Grab your spot at the free arXiv Accessibility Forum
Help | Advanced Search
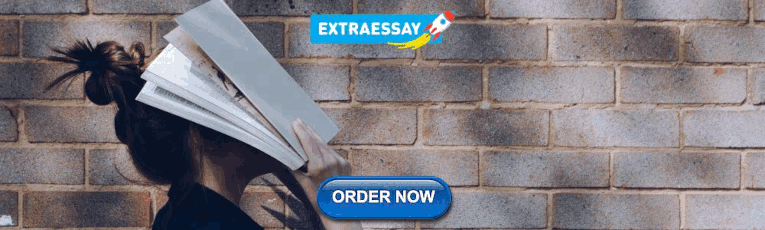
Computer Science > Computation and Language
Title: information redundancy and biases in public document information extraction benchmarks.
Abstract: Advances in the Visually-rich Document Understanding (VrDU) field and particularly the Key-Information Extraction (KIE) task are marked with the emergence of efficient Transformer-based approaches such as the LayoutLM models. Despite the good performance of KIE models when fine-tuned on public benchmarks, they still struggle to generalize on complex real-life use-cases lacking sufficient document annotations. Our research highlighted that KIE standard benchmarks such as SROIE and FUNSD contain significant similarity between training and testing documents and can be adjusted to better evaluate the generalization of models. In this work, we designed experiments to quantify the information redundancy in public benchmarks, revealing a 75% template replication in SROIE official test set and 16% in FUNSD. We also proposed resampling strategies to provide benchmarks more representative of the generalization ability of models. We showed that models not suited for document analysis struggle on the adjusted splits dropping on average 10,5% F1 score on SROIE and 3.5% on FUNSD compared to multi-modal models dropping only 7,5% F1 on SROIE and 0.5% F1 on FUNSD.
Comments: | 15 pages, ICDAR 2023 (17th International Conference on Document Analysis and Recognition) |
Subjects: | Computation and Language (cs.CL); Artificial Intelligence (cs.AI) |
Cite as: | [cs.CL] |
(or [cs.CL] for this version) | |
Focus to learn more arXiv-issued DOI via DataCite |
Submission history
Access paper:.
- Other Formats

References & Citations
- Google Scholar
- Semantic Scholar
BibTeX formatted citation

Bibliographic and Citation Tools
Code, data and media associated with this article, recommenders and search tools.
- Institution
arXivLabs: experimental projects with community collaborators
arXivLabs is a framework that allows collaborators to develop and share new arXiv features directly on our website.
Both individuals and organizations that work with arXivLabs have embraced and accepted our values of openness, community, excellence, and user data privacy. arXiv is committed to these values and only works with partners that adhere to them.
Have an idea for a project that will add value for arXiv's community? Learn more about arXivLabs .
information redundancy Recently Published Documents
Total documents.
- Latest Documents
- Most Cited Documents
- Contributed Authors
- Related Sources
- Related Keywords
Information redundancy across spatial scales modulates early visual cortical processing
Graph transformer for drug response prediction.
Previous models have shown that learning drug features from their graph representation is more efficient than learning from their strings or numeric representations. Furthermore, integrating multi-omics data of cell lines increases the performance of drug response prediction. However, these models showed drawbacks in extracting drug features from graph representation and incorporating redundancy information from multi-omics data. This paper proposes a deep learning model, GraTransDRP, to better drug representation and reduce information redundancy. First, the Graph transformer was utilized to extract the drug representation more efficiently. Next, Convolutional neural networks were used to learn the mutation, meth, and transcriptomics features. However, the dimension of transcriptomics features is up to 17737. Therefore, KernelPCA was applied to transcriptomics features to reduce the dimension and transform them into a dense presentation before putting them through the CNN model. Finally, drug and omics features were combined to predict a response value by a fully connected network. Experimental results show that our model outperforms some state-of-the-art methods, including GraphDRP, GraOmicDRP.
TF-IDF Method and Vector Space Model Regarding the Covid-19 Vaccine on Online News
Advances in information and technology have caused the use of the internet to be a concern of the general public. Online news sites are one of the technologies that have developed as a means of disseminating the latest information in the world. When viewed in terms of numbers, newsreaders are very sufficient to get the desired information. However, with this, the amount of information collected will result in an explosion of information and the possibility of information redundancy. The search system is one of the solutions which expected to help in finding the desired or relevant information by the input query. The methods commonly used in this case are TF-IDF and VSM (Vector Space Model) which are used in weighting to measure statistics from a collection of documents on the search for some information about the Covid 19 vaccine on kompas.com news then tokenizing it to separate the text, stopword removal or filtering to remove unnecessary words which usually consist of conjunctions and others. The next step is sentence stemming which aims to eliminate word inflection to its basic form. Then the TF-IDF and VSM calculations were carried out and the final result are news documents 3 (DOC 3) with a weight of 5.914226424; news documents 2 (DOC 2) with a weight of 1.767692186; news documents 5 (DOC 5) with weights 1.550165096; news document 4 (DOC 4) with a weight of 1.17141223;, and the last is news document 1 (DOC 1) with a weight of 0.5244103739.
Multi-Scale Guided Attention Network for Crowd Counting
The CNN-based crowd counting method uses image pyramid and dense connection to fuse features to solve the problems of multiscale and information loss. However, these operations lead to information redundancy and confusion between crowd and background information. In this paper, we propose a multi-scale guided attention network (MGANet) to solve the above problems. Specifically, the multilayer features of the network are fused by a top-down approach to obtain multiscale information and context information. The attention mechanism is used to guide the acquired features of each layer in space and channel so that the network pays more attention to the crowd in the image, ignores irrelevant information, and further integrates to obtain the final high-quality density map. Besides, we propose a counting loss function combining SSIM Loss, MAE Loss, and MSE Loss to achieve effective network convergence. We experiment on four major datasets and obtain good results. The effectiveness of the network modules is proved by the corresponding ablation experiments. The source code is available at https://github.com/lpfworld/MGANet.
Predicting reposting latency of news content in social media: A focus on issue attention, temporal usage pattern, and information redundancy
Information redundancy across spatial scales modulates early visual cortex responses, optimising aircraft taxi speed: design and evaluation of new means to present information on a head-up display.
Abstract The objective of this study was to design and evaluate new means of complying to time constraints by presenting aircraft target taxi speeds on a head-up display (HUD). Four different HUD presentations were iteratively developed from paper sketches into digital prototypes. Each HUD presentation reflected different levels of information presentation. A subsequent evaluation included 32 pilots, with varying flight experience, in usability tests. The participants subjectively assessed which information was most useful to comply with time constraints. The assessment was based on six themes including information, workload, situational awareness, stress, support and usability. The evaluation consisted of computer-simulated taxi-runs, self-assessments and statistical analysis. Information provided by a graphical vertical tape descriptive/predictive HUD presentation, including alpha-numerical information redundancy, was rated most useful. Differences between novice and expert pilots can be resolved by incorporating combinations of graphics and alpha-numeric presentations. The findings can be applied for further studies of combining navigational and time-keeping HUD support during taxi.
Information Redundancy Neglect versus Overconfidence: A Social Learning Experiment
We study social learning in a continuous action space experiment. Subjects, acting in sequence, state their beliefs about the value of a good after observing their predecessors’ statements and a private signal. We compare the behavior in the laboratory with the Perfect Bayesian Equilibrium prediction and the predictions of bounded rationality models of decision-making: the redundancy of information neglect model and the overconfidence model. The results of our experiment are in line with the predictions of the overconfidence model and at odds with the others’. (JEL C91, D12, D82, D83)
Visual images contain redundant information across spatial scales where low spatial frequency contrast is informative towards the location and likely content of high spatial frequency detail. Previous research suggests that the visual system makes use of those redundancies to facilitate efficient processing. In this framework, a fast, initial analysis of low-spatial frequency (LSF) information guides the slower and later processing of high spatial frequency (HSF) detail. Here, we used multivariate classification as well as time-frequency analysis of MEG responses to the viewing of intact and phase scrambled images of human faces to demonstrate that the availability of redundant LSF information, as found in broadband intact images, correlates with a reduction in HSF representational dominance in both early and higher-level visual areas as well as a reduction of gamma-band power in early visual cortex. Our results indicate that the cross spatial frequency information redundancy that can be found in all natural images might be a driving factor in the efficient integration of fine image details.
THE DATA DIAGNOSTIC METHOD OF IN THE SYSTEM OF RESIDUE CLASSES
The subject of the article is the development of a method for diagnosing data that are presented in the system of residual classes (SRC). The purpose of the article is to develop a method for fast diagnostics of data in the SRC when entering the minimum information redundancy. Tasks: to analyze and identify possible shortcomings of existing methods for diagnosing data in the SRC, to explore possible ways to eliminate the identified shortcomings, to develop a method for prompt diagnosis of data in SRC. Research methods: methods of analysis and synthesis of computer systems, number theory, coding theory in SRC. The following results were obtained. It is shown that the main disadvantage of the existing methods is the significant time of data diagnostics when it is necessary to introduce significant information redundancy into the non-positional code structure (NCS). The method considered in the article makes it possible to increase the efficiency of the diagnostic procedure when introducing minimal information redundancy into the NCS. The data diagnostics time, in comparison with the known methods, is reduced primarily due to the elimination of the procedure for converting numbers from the NCS to the positional code, as well as the elimination of the positional operation of comparing numbers. Secondly, the data diagnostics time is reduced by reducing the number of SRC bases in which errors can occur. Third, the data diagnostics time is reduced due to the presentation of the set of values of the alternative set of numbers in a tabular form and the possibility of sampling them in one machine cycle. The amount of additionally introduced information redundancy is reduced due to the effective use of the internal information redundancy that exists in the SRC. An example of using the proposed method for diagnosing data in SRC is given. Conclusions. Thus, the proposed method makes it possible to reduce the time for diagnosing data errors that are presented in the SRC, which increases the efficiency of diagnostics with the introduction of minimal information redundancy.
Export Citation Format
Share document.
- Search Menu
- Sign in through your institution
- Author Guidelines
- Submission Site
- Self-Archiving Policy
- Why Submit?
- About Journal of Computer-Mediated Communication
- About International Communication Association
- Editorial Board
- Advertising & Corporate Services
- Journals Career Network
- Journals on Oxford Academic
- Books on Oxford Academic
Article Contents
Conclusion and discussion, about the authors.
