Quantum Computing Review: A Decade of Research
Ieee account.
- Change Username/Password
- Update Address
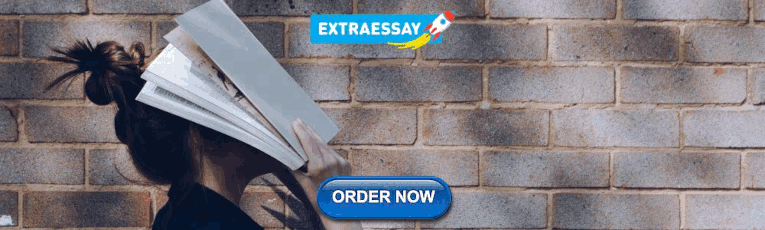
Purchase Details
- Payment Options
- Order History
- View Purchased Documents
Profile Information
- Communications Preferences
- Profession and Education
- Technical Interests
- US & Canada: +1 800 678 4333
- Worldwide: +1 732 981 0060
- Contact & Support
- About IEEE Xplore
- Accessibility
- Terms of Use
- Nondiscrimination Policy
- Privacy & Opting Out of Cookies
A not-for-profit organization, IEEE is the world's largest technical professional organization dedicated to advancing technology for the benefit of humanity. © Copyright 2024 IEEE - All rights reserved. Use of this web site signifies your agreement to the terms and conditions.
Advertisement
A review on quantum computing and deep learning algorithms and their applications
- Published: 07 April 2022
- Volume 27 , pages 13217–13236, ( 2023 )
Cite this article
- Fevrier Valdez ORCID: orcid.org/0000-0002-0159-0407 1 &
- Patricia Melin 1
7498 Accesses
1 Altmetric
Explore all metrics
In this paper, we describe a review concerning the Quantum Computing (QC) and Deep Learning (DL) areas and their applications in Computational Intelligence (CI). Quantum algorithms (QAs), engage the rules of quantum mechanics to solve problems using quantum information, where the quantum information is concerning the state of a quantum system, which can be manipulated using quantum information algorithms and other processing techniques. Nowadays, many QAs have been proposed, whose general conclusion is that using the effects of quantum mechanics results in a significant speedup (exponential, polynomial, super polynomial) over the traditional algorithms. This implies that some complex problems currently intractable with traditional algorithms can be solved with QA. On the other hand, DL algorithms offer what is known as machine learning techniques. DL is concerned with teaching a computer to filter inputs through layers to learn how to predict and classify information. Observations can be in the form of plain text, images, or sound. The inspiration for deep learning is the way that the human brain filters information. Therefore, in this research, we analyzed these two areas to observe the most relevant works and applications developed by the researchers in the world.
Similar content being viewed by others
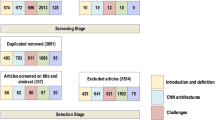
Review of deep learning: concepts, CNN architectures, challenges, applications, future directions
Laith Alzubaidi, Jinglan Zhang, … Laith Farhan
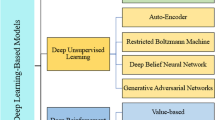
Deep learning: systematic review, models, challenges, and research directions
Tala Talaei Khoei, Hadjar Ould Slimane & Naima Kaabouch
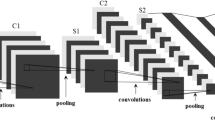
A review of convolutional neural network architectures and their optimizations
Shuang Cong & Yang Zhou
Avoid common mistakes on your manuscript.
1 Introduction
Nowadays, many intelligent algorithms have been proposed to solve complex problems, some are based on nature Baskaran et al. ( 2015 ), evolution Valdez ( 2020 ), brain behavior Krizhevsky et al. ( 2012 ), physics Rere et al. ( 2015 ), etc; which can be found in the literature, it is calculated there are more than 100 different algorithms, and improved algorithms for finding the best results on the complex problems. However, it is not our aim to analyze all existent methods. Instead, our approach will be on the quantum and deep learning algorithms and their applications. Therefore, we have selected the most relevant applications in this work. Although, we have worked with different algorithms in different ways, for example, with neural networks, fuzzy logic, evolutionary computing, QA and DL have demonstrated be two areas in Computational Intelligence to solve several problems as pattern recognition, optimization problems and can be combined with other methods to improve the performance. Therefore, we focused with the applications about quantum and deep learning algorithms.
Quantum computing works with machines that use the properties of quantum physics to store data and perform computations. This can be extremely advantageous for certain tasks where they could vastly outperform even our best supercomputers. In Montiel-Ross ( 2020 ) was made a review of quantum-inspired population-based metaheuristics, in this work, the authors reviewed which quantum-inspired metaheuristics could be translated to be used in the existing quantum computers based on the circuit model programming paradigm. Also, in Montiel-Ross et al. ( 2019 ), was proposed the quantum-inspired Acromyrmex evolutionary algorithm as a highly efficient global optimization method for complex systems.
A review of quantum neural networks is shown in Zhao and Wang ( 2021 ), where the authors observed that the quantum neural networks are higher storage capacity and computational efficiency compared to its classical counterparts. Also, in Beer et al. ( 2020 ), was proposed a truly quantum analogue of classical neurons, which form quantum feedforward neural networks capable of universal quantum computation.
In Figure 1 , is appreciated the domain which belong deep learning. In the figure, we can observe the relationship with machine learning and the artificial intelligence and DL is shown as a sub-field of artificial intelligence, and DL is a specialization of Machine Learning. Therefore, DL represents the advance stage of machine learning which mainly uses neural networks for learning and prediction of data. It is a group of different algorithms. These are used to design complex systems that can take any type of problems and give predictions. It uses the deep graph with numerous processing layers, made up of many linear and nonlinear conversions, (Schmidhuber ( 2015 ), Shinde and Shah ( 2018 )).
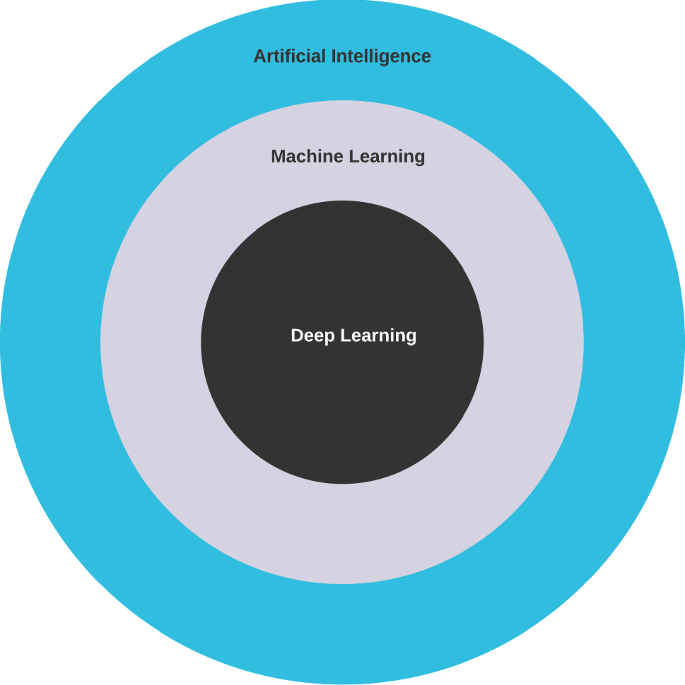
Domains of deep learning, machine learning and artificial intelligence
The main contribution in this paper, is to visualize the evolution that QC and DL algorithms have had in the last years. For this reason, we made several queries in Scopus and Web of Science to validate in the best way the obtained results. After, we collected the data in plain text to generate the clusters, networks and relations of works around the world with specific queries of the analyzed applications. To achieve obtain the graphics and presented results corresponding to queries, the VosViewer software was used in this research. VOSviewer is a software tool for constructing and visualizing bibliometric networks. These networks may for instance include journals, researchers, or individual publications, and they can be constructed based on citation, bibliographic coupling, co-citation, or co-authorship relations (Perianes-Rodriguez et al. ( 2016 ), Van Eck and Waltman ( 2014 )). The parameters used in VosViewer to build the networks, clusters and relations presented in the paper are shown in Table 1 .
This paper is organized as follows: in Section 1 a brief introduction about of main contribution is presented, Section 2 describes with detail the literature review analyzed in the paper, Section 3 shows the Applications with Quantum Computing Algorithms, in Section 4 the Applications with Deep Learning are presented, and the following Section presents the conclusions and future works and finally the conclusions in the last section are included.
2 Literature review
In this section, we made an exhaustive review about the topics described above. In this case, the consulted papers were collected from the Scopus database. Also, we made a search in Web of Science (WoS) to analyze the published journals papers with these two areas. In WoS, we made queries with the topic ’quantum computing’. With this query, were obtained as result from WoS 33,230 journal papers. However, is a big number of works with difficulty for analyzing. But, with this query is possible to understand the areas, quantity of paper by areas, authors and countries developing works with quantum computing as is shown in Figure 2 , where, it can be appreciated the number of papers by areas. The Figure only shows the first 10 areas.
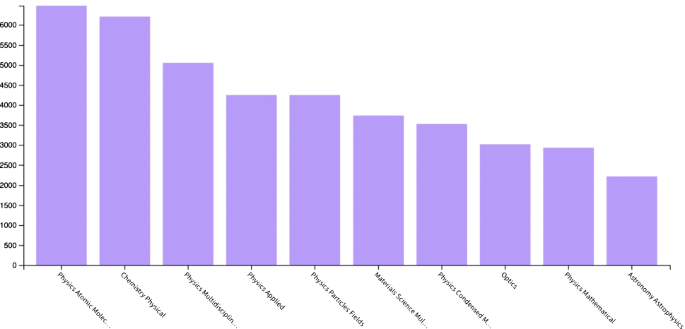
Topic ‘quantum computing’. collected data from WoS
Also, we made a query from WoS search is with the topic ’Deep Learning’, and were found 86,829 papers, that represent a major number of works with respect to QC. But, this is because the search was very general. Therefore, in the other section, we made specific queries to filter and refine in the best way this data. Figure 3 , shows the areas with the higher number of published journal papers, where we can highlight the number of works in the area Engineering Electrical Electronic with more than 20,000 papers. Also, this figure only shows the first ten areas.
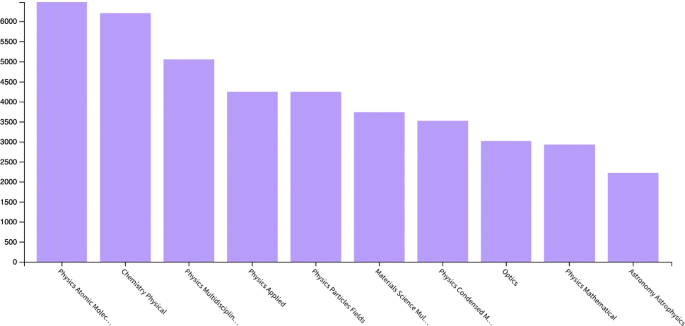
Topic ‘deep learning’. collected data from WoS
In this part, we presented related works about these two topics (QC and DL) algorithms. The source of collected data were reviewed in Scopus database. The search was made with the topic ’Quantum Computing Algorithms’, the obtained results were 5785 papers in total. However, we are presenting a brief description only of the most relevant and recent works in this area. But, with the topic search above described is possible to find at any time the updated documents. Also, we presented a review about applications using Quantum Computing Algorithms. In this case, we decide to include applications based in Computational Intelligence, such has neural networks, fuzzy logic, intelligent control, robotic, medicine, etc.
Before doing the separate queries, with the topics of QC and DL. A query was made in the Scopus database with the two topics together; this was, to know the authors who in the last 10 years have been working in this field of computational intelligence. Figure 4 shows the result of this query.
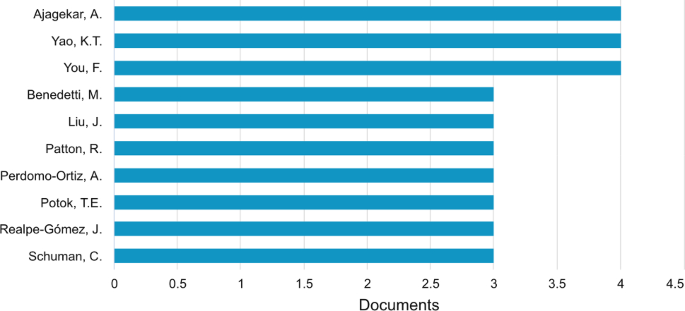
Scopus authors working with QC and DL algorithms
From Scopus, with the topic QC and DL we made a query to know the classification of works according to type of document. In Figure 5 it can be appreciated, the types of work carried out in the last 10 years. In Figure 6 it can be noted in that the numbers of papers developed to date is increasing every day.
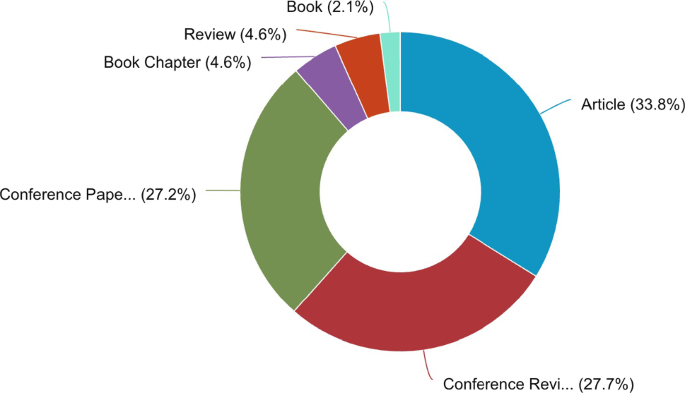
Document types from Scopus database
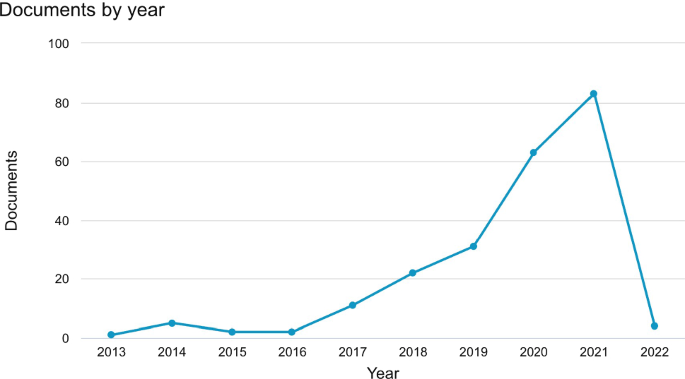
Documents from Scopus database in the last 10 years
In a recent work, Pathak et al. ( 2022 ), presented an Algorithm of Quantum Computing During Pandemic Situation of COVID-19. Further, this work presents fundamental about quantum properties such as superposition, entanglement, and quantum programming tools such as Qiskit (IBM), pyQuil (Google), etc. In Potempa and Porebski ( 2022 ) the authors Comparing Concepts of Quantum and Classical Neural Network Models for Image Classification Task. The comparative results of two models: classical and quantum neural networks of a similar number of training parameters, indicate that the quantum network, although its simulation is time-consuming, overcomes the classical network it has better convergence and achieves higher training and testing accuracy. On the other hand, an interesting work was proposed for Enhancing Security Using Quantum Computing. Were, the authors, developed quantum algorithms using qubit that run faster than classical algorithms and these algorithms reduce the time complexity and also it is impossible for the attackers to attack Peelam and Johari ( 2022 ), with this work is highlighting the importance for using quantum algorithms in security problems in the last years. Other use of QC can be seen in Ong and Tan ( 2022 ), where the authors proposed a work using a quantum circuit program generation with a genetic algorithm for the Open Quantum Assembly Language. In other research, was proposed a Supervised Machine Learning Strategies for Investigation of Weird Pattern Formulation from Large Volume Data Using Quantum Computing, in this work, Quantum machine learning accelerated the supervised, unsupervised, and reinforcement learning methods obtained better results than the classical machi Nivelkar and Bhirud ( 2022 ).
In Xiao et al. ( 2021 ) was proposed a stochastic quantum program synthesis framework based on Bayesian optimization, where Quantum computers and algorithms offered an exponential performance improvement over some NP-complete programs which cannot be run efficiently through a Von Neumann computing approach. On the other hand, a classical simulation of the Quantum Approximate Optimization Algorithm was presented, in this work Medvidović and Carleo ( 2021 ), was developed A neural-network of the many qubit wave function, focusing on states relevant for the Quantum Approximate Optimization Algorithm. A practical application can be seen in Dalyac et al. ( 2021 ) where CA was used for hard industrial optimization problems. The case study in the field of smart-charging of electric vehicles. Also, an important application in the cryptography area was presented in Gaj ( 2018 ).
Other important and recent works can be seen in Singh et al. ( 2021a ), Wang et al. ( 2021 ), Yunakovsky et al. ( 2021 ), Gao et al. ( 2021 ), Huber et al. ( 2021 ), Kulkarni et al. ( 2021 ), Ajagekar and You ( 2021 ), Alberts et al. ( 2021 ), Medvidović and Carleo ( 2021 ), Singh et al. ( 2021b ), Im et al. ( 2021 ) Liu et al. ( 2021 ).
3 Applications with quantum computing algorithms
In this section, we presented the most relevant applications in Medicine, Intelligent Control and Robotic. In each area, we made a brief description about the analyzed works. Also, in this part, we are presenting the most cited paper in each field. Table 2 shows the 10 most cited works in the medicine area using QC.
In Table 3 , the 10 most cited works in the area of intelligent control with QC is presented. In this table is shown the difference with respect to the medicine area, where the number of citations is bigger than intelligent control.
Finally, in Table 4 is shown the most cited works in robotic using QC. Here, it is shown that the number of citations is greater than the intelligent control topic, but less than that of medicine.
3.1 Medicine quantum computing
In this section, a review with QA algorithms applied to medicine are shown. We used the tool VosViewer Perianes-Rodriguez et al. ( 2016 ) to appreciate the formed networks and relations in medicine. From Scopus database, was made a query to calculate the network, relations, clusters with the topic’Medicine Quantum Computing’. We found 114 linked papers considering title, abstract and keyword. The collected data from Scopus, were used in VosViewer to obtain in graph form the obtained results. First, we create a map based on bibliographic data from Scopus.
The data were introduced in a csv format. The type of analysis was by Co-occurrence, the counting method was full counting. Finally, the measure unit used was by keywords with a minimum number of occurrences equal to 2. Where, we obtained 1675 keywords, which 292 meet the threshold. For each of the 292 keywords, the total strength of the co-occurrence links with other keywords was calculated. The keywords with the greatest total link strength were selected. In total 292 keywords were selected to obtain the final results. In Fig. 8 , is shown the network, relations, clusters and links in a form graph of this query using data from Scopus. Figure 7 shows the citations in the last years. In this figure, we can appreciate how the number of citations has increased significantly each year (Fig. 8 ).
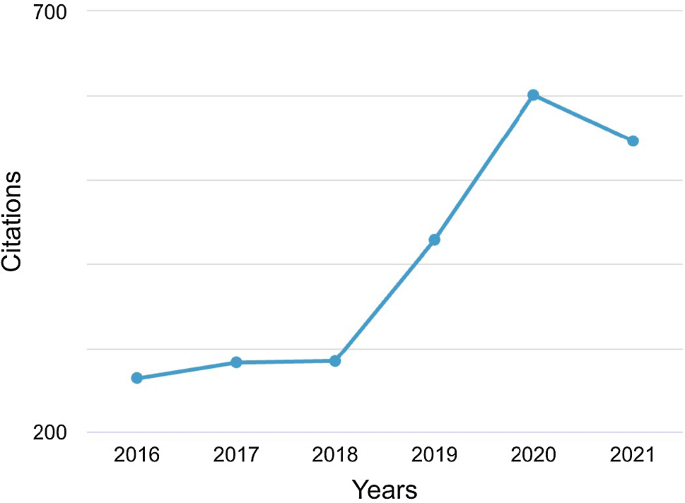
Scopus citations with the topic medicine quantum computing
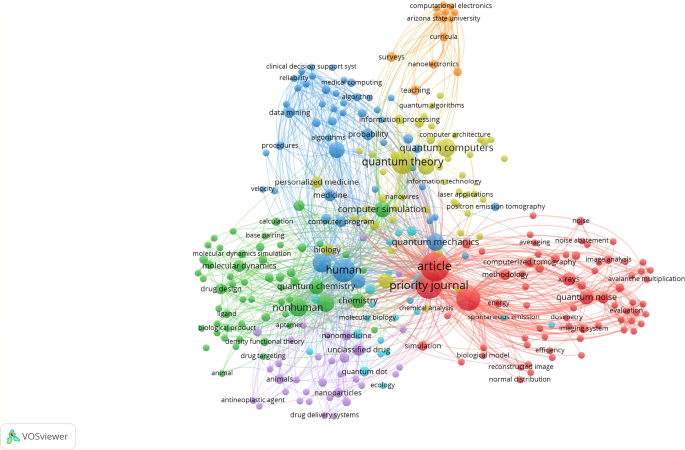
Network with the topic medicine quantum computing
Also, in this section, are presented a brief description about relevant works with the analyzed topic.
In Boev et al. ( 2021 ), the authors developed a method for solving genome assembly tasks with the use of quantum and quantum-inspired optimization techniques. Within this method, we present experimental results on genome assembly using quantum annealers both for simulated data and the φ X 174 bacteriophages. The results pave a way for a significant increase in the efficiency of solving bioinformatics problems with the use of quantum computing technologies.
Also, in Thomasian and Adashi ( 2021 ), a qualitative review of the medical cybersecurity literature was presented with collation of federal and international legal documents, policy reports, industry frameworks, cyberbreach analyses, and scientific journal papers. On the other hand, fuzzy logic with quantum computing was used in Kumar et al. ( 2020 ), in this the authors proposed a health analytics system by forming a knowledge repository of patient’s symptoms and medicines dosage to prescribe the precise quantum of medicine to cure an ailment and also prevent drug abuse. Also, in this field, Bianconi and Mohseni ( 2020 ), an Infrared detection and imaging are key enabling technologies for a vast number of applications, ranging from communication, to medicine and astronomy, were proposed using quantum computing. In big data applied to medicine have been developed some works with QC, for example, in the healthcare industry, various sources for big data include hospital records, medical records of patients, results of medical examinations, and devices that are a part of internet of things. The authors used QC to manage this quantity of data Dash et al. ( 2019 ).
