Have a language expert improve your writing
Run a free plagiarism check in 10 minutes, generate accurate citations for free.
- Knowledge Base
Methodology
- Random Assignment in Experiments | Introduction & Examples
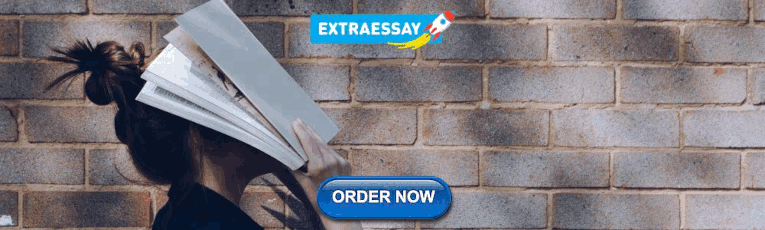
Random Assignment in Experiments | Introduction & Examples
Published on March 8, 2021 by Pritha Bhandari . Revised on June 22, 2023.
In experimental research, random assignment is a way of placing participants from your sample into different treatment groups using randomization.
With simple random assignment, every member of the sample has a known or equal chance of being placed in a control group or an experimental group. Studies that use simple random assignment are also called completely randomized designs .
Random assignment is a key part of experimental design . It helps you ensure that all groups are comparable at the start of a study: any differences between them are due to random factors, not research biases like sampling bias or selection bias .
Table of contents
Why does random assignment matter, random sampling vs random assignment, how do you use random assignment, when is random assignment not used, other interesting articles, frequently asked questions about random assignment.
Random assignment is an important part of control in experimental research, because it helps strengthen the internal validity of an experiment and avoid biases.
In experiments, researchers manipulate an independent variable to assess its effect on a dependent variable, while controlling for other variables. To do so, they often use different levels of an independent variable for different groups of participants.
This is called a between-groups or independent measures design.
You use three groups of participants that are each given a different level of the independent variable:
- a control group that’s given a placebo (no dosage, to control for a placebo effect ),
- an experimental group that’s given a low dosage,
- a second experimental group that’s given a high dosage.
Random assignment to helps you make sure that the treatment groups don’t differ in systematic ways at the start of the experiment, as this can seriously affect (and even invalidate) your work.
If you don’t use random assignment, you may not be able to rule out alternative explanations for your results.
- participants recruited from cafes are placed in the control group ,
- participants recruited from local community centers are placed in the low dosage experimental group,
- participants recruited from gyms are placed in the high dosage group.
With this type of assignment, it’s hard to tell whether the participant characteristics are the same across all groups at the start of the study. Gym-users may tend to engage in more healthy behaviors than people who frequent cafes or community centers, and this would introduce a healthy user bias in your study.
Although random assignment helps even out baseline differences between groups, it doesn’t always make them completely equivalent. There may still be extraneous variables that differ between groups, and there will always be some group differences that arise from chance.
Most of the time, the random variation between groups is low, and, therefore, it’s acceptable for further analysis. This is especially true when you have a large sample. In general, you should always use random assignment in experiments when it is ethically possible and makes sense for your study topic.
Prevent plagiarism. Run a free check.
Random sampling and random assignment are both important concepts in research, but it’s important to understand the difference between them.
Random sampling (also called probability sampling or random selection) is a way of selecting members of a population to be included in your study. In contrast, random assignment is a way of sorting the sample participants into control and experimental groups.
While random sampling is used in many types of studies, random assignment is only used in between-subjects experimental designs.
Some studies use both random sampling and random assignment, while others use only one or the other.
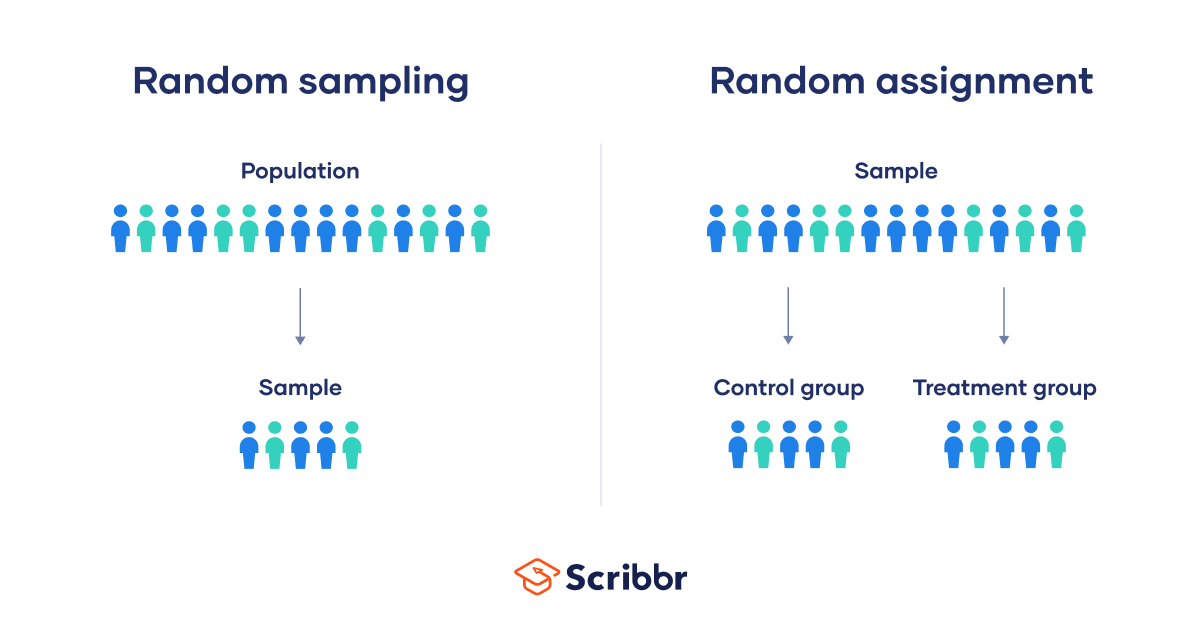
Random sampling enhances the external validity or generalizability of your results, because it helps ensure that your sample is unbiased and representative of the whole population. This allows you to make stronger statistical inferences .
You use a simple random sample to collect data. Because you have access to the whole population (all employees), you can assign all 8000 employees a number and use a random number generator to select 300 employees. These 300 employees are your full sample.
Random assignment enhances the internal validity of the study, because it ensures that there are no systematic differences between the participants in each group. This helps you conclude that the outcomes can be attributed to the independent variable .
- a control group that receives no intervention.
- an experimental group that has a remote team-building intervention every week for a month.
You use random assignment to place participants into the control or experimental group. To do so, you take your list of participants and assign each participant a number. Again, you use a random number generator to place each participant in one of the two groups.
To use simple random assignment, you start by giving every member of the sample a unique number. Then, you can use computer programs or manual methods to randomly assign each participant to a group.
- Random number generator: Use a computer program to generate random numbers from the list for each group.
- Lottery method: Place all numbers individually in a hat or a bucket, and draw numbers at random for each group.
- Flip a coin: When you only have two groups, for each number on the list, flip a coin to decide if they’ll be in the control or the experimental group.
- Use a dice: When you have three groups, for each number on the list, roll a dice to decide which of the groups they will be in. For example, assume that rolling 1 or 2 lands them in a control group; 3 or 4 in an experimental group; and 5 or 6 in a second control or experimental group.
This type of random assignment is the most powerful method of placing participants in conditions, because each individual has an equal chance of being placed in any one of your treatment groups.
Random assignment in block designs
In more complicated experimental designs, random assignment is only used after participants are grouped into blocks based on some characteristic (e.g., test score or demographic variable). These groupings mean that you need a larger sample to achieve high statistical power .
For example, a randomized block design involves placing participants into blocks based on a shared characteristic (e.g., college students versus graduates), and then using random assignment within each block to assign participants to every treatment condition. This helps you assess whether the characteristic affects the outcomes of your treatment.
In an experimental matched design , you use blocking and then match up individual participants from each block based on specific characteristics. Within each matched pair or group, you randomly assign each participant to one of the conditions in the experiment and compare their outcomes.
Sometimes, it’s not relevant or ethical to use simple random assignment, so groups are assigned in a different way.
When comparing different groups
Sometimes, differences between participants are the main focus of a study, for example, when comparing men and women or people with and without health conditions. Participants are not randomly assigned to different groups, but instead assigned based on their characteristics.
In this type of study, the characteristic of interest (e.g., gender) is an independent variable, and the groups differ based on the different levels (e.g., men, women, etc.). All participants are tested the same way, and then their group-level outcomes are compared.
When it’s not ethically permissible
When studying unhealthy or dangerous behaviors, it’s not possible to use random assignment. For example, if you’re studying heavy drinkers and social drinkers, it’s unethical to randomly assign participants to one of the two groups and ask them to drink large amounts of alcohol for your experiment.
When you can’t assign participants to groups, you can also conduct a quasi-experimental study . In a quasi-experiment, you study the outcomes of pre-existing groups who receive treatments that you may not have any control over (e.g., heavy drinkers and social drinkers). These groups aren’t randomly assigned, but may be considered comparable when some other variables (e.g., age or socioeconomic status) are controlled for.
Here's why students love Scribbr's proofreading services
Discover proofreading & editing
If you want to know more about statistics , methodology , or research bias , make sure to check out some of our other articles with explanations and examples.
- Student’s t -distribution
- Normal distribution
- Null and Alternative Hypotheses
- Chi square tests
- Confidence interval
- Quartiles & Quantiles
- Cluster sampling
- Stratified sampling
- Data cleansing
- Reproducibility vs Replicability
- Peer review
- Prospective cohort study
Research bias
- Implicit bias
- Cognitive bias
- Placebo effect
- Hawthorne effect
- Hindsight bias
- Affect heuristic
- Social desirability bias
In experimental research, random assignment is a way of placing participants from your sample into different groups using randomization. With this method, every member of the sample has a known or equal chance of being placed in a control group or an experimental group.
Random selection, or random sampling , is a way of selecting members of a population for your study’s sample.
In contrast, random assignment is a way of sorting the sample into control and experimental groups.
Random sampling enhances the external validity or generalizability of your results, while random assignment improves the internal validity of your study.
Random assignment is used in experiments with a between-groups or independent measures design. In this research design, there’s usually a control group and one or more experimental groups. Random assignment helps ensure that the groups are comparable.
In general, you should always use random assignment in this type of experimental design when it is ethically possible and makes sense for your study topic.
To implement random assignment , assign a unique number to every member of your study’s sample .
Then, you can use a random number generator or a lottery method to randomly assign each number to a control or experimental group. You can also do so manually, by flipping a coin or rolling a dice to randomly assign participants to groups.
Cite this Scribbr article
If you want to cite this source, you can copy and paste the citation or click the “Cite this Scribbr article” button to automatically add the citation to our free Citation Generator.
Bhandari, P. (2023, June 22). Random Assignment in Experiments | Introduction & Examples. Scribbr. Retrieved August 12, 2024, from https://www.scribbr.com/methodology/random-assignment/
Is this article helpful?
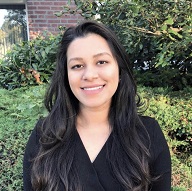
Pritha Bhandari
Other students also liked, guide to experimental design | overview, steps, & examples, confounding variables | definition, examples & controls, control groups and treatment groups | uses & examples, get unlimited documents corrected.
✔ Free APA citation check included ✔ Unlimited document corrections ✔ Specialized in correcting academic texts
- Open access
- Published: 16 August 2021
A roadmap to using randomization in clinical trials
- Vance W. Berger 1 ,
- Louis Joseph Bour 2 ,
- Kerstine Carter 3 ,
- Jonathan J. Chipman ORCID: orcid.org/0000-0002-3021-2376 4 , 5 ,
- Colin C. Everett ORCID: orcid.org/0000-0002-9788-840X 6 ,
- Nicole Heussen ORCID: orcid.org/0000-0002-6134-7206 7 , 8 ,
- Catherine Hewitt ORCID: orcid.org/0000-0002-0415-3536 9 ,
- Ralf-Dieter Hilgers ORCID: orcid.org/0000-0002-5945-1119 7 ,
- Yuqun Abigail Luo 10 ,
- Jone Renteria 11 , 12 ,
- Yevgen Ryeznik ORCID: orcid.org/0000-0003-2997-8566 13 ,
- Oleksandr Sverdlov ORCID: orcid.org/0000-0002-1626-2588 14 &
- Diane Uschner ORCID: orcid.org/0000-0002-7858-796X 15
for the Randomization Innovative Design Scientific Working Group
BMC Medical Research Methodology volume 21 , Article number: 168 ( 2021 ) Cite this article
30k Accesses
40 Citations
14 Altmetric
Metrics details
Randomization is the foundation of any clinical trial involving treatment comparison. It helps mitigate selection bias, promotes similarity of treatment groups with respect to important known and unknown confounders, and contributes to the validity of statistical tests. Various restricted randomization procedures with different probabilistic structures and different statistical properties are available. The goal of this paper is to present a systematic roadmap for the choice and application of a restricted randomization procedure in a clinical trial.
We survey available restricted randomization procedures for sequential allocation of subjects in a randomized, comparative, parallel group clinical trial with equal (1:1) allocation. We explore statistical properties of these procedures, including balance/randomness tradeoff, type I error rate and power. We perform head-to-head comparisons of different procedures through simulation under various experimental scenarios, including cases when common model assumptions are violated. We also provide some real-life clinical trial examples to illustrate the thinking process for selecting a randomization procedure for implementation in practice.
Restricted randomization procedures targeting 1:1 allocation vary in the degree of balance/randomness they induce, and more importantly, they vary in terms of validity and efficiency of statistical inference when common model assumptions are violated (e.g. when outcomes are affected by a linear time trend; measurement error distribution is misspecified; or selection bias is introduced in the experiment). Some procedures are more robust than others. Covariate-adjusted analysis may be essential to ensure validity of the results. Special considerations are required when selecting a randomization procedure for a clinical trial with very small sample size.
Conclusions
The choice of randomization design, data analytic technique (parametric or nonparametric), and analysis strategy (randomization-based or population model-based) are all very important considerations. Randomization-based tests are robust and valid alternatives to likelihood-based tests and should be considered more frequently by clinical investigators.
Peer Review reports
Various research designs can be used to acquire scientific medical evidence. The randomized controlled trial (RCT) has been recognized as the most credible research design for investigations of the clinical effectiveness of new medical interventions [ 1 , 2 ]. Evidence from RCTs is widely used as a basis for submissions of regulatory dossiers in request of marketing authorization for new drugs, biologics, and medical devices. Three important methodological pillars of the modern RCT include blinding (masking), randomization, and the use of control group [ 3 ].
While RCTs provide the highest standard of clinical evidence, they are laborious and costly, in terms of both time and material resources. There are alternative designs, such as observational studies with either a cohort or case–control design, and studies using real world evidence (RWE). When properly designed and implemented, observational studies can sometimes produce similar estimates of treatment effects to those found in RCTs, and furthermore, such studies may be viable alternatives to RCTs in many settings where RCTs are not feasible and/or not ethical. In the era of big data, the sources of clinically relevant data are increasingly rich and include electronic health records, data collected from wearable devices, health claims data, etc. Big data creates vast opportunities for development and implementation of novel frameworks for comparative effectiveness research [ 4 ], and RWE studies nowadays can be implemented rapidly and relatively easily. But how credible are the results from such studies?
In 1980, D. P. Byar issued warnings and highlighted potential methodological problems with comparison of treatment effects using observational databases [ 5 ]. Many of these issues still persist and actually become paramount during the ongoing COVID-19 pandemic when global scientific efforts are made to find safe and efficacious vaccines and treatments as soon as possible. While some challenges pertinent to RWE studies are related to the choice of proper research methodology, some additional challenges arise from increasing requirements of health authorities and editorial boards of medical journals for the investigators to present evidence of transparency and reproducibility of their conducted clinical research. Recently, two top medical journals, the New England Journal of Medicine and the Lancet, retracted two COVID-19 studies that relied on observational registry data [ 6 , 7 ]. The retractions were made at the request of the authors who were unable to ensure reproducibility of the results [ 8 ]. Undoubtedly, such cases are harmful in many ways. The already approved drugs may be wrongly labeled as “toxic” or “inefficacious”, and the reputation of the drug developers could be blemished or destroyed. Therefore, the highest standards for design, conduct, analysis, and reporting of clinical research studies are now needed more than ever. When treatment effects are modest, yet still clinically meaningful, a double-blind, randomized, controlled clinical trial design helps detect these differences while adjusting for possible confounders and adequately controlling the chances of both false positive and false negative findings.
Randomization in clinical trials has been an important area of methodological research in biostatistics since the pioneering work of A. Bradford Hill in the 1940’s and the first published randomized trial comparing streptomycin with a non-treatment control [ 9 ]. Statisticians around the world have worked intensively to elaborate the value, properties, and refinement of randomization procedures with an incredible record of publication [ 10 ]. In particular, a recent EU-funded project ( www.IDeAl.rwth-aachen.de ) on innovative design and analysis of small population trials has “randomization” as one work package. In 2020, a group of trial statisticians around the world from different sectors formed a subgroup of the Drug Information Association (DIA) Innovative Designs Scientific Working Group (IDSWG) to raise awareness of the full potential of randomization to improve trial quality, validity and rigor ( https://randomization-working-group.rwth-aachen.de/ ).
The aims of the current paper are three-fold. First, we describe major recent methodological advances in randomization, including different restricted randomization designs that have superior statistical properties compared to some widely used procedures such as permuted block designs. Second, we discuss different types of experimental biases in clinical trials and explain how a carefully chosen randomization design can mitigate risks of these biases. Third, we provide a systematic roadmap for evaluating different restricted randomization procedures and selecting an “optimal” one for a particular trial. We also showcase application of these ideas through several real life RCT examples.
The target audience for this paper would be clinical investigators and biostatisticians who are tasked with the design, conduct, analysis, and interpretation of clinical trial results, as well as regulatory and scientific/medical journal reviewers. Recognizing the breadth of the concept of randomization, in this paper we focus on a randomized, comparative, parallel group clinical trial design with equal (1:1) allocation, which is typically implemented using some restricted randomization procedure, possibly stratified by some important baseline prognostic factor(s) and/or study center. Some of our findings and recommendations are generalizable to more complex clinical trial settings. We shall highlight these generalizations and outline additional important considerations that fall outside the scope of the current paper.
The paper is organized as follows. The “ Methods ” section provides some general background on the methodology of randomization in clinical trials, describes existing restricted randomization procedures, and discusses some important criteria for comparison of these procedures in practice. In the “ Results ” section, we present our findings from four simulation studies that illustrate the thinking process when evaluating different randomization design options at the study planning stage. The “ Conclusions ” section summarizes the key findings and important considerations on restricted randomization procedures, and it also highlights some extensions and further topics on randomization in clinical trials.
What is randomization and what are its virtues in clinical trials?
Randomization is an essential component of an experimental design in general and clinical trials in particular. Its history goes back to R. A. Fisher and his classic book “The Design of Experiments” [ 11 ]. Implementation of randomization in clinical trials is due to A. Bradford Hill who designed the first randomized clinical trial evaluating the use of streptomycin in treating tuberculosis in 1946 [ 9 , 12 , 13 ].
Reference [ 14 ] provides a good summary of the rationale and justification for the use of randomization in clinical trials. The randomized controlled trial (RCT) has been referred to as “the worst possible design (except for all the rest)” [ 15 ], indicating that the benefits of randomization should be evaluated in comparison to what we are left with if we do not randomize. Observational studies suffer from a wide variety of biases that may not be adequately addressed even using state-of-the-art statistical modeling techniques.
The RCT in the medical field has several features that distinguish it from experimental designs in other fields, such as agricultural experiments. In the RCT, the experimental units are humans, and in the medical field often diagnosed with a potentially fatal disease. These subjects are sequentially enrolled for participation in the study at selected study centers, which have relevant expertise for conducting clinical research. Many contemporary clinical trials are run globally, at multiple research institutions. The recruitment period may span several months or even years, depending on a therapeutic indication and the target patient population. Patients who meet study eligibility criteria must sign the informed consent, after which they are enrolled into the study and, for example, randomized to either experimental treatment E or the control treatment C according to the randomization sequence. In this setup, the choice of the randomization design must be made judiciously, to protect the study from experimental biases and ensure validity of clinical trial results.
The first virtue of randomization is that, in combination with allocation concealment and masking, it helps mitigate selection bias due to an investigator’s potential to selectively enroll patients into the study [ 16 ]. A non-randomized, systematic design such as a sequence of alternating treatment assignments has a major fallacy: an investigator, knowing an upcoming treatment assignment in a sequence, may enroll a patient who, in their opinion, would be best suited for this treatment. Consequently, one of the groups may contain a greater number of “sicker” patients and the estimated treatment effect may be biased. Systematic covariate imbalances may increase the probability of false positive findings and undermine the integrity of the trial. While randomization alleviates the fallacy of a systematic design, it does not fully eliminate the possibility of selection bias (unless we consider complete randomization for which each treatment assignment is determined by a flip of a coin, which is rarely, if ever used in practice [ 17 ]). Commonly, RCTs employ restricted randomization procedures which sequentially balance treatment assignments while maintaining allocation randomness. A popular choice is the permuted block design that controls imbalance by making treatment assignments at random in blocks. To minimize potential for selection bias, one should avoid overly restrictive randomization schemes such as permuted block design with small block sizes, as this is very similar to alternating treatment sequence.
The second virtue of randomization is its tendency to promote similarity of treatment groups with respect to important known, but even more importantly, unknown confounders. If treatment assignments are made at random, then by the law of large numbers, the average values of patient characteristics should be approximately equal in the experimental and the control groups, and any observed treatment difference should be attributed to the treatment effects, not the effects of the study participants [ 18 ]. However, one can never rule out the possibility that the observed treatment difference is due to chance, e.g. as a result of random imbalance in some patient characteristics [ 19 ]. Despite that random covariate imbalances can occur in clinical trials of any size, such imbalances do not compromise the validity of statistical inference, provided that proper statistical techniques are applied in the data analysis.
Several misconceptions on the role of randomization and balance in clinical trials were documented and discussed by Senn [ 20 ]. One common misunderstanding is that balance of prognostic covariates is necessary for valid inference. In fact, different randomization designs induce different extent of balance in the distributions of covariates, and for a given trial there is always a possibility of observing baseline group differences. A legitimate approach is to pre-specify in the protocol the clinically important covariates to be adjusted for in the primary analysis, apply a randomization design (possibly accounting for selected covariates using pre-stratification or some other approach), and perform a pre-planned covariate-adjusted analysis (such as analysis of covariance for a continuous primary outcome), verifying the model assumptions and conducting additional supportive/sensitivity analyses, as appropriate. Importantly, the pre-specified prognostic covariates should always be accounted for in the analysis, regardless whether their baseline differences are present or not [ 20 ].
It should be noted that some randomization designs (such as covariate-adaptive randomization procedures) can achieve very tight balance of covariate distributions between treatment groups [ 21 ]. While we address randomization within pre-specified stratifications, we do not address more complex covariate- and response-adaptive randomization in this paper.
Finally, randomization plays an important role in statistical analysis of the clinical trial. The most common approach to inference following the RCT is the invoked population model [ 10 ]. With this approach, one posits that there is an infinite target population of patients with the disease, from which \(n\) eligible subjects are sampled in an unbiased manner for the study and are randomized to the treatment groups. Within each group, the responses are assumed to be independent and identically distributed (i.i.d.), and inference on the treatment effect is performed using some standard statistical methodology, e.g. a two sample t-test for normal outcome data. The added value of randomization is that it makes the assumption of i.i.d. errors more feasible compared to a non-randomized study because it introduces a real element of chance in the allocation of patients.
An alternative approach is the randomization model , in which the implemented randomization itself forms the basis for statistical inference [ 10 ]. Under the null hypothesis of the equality of treatment effects, individual outcomes (which are regarded as not influenced by random variation, i.e. are considered as fixed) are not affected by treatment. Treatment assignments are permuted in all possible ways consistent with the randomization procedure actually used in the trial. The randomization-based p- value is the sum of null probabilities of the treatment assignment permutations in the reference set that yield the test statistic values greater than or equal to the experimental value. A randomization-based test can be a useful supportive analysis, free of assumptions of parametric tests and protective against spurious significant results that may be caused by temporal trends [ 14 , 22 ].
It is important to note that Bayesian inference has also become a common statistical analysis in RCTs [ 23 ]. Although the inferential framework relies upon subjective probabilities, a study analyzed through a Bayesian framework still relies upon randomization for the other aforementioned virtues [ 24 ]. Hence, the randomization considerations discussed herein have broad application.
What types of randomization methodologies are available?
Randomization is not a single methodology, but a very broad class of design techniques for the RCT [ 10 ]. In this paper, we consider only randomization designs for sequential enrollment clinical trials with equal (1:1) allocation in which randomization is not adapted for covariates and/or responses. The simplest procedure for an RCT is complete randomization design (CRD) for which each subject’s treatment is determined by a flip of a fair coin [ 25 ]. CRD provides no potential for selection bias (e.g. based on prediction of future assignments) but it can result, with non-negligible probability, in deviations from the 1:1 allocation ratio and covariate imbalances, especially in small samples. This may lead to loss of statistical efficiency (decrease in power) compared to the balanced design. In practice, some restrictions on randomization are made to achieve balanced allocation. Such randomization designs are referred to as restricted randomization procedures [ 26 , 27 ].
Suppose we plan to randomize an even number of subjects \(n\) sequentially between treatments E and C. Two basic designs that equalize the final treatment numbers are the random allocation rule (Rand) and the truncated binomial design (TBD), which were discussed in the 1957 paper by Blackwell and Hodges [ 28 ]. For Rand, any sequence of exactly \(n/2\) E’s and \(n/2\) C’s is equally likely. For TBD, treatment assignments are made with probability 0.5 until one of the treatments receives its quota of \(n/2\) subjects; thereafter all remaining assignments are made deterministically to the opposite treatment.
A common feature of both Rand and TBD is that they aim at the final balance, whereas at intermediate steps it is still possible to have substantial imbalances, especially if \(n\) is large. A long run of a single treatment in a sequence may be problematic if there is a time drift in some important covariate, which can lead to chronological bias [ 29 ]. To mitigate this risk, one can further restrict randomization so that treatment assignments are balanced over time. One common approach is the permuted block design (PBD) [ 30 ], for which random treatment assignments are made in blocks of size \(2b\) ( \(b\) is some small positive integer), with exactly \(b\) allocations to each of the treatments E and C. The PBD is perhaps the oldest (it can be traced back to A. Bradford Hill’s 1951 paper [ 12 ]) and the most widely used randomization method in clinical trials. Often its choice in practice is justified by simplicity of implementation and the fact that it is referenced in the authoritative ICH E9 guideline on statistical principles for clinical trials [ 31 ]. One major challenge with PBD is the choice of the block size. If \(b=1\) , then every pair of allocations is balanced, but every even allocation is deterministic. Larger block sizes increase allocation randomness. The use of variable block sizes has been suggested [ 31 ]; however, PBDs with variable block sizes are also quite predictable [ 32 ]. Another problematic feature of the PBD is that it forces periodic return to perfect balance, which may be unnecessary from the statistical efficiency perspective and may increase the risk of prediction of upcoming allocations.
More recent and better alternatives to the PBD are the maximum tolerated imbalance (MTI) procedures [ 33 , 34 , 35 , 36 , 37 , 38 , 39 , 40 , 41 ]. These procedures provide stronger encryption of the randomization sequence (i.e. make it more difficult to predict future treatment allocations in the sequence even knowing the current sizes of the treatment groups) while controlling treatment imbalance at a pre-defined threshold throughout the experiment. A general MTI procedure specifies a certain boundary for treatment imbalance, say \(b>0\) , that cannot be exceeded. If, at a given allocation step the absolute value of imbalance is equal to \(b\) , then one next allocation is deterministically forced toward balance. This is in contrast to PBD which, after reaching the target quota of allocations for either treatment within a block, forces all subsequent allocations to achieve perfect balance at the end of the block. Some notable MTI procedures are the big stick design (BSD) proposed by Soares and Wu in 1983 [ 37 ], the maximal procedure proposed by Berger, Ivanova and Knoll in 2003 [ 35 ], the block urn design (BUD) proposed by Zhao and Weng in 2011 [ 40 ], just to name a few. These designs control treatment imbalance within pre-specified limits and are more immune to selection bias than the PBD [ 42 , 43 ].
Another important class of restricted randomization procedures is biased coin designs (BCDs). Starting with the seminal 1971 paper of Efron [ 44 ], BCDs have been a hot research topic in biostatistics for 50 years. Efron’s BCD is very simple: at any allocation step, if treatment numbers are balanced, the next assignment is made with probability 0.5; otherwise, the underrepresented treatment is assigned with probability \(p\) , where \(0.5<p\le 1\) is a fixed and pre-specified parameter that determines the tradeoff between balance and randomness. Note that \(p=1\) corresponds to PBD with block size 2. If we set \(p<1\) (e.g. \(p=2/3\) ), then the procedure has no deterministic assignments and treatment allocation will be concentrated around 1:1 with high probability [ 44 ]. Several extensions of Efron’s BCD providing better tradeoff between treatment balance and allocation randomness have been proposed [ 45 , 46 , 47 , 48 , 49 ]; for example, a class of adjustable biased coin designs introduced by Baldi Antognini and Giovagnoli in 2004 [ 49 ] unifies many BCDs in a single framework. A comprehensive simulation study comparing different BCDs has been published by Atkinson in 2014 [ 50 ].
Finally, urn models provide a useful mechanism for RCT designs [ 51 ]. Urn models apply some probabilistic rules to sequentially add/remove balls (representing different treatments) in the urn, to balance treatment assignments while maintaining the randomized nature of the experiment [ 39 , 40 , 52 , 53 , 54 , 55 ]. A randomized urn design for balancing treatment assignments was proposed by Wei in 1977 [ 52 ]. More novel urn designs, such as the drop-the-loser urn design developed by Ivanova in 2003 [ 55 ] have reduced variability and can attain the target treatment allocation more efficiently. Many urn designs involve parameters that can be fine-tuned to obtain randomization procedures with desirable balance/randomness tradeoff [ 56 ].
What are the attributes of a good randomization procedure?
A “good” randomization procedure is one that helps successfully achieve the study objective(s). Kalish and Begg [ 57 ] state that the major objective of a comparative clinical trial is to provide a precise and valid comparison. To achieve this, the trial design should be such that it: 1) prevents bias; 2) ensures an efficient treatment comparison; and 3) is simple to implement to minimize operational errors. Table 1 elaborates on these considerations, focusing on restricted randomization procedures for 1:1 randomized trials.
Before delving into a detailed discussion, let us introduce some important definitions. Following [ 10 ], a randomization sequence is a random vector \({{\varvec{\updelta}}}_{n}=({\delta }_{1},\dots ,{\delta }_{n})\) , where \({\delta }_{i}=1\) , if the i th subject is assigned to treatment E or \({\delta }_{i}=0\) , if the \(i\) th subject is assigned to treatment C. A restricted randomization procedure can be defined by specifying a probabilistic rule for the treatment assignment of the ( i +1)st subject, \({\delta }_{i+1}\) , given the past allocations \({{\varvec{\updelta}}}_{i}\) for \(i\ge 1\) . Let \({N}_{E}\left(i\right)={\sum }_{j=1}^{i}{\delta }_{j}\) and \({N}_{C}\left(i\right)=i-{N}_{E}\left(i\right)\) denote the numbers of subjects assigned to treatments E and C, respectively, after \(i\) allocation steps. Then \(D\left(i\right)={N}_{E}\left(i\right)-{N}_{C}(i)\) is treatment imbalance after \(i\) allocations. For any \(i\ge 1\) , \(D\left(i\right)\) is a random variable whose probability distribution is determined by the chosen randomization procedure.
Balance and randomness
Treatment balance and allocation randomness are two competing requirements in the design of an RCT. Restricted randomization procedures that provide a good tradeoff between these two criteria are desirable in practice.
Consider a trial with sample size \(n\) . The absolute value of imbalance, \(\left|D(i)\right|\) \((i=1,\dots,n)\) , provides a measure of deviation from equal allocation after \(i\) allocation steps. \(\left|D(i)\right|=0\) indicates that the trial is perfectly balanced. One can also consider \(\Pr(\vert D\left(i\right)\vert=0)\) , the probability of achieving exact balance after \(i\) allocation steps. In particular \(\Pr(\vert D\left(n\right)\vert=0)\) is the probability that the final treatment numbers are balanced. Two other useful summary measures are the expected imbalance at the \(i\mathrm{th}\) step, \(E\left|D(i)\right|\) and the expected value of the maximum imbalance of the entire randomization sequence, \(E\left(\underset{1\le i\le n}{\mathrm{max}}\left|D\left(i\right)\right|\right)\) .
Greater forcing of balance implies lack of randomness. A procedure that lacks randomness may be susceptible to selection bias [ 16 ], which is a prominent issue in open-label trials with a single center or with randomization stratified by center, where the investigator knows the sequence of all previous treatment assignments. A classic approach to quantify the degree of susceptibility of a procedure to selection bias is the Blackwell-Hodges model [ 28 ]. Let \({G}_{i}=1\) (or 0), if at the \(i\mathrm{th}\) allocation step an investigator makes a correct (or incorrect) guess on treatment assignment \({\delta }_{i}\) , given past allocations \({{\varvec{\updelta}}}_{i-1}\) . Then the predictability of the design at the \(i\mathrm{th}\) step is the expected value of \({G}_{i}\) , i.e. \(E\left(G_i\right)=\Pr(G_i=1)\) . Blackwell and Hodges [ 28 ] considered the expected bias factor , the difference between expected total number of correct guesses of a given sequence of random assignments and the similar quantity obtained from CRD for which treatment assignments are made independently with equal probability: \(E(F)=E\left({\sum }_{i=1}^{n}{G}_{i}\right)-n/2\) . This quantity is zero for CRD, and it is positive for restricted randomization procedures (greater values indicate higher expected bias). Matts and Lachin [ 30 ] suggested taking expected proportion of deterministic assignments in a sequence as another measure of lack of randomness.
In the literature, various restricted randomization procedures have been compared in terms of balance and randomness [ 50 , 58 , 59 ]. For instance, Zhao et al. [ 58 ] performed a comprehensive simulation study of 14 restricted randomization procedures with different choices of design parameters, for sample sizes in the range of 10 to 300. The key criteria were the maximum absolute imbalance and the correct guess probability. The authors found that the performance of the designs was within a closed region with the boundaries shaped by Efron’s BCD [ 44 ] and the big stick design [ 37 ], signifying that the latter procedure with a suitably chosen MTI boundary can be superior to other restricted randomization procedures in terms of balance/randomness tradeoff. Similar findings confirming the utility of the big stick design were recently reported by Hilgers et al. [ 60 ].
Validity and efficiency
Validity of a statistical procedure essentially means that the procedure provides correct statistical inference following an RCT. In particular, a chosen statistical test is valid, if it controls the chance of a false positive finding, that is, the pre-specified probability of a type I error of the test is achieved but not exceeded. The strong control of type I error rate is a major prerequisite for any confirmatory RCT. Efficiency means high statistical power for detecting meaningful treatment differences (when they exist), and high accuracy of estimation of treatment effects.
Both validity and efficiency are major requirements of any RCT, and both of these aspects are intertwined with treatment balance and allocation randomness. Restricted randomization designs, when properly implemented, provide solid ground for valid and efficient statistical inference. However, a careful consideration of different options can help an investigator to optimize the choice of a randomization procedure for their clinical trial.
Let us start with statistical efficiency. Equal (1:1) allocation frequently maximizes power and estimation precision. To illustrate this, suppose the primary outcomes in the two groups are normally distributed with respective means \({\mu }_{E}\) and \({\mu }_{C}\) and common standard deviation \(\sigma >0\) . Then the variance of an efficient estimator of the treatment difference \({\mu }_{E}-{\mu }_{C}\) is equal to \(V=\frac{4{\sigma }^{2}}{n-{L}_{n}}\) , where \({L}_{n}=\frac{{\left|D(n)\right|}^{2}}{n}\) is referred to as loss [ 61 ]. Clearly, \(V\) is minimized when \({L}_{n}=0\) , or equivalently, \(D\left(n\right)=0\) , i.e. the balanced trial.
When the primary outcome follows a more complex statistical model, optimal allocation may be unequal across the treatment groups; however, 1:1 allocation is still nearly optimal for binary outcomes [ 62 , 63 ], survival outcomes [ 64 ], and possibly more complex data types [ 65 , 66 ]. Therefore, a randomization design that balances treatment numbers frequently promotes efficiency of the treatment comparison.
As regards inferential validity, it is important to distinguish two approaches to statistical inference after the RCT – an invoked population model and a randomization model [ 10 ]. For a given randomization procedure, these two approaches generally produce similar results when the assumption of normal random sampling (and some other assumptions) are satisfied, but the randomization model may be more robust when model assumptions are violated; e.g. when outcomes are affected by a linear time trend [ 67 , 68 ]. Another important issue that may interfere with validity is selection bias. Some authors showed theoretically that PBDs with small block sizes may result in serious inflation of the type I error rate under a selection bias model [ 69 , 70 , 71 ]. To mitigate risk of selection bias, one should ideally take preventative measures, such as blinding/masking, allocation concealment, and avoidance of highly restrictive randomization designs. However, for already completed studies with evidence of selection bias [ 72 ], special statistical adjustments are warranted to ensure validity of the results [ 73 , 74 , 75 ].
Implementation aspects
With the current state of information technology, implementation of randomization in RCTs should be straightforward. Validated randomization systems are emerging, and they can handle randomization designs of increasing complexity for clinical trials that are run globally. However, some important points merit consideration.
The first point has to do with how a randomization sequence is generated and implemented. One should distinguish between advance and adaptive randomization [ 16 ]. Here, by “adaptive” randomization we mean “in-real-time” randomization, i.e. when a randomization sequence is generated not upfront, but rather sequentially, as eligible subjects enroll into the study. Restricted randomization procedures are “allocation-adaptive”, in the sense that the treatment assignment of an individual subject is adapted to the history of previous treatment assignments. While in practice the majority of trials with restricted and stratified randomization use randomization schedules pre-generated in advance, there are some circumstances under which “in-real-time” randomization schemes may be preferred; for instance, clinical trials with high cost of goods and/or shortage of drug supply [ 76 ].
The advance randomization approach includes the following steps: 1) for the chosen randomization design and sample size \(n\) , specify the probability distribution on the reference set by enumerating all feasible randomization sequences of length \(n\) and their corresponding probabilities; 2) select a sequence at random from the reference set according to the probability distribution; and 3) implement this sequence in the trial. While enumeration of all possible sequences and their probabilities is feasible and may be useful for trials with small sample sizes, the task becomes computationally prohibitive (and unnecessary) for moderate or large samples. In practice, Monte Carlo simulation can be used to approximate the probability distribution of the reference set of all randomization sequences for a chosen randomization procedure.
A limitation of advance randomization is that a sequence of treatment assignments must be generated upfront, and proper security measures (e.g. blinding/masking) must be in place to protect confidentiality of the sequence. With the adaptive or “in-real-time” randomization, a sequence of treatment assignments is generated dynamically as the trial progresses. For many restricted randomization procedures, the randomization rule can be expressed as \(\Pr(\delta_{i+1}=1)=\left|F\left\{D\left(i\right)\right\}\right|\) , where \(F\left\{\cdot \right\}\) is some non-increasing function of \(D\left(i\right)\) for any \(i\ge 1\) . This is referred to as the Markov property [ 77 ], which makes a procedure easy to implement sequentially. Some restricted randomization procedures, e.g. the maximal procedure [ 35 ], do not have the Markov property.
The second point has to do with how the final data analysis is performed. With an invoked population model, the analysis is conditional on the design and the randomization is ignored in the analysis. With a randomization model, the randomization itself forms the basis for statistical inference. Reference [ 14 ] provides a contemporaneous overview of randomization-based inference in clinical trials. Several other papers provide important technical details on randomization-based tests, including justification for control of type I error rate with these tests [ 22 , 78 , 79 ]. In practice, Monte Carlo simulation can be used to estimate randomization-based p- values [ 10 ].