- < Previous
Information Overload, Similarity, and Redundancy: Unsubscribing Information Sources on Twitter

- Article contents
- Figures & tables
- Supplementary Data
Hai Liang, King-wa Fu, Information Overload, Similarity, and Redundancy: Unsubscribing Information Sources on Twitter, Journal of Computer-Mediated Communication , Volume 22, Issue 1, 1 January 2017, Pages 1–17, https://doi.org/10.1111/jcc4.12178
- Permissions Icon Permissions
The emergence of social media has changed individuals' information consumption patterns. The purpose of this study is to explore the role of information overload, similarity, and redundancy in unsubscribing information sources from users' information repertoires. In doing so, we randomly selected nearly 7,500 ego networks on Twitter and tracked their activities in 2 waves. A multilevel logistic regression model was deployed to test our hypotheses. Results revealed that individuals (egos) obtain information by following a group of stable users (alters). An ego's likelihood of unfollowing alters is negatively associated with their information similarity, but is positively associated with both information overload and redundancy. Furthermore, relational factors can modify the impact of information redundancy on unfollowing.
Social media have changed the current media environment and can consequently influence individuals' selection of information sources. First, social media offer users a large number of information choices. All social media users can provide some kinds of content, and thus could be considered as information sources. However, the available human attention to consume information is always limited ( Webster, 2010 ), leading to varying degrees of information overload on social media ( Holton & Chyi, 2012 ). To cope with information overload, users will rely upon relatively small subsets or “repertoires” of their preferred channels (e.g., Kim, 2014 ; Taneja, Webster, Malthouse, & Ksiazek, 2012 ; Yuan, 2011 ).
Second, social media users are usually embedded in online social networks. They select information sources by “following” other users. Those users being followed are called followees. In social networks, users are inclined to follow other users who appear to be similar to themselves with respect to many attributes ( McPherson, Smith-Lovin, & Cook, 2001 ). Given the increasing number of available choices on social media, this homophilous selectivity is reinforced in computer-mediated communication and potentially results in audience fragmentation ( Sunstein, 2009 ). If users follow many content-similar followees, they are likely to receive many duplicated messages in their personal information streams, and thus lead to information redundancy ( Harrigan, Achananuparp, & Lim, 2012 ).
Although the repertoire approach to media use provides an important framework to capture information consumption patterns under information overload ( Kim, 2014 ; Taneja et al., 2012 ; Yuan, 2011 ), few studies have examined the dynamic process of repertoire formation and the role of content similarity and redundancy. In a networked environment on social media, information overload could increase the tendency of similarity-based selection and further leads to information redundancy, which in turn might increase information overload. Besides, little attention has been paid to the removal of information sources from personal repertoires. The deselection process helps reduce information overload ( Webster, 2010 ) and stabilize personal information repertoires ( Kwak, Chun, & Moon, 2011 ). In order to investigate the role of information overload, similarity, and redundancy in structuring information consumption patterns, this study extends the repertoire approach by incorporating media choice theories and social network analysis.
Followees as Information Repertoire on Social Media
Contemporary social media are usually conceived as a combination of information platforms and social network services, wherein “ordinary” users (as well as media organizations, journalists, and other “elite” users) create, share, and consume user-generated content (as well as professional news content) in social networks (e.g., Kwak, Lee, Park, & Moon, 2010 ; Murthy, 2012 ). This definition captures two essential aspects of the character of current social media platforms. First, ordinary people have turned themselves into media content providers on social media by producing and sharing news messages ( Murthy, 2012 ). Second, people's reception of information is basically constrained by their personal social networks online. Social media users decide whose messages they wish to receive by following other users. By creating such following connections, users receive the content that their followees post. Thus, as users choose whom to follow, they also choose the information to which they will have access.
Twitter is one of the most popular social media platforms. Figure 1 illustrates the information consumption structure on Twitter. An individual (e.g., Ego 1) can subscribe to receive the tweets of another user (e.g., Alter 2). We say Alter 2 is a followee of Ego 1 while Ego 1 is a follower of Alter 2. On Twitter, information seeking takes the form of following relationships ( Himelboim, Hansen, & Bowser, 2013 ). Egos receive messages from followees but do not receive them from followers. In Figure 1 , Ego 1 is following Alters 1–3. Therefore, Ego 1 receives all tweets posted by these alters. The following relationships on Twitter may not be reciprocal. Users may rebroadcast a tweet by retweeting the message to their followers. They can also converse with any other users by replying to their tweets or mentioning other users using the “@” sign. All original tweets, retweets, and replies are displayed in users' timelines.

An ego network approach to conceiving followees as information repertoires. An ego network consists of a focal node (“ego”) and the nodes to whom ego is directly connected to (alters) plus the ties (arrows in the figure) among the alters. The arrow pointing from ego 1 to alter 2 indicates that ego 1 follows alter 2. It also suggests information flows from alter 2 to ego 1.
The emerging characteristics of social media have several implications for existing theories about the choice of information sources. First, all social media users, including ordinary people, journalists, and media organizations, can be their followers' information sources. Hermida, Fletcher, Korell, & Logan ( 2012 ) found that social media users are more likely to receive information from the individuals than from news organizations and journalists followed on social media. Even for media content, Twitter users get roughly half of their media referrals via intermediaries ( Wu, Hofman, Mason, & Watts, 2011 ). In this sense, the selection of information sources partly becomes the choice of followees on social media. Therefore, alters, followees, and information sources are interchangeable terms in this study.
Second, users' followees serve as their information repertoires to help cope with information overload on social media. The conventional notion about media choice is that people assess the available resources and choose among them in an effort to achieve their purposes rationally. However, that rationality is bounded by the overabundance of choice and the limited human attention available on social media. It is impossible to follow all available information sources or select relevant alters by examining every single message posted by these alters. One technique many people use to manage their choices is to limit the number of choices by paring down their options to a more manageable repertoire of preferred sources ( Webster, 2010 , 2014 ). On Twitter, that usually means following a small number of followees/alters.
Third, repertoire is a subset of available media that an individual uses regularly or frequently (e.g., Webster, 2014 ; Webster & Ksiazek, 2012 ), which actually reflects the habitual nature of media consumption. However, most studies analyze media repertoires in a static way. Building information repertoires on social media by following other users is a dynamic process. On Twitter, users can take two steps to establish and maintain their followees. When joining Twitter, users may subscribe to other users. Later on, users are free to unsubscribe and remove users from their following lists (i.e., unfollow). Kwak et al. ( 2011 ) have documented a relationship stabilization process on Twitter—users are less likely to unfollow those whom the users have been following for a long time. Finally, this process will result in stable repertories of information sources, which users rely on heavily for daily information consumption. It suggests that researchers should pay more attention to the unfollowing process in the dynamic analysis of repertoire formation.
Finally, previous studies focus on explaining the absolute size of repertoires, but often say little about their composition (see Webster & Ksiazek, 2012 ). An information repertoire is introduced to cope with information overload by selecting preferred sources. By taking the composition into consideration, similarity-based selection may create redundant messages in personal repertoires ( Himelboim et al., 2013 ) and exacerbate information overload ( Franz, 1999 ). It remains largely unknown to what extent users unfollow their information sources based on individual preference of certain content category, information overload, and information redundancy.
Building Repertoires: Informational Factors
The above discussion suggests three intertwined variables that are relevant to the formation of user repertoires on social media platforms. Unlike previous repertoire studies that focus on the structure of media use, the variables are related to the communication content. First, the primary purpose of building information repertoires is to cope with information overload. Information overload has traditionally been conceived as a subjective experience in which users are overwhelmed by a large supply of information in a given period of time (e.g., Savolainen, 2007 ). Although many reasons can cause information overload, the core component is the volume of incoming information ( Franz, 1999 ). The growing number of information sources could have negative consequences for information seeking. Holton and Chyi ( 2012 ) found that 72.8% of respondents felt at least somewhat overloaded with the amount of news available today. In addition, the study reports that perceived overload depends on platforms: Use of Facebook is positively associated with information overload, whereas the use of Twitter is not significantly correlated with information overload. To cope with information overload, media users usually maintain a repertoire by limiting the number of sources. For instance, TV studies have demonstrated that people watch a small fraction of available channels in the US and across the world (see Webster, 2014 ).
H1: Users are more likely to unfollow the alters who post more messages during a given period of time.
Second, building information repertoires is closely related to the choice of specific information sources. Previous studies have used content preferences to explain cross-platform media repertoires (see Taneja et al., 2012 ). They found that information sources providing similar content are likely to be used together. For example, researchers have evidenced a news repertoire that combined Internet and television news (e.g., Dutta-Bergman, 2004 ; Yuan, 2011 ). This findings are consistent with the theory of media complementarity: Individuals who are interested in a particular content type expose themselves to various information channels that correspond with their area of interest ( Dutta-Bergman, 2004 ).
Although the original purpose was to explain cross-platform media consumption, Himelboim et al. ( 2013 ) have extended the theory of channel complementarity by considering the complementary selection of information sources that occur within a single social media platform. They found that Twitter users in different clusters follow different sets of sources that cover different areas of content (i.e., local versus national news). In a similar way, if users show consistent interest in posting a type of tweet, they are more likely to follow those users posting messages on a similar topic ( Weng, Lim, Jiang, & He, 2010 ).
H2: Users are less likely to unfollow the alters who post similar hashtags.
H3: The positive association between unfollowing and tweeting frequency is weaker for the alters who post similar hashtags to their egos.
Third, selecting information sources based on information similarity in a networked communication environment can lead to information redundancy in one's personal repertoire. If users select the sources posting similar topics (using similar hashtags) consistently, they will be expected to receive lots of redundant messages. Information redundancy refers to message repetition in a series of received messages ( Stephens, Barrett, & Mahometa, 2013 ). It does not mean posting repeated messages by a single alter user. As illustrated in Figure 1 , information redundancy quantifies the extent to which an alter posted a type of content (i.e., hashtag) similar to other alters in an ego network, while information similarity is related to the similarity of hashtags between egos and alters. Even though there are no duplicated tweets within an alter's timeline, the tweets by alters could be totally redundant to their egos, because other alters may post similar tweets in the ego network.
The role of information redundancy in media choice has been only implicitly mentioned. For example, previous studies suggest that use of one medium will displace the use of functionally alternative media, because the time available in any day is fixed (e.g., Ferguson & Perse, 2000 ). It implies that people are less likely to choose media with redundant information relative to their current media repertoires under information overload conditions. Another relevant argument is based on the theory of media complementarity. It predicts that people who select one type of information from one channel will also select the same type of information from other channels ( Dutta-Bergman, 2004 ; Himelboim et al., 2013 ). Eventually, that will cause duplicated messages received from different media platforms ( Jenkins, 2006 ). It implies that redundancy might be acceptable if people are interested in only a few types of content. For example, users who are interested in pop music may follow many pop stars on social media. However, empirical studies found that people are actually interested in different types of content and are likely to possess a complementary architecture in their information repertoires (e.g., Chaffee, 1982 ; Webster, 2014 ; Yuan, 2011 ). That means if the users are interested in both pop music and sports, they may unfollow a few pop stars and then follow some sports stars to avoid information overload, even though the unfollowed pop stars were posting unique tweets concerning their own stories.
H4: Users are more likely to unfollow those alters who post more redundant hashtags relative to what the users receive from all other alters.
