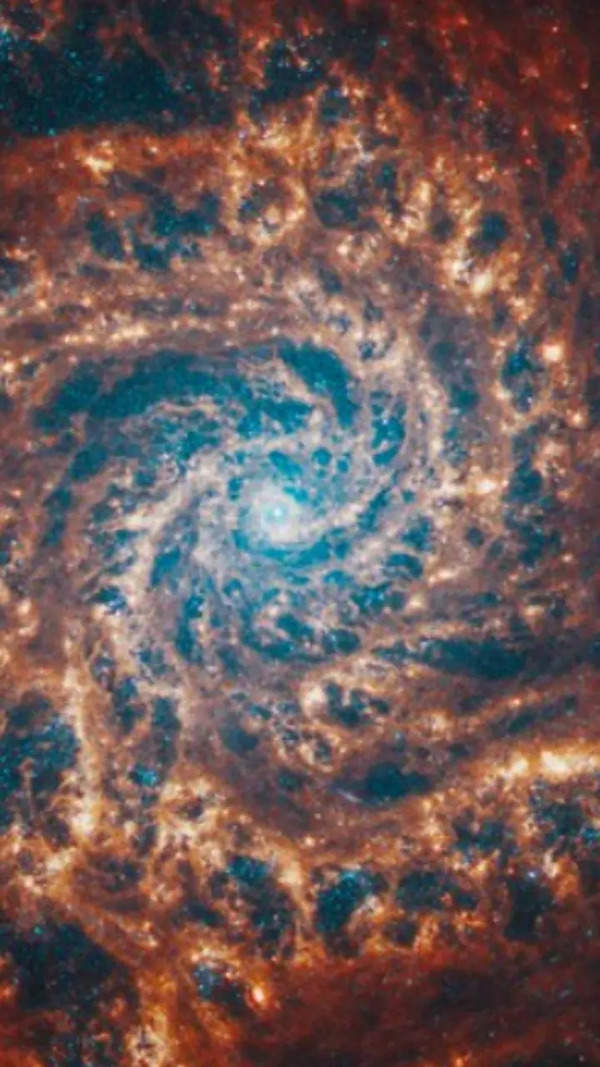
- You Are At:
Air Pollution exacerbates Sleep Apnea risk and severity, finds new study
For people living in areas with high pollution levels, the study suggests prioritising strategies to reduce their exposure indoors, such as using air purifiers. If you suspect you might have sleep apnea, regardless of where you live, talking to your doctor is crucial for diagnosis and treatment.

The research, published in the International Neurotoxicology Association journal, looked at data from twelve different studies to understand the link. While the evidence isn't conclusive yet, the researchers found a reason to believe that air pollutants, particularly nitrogen dioxide (NO2), might be contributing to a higher risk and severity of OSA.
NO2 is a common gas pollutant produced by traffic fumes and the burning of fossil fuels. The study suggests that exposure to NO2 could worsen existing sleep apnea or even increase the chances of developing it altogether.
The researchers haven't pinpointed the exact mechanism behind this link, but they propose a few possibilities. Air pollution can irritate and inflame the upper airway, making it more likely to collapse during sleep, a hallmark of OSA. Additionally, pollutants might disrupt the nervous system's control of breathing.
"These pathophysiological changes are known to contribute to the development and exacerbation of respiratory disorders. In the context of OSA, air pollution may aggravate upper airway inflammation and dysfunction, thereby increasing the propensity for airway collapse during sleep," the study reads. "It is important to note that effects of air pollutants may vary based on the type of pollutant, duration of exposure, and individual susceptibility factors, including age, gender, and underlying health conditions," it adds.
This study adds to a growing body of research suggesting that air pollution can have a wider impact on our health than previously thought. More work is needed to confirm the link between pollution and OSA, but these findings highlight the potential dangers of breathing dirty air.
ALSO READ: Anti-Pollution Diet: 11 top foods to cleanse your system
Read all the Breaking News Live on indiatvnews.com and Get Latest English News & Updates from Health
- Air pollution
- sleep apnea
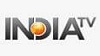
Balasore Lok Sabha Election 2024: Sitting BJP MP Pratap Sarangi to challenge BJD's L Samantsinghar
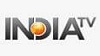
Mohanlal invites Shah Rukh Khan for a 'Zinda Banda' session, 'Jawan' star asks 'Your place or mine?'