A roadmap for comparison of restricted randomization procedures
The design of any RCT starts with formulation of the trial objectives and research questions of interest [ 3 , 31 ]. The choice of a randomization procedure is an integral part of the study design. A structured approach for selecting an appropriate randomization procedure for an RCT was proposed by Hilgers et al. [ 60 ]. Here we outline the thinking process one may follow when evaluating different candidate randomization procedures. Our presented roadmap is by no means exhaustive; its main purpose is to illustrate the logic behind some important considerations for finding an “optimal” randomization design for the given trial parameters.
Throughout, we shall assume that the study is designed as a randomized, two-arm comparative trial with 1:1 allocation, with a fixed sample size \(n\) that is pre-determined based on budgetary and statistical considerations to obtain a definitive assessment of the treatment effect via the pre-defined hypothesis testing. We start with some general considerations which determine the study design:
Sample size ( \(n\) ). For small or moderate studies, exact attainment of the target numbers per group may be essential, because even slight imbalance may decrease study power. Therefore, a randomization design in such studies should equalize well the final treatment numbers. For large trials, the risk of major imbalances is less of a concern, and more random procedures may be acceptable.
The length of the recruitment period and the trial duration. Many studies are short-term and enroll participants fast, whereas some other studies are long-term and may have slow patient accrual. In the latter case, there may be time drifts in patient characteristics, and it is important that the randomization design balances treatment assignments over time.
Level of blinding (masking): double-blind, single-blind, or open-label. In double-blind studies with properly implemented allocation concealment the risk of selection bias is low. By contrast, in open-label studies the risk of selection bias may be high, and the randomization design should provide strong encryption of the randomization sequence to minimize prediction of future allocations.
Number of study centers. Many modern RCTs are implemented globally at multiple research institutions, whereas some studies are conducted at a single institution. In the former case, the randomization is often stratified by center and/or clinically important covariates. In the latter case, especially in single-institution open-label studies, the randomization design should be chosen very carefully, to mitigate the risk of selection bias.
An important point to consider is calibration of the design parameters. Many restricted randomization procedures involve parameters, such as the block size in the PBD, the coin bias probability in Efron’s BCD, the MTI threshold, etc. By fine-tuning these parameters, one can obtain designs with desirable statistical properties. For instance, references [ 80 , 81 ] provide guidance on how to justify the block size in the PBD to mitigate the risk of selection bias or chronological bias. Reference [ 82 ] provides a formal approach to determine the “optimal” value of the parameter \(p\) in Efron’s BCD in both finite and large samples. The calibration of design parameters can be done using Monte Carlo simulations for the given trial setting.
Another important consideration is the scope of randomization procedures to be evaluated. As we mentioned already, even one method may represent a broad class of randomization procedures that can provide different levels of balance/randomness tradeoff; e.g. Efron’s BCD covers a wide spectrum of designs, from PBD(2) (if \(p=1\) ) to CRD (if \(p=0.5\) ). One may either prefer to focus on finding the “optimal” parameter value for the chosen design, or be more general and include various designs (e.g. MTI procedures, BCDs, urn designs, etc.) in the comparison. This should be done judiciously, on a case-by-case basis, focusing only on the most reasonable procedures. References [ 50 , 58 , 60 ] provide good examples of simulation studies to facilitate comparisons among various restricted randomization procedures for a 1:1 RCT.
In parallel with the decision on the scope of randomization procedures to be assessed, one should decide upon the performance criteria against which these designs will be compared. Among others, one might think about the two competing considerations: treatment balance and allocation randomness. For a trial of size \(n\) , at each allocation step \(i=1,\dots ,n\) one can calculate expected absolute imbalance \(E\left|D(i)\right|\) and the probability of correct guess \(\Pr(G_i=1)\) as measures of lack of balance and lack of randomness, respectively. These measures can be either calculated analytically (when formulae are available) or through Monte Carlo simulations. Sometimes it may be useful to look at cumulative measures up to the \(i\mathrm{th}\) allocation step ( \(i=1,\dots ,n\) ); e.g. \(\frac{1}{i}{\sum }_{j=1}^{i}E\left|D(j)\right|\) and \(\frac1i\sum\nolimits_{j=1}^i\Pr(G_j=1)\) . For instance, \(\frac{1}{n}{\sum }_{j=1}^{n}{\mathrm{Pr}}({G}_{j}=1)\) is the average correct guess probability for a design with sample size \(n\) . It is also helpful to visualize the selected criteria. Visualizations can be done in a number of ways; e.g. plots of a criterion vs. allocation step, admissibility plots of two chosen criteria [ 50 , 59 ], etc. Such visualizations can help evaluate design characteristics, both overall and at intermediate allocation steps. They may also provide insights into the behavior of a particular design for different values of the tuning parameter, and/or facilitate a comparison among different types of designs.
Another way to compare the merits of different randomization procedures is to study their inferential characteristics such as type I error rate and power under different experimental conditions. Sometimes this can be done analytically, but a more practical approach is to use Monte Carlo simulation. The choice of the modeling and analysis strategy will be context-specific. Here we outline some considerations that may be useful for this purpose:
Data generating mechanism . To simulate individual outcome data, some plausible statistical model must be posited. The form of the model will depend on the type of outcomes (e.g. continuous, binary, time-to-event, etc.), covariates (if applicable), the distribution of the measurement error terms, and possibly some additional terms representing selection and/or chronological biases [ 60 ].
True treatment effects . At least two scenarios should be considered: under the null hypothesis ( \({H}_{0}\) : treatment effects are the same) to evaluate the type I error rate, and under an alternative hypothesis ( \({H}_{1}\) : there is some true clinically meaningful difference between the treatments) to evaluate statistical power.
Randomization designs to be compared . The choice of candidate randomization designs and their parameters must be made judiciously.
Data analytic strategy . For any study design, one should pre-specify the data analysis strategy to address the primary research question. Statistical tests of significance to compare treatment effects may be parametric or nonparametric, with or without adjustment for covariates.
The approach to statistical inference: population model-based or randomization-based . These two approaches are expected to yield similar results when the population model assumptions are met, but they may be different if some assumptions are violated. Randomization-based tests following restricted randomization procedures will control the type I error at the chosen level if the distribution of the test statistic under the null hypothesis is fully specified by the randomization procedure that was used for patient allocation. This is always the case unless there is a major flaw in the design (such as selection bias whereby the outcome of any individual participant is dependent on treatment assignments of the previous participants).
Overall, there should be a well-thought plan capturing the key questions to be answered, the strategy to address them, the choice of statistical software for simulation and visualization of the results, and other relevant details.
In this section we present four examples that illustrate how one may approach evaluation of different randomization design options at the study planning stage. Example 1 is based on a hypothetical 1:1 RCT with \(n=50\) and a continuous primary outcome, whereas Examples 2, 3, and 4 are based on some real RCTs.
Example 1: Which restricted randomization procedures are robust and efficient?
Our first example is a hypothetical RCT in which the primary outcome is assumed to be normally distributed with mean \({\mu }_{E}\) for treatment E, mean \({\mu }_{C}\) for treatment C, and common variance \({\sigma }^{2}\) . A total of \(n\) subjects are to be randomized equally between E and C, and a two-sample t-test is planned for data analysis. Let \(\Delta ={\mu }_{E}-{\mu }_{C}\) denote the true mean treatment difference. We are interested in testing a hypothesis \({H}_{0}:\Delta =0\) (treatment effects are the same) vs. \({H}_{1}:\Delta \ne 0\) .
The total sample size \(n\) to achieve given power at some clinically meaningful treatment difference \({\Delta }_{c}\) while maintaining the chance of a false positive result at level \(\alpha\) can be obtained using standard statistical methods [ 83 ]. For instance, if \({\Delta }_{c}/\sigma =0.95\) , then a design with \(n=50\) subjects (25 per arm) provides approximately 91% power of a two-sample t-test to detect a statistically significant treatment difference using 2-sided \(\alpha =\) 5%. We shall consider 12 randomization procedures to sequentially randomize \(n=50\) subjects in a 1:1 ratio.
Random allocation rule – Rand.
Truncated binomial design – TBD.
Permuted block design with block size of 2 – PBD(2).
Permuted block design with block size of 4 – PBD(4).
Big stick design [ 37 ] with MTI = 3 – BSD(3).
Biased coin design with imbalance tolerance [ 38 ] with p = 2/3 and MTI = 3 – BCDWIT(2/3, 3).
Efron’s biased coin design [ 44 ] with p = 2/3 – BCD(2/3).
Adjustable biased coin design [ 49 ] with a = 2 – ABCD(2).
Generalized biased coin design (GBCD) with \(\gamma =1\) [ 45 ] – GBCD(1).
GBCD with \(\gamma =2\) [ 46 ] – GBCD(2).
GBCD with \(\gamma =5\) [ 47 ] – GBCD(5).
Complete randomization design – CRD.
These 12 procedures can be grouped into five major types. I) Procedures 1, 2, 3, and 4 achieve exact final balance for a chosen sample size (provided the total sample size is a multiple of the block size). II) Procedures 5 and 6 ensure that at any allocation step the absolute value of imbalance is capped at MTI = 3. III) Procedures 7 and 8 are biased coin designs that sequentially adjust randomization according to imbalance measured as the difference in treatment numbers. IV) Procedures 9, 10, and 11 (GBCD’s with \(\gamma =\) 1, 2, and 5) are adaptive biased coin designs, for which randomization probability is modified according to imbalance measured as the difference in treatment allocation proportions (larger \(\gamma\) implies greater forcing of balance). V) Procedure 12 (CRD) is the most random procedure that achieves balance for large samples.
Balance/randomness tradeoff
We first compare the procedures with respect to treatment balance and allocation randomness. To quantify imbalance after \(i\) allocations, we consider two measures: expected value of absolute imbalance \(E\left|D(i)\right|\) , and expected value of loss \(E({L}_{i})=E{\left|D(i)\right|}^{2}/i\) [ 50 , 61 ]. Importantly, for procedures 1, 2, and 3 the final imbalance is always zero, thus \(E\left|D(n)\right|\equiv 0\) and \(E({L}_{n})\equiv 0\) , but at intermediate steps one may have \(E\left|D(i)\right|>0\) and \(E\left({L}_{i}\right)>0\) , for \(1\le i<n\) . For procedures 5 and 6 with MTI = 3, \(E\left({L}_{i}\right)\le 9/i\) . For procedures 7 and 8, \(E\left({L}_{n}\right)\) tends to zero as \(n\to \infty\) [ 49 ]. For procedures 9, 10, 11, and 12, as \(n\to \infty\) , \(E\left({L}_{n}\right)\) tends to the positive constants 1/3, 1/5, 1/11, and 1, respectively [ 47 ]. We take the cumulative average loss after \(n\) allocations as an aggregate measure of imbalance: \(Imb\left(n\right)=\frac{1}{n}{\sum }_{i=1}^{n}E\left({L}_{i}\right)\) , which takes values in the 0–1 range.
To measure lack of randomness, we consider two measures: expected proportion of correct guesses up to the \(i\mathrm{th}\) step, \(PCG\left(i\right)=\frac1i\sum\nolimits_{j=1}^i\Pr(G_j=1)\) , \(i=1,\dots ,n\) , and the forcing index [ 47 , 84 ], \(FI(i)=\frac{{\sum }_{j=1}^{i}E\left|{\phi }_{j}-0.5\right|}{i/4}\) , where \(E\left|{\phi }_{j}-0.5\right|\) is the expected deviation of the conditional probability of treatment E assignment at the \(j\mathrm{th}\) allocation step ( \({\phi }_{j}\) ) from the unconditional target value of 0.5. Note that \(PCG\left(i\right)\) takes values in the range from 0.5 for CRD to 0.75 for PBD(2) assuming \(i\) is even, whereas \(FI(i)\) takes values in the 0–1 range. At the one extreme, we have CRD for which \(FI(i)\equiv 0\) because for CRD \({\phi }_{i}=0.5\) for any \(i\ge 1\) . At the other extreme, we have PBD(2) for which every odd allocation is made with probability 0.5, and every even allocation is deterministic, i.e. made with probability 0 or 1. For PBD(2), assuming \(i\) is even, there are exactly \(i/2\) pairs of allocations, and so \({\sum }_{j=1}^{i}E\left|{\phi }_{j}-0.5\right|=0.5\cdot i/2=i/4\) , which implies that \(FI(i)=1\) for PBD(2). For all other restricted randomization procedures one has \(0<FI(i)<1\) .
A “good” randomization procedure should have low values of both loss and forcing index. Different randomization procedures can be compared graphically. As a balance/randomness tradeoff metric, one can calculate the quadratic distance to the origin (0,0) for the chosen sample size, e.g. \(d(n)=\sqrt{{\left\{Imb(n)\right\}}^{2}+{\left\{FI(n)\right\}}^{2}}\) (in our example \(n=50\) ), and the randomization designs can then be ranked such that designs with lower values of \(d(n)\) are preferable.
We ran a simulation study of the 12 randomization procedures for an RCT with \(n=50\) . Monte Carlo average values of absolute imbalance, loss, \(Imb\left(i\right)\) , \(FI\left(i\right)\) , and \(d(i)\) were calculated for each intermediate allocation step ( \(i=1,\dots ,50\) ), based on 10,000 simulations.
Figure 1 is a plot of expected absolute imbalance vs. allocation step. CRD, GBCD(1), and GBCD(2) show increasing patterns. For TBD and Rand, the final imbalance (when \(n=50\) ) is zero; however, at intermediate steps is can be quite large. For other designs, absolute imbalance is expected to be below 2 at any allocation step up to \(n=50\) . Note the periodic patterns of PBD(2) and PBD(4); for instance, for PBD(2) imbalance is 0 (or 1) for any even (or odd) allocation.
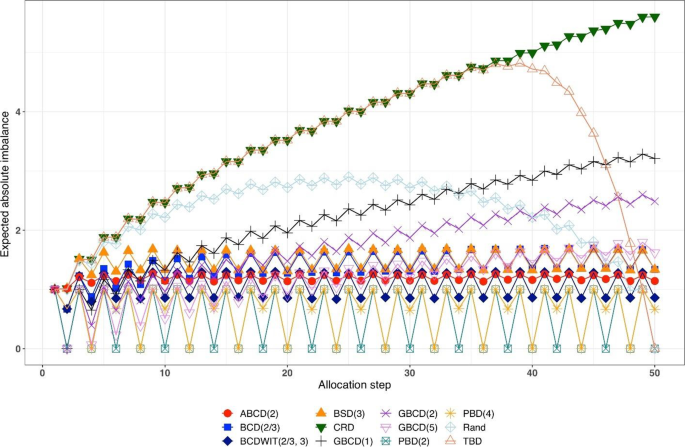
Simulated expected absolute imbalance vs. allocation step for 12 restricted randomization procedures for n = 50. Note: PBD(2) and PBD(4) have forced periodicity absolute imbalance of 0, which distinguishes them from MTI procedures
Figure 2 is a plot of expected proportion of correct guesses vs. allocation step. One can observe that for CRD it is a flat pattern at 0.5; for PBD(2) it fluctuates while reaching the upper limit of 0.75 at even allocation steps; and for ten other designs the values of proportion of correct guesses fall between those of CRD and PBD(2). The TBD has the same behavior up to ~ 40 th allocation step, at which the pattern starts increasing. Rand exhibits an increasing pattern with overall fewer correct guesses compared to other randomization procedures. Interestingly, BSD(3) is uniformly better (less predictable) than ABCD(2), BCD(2/3), and BCDWIT(2/3, 3). For the three GBCD procedures, there is a rapid initial increase followed by gradual decrease in the pattern; this makes good sense, because GBCD procedures force greater balance when the trial is small and become more random (and less prone to correct guessing) as the sample size increases.
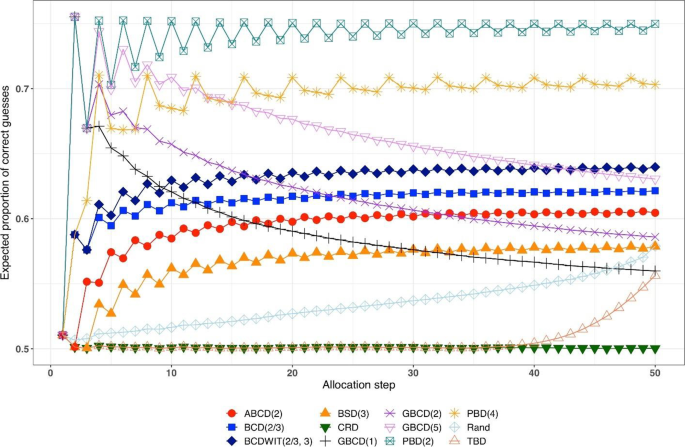
Simulated expected proportion of correct guesses vs. allocation step for 12 restricted randomization procedures for n = 50
Table 2 shows the ranking of the 12 designs with respect to the overall performance metric \(d(n)=\sqrt{{\left\{Imb(n)\right\}}^{2}+{\left\{FI(n)\right\}}^{2}}\) for \(n=50\) . BSD(3), GBCD(2) and GBCD(1) are the top three procedures, whereas PBD(2) and CRD are at the bottom of the list.
Figure 3 is a plot of \(FI\left(n\right)\) vs. \(Imb\left(n\right)\) for \(n=50\) . One can see the two extremes: CRD that takes the value (0,1), and PBD(2) with the value (1,0). The other ten designs are closer to (0,0).
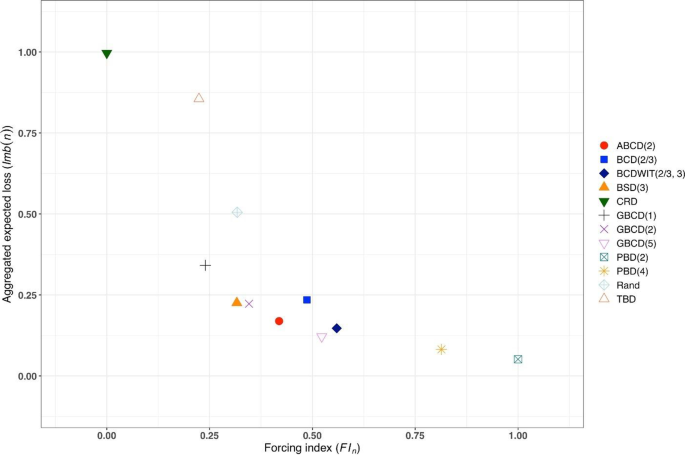
Simulated forcing index (x-axis) vs. aggregate expected loss (y-axis) for 12 restricted randomization procedures for n = 50
Figure 4 is a heat map plot of the metric \(d(i)\) for \(i=1,\dots ,50\) . BSD(3) seems to provide overall best tradeoff between randomness and balance throughout the study.
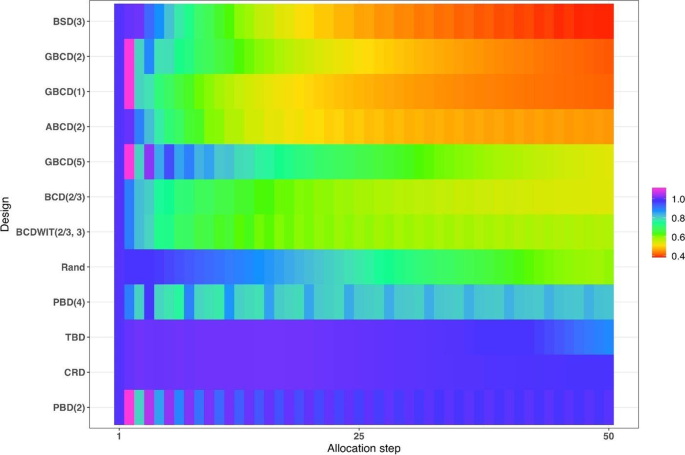
Heatmap of the balance/randomness tradeoff \(d\left(i\right)=\sqrt{{\left\{Imb(i)\right\}}^{2}+{\left\{FI(i)\right\}}^{2}}\) vs. allocation step ( \(i=1,\dots ,50\) ) for 12 restricted randomization procedures. The procedures are ordered by value of d(50), with smaller values (more red) indicating more optimal performance
Inferential characteristics: type I error rate and power
Our next goal is to compare the chosen randomization procedures in terms of validity (control of the type I error rate) and efficiency (power). For this purpose, we assumed the following data generating mechanism: for the \(i\mathrm{th}\) subject, conditional on the treatment assignment \({\delta }_{i}\) , the outcome \({Y}_{i}\) is generated according to the model
where \({u}_{i}\) is an unknown term associated with the \(i\mathrm{th}\) subject and \({\varepsilon }_{i}\) ’s are i.i.d. measurement errors. We shall explore the following four models:
M1: Normal random sampling : \({u}_{i}\equiv 0\) and \({\varepsilon }_{i}\sim\) i.i.d. N(0,1), \(i=1,\dots ,n\) . This corresponds to a standard setup for a two-sample t-test under a population model.
M2: Linear trend : \({u}_{i}=\frac{5i}{n+1}\) and \({\varepsilon }_{i}\sim\) i.i.d. N(0,1), \(i=1,\dots ,n\) . In this model, the outcomes are affected by a linear trend over time [ 67 ].
M3: Cauchy errors : \({u}_{i}\equiv 0\) and \({\varepsilon }_{i}\sim\) i.i.d. Cauchy(0,1), \(i=1,\dots ,n\) . In this setup, we have a misspecification of the distribution of measurement errors.
M4: Selection bias : \({u}_{i+1}=-\nu \cdot sign\left\{D\left(i\right)\right\}\) , \(i=0,\dots ,n-1\) , with the convention that \(D\left(0\right)=0\) . Here, \(\nu >0\) is the “bias effect” (in our simulations we set \(\nu =0.5\) ). We also assume that \({\varepsilon }_{i}\sim\) i.i.d. N(0,1), \(i=1,\dots ,n\) . In this setup, at each allocation step the investigator attempts to intelligently guess the upcoming treatment assignment and selectively enroll a patient who, in their view, would be most suitable for the upcoming treatment. The investigator uses the “convergence” guessing strategy [ 28 ], that is, guess the treatment as one that has been less frequently assigned thus far, or make a random guess in case the current treatment numbers are equal. Assuming that the investigator favors the experimental treatment and is interested in demonstrating its superiority over the control, the biasing mechanism is as follows: at the \((i+1)\) st step, a “healthier” patient is enrolled, if \(D\left(i\right)<0\) ( \({u}_{i+1}=0.5\) ); a “sicker” patient is enrolled, if \(D\left(i\right)>0\) ( \({u}_{i+1}=-0.5\) ); or a “regular” patient is enrolled, if \(D\left(i\right)=0\) ( \({u}_{i+1}=0\) ).
We consider three statistical test procedures:
T1: Two-sample t-test : The test statistic is \(t=\frac{{\overline{Y} }_{E}-{\overline{Y} }_{C}}{\sqrt{{S}_{p}^{2}\left(\frac{1}{{N}_{E}\left(n\right)}+\frac{1}{{N}_{C}\left(n\right)}\right)}}\) , where \({\overline{Y} }_{E}=\frac{1}{{N}_{E}\left(n\right)}{\sum }_{i=1}^{n}{{\delta }_{i}Y}_{i}\) and \({\overline{Y} }_{C}=\frac{1}{{N}_{C}\left(n\right)}{\sum }_{i=1}^{n}{(1-\delta }_{i}){Y}_{i}\) are the treatment sample means, \({N}_{E}\left(n\right)={\sum }_{i=1}^{n}{\delta }_{i}\) and \({N}_{C}\left(n\right)=n-{N}_{E}\left(n\right)\) are the observed group sample sizes, and \({S}_{p}^{2}\) is a pooled estimate of variance, where \({S}_{p}^{2}=\frac{1}{n-2}\left({\sum }_{i=1}^{n}{\delta }_{i}{\left({Y}_{i}-{\overline{Y} }_{E}\right)}^{2}+{\sum }_{i=1}^{n}(1-{\delta }_{i}){\left({Y}_{i}-{\overline{Y} }_{C}\right)}^{2}\right)\) . Then \({H}_{0}:\Delta =0\) is rejected at level \(\alpha\) , if \(\left|t\right|>{t}_{1-\frac{\alpha }{2}, n-2}\) , the 100( \(1-\frac{\alpha }{2}\) )th percentile of the t-distribution with \(n-2\) degrees of freedom.
T2: Randomization-based test using mean difference : Let \({{\varvec{\updelta}}}_{obs}\) and \({{\varvec{y}}}_{obs}\) denote, respectively the observed sequence of treatment assignments and responses, obtained from the trial using randomization procedure \(\mathfrak{R}\) . We first compute the observed mean difference \({S}_{obs}=S\left({{\varvec{\updelta}}}_{obs},{{\varvec{y}}}_{obs}\right)={\overline{Y} }_{E}-{\overline{Y} }_{C}\) . Then we use Monte Carlo simulation to generate \(L\) randomization sequences of length \(n\) using procedure \(\mathfrak{R}\) , where \(L\) is some large number. For the \(\ell\mathrm{th}\) generated sequence, \({{\varvec{\updelta}}}_{\ell}\) , compute \({S}_{\ell}=S({{\varvec{\updelta}}}_{\ell},{{\varvec{y}}}_{obs})\) , where \({\ell}=1,\dots ,L\) . The proportion of sequences for which \({S}_{\ell}\) is at least as extreme as \({S}_{obs}\) is computed as \(\widehat{P}=\frac{1}{L}{\sum }_{{\ell}=1}^{L}1\left\{\left|{S}_{\ell}\right|\ge \left|{S}_{obs}\right|\right\}\) . Statistical significance is declared, if \(\widehat{P}<\alpha\) .
T3: Randomization-based test based on ranks : This test procedure follows the same logic as T2, except that the test statistic is calculated based on ranks. Given the vector of observed responses \({{\varvec{y}}}_{obs}=({y}_{1},\dots ,{y}_{n})\) , let \({a}_{jn}\) denote the rank of \({y}_{j}\) among the elements of \({{\varvec{y}}}_{obs}\) . Let \({\overline a}_n\) denote the average of \({a}_{jn}\) ’s, and let \({\boldsymbol a}_n=\left(a_{1n}-{\overline a}_n,...,\alpha_{nn}-{\overline a}_n\right)\boldsymbol'\) . Then a linear rank test statistic has the form \({S}_{obs}={{\varvec{\updelta}}}_{obs}^{\boldsymbol{^{\prime}}}{{\varvec{a}}}_{n}={\sum }_{i=1}^{n}{\delta }_{i}({a}_{in}-{\overline{a} }_{n})\) .
We consider four scenarios of the true mean difference \(\Delta ={\mu }_{E}-{\mu }_{C}\) , which correspond to the Null case ( \(\Delta =0\) ), and three choices of \(\Delta >0\) which correspond to Alternative 1 (power ~ 70%), Alternative 2 (power ~ 80%), and Alternative 3 (power ~ 90%). In all cases, \(n=50\) was used.
Figure 5 summarizes the results of a simulation study comparing 12 randomization designs, under 4 models for the outcome (M1, M2, M3, and M4), 4 scenarios for the mean treatment difference (Null, and Alternatives 1, 2, and 3), using 3 statistical tests (T1, T2, and T3). The operating characteristics of interest are the type I error rate under the Null scenario and the power under the Alternative scenarios. Each scenario was simulated 10,000 times, and each randomization-based test was computed using \(L=\mathrm{10,000}\) sequences.
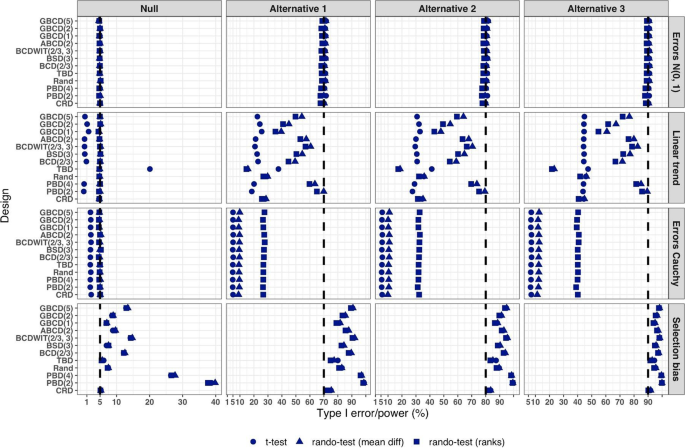
Simulated type I error rate and power of 12 restricted randomization procedures. Four models for the data generating mechanism of the primary outcome (M1: Normal random sampling; M2: Linear trend; M3: Errors Cauchy; and M4: Selection bias). Four scenarios for the treatment mean difference (Null; Alternatives 1, 2, and 3). Three statistical tests (T1: two-sample t-test; T2: randomization-based test using mean difference; T3: randomization-based test using ranks)
From Fig. 5 , under the normal random sampling model (M1), all considered randomization designs have similar performance: they maintain the type I error rate and have similar power, with all tests. In other words, when population model assumptions are satisfied, any combination of design and analysis should work well and yield reliable and consistent results.
Under the “linear trend” model (M2), the designs have differential performance. First of all, under the Null scenario, only Rand and CRD maintain the type I error rate at 5% with all three tests. For TBD, the t-test is anticonservative, with type I error rate ~ 20%, whereas for nine other procedures the t-test is conservative, with type I error rate in the range 0.1–2%. At the same time, for all 12 designs the two randomization-based tests maintain the nominal type I error rate at 5%. These results are consistent with some previous findings in the literature [ 67 , 68 ]. As regards power, it is reduced significantly compared to the normal random sampling scenario. The t-test seems to be most affected and the randomization-based test using ranks is most robust for a majority of the designs. Remarkably, for CRD the power is similar with all three tests. This signifies the usefulness of randomization-based inference in situations when outcome data are subject to a linear time trend, and the importance of applying randomization-based tests at least as supplemental analyses to likelihood-based test procedures.
Under the “Cauchy errors” model (M3), all designs perform similarly: the randomization-based tests maintain the type I error rate at 5%, whereas the t-test deflates the type I error to 2%. As regards power, all designs also have similar, consistently degraded performance: the t-test is least powerful, and the randomization-based test using ranks has highest power. Overall, under misspecification of the error distribution a randomization-based test using ranks is most appropriate; yet one should acknowledge that its power is still lower than expected.
Under the “selection bias” model (M4), the 12 designs have differential performance. The only procedure that maintained the type I error rate at 5% with all three tests was CRD. For eleven other procedures, inflations of the type I error were observed. In general, the more random the design, the less it was affected by selection bias. For instance, the type I error rate for TBD was ~ 6%; for Rand, BSD(3), and GBCD(1) it was ~ 7.5%; for GBCD(2) and ABCD(2) it was ~ 8–9%; for Efron’s BCD(2/3) it was ~ 12.5%; and the most affected design was PBD(2) for which the type I error rate was ~ 38–40%. These results are consistent with the theory of Blackwell and Hodges [ 28 ] which posits that TBD is least susceptible to selection bias within a class of restricted randomization designs that force exact balance. Finally, under M4, statistical power is inflated by several percentage points compared to the normal random sampling scenario without selection bias.
We performed additional simulations to assess the impact of the bias effect \(\nu\) under selection bias model. The same 12 randomization designs and three statistical tests were evaluated for a trial with \(n=50\) under the Null scenario ( \(\Delta =0\) ), for \(\nu\) in the range of 0 (no bias) to 1 (strong bias). Figure S1 in the Supplementary Materials shows that for all designs but CRD, the type I error rate is increasing in \(\nu\) , with all three tests. The magnitude of the type I error inflation is different across the restricted randomization designs; e.g. for TBD it is minimal, whereas for more restrictive designs it may be large, especially for \(\nu \ge 0.4\) . PBD(2) is particularly vulnerable: for \(\nu\) in the range 0.4–1, its type I error rate is in the range 27–90% (for the nominal \(\alpha =5\) %).
In summary, our Example 1 includes most of the key ingredients of the roadmap for assessment of competing randomization designs which was described in the “ Methods ” section. For the chosen experimental scenarios, we evaluated CRD and several restricted randomization procedures, some of which belonged to the same class but with different values of the parameter (e.g. GBCD with \(\gamma =1, 2, 5\) ). We assessed two measures of imbalance, two measures of lack of randomness (predictability), and a metric that quantifies balance/randomness tradeoff. Based on these criteria, we found that BSD(3) provides overall best performance. We also evaluated type I error and power of selected randomization procedures under several treatment response models. We have observed important links between balance, randomness, type I error rate and power. It is beneficial to consider all these criteria simultaneously as they may complement each other in characterizing statistical properties of randomization designs. In particular, we found that a design that lacks randomness, such as PBD with blocks of 2 or 4, may be vulnerable to selection bias and lead to inflations of the type I error. Therefore, these designs should be avoided, especially in open-label studies. As regards statistical power, since all designs in this example targeted 1:1 allocation ratio (which is optimal if the outcomes are normally distributed and have between-group constant variance), they had very similar power of statistical tests in most scenarios except for the one with chronological bias. In the latter case, randomization-based tests were more robust and more powerful than the standard two-sample t-test under the population model assumption.
Overall, while Example 1 is based on a hypothetical 1:1 RCT, its true purpose is to showcase the thinking process in the application of our general roadmap. The following three examples are considered in the context of real RCTs.
Example 2: How can we reduce predictability of a randomization procedure and lower the risk of selection bias?
Selection bias can arise if the investigator can intelligently guess at least part of the randomization sequence yet to be allocated and, on that basis, preferentially and strategically assigns study subjects to treatments. Although it is generally not possible to prove that a particular study has been infected with selection bias, there are examples of published RCTs that do show some evidence to have been affected by it. Suspect trials are, for example, those with strong observed baseline covariate imbalances that consistently favor the active treatment group [ 16 ]. In what follows we describe an example of an RCT where the stratified block randomization procedure used was vulnerable to potential selection biases, and discuss potential alternatives that may reduce this vulnerability.
Etanercept was studied in patients aged 4 to 17 years with polyarticular juvenile rheumatoid arthritis [ 85 ]. The trial consisted of two parts. During the first, open-label part of the trial, patients received etanercept twice weekly for up to three months. Responders from this initial part of the trial were then randomized, at a 1:1 ratio, in the second, double-blind, placebo-controlled part of the trial to receive etanercept or placebo for four months or until a flare of the disease occurred. The primary efficacy outcome, the proportion of patients with disease flare, was evaluated in the double-blind part. Among the 51 randomized patients, 21 of the 26 placebo patients (81%) withdrew because of disease flare, compared with 7 of the 25 etanercept patients (28%), yielding a p- value of 0.003.
Regulatory review by the Food and Drug Administrative (FDA) identified vulnerability to selection biases in the study design of the double-blind part and potential issues in study conduct. These findings were succinctly summarized in [ 16 ] (pp.51–52).
Specifically, randomization was stratified by study center and number of active joints (≤ 2 vs. > 2, referred to as “few” or “many” in what follows), with blocked randomization within each stratum using a block size of two. Furthermore, randomization codes in corresponding “few” and “many” blocks within each study center were mirror images of each other. For example, if the first block within the “few” active joints stratum of a given center is “placebo followed by etanercept”, then the first block within the “many” stratum of the same center would be “etanercept followed by placebo”. While this appears to be an attempt to improve treatment balance in this small trial, unblinding of one treatment assignment may lead to deterministic predictability of three upcoming assignments. While the double-blind nature of the trial alleviated this concern to some extent, it should be noted that all patients did receive etanercept previously in the initial open-label part of the trial. Chances of unblinding may not be ignorable if etanercept and placebo have immediately evident different effects or side effects. The randomized withdrawal design was appropriate in this context to improve statistical power in identifying efficacious treatments, but the specific randomization procedure used in the trial increased vulnerability to selection biases if blinding cannot be completely maintained.
FDA review also identified that four patients were randomized from the wrong “few” or “many” strata, in three of which (3/51 = 5.9%) it was foreseeable that the treatment received could have been reversed compared to what the patient would have received if randomized in the correct stratum. There were also some patients randomized out of order. Imbalance in baseline characteristics were observed in age (mean ages of 8.9 years in the etanercept arm vs. that of 12.2 years in the placebo arm) and corticosteroid use at baseline (50% vs. 24%).
While the authors [ 85 ] concluded that “The unequal randomization did not affect the study results”, and indeed it was unknown whether the imbalance was a chance occurrence or in part caused by selection biases, the trial could have used better alternative randomization procedures to reduce vulnerability to potential selection bias. To illustrate the latter point, let us compare predictability of two randomization procedures – permuted block design (PBD) and big stick design (BSD) for several values of the maximum tolerated imbalance (MTI). We use BSD here for the illustration purpose because it was found to provide a very good balance/randomness tradeoff based on our simulations in Example 1 . In essence, BSD provides the same level of imbalance control as PBD but with stronger encryption.
Table 3 reports two metrics for PBD and BSD: proportion of deterministic assignments within a randomization sequence, and excess correct guess probability. The latter metric is the absolute increase in proportion of correct guesses for a given procedure over CRD that has 50% probability of correct guesses under the “optimal guessing strategy”. Footnote 1 Note that for MTI = 1, BSD is equivalent to PBD with blocks of two. However, by increasing MTI, one can substantially decrease predictability. For instance, going from MTI = 1 in the BSD to an MTI of 2 or 3 (two bottom rows), the proportion of deterministic assignments decreases from 50% to 25% and 16.7%, respectively, and excess correct guess probability decreases from 25% to 12.5% and 8.3%, which is a substantial reduction in risk of selection bias. In addition to simplicity and lower predictability for the same level of MTI control, BSD has another important advantage: investigators are not accustomed to it (as they are to the PBD), and therefore it has potential for complete elimination of prediction through thwarting enough early prediction attempts.
Our observations here are also generalizable to other MTI randomization methods, such as the maximal procedure [ 35 ], Chen’s designs [ 38 , 39 ], block urn design [ 40 ], just to name a few. MTI randomization procedures can be also used as building elements for more complex stratified randomization schemes [ 86 ].
Example 3: How can we mitigate risk of chronological bias?
Chronological bias may occur if a trial recruitment period is long, and there is a drift in some covariate over time that is subsequently not accounted for in the analysis [ 29 ]. To mitigate risk of chronological bias, treatment assignments should be balanced over time. In this regard, the ICH E9 guideline has the following statement [ 31 ]:
“...Although unrestricted randomisation is an acceptable approach, some advantages can generally be gained by randomising subjects in blocks. This helps to increase the comparability of the treatment groups, particularly when subject characteristics may change over time, as a result, for example, of changes in recruitment policy. It also provides a better guarantee that the treatment groups will be of nearly equal size...”
While randomization in blocks of two ensures best balance, it is highly predictable. In practice, a sensible tradeoff between balance and randomness is desirable. In the following example, we illustrate the issue of chronological bias in the context of a real RCT.
Altman and Royston [ 87 ] gave several examples of clinical studies with hidden time trends. For instance, an RCT to compare azathioprine versus placebo in patients with primary biliary cirrhosis (PBC) with respect to overall survival was an international, double-blind, randomized trial including 248 patients of whom 127 received azathioprine and 121 placebo [ 88 ]. The study had a recruitment period of 7 years. A major prognostic factor for survival was the serum bilirubin level on entry to the trial. Altman and Royston [ 87 ] provided a cusum plot of log bilirubin which showed a strong decreasing trend over time – patients who entered the trial later had, on average, lower bilirubin levels, and therefore better prognosis. Despite that the trial was randomized, there was some evidence of baseline imbalance with respect to serum bilirubin between azathioprine and placebo groups. The analysis using Cox regression adjusted for serum bilirubin showed that the treatment effect of azathioprine was statistically significant ( p = 0.01), with azathioprine reducing the risk of dying to 59% of that observed during the placebo treatment.
The azathioprine trial [ 88 ] provides a very good example for illustrating importance of both the choice of a randomization design and a subsequent statistical analysis. We evaluated several randomization designs and analysis strategies under the given time trend through simulation. Since we did not have access to the patient level data from the azathioprine trial, we simulated a dataset of serum bilirubin values from 248 patients that resembled that in the original paper (Fig. 1 in [ 87 ]); see Fig. 6 below.