H5: The positive association between information redundancy and unfollowing is stronger for the alters who post more messages during a given period of time.
H6: The negative association between hashtag similarity and unfollowing is stronger for the alters who post less redundant hashtags.
The Role of Relational Factors
In addition to these informational factors, relational factors could be another set of factors structuring the dynamic process of repertoire building on social media. Relationship building (e.g., maintaining friendship online) and information seeking are the two major motivations of Twitter use ( Kwak et al., 2010 ; Myers, Sharma, Gupta, & Lin, 2014 ; Wu et al., 2011 ). Information consumption on social media could be a byproduct of social networking behaviors. Many ordinary users socialize with their friends, family, and coworkers on Twitter. They establish ties for relational purposes, while the ties also serve as the conduits of information flow ( Golder & Yardi, 2010 ; Myers & Leskovec, 2014 ). The network structure can influence what information users will receive. For example, users in densely connected communities are expected to receive more redundant information ( Harrigan et al., 2012 ). That suggests that the relational factors might modify the relationships between the informational factors and unfollowing.
RQ: How will the relational factors influence the impacts of informational factors on unfollowing?
Data Collection
By using Twitter's REST APIs, we collected a two-wave panel dataset. To overcome the representativeness problem, this study sampled the panel users randomly from the population. First, we employed a method reported in Liang and Fu ( 2015 ) to generate random Twitter user IDs. We generated 90,000 random numbers. And then, we searched these numbers via the official API to check the existence of these Twitter IDs. Using this method, we obtained 34,006 valid Twitter user accounts (egos).
Second, we obtained the egos' user profiles, their followees' IDs, and up to 3,200 tweets and retweets (timeline) for each ego user. We collected the first wave of data in December 2014 and the second wave in March 2015. In the second wave, 33,774 egos still exist. Due to the privacy settings on Twitter, we could only get the tweets from the public accounts. In addition, since we are only interested in the unfollowing behavior, we exclude those users who are totally inactive during the period of data collection. Finally, we got 7,609 ego users who are both active and publicly available.
Third, we constructed ego networks in which nodes are users and ties are the following relationships between egos and followees. In the first wave, there are 1,314,156 nodes (including 7,360 ego users) and 1,766,269 ties in the ego networks. In the second wave, there are 1,403,291 nodes (including 7,464 ego users) and 1,888,039 ties in the ego networks. We further collected the followees' profiles and tweets, and their followees. We excluded the followees whose tweets and following relationships are kept private. The final dataset for our analyses include 7,449 ego networks with 1,180,903 nodes and 1,658,069 ties by combining the two waves.
Unfollowing was measured by comparing the ego networks between Wave 1 and Wave 2. If a followee in Wave 1 has not been observed in the followee list of Wave 2, we consider it was unfollowed during the two waves. Among the 1,658,069 ties, 2.89% (47,962) were removed during the two waves. Among the 7,449 egos, 38.44% (2,864) have deleted at least one followee. We note that most independent variables were extracted from the first wave only. Therefore, we only included the users (egos and followees) who appeared in Wave 1 (7,326 egos and 1,613,735 ties) for formal analyses.
In order to measure information similarity and information redundancy, we employed text mining techniques. First, we created a term-document matrix for each ego and its followees (i.e., 7,326 term-document matrices in total). In each term-document matrix, rows are the users (including an ego and their alters) and columns are the unique hashtags in the users' tweets (all available tweets). Given that our sample consists of active users, about 90% of the alters have posted at least one hashtag. Second, the hashtags used by user u were encoded into a feature vector of term frequency–inverse document frequency (tf-idf) ϕ( u ). The i th element ϕ i ( u ) represents the frequency of the hashtag indexed by i in all the hashtags used by u , scaled by the inverse document frequency (see Salton, Wong, & Yang, 1975 ). The tf-idf value increases proportionally to the number of times a word appears in the document, but is offset by the frequency of the word in the corpus.
Hashtag similarity was measured at the dyadic level by the semantic similarity between the hashtags used by the egos and those used by their alters. As a result, the score quantifying the similarity of information posted by two users u 1 and u 2 is given by a cosine similarity measure: ⟨ϕ i ( u 1 ), ϕ i ( u 2 )⟩/(||ϕ( u 1 )|| · ||ϕ( u 2 )||). Theoretically, hashtag similarity ranges from 0 (completely dissimilar) to 1 (actually the same). The mean of the hashtag similarity score in our data is 0.009 ( SD = 0.047). Since the hashtag similarity was calculated using the semantic distance based on the tf-idf values, if two hashtags are referring the same topic (e.g., #politics and #Obama), users are inclined to use them together, and thus the semantic similarity score between them will be high.
Hashtag redundancy was also measured based on the tf-idf values. Words are not equally important in terms of their uniqueness. In fact, some words have little or no discriminating power. For example, one's followees are all university researchers. It is likely that all followees may include “research” as a hashtag. This word is purely redundant. TF-IDF is a numerical statistic that is intended to reflect how important a word is to a document in a collection or corpus ( Robertson, 2004 ). It quantifies the word importance relative to all words used by alters in an ego network. If a followee has many hashtags with high tf-idf values, it means that the user included many unique hashtags and showed less redundancy relative to other alters in the same ego network. Therefore, we calculated the sum of all tf-idf values for each followee as an indicator of information uniqueness ( IU ). And then we subtract the value using the maximum value to measure information redundancy ( IR = min ( IR ))/(max( IR ) − min( IR )). We further normalized the raw score to range from 0 to 1 by using the formula: ( IR = min ( IR ))/(max( IR ) − min( IR )). As a result, the mean of information redundancy is in our data 0.368 ( SD = 0.283).
Information overload was measured at the alter level by calculating the tweeting frequency—the number of messages posted by an alter during the two waves. On average, a followee posted 984 ( Mdn = 82, SD = 5,609) messages. Although the information overload is a subjective feeling, we measured it in a more objective way. In order to control this individual (subjective) heterogeneity, we modeled its effect in a multilevel framework with other control variables.
Popularity of a followee was measured by the ratio of the number of followers to the number of followees at Wave 1. The numbers are directly provided by Twitter's profile API. The average of popularity is 19,880 ( Mdn = 2, SD = 332,295). Similarly, we calculated the popularity score for each ego user ( M = 0.90, Mdn = 0.45, SD = 12.06).
Reciprocity was measured by a binary variable. If a followee of an ego was also a follower of the ego at Wave 1, we said the tie was reciprocal at Wave 1. We calculated the reciprocity only at Wave 1 and use it as a time-lag predictor of the unfollowing behavior in Wave 2. In our data, 36.8% of the ties are reciprocal.
To calculate the number of common followees between the ego and their alters at Wave 1, we collected the followees of the egos and the followees of the alters. We then compared the followee lists of the egos and the followee lists of the alters to determine the number of shared followees. In Figure 1 , Ego 2 and Alter 5 shared a common followee—Alter 4. On average, the egos and alters shared 62 ( Mdn = 12, SD = 208) followees in our dataset.
We included two types of control variables that have been investigated in previous studies ( Kivran-Swaine et al., 2011 ; Kwak et al., 2012 ; Xu et al., 2013 ). The ego specific predictors are the characteristics of ego users, whereas alter specific predictors are the characteristics of the followees. Ego specific variables include ego popularity, the number of tweets, and years since registration, all of which are directly provided by Twitter's profile API. Alter specific variables include the number of tweets, year since registration, hashtag rate (the proportion of the tweets containing at least a hashtag), and interaction frequency. Interaction frequency was measured by the sum of the frequency of the ego retweeting its followees' tweets, the frequency of the ego replying to its followees, and the frequency of mentioning.
Finally, we include the order of follow as an alter-specific control variable. Twitter does not offer information about the establishment time of each relationship. However, it does provide the temporal order of the establishment of relationships in the personal network ( Kwak et al., 2011 ). Similarly, we constructed the relative order in relationship establishment of followees for each ego user by breaking the followees into 10 groups. The final score ranges from 10% (the most recent 10% of followees) to 100% (the oldest 10% of followees).
Data Analysis
We used multilevel logistic regression ( Snijders & Bosker, 2012 ) to test our hypotheses. The multilevel framework has been successfully employed to model (ego-centric) network formation problems (e.g., Golder & Yardi, 2010 ; Kivran-Swaine et al., 2011 ). In our study, the unit of analysis is the tie between egos and alters. Each following relationship nested under the same ego user could be influenced by the unique characteristics of that particular ego. We choose logistic as the link function because our dependent variables are binary responses (i.e., unfollowing or not). All alter-specific measures are Level-1 variables. All ego-level predictors are Level-2 variables.