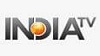
IPL Rising Star: Yash Thakur, from Vidarbha to Lucknow Super Giants
Related Health News
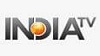
Who can take Insulin? How to store, when to take it, precautions, side effects and more
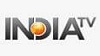
Ghazipur Landfill Fire: Know how to protect yourself from respiratory issues due to poisonous gas
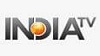
What are symptoms of heat stroke during peak summer season? Know preventive measures
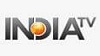
Balanced Diet to Adequate Sleep: 5 simple tips to manage pre-diabetic conditions
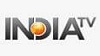
Superfood Ginseng: Know THESE 5 benefits of this Herbal Medicine
Latest News
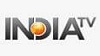
Sensex rises over 200 points, Nifty tops 22,400
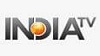
What is Nomophobia? Know its causes, symptoms, treatment and prevention
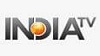
Major blow to TikTok: US Senate passes bill forcing parent company ByteDance to sell or face ban
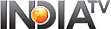
- Aap Ki Adalat
- Aaj Ki Baat
- Kurukshetra
- Haqiqat Kya Hai
- Entertainment
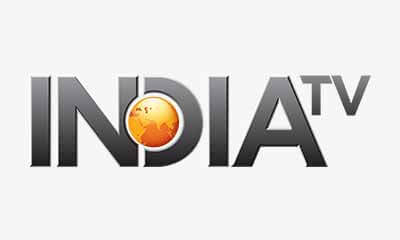
Modi Aur Musalman: Will 70% Muslim votes be divided or will it go directly to Mamata?
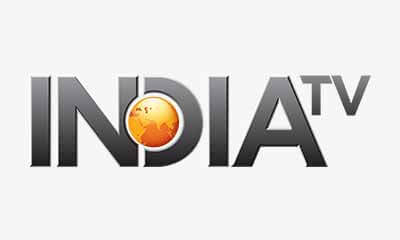
Aaj Ki Baat: Congress's 'Pilot Project'...what is the fact?
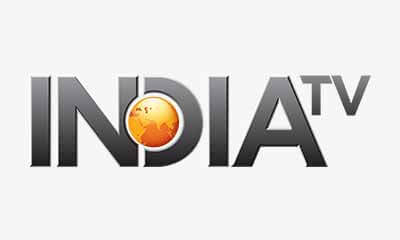
Haqiqat Kya Hai: If Modi does not become the Prime Minister, will there be reservation for Muslims?
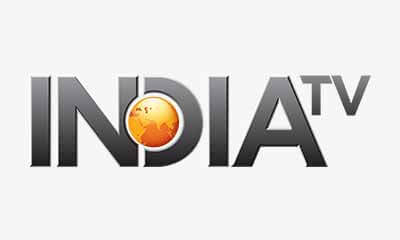
Bike Reporter: The royal family of Rajgarh...roar in the election field
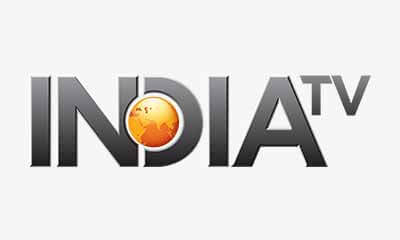
Hema Malini Interview: Election EXCLUSIVE with Hema Malini
- Maharashtra
- Uttar Pradesh