3.2 Intelligent control quantum computing applications
In the same way that the previous topic, in this section, a review with QC algorithms applied to intelligent control are presented. Also, was used the tool VosViewer Perianes-Rodriguez et al. ( 2016 ) to distinguish the formed networks and relations in intelligent control. From Scopus database, was made a query to calculate the network, relations, clusters with the topic ‘Intelligent Control Quantum Computing’. We found 141 linked papers considering title, abstract and keyword. The collected data from Scopus, were used in VosViewer to obtain in graph form the obtained results. First, we create a map based on bibliographic data from Scopus. The data were introduced in a csv format. The type of analysis was by Co-occurrence, the counting method was full counting. Finally, the measure unit used was by keywords with a minimum number of occurrences equal to 2. Where, we obtained 1329 keywords, which 301 meet the threshold. For each of the 301 keywords, the total strength of the co-occurrence links with other keywords was calculated. The keywords with the greatest total link strength were selected. In total 301 keywords were selected to obtain the presented results. In Fig. 9 , is shown the network, relations, clusters and links in a form graph of this query using data from Scopus. Figure 10 shows the density of this network, highlighting with yellow color the area of quantum computing and intelligent control with more strength that other analyzed keywords. Figure 11 shows the citations in the last years from Scopus. In this figure, we can appreciate how the number of citations has increased significantly each year. Also, in this section, are presented a brief description about relevant works with the analyzed topic. In Guan et al. ( 2020 ) proposed new Lyapunov control scheme for quantum systems using a Particle Swarm Optimization algorithm. Also, in Barchatova et al. ( 2015 ) was proposed an Intelligent robust control system based on quantum KB-selforganization. The authors, considered Quantum soft computing and Kansei/affective engineering technologies in this research. On the other hand, Mohanty and Rout ( 2015 ) presented a motion control method for mobile robots in indoor environments based on color object detection using quantum computing. Also, Han and Yuan ( 2014 ), proposed a Multivariable system identification based on double quantum particle swarm optimization and big data, in this case, the authors used an optimization method combined with quantum computing. Finally, in this section, we presented the application of an adaptive quantum particle swarm optimization algorithm for optimal dispatching of cascaded hydro power stations proposed by Zhang et al. ( 2012 )
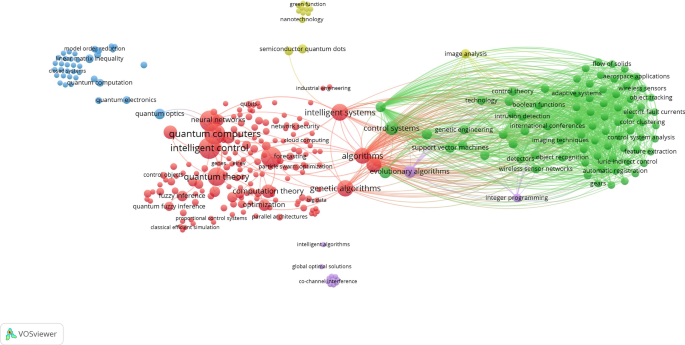
Network with the topic intelligent control quantum computing
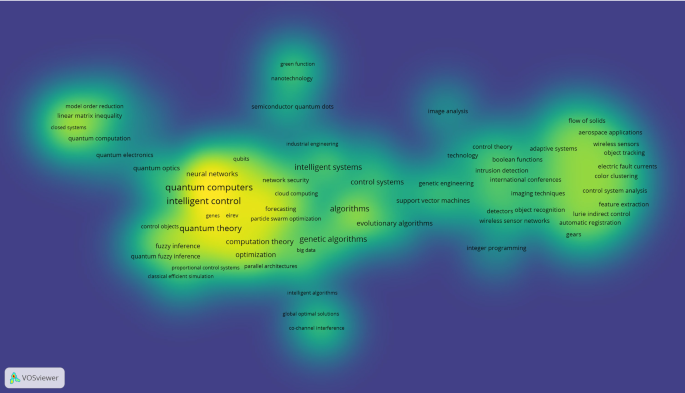
Density with the topic intelligent control quantum computing
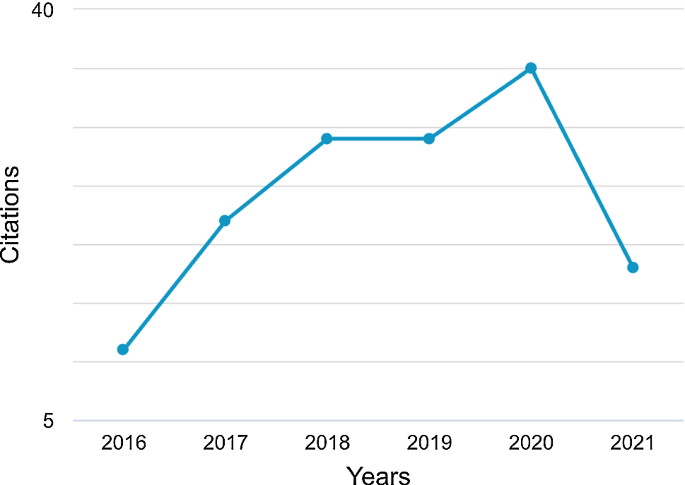
Citations from Scopus Scopus citations with the topic ‘Intelligent Control Quantum Computing’
3.3 Robotic control quantum computing applications
Finally, in this section, a review with QC algorithms applied to Robotic are presented. Also, was used the tool VosViewer Perianes-Rodriguez et al. ( 2016 ) to distinguish the formed networks and relations in Robotic. From Scopus database, was made a query to calculate the network, relations, clusters with the topic’Robotic Control Quantum Computing’. We found 93 linked papers considering title, abstract and keyword. The collected data from Scopus, were used in VosViewer to obtain in graph form the obtained results. First, we create a map based on bibliographic data from Scopus. The data were introduced in a csv format. The type of analysis was by Co-occurrence, the counting method was full counting. Finally, the measure unit used was by keywords with a minimum number of occurrences equal to 2. Where, we obtained 1013 keywords, which 197 meet the threshold. For each of the 197 keywords, the total strength of the co-occurrence links with other keywords was calculated. The keywords with the greatest total link strength were selected. In total, 197 keywords were selected to obtain the final results. In Fig. 12 , is shown the network, relations, clusters and links in a form graph of this query using data from Scopus. Figure 13 shows the overlay visualization to appreciate clearly how are distributed each one the keywords. In Fig. 14 the density of this network is presented, highlighting with yellow color the area of quantum computing and robotic with more strength that other analyzed keywords. Figure 15 shows the citations in the last years from Scopus. In this figure, we can appreciate how the number of citations has increased significantly each year.
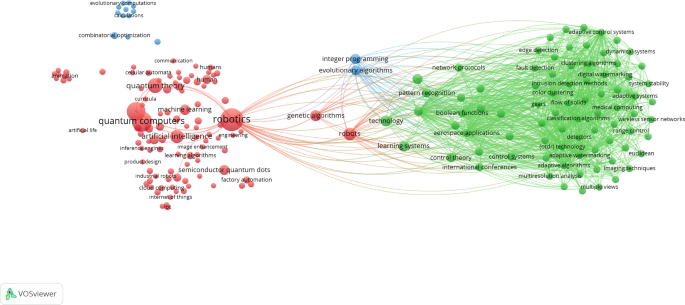
Network with the topic robotic quantum computing
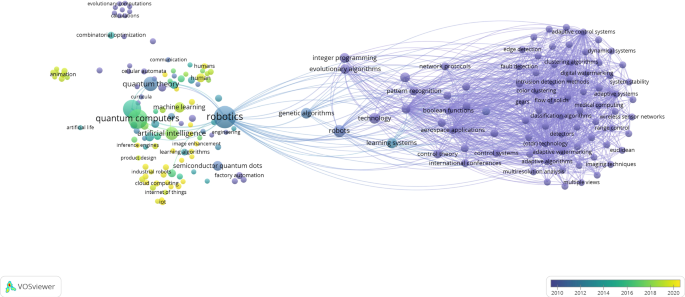
Overlay with the topic robotic quantum computing
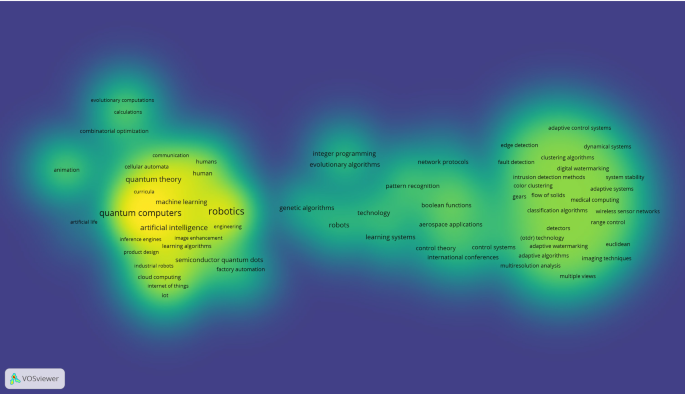
Density with the topic robotic quantum computing
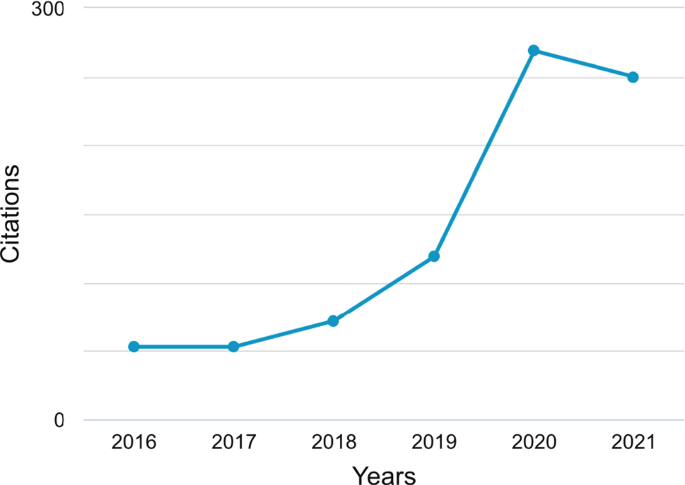
Scopus citations with the topic’Robotic Quantum Computing’
Also, in this section are presented some important applications with robotic using QC. Atchade-Adelomou et al. ( 2021 ) proposed a quantum computing approach in mobile robot order picking and batching problem solver optimization, the authors developed a quantum algorithm to minimize the distance traveled in warehouses and distribution centers where order picking is applied. Also, in this field A New Quantum-computing based Algorithm for Robotic Arms and Rigid Bodies’ Orientation was proposed by Zioui et al. ( 2021 ). Also, in Post Quantum Secure Command and Control of Mobile Agents Inserting quantum-resistant encryption schemes in the Secure Robot Operating System Varma et al. ( 2020 ). On the other hand, in Korenkov et al. ( 2020 ) quantum software engineering supremacy in Intelligent robotics was developed. Also, in Ulyanov ( 2020 ) Quantum fuzzy inference based on quantum genetic algorithm: Quantum simulator in intelligent robotics.
4 Applications with deep learning algorithms
In this section, we presented a review about applications using Deep Learning Algorithms. In this case, we decide to include applications based in Computational Intelligence, such has neural networks, fuzzy logic, etc. Also, in this section, we are presenting the most cited paper in each field. Table 5 shows the 10 most cited works in the medicine area using DL. Here, is observed how in the last years, the number of citations is increasing in this field.
In Table 6 , are presented the most cited works in intelligent control with DL. Also, is shown as in the last years this topic is highly used by the researchers.
Finally, in Table 7 , are presented the most cited works in robotic with DL. Also, in this topic the number of citations is increasing in the last years.
4.1 Medicine deep learning applications
Also, in this section, a review with DL algorithms applied to Medicine are presented. Also, was used the tool VosViewer Perianes-Rodriguez et al. ( 2016 ) to distinguish the formed networks and relations in medicine. From Scopus database, was made a query to calculate the network, relations, clusters with the topic’Robotic Control Quantum Computing’. We found 1011 linked papers considering title, abstract and keyword. The collected data from Scopus, were used in VosViewer to obtain in graph form the obtained results. First, we create a map based on bibliographic data from Scopus. The data were introduced in a csv format. The type of analysis was by Co-occurrence, the counting method was full counting. Finally, the measure unit used was by keywords with a minimum number of occurrences equal to 2. Where, we obtained 7782 keywords, which 2355 meet the threshold. For each of the 2355 keywords, the total strength of the co-occurrence links with other keywords was calculated. The keywords with the greatest total link strength were selected. In total 1000 keywords were selected to obtain the final results. In Fig. 16 , is shown the network, relations, clusters and links in a form graph of this query using data from Scopus. In Fig. 17 the density of this network is presented, highlighting with yellow color the area of deep learning and human with more strength that other analyzed keywords. Figure 18 shows the citations in the last years from Scopus. In this figure, we can appreciate how the number of citations has increased significantly each year. Also, in this section are presented some applications with deep learning techniques as, neural networks, machine learning, convolutional neural networks, etc., applied to medicine.
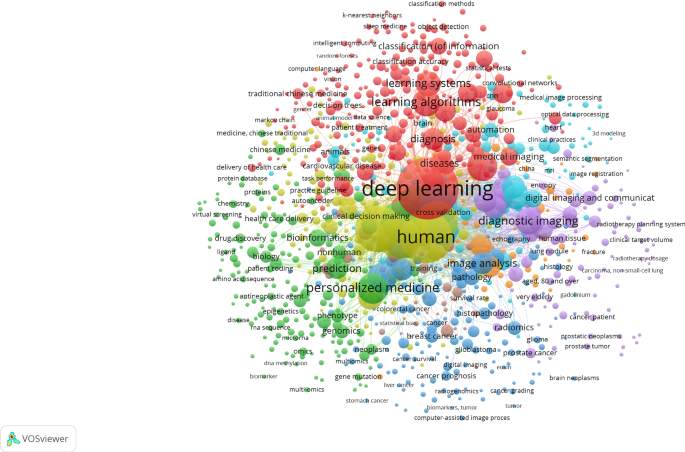
Network with the topic medicine deep learning algorithms
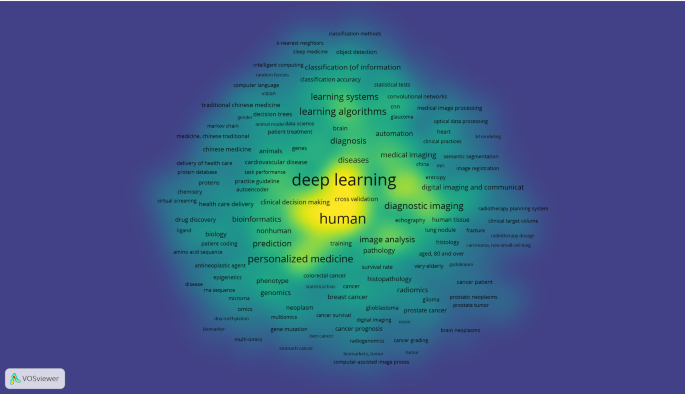
Density with the topic medicine deep learning algorithms
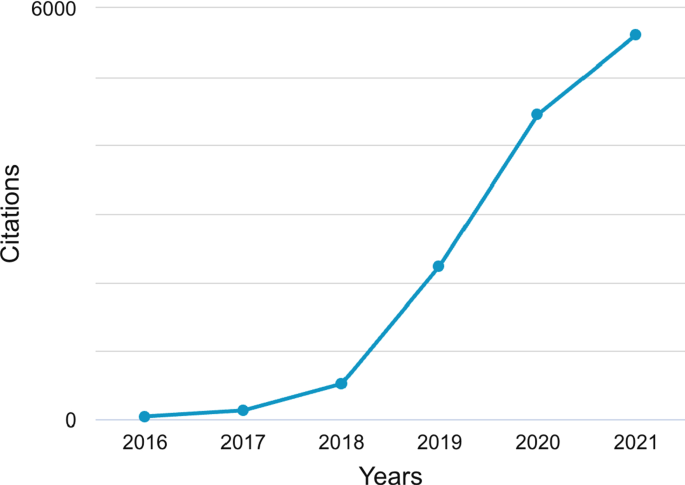
Scopus citations with the topic ‘Medicine Deep Learning Algorithms’
Nowadays, several web medical applications evolved in the field of medicine, there is need for an intelligent and efficient extraction technique. Therefore, neural networks, machine learning can be used to improve the obtained results with other classical techniques. For example, in Deepika and Radha ( 2022 ) was presented a study of Abstract-Based Classification of Medical Journals Using Machine Learning Techniques. Other relevant application using deep learning is presented in Gaxiola et al. ( 2018 ), in this work, the authors used modular neural networks for iris recognition. The authors used the human iris database improved with image preprocessing methods. Also, a recent work in the medical area with deep learning techniques is presented by Varela-Santos and Melin ( 2021 ). In this case, the authors used neural networks for classifying coronavirus based on its manifestation on chest X-rays using texture features. In González et al. ( 2015 ), Fuzzy logic with the optimization method gravitational search was used to optimize the architecture of a modular neural networks in echocardiogram recognition. In the same way, the authors presented a work but using type-2 fuzzy logic with pattern recognition with modular neural networks González et al. ( 2016 ). On the other hand, an overview of deep learning in medical imaging was presented by Lundervold and Lundervold ( 2019 ). In this work, the authors review the deep artificial neural networks, machine learning models to image analysis to natural language processing.
4.2 Intelligent control deep learning application
Also, in this section, a review with DL algorithms applied to Medicine are presented. Also, was used the tool VosViewer Perianes-Rodriguez et al. ( 2016 ) to distinguish the formed networks and relations in medicine. From Scopus database, was made a query to calculate the network, relations, clusters with the topic’Robotic Control Quantum Computing’. We found 1160 linked papers considering title, abstract and keyword. The collected data from Scopus, were used in VosViewer to obtain in graph form the obtained results. First, we create a map based on bibliographic data from Scopus. The data were introduced in a csv format. The type of analysis was by Co-occurrence, the counting method was full counting. Finally, the measure unit used was by keywords with a minimum number of occurrences equal to 2. Where, we obtained 8721 keywords, which 2131 meet the threshold. For each of the 2131 keywords, the total strength of the co-occurrence links with other keywords was calculated. The keywords with the greatest total link strength were selected. In total 1000 keywords were selected to obtain the final results. In Fig. 19 , is shown the network, relations, clusters and links in a form graph of this query using data from Scopus. In Fig. 20 the density of this network is presented, highlighting with yellow color the area of intelligent control and deep learning with more strength that other analyzed keywords. Figure 21 shows the citations in the last years from Scopus. In this figure, we can appreciate how the number of citations has increased significantly each year.
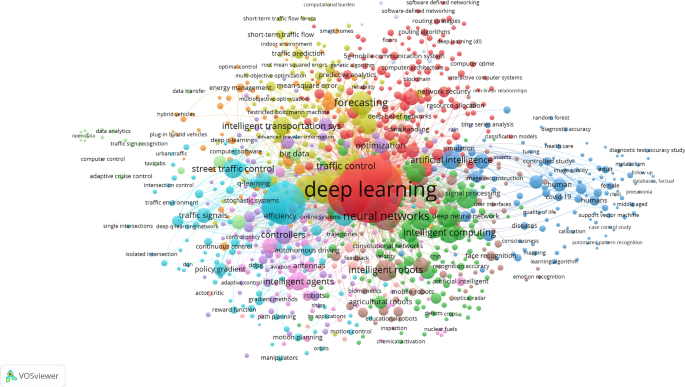
Network with the topic ‘intelligent control deep learning algorithms’
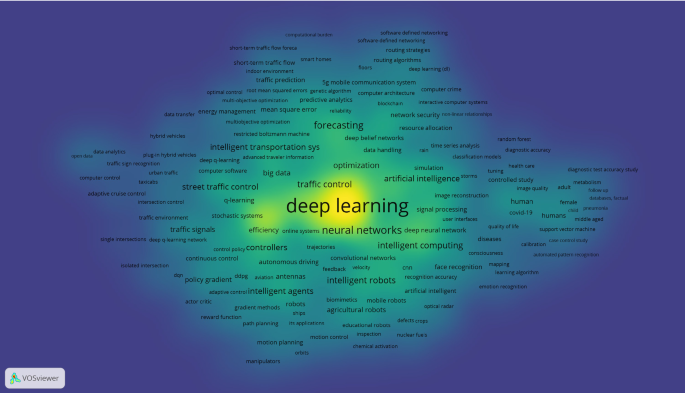
Density with the topic ‘intelligent control deep learning algorithms’
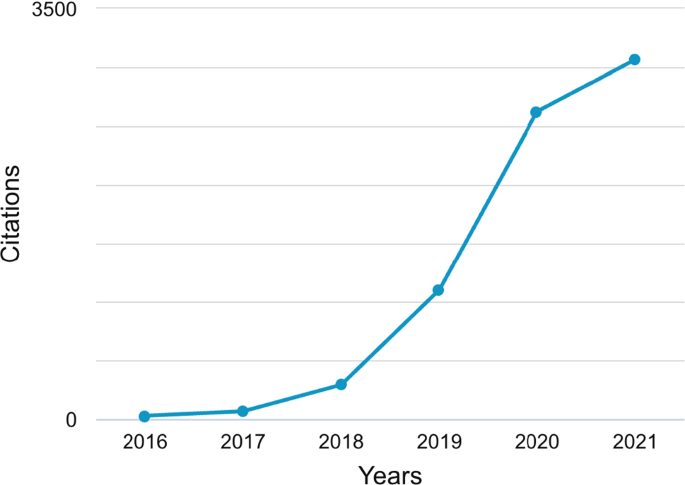
Scopus citations with the topic ‘Intelligent Control Deep Learning Algorithms’
4.3 Robotic deep learning applications
Also, in this section, a review with DL algorithms applied to Medicine are presented. Also, was used the tool VosViewer Perianes-Rodriguez et al. ( 2016 ) to distinguish the formed networks and relations in medicine. From Scopus database, was made a query to calculate the network, relations, clusters with the topic’Robotic Control Quantum Computing’. We found 516 linked papers considering title, abstract and keyword. The collected data from Scopus, were used in VosViewer to obtain in graph form the obtained results. First, we create a map based on bibliographic data from Scopus. The data were introduced in a csv format. The type of analysis was by Co-occurrence, the counting method was full counting. Finally, the measure unit used was by keywords with a minimum number of occurrences equal to 2. Where, we obtained 4010 keywords, which 990 meet the threshold. For each of the 990 keywords, the total strength of the co-occurrence links with other keywords was calculated. The keywords with the greatest total link strength were selected. In total, 990 keywords were selected to obtain the final results. In Fig. 22 , is shown the network, relations, clusters and links in a form graph of this query using data from Scopus. In Fig. 23 the density of this network is presented, highlighting with yellow color the area of deep learning and learning algorithms with more strength that other analyzed keywords. Figure 24 shows the citations in the last years from Scopus. In this figure, we can appreciate how the number of citations has increased significantly each year.
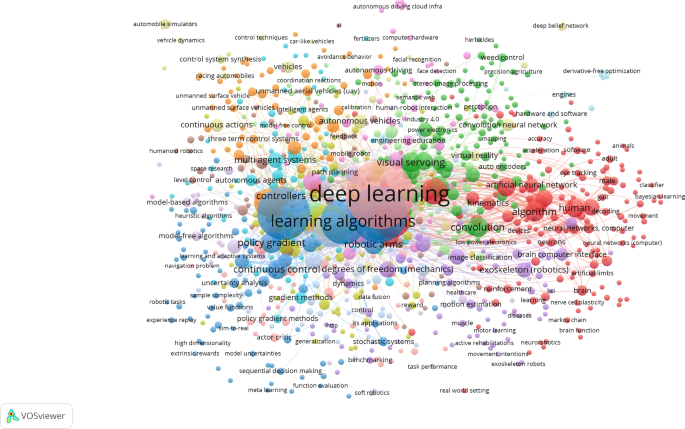
Network with the topic ‘robotic deep learning algorithms’
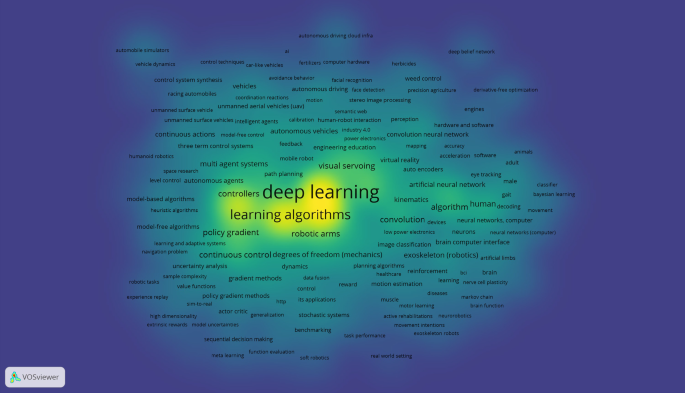
Density with the topic ‘robotic deep learning algorithms’
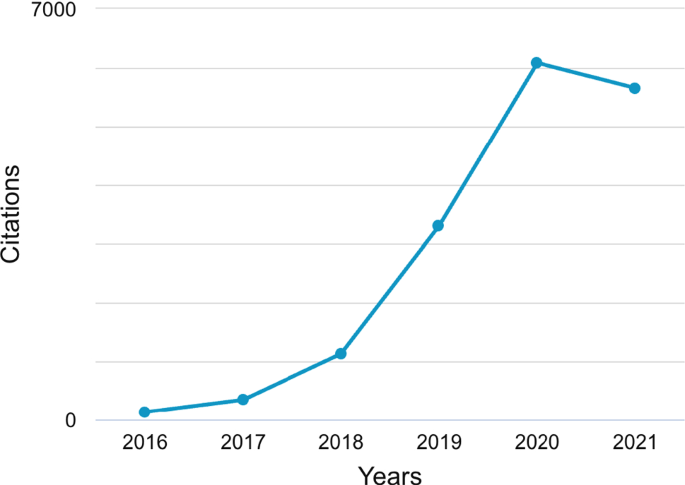
Scopus citations with the topic ‘Robotic Deep Learning Algorithms’
In this field, we can see many works applying robotics with deep learning methods. Therefore, in this section are presented the most relevant and recent in this area. For example, in Wu et al. ( 2019 ) implemented a robot to make an automatic choreography system based on the deep learning technology. On the other hand, King and Hwang ( 1989 ). The authors proposed a ring VLSI systolic architecture for implementing neural networks with applications to robotic processing. Also, Liao et al. ( 2021 ) proposed an unsupervised fault detection and recovery for intelligent robotic rollators using deep neural networks. The experiments under several conditions confirmed that the method, which leverages machine learning-enhanced algorithms, exhibits reliable performance. Also, Chen et al. ( 2020 ) proposed a robotic device capable of introducing needles and catheters into deformable tissues such as blood vessels to draw blood or deliver fluids autonomously. Robotic cannulation is driven by predictions from a series of deep convolutional neural networks that encode information from multimodal image sequences to guide real-time serving. Also, in Alzubaidi et al. ( 2021 ) was made a review about applications in robotic with deep learning, convolutional neural networks architectures, challenges, etc. In this study, the authors attempt to provide a more comprehensive survey of the most important aspects of Deep Learning and including those enhancements recently added to the field.