Repertoire Stabilization
Table 1 presents the formal models to predict the unfollowing behavior on Twitter. We calculated two types of R 2 for multilevel models ( Nakagawa & Schielzeth, 2013 ): Marginal R 2 is concerned with variance explained by fixed factors, and conditional R 2 is concerned with variance explained by both fixed and random factors. The full model (the second column in Table 1 ) can explain 69.7% of the variance. We should note that much of the variance comes from the ego level. The Intraclass Correlation Coefficient for a null model without any predictors is 95.5%. In our data, nearly two-thirds of users didn't unfollow any users during our observations. The marginal R 2 of the full model is 6.1%
Multilevel Logistic Regression Models Predicting Unfollowing
. | Model 1 . | Full Model . | Raw Text Measures . | |||
---|---|---|---|---|---|---|
. | ||||||
. | Estimate (SE) . | Z . | Estimate (SE) . | Z . | Estimate (SE) . | Z . |
Similarity | −2.312 (.313) | −7.39 | −1.700 (.339) | −5.02 | −2.349 (.604) | −3.89 |
Overload | 0.102 (.023) | 11.55 | 0.083 (.009) | 9.18 | 0.038 (.013) | 2.87 |
Redundancy | −0.019 (.002) | −0.80 | 0.120 (.024) | 5.00 | 0.071 (.031) | 2.26 |
Overload × Similarity | −0.353 (.141) | −2.50 | −0.458 (.139) | −3.28 | 0.201 (0.77) | 2.63 |
Overload × Redundancy | −0.068 (.018) | −3.82 | −0.032 (.018) | −1.79 | 0.049 (.020) | 2.47 |
Similarity × Redundancy | 1.489 (.502) | 2.97 | 0.953 (.562) | 1.70 | 1.868 (1.056) | 1.77 |
Alter popularity | −0.036 (.009) | −4.14 | −0.036 (.009) | −4.19 | ||
Reciprocity | −1.518 (.017) | −89.58 | −1.513 (.0170) | −89.43 | ||
Shared followees | −0.151 (.007) | −21.23 | −0.151 (.007) | −21.16 | ||
Order of follow (old) | −1.555 (.021) | −72.95 | −1.452 (.022) | −66.80 | −1.456 (.022) | −66.95 |
Interaction frequency | −0.259 (.0130) | −19.95 | −0.219 (.013) | −17.13 | −0.219 (.013) | −17.13 |
of tweets | 0.004 (.007) | 0.57 | 0.013 (.007) | 1.72 | 0.019 (.007) | 2.59 |
Year since registration | 0.005 (.004) | 1.26 | −0.060 (.004) | −13.48 | −0.062 (.004) | −14.13 |
Hashtag rate | 0.006 (.052) | 0.11 | 0.106 (.052) | 2.01 | 0.021 (.052) | 0.41 |
Ego popularity | 0.034 (.012) | 2.79 | −0.036 (.013) | −2.85 | 0.034 (.011) | 2.96 |
of tweets | 0.143 (.034) | 4.19 | 0.192 (.036) | 5.35 | 0.173 (.032) | 5.41 |
Year since registration | −0.031 (.026) | −1.20 | 0.003 (.027) | 0.10 | 0.004 (.026) | 0.15 |
Intercept | −5.357 (.096) | −55.83 | −5.119 (.101) | −50.69 | −4.746 (.093) | −50.82 |
8.138 (2.853) | 9.021 (3.003) | 7.177 (2.679) | ||||
−119,115.4 | −113,763.1 | −113,979.6 | ||||
67.8% | 69.7% | 65.2% | ||||
2.6% | 6.1% | 7.0% | ||||
1,613,735 | ||||||
7,326 |
. | Model 1 . | Full Model . | Raw Text Measures . | |||
---|---|---|---|---|---|---|
. | ||||||
. | Estimate (SE) . | Z . | Estimate (SE) . | Z . | Estimate (SE) . | Z . |
Similarity | −2.312 (.313) | −7.39 | −1.700 (.339) | −5.02 | −2.349 (.604) | −3.89 |
Overload | 0.102 (.023) | 11.55 | 0.083 (.009) | 9.18 | 0.038 (.013) | 2.87 |
Redundancy | −0.019 (.002) | −0.80 | 0.120 (.024) | 5.00 | 0.071 (.031) | 2.26 |
Overload × Similarity | −0.353 (.141) | −2.50 | −0.458 (.139) | −3.28 | 0.201 (0.77) | 2.63 |
Overload × Redundancy | −0.068 (.018) | −3.82 | −0.032 (.018) | −1.79 | 0.049 (.020) | 2.47 |
Similarity × Redundancy | 1.489 (.502) | 2.97 | 0.953 (.562) | 1.70 | 1.868 (1.056) | 1.77 |
Alter popularity | −0.036 (.009) | −4.14 | −0.036 (.009) | −4.19 | ||
Reciprocity | −1.518 (.017) | −89.58 | −1.513 (.0170) | −89.43 | ||
Shared followees | −0.151 (.007) | −21.23 | −0.151 (.007) | −21.16 | ||
Order of follow (old) | −1.555 (.021) | −72.95 | −1.452 (.022) | −66.80 | −1.456 (.022) | −66.95 |
Interaction frequency | −0.259 (.0130) | −19.95 | −0.219 (.013) | −17.13 | −0.219 (.013) | −17.13 |
of tweets | 0.004 (.007) | 0.57 | 0.013 (.007) | 1.72 | 0.019 (.007) | 2.59 |
Year since registration | 0.005 (.004) | 1.26 | −0.060 (.004) | −13.48 | −0.062 (.004) | −14.13 |
Hashtag rate | 0.006 (.052) | 0.11 | 0.106 (.052) | 2.01 | 0.021 (.052) | 0.41 |
Ego popularity | 0.034 (.012) | 2.79 | −0.036 (.013) | −2.85 | 0.034 (.011) | 2.96 |
of tweets | 0.143 (.034) | 4.19 | 0.192 (.036) | 5.35 | 0.173 (.032) | 5.41 |
Year since registration | −0.031 (.026) | −1.20 | 0.003 (.027) | 0.10 | 0.004 (.026) | 0.15 |
Intercept | −5.357 (.096) | −55.83 | −5.119 (.101) | −50.69 | −4.746 (.093) | −50.82 |
8.138 (2.853) | 9.021 (3.003) | 7.177 (2.679) | ||||
−119,115.4 | −113,763.1 | −113,979.6 | ||||
67.8% | 69.7% | 65.2% | ||||
2.6% | 6.1% | 7.0% | ||||
1,613,735 | ||||||
7,326 |
p < .01,
Variables were rescaled using Z-scores ( M = 0, SD = 1) for multilevel analyses.
Variables that were measured at the ego level (i.e., level-2 variables).
All tie measures and alter-specific measures are Level-1 variables. All ego-level predictors are Level-2 variables.
The first two models were based on the hashtag measures, while the last model was based on the raw text measures of similarity and redundancy.
If we only focused on the users who had unfollowed at least once, the same model could explain 10.4% of the variance by the fixed factors.
The order of follow shows a significant effect on unfollowing, suggesting that people are less likely to unfollow the users who have connected for a relatively long time. In this way, users re-examine their recent followees and decide whether to keep them in their information repertoires. Finally, the repertoire becomes more and more stable. An alternative explanation is that the older followees might imply strong ties, thus are less likely to be unfollowed. Figure 2 shows that even we controlled for the interaction frequency and other variables, the negative relationship between the order of follow and unfollowing probability still holds. The result implies users are intentionally stabilizing their information repertoires over time.

The observed and estimated probabilities of unfollowing as a function of the order of follow. The estimated probability was calculated based on the full model in Table 1 .
Informational Predictors
The major concern of the current study is to examine the role of informational factors in building personal repertoires of information sources. H1 stated that people are more likely to unfollow when they receive overloaded information. The full model in Table 1 shows that information overload is significantly associated with unfollowing, which means that users are more likely to unfollow the followees who posted too many tweets during the two waves ( B = 0.071, SE = .006, p < .01). Therefore, H1 is supported.
H2 stated that users are less likely to unfollow the users sharing similar hashtags. The full model shows that hashtag similarity is negatively associated with unfollowing. That means users are more likely to keep the followees who tweeted similar hashtags ( B = −1.165, SE = .137, p < .01). Therefore, H2 is supported.
H3 stated that hashtag similarity moderates the impact of information overload on unfollowing other users. Table 1suggests that the interaction effect of hashtag similarity and information overload on unfollowing is statistically significant ( B = −0.458, SE = .139, p < .01). Figure 3 illustrates that the positive association between information overload and unfollowing is stronger when hashtag similarity between the ego and alter is low. From Figure 3 , we also note that the difference of unfollowing probability between high and low similarity increases exponentially as information overload increases, indicating that information overload reinforces similarity-based selection. Therefore, H3 is supported.

The interaction effect of information overload and similarity on unfollowing. The estimated probability was calculated based on the full model in Table 1 .
H4 stated that users are more likely to unfollow the users whose tweets included many redundant hashtags. In the full model, hashtag redundancy is positively associated with unfollowing in the full model ( B = 0.120, SE = .036, p < .01), suggesting that the increase of 1 hashtag redundancy will increase the probability of being unfollowed by 3%. Therefore, H4 is supported.
Concerning H5 and H6, the full model suggests that both interaction effects are not significant at all. It suggests that the redundancy effect is not conditional on information overload and similarity when relational factors are controlled for. Therefore, H5 and H6 are not supported.
Relational Predictors
According to the full model in Table 1 , all relational factors show significant impacts on unfollowing behavior. Consistent with previous studies, popularity is negatively associated with unfollowing ( B = −0.036, SE = .009, p < .01). Ego users are less likely to unfollow users with more followers. Reciprocity is also negatively correlated with unfollowing ( B = −1.518, SE = .017, p < .01). For followees with reciprocal ties with their egos at Wave 1, the probability of being unfollowed is 64% lower than the probability for the followees without reciprocal ties (82% versus 18%). Finally, the number of shared followees is negatively associated with unfollowing ( B = −0.151, SE = .017, p < .01), indicating that the followees who share more followees with the egos are less likely to be unfollowed by the egos.
To answer the RQ, Model 1 in Table 1 excluded all relational factors. The inconsistency between Model 1 and the full model is caused by the exclusion of relational variables. First, the redundancy effect is no longer significant in Model 1. It indicates that relational factors are suppressors. In our data, information redundancy is positively correlated with reciprocity and the number of shared followees. For reciprocal ties, the followees' information redundancy is 0.43 on average, whereas the average information redundancy is 0.33 for nonreciprocal ties, χ 2 (1, N = 1,613,733) = 50,676, p < .001. The Spearman's rank correlation between the number of followees and information redundancy is 0.17 ( p < .001). This means that high information redundancy implies dense connections (i.e., reciprocal and sharing more followees), which in turn decreases the unfollowing probability in Model 1. In addition, without considering the relational factors, the interaction effects with hashtag redundancy are significant in Model 1. The model suggests that users are less likely to unfollow the alters with redundant hashtags when information overload is high. It implies that users prefer information redundancy, which was produced by the relational factors.
This study conceptualized social media followees as information source repertoires and examined the dynamics of repertoire formation using panel data from Twitter. First, this study suggests that users maintain relatively stable information repertoires to cope with information overload. During the 3 months of observation, only 5.56% of the following ties have been changed. Despite that, our findings suggest that some users actively and continuously adjust their information source repertoires over time. It is consistent with previous research ( Kwak et al., 2011 ) that the new followees are most likely to be unfollowed, even when competing factors are controlled for. It implies that users are intentionally stabilizing their personal repertoires for daily information other than receiving it passively.
In our dataset, nearly two-thirds of users did not unfollow any users during our observations. This indicates that unfollowing actually is not a popular behavior on Twitter. However, it does not mean that unfollowing is a rare phenomenon or it lacks theoretical significance. We tracked the unfollowing behavior in a relatively short period of time. The number of users who have unfollowed other users should be much larger than 1/3. If we consider the frequency of unfollowing as an indicator for rational selection of information sources, the current study suggests that most users are not rational but habitual information consumers ( Wood, Quinn, & Kashy, 2002 ). Instead of browsing all information channels, users would like to check information from a few sources repeatedly.
Second, this study extended the repertoire approach by examining the role of information overload, similarity, and redundancy in structuring information consumption patterns on a single social media platform. We found that seeking information similarity and reducing information redundancy could coexist in the process of optimizing information repertoires. One popular argument states that users are increasingly seeking content similar sources on social media. This is one of the important coping strategies people have for finding preferred content in an increasingly complex media environment ( Webster & Ksiazek, 2012 ). Following this tendency, individuals would like to consume a steady diet of their preferred type of information sources. Finally, users with similar interests will cluster together ( Himelboim et al., 2013 ) and cause information redundancy.
The current study indeed found that Twitter users are more inclined to keep those followees sharing similar hashtags. Under the information overload situations, the tendency of selecting content similar alters is reinforced (see Figure 3 ). However, the average hashtag similarity between egos and followees is only weakly associated with the average redundancy among the followees ( r = 0.038, t = 3.27, df = 7,267, p < .01). The reason is that, as Table 1 suggests, people intentionally unfollowed the users with redundant information, even though they kept the similar alters at the same time. As a balance, their information repertoires contain the messages they are interested in and with very little redundancy. This also implies that people do have diverse interests and try to sample a diverse range of sources to build their information repertoires.