- Madhya Pradesh
- West Bengal
- Jammu & Kashmir
- Chhattisgarh
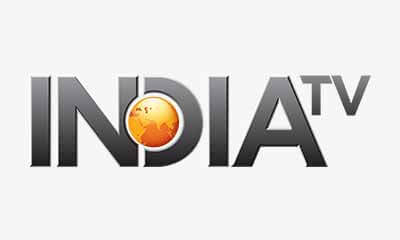
DRDO develops lightest bulletproof jacket for protection against highest threat level
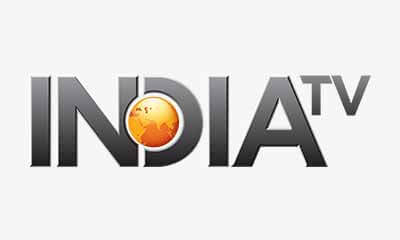
Congress candidate says ‘Constitution forced on Goa’, PM Modi calls it ‘ploy to break country’
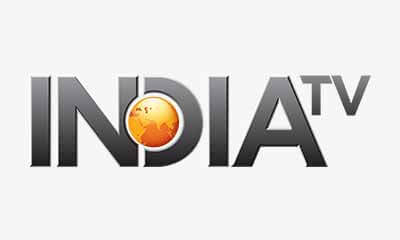
India successfully tests new version of air-launched medium-range ballistic missile
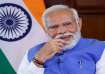
PM Modi’s crackdown on VIP treatment: Stories that show rise of 'every person is important' culture
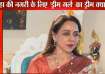
'Modi, Amit Shah are Jai-Veeru of Indian politics, entire opposition is Gabbar': Hema Malini | VIDEO
- Constituencies
- Key Candidates
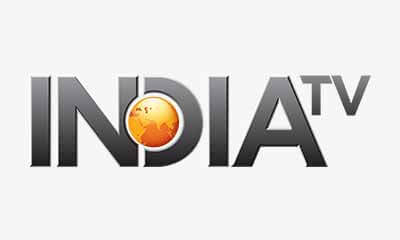
Lok Sabha Elections 2024: Amit Shah holds massive roadshow in Bengaluru | WATCH
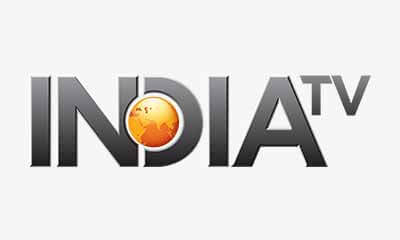
Congress fields Meira Kumar's son Anshul Avijit from Patna Sahib seat against Ravi Shankar Prasad
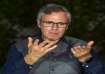
Omar Abdullah dubs Mehbooba's PDP as BJP’s ‘C-team’, urges people to ‘only look towards’ INDIA bloc
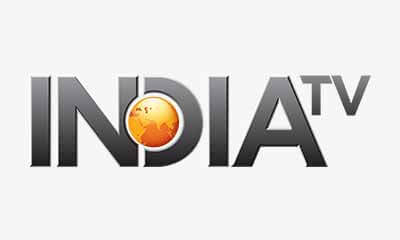
Marcus Stoinis shatters all-time record in IPL as LSG become first team to beat CSK at home in 2024
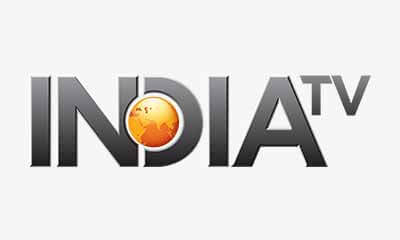
CSK vs LSG IPL 2024 Highlights: Marcus Stoinis' ton powers Lucknow Super Giants to six-wicket win
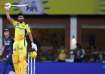
Ruturaj Gaikwad becomes first Chennai Super Kings skipper to hit IPL century, breaks Dhoni's record
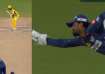
Flying KL Rahul takes a one-handed stunner to remove Ajinkya Rahane in CSK vs LSG clash | WATCH
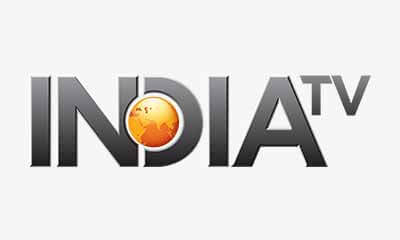
BIG relief to Ukraine, Israel and Taiwan as US Senate overwhelmingly passes USD 95 billion aid
