Social Work Research Methods That Drive the Practice
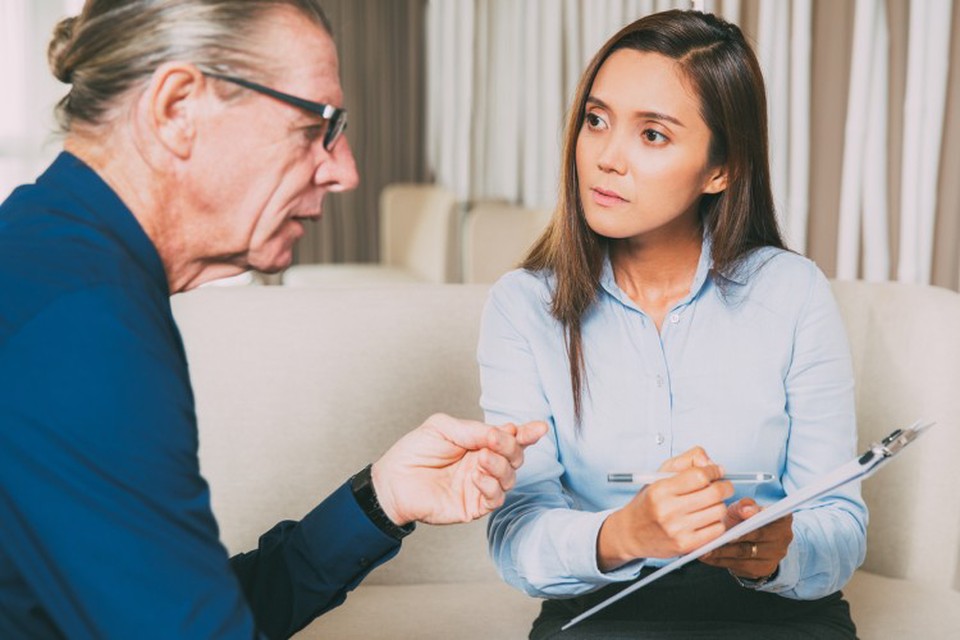
Social workers advocate for the well-being of individuals, families and communities. But how do social workers know what interventions are needed to help an individual? How do they assess whether a treatment plan is working? What do social workers use to write evidence-based policy?
Social work involves research-informed practice and practice-informed research. At every level, social workers need to know objective facts about the populations they serve, the efficacy of their interventions and the likelihood that their policies will improve lives. A variety of social work research methods make that possible.
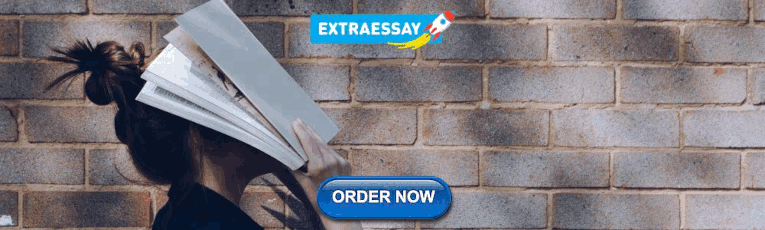
Data-Driven Work
Data is a collection of facts used for reference and analysis. In a field as broad as social work, data comes in many forms.
Quantitative vs. Qualitative
As with any research, social work research involves both quantitative and qualitative studies.
Quantitative Research
Answers to questions like these can help social workers know about the populations they serve — or hope to serve in the future.
- How many students currently receive reduced-price school lunches in the local school district?
- How many hours per week does a specific individual consume digital media?
- How frequently did community members access a specific medical service last year?
Quantitative data — facts that can be measured and expressed numerically — are crucial for social work.
Quantitative research has advantages for social scientists. Such research can be more generalizable to large populations, as it uses specific sampling methods and lends itself to large datasets. It can provide important descriptive statistics about a specific population. Furthermore, by operationalizing variables, it can help social workers easily compare similar datasets with one another.
Qualitative Research
Qualitative data — facts that cannot be measured or expressed in terms of mere numbers or counts — offer rich insights into individuals, groups and societies. It can be collected via interviews and observations.
- What attitudes do students have toward the reduced-price school lunch program?
- What strategies do individuals use to moderate their weekly digital media consumption?
- What factors made community members more or less likely to access a specific medical service last year?
Qualitative research can thereby provide a textured view of social contexts and systems that may not have been possible with quantitative methods. Plus, it may even suggest new lines of inquiry for social work research.
Mixed Methods Research
Combining quantitative and qualitative methods into a single study is known as mixed methods research. This form of research has gained popularity in the study of social sciences, according to a 2019 report in the academic journal Theory and Society. Since quantitative and qualitative methods answer different questions, merging them into a single study can balance the limitations of each and potentially produce more in-depth findings.
However, mixed methods research is not without its drawbacks. Combining research methods increases the complexity of a study and generally requires a higher level of expertise to collect, analyze and interpret the data. It also requires a greater level of effort, time and often money.
The Importance of Research Design
Data-driven practice plays an essential role in social work. Unlike philanthropists and altruistic volunteers, social workers are obligated to operate from a scientific knowledge base.
To know whether their programs are effective, social workers must conduct research to determine results, aggregate those results into comprehensible data, analyze and interpret their findings, and use evidence to justify next steps.
Employing the proper design ensures that any evidence obtained during research enables social workers to reliably answer their research questions.
Research Methods in Social Work
The various social work research methods have specific benefits and limitations determined by context. Common research methods include surveys, program evaluations, needs assessments, randomized controlled trials, descriptive studies and single-system designs.
Surveys involve a hypothesis and a series of questions in order to test that hypothesis. Social work researchers will send out a survey, receive responses, aggregate the results, analyze the data, and form conclusions based on trends.
Surveys are one of the most common research methods social workers use — and for good reason. They tend to be relatively simple and are usually affordable. However, surveys generally require large participant groups, and self-reports from survey respondents are not always reliable.
Program Evaluations
Social workers ally with all sorts of programs: after-school programs, government initiatives, nonprofit projects and private programs, for example.
Crucially, social workers must evaluate a program’s effectiveness in order to determine whether the program is meeting its goals and what improvements can be made to better serve the program’s target population.
Evidence-based programming helps everyone save money and time, and comparing programs with one another can help social workers make decisions about how to structure new initiatives. Evaluating programs becomes complicated, however, when programs have multiple goal metrics, some of which may be vague or difficult to assess (e.g., “we aim to promote the well-being of our community”).
Needs Assessments
Social workers use needs assessments to identify services and necessities that a population lacks access to.
Common social work populations that researchers may perform needs assessments on include:
- People in a specific income group
- Everyone in a specific geographic region
- A specific ethnic group
- People in a specific age group
In the field, a social worker may use a combination of methods (e.g., surveys and descriptive studies) to learn more about a specific population or program. Social workers look for gaps between the actual context and a population’s or individual’s “wants” or desires.
For example, a social worker could conduct a needs assessment with an individual with cancer trying to navigate the complex medical-industrial system. The social worker may ask the client questions about the number of hours they spend scheduling doctor’s appointments, commuting and managing their many medications. After learning more about the specific client needs, the social worker can identify opportunities for improvements in an updated care plan.
In policy and program development, social workers conduct needs assessments to determine where and how to effect change on a much larger scale. Integral to social work at all levels, needs assessments reveal crucial information about a population’s needs to researchers, policymakers and other stakeholders. Needs assessments may fall short, however, in revealing the root causes of those needs (e.g., structural racism).
Randomized Controlled Trials
Randomized controlled trials are studies in which a randomly selected group is subjected to a variable (e.g., a specific stimulus or treatment) and a control group is not. Social workers then measure and compare the results of the randomized group with the control group in order to glean insights about the effectiveness of a particular intervention or treatment.
Randomized controlled trials are easily reproducible and highly measurable. They’re useful when results are easily quantifiable. However, this method is less helpful when results are not easily quantifiable (i.e., when rich data such as narratives and on-the-ground observations are needed).
Descriptive Studies
Descriptive studies immerse the researcher in another context or culture to study specific participant practices or ways of living. Descriptive studies, including descriptive ethnographic studies, may overlap with and include other research methods:
- Informant interviews
- Census data
- Observation
By using descriptive studies, researchers may glean a richer, deeper understanding of a nuanced culture or group on-site. The main limitations of this research method are that it tends to be time-consuming and expensive.
Single-System Designs
Unlike most medical studies, which involve testing a drug or treatment on two groups — an experimental group that receives the drug/treatment and a control group that does not — single-system designs allow researchers to study just one group (e.g., an individual or family).
Single-system designs typically entail studying a single group over a long period of time and may involve assessing the group’s response to multiple variables.
For example, consider a study on how media consumption affects a person’s mood. One way to test a hypothesis that consuming media correlates with low mood would be to observe two groups: a control group (no media) and an experimental group (two hours of media per day). When employing a single-system design, however, researchers would observe a single participant as they watch two hours of media per day for one week and then four hours per day of media the next week.
These designs allow researchers to test multiple variables over a longer period of time. However, similar to descriptive studies, single-system designs can be fairly time-consuming and costly.
Learn More About Social Work Research Methods
Social workers have the opportunity to improve the social environment by advocating for the vulnerable — including children, older adults and people with disabilities — and facilitating and developing resources and programs.
Learn more about how you can earn your Master of Social Work online at Virginia Commonwealth University . The highest-ranking school of social work in Virginia, VCU has a wide range of courses online. That means students can earn their degrees with the flexibility of learning at home. Learn more about how you can take your career in social work further with VCU.
From M.S.W. to LCSW: Understanding Your Career Path as a Social Worker
How Palliative Care Social Workers Support Patients With Terminal Illnesses
How to Become a Social Worker in Health Care
Gov.uk, Mixed Methods Study
MVS Open Press, Foundations of Social Work Research
Open Social Work Education, Scientific Inquiry in Social Work
Open Social Work, Graduate Research Methods in Social Work: A Project-Based Approach
Routledge, Research for Social Workers: An Introduction to Methods
SAGE Publications, Research Methods for Social Work: A Problem-Based Approach
Theory and Society, Mixed Methods Research: What It Is and What It Could Be
READY TO GET STARTED WITH OUR ONLINE M.S.W. PROGRAM FORMAT?
Bachelor’s degree is required.
VCU Program Helper
This AI chatbot provides automated responses, which may not always be accurate. By continuing with this conversation, you agree that the contents of this chat session may be transcribed and retained. You also consent that this chat session and your interactions, including cookie usage, are subject to our privacy policy .
- Architecture and Design
- Asian and Pacific Studies
- Business and Economics
- Classical and Ancient Near Eastern Studies
- Computer Sciences
- Cultural Studies
- Engineering
- General Interest
- Geosciences
- Industrial Chemistry
- Islamic and Middle Eastern Studies
- Jewish Studies
- Library and Information Science, Book Studies
- Life Sciences
- Linguistics and Semiotics
- Literary Studies
- Materials Sciences
- Mathematics
- Social Sciences
- Sports and Recreation
- Theology and Religion
- Publish your article
- The role of authors
- Promoting your article
- Abstracting & indexing
- Publishing Ethics
- Why publish with De Gruyter
- How to publish with De Gruyter
- Our book series
- Our subject areas
- Your digital product at De Gruyter
- Contribute to our reference works
- Product information
- Tools & resources
- Product Information
- Promotional Materials
- Orders and Inquiries
- FAQ for Library Suppliers and Book Sellers
- Repository Policy
- Free access policy
- Open Access agreements
- Database portals
- For Authors
- Customer service
- People + Culture
- Journal Management
- How to join us
- Working at De Gruyter
- Mission & Vision
- De Gruyter Foundation
- De Gruyter Ebound
- Our Responsibility
- Partner publishers

Your purchase has been completed. Your documents are now available to view.