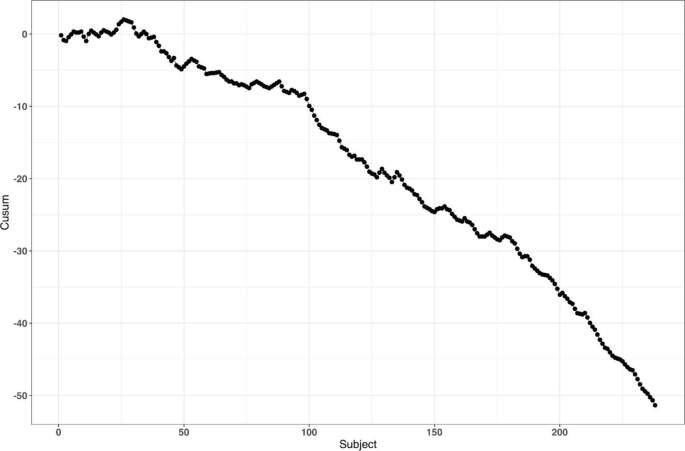
reproduced from Fig. 1 of Altman and Royston [ 87 ]
Cusum plot of baseline log serum bilirubin level of 248 subjects from the azathioprine trial,
For the survival outcomes, we use the following data generating mechanism [ 71 , 89 ]: let \({h}_{i}(t,{\delta }_{i})\) denote the hazard function of the \(i\mathrm{th}\) patient at time \(t\) such that
where \({h}_{c}(t)\) is an unspecified baseline hazard, \(\log HR\) is the true value of the log-transformed hazard ratio, and \({u}_{i}\) is the log serum bilirubin of the \(i\mathrm{th}\) patient at study entry.
Our main goal is to evaluate the impact of the time trend in bilirubin on the type I error rate and power. We consider seven randomization designs: CRD, Rand, TBD, PBD(2), PBD(4), BSD(3), and GBCD(2). The latter two designs were found to be the top two performing procedures based on our simulation results in Example 1 (cf. Table 2 ). PBD(4) is the most commonly used procedure in clinical trial practice. Rand and TBD are two designs that ensure exact balance in the final treatment numbers. CRD is the most random design, and PBD(2) is the most balanced design.
To evaluate both type I error and power, we consider two values for the true treatment effect: \(HR=1\) (Null) and \(HR=0.6\) (Alternative). For data analysis, we use the Cox regression model, either with or without adjustment for serum bilirubin. Furthermore, we assess two approaches to statistical inference: population model-based and randomization-based. For the sake of simplicity, we let \({h}_{c}\left(t\right)\equiv 1\) (exponential distribution) and assume no censoring when simulating the data.
For each combination of the design, experimental scenario, and data analysis strategy, a trial with 248 patients was simulated 10,000 times. Each randomization-based test was computed using \(L=\mathrm{1,000}\) sequences. In each simulation, we used the same time trend in serum bilirubin as described. Through simulation, we estimated the probability of a statistically significant baseline imbalance in serum bilirubin between azathioprine and placebo groups, type I error rate, and power.
First, we observed that the designs differ with respect to their potential to achieve baseline covariate balance under the time trend. For instance, probability of a statistically significant group difference on serum bilirubin (two-sided P < 0.05) is ~ 24% for TBD, ~ 10% for CRD, ~ 2% for GBCD(2), ~ 0.9% for Rand, and ~ 0% for BSD(3), PBD(4), and PBD(2).
Second, a failure to adjust for serum bilirubin in the analysis can negatively impact statistical inference. Table 4 shows the type I error and power of statistical analyses unadjusted and adjusted for serum bilirubin, using population model-based and randomization-based approaches.
If we look at the type I error for the population model-based, unadjusted analysis, we can see that only CRD and Rand are valid (maintain the type I error rate at 5%), whereas TBD is anticonservative (~ 15% type I error) and PBD(2), PBD(4), BSD(3), and GBCD(2) are conservative (~ 1–2% type I error). These findings are consistent with the ones for the two-sample t-test described earlier in the current paper, and they agree well with other findings in the literature [ 67 ]. By contrast, population model-based covariate-adjusted analysis is valid for all seven randomization designs. Looking at the type I error for the randomization-based analyses, all designs yield consistent valid results (~ 5% type I error), with or without adjustment for serum bilirubin.
As regards statistical power, unadjusted analyses are substantially less powerful then the corresponding covariate-adjusted analysis, for all designs with either population model-based or randomization-based approaches. For the population model-based, unadjusted analysis, the designs have ~ 59–65% power, whereas than the corresponding covariate-adjusted analyses have ~ 97% power. The most striking results are observed with the randomization-based approach: the power of unadjusted analysis is quite different across seven designs: it is ~ 37% for TBD, ~ 60–61% for CRD and Rand, ~ 80–87% for BCD(3), GBCD(2), and PBD(4), and it is ~ 90% for PBD(2). Thus, PBD(2) is the most powerful approach if a time trend is present, statistical analysis strategy is randomization-based, and no adjustment for time trend is made. Furthermore, randomization-based covariate-adjusted analyses have ~ 97% power for all seven designs. Remarkably, the power of covariate-adjusted analysis is identical for population model-based and randomization-based approaches.
Overall, this example highlights the importance of covariate-adjusted analysis, which should be straightforward if a covariate affected by a time trend is known (e.g. serum bilirubin in our example). If a covariate is unknown or hidden, then unadjusted analysis following a conventional test may have reduced power and distorted type I error (although the designs such as CRD and Rand do ensure valid statistical inference). Alternatively, randomization-based tests can be applied. The resulting analysis will be valid but may be potentially less powerful. The degree of loss in power following randomization-based test depends on the randomization design: designs that force greater treatment balance over time will be more powerful. In fact, PBD(2) is shown to be most powerful under such circumstances; however, as we have seen in Example 1 and Example 2, a major deficiency of PBD(2) is its vulnerability to selection bias. From Table 4 , and taking into account the earlier findings in this paper, BSD(3) seems to provide a very good risk mitigation strategy against unknown time trends.
Example 4: How do we design an RCT with a very small sample size?
In our last example, we illustrate the importance of the careful choice of randomization design and subsequent statistical analysis in a nonstandard RCT with small sample size. Due to confidentiality and because this study is still in conduct, we do not disclose all details here except for that the study is an ongoing phase II RCT in a very rare and devastating autoimmune disease in children.
The study includes three periods: an open-label single-arm active treatment for 28 weeks to identify treatment responders (Period 1), a 24-week randomized treatment withdrawal period to primarily assess the efficacy of the active treatment vs. placebo (Period 2), and a 3-year long-term safety, open-label active treatment (Period 3). Because of a challenging indication and the rarity of the disease, the study plans to enroll up to 10 male or female pediatric patients in order to randomize 8 patients (4 per treatment arm) in Period 2 of the study. The primary endpoint for assessing the efficacy of active treatment versus placebo is the proportion of patients with disease flare during the 24-week randomized withdrawal phase. The two groups will be compared using Fisher’s exact test. In case of a successful outcome, evidence of clinical efficacy from this study will be also used as part of a package to support the claim for drug effectiveness.
Very small sample sizes are not uncommon in clinical trials of rare diseases [ 90 , 91 ]. Naturally, there are several methodological challenges for this type of study. A major challenge is generalizability of the results from the RCT to a population. In this particular indication, no approved treatment exists, and there is uncertainty on disease epidemiology and the exact number of patients with the disease who would benefit from treatment (patient horizon). Another challenge is the choice of the randomization procedure and the primary statistical analysis. In this study, one can enumerate upfront all 25 possible outcomes: {0, 1, 2, 3, 4} responders on active treatment, and {0, 1, 2, 3, 4} responders on placebo, and create a chart quantifying the level of evidence ( p- value) for each experimental outcome, and the corresponding decision. Before the trial starts, a discussion with the regulatory agency is warranted to agree upon on what level of evidence must be achieved in order to declare the study a “success”.
Let us perform a hypothetical planning for the given study. Suppose we go with a standard population-based approach, for which we test the hypothesis \({H}_{0}:{p}_{E}={p}_{C}\) vs. \({H}_{0}:{p}_{E}>{p}_{C}\) (where \({p}_{E}\) and \({p}_{C}\) stand for the true success rates for the experimental and control group, respectively) using Fisher’s exact test. Table 5 provides 1-sided p- values of all possible experimental outcomes. One could argue that a p- value < 0.1 may be viewed as a convincing level of evidence for this study. There are only 3 possibilities that can lead to this outcome: 3/4 vs. 0/4 successes ( p = 0.0714); 4/4 vs. 0/4 successes ( p = 0.0143); and 4/4 vs. 1/4 successes ( p = 0.0714). For all other outcomes, p ≥ 0.2143, and thus the study would be regarded as a “failure”.
Now let us consider a randomization-based inference approach. For illustration purposes, we consider four restricted randomization procedures—Rand, TBD, PBD(4), and PBD(2)—that exactly achieve 4:4 allocation. These procedures are legitimate choices because all of them provide exact sample sizes (4 per treatment group), which is essential in this trial. The reference set of either Rand or TBD includes \(70=\left(\begin{array}{c}8\\ 4\end{array}\right)\) unique sequences though with different probabilities of observing each sequence. For Rand, these sequences are equiprobable, whereas for TBD, some sequences are more likely than others. For PBD( \(2b\) ), the size of the reference set is \({\left\{\left(\begin{array}{c}2b\\ b\end{array}\right)\right\}}^{B}\) , where \(B=n/2b\) is the number of blocks of length \(2b\) for a trial of size \(n\) (in our example \(n=8\) ). This results in in a reference set of \({2}^{4}=16\) unique sequences with equal probability of 1/16 for PBD(2), and of \({6}^{2}=36\) unique sequences with equal probability of 1/36 for PBD(4).
In practice, the study statistician picks a treatment sequence at random from the reference set according to the chosen design. The details (randomization seed, chosen sequence, etc.) are carefully documented and kept confidential. For the chosen sequence and the observed outcome data, a randomization-based p- value is the sum of probabilities of all sequences in the reference set that yield the result at least as large in favor of the experimental treatment as the one observed. This p- value will depend on the randomization design, the observed randomization sequence and the observed outcomes, and it may also be different from the population-based analysis p- value.
To illustrate this, suppose the chosen randomization sequence is CEECECCE (C stands for control and E stands for experimental), and the observed responses are FSSFFFFS (F stands for failure and S stands for success). Thus, we have 3/4 successes on experimental and 0/4 successes on control. Then, the randomization-based p- value is 0.0714 for Rand; 0.0469 for TBD, 0.1250 for PBD(2); 0.0833 for PBD(4); and it is 0.0714 for the population-based analysis. The coincidence of the randomization-based p- value for Rand and the p- value of the population-based analysis is not surprising. Fisher's exact test is a permutation test and in the case of Rand as randomization procedure, the p- value of a permutation test and of a randomization test are always equal. However, despite the numerical equality, we should be mindful of different assumptions (population/randomization model).
Likewise, randomization-based p- values can be derived for other combinations of observed randomization sequences and responses. All these details (the chosen randomization design, the analysis strategy, and corresponding decisions) would have to be fully specified upfront (before the trial starts) and agreed upon by both the sponsor and the regulator. This would remove any ambiguity when the trial data become available.
As the example shows, the level of evidence in the randomization-based inference approach depends on the chosen randomization procedure and the resulting decisions may be different depending on the specific procedure. For instance, if the level of significance is set to 10% as a criterion for a “successful trial”, then with the observed data (3/4 vs. 0/4), there would be a significant test result for TBD, Rand, PBD(4), but not for PBD(2).
Summary and discussion
Randomization is the foundation of any RCT involving treatment comparison. Randomization is not a single technique, but a very broad class of statistical methodologies for design and analysis of clinical trials [ 10 ]. In this paper, we focused on the randomized controlled two-arm trial designed with equal allocation, which is the gold standard research design to generate clinical evidence in support of regulatory submissions. Even in this relatively simple case, there are various restricted randomization procedures with different probabilistic structures and different statistical properties, and the choice of a randomization design for any RCT must be made judiciously.
For the 1:1 RCT, there is a dual goal of balancing treatment assignments while maintaining allocation randomness. Final balance in treatment totals frequently maximizes statistical power for treatment comparison. It is also important to maintain balance at intermediate steps during the trial, especially in long-term studies, to mitigate potential for chronological bias. At the same time, a procedure should have high degree of randomness so that treatment assignments within the sequence are not easily predictable; otherwise, the procedure may be vulnerable to selection bias, especially in open-label studies. While balance and randomness are competing criteria, it is possible to find restricted randomization procedures that provide a sensible tradeoff between these criteria, e.g. the MTI procedures, of which the big stick design (BSD) [ 37 ] with a suitably chosen MTI limit, such as BSD(3), has very appealing statistical properties. In practice, the choice of a randomization procedure should be made after a systematic evaluation of different candidate procedures under different experimental scenarios for the primary outcome, including cases when model assumptions are violated.
In our considered examples we showed that the choice of randomization design, data analytic technique (e.g. parametric or nonparametric model, with or without covariate adjustment), and the decision on whether to include randomization in the analysis (e.g. randomization-based or population model-based analysis) are all very important considerations. Furthermore, these examples highlight the importance of using randomization designs that provide strong encryption of the randomization sequence, importance of covariate adjustment in the analysis, and the value of statistical thinking in nonstandard RCTs with very small sample sizes and small patient horizon. Finally, in this paper we have discussed randomization-based tests as robust and valid alternatives to likelihood-based tests. Randomization-based inference is a useful approach in clinical trials and should be considered by clinical researchers more frequently [ 14 ].
Further topics on randomization
Given the breadth of the subject of randomization, many important topics have been omitted from the current paper. Here we outline just a few of them.
In this paper, we have focused on the 1:1 RCT. However, clinical trials may involve more than two treatment arms. Extensions of equal randomization to the case of multiple treatment arms is relatively straightforward for many restricted randomization procedures [ 10 ]. Some trials with two or more treatment arms use unequal allocation (e.g. 2:1). Randomization procedures with unequal allocation ratios require careful consideration. For instance, an important and desirable feature is the allocation ratio preserving property (ARP). A randomization procedure targeting unequal allocation is said to be ARP, if at each allocation step the unconditional probability of a particular treatment assignment is the same as the target allocation proportion for this treatment [ 92 ]. Non-ARP procedures may have fluctuations in the unconditional randomization probability from allocation to allocation, which may be problematic [ 93 ]. Fortunately, some randomization procedures naturally possess the ARP property, and there are approaches to correct for a non-ARP deficiency – these should be considered in the design of RCTs with unequal allocation ratios [ 92 , 93 , 94 ].
In many RCTs, investigators may wish to prospectively balance treatment assignments with respect to important prognostic covariates. For a small number of categorical covariates one can use stratified randomization by applying separate MTI randomization procedures within strata [ 86 ]. However, a potential advantage of stratified randomization decreases as the number of stratification variables increases [ 95 ]. In trials where balance over a large number of covariates is sought and the sample size is small or moderate, one can consider covariate-adaptive randomization procedures that achieve balance within covariate margins, such as the minimization procedure [ 96 , 97 ], optimal model-based procedures [ 46 ], or some other covariate-adaptive randomization technique [ 98 ]. To achieve valid and powerful results, covariate-adaptive randomization design must be followed by covariate-adjusted analysis [ 99 ]. Special considerations are required for covariate-adaptive randomization designs with more than two treatment arms and/or unequal allocation ratios [ 100 ].
In some clinical research settings, such as trials for rare and/or life threatening diseases, there is a strong ethical imperative to increase the chance of a trial participant to receive an empirically better treatment. Response-adaptive randomization (RAR) has been increasingly considered in practice, especially in oncology [ 101 , 102 ]. Very extensive methodological research on RAR has been done [ 103 , 104 ]. RAR is increasingly viewed as an important ingredient of complex clinical trials such as umbrella and platform trial designs [ 105 , 106 ]. While RAR, when properly applied, has its merit, the topic has generated a lot of controversial discussions over the years [ 107 , 108 , 109 , 110 , 111 ]. Amid the ongoing COVID-19 pandemic, RCTs evaluating various experimental treatments for critically ill COVID-19 patients do incorporate RAR in their design; see, for example, the I-SPY COVID-19 trial ( https://clinicaltrials.gov/ct2/show/NCT04488081 ).
Randomization can also be applied more broadly than in conventional RCT settings where randomization units are individual subjects. For instance, in a cluster randomized trial, not individuals but groups of individuals (clusters) are randomized among one or more interventions or the control [ 112 ]. Observations from individuals within a given cluster cannot be regarded as independent, and special statistical techniques are required to design and analyze cluster-randomized experiments. In some clinical trial designs, randomization is applied within subjects. For instance, the micro-randomized trial (MRT) is a novel design for development of mobile treatment interventions in which randomization is applied to select different treatment options for individual participants over time to optimally support individuals’ health behaviors [ 113 ].
Finally, beyond the scope of the present paper are the regulatory perspectives on randomization and practical implementation aspects, including statistical software and information systems to generate randomization schedules in real time. We hope to cover these topics in subsequent papers.
Availability of data and materials
All results reported in this paper are based either on theoretical considerations or simulation evidence. The computer code (using R and Julia programming languages) is fully documented and is available upon reasonable request.
Guess the next allocation as the treatment with fewest allocations in the sequence thus far, or make a random guess if the treatment numbers are equal.
Byar DP, Simon RM, Friedewald WT, Schlesselman JJ, DeMets DL, Ellenberg JH, Gail MH, Ware JH. Randomized clinical trials—perspectives on some recent ideas. N Engl J Med. 1976;295:74–80.
Article CAS PubMed Google Scholar
Collins R, Bowman L, Landray M, Peto R. The magic of randomization versus the myth of real-world evidence. N Engl J Med. 2020;382:674–8.
Article PubMed Google Scholar
ICH Harmonised tripartite guideline. General considerations for clinical trials E8. 1997.
Hernán MA, Robins JM. Using big data to emulate a target trial when a randomized trial is not available. Am J Epidemiol. 2016;183(8):758–64.
Article PubMed PubMed Central Google Scholar
Byar DP. Why data bases should not replace randomized clinical trials. Biometrics. 1980;36:337–42.
Mehra MR, Desai SS, Kuy SR, Henry TD, Patel AN. Cardiovascular disease, drug therapy, and mortality in Covid-19. N Engl J Med. 2020;382:e102. https://www.nejm.org/doi/10.1056/NEJMoa2007621 .
Mehra MR, Desai SS, Ruschitzka F, Patel AN. Hydroxychloroquine or chloroquine with or without a macrolide for treatment of COVID-19: a multinational registry analysis. Lancet. 2020. https://www.sciencedirect.com/science/article/pii/S0140673620311806?via%3Dihub .
Mehra MR, Desai SS, Kuy SR, Henry TD, Patel AN. Retraction: Cardiovascular disease, drug therapy, and mortality in Covid-19. N Engl J Med. 2020. https://doi.org/10.1056/NEJMoa2007621 . https://www.nejm.org/doi/10.1056/NEJMc2021225 .
Medical Research Council. Streptomycin treatment of pulmonary tuberculosis. BMJ. 1948;2:769–82.
Article Google Scholar
Rosenberger WF, Lachin J. Randomization in clinical trials: theory and practice. 2nd ed. New York: Wiley; 2015.
Google Scholar
Fisher RA. The design of experiments. Edinburgh: Oliver and Boyd; 1935.
Hill AB. The clinical trial. Br Med Bull. 1951;7(4):278–82.
Hill AB. Memories of the British streptomycin trial in tuberculosis: the first randomized clinical trial. Control Clin Trials. 1990;11:77–9.
Rosenberger WF, Uschner D, Wang Y. Randomization: The forgotten component of the randomized clinical trial. Stat Med. 2019;38(1):1–30 (with discussion).
Berger VW. Trials: the worst possible design (except for all the rest). Int J Person Centered Med. 2011;1(3):630–1.
Berger VW. Selection bias and covariate imbalances in randomized clinical trials. New York: Wiley; 2005.
Book Google Scholar
Berger VW. The alleged benefits of unrestricted randomization. In: Berger VW, editor. Randomization, masking, and allocation concealment. Boca Raton: CRC Press; 2018. p. 39–50.
Altman DG, Bland JM. Treatment allocation in controlled trials: why randomise? BMJ. 1999;318:1209.
Article CAS PubMed PubMed Central Google Scholar
Senn S. Testing for baseline balance in clinical trials. Stat Med. 1994;13:1715–26.
Senn S. Seven myths of randomisation in clinical trials. Stat Med. 2013;32:1439–50.
Rosenberger WF, Sverdlov O. Handling covariates in the design of clinical trials. Stat Sci. 2008;23:404–19.
Proschan M, Dodd L. Re-randomization tests in clinical trials. Stat Med. 2019;38:2292–302.
Spiegelhalter DJ, Freedman LS, Parmar MK. Bayesian approaches to randomized trials. J R Stat Soc A Stat Soc. 1994;157(3):357–87.
Berry SM, Carlin BP, Lee JJ, Muller P. Bayesian adaptive methods for clinical trials. Boca Raton: CRC Press; 2010.
Lachin J. Properties of simple randomization in clinical trials. Control Clin Trials. 1988;9:312–26.
Pocock SJ. Allocation of patients to treatment in clinical trials. Biometrics. 1979;35(1):183–97.
Simon R. Restricted randomization designs in clinical trials. Biometrics. 1979;35(2):503–12.
Blackwell D, Hodges JL. Design for the control of selection bias. Ann Math Stat. 1957;28(2):449–60.
Matts JP, McHugh R. Analysis of accrual randomized clinical trials with balanced groups in strata. J Chronic Dis. 1978;31:725–40.
Matts JP, Lachin JM. Properties of permuted-block randomization in clinical trials. Control Clin Trials. 1988;9:327–44.
ICH Harmonised Tripartite Guideline. Statistical principles for clinical trials E9. 1998.
Shao H, Rosenberger WF. Properties of the random block design for clinical trials. In: Kunert J, Müller CH, Atkinson AC, eds. mODa 11 – Advances in model-oriented design and analysis. Springer International Publishing Switzerland; 2016. 225–233.
Zhao W. Evolution of restricted randomization with maximum tolerated imbalance. In: Berger VW, editor. Randomization, masking, and allocation concealment. Boca Raton: CRC Press; 2018. p. 61–81.
Bailey RA, Nelson PR. Hadamard randomization: a valid restriction of random permuted blocks. Biom J. 2003;45(5):554–60.
Berger VW, Ivanova A, Knoll MD. Minimizing predictability while retaining balance through the use of less restrictive randomization procedures. Stat Med. 2003;22:3017–28.
Zhao W, Berger VW, Yu Z. The asymptotic maximal procedure for subject randomization in clinical trials. Stat Methods Med Res. 2018;27(7):2142–53.
Soares JF, Wu CFJ. Some restricted randomization rules in sequential designs. Commun Stat Theory Methods. 1983;12(17):2017–34.
Chen YP. Biased coin design with imbalance tolerance. Commun Stat Stochastic Models. 1999;15(5):953–75.
Chen YP. Which design is better? Ehrenfest urn versus biased coin. Adv Appl Probab. 2000;32:738–49.
Zhao W, Weng Y. Block urn design—A new randomization algorithm for sequential trials with two or more treatments and balanced or unbalanced allocation. Contemp Clin Trials. 2011;32:953–61.
van der Pas SL. Merged block randomisation: A novel randomisation procedure for small clinical trials. Clin Trials. 2019;16(3):246–52.
Zhao W. Letter to the Editor – Selection bias, allocation concealment and randomization design in clinical trials. Contemp Clin Trials. 2013;36:263–5.
Berger VW, Bejleri K, Agnor R. Comparing MTI randomization procedures to blocked randomization. Stat Med. 2016;35:685–94.
Efron B. Forcing a sequential experiment to be balanced. Biometrika. 1971;58(3):403–17.
Wei LJ. The adaptive biased coin design for sequential experiments. Ann Stat. 1978;6(1):92–100.
Atkinson AC. Optimum biased coin designs for sequential clinical trials with prognostic factors. Biometrika. 1982;69(1):61–7.
Smith RL. Sequential treatment allocation using biased coin designs. J Roy Stat Soc B. 1984;46(3):519–43.
Ball FG, Smith AFM, Verdinelli I. Biased coin designs with a Bayesian bias. J Stat Planning Infer. 1993;34(3):403–21.
BaldiAntognini A, Giovagnoli A. A new ‘biased coin design’ for the sequential allocation of two treatments. Appl Stat. 2004;53(4):651–64.
Atkinson AC. Selecting a biased-coin design. Stat Sci. 2014;29(1):144–63.
Rosenberger WF. Randomized urn models and sequential design. Sequential Anal. 2002;21(1&2):1–41 (with discussion).
Wei LJ. A class of designs for sequential clinical trials. J Am Stat Assoc. 1977;72(358):382–6.
Wei LJ, Lachin JM. Properties of the urn randomization in clinical trials. Control Clin Trials. 1988;9:345–64.
Schouten HJA. Adaptive biased urn randomization in small strata when blinding is impossible. Biometrics. 1995;51(4):1529–35.
Ivanova A. A play-the-winner-type urn design with reduced variability. Metrika. 2003;58:1–13.
Kundt G. A new proposal for setting parameter values in restricted randomization methods. Methods Inf Med. 2007;46(4):440–9.
Kalish LA, Begg CB. Treatment allocation methods in clinical trials: a review. Stat Med. 1985;4:129–44.
Zhao W, Weng Y, Wu Q, Palesch Y. Quantitative comparison of randomization designs in sequential clinical trials based on treatment balance and allocation randomness. Pharm Stat. 2012;11:39–48.
Flournoy N, Haines LM, Rosenberger WF. A graphical comparison of response-adaptive randomization procedures. Statistics in Biopharmaceutical Research. 2013;5(2):126–41.
Hilgers RD, Uschner D, Rosenberger WF, Heussen N. ERDO – a framework to select an appropriate randomization procedure for clinical trials. BMC Med Res Methodol. 2017;17:159.
Burman CF. On sequential treatment allocations in clinical trials. PhD Thesis Dept. Mathematics, Göteborg. 1996.
Azriel D, Mandel M, Rinott Y. Optimal allocation to maximize the power of two-sample tests for binary response. Biometrika. 2012;99(1):101–13.
Begg CB, Kalish LA. Treatment allocation for nonlinear models in clinical trials: the logistic model. Biometrics. 1984;40:409–20.
Kalish LA, Harrington DP. Efficiency of balanced treatment allocation for survival analysis. Biometrics. 1988;44(3):815–21.
Sverdlov O, Rosenberger WF. On recent advances in optimal allocation designs for clinical trials. J Stat Theory Practice. 2013;7(4):753–73.
Sverdlov O, Ryeznik Y, Wong WK. On optimal designs for clinical trials: an updated review. J Stat Theory Pract. 2020;14:10.
Rosenkranz GK. The impact of randomization on the analysis of clinical trials. Stat Med. 2011;30:3475–87.
Galbete A, Rosenberger WF. On the use of randomization tests following adaptive designs. J Biopharm Stat. 2016;26(3):466–74.
Proschan M. Influence of selection bias on type I error rate under random permuted block design. Stat Sin. 1994;4:219–31.
Kennes LN, Cramer E, Hilgers RD, Heussen N. The impact of selection bias on test decisions in randomized clinical trials. Stat Med. 2011;30:2573–81.
PubMed Google Scholar
Rückbeil MV, Hilgers RD, Heussen N. Assessing the impact of selection bias on test decisions in trials with a time-to-event outcome. Stat Med. 2017;36:2656–68.
Berger VW, Exner DV. Detecting selection bias in randomized clinical trials. Control Clin Trials. 1999;25:515–24.
Ivanova A, Barrier RC, Berger VW. Adjusting for observable selection bias in block randomized trials. Stat Med. 2005;24:1537–46.
Kennes LN, Rosenberger WF, Hilgers RD. Inference for blocked randomization under a selection bias model. Biometrics. 2015;71:979–84.
Hilgers RD, Manolov M, Heussen N, Rosenberger WF. Design and analysis of stratified clinical trials in the presence of bias. Stat Methods Med Res. 2020;29(6):1715–27.
Hamilton SA. Dynamically allocating treatment when the cost of goods is high and drug supply is limited. Control Clin Trials. 2000;21(1):44–53.
Zhao W. Letter to the Editor – A better alternative to the inferior permuted block design is not necessarily complex. Stat Med. 2016;35:1736–8.
Berger VW. Pros and cons of permutation tests in clinical trials. Stat Med. 2000;19:1319–28.
Simon R, Simon NR. Using randomization tests to preserve type I error with response adaptive and covariate adaptive randomization. Statist Probab Lett. 2011;81:767–72.
Tamm M, Cramer E, Kennes LN, Hilgers RD. Influence of selection bias on the test decision. Methods Inf Med. 2012;51:138–43.
Tamm M, Hilgers RD. Chronological bias in randomized clinical trials arising from different types of unobserved time trends. Methods Inf Med. 2014;53:501–10.
BaldiAntognini A, Rosenberger WF, Wang Y, Zagoraiou M. Exact optimum coin bias in Efron’s randomization procedure. Stat Med. 2015;34:3760–8.
Chow SC, Shao J, Wang H, Lokhnygina. Sample size calculations in clinical research. 3rd ed. Boca Raton: CRC Press; 2018.
Heritier S, Gebski V, Pillai A. Dynamic balancing randomization in controlled clinical trials. Stat Med. 2005;24:3729–41.
Lovell DJ, Giannini EH, Reiff A, et al. Etanercept in children with polyarticular juvenile rheumatoid arthritis. N Engl J Med. 2000;342(11):763–9.
Zhao W. A better alternative to stratified permuted block design for subject randomization in clinical trials. Stat Med. 2014;33:5239–48.
Altman DG, Royston JP. The hidden effect of time. Stat Med. 1988;7:629–37.
Christensen E, Neuberger J, Crowe J, et al. Beneficial effect of azathioprine and prediction of prognosis in primary biliary cirrhosis. Gastroenterology. 1985;89:1084–91.
Rückbeil MV, Hilgers RD, Heussen N. Randomization in survival trials: An evaluation method that takes into account selection and chronological bias. PLoS ONE. 2019;14(6):e0217964.
Article CAS Google Scholar
Hilgers RD, König F, Molenberghs G, Senn S. Design and analysis of clinical trials for small rare disease populations. J Rare Dis Res Treatment. 2016;1(3):53–60.
Miller F, Zohar S, Stallard N, Madan J, Posch M, Hee SW, Pearce M, Vågerö M, Day S. Approaches to sample size calculation for clinical trials in rare diseases. Pharm Stat. 2017;17:214–30.
Kuznetsova OM, Tymofyeyev Y. Preserving the allocation ratio at every allocation with biased coin randomization and minimization in studies with unequal allocation. Stat Med. 2012;31(8):701–23.
Kuznetsova OM, Tymofyeyev Y. Brick tunnel and wide brick tunnel randomization for studies with unequal allocation. In: Sverdlov O, editor. Modern adaptive randomized clinical trials: statistical and practical aspects. Boca Raton: CRC Press; 2015. p. 83–114.
Kuznetsova OM, Tymofyeyev Y. Expansion of the modified Zelen’s approach randomization and dynamic randomization with partial block supplies at the centers to unequal allocation. Contemp Clin Trials. 2011;32:962–72.
EMA. Guideline on adjustment for baseline covariates in clinical trials. 2015.
Taves DR. Minimization: A new method of assigning patients to treatment and control groups. Clin Pharmacol Ther. 1974;15(5):443–53.
Pocock SJ, Simon R. Sequential treatment assignment with balancing for prognostic factors in the controlled clinical trial. Biometrics. 1975;31(1):103–15.
Hu F, Hu Y, Ma Z, Rosenberger WF. Adaptive randomization for balancing over covariates. Wiley Interdiscipl Rev Computational Stat. 2014;6(4):288–303.
Senn S. Statistical issues in drug development. 2nd ed. Wiley-Interscience; 2007.
Kuznetsova OM, Tymofyeyev Y. Covariate-adaptive randomization with unequal allocation. In: Sverdlov O, editor. Modern adaptive randomized clinical trials: statistical and practical aspects. Boca Raton: CRC Press; 2015. p. 171–97.
Berry DA. Adaptive clinical trials: the promise and the caution. J Clin Oncol. 2011;29(6):606–9.
Trippa L, Lee EQ, Wen PY, Batchelor TT, Cloughesy T, Parmigiani G, Alexander BM. Bayesian adaptive randomized trial design for patients with recurrent glioblastoma. J Clin Oncol. 2012;30(26):3258–63.
Hu F, Rosenberger WF. The theory of response-adaptive randomization in clinical trials. New York: Wiley; 2006.
Atkinson AC, Biswas A. Randomised response-adaptive designs in clinical trials. Boca Raton: CRC Press; 2014.
Rugo HS, Olopade OI, DeMichele A, et al. Adaptive randomization of veliparib–carboplatin treatment in breast cancer. N Engl J Med. 2016;375:23–34.
Berry SM, Petzold EA, Dull P, et al. A response-adaptive randomization platform trial for efficient evaluation of Ebola virus treatments: a model for pandemic response. Clin Trials. 2016;13:22–30.
Ware JH. Investigating therapies of potentially great benefit: ECMO. (with discussion). Stat Sci. 1989;4(4):298–340.
Hey SP, Kimmelman J. Are outcome-adaptive allocation trials ethical? (with discussion). Clin Trials. 2005;12(2):102–27.
Proschan M, Evans S. Resist the temptation of response-adaptive randomization. Clin Infect Dis. 2020;71(11):3002–4. https://doi.org/10.1093/cid/ciaa334 .
Villar SS, Robertson DS, Rosenberger WF. The temptation of overgeneralizing response-adaptive randomization. Clinical Infectious Diseases. 2020; ciaa1027; doi: https://doi.org/10.1093/cid/ciaa1027 .
Proschan M. Reply to Villar, et al. Clinical infectious diseases. 2020; ciaa1029; doi: https://doi.org/10.1093/cid/ciaa1029 .
Donner A, Klar N. Design and Analysis of Cluster Randomization Trials in Health Research. London: Arnold Publishers Limited; 2000.
Klasnja P, Hekler EB, Shiffman S, Boruvka A, Almirall D, Tewari A, Murphy SA. Micro-randomized trials: An experimental design for developing just-in-time adaptive interventions. Health Psychol. 2015;34:1220–8.
Article PubMed Central Google Scholar
Download references
Acknowledgements
The authors are grateful to Robert A. Beckman for his continuous efforts coordinating Innovative Design Scientific Working Groups, which is also a networking research platform for the Randomization ID SWG. We would also like to thank the editorial board and the two anonymous reviewers for the valuable comments which helped to substantially improve the original version of the manuscript.
None. The opinions expressed in this article are those of the authors and may not reflect the opinions of the organizations that they work for.
Author information
Authors and affiliations.
National Institutes of Health, Bethesda, MD, USA
Vance W. Berger
Boehringer Ingelheim Pharma GmbH & Co. KG, Biberach, Germany
Louis Joseph Bour
Boehringer-Ingelheim Pharmaceuticals Inc, Ridgefield, CT, USA
Kerstine Carter
Population Health Sciences, University of Utah School of Medicine, Salt Lake City UT, USA
Jonathan J. Chipman
Cancer Biostatistics, University of Utah Huntsman Cancer Institute, Salt Lake City UT, USA
Clinical Trials Research Unit, University of Leeds, Leeds, UK
Colin C. Everett
RWTH Aachen University, Aachen, Germany
Nicole Heussen & Ralf-Dieter Hilgers
Medical School, Sigmund Freud University, Vienna, Austria
Nicole Heussen
York Trials Unit, Department of Health Sciences, University of York, York, UK
Catherine Hewitt
Food and Drug Administration, Silver Spring, MD, USA
Yuqun Abigail Luo
Open University of Catalonia (UOC) and the University of Barcelona (UB), Barcelona, Spain
Jone Renteria
Department of Human Development and Quantitative Methodology, University of Maryland, College Park, MD, USA
BioPharma Early Biometrics & Statistical Innovations, Data Science & AI, R&D BioPharmaceuticals, AstraZeneca, Gothenburg, Sweden
Yevgen Ryeznik
Early Development Analytics, Novartis Pharmaceuticals Corporation, NJ, East Hanover, USA
Oleksandr Sverdlov
Biostatistics Center & Department of Biostatistics and Bioinformatics, George Washington University, DC, Washington, USA
Diane Uschner
You can also search for this author in PubMed Google Scholar
- Robert A Beckman
Contributions
Conception: VWB, KC, NH, RDH, OS. Writing of the main manuscript: OS, with contributions from VWB, KC, JJC, CE, NH, and RDH. Design of simulation studies: OS, YR. Development of code and running simulations: YR. Digitization and preparation of data for Fig. 5 : JR. All authors reviewed the original manuscript and the revised version. The authors read and approved the final manuscript.
Corresponding author
Correspondence to Oleksandr Sverdlov .
Ethics declarations
Ethics approval and consent to participate.
Not applicable.
Consent for publication
Competing interests, additional information, publisher's note.
Springer Nature remains neutral with regard to jurisdictional claims in published maps and institutional affiliations.
Supplementary Information
Additional file 1: figure s1.
. Type I error rate under selection bias model with bias effect ( \(\nu\) ) in the range 0 (no bias) to 1 (strong bias) for 12 randomization designs and three statistical tests.
Rights and permissions
Open Access This article is licensed under a Creative Commons Attribution 4.0 International License, which permits use, sharing, adaptation, distribution and reproduction in any medium or format, as long as you give appropriate credit to the original author(s) and the source, provide a link to the Creative Commons licence, and indicate if changes were made. The images or other third party material in this article are included in the article's Creative Commons licence, unless indicated otherwise in a credit line to the material. If material is not included in the article's Creative Commons licence and your intended use is not permitted by statutory regulation or exceeds the permitted use, you will need to obtain permission directly from the copyright holder. To view a copy of this licence, visit http://creativecommons.org/licenses/by/4.0/ . The Creative Commons Public Domain Dedication waiver ( http://creativecommons.org/publicdomain/zero/1.0/ ) applies to the data made available in this article, unless otherwise stated in a credit line to the data.
Reprints and permissions
About this article
Cite this article.
Berger, V., Bour, L., Carter, K. et al. A roadmap to using randomization in clinical trials. BMC Med Res Methodol 21 , 168 (2021). https://doi.org/10.1186/s12874-021-01303-z
Download citation
Received : 24 December 2020
Accepted : 14 April 2021
Published : 16 August 2021
DOI : https://doi.org/10.1186/s12874-021-01303-z
Share this article
Anyone you share the following link with will be able to read this content:
Sorry, a shareable link is not currently available for this article.
Provided by the Springer Nature SharedIt content-sharing initiative
- Randomization-based test
- Restricted randomization design
BMC Medical Research Methodology
ISSN: 1471-2288
- General enquiries: [email protected]
- Download PDF
- CME & MOC
- Share X Facebook Email LinkedIn
- Permissions
Sequential, Multiple Assignment, Randomized Trial Designs
- 1 Department of Biostatistics, School of Public Health, University of Michigan, Ann Arbor
- 2 Survey Research Center, Institute for Social Research, University of Michigan, Ann Arbor
- 3 Department of Statistics, University of Michigan, Ann Arbor
- JAMA Guide to Statistics and Methods Collider Bias Mathias J. Holmberg, MD, MPH, PhD; Lars W. Andersen, MD, MPH, PhD, DMSc JAMA
- Special Communication Reporting of Factorial Randomized Trials Brennan C. Kahan, PhD; Sophie S. Hall, PhD; Elaine M. Beller, MAppStat; Megan Birchenall, BSc; An-Wen Chan, MD, DPhil; Diana Elbourne, PhD; Paul Little, MD; John Fletcher, MPH; Robert M. Golub, MD; Beatriz Goulao, PhD; Sally Hopewell, DPhil; Nazrul Islam, PhD; Merrick Zwarenstein, MBBCh, PhD; Edmund Juszczak, MSc; Alan A. Montgomery, PhD JAMA
- Original Investigation Comparison of Teleintegrated Care and Telereferral Care for Treating Complex Psychiatric Disorders in Primary Care John C. Fortney, PhD; Amy M. Bauer, MS, MD; Joseph M. Cerimele, MPH, MD; Jeffrey M. Pyne, MD; Paul Pfeiffer, MD; Patrick J. Heagerty, PhD; Matt Hawrilenko, PhD; Melissa J. Zielinski, PhD; Debra Kaysen, PhD; Deborah J. Bowen, PhD; Danna L. Moore, PhD; Lori Ferro, MHA; Karla Metzger, MSW; Stephanie Shushan, MHA; Erin Hafer, MPH; John Paul Nolan, AAS; Gregory W. Dalack, MD; Jürgen Unützer, MPH, MD JAMA Psychiatry
An adaptive intervention is a set of diagnostic, preventive, therapeutic, or engagement strategies that are used in stages, and the selection of the intervention at each stage is based on defined decision rules. At the beginning of each stage in care, treatment may be changed by the clinician to suit the needs of the patient. Typical adaptations include intensifying an ongoing treatment or adding or switching to another treatment. These decisions are made in response to changes in the patient’s status, such as a patient’s early response to, or engagement with, a prior treatment. The patient experiences an adaptive intervention as a sequence of personalized treatments.