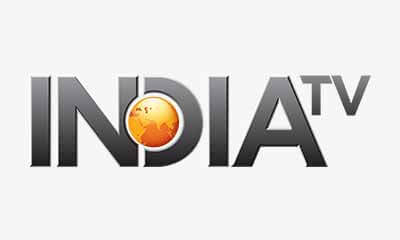
US's stern warning to Pakistan: 'Anyone considering business deal with Iran, will face sanctions'
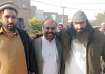
Syed Salahuddin, chief of Hizbul Mujahideen, makes rare appearance in PoK's Muzaffarabad
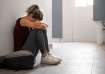
China: 18-year-old girl diagnosed with 'love brain' after calling boyfriend over 100 times daily
- Celebrities
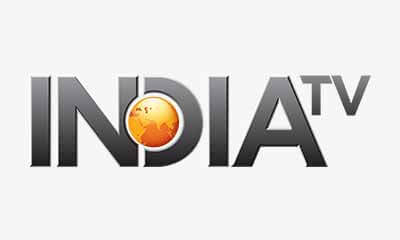
Preity Zinta joins Sunny Deol in Rajkumar Santoshi's Lahore 1947, drops BTS pics
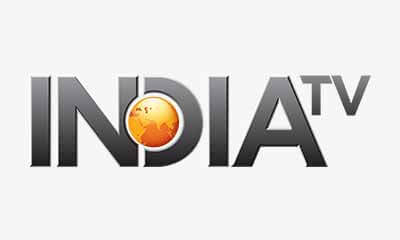
Ranveer Singh Deepfake Video: Mumbai's cyber police registers FIR against X user
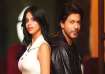
Shah Rukh Khan to again play 'Don' in Suhana Khan starrer 'King' | Deets Inside
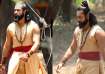
Vicky Kaushal's look from Chhaava leaked! actor spotted in avatar of Chhatrapati Sambhaji Maharaj
- Live Scores
- Other Sports
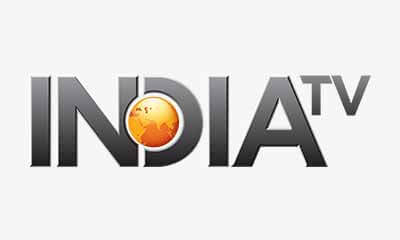
Apple to host 'Let Loose' event on May 7: Here's what to expect
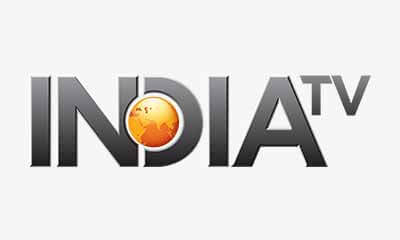
OnePlus Nord CE 3 gets price cut in India: Check out new price here
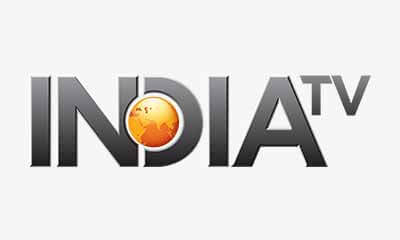
Tinder rollouts 'Share My Date' feature: Here's what's it, how it works
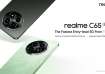
Realme C65 5G to launch in India later this week: Here's what to expect
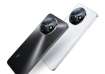
Itel S24 launched in India: Check price, specifications, availability
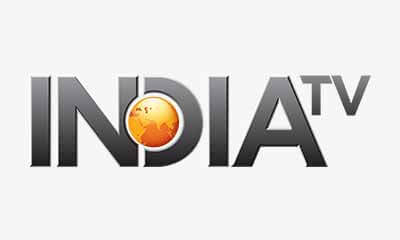
What is UK's Rwanda deportation bill and why is it such a big issue for Rishi Sunak? Explained
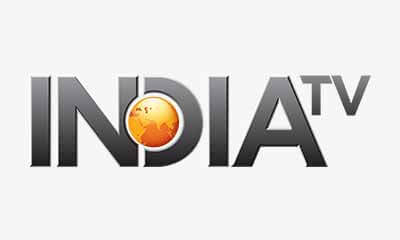
Muizzu's party wins Maldives polls despite geopolitical turbulence: What does this mean for India?