Third, we note that the formation process is significantly constrained by relational factors (i.e., popularity, reciprocity, and the number of common followees). In addition to their direct effects on unfollowing, the relational factors can alter the impacts of informational factors. We found that relational variables are suppressors of the redundancy effect. This implies that some users received unexpected and redundant information from their networked users. This relational constraint can also explain why previous research found that information overload is higher on Facebook than that on Twitter ( Holton & Chyi, 2012 ), because relational constraint on Twitter is expected to be lower (e.g., Marwick & boyd, 2011 ). In addition, we hypothesized that the informational effects are conditional on each other. However, our results suggest that the redundancy effect is not dependent on information overload and similarity when the relational factors are controlled for.
Furthermore, although we focused on the information variables in building information repertoires, it does not mean that alternative explanations are impossible. On the contrary, our study is consistent with previous repertoire studies that the structural factors are more important that other factors (see Webster, 2014 ). The structural factors in the present study include the relational variables that characterize the online social networks and the control variables. For example, the low ratio of the number of followers to the number of followees (popularity) indicates that the users are inclined to keep more information sources. This finding is consistent with the idea of audience availability in television program choice ( Webster & Wakshlag, 1983 ). Following many sources may suggest the users' availability in viewing new messages. However, these variables are at the microlevel or mesolevel in general. Future studies can explore the impacts of more macrolevel variables on the unfollowing behavior. As suggested by Webster ( 2014 ), the aggregate network level analysis would be beneficial to understand the bounded rationality of online user behaviors.
Limitations and Future Research
Several limitations can be associated with this study. First, when considering followees as information repertoire, we assume that users actually only read the messages posted by their followees. This assumption might not be accurate. Users can simply ignore the messages that they are not interested in to reduce information overload ( Savolainen, 2007 ). In addition, users can receive messages beyond their immediate following networks. Social networks are not the only mechanisms through which users are directed to media. The recommender system and search engine are commonly used for direct audience attention on social media platforms ( Webster, 2010 ). However, the following relationships do indicate awareness of the presence of the followees ( Himelboim et al., 2013 ). Future studies can track users' browsing history on social websites to examine patterns of consuming specific messages other than sources.
Second, Twitter provides researchers with the unique opportunity to track patterns of individual selection of information sources. Although, the unobtrusive approach provides more objective measures, it lacks information on both demographic and psychological variables. Previous studies have found that demographic variables, such as gender and age, show significant impact on the composition of media repertoires (e.g., Yuan, 2011 ). In addition, users with different psychological characteristics may prefer different information-seeking approaches ( Stefanone, Hurley, & Yang, 2013 ). Future studies need to further control these variables and examine the interaction effects between the self-reported and objective measures employed to build information repertoires.
In addition, the unobtrusive approach can cause potential measurement errors. For example, using tweeting frequency to measure information overload might be problematic. Even receiving the same amount of messages, some users may perceive more overload than would other users. We could not measure this subjective feeling directly. Instead, we employed the multilevel framework to control this individual heterogeneity carefully. First, the impact of tweeting frequency on the probability of being unfollowed by egos was considered separately for each ego. Furthermore, we included the potential compounding variables to control the individual differences. For example, Table 1 suggests that egos with more followees actually are less likely to unfollow other users, indicating that those users may have a higher threshold of information overload.
We measured information similarity and redundancy based on hashtags. This kind of operationalization was based on the repertoire approach to studying the user-defined channel types. Although the hashtag provides a convenient way to measure the content topics, 10% of followees did not post any hashtags in our sample. In the current study we considered them as “no preference” cases, i.e., the similarity and redundancy scores are zero. Another way to measure information similarity and redundancy is to calculate the variables based on the raw text. However, we think they are conceptually different things. The purpose of the current study is to demonstrate that the user's choice of information sources is based on content topics other than using similar or unique words. As a robustness check, we conducted a post hoc analysis based on the raw text measures (see the last column in Table 1 : Raw Text Measures). We found that the main effects are similar, whereas the interaction effects are slightly different. Future studies should use more advanced techniques to detect user-defined categories, such as the topic modeling approach, which is similar to factor analysis in media repertoire studies (see Weng et al., 2010 ).
Finally, social media platforms emphasize different technological characteristics. Our results rely on Twitter, which puts a greater emphasis on news sharing. For other social media platforms, like Facebook, studies may emphasize social networking. In this sense, users might be less susceptible to the information variables than was the case in our study. Furthermore, previous repertoire studies have demonstrated that people could build their personal repertoires across media platforms or rely on one of them. The choice of different repertoires is associated with user background characteristics (e.g., Kim, 2014 ). For studies based on a single platform, it is difficult to capture more general media use patterns. For example, watching TV news intensively may cause information overload or redundancy on Twitter. Therefore, future studies are encouraged to test our hypotheses across different social media platforms.
This study was supported by the Public Policy Research Funding Scheme of the Central Policy Unit of the Government of the Hong Kong Special Administrative Region (2013.A8.009.14A) and the Small Project Funding from The University of Hong Kong (201409176011).
Barabasi , A. L. , & Albert , R. ( 1999 ). Emergence of scaling in random networks . Science , 286 ( 5439 ), 509 – 512 . doi: 10.1126/science.286.5439.509
Google Scholar
Chaffee , S. H. ( 1982 ). Mass media and interpersonal channels: Competitive, convergent, or complementary . In G. Gumpert & R. Cathcart (Eds.), Inter/media: Interpersonal communication in a media world (3rd ed., pp. 62 – 80 ). New York, NY : Oxford University Press .
Google Preview
Dutta-Bergman , M. J. ( 2004 ). Complementarity in consumption of news types across traditional and new media . Journal of Broadcasting & Electronic Media , 48 ( 1 ), 41 – 60 . doi: 10.1207/s15506878jobem4801_3
Farhoomand , A. F. , & Drury , D. H. ( 2002 ). Managerial information overload . Communications of the ACM , 45 ( 10 ), 127 – 131 . doi: 10.1145/570907.570909
Ferguson , D. A. , & Perse , E. M. ( 2000 ). The World Wide Web as a functional alternative to television . Journal of Broadcasting & Electronic Media , 44 ( 2 ), 155 – 174 . doi: 10.1207/s15506878jobem4402_1
Franz , H. ( 1999 ). The impact of computer mediated communication on information overload in distributed teams . Proceedings of the 32nd Annual Hawaii International Conference on System Sciences, 1999, HICSS-32, 6182030 . doi: 10.1109/HICSS.1999.772712
Golder , S. A. , & Yardi , S. ( 2010 ). Structural predictors of tie formation in twitter: Transitivity and mutuality . Paper presented at the 2010 IEEE Second International Conference on Social Computing (SocialCom).
Harrigan , N. , Achananuparp , P. , & Lim , E. P. ( 2012 ). Influentials, novelty, and social contagion: The viral power of average friends, close communities, and old news . Social Networks , 34 ( 4 ), 470 – 480 . doi: 10.1016/j.socnet.2012.02.005
Hermida , A. , Fletcher , F. , Korell , D. , & Logan , D. ( 2012 ). Share, like, recommend: Decoding the social media news consumer . Journalism Studies , 13 ( 5–6 ), 815 – 824 . doi: 10.1080/1461670x.2012.664430
Himelboim , I. , Hansen , D. , & Bowser , A. ( 2013 ). Playing the same Twitter network: Political information seeking in the 2010 US gubernatorial elections . Information Communication & Society , 16 ( 9 ), 1373 – 1396 . doi: 10.1080/1369118x.2012.706316
Holton , A. E. , & Chyi , H. I. ( 2012 ). News and the overloaded consumer: Factors influencing information overload among news consumers . Cyberpsychology Behavior and Social Networking , 15 ( 11 ), 619 – 624 . doi: 10.1089/cyber.2011.0610
Jenkins , H. ( 2006 ). Convergence culture: Where old and new media collide . New York, NY : New York University Press .
Kim , S. J. ( 2014 ). A repertoire approach to cross-platform media use behavior . New Media & Society , 1 – 20 . doi: 10.1177/1461444814543162
Kivran-Swaine , F. , Govindan , P. , & Naaman , M. ( 2011 ). The impact of network structure on breaking ties in online social networks: Unfollowing on Twitter . Paper presented at the Proceedings of the SIGCHI conference on human factors in computing systems.
Kwak , H. , Chun , H. , & Moon , S. ( 2011 ). Fragile online relationship: A first look at unfollow dynamics in twitter . Paper presented at the Proceedings of the SIGCHI Conference on Human Factors in Computing Systems.
Kwak , H. , Lee , C. , Park , H. , & Moon , S. ( 2010 ). What is Twitter, a social network or a news media? Paper presented at the Proceedings of the 19th international conference on World wide web.
Kwak , H. , Moon , S. B. , & Lee , W. ( 2012 ). More of a receiver than a giver: Why do people unfollow in Twitter? Paper presented at the ICWSM.
Liang , H. , & Fu , K. W. ( 2015 ). Testing propositions derived from Twitter studies: Generalization and replication in computational social science . Plos One . doi: 10.1371/journal.pone.0134270
Marwick , A. E. , & boyd , d. ( 2011 ). I tweet honestly, I tweet passionately: Twitter users, context collapse, and the imagined audience . New Media & Society , 13 ( 1 ), 114 – 133 . doi: 10.1177/1461444810365313
McPherson , M. , Smith-Lovin , L. , & Cook , J. M. ( 2001 ). Birds of a feather: Homophily in social networks . Annual Review of Sociology , 27 , 415 – 444 . doi: 10.1146/annurev.soc.27.1.415
Murthy , D. ( 2012 ). Towards a sociological understanding of social media: Theorizing Twitter . Sociology-the Journal of the British Sociological Association , 46 ( 6 ), 1059 – 1073 . doi: 10.1177/0038038511422553
Myers , S. A. , & Leskovec , J. ( 2014 ). The bursty dynamics of the twitter information network . Paper presented at the Proceedings of the 23rd International Conference on World Wide Web.
Myers , S. A. , Sharma , A. , Gupta , P. , & Lin , J. ( 2014 ). Information network or social network?: The structure of the twitter follow graph . Paper presented at the Proceedings of the Companion Publication of the 23rd International Conference on World Wide Web.
Nakagawa , S. , & Schielzeth , H. ( 2013 ). A general and simple method for obtaining R2 from generalized linear mixed-effects models . Methods in Ecology and Evolution , 4 ( 2 ), 133 – 142 . doi: 10.1111/j.2041-210x.2012.00261.x
Quercia , D. , Bodaghi , M. , & Crowcroft , J. ( 2012 ). Loosing friends on Facebook . Paper presented at the Proceedings of the 4th Annual ACM Web Science Conference.