Read More About
Kidwell KM , Almirall D. Sequential, Multiple Assignment, Randomized Trial Designs. JAMA. 2023;329(4):336–337. doi:10.1001/jama.2022.24324
Manage citations:
© 2024
Artificial Intelligence Resource Center
Cardiology in JAMA : Read the Latest
Browse and subscribe to JAMA Network podcasts!
Others Also Liked
Select your interests.
Customize your JAMA Network experience by selecting one or more topics from the list below.
- Academic Medicine
- Acid Base, Electrolytes, Fluids
- Allergy and Clinical Immunology
- American Indian or Alaska Natives
- Anesthesiology
- Anticoagulation
- Art and Images in Psychiatry
- Artificial Intelligence
- Assisted Reproduction
- Bleeding and Transfusion
- Caring for the Critically Ill Patient
- Challenges in Clinical Electrocardiography
- Climate and Health
- Climate Change
- Clinical Challenge
- Clinical Decision Support
- Clinical Implications of Basic Neuroscience
- Clinical Pharmacy and Pharmacology
- Complementary and Alternative Medicine
- Consensus Statements
- Coronavirus (COVID-19)
- Critical Care Medicine
- Cultural Competency
- Dental Medicine
- Dermatology
- Diabetes and Endocrinology
- Diagnostic Test Interpretation
- Drug Development
- Electronic Health Records
- Emergency Medicine
- End of Life, Hospice, Palliative Care
- Environmental Health
- Equity, Diversity, and Inclusion
- Facial Plastic Surgery
- Gastroenterology and Hepatology
- Genetics and Genomics
- Genomics and Precision Health
- Global Health
- Guide to Statistics and Methods
- Hair Disorders
- Health Care Delivery Models
- Health Care Economics, Insurance, Payment
- Health Care Quality
- Health Care Reform
- Health Care Safety
- Health Care Workforce
- Health Disparities
- Health Inequities
- Health Policy
- Health Systems Science
- History of Medicine
- Hypertension
- Images in Neurology
- Implementation Science
- Infectious Diseases
- Innovations in Health Care Delivery
- JAMA Infographic
- Law and Medicine
- Leading Change
- Less is More
- LGBTQIA Medicine
- Lifestyle Behaviors
- Medical Coding
- Medical Devices and Equipment
- Medical Education
- Medical Education and Training
- Medical Journals and Publishing
- Mobile Health and Telemedicine
- Narrative Medicine
- Neuroscience and Psychiatry
- Notable Notes
- Nutrition, Obesity, Exercise
- Obstetrics and Gynecology
- Occupational Health
- Ophthalmology
- Orthopedics
- Otolaryngology
- Pain Medicine
- Palliative Care
- Pathology and Laboratory Medicine
- Patient Care
- Patient Information
- Performance Improvement
- Performance Measures
- Perioperative Care and Consultation
- Pharmacoeconomics
- Pharmacoepidemiology
- Pharmacogenetics
- Pharmacy and Clinical Pharmacology
- Physical Medicine and Rehabilitation
- Physical Therapy
- Physician Leadership
- Population Health
- Primary Care
- Professional Well-being
- Professionalism
- Psychiatry and Behavioral Health
- Public Health
- Pulmonary Medicine
- Regulatory Agencies
- Reproductive Health
- Research, Methods, Statistics
- Resuscitation
- Rheumatology
- Risk Management
- Scientific Discovery and the Future of Medicine
- Shared Decision Making and Communication
- Sleep Medicine
- Sports Medicine
- Stem Cell Transplantation
- Substance Use and Addiction Medicine
- Surgical Innovation
- Surgical Pearls
- Teachable Moment
- Technology and Finance
- The Art of JAMA
- The Arts and Medicine
- The Rational Clinical Examination
- Tobacco and e-Cigarettes
- Translational Medicine
- Trauma and Injury
- Treatment Adherence
- Ultrasonography
- Users' Guide to the Medical Literature
- Vaccination
- Venous Thromboembolism
- Veterans Health
- Women's Health
- Workflow and Process
- Wound Care, Infection, Healing
- Register for email alerts with links to free full-text articles
- Access PDFs of free articles
- Manage your interests
- Save searches and receive search alerts
- Skip to secondary menu
- Skip to main content
- Skip to primary sidebar
Statistics By Jim
Making statistics intuitive
Random Assignment in Experiments
By Jim Frost 4 Comments
Random assignment uses chance to assign subjects to the control and treatment groups in an experiment. This process helps ensure that the groups are equivalent at the beginning of the study, which makes it safer to assume the treatments caused any differences between groups that the experimenters observe at the end of the study.

Huh? That might be a big surprise! At this point, you might be wondering about all of those studies that use statistics to assess the effects of different treatments. There’s a critical separation between significance and causality:
- Statistical procedures determine whether an effect is significant.
- Experimental designs determine how confidently you can assume that a treatment causes the effect.
In this post, learn how using random assignment in experiments can help you identify causal relationships.
Correlation, Causation, and Confounding Variables
Random assignment helps you separate causation from correlation and rule out confounding variables. As a critical component of the scientific method , experiments typically set up contrasts between a control group and one or more treatment groups. The idea is to determine whether the effect, which is the difference between a treatment group and the control group, is statistically significant. If the effect is significant, group assignment correlates with different outcomes.
However, as you have no doubt heard, correlation does not necessarily imply causation. In other words, the experimental groups can have different mean outcomes, but the treatment might not be causing those differences even though the differences are statistically significant.
The difficulty in definitively stating that a treatment caused the difference is due to potential confounding variables or confounders. Confounders are alternative explanations for differences between the experimental groups. Confounding variables correlate with both the experimental groups and the outcome variable. In this situation, confounding variables can be the actual cause for the outcome differences rather than the treatments themselves. As you’ll see, if an experiment does not account for confounding variables, they can bias the results and make them untrustworthy.
Related posts : Understanding Correlation in Statistics , Causation versus Correlation , and Hill’s Criteria for Causation .
Example of Confounding in an Experiment

- Control group: Does not consume vitamin supplements
- Treatment group: Regularly consumes vitamin supplements.
Imagine we measure a specific health outcome. After the experiment is complete, we perform a 2-sample t-test to determine whether the mean outcomes for these two groups are different. Assume the test results indicate that the mean health outcome in the treatment group is significantly better than the control group.
Why can’t we assume that the vitamins improved the health outcomes? After all, only the treatment group took the vitamins.
Related post : Confounding Variables in Regression Analysis
Alternative Explanations for Differences in Outcomes
The answer to that question depends on how we assigned the subjects to the experimental groups. If we let the subjects decide which group to join based on their existing vitamin habits, it opens the door to confounding variables. It’s reasonable to assume that people who take vitamins regularly also tend to have other healthy habits. These habits are confounders because they correlate with both vitamin consumption (experimental group) and the health outcome measure.
Random assignment prevents this self sorting of participants and reduces the likelihood that the groups start with systematic differences.
In fact, studies have found that supplement users are more physically active, have healthier diets, have lower blood pressure, and so on compared to those who don’t take supplements. If subjects who already take vitamins regularly join the treatment group voluntarily, they bring these healthy habits disproportionately to the treatment group. Consequently, these habits will be much more prevalent in the treatment group than the control group.
The healthy habits are the confounding variables—the potential alternative explanations for the difference in our study’s health outcome. It’s entirely possible that these systematic differences between groups at the start of the study might cause the difference in the health outcome at the end of the study—and not the vitamin consumption itself!
If our experiment doesn’t account for these confounding variables, we can’t trust the results. While we obtained statistically significant results with the 2-sample t-test for health outcomes, we don’t know for sure whether the vitamins, the systematic difference in habits, or some combination of the two caused the improvements.
Learn why many randomized clinical experiments use a placebo to control for the Placebo Effect .
Experiments Must Account for Confounding Variables
Your experimental design must account for confounding variables to avoid their problems. Scientific studies commonly use the following methods to handle confounders:
- Use control variables to keep them constant throughout an experiment.
- Statistically control for them in an observational study.
- Use random assignment to reduce the likelihood that systematic differences exist between experimental groups when the study begins.
Let’s take a look at how random assignment works in an experimental design.
Random Assignment Can Reduce the Impact of Confounding Variables
Note that random assignment is different than random sampling. Random sampling is a process for obtaining a sample that accurately represents a population .

Random assignment uses a chance process to assign subjects to experimental groups. Using random assignment requires that the experimenters can control the group assignment for all study subjects. For our study, we must be able to assign our participants to either the control group or the supplement group. Clearly, if we don’t have the ability to assign subjects to the groups, we can’t use random assignment!
Additionally, the process must have an equal probability of assigning a subject to any of the groups. For example, in our vitamin supplement study, we can use a coin toss to assign each subject to either the control group or supplement group. For more complex experimental designs, we can use a random number generator or even draw names out of a hat.
Random Assignment Distributes Confounders Equally
The random assignment process distributes confounding properties amongst your experimental groups equally. In other words, randomness helps eliminate systematic differences between groups. For our study, flipping the coin tends to equalize the distribution of subjects with healthier habits between the control and treatment group. Consequently, these two groups should start roughly equal for all confounding variables, including healthy habits!
Random assignment is a simple, elegant solution to a complex problem. For any given study area, there can be a long list of confounding variables that you could worry about. However, using random assignment, you don’t need to know what they are, how to detect them, or even measure them. Instead, use random assignment to equalize them across your experimental groups so they’re not a problem.
Because random assignment helps ensure that the groups are comparable when the experiment begins, you can be more confident that the treatments caused the post-study differences. Random assignment helps increase the internal validity of your study.
Comparing the Vitamin Study With and Without Random Assignment
Let’s compare two scenarios involving our hypothetical vitamin study. We’ll assume that the study obtains statistically significant results in both cases.
Scenario 1: We don’t use random assignment and, unbeknownst to us, subjects with healthier habits disproportionately end up in the supplement treatment group. The experimental groups differ by both healthy habits and vitamin consumption. Consequently, we can’t determine whether it was the habits or vitamins that improved the outcomes.
Scenario 2: We use random assignment and, consequently, the treatment and control groups start with roughly equal levels of healthy habits. The intentional introduction of vitamin supplements in the treatment group is the primary difference between the groups. Consequently, we can more confidently assert that the supplements caused an improvement in health outcomes.
For both scenarios, the statistical results could be identical. However, the methodology behind the second scenario makes a stronger case for a causal relationship between vitamin supplement consumption and health outcomes.
How important is it to use the correct methodology? Well, if the relationship between vitamins and health outcomes is not causal, then consuming vitamins won’t cause your health outcomes to improve regardless of what the study indicates. Instead, it’s probably all the other healthy habits!
Learn more about Randomized Controlled Trials (RCTs) that are the gold standard for identifying causal relationships because they use random assignment.
Drawbacks of Random Assignment
Random assignment helps reduce the chances of systematic differences between the groups at the start of an experiment and, thereby, mitigates the threats of confounding variables and alternative explanations. However, the process does not always equalize all of the confounding variables. Its random nature tends to eliminate systematic differences, but it doesn’t always succeed.
Sometimes random assignment is impossible because the experimenters cannot control the treatment or independent variable. For example, if you want to determine how individuals with and without depression perform on a test, you cannot randomly assign subjects to these groups. The same difficulty occurs when you’re studying differences between genders.
In other cases, there might be ethical issues. For example, in a randomized experiment, the researchers would want to withhold treatment for the control group. However, if the treatments are vaccinations, it might be unethical to withhold the vaccinations.
Other times, random assignment might be possible, but it is very challenging. For example, with vitamin consumption, it’s generally thought that if vitamin supplements cause health improvements, it’s only after very long-term use. It’s hard to enforce random assignment with a strict regimen for usage in one group and non-usage in the other group over the long-run. Or imagine a study about smoking. The researchers would find it difficult to assign subjects to the smoking and non-smoking groups randomly!
Fortunately, if you can’t use random assignment to help reduce the problem of confounding variables, there are different methods available. The other primary approach is to perform an observational study and incorporate the confounders into the statistical model itself. For more information, read my post Observational Studies Explained .
Read About Real Experiments that Used Random Assignment
I’ve written several blog posts about studies that have used random assignment to make causal inferences. Read studies about the following:
- Flu Vaccinations
- COVID-19 Vaccinations
Sullivan L. Random assignment versus random selection . SAGE Glossary of the Social and Behavioral Sciences, SAGE Publications, Inc.; 2009.
Share this:

Reader Interactions
November 13, 2019 at 4:59 am
Hi Jim, I have a question of randomly assigning participants to one of two conditions when it is an ongoing study and you are not sure of how many participants there will be. I am using this random assignment tool for factorial experiments. http://methodologymedia.psu.edu/most/rannumgenerator It asks you for the total number of participants but at this point, I am not sure how many there will be. Thanks for any advice you can give me, Floyd
May 28, 2019 at 11:34 am
Jim, can you comment on the validity of using the following approach when we can’t use random assignments. I’m in education, we have an ACT prep course that we offer. We can’t force students to take it and we can’t keep them from taking it either. But we want to know if it’s working. Let’s say that by senior year all students who are going to take the ACT have taken it. Let’s also say that I’m only including students who have taking it twice (so I can show growth between first and second time taking it). What I’ve done to address confounders is to go back to say 8th or 9th grade (prior to anyone taking the ACT or the ACT prep course) and run an analysis showing the two groups are not significantly different to start with. Is this valid? If the ACT prep students were higher achievers in 8th or 9th grade, I could not assume my prep course is effecting greater growth, but if they were not significantly different in 8th or 9th grade, I can assume the significant difference in ACT growth (from first to second testing) is due to the prep course. Yes or no?
May 26, 2019 at 5:37 pm
Nice post! I think the key to understanding scientific research is to understand randomization. And most people don’t get it.
May 27, 2019 at 9:48 pm
Thank you, Anoop!
I think randomness in an experiment is a funny thing. The issue of confounding factors is a serious problem. You might not even know what they are! But, use random assignment and, voila, the problem usually goes away! If you can’t use random assignment, suddenly you have a whole host of issues to worry about, which I’ll be writing about in more detail in my upcoming post about observational experiments!
Comments and Questions Cancel reply
What is a Randomized Control Trial (RCT)?
Julia Simkus
Editor at Simply Psychology
BA (Hons) Psychology, Princeton University
Julia Simkus is a graduate of Princeton University with a Bachelor of Arts in Psychology. She is currently studying for a Master's Degree in Counseling for Mental Health and Wellness in September 2023. Julia's research has been published in peer reviewed journals.
Learn about our Editorial Process
Saul McLeod, PhD
Editor-in-Chief for Simply Psychology
BSc (Hons) Psychology, MRes, PhD, University of Manchester
Saul McLeod, PhD., is a qualified psychology teacher with over 18 years of experience in further and higher education. He has been published in peer-reviewed journals, including the Journal of Clinical Psychology.
Olivia Guy-Evans, MSc
Associate Editor for Simply Psychology
BSc (Hons) Psychology, MSc Psychology of Education
Olivia Guy-Evans is a writer and associate editor for Simply Psychology. She has previously worked in healthcare and educational sectors.
On This Page:
A randomized control trial (RCT) is a type of study design that involves randomly assigning participants to either an experimental group or a control group to measure the effectiveness of an intervention or treatment.
Randomized Controlled Trials (RCTs) are considered the “gold standard” in medical and health research due to their rigorous design.
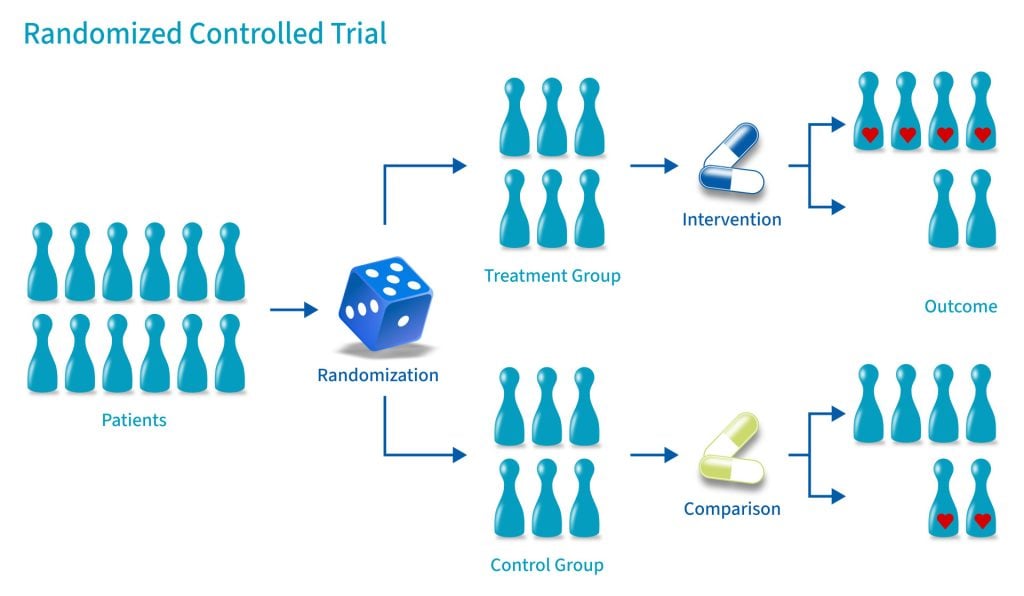
Control Group
A control group consists of participants who do not receive any treatment or intervention but a placebo or reference treatment. The control participants serve as a comparison group.
The control group is matched as closely as possible to the experimental group, including age, gender, social class, ethnicity, etc.
Because the participants are randomly assigned, the characteristics between the two groups should be balanced, enabling researchers to attribute any differences in outcome to the study intervention.
Since researchers can be confident that any differences between the control and treatment groups are due solely to the effects of the treatments, scientists view RCTs as the gold standard for clinical trials.
Random Allocation
Random allocation and random assignment are terms used interchangeably in the context of a randomized controlled trial (RCT).
Both refer to assigning participants to different groups in a study (such as a treatment group or a control group) in a way that is completely determined by chance.
The process of random assignment controls for confounding variables , ensuring differences between groups are due to chance alone.
Without randomization, researchers might consciously or subconsciously assign patients to a particular group for various reasons.
Several methods can be used for randomization in a Randomized Control Trial (RCT). Here are a few examples:
- Simple Randomization: This is the simplest method, like flipping a coin. Each participant has an equal chance of being assigned to any group. This can be achieved using random number tables, computerized random number generators, or drawing lots or envelopes.
- Block Randomization: In this method, participants are randomized within blocks, ensuring that each block has an equal number of participants in each group. This helps to balance the number of participants in each group at any given time during the study.
- Stratified Randomization: This method is used when researchers want to ensure that certain subgroups of participants are equally represented in each group. Participants are divided into strata, or subgroups, based on characteristics like age or disease severity, and then randomized within these strata.
- Cluster Randomization: In this method, groups of participants (like families or entire communities), rather than individuals, are randomized.
- Adaptive Randomization: In this method, the probability of being assigned to each group changes based on the participants already assigned to each group. For example, if more participants have been assigned to the control group, new participants will have a higher probability of being assigned to the experimental group.
Computer software can generate random numbers or sequences that can be used to assign participants to groups in a simple randomization process.
For more complex methods like block, stratified, or adaptive randomization, computer algorithms can be used to consider the additional parameters and ensure that participants are assigned to groups appropriately.
Using a computerized system can also help to maintain the integrity of the randomization process by preventing researchers from knowing in advance which group a participant will be assigned to (a principle known as allocation concealment). This can help to prevent selection bias and ensure the validity of the study results .
Allocation Concealment
Allocation concealment is a technique to ensure the random allocation process is truly random and unbiased.
RCTs use allocation concealment to decide which patients get the real medicine and which get a placebo (a fake medicine)
It involves keeping the sequence of group assignments (i.e., who gets assigned to the treatment group and who gets assigned to the control group next) hidden from the researchers before a participant has enrolled in the study.
This helps to prevent the researchers from consciously or unconsciously selecting certain participants for one group or the other based on their knowledge of which group is next in the sequence.
Allocation concealment ensures that the investigator does not know in advance which treatment the next person will get, thus maintaining the integrity of the randomization process.
Blinding (Masking)
Binding, or masking, refers to withholding information regarding the group assignments (who is in the treatment group and who is in the control group) from the participants, the researchers, or both during the study .
A blinded study prevents the participants from knowing about their treatment to avoid bias in the research. Any information that can influence the subjects is withheld until the completion of the research.
Blinding can be imposed on any participant in an experiment, including researchers, data collectors, evaluators, technicians, and data analysts.
Good blinding can eliminate experimental biases arising from the subjects’ expectations, observer bias, confirmation bias, researcher bias, observer’s effect on the participants, and other biases that may occur in a research test.
In a double-blind study , neither the participants nor the researchers know who is receiving the drug or the placebo. When a participant is enrolled, they are randomly assigned to one of the two groups. The medication they receive looks identical whether it’s the drug or the placebo.
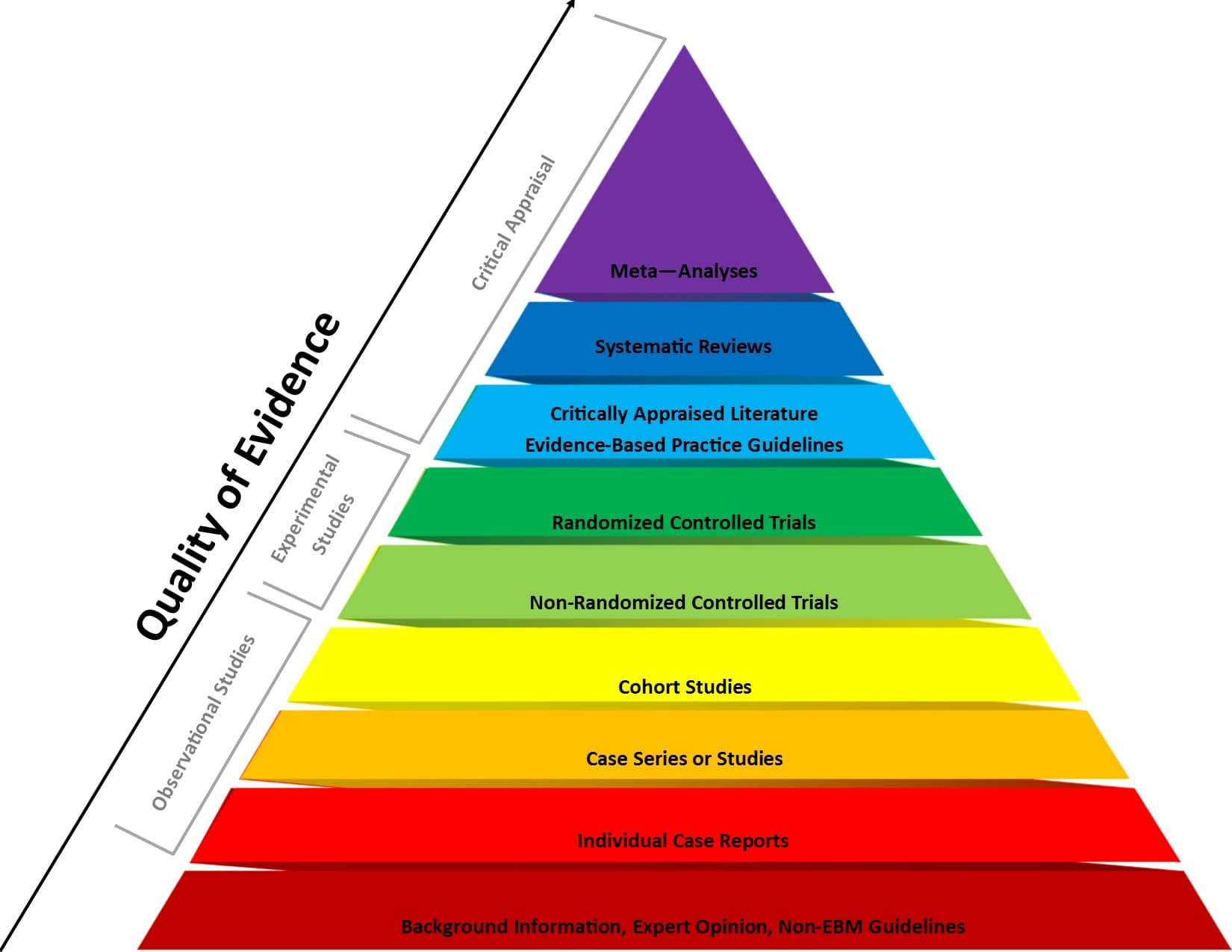
Figure 1 . Evidence-based medicine pyramid. The levels of evidence are appropriately represented by a pyramid as each level, from bottom to top, reflects the quality of research designs (increasing) and quantity (decreasing) of each study design in the body of published literature. For example, randomized control trials are higher quality and more labor intensive to conduct, so there is a lower quantity published.
Resesearch Designs
The choice of design should be guided by the research question, the nature of the treatments or interventions being studied, practical considerations (like sample size and resources), and ethical considerations (such as ensuring all participants have access to potentially beneficial treatments).
The goal is to select a design that provides the most valid and reliable answers to your research questions while minimizing potential biases and confounds.
1. Between-participants randomized designs
Between-participant design involves randomly assigning participants to different treatment conditions. In its simplest form, it has two groups: an experimental group receiving the treatment and a control group.
With more than two levels, multiple treatment conditions are compared. The key feature is that each participant experiences only one condition.
This design allows for clear comparison between groups without worrying about order effects or carryover effects.
It’s particularly useful for treatments that have lasting impacts or when experiencing one condition might influence how participants respond to subsequent conditions.
A study testing a new antidepressant medication might randomly assign 100 participants to either receive the new drug or a placebo.
The researchers would then compare depression scores between the two groups after a specified treatment period to determine if the new medication is more effective than the placebo.
Use this design when:
- You want to compare the effects of different treatments or interventions
- Carryover effects are likely (e.g., learning effects or lasting physiological changes)
- The treatment effect is expected to be permanent
- You have a large enough sample size to ensure groups are equivalent through randomization
2. Factorial designs
Factorial designs investigate the effects of two or more independent variables simultaneously. They allow researchers to study both main effects of each variable and interaction effects between variables.
These can be between-participants (different groups for each combination of conditions), within-participants (all participants experience all conditions), or mixed (combining both approaches).
Factorial designs allow researchers to examine how different factors combine to influence outcomes, providing a more comprehensive understanding of complex phenomena.
They’re more efficient than running separate studies for each variable and can reveal important interactions that might be missed in simpler designs.
A study examining the effects of both exercise intensity (high vs. low) and diet type (high-protein vs. high-carb) on weight loss might use a 2×2 factorial design.
Participants would be randomly assigned to one of four groups: high-intensity exercise with high-protein diet, high-intensity exercise with high-carb diet, low-intensity exercise with high-protein diet, or low-intensity exercise with high-carb diet.
- You want to study the effects of multiple independent variables simultaneously
- You’re interested in potential interactions between variables
- You want to increase the efficiency of your study by testing multiple hypotheses at once
3. Cluster randomized designs
In cluster randomized trials, groups or “clusters” of participants are randomized to treatment conditions, rather than individuals.
This is often used when individual randomization is impractical or when the intervention is naturally applied at a group level.
It’s particularly useful in educational or community-based research where individual randomization might be disruptive or lead to treatment diffusion.
A study testing a new teaching method might randomize entire classrooms to either use the new method or continue with the standard curriculum.
The researchers would then compare student outcomes between the classrooms using the different methods, rather than randomizing individual students.
- You have a smaller sample size available
- Individual differences are likely to be large
- The effects of the treatment are temporary
- You can effectively control for order and carryover effects
4. Within-participants (repeated measures) designs
In these designs, each participant experiences all treatment conditions, serving as their own control.
Within-participants designs are more statistically powerful as they control for individual differences. They require fewer participants, making them more efficient.
However, they’re only appropriate when the treatment effects are temporary and when you can effectively counterbalance to control for order effects.
A study on the effects of caffeine on cognitive performance might have participants complete cognitive tests on three separate occasions: after consuming no caffeine, a low dose of caffeine, and a high dose of caffeine.
The order of these conditions would be counterbalanced across participants to control for order effects.
5. Crossover designs
Crossover designs are a specific type of within-participants design where participants receive different treatments in different time periods.
This allows each participant to serve as their own control and can be more efficient than between-participants designs.
Crossover designs combine the benefits of within-participants designs (increased power, control for individual differences) with the ability to compare different treatments.
They’re particularly useful in clinical trials where you want each participant to experience all treatments, but need to ensure that the effects of one treatment don’t carry over to the next.
A study comparing two different pain medications might have participants use one medication for a month, then switch to the other medication for another month after a washout period.
Pain levels would be measured during both treatment periods, allowing for within-participant comparisons of the two medications’ effectiveness.
- You want to compare the effects of different treatments within the same individuals
- The treatments have temporary effects with a known washout period
- You want to increase statistical power while using a smaller sample size
- You want to control for individual differences in response to treatment
Prevents bias
In randomized control trials, participants must be randomly assigned to either the intervention group or the control group, such that each individual has an equal chance of being placed in either group.
This is meant to prevent selection bias and allocation bias and achieve control over any confounding variables to provide an accurate comparison of the treatment being studied.
Because the distribution of characteristics of patients that could influence the outcome is randomly assigned between groups, any differences in outcome can be explained only by the treatment.
High statistical power
Because the participants are randomized and the characteristics between the two groups are balanced, researchers can assume that if there are significant differences in the primary outcome between the two groups, the differences are likely to be due to the intervention.
This warrants researchers to be confident that randomized control trials will have high statistical power compared to other types of study designs.
Since the focus of conducting a randomized control trial is eliminating bias, blinded RCTs can help minimize any unconscious information bias.
In a blinded RCT, the participants do not know which group they are assigned to or which intervention is received. This blinding procedure should also apply to researchers, health care professionals, assessors, and investigators when possible.
“Single-blind” refers to an RCT where participants do not know the details of the treatment, but the researchers do.
“ Double-blind ” refers to an RCT where both participants and data collectors are masked of the assigned treatment.
Limitations
Costly and timely.
Some interventions require years or even decades to evaluate, rendering them expensive and time-consuming.
It might take an extended period of time before researchers can identify a drug’s effects or discover significant results.
Requires large sample size
There must be enough participants in each group of a randomized control trial so researchers can detect any true differences or effects in outcomes between the groups.
Researchers cannot detect clinically important results if the sample size is too small.
Change in population over time
Because randomized control trials are longitudinal in nature, it is almost inevitable that some participants will not complete the study, whether due to death, migration, non-compliance, or loss of interest in the study.
This tendency is known as selective attrition and can threaten the statistical power of an experiment.
Randomized control trials are not always practical or ethical, and such limitations can prevent researchers from conducting their studies.
For example, a treatment could be too invasive, or administering a placebo instead of an actual drug during a trial for treating a serious illness could deny a participant’s normal course of treatment. Without ethical approval, a randomized control trial cannot proceed.
Fictitious Example
An example of an RCT would be a clinical trial comparing a drug’s effect or a new treatment on a select population.
The researchers would randomly assign participants to either the experimental group or the control group and compare the differences in outcomes between those who receive the drug or treatment and those who do not.
Real-life Examples
- Preventing illicit drug use in adolescents: Long-term follow-up data from a randomized control trial of a school population (Botvin et al., 2000).
- A prospective randomized control trial comparing medical and surgical treatment for early pregnancy failure (Demetroulis et al., 2001).
- A randomized control trial to evaluate a paging system for people with traumatic brain injury (Wilson et al., 2009).
- Prehabilitation versus Rehabilitation: A Randomized Control Trial in Patients Undergoing Colorectal Resection for Cancer (Gillis et al., 2014).
- A Randomized Control Trial of Right-Heart Catheterization in Critically Ill Patients (Guyatt, 1991).
- Berry, R. B., Kryger, M. H., & Massie, C. A. (2011). A novel nasal excitatory positive airway pressure (EPAP) device for the treatment of obstructive sleep apnea: A randomized controlled trial. Sleep , 34, 479–485.
- Gloy, V. L., Briel, M., Bhatt, D. L., Kashyap, S. R., Schauer, P. R., Mingrone, G., . . . Nordmann, A. J. (2013, October 22). Bariatric surgery versus non-surgical treatment for obesity: A systematic review and meta-analysis of randomized controlled trials. BMJ , 347.
- Streeton, C., & Whelan, G. (2001). Naltrexone, a relapse prevention maintenance treatment of alcohol dependence: A meta-analysis of randomized controlled trials. Alcohol and Alcoholism, 36 (6), 544–552.
How Should an RCT be Reported?
Reporting of a Randomized Controlled Trial (RCT) should be done in a clear, transparent, and comprehensive manner to allow readers to understand the design, conduct, analysis, and interpretation of the trial.
The Consolidated Standards of Reporting Trials ( CONSORT ) statement is a widely accepted guideline for reporting RCTs.
Further Information
- Cocks, K., & Torgerson, D. J. (2013). Sample size calculations for pilot randomized trials: a confidence interval approach. Journal of clinical epidemiology, 66(2), 197-201.
- Kendall, J. (2003). Designing a research project: randomised controlled trials and their principles. Emergency medicine journal: EMJ, 20(2), 164.
Akobeng, A.K., Understanding randomized controlled trials. Archives of Disease in Childhood , 2005; 90: 840-844.
Bell, C. C., Gibbons, R., & McKay, M. M. (2008). Building protective factors to offset sexually risky behaviors among black youths: a randomized control trial. Journal of the National Medical Association, 100 (8), 936-944.
Bhide, A., Shah, P. S., & Acharya, G. (2018). A simplified guide to randomized controlled trials. Acta obstetricia et gynecologica Scandinavica, 97 (4), 380-387.
Botvin, G. J., Griffin, K. W., Diaz, T., Scheier, L. M., Williams, C., & Epstein, J. A. (2000). Preventing illicit drug use in adolescents: Long-term follow-up data from a randomized control trial of a school population. Addictive Behaviors, 25 (5), 769-774.
Demetroulis, C., Saridogan, E., Kunde, D., & Naftalin, A. A. (2001). A prospective randomized control trial comparing medical and surgical treatment for early pregnancy failure. Human Reproduction, 16 (2), 365-369.
Gillis, C., Li, C., Lee, L., Awasthi, R., Augustin, B., Gamsa, A., … & Carli, F. (2014). Prehabilitation versus rehabilitation: a randomized control trial in patients undergoing colorectal resection for cancer. Anesthesiology, 121 (5), 937-947.
Globas, C., Becker, C., Cerny, J., Lam, J. M., Lindemann, U., Forrester, L. W., … & Luft, A. R. (2012). Chronic stroke survivors benefit from high-intensity aerobic treadmill exercise: a randomized control trial. Neurorehabilitation and Neural Repair, 26 (1), 85-95.
Guyatt, G. (1991). A randomized control trial of right-heart catheterization in critically ill patients. Journal of Intensive Care Medicine, 6 (2), 91-95.
MediLexicon International. (n.d.). Randomized controlled trials: Overview, benefits, and limitations. Medical News Today. Retrieved from https://www.medicalnewstoday.com/articles/280574#what-is-a-randomized-controlled-trial
Wilson, B. A., Emslie, H., Quirk, K., Evans, J., & Watson, P. (2005). A randomized control trial to evaluate a paging system for people with traumatic brain injury. Brain Injury, 19 (11), 891-894.

Principles of Clinical Trials: Bias and Precision Control
Randomization, Stratification, and Minimization
- Reference work entry
- First Online: 20 July 2022
- Cite this reference work entry
- Fan-fan Yu 3
357 Accesses
The fundamental difference distinguishing observational studies from clinical trials is randomization. This chapter provides a practical guide to concepts of randomization that are widely used in clinical trials. It starts by describing bias and potential confounding arising from allocating people to treatment groups in a predictable way. It then presents the concept of randomization, starting from a simple coin flip, and sequentially introduces methods with additional restrictions to account for better balance of the groups with respect to known (measured) and unknown (unmeasured) variables. These include descriptions and examples of complete randomization and permuted block designs. The text briefly describes biased coin designs that extend this family of designs. Stratification is introduced as a way to provide treatment balance on specific covariates and covariate combinations, and an adaptive counterpart of biased coin designs, minimization, is described. The chapter concludes with some practical considerations when creating and implementing randomization schedules.
By the chapter’s end, statistician or clinicians designing a trial may distinguish generally what assignment methods may fit the needs of their trial and whether or not stratifying by prognostic variables may be appropriate. The statistical properties of the methods are left to the individual references at the end.
This is a preview of subscription content, log in via an institution to check access.
Access this chapter
Subscribe and save.
- Get 10 units per month
- Download Article/Chapter or eBook
- 1 Unit = 1 Article or 1 Chapter
- Cancel anytime
- Available as PDF
- Read on any device
- Instant download
- Own it forever
- Available as EPUB and PDF
- Durable hardcover edition
- Dispatched in 3 to 5 business days
- Free shipping worldwide - see info
Tax calculation will be finalised at checkout
Purchases are for personal use only
Institutional subscriptions
Similar content being viewed by others
The Randomized Controlled Trial: Methodological Perspectives
General Overview of the Statistical Issues in Clinical Study Designs
Buyse M (2000) Centralized treatment allocation in comparative clinical trials. Applied Clinical Trials 9:32–37
Google Scholar
Byar D, Simon R, Friendewald W, Schlesselman J, DeMets D, Ellenberg J, Gail M, Ware J (1976) Randomized clinical trials – perspectives on some recent ideas. N Engl J Med 295:74–80
Article Google Scholar
Hennekens C, Buring J, Manson J, Stampfer M, Rosner B, Cook NR, Belanger C, LaMotte F, Gaziano J, Ridker P, Willett W, Peto R (1996) Lack of effect of long-term supplementation with beta carotene on the incidence of malignant neoplasms and cardiovascular disease. N Engl J Med 334:1145–1149
Ivanova A (2003) A play-the-winner type urn model with reduced variability. Metrika 58:1–13
Article MathSciNet Google Scholar
Kahan B, Morris T (2012) Improper analysis of trials randomized using stratified blocks or minimisation. Stat Med 31:328–340
Lachin J (1988a) Statistical properties of randomization in clinical trials. Control Clin Trials 9:289–311
Lachin J (1988b) Properties of simple randomization in clinical trials. Control Clin Trials 9:312–326
Lachin JM, Matts JP, Wei LJ (1988) Randomization in clinical trials: Conclusions and recommendations. Control Clin Trials 9(4):365–374
Leyland-Jones B, Bondarenko I, Nemsadze G, Smirnov V, Litvin I, Kokhreidze I, Abshilava L, Janjalia M, Li R, Lakshmaiah KC, Samkharadze B, Tarasova O, Mohapatra RK, Sparyk Y, Polenkov S, Vladimirov V, Xiu L, Zhu E, Kimelblatt B, Deprince K, Safonov I, Bowers P, Vercammen E (2016) A randomized, open-label, multicenter, phase III study of epoetin alfa versus best standard of care in anemic patients with metastatic breast cancer receiving standard chemotherapy. J Clin Oncol 34:1197–1207
Matthews J (2000) An introduction to randomized controlled clinical trials. Oxford University Press, Inc., New York
MATH Google Scholar
Matts J, Lachin J (1988) Properties of permuted-block randomization in clinical trials. Control Clin Trials 9:345–364
Pocock S, Simon R (1975) Sequential treatment assignment with balancing for prognostic factors in the controlled clinical trial. Biometrics 31:103–115
Proschan M, Brittain E, Kammerman L (2011) Minimize the use of minimization with unequal allocation. Biometrics 67(3):1135–1141. https://doi.org/10.1111/j.1541-0420.2010.01545.x
Article MathSciNet MATH Google Scholar
Rosenberger W, Uschner D, Wang Y (2018) Randomization: the forgotten component of the randomized clinical trial. Stat Med 38(1):1–12
Russell S, Bennett J, Wellman J, Chung D, Yu Z, Tillman A, Wittes J, Pappas J, Elci O, McCague S, Cross D, Marshall K, Walshire J, Kehoe T, Reichert H, Davis M, Raffini L, Lindsey G, Hudson F, Dingfield L, Zhu X, Haller J, Sohn E, Mahajin V, Pfeifer W, Weckmann M, Johnson C, Gewaily D, Drack A, Stone E, Wachtel K, Simonelli F, Leroy B, Wright J, High K, Maguire A (2017) Efficacy and safety of voretigene neparvovec (AAV2-hRPE65v2) in patients with REP65-mediated inherited retinal dystrophy: a randomised, controlled, open-label, phase 3 trial. Lancet 390:849–860
Scott N, McPherson G, Ramsay C (2002) The method of minimization for allocation to clinical trials: a review. Control Clin Trials 23:662–674
Taves DR (1974) Minimization: a new method of assigning patients to treatment and control groups. Clin Pharmacol Ther 15:443–453
Wei L, Durham S (1978) The randomized play-the-winner rule in medical trials. J Am Stat Assoc 73(364):840–843
Download references
Author information
Authors and affiliations.