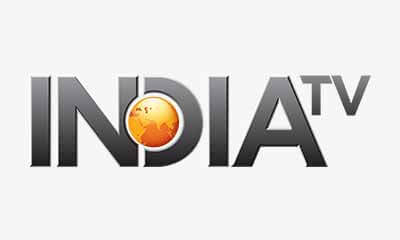
Imbalance between bat and ball? 3 reasons why impact player rule has overstayed its welcome
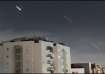
Iran and Israel: How the two countries went from allies to arch-enemies? History EXPLAINED
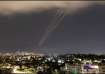
What are Israeli PM Netanyahu's options after Iran's unprecedented direct attack? Explained
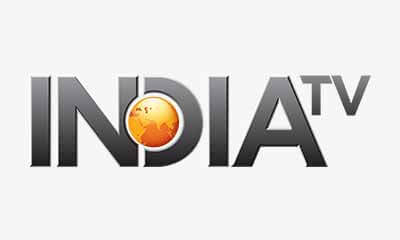
Horoscope Today, April 24: Day of enthusiasm for Pisces; know about other zodiac signs
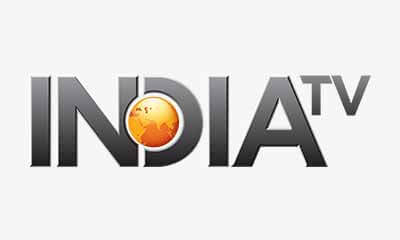
Horoscope Today, April 23: Capricorns to avoid unnecessary thinking; know about other zodiac signs
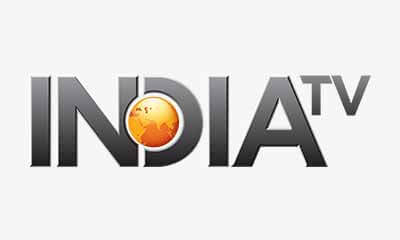
Horoscope Today, April 22: Luck will favour Pisces; know about other zodiac signs
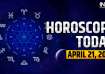
Horoscope Today, April 21: Stars of fortune high for Taurus; know about other zodiac signs
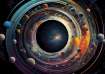
Weekly Horoscope (April 22-April 28): Aquarius needs to prioritise health; know about your sign
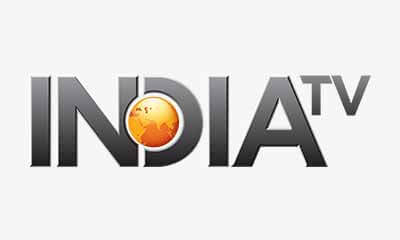
Superfood Wild Rice: Know THESE 5 benefits of this Water Oats
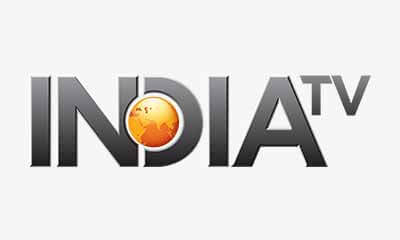
- Relationships
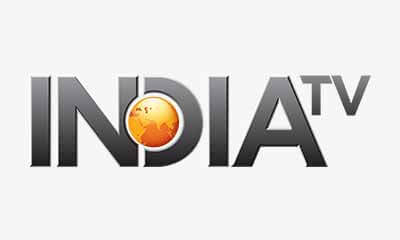
World Immunisation Week 2024: Date, theme, history, significance and all you need to know
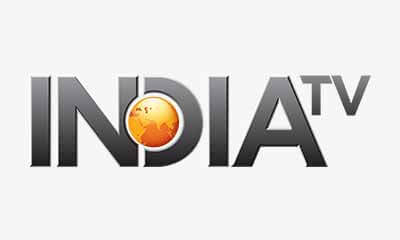
Indian passport holders can get multi-year Schengen visa: How to apply for it?
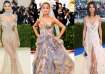
Met Gala 2024: Date, theme, ticket price, co-chairs, guest list and more
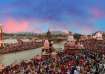
Spike in spiritual tourism, Ayodhya and Ujjain top Indian travelling destinations
Customer Reviews
is a “rare breed” among custom essay writing services today
All the papers delivers are completely original as we check every single work for plagiarism via advanced plagiarism detection software. As a double check of the paper originality, you are free to order a full plagiarism PDF report while placing the order or afterwards by contacting our Customer Support Team.
Being tempted by low prices and promises of quick paper delivery, you may choose another paper writing service. The truth is that more often than not their words are hollow. While the main purpose of such doubtful companies is to cash in on credulity of their clients, the prime objective of is clients’ satisfaction. We do fulfill our guarantees, and if a customer believes that initial requirements were not met or there is plagiarism found and proved in paper, they can request revision or refund. However, a refund request is acceptable only within 14 days of the initial deadline.