Statistics in Social Work
An introduction to practical applications.
- Amy Batchelor
- X / Twitter
Please login or register with De Gruyter to order this product.
- Language: English
- Publisher: Columbia University Press
- Copyright year: 2019
- Audience: Professional and scholarly;
- Other: 50 figures
- Published: November 19, 2019
- ISBN: 9780231550222

Site Content
Statistics in social work.
An Introduction to Practical Applications
Amy Batchelor
Columbia University Press
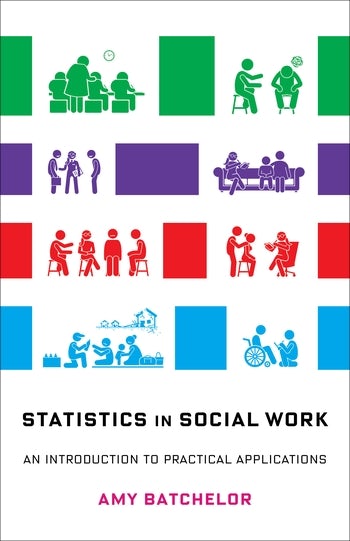
Pub Date: November 2019
ISBN: 9780231193276
Format: Paperback
List Price: $30.00 £25.00
Shipping Options
Purchasing options are not available in this country.
ISBN: 9780231193269
Format: Hardcover
List Price: $90.00 £75.00
ISBN: 9780231550222
Format: E-book
List Price: $29.99 £25.00
- EPUB via the Columbia UP App
- PDF via the Columbia UP App
Statistics in Social Work is a practical and effective resource for social work students. Batchelor requires no prior knowledge of statistics from her readers and explains topics in plain language with relatable examples. Most importantly, she offers a social justice perspective that emphasizes and integrates the core value of the social work profession. Ashley Davis, Boston University
This is an excellent introduction to statistics for both students and practitioners in social work—it demystifies terms and procedures and uses real world examples to help the reader to see the everyday applicability of statistical knowledge, whether in practice or in study. John Devaney, coauthor of Quantitative Research Methods for Social Work: Making Social Work Count
About the Author
- Social Work
- Mathematics
- Social Sciences
- Social Work: Research Methods
(Stanford users can avoid this Captcha by logging in.)
- Send to text email RefWorks EndNote printer
Statistics in social work : an introduction to practical applications
Available online.
- EBSCO University Press
More options
- Find it at other libraries via WorldCat
- Contributors
Description
Creators/contributors, contents/summary.
- Acknowledgments 1. Introduction 2. Creating Useful Data 3. Understanding People and Populations 4. Variance: The Distance Between Us 5. The Statistics of Relationships 6. Sampling: The Who and the How 7. What Works? Hypothesis Testing and Inferential Statistics 8. When Two Is Not Enough: Testing with Multiple Groups 9. An Introduction to Advanced Concepts
- Appendix I: Glossary
- Appendix II: Answer Key for Review Questions
- Appendix III: Equations Cheat Sheet References Index.
- (source: Nielsen Book Data)
Bibliographic information
Browse related items.

- Stanford Home
- Maps & Directions
- Search Stanford
- Emergency Info
- Terms of Use
- Non-Discrimination
- Accessibility
© Stanford University , Stanford , California 94305 .
- Skip to header navigation
- Skip to primary navigation
- Skip to main content
- Skip to primary sidebar
- Skip to footer
- Member login
International Federation of Social Workers
Global Online conference
Statistics in Social Work: An Introduction to Practical Application
April 1, 2020
Author: Amy Batchelor Paperback: 191 pages Publisher: Columbia University Press, New York Language: English ISBN: 9780231193276
Review author : Ian Harris
Batchelor presents a concise and approachable introduction to statistics relevant to social workers. She uses a systematic chapter structure that opens with a brief overview of the material to be considered followed by a list of specific learning objectives. The material is presented as a series of responses to practical questions and summaries of key concepts making it easy for the reader to identify content relevant to their particular interests. Discussion is frequently illustrated with examples showing arithmetic working and specific cases related to practice issues. The chapters close with a key take away summary and short questions that the reader can use to test themselves.
The topics covered start with basic concepts like ‘variable’, ‘level of measurement’ and ‘population’ before progressing to descriptive measures of dispersion, central tendency and relatedness. These are followed by an examination of experimental methods, hypothesis testing and inferential statistics including some work on the analysis of results from multiple groups using F-tests and ANOVA. The appendices comprise a glossary of more than seventy key terms, answers to end of chapter review questions and a cheat sheet for descriptive techniques.
Detailed instructions on calculating descriptive statistics are supplied but the more extended calculations for inference are not. Instead, there is guidance on reading the results of such calculations as they are usually reported in research literature, e.g. test values for Student’s t, ANOVA and χ 2 ; degrees of freedom, and alpha or ‘p’ values. Curiously, given that is relatively easier to collect ordinal level data, there is no discussion of Wilcoxon-Mann-Whitney type tests based on the summing of ranks. Also, the otherwise very useful decision tree for selecting the appropriate tests neglects the possibility of drawing inferences from ordinal level data and only indicates χ 2 as an alternative to tests requiring ratio or interval level data. It is, of course possible to treat ordinal level data as categorical and so count the frequencies needed for χ 2 but this is not discussed either.
The discussion of experimental design and the selection of the appropriate statistics to calculate when testing hypotheses was brief and not as extensively illustrated as the earlier content on descriptive statistics. However, it could be a useful source of support for readers trying to think critically about methodological issues in research reports. This is consistent with the larger ambition of equipping the practitioner with critical insights necessary for the recognition of biases and limitations otherwise obscured by complex models and the appearance of scientific neutrality. To this end there are frequent illustrations of sources of invalidity including sampling biases, neglect of error margins and related distortions of confidence.
This goes some way to counterbalance claims made about the power of statistical procedures to reliably indicate what works and focus interventions and activities to greatest effect. These claims are made on the basis of a commitment to Evidence Based Practice (EBP) in the sense given by the NASW definition [i] . It is hard to ignore the extent to which EBP has become associated with a particular approach to welfare policy and practice [ii] . It is something of a truism to claim that EBP’s positivism originates in narratives that privilege a technical-rational conception of science and a preference for the bureaucratic-legal organisation of the state. These narratives and values are arguably deeply rooted in the Enlightenment traditions of the West and are consequently open to criticisms of potential cultural bias. Therefore, the relatively unexplored assertion of the validity of EBP appears somewhat inconsistent with the advocacy of critical exploration of biases obscured by complexity and the appearance of neutrality. Clearly, it would be outside the scope of an introductory instructional manual to rehearse all such considerations but some acknowledgement of the issue might have been valuable.
That said, the intent to equip and promote critical engagement with the often obscure reporting of research evidence is laudable and timely. If you are looking for a source that will help you overcome a sense of statistics as alien and inaccessible then this book would provide you with a good starting point.
[i] See the American National Association of Social Workers website at https://www.socialworkers.org/News/Research-Data/Social-Work-Policy-Research/Evidence-Based-Practice
[ii] See Witkin, S., & Harrison, W. (2001). Editorial: Whose Evidence and for What Purpose? Social Work, 46(4), 293-296, for an early overview of the range of critical and supportive perspectives taken on EBP. For more recent contributions see Betts Adams, K., Matto, H. & Winston LeCroy, C. (2009) Limitations of Evidence-Based Practice for Social Work Education: Unpacking the Complexity, Journal of Social Work Education, 45:2, 165-186 and Bergmark, A. & Lundström, T. (2011) Guided or independent? Social workers, central bureaucracy and evidence-based practice, European Journal of Social Work, 14:3, 323-337
Review author : Ian Harris is a lecturer in social work and teaches at the University of Essex. He specialises in curriculum design and professional education particularly in the practice of assessing risks, vulnerabilities and exploitation. Ian has a long-standing interest in the relationships between vocation and knowledge which he explores from critical perspectives in the study of culture.
- Login / Account
- Documentation
- Online Participation
- Constitution/ Governance
- Secretariat
- Our members
- General Meetings
- Executive Meetings
- Meeting papers 2018
- Global Definition of Social Work
- Meet Social Workers from around the world
- Frequently Asked Questions
- IFSW Africa
- IFSW Asia and Pacific
- IFSW Europe
- IFSW Latin America and Caribbean
- IFSW North America
- Education Commission
- Ethics Commission
- Indigenous Commission
- United Nations Commission
- Information Hub
- Upcoming Events
- Keynote Speakers
- Global Agenda
- Archive: European DM 2021
- The Global Agenda
- World Social Work Day
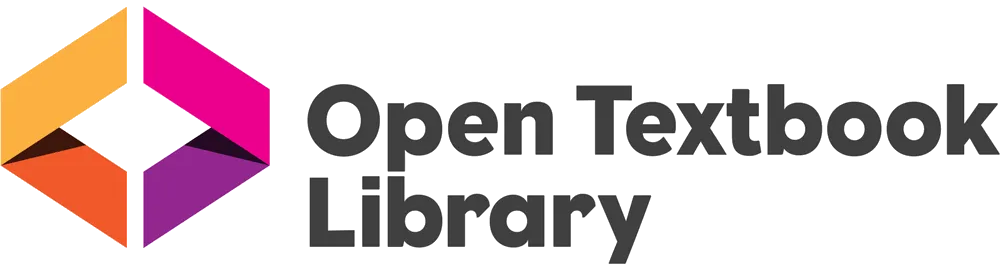
Foundations of Social Work Research
(4 reviews)

Rebecca L. Mauldin
Copyright Year: 2020
ISBN 13: 9781648169915
Publisher: Mavs Open Press
Language: English
Formats Available
Conditions of use.

Learn more about reviews.
Reviewed by LaToya Smith-Jones, Adjunct Professor, University of Texas at Arlington on 3/26/24
The textbook covers various topics that are familiar to the Social Work profession. There are relatable examples given within the book, which allow Social Work students to understand discussions through the lens of an actual practitioner. Each... read more
Comprehensiveness rating: 5 see less
The textbook covers various topics that are familiar to the Social Work profession. There are relatable examples given within the book, which allow Social Work students to understand discussions through the lens of an actual practitioner. Each section provides an area where research vocabulary is listed and reviewed, as well as examples to deepen the understanding of the vocabulary used.
Content Accuracy rating: 5
The information presented in the textbook is presented with accuracy. Bias was not noticed within the text.
Relevance/Longevity rating: 5
The information presented within the textbook was up-to-date. Classical studies were also included in the textbook. The classical studies allow the students to understand the historical influence regarding the research process.
Clarity rating: 5
The textbook provides examples and a separate vocabulary section in order to understand the jargon and technical terminology. individuals who do not have a research background will be able to comprehend the information written.
Consistency rating: 5
The textbook is consistent regarding terminology and framework. Each section builds upon the previous section.
Modularity rating: 5
Each section is broken up according to the topic of the chapter. Each chapter is broken up in sections, which allows for an easier read.
Organization/Structure/Flow rating: 5
The chapters are presented in a logical and clear fashion. The information presented within the textbook builds upon itself. Students are first introduced to background information regarding the topic and then they are given information regarding the application of the information shared.
Interface rating: 5
There were not any interface issues.
Grammatical Errors rating: 5
There were not any grammatical errors noted.
Cultural Relevance rating: 5
Information within the text was inclusive and included examples of various ethnicities and backgrounds.
The textbook is excellent to use for students who do not have a research background. The manner in which the information is presented and laid out assists with aiding students' understanding.
Reviewed by Quentin Maynard, Assistant Professor, University of Southern Indiana on 11/30/22
This text covers topics that social work students need to understand to be consumers of research. The author and contributors include current real work examples to help emphasize the different topics. Integrating the chapter on Real World Research... read more
This text covers topics that social work students need to understand to be consumers of research. The author and contributors include current real work examples to help emphasize the different topics. Integrating the chapter on Real World Research throughout the text might help emphasize to students that engaging in research is necessary to our profession, even as practitioners.