Statistics Collaborative, Inc., Washington, DC, USA
You can also search for this author in PubMed Google Scholar
Corresponding author
Correspondence to Fan-fan Yu .
Editor information
Editors and affiliations.
Department of Surgery, Division of Surgical Oncology, Brigham and Women’s Hospital, Harvard Medical School, Boston, MA, USA
Steven Piantadosi
Department of Epidemiology, School of Public Health, Johns Hopkins University, Baltimore, MD, USA
Curtis L. Meinert
Section Editor information
Department of Medicine, University of Alabama, Birmingham, AL, USA
O. Dale Williams
Rights and permissions
Reprints and permissions
Copyright information
© 2022 Springer Nature Switzerland AG
About this entry
Cite this entry.
Yu, Ff. (2022). Principles of Clinical Trials: Bias and Precision Control. In: Piantadosi, S., Meinert, C.L. (eds) Principles and Practice of Clinical Trials. Springer, Cham. https://doi.org/10.1007/978-3-319-52636-2_211
Download citation
DOI : https://doi.org/10.1007/978-3-319-52636-2_211
Published : 20 July 2022
Publisher Name : Springer, Cham
Print ISBN : 978-3-319-52635-5
Online ISBN : 978-3-319-52636-2
eBook Packages : Mathematics and Statistics Reference Module Computer Science and Engineering
Share this entry
Anyone you share the following link with will be able to read this content:
Sorry, a shareable link is not currently available for this article.
Provided by the Springer Nature SharedIt content-sharing initiative
- Publish with us
Policies and ethics
- Find a journal
- Track your research
Purpose and Limitations of Random Assignment
In an experimental study, random assignment is a process by which participants are assigned, with the same chance, to either a treatment or a control group. The goal is to assure an unbiased assignment of participants to treatment options.
Random assignment is considered the gold standard for achieving comparability across study groups, and therefore is the best method for inferring a causal relationship between a treatment (or intervention or risk factor) and an outcome.
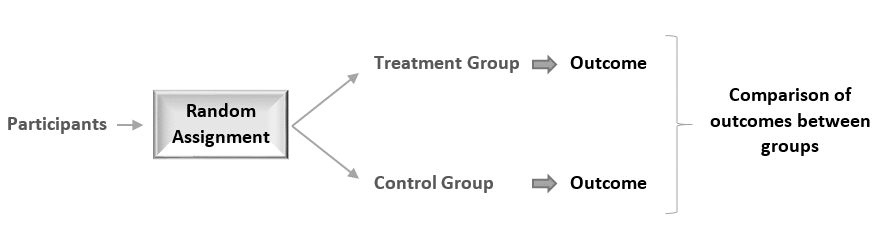
Random assignment of participants produces comparable groups regarding the participants’ initial characteristics, thereby any difference detected in the end between the treatment and the control group will be due to the effect of the treatment alone.
How does random assignment produce comparable groups?
1. random assignment prevents selection bias.
Randomization works by removing the researcher’s and the participant’s influence on the treatment allocation. So the allocation can no longer be biased since it is done at random, i.e. in a non-predictable way.
This is in contrast with the real world, where for example, the sickest people are more likely to receive the treatment.
2. Random assignment prevents confounding
A confounding variable is one that is associated with both the intervention and the outcome, and thus can affect the outcome in 2 ways:
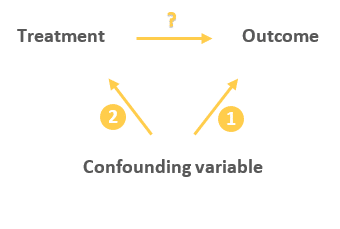
Either directly:
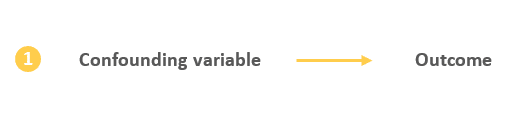
Or indirectly through the treatment:

This indirect relationship between the confounding variable and the outcome can cause the treatment to appear to have an influence on the outcome while in reality the treatment is just a mediator of that effect (as it happens to be on the causal pathway between the confounder and the outcome).
Random assignment eliminates the influence of the confounding variables on the treatment since it distributes them at random between the study groups, therefore, ruling out this alternative path or explanation of the outcome.
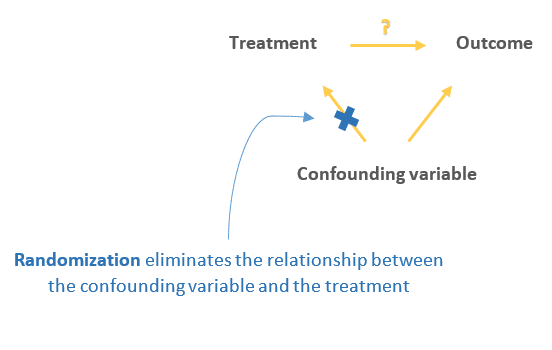
3. Random assignment also eliminates other threats to internal validity
By distributing all threats (known and unknown) at random between study groups, participants in both the treatment and the control group become equally subject to the effect of any threat to validity. Therefore, comparing the outcome between the 2 groups will bypass the effect of these threats and will only reflect the effect of the treatment on the outcome.
These threats include:
- History: This is any event that co-occurs with the treatment and can affect the outcome.
- Maturation: This is the effect of time on the study participants (e.g. participants becoming wiser, hungrier, or more stressed with time) which might influence the outcome.
- Regression to the mean: This happens when the participants’ outcome score is exceptionally good on a pre-treatment measurement, so the post-treatment measurement scores will naturally regress toward the mean — in simple terms, regression happens since an exceptional performance is hard to maintain. This effect can bias the study since it represents an alternative explanation of the outcome.
Note that randomization does not prevent these effects from happening, it just allows us to control them by reducing their risk of being associated with the treatment.
What if random assignment produced unequal groups?
Question: What should you do if after randomly assigning participants, it turned out that the 2 groups still differ in participants’ characteristics? More precisely, what if randomization accidentally did not balance risk factors that can be alternative explanations between the 2 groups? (For example, if one group includes more male participants, or sicker, or older people than the other group).
Short answer: This is perfectly normal, since randomization only assures an unbiased assignment of participants to groups, i.e. it produces comparable groups, but it does not guarantee the equality of these groups.
A more complete answer: Randomization will not and cannot create 2 equal groups regarding each and every characteristic. This is because when dealing with randomization there is still an element of luck. If you want 2 perfectly equal groups, you better match them manually as is done in a matched pairs design (for more information see my article on matched pairs design ).
This is similar to throwing a die: If you throw it 10 times, the chance of getting a specific outcome will not be 1/6. But it will approach 1/6 if you repeat the experiment a very large number of times and calculate the average number of times the specific outcome turned up.
So randomization will not produce perfectly equal groups for each specific study, especially if the study has a small sample size. But do not forget that scientific evidence is a long and continuous process, and the groups will tend to be equal in the long run when a meta-analysis aggregates the results of a large number of randomized studies.
So for each individual study, differences between the treatment and control group will exist and will influence the study results. This means that the results of a randomized trial will sometimes be wrong, and this is absolutely okay.
BOTTOM LINE:
Although the results of a particular randomized study are unbiased, they will still be affected by a sampling error due to chance. But the real benefit of random assignment will be when data is aggregated in a meta-analysis.
Limitations of random assignment
Randomized designs can suffer from:
1. Ethical issues:
Randomization is ethical only if the researcher has no evidence that one treatment is superior to the other.
Also, it would be unethical to randomly assign participants to harmful exposures such as smoking or dangerous chemicals.
2. Low external validity:
With random assignment, external validity (i.e. the generalizability of the study results) is compromised because the results of a study that uses random assignment represent what would happen under “ideal” experimental conditions, which is in general very different from what happens at the population level.
In the real world, people who take the treatment might be very different from those who don’t – so the assignment of participants is not a random event, but rather under the influence of all sort of external factors.
External validity can be also jeopardized in cases where not all participants are eligible or willing to accept the terms of the study.
3. Higher cost of implementation:
An experimental design with random assignment is typically more expensive than observational studies where the investigator’s role is just to observe events without intervening.
Experimental designs also typically take a lot of time to implement, and therefore are less practical when a quick answer is needed.
4. Impracticality when answering non-causal questions:
A randomized trial is our best bet when the question is to find the causal effect of a treatment or a risk factor.
Sometimes however, the researcher is just interested in predicting the probability of an event or a disease given some risk factors. In this case, the causal relationship between these variables is not important, making observational designs more suitable for such problems.
5. Impracticality when studying the effect of variables that cannot be manipulated:
The usual objective of studying the effects of risk factors is to propose recommendations that involve changing the level of exposure to these factors.
However, some risk factors cannot be manipulated, and so it does not make any sense to study them in a randomized trial. For example it would be impossible to randomly assign participants to age categories, gender, or genetic factors.
6. Difficulty to control participants:
These difficulties include:
- Participants refusing to receive the assigned treatment.
- Participants not adhering to recommendations.
- Differential loss to follow-up between those who receive the treatment and those who don’t.
All of these issues might occur in a randomized trial, but might not affect an observational study.
- Shadish WR, Cook TD, Campbell DT. Experimental and Quasi-Experimental Designs for Generalized Causal Inference . 2nd edition. Cengage Learning; 2001.
- Friedman LM, Furberg CD, DeMets DL, Reboussin DM, Granger CB. Fundamentals of Clinical Trials . 5th ed. 2015 edition. Springer; 2015.
Further reading
- Posttest-Only Control Group Design
- Pretest-Posttest Control Group Design
- Randomized Block Design
Your session is about to expire
Clinical trial basics: randomization in clinical trials, introduction.
Clinical trials represent a core pillar of advancing patient care and medical knowledge. Clinical trials are designed to thoroughly assess the effectiveness and safety of new drugs and treatments in the human population. There are 4 main phases of clinical trials, each with its own objectives and questions, and they can be designed in different ways depending on the study population, the treatment being tested, and the specific research hypotheses. The “gold standard” of clinical research are randomized controlled trials (RCTs), which aim to avoid bias by randomly assigning patients into different groups, which can then be compared to evaluate the new drug or treatment. The process of random assignment of patients to groups is called randomization.
Randomization in clinical trials is an essential concept for minimizing bias, ensuring fairness, and maximizing the statistical power of the study results. In this article, we will discuss the concept of randomization in clinical trials, why it is important, and go over some of the different randomization methods that are commonly used.
What does randomization mean in clinical trials?
Randomization in clinical trials involves assigning patients into two or more study groups according to a chosen randomization protocol (randomization method). Randomizing patients allows for directly comparing the outcomes between the different groups, thereby providing stronger evidence for any effects seen being a result of the treatment rather than due to chance or random variables.
What is the main purpose of randomization?
Randomization is considered a key element in clinical trials for ensuring unbiased treatment of patients and obtaining reliable, scientifically valuable results. [1] Randomization is important for generating comparable intervention groups and for ensuring that all patients have an equal chance of receiving the novel treatment under study. The systematic rule for the randomization process (known as “sequence generation”) reduces selection bias that could arise if researchers were to manually assign patients with better prognoses to specific study groups; steps must be taken to further ensure strict implementation of the sequence by preventing researchers and patients from knowing beforehand which group patients are destined for (known as “allocation sequence concealment”). [2]
Randomization also aims to remove the influence of external and prognostic variables to increase the statistical power of the results. Some researchers are opposed to randomization, instead supporting the use of statistical techniques such as analysis of covariance (ANCOVA) and multivariate ANCOVA to adjust for covariate imbalance after the study is completed, in the analysis stage. However, this post-adjustment approach might not be an ideal fit for every clinical trial because the researcher might be unaware of certain prognostic variables that could lead to unforeseen interaction effects and contaminate the data. Thus, the best way to avoid bias and the influence of external variables and thereby ensure the validity of statistical test results is to apply randomization in the clinical trial design stage.
Randomized controlled trials (RCTs): The ‘gold standard’
Randomized controlled trials, or RCTs, are considered the “gold standard” of clinical research because, by design, they feature minimized bias, high statistical power, and a strong ability to provide evidence that any clinical benefit observed results specifically from the study intervention (i.e., identifying cause-effect relationships between the intervention and the outcome).[3] A randomized controlled trial is one of the most effective studies for measuring the effectiveness of a new drug or intervention.
How are participants randomized? An introduction to randomization methods
Randomization includes a broad class of design techniques for clinical trials, and is not a single methodology. For randomization to be effective and reduce (rather than introduce) bias, a randomization schedule is required for assigning patients in an unbiased and systematic manner. Below is a brief overview of the main randomization techniques commonly used; further detail is given in the next sections.
Fixed vs. adaptive randomization
Randomization methods can be divided into fixed and adaptive randomization. Fixed randomization involves allocating patients to interventions using a fixed sequence that doesn’t change throughout the study. On the other hand, adaptive randomization involves assigning patients to groups in consideration of the characteristics of the patients already in the trial, and the randomization probabilities can change over the course of the study. Each of these techniques can be further subdivided:
Fixed allocation randomization methods:
- Simple randomization : the simplest method of randomization, in which patient allocation is based on a single sequence of random assignments.
- Block randomization : patients are first assigned to blocks of equal size, and then randomized within each block. This ensures balance in group sizes.
Stratified randomization : patients are first allocated to blocks (strata) designed to balance combinations of specific covariates (subject’s baseline characteristics), and then randomization is performed within each stratum.
Adaptive randomization methods:
- Outcome-adaptive/results-adaptive randomization : involves allocating patients to study groups in consideration of other patients’ responses to the ongoing trial treatment. Minimization: involves minimizing imbalance amongst covariates by allocating new enrollments as a function of prior allocations
Below is a graphic summary of the breakdown we’ve just covered.
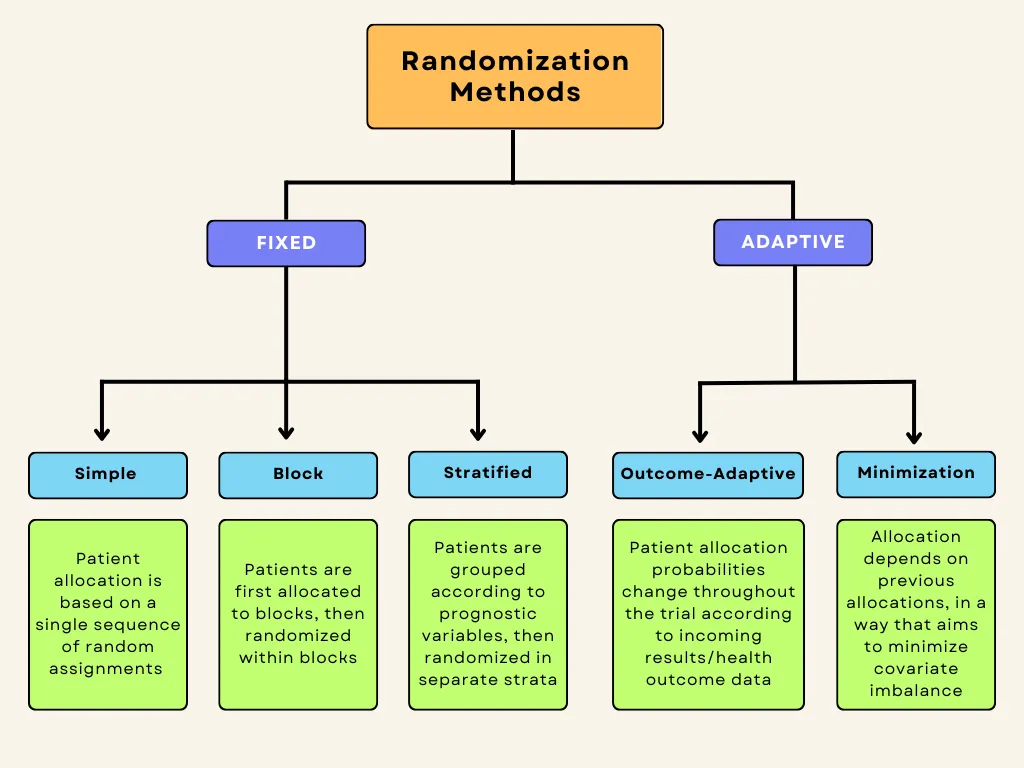
Fixed-allocation randomization in clinical trials
Here, we will discuss the three main fixed-allocation randomization types in more detail.
Simple Randomization
Simple randomization is the most commonly used method of fixed randomization, offering completely random patient allocation into the different study groups. It is based on a single sequence of random assignments and is not influenced by previous assignments. The benefits are that it is simple and it fulfills the allocation concealment requirement, ensuring that researchers, sponsors, and patients are unaware of which patient will be assigned to which treatment group. Simple randomization can be conceptualized, or even performed, by the following chance actions:
- Flipping a coin (e.g., heads → control / tails → intervention)
- Throwing a dice (e.g., 1-3 → control / 4-6 → intervention)
- Using a deck of shuffled cards (e.g., red → control / black → intervention)
- Using a computer-generated random number sequence
- Using a random number table from a statistics textbook
There are certain disadvantages associated with simple randomization, namely that it does not take into consideration the influence of covariates, and it may lead to unequal sample sizes between groups. For clinical research studies with small sample sizes, the group sizes are more likely to be unequal.
Especially in smaller clinical trials, simple randomization can lead to covariate imbalance. It has been suggested that clinical trials enrolling at least 1,000 participants can essentially avoid random differences between treatment groups and minimize bias by using simple randomization. [4] On the other hand, the risks posed by imbalances in covariates and prognostic factors are more relevant in smaller clinical trials employing simple randomization, and thus, other methods such as blocking should be considered for such trials.
Block Randomization
Block randomization is a type of “constrained randomization” that is preferred for achieving balance in the sizes of treatment groups in smaller clinical trials. The first step is to select a block size. Blocks represent “subgroups” of participants who will be randomized in these subgroups, or blocks. Block size should be a multiple of the number of groups; for instance, if there are two groups, the block size can be 4, 6, 8, etc. Once block size is determined, then all possible different combinations (permutations) of assignment within the block are identified. Each block is then randomly assigned one of these permutations, and individuals in the block are allocated according to the specific pattern of the permuted block.[5]
Let’s consider a small clinical trial with two study groups (control and treatment) and 20 participants. In this situation, an allocation sequence based on blocked randomization would involve the following steps:
1. The researcher chooses block size: In this case, we will use a block size of 4 (which is a multiple of the number of study groups, 2).
2. All 6 possible balanced combinations of control (C) and treatment (T) allocations within each block are shown as follows:
3. These allocation sequences are randomly assigned to the blocks, which then determine the assignment of the 4 participants within each block. Let’s say the sequence TCCT is selected for block 1. The allocation would then be as follows:
- Participant 1 → Treatment (T)
- Participant 2 → Control (C)
- Participant 3 → Control (C)
- Participant 4 → Treatment (T)
We can see that blocked randomization ensures equal (or nearly equal, if for example enrollment is terminated early or the final target is not quite met) assignment to treatment groups.
There are disadvantages to blocked randomization. For one, if the investigators/researchers are not blinded (masked), then the condition of allocation concealment is not met, which could lead to selection bias. To illustrate this, let’s say that two of four participants have enrolled in block 2 of the above example, for which the randomly selected sequence is CCTT. In this case, the investigator would know that the next two participants for the current block would be assigned to the treatment group (T), which could influence his/her selection process. Keeping the investigator masked (blinded) or utilizing random block sizes are potential solutions for preventing this issue. Another drawback is that the determined blocks may still contain covariate imbalances. For instance, one block might have more participants with chronic or secondary illnesses.
Despite these drawbacks, block randomization is simple to implement and better than simple randomization for smaller clinical trials in that treatment groups will have an equal number of participants. Blinding researchers to block size or randomizing block size can reduce potential selection bias. [5]
Stratified Randomization
Stratified randomization aims to prevent imbalances amongst prognostic variables (or the patients’ baseline characteristics, also known as covariates) in the study groups. Stratified randomization is another type of constrained randomization, where participants are first grouped (“stratified”) into strata based on predetermined covariates, which could include such things as age, sex, comorbidities, etc. Block randomization is then applied within each of these strata separately, ensuring balance amongst prognostic variables as well as in group size.
The covariates of interest are determined by the researchers before enrollment begins, and are chosen based on the potential influence of each covariate on the dependent variable. Each covariate will have a given number of levels, and the product of the number of levels of all covariates determines the number of strata for the trial. For example, if two covariates are identified for a trial, each with two levels (let’s say age, divided into two levels [<50 and 50+], and height [<175 cm and 176+ cm]), a total of 4 strata will be created (2 x 2 = 4).
Patients are first assigned to the appropriate stratum according to these prognostic classifications, and then a randomization sequence is applied within each stratum. Block randomization is usually applied in order to guarantee balance between treatment groups in each stratum.
Stratified randomization can thus prevent covariate imbalance, which can be especially important in smaller clinical trials with few participants. [6] Nonetheless, stratification and imbalance control can become complex if too many covariates are considered, because an overly large number of strata can lead to imbalances in patient allocation due to small sample sizes within individual strata,. Thus, the number of strata should be kept to a minimum for the best results – in other words, only covariates with potentially important influences on study outcomes and results should be included. [6] Stratified randomization also reduces type I error, which describes “false positive” results, wherein differences in treatment outcomes are observed between groups despite the treatments being equal (for example, if the intervention group contained participants with overall better prognosis, it could be concluded that the intervention was effective, although in reality the effect was only due to their better initial prognosis and not the treatment). [5] Type II errors are also reduced, which describe “false negatives,” wherein actual differences in outcomes between groups are not noticed. The “power” of a trial to identify treatment effects is inversely related to these errors, which are related to variance between groups being compared; stratification reduces variance between groups and thus theoretically increases power. The required sample size decreases as power increases, which can also be used to explain why stratification is relatively more impactful with smaller sample sizes.
A major drawback of stratified randomization is that it requires identification of all participants before they can be allocated. Its utility is also disputed by some researchers, especially in the context of trials with large sample sizes, wherein covariates are more likely to be balanced naturally even when using simpler randomization techniques. [6]
Adaptive randomization in clinical trials
Adaptive randomization describes schemes in which treatment allocation probabilities are adjusted as the trial progresses.In adaptive randomization,allocation probabilities can be altered in order to either minimize imbalances in prognostic variables (covariate-adaptive randomization, or “minimization”), or to increase the allocation of patients to the treatment arm(s) showing better patient outcomes (“response-adaptive randomization”). [7] Adaptive randomization methods can thus address the issue of covariate imbalance, or can be employed to offer a unique ethical advantage in studies wherein preliminary or interim analyses indicate that one treatment arm is significantly more effective, maximizing potential therapeutic benefit for patients by increasing allocation to the most-effective treatment arm.
One of the main disadvantages associated with adaptive randomization methods is that they are time-consuming and complex; recalculation is necessary for each new patient or when any treatment arm is terminated.
Outcome-adaptive (response-adaptive) randomization
Outcome-adaptive randomization was first proposed in 1969 as “play-the-winner” treatment assignments. [8] This method involves adjusting the allocation probabilities based on the data and results being collected in the ongoing trial. The aim is to increase the ratio of patients being assigned to the more effective treatment arm, representing a significant ethical advantage especially for trials in which one or more treatments are clearly demonstrating promising therapeutic benefit. The maximization of therapeutic benefit for participants comes at the expense of statistical power, which is one of the major drawbacks of this randomization method.
Outcome-adaptive randomization can decrease power because, by increasing the allocation of participants to the more-effective treatment arm, which then in turn demonstrates better outcomes, an increasing bias toward that treatment arm is created. Thus, outcome-adaptive randomization is unsuitable for long-term phase III clinical trials requiring high statistical power, and some argue that the high design complexity is not warranted as the benefits offered are minimal (or can be achieved through other designs). [8]
Covariate-adaptive randomization (Minimization)
Minimization is a complex form of adaptive randomization which, similarly to stratified randomization, aims to maximize the balance amongst covariates between treatment groups. Rather than achieving this by initially stratifying participants into separate strata based on covariates and then randomizing, the first participants are allocated randomly and then each new allocation involves hypothetically allocating the new participant to all groups and calculating a resultant “imbalance score.” The participant will then be assigned in such a way that this covariate imbalance is minimized (hence the name minimization). [9]
A principal drawback of minimization is that it is labor-intensive due to frequent recalculation of imbalance scores as new patients enroll in the trial. However, there are web-based tools and computer programs that can be used to facilitate the recalculation and allocation processes. [10]
Randomization in clinical trials is important as it ensures fair allocation of patients to study groups and enables researchers to make accurate and valid comparisons. The choice of the specific randomization schedule will depend on the trial type/phase, sample size, research objectives, and the condition being treated. A balance should be sought between ease of implementation, prevention of bias, and maximization of power. To further minimize bias, considerations such as blinding and allocation concealment should be combined with randomization techniques.
Other Trials to Consider
Renal Autologous Cell Therapy (REACT)
Dose escalation, order: period c, period a, period b, varian ethos adaptive radiation therapy, immersive soundscapes environment, 900 +/- 15nm/ 770 +/- 9nm/ 830 +/- 15nm, popular categories.
Tymlos Clinical Trials
Paid Clinical Trials in Cincinnati, OH
Paid Clinical Trials in Omaha, NE
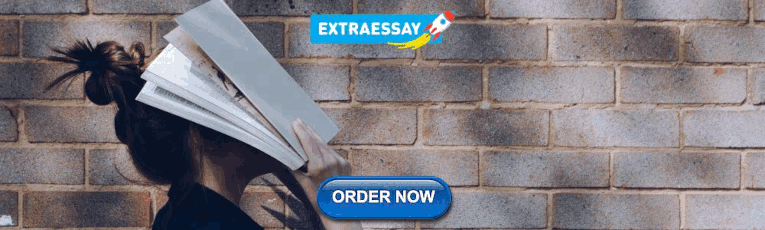
Paid Clinical Trials in Meridian, ID
Paid Clinical Trials in New York
Paid Clinical Trials in Tennessee
Paid Clinical Trials in New Mexico
Paid Clinical Trials in Alaska
Paid Clinical Trials in Wyoming
Forteo Clinical Trials
Popular guides.
Thank you for visiting nature.com. You are using a browser version with limited support for CSS. To obtain the best experience, we recommend you use a more up to date browser (or turn off compatibility mode in Internet Explorer). In the meantime, to ensure continued support, we are displaying the site without styles and JavaScript.
- View all journals
- Explore content
- About the journal
- Publish with us
- Sign up for alerts
- Open access
- Published: 15 August 2024
What differentiates clinical trial statistics from preclinical methods and why robust approaches matter
Nature Communications volume 15 , Article number: 7035 ( 2024 ) Cite this article
Metrics details
Clinical trial statistics underlie the central decision-making process for whether a therapeutic approach can enter the clinic, but the nuances of this field may not be widely understood. Furthermore, how the statistics used in clinical trials differ from preclinical approaches and why they differ is not always clear. Here, three experts discuss the intricacies of clinical trial statistical planning and analysis as well as common issues that arise and emerging trends. The experts are Dr Tao Chen (Senior lecturer in Biostatistics at the Liverpool School of Tropical medicine), Professor Li Chao (Professor in Biostatistics at Xi’an Jiaotong University) and Professor Yang Wang (Professor in Biostatistics at the Chinese Academy of Medical Sciences and Peking Union Medical College). They have a diverse range of backgrounds across biostatistics and have been involved in numerous clinical trials of varying types.
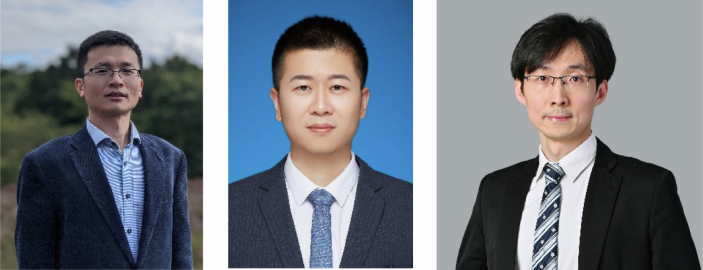
1. Statistics for clinical trials is a speciality in and of itself: could you please start by highlighting what differentiates clinical trial statistical approaches and practises from those applied in other areas of life sciences?
While statistics is a fundamental tool across all areas of life sciences, its application in clinical trials is characterized by the specific challenges and requirements associated with testing medical interventions in human populations. Key considerations that distinguish statistics applied to clinical trials include the incorporation of stringent ethical considerations to establish the balance between benefit and risk from the investigational treatment, the need for meticulous regulatory compliance (e.g., The International Council for Harmonisation of Technical Requirements for Pharmaceuticals for Human Use (ICH)), the systematic use of randomization and blinding to eliminate selection bias and confounding effects, the focus on clinically relevant outcomes directly related to the primary objective of the trial, a predetermined sample size calculation to ensure a study is sufficiently powered to assess the desired outcomes (an underpowered study, with limited sample size, may lead to false negative results), and prespecified statistical analysis plans to avoid selective reporting. Collectively, these elements aim to provide robust evidence regarding the safety and efficacy of medical interventions. Meanwhile, they also shape the statistical approaches in clinical trials, setting them apart from methodologies applied in other areas of life sciences research.
2. Clinical trials are separated into different phases and types, how do the statistics differ between phases or types?
Clinical trials are typically organized into different phases (e.g., Phase I to Phase IV) and types (e.g., exploratory trials looking at new effects and confirmatory trials to support previous results), which form a structured framework for evaluating the safety, efficacy, and effectiveness of medical treatments and guide the progression of treatments from initial testing to regulatory approval and post-market surveillance. As such, the emphasis on statistical aspects including sample size, study design, and choice of statistical methods varies to address the unique objectives and challenges of each phase of clinical trials.
For example, a dose escalation design with a small sample size of healthy volunteers is often employed to descriptively characterise the preliminary safety profile in phase I trials before advancing to subsequent phases of clinical trials. Whereas larger-scale trials with complex designs, may be used to confirm efficacy and safety assessments involving rigorous hypothesis testing - such as the establishment of clear and predefined hypotheses (e.g., testing for superiority or non-inferiority), selection of appropriate statistical test and control of type I error rate—These ultimately ensure the reliability and validity of study findings, leading to more informed decisions in clinical practice and healthcare policy.
3. Statistics play an important role in both the analysis and design of clinical trials, could you please define these different roles?
Statistics is integral to both the analysis and design of clinical trials. In the design phase, statistics is employed to plan and structure the trial to prevent bias to ensure validity, efficiency, and ethical conduct and optimize the chances of obtaining meaningful results. Various types of biases could be incurred if deviations from the protocol occurs. These include attrition bias due to differential loss of participants from different groups (e.g., a higher dropout rate in the control group) leading to biased estimates of treatment effects, ascertainment bias when knowing the treatment allocation prematurely, which inadvertently influence the assessment of outcomes (e.g., giving more attention or ancillary treatments/test for the subjects from intervention group), reporting bias introduced from outcome switching or failure to adjust for the multiplicity which can occur when multiple outcomes are being assessed, and selection bias when there is systematic differences in characteristics between groups being compared in a study(e.g., poor randomisation) as in these situations the probability of obtaining false positive associations increases.
In the analysis phase, the primary goal is to draw valid and reliable conclusions from the collected data, which involves assessing the efficacy and safety of the investigational treatment and making inferences from the trial population to the broader target population but with cautiousness. It remains imperative to consistently consider the prespecified analysis plan throughout the trial design process and ensure alignment with the overall trial design to maintain scientific integrity, prevent data fishing, facilitate interpretation and reproducibility, as well as comply with regulations and uphold ethical standards.
4. Could you please highlight some of the key issues with statistics in the design of trials that authors, reviewers, and editors should be aware of when assessing manuscripts?
Several critical issues related to statistics in the design of trials should be considered during the manuscript assessment process by authors, reviewers, and editors. These issues may include failure to prospectively register the trial, the absence of the pre-specified statistical analysis plan, ethical lapses, poor randomization, unclear allocation concealment (e.g., Interactive web response system to reduce the chance of guessing the randomisation sequence correctly), unjustified sample sizes calculation, inappropriate selection of endpoints that are not clinically relevant and validated, and imbalanced baseline characteristics or confounding factors between groups. Failure to comply with best practice in clinical trials would result in biased, unreliable, or misleading findings, compromising the validity, integrity, and ethical conduct of the research. For example, absence of a predefined analysis plan can lead to publication bias, selective reporting of outcomes, and incomplete dissemination of study results, hindering transparency and misleading conclusions.
5. Specifically, how does trial design influence whether data obtained from a clinical trial is meaningful?
The design exerts influence over various aspects of clinical trials. For instance, meaningful data relies on the careful selection of clinically relevant endpoints that directly measure the outcomes of interest. The use of inappropriate endpoints may lead to results that are inconclusive or even misleading. Similarly, the relevance of the data depends on how well the study population mirrors the target patient population. Excessive restrictions or inappropriate inclusion criteria have the potential to limit the generalizability of the results. Likewise, inadequate sample sizes can result in underpowered studies, diminishing the ability to detect true treatment effects and possibly yielding inconclusive or misleading results. Therefore, a well-thought-out design, aligned with study objectives, ethical standards, and robust statistical methodologies, enhances the validity and relevance of the trial outcomes.
6. It is well known that there are abundant issues with statistical analyses of preclinical studies, could you comment on how these preclinical issues carry over and affect clinical trials?
Issues stemming from preclinical study can significantly influence the planning, execution, and interpretation of subsequent clinical trials. These challenges are multifaceted, including the translatability of preclinical findings, biological variability between animal models and humans, lack of methodological rigors (e.g., randomisation and blinding), potential false positives from multiplicity, hypothesis driven research with small sample size and ethical concerns regarding the applicability of interventions to human subjects. The exploratory nature and challenges inherent in preclinical research findings increase the complexities and uncertainties while designing and analysing in further clinical trials. Therefore, by promoting transparency, judiciously leveraging information from preclinical studies and integrating this prior information into subsequent translational research through rigorous methodologies like Bayesian approaches, and fostering collaborations among researchers to which better align preclinical studies with real-world needs and maximize the translational potential of their findings, we can improve the reliability and translatability of findings throughout the translational process.
7. Could you please highlight some of the key issues with statistics in the analysis of trials that authors, reviewers, and editors should be aware of when assessing manuscripts?
Authors, reviewers, and editors must maintain vigilance to safeguard the credibility, transparency, and reliability of statistical analyses in clinical trial manuscripts. Key concerns over the statistical analysis particularly include the inconsistency between the statistical analysis plan and the reported analyses, departure from the original randomised assignment in the final analysis of a clinical trial, failure to adjust for multiple testing, inadequate handling of missing data, overemphasis on P-values, inappropriate subgroup analyses, outcome switching based on observed result, and change to the hypothesis (e.g., from non-inferiority to superiority) without pre-specification. These issues would introduce the risk of reporting or publication bias and erroneous conclusions. For example, overemphasis on p-values to draw conclusions without considering effect sizes, clinical significance, or context can lead to misinterpretation and undermine the reliability of study results.
Addressing these concerns by collaborating with statisticians at the early stage of the trial and maintaining adherence to methodological standards are essential for establishing robustness and integrity of clinical trial research.
8. Further to their implementation, clear and open reporting is vital for clinical trials: could you please comment on what are some of the major things that should be discussed and reported?
Comprehensive reporting allows researchers, clinicians, and the public to understand the study’s methods and results. Also, clear and open reporting ensures that the scientific community can critically evaluate the study, reproduce the research, and consider its findings in the broader context of existing evidence and the limitations of the trial design. The SPIRIT (Standard Protocol Items: Recommendations for Interventional Trials) guideline serves as a valuable resource for researchers and research teams to ensure that clinical trial protocols are comprehensive, transparent, and adhere to best practices with a set of recommendations, such as prospective trial registration, safety monitoring and study design. Correspondingly, researchers are encouraged to refer to the CONSORT (Consolidated Standards of Reporting Trials) and extensions (for specific types of trials, such as non-pharmacological interventions) for the complete checklist and guidance on reporting randomized controlled trials. Some key elements such as the strategies to control Type I error, maintenance of blindness, randomisation procedures, and justification for the non-inferiority margin-a predefined threshold used in non-inferiority clinical trials to determine whether a new treatment is not unacceptably worse than an active comparator treatment, should always be described in detail to strengthen the completeness and transparency of clinical trial reports.
9. In addition to their use in primary reporting of trials, statistical analysis of post-hoc trials is also important; could you please comment on the value of these analyses and describe how these approaches differ from the original analyses?
While post-hoc analyses can provide valuable insights and generate hypotheses for further exploration, it’s crucial to acknowledge their distinct characteristics and implications compared to the original analyses, necessitating a cautious approach. Generally, post-hoc analyses involve exploring data without predefined hypotheses, rendering them exploratory in nature and may be considered as supplementary or as validation for the original analysis. Additionally, statistical methods for post-hoc analysis may be less stringent, requiring adjustments for multiple comparisons to mitigate the increased risk of false positives. Overall, there is a risk of data-driven analyses, post hoc changes, or selective reporting of statistically significant results, leading to biased or misleading conclusions for post hoc analysis of trial data without a predefined analysis plan. Therefore, transparency regarding the exploratory nature of these analyses, stating them as such in the manuscripts, timing for conducting the analyses, appropriate statistical adjustments, and validation in independent studies are essential to ensure the reliability and robustness of the findings. Researchers should distinctly delineate between pre-specified analyses and post-hoc exploratory analyses when reporting trial results.
10. Finally, as with all fields of academia, statistics is constantly evolving and changing, could you please mention some of the changes that are happening currently which may influence clinical trial statistical design/analysis in the future?
Statistics, encompassing its application in clinical trial design and analysis, is continually evolving with ongoing technological advancements (e.g., artificial intelligence, digital health and telemedicine) and emerging changes (e.g., precision medicine) that are transforming the future of medicine and improving the quality of life for individuals. Several trends and developments are currently shaping the landscape of statistical approaches in clinical trials. Bayesian methodologies offer flexibility by incorporating prior information, updating analyses as data accumulate, and providing more informative posterior distributions after combining information from the observed data and any prior beliefs. Real-world evidence is gaining importance as it complements traditional clinical trial data, offering insights into long-term outcomes, patient perspectives, and treatment effectiveness in real-world settings. The integration of machine learning and artificial intelligence technologies holds promise for identifying intricate data patterns, optimizing patient recruitment strategies, and enhancing predictive modelling for patient outcomes. Precision medicine approaches, guided by genetic information, are advancing, leading to more targeted and personalized treatments. Clinical trial simulations are gaining recognition as an important component of clinical development programs, offering valuable insights to enhance understanding and inform decision-making at various stages of drug development. These trends collectively underscore the dynamic nature of statistical methodologies in clinical trials, driven by technological advancements, shifts in regulatory landscapes, and a commitment to improving the efficiency and ethical conduct of clinical trials.