Robertson , S. ( 2004 ). Understanding inverse document frequency: On theoretical arguments for IDF . Journal of Documentation , 60 ( 5 ), 503 – 520 . doi: 10.1108/00220410560582
Salton , G. , Wong , A. , & Yang , C.-S. ( 1975 ). A vector space model for automatic indexing . Communications of the ACM , 18 ( 11 ), 613 – 620 . doi: 10.1145/361219.361220
Savolainen , R. ( 2007 ). Filtering and withdrawing: Strategies for coping with information overload in everyday contexts . Journal of Information Science , 33 ( 5 ), 611 – 621 . doi: 10.1177/0165551506077418
Snijders , T. A. B. , & Bosker , R. J. ( 2012 ). Multilevel analysis: An introduction to basic and advanced multilevel modeling (2nd ed.). London : Sage .
Stefanone , M. A. , Hurley , C. M. , & Yang , Z. J. ( 2013 ). Antecedents of online information seeking . Information Communication & Society , 16 ( 1 ), 61 – 81 . doi: 10.1080/1369118x.2012.656137
Stephens , K. K. , Barrett , A. K. , & Mahometa , M. J. ( 2013 ). Organizational communication in emergencies: Using multiple channels and sources to combat noise and capture attention . Human Communication Research , 39 ( 2 ), 230 – 251 . doi: 10.1111/hcre.12002
Sunstein , C. R. ( 2009 ). Republic.com 2.0 . Princeton, NJ : Princeton University Press .
Taneja , H. , Webster , J. G. , Malthouse , E. C. , & Ksiazek , T. B. ( 2012 ). Media consumption across platforms: Identifying user-defined repertoires . New Media & Society , 14 ( 6 ), 951 – 968 . doi: 10.1177/1461444811436146
Watson-Manheim , M. B. , & Belanger , F. ( 2007 ). Communication media repertoires: Dealing with the multiplicity of media choices . Mis Quarterly , 31 ( 2 ), 267 – 293 .
Webster , J. G. ( 2010 ). User information regimes: How social media shape patterns of consumption . Northwestern University Law Review , 104 ( 2 ), 593 – 612 .
Webster , J. G. ( 2014 ). The marketplace of attention: How audiences take shape in a digital age : MIT Press .
Webster , J. G. , & Ksiazek , T. B. ( 2012 ). The dynamics of audience fragmentation: Public attention in an age of digital media . Journal of Communication , 62 ( 1 ), 39 – 56 . doi: 10.1111/j.1460-2466.2011.01616.x
Webster , J. G. , & Wakshlag , J. J. ( 1983 ). A theory of television program choice . Communication Research , 10 ( 4 ), 430 – 446 . doi: 10.1177/009365083010004002
Weng , J. , Lim , E.-P. , Jiang , J. , & He , Q. ( 2010 ). Twitterrank: finding topic-sensitive influential twitterers . Paper presented at the Proceedings of the third ACM international conference on Web search and data mining.
Wood , W. , Quinn , J. M. , & Kashy , D. A. ( 2002 ). Habits in everyday life: Thought, emotion, and action . Journal of Personality and Social Psychology , 83 ( 6 ), 1281 – 1297 . doi: 10.1037//0022-3514.83.6.1281
Wu , S. , Hofman , J. M. , Mason , W. A. , & Watts , D. J. ( 2011 ). Who says what to whom on Twitter . Paper presented at the Proceedings of the 20th international conference on World Wide Web.
Xu , B. , Huang , Y. , Kwak , H. , & Contractor , N. ( 2013 ). Structures of broken ties: Exploring unfollow behavior on twitter . Paper presented at the Proceedings of the 2013 conference on Computer supported cooperative work.
Yuan , E. ( 2011 ). News consumption across multiple media platforms: A repertoire approach . Information Communication & Society , 14 ( 7 ), 998 – 1016 . doi: 10.1080/1369118x.2010.549235
Hai Liang is Assistant Professor in the School of Journalism and Communication at the Chinese University of Hong Kong. His research interests include political communication, dynamic communication process, social media analytics, and computational social science. E-mail: [email protected] . Address: School of Journalism and Communication, Room 424, Humanities Building, New Asia College, The Chinese University of Hong Kong, Shatin, N.T., Hong Kong
King-wa Fu is Associate Professor at the Journalism and Media Studies Centre, The University of Hong Kong. His research interests cover political participation and media use, computational media studies, health and the media, and younger generation's Internet use. E-mail: [email protected] . Address: Journalism and Media Studies Centre, Room 206, Eliot Hall, Pokfulam Road, The University of Hong Kong, Hong Kong.
Month: | Total Views: |
---|---|
December 2017 | 7 |
January 2018 | 25 |
February 2018 | 30 |
March 2018 | 67 |
April 2018 | 75 |
May 2018 | 98 |
June 2018 | 53 |
July 2018 | 57 |
August 2018 | 99 |
September 2018 | 113 |
October 2018 | 103 |
November 2018 | 136 |
December 2018 | 76 |
January 2019 | 63 |
February 2019 | 83 |
March 2019 | 107 |
April 2019 | 129 |
May 2019 | 68 |
June 2019 | 68 |
July 2019 | 68 |
August 2019 | 87 |
September 2019 | 56 |
October 2019 | 97 |
November 2019 | 77 |
December 2019 | 77 |
January 2020 | 74 |
February 2020 | 114 |
March 2020 | 108 |
April 2020 | 152 |
May 2020 | 88 |
June 2020 | 154 |
July 2020 | 106 |
August 2020 | 82 |
September 2020 | 130 |
October 2020 | 171 |
November 2020 | 165 |
December 2020 | 113 |
January 2021 | 116 |
February 2021 | 148 |
March 2021 | 159 |
April 2021 | 158 |
May 2021 | 126 |
June 2021 | 104 |
July 2021 | 108 |
August 2021 | 150 |
September 2021 | 111 |
October 2021 | 140 |
November 2021 | 114 |
December 2021 | 82 |
January 2022 | 73 |
February 2022 | 89 |
March 2022 | 114 |
April 2022 | 110 |
May 2022 | 74 |
June 2022 | 95 |
July 2022 | 97 |
August 2022 | 72 |
September 2022 | 61 |
October 2022 | 109 |
November 2022 | 64 |
December 2022 | 56 |
January 2023 | 55 |
February 2023 | 70 |
March 2023 | 64 |
April 2023 | 125 |
May 2023 | 61 |
June 2023 | 44 |
July 2023 | 54 |
August 2023 | 71 |
September 2023 | 72 |
October 2023 | 61 |
November 2023 | 101 |
December 2023 | 63 |
January 2024 | 86 |
February 2024 | 67 |
March 2024 | 78 |
April 2024 | 107 |
May 2024 | 90 |
June 2024 | 109 |
July 2024 | 97 |
August 2024 | 51 |
Email alerts
Citing articles via.
- Recommend to Your Librarian
- Advertising and Corporate Services
Affiliations
- Online ISSN 1083-6101
- Copyright © 2024 International Communication Association
- About Oxford Academic
- Publish journals with us
- University press partners
- What we publish
- New features
- Open access
- Institutional account management
- Rights and permissions
- Get help with access
- Accessibility
- Advertising
- Media enquiries
- Oxford University Press
- Oxford Languages
- University of Oxford
Oxford University Press is a department of the University of Oxford. It furthers the University's objective of excellence in research, scholarship, and education by publishing worldwide
- Copyright © 2024 Oxford University Press
- Cookie settings
- Cookie policy
- Privacy policy
- Legal notice
This Feature Is Available To Subscribers Only
Sign In or Create an Account
This PDF is available to Subscribers Only
For full access to this pdf, sign in to an existing account, or purchase an annual subscription.
Have a language expert improve your writing
Run a free plagiarism check in 10 minutes, generate accurate citations for free.
- Knowledge Base
- Academic writing
- How to avoid repetition and redundancy
How to Avoid Repetition and Redundancy in Academic Writing
Published on March 15, 2019 by Kristin Wieben . Revised on July 23, 2023.
Repetition and redundancy can cause problems at the level of either the entire paper or individual sentences. However, repetition is not always a problem as, when used properly, it can help your reader follow along. This article shows how to streamline your writing.
Instantly correct all language mistakes in your text
Upload your document to correct all your mistakes in minutes
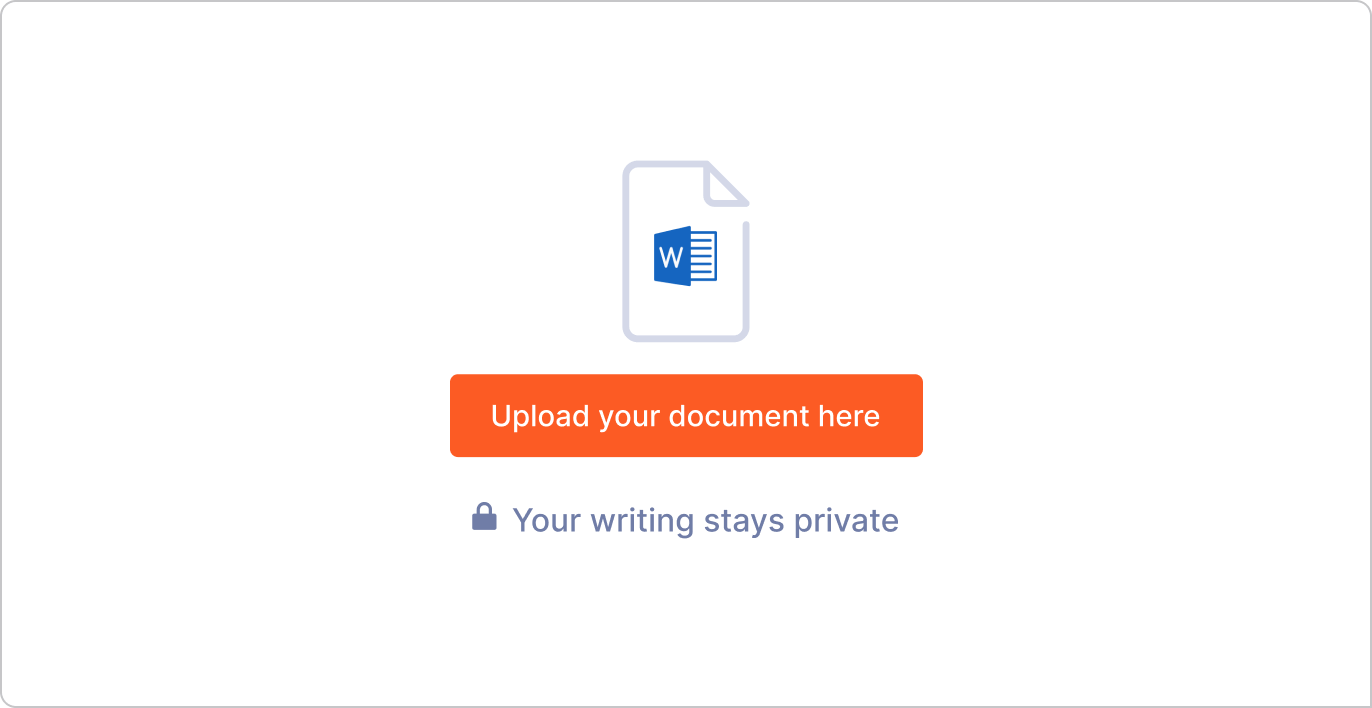
Table of contents
Avoiding repetition at the paper level, avoiding repetition at the sentence level, when is repetition not a problem, other interesting articles.