5 Conclusions
After performing an analysis from Scopus, an undertaking an exhaustive study about the applications and importance of Quantum Computing and Deep Learning; we can conclude that these areas have achieved an important advancement in the last years. We can observe, the increment of number of citations, for example, in Scopus, the tendency is developed every day more works based on these two fields of the computational intelligence. Also, we have found, the networks, clusters, and built relationships around the world by many researches that are working with the analyzed topics. As future works, other topics of WoS, Google Scholar, or other databases can be analyzed with this software to compare the number of citations or relevance considering other sources. Also, specific queries with other bibliometric software such as CiteSpace, Zhang et al. ( 2010 ), that is a free software for visualizing and analyzing trends and patterns in the scientific literature can be used. The relevance of the review is that with this paper, the researchers can observe the trends for using QC and DL in several areas. However, the paper can serve as reference to make other queries, with other topics from different databases. Also, the same searches can be explored to view the updated information about the analyzed topics in this review. Although, only were reviewed medicine, robotic and intelligent control to make the research, the paper can be extended with other important areas. We decided to explore these areas because we have some works in computational intelligence with these topics.
Data availability
Not applicable
Ajagekar A, You F (2021) Quantum computing based hybrid deep learning for fault diagnosis in electrical power systems. Scopus. https://doi.org/10.1016/j.apenergy.2021.117628
Article Google Scholar
Alberts GJN, Rol MA, Last T, Broer BW, Bultink CC, Rijlaarsdam MSC, Hauwermeiren AEV (2021) Accelerating quantum computer developments. Scopus. https://doi.org/10.1140/epjqt/s40507-021-00107-w
Alzubaidi L, Zhang J, Humaidi AJ, Al-Dujaili A, Duan Y, Al-Shamma O, Santamaría J, Fadhel MA, Al-Amidie M, Farhan L (2021) Review of deep learning: concepts, CNN architectures, challenges, applications, future directions. Scopus. https://doi.org/10.1186/s40537-021-00444-8
Ankita S, Shikha A, Jitendra A, Sanjeev S (2013) A review on application of particle swarm optimization in association rule mining. Scopus 199:405–414. https://doi.org/10.1007/978-3-642-35314-7_46
Arulkumaran K, Deisenroth MP, Brundage M, Bharath AA (2017) Deep reinforcement learning: a brief survey. Scopus 34(6):26–38. https://doi.org/10.1109/MSP.2017.2743240
Atchade-Adelomou P, Alonso-Linaje G, Albo-Canals J, Casado-Fauli D (2021) Qrobot: a quantum computing approach in mobile robot order picking and batching problem solver optimization. Scopus. https://doi.org/10.3390/a14070194
Barchatova IA, Ulyanov SV, Albu VA (2015) Intelligent robust control system based on quantum KB-self-organization: quantum soft computing and Kansei/affective engineering technologies. Scopus 323:37–48. https://doi.org/10.1007/978-3-319-11310-4_4
Article MATH Google Scholar
Baskaran A, Balaji N, Basha S, Vengattaraman T (2015) A survey of nature inspired algorithms. Int J Appl Eng Res 10:19313–19324
Google Scholar
Beer K, Bondarenko D, Farrelly T, Osborne T, Salzmann R, Scheiermann D, Wolf R (2020) Training deep quantum neural networks. Nat Commun 11:808. https://doi.org/10.1038/s41467-020-14454-2
Bianconi S, Mohseni H (2020) Recent advances in infrared imagers: toward thermodynamic and quantum limits of photon sensitivity. Scopus. https://doi.org/10.1088/1361-6633/ab72e5
Boev AS, Rakitko AS, Usmanov SR (2021) Genome assembly using quantum and quantum-inspired annealing. Sci Rep 11:13183–13183
Chen AI, Balter ML, Maguire TJ (2020) Deep learning robotic guidance for autonomous vascular access. Nat Mach Intell 2:104–115
Cheng L, Wang C, Feng L, Yang K, Liu Z (2014) Functional nanomaterials for phototherapies of cancer. Chem Rev. https://doi.org/10.1021/cr400532z
Ching T, Himmelstein DS, Beaulieu-Jones BK, Kalinin AA, Do BT, Way GP, Ferrero E, Agapow PM, Zietz M, Hoffman MM, Xie W, Rosen GL, Lengerich BJ, Israeli J, Lanchantin J, Woloszynek S, Carpenter AE, Shrikumar A, Xu J, Cofer EM, Lavender CA, Turaga SC, Alexandari AM, Lu Z, Harris DJ, Decaprio D, Qi Y, Kundaje A, Peng Y, Wiley LK, Segler MHS, Boca SM, Swamidass SJ, Huang A, Gitter A, Greene CS (2018) Opportunities and obstacles for deep learning in biology and medicine. Scopus. https://doi.org/10.1098/rsif.2017.0387
Cully A, Clune J, Tarapore D, Mouret JB (2015) Robots that can adapt like animals. Scopus 521(7553):503–507. https://doi.org/10.1038/nature14422
Dalyac C, Henriet L, Jeandel E, Lechner W, Perdrix S, Porcheron M, Veshchezerova M (2021) Qualifying quantum approaches for hard industrial optimization problems. A case study in the field of smart-charging of electric vehicles. Scopus. https://doi.org/10.1140/epjqt/s40507-021-00100-3
Das R, Baker D (2008) Macromolecular modeling with Rosetta. Ann Rev Biochem 77:363–382. https://doi.org/10.1146/annurev.biochem.77.062906.171838
Dash S, Shakyawar SK, Sharma M, Kaushik S (2019) Big data in healthcare: management, analysis and future prospects. Scopus. https://doi.org/10.1186/s40537-019-0217-0
Deepika A, Radha N (2022) Performance analysis of abstract-based classification of medical journals using machine learning techniques. Scopus 75:613–626. https://doi.org/10.1007/978-981-16-3728-5_47
Dong D, Chen C, Zhang C, Chen Z (2006) Quantum robot: structure, algorithms and applications. Scopus 24(4):513–521. https://doi.org/10.1017/S0263574705002596
Fadlullah ZM, Tang F, Mao B, Kato N, Akashi O, Inoue T, Mizutani K (2017) State-of-the-art deep learning: evolving machine intelligence to-ward tomorrow’s intelligent network traffic control systems. Scopus 19(4):2432–2455. https://doi.org/10.1109/COMST.2017.2707140
Gaj K (2018) Challenges and rewards of implementing and benchmarking post-Quantum cryptography in hardware. doi: https://doi.org/10.1145/3194554.3194615
Gao H, Zhang S, Du Y, Wang Y, Diao M (2017) Relay selection scheme based on quantum differential evolution algorithm in relay networks. Scopus 11(7):3501–3523. https://doi.org/10.3837/tiis.2017.07.011
Gao Q, Jones GO, Motta M, Sugawara M, Watanabe HC, Kobayashi T, Watanabe E, Ohnishi YY, Nakamura H, Yamamoto N (2021) Applications of quantum computing for investigations of electronic transitions in phenylsulfonyl-carbazole TADF emitters. Scopus. https://doi.org/10.1038/s41524-021-00540-6
Gaxiola F, Melin P, Valdez F, Castro JR (2018) Person recognition with modular deep neural network using the Iris biometric measure. doi: https://doi.org/10.1007/978-3-319-71008-2_6
Giusti A, Guzzi J, Ciresan DC, He FL, Rodriguez JP, Fontana F, Faessler M, Forster C, Schmidhuber J, Caro GD, Scaramuzza D, Gambardella LM (2016) A machine learning approach to visual perception of forest trails for mobile robots. Scopus 1(2):661–667. https://doi.org/10.1109/LRA.2015.2509024
González B, Valdez F, Melin P, Prado-Arechiga G (2015) Fuzzy logic in the gravitational search algorithm enhanced using fuzzy logic with dynamic alpha parameter value adaptation for the optimization of modular neural networks in echocardiogram recognition. Appl Soft Comput 37:245–254. https://doi.org/10.1016/j.asoc.2015.08.034
González B, Melin P, Valdez F, Prado-Arechiga G (2016) Interval type-2 fuzzy logic gravitational search algorithm for the optimization of modular neural networks in echocardiogram recognition. In: Proceedings of the 2016 {IEEE} symposium series on computational intelligence, {SSCI} 2016, Athens, Greece, December 6–9, 2016, IEEE, pp 1–7, doi: https://doi.org/10.1109/SSCI.2016.7850134
Gu S, Holly E, Lillicrap T, Levine S (2017) Deep reinforcement learning for robotic manipulation with asynchronous off-policy updates. doi: https://doi.org/10.1109/ICRA.2017.7989385
Guan X, Kuang S, Dong D (2020) PSO-assisted Lyapunov control design for quantum systems. doi: https://doi.org/10.1109/SSCI47803.2020.9308347
Häffner H, Roos CF, Blatt R (2008) Quantum computing with trapped ions. Phys Rep 469(4):155–203. https://doi.org/10.1016/j.physrep.2008.09
Article MathSciNet Google Scholar
Hamet P, Tremblay J (2017) Artificial intelligence in medicine. Metabolism 69S:S36–S40. https://doi.org/10.1016/j.metabol.2017.01.011
Hammernik K, Klatzer T, Kobler E, Recht MP, Sodickson DK, Pock T, Knoll F (2018) Learning a variational network for reconstruction of accelerated MRI data. Scopus 79(6):3055–3071. https://doi.org/10.1002/mrm.26977
Han P, Yuan S (2014) Multivariable system identification based on double quantum particle swarm optimization and big data. Scopus 34(32):5779–5787. https://doi.org/10.13334/j.0258-8013.pcsee.2014.32.012
Hu C, Wu Q, Li H, Jian S, Li N, Lou Z (2018) Deep learning with a long short-term memory networks approach for rainfall-runoff simulation. Scopus. https://doi.org/10.3390/w10111543
Huang Y, Tang C, Wang S (2007) Quantum-inspired swarm evolution algorithm
Huber SP, Bosoni E, Bercx M, Bröder J, Degomme A, Dikan V, Eimre K, Flage-Larsen E, Garcia A, Genovese L, Gresch D, Johnston C, Petretto G, Poncé S, Rignanese GM, Sewell CJ, Smit B, Tseplyaev V, Uhrin M, Wortmann D, Yakutovich AV, Zadoks A, Zarabadi-Poor P, Zhu B, Marzari N, Pizzi G (2021) Common workflows for computing material properties using different quantum engines. Scopus. https://doi.org/10.1038/s41524-021-00594-6
Im DG, Lee CH, Kim Y, Nha H, Kim MS, Lee SW, Kim YH (2021) Optimal teleportation via noisy quantum channels without additional qubit resources. Scopus. https://doi.org/10.1038/s41534-021-00426-x
Jiang F, Jiang Y, Zhi H, Dong Y, Li H, Ma S, Wang Y, Dong Q, Shen H, Wang Y (2017) Artificial intelligence in healthcare: past, present and future. Stroke Vasc Neurol 2(4):230–243. https://doi.org/10.1136/svn-2017-000101
Johnson KW, Soto JT, Glicksberg BS, Shameer K, Miotto R, Ali M, Ashley E, Dudley JT (2018) Artificial intelligence in cardiology. Scopus 71(23):2668–2679. https://doi.org/10.1016/j.jacc.2018.03.521
Kasabov N (2007) Evolving connectionist systems: the knowledge engineering approach. doi: https://doi.org/10.1007/978-1-84628-347-5
Kendall A, Cipolla R (2016) Modelling uncertainty in deep learning for camera relocalization. doi: https://doi.org/10.1109/ICRA.2016.7487679
Kim DH, Kim TJY, Wang X, Kim M, Quan YJ, Oh JW, Min SH, Kim H, Bhandari B, Yang I, Ahn SH (2018) Smart machining process using machine learning: a review and perspective on machining industry. Scopus 5(4):555–568. https://doi.org/10.1007/s40684-018-0057-y
King SY, Hwang JN (1989) Neural network architectures for robotic applications. IEEE Trans Robot Autom 5(5):641–657. https://doi.org/10.1109/70.88082
Kok P, Munro WJ, Nemoto K, Ralph TC, Dowling JP, Milburn GJ (2007) Linear optical quantum computing with photonic qubits. Rev Mod Phys 79(1):135–174. https://doi.org/10.1103/RevModPhys.79.135
Korenkov VV, Reshetnikov AG, Ulyanov SV (2020) Quantum software engineering supremacy in intelligent robotics. doi: https://doi.org/10.1109/MoNeTeC49726.2020.9258000
Koza JR (2010) Human-competitive results produced by genetic programming. Scopus 11(3–4):251–284. https://doi.org/10.1007/s10710-010-9112-3
Krittanawong C, Zhang H, Wang Z, Aydar M, Kitai T (2017) Artificial intelligence in precision cardiovascular medicine. Scopus 69(21):2657–2664. https://doi.org/10.1016/j.jacc.2017.03.571
Krizhevsky A, Sutskever I, Hinton GE (2012) ImageNet classification with deep convolutional neural networks. In: Pereira F, Burges CJC, Bottou L, Weinberger KQ (eds) Advances in neural information processing systems, Curran Associates, Inc., vol 25
Kulkarni V, Kulkarni M, Pant A (2021) Quantum computing methods for supervised learning. Scopus. https://doi.org/10.1007/s42484-021-00050-0
Kumar KP, Dhinakaran K, Vinod D (2020) Fuzzy quantum computing model for health analytics. Scopus 10(5):2006–2024
Lang AH, Vora S, Caesar H, Zhou L, Yang J, Beijbom O (2019) Pointpillars: fast encoders for object detection from point clouds. doi: https://doi.org/10.1109/CVPR.2019.01298
Lee JG, Jun S, Cho YW, Lee H, Kim GB, Seo JB, Kim N (2017) Deep learning in medical imaging: general overview. Scopus 18(4):570–584. https://doi.org/10.3348/kjr.2017.18.4.570
Leibfried D, Knill E, Seidelin S, Britton J, Blakestad R, Chiaverini J, Hume D, Itano W, Jost J, Langer C, Ozeri R, Reichle R, Wineland D (2006) Creation of a six-atom ’Schrodinger cat’ state. Nature 438:639–642. https://doi.org/10.1038/nature04251
Li J, Lu Y, Xu Y, Liu C, Tu Y, Ye S, Liu H, Xie Y, Qian H, Zhu X (2018) AIR-Chem: authentic intelligent robotics for chemistry. Scopus 122(46):9142–9148. https://doi.org/10.1021/acs.jpca.8b10680
Liao Y, Yeaser A, Yang B, Tung J, Hashemi E (2021) Unsupervised fault detection and recovery for intelligent robotic rollators. Robot Autonom Syst. https://doi.org/10.1016/j.robot.2021.103876
Limonov M, Rybin M, Poddubny A, Kivshar Y (2017) Fano resonances in photonics. Nat Photon 11:543–554. https://doi.org/10.1038/nphoton.2017.142
Liu CY, Spicer M, Apuzzo MLJ, Kobayashi S, Hongo K, Black PML, Rutka JT, Benabid AL, Kelly PJ, Schramm J (2003) The genesis of neuro-surgery and the evolution of the neurosurgical operative environment: Part II-concepts for future development, 2003 and beyond. Scopus 52(1):20–35. https://doi.org/10.1097/00006123-200301000-00002
Liu W, Zhang Y, Deng Z, Zhao J, Tong L (2021) A hybrid quantum-classical conditional generative adversarial network algorithm for human-centered paradigm in cloud. Scopus. https://doi.org/10.1186/s13638-021-01898-3
Lundervold AS, Lundervold A (2019) An overview of deep learning in medical imaging focusing on MRI. Z Med Phys 29(2):102–127. https://doi.org/10.1016/j.zemedi.2018.11.002
Madjarov IS, Cooper A, Shaw AL, Covey JP, Schkolnik V, Yoon TH, Williams JR, Endres M (2019) An atomic-array optical clock with single-atom readout. Scopus. https://doi.org/10.1103/PhysRevX.9.041052
Mahler J, Liang J, Niyaz S, Laskey M, Doan R, Liu X, Ojea JA, Goldberg K (2017) Dex-Net 2.0: deep learning to plan Robust grasps with synthetic point clouds and analytic grasp metrics. Doi: https://doi.org/10.15607/rss.2017.xiii.058
Mao Q, Hu F, Hao Q (2018) Deep learning for intelligent wireless networks: a comprehensive survey. Scopus 20(4):2595–2621. https://doi.org/10.1109/COMST.2018.2846401
Medvidović M, Carleo G (2021) Classical variational simulation of the quantum approximate optimization algorithm. Scopus. https://doi.org/10.1038/s41534-021-00440-z
Mobadersany P, Yousefi S, Amgad M, Gutman DA, Barnholtz-Sloan JS, Vega JEV, Brat DJ, Cooper LAD (2018) Predicting cancer outcomes from histology and genomics using convolutional networks. Scopus 115(13):2970–2979. https://doi.org/10.1073/pnas.1717139115
Mohanty MN, Rout S (2015) An intelligent method for moving object detection. Scopus 309(2):343–351. https://doi.org/10.1007/978-81-322-2009-1_39
Montiel-Ross OH (2020) A review of quantum-inspired metaheuristics: going from classical computers to real quantum computers. IEEE Access 8:814–838. https://doi.org/10.1109/ACCESS.2019.2962155
Montiel-Ross O, Rubio Y, Olvera C, Rivera A (2019) Quantum-inspired acromyrmex evolutionary algorithm. Sci Rep. https://doi.org/10.1038/s41598-019-48409-5
Nivelkar M, Bhirud SG (2022) Supervised machine learning strategies for investigation of weird pattern formulation from large volume data using quantum computing. Scopus 218:569–576. https://doi.org/10.1007/978-981-16-2164-2_45
Nweke HF, Teh YW, Al-garadi MA, Alo UR (2018) Deep learning algorithms for human activity recognition using mobile and wearable sensor networks: state of the art and research challenges. Scopus 105:233–261. https://doi.org/10.1016/j.eswa.2018.03.056
O’Brien JL (2007) Optical quantum computing. Science 318(5856):1567–1570. https://doi.org/10.1126/science.1142892
Ong TJ, Tan CC (2022) A genetic algorithm for quantum circuit generation in OpenQASM. Scopus 295:97–114. https://doi.org/10.1007/978-3-030-82196-8_8
Pathak N, Misra NK, Bhoi BK, Kumar S (2022) Concept and algorithm of quantum computing during pandemic situation of COVID-19. Scopus 235:523–535. https://doi.org/10.1007/978-981-16-2877-1_48
Peelam MS, Johari R (2022) Enhancing security using quantum computing (ESUQC). Scopus 768:227–235. https://doi.org/10.1007/978-981-16-2354-7_21
Peng X, Zhang Y, Xiao S, Zheng W, Cui JQ, Chen L, Xiao D (2008) An alert correlation method based on improved cluster algorithm. doi: https://doi.org/10.1109/PACIIA . 2008.285
Perianes-Rodriguez A, Waltman L, van Eck NJ (2016) Constructing bibliometric networks: a comparison between full and fractional counting. J Inform 10(4):1178–1195. https://doi.org/10.1016/j.joi.2016.10.006
Potempa R, Porebski S (2022) Comparing concepts of quantum and classical neural network models for image classification task. Scopus 255:61–71. https://doi.org/10.1007/978-3-030-81523-3_6
Rere LR, Fanany MI, Arymurthy AM (2015) Simulated annealing algorithm for deep learning. Proc Comput Sci 72:137–144. https://doi.org/10.1016/j.procs.2015.12.114
Resconi G, der Wal AJV (2002) Morphogenic neural networks encode abstract rules by data. Scopus 142(1–4):249–273. https://doi.org/10.1016/S0020-0255(02)00168-8
Sa I, Ge Z, Dayoub F, Upcroft B, Perez T, McCool C (2016) Deepfruits: a fruit detection system using deep neural networks. Scopus. https://doi.org/10.3390/s16081222
Saffman M, Walker TG, M∅lmer K (2010) Quantum information with Rydberg atoms. Rev Mod Phys 82(3):2313–2363. doi: https://doi.org/10.1103/RevModPhys.82.2313
Schmidhuber J (2015) Deep learning in neural networks: an overview. Neural Netw 61:85–117. https://doi.org/10.1016/j.neunet.2014.09.003
Shinde PP, Shah S (2018) A review of machine learning and deep learning applications. In: Proceedings of the 2018 fourth international conference on computing communication control and automation (ICCUBEA), pp 1–6. Doi: https://doi.org/10.1109/ICCUBEA.2018.8697857
Shrestha A, Mahmood A (2019) Review of deep learning algorithms and architectures. Scopus 7:53040–53065. https://doi.org/10.1109/ACCESS.2019.2912200
Singh AK, Saxena D, Kumar J, Gupta V (2021a) A quantum approach towards the adaptive prediction of cloud workloads. Scopus 32(12):2893–2905. https://doi.org/10.1109/TPDS.2021.3079341
Singh S, Chawla P, Sarkar A, Chandrashekar CM (2021b) Universal quantum computing using single-particle discrete-time quantum walk. Scopus. https://doi.org/10.1038/s41598-021-91033-5
Song T, Wang S, Wang X (2008) The design of reversible gate and reversible sequential circuit based on DNA computing. Doi: https://doi.org/10.1109/ISKE.2008.4730909
Sze V, Chen YH, Yang TJ, Emer JS (2017) Efficient processing of deep neural networks: a tutorial and survey. Scopus 105(12):2295–2329. https://doi.org/10.1109/JPROC.2017.2761740
Tang F, Fadlullah ZM, Mao B, Kato N (2018) An intelligent traffic load prediction-based adaptive channel assignment algorithm in SDN-IoT: a deep learning approach. Scopus 5(6):5141–5154. https://doi.org/10.1109/JIOT.2018.2838574
Thomasian NM, Adashi EY (2021) Cybersecurity in the internet of medical things. Scopus. https://doi.org/10.1016/j.hlpt.2021.100549
Thomford NE, Senthebane DA, Rowe A, Munro D, Seele P, Maroyi A, Dzobo K (2018) Natural products for drug discovery in the 21st century: innovations for novel drug discovery. Scopus. https://doi.org/10.3390/ijms19061578
Topol EJ (2019) High-performance medicine: the convergence of human and artificial intelligence. Scopus 25(1):44–56. https://doi.org/10.1038/s41591-018-0300-7
Tuyls K, Weiss G (2012) Multiagent learning: Basics, challenges, and prospects. Scopus 33(3):41–52. https://doi.org/10.1609/aimag.v33i3.2426
Ulyanov SV (2020) Quantum fuzzy inference based on quantum genetic algorithm: quantum simulator in intelligent robotics. Scopus 1095:78–85. https://doi.org/10.1007/978-3-030-35249-3_9
Ulyanov SV (2004) Quantum soft computing in control process design: quantum genetic algorithms and quantum neural network approaches
Valdez F (2020) A review of optimization swarm intelligence-inspired algorithms with type-2 fuzzy logic parameter adaptation. Soft Comput 24(1):215–226. https://doi.org/10.1007/s00500-019-04290-y
Varela-Santos S, Melin P (2021) A new approach for classifying coronavirus COVID-19 based on its manifestation on chest X-rays using texture features and neural networks. Inf Sci 545:403–414. https://doi.org/10.1016/j.ins.2020.09.041
Van Eck NJ, Waltman L (2014) Visualizing bibliometric networks. In: Measuring scholarly impact, vol 1. Springer, New York. DOI https://doi.org/10.1007/978-3-319-10377-8
Varma R, Melville C, Pinello C, Sahai T (2020) Post quantum secure command and control of mobile agents inserting quantum-resistant encryption schemes in the secure robot operating system. Doi: https://doi.org/10.1109/IRC.2020.00012
Wang S, Clark R, Wen H, Trigoni N (2017) DeepVO: towards end-to-end visual odometry with deep re-current. Convolut Neural Netw. https://doi.org/10.1109/ICRA.2017.7989236
Wang Q, Chumak AV, Pirro P (2021) Inverse-design magnonic devices. Scopus. https://doi.org/10.1038/s41467-021-22897-4
Wei J, He J, Chen K, Zhou Y, Tang Z (2017a) Collaborative filtering and deep learning based recommendation system for cold start items. Scopus 69:1339–1351. https://doi.org/10.1016/j.eswa.2016.09.040
Wei J, Luna PD, Bengio Y, Aspuru-Guzik A, Sargent E (2017b) Use machine learning to find energy materials. Scopus 552(7683):23–25. https://doi.org/10.1038/d41586-017-07820-6
Wei T, Wang Y, Zhu Q (2017c) Deep reinforcement learning for building HVAC control. doi: https://doi.org/10.1145/3061639.3062224
Werbos P, Dolmatova L (2016) Analog quantum computing (AQC) and the need for time-symmetric physics. Quant Inform Process. https://doi.org/10.1007/s11128-015-1146-2
Article MathSciNet MATH Google Scholar
Wu R, Peng W, Zhou C, Chao F, Yang L, Lin CM, Shang C (2019) Towards deep learning based robot automatic choreography system. In: Yu H, Liu J, Liu L, Ju Z, Liu Y, Zhou D (eds) Intelligent robotics and applications 12th international conference, {ICIRA} 2019, Shenyang, China, August 8–11, 2019, Proceedings, Part {IV}, Springer, vol 11743, pp 629–640. Doi: https://doi.org/10.1007/978-3-030-27538-9_54
Xiao Y, Nazarian S, Bogdan P (2021) A stochastic quantum program synthesis framework based on Bayesian optimization. Scopus. https://doi.org/10.1038/s41598-021-91035-3
Yang HF, Dillon TS, Chen YPP (2017) Optimized structure of the traffic flow forecasting model with a deep learning approach. Scopus 28(10):2371–2381. https://doi.org/10.1109/TNNLS.2016.2574840
Yunakovsky SE, Kot M, Pozhar N, Nabokov D, Kudinov M, Guglya A, Kiktenko EO, Kolycheva E, Borisov A, Fedorov AK (2021) Towards security recommendations for public-key infrastructures for production environments in the post-quantum era. Scopus. https://doi.org/10.1140/epjqt/s40507-021-00104-z
Zhang G, Wu Y, Zhang F, Liu X (2012) Application of adaptive quantum particle swarm optimization algorithm for optimal dispatching of cascaded hydropower stations. Scopus 7390:463–470. https://doi.org/10.1007/978-3-642-31576-3_59
Zhang J, Chen C, Vogeley MSE (2010) The use of scientific data: a content analysis. In: Proceedings of the 73rd navigating streams in an information ecosystem ASIS{&}T annual meeting, {ASIST} 2010, Pittsburgh, PA, USA, October 22–27, 2010, Wiley, vol 47, pp 1–2, DOI: https://doi.org/10.1002/meet.14504701319
Zhao R, Wang S (2021) A review of quantum neural networks: methods, models, dilemma
Zhu M, Wang X, Wang Y (2018) Human-like autonomous car-following model with deep reinforcement learning. Scopus 97:348–368. https://doi.org/10.1016/j.trc.2018.10.024
Zhu K, Jiang M (2010) Quantum artificial fish swarm algorithm. Doi: https://doi.org/10.1109/WCICA.2010.5553761
Zioui N, Mahmoudi Y, Mahmoudi A, Tadjine M, Bentouba S (2021) A new quantum-computing-based algorithm for robotic arms and rigid bodies’ orientation. Scopus 7(3):1836–1846. https://doi.org/10.22055/jacm.2021.37611.3048
Download references
Acknowledgements
The authors would like to thank CONACYT and Tecnológico Nacional de Mexico/Tijuana Institute of Technology for the support during this research work.