Rights and permissions
Open Access This article is licensed under a Creative Commons Attribution-NonCommercial-NoDerivatives 4.0 International License, which permits any non-commercial use, sharing, distribution and reproduction in any medium or format, as long as you give appropriate credit to the original author(s) and the source, provide a link to the Creative Commons licence, and indicate if you modified the licensed material. You do not have permission under this licence to share adapted material derived from this article or parts of it. The images or other third party material in this article are included in the article’s Creative Commons licence, unless indicated otherwise in a credit line to the material. If material is not included in the article’s Creative Commons licence and your intended use is not permitted by statutory regulation or exceeds the permitted use, you will need to obtain permission directly from the copyright holder. To view a copy of this licence, visit http://creativecommons.org/licenses/by-nc-nd/4.0/ .
Reprints and permissions
About this article
Cite this article.
What differentiates clinical trial statistics from preclinical methods and why robust approaches matter. Nat Commun 15 , 7035 (2024). https://doi.org/10.1038/s41467-024-51486-4
Download citation
Published : 15 August 2024
DOI : https://doi.org/10.1038/s41467-024-51486-4
Share this article
Anyone you share the following link with will be able to read this content:
Sorry, a shareable link is not currently available for this article.
Provided by the Springer Nature SharedIt content-sharing initiative
Quick links
- Explore articles by subject
- Guide to authors
- Editorial policies
Sign up for the Nature Briefing newsletter — what matters in science, free to your inbox daily.


An official website of the United States government
The .gov means it’s official. Federal government websites often end in .gov or .mil. Before sharing sensitive information, make sure you’re on a federal government site.
The site is secure. The https:// ensures that you are connecting to the official website and that any information you provide is encrypted and transmitted securely.
- Publications
- Account settings
Preview improvements coming to the PMC website in October 2024. Learn More or Try it out now .
- Advanced Search
- Journal List
- Korean J Anesthesiol
- v.72(3); 2019 Jun
Randomization in clinical studies
Chi-yeon lim.
1 Department of Biostatistics, Dongguk University College of Medicine, Goyang, Korea
2 Department of Anesthesiology and Pain Medicine, Dongguk University Ilsan Hospital, Goyang, Korea
Randomized controlled trial is widely accepted as the best design for evaluating the efficacy of a new treatment because of the advantages of randomization (random allocation). Randomization eliminates accidental bias, including selection bias, and provides a base for allowing the use of probability theory. Despite its importance, randomization has not been properly understood. This article introduces the different randomization methods with examples: simple randomization; block randomization; adaptive randomization, including minimization; and response-adaptive randomization. Ethics related to randomization are also discussed. The study is helpful in understanding the basic concepts of randomization and how to use R software.
Introduction
Statistical inference in clinical trials is a mandatory process to verify the efficacy and safety of drugs, medical devices, and procedures. It allows for generalizing the results observed through sample, so the sample by random sampling is very important. A randomized controlled trial (RCT) comparing the effects among study groups carry out to avoid any bias at the stage of the planning a study protocol. Randomization (or random allocation of subjects) can mitigate these biases with its randomness, which implies no rule or predictability for allocating subjects to treatment and control groups.
Another property of randomization is that it promotes comparability of the study groups and serves as a basis for statistical inference for quantitative evaluation of the treatment effect. Randomization can be used to create similarity of groups. In other words, all factors, whether known or unknown, that may affect the outcome can be similarly distributed among groups. This similarity is very important and allows for statistical inferences on the treatment effects. Also, it ensures that other factors except treatment do not affect the outcome. If the outcomes of the treatment group and control group show differences, this will be the only difference between the groups, leading to the conclusion that the difference is treatment induced [ 1 ].
CONSORT 1 ) , a set of guidelines proposed to improve completeness of the clinical study report, also includes randomization. Randomization plays a crucial role in increasing the quality of evidence-based studies by minimizing the selection bias that could affect the outcomes. In general, randomization places programming for random number generation, random allocation concealment for security, and a separate random code manager. After then, the generated randomization is implemented to the study [ 2 ]. Randomization is based on probability theory and hence difficult to understand. Moreover, its reproducibility problem requires the use of computer programming language. This study tries to alleviate these difficulties by enabling even a non-statistician to understand randomization for a comparative RCT design.
Methods of Randomization
The method of randomization applied must be determined at the planning stage of a study. “Randomness” cannot be predicted because it involves no rule, constraint, or characteristic. Randomization can minimize the predictability of which treatment will be performed. The method described here is called simple randomization (or complete randomization). However, the absence of rules, constraints, or characteristics does not completely eliminate imbalances by chance. For example, assume that in a multicenter study, all subjects are randomly allocated to treatment or control groups. If subjects from center A are mainly allocated to the control group and lots of subjects from center B are allocated to the treatment group, even though this is allocated with simple randomization, can we ignore the imbalance of the randomization rate in each center?
For another example, if the majority of subjects in the control group were recruited early in the study and/or the majority of those in the treatment group were recruited later in the study, can the chronological bias be ignored? The imbalance in simple randomization is often resolved through restrictive randomization, which is a slightly restricted method [ 3 , 4 ]. Furthermore, adaptive randomization can change the allocation of subjects to reflect the prognostic factors or the response to therapy during the study. The use of adaptive randomization has been increasing in recent times, but simple or restrictive randomization continues to be widely used [ 4 ]. In the Appendix , the R commands are prepared for the various randomization methods described below.
Simple randomization
In simple randomization, a coin or a die roll, for example, may be used to allocate subjects to a group. The best part of simple randomization is that it minimizes any bias by eliminating predictability. Furthermore, each subject can maintain complete randomness and independence with regard to the treatment administered [ 5 ]. This method is easy to understand and apply, 2 ) but it cannot prevent the imbalances in the sample size or prognostic factors that are likely to occur as the number of subjects participating in the study decreases. If the ratio of number of subjects shows an imbalance, that is, it is not 1 : 1, even with the same number of subjects participating, the power of the study will fall. In a study involving a total of 40 subjects in two groups, if 20 subjects are allocated to each group, the power is 80%; this will be 77% for a 25/15 subject allocation and 67% for a 30/10 subject allocation ( Fig. 1 ). 3 ) In addition, it would be difficult to consider a 25/15 or 30/10 subject allocation as aesthetically balanced. 4 ) In other words, the balancing of subjects seems plausible to both researchers and readers. Unfortunately, the nature of simple randomization rarely lets the number of subjects in both groups to be equal [ 6 ]. Therefore, if it is not out of the range of the assignment ratio (e.g., 45%–55%), 5 ) it is balanced. As the total number of subjects increases, the probability of departing from the assignment ratio, that is, the probability of imbalance, decreases. In the following, the total number of subjects and the probability of imbalance were examined in the two-group study with an assignment ratio of 45%–55% ( Fig. 2 ). If the total number of subjects is 40, the probability of the imbalance is 52.7% ( Fig. 2 , point A), but this decreases to 15.7% for 200 subjects ( Fig. 2 , point B) and 4.6% for 400 subjects ( Fig. 2 , point C). This is the randomization method recommended for large-scale clinical trials, because the likelihood of imbalance in trials with a small number of subjects is high [ 6 – 8 ]. 6 ) However, as the number of subjects does not always increase, other solutions need to be considered. A block randomization is helpful to resolve the imbalance in number of subjects, while a stratified randomization and an adaptive randomization can help resolve the imbalance in prognostic factors.

Influence of sample size ratio in two groups on power (difference (d) = 0.9, two-tailed, significant level = 0.05). The dashed line indicates the same sample size in two groups (n = 20) and maximized power.

Probability curves of imbalance between two groups for complete randomization as a function of total sample size (n). When n = 40, there is a 52.7% chance of imbalance beyond 10% (allocation ratio 45%–55%) (point A). When n = 200, there is a 15.7% chance of imbalance (point B), but n = 400 results in only 4.6% chance of imbalance (point C).
Block randomization
If we consider only the balance in number of subjects in a study involving two treatment groups A and B, then A and B can be repeatedly allocated in a randomized block design with predefined block size. Here, a selection bias is inevitable because a researcher or subject can easily predict the allocation of the group. For a small number of subjects, their number in the treatment groups will not remain the same as the study progresses, and the statistical analysis may show the problem of poor power. To avoid this, we set blocks for randomization and balance the number of subjects in each block. 7 ) When using blocks, we need to apply multiple blocks and randomize within each block. At the end of block randomization, the number of subjects can easily be balanced, and the maximum imbalance in the study can be limited to an appropriate level. That is, block randomization has the advantage of increasing the comparability between groups by keeping the ratio of the number of subjects between groups almost the same. However, if the block size is 2, the allocation result of the second subject in the block can be easily predicted with a high risk of observation bias. 8 ) Therefore, the block size used should preferably be 4 or more. However, note that even when the block size is large, if the block size is known to the researcher, the risk of selection bias will increase because the treatment of the last subject in the block will be revealed. To reduce the risk of predictability from the use of one block size, the size may be varied. 9 )
Restricted randomization for unbalanced allocation
Sometimes unbalanced allocation becomes necessary for ethical or cost reasons [ 9 ]. Furthermore, if you expect a high dropout rate in a particular group, you have to allocate more subjects. For example, for patients with terminal cancer who are not treated with conventional anticancer agents, it would be both ethical and helpful to recruit those who would be more likely to receive a newly developed anticancer drug [ 10 ] (of course, contrary to expectations, the drug could be harmful).
As for simple randomization, the probability is first determined according to the ratio between the groups, and then the subjects are allocated. If the ratio between group A and group B is 2 : 1, the probability of group A is 2/3 and that of group B is 1/3. Block randomization often uses a jar model with a random allocation rule. To consider the method, first drop as many balls as the number of subjects into the jar according to the group allocation ratio (of course, the balls have different colors depending on the group). Whenever you allocate a subject, take out one ball randomly and confirm it, and do not place the ball back into the jar (random sampling without replacement). Repeat this allocation for each block.
Stratified randomization
Some studies have prognostic factors or covariates affecting the study outcome as well as treatment. Researchers hope to balance the prognostic factors between the study groups, but randomization does not eliminate all the imbalances in prognostic factors. Stratified randomization refers to the situation where the strata are based on level of prognostic factors or covariates. For example, if “sex” is the chosen prognostic factor, the number of strata is two (male and female), and randomization is applied to each stratum. When a male subject participates, the subject is first allocated to the male strata, and the group (treatment group, control group, etc.) is determined through randomization applied to the male strata. In a multicenter study, one typical prognostic factor is the “site.” This may be due to the differences in characteristics between the subjects and the manner and procedure in which the patients are treated in each hospital.
Stratification can reduce imbalances and increase statistical power, but it has certain problems. If several important prognostic factors affect the outcome, the number of strata would increase [ 11 ]. For example, 12 (2 × 2 × 3) strata are formed solely from recruitment hospitals (sites 1 and 2), sex (male and female), and age group (under 20 years, 20–64 years, and 65 years and older) ( Fig. 3 ). In case of several strata in relation to the target sample size, the number of subjects allocated to a few strata may be empty or sparse. This causes an imbalance10) 10 ) in the number of subjects allocated to the treatment group. To reduce this risk, the prognostic factors should be carefully selected. These prognostic factors should be considered again during the statistical analysis and at the end of the study.

Example of stratification with three prognostic factors (site, sex, and age band). Eventually, randomization with 12 strata should be accomplished using 12 separate randomization processes. C: control group, T: treatment group.
Adaptive randomization
Adaptive randomization is a method of changing the allocation probability according to the progress and position of the study. It may be used to minimize the imbalance between treatment groups as well as to change the allocation probability based on the therapeutic effect. Covariate-adaptive randomization adjusts the allocation of each subject to reduce the imbalance, taking into account the imbalance of the prognostic factors. One example is the “minimization technique of randomization (minimization)” to develop indicators that collectively determine the distributional imbalance of various prognostic factors and allocates them to minimize the imbalance.
Minimization 11 )
Minimization was first introduced as a covariate adaptive method to balance the prognostic factors [ 12 , 13 ]. The first subject is allocated through simple randomization, and the subsequent ones are allocated to balance the prognostic factors. In other words, the information of the subjects who have already participated in the study is used to allocate the newly recruited subjects and minimize the imbalance of the prognostic factors [ 14 ].
Several methods have emerged following Taves [ 13 ]. Pocock and Simon define a more general method [ 12 ]. 12 ) First, the total number of imbalances is calculated after virtually allocating a newly recruited subject to all groups, respectively. Then, each group has its own the total number of imbalances. Here, this subject will be allocated to the group with lowest total number of imbalances.
We next proceed with a virtual allocation to the recruitment hospitals (Sites 1 and 2), sex (male and female), and age band (under 20 years, 20–64 years, and 65 years or older) as prognostic factors. This study has two groups: a treatment group and a control group.
Assume that the first subject (male, 52-years-old) was recruited from Site 2. Because this subject is the first one, the allocation is determined by simple randomization.
Further, assume that the subject is allocated to a treatment group. In this group, scores are added to Site 2 of the recruiting hospital, sex Male, and the 20–64 age band ( Table 1 ). Next, assume that the second subject (female, 25-years-old) was recruited through Site 2. Calculate the total number of imbalances when this subject is allocated to the treatment group and to the control group. Add the appropriate scores to the area within each group, and sum the differences between the areas.
How Adaptive Randomization Using Minimization Works
Prognostic factor | Control group | Treatment group |
---|---|---|
Site | ||
Site 1 | 0 | 0 |
Site 2 | 0 | 1 |
Sex | ||
Male | 0 | 1 |
Female | 0 | 0 |
Age band | ||
< 20 | 0 | 0 |
20–64 | 0 | 1 |
≥ 65 | 0 | 0 |
The score in each factor is 0. The first patient (sex male, 52 yr, from site 2) is allocated to the treatment group through simple randomization. Therefore, site 2, sex male, and the 20–64 years age band in the treatment group receive the score.
First, the total number of imbalances when the subject is allocated to the control group is
The total number of imbalances when the subject is allocated to the treatment group is
Since the total number of imbalances when the subject is allocated to the control group has 1 point (< 5), the second subject is allocated to the control group, and the score is added to Site 2 of the recruiting hospital, Sex female, and the 20–64 age band in the control group ( Table 2 ). Next, the third subject (Site 1, Sex male, 17-years-old) is recruited.
Prognostic factor | Control group | Treatment group | |
---|---|---|---|
If allocated to control group | Site | ||
Site 1 | 0 | 0 | |
Site 2 | 1 | 1 | |
Sex | |||
Male | 0 | 1 | |
Female | 1 | 0 | |
Age band | |||
< 20 | 0 | 0 | |
20–64 | 1 | 1 | |
≥ 65 | 0 | 0 | |
Total number of imbalances | [(1 − 1) + (1 − 0) + (1 − 1)] = 1 | ||
If allocated to treatment group | Site | ||
Site 1 | 0 | 0 | |
Site 2 | 0 | 2 | |
Sex | |||
Male | 0 | 1 | |
Female | 0 | 1 | |
Age band | |||
< 20 | 0 | 0 | |
20–64 | 0 | 2 | |
≥ 65 | 0 | 0 | |
Total number of imbalances | [(2 − 0) + (1 − 0) + (2 − 0)] = 5 |
The second patient has factors sex female, 25 yr, and site 2. If this patient is allocated to the control group, the total imbalance is 1. If this patient is allocated to the treatment group, the total imbalance is 5. Therefore, this patient is allocated to the control group, and site 2, sex female, and the 20–64 years age band in the control group receive the score.
Now, the total number of imbalances when the subject is allocated to the control group is
The total number of imbalances when the subject is allocated to the control group is 2 point (< 4). Therefore, the third subject is allocated to the control group, and the score is added to Site 1 of the recruiting hospital, sex male, and the < 20 age band ( Table 3 ). The subjects are allocated and scores added in this manner. Now, assume that the study continues, and the 15th subject (female, 74-years-old) is recruited from Site 2.
Prognostic factor | Control group | Treatment group | |
---|---|---|---|
If allocated to control group | Site | ||
Site 1 | 1 | 0 | |
Site 2 | 1 | 1 | |
Sex | |||
Male | 1 | 1 | |
Female | 1 | 0 | |
Age band | |||
< 20 | 1 | 0 | |
20–64 | 1 | 1 | |
≥ 65 | 0 | 0 | |
Total number of imbalances | [(1 − 0) + (1 − 1) + (1 − 0)] = 2 | ||
If allocated to treatment group | Site | ||
Site 1 | 0 | 1 | |
Site 2 | 1 | 1 | |
Sex | |||
Male | 0 | 2 | |
Female | 1 | 0 | |
Age band | |||
< 20 | 0 | 1 | |
20–64 | 1 | 1 | |
≥ 65 | 0 | 0 | |
Total number of imbalances | [(1 − 0) + (2 − 0) + (1 − 0)] = 4 |
The third patient has factors sex male, 17 yr, and site 1. If this patient is allocated to the control group, the total imbalance is 2. If this patient is allocated to the treatment group, the total imbalance is 4. Therefore,this patient is allocated to the control group, and then site 1, sex male, and the < 20 age band in the control group receive the score.
Here, the total number of imbalances when the subject is allocated to the control group is
The total number of imbalances when the subject is allocated to the control group is lower than that when the allocation is to the treatment group (3 < 5). Therefore, the 15th subject is allocated to the control group, and the score is added to Site 2 of the recruiting hospital, female sex, and the ≥ 65 age band ( Table 4 ). If the total number of imbalances during the minimization technique is the same, the allocation is determined by simple randomization.
Prognostic factor | Control group | Treatment group | |
---|---|---|---|
If allocated to control group | Site | ||
Site 1 | 4 | 2 | |
Site 2 | 4 | 5 | |
Sex | |||
Male | 4 | 4 | |
Female | 4 | 3 | |
Age band | |||
< 20 | 2 | 2 | |
20–64 | 2 | 2 | |
≥ 65 | 4 | 3 | |
Total number of imbalances | [(5 − 4) + (4 − 3) + (4 − 3)] = 3 | ||
If allocated to treatment group | Site | ||
Site 1 | 4 | 2 | |
Site 2 | 3 | 6 | |
Sex | |||
Male | 4 | 4 | |
Female | 3 | 4 | |
Age band | |||
< 20 | 2 | 2 | |
20–64 | 2 | 2 | |
≥ 65 | 3 | 4 | |
Total number of imbalances | [(6 − 3) + (4 − 3) + (4 − 3)] = 5 |
The 15th patient has factors sex female, 74 yr, and site 2. If this patient is allocated to the control group, the total imbalance is 3. If this patient is allocated to the treatment group, the total imbalance is 5. Therefore, this patient is allocated to the control group, and site 2, sex female, and the ≥ 65 age band in the control group receive the score.
Although minimization is designed to overcome the disadvantages of stratified randomization, this method also has drawbacks. A concern from a statistical point of view is that it does not satisfy randomness, which is the basic assumption of statistical inference [ 15 , 16 ]. For this reason, the analysis of covariance or permutation test are proposed [ 13 ]. Furthermore, exposure of the subjects’ information can lead to a certain degree of allocation prediction for the next subjects. The calculation process is complicated, but can be carried out through various programs.
Response-adaptive randomization
So far, the randomization methods is assumed that the variances of treatment effects are equal in each group. Thus, the number of subjects in both groups is determined under this assumption. However, when analyzing the data accruing as the study progresses, what happens if the variance in treatment effects is not the same? In this case, would it not reduce the number of subjects initially determined rather than the statistical power? In other words, should the allocation probabilities determined prior to the study remain constant throughout the study? Alternatively, is it possible to change the allocation probability during the study by using the data accruing as the study progresses? If the treatment effects turn out to be inferior during the study, would it be advisable to reduce the number of subjects allocated to this group [ 17 , 18 ]?
An example of response-adaptive randomization is the randomized play-the-winner rule. Here, the first subject is allocated by predefined randomization, and if this patient’s response is “success,” the next patient will be allocated to the same treatment group; otherwise, the patient will be allocated to another treatment. That is, this method is based on statistical reasoning that is not possible under a fixed allocation probability and on the ethics of allowing more patients to be allocated to treatments that benefit the patients. However, the method can lead to imbalances between the treatment groups. In addition, if clinical studies take a very long time to obtain the results of patient responses, this method cannot be recommended.
Ethics of Randomization
As noted earlier, RCT is a scientific study design based on the probability of allocating subjects to treatment groups in order to ensure comparability, form the basis of statistical inference, and identify the effects of treatment. However, an ethical debate needs to examine whether the treatment method for the subjects, especially for patients, should be determined by probability rather than by the physician. Nonetheless, the decisions should preferably be made by probability because clinical trials have the distinct goals of investigating the efficacy and safety of new medicines, medical devices, and procedures, rather than merely reach therapeutic conclusions. The purpose of the study is therefore to maintain objectivity, which is why prejudice and bias should be excluded. That is, only an unconstrained attitude during the study can confirm that a particular medicine, medical device, or procedure is effective or safe.
Consider this from another perspective. If the researcher maintains an unconstrained attitude, and the subject receives all the information, understands it, and decides to voluntarily participate, is the clinical study ethical? Unfortunately, this is not so easy to answer. Participation in a clinical study may provide the subject with the benefit of treatment, but it could be risky. Furthermore, the subjects may be given a placebo, and not treatment. Eventually, the subject may be forced to make personal sacrifices for ambiguous benefit. In other words, some subjects have to undergo further treatment, representing the cost that society has to pay for the benefit of future subjects or for a larger number of subjects [ 4 , 19 ]. This ethical dilemma on the balance between individual ethics and collective ethics [ 20 ] is still spawning much controversy. If, additionally, the researcher is biased, the controversy over this dilemma will obviously become more confused and the reliability of the study will be lowered. Therefore, randomization is a key factor in a study having to clarify causality through comparison.
Conclusions
Studies have described a random table with subsequent randomization. However, if accurate information on randomization is not provided, it would be difficult to gain enough confidence to proceed with the study and arrive at conclusions. Furthermore, probability-based treatment is allowed with the hope that the trial will be conducted through proper processes, and that the outcome will ultimately benefit the medical profession. Concurrently, it should be fully appreciated that the contribution of the subjects involved in this process is a social cost.
1) http://www.consort-statement.org/
2) However, since the results and process of randomization cannot be easily recorded, the audit of randomization is difficult.
3) Two-tailed test with difference (d) = 0.91 and type 1 error of 0.05.
4) “Cosmetic credibility” is often used.
5) The difference in number of subjects does not exceed 10% of the total number of subjects. This range is determined by a researcher, who is also able to choose 20% instead of 10%.
6) These references recommend 200 or more subjects, but it is not possible to determine the exact number.
7) Random allocation rule, truncated binomial randomization, Hadamard randomization, and the maximal procedure are forced balance randomization methods within blocks, and one of them is applied to the block. The details are beyond the scope of this study, and are therefore not covered.
8) The block size of 2 applies mainly to a study of allocating a pair at the same time.
9) Strictly speaking, the block size is randomly selected from a discrete uniform distribution, and so the use of a random block design rather than a “varying” block size would be a more formal procedure.
10) As the number of strata increases, the imbalance increases due to various factors. The details are beyond the scope of this study.
11) This paragraph introduces how to allocate “two” groups.
12) We can set the weights on the variables or the allowable range for the total number of imbalance, but in this study, we did not set any weights or allowable range for the total number of imbalances.
No potential conflict of interest relevant to this article was reported.
Authors’ contribution
Chi-Yeon Lim (Software; Supervision; Validation; Writing – review & editing)
Junyong In (Conceptualization; Software; Visualization; Writing – original draft; Writing – review & editing)
Click through the PLOS taxonomy to find articles in your field.
For more information about PLOS Subject Areas, click here .
Loading metrics
Open Access
Study Protocol
Relative motion splints versus metacarpophalangeal joint blocking splints in the management of trigger finger: Study protocol for a randomized comparative trial
Roles Investigation, Writing – original draft, Writing – review & editing
Affiliations Faculty of Health Sciences, Occupational Therapy Programme, Centre for Rehabilitation & Special Needs Studies, Universiti Kebangsaan Malaysia, Kuala Lumpur, Malaysia, Occupational Therapy Unit, Hospital Sultan Haji Ahmad Shah, Ministry of Health of Malaysia, Putrajaya, Malaysia

Roles Conceptualization, Methodology, Supervision, Writing – review & editing
* E-mail: [email protected]
Affiliation Faculty of Health Sciences, Occupational Therapy Programme, Centre for Rehabilitation & Special Needs Studies, Universiti Kebangsaan Malaysia, Kuala Lumpur, Malaysia
Roles Conceptualization, Methodology, Writing – review & editing
Affiliation Self-Employed Hand and Upper Extremity Therapy Consultant, Saint Joseph, Michigan, United States of America
Contributed equally to this work with: Hanif Farhan Mohd Rasdi, Nur Rahimawati Abdul Rahman
Roles Formal analysis, Methodology, Supervision
Roles Methodology, Supervision
Affiliation Orthopaedic Department, Hospital Sultan Haji Ahmad Shah, Ministry of Health of Malaysia, Putrajaya, Malaysia
- Li Xian Leong,
- Siaw Chui Chai,
- Julianne W. Howell,
- Hanif Farhan Mohd Rasdi,
- Nur Rahimawati Abdul Rahman
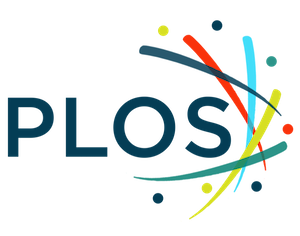
- Published: August 13, 2024
- https://doi.org/10.1371/journal.pone.0307033
- Reader Comments
Evidence supports the use of hand-based metacarpophalangeal joint (MCPJ) blocking splints as an intervention for trigger finger (TF). In practice, finger-based relative motion (RM) splints are also implemented without evidence.
This randomized comparative trial (RCT) aims to evaluate implementation of MCPJ blocking and RM splints for effectiveness, function, occupational performance and wearability after 6 weeks of TF management.
Methods and analysis
Priori analysis determined 36 individuals were needed for random assignment to the RM or MCPJ blocking splint groups. Individuals must be aged ≥21 years, and diagnosed with TF involving ≥1 finger. For blinding purposes, the primary author screens for eligibility, fabricates the splints and educates. Therapist A administers the primary outcome measures Week-1 and Week-6—stage of stenosing tenosynovitis and secondary outcome measures- number of triggering events in 10 active fists, visual analog scales (VAS) for pain, splint comfort and satisfaction, Disabilities of the Arm, Shoulder and Hand, and Canadian Occupational Performance Measure. Therapist B in Week-3 instructs participants in deep tissue massage and administers splint wearability VASs. The RM pencil test is used to determine the affected finger(s) MCPJ splint position i.e., more extension or flexion based on participant response. The MCPJ blocking splint holds the MCPJ in a neutral position. Analysis involves a mixed-effects ANOVA to compare Week-1 and Week-6 primary and secondary outcomes.
Recruitment and data collection are ongoing.
Biomechanically RM splints control tendon excursion and reduce passive tendon tension while allowing unencumbered finger motion and hand function. Hence clinicians use RM splints as an intervention for TF, despite the lack of implementation evidence. This RCT implements a function-focused as well as patient-centered approach with partial blinding of assessors and participants.
We anticipate that this study will provide evidence for the implementation of RM splints to manage adults with TF.
Trial registration
Clinical trial registration This trial is registered with ClinicalTrials.gov ( NCT05763017 ).
Citation: Leong LX, Chai SC, Howell JW, Mohd Rasdi HF, Abdul Rahman NR (2024) Relative motion splints versus metacarpophalangeal joint blocking splints in the management of trigger finger: Study protocol for a randomized comparative trial. PLoS ONE 19(8): e0307033. https://doi.org/10.1371/journal.pone.0307033
Editor: Aliah Faisal Shaheen, Brunel University London, UNITED KINGDOM OF GREAT BRITAIN AND NORTHERN IRELAND
Received: May 28, 2023; Accepted: May 15, 2024; Published: August 13, 2024
Copyright: © 2024 Leong et al. This is an open access article distributed under the terms of the Creative Commons Attribution License , which permits unrestricted use, distribution, and reproduction in any medium, provided the original author and source are credited.
Data Availability: All relevant data are within Supporting Information files.
Funding: The authors received no specific funding for this work.
Competing interests: The authors have declared that no competing interests exist.
Introduction
Trigger finger (TF) is a common condition that interferes with gliding of the digital flexor tendon and tendon sheath through the pulley system of the finger [ 1 ]. This condition may result in pain, clicking, catching and loss of motion, and is thought to be due to inflammation with the most common site near the metacarpophalangeal joint (MCPJ) at the first annular pulley (A1) [ 2 ]. TF can affect activities of daily living, notably hand functions that require precision, speed, gross power grip, 3-jaw chuck pinch, and manipulation [ 3 , 4 ] and for some, TF has been reported to contribute significantly to disability [ 3 ].
Interventions for TF can include surgery [ 2 ], corticosteroid injection [ 1 ] or splinting [ 4 ]. Generally splinting and/or injection are the first line of treatment [ 5 ]. The primary purpose for using a splint is to limit flexor tendon and sheath excursion through the A1 pulley [ 6 ]. Various hand-based and finger-based splints have been described [ 7 ]. These splints usually immobilize one finger joint, either the MCPJ, proximal interphalangeal joint (PIPJ), or distal interphalangeal joint (DIPJ). Per review of the literature [ 7 ], the most frequently cited type of splint blocks the MCPJ [ 6 , 8 – 13 ], while others block the PIPJ [ 8 , 14 , 15 ] or the DIPJ [ 10 , 16 ]. When compared with a DIPJ blocking splint, Tarbhai et al. [ 10 ] noted that the MCPJ blocking splint was preferred by patients because it felt more stable, comfortable, and their fingers were less stiff. These authors [ 10 ] reported the DIPJ blocking splint to be troublesome because it slipped off the finger easily and interfered with fingertip prehension.
Another finger-based splint which holds potential for managing TF is the relative motion (RM) splint. Originally designed to protect extensor tendon repairs of the fingers [ 17 ], the RM splint is low profile [ 17 ], small in size [ 18 ], easy to fabricate [ 18 ], and allows full mobility of the fingers minus 20–25° of MCPJ motion [ 19 ]. RM splints are named by the direction in which the affected finger’s MCPJ is positioned, either in greater extension (RME) or more flexion (RMF) [ 19 ]. The extensor tendon repair literature suggests RM splints support hand function [ 17 , 18 , 20 ], which can contribute to patient adherence [ 18 ]. Clinician experts advocate the use RM splints to manage TF [ 19 ] although evidence is lacking.
For this study, we are comparing a MCPJ blocking splint and a RM splint that limits motion of the MCPJ of the involved finger(s) by at least 20–25°.
We propose two reasons why RM splints may work as an intervention for TF at the A1 pulley: 1) the position of the affected finger in a RME splint decreases passive tension of the extensor tendon(s), which biomechanically lessens the force of flexion during active finger motion [ 21 , 22 ] and subsequently reducing the force of flexion exerted on the pulley system; and 2) the design of the RME and RMF splints limits MCPJ motion by at least 20–25° to reduce excursion of the flexor tendon and sheath through the A1 pulley without the need to fully block MCPJ motion [ 23 , 24 ].
The primary objective of this study is to compare the effectiveness of wearing either a RM or a MCPJ blocking splint for 6 weeks on therapist-observed signs and patient-report of symptoms. The secondary aim is to compare the effect of these two splints on the patient- report of hand function, occupational performance, splint comfort and satisfaction after 6-weeks of wear.
Research design
This randomized comparative trial (RCT) allocates participants into 2 splint groups: RM and MCPJ blocking. The RCT protocol is guided by the Standard Protocol Items: Recommendations for Interventional Trials (SPIRIT) [ 25 ] checklist consisting of a 33-items ( S1 File ). The flow of study begins with participant enrollment, splint group allocation, Week-1 pre-intervention assessment, splinting intervention, Week-3 mid-intervention assessment and deep tissue massage intervention and Week-6 post-intervention assessment as detailed in Figs 1 and 2 .
- PPT PowerPoint slide
- PNG larger image
- TIFF original image
Abbreviations: TF—Trigger finger; MCPJ—metacarpophalangeal joint; SST—Stages of Stenosing Tenosynovitis; VAS—Visual Analogue Scale; NTE—number of triggering events in ten active fists; DASH—Disabilities of the Arm, Shoulder and Hand outcome measure; COPM—Canadian Occupational Performance Measure and RM—relative motion.
https://doi.org/10.1371/journal.pone.0307033.g001
https://doi.org/10.1371/journal.pone.0307033.g002
Participants: Recruitment and eligibility criteria
Potential participants are recruited from Hospital Sultan Haji Ahmad Shah, Temerloh, Pahang, Malaysia. Those individuals diagnosed by an orthopedic surgeon with TF involving the A1 pulley of the finger(s) and referred to the Hospital’s Occupational Therapy Unit. For those referred to be included, the following criteria must be satisfied: at least 21 years old; one or more trigger fingers; one or both hands involved; and passive MCPJ extension to neutral of the involved finger(s). Potential participants are excluded for the following reasons:
- trigger finger involving the thumb
- steroid injection involving the affected finger within the previous 6 months
- prior surgical release of the A1 pulley involving the affected finger
- a history of fracture, tendon or nerve injury, Dupuytren’s, other soft tissue injuries involving the affected or adjacent fingers
Sampling size
G*Power was used to compute statistical power analyses and to determine the sample size. Based on a prior TF study [ 14 ] which reported a pre-intervention pain score of 4.65 (SD 2.39) and post-intervention pain score of 3.40 (SD 2.44), we calculated the Cohen-d effect size to be 0.518. The Cohen-d effect size was converted to a Cohen-f to determine the sample size needed for this mixed-effect ANOVA study. After digital conversion, the Cohen-f effect size was 0.259. With a Cohen-f effect size of 0.26, confidence level of 95%, significant level of 5%, and statistical power of 80%, 32 participants are required. Since a 10% dropout rate is anticipated, a total of 36 participants are needed with 18 patients allocated to the RM group and 18 patients to the MCPJ blocking group.
Randomization
A simple random method is used to assign participants to a splint group on Week-1 by a protocol-trained front staff person. This person selects one envelope from a group of sealed envelopes each containing a sheet of paper that designates the participant’s assignment to the RM or the MCPJ blocking group ( Fig 1 ).
Eligibility screening of potential participants is done by the Researcher (primary author LXL) prior to allocating the person to any splint group ( Fig 1 ). This strategy preserves the blinding of Therapist A and the participant during Week-1 pre-intervention assessment. Once the participant is assigned to a splint group, Therapist A, the participant, and the Researcher are no longer blinded to the type of splint. Given the nature of the study and the visibility of splint wearing, ensuring blinding of participants and assessors in splinting studies is nearly methodologically impossible [ 26 ]. Given the participant information sheet does not specify which splint group serves as the experimental treatment or comparator group, participants are considered partially blinded.
Research team
The research team consists of three occupational therapists (the first, second, and fourth authors), a certified hand therapist (the third author), and an orthopedic surgeon (the fifth author). Besides, the study also includes a front office staff person and two Occupational Therapists-Therapist A and Therapist B during the data collection process ( Fig 1 ). Eligibility screening and splint fabrication is done by the Researcher (the first author). Therapist A administers Week-1 pre-intervention and Week-6 post-intervention assessments ( Fig 1 ). Therapist B provides deep tissue massage intervention for each participant and performs the Week-3 mid-intervention assessment ( Fig 1 ).
Informed consent, registration, baseline assessment and group allocation
Once the person’s eligibility is confirmed, the Researcher issues the participant information sheet ( S1 File ) and explains the flow of the study ( Fig 1 ). If the person agrees to participate, he/she is assigned an anonymous identity number and asked to sign the informed consent form. Therapist A then administers the demographic questionnaire ( S2 File ) and completes the Week-1 pre-intervention assessment. Lastly, on Week-1, the participant is randomly assigned by a front office staff person to the RM or MCPJ blocking group ( Fig 1 ).
Splint fabrication, comfort and satisfaction rating
Participants assigned to the RM group have either a RME or RMF splint fabricated by the Researcher ( Fig 1 ). The design of the RM splint depends on the results of the pencil test [ 27 ] conducted by the Researcher ( Fig 3 ). The pencil test is used in conjunction with active finger flexion and extension to assess which position (more extension or flexion) of the MCPJ of the affected finger(s) that best reduces the participant’s reported of symptoms [ 27 ]. For the RME pencil test, the Researcher weaves a pencil under the proximal phalanx of the affected finger(s) and over the dorsum of the proximal phalanx of the adjacent fingers [ 28 ]. For the RMF pencil test, the Researcher weaves the pencil over the dorsum of the proximal phalanx of the affected finger(s) and under the proximal phalanx of the adjacent fingers [ 28 ]. With the pencil in place, for this study the Researcher asks the participant to 1) actively open and close his/her fingers several times and 2) compare the effect of the RME and RMF pencil test on his/her symptom(s). If the participant’s answer is the RME pencil test position, then a RME splint is fabricated. Conversely, if the participant’s answer is the RMF pencil test position, then a RMF splint is made. In using the RM pencil test for assessment, the trial and error splint fabrication method to determine which RM splint design (RME or RMF) is eliminated.
a) RM extension (RME) fingers open, b) RME fingers closed, c) RM flexion (RMF) fingers open and d) RMF fingers closed.
https://doi.org/10.1371/journal.pone.0307033.g003
Each RME splint includes 4 fingers and is fabricated from a 3.2mm sheet of thermoplastic. The cut width is approximately three quarters the length of the proximal phalanx and the cut length is the circumference measured around the base of all 4 proximal phalanges. For the 4-finger RME splint, the cut strip of thermoplastic is woven around the proximal phalanx of all fingers with the MCPJ of the affected finger(s) positioned in approximately 20–25° more extension than MCPJ of the adjacent fingers ( Fig 4 ). For the 4-finger RMF splint, the thermoplastic is cut the same way but the thermoplastic strip is woven in reverse with the MCPJ of the affected finger(s) positioned in approximately 20–25° more flexion ( Fig 5 ). As necessary, the differential angle of the MCPJ in either flexion or extension may be increased, if 20–25° flexion/extension is not sufficient to reduce/eliminate the participant-reported symptom(s).
https://doi.org/10.1371/journal.pone.0307033.g004
https://doi.org/10.1371/journal.pone.0307033.g005
To fabricate the MCPJ blocking splint, a T-shape pattern is cut from the sheet of 3.2 mm thermoplastic. The vertical line of T is molded to contour the palm over the volar aspect of the MCPJ, while the horizontal line of T is formed into a circumferential “ring” around the proximal phalanx of the affected finger(s) taking care not to block PIPJ motion ( Fig 6 ).
https://doi.org/10.1371/journal.pone.0307033.g006
After either the RM or MCPJ blocking splint is fabricated, the participant is asked to wear it for 15 minutes to ensure the fit is good with no pressure points. If there is pain and/or skin irritation, the splint is modified and the process repeated. Once the participant and therapist are satisfied with the fit of the splint, the participant completes Week-1 VAS for splint comfort and splint satisfaction ( S2 File ) ( Fig 1 ).
Instruction and education of participants
All participants will have the usual therapy instruction and education provided. Each participant is instructed by the Researcher to wear their splint full time, 24 hours per day, every day and night for 6 weeks ( Fig 1 ). During this time, the participant is advised to avoid finger motion that exacerbate their symptoms. Each participant was asked to keep a daily diary to report the number of hours the splint was worn during a 24-hour day and to list task(s) for which the splint is removed ( Fig 1 ).
Each participant is educated by the Researcher about the condition of TF including symptoms, cause, risk factors, and possible future interventions if splinting is not successful. Each participant is encouraged to modify or avoid activities that provoke his/her TF symptoms while in or out of the splint. Each participant is asked to contact the Researcher regarding splint fit issues or if the splint has been lost so that the problem can be addressed and documented.
Therapy intervention and follow up
Each eligible participant is required to attend 3 sessions of therapy, Week-1, Week-3, and Week-6 for this 6-week study ( Fig 1 ). During Week-3, Therapist B administers the splint satisfaction and comfort assessments and instructs participants in deep circular massage over the A1 pulley with the splint removed. After this instruction, the participant is directed to do the deep tissue massage for 5 minutes, 3 times per day at home. If at this time the splint requires modification, Therapist B will assist with this. On Week-6, Therapist A administers the post-intervention assessments to each participant ( Fig 1 ).