The content was accurate and error-free.
The content of the text was up-to-date and included information relevant to social work research. Since the main author solicited contributions from colleagues at their institution, updates and changes would likely be relatively straightforward.
The book seemed accessible for individuals with limited research experience. Key words were defined in the text and included in a glossary at the end of each section and the text.
The text was consistent in style and organization. Chapter subsections have specific learning objectives allowing students to know what will be covered in each chapter. Doing this reduces bloat and increases clarity for readers.
The text did not appear to be structured in a way that was overwhelming or difficult to follow.
The structure of the book was logical.
The digital pdf and the online versions of the text were intuitive and easy to navigate. I did not notice any issues with the interface in either format.
No writing or grammar errors noted.
The text is culturally sensitive. It includes a content advisory at the beginning of each chapter which allows students to be aware of specific topics (e.g., racism, sexism, and poverty) discussed or mentioned in the chapter. While this text was adapted for students at a specific university, the authors include topics that reach much farther than that audience. The examples included cover a diverse set of people and situations.
This is a comprehensive text that allows students the opportunity to learn how to be consumers of social work research. While practice evaluation might not be the scope of this text, other than the chapter on Real World Research, including discussions about how students might apply the concepts of each chapter in social work practice. The structure of the book allows students to see the research that their professors are engaging in and might make research more accessible to social work students and practitioners
Reviewed by Matt Walsh, Assistant Professor of Social Work, Marian University on 12/30/21
This textbook covers all the aspects of research you would expect for an introduction to social work research. It uses classic examples of past research to highlight the importance of ethics in research. It also does a good job of discussing... read more
This textbook covers all the aspects of research you would expect for an introduction to social work research. It uses classic examples of past research to highlight the importance of ethics in research. It also does a good job of discussing both quantitative and qualitative research as well as single system designs and program evaluation. My one critique as someone who does qualitative research is that it mentions the importance of trustworthiness and rigor in qualitative research but does not mention how a research can achieve this. However, it does go into other elements like coding and it would not be hard to provide student with supplemental materials about memoing or peer debriefing as examples and to be fair, it is hard to put everything in just one chapter.
All components are accurately described and well-written. The glossary at the end of each section is helpful for key words. The text appears to be error-free and unbiased.
There are links to recent examples which highlights the real world aspect of research.
This text is clear in its description of research and its major components. Certain aspects like causality get a little advanced for a introduction to research book but there are good visual to aid in students' understanding of some of the more complicated concepts. (Please note that I am reviewing this with BSW students in mind, MSW students may not find some of these sections as overwhelming as I suspect my students might).
The book is very well structured and consistent throughout.
The text is well structured and organized as a whole and in terms of each chapter and each section with the chapters.
The topics follow the order of most other foundational research books I have seen and have a logical flow to them.
I did not find any interface issues.
I could not see any grammatical errors.
There are good examples throughout that display an effort to have inclusivity, diversity, and equity in this text.
I feel like this book would provide students with a good understanding about research and could be used interchangeably with other foundational/introduction books on the market, especially if the professor is familiar with teaching research and has already established a good foundation (quizzes, lecture slides, assignments, activities, etc.).
Reviewed by Vivian Miller, Assistant Professor in Social Work, Bowling Green State University on 1/5/21
The text Foundations of Social Work Research covers social work research comprehensively and appropriately. Across twelve chapters, the author begins by introducing research, the science behind research and how this translates to the profession of... read more
The text Foundations of Social Work Research covers social work research comprehensively and appropriately. Across twelve chapters, the author begins by introducing research, the science behind research and how this translates to the profession of social work, and the importance of understanding research as it applies to social work practice across all system levels. In addition to comprehensive chapters, the text contains a glossary, practice behavior indices, bibliography, derivative notes, and links by each chapter.
This text is an accurate text that is error free. This text is extremely well-written and includes real-life examples, drawing on written contributions from social work faculty across practice settings and populations, as well as students at the masters and doctoral levels.
Much of research methods and the process is overall static, however the author does an incredible job to provide timely, relevant, and applicable examples throughout the text to ensure that this version will not be obsolete within a short period of time.
This text is clearly written and is easy to move through. This text contains chapters and sub-chapters. I’d recommend this book for a higher-level undergraduate program or graduate program (e.g., MSW), as there is technical terminology used. Additionally, the author provides a glossary at the back of the text, hyperlinked to each chapter on the web-version. Moreover, there are definitions highlighted at center page throughout the text.
This text is very consistent. Chapters build on one another and are written in clear order.
The use of subheadings throughout allows this text to be separated into smaller reading sections. For instance, if an instructor wanted to assign reading for “Probability sampling,” this topic can be readily extracted from the full text. A student can understand this topic area despite being separated from the text as context is provided to the reader in each sub-chapter. The use of bolded words, images, examples, and hyperlinks throughout make the text easy to separate and digest.
This text is very well-organized and moves through each section in a step-wise process building on each previous content area.
There are no interface issues in the text. Images display well, as well as key takeaway and glossary charts throughout each chapter.
The text contains no grammatical errors.
This text is culturally sensitive. Examples across all system levels (e.g., micro, messo, and macro) are inclusive of a variety of races, ethnicities, and backgrounds.
Highly recommend this text for a Social Work research course.
Table of Contents
- Chapter One: Introduction to research
- Chapter Two: Linking methods with theory
- Chapter Three: Ethics in social work research
- Chapter Four: Design and causality
- Chapter Five: Defining and measuring concepts
- Chapter Six: Sampling
- Chapter Seven: Survey research
- Chapter Eight: Experimental design
- Chapter Nine: Unique features of qualitative research
- Chapter Ten: Unobtrusive research
- Chapter Eleven: Real-world research
- Chapter Twelve: Reporting research
Ancillary Material
About the book.
This textbook was created to provide an introduction to research methods for BSW and MSW students, with particular emphasis on research and practice relevant to students at the University of Texas at Arlington. It provides an introduction to social work students to help evaluate research for evidence-based practice and design social work research projects. It can be used with its companion, A Guidebook for Social Work Literature Reviews and Research Questions by Rebecca L. Mauldin and Matthew DeCarlo, or as a stand-alone textbook.
About the Contributors
Rebecca L. Mauldin , Ph.D
Contribute to this Page
The use of statistics in social sciences
Journal of Humanities and Applied Social Sciences
ISSN : 2632-279X
Article publication date: 17 October 2019
Issue publication date: 30 October 2019
The purpose this paper is to review some of the statistical methods used in the field of social sciences.
Design/methodology/approach
A review of some of the statistical methodologies used in areas like survey methodology, official statistics, sociology, psychology, political science, criminology, public policy, marketing research, demography, education and economics.
Several areas are presented such as parametric modeling, nonparametric modeling and multivariate methods. Focus is also given to time series modeling, analysis of categorical data and sampling issues and other useful techniques for the analysis of data in the social sciences. Indicative references are given for all the above methods along with some insights for the application of these techniques.
Originality/value
This paper reviews some statistical methods that are used in social sciences and the authors draw the attention of researchers on less popular methods. The purpose is not to give technical details and also not to refer to all the existing techniques or to all the possible areas of statistics. The focus is mainly on the applied aspect of the techniques and the authors give insights about techniques that can be used to answer problems in the abovementioned areas of research.
- Time series
- Social sciences
- Non-parametric
- Multivariate
- Statistical modeling
Maravelakis, P. (2019), "The use of statistics in social sciences", Journal of Humanities and Applied Social Sciences , Vol. 1 No. 2, pp. 87-97. https://doi.org/10.1108/JHASS-08-2019-0038
Emerald Publishing Limited
Copyright © 2019, Petros Maravelakis.
Published in Journal of Humanities and Applied Social Sciences . Published by Emerald Publishing Limited. This article is published under the Creative Commons Attribution (CC BY 4.0) licence. Anyone may reproduce, distribute, translate and create derivative works of this article (for both commercial and non-commercial purposes), subject to full attribution to the original publication and authors. The full terms of this licence may be seen at http://creativecommons.org/licences/by/4.0/legalcode .
1. Introduction
According to Dodge (2008) , Statistics:
[…] is made up of a set of techniques for obtaining knowledge from incomplete data, from a rigorous scientific system for managing data collection, their organization, analysis, and interpretation, when it is possible to present them in numeric form.
The purpose of this paper is to try to review the statistical techniques in the field of social sciences in other words social statistics.
Social statistics is the field of statistical science that deals with the study of social phenomena and in particular human behavior in a social environment. Such phenomena are any kind of human activities, including activities of groups of people like households, societies and nations and their impacts on culture, education and other areas. Generally, we can say that social statistics deal with the application of statistical methodology in areas like survey methodology, official statistics, sociology, psychology, political science, criminology, public policy, marketing research, demography, education, economics and others.
Due to the nature of social sciences it is common to study indicators that cannot be measured directly. Moreover, data that is unobservable, informal, illegal or “too personal” are often studied in this area ( Lovric (2011) ). For example, a social researcher may be interested on the data (answers) of the question “Do you participate in illegal gambling?”. Other similar questions may be asking, for example, about the sexual behavior of a respondent, possible addictions etc.
In this paper, we review some statistical methods that are used in social sciences and we draw the attention of researchers on less popular methods. Our purpose is not to give technical details and also not to refer to all the existing techniques or to all the possible areas of statistics. We focus mainly on the applied aspect of the techniques and we give insights about techniques that can be used to answer problems in the abovementioned areas of research.
The remaining of the paper is organized as follows. In Section 2, we refer to the sampling issues which are of fundamental importance to gather data that are representative of the population under study. Section 3 gives an overview of descriptive statistics, regression analysis and analysis of variance. Since regression is a method heavily used to model quantitative data it is of great importance in social sciences. Analysis of variance can be used to identify if one or more categorical variables have a statistically significant effect on a continuous (dependent) variable. Parametric models are presented in Section 4. It is practically impossible to cover all the available models but a number of important models are outlined. Non-parametric methods are given in Section 5. These methods are particularly important because assumptions in parametric models are frequently questionable in practice. Some multivariate techniques are described in Section 6. Since most of the data contain several different variables that are related multivariate techniques are a necessary tool. Usual practice in questionnaires is to collect categorical data with inherent order and without order. In Section 7, we describe some of the popular techniques that analyze such data. Some of the time series methods to analyze data that are dependent over time are given in Section 8. In Section 9, we present the data mining methods that can be used to identify patterns in large data sets. Finally, we give some conclusions and recommendations.
2. Sampling
The first issue before the use of any statistical method is the collection of the sample. We may say that sampling is a collection of techniques on how to select a number of individuals from the target population to estimate certain characteristics of the population that we want to study. There are two ways to select a sample, either using a probability or a nonprobability sample. In probability sampling every unit of the population has a chance of being selected in the sample. Moreover, this probability is greater than zero and it can be computed. The methods that are used are simple random sampling, systematic sampling, stratified sampling, cluster sampling and multistage sampling (or combinations of these methods). On the other hand, in nonprobability sampling some of the units of the population have zero probability of being selected or the probability of being selected cannot be computed. The most known non-probability sampling methods are intentional sampling, convenience sampling, quota sampling and snowball sampling.
The main difference between probability and non-probability sampling is the fact that with probability sampling we draw a random sample. This assertion is extremely important due to the fact that using statistical theory we can extend the results from a random sample to draw conclusions about the population. This is not allowed in non-probability sampling.
The way to select a random sample is apparently very important. However one may observe that in papers published in social sciences this fact is overlooked. Specifically, emphasis is given to the conclusions of the research effort and not on the way data was gathered. This fact is surely a very important factor on the reliability of the results. In the same issue, another statistical question that needs special attention is the determination of the sample size. In many published papers the authors just refer to the size of the sample without referring to the technical details of its computation. This is another serious problem that affects the credibility of the results in a survey.