On the most basic level, avoid copy-and-pasting entire sentences or paragraphs into multiple sections of the paper. Readers generally don’t enjoy repetition of this type.
Don’t restate points you’ve already made
It’s important to strike an appropriate balance between restating main ideas to help readers follow along and avoiding unnecessary repetition that might distract or bore readers.
For example, if you’ve already covered your methods in a dedicated methodology chapter , you likely won’t need to summarize them a second time in the results chapter .
If you’re concerned about readers needing additional reminders, you can add short asides pointing readers to the relevant section of the paper (e.g. “For more details, see Chapter 4”).
Don’t use the same heading more than once
It’s important for each section to have its own heading so that readers skimming the text can easily identify what information it contains. If you have two conclusion sections, try making the heading more descriptive – for instance, “Conclusion of X.”
Are all sections relevant to the main goal of the paper?
Try to avoid providing redundant information. Every section, example and argument should serve the main goal of your paper and should relate to your thesis statement or research question .
If the link between a particular piece of information and your broader purpose is unclear, then you should more explicitly draw the connection or otherwise remove that information from your paper.
Receive feedback on language, structure, and formatting
Professional editors proofread and edit your paper by focusing on:
- Academic style
- Vague sentences
- Style consistency
See an example
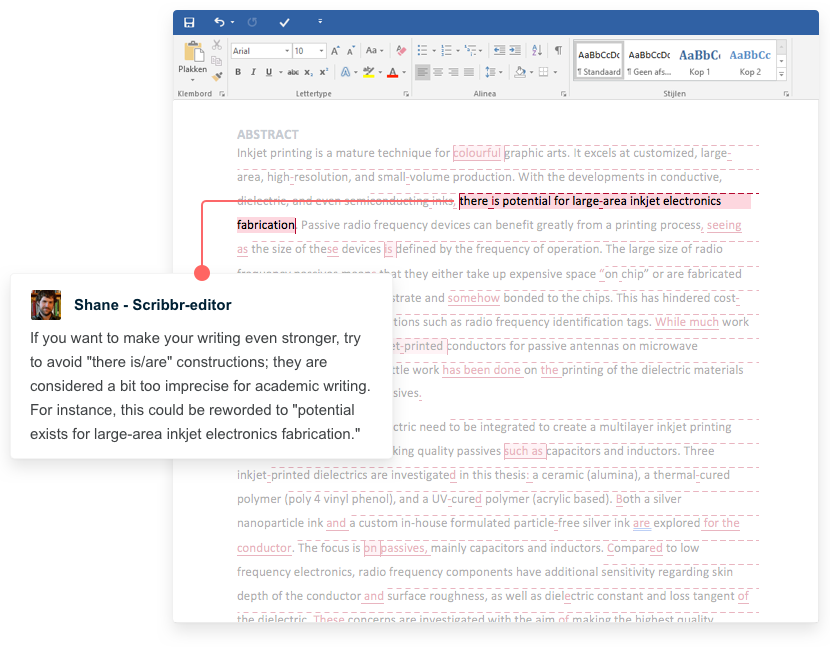
Keep an eye out for lengthy introductory clauses that restate the main point of the previous sentence. This sort of sentence structure can bury the new point you’re trying to make. Try to keep introductory clauses relatively short so that readers are still focused by the time they encounter the main point of the sentence.
In addition to paying attention to these introductory clauses, you might want to read your paper aloud to catch excessive repetition. Below we listed some tips for avoiding the most common forms of repetition.
- Use a variety of different transition words
- Vary the structure and length of your sentences
- Don’t use the same pronoun to reference more than one antecedent (e.g. “ They asked whether they were ready for them”)
- Avoid repetition of particular sounds or words (e.g. “ Several shelves sheltered similar sets of shells ”)
- Avoid redundancies (e.g “ In the year 2019 ” instead of “ in 2019 ”)
- Don’t state the obvious (e.g. “The conclusion chapter contains the paper’s conclusions”)
It’s important to stress that repetition isn’t always problematic. Repetition can help your readers follow along. However, before adding repetitive elements to your paper, be sure to ask yourself if they are truly necessary.
Restating key points
Repeating key points from time to time can help readers follow along, especially in papers that address highly complex subjects. Here are some good examples of when repetition is not a problem:
Restating the research question in the conclusion This will remind readers of exactly what your paper set out to accomplish and help to demonstrate that you’ve indeed achieved your goal.
Referring to your key variables or themes Rather than use varied language to refer to these key elements of the paper, it’s best to use a standard set of terminology throughout the paper, as this can help your readers follow along.
Underlining main points
When used sparingly, repetitive sentence and paragraph structures can add rhetorical flourish and help to underline your main points. Here are a few famous examples:
“ Ask not what your country can do for you – ask what you can do for your country” – John F. Kennedy, inaugural address
“…and that government of the people , by the people , for the people shall not perish from the earth.” – Abraham Lincoln, Gettysburg Address
If you want to know more about AI for academic writing, AI tools, or fallacies make sure to check out some of our other articles with explanations and examples or go directly to our tools!
- Ad hominem fallacy
- Post hoc fallacy
- Appeal to authority fallacy
- False cause fallacy
- Sunk cost fallacy
- Deep learning
- Generative AI
- Machine learning
- Reinforcement learning
- Supervised vs. unsupervised learning
(AI) Tools
- Grammar Checker
- Paraphrasing Tool
- Text Summarizer
- AI Detector
- Plagiarism Checker
- Citation Generator
Cite this Scribbr article
If you want to cite this source, you can copy and paste the citation or click the “Cite this Scribbr article” button to automatically add the citation to our free Citation Generator.
Wieben, K. (2023, July 23). How to Avoid Repetition and Redundancy in Academic Writing. Scribbr. Retrieved August 19, 2024, from https://www.scribbr.com/academic-writing/repetition-redundancy/
Is this article helpful?
Kristin Wieben
Other students also liked, transition words & phrases | list & examples, how to write more concisely, how to write effective headings, what is your plagiarism score.
Subscribe to the PwC Newsletter
Join the community, edit social preview.

Add a new code entry for this paper
Remove a code repository from this paper, mark the official implementation from paper authors, add a new evaluation result row.
TASK | DATASET | MODEL | METRIC NAME | METRIC VALUE | GLOBAL RANK | REMOVE |
---|
- DOCUMENT UNDERSTANDING
- KEY INFORMATION EXTRACTION
Remove a task
Add a method, remove a method, edit datasets, information redundancy and biases in public document information extraction benchmarks.
28 Apr 2023 · Seif Laatiri , Pirashanth Ratnamogan , Joel Tang , Laurent Lam , William Vanhuffel , Fabien Caspani · Edit social preview
Advances in the Visually-rich Document Understanding (VrDU) field and particularly the Key-Information Extraction (KIE) task are marked with the emergence of efficient Transformer-based approaches such as the LayoutLM models. Despite the good performance of KIE models when fine-tuned on public benchmarks, they still struggle to generalize on complex real-life use-cases lacking sufficient document annotations. Our research highlighted that KIE standard benchmarks such as SROIE and FUNSD contain significant similarity between training and testing documents and can be adjusted to better evaluate the generalization of models. In this work, we designed experiments to quantify the information redundancy in public benchmarks, revealing a 75% template replication in SROIE official test set and 16% in FUNSD. We also proposed resampling strategies to provide benchmarks more representative of the generalization ability of models. We showed that models not suited for document analysis struggle on the adjusted splits dropping on average 10,5% F1 score on SROIE and 3.5% on FUNSD compared to multi-modal models dropping only 7,5% F1 on SROIE and 0.5% F1 on FUNSD.
Code Edit Add Remove Mark official
Tasks edit add remove, datasets edit.

Results from the Paper Edit
Methods edit add remove.
Fault-Tolerant Systems by Israel Koren, C. Mani Krishna
Get full access to Fault-Tolerant Systems and 60K+ other titles, with a free 10-day trial of O'Reilly.
There are also live events, courses curated by job role, and more.
CHAPTER 3 Information Redundancy
Errors in data may occur when the data are being transferred from one unit to another, from one system to another, or even while the data are stored in a memory unit. To tolerate such errors, we introduce redundancy into the data: this is called information redundancy . The most common form of information redundancy is coding , which adds check bits to the data, allowing us to verify the correctness of the data before using it and, in some cases, even allowing the correction of the erroneous data bits. Several commonly used error-detecting and error-correcting codes are discussed in Section 3.1.
Introducing information redundancy through coding is not limited to the level of individual data words but can be extended ...
Get Fault-Tolerant Systems now with the O’Reilly learning platform.
O’Reilly members experience books, live events, courses curated by job role, and more from O’Reilly and nearly 200 top publishers.
Don’t leave empty-handed
Get Mark Richards’s Software Architecture Patterns ebook to better understand how to design components—and how they should interact.
It’s yours, free.
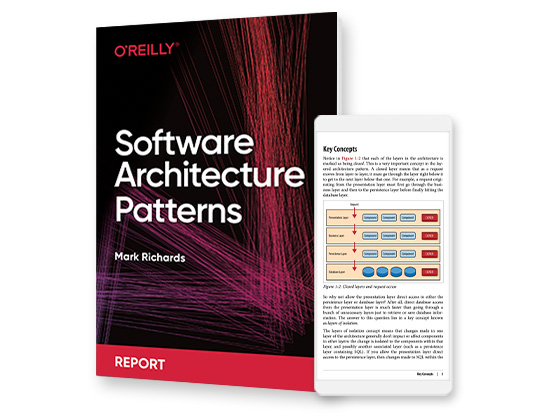
Check it out now on O’Reilly
Dive in for free with a 10-day trial of the O’Reilly learning platform—then explore all the other resources our members count on to build skills and solve problems every day.