Outcome measures
This RCT is using the severity of triggering as the primary outcome measure to compare the effectiveness of the RM and MCPJ blocking splints. Severity is graded with the stages of stenosing tenosynovitis (SST) classification system [ 11 ]. The grading system is divided into six stages: Stage 1: normal finger movement; Stage 2: uneven finger movement, Stage 3: triggering, clicking or catching, Stage 4: finger locked in flexion or extension that can be unlocked by active finger movement, Stage 5: finger locked in flexion or extension that requires passive force to unlock, and Stage 6: finger locked in flexion or extension [ 11 ]. Therapist A will assess and record the stage of severity of triggering on Week-1 and Week-6.
Patient-report of pain is a secondary outcome measured using a visual analog scale (VAS). For this study, the 10cm VAS is labelled ‘no pain’ on the left end of the scale and ‘extreme pain’ on the other end. Week-1, Therapist A asks each participant to rate their pain by marking the VAS line and measures the distance in centimeters from the mark to the left end of the line. The participant’s perception of splint wearability i.e., comfort and satisfaction are assessed using two separate VASs. For comfort, the left end is labelled ‘not at all comfortable’ and the other end ‘extremely comfortable’. For satisfaction, the left end is labelled ‘not at all satisfied’ and the other end ‘extremely satisfied’. Participants are asked to mark each scale regarding their splint wear comfort or satisfaction. The centimeter distance from the left end of the scales to the marks are recorded as the splint comfort and satisfaction scores. The wearability VASs are administered and documented by Therapist A on Week-1 and Week-6, by Therapist B on Week-3 ( Fig 1 ).
Another secondary outcome measure to assess frequency of triggering is the number of triggering events in ten active fists (NTE) [ 29 ]. The number of triggering events is determined by Therapist A who counts and records the number of times the participant’s finger triggers during 10 repetitions of full active opening and closing of the fingers [ 29 ] ( Fig 1 ). If at any time the finger locks during this test, Therapist A will automatically assign a score of 10/10. If multiple fingers are involved, only 10 finger opening and closing will be required and the score will be that of the worst finger.
The Disabilities of the Arm, Shoulder and Hand (DASH) outcome measure is used to assess hand function. The DASH is a 30-item, self-report questionnaire designed to assess the participant’s health status during the previous week and consists of items that assess performance of select activities (21 items), severity of symptoms (5 items) and the impact of the problem on social function, work, sleep and self-image (4 items). There are two optional modules specific to work, sports and/or performing arts that are not being used in this study. The DASH has a total possible score of 100 with a higher score indicating more disability or poorer hand function [ 30 ]. Therapist A administers and records the DASH score on Week-1 and Week-6.
The Canadian Occupational Performance Measure (COPM) is used as a secondary outcome measure to compare the effect of each splint on the participant’s occupational performance [ 31 ]. Therapist A interviews and records participant’s answers on Week-1 and Week-6 to identify his/her occupation performance problem(s). Each participant is asked to prioritize activities and use a 10-point scale to rate performance and satisfaction. A higher number indicates a better performance and satisfaction rating.
Statistical analysis
Descriptive statistics will be used to summarize the demographic data including age, gender, occupation, hand dominance, digit(s) involved, associated medical conditions, and duration of triggering. Data will be analyzed and described in total numbers, mean, and the pattern of data. Descriptive statistics will also be used for the primary outcome measures of SST; and secondary outcome measures including DASH, COPM, VAS (pain, comfort, and satisfaction) and NTE. A mixed-effects ANOVA will be used to compare the splint groups Week-1 and Week-6 SST, NTE, VAS (pain, comfort and satisfaction), DASH and COPM data. The minimal dataset is shown in the S3 File .
Monitoring, ethics and dissemination of information
Potential participants for this study are recruited from a public hospital and monitored by the Clinical Research Centre (CRC) Pahang, Malaysia, a research institute under the guise of the National Institutes of Health, Ministry of Health Malaysia. Officers of the CRC conduct visits to monitor the study and issue reports according to the Centre’s protocol.
This study has been registered on ClinicalTrials.gov with the identifier NCT05763017. Ethics approval was obtained from the Medical Research and Ethics Committee of Ministry of Health (NMRR ID-22-00204-BWM) on 11 th May 2022 and the Research Ethics Committee of Universiti Kebangsaan Malaysia (JEP-2022-319) on 15 th July 2022.
Prior to becoming a study participant, each patient is given and asked to read an information sheet ( S2 File ) describing the purpose and design of the study. To participate, an informed consent is signed.
Each participant’s name is kept in a password-protected database, linked only by a study identification number and used on all participant data sheets. The data from this study will be made into a report and disseminated to Hospital Haji Ahmad Shah, the Ministry of Health, and Universiti Kebangsaan Malaysia. Once the data are gathered and the research completed, the raw materials will be stored in the locked file cabinet in the office of the Researcher. Electronic data will be secured and only accessible by a password known to the Researcher. After 5 years from study completion, all materials will be shredded and discarded by the Researcher.
The trial findings will be reported according to the Consolidated Standard of Reporting Trials (CONSORT) guidelines. The manuscript of this trial will be submitted to a peer-reviewed international scientific journal for publishing. The findings may be presented at national conferences and scientific meetings.
This study was initiated on 28 th June 2022 and is currently in the data collection phase where recruitment of participants and implementation of the intervention is ongoing.
Therapists who manage patients with hand and upper limb conditions aim to optimize the patient’s function and ability to participate in all areas of their life. Therapy intervention in this study involves two different splints designed to lessen/eliminate the symptoms of TF with minimal impact on hand function and lifestyle. Splinting with or without steroid injection is described as the first line of intervention for TF with surgery a later option if needed [ 5 ]. This study is important as the results will help to fill the knowledge gap identified by literature review [ 4 , 7 ] regarding the role of splinting as an intervention for TF. Three areas were identified as lacking [ 4 , 7 ]: 1) sufficient quality of evidence to inform practice; 2) comparative evidence between different splint deigns as related to efficacy, hand function, and occupational performance; and 3) patient-report about splint satisfaction and comfort (wearability). A review of 11 studies [ 7 ] implementing splints to manage TF included 2 that were level-1 evidence [ 10 , 32 ] as described Sackett [ 33 ], 5 were level-2 evidence [ 8 , 12 , 13 , 15 , 34 ], and 4 were level-3 evidence [ 6 , 11 , 16 , 35 ]. Of importance is that the same MCPJ blocking splint used in this RCT was also used in the level-1 studies [ 10 , 14 ]. In these studies, comparison of the MCPJ blocking splint was to finger-based splints that blocked motion at the PIPJ [ 14 ] or a DIPJ [ 10 ]. In this RCT, comparison is also made to finger-based RM splints that do not block joint motion but permit full finger mobility minus 20–25° of MCPJ motion.
A comparative study [ 20 ] between hand-based splints and finger-based RM splints implemented for protecting extensor tendon repairs concluded that the finger-based RM splints positively affected function, patient satisfaction, comfort and confirm non-comparative reports by others [ 17 , 36 ]. This RCT is different from other studies reviewed [ 7 ] as it includes patient-report of function and splint wearability. The importance of this inclusion is that patient perception has been linked to patient adherence [ 37 ]. Similarly, as noted by Tarbhai and colleagues [ 10 ] compared of MCPJ blocking splints to finger-based splints, our study also measures patient-report of function as assessed by the COPM.
The functionality of a RM splint over a hand-based splint has been evaluated with the Sollerman Test [ 20 ] and the photo-voice method [ 38 ]. For this RCT, the participants have been asked to describe their 6 weeks of splint wear experience via daily diaries. Generally, TF splint management studies [ 12 , 14 , 15 ] have used the DASH or quick-DASH to assess patient-report of function. The DASH has been included in this RCT so that comparison can be made with other studies that have used the DASH as an outcome measure.
The 2016 RM scoping review [ 19 ] informed the evidence that RM splints are being used clinically for a variety of purposes including TF despite the lack of evidence. This described scenario of practice application prior to substantiating evidence is not uncommon, thus confirming the need for this RCT.
A few strengths and limitations have been previously discussed, until this RCT is complete, there are a few additional points to consider-
- The sample size- although a power analysis was done, the participants in this study, may not fully represent the greater population of people with trigger finger.
- The risk of bias- given this is a clinical research study we did our best to minimize the risk of bias by using blinding methodology. However, at some point, the therapists, researcher, and participant will see the splint worn by each participant.
- The inclusion criteria did not exclude potential participants with co-morbidities such as carpal tunnel syndrome, diabetes mellitus, and rheumatoid arthritis. In our experience, these co-morbidities resemble the characteristics of patients with TF frequently managed by therapists.
- Patients with these aforementioned metabolic and inflammatory co-morbidities have a higher propensity to develop TF [ 32 ], which may indicate a more severe condition, which may subsequently be associated with worse health outcomes.
- Therapy contact of 3 therapy sessions was designed to reflect practice for which wearing the splint is the primary intervention. Different results may have been observed with more frequent therapy sessions and stricter controls; however, this would not have mirrored usual therapy practice.
- The Researchers of this study are relying on honest daily diary comments from participants, just as therapists do when interacting with patients in the clinical setting to evaluate the effectiveness of an intervention.
Supporting information
S1 file. spirit 2013 checklist..
https://doi.org/10.1371/journal.pone.0307033.s001
S2 File. Study protocol.
Study protocol with information sheet, consent form, demographic questionnaire, and data collection form.
https://doi.org/10.1371/journal.pone.0307033.s002
S3 File. Database.
https://doi.org/10.1371/journal.pone.0307033.s003
Acknowledgments
We would like to thank the Director General of Health Malaysia for his permission to publish this article.
- View Article
- PubMed/NCBI
- Google Scholar
- Study protocol
- Open access
- Published: 16 August 2024
Efficacy of personalized repetitive transcranial magnetic stimulation based on functional reserve to enhance ambulatory function in patients with Parkinson’s disease: study protocol for a randomized controlled trial
- Seo Jung Yun 1 , 2 , 3 ,
- Ho Seok Lee 4 ,
- Dae Hyun Kim 4 ,
- Yeun Jie Yoo 6 ,
- Na Young Kim 7 ,
- Jungsoo Lee 8 ,
- Donghyeon Kim 9 ,
- Hae-Yeon Park 5 ,
- Mi-Jeong Yoon 6 ,
- Young Seok Kim 7 ,
- Won Hyuk Chang 4 , 10 &
- Han Gil Seo ORCID: orcid.org/0000-0001-6904-7542 1 , 2
Trials volume 25 , Article number: 543 ( 2024 ) Cite this article
Metrics details
Repetitive transcranial magnetic stimulation (rTMS) is one of the non-invasive brain stimulations that modulate cortical excitability through magnetic pulses. However, the effects of rTMS on Parkinson’s disease (PD) have yielded mixed results, influenced by factors including various rTMS stimulation parameters as well as the clinical characteristics of patients with PD. There is no clear evidence regarding which patients should be applied with which parameters of rTMS. The study aims to investigate the efficacy and safety of personalized rTMS in patients with PD, focusing on individual functional reserves to improve ambulatory function.
This is a prospective, exploratory, multi-center, single-blind, parallel-group, randomized controlled trial. Sixty patients with PD will be recruited for this study. This study comprises two sub-studies, each structured as a two-arm trial. Participants are classified into sub-studies based on their functional reserves for ambulatory function, into either the motor or cognitive priority group. The Timed-Up and Go (TUG) test is employed under both single and cognitive dual-task conditions (serial 3 subtraction). The motor dual-task effect, using stride length, and the cognitive dual-task effect, using the correct response rate of subtraction, are calculated. In the motor priority group, high-frequency rTMS targets the primary motor cortex of the lower limb, whereas the cognitive priority group receives rTMS over the left dorsolateral prefrontal cortex. The active comparator for each sub-study is bilateral rTMS of the primary motor cortex of the upper limb. Over 4 weeks, the participants will undergo 10 rTMS sessions, with evaluations conducted pre-intervention, mid-intervention, immediately post-intervention, and at 2-month follow-up. The primary outcome is a change in TUG time between the pre- and immediate post-intervention evaluations. The secondary outcome variables are the TUG under cognitive dual-task conditions, Movement Disorder Society-Unified Parkinson’s Disease Rating Scale Part III, New Freezing of Gait Questionnaire, Digit Span, trail-making test, transcranial magnetic stimulation-induced motor-evoked potentials, diffusion tensor imaging, and resting state functional magnetic resonance imaging.
The study will reveal the effect of personalized rTMS based on functional reserve compared to the conventional rTMS approach in PD. Furthermore, the findings of this study may provide empirical evidence for an rTMS protocol tailored to individual functional reserves to enhance ambulatory function in patients with PD.
Trial registration
ClinicalTrials.gov NCT06350617. Registered on 5 April 2024.
Peer Review reports
Introduction
Background and rationale {6a}.
Parkinson’s disease (PD) is the second most common neurodegenerative disorder, characterized by cardinal symptoms including resting tremor, bradykinesia, and rigidity, alongside non-motor symptoms such as cognitive impairment, depression, and autonomic dysfunction [ 1 ]. The current gold standard treatment for PD involves dopaminergic medications, which alleviate symptoms without impeding disease progression [ 2 ]. However, prolonged use of these medications may lead to complications such as levodopa-induced dyskinesia [ 3 ]. Surgical interventions, such as deep brain stimulation of the subthalamic nucleus or globus pallidus interna, are available for some patients, although eligibility is limited [ 4 ]. The global prevalence of PD is increasing, which is attributable to the rapid expansion of the aging population [ 5 ]. Therefore, ongoing research into disease-modifying therapies is necessary to manage symptoms and slow disease progression.
Repetitive transcranial magnetic stimulation (rTMS) is a non-invasive brain stimulation that modulates cortical excitability through magnetic pulses [ 6 ]. In PD, rTMS has been employed to enhance motor and gait function by targeting areas such as the primary motor cortex (M1), dorsolateral prefrontal cortex (DLPFC), supplementary motor area (SMA), and cerebellum using various stimulation parameters [ 7 , 8 , 9 ]. High-frequency rTMS over the M1, DLPFC, and SMA has demonstrated positive effects on overall motor symptoms in PD [ 9 ]. While an increase in dopamine secretion in the basal ganglia via the cortico-striatal pathways may contribute to improvements in motor function, the precise underlying mechanism of rTMS in PD remains to be elucidated [ 10 , 11 ]. Conversely, intermittent theta burst stimulation of the M1 and DLPFC, or high-frequency rTMS of the bilateral motor cortex, does not significantly benefit motor function in PD [ 12 , 13 ]. Likewise, research on the application of varied rTMS methodologies to enhance motor function in PD has been conducted, yielding diverse outcomes based on these rTMS approaches (Table 1 ).
rTMS applied to the DLPFC also alleviates the non-motor symptoms of PD, such as depression and cognitive impairment [ 29 ]. Multiple sessions of high-frequency rTMS targeted at the DLPFC could enhance executive function in PD [ 26 , 30 ]. In contrast, high-frequency rTMS of the left DLPFC leads to a non-significant reduction in depressive mood among patients with PD [ 14 ]. The exact rationale behind these varied responses in PD to rTMS treatment remains speculative, highlighting the need for further research to uncover the underlying mechanisms thereof. The effects of rTMS in PD have yielded mixed results, influenced by factors including the rTMS stimulation site, frequency, intensity, total number of pulses, and the number of sessions, as well as clinical subtypes of patients with PD [ 15 , 31 ]. Furthermore, variability in the extent of basal ganglia damage among patients presents challenges in achieving consistent outcomes with standardized rTMS treatment protocols. A personalized rTMS approach targeting heterogeneous patient’ characteristics, including the presence of tremors, freezing, motor fluctuation, and dyskinesia may be necessary to maximize the effect of rTMS [ 7 ].
Recently, the concept of functional reserve has been proposed for patients with PD [ 32 ]. This concept emerged from the manifestation of inconsistent symptoms in patients with similar degrees of nigrostriatal dopaminergic deficits from dopamine transporter (DAT) imaging [ 23 ]. In a study assessing motor functional reserve using the Movement Disorder Society-Unified Parkinson’s Disease Rating Scale (MDS-UPDRS) Part III and DAT, functional connectivity analysis using resting-state functional magnetic resonance imaging (rsfMRI) confirmed that motor functional reserve was associated with the functional connectivity of brain networks in PD, involving structures such as the basal ganglia and inferior frontal lobe. Another study found an association between motor functional reserve in PD and striatal volume [ 33 ]. Hence, it is conceivable that the motor function reserve in PD is related to the neural network connectivity in the basal ganglia and frontal lobe. Additionally, the concept of resilience in patients with PD includes the cognitive functional reserve. Therefore, targeting functional improvement based on individual functional reserve, which encompasses motor and cognitive functions, as well as the degree of structural damage to the brain, is necessary for the management of PD [ 32 ].
Single-and dual-task assessments are primarily utilized to discern functional reserves in patients with PD [ 34 ]. In the early stages of PD, there is a reduction in gait automaticity due to impairment of the sensorimotor circuit of the basal ganglia [ 35 ]. Therefore, patients with PD compensate by engaging in goal-directed networks to perform dual-tasks, instead of relying on the negatively affected habitual control pathway [ 36 ]. Discrepancies in performance between single- and dual-tasks could shed light on the underlying functional adaptation mechanisms, whether motor or cognitive. One study compared brain activity patterns in groups that focused on motor and cognitive functions, revealing increased activity in the prefrontal and parietal cortex of the cerebrum among the participants in the cognitive function-focused group [ 37 ]. This suggests that patients who prioritize cognitive function may leverage prefrontal cortex functions such as coordination, concentration, and execution in their efforts for behavioral enhancement. In such patients, enhancing the cognitive network may prove to be a more efficient strategy to improve gait and daily functional abilities than attempting to restore already lost motor functions. In PD, a higher cognitive reserve is associated with a lower overall cognitive impairment and reduced severity of motor symptoms [ 38 , 39 ]. Motor reserves in PD explain the variations in motor deficits observed among patients despite having comparable levels of striatal dopamine depletion [ 40 ]. These concepts are expected to provide significant insights into the implementation of personalized rTMS interventions aimed at enhancing resilience against neurodegenerative changes.
Gait impairment in PD is one of the most disabling conditions and is associated with an increased risk of falls, reduced independence, and diminished quality of life [ 41 , 42 ]. Improvements in simple motor symptoms, while beneficial, may not directly translate into functional enhancements that offer immediate benefits to patients with PD. The Timed-Up and Go (TUG) test in patients with PD is as an invaluable instrument to evaluate transitions, balance, and gait. In addition, when combined with gait analysis under both single- and dual-task conditions, the TUG test facilitates the quantitative assessment of overall gait function [ 42 ].
Hence, the variability in symptom improvement among patients with PD could be attributed to individual differences in motor and cognitive functional reserves. Consequently, designing effective rTMS treatment protocols necessitates a thorough assessment of each patient’s functional impairment and reserve capacity. Incorporating the concepts of motor and cognitive reserves into treatment planning allows for the tailoring of individualized rTMS protocols, optimizing treatment outcomes for patients with PD.
Objectives {7}
The study aims to investigate the efficacy and safety of personalized rTMS in patients with PD, focusing on individual functional reserves to improve ambulatory function. The participants are categorized into motor or cognitive priority groups based on their functional reserve determined through single- and dual-task assessments, and specific rTMS strategies implemented that reflect their unique characteristics.
Trial design {8}
This is a prospective, exploratory, multi-center, single-blind, parallel-group, randomized controlled trial.
Methods: participants, interventions, and outcomes
Study setting {9}.
The study will be conducted across five tertiary hospitals in the Republic of Korea, including Samsung Medical Center, Seoul National University Hospital, and Yongin Severance Hospital as well as Bucheon St. Mary’s Hospital and St. Vincent’s Hospital, both of which are branches of the Catholic Medical Center. The study will be performed in accordance with the principles of Good Clinical Practice and the Declaration of Helsinki.
Eligibility criteria {10}
The inclusion criteria are as follows:
Patients clinically diagnosed with idiopathic PD following the UK Parkinson’s Disease Society Brain Bank Diagnostic Criteria
Modified Hoehn and Yahr scale 2 to 4
Patients capable of walking on level ground without the use of a gait aid
Aged ≥ 50 years
Patients who have provided informed consent and voluntarily signed the written consent form for participation in the study
The exclusion criteria include:
Patients with contraindications for rTMS, a history of epilepsy, any metal inserted into the head, or who had undergone cranial surgery
Patients exhibiting cognitive impairment based on the Korean-Montreal Cognitive Assessment test, with the following cutoff scores [ 43 ]:
< 7 points: Illiterate,
< 13 points: Education duration 0.5–3 years,
< 16 points: Education duration of 4–6 years,
< 19 points: Education duration of 7–9 years, and
< 20 points: Education duration 10 years or more
Concurrent major neurological conditions, such as spinal cord injury and stroke
Existing significant psychiatric disorders requiring continuous medication, such as major depressive disorder, schizophrenia, bipolar disorder, or dementia
Severe dyskinesia or severe on–off phenomenon
Pregnancy and lactation
Participants with contraindications for MRI, such as those with implanted devices like pacemakers
Refuse to participate in the study
Who will take informed consent? {26a}
The Korean Pharmacists Act mandates that a physician serving as the investigator should obtain informed consent from prospective clinical trial participants or their authorized representatives. Investigators are obliged to elucidate the contents of the finalized Institutional Review Board (IRB)-approved informed consent form to ensure a comprehensive understanding among potential research participants. This includes explaining the purpose of the study, the benefits, and harms involved and providing clear channels to contact both the investigator and the IRB for any questions that may arise during participation. Following consent acquisition, the investigator should promptly provide the participants with a copy of the consent form.
Additional consent provisions for collection and use of participant data and biological specimens {26b}
Not applicable. No biological specimens are collected.
Interventions
Explanation for the choice of comparators {6b}.
The comparators of both sub-studies are the active control groups. The effectiveness of high-frequency rTMS applied to bilateral primary motor cortex in the upper limb (M1-UL) in patients with PD has been previously demonstrated to enhance general motor performance and alleviate depression and anxiety, aligning with evidence-based rTMS guidelines [ 9 , 44 ]. Additionally, high-frequency stimulation of M1 has been shown to relieve musculoskeletal pain and improve the quality of life in patients with PD. Therefore, stimulation applied to bilateral M1 regions, as a conventional approach, is chosen as the comparative protocol to validate the superiority of this novel personalized rTMS.
Intervention description {11a}
This study comprises two sub-studies, each designed as a two-arm trial. The participants are classified into sub-studies based on their functional reserves as follows:
Motor priority group (sub-study 1): Patients with well-preserved motor function in whom motor skills have a significant impact on overall functioning; and
Cognitive priority group (sub-study 2): Patients with well-preserved cognitive function and notable impairment in motor function in which cognitive abilities substantially affect overall functioning.
As part of the pre-intervention assessment, the TUG and the TUG under cognitive dual-task condition (TUG-Cog) are administered. The TUG-Cog involves performing the TUG test concurrently with a cognitive task of serially subtracting the number 3. Additionally, serial 3 subtraction as a single cognitive task is performed from a randomly selected number between 80 and 100 for 20 s in the sitting position [ 34 ]. For both tasks, the correct response rate for subtraction is calculated as the time spent in seconds divided by the number of correct responses. Task-specific interference is calculated using the equation for dual-task effect (DTE) (Eq. 1 ) [ 37 ]. The motor dual-task effect (mDTE) is computed using stride length and the cognitive dual-task effect (cogDTE) is based on the correct response rate of TUG and TUG-Cog.
For evaluation of task prioritization during dual-task conditions, the modified Attention Allocation Index (mAAI) is employed (Eq. 2 ) [ 34 ]. The mAAI is calculated by subtracting the cogDTE from the mDTE, where negative values indicate an attention shift toward the motor task (motor priority), while positive values suggest an attention shift toward the cognitive task (cognitive priority).
Patients demonstrating motor priority will undergo high-frequency rTMS over the primary motor cortex in the lower limb (M1-LL), whereas those showing cognitive priority receive high-frequency rTMS over the left DLPFC. Given the substantial impairment of the motor network in the latter group, we envision a heightened reinforcement of compensatory mechanisms utilizing the cognitive network.
In the experimental group of sub-study 1, the more affected M1-LL is stimulated using a double-cone coil with a frequency of 10 Hz and an intensity set at 90% of the participant’s resting motor threshold (RMT) measured in the more affected M1-LL. The RMT in the M1-LL is determined at rest with the tibialis anterior muscle on the more affected side. The RMT is defined as the minimum intensity at which responses of 50 uV or greater are elicited in at least five out of ten trials, measured in a resting state of full relaxation. The more affected side will be determined based on the findings of the MDS-UPDRS Part III performed during at the pre-intervention evaluation. In instances where the assessment does not conclusively identify the more affected side, the onset side of PD symptoms is considered. If the side of onset remains unclear, the non-dominant side is designated as the more affected side. The stimulation protocol consisted of 5 s of stimulation followed by a 25-s rest period, repeated for a total of 20 cycles, resulting in the administration of 1000 stimuli per session. Using this protocol, each session lasts a total of 10 min.
In sub-study 2, the hot spot for the experimental group is the left DLPFC. The left DLPFC will be manually designated based on anatomical landmarks [ 45 ]. Once the left DLPFC is determined, the Neurophet tES LAB (Neurophet Inc., Seoul, Republic of Korea) will be used to obtain individual guide information relative to Cz. The software segments each individual’s T1-weighted brain image acquired at pre-intervention evaluation, reconstructs it into a three-dimensional brain model, and provides guidance for coil placement on the scalp. Investigators use the skull caps to apply stimulation at precise locations. The stimulation intensity is set at 100% of the participant’s RMT in the more affected M1-UL. Stimulation frequency, duration, cycles, and total stimuli are the same as those used in the experimental group in sub-study 1.
The bilateral M1-UL is stimulated in the control groups in both sub-studies. The stimulation intensity is adjusted to 90% of the participants’ RMT in the more affected M1-UL. The RMT of the M1-UL is determined at rest with the first dorsal interosseus muscle on the more affected side. Bilateral stimulation is conducted sequentially, starting with the more affected side, followed by the less affected side. A figure-eight coil will be used to stimulate the DLPFC and M1-UL. Other stimulation protocols are consistent with those used in the experimental groups. The stimulation intensity, frequency, duration, and total stimuli were determined based on previous study guidelines [ 44 ].
The intervention will employ a Magstim Rapid2 (Magstim Co. Ltd., UK) or MagPro X100 magnetic stimulator (MagVenture, Lucernemarken, Denmark). All participants will undergo 10 rTMS sessions, 2–3 times a week, for 10 min per session over a period of 4 weeks. After completing the initial five sessions within a 2-week period, a mid-intervention evaluation will be conducted. During each session, even-level gait training or treadmill training will be also performed for 10 min immediately after rTMS.
Criteria for discontinuing or modifying allocated interventions {11b}
The criteria for discontinuing the intervention are as follows:
Voluntary discontinuation by the participant: Participants have the freedom to withdraw from the trial at any time without providing an explanation, and this will not affect their future treatment.
Missed follow-up visits.
Discontinuation due to a significant adverse event or if the participant or legal representative requests termination due to adverse events.
Based on the investigator’s judgment, the progression of a clinical trial may be deemed unsuitable.
Significant protocol violation or deviation from inclusion/exclusion criteria during the clinical trial.
Study adherence < 80%.
Modifications of allocated interventions are not considered.
Strategies to improve adherence to interventions {11c}
Stimulation protocols for all interventions have been already established for their effectiveness in patients with PD [ 9 , 44 ]. Participants will be informed that, regardless of their assigned study group, they could expect to experience several known benefits of rTMS. Furthermore, given that all interventions will occur within the hospital, clinical research coordinators will maintain periodic contact through phone calls or text messages to remind the participants of upcoming interventions.
Relevant concomitant care permitted or prohibited during the trial {11d}
Participants are required to maintain a consistent dosage of their antiparkinsonian medication throughout the study. In addition, the same dose of physical therapy is permitted. Any changes are prohibited.
Provisions for post-trial care {30}
In this study, participants will receive compensation through the clinical trial insurance coverage held by the investigator in adherence to the study’s victim compensation protocol. This coverage extends to physical damage incurred as a result of the investigational medical device and any adverse, unintended reactions arising from the study procedures. Nevertheless, compensation is not applicable in scenarios where the investigational medical device fails to yield valid results or prove beneficial, or in cases of damage resulting from negligence on the part of the participant, among other specified exclusions.
Outcomes {12}
Pre-intervention evaluations will be conducted within 3 days before the initial intervention session. Post-intervention evaluations will be conducted within 48 h after the final intervention session. Follow-up evaluations will be conducted 2 months after the intervention concludes. The primary outcome is the difference in TUG time between the pre- and post-intervention evaluations. Secondary outcome variables include the TUG measured at follow-up evaluations. Additionally, the TUG-Cog, MDS-UPDRS Part III, New Freezing of Gait Questionnaire (NFoGQ), Digit Span, trail-making test, transcranial magnetic stimulation-induced motor-evoked potential (TMS-induced MEP), diffusion tensor imaging (DTI), and rsfMRI are assessed pre-intervention, post-intervention, and at follow-up to investigate the effectiveness of personalized rTMS.
The TUG assesses ambulatory functions, including gait, balance, mobility, and fall risk. In the TUG, the participants are instructed to rise from a chair, walk to a traffic cone located 3 m away at a comfortable pace, return to the chair, and sit down. The TUG-Cog involves the simultaneous execution of the TUG test and serial subtraction in three, starting from a randomly selected number between 80 and 100 [ 34 ]. The TUG-Cog is designed to provide a comprehensive assessment by incorporating both motor and cognitive tasks. The TUG and TUG-Cog are each measured twice, and the mean values are utilized for analysis. During the TUG and TUG-Cog, the participants wore shoes equipped with a smart insole (Gilon Gait Data Collector & Analyze MD, Gilon Inc., Gyeonggi, Republic of Korea). The smart insole measures gait parameters including stride length, step count, cadence, velocity, distance, swing ratio, and foot plantar pressure (heel/mid/toe). The stride lengths of the TUG and TUG-Cog are utilized to assign sub-studies and evaluate the efficacy of the intervention. During the mid-intervention evaluation, the TUG is conducted without smart insoles.
The MDS-UPDRS part III assesses the motor symptoms of PD using 18 items, with each item scored on a scale of 0 to 4 [ 46 ]. Higher scores indicate more severe symptoms. The NFoGQ consists of nine items designed to assess the severity of freezing of gait (FoG) and gait disturbance [ 47 ]. The NFoGQ has demonstrated reliability in measuring both the severity of FoG and its functional impact in patients with PD.
Cognitive function is assessed using the Digit Span and trail-making test. The Digit Span evaluates attention and working memory. Participants are instructed to repeat a sequence of numbers, either in the same order (forward) or in the reverse order (backward). The sequence starts with three numbers in the forward task and progresses to nine numbers, whereas the backward task involves sequences from two numbers up to eight numbers. The trail-making test evaluates cognitive functions, such as cognitive processing speed and executive function. In trail-making test A, the participant connects numbers from 1 to 15, while in trail-making test B, the task involves connecting eight numbers and seven letters (Monday to Sunday in Korean) in alternating ascending order. The examiner measures the time taken by the participants to complete the task.
Cortical excitability through TMS-induced MEP from the bilateral first dorsal interosseous muscles is utilized to evaluate the efficacy of the intervention. The intensity is set at 120% of the RMT at intervals of 5 s or more, and repeated 10 times. The average amplitude of the top five responses is measured and evaluated. Brain imaging data, including rsfMRI, DTI, and T1-weighted structural images, will be acquired using 3-T scanners (Philips Ingenia CX, Philips Elition, Siemens Magnetom Trio, and Siemens Magnetom Vida). rsfMRI will be utilized to extract brain networks based on their functional connectivity. Changes in brain network characteristics due to the intervention will be examined through connectivity strength, graph theory, and large-scale network analyses of global and local networks, as well as intrahemispheric and interhemispheric networks. During the resting-state scan, participants will be instructed to keep their eyes closed and remain motionless. A total of 180 whole-brain images will be collected at each session using the following metrics: 75 axial slices, slice thickness = 2 mm, no gap, matrix size = 112 × 112 or 124 × 124, and repetition time = 2000 ms. DTI will be employed to extract the integrity of major neural pathways and structural networks using fiber tractography and to examine changes in the characteristics of the integrity and networks due to the intervention. Each session will acquire more than 30 diffusion-weighted images with b = 1000 s/mm 2 , ensuring a minimum of 75 axial slices, a slice thickness = 2 mm, no gaps, and a matrix size of = 112 × 112 or 128 × 128. T1-weighted structural images will be used to determine the individual target positions in the DLPFC. The images will be acquired with a resolution and slice thickness of 1 mm or less, following the recommendations of the Neurophet software for 3D modeling-based target positioning.
All evaluations will be conducted in the “on” state, representing the peak effect of PD medication. Outcome measures will be assessed at pre-, post-intervention, and follow-up evaluations. Evaluation during the intervention will only proceed for the TUG, TUG-Cog, and TMS-induced MEP.
Participant timeline {13}
Figure 1 presents a comprehensive flowchart of the study process, including the allocation phase. After enrollment and screening, a pre-intervention evaluation is conducted. Based on the pre-intervention evaluation, participants are classified into sub-studies and allocated to either the experimental or control group. The first rTMS session takes place within 3 days of the pre-intervention evaluation. The initial 5 interventions are administered within 2 weeks, with a mid-intervention evaluation employed within 24 h following the fifth session. Subsequently, an additional 5 rTMS sessions are administered over the next 2 weeks, and within 48 h of completing all intervention sessions, an immediate post-intervention evaluation is conducted. Follow-up evaluation is performed 2 months after the completion of the intervention, marking the end of the study. The total study duration is anticipated to be approximately 12–14 weeks. The timelines for both the experimental and control groups in the sub-studies are identical (Table 2 ).
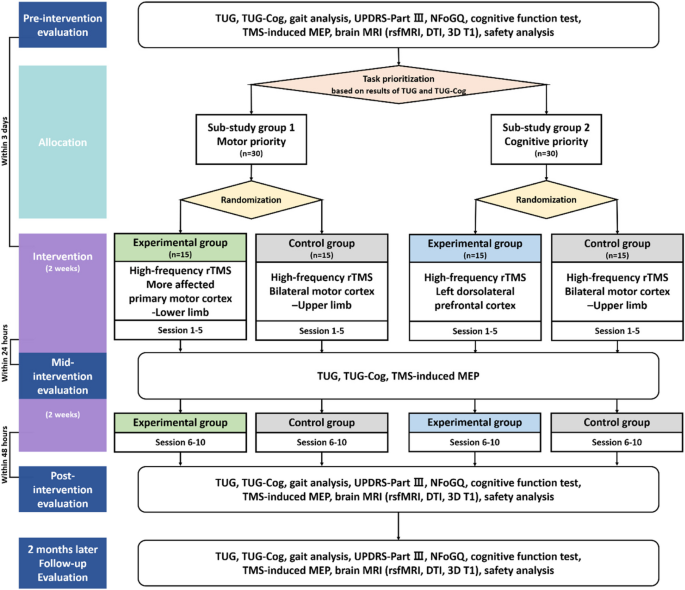
Flowchart through the entire study process TUG Timed-Up and Go, TUG-Cog Timed-Up and Go under cognitive dual-task condition, UPDRS Unified Parkinson’s Disease Rating Scale, NFoGQ New Freezing of Gait Questionnaire, TMS-induced MEP Transcranial magnetic stimulation-induced motor-evoked potential, MRI Magnetic resonance imaging, rsfMRI Resting state functional magnetic resonance imaging, DTI Diffusion tensor imaging
Sample size {14}
The primary outcome is the change in TUG time between pre- and post-intervention evaluations. The power of the study was set at 80%, with a significance level (α) of 5%. The clinically significant effect size (δ) was determined to be 4.9, and the expected standard deviation (σ) is estimated to be 4.0 [ 48 , 49 ]. The analysis was conducted using Lehr’s formula, resulting in a required sample size of 10.6 [ 50 ].
The follow-up rate was targeted at 75%, based on the conventional outpatient rehabilitation treatment criteria over a 4-week period. Therefore, the sample size for each sub-study was determined to be 30.
Recruitment {15}
Study participants will be recruited by posting notices on the bulletin boards of respective 5 hospitals. The investigators will not exclude potential participants based on race or socioeconomic status. If eligible according to the study criteria, every effort will be made to facilitate the participation of eligible patients in this research. Additionally, patients will be informed about the purpose of the study to ensure representation of the entire PD patient population receiving treatment at each institution.
Assignment of interventions: allocation
Sequence generation {16a}.
Participants are randomly allocated to the experimental and control groups of each sub-study. A designated individual, who is not involved in this study, utilizes the www.randomization.com to generate a randomization table, ensuring a 1:1 allocation between the experimental and control groups before the enrollment of the first participant. The chief investigator (CI) maintains the confidentiality of the randomization table matching list and refrains from revealing it until the completion of the final statistical analysis, managing it securely as per protocol.
Concealment mechanism {16b}
The order of assignment is concealed using an electronic data capture system until each participant is assigned.
Implementation {16c}
The allocation sequence is generated by a third party who is not involved in the study. The physician investigators enroll the participants, and based on the results of the pre-intervention evaluation, the participants are assigned to the sub-studies. The rTMS administrator verifies the electronic data capture system to determine the participant’s assigned group.
Assignment of interventions: blinding
Who will be blinded {17a}.
This study is conducted as a single-blind clinical trial with a blinded observer; the rTMS administrator is aware of the participant’s assigned group, while both the participant and assessor are unaware of which treatment is being implemented. The evaluation is performed by an investigator who does not administer rTMS, to ensure that the assessor remains blinded.
Procedure for unblinding if needed {17b}
This is a single-blind study; therefore, the assessors and participants will not be informed of the study arm. However, unblinding should be considered in cases of serious medical emergencies. In the event of a serious medical emergency, unblinding will be performed only if information regarding the stimulation protocol affects the participant’s treatment. Unblinded participants will not be permitted to continue within the study.
Data collection and management
Plans for assessment and collection of outcomes {18a}.
This multi-center study is conducted across five tertiary hospitals in the Republic of Korea. To ensure robust data quality, researchers from all the institutions convened multiple meetings to discuss and establish standardized assessment methods. Following these discussions, comprehensive training sessions were conducted to ensure that the assessors were well-versed in standardized methodologies. Most of the assessments used in this study are validated for both reliability and validity. Additionally, we meticulously document and disseminate protocols to conduct research evaluations, allowing for the ongoing scrutiny of evaluation methods and data collection forms.
Plans to promote participant retention and complete follow-up {18b}
Clinical research coordinators in each hospital will communicate with the participants via phone calls or text messages to ensure their awareness and participation in upcoming evaluations and interventions.
Data management {19}
To ensure data quality, the CI selected the clinical research organization responsible for overseeing various aspects of data management. The contract encompasses tasks, such as developing an electronic case report form (eCRF) database and implementing data management protocols. The database system is tasked with query programming, performing range checks for data values, and managing data archiving. Additionally, the data management team is responsible for creating a data validation plan, overseeing query management, coding adverse events, and reconciling serious adverse events.
Confidentiality {27}
In accordance with the ethical guidelines, personal information and research outcomes of participants will be documented on the designated eCRF without exposing personal details such as the obligation record number and name of the participant. Access to these records is restricted to registered researchers to ensure confidentiality. The identities of the participants will be kept confidential in all instances of research presentation or publication. Additionally, any research data, including imaging data and documents, will be stored in password-protected files in a secure, locked facility. Researchers are required to retain all clinical trial-related records and informed consent forms for a period of 3 years from the conclusion of the research (Bioethics and Safety Act of the Republic of Korea), and documents beyond this retention period will be disposed of in accordance with the regulations outlined in the Personal Information Protection Act of the Republic of Korea.
Plans for collection, laboratory evaluation, and storage of biological specimens for genetic or molecular analysis in this trial/future use {33}
Statistical methods, statistical methods for primary and secondary outcomes {20a}.