These two problems are not the only that appear in surveys in social sciences but surely they are the most usual. Other issues that occur are blurred definition of the population, problems during the collection of the data that are rarely mentioned (for example replacement of selected units), non-sampling errors (for example non-response, over or under coverage) and others.
Generally, we can say that in many cases sampling is treated with less attention than what is needed. Researchers in the field and practitioners may refer to the classical book by Kish (1995) . Other useful references are Cohen (1988) , Joseph et al. (1997) , Lenth (2001) and Shuster (1990) .
3. Descriptive statistics, regression and analysis of variance
The first step in any statistical analysis is the use of descriptive statistics to present the data and try to identify any kind of trends, relationships or abnormal behavior. Analysis based on descriptive statistics or exploratory data analysis usually makes no stochastic assumptions. A first approach in parametric tests is to use the classic hypothesis tests and confidence intervals. Apart from that there are also other statistical methods that can be employed in social sciences. Regression analysis and analysis of variance (ANOVA) are some of the classical methods.
3.1 Regression
Regression is one of the most known methods used for analyzing relationships between variables. The main objectives of a regression analysis is to check if there is an association between variables, to identify the strength of this relationship and to conclude to a regression equation that is used to describe this relationship.
There are several forms of regression modeling, for example, linear regression, logistic regression and regression discontinuity. There are also other aspects of the regression methodology but we confine ourselves to these cases. All these methodologies have been extensively used in real cases of social sciences.
Linear regression is the simplest of these methods since it is used to model the relationship between one dependent variable and one or more explanatory variables. In this methodology, we try to find a function to fit the values of the explanatory variable that vary linearly with the target variable. Linear regression is particularly useful since it is able to predict the value of the dependent variable given the value of the explanatory variable or variables. We have to stress that in this method the target variable (dependent variable) is continuous.
In logistic regression we want to obtain a nonlinear curve to fit the data when the target variable is discrete. This methodology is particularly useful in modeling a target variable having value for example Yes (0) or No (1). More formally we can say that the target variable is binomial. Our aim is to find an equation that functionally connects the values of the explanatory variables to the values of the target variable. The explanatory variables can be either continuous or categorical. Since the range of the explanatory variables can be between -∞ and ∞ a proper transformation is applied to the target variable. If we transform the target variable to the logarithm of the odds of its values then the transformed target variable is linearly related to the explanatory variables. For more details about the linear and the logistic regression the interested reader can refer to Kutner et al. (2005) .
Regression discontinuity is used to compute the effect of an intervention. This methodology is able to give unbiased estimates of this intervention. In a regression discontinuity design we use a rule to assign the intervention to a unit. This methodology is extensively used in education. Specifically, a scoring rule is used after a test is given in a class to select the students that need more effort on the specific course. Students with scores below a cutoff value are assigned to the group that will spend more time studying and students with scores above the cutoff value are assigned to the comparison group, or vice versa.
The effect of the intervention is estimated as the difference in the mean outcome of the treatment group and the comparison group. A regression line or curve is estimated for the two groups (treatment and comparison groups), and the difference in the mean of these regression lines at the cutoff value of the measured variable is the estimate of the effect of the intervention. We conclude that there was an effect of the intervention if a “discontinuity” appears between the two regression lines at the cutoff value. A detailed description of regression discontinuity is given in Riley-Tilman and Burns (2009) and Jacob and Zhu (2012) .
3.2 Analysis of variance
Analysis of variance (ANOVA) is a well-known method used to compare several means at the same time using a fixed confidence level. The data used are the results of an experiment. There is a continuous dependent variable, and one or more qualitative independent variables (categorical or nominal variables). The design of the experiment must be done in such a way that it will not affect its results. For example, a completely randomized experiment does not affect the output of the experiment. However, the choice of the design of the experiment affects which analysis of variance method will be used. There are a lot of different designs of experiments and analysis of variance methods for several different cases.
Regression analysis and analysis of variance are closely related. If we use dummy variables as independent variables in analysis of variance then the analysis becomes regression analysis. However, there is a serious difference between the two methods. In the analysis of variance if the design of the experiment is properly done, we may conclude that there is causality (the independent variable has a causal effect on the dependent variable). On the other hand, in regression analysis a statistically significant effect may mean causality or not (a statistically significant result does not necessarily mean causal effect).
The analysis of variance tests the independence of the response and explanatory variables. If we decide that there is this type of dependence then we have to do extra analysis to identify which means are different and to what extent.
The analysis of variance assumes that the samples in the groups (categories of the independent variable) are independent. This means that each group has a different sample of subjects. However, there are cases where each group has the same sample of subjects. Apparently, the samples are then dependent and of course we have to take this fact into consideration to reach credible results. This case is called repeated measures analysis of variance.
For more information on this topic, see Agresti and Finlay (2009) and Cohen and Lea (2004) .
4. Parametric methods
Assume that a researcher wants to use the ANOVA and apart from the dependent variable and a categorical variable (factor), data for one or more quantitative variables measured on each experimental unit are available. Then, if these variables have an effect on the outcome of the experiment, they can be used in the model as independent variables. Such variables are called covariates or concomitant variables. The analysis involving all these variables is called analysis of covariance. Although the model is more complex by including the extra variables, the profit is that the error variance is reduced.
Another very useful class of models is mixed models. Mixed models contain both fixed and random effects. They are particularly useful in social sciences when we have repeated measurements. Moreover, in the case of missing data, which are very common in sample surveys, mixed models offer a strong alternative to methods like ANOVA for repeated measures. Their drawback is that estimation is more difficult along with the fact that we end up to have a more complex model.
A useful class of models is also the semiparametric models (or even better the semiparametric regression models). These regression models include both parametric and nonparametric components. They are used when the usual parametric models do not have a satisfactory performance. More about nonparametric methods are given in Section 5.
Another very useful method is robust regression. Keeping in mind the usefulness of linear regression, its wide applicability and acceptance between the researchers it is natural to propose a method that overcomes the difficulty to fulfill its assumptions. Robust regression is used to avoid the effect of outliers. One approach is to use the M-estimators and another one is to replace the normal distribution in the assumptions with a heavy-tailed distribution.
Undoubtedly methods like linear regression and ANOVA have been used to an enormous extent in social sciences but many times without the proper accuracy in the details. We believe that much of the work done could be improved using the more advanced models presented in this section. For more details the reader could refer to Christensen (2011) and Rencher and Schaalje (2008) . For robust regression a useful reference is Rousseeuw and Leroy (1987) .
5. Nonparametric methods
In social statistics the vast amount of research is based on parametric methods. However, many parametric methods are based on strong assumptions that are disregarded most of the times. This has serious effect on the justification of the results.
The alternative in this case is to use nonparametric statistical methods. Nonparametric statistics do not rely on a specific family of probability distributions and there is no assumption about the probability distributions of the variables used. Therefore it is an ideal collection of methods for handling real data that most of the times fail to follow these strong assumptions of parametric inference.
There is a number of techniques that are already popular among the researchers in social sciences. Such techniques are certain hypothesis tests like Wilcoxon Signed rank test, Mann-Whitney test and Kruskal-Wallis tests. Other used techniques are the Spearman correlation coefficient, the runs test and normality tests. For a detailed review of such techniques the interested reader can refer to Corder and Foreman (2009) .
However, there is a number of other nonparametric methods that have been developed and are already famous among statisticians that have not gained much attention between the researchers in social sciences. Such methods are the jackknife and the bootstrap methods. Jacknife can be used to compute the bias and the variance of an estimator whereas bootstrap estimates the variance and the distribution of a statistic or it is used to construct confidence intervals. It must be noted that both these methods are computationally demanding. Nevertheless, they can be very useful in social sciences especially in the cases of complex estimators of parameters that need to be further studied.
Another useful method is nonparametric regression. The usual linear regression is a heavily used method in social sciences. However, its assumptions are very rarely referred due to the fact that they rarely hold. Nonparametric regression is a solution in that case. It is able to answer the initially stated problem that led to regression with flexibility in terms of the assumed model. Other interesting nonparametric methods are the ones used for density estimation like cross-validation and density estimation. These methods estimate the probability distribution function using just the data. They can be used in cases where the distribution of the data is unknown and difficult to be computed analytically. If a researcher is able to compute the distribution function of the variable or variables under study then he/she can obtain statistical methodologies like confidence intervals or hypothesis testing making the decision process easier and credible. For more details about these methods the interested reader could refer to Wasserman (2006) .
6. Multivariate methods
Usually in social sciences and generally in real problems more than one variable is involved. These variables need to be considered together since most of the times they are related. Several methods have been developed for the analysis of such data. These methods include among others cluster analysis, correspondence analysis, principal component analysis and factor analysis.
One of the main goals of multivariate analysis is classification. Cluster analysis is a method of classification which aims to group individuals (objects) so that those allocated to a particular group are, in a way, considered to be close together. The data used in cluster analysis are a data matrix where the columns are used for the objects and the rows for the attributes that describe the object. The output of a cluster analysis is the clusters that are used to characterize objects as similar or not. In hierarchical cluster analysis the clusters appear as a tree (they have hierarchy). In nonhierarchical cluster analysis, the number of clusters are determined by the researcher which have to be less than the number of objects. Both of these techniques are processed through statistical software. The allocation of people in similar groups is very important for a social scientist since it gives him the ability to pin point the special characteristics of these groups.
Correspondence analysis is an exploratory technique that helps a scientist to analyze multi-way frequency tables. Its main goal is to plot the data using less dimensions to identify their key features. The data used in this method have to be nonnegative and they should appear in a data table. Correspondence analysis aims to display data tables in two-dimensional spaces, called maps. The idea behind this method is that the model must follow the data, and not the opposite. In its simplest form we have a variable that we want to model and several explanatory variables. All these variables are frequencies appearing in one or more contingency tables. We use cross-tabulation for each of the explanatory variables and the variable we want to model to identify the level of their association. A technique which is also used, is to stack the tables before the application of correspondence analysis to reveal the relationship of the variable we want to model with the explanatory variables in the same map.
Principal component analysis is used to summarize p -correlated variables by a smaller number of uncorrelated variables. These variables contain most of the information that exist in the original set of variables. Keeping in mind the vast amount of data a social scientist has at hand today, we may conjecture that this technique is very important. The fact that we end up with a smaller number of variables, demands less computational power to perform the analysis of the remaining variables. Moreover, the fact that the variables are uncorrelated makes the analysis easier since the techniques used do not have to consider a relationship between the variables used. However, there a number of drawbacks. First of all, the fact that a piece of information is lost may affect the conclusions of the analysis. Moreover, if we begin with thousands of variables (which is not rare today) we may have to work with a lot of variables even after the application of principal component analysis to retain most of the information in the data.
As we already stated in the introduction sometimes in social science research, we cannot measure the variable or variables that we are interested in a direct way. These variables are called latent variables and commonly they are called factors. An example of a latent variable is human intelligence. In Factor analysis we try to relate the observable to the unobservable variables by a probability model to make statistical inference. The main objective of the analysis is to select the number of the latent variables that have to be used to explain the correlations between the unobservable variable and to interpret them. Another objective is to predict the values of the latent variables that produced the observable variables. In factor analysis the researcher regresses each of the observed variables on the set of the latent variables. Usually after the computation of the factors a social scientist tries to “name” them based on the numerical findings. However, since there is not a specific way to perform this action, the result of this step is sometimes not properly elaborated. For all the above methods indicative references are Everitt (1993) , Greenacre (2007) , Jolliffe (2002) and Bartholomew and Knott (2011) .
Apart from these well-known methods there are also some other methods equally important but less used. These methods are path analysis, structural equation modeling and multilevel modeling.