Our paper writing service is the best choice for those who cannot handle writing assignments themselves for some reason. At , you can order custom written essays, book reviews, film reports, research papers, term papers, business plans, PHD dissertations and so forth. No matter what academic level or timeframe requested is – we will produce an excellent work for you!
Customers usually want to be informed about how the writer is progressing with their paper and we fully understand that – he who pays the piper calls the tune. Therefore, with you have a possibility to get in touch with your writer any time you have some concerns or want to give additional instructions. Our customer support staff is there for you 24/7 to answer all your questions and deal with any problems if necessary.
Of course, the best proof of the premium quality of our services is clients’ testimonials. Just take a few minutes to look through the customer feedback and you will see that what we offer is not taking a gamble.
is a company you can trust. Share the burden of academic writing with us. Your future will be in safe hands! Buy essays, buy term papers or buy research papers and economize your time, your energy and, of course, your money!
Customer Reviews
Specifically, buying papers from us you can get 5%, 10%, or 15% discount.
There are questions about essay writing services that students ask about pretty often. So we’ve decided to answer them in the form of an F.A.Q.
Is essay writing legitimate?
As writing is a legit service as long as you stick to a reliable company. For example, is a great example of a reliable essay company. Choose us if you’re looking for competent helpers who, at the same time, don’t charge an arm and a leg. Also, our essays are original, which helps avoid copyright-related troubles.
Are your essay writers real people?
Yes, all our writers of essays and other college and university research papers are real human writers. Everyone holds at least a Bachelor’s degree across a requested subject and boats proven essay writing experience. To prove that our writers are real, feel free to contact a writer we’ll assign to work on your order from your Customer area.
Is there any cheap essay help?
You can have a cheap essay writing service by either of the two methods. First, claim your first-order discount – 15%. And second, order more essays to become a part of the Loyalty Discount Club and save 5% off each order to spend the bonus funds on each next essay bought from us.
Can I reach out to my essay helper?
Contact your currently assigned essay writer from your Customer area. If you already have a favorite writer, request their ID on the order page, and we’ll assign the expert to work on your order in case they are available at the moment. Requesting a favorite writer is a free service.
Meeting Deadlines

Finished Papers
We suggest our customers use the original top-level work we provide as a study aid and not as final papers to be submitted in class. Order your custom work and get straight A's.
260 King Street, San Francisco
Updated Courtyard facing Unit at the Beacon! This newly remodeled…
Customer Reviews
What if I can’t write my essay?
Essay Service Features That Matter

- Individual approach
- Fraud protection
Our team of paper writers consists only of native speakers coming from countries such as the US or Canada. But being proficient in English isn't the only requirement we have for an essay writer. All professionals working for us have a higher degree from a top institution or are current university professors. They go through a challenging hiring process which includes a diploma check, a successful mock-task completion, and two interviews. Once the writer passes all of the above, they begin their training, and only after its successful completion do they begin taking "write an essay for me" orders.
Finished Papers
Finished Papers
What is the best custom essay writing service?
In the modern world, there is no problem finding a person who will write an essay for a student tired of studying. But you must understand that individuals do not guarantee you the quality of work and good writing. They can steal your money at any time and disappear from sight.
The best service of professional essay writing companies is that the staff give you guarantees that you will receive the text at the specified time at a reasonable cost. You have the right to make the necessary adjustments and monitor the progress of the task at all levels.
Clients are not forced to pay for work immediately; money is transferred to a bank card only after receiving a document.
The services guarantee the uniqueness of scientific work, because the employees have special education and are well versed in the topics of work. They do not need to turn to third-party sites for help. All files are checked for plagiarism so that your professors cannot make claims. Nobody divulges personal information and cooperation between the customer and the contractor remains secret.
The various domains to be covered for my essay writing.
If you are looking for reliable and dedicated writing service professionals to write for you, who will increase the value of the entire draft, then you are at the right place. The writers of PenMyPaper have got a vast knowledge about various academic domains along with years of work experience in the field of academic writing. Thus, be it any kind of write-up, with multiple requirements to write with, the essay writer for me is sure to go beyond your expectations. Some most explored domains by them are:
- Project management
- Password reminder
- Registration
- Paraphrasing
- Research Paper
- Research Proposal
- Scholarship Essay
- Speech Presentation
- Statistics Project
- Thesis Proposal
Parents Are Welcome
No one cares about your academic progress more than your parents. That is exactly why thousands of them come to our essay writers service for an additional study aid for their children. By working with our essay writers, you can get a high-quality essay sample and use it as a template to help them succeed. Help your kids succeed and order a paper now!