This paper did not receive funding.
Author information
Authors and affiliations.
Tijuana Institute of Technology, Calzada Tecnologico S/N, 22414, Tijuana, BC, Mexico
Fevrier Valdez & Patricia Melin
You can also search for this author in PubMed Google Scholar
Contributions
Conceptualization, F.V. and P.M.; methodology, F.V.; software, F.V.; validation, F.V. and P.M.; formal analysis, P.M.; investigation, P.M.; resources, P.M.; writing—original draft preparation, F.V. and P.M.; writing—review and editing, P.M.; visualization, F.V.; supervision, F.V. and P.M.; project administration. All authors have read and agreed to the published version of the manuscript.
Corresponding author
Correspondence to Fevrier Valdez .
Ethics declarations
Conflict of interest.
All the authors in the paper have no conflict of interest.
Ethical approval
This article does not contain any studies with human participants or animals performed by any of the authors.
Informed Consent Statement
Additional information.
Communicated by Oscar Castillo.
Publisher's Note
Springer Nature remains neutral with regard to jurisdictional claims in published maps and institutional affiliations.
Rights and permissions
Reprints and permissions
About this article
Valdez, F., Melin, P. A review on quantum computing and deep learning algorithms and their applications. Soft Comput 27 , 13217–13236 (2023). https://doi.org/10.1007/s00500-022-07037-4
Download citation
Accepted : 09 March 2022
Published : 07 April 2022
Issue Date : September 2023
DOI : https://doi.org/10.1007/s00500-022-07037-4
Share this article
Anyone you share the following link with will be able to read this content:
Sorry, a shareable link is not currently available for this article.
Provided by the Springer Nature SharedIt content-sharing initiative
- Quantum computing
- Deep learning
- Neural networks
- Fuzzy logic
- Intelligent
- Find a journal
- Publish with us
- Track your research
Suggestions or feedback?
MIT News | Massachusetts Institute of Technology
- Machine learning
- Social justice
- Black holes
- Classes and programs
Departments
- Aeronautics and Astronautics
- Brain and Cognitive Sciences
- Architecture
- Political Science
- Mechanical Engineering
Centers, Labs, & Programs
- Abdul Latif Jameel Poverty Action Lab (J-PAL)
- Picower Institute for Learning and Memory
- Lincoln Laboratory
- School of Architecture + Planning
- School of Engineering
- School of Humanities, Arts, and Social Sciences
- Sloan School of Management
- School of Science
- MIT Schwarzman College of Computing
A blueprint for making quantum computers easier to program
Press contact :.
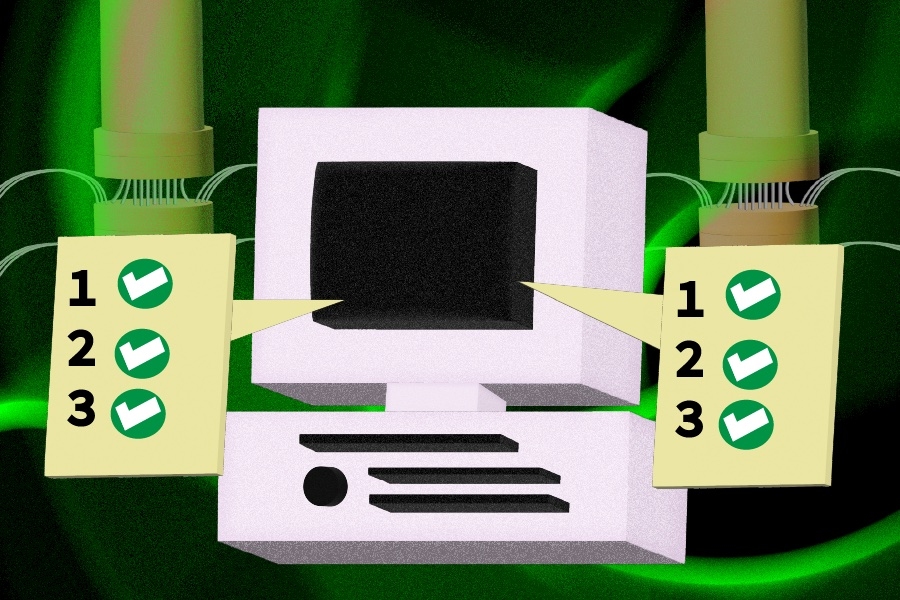
Previous image Next image
When MIT professor and now Computer Science and Artificial Intelligence Laboratory (CSAIL) member Peter Shor first demonstrated the potential of quantum computers to solve problems faster than classical ones, he inspired scientists to imagine countless possibilities for the emerging technology. Thirty years later, though, the quantum edge remains a peak not yet reached. Unfortunately, the technology of quantum computing isn’t fully operational yet. One major challenge lies in translating quantum algorithms from abstract mathematical concepts into concrete code that can run on a quantum computer. Whereas programmers for regular computers have access to myriad languages such as Python and C++ with constructs that align with standard classical computing abstractions, quantum programmers have no such luxury; few quantum programming languages exist today, and they are comparatively difficult to use because quantum computing abstractions are still in flux. In their recent work, MIT researchers highlight that this disparity exists because quantum computers don’t follow the same rules for how to complete each step of a program in order — an essential process for all computers called control flow — and present a new abstract model for a quantum computer that could be easier to program.
In a paper soon to be presented at the ACM Conference on Object-oriented Programming, Systems, Languages, and Applications, the group outlines a new conceptual model for a quantum computer, called a quantum control machine, that could bring us closer to making programs as easy to write as those for regular classical computers. Such an achievement would help turbocharge tasks that are impossible for regular computers to efficiently complete, like factoring large numbers, retrieving information in databases, and simulating how molecules interact for drug discoveries. “Our work presents the principles that govern how you can and cannot correctly program a quantum computer,” says lead author and CSAIL PhD student Charles Yuan SM ’22. “One of these laws implies that if you try to program a quantum computer using the same basic instructions as a regular classical computer, you’ll end up turning that quantum computer into a classical computer and lose its performance advantage. These laws explain why quantum programming languages are tricky to design and point us to a way to make them better.” Old school vs. new school computing One reason why classical computers are relatively easier to program today is that their control flow is fairly straightforward. The basic ingredients of a classical computer are simple: binary digits or bits, a simple collection of zeros and ones. These ingredients assemble into the instructions and components of the computer’s architecture. One important component is the program counter, which locates the next instruction in a program much like a chef following a recipe, by recalling the next direction from memory. As the algorithm sequentially navigates through the program, a control flow instruction called a conditional jump updates the program counter to make the computer either advance forward to the next instruction or deviate from its current steps. By contrast, the basic ingredient of a quantum computer is a qubit, which is a quantum version of a bit. This quantum data exists in a state of zero and one at the same time, known as a superposition. Building on this idea, a quantum algorithm can choose to execute a superposition of two instructions at the same time — a concept called quantum control flow.
The problem is that existing designs of quantum computers don’t include an equivalent of the program counter or a conditional jump. In practice, that means programmers typically implement control flow by manually arranging logical gates that describe the computer’s hardware, which is a tedious and error-prone procedure. To provide these features and close the gap with classical computers, Yuan and his coauthors created the quantum control machine — an instruction set for a quantum computer that works like the classical idea of a virtual machine. In their paper, the researchers envision how programmers could use this instruction set to implement quantum algorithms for problems such as factoring numbers and simulating chemical interactions.
As the technical crux of this work, the researchers prove that a quantum computer cannot support the same conditional jump instruction as a classical computer, and show how to modify it to work correctly on a quantum computer. Specifically, the quantum control machine features instructions that are all reversible — they can run both forward and backward in time. A quantum algorithm needs all instructions, including those for control flow, to be reversible so that it can process quantum information without accidentally destroying its superposition and producing a wrong answer.
The hidden simplicity of quantum computers According to Yuan, you don’t need to be a physicist or mathematician to understand how this futuristic technology works. Quantum computers don’t necessarily have to be arcane machines, he says, that require scary equations to understand. With the quantum control machine, the CSAIL team aims to lower the barrier to entry for people to interact with a quantum computer by raising the unfamiliar concept of quantum control flow to a level that mirrors the familiar concept of control flow in classical computers. By highlighting the dos and don’ts of building and programming quantum computers, they hope to educate people outside of the field about the power of quantum technology and its ultimate limits.
Still, the researchers caution that as is the case for many other designs, it’s not yet possible to directly turn their work into a practical hardware quantum computer due to the limitations of today’s qubit technology. Their goal is to develop ways of implementing more kinds of quantum algorithms as programs that make efficient use of a limited number of qubits and logic gates. Doing so would bring us closer to running these algorithms on the quantum computers that could come online in the near future.
“The fundamental capabilities of models of quantum computation has been a central discussion in quantum computation theory since its inception,” says MIT-IBM Watson AI Lab researcher Patrick Rall, who was not involved in the paper. “Among the earliest of these models are quantum Turing machines which are capable of quantum control flow. However, the field has largely moved on to the simpler and more convenient circuit model, for which quantum lacks control flow. Yuan, Villanyi, and Carbin successfully capture the underlying reason for this transition using the perspective of programming languages. While control flow is central to our understanding of classical computation, quantum is completely different! I expect this observation to be critical for the design of modern quantum software frameworks as hardware platforms become more mature.” The paper lists two additional CSAIL members as authors: PhD student Ági Villányi ’21 and Associate Professor Michael Carbin. Their work was supported, in part, by the National Science Foundation and the Sloan Foundation.
Share this news article on:
Related links.
- Michael Carbin
- Charles Yuan
- Computer Science and Artificial Intelligence Laboratory (CSAIL)
- Department of Electrical Engineering and Computer Science
Related Topics
- Electrical Engineering & Computer Science (eecs)
- MIT-IBM Watson AI Lab
- Computer science and technology
- Quantum computing
- Programming
Related Articles
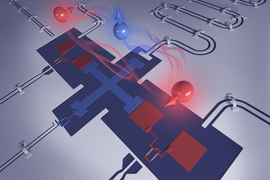
New qubit circuit enables quantum operations with higher accuracy
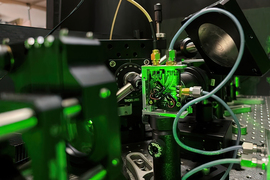
Canceling noise to improve quantum devices
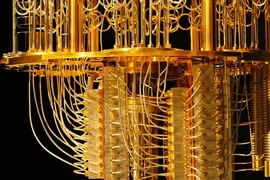
A new language for quantum computing
Previous item Next item
More MIT News
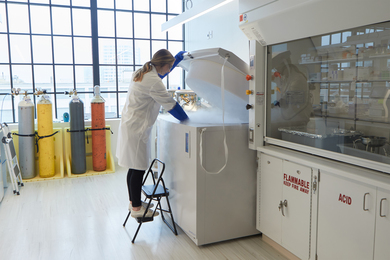
A home where world-changing innovations take flight
Read full story →
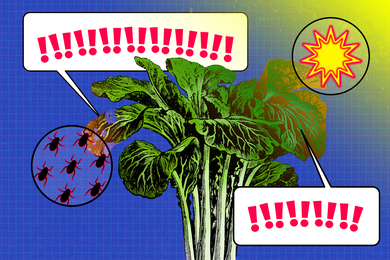
Plant sensors could act as an early warning system for farmers
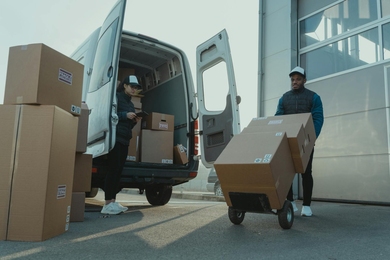
3 Questions: Enhancing last-mile logistics with machine learning
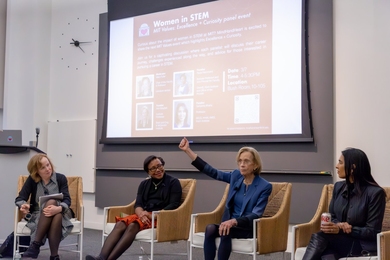
Women in STEM — A celebration of excellence and curiosity
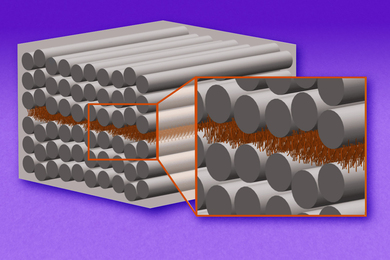
“Nanostitches” enable lighter and tougher composite materials
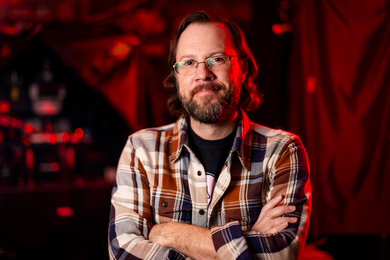
From neurons to learning and memory
- More news on MIT News homepage →
Massachusetts Institute of Technology 77 Massachusetts Avenue, Cambridge, MA, USA
- Map (opens in new window)
- Events (opens in new window)
- People (opens in new window)
- Careers (opens in new window)
- Accessibility
- Social Media Hub
- MIT on Facebook
- MIT on YouTube
- MIT on Instagram
Inventing what’s next in quantum
Latest news.
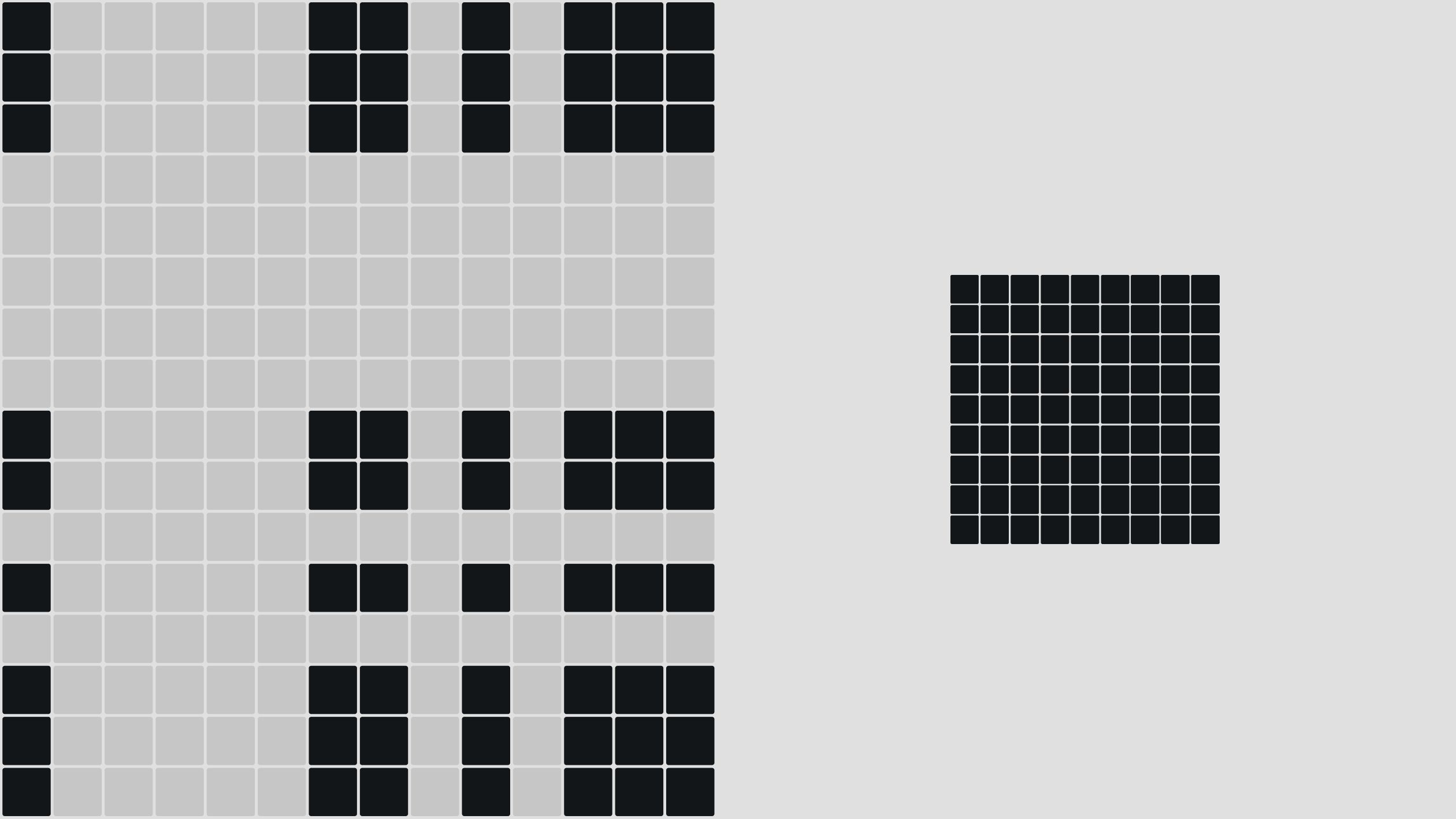
Exploring measurement error mitigation with the Mthree Qiskit extension
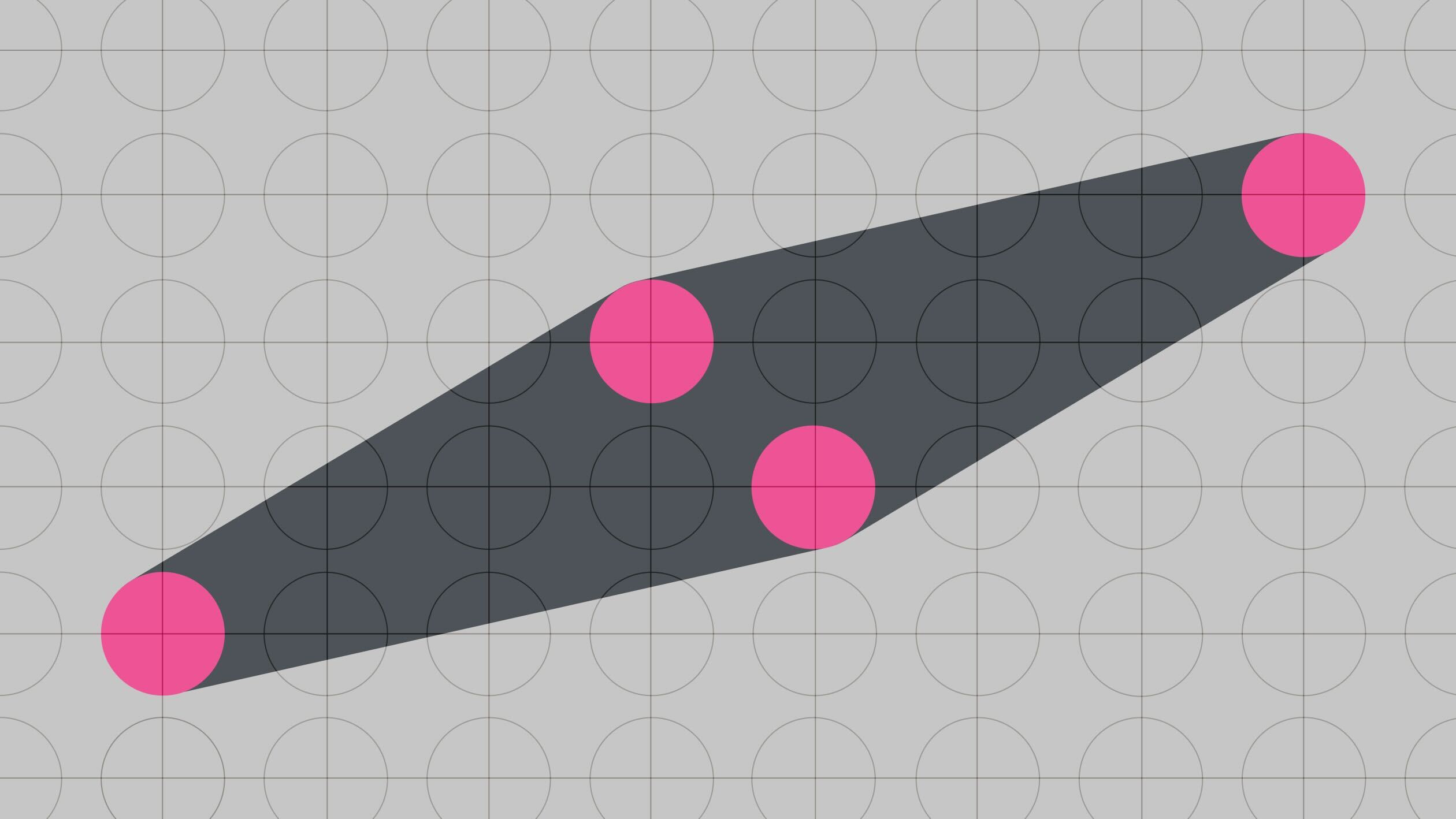
Landmark IBM error correction paper published on the cover of Nature
Best practices for transitioning to qiskit sdk v1.0.