Path analysis is concerned with causation. Specifically it uses regression methods to identify patterns of causation in networks. In the beginning path analysis starts with a network of variables to specify the paths of causation. Usually, a cause and effect relationship assumes that there are a number of relationships and some variables that are believed to be caused by others, appear to affect other variables. A regression model cannot identify such a case because it can merely use one dependent variable. In path analysis all the necessary regression models considered, account for all the relationships needed.
Structural theory tries to give the structural relationships between constructs. This theory is represented by a structural model using a number of equations. These equations are usually accompanied by a proper diagram indicating the relationships. In other words, structural equation modeling is a method that tries to estimate the relationship between latent variables. This relationship can be linear or non-linear. The advantage of this method is that it allows us to test hypotheses on the relationships between observed variables and latent variables and also between the latent variables themselves.
Multilevel modeling is used to analyze data involving clusters. Specifically, in social research we are often concerned with the relationship between individuals and the groups they belong. This relationship actually leads to nested data, that is individuals nested within groups. For example in education students are nested within schools. The performance of a student in a series of exams could be affected by both characteristics of the student and of the school he/she attended.
For path analysis, structural equation modeling and multilevel modeling, the interested reader can refer to Agresti and Finlay (2009) , Bartholomew et al. (2008) and Timm (2002) .
7. Categorical data
Usually in social sciences researchers have to analyze categorical data. A categorical variable can take a limited number of specific discrete values. Usually such values occur for example when respondents are assigned in groups or when a property holds or not. In social sciences the different categories of a categorical variable often measures attitudes and opinions.
Categorical variables with a natural ordering are called ordinal variables. Categorical variables without ordering are called nominal variables. Methods designed for ordinal variables cannot be used with nominal variables due to the fact that nominal variables do not have ordered categories. Methods designed for nominal variables can be used with nominal or ordinal variables, since they only require a categorical scale.
The most famous models for analyzing categorical data are logistic regression models. Logistic regression can be used with continuous and discrete predictors ( Agresti (2007) ). Loglinear models are used to analyze associations among multiple categorical response variables. A log-linear model can be transformed using logarithm to a polynomial function of the parameters of the model. This is very helpful since the researcher can use linear regression ( Azen and Walker (2011) ).
A broad class of models is the generalized linear models. These models are a generalization of ordinary linear regression in the sense that it allows the distribution of the error to be different from the normal distribution. Another class of models is those that are used to analyze repeated measures data or longitudinal data. That kind of data is repeated observations of the same variables over several periods of time. One feature that must be taken into consideration is that data are correlated since the same subjects are measured over time ( Lawal (2003) ).
We may say that in general researchers in social sciences could rely more on the abovementioned models for the analysis of categorical data. These models are not very popular among researchers who tend to rely more on descriptive measures. We believe that the practitioners in the area could benefit a lot from the already developed methods.
8. Time series
Time series is a sequence of observations on a variable of interest with chronological order. That kind of data is quite natural in some of the fields in social sciences like economics. The observations in a time series are considered dependent. Time series analysis is a collection of techniques for the analysis of this time dependence.
There are a lot of different approaches to handle time series data. A first approach is to use the autoregressive models or the moving average models. The autoregressive model (AR) assumes that there is linear dependence of the variable we study with its own previous values. The moving average (MA) model is a linear regression of the current value of the series against current and previous (in terms of time) error terms.
Another class of models are the autoregressive moving average (ARMA) models. We use the notation ARMA( p , q ) to define a model with p autoregressive terms and q moving-average terms. A generalization of this model is the autoregressive integrated moving average (ARIMA) model. This model is generally referred as ARIMA( p , d, q ) where parameters p , d, and q are non-negative integers that refer to the order of the autoregressive, integrated and moving average parts of the model respectively. All the above mentioned models (AR, MA, ARMA, ARIMA) form among other techniques the Box-Jenkins method for modeling time series. For more details Box et al. (2008) .
Another class of time series models, especially useful in econometrics, are the autoregressive conditional heteroskedasticity (ARCH) models. In ARCH models we assume that the variance of the current error term is a function of the actual sizes of the previous time periods’ error terms. ARCH models have been extensively used to model financial time series. A generalization of the ARCH models is the generalized autoregressive conditional heteroskedasticity models (GARCH). In GARCH models we assume that the error variance is modeled by an ARMA model. There are a number of newer model proposals based on ARCH and GARCH models. The interested reader about ARCH models can refer to Xekalaki and Degiannakis (2010) .
Another interesting characteristic in time series is forecasts. Apparently, it has attracted the interest of researchers in various fields. Several techniques on this very interesting issue have been proposed. Methods and examples of applications are given in Bisgaard and Kulahci (2011) .
The research in social sciences, using the already stated models for time series, mainly appears in economics and marketing. We strongly believe that researchers in other areas of social sciences could benefit from these models also.
9. Data mining
Data mining is a collection of techniques used to find patterns in a set of data. They are extremely important in the analysis of large data sets of social phenomena. Other names that refer to the same collection of techniques are machine learning and predictive analytics. During the last years there is an increasing interest in these techniques although most of them are known for decades. We have to note here that the use of a computer is compulsory to run these techniques and moreover that if we have large data sets the larger the amount of data the more computational power we need.
The computational methods that comprise the field of data mining derive from the areas of statistics and artificial intelligence. These techniques are used to find meaningful associations between related variables usually between a large number of variables. These structures help the practitioner to draw useful conclusions about his/her research questions.
An important feature that is one of the objectives of a data mining analysis is the generalization of the results. To be more specific, if after an analysis of the data at hand using data mining techniques we conclude that there are some important patterns, then we would also like to find that these patterns exist and in the data that we will gather in future. This generalization is very important for drawing conclusions that are irrespective of the collected data the specific time we run the analysis.
If we consider the predictive dimension of data mining we can refer to the two important conclusions of such an analysis. The first conclusion is that after we reach a useful and meaningful model we can use it to predict the variable under study using some or all the remaining variables. Obviously, such a conclusion gives the researcher the ability to compute the values of the dependent variable given the values of the independent variables. The second conclusion is that the researcher is able to comment about the relationship between the dependent and the independent variables.
Another characteristic we need to highlight is the need to know as much as possible about the data and the process. The definition of the variables, the way they are measured and their interrelation in terms of the case studied are extremely important to the researcher to assist him reach a meaningful conclusion. Additionally, since the data are most of the times in vast numbers there is the need to store, process and compute them. Therefore, it is highly probable that knowledge of databases and parallel computing will be compulsory for the application of data mining techniques.
Keeping in mind the vast amount of social data that are gathered in today’s world using classical ways (e.g. questionnaires) along with the use of mobile technologies, social networks, texts, photographs, videos and all the different types of human activities that are transformed to data we can easily conclude that it is not a rare event to have to analyze thousands of variables with many cases in each of them. In such cases we can say that we end up with big data (data with high volume, high velocity and high variety). This fact highlights the need to use data mining techniques that can handle such amount of data. More information and detailed representation of data mining techniques can be found in Hastie et al. (2009) and Azzalini and Scarpa (2010) .
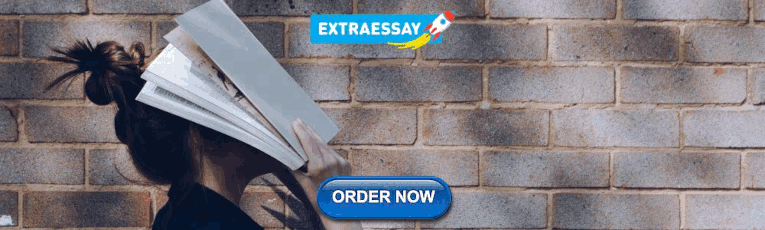
10. Conclusions
In this paper, we reviewed some statistical methods useful in the area of social sciences. Sampling techniques, regression analysis, analysis of variance, parametric and nonparametric models along with multivariate methods were presented. Categorical data analysis techniques, time series methods and data mining were also presented. Indicative references in all of these areas are also given.
Statistical methods have played a very important role in social sciences. In every applied research effort statistical techniques are compulsory to reach a non-questionable conclusion. We strongly believe that advanced statistical methods can be employed heavily in this area. It seems that researchers rely more on classical statistical methods although they could benefit from the use of newer and advanced techniques.
Agresti , A. ( 2007 ), An Introduction to Categorical Data Analysis , John Wiley , New York, NY .
Agresti , A. and Finlay , B. ( 2009 ), Statistical Methods for the Social Sciences , 4th ed. , Pearson/Prentice Hall , NJ .
Azen , R. and Walker , C.M. ( 2011 ), Categorical Data Analysis for the Behavioral and Social Sciences , Routledge , New York, NY .
Azzalini , A. and Scarpa , B. ( 2010 ), Data Analysis and Data Mining , Oxford University Press , New York, NY .
Bartholomew , D.J. and Knott , M. ( 2011 ), Latent Variable Models and Factor Analysis , 2nd ed. , Vol. 7 , Kendall’s Library of Statistics , Arnold .
Bartholomew , D.J. , Steele , F. , Moustaki , I. and Galbraith , J.I. ( 2008 ), Analysis of Multivariate Social Science Data , 2nd ed. , CRC Press , New York, NY .
Bisgaard , S. and Kulahci , M. ( 2011 ), Time Series Analysis and Forecasting by Example , John Wiley , New York, NY .
Box , G.E.P. , Jenkins , G.M. and Reinsel , G.C. ( 2008 ), Time Series Analysis: forecasting and Control , 4th ed. , John Wiley , New York, NY .
Christensen , R. ( 2011 ), Plane Answers to Complex Questions: The Theory of Linear Models , Springer .
Cohen , J. ( 1988 ), Statistical Power for Behavioral Sciences , Lawrence Erlbaum Assoc ., Mahwah, NJ .
Cohen , B.H. and Lea , R.B. ( 2004 ), Essentials of Statistics for the Social and Behavioral Sciences , John Wiley , New York, NY .
Corder , G.W. and Foreman , D.I. ( 2009 ), Nonparametric Statistics for Non-Statisticians: A Step-by-Step Approach , John Wiley , New York, NY .
Dodge , Y. ( 2008 ), The Concise Encyclopedia of Statistics , Springer .
Everitt , B. ( 1993 ), Cluster Analysis , Arnold , London .
Greenacre , M.J. ( 2007 ), Correspondence Analysis in Practice , Chapman and Hall , Boca Raton .
Hastie , T. , Tibshirani , R. and Friedman , J. ( 2009 ), The Elements of Statistical Learning: data Mining, Inference, and Prediction , Springer , New York, NY .
Jacob , R. and Zhu , P. ( 2012 ), A Practical Guide to Regression Discontinuity , mdrc .
Jolliffe , I.T. ( 2002 ), Principal Component Analysis , 2nd ed. , Springer , New York, NY .
Joseph , L. , Burger , R.D. and Belisle , P. ( 1997 ), “ Bayesian and mixed bayesian/likelihood criteria for sample size determination ”, Statistics in Medicine , Vol. 16 No. 7 , pp. 769 - 789 .
Kish , L. ( 1995 ), Survey Sampling , John Wiley , New York, NY .
Kutner , M.H. , Nachtsheim , C.J. , Neter , J. and Li , W. ( 2005 ), Applied Linear Statistical Models , McGraw-Hill/Irwin , New York, NY .
Lawal , B.H. ( 2003 ), Categorical Data Analysis with SAS and SPSS Applications , Lawrence Erlbaum Associates , NJ .
Lenth , R.V. ( 2001 ), “ Some practical guidelines for effective sample size calculations ”, American Statistician , Vol. 55 No. 3 , pp. 187 - 193 .
Lovric , M. ( 2011 ), International Encyclopedia of Statistical Science , Springer .
Rencher , A.C. and Schaalje , G.B. ( 2008 ), Linear Models in Statistics , John Wiley , New York, NY .