What We Guarantee
- No Plagiarism
- On Time Delevery
- Privacy Policy
- Complaint Resolution
Johan Wideroos

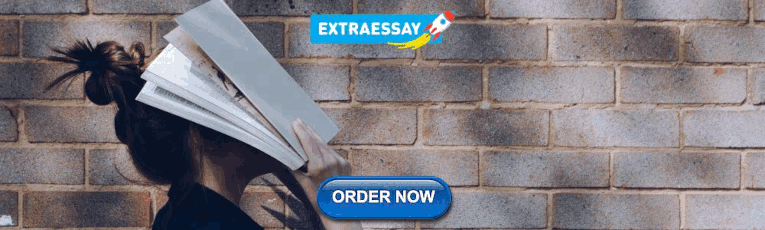
IMAGES
COMMENTS
Air pollutant concentration of Trivandrum, the capital of Kerala, exceeded the limits of National Ambient Air Quality (NAAQ) standards, according to a study conducted in 2015 by NATPAC. These polluted corridors harbour vegetation on roadsides and traffic islands, planted solely for aesthetic appeal. Analysis of air pollution tolerance levels of existing plants can act as a scientific basis for ...
This study quantifies air pollution using a parameter - Air Quality Index and compare the pollution of air in six major sites across Kerala, India (South over bridge and Eloor in Ernakulam ...
Fig 1: Air pollutants in Kerala during 2008-2016. The values of SPM were available only for three years during 2008-10. We can see that SPM values were highest during the year 2008 with a value of 79.21 μg/m 3 followed by 2009 (76.17 μg/m 3 ) and least in the year 2010 with a value of 64.03 μg/m 3. There is a gradual decrease in SPM in the ...
The cardiovascular burden would increase to 53,930 ( CI: 28,405-69,951) YLLs. 3.2. Discussion. The aim of the study was to test the feasibility of the environmental burden of disease approach at state level in Kerala, India, and to quantify a first set of disease burden estimates due to ambient air pollution by PM 2.5.
Air pollutant concentration of Trivandrum, the capital of Kerala, exceeded the limits of National Ambient Air Quality (NAAQ) standards, according to a study conducted in 2015 by NATPAC. ... Phytoremediation for urban landscaping and air pollution control-a case study in Trivandrum city, Kerala, India Environ Sci Pollut Res Int. 2021 Feb;28(8 ...
Phytoremediation for urban landscaping and air pollution control—a case study in Trivandrum city, Kerala, India Ancy S Watson1 & Sudha Bai R1 Received: 18 April 2020 /Accepted: 4 October 2020 ... from Kerala State Pollution Control Board (KSPCB) (NATPAC 2016)(Fig.1). Table 1 Corridors based on vehicular emission (NATPAC 2016) Rank Corridor
Air pollutant concentration of Trivandrum, the capital of Kerala, exceeded the limits of National Ambient Air Quality (NAAQ) standards, according to a study conducted in 2015 by NATPAC.
The study area of Kerala. ... Thomas J.J. Kerala's industrial backwardness: A case of path dependence in industrialization? World Dev. 2005; 33 (5) ... Tobollik M., Razum O., Wintermeyer D., Plass D. Burden of outdoor air pollution in Kerala, India—a first health risk assessment at state level. Int. J. Environ. Res. Public Health.
An expert body like NEERI was entrusted with the work of study of the air pollution. The Government of Kerala had already notified under Sec.19(1) of the air (Prevention and Control of Pollution) Act, 1981 dated 31-7-1984 declaring the area coming under the Corporation of Cochin as an Air Pollution Control Area KEA No.1.
The results of this study indicate a reduction in the different air pollutants and an overall improvement in the ambient air quality in the coastal urban regions of Kerala in the LD period of 2021. Air pollution in Kerala is primarily contributed by vehicular traffic, industries, and construction works (Jyothi et al. 2019). Since the lockdown ...