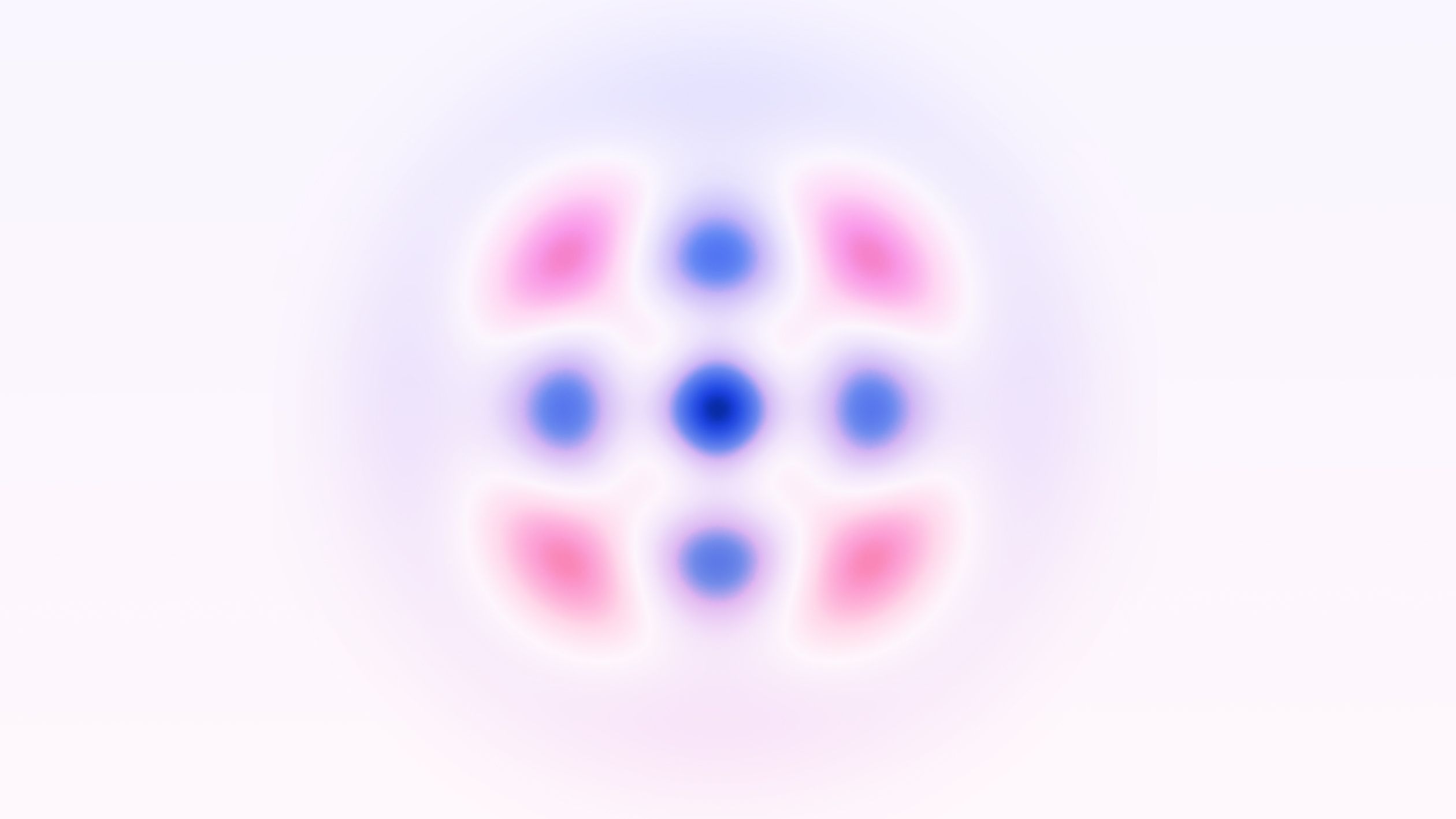
Simulating a bosonic error correcting code using Bosonic Qiskit
Research papers.
Papers leveraging Qiskit® and IBM® quantum services
IBM quantum publications
Encoding a magic state with beyond break-even fidelity
Gupta, R.S., Sundaresan, N., Alexander, T. et al. | Nature 625, 259-263 (2024)
Evidence for the utility of quantum computing before fault tolerance
Kim, Y., Eddins, A., Anand, S. et al. | Nature 618, 500–505 (2023)
Quantum-enhanced Markov chain Monte Carlo
Layden, D., Mazzola, G., Mishmash, R.V. et al. | Nature 619, 282–287 (2023)
Partner publications
Simulating large-size quantum spin chains on cloud-based superconducting quantum computers
Yu, H., Zhao, Y., & Wei, T. C. | Physical Review Research, 5(1), 013183 (2023)
Scalable circuits for preparing ground states on digital quantum computers: the Schwinger model vacuum on 100 qubits
Farrell, R. C., Illa, M., Ciavarella, A. N., & Savage, M. J. | arXiv:2308.04481 (2023)
Quantum algorithm for high energy physics simulations
Bauer, C. W., De Jong, W. A., Nachman, B., & Provasoli, D. | arXiv:1904.03196 (2019)
Join our team
Explore a quantum career at ibm, intern with us.
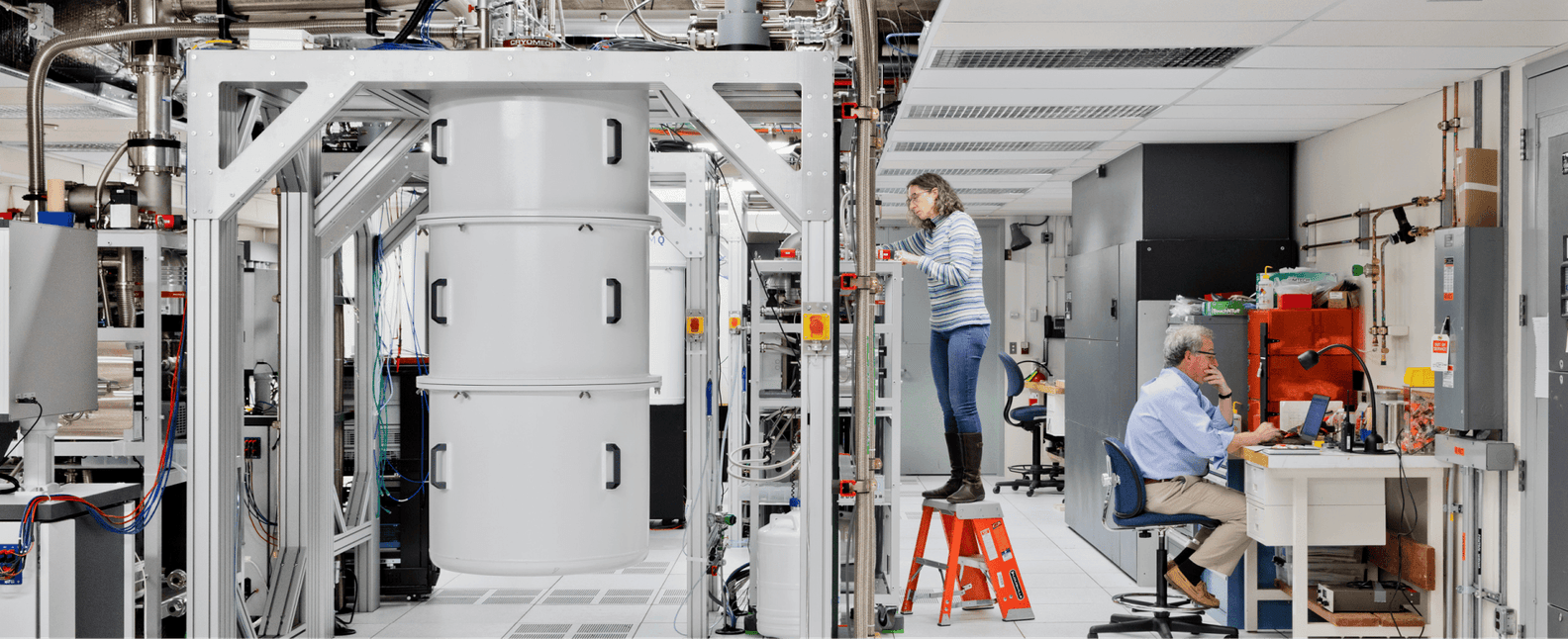
Quantum Computing
Quantum Computing merges two great scientific revolutions of the 20th century: computer science and quantum physics. Quantum physics is the theoretical basis of the transistor, the laser, and other technologies which enabled the computing revolution. But on the algorithmic level, today's computing machinery still operates on ""classical"" Boolean logic. Quantum Computing is the design of hardware and software that replaces Boolean logic by quantum law at the algorithmic level. For certain computations such as optimization, sampling, search or quantum simulation this promises dramatic speedups. We are particularly interested in applying quantum computing to artificial intelligence and machine learning. This is because many tasks in these areas rely on solving hard optimization problems or performing efficient sampling.
Recent Publications
Some of our teams.
Applied science
We're always looking for more talented, passionate people.
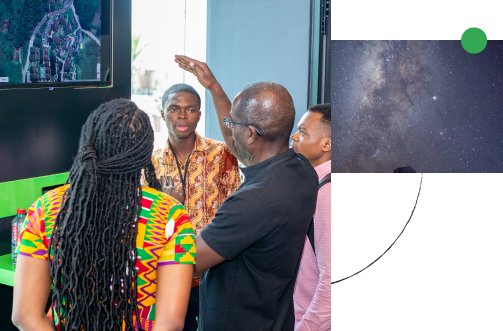
quantum computing Recently Published Documents
Total documents.
- Latest Documents
- Most Cited Documents
- Contributed Authors
- Related Sources
- Related Keywords
Improving the Variational Quantum Eigensolver Using Variational Adiabatic Quantum Computing
The variational quantum eigensolver (VQE) is a hybrid quantum-classical algorithm for finding the minimum eigenvalue of a Hamiltonian that involves the optimization of a parameterized quantum circuit. Since the resulting optimization problem is in general nonconvex, the method can converge to suboptimal parameter values that do not yield the minimum eigenvalue. In this work, we address this shortcoming by adopting the concept of variational adiabatic quantum computing (VAQC) as a procedure to improve VQE. In VAQC, the ground state of a continuously parameterized Hamiltonian is approximated via a parameterized quantum circuit. We discuss some basic theory of VAQC to motivate the development of a hybrid quantum-classical homotopy continuation method. The proposed method has parallels with a predictor-corrector method for numerical integration of differential equations. While there are theoretical limitations to the procedure, we see in practice that VAQC can successfully find good initial circuit parameters to initialize VQE. We demonstrate this with two examples from quantum chemistry. Through these examples, we provide empirical evidence that VAQC, combined with other techniques (an adaptive termination criteria for the classical optimizer and a variance-based resampling method for the expectation evaluation), can provide more accurate solutions than “plain” VQE, for the same amount of effort.
First Step to Quantum Computing
Quantum computing for chemical and biomolecular product design, quantum computing for the brain, introduction to the special issue on design automation for quantum computing, quantum fourier transform to estimate drive cycles.
AbstractDrive cycles in vehicle systems are important determinants for energy consumption, emissions, and safety. Estimating the frequency of the drive cycle quickly is important for control applications related to fuel efficiency, emission reduction and improving safety. Quantum computing has established the computational efficiency that can be gained. A drive cycle frequency estimation algorithm based on the quantum Fourier transform is exponentially faster than the classical Fourier transform. The algorithm is applied on real world data set. We evaluate the method using a quantum computing simulator, demonstrating remarkable consistency with the results from the classical Fourier transform. Current quantum computers are noisy, a simple method is proposed to mitigate the impact of the noise. The method is evaluated on a 15 qubit IBM-q quantum computer. The proposed method for a noisy quantum computer is still faster than the classical Fourier transform.
Quantum Computing for Dealing with Inaccurate Knowledge Related to the Certainty Factors Model
In this paper, we illustrate that inaccurate knowledge can be efficiently implemented in a quantum environment. For this purpose, we analyse the correlation between certainty factors and quantum probability. We first explore the certainty factors approach for inexact reasoning from a classical point of view. Next, we introduce some basic aspects of quantum computing, and we pay special attention to quantum rule-based systems. In this context, a specific use case was built: an inferential network for testing the behaviour of the certainty factors approach in a quantum environment. After the design and execution of the experiments, the corresponding analysis of the obtained results was performed in three different scenarios: (1) inaccuracy in declarative knowledge, or imprecision, (2) inaccuracy in procedural knowledge, or uncertainty, and (3) inaccuracy in both declarative and procedural knowledge. This paper, as stated in the conclusions, is intended to pave the way for future quantum implementations of well-established methods for handling inaccurate knowledge.
Edge Caching in Fog-Based Sensor Networks through Deep Learning-Associated Quantum Computing Framework
Fog computing (FC) based sensor networks have emerged as a propitious archetype for next-generation wireless communication technology with caching, communication, and storage capacity services in the edge. Mobile edge computing (MEC) is a new era of digital communication and has a rising demand for intelligent devices and applications. It faces performance deterioration and quality of service (QoS) degradation problems, especially in the Internet of Things (IoT) based scenarios. Therefore, existing caching strategies need to be enhanced to augment the cache hit ratio and manage the limited storage to accelerate content deliveries. Alternatively, quantum computing (QC) appears to be a prospect of more or less every typical computing problem. The framework is basically a merger of a deep learning (DL) agent deployed at the network edge with a quantum memory module (QMM). Firstly, the DL agent prioritizes caching contents via self organizing maps (SOMs) algorithm, and secondly, the prioritized contents are stored in QMM using a Two-Level Spin Quantum Phenomenon (TLSQP). After selecting the most appropriate lattice map (32 × 32) in 750,000 iterations using SOMs, the data points below the dark blue region are mapped onto the data frame to get the videos. These videos are considered a high priority for trending according to the input parameters provided in the dataset. Similarly, the light-blue color region is also mapped to get medium-prioritized content. After the SOMs algorithm’s training, the topographic error (TE) value together with quantization error (QE) value (i.e., 0.0000235) plotted the most appropriate map after 750,000 iterations. In addition, the power of QC is due to the inherent quantum parallelism (QP) associated with the superposition and entanglement principles. A quantum computer taking “n” qubits that can be stored and execute 2n presumable combinations of qubits simultaneously reduces the utilization of resources compared to conventional computing. It can be analyzed that the cache hit ratio will be improved by ranking the content, removing redundant and least important content, storing the content having high and medium prioritization using QP efficiently, and delivering precise results. The experiments for content prioritization are conducted using Google Colab, and IBM’s Quantum Experience is considered to simulate the quantum phenomena.
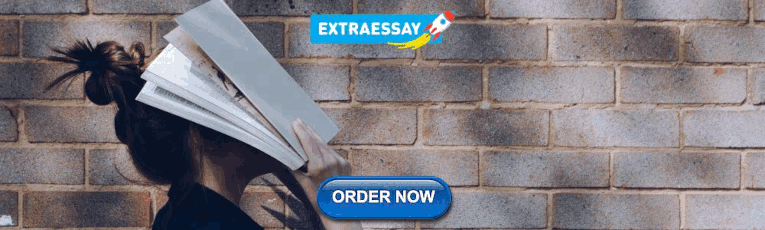
Quantum computing formulation of some classical Hadamard matrix searching methods and its implementation on a quantum computer
AbstractFinding a Hadamard matrix (H-matrix) among all possible binary matrices of corresponding order is a hard problem that can be solved by a quantum computer. Due to the limitation on the number of qubits and connections in current quantum processors, only low order H-matrix search of orders 2 and 4 were implementable by previous method. In this paper, we show that by adopting classical searching techniques of the H-matrices, we can formulate new quantum computing methods for finding higher order ones. We present some results of finding H-matrices of order up to more than one hundred and a prototypical experiment of the classical-quantum resource balancing method that yields a 92-order H-matrix previously found by Jet Propulsion Laboratory researchers in 1961 using a mainframe computer. Since the exactness of the solutions can be verified by an orthogonality test performed in polynomial time; which is untypical for optimization of hard problems, the proposed method can potentially be used for demonstrating practical quantum supremacy in the near future.
Analysis of Carleman Linearization of Lattice Boltzmann
We explore the Carleman linearization of the collision term of the lattice Boltzmann formulation, as a first step towards formulating a quantum lattice Boltzmann algorithm. Specifically, we deal with the case of a single, incompressible fluid with the Bhatnagar Gross and Krook equilibrium function. Under this assumption, the error in the velocities is proportional to the square of the Mach number. Then, we showcase the Carleman linearization technique for the system under study. We compute an upper bound to the number of variables as a function of the order of the Carleman linearization. We study both collision and streaming steps of the lattice Boltzmann formulation under Carleman linearization. We analytically show why linearizing the collision step sacrifices the exactness of streaming in lattice Boltzmann, while also contributing to the blow up in the number of Carleman variables in the classical algorithm. The error arising from Carleman linearization has been shown analytically and numerically to improve exponentially with the Carleman linearization order. This bodes well for the development of a corresponding quantum computing algorithm based on the Lattice Boltzmann equation.
Export Citation Format
Share document.
What’s Next in Quantum is quantum-centric supercomputing
A key factor in classical supercomputing is the intersection of communication and computation. The same holds true for quantum. Quantum-centric supercomputing utilizes a modular architecture to enable scaling. It combines quantum communication and computation to increase system capacity, and uses a hybrid cloud middleware to seamlessly integrate quantum and classical workflows. To realize this next wave in quantum we are building a new system called Quantum System Two. See our quantum development roadmap
- Quantum Circuits and Software
- Quantum Chemistry
- Quantum Machine Learning
- Quantum Community
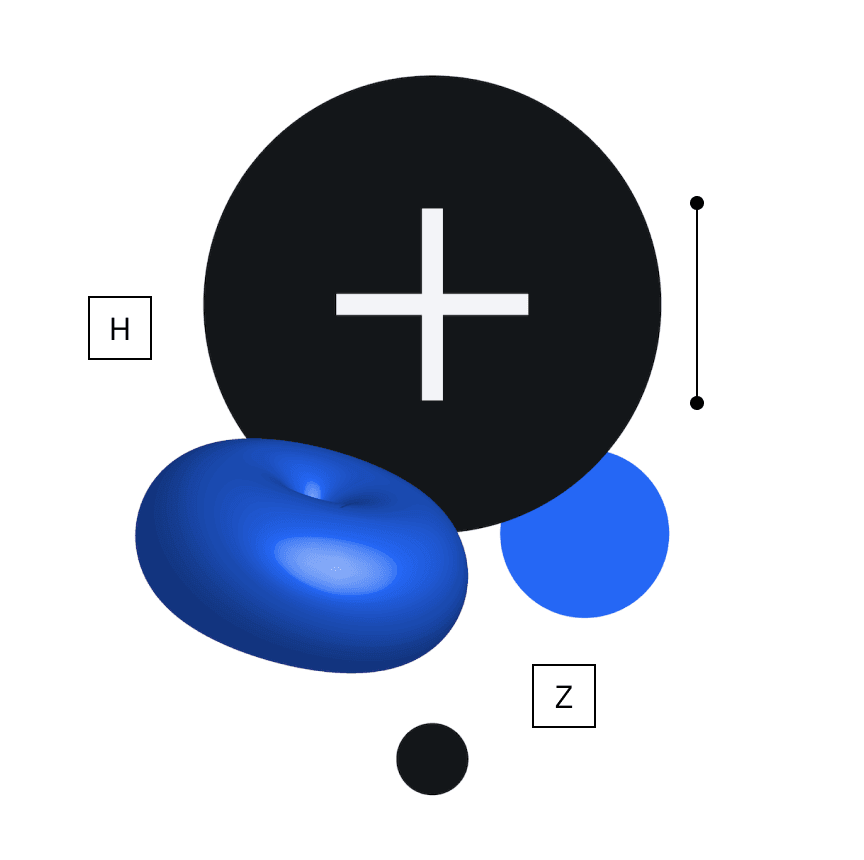
Program real quantum systems
IBM offers cloud access to the most advanced quantum computers available. Learn, develop, and run programs with our quantum applications and systems.
The first IBM quantum computer installed on a university campus comes to RPI
Exploring measurement error mitigation with the mthree qiskit extension.