Riley-Tilman , T.C. and Burns , M.K. ( 2009 ), Evaluating Educational Interventions , The Guilford Press , New York, NY .
Rousseeuw , P.J. and Leroy , A.M. ( 1987 ), Robust Regression and Outlier Detection , John Wiley , New York, NY .
Shuster , J.J. ( 1990 ), Handbook of Sample Size Guidelines for Clinical Trials , CRC Press , Boca Raton, FL .
Timm , N.H. ( 2002 ), Applied Multivariate Analysis , Springer , New York, NY .
Wasserman , L. ( 2006 ), All of Nonparametric Statistics , Springer , New York, NY .
Xekalaki , E. and Degiannakis , S. ( 2010 ), ARCH Models for Financial Applications , John Wiley , New York, NY .
Acknowledgements
This article is an invited submission and was not peer reviewed.
Corresponding author
Related articles, we’re listening — tell us what you think, something didn’t work….
Report bugs here
All feedback is valuable
Please share your general feedback
Join us on our journey
Platform update page.
Visit emeraldpublishing.com/platformupdate to discover the latest news and updates
Questions & More Information
Answers to the most commonly asked questions here
Using Statistics in Social Research
A Concise Approach
- © 2013
- Scott M. Lynch 0
Princeton University Department of Sociology, Princeton, USA
You can also search for this author in PubMed Google Scholar
- A more compact and concise approach to interpreting statistical survey research data
- Details in an accessible way integral relationship between probabilistic reasoning and statistical inference
- Uses simulation exercises to demonstrate key points, such as Central Limit Theorem ?
- Includes supplementary material: sn.pub/extras
113k Accesses
18 Citations
This is a preview of subscription content, log in via an institution to check access.
Access this book
- Available as EPUB and PDF
- Read on any device
- Instant download
- Own it forever
- Compact, lightweight edition
- Dispatched in 3 to 5 business days
- Free shipping worldwide - see info
- Durable hardcover edition
Tax calculation will be finalised at checkout
Other ways to access
Licence this eBook for your library
Institutional subscriptions
Table of contents (12 chapters)
Front matter, introduction.
Scott M. Lynch
Overview of the Research Process
Data and its acquisition, summarizing data with descriptive statistics, probability theory, statistical inference, statistical approaches for nominal data: chi-square tests, comparing means across multiple groups: analysis of variance (anova), correlation and simple regression, introduction to multiple regression, presenting results of statistical analysis, back matter.
- applied statistics in social science
- psychometrics
- quantitative social research
- social science research
- statistical methods
- statistics in sociology
About this book
This book covers applied statistics for the social sciences with upper-level undergraduate students in mind. The chapters are based on lecture notes from an introductory statistics course the author has taught for a number of years. The book integrates statistics into the research process, with early chapters covering basic philosophical issues underpinning the process of scientific research. These include the concepts of deductive reasoning and the falsifiability of hypotheses, the development of a research question and hypotheses, and the process of data collection and measurement. Probability theory is then covered extensively with a focus on its role in laying the foundation for statistical reasoning and inference. After illustrating the Central Limit Theorem, later chapters address the key, basic statistical methods used in social science research, including various z and t tests and confidence intervals, nonparametric chi square tests, one-way analysis of variance, correlation, simple regression, and multiple regression, with a discussion of the key issues involved in thinking about causal processes. Concepts and topics are illustrated using both real and simulated data. The penultimate chapter presents rules and suggestions for the successful presentation of statistics in tabular and graphic formats, and the final chapter offers suggestions for subsequent reading and study.
Authors and Affiliations
About the author.
Scott M. Lynch is a professor in the Department of Sociology and Office of Population Research at Princeton University. His substantive research interests are in changes in racial and socioeconomic inequalities in health and mortality across age and time, as well as in understanding the sources of regional disparities in health in the U.S. His methodological interests are in the application of Bayesian statistics and estimation methods to problems that cannot be easily addressed with classical statistical methods in sociology and demography.
Bibliographic Information
Book Title : Using Statistics in Social Research
Book Subtitle : A Concise Approach
Authors : Scott M. Lynch
DOI : https://doi.org/10.1007/978-1-4614-8573-5
Publisher : Springer New York, NY
eBook Packages : Mathematics and Statistics , Mathematics and Statistics (R0)
Copyright Information : Springer Science+Business Media New York 2013
Hardcover ISBN : 978-1-4614-8572-8
Softcover ISBN : 978-1-4939-5306-6
eBook ISBN : 978-1-4614-8573-5
Edition Number : 1
Number of Pages : XXIII, 229
Number of Illustrations : 44 b/w illustrations
Topics : Statistics for Social Sciences, Humanities, Law , Statistical Theory and Methods
- Publish with us
Policies and ethics
- Find a journal
- Track your research
- USC Libraries
- Research Guides
- SOWK 546: The Science of Social Work
- Demographics, Data & Statistics for Social Work
SOWK 546: The Science of Social Work: Demographics, Data & Statistics for Social Work
- Finding Scholarly Articles
- Developing a Search Strategy
- Research Designs and Methods
- Evaluating Research Effectively
- Appraising Research
- Evidence-based Practice Resources
- Understanding Journal Impact Factors
- Policy and Legislation Resources
- Tests & Measurements
- Background on Theories/Reference Sources
- APA 7th Edition
Finding, Using and Managing Data
- Data Reference Toolkit by Andy Rutkowski Last Updated Jan 11, 2024 310 views this year
- Statistics & Data by Eimmy Solis Last Updated Mar 26, 2024 1783 views this year
Finding Statistics and Data
Data is the raw information that is analyzed to create statistics, or interpreted data. Data can be numerical, or qualitative (interviews, opinion data, etc).

- Roper Center The Roper Center maintains an archive of public opinion survey data--including the General Social Survey (1972-2008). Allows users to search survey questions or download data directly. Users will need to enter their USC email address to access data.
- National Council on Disabilility NCD is an independent federal agency charged with advising the President, Congress, and other federal agencies regarding policies, programs, practices, and procedures that affect people with disabilities.
International/Global Data and Statistics
- International Education Statistics National Center for Education Statistics (NCES), Institute of Education Sciences (IES)
- Pan American Health Organization PAHO is the specialized international health agency for the Americas. It works with countries throughout the region to improve and protect people's health
- UNICEF Statistics United Nations International Children's Emergency Fund (UNICEF)
- United Nations Statistics Provides data on international trade, national accounts, energy, industry, environment and demographic and social statistics gathered from national and international sources.
- World Health Organization WHO's annual World Health Statistics reports present the most recent health statistics for the WHO Member States.
- Inter-university Consortium for Political and Social Research This link opens in a new window The Inter-university Consortium for Political and Social Research (ICPSR), established in 1962, is an integral part of the infrastructure of social science research. more... less... ICPSR maintains and provides access to a vast archive of social science data for research and instruction.
Finding Community Demographics
- U.S. Census Bureau Data The Census Bureau is the leading source of quality data about the nation's people and economy. You have two options to start your search on the landing page of data.census.gov: a free-form single search bar or an advanced search filtering experience.
- Census Data Tutorials These tutorials are from the U.S. Census Bureau and will help with navigating the new database.
- Homelessness Data These raw data sets contain Point-in-Time (PIT) estimates and national PIT estimates of homelessness as well as national estimates of homelessness by state and estimates of chronic homelessness from 2007 - 2019
- Pew Research Center Pew Research Center is a nonpartisan fact tank that informs the public about the issues, attitudes and trends shaping the world.
- Social Explorer This link opens in a new window Visually display demographic data in the U.S. from 1790-present at a variety of geographic levels. Create reports, maps and animations that can be downloaded.
View the videos below for more info on how to use social explorer.
Open and Free Data
- CHHS Open Data California Health and Human Services (CHHS) Open Data Portal
- Data.gov Data.gov is the homepage for the U.S. Government's open data. You will find Federal, state, and local data.
- HealthData.gov This information includes clinical care provider quality information, nationwide health service provider directories, databases of the latest medical and scientific knowledge, consumer product data, community health performance information, government spending data.
- Journal of Open Psychology Data The Journal of Open Psychology Data (JOPD) features peer reviewed data papers describing psychology datasets with high reuse potential. Data papers may describe data from unpublished work, including replication research, or from papers published previously in a traditional journal.
- Kids Count Data Center Annie E. Casey Foundation database including over 100 indicators of child well-being, including economic status, health, safety, and risk factors on the local community, city, state, and national level.
- Los Angeles Open Data A portal to find information, insights and analysis from the city of Los Angeles.
- openICPSR openICPSR provides a place for researchers to archive, store and share their data. All data included in OpenICPSR is free for public use and primarily focuses on behavioral health and social sciences.
Los Angeles and California

- California Department of Education, Data and Statistics Data and statistics collected from California schools and learning support resources to identify trends and educational needs and to measure performance
- California Department of Rehabilitation The California Department of Rehabilitation (DOR) is an employment and independent living resource for people with disabilities.
- California Department of Social Services (CDSS) Data Portal California Department of Social Services (CDSS)
- Healthy City Healthycity.org is an online platform for accessing data, creating maps, and working collaboratively. It allows users to theme and filter data, conduct research, and connect with their communities. From the Advancement Project California.
- Los Angeles Homeless Services Authority
- Know Your Community: LA City The City of Los Angeles hub for information, data, and resources.
- << Previous: Policy and Legislation Resources
- Next: Tests & Measurements >>
- Last Updated: Apr 11, 2024 10:03 AM
- URL: https://libguides.usc.edu/sowk546

- University of Texas Libraries
- UT Libraries
Social Work Research
- Data and Statistics
- Getting Started
- Encyclopedias & Handbooks
- Evidence Based Practice
- Peer Review & Evaluation
- Reading Scholarly Articles
- Books & Dissertations
- Film & Video
- Policy & Government Documents
- Trabajo Social
- Citing & Citation Management
- Research Funding
- Open Access
- Choosing & Assessing Journals
- Increasing Access to Your Work
- Tracking Your Impact
- Systematic Reviews
- Tests & Measures
Recommended Sources
- Children and Family
- Criminal Justice
- Immigration
Please either create a free account or use the Guest Login to enter the site.
We have a limited number of users for this database. Please select the logout option before you leave your session. When the limit is reached, a username and password prompt will probably be displayed. If this happens, simply wait 15 minutes and then try the link again.
- Wikidata the free knowledge base with 75,231,432 data items that anyone can edit.
- AARP State Data Center This site provides statistics and reports on Social Security, Medicare, housing, long-term care, poverty level, caregiver estimates and more on a state-by-state level.
- AoA - Profile on Older Americans Each year, the Administration on Aging (AoA) compiles the latest statistics on the older population compiled primarily from U.S. Census data into a Profile of Older Americans. The profile provides data on changes in demographics of the population over age 65, including 15 topical areas (such as population, income and poverty, living arrangements, education, health, and caregiving).
- CDC Healthy Aging Data The Healthy Aging Data Portal provides easy access to CDC data on a range of key indicators of health and well-being, screenings and vaccinations, caregiving, and mental health, including subjective cognitive decline among older adults at national and state levels.
- ChildStats.gov The Federal Interagency Forum on Child and Family Statistics's annual report, America's Children: Key National Indicators of Well-Being, provides the Nation with a summary of national indicators of child well-being and monitors changes in these indicators over time.
- Child Welfare Information Gateway Find reports, databases, and other sources of statistics and demographic data on children and families in the United States, child abuse and neglect, child welfare services, children and youth in foster care, and domestic and international adoption.
- Kids Count Data Center Data by state, across states and more.
- Texas DFPS Data Book Data collection and published by the Texas Department of Family and Protective Services.