According to a new study released jointly by a UK-based non-profit management firm, Dalberg Advisors and Industrial Development Corporation, air pollution in India caused annual losses of up to Rs 7 lakh crore ($95 billion) (Dalberg 2019). The main pollutant emissions in India are due to the energy production industry, vehicle traffic on roads ...
The State Pollution Control Board (PCB) has proposed a year-long study to identify the sources and extent of air pollution in Kochi and Thiruvananthapuram. The recommendation was placed before the ...
The PCB report says that the annual average values of RSPM was found to be more than the permissible limit at seven stations in 2012. Irumpanam, a suburb in Kochi, recorded 664 ug/m3 in May 2012 ...
Mounting evidence have linked ambient air pollution and temperature with childhood pneumonia, but it is unclear whether there is an interaction between air pollution and temperature on childhood pneumonia. We aim to assess the combined effect of ambient air pollution and temperature exposure during preconception and pregnancy on pneumonia by a case-control study of 1510 children aged 0-14 ...
For people living in areas with high pollution levels, the study suggests prioritising strategies to reduce their exposure indoors, such as using air purifiers. If you suspect you might have sleep ...
Air Pollution: A Real Case Scenario in Patna Key t Air pollution is a significant environmental issue faced by the city of Patna, located in To address this issue, the government of Bihar has implemented various measures to control air ... The study will focus on various aspects of air pollution control, including the installation of air quality
This study assesses Delhi's air pollution scenario in the winter of 2021 and the actions to tackle it. Winter 2021 was unlike previous winters as the control measures mandated by the Commission of Air Quality Management (CAQM) in Delhi National Capital Region and adjoining areas were rolled out. These measures included the Graded Response ...
The strongest association with LD frequency in the ecological model was found for large-scale factors such as weather and air pollution. The case-crossover study confirmed the strong association of elevated daily mean temperature (OR 2.83; CI: 1.70, 4.70) and mean daily vapour pressure (OR: 1.52, CI: 1.15, 2.01) 6-14 days before LD occurrence.
The figure shows that during the year 2008 API value was highest ie.,70.89 followed by the year 2009 with a value of 68.18 and least in the year 2010 with a value of 59.34. The values of API fall within the range of 50-75 during three years. Hence we can say that in Kerala as a whole, moderate air pollution existed during the years 2008-10.
Quality is the most important aspect in our work! 96% Return clients; 4,8 out of 5 average quality score; strong quality assurance - double order checking and plagiarism checking. Hire a Writer. Case Study Of Air Pollution In Kerala, Kate Garraway Wedding Speech, What Traits Make A Good Leader Essay, Custom Scholarship Essay Writer Websites For ...
Case Study Of Air Pollution In Kerala. A standard essay helper is an expert we assign at no extra cost when your order is placed. Within minutes, after payment has been made, this type of writer takes on the job. A standard writer is the best option when you're on a budget but the deadline isn't burning. Within a couple of days, a new ...
Contact your currently assigned essay writer from your Customer area. If you already have a favorite writer, request their ID on the order page, and we'll assign the expert to work on your order in case they are available at the moment. Requesting a favorite writer is a free service. 1 (888)814-4206 1 (888)499-5521.
We hire only professional academic writers and editors with Ph.D. degrees. Sophia Melo Gomes. #24 in Global Rating. Case Study Of Air Pollution In Kerala. Choose a writer for your task among hundreds of professionals. Urgency. 741Orders prepared. 4240Orders prepared. 4.8/5.
Case Study Of Air Pollution In Kerala - Lydia A. BA/MA/MBA/PhD writers. A writer who is an expert in the respective field of study will be assigned. 1(888)814-4206 1(888)499-5521. Informative Category. Place an order. 4.9/5.
Case Study Of Air Pollution In Kerala - 4.7/5 - Agnes Malkovych, Canada. 100% Success rate 695 . Finished Papers. Only a Ph.D. professional can handle such a comprehensive project as a dissertation. The best experts are ready to do your dissertation from scratch and guarantee the best result. Thoroughly researched, expertly written, and styled ...
Case Study Of Air Pollution In Kerala. The narration in my narrative work needs to be smooth and appealing to the readers while writing my essay. Our writers enhance the elements in the writing as per the demand of such a narrative piece that interests the readers and urges them to read along with the entire writing.