Demographic data are presented as means and standard deviations for continuous variables, while frequencies and percentages are used for categorical variables. Efficacy analysis are based on the assessment of the change from pre-intervention evaluation within each sub-study. To compare the baseline characteristics between the experimental and control groups in each sub-study, Student’s t -test for normally distributed variables or the Wilcoxon singed-rank test for non-normally distributed variables is employed. The Shapiro–Wilk test is used to examine the normal distribution of the variables.
To evaluate the effects of time, group, and the interaction of time with the group, we employ repeated-measures analysis of variance and repeated-measures analysis of covariance for variables exhibiting a normal distribution. Non-parametric variables are analyzed using a generalized estimating equation. Statistical significance is set at P < 0.05. A comparison between the sub-studies is not considered.
Interim analyses {21b}
Not applicable. No formal interim analysis has been planned.
Methods for additional analyses (e.g., subgroup analyses) {20b}
Not applicable. No subgroup analysis has been planned.
Methods in analysis to handle protocol non-adherence and any statistical methods to handle missing data {20c}
All participants involved in this study and those undergoing intervention are included in the intention-to-treat (ITT) set. Safety analyses are conducted based on the ITT dataset. Participants in the ITT set who undergo the TUG test at both pre- and post-intervention evaluations are categorized as the full analysis set (FAS). Efficacy analysis will be based on the FAS. Those in the ITT set who successfully complete the study with no significant protocol violations are classified into the per-protocol (PP) set. Efficacy analyses within the PP set are conducted alongside the FAS. In the event of disparities between the FAS and PP analyses, the reasons behind such difference will be investigated. For missing values, data will be analyzed using the last-observation-carried-forward method, assuming that the most recent observation was obtained at that time point.
Plans to give access to the full protocol, participant-level data and statistical code {31c}
Not applicable. The datasets analyzed during the current study and statistical code are available from the corresponding authors on reasonable request, as is the full protocol.
Oversight and monitoring
Composition of the coordinating center and trial steering committee {5d}.
To monitor trial progress, a monthly meeting will be convened by the coordinating center, consisting of the CI and Principal Investigators (PIs) from the five clinical trial sites. There is no independent trial steering committee, but each site has a Human Research Protection Program (HRPP) and a Quality Assurance (QA) department. The HRPP is responsible for protecting the rights and welfare of participants. The QA department ensures that research complies with applicable regulations, ethical principles, institutional policies, and approved protocols by conducting internal audits, managing non-compliance issues, assisting with researcher training, and implementing quality improvement measures.
Composition of the data monitoring committee, its role and reporting structure {21a}
The Data Monitoring Committee, consisting of the CI and monitoring agents from each hospital, conducts monitoring every 6 months, with additional irregular monitoring in the event of serious adverse events. The sponsor has played no role in the study’s design and not involved in the collection, analysis, interpretation of data, or writing of the manuscripts.
Adverse event reporting and harms {22}
During the clinical trial, personnel record adverse reaction details, including symptoms, onset dates, and resolution dates, in an adverse reaction record form. Severity is assessed using a scale ranging from negligible to critical. Causality is evaluated for obvious relevance, probable relevance, suspected relevance, low relevance, lack of relevance, or indeterminable status. Interventions for medical devices are categorized as discontinuation, reduction, increase, no change in dosage, unknown, or not applicable. The results of the interventions, specifying the resolution or worsening of adverse reactions, are also managed.
When reporting the study results, the PI provides a comprehensive description and assessment of all symptoms that occurred during the clinical trial. In case of a serious adverse event, the PI reports it to the IRB to determine the continuation or discontinuation of the study. Critical incidents, such as death or life-threatening events, necessitate reporting to the Ministry of Food and Drug Safety of the Republic of Korea within 7 days. Additionally, events requiring hospitalization or an extension of hospitalization resulting in irreparable damage, severe disability, or dysfunction must be reported within 15 days. The results of the interventions are methodically recorded and managed according to recovery/resolution status, ongoing recovery/resolution, non-recovery/non-resolution, recovery with residual effects, death, or unknown outcomes.
Frequency and plans for auditing trial conduct {23}
Regularly scheduled plans for auditing trials are not in place. However, audits may be conducted at any time by the Ministry of Food and Drug Safety of the Republic of Korea or by an internal auditing organization within the institution where the clinical trial is being conducted. The audit process will be independent of the investigators and sponsor.
Plans for communicating important protocol amendments to relevant parties (e.g., trial participants, ethical committees) {25}
Regarding important protocol amendments, the CI at Samsung Medical Center informs the PIs at each institution conducting the clinical trial. The CI is obligated to report these amendments to the Ministry of Food and Drug Safety of the Republic of Korea and the IRB of Samsung Medical Center. The PIs at each institution report these amendments to their respective IRBs. Additionally, PIs inform their research teams in detail about significant protocol modifications, and if necessary, notify the research participants.
Dissemination plans {31a}
The trial has been registered at ClinicalTrials.gov (NCT06350617) and we will continuously update the trial status on the site throughout the study. The publication of academic papers is scheduled within 2 years of the completion of all data collection.
This study delineates a novel investigation into the administration of personalized rTMS aimed at enhancing ambulatory function by reinforcing the preserved functional reserves in patients with PD. Based on the assessments of patient functional capacity through single- and dual-task assessments, this study aims to classify patients with PD into distinct groups according to their motor or cognitive functional reserves, with the subsequent application of rTMS targeted at specific cerebral sites. The principal objective of both the motor and cognitive priority groups is to improve of ambulatory function. In the motor priority group, the goal is achieved by enhancing motor capacity through the application of high-frequency rTMS to the M1-LL. In contrast, in the cognitive priority group, a compensatory enhancement of ambulatory function is sought by strengthening cognitive abilities facilitated by the application of high-frequency rTMS to the left DLPFC.
This study has several limitations. First, as the study is conducted across five tertiary hospitals, two different rTMS stimulators are employed, which could potentially introduce variability in the stimulation parameters and outcomes. Second, the lack of a gold standard to determine functional priorities poses a challenge in precisely categorizing patients, potentially affecting the specificity and applicability of rTMS protocol tailored to individual needs. Nevertheless, the DTE battery based on TUG and TUG-Cog has established validity and reliability [ 34 ]. Additionally, both the TUG and TUG-Cog can be easily administered without the need for specialized equipment or tools, making them feasible for widespread use in clinical settings.
In conclusion, this study will reveal the effect of personalized rTMS compared to the conventional rTMS approach in PD. Furthermore, the findings of this study may provide empirical evidence for high-frequency rTMS protocol tailored to individual functional reserves to enhance ambulatory function in patients with PD.
Trial status
Protocol version: 1.2 (26 JAN 2024).
Study start: 20 FEB 2024 (actual).
Study completion: 31 DEC 2025 (estimated).
Availability of data and materials {29}
Not applicable. Any data required to support the protocol can be supplied from the corresponding authors on request.
Abbreviations
Chief investigator
Dopamine transporter
Dorsolateral prefrontal cortex
Dual-task effect
Diffusion tensor imaging
Electronic Case Report Form
Institutional Review Board
Primary motor cortex
Primary motor cortex in the lower limb
Primary motor cortex in the upper limb
Movement Disorder Society-Unified Parkinson’s Disease Rating Scale
Magnetic resonance imaging
New Freezing of Gait Questionnaire
- Parkinson’s disease
Principal investigator
Resting motor threshold
Resting state functional magnetic resonance imaging
Repetitive transcranial direct magnetic stimulation
Transcranial direct magnetic stimulation-induced motor evoked potential
Timed-Up and Go
Timed-Up and Go under cognitive dual-task condition
Robert C, Wilson CS, Lipton RB, Arreto CD. Parkinson’s disease: evolution of the scientific literature from 1983 to 2017 by countries and journals. Parkinsonism Relat Disord. 2019;61:10–8. https://doi.org/10.1016/j.parkreldis.2018.11.011 .
Article CAS PubMed Google Scholar
Fahn S, Oakes D, Shoulson I, Kieburtz K, Rudolph A, Lang A, et al. Levodopa and the progression of Parkinson’s disease. N Engl J Med. 2004;351:2498–508. https://doi.org/10.1056/NEJMoa033447 .
Espay AJ, Morgante F, Merola A, Fasano A, Marsili L, Fox SH, et al. Levodopa-induced dyskinesia in Parkinson disease: current and evolving concepts. Ann Neurol. 2018;84:797–811. https://doi.org/10.1002/ana.25364 .
Article PubMed Google Scholar
Limousin P, Martinez-Torres I. Deep brain stimulation for Parkinson’s disease. Neurotherapeutics. 2008;5:309–19. https://doi.org/10.1016/j.nurt.2008.01.006 .
Article PubMed PubMed Central Google Scholar
Ou Z, Pan J, Tang S, Duan D, Yu D, Nong H, et al. Global trends in the incidence, prevalence, and years lived with disability of Parkinson’s disease in 204 countries/territories from 1990 to 2019. Front Public Health. 2021;9: 776847. https://doi.org/10.3389/fpubh.2021.776847 .
Chail A, Saini RK, Bhat PS, Srivastava K, Chauhan V. Transcranial magnetic stimulation: a review of its evolution and current applications. Ind Psychiatry J. 2018;27:172–80. https://doi.org/10.4103/ipj.ipj_88_18 .
Madrid J, Benninger DH. Non-invasive brain stimulation for Parkinson’s disease: clinical evidence, latest concepts and future goals: A systematic review. J Neurosci Methods. 2021;347: 108957. https://doi.org/10.1016/j.jneumeth.2020.108957 .
Chou YH, Hickey PT, Sundman M, Song AW, Chen NK. Effects of repetitive transcranial magnetic stimulation on motor symptoms in Parkinson disease: a systematic review and meta-analysis. JAMA Neurol. 2015;72:432–40. https://doi.org/10.1001/jamaneurol.2014.4380 .
Li R, He Y, Qin W, Zhang Z, Su J, Guan Q, et al. Effects of repetitive transcranial magnetic stimulation on motor symptoms in Parkinson’s disease: A meta-analysis. Neurorehab Neural Repair. 2022;36:395–404. https://doi.org/10.1177/15459683221095034 .
Article Google Scholar
Strafella AP, Ko JH, Monchi O. Therapeutic application of transcranial magnetic stimulation in Parkinson’s disease: the contribution of expectation. Neuroimage. 2006;31:1666–72. https://doi.org/10.1016/j.neuroimage.2006.02.005 .
González-García N, Armony JL, Soto J, Trejo D, Alegría MA, Drucker-Colín R. Effects of rTMS on Parkinson’s disease: a longitudinal fMRI study. J Neurol. 2011;258:1268–80. https://doi.org/10.1007/s00415-011-5923-2 .
Benninger DH, Iseki K, Kranick S, Luckenbaugh DA, Houdayer E, Hallett M. Controlled study of 50-Hz repetitive transcranial magnetic stimulation for the treatment of Parkinson disease. Neurorehab Neural Repair. 2012;26:1096–105. https://doi.org/10.1177/1545968312445636 .
Benninger DH, Berman BD, Houdayer E, Pal N, Luckenbaugh DA, Schneider L, et al. Intermittent theta-burst transcranial magnetic stimulation for treatment of Parkinson disease. Neurology. 2011;76:601–9. https://doi.org/10.1212/WNL.0b013e31820ce6bb .
Article CAS PubMed PubMed Central Google Scholar
Brys M, Fox MD, Agarwal S, Biagioni M, Dacpano G, Kumar P, et al. Multifocal repetitive TMS for motor and mood symptoms of Parkinson disease: a randomized trial. Neurology. 2016;87:1907–15. https://doi.org/10.1212/WNL.0000000000003279 .
Khedr EM, Al-Fawal B, Abdel Wraith A, Saber M, Hasan AM, Bassiony A, et al. The effect of 20 Hz versus 1 Hz repetitive transcranial magnetic stimulation on motor dysfunction in Parkinson’s disease: which is more beneficial? J Parkinsons Dis. 2019;9:379–87. https://doi.org/10.3233/JPD-181540 .
Spagnolo F, Fichera M, Chieffo R, Dalla Costa G, Pisa M, Volonté MA, et al. Bilateral repetitive transcranial magnetic stimulation with the H-coil in Parkinson's disease: A randomized, Sham-controlled study. Front neurol. 2021;11;584713. https://doi.org/10.3389/fneur.2020.584713 .
Makkos A, Pál E, Aschermann Z, Janszky J, Balázs É, Takács K, et al. High-frequency repetitive transcranial magnetic stimulation can improve depression in Parkinson's disease: a randomized, double-blind, placebo-controlled study. Neuropsychobiology. 2016;73;169–77. https://doi.org/10.1159/000445296 .
Yokoe M, Mano T, Maruo T, Hosomi K, Shimokawa T, Kishima H, et al. The optimal stimulation site for high-frequency repetitive transcranial magnetic stimulation in Parkinson’s disease: A double-blind crossover pilot study. J Clin Neurosci. 2018;47;72–8. https://doi.org/10.1016/j.jocn.2017.09.023 .
Li J, Mi TM, Zhu BF, Ma JH, Han C, Li Y, et al. High-frequency repetitive transcranial magnetic stimulation over the primary motor cortex relieves musculoskeletal pain in patients with Parkinson's disease: a randomized controlled trial. Parkinsonism relat disord. 2020;80;113–9. https://doi.org/10.1016/j.brs.2013.05.002 .
Chang WH, Kim MS, Cho JW, Youn J, Kim YK, Kim SW, et al. Effect of cumulative repetitive transcranial magnetic stimulation on freezing of gait in patients with atypical Parkinsonism: A pilot study. J rehabil med. 2016;48;824–8. https://doi.org/10.2340/16501977-2140 .
Kim MS, Chang WH, Cho JW, Youn J, Kim YK, Kim SW, et al. Efficacy of cumulative high-frequency rTMS on freezing of gait in Parkinson’s disease. Restor Neurol Neurosci. 2015;33;521–30. https://doi.org/10.3233/RNN-140489 .
Maruo T, Hosomi K, Shimokawa T, Kishima H, Oshino S, Morris S, et al. High-frequency repetitive transcranial magnetic stimulation over the primary foot motor area in Parkinson's disease. Brain stimul. 2013;6;884–91. https://doi.org/10.1016/j.brs.2013.05.002 .
Chung SJ, Kim HR, Jung JH, Lee PH, Jeong Y, Sohn YH. Identifying the functional brain network of motor reserve in early Parkinson’s disease. Mov Disord. 2020;35:577–86. https://doi.org/10.1002/mds.28012 .
Yang YR, Tseng CY, Chiou SY, Liao KK, Cheng SJ, Lai KL, et al. Combination of rTMS and treadmill training modulates corticomotor inhibition and improves walking in Parkinson disease: a randomized trial. Neurorehabil neural repair. 2013;27;79–86. https://doi.org/10.1177/1545968312451915 .
Khedr EM, Farweez HM, Islam H. Therapeutic effect of repetitive transcranial magnetic stimulation on motor function in Parkinson's disease patients. Eur J Neurol. 2003;10;567–72. https://doi.org/10.1046/j.1468-1331.2003.00649.x .
Pal E, Nagy F, Aschermann Z, Balazs E, Kovacs N. The impact of left prefrontal repetitive transcranial magnetic stimulation on depression in Parkinson’s disease: a randomized, double-blind, placebo-controlled study. Mov Disord. 2010;25:2311–7. https://doi.org/10.1002/mds.23270 .
del Olmo MF, Bello O, Cudeiro J. Transcranial magnetic stimulation over dorsolateral prefrontal cortex in Parkinson’s disease. Clin Neurophysiol. 2007;118;131–9. https://doi.org/10.1016/j.clinph.2006.09.002 .
Lomarev MP, Kanchana S, Bara‐Jimenez W, Iyer M, Wassermann EM, Hallett M. Placebo‐controlled study of rTMS for the treatment of Parkinson's disease. Mov Disord. 2006;21;325–31. https://doi.org/10.1002/mds.20713 .
Hai-Jiao W, Ge T, Li-Na Z, Deng C, Da X, Shan-Shan C, et al. The efficacy of repetitive transcranial magnetic stimulation for Parkinson disease patients with depression. Int J Neurosci. 2020;130:19–27. https://doi.org/10.1080/00207454.2018.1495632 .
Jiang Y, Guo Z, McClure MA, He L, Mu Q. Effect of rTMS on Parkinson’s cognitive function: a systematic review and meta-analysis. BMC Neurol. 2020;20:377. https://doi.org/10.1186/s12883-020-01953-4 .
Shirota Y, Hamada M, Ugawa Y. Clinical applications of rTMS in Parkinson’s disease. In: Platz T, editor. Therapeutic rTMS in Neurology. Cham: Springer. https://doi.org/10.1007/978-3-319-25721-1_9 .
Hoenig MC, Dzialas V, Drzezga A, van Eimeren T. The concept of motor reserve in Parkinson’s disease: new wine in old bottles? Mov Disord. 2023;38(FZJ-2023–00164):16–20. https://doi.org/10.1002/mds.29266 .
Jeong SH, Lee EC, Chung SJ, Lee HS, Jung JH, Sohn YH, et al. Local striatal volume and motor reserve in drug-naïve Parkinson’s disease. npj Parkinsons Dis. 2022;8:168. https://doi.org/10.1038/s41531-022-00429-1 .
Longhurst JK, Rider JV, Cummings JL, John SE, Poston B, Held Bradford EC, et al. A novel way of measuring dual-task interference: the reliability and construct validity of the dual-task effect battery in neurodegenerative disease. Neurorehab Neural Repair. 2022;36:346–59. https://doi.org/10.1177/15459683221088864 .
Rodriguez-Oroz MC, Jahanshahi M, Krack P, Litvan I, Macias R, Bezard E, et al. Initial clinical manifestations of Parkinson’s disease: features and pathophysiological mechanisms. Lancet Neurol. 2009;8:1128–39. https://doi.org/10.1016/S1474-4422(09)70293-5 .
Wu T, Hallett M, Chan P. Motor automaticity in Parkinson’s disease. Neurobiol Dis. 2015;82:226–34. https://doi.org/10.1016/j.nbd.2015.06.014 .
Zhang X, Wang Y, Lu J, Wang J, Shu Z, Cheng Y, et al. Fronto-parietal cortex activation during walking in patients with Parkinson’s disease adopting different postural strategies. Front Neurol. 2022;13: 998243. https://doi.org/10.3389/fneur.2022.998243 .
Loftus AM, Gasson N, Lopez N, Sellner M, Reid C, Cocks N, et al. Cognitive reserve, executive function, and memory in Parkinson’s disease. Brain Sci. 2021;11: 992. https://doi.org/10.3390/brainsci11080992 .
Guzzetti S, Mancini F, Caporali A, Manfredi L, Daini R. The association of cognitive reserve with motor and cognitive functions for different stages of Parkinson’s disease. Exp Gerontol. 2019;115:79–87. https://doi.org/10.1016/j.exger.2018.11.020 .
Chung SJ, Lee JJ, Lee PH, Sohn YH. Emerging concepts of motor reserve in Parkinson’s disease. J Mov Disord. 2020;13:171–84. https://doi.org/10.14802/jmd.20029 .
Vallabhajosula S, Buckley TA, Tillman MD, Hass CJ. Age and Parkinson’s disease related kinematic alterations during multi-directional gait initiation. Gait Posture. 2013;37:280–6. https://doi.org/10.1016/j.gaitpost.2012.07.018 .
Mirelman A, Bonato P, Camicioli R, Ellis TD, Giladi N, Hamilton JL, et al. Gait impairments in Parkinson’s disease. Lancet Neurol. 2019;18:697–708. https://doi.org/10.1016/S1474-4422(19)30044-4 .
Kim JI, Sunwoo MK, Sohn YH, Lee PH, Hong JY. The MMSE and MoCA for screening cognitive impairment in less educated patients with Parkinson’s disease. J Mov Disord. 2016;9:152–9.
Lefaucheur JP, Aleman A, Baeken C, Benninger DH, Brunelin J, Di Lazzaro V, et al. Evidence-based guidelines on the therapeutic use of repetitive transcranial magnetic stimulation (rTMS): an update (2014–2018). Clin Neurophysiol. 2020;131:474–528. https://doi.org/10.1016/j.clinph.2019.11.002 .
Mylius V, Ayache SS, Ahdab R, Farhat WH, Zouari HG, Belke M, Brugières P, Wehrmann E, Krakow K, Timmesfeld N, Schmidt S. Definition of DLPFC and M1 according to anatomical landmarks for navigated brain stimulation: inter-rater reliability, accuracy, and influence of gender and age. Neuroimage. 2013;78:224–32. https://doi.org/10.1016/j.neuroimage.2013.03.061 .
Goetz CG, Tilley BC, Shaftman SR, Stebbins GT, Fahn S, Martinez-Martin P, et al. Movement Disorder Society-sponsored revision of the Unified Parkinson’s disease Rating Scale (MDS-UPDRS): scale presentation and clinimetric testing results. Mov Disord. 2008;23:2129–70. https://doi.org/10.1002/mds.22340 .
Nieuwboer A, Rochester L, Herman T, Vandenberghe W, Emil GE, Thomaes T, et al. Reliability of the new freezing of gait questionnaire: agreement between patients with Parkinson’s disease and their carers. Gait Posture. 2009;30:459–63. https://doi.org/10.1016/j.gaitpost.2009.07.108 .
Dal Bello-Haas V, Klassen L, Sheppard MS, Metcalfe A. Psychometric properties of activity, self-efficacy, and quality-of-life measures in individuals with Parkinson disease. Physiother Can. 2011;63:47–57. https://doi.org/10.3138/ptc.2009-08 .
Chung CLH, Mak MKY, Hallett M. Transcranial magnetic stimulation promotes gait training in Parkinson disease. Ann Neurol. 2020;88:933–45. https://doi.org/10.1002/ana.25881 .
Lehr R. Sixteen S-squared over D-squared: A relation for crude sample size estimates. Stat Med. 1992;11:1099–102. https://doi.org/10.1002/sim.4780110811 .
Download references
Acknowledgements
Not applicable.
This research was supported by the K-Brain Project of the National Research Foundation (NRF) funded by the Korean government (Ministry of Science and ICT, MSIT) (No. RS-2023–00265824).
Author information
Authors and affiliations.
Department of Rehabilitation Medicine, Seoul National University Hospital, Seoul, Republic of Korea
Seo Jung Yun & Han Gil Seo
Department of Rehabilitation Medicine, Seoul National University College of Medicine, Seoul, Republic of Korea
Department of Human Systems Medicine, Seoul National University College of Medicine, Seoul, Republic of Korea
Seo Jung Yun
Department of Physical and Rehabilitation Medicine, Center for Prevention and Rehabilitation, Heart Vascular Stroke Institute, Samsung Medical Center, Sungkyunkwan University School of Medicine, Seoul, Republic of Korea
Ho Seok Lee, Dae Hyun Kim & Won Hyuk Chang
Department of Rehabilitation Medicine, Bucheon St. Mary’s Hospital, College of Medicine, The Catholic University of Korea, Seoul, Republic of Korea
Sun Im & Hae-Yeon Park
Department of Rehabilitation Medicine, St. Vincent’s Hospital, College of Medicine, The Catholic University of Korea, Seoul, Republic of Korea
Yeun Jie Yoo & Mi-Jeong Yoon
Department of Rehabilitation Medicine, Yongin Severance Hospital, Yonsei University College of Medicine, Yongin, Republic of Korea
Na Young Kim & Young Seok Kim
Department of Medical IT Convergence Engineering, Kumoh National Institute of Technology, Gumi, Republic of Korea
Jungsoo Lee
Research Institute, NEUROPHET Inc, Seoul, Republic of Korea
Donghyeon Kim
Department of Health Science and Technology, Department of Medical Device Management and Research, SAIHST, Sungkyunkwan University, Seoul, Republic of Korea
Won Hyuk Chang
You can also search for this author in PubMed Google Scholar
Contributions
SJY contributed to the development of the protocol, organized the protocol, and wrote the first draft of the manuscript. HSL, DHK, YJY, JL, DK, H-YP, M-JY, and YSK organized the protocol. SI and NYK conceptualized and organized the protocol. WHC and HGS, as the corresponding authors, conceived the study, and conceptualized, developed, and organized the protocol. All authors read and approved the final manuscript.
Corresponding authors
Correspondence to Won Hyuk Chang or Han Gil Seo .
Ethics declarations
Ethics approval and consent to participate {24}.
The study protocol was approved by the Institutional Review Board of the Samsung Medical Center, Seoul National University Hospital, Yongin Severance Hospital, and Catholic Medical Center (IRB No. 2023-12-028, 2402-073-1511, 9-2024-0015, and XC240NDS0007, respectively). Written, informed consent to participate will be obtained from all participants.
Consent for publication {32}
Not applicable. No identifying images or other personal or clinical details of participants are presented here or will be presented in reports of the trial results. The participant information materials and informed consent form are available from the corresponding authors on request.
Competing interests {28}
DK is the CTO at NEUROPHET INC., Seoul, Republic of Korea, whose neuroimaging software was used in this study. Otherwise, there are no conflicts of interest to declare.
Additional information
Publisher’s note.
Springer Nature remains neutral with regard to jurisdictional claims in published maps and institutional affiliations.
Supplementary Information
Supplementary material 1., rights and permissions.
Open Access This article is licensed under a Creative Commons Attribution 4.0 International License, which permits use, sharing, adaptation, distribution and reproduction in any medium or format, as long as you give appropriate credit to the original author(s) and the source, provide a link to the Creative Commons licence, and indicate if changes were made. The images or other third party material in this article are included in the article's Creative Commons licence, unless indicated otherwise in a credit line to the material. If material is not included in the article's Creative Commons licence and your intended use is not permitted by statutory regulation or exceeds the permitted use, you will need to obtain permission directly from the copyright holder. To view a copy of this licence, visit http://creativecommons.org/licenses/by/4.0/ . The Creative Commons Public Domain Dedication waiver ( http://creativecommons.org/publicdomain/zero/1.0/ ) applies to the data made available in this article, unless otherwise stated in a credit line to the data.
Reprints and permissions
About this article
Cite this article.
Yun, S.J., Lee, H.S., Kim, D.H. et al. Efficacy of personalized repetitive transcranial magnetic stimulation based on functional reserve to enhance ambulatory function in patients with Parkinson’s disease: study protocol for a randomized controlled trial. Trials 25 , 543 (2024). https://doi.org/10.1186/s13063-024-08385-2
Download citation
Received : 28 April 2024
Accepted : 06 August 2024
Published : 16 August 2024
DOI : https://doi.org/10.1186/s13063-024-08385-2
Share this article
Anyone you share the following link with will be able to read this content:
Sorry, a shareable link is not currently available for this article.
Provided by the Springer Nature SharedIt content-sharing initiative
- Functional reserve
- Repetitive transcranial magnetic stimulation
ISSN: 1745-6215
- Submission enquiries: Access here and click Contact Us
- General enquiries: [email protected]

An official website of the United States government
The .gov means it’s official. Federal government websites often end in .gov or .mil. Before sharing sensitive information, make sure you’re on a federal government site.
The site is secure. The https:// ensures that you are connecting to the official website and that any information you provide is encrypted and transmitted securely.
- Publications
- Account settings
- My Bibliography
- Collections
- Citation manager
Save citation to file
Email citation, add to collections.
- Create a new collection
- Add to an existing collection
Add to My Bibliography
Your saved search, create a file for external citation management software, your rss feed.
- Search in PubMed
- Search in NLM Catalog
- Add to Search
A note on stratifying versus complete random assignment in clinical trials
- PMID: 7160192
- DOI: 10.1016/0197-2456(82)90026-5
The efficiency of stratifying on a risk factor before randomization as opposed to complete randomization and adjustment for the risk factor by post-randomization analysis is examined by calculating the variance of the estimated difference between two treatment means for the two design strategies. This analysis shows that in small samples relying on post-randomization stratification can lead to significant loss in efficiency when compared to prerandomization stratification. In moderate and large samples the two design strategies should have similar variances.
PubMed Disclaimer
Similar articles
- Designing for cancer clinical trials: selection of prognostic factors. Brown BW Jr. Brown BW Jr. Cancer Treat Rep. 1980 Feb-Mar;64(2-3):499-502. Cancer Treat Rep. 1980. PMID: 7407790
- Prognostic factors for randomization and stratification and endpoints for the evaluation of trials. Sylvester RJ, Suciu S, Yamanaka H. Sylvester RJ, et al. Prog Clin Biol Res. 1988;269:275-87. Prog Clin Biol Res. 1988. PMID: 3293065 Review. No abstract available.
- Adaptive allocation in randomized controlled trials. Birkett NJ. Birkett NJ. Control Clin Trials. 1985 Jun;6(2):146-55. doi: 10.1016/0197-2456(85)90120-5. Control Clin Trials. 1985. PMID: 4006488
- Randomization designs in comparative clinical trials. Ellenberg SS. Ellenberg SS. N Engl J Med. 1984 May 24;310(21):1404-8. doi: 10.1056/NEJM198405243102141. N Engl J Med. 1984. PMID: 6717522 No abstract available.
- Randomization in substance abuse clinical trials. Hedden SL, Woolson RF, Malcolm RJ. Hedden SL, et al. Subst Abuse Treat Prev Policy. 2006 Feb 6;1:6. doi: 10.1186/1747-597X-1-6. Subst Abuse Treat Prev Policy. 2006. PMID: 16722591 Free PMC article. Review.
- A multidomain lifestyle intervention to maintain optimal cognitive functioning in Dutch older adults-study design and baseline characteristics of the FINGER-NL randomized controlled trial. Deckers K, Zwan MD, Soons LM, Waterink L, Beers S, van Houdt S, Stiensma B, Kwant JZ, Wimmers SCPM, Heutz RAM, Claassen JAHR, Oosterman JM, de Heus RAA, van de Rest O, Vermeiren Y, Voshaar RCO, Smidt N, Broersen LM, Sikkes SAM, Aarts E; MOCIA consortium; FINGER-NL consortium; Köhler S, van der Flier WM. Deckers K, et al. Alzheimers Res Ther. 2024 Jun 13;16(1):126. doi: 10.1186/s13195-024-01495-8. Alzheimers Res Ther. 2024. PMID: 38872204 Free PMC article. Clinical Trial.
- Study design and methods: U.S. study to protect brain health through lifestyle intervention to reduce risk (U.S. POINTER). Baker LD, Snyder HM, Espeland MA, Whitmer RA, Kivipelto M, Woolard N, Katula J, Papp KV, Ventrelle J, Graef S, Hill MA, Rushing S, Spell J, Lovato L, Felton D, Williams BJ, Ghadimi Nouran M, Raman R, Ngandu T, Solomon A, Wilmoth S, Cleveland ML, Williamson JD, Lambert KL, Tomaszewski Farias S, Day CE, Tangney CC, Gitelman DR, Matongo O, Reynolds T, Pavlik VN, Yu MM, Alexander AS, Elbein R, McDonald AM, Salloway S, Wing RR, Antkowiak S, Morris MC, Carrillo MC; U.S. POINTER Study Group. Baker LD, et al. Alzheimers Dement. 2024 Feb;20(2):769-782. doi: 10.1002/alz.13365. Epub 2023 Sep 30. Alzheimers Dement. 2024. PMID: 37776210 Free PMC article. Clinical Trial.
- Time-trend impact on treatment estimation in two-arm clinical trials with a binary outcome and Bayesian response adaptive randomization. Jiang Y, Zhao W, Durkalski-Mauldin V. Jiang Y, et al. J Biopharm Stat. 2020;30(1):69-88. doi: 10.1080/10543406.2019.1607368. Epub 2019 Apr 24. J Biopharm Stat. 2020. PMID: 31017843 Free PMC article.
- Design and baseline characteristics of the CARdiovascular Outcome Trial of LINAgliptin Versus Glimepiride in Type 2 Diabetes (CAROLINA®). Marx N, Rosenstock J, Kahn SE, Zinman B, Kastelein JJ, Lachin JM, Espeland MA, Bluhmki E, Mattheus M, Ryckaert B, Patel S, Johansen OE, Woerle HJ. Marx N, et al. Diab Vasc Dis Res. 2015 May;12(3):164-74. doi: 10.1177/1479164115570301. Epub 2015 Mar 15. Diab Vasc Dis Res. 2015. PMID: 25780262 Free PMC article. Clinical Trial.
- Healthy Living Partnerships to Prevent Diabetes (HELP PD): design and methods. Katula JA, Vitolins MZ, Rosenberger EL, Blackwell C, Espeland MA, Lawlor MS, Rejeski WJ, Goff DC. Katula JA, et al. Contemp Clin Trials. 2010 Jan;31(1):71-81. doi: 10.1016/j.cct.2009.09.002. Epub 2009 Sep 13. Contemp Clin Trials. 2010. PMID: 19758580 Free PMC article. Clinical Trial.
Publication types
- Search in MeSH
Related information
- Cited in Books
Grants and funding
- 71-2243/PHS HHS/United States
LinkOut - more resources
Full text sources.
- Elsevier Science
- MedlinePlus Health Information
- Citation Manager
NCBI Literature Resources
MeSH PMC Bookshelf Disclaimer
The PubMed wordmark and PubMed logo are registered trademarks of the U.S. Department of Health and Human Services (HHS). Unauthorized use of these marks is strictly prohibited.
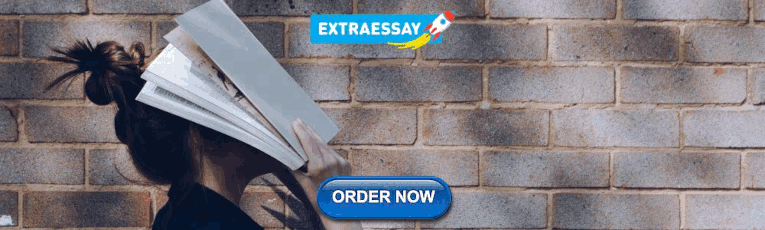
IMAGES
COMMENTS
In experimental research, random assignment is a way of placing participants from your sample into different treatment groups using randomization. ... Example: Non-random assignment In your clinical study, you recruit participants using flyers. at gyms, cafes, and local community centers. You use a haphazard method to assign participants to ...
Background. Various research designs can be used to acquire scientific medical evidence. The randomized controlled trial (RCT) has been recognized as the most credible research design for investigations of the clinical effectiveness of new medical interventions [1, 2].Evidence from RCTs is widely used as a basis for submissions of regulatory dossiers in request of marketing authorization for ...
Various research designs can be used to acquire scientific medical evidence. The randomized controlled trial (RCT) has been recognized as the most credible research design for investigations of the clinical effectiveness of new medical interventions [1, 2].Evidence from RCTs is widely used as a basis for submissions of regulatory dossiers in request of marketing authorization for new drugs ...
In RCT, random assignment is important and performing it is easy if you know how to do it. Besides the practice of randomization, correct reporting of the randomization process is also important and it should be done very accurately. ... Montané E, Vallano A, Vidal X, Aguilera C, Laporte JR. Reporting randomised clinical trials of analgesics ...
This JAMA Guide to Statistics and Methods explains sequential, multiple assignment, randomized trial (SMART) study designs, in which some or all participants are randomized at 2 or more decision points depending on the participant's response to prior treatment.
The primary goal of comparative clinical trials is to provide comparisons of treatments with maximum precision and validity. 4 One critical component of clinical trials is random assignment of participants into groups. Randomizing participants helps remove the effect of extraneous variables (eg, age, injury history) and minimizes bias ...
Abstract. In this article, we illustrate a new method for random selection and random assignment that we developed in a pilot study for a randomized clinical trial. The randomization database is supported by a commonly available spreadsheet. Formulas were written for randomizing participants and for creating a "shadow" system to verify ...
By Jim Frost 4 Comments. Random assignment uses chance to assign subjects to the control and treatment groups in an experiment. This process helps ensure that the groups are equivalent at the beginning of the study, which makes it safer to assume the treatments caused any differences between groups that the experimenters observe at the end of ...
A Randomized Control Trial (RCT) is a type of scientific experiment that randomly assigns participants to an experimental group or a control group to measure the effectiveness of an intervention or treatment. ... scientists view RCTs as the gold standard for clinical trials. Random Allocation. Random allocation and random assignment are terms ...
Clinical trials aiming for a 1:1 assignment seek to attain equal experience with both treatments. As discussed above, using simple randomization may not guarantee that assignment when the sample size is small. ... For randomized clinical trials, tables of baseline characteristics also help show whether randomization is doing its job. Data and ...
randomization. Large clinical trials don't use stratification. It is unlikely to get imbalance in subject characteristics in a large randomized trial. 4. Unequal Randomization Most randomized trials allocate equal numbers of patients to experimental and control groups. This is the most statistically efficient randomization ratio as it maximizes
14 Statistical Methods for Use in the Analysis of Randomized Clinical Trials Utilizing a Pretreatment, Posttreatment, Follow-up (PPF) Paradigm Notes. Notes. 15 ... random assignment, the evaluation of treatment response across time, participant selection, study setting, properly defining and checking the integrity of the independent variable (i ...
Random assignment, blinding, and controlling are key aspects of the design of experiments because they help ensure that the results are not spurious or deceptive via confounding. This is why randomized controlled trials are vital in clinical research, especially ones that can be double-blinded and placebo-controlled.
Randomized controlled trials (RCTs) have traditionally been viewed as the gold standard of clinical trial design, residing at the top of the hierarchy of levels of evidence in clinical study; this is because the process of randomization can minimize differences in characteristics of the groups that may influence the outcome, thus providing the ...
In an experimental study, random assignment is a process by which participants are assigned, with the same chance, to either a treatment or a control group. The goal is to assure an unbiased assignment of participants to treatment options. ... Fundamentals of Clinical Trials. 5th ed. 2015 edition. Springer; 2015. Further reading. Posttest-Only ...
The "gold standard" of clinical research are randomized controlled trials (RCTs), which aim to avoid bias by randomly assigning patients into different groups, which can then be compared to evaluate the new drug or treatment. The process of random assignment of patients to groups is called randomization. Randomization in clinical trials is ...
A randomized controlled trial (or randomized control trial; [ 2] RCT) is a form of scientific experiment used to control factors not under direct experimental control. Examples of RCTs are clinical trials that compare the effects of drugs, surgical techniques, medical devices, diagnostic procedures, diets or other medical treatments. [ 3][ 4]
Abstract. Random assignment of treatments is an essential feature of experimental design in general and clinical trials in particular. It provides broad comparability of treatment groups and validates the use of statistical methods for the analysis of results. Various devices are available for improving the balance of prognostic factors across ...
Key concerns over the statistical analysis particularly include the inconsistency between the statistical analysis plan and the reported analyses, departure from the original randomised assignment ...
In clinical research, randomized trials are widely accepted as the definitive method of evaluating the efficacy of therapies. Random assignment of patients to treatment ensures internal validity of the comparison of new treatments with controls. An assessment of external validity can best be achieve …
Many procedures have been proposed for the random assignment of participants to treatment groups in clinical trials. In this article, common randomization techniques, including simple randomization, block randomization, stratified randomization, and covariate adaptive randomization, are reviewed.
Randomized controlled trial is widely accepted as the best design for evaluating the efficacy of a new treatment because of the advantages of randomization (random allocation). ... if it is not out of the range of the assignment ratio (e.g ... Ethics and practice: alternative designs for phase III randomized clinical trials. Control Clin Trials ...
Background Evidence supports the use of hand-based metacarpophalangeal joint (MCPJ) blocking splints as an intervention for trigger finger (TF). In practice, finger-based relative motion (RM) splints are also implemented without evidence. Purpose This randomized comparative trial (RCT) aims to evaluate implementation of MCPJ blocking and RM splints for effectiveness, function, occupational ...
Random Allocation*. Research Design*. Statistics as Topic. This article is intended as a practical guide to the various methods of patient assignment in clinical trials. Topics discussed include a critical appraisal of non-randomized studies, methods of restricted randomization such as random permuted blocks and the biased coin technique, the ...
Background Repetitive transcranial magnetic stimulation (rTMS) is one of the non-invasive brain stimulations that modulate cortical excitability through magnetic pulses. However, the effects of rTMS on Parkinson's disease (PD) have yielded mixed results, influenced by factors including various rTMS stimulation parameters as well as the clinical characteristics of patients with PD. There is ...
The efficiency of stratifying on a risk factor before randomization as opposed to complete randomization and adjustment for the risk factor by post-randomization analysis is examined by calculating the variance of the estimated difference between two treatment means for the two design strategies. This analysis shows that in small samples ...