- U.S. Census - Families & Living Arrangements The Census Bureau collects data about American families for the nation, states and communities. Our statistics describe trends in household and family composition, and show the number of children, young adults and couples living in the United States.
- Bureau of Justice Statistics The primary source for United States’ criminal justice statistics. Information on crime, criminal offenders, victims of crime, and the operation of justice systems at all levels of government can be found on this site.
- Global and regional estimates of violence against women - WHO The report presents the first global systematic review of scientific data on the prevalence of two forms of violence against women: violence by an intimate partner (intimate partner violence) and sexual violence by someone other than a partner (non-partner sexual violence).
- Sourcebook of Criminal Justice Statistics The Sourcebook of Criminal Justice Statistics brings together data from more than 100 published and unpublished sources about many aspects of criminal justice in the United States.
- Office of Juvenile Justice and Delinquency Prevention Statistical Briefing Book Enables users to access online information via OJJDP's Web site to learn more about juvenile crime and victimization and about youth involved in the juvenile justice system
- Bureau of Justice Statistics - Violent Crime Includes statistics on crimes against youth, female victims of sexual assault, and crimes against persons with disabilities.
- NCES Data Tools
- OECD Education GPS Provides statistics on education from 68 countries such as average performance in mathematics, science literacy and reading by 15-year-old students, adult literacy rates and literacy averages across varying age ranges.
- U.S. Census - Educational Attainment The United States Census Bureau provides tables and reports on educational attainment from the American Community Survey, Current Population Survey and other Census data sources.
- World Bank Education Statistics The World Bank EdStats All Indicator Query holds over 4,000 internationally comparable indicators that describe education access, progression, completion, literacy, teachers, population, and expenditures.
- ED Data Express Updated annually, Ed Data Express is focused on student achievement, demographics, accountability, and performance data from grant programs administered in the Office of Elementary and Secondary Education.
- Texas Education Agency State education database that includes information about 1,200 Texas school districts and charters, as well as TEA.
- Additional Education Data Sources
- National Center for Health Statistics The National Center for Health Statistics is part of the Centers for Disease Control and Prevention, Department of Health and Human Services.
- MedLine Plus: Health Information by Population Group Produced by the National Library of Medicine, MedLine Plus is the world’s largest medical library. It includes information about diseases, conditions, and wellness issues broken down by population group.
- Disability Statistics Maintained by the Employment and Disability Institute, Cornell University. Includes national and state level data on a variety of topics related to people with disabilities and includes an interactive search tool.
- National Institute of Mental Health The information on these pages includes currently available statistics on the prevalence and treatment of mental illnesses among the U.S. population. In addition, information is provided about possible consequences of mental illnesses, such as suicide and disability.
- Health Disparity Widget From the US Department of Health and Human Services Office of Minority Health, easily search by health indicator and disparity type.
- Substance Abuse and Mental Health Services Administration Congress established the Substance Abuse and Mental Health Services Administration (SAMHSA) in 1992 to make substance use and mental disorder information, services, and research more accessible.
- Additional Health Data Sources
- Immigration Data & Statistics US data published by the Department of Homeland Security's Office of Immigration Statistics (OIS)
- Migration Data Hub International data on immigration statistics, maps, and numbers from the Migration Policy Institute
- US Census Bureau Foreign Born Population The foreign-born population includes anyone who is not a U.S. citizen at birth, including those who become U.S. citizens through naturalization.
- Department of Justice Executive Office for Immigration Review Scroll down to Executive Office of Immigration Review (EOIR) section.
- Pew Research Center Hispanic Trends This Pew Research Center project provides a wealth of information on the Hispanic population in the United States as well as statistics on U.S. immigration trends and trends in unauthorized immigration.
- Additional Immigration Resources
- National Center for Children in Poverty State profiles, data tools and more are available.
- US Census Bureau Poverty The Census Bureau provides poverty data from several household surveys and programs. Here you can find poverty estimates, learn about these surveys and programs, and get guidance on how to choose the right estimate for your needs.
- Poverty and Equity Data Portal The portal allows you to explore several poverty and inequality indicators for countries and regions as well as explore countries by various income levels – low income, lower middle income, and upper middle income, and access poverty and inequality data for fragile, IDA and other country groupings.
- State of the Nation's Housing This report is produced annually by the Joint Center for Housing Studies, Harvard University. Informative tables and figures are included.
- Food and Nutrition Services Data & Statistics Provided data and research regarding USDA Nutrition Assistance programs including Supplemental Nutrition Assistance Program (SNAP), School Meals, and WIC.
Research and Industry
Applications in the social sciences.
Statistics plays a central role in the social sciences, applying rigorous quantitative methods to understand and solve some of the greatest challenges affecting human societies. Statistics is especially critical at a time when the volume and type of available data are transforming the social sciences.
Berkeley statistics faculty study pressing problems from across the social sciences: economics, policy, and quantitative finance; the census, official statistics, and demography; political science and election integrity and security; sociology, social network analysis, and computational social science; and education and psychometrics. Faculty have close connections and joint appointments with other UC Berkeley departments (including Demography, Political Science, Public Policy) and are core members of the campus’s new computational social science training program. Faculty also have active collaborations with social science researchers, non-profit organizations, companies, and government agencies from across the world.
The innovative work today builds on the Berkeley statistics department’s long history at the intersection of statistics and the social sciences. This is particularly true in the use of statistics in the law—in a landmark case on census adjustment in 1990, the US Supreme Court ruled unanimously in favor of an analysis from faculty Ken Wachter and (the late) David Freedman. Berkeley Statistics faculty continue to serve as leading experts on related matters.
Researchers
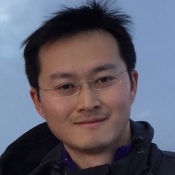
causal inference in experiments and observational studies, with applications to biomedical and social sciences; contaminated data including missing data, measurement error, and selection bias
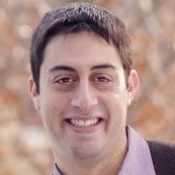
applied statistics, theoretical statistics, Bayesian statistics, machine learning, statistics in social sciences
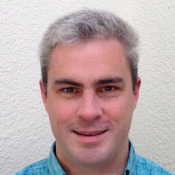
Michael Mahoney
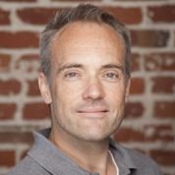
Jon McAuliffe
machine learning, statistical prediction, variational inference, statistical computing, optimization
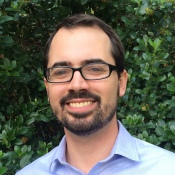
Sam Pimentel
causal inference, health services & policy analysis, biostatistics, discrete optimization
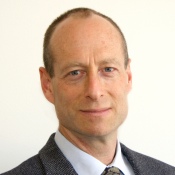
Philip B. Stark
uncertainty quantification and inference, inverse problems, nonparametrics, risk assessment, earthquake prediction, election auditing, geomagnetism, cosmology, litigation, food/nutrition

Ken Wachter
Mathematical demography, statistical models for the evolution of aging, systematic constraints and random influences that shape the structure of human populations, computer simulation methods.
Purdue Online Writing Lab Purdue OWL® College of Liberal Arts
Welcome to the Purdue Online Writing Lab
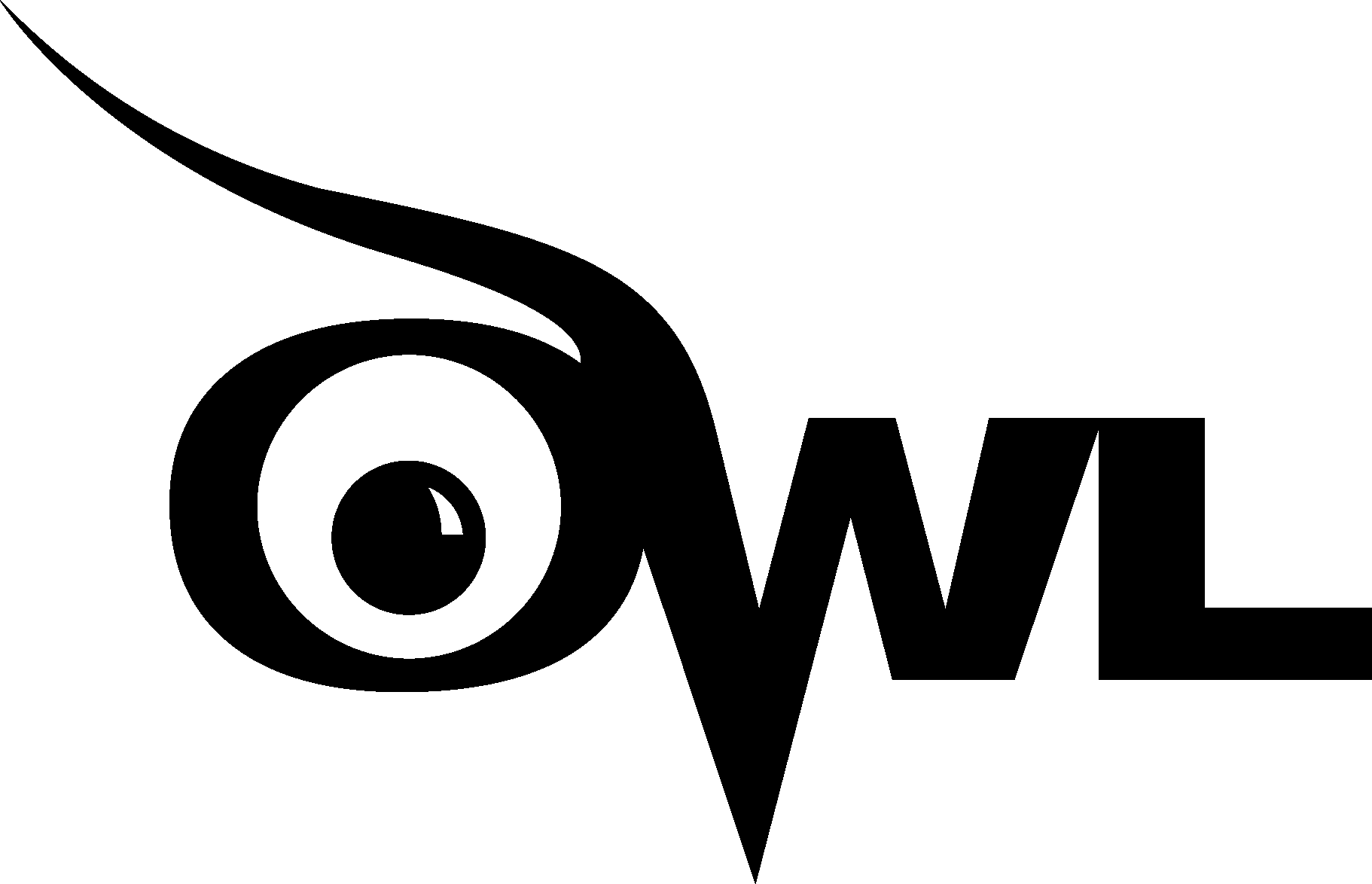
Welcome to the Purdue OWL
This page is brought to you by the OWL at Purdue University. When printing this page, you must include the entire legal notice.
Copyright ©1995-2018 by The Writing Lab & The OWL at Purdue and Purdue University. All rights reserved. This material may not be published, reproduced, broadcast, rewritten, or redistributed without permission. Use of this site constitutes acceptance of our terms and conditions of fair use.
The Online Writing Lab at Purdue University houses writing resources and instructional material, and we provide these as a free service of the Writing Lab at Purdue. Students, members of the community, and users worldwide will find information to assist with many writing projects. Teachers and trainers may use this material for in-class and out-of-class instruction.
The Purdue On-Campus Writing Lab and Purdue Online Writing Lab assist clients in their development as writers—no matter what their skill level—with on-campus consultations, online participation, and community engagement. The Purdue Writing Lab serves the Purdue, West Lafayette, campus and coordinates with local literacy initiatives. The Purdue OWL offers global support through online reference materials and services.