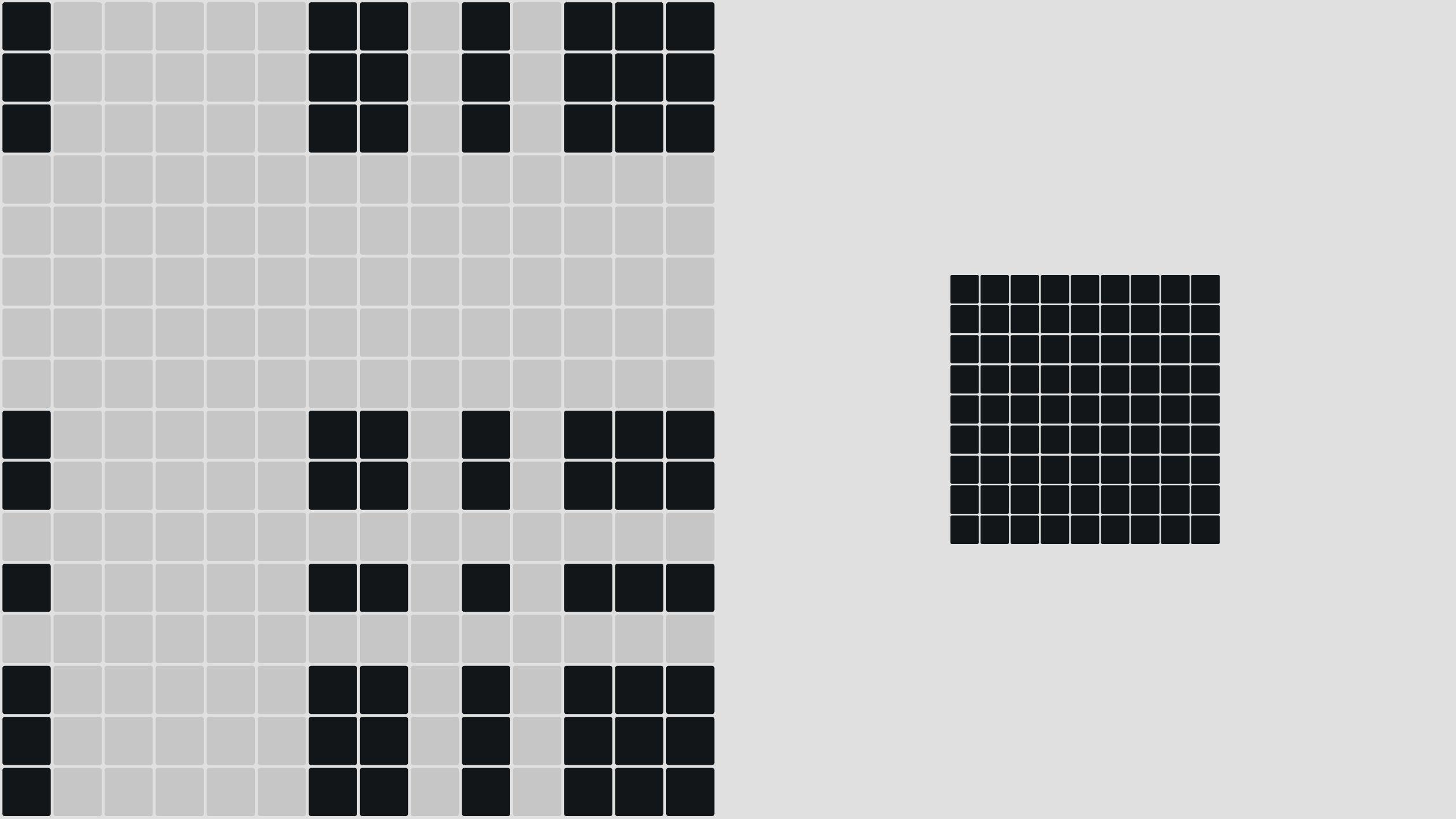
- Quantum Error Correction & Mitigation
Landmark IBM error correction paper published on the cover of Nature
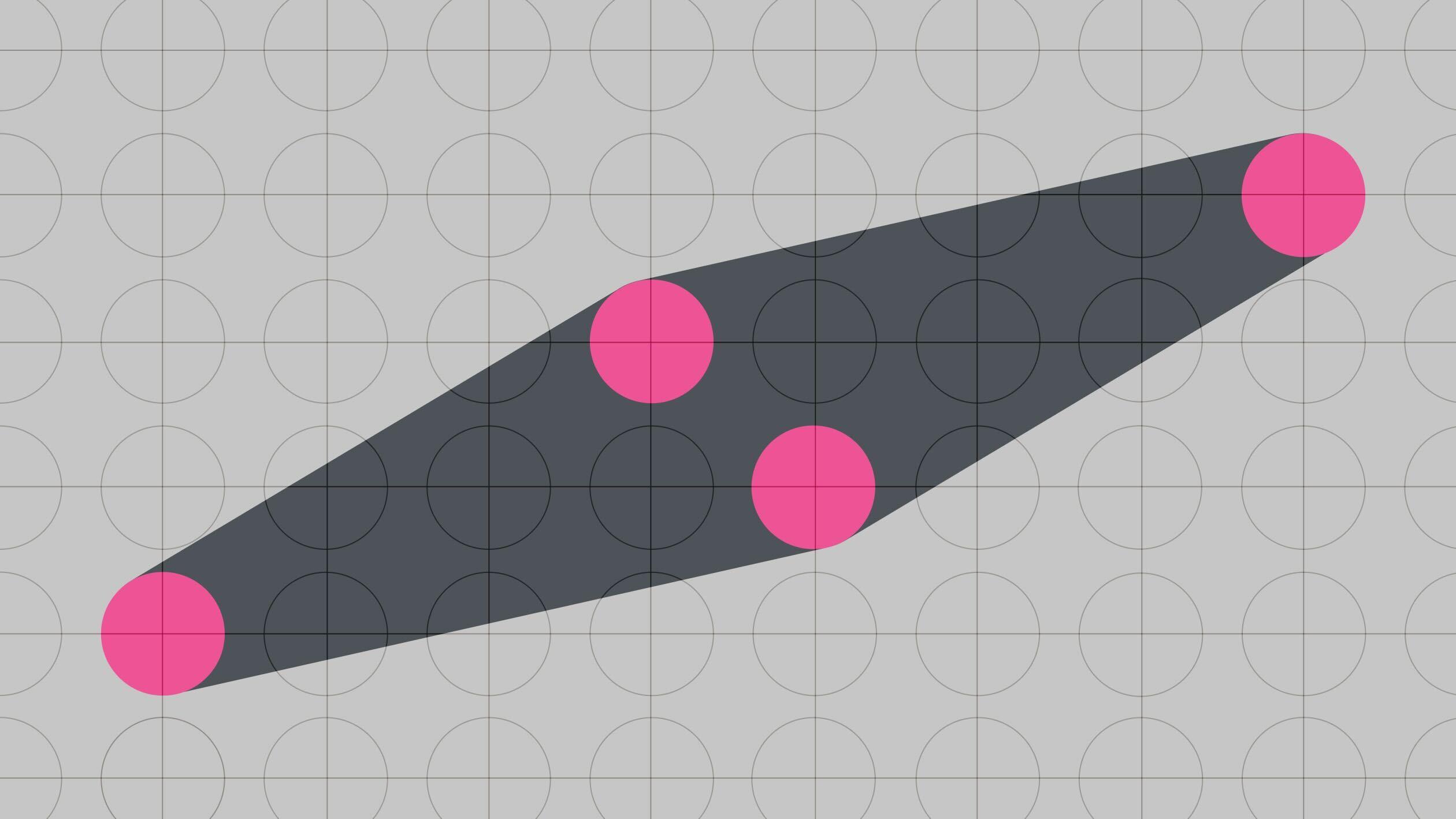
Computing with error-corrected quantum computers
- Quantum Research
The 2023 IBM Research annual letter
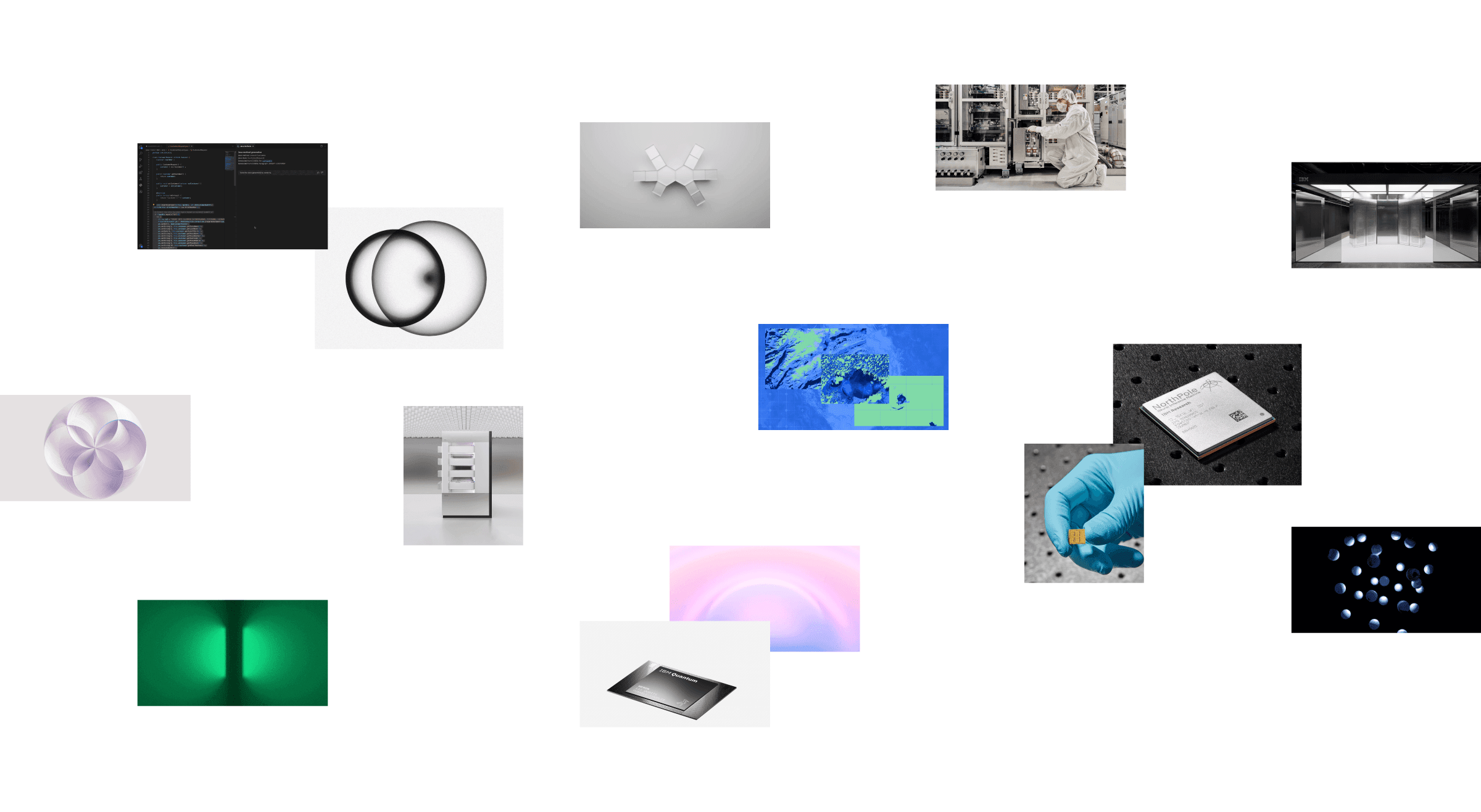
- Accelerated Discovery
- Hybrid Cloud
- Semiconductors
The era of quantum utility must also be the era of responsible quantum computing
- Quantum Safe
- See more of our work on Quantum
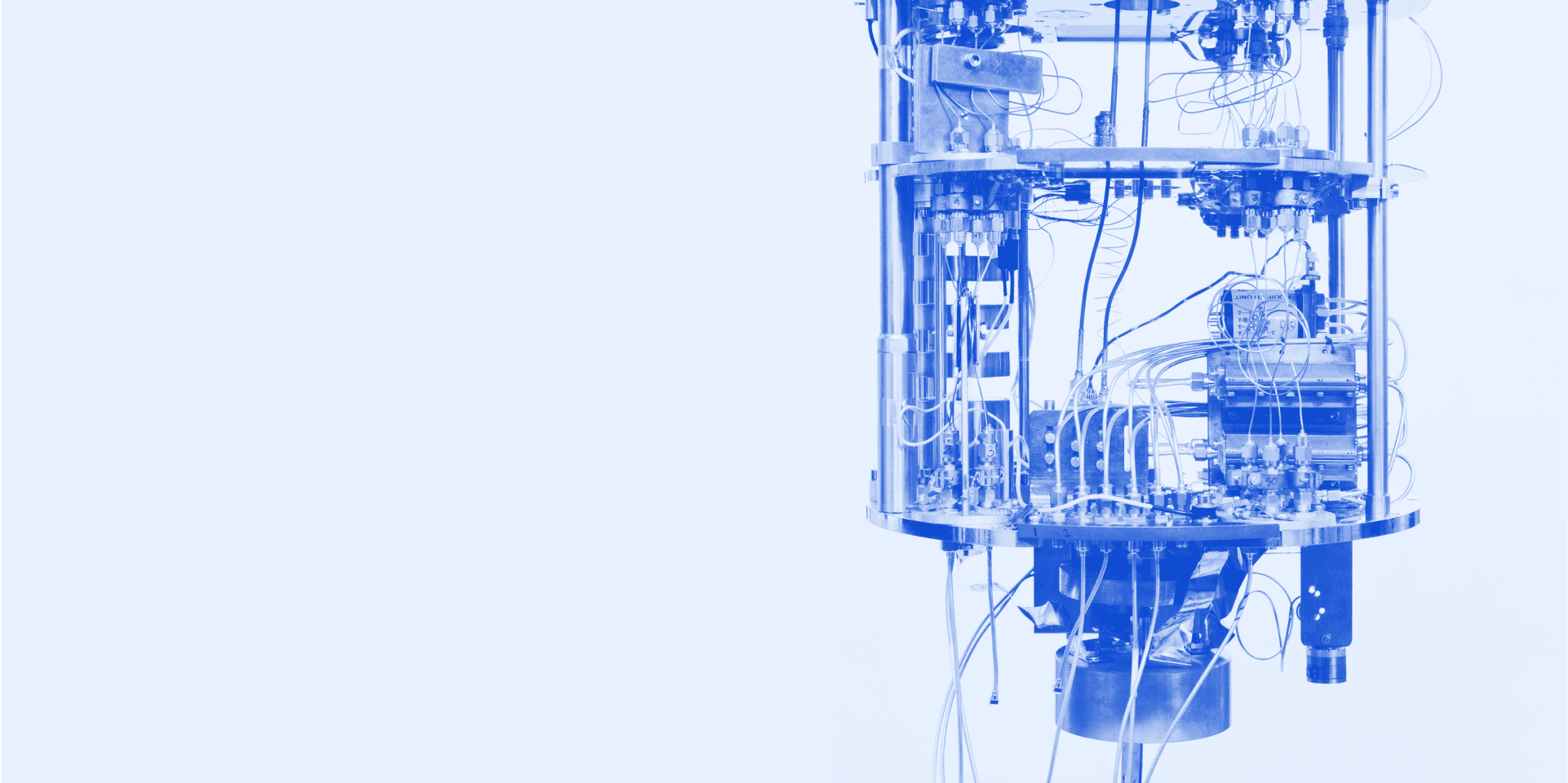
Qiskit: Open-Source Quantum Development
Qiskit is an open-source SDK for working with quantum computers at the level of pulses, circuits, and application modules.
Publication collections
Acs spring 2024.
American Chemical Society (ACS) Spring Meeting
APS March Meeting 2024
American Physical Society (March Meeting)
Quantum Information Processing Conference
ACS Fall 2023
American Chemical Society (ACS) Fall Meeting
- Quantum Error Correction
- Quantum Finance
- Quantum Hardware
- Quantum Information Science
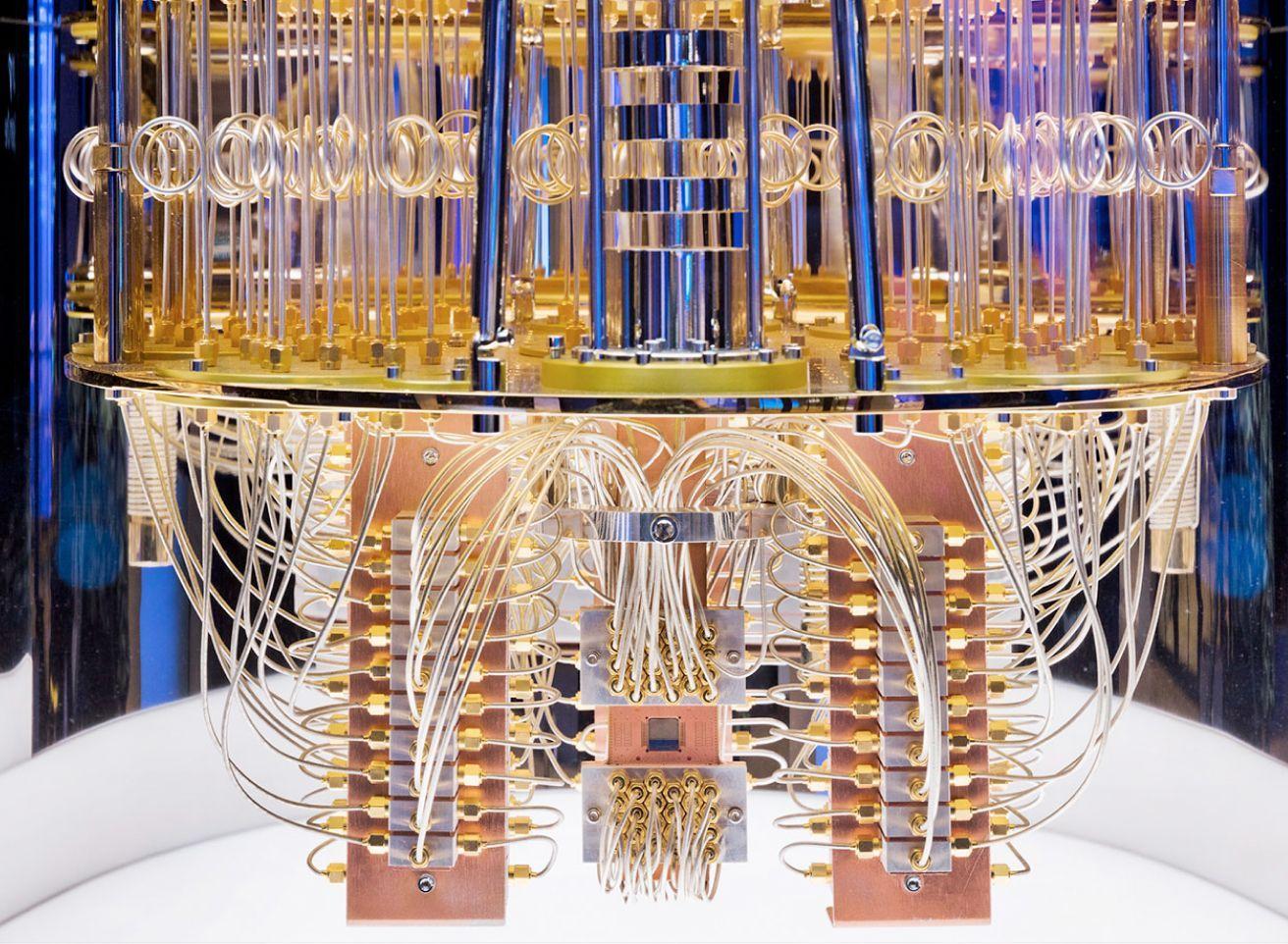
Collaborate with us
IBM Quantum Network is a community of Fortune 500 companies, academic institutions, startups and national research labs working with IBM to advance quantum computing.
- IBM Quantum Network

Breakthrough promises secure quantum computing at home
The full power of next-generation quantum computing could soon be harnessed by millions of individuals and companies, thanks to a breakthrough by scientists at Oxford University Physics guaranteeing security and privacy. This advance promises to unlock the transformative potential of cloud-based quantum computing and is detailed in a new study published in the influential U.S. scientific journal Physical Review Letters .
Quantum computing is developing rapidly, paving the way for new applications which could transform services in many areas like healthcare and financial services. It works in a fundamentally different way to conventional computing and is potentially far more powerful. However, it currently requires controlled conditions to remain stable and there are concerns around data authenticity and the effectiveness of current security and encryption systems.
Several leading providers of cloud-based services, like Google, Amazon, and IBM, already separately offer some elements of quantum computing. Safeguarding the privacy and security of customer data is a vital precursor to scaling up and expending its use, and for the development of new applications as the technology advances. The new study by researchers at Oxford University Physics addresses these challenges.
"We have shown for the first time that quantum computing in the cloud can be accessed in a scalable, practical way which will also give people complete security and privacy of data, plus the ability to verify its authenticity," said Professor David Lucas, who co-heads the Oxford University Physics research team and is lead scientist at the UK Quantum Computing and Simulation Hub, led from Oxford University Physics.
In the new study, the researchers use an approach dubbed "blind quantum computing," which connects two totally separate quantum computing entities -- potentially an individual at home or in an office accessing a cloud server -- in a completely secure way. Importantly, their new methods could be scaled up to large quantum computations.
"Using blind quantum computing, clients can access remote quantum computers to process confidential data with secret algorithms and even verify the results are correct, without revealing any useful information. Realising this concept is a big step forward in both quantum computing and keeping our information safe online'' said study lead Dr Peter Drmota, of Oxford University Physics.
The researchers created a system comprising a fibre network link between a quantum computing server and a simple device detecting photons, or particles of light, at an independent computer remotely accessing its cloud services. This allows so-called blind quantum computing over a network. Every computation incurs a correction which must be applied to all that follow and needs real-time information to comply with the algorithm. The researchers used a unique combination of quantum memory and photons to achieve this.
"Never in history have the issues surrounding privacy of data and code been more urgently debated than in the present era of cloud computing and artificial intelligence," said Professor David Lucas. "As quantum computers become more capable, people will seek to use them with complete security and privacy over networks, and our new results mark a step change in capability in this respect."
The results could ultimately lead to commercial development of devices to plug into laptops, to safeguard data when people are using quantum cloud computing services.
Researchers exploring quantum computing and technologies at Oxford University Physics have access to the state-of-the-art Beecroft laboratory facility, specially constructed to create stable and secure conditions including eliminating vibration.
Funding for the research came from the UK Quantum Computing and Simulation (QCS) Hub, with scientists from the UK National Quantum Computing Centre, the Paris-Sorbonne University, the University of Edinburgh, and the University of Maryland, collaborating on the work.
- Computers and Internet
- Quantum Computers
- Information Technology
- Distributed Computing
- Computer Science
- Spintronics Research
- Quantum computer
- Quantum entanglement
- Computing power everywhere
- Grid computing
- Quantum tunnelling
- Computer security
- Quantum dot
Story Source:
Materials provided by University of Oxford . Note: Content may be edited for style and length.
Journal Reference :
- P. Drmota, D. P. Nadlinger, D. Main, B. C. Nichol, E. M. Ainley, D. Leichtle, A. Mantri, E. Kashefi, R. Srinivas, G. Araneda, C. J. Ballance, D. M. Lucas. Verifiable Blind Quantum Computing with Trapped Ions and Single Photons . Physical Review Letters , 2024; 132 (15) DOI: 10.1103/PhysRevLett.132.150604
Cite This Page :
Explore More
- Plastic Pollution Kills Ocean Embryos
- Most Massive Stellar Black Hole in Our Galaxy
- Coffee's Prehistoric Origin and It's Future
- Can Animals Count? New Rat Study
- A Single Atom Layer of Gold: Goldene
- Fool's Gold May Contain Valuable Lithium
- Exercise Cuts Stress-Related Brain Activity
- Microplastics Go from Gut to Other Organs
- Epilepsy Drug May Prevent Brain Tumors
- Evolution's Recipe Book
Trending Topics
Strange & offbeat.