A Message From the Assistant Director of Content Development
The Purdue OWL® is committed to supporting students, instructors, and writers by offering a wide range of resources that are developed and revised with them in mind. To do this, the OWL team is always exploring possibilties for a better design, allowing accessibility and user experience to guide our process. As the OWL undergoes some changes, we welcome your feedback and suggestions by email at any time.
Please don't hesitate to contact us via our contact page if you have any questions or comments.
All the best,
Social Media
Facebook twitter.
Scam statistics
We’re testing a new way of providing current scam statistics that lets you filter and break down the data.
Error message
- An error occurred while fetching statistics data
Showing All scam types stats for 2024
Note: This scam type has been removed. Historical data is still available.
Note: This scam type was added in April 2018. No data is available before this date.
Amount lost
Number of reports, reports with financial losses, amount lost and number of reports, top 10 scams by amount lost, top 10 scams by reports, delivery method, gender - amount lost, gender - number of reports.
This data is based on reports provided to the ACCC by web form and over the phone.
The data is published on a monthly basis. Our quality assurance processes may mean the data changes from time to time.
Some upper level categories include scam reports classified under ‘Other’ or reports without a lower level classification due to insufficient detail provided. Consequently, upper level data is not an aggregation of lower level scam categories.
Note: Due to a technical error, some scam reports from previous months are included in July 2016 causing an increase in reports for some categories. This error has been fixed for future months.
Is this page useful?
Thanks for your feedback.
By continuing, you will be directed to a site intended only for residents of the United States and Canada. We are called MSD everywhere, except in the United States and Canada where we are known as Merck & Co., Inc., Rahway, NJ, USA.
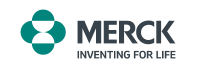
The link you have selected will take you to a third-party website, owned and operated by AgileOne. Our company does not control, and is not responsible for, the accuracy, content, practices, or standards of the pharmatempjobs.com website and/or AgileOne.
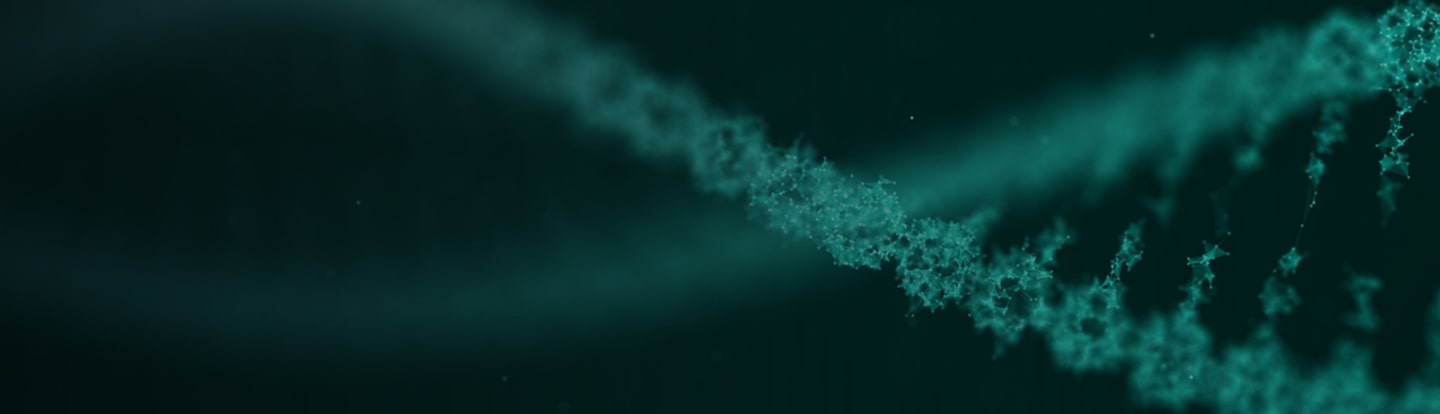
We’re sorry – the job you are trying to apply for is no longer available.
Maybe you would like to consider the Categories below:
Hybrid work model
Benefits & well-being, be yourself, curious about our hiring process.
- Reasonable accommodations for qualified individuals who require assistance due to disability
- Job Search, Application & Interview Tips
- Frequently Asked Questions
- Applicant Notice
- The Physician Payment Sunshine Act (PPSA)
Not ready to apply?
Connect with us on social, beware of hiring scams. review our applicant notice ..
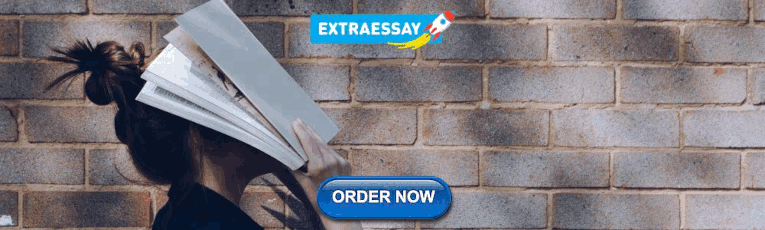
COMMENTS
Statistics in Social Work guides students through concepts and procedures from descriptive statistics and correlation to hypothesis testing and inferential statistics. Besides presenting key concepts, it focuses on real-world examples that students will encounter in a social work practice. Using concrete examples from a variety of potential ...
Based on a review of economists' debates on mathematical economics, this article discusses a key issue for shaping the science of social work—research methodology. The article describes three important tasks quantitative researchers need to fulfill in order to enhance the scientific rigor of social work research.
Social work researchers will send out a survey, receive responses, aggregate the results, analyze the data, and form conclusions based on trends. Surveys are one of the most common research methods social workers use — and for good reason. They tend to be relatively simple and are usually affordable.
John Devaney, coauthor of Quantitative Research Methods for Social Work: Making Social Work Count: This is an excellent introduction to statistics for both students and practitioners in social work—it demystifies terms and procedures and uses real world examples to help the reader to see the everyday applicability of statistical knowledge, whether in practice or in study.
Abstract. Data science is merging of several techniques that include statistics, computer programming, hacking skills, and a solid expertise in specific fields, among others. This approach represents opportunities for social work research and intervention. Thus, practitioners can take advantage of data science methods and reach new standards ...
Statistics in Social Work guides students through concepts and procedures from descriptive statistics and correlation to hypothesis testing and inferential statistics. Besides presenting key concepts, it focuses on real-world examples that students will encounter in a social work practice. Using concrete illustrations from a variety of ...
Understanding statistical concepts is essential for social work professionals. It is key to understanding research and reaching evidence-based decisions in your own practice-but that is only the beginning. If you understand statistics, you can determine the best interventions for your clients. You can use new tools to monitor and evaluate the ...
Social workers, central bureaucracy and evidence-based practice, European Journal of Social Work, 14:3, 323-337. Review author: Ian Harris is a lecturer in social work and teaches at the University of Essex. He specialises in curriculum design and professional education particularly in the practice of assessing risks, vulnerabilities and ...
This textbook was created to provide an introduction to research methods for BSW and MSW students, with particular emphasis on research and practice relevant to students at the University of Texas at Arlington. It provides an introduction to social work students to help evaluate research for evidence-based practice and design social work research projects. It can be used with its companion, A ...
The purpose this paper is to review some of the statistical methods used in the field of social sciences.,A review of some of the statistical methodologies used in areas like survey methodology, official statistics, sociology, psychology, political science, criminology, public policy, marketing research, demography, education and economics ...
The book integrates statistics into the research process, with early chapters covering basic philosophical issues underpinning the process of scientific research. These include the concepts of deductive reasoning and the falsifiability of hypotheses, the development of a research question and hypotheses, and the process of data collection and ...
Data papers may describe data from unpublished work, including replication research, or from papers published previously in a traditional journal. Kids Count Data Center Annie E. Casey Foundation database including over 100 indicators of child well-being, including economic status, health, safety, and risk factors on the local community, city ...
Published: October 2016. From $44.00. Review copy available. Instructor Teaching Site. Textbook. An Introduction to Statistics and Data Analysis Using Stata®. From Research Design to Final Report,First Edition. Lisa Daniels, Nicholas Minot. Published: January 2019.
Google Public Data Directory. Google collects publicly available data from organizations like Eurostat, OECD, U.S. Census Bureau and World Bank and offers visualizations of this data. Wikidata. the free knowledge base with 75,231,432 data items that anyone can edit.
full-time faculty members held research-focused doctorates in social work as their highest earned degree (48.9%). 89.8% held a master's degree in social work, and 51.4% held a license in social work. The average annual salary for assistant professors in social work programs
The history of social work education may have also contributed to making it difficult for those teaching on university social work courses to engage routinely in research (Orme and Powell, 2007).
Abstract. Purpose -The purpose this paper is to review some of the statistical methods used in the field of social. sciences. Design/methodology/approach -A review of some of the statistical ...
Abstract: The main objective of the article is to orient the possibilities of using statistical methods both in. education and in research in the areas of Social Work. Social Work and related ...
Understanding statistical concepts is essential for social work professionals. It is key to understanding research and reaching evidence-based decisions in your own practice—but that is only the beginning. If you understand statistics, you can determine the best interventions for your clients. You can use new tools to monitor and evaluate the progress of your client or team.
minority social work graduate students. While anxiety is a common barrier to learning statistics, a typical social work graduate course includes a "blend of students from more to less interested, anxious, and capable" (Bolen, 2006, p. 19) with statistics, and instructors often find it challenging to teach to such
Applications in the Social Sciences. Statistics plays a central role in the social sciences, applying rigorous quantitative methods to understand and solve some of the greatest challenges affecting human societies. Statistics is especially critical at a time when the volume and type of available data are transforming the social sciences ...
Inferential Statistics 332 16. Reporting of Research 357. 1 Introduction to Social Work Research *D.K. Lal Das Introduction Research is a process by which one acquires authentic and reliable information about a phenomenon. It may be ... its application in social work, its assumptions and finally to take a close
Social work study and research involve extensive use of statistical analysis. For example; Regression models are typically used to study the relationships between various social parameters to conclude how or why a social phenomenon occurs. Mathematical models in Social work research used to study social phenomena, which are used to study the ...
"Experimental research designs in social work: theory and applications." Social Work Education, ahead-of-print(ahead-of-print), pp. 1-2
2024 AP Exam Dates. The 2024 AP Exams will be administered in schools over two weeks in May: May 6-10 and May 13-17. AP coordinators are responsible for notifying students when and where to report for the exams. Early testing or testing at times other than those published by College Board is not permitted under any circumstances.
Mission. The Purdue On-Campus Writing Lab and Purdue Online Writing Lab assist clients in their development as writers—no matter what their skill level—with on-campus consultations, online participation, and community engagement. The Purdue Writing Lab serves the Purdue, West Lafayette, campus and coordinates with local literacy initiatives.
Research and resources Expand submenu for "Research and resources" Scam statistics. Scam statistics - public beta; The Little Black Book of Scams; Scams Awareness Network ... The ACCC acknowledges the Traditional Custodians of the lands across Australia on which we live and work. We acknowledge their connection to this Country and pay our ...
Apply for 2024 University Recruiting - Clinical Trial Slide Files Co-op - Fall 2024 - Spring 2025 job with Merck in North Wales, Pennsylvania, United States of America. Browse and apply for the Business Support jobs at Merck
Posted By Tim Hains On Date May 7, 2024. Via Open To Debate -- High school students have breathed a sigh of relief for the last couple of years as some colleges decided to make their applications ...
New research into B2B content marketing trends for 2024 reveals specifics of AI implementation, social media use, and budget forecasts, plus content success factors. ... Here's the good news: All that non-sexy work frees time and resources (human and tech) you can apply to bring your brand's strategies and plans to life. But in many ...