- IEEE CS Standards
- Career Center
- Subscribe to Newsletter
- IEEE Standards
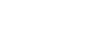
- For Industry Professionals
- For Students
- Launch a New Career
- Membership FAQ
- Membership FAQs
- Membership Grades
- Special Circumstances
- Discounts & Payments
- Distinguished Contributor Recognition
- Grant Programs
- Find a Local Chapter
- Find a Distinguished Visitor
- Find a Speaker on Early Career Topics
- Technical Communities
- Collabratec (Discussion Forum)
- Start a Chapter
- My Subscriptions
- My Referrals
- Computer Magazine
- ComputingEdge Magazine
- Let us help make your event a success. EXPLORE PLANNING SERVICES
- Events Calendar
- Calls for Papers
- Conference Proceedings
- Conference Highlights
- Top 2024 Conferences
- Conference Sponsorship Options
- Conference Planning Services
- Conference Organizer Resources
- Virtual Conference Guide
- Get a Quote
- CPS Dashboard
- CPS Author FAQ
- CPS Organizer FAQ
- Find the latest in advanced computing research. VISIT THE DIGITAL LIBRARY
- Open Access
- Tech News Blog
- Author Guidelines
- Reviewer Information
- Guest Editor Information
- Editor Information
- Editor-in-Chief Information
- Volunteer Opportunities
- Video Library
- Member Benefits
- Institutional Library Subscriptions
- Advertising and Sponsorship
- Code of Ethics
- Educational Webinars
- Online Education
- Certifications
- Industry Webinars & Whitepapers
- Research Reports
- Bodies of Knowledge
- CS for Industry Professionals
- Resource Library
- Newsletters
- Women in Computing
- Digital Library Access
- Organize a Conference
- Run a Publication
- Become a Distinguished Speaker
- Participate in Standards Activities
- Peer Review Content
- Author Resources
- Publish Open Access
- Society Leadership
- Boards & Committees
- Local Chapters
- Governance Resources
- Conference Publishing Services
- Chapter Resources
- About the Board of Governors
- Board of Governors Members
- Diversity & Inclusion
- Open Volunteer Opportunities
- Award Recipients
- Student Scholarships & Awards
- Nominate an Election Candidate
- Nominate a Colleague
- Corporate Partnerships
- Conference Sponsorships & Exhibits
- Advertising
- Recruitment
- Publications
- Education & Career
The Current State of Quantum Machine Learning
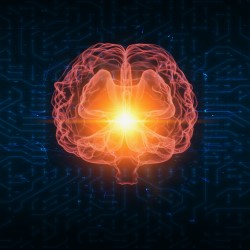
Given the recent advances in quantum computing, researchers attending the 2023 IEEE International Conference on Quantum Computing and Engineering (QCE) investigated how to expand the number of use cases for QML in the workshop ‘ Quantum Machine Learning: From Foundations to Applications .’
Researchers Volker Tresp, Steffen Udluft, Daniel Hein, and Werner Hauptmann from Siemens AG, Technology in Munich, Germany, Martin Leib from IQM in Munich, Christopher Mutschler and Daniel D. Scherer from Fraunhofer IIS in Nuremberg, and Wolfgang Mauerer of Technical University of Applied Sciences Regensburg Siemens AG, Technology in Regensburg/Munich, Germany outlined the purpose and outcomes of the workshop.
The Goals of the Workshop
The workshop brought together researchers and industry practitioners from AI, ML, software and systems engineering, physics, and other disciplines to discuss the challenges QML currently faces and how it can be used to support the advancement of QML as an effective tool with diverse applications.
Currently, QML shows the potential to operate using fewer data points than traditional ML, as is the case with quantum support vector machines.
At the same time, subtle issues present limitations. Many of the challenges stem from the fact that quantum computers are sensitive to noise, and since qubits can assume an infinite number of states, errors can be hard to correct.
To best address QML’s challenges, the researchers felt it was most suitable to raise the topic in the quantum community instead of the ML community, which is already crowded with discourse. This made IEEE’s International Conference on Quantum Computing and Engineering (QCE) an ideal venue for the workshop.
A Call for Contributions
To bring more ideas to the table and encourage a diverse, international array of voices, the team encouraged participants to submit four different kinds of papers:
- Research papers
- System papers
- Experiments and analysis papers
- Application papers
The desired outcomes included:
- Publishing, presenting, and discussing research
- Allowing participants to form interest groups
- Enhance understanding regarding how to compare QML solutions holistically
- Identify research and application opportunities for QML
- Form relationships between those from industry and those from academia around the potential of QML
- Share ideas between the machine learning and quantum computing communities.
The target audience for the workshop was broad: Anyone interested in learning about the challenges faced and opportunities presented by QML, regardless of whether they hail from computer science, physics, or engineering.
Eight Papers Accepted
The team used a triple-anonymous peer review process that evaluated submissions according to their relevance, novelty, technical soundness, appropriateness, depth of literary coverage, and presentation.
The process resulted in eight papers being accepted. These addressed three primary topics:
- Applications of QML, such as for sentiment analysis in the finance sector
- Quantum reinforcement learning can improve sampling efficiency, optimize circuit compilation, and involve multiple quantum processing units (QPUs) in distributed training.
- General quantum machine learning, including computing the principle components of a matrix, Betti numbers, and persistent Bettis numbers.
Results: An Accurate Picture of the Current State of QML
When looked at as a whole, the papers depict an accurate vista of the QML field. It’s still very difficult to execute unconditional speedups in a QML setting due to a lack of understanding regarding QML and the wide range of approaches already driving classical ML systems.
The small size, as well as the noise and imperfections of existing QML machines, makes it difficult to extrapolate larger-scale uses of QML.
These difficulties are exacerbated by a few different factors. Classical ML research is based on computational models and doesn’t depend on physical structures, while QML needs to be run and tested in a physical environment due to a lack of reliable error correction techniques. In addition, the relatively small size of the QML community limits the number of people available to address these challenges, which inhibits the pace of progress.
At the same time, the workshop was a significant step forward towards overcoming these and other challenges. It surfaced papers that broadened the understanding of QML’s potential and united stakeholders from a variety of backgrounds around the advancement of QML. For a deeper dive into the workshop and the contributions of participants and authors, download the full paper
Download Report
" * " indicates required fields
Registration is now open for IEEE International Conference on Quantum Computing and Engineering (QCE)
Join us 15-20 September at the Palais des Congrès in Montreal for a transformative week of Quantum Computing. Meet and learn from leading researchers, innovators, and peers in this dynamic field. Don’t just watch the future unfold—be a part of it. REGISTER NOW and mark your calendars for IEEE International Conference on Quantum Computing and Engineering (QCE)
Recommended by IEEE Computer Society
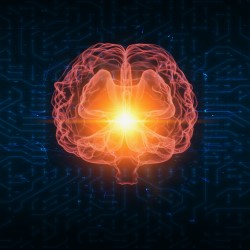
Unveiling the Challenges of Quantum Software Engineering: A Developer's Perspective
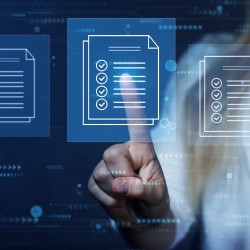
Demystifying Quantum Software Development: A Look at Requirements Engineering
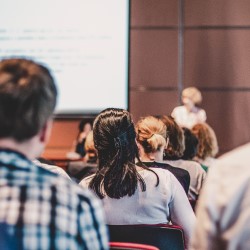
Toward a Holistic STEM Faculty Development Research Agenda
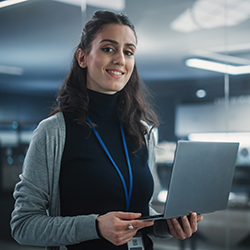
Engineering Doctoral Students: Identity Formation and Career Support
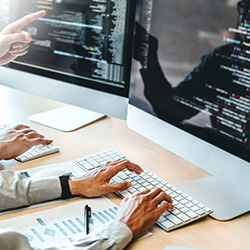
A Question (and an Answer) about Expertise in the Stack Overflow Universe
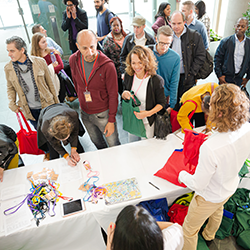
Diversity in Computing: The Role of Interinstitutional Faculty Learning Communities
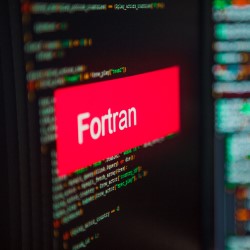
Modernizing the Fortran Ecosystem
Thank you for visiting nature.com. You are using a browser version with limited support for CSS. To obtain the best experience, we recommend you use a more up to date browser (or turn off compatibility mode in Internet Explorer). In the meantime, to ensure continued support, we are displaying the site without styles and JavaScript.
- View all journals
- Explore content
- About the journal
- Publish with us
- Sign up for alerts
- Review Article
- Published: 04 March 2010
Quantum computers
- T. D. Ladd 1 nAff11 ,
- F. Jelezko 2 ,
- R. Laflamme 3 , 4 , 5 ,
- Y. Nakamura 6 , 7 ,
- C. Monroe 8 , 9 &
- J. L. O’Brien 10
Nature volume 464 , pages 45–53 ( 2010 ) Cite this article
78k Accesses
2491 Citations
60 Altmetric
Metrics details
- Computer science
- Quantum information
- Quantum mechanics
Over the past several decades, quantum information science has emerged to seek answers to the question: can we gain some advantage by storing, transmitting and processing information encoded in systems that exhibit unique quantum properties? Today it is understood that the answer is yes, and many research groups around the world are working towards the highly ambitious technological goal of building a quantum computer, which would dramatically improve computational power for particular tasks. A number of physical systems, spanning much of modern physics, are being developed for quantum computation. However, it remains unclear which technology, if any, will ultimately prove successful. Here we describe the latest developments for each of the leading approaches and explain the major challenges for the future.
This is a preview of subscription content, access via your institution
Access options
Subscribe to this journal
Receive 51 print issues and online access
185,98 € per year
only 3,65 € per issue
Buy this article
- Purchase on Springer Link
- Instant access to full article PDF
Prices may be subject to local taxes which are calculated during checkout
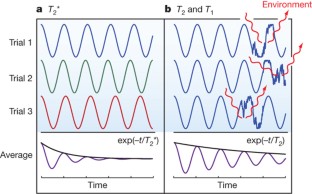
Similar content being viewed by others
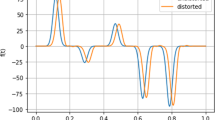
QDataSet, quantum datasets for machine learning
Elija Perrier, Akram Youssry & Chris Ferrie
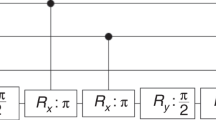
Quantum advantage for computations with limited space
Dmitri Maslov, Jin-Sung Kim, … Sarah Sheldon
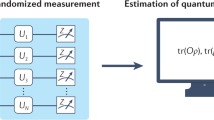
The randomized measurement toolbox
Andreas Elben, Steven T. Flammia, … Peter Zoller
Nielsen, M. A. & Chuang, I. L. Quantum Computation and Quantum Information (Cambridge University Press, 2000)
MATH Google Scholar
Knill, E. Quantum computing with realistically noisy devices. Nature 434 , 39–44 (2005)
ADS CAS PubMed Google Scholar
DiVincenzo, D. P. The physical implementation of quantum computation. Fortschr. Phys. 48 , 771–783 (2000)
Mizel, A., Lidar, D. A. & Mitchell, M. Simple proof of equivalence between adiabatic quantum computation and the circuit model. Phys. Rev. Lett. 99 , 070502 (2007)
ADS PubMed Google Scholar
Raussendorf, R. & Briegel, H. J. A one-way quantum computer. Phys. Rev. Lett. 86 , 5188–5191 (2001)
Cory, D. G., Fahmy, A. F. & Havel, T. F. Ensemble quantum computing by NMR-spectroscopy. Proc. Natl Acad. Sci. USA 94 , 1634–1639 (1997)
ADS CAS PubMed PubMed Central Google Scholar
Gershenfeld, N. A. & Chuang, I. L. Bulk spin resonance quantum computation. Science 275 , 350–356 (1997)
MathSciNet CAS PubMed MATH Google Scholar
Ryan, C. A., Moussa, O., Baugh, J. & Laflamme, R. Spin based heat engine: demonstration of multiple rounds of algorithmic cooling. Phys. Rev. Lett. 100 , 140501 (2008)
Shor, P. W. & Jordan, S. P. Estimating Jones polynomials is a complete problem for one clean qubit. Quant. Inform. Comput. 8 , 681–714 (2008)
MathSciNet MATH Google Scholar
Braunstein, S. L. & van Loock, P. Quantum information with continuous variables. Rev. Mod. Phys. 77 , 513–577 (2005)
ADS MathSciNet MATH Google Scholar
Schmidt, H. & Imamoglu, A. Giant Kerr nonlinearities obtained by electromagnetically induced transparency. Opt. Lett. 21 , 1936–1938 (1996)
Duan, L. M. & Kimble, H. J. Scalable photonic quantum computation through cavity-assisted interactions. Phys. Rev. Lett. 92 , 127902 (2004)
Knill, E., Laflamme, R. & Milburn, G. J. A scheme for efficient quantum computation with linear optics. Nature 409 , 46–52 (2001)
Politi, A., Matthews, J. C. F. & O’Brien, J. L. Shor’s quantum factoring algorithm on a photonic chip. Science 325 , 1221 (2009)
ADS MathSciNet CAS PubMed MATH Google Scholar
O’Brien, J. L. Optical quantum computing. Science 318 , 1567–1570 (2007)
Migdal, A. & Dowling, J. eds. Single-photon detectors, applications, and measurement. J. Mod. Opt. 51 , (2004)
Hadfield, R. H. Single-photon detectors for optical quantum information applications. Nature Photon. 3 , 696–705 (2009)
ADS CAS Google Scholar
Grangier, P., Sanders, B. & Vuckovic, J. eds. Focus on single photons on demand. New J. Phys. 6 , (2004)
Shields, A. J. Semiconductor quantum light sources. Nature Photon. 1 , 215–223 (2007)
Matthews, J. C. F., Politi, A., Stefanov, A. & O’Brien, J. L. Manipulation of multiphoton entanglement in waveguide quantum circuits. Nature Photon. 3 , 346–350 (2009)
Kistner, C. et al. Demonstration of strong coupling via electro-optical tuning in high-quality QD-micropillar systems. Opt. Express 16 , 15006–15012 (2008)
Fushman, I. et al. Controlled phase shifts with a single quantum dot. Science 320 , 769–772 (2008)
Gruber, A. et al. Scanning confocal optical microscopy and magnetic resonance on single defect centers. Science 276 , 2012–2014 (1997)
CAS Google Scholar
Devitt, S. J. et al. Photonic module: an on-demand resource for photonic entanglement. Phys. Rev. A 76 , 052312 (2007)
ADS Google Scholar
Wineland, D. J. et al. Experimental issues in coherent quantum-state manipulation of trapped atomic ions. J. Res. Natl. Inst. Stand. Technol. 103 , 259–328 (1998)
CAS PubMed PubMed Central Google Scholar
Wineland, D. & Blatt, R. Entangled states of trapped atomic ions. Nature 453 , 1008–1014 (2008)
Ospelkaus, C. et al. Trapped-ion quantum logic gates based on oscillating magnetic fields. Phys. Rev. Lett. 101 , 090502 (2008)
Garcia-Ripoll, J. J., Zoller, P. & Cirac, J. I. Speed optimized two-qubit gates with laser coherent control techniques for ion trap quantum computing. Phys. Rev. Lett. 91 , 157901 (2003)
Leibfried, D., Blatt, R., Monroe, C. & Wineland, D. Quantum dynamics of single trapped ions. Rev. Mod. Phys. 75 , 281–324 (2003)
Home, J. P. et al. Complete methods set for scalable ion trap quantum information processing. Science 325 , 1227–1230 (2009)
Olmschenk, S. et al. Quantum teleportation between distant matter qubits. Science 323 , 486–489 (2009)
Dür, W., Briegel, H. J., Cirac, J. I. & Zoller, P. Quantum repeaters based on entanglement purification. Phys. Rev. A 59 , 169–181 (1999)
Duan, L.-M. & Raussendorf, R. Efficient quantum computation with probabilistic quantum gates. Phys. Rev. Lett. 95 , 080503 (2005)
ADS MathSciNet PubMed Google Scholar
Morsch, O. & Oberthaler, M. Dynamics of Bose-Einstein condensates in optical lattices. Rev. Mod. Phys. 78 , 179–215 (2006)
Anderlini, M. et al. Controlled exchange interaction between pairs of neutral atoms in an optical lattice. Nature 448 , 452–456 (2007)
Urban, E. et al. Observation of Rydberg blockade between two atoms. Nature Phys. 5 , 110–114 (2009)
Gaëtan, A. et al. Observation of collective excitation of two individual atoms in the Rydberg blockade regime. Nature Phys. 5 , 115–118 (2009)
Negrevergne, C. et al. Benchmarking quantum control methods on a 12-qubit system. Phys. Rev. Lett. 96 , 170501 (2006)
Vandersypen, L. M. K. et al. Experimental realization of Shor’s quantum factoring algorithm using nuclear magnetic resonance. Nature 414 , 883–887 (2001)
Khaneja, N., Reiss, T., Kehlet, C., Schulte-Herbruggen, T. & Glaser, S. J. Optimal control of coupled spin dynamics: design of NMR pulse sequences by gradient ascent algorithms. J. Magn. Reson. 172 , 296–305 (2005)
Braunstein, S. L. et al. Separability of very noisy mixed states and implications for NMR quantum computing. Phys. Rev. Lett. 83 , 1054–1057 (1999)
Mehring, M., Mende, J. & Scherer, W. Entanglement between an electron and a nuclear spin 1/2. Phys. Rev. Lett. 90 , 153001 (2003)
Hanson, R., Kouwenhoven, L. P., Petta, J. R., Tarucha, S. & Vandersypen, L. M. K. Spins in few-electron quantum dots. Rev. Mod. Phys. 79 , 1217–1265 (2007)
Uhrig, S. G. Keeping a quantum bit alive by optimized π-pulse sequences. Phys. Rev. Lett. 98 , 100504 (2007)
Liu, H. W. et al. A gate-defined silicon quantum dot molecule. Appl. Phys. Lett. 92 , 222104 (2008)
Simmons, C. B. et al. Charge sensing and controllable tunnel coupling in a Si/SiGe double quantum dot. Nano Lett. 9 , 3234–3238 (2009)
Kane, B. E. A silicon-based nuclear spin quantum computer. Nature 393 , 133–137 (1998)
Vrijen, R. et al. Electron-spin-resonance transistors for quantum computing in silicon-germanium heterostructures. Phys. Rev. A 62 , 012306 (2000)
Tyryshkin, A. M. & Lyon, S. A. Data presented at the Silicon Qubit Workshop, 24–25 August (University of California, Berkeley; sponsored by Lawrence Berkeley National Laboratory and Sandia National Laboratory, 2009)
Ladd, T. D., Maryenko, D., Yamamoto, Y., Abe, E. & Itoh, K. M. Coherence time of decoupled nuclear spins in silicon. Phys. Rev. B 71 , 14401 (2005)
Yang, A. et al. Simultaneous subsecond hyperpolarization of the nuclear and electron spins of phosphorus in silicon by optical pumping of exciton transitions. Phys. Rev. Lett. 102 , 257401 (2009)
Batra, A., Weis, C. D., Reijonen, J., Persaud, A. & Schenkel, T. Detection of low energy single ion impacts in micron scale transistors at room temperature. Appl. Phys. Lett. 91 , 193502 (2007)
O’Brien, J. L. et al. Towards the fabrication of phosphorus qubits for a silicon quantum computer. Phys. Rev. B 64 , 161401 (2001)
Schneider, C. et al. Lithographic alignment to site-controlled quantum dots for device integration. Appl. Phys. Lett. 92 , 183101 (2008)
Atatüre, M. et al. Quantum-dot spin-state preparation with near-unity fidelity. Science 312 , 551–553 (2006)
Gerardot, B. D. et al. Optical pumping of a single hole spin in a quantum dot. Nature 451 , 441–444 (2008)
Press, D., Ladd, T. D., Zhang, B. Y. & Yamamoto, Y. Complete quantum control of a single quantum dot spin using ultrafast optical pulses. Nature 456 , 218–221 (2008)
Berezovsky, J. et al. Nondestructive optical measurements of a single electron spin in a quantum dot. Science 314 , 1916–1920 (2006)
Harrison, J., Sellars, M. J. & Manson, N. B. Measurement of the optically induced spin polarisation of N-V centres in diamond. Diamond Related Mater. 15 , 586–588 (2006)
Dutt, M. V. G. et al. Quantum register based on individual electronic and nuclear spin qubits in diamond. Science 316 , 1312–1316 (2007)
PubMed Google Scholar
Neumann, P. et al. Multipartite entanglement among single spins in diamond. Science 320 , 1326–1329 (2008)
Jiang, L. et al. Repetitive readout of a single electronic spin via quantum logic with nuclear spin ancillae. Science 326 , 267–272 (2009)
Hanson, R., Dobrovitski, V. V., Feiguin, A. E., Gywat, O. & Awschalom, D. D. Coherent dynamics of a single spin interacting with an adjustable spin bath. Science 320 , 352–355 (2008)
Takahashi, S., Hanson, R., van Tol, J., Sherwin, M. S. & Awschalom, D. D. Quenching spin decoherence in diamond through spin bath polarization. Phys. Rev. Lett. 101 , 047601 (2008)
Balasubramanian, G. et al. Ultralong spin coherence time in isotopically engineered diamond. Nature Mater. 8 , 383–387 (2009)
Neumann, P. et al. Scalable quantum register based on coupled electron spins in a room temperature solid. Nature Phys. 10.1038/nphys1536 (in the press)
Wang, C. F. et al. Fabrication and characterization of two-dimensional photonic crystal microcavities in nanocrystalline diamond. Appl. Phys. Lett. 91 , 201112 (2007)
Wu, E. et al. Room temperature triggered single-photon source in the near infrared. New J. Phys. 9 , 434 (2007)
Wang, C., Kurtsiefer, C., Weinfurter, H. & Burchard, B. Single photon emission from SiV centres in diamond produced by ion implantation. J. Phys. At. Mol. Opt. Phys. 39 , 37–41 (2006)
Sanaka, K., Pawlis, A., Ladd, T. D., Lischka, K. & Yamamoto, Y. Indistinguishable photons from independent semiconductor nanostructures. Phys. Rev. Lett. 103 , 053601 (2009)
Nakamura, Y., Pashkin, Yu. A. & Tsai, J. S. Coherent control of macroscopic quantum states in a single-Cooper-pair box. Nature 398 , 786–788 (1999)
Vion, D. et al. Manipulating the quantum state of an electrical circuit. Science 296 , 886–889 (2002)
Schreier, J. A. et al. Suppressing charge noise decoherence in superconducting charge qubits. Phys. Rev. B 77 , 180502 (2008)
Chiorescu, I., Nakamura, Y., Harmans, C. J. P. M. & Mooij, J. E. Coherent quantum dynamics of a superconducting flux qubit. Science 299 , 1869–1871 (2003)
Martinis, J. M., Nam, S., Aumentado, J. & Urbina, C. Rabi oscillations in a large Josephson-junction qubit. Phys. Rev. Lett. 89 , 117901 (2002)
Niskanen, A. O. et al. Quantum coherent tunable coupling of superconducting qubits. Science 316 , 723–726 (2007)
Harris, R. et al. Experimental demonstration of a robust and scalable flux qubit. Preprint at 〈 http://arxiv.org/abs/0909.4321 〉 (2009)
Wallraff, A. et al. Strong coupling of a single photon to a superconducting qubit using circuit quantum electrodynamics. Nature 431 , 162–167 (2004)
DiCarlo, L. et al. Demonstration of two-qubit algorithms with a superconducting quantum processor. Nature 260 , 240–244 (2009)
Ansmann, M. et al. Violation of Bell’s inequality in Josephson phase qubits. Nature 461 , 504–506 (2009)
Chow, J. M. et al. Entanglement metrology using a joint readout of superconducting qubits. Preprint at 〈 http://arxiv.org/abs/0908.1955 〉 (2009)
Lupascu, A. et al. Quantum non-demolition measurement of a superconducting two-level system. Nature Phys. 3 , 119–123 (2007)
Micheli, A., Brennen, G. K. & Zoller, P. A toolbox for lattice-spin models with polar molecules. Nature Phys. 2 , 341–347 (2006)
Rippe, L., Julsgaard, B., Walther, A., Ying, Y. & Kroll, S. Experimental quantum-state tomography of a solid-state qubit. Phys. Rev. A 77 , 022307 (2008)
de Riedmatten, H., Afzelius, M., Staudt, M. U., Simon, C. & Gisin, N. A solid-state light-matter interface at the single-photon level. Nature 456 , 773–777 (2008)
Morton, J. J. L. et al. Bang-bang control of fullerene qubits using ultrafast phase gates. Nature Phys. 2 , 40–43 (2006)
Mason, N., Biercuk, M. J. & Marcus, C. M. Local gate control of a carbon nanotube double quantum dot. Science 303 , 655–658 (2004)
Trauzettel, B., Bulaev, D. V., Loss, D. & Burkard, G. Spin qubits in graphene quantum dots. Nature Phys. 3 , 192–196 (2007)
Platzman, P. M. & Dykman, M. I. Quantum computing with electrons floating on liquid helium. Science 284 , 1967–1969 (1999)
CAS PubMed Google Scholar
Leuenberger, M. N. & Loss, D. Quantum computing in molecular magnets. Nature 410 , 789–793 (2001)
Tian, L., Rabl, P., Blatt, R. & Zoller, P. Interfacing quantum-optical and solid-state qubits. Phys. Rev. Lett. 92 , 247902 (2004)
Andre, A. et al. A coherent all-electrical interface between polar molecules and mesoscopic superconducting resonators. Nature Phys. 2 , 636–642 (2006)
Recher, P., Sukhorukov, E. V. & Loss, D. Andreev tunneling, Coulomb blockade, and resonant transport of nonlocal spin-entangled electrons. Phys. Rev. B 63 , 165314 (2001)
Privman, V., Vagner, I. D. & Kventsel, G. Quantum computation in quantum-Hall systems. Phys. Lett. A 239 , 141–146 (1998)
ADS MathSciNet CAS MATH Google Scholar
Smelyanskiy, V. N., Petukhov, A. G. & Osipov, V. V. Quantum computing on long-lived donor states of Li in Si. Phys. Rev. B 72 , 081304 (2005)
Tian, L. & Zoller, P. Coupled ion-nanomechanical systems. Phys. Rev. Lett. 93 , 266403 (2004)
Piermarocchi, C., Chen, P., Sham, L. J. & Steel, D. G. Optical RKKY interaction between charged semiconductor quantum dots. Phys. Rev. Lett. 89 , 167402 (2002)
Quinteiro, G. F., Fernandez-Rossier, J. & Piermarocchi, C. Long-range spin-qubit interaction mediated by microcavity polaritons. Phys. Rev. Lett. 97 , 097401 (2006)
Khitun, A., Ostroumov, R. & Wang, K. L. Spin-wave utilization in a quantum computer. Phys. Rev. A 64 , 062304 (2001)
Barnes, C. H. W., Shilton, J. M. & Robinson, A. M. Quantum computation using electrons trapped by surface acoustic waves. Phys. Rev. B 62 , 8410–8419 (2000)
Chang, D. E., Sørensen, A. S., Hemmer, P. R. & Lukin, M. D. Quantum optics with surface plasmons. Phys. Rev. Lett. 97 , 053002 (2006)
Raussendorf, R. & Harrington, J. Fault-tolerant quantum computation with high threshold in two dimensions. Phys. Rev. Lett. 98 , 190504 (2007)
Nayak, C., Simon, S. H., Stern, A., Freedman, M. & Das Sarma, S. Non-abelian anyons and topological quantum computation. Rev. Mod. Phys. 80 , 1083–1159 (2008)
Langer, C. et al. Long-lived qubit memory using atomic ions. Phys. Rev. Lett. 95 , 060502 (2005)
Knill, E. et al. Randomized benchmarking of quantum gates. Phys. Rev. A 77 , 012307 (2008)
Benhelm, J., Kirchmair, G., Roos, C. F. & Blatt, R. Towards fault-tolerant quantum computing with trapped ions. Nature Phys. 4 , 463–466 (2008)
Treutlein, P., Hommelhoff, P., Steinmetz, T., Hänsch, T. W. & Reichel, J. Coherence in microchip traps. Phys. Rev. Lett. 92 , 203005 (2004)
Ryan, C. A., Laforest, M. & Laflamme, R. Randomized benchmarking of single- and multi-qubit control in liquid-state NMR quantum information processing. New J. Phys. 11 , 013034 (2009)
Bertet, P. et al. Dephasing of a superconducting qubit induced by photon noise. Phys. Rev. Lett. 95 , 257002 (2005)
Emerson, J. et al. Symmetrized characterization of noisy quantum processes. Science 317 , 1893–1896 (2007)
Hanson, R. & Awschalom, D. D. Coherent manipulation of single spins in semiconductors. Nature 453 , 1043–1049 (2008)
Download references
Acknowledgements
We thank R. Hanson, M. D. Lukin, and W. D. Oliver for comments. We acknowledge support from NSF, EPSRC, QIP IRC, IARPA, ERC, the Leverhulme Trust, CREST-JST, DFG, BMBF and Landesstiftung BW. J.L.O’B. acknowledges a Royal Society Wolfson Merit Award.
Author Contributions All authors contributed to all aspects of this work.
Author information
Present address: Present address: HRL Laboratories, LLC, 3011 Malibu Canyon Road, Malibu, California 90265, USA.,
Authors and Affiliations
Edward L. Ginzton Laboratory, Stanford University, Stanford, California 94305-4088, USA ,
3. Physikalisches Institut, Universität Stuttgart, Pfaffenwaldring 57, D-70550, Germany ,
Institute for Quantum Computing,,
R. Laflamme
Department of Physics and Astronomy, University of Waterloo, 200 University Avenue West, Waterloo, Ontario, N2L 3G1, Canada,
Perimeter Institute, 31 Caroline Street North, Waterloo, Ontario, N2L 2Y5, Canada ,
Nano Electronics Research Laboratories, NEC Corporation, Tsukuba, Ibaraki 305-8501, Japan ,
Y. Nakamura
The Institute of Physical and Chemical Research (RIKEN), Wako, Saitama 351-0198, Japan ,
University of Maryland Department of Physics,, Joint Quantum Institute,
National Institute of Standards and Technology, College Park, Maryland 20742, USA ,
H. H. Wills Physics Laboratory and Department of Electrical and Electronic Engineering, Centre for Quantum Photonics, University of Bristol, Merchant Venturers Building, Woodland Road, Bristol, BS8 1UB, UK,
J. L. O’Brien
You can also search for this author in PubMed Google Scholar
Corresponding author
Correspondence to J. L. O’Brien .
Ethics declarations
Competing interests.
The authors declare no competing financial interests.
PowerPoint slides
Powerpoint slide for fig. 1, powerpoint slide for fig. 2, powerpoint slide for fig. 3, powerpoint slide for fig. 4, powerpoint slide for fig. 5, rights and permissions.
Reprints and permissions
About this article
Cite this article.
Ladd, T., Jelezko, F., Laflamme, R. et al. Quantum computers. Nature 464 , 45–53 (2010). https://doi.org/10.1038/nature08812
Download citation
Issue Date : 04 March 2010
DOI : https://doi.org/10.1038/nature08812
Share this article
Anyone you share the following link with will be able to read this content:
Sorry, a shareable link is not currently available for this article.
Provided by the Springer Nature SharedIt content-sharing initiative
This article is cited by
An integrated microwave-to-optics interface for scalable quantum computing.
- Matthew J. Weaver
- Pim Duivestein
- Robert Stockill
Nature Nanotechnology (2024)
Quantum-walk search in motion
- Himanshu Sahu
Scientific Reports (2024)
Thermal multiferroics in all-inorganic quasi-two-dimensional halide perovskites
- Xue-Zeng Lu
- Hiroshi Kageyama
Nature Materials (2024)
Electron charge qubit with 0.1 millisecond coherence time
- Xianjing Zhou
Nature Physics (2024)
Heralded entanglement between error-protected logical qubits for fault-tolerant distributed quantum computing
Science China Physics, Mechanics & Astronomy (2024)
By submitting a comment you agree to abide by our Terms and Community Guidelines . If you find something abusive or that does not comply with our terms or guidelines please flag it as inappropriate.
Quick links
- Explore articles by subject
- Guide to authors
- Editorial policies
Sign up for the Nature Briefing: AI and Robotics newsletter — what matters in AI and robotics research, free to your inbox weekly.


- Follow us on Facebook
- Follow us on Twitter
- Follow us on LinkedIn
- Watch us on Youtube
- Latest Explore all the latest news and information on Physics World
- Research updates Keep track of the most exciting research breakthroughs and technology innovations
- News Stay informed about the latest developments that affect scientists in all parts of the world
- Features Take a deeper look at the emerging trends and key issues within the global scientific community
- Opinion and reviews Find out whether you agree with our expert commentators
- Interviews Discover the views of leading figures in the scientific community
- Analysis Discover the stories behind the headlines
- Blog Enjoy a more personal take on the key events in and around science
- Physics World Live
- Impact Explore the value of scientific research for industry, the economy and society
- Events Plan the meetings and conferences you want to attend with our comprehensive events calendar
- Innovation showcases A round-up of the latest innovation from our corporate partners
- Collections Explore special collections that bring together our best content on trending topics
- Artificial intelligence Explore the ways in which today’s world relies on AI, and ponder how this technology might shape the world of tomorrow
- #BlackInPhysics Celebrating Black physicists and revealing a more complete picture of what a physicist looks like
- Nanotechnology in action The challenges and opportunities of turning advances in nanotechnology into commercial products
- The Nobel Prize for Physics Explore the work of recent Nobel laureates, find out what happens behind the scenes, and discover some who were overlooked for the prize
- Revolutions in computing Find out how scientists are exploiting digital technologies to understand online behaviour and drive research progress
- The science and business of space Explore the latest trends and opportunities associated with designing, building, launching and exploiting space-based technologies
- Supercool physics Experiments that probe the exotic behaviour of matter at ultralow temperatures depend on the latest cryogenics technology
- Women in physics Celebrating women in physics and their contributions to the field
- Audio and video Explore the sights and sounds of the scientific world
- Podcasts Our regular conversations with inspiring figures from the scientific community
- Video Watch our specially filmed videos to get a different slant on the latest science
- Webinars Tune into online presentations that allow expert speakers to explain novel tools and applications
- IOP Publishing
- Enter e-mail address
- Show Enter password
- Remember me Forgot your password?
- Access more than 20 years of online content
- Manage which e-mail newsletters you want to receive
- Read about the big breakthroughs and innovations across 13 scientific topics
- Explore the key issues and trends within the global scientific community
- Choose which e-mail newsletters you want to receive
Reset your password
Please enter the e-mail address you used to register to reset your password
Registration complete
Thank you for registering with Physics World If you'd like to change your details at any time, please visit My account
Get set for World Quantum Day 2024
Matin Durrani explains how you can join in the celebrations for the third World Quantum Day, which takes place on Sunday 14 April 2024
Sunday 14 April 2024 will be the third World Quantum Day . An annual celebration “promoting public awareness and understanding of quantum science and technology around the world”, it’s a bottom-up initiative from scientists in more than 65 nations from Algeria to Zambia. The day of 14 April has been chosen because “4.14” are the first three digits of Planck’s constant when rounded up: 4.14 × 10 –15 eVs.
The pedant in me is irked a bit by the date. Unless you live in the US, 14 April is surely 14.4 not 4.14. Plus, I’ve always known Planck’s constant as 6.63 × 10 –34 Js not 4.14 × 10 –15 eVs. Putting that aside, World Quantum Day is a brilliant initiative. These are great times for quantum physics, with burgeoning applications of “quantum 2.0” technology such as quantum computing, sensing and cryptography.
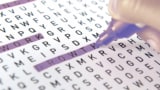
Can you solve this quantum cryptic word search?
As James McKenzie describes in his recent feature article , overall private investment in quantum tech stood at $1.2bn in 2023 according to the State of Quantum 2024 report. Sure, that figure has dropped sharply since 2022, but up to $50bn in public cash has already been ploughed into quantum science. In fact, 33 countries around the world have government initiatives in quantum technology, including 20 with full-scale national strategies.
One person with an overview of the quantum-technology landscape is Mauro Paternostro from Queens’ University Belfast, editor-in-chief of the IOP Publishing journal Quantum Science and Technology . In a wide-ranging interview to be published next week, he argues that the most advanced quantum technology is actually quantum sensing.
In fact, as Steve Brierley – chief executive of Riverlane – points out in his recent opinion article , quantum computers will only ever be properly useful once we can properly deal with error correction.
But quantum tech holds great promise, including in Africa, where “steady progress” is being made, according to Farai Mazhandu and Mhlambululi Mafu . Output from African researchers in quantum-related fields is relatively small, but with a young, digitally native population and a burgeoning quantum workforce, they believe the continent is “poised to take advantage of the coming ‘second quantum revolution’”.
If all this talk of technology is too much, check out the feature by Philip Ball , who looks at the work of “quantum plumbers”. Meanwhile, Robert P Crease, Jennifer Carter and Gino Elia examine “Wigner’s friend” – a quantum thought experiment that has stumped physicists and philosophers for more than 60 years. And why not try our quantum-physics cryptic word search and see if you can reveal the hidden message.
Finally, do check out the Physics World quantum channel as well as a special collection of quantum-themed research articles, focus issues and ebooks from IOP Publishing, which publishes Physics World .
And don’t forget to sign up for the first Physics World Live event – an online panel debate in partnership with Reports on Progress in Physics where you can hear from leading researchers, including Nicole Metje from the University of Birmingham in the UK, discuss the latest on quantum sensors.
Want to read more?
- E-mail Address
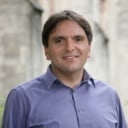
Download your publishing guide
A step-by-step guide unlocking your research’s scientific impact
- Stars and solar physics
Dispatches from the Great North American eclipse of 2024
Iop publishing and physics world celebrate world quantum day, discover more from physics world.
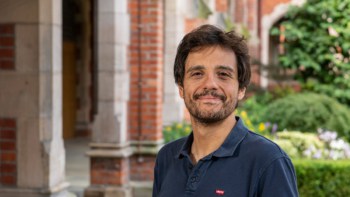
Mauro Paternostro: a vision of the quantum landscape
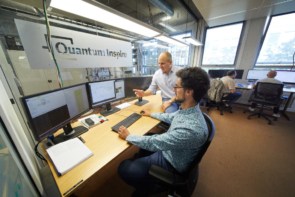
- Quantum computing
Europe plans to build 100-qubit quantum computer by 2026
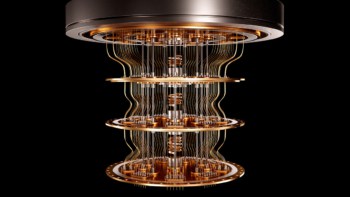
Related jobs
Senior quantum scientist, university professorship (w3) "quantum materials", principle physicist, related events.
- Quantum | Conference IQT The Hague 22—24 April 2024 | The Hague, Netherlands
- Quantum | Conference Quantum.Tech USA 24—26 April 2024 | Washington DC, US
- Quantum | Conference IQT Vancouver-Pacific Rim 4—6 June 2024 | Vancouver, Canada
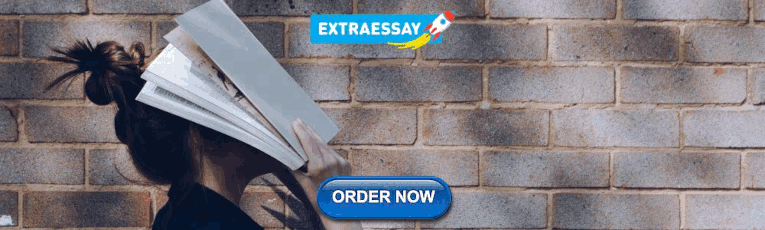
IMAGES
VIDEO
COMMENTS
Quantum computing (QC) has the potential to be the next abstruse technology, with a wide range of possible applications and ramifications for organizations and markets. QC provides an exponential speedup by employing quantum mechanics principles, including superposition and entanglement. The potential advantages offered by the revolutionary paradigm have propelled scientific productions ...
Nature Reviews Physics 4 , 1 ( 2022) Cite this article. This year we celebrate four decades of quantum computing by looking back at the milestones of the field and forward to the challenges and ...
To better understand quantum computing, this paper examines the foundations and vision based on current research in this area. We discuss cutting-edge developments in quantum computer hardware advancement, and subsequent advances in quantum cryptography, quantum software, and high-scalability quantum computers. Many potential challenges and ...
ACM Transactions on Quantum Computing publishes high-impact, original research papers and selected surveys on topics in quantum computing and quantum information science. The journal targets the quantum computer science community with a focus on the theory and practice of quantum computing including but not limited to: models of quantum computing, quantum algorithms and complexity, quantum ...
Quantum computers can calculate and test extensive combinations of hypotheses simultaneously instead of sequentially (S.‐S. Li et al., 2001). Furthermore, some quantum algorithms can be designed in a way that they can solve problems in much fewer steps than their classical counterparts (their complex‐ ity is lower).
This paper attempts to cover as many current state-of-the-art models of quantum computing in various domains and to contribute vital knowledge to the quantum field, readers, and researchers. ... Tom Garlinghouse for the office of the Dean for Research: Quantum computing: Opening new realms of possibilities. Jan. 21, 2020. https://www.princeton ...
A new generation of quantum applications has begun to transform research practices in chemistry, material sciences, and optimization, often combined with machine learning and data science 1.In ...
First, motivated by quantum applications in optimization 2,3,4, the power of quantum computing could, in principle, be used to help improve the training process of existing classical models 5,6 ...
Quantum computing documents by year, 1982-2020. Scopus search results show a steady increase in research output in quantum computing and related areas, really kicking off in 1994. The growth increases steadily resulting in over 48,000 publication. Especially from 2015 onward there is sa steeper rate of publication.
Quantum Computing is a rapidly arising innovation that tackles the laws of quantum mechanics to take care of issues excessively complex for already available classical computers. ... Grassman Space & so on), is a quantum computer" [1]. This paper includes the study of various topics related with quantum computing as well as quantum computers ...
This paper is organized as follows: in Section 1 a brief introduction about of main contribution is presented, Section 2 describes with detail the literature review analyzed in the paper, Section 3 shows the Applications with Quantum Computing Algorithms, in Section 4 the Applications with Deep Learning are presented, and the following Section presents the conclusions and future works and ...
In a paper soon to be presented at the ACM Conference on Object-oriented Programming, Systems, Languages, and Applications, the group outlines a new conceptual model for a quantum computer, called a quantum control machine, that could bring us closer to making programs as easy to write as those for regular classical computers. Such an ...
View a PDF of the paper titled Quantum Computers, Quantum Computing and Quantum Thermodynamics, by Fabrizio Cleri. View PDF Abstract: Quantum thermodynamics aims at extending standard thermodynamics and non-equilibrium statistical physics to systems with sizes well below the thermodynamic limit. A rapidly evolving research field, which promises ...
A comprehensive overview of the promise and challenges of quantum computing and quantum technology, with a focus on computing. The paper covers the latest results, trends, and applications of quantum physics and computing, and provides a map to navigate the hype and literature.
Simulating large-size quantum spin chains on cloud-based superconducting quantum computers Yu, H., Zhao, Y., & Wei, T. C. | Physical Review Research, 5(1), 013183 (2023) Scalable circuits for preparing ground states on digital quantum computers: the Schwinger model vacuum on 100 qubits
Abstract. Quantum computing is computing using quantum-mechanical phenomena, such as superposition and entanglement. A quantum computer is a device that performs quantum computing. Such a computer ...
Quantum computing takes flight. A programmable quantum computer has been reported to outperform the most powerful conventional computers in a specific task — a milestone in computing comparable ...
Quantum Computing is the design of hardware and software that replaces Boolean logic by quantum law at the algorithmic level. For certain computations such as optimization, sampling, search or quantum simulation this promises dramatic speedups. We are particularly interested in applying quantum computing to artificial intelligence and machine ...
Quantum key distribution, quantum communication, quantum computing, and post-quantum cryptography are all the focus areas. In July of 2021, a Memorandum of Understanding (MoU) was signed between the Defence Institute of Advanced Technology and the Centre for the Development of Advanced Computing (C-DAC) to collaborate on the study and ...
1. INTRODUCTION. As of 2016, actual quantum computers are yet to be. developed, but using sma ll number of bits several. experiments a re carri ed out. Research in the field of. Quantum Computing ...
2.2 Limitations of Classical Computers and birth of art of Quantum Computing 2.2.1 Public key Cryptography and Classical factoring of big integers. 2.2.2 Quantum Factoring 2.2.3 Searching of an item with desired property. 2.2.4 Simulation of quantum system by classical computer. 2.3 Quantum Computing: A whole new concept in Parallelism
The algorithm is applied on real world data set. We evaluate the method using a quantum computing simulator, demonstrating remarkable consistency with the results from the classical Fourier transform. Current quantum computers are noisy, a simple method is proposed to mitigate the impact of the noise. The method is evaluated on a 15 qubit IBM-q ...
IBM Quantum Network is a community of Fortune 500 companies, academic institutions, startups and national research labs working with IBM to advance quantum computing. IBM Quantum Network. We're inventing what's next in quantum research. Explore our recent work, access unique toolkits, and discover the breadth of topics that matter to us.
Quantum neural networks (QNNs) leverage the strengths of both quantum computing and neural networks, offering solutions to challenges that are often beyond the reach of traditional neural networks. QNNs are being used in areas such as computer games, function approximation, and big data processing. Moreover, quantum neural network algorithms are finding utility in social network modeling ...
Funding for the research came from the UK Quantum Computing and Simulation (QCS) Hub, with scientists from the UK National Quantum Computing Centre, the Paris-Sorbonne University, the University ...
This paper does not touch on the physics of the devices, and therefore does not require any notion of quan-tum mechanics. Numerical examples on an implementation of Grover's algorithm using open-source software are provided. 1 Introduction Quantum computing is a relatively new area of computing that has the potential to greatly speed up the ...
Quantum machine learning (QML), on the other hand, is relatively new and generally underutilized in both industry and research. Given the recent advances in quantum computing, researchers attending the 2023 IEEE International Conference on Quantum Computing and Engineering (QCE) investigated how to expand the number of use cases for QML in the ...
Initialization is an important challenge for nuclear magnetic resonance quantum computers. The first proposals employed pseudo-pure-state techniques, which isolate the signal of an initialized ...
But quantum tech holds great promise, including in Africa, where "steady progress" is being made, according to Farai Mazhandu and Mhlambululi Mafu.Output from African researchers in quantum-related fields is relatively small, but with a young, digitally native population and a burgeoning quantum workforce, they believe the continent is "poised to take advantage of the coming 'second ...