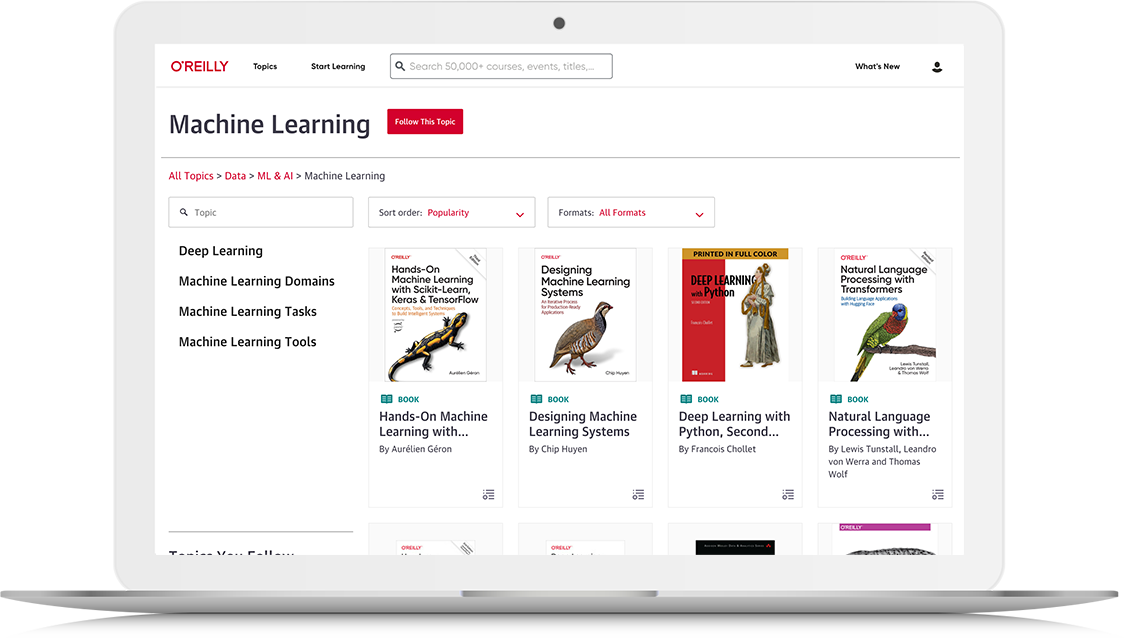

An official website of the United States government
The .gov means it’s official. Federal government websites often end in .gov or .mil. Before sharing sensitive information, make sure you’re on a federal government site.
The site is secure. The https:// ensures that you are connecting to the official website and that any information you provide is encrypted and transmitted securely.
- Publications
- Account settings
Preview improvements coming to the PMC website in October 2024. Learn More or Try it out now .
- Advanced Search
- Journal List
- BMC Bioinformatics

Redundancy in electronic health record corpora: analysis, impact on text mining performance and mitigation strategies
Raphael cohen.
1 Department of Computer Science, Ben-Gurion University in the Negev, Beer-Sheva, Israel
Michael Elhadad
Noémie elhadad.
2 Department of Biomedical Informatics, Columbia University, New York, NY, USA
The increasing availability of Electronic Health Record (EHR) data and specifically free-text patient notes presents opportunities for phenotype extraction. Text-mining methods in particular can help disease modeling by mapping named-entities mentions to terminologies and clustering semantically related terms. EHR corpora, however, exhibit specific statistical and linguistic characteristics when compared with corpora in the biomedical literature domain. We focus on copy-and-paste redundancy: clinicians typically copy and paste information from previous notes when documenting a current patient encounter. Thus, within a longitudinal patient record, one expects to observe heavy redundancy. In this paper, we ask three research questions: (i) How can redundancy be quantified in large-scale text corpora? (ii) Conventional wisdom is that larger corpora yield better results in text mining. But how does the observed EHR redundancy affect text mining? Does such redundancy introduce a bias that distorts learned models? Or does the redundancy introduce benefits by highlighting stable and important subsets of the corpus? (iii) How can one mitigate the impact of redundancy on text mining?
We analyze a large-scale EHR corpus and quantify redundancy both in terms of word and semantic concept repetition. We observe redundancy levels of about 30% and non-standard distribution of both words and concepts. We measure the impact of redundancy on two standard text-mining applications: collocation identification and topic modeling. We compare the results of these methods on synthetic data with controlled levels of redundancy and observe significant performance variation. Finally, we compare two mitigation strategies to avoid redundancy-induced bias: (i) a baseline strategy, keeping only the last note for each patient in the corpus; (ii) removing redundant notes with an efficient fingerprinting-based algorithm. a For text mining, preprocessing the EHR corpus with fingerprinting yields significantly better results.
Conclusions
Before applying text-mining techniques, one must pay careful attention to the structure of the analyzed corpora. While the importance of data cleaning has been known for low-level text characteristics (e.g., encoding and spelling), high-level and difficult-to-quantify corpus characteristics, such as naturally occurring redundancy, can also hurt text mining. Fingerprinting enables text-mining techniques to leverage available data in the EHR corpus, while avoiding the bias introduced by redundancy.
The Electronic Health Record (EHR) contains valuable information entered by clinicians. Besides its immediate clinical use at the point of care, the EHR, when treated as a repository of medical information across many patients, provides rich data waiting to be analyzed and mined for clinical discovery. Patient notes, in particular, convey an abundance of information about the patient’s medical history and treatments, as well as signs and symptoms, which, often, are not captured in the structured part of the EHR. The information in notes can be found in the form of narrative and semi-structured format through lists or templates with free-text fields. As such, much research has been devoted to parsing and information extraction of clinical notes [ 1 - 3 ] with the goal of improving both health care and clinical research.
Two promising areas of research in mining the EHR concern phenotype extraction, or more generally the modeling of disease based on clinical documentation [ 4 - 6 ] and drug-related discovery [ 7 , 8 ]. With these goals in mind, one might want to identify concepts that are associated by looking for frequently co-occurring pairs of concepts or phrases in patient notes, or cluster concepts across patients to identify latent variables corresponding to clinical models. In these types of scenarios, standard text-mining methods can be applied to large-scale corpora of patient notes. Collocation discovery can help identify lexical variants of medical concepts that are specific to the genre of clinical notes and are not covered by existing terminologies. Topic modeling, another text-mining technique, can help cluster terms often mentioned in the same documents across many patients. This technique can bring us one step closer to identifying a set of terms representative of a particular condition, be it symptoms, drugs, comorbidities or even lexical variants of a given condition.
EHR corpora, however, exhibit specific characteristics when compared with corpora in the biomedical literature domain or the general English domain. This paper is concerned with the inherent characteristics of corpora composed of longitudinal records in particular and their impact on text-mining techniques. Each patient is represented by a set of notes. There is a wide variation in the number of notes per patient, either because of their health status, or because some patients go to different health providers while others have all their visits in the same institution. Furthermore, clinicians typically copy and paste information from previous notes when documenting a current patient encounter. As a consequence, for a given longitudinal patient record, one expects to observe heavy redundancy. In this paper, we ask three research questions: (i) how can redundancy be quantified in large-scale text corpora? (ii) Conventional wisdom is that larger corpora yield better results in text mining. But how does the observed text redundancy in EHR affect text mining? Does the observed redundancy introduce a bias that distorts learned models? Or does the redundancy introduce benefits by highlighting stable and important subsets of the corpus? (iii) How can one mitigate the impact of redundancy on text mining?
Before presenting results of our experiments and methods, we first review previous work in assessing redundancy in the EHR, two standard text-mining techniques of interest for data-driven disease modeling, and current work in how to mitigate presence of information redundancy.
Redundancy in the EHR
Along with the advent of EHR comes the ability to copy and paste from one note to another. While this functionality has definite benefits for clinicians, among them more efficient documentation, it has been noted that it might impact the quality of documentation as well as introduce errors in the documentation process [ 9 - 13 ].
Wrenn et al. [ 14 ] examined 1,670 patient notes of four types (resident sign-out note, progress note, admission note and discharge note) and assessed the amount of redundancy in these notes through time. Redundancy was defined through alignment of information in notes at the line level, using the Levenshtein edit distance. They showed redundancy of 78% within sign-out notes and 54% within progress notes of the same patient. Admission notes showed a redundancy of 30% compared to the progress, discharge and sign-out notes of the same patient. More recently, Zhang et al . [ 15 ] experimented with different metrics to assess redundancy in outpatient notes. They analyzed a corpus of notes from 178 patients. They confirm that in outpatient notes, like for inpatient notes, there is a large amount of redundancy.
Different metrics for quantifying redundancy exist for text. Sequence alignment methods such as the one proposed by Zhang et al . [ 15 ] are accurate yet expensive due to high complexity of string alignment even when optimized. Less stringent metrics include: amount of shared words, amount of shared concepts or amount of overlapping bi-grams [ 16 ]. While these methods have been shown to identify semantic similarity of texts, they do not specifically capture instances of copy-paste operations, which reproduce whole paragraphs.
BLAST [ 17 ], the most popular sequence similarity algorithm in bioinformatics, is based on hashing of short sub-strings within the genetic sequence and then using the slower optimized dynamic programming alignment for sequences found to share enough sub-sequences.
The algorithm we present in this paper for building a sub-corpus with reduced redundancy is based on a finger-printing method similar to BLAST. We show that this algorithm does not require the slower alignment stage of BLAST and that it accurately identifies instances of copy-paste operations.
Text mining techniques
We review two established text-mining techniques: collocation identification and topic modeling. Both techniques have been used in many different domains and do not require any supervision. They both rely on patterns of co-occurrence of words.
Collocations are word sequences that co-occur more often than expected by chance. Collocations, such as “heart attack” and “mineral water,” carry more information than the individual words comprising them. Extraction of collocation is a basic NLP method [ 18 ] and is particularly useful for extracting salient phrases in a corpus. The NSP package we use in our experiments is widely used for collocation and n-gram extraction in the clinical domain [ 19 - 22 ].
Collocations in a corpus of clinical notes are prime candidates to be mapped to meaningful phenotypes [ 19 - 21 ]. Collocations can also help uncover multi-word terms that are not covered by medical terminologies. For instance, the phrase “hip rplc” is a common phrase used to refer to the hip replacement procedure, which does not match any concept on its own in the UMLS. When gathering counts or co-occurrence patterns for association studies with the goal of high-level applications, like detection of adverse drug events or disease modeling, augmenting existing terminologies with such collocations can be beneficial.
Collocations and n-grams are also used for various NLP applications such as domain adaptation of syntactic parsers [ 23 ], translation of medical summaries [ 24 ], semantic classification [ 25 ]or automatically labeling topics extracted using topic modeling [ 26 ].
State of the art articles (as cited above) and libraries (such as the NSP package) do not include any form of redundancy control or noise reduction. Redundancy mitigation is currently not a standard practice within the field of collocation extraction.
Topic modeling aims to identify common topics of discussion in a collection of documents (in our case, patient notes). Latent Dirichlet Allocation (LDA), introduced by Blei et al . [ 27 ], is an unsupervised generative probabilistic graphical model for topic modeling. Documents are represented as random mixtures over latent topics, where each topic is characterized by a distribution over words. The words in a document are generated one after the other by repeatedly sampling a topic according to the topic distribution and selecting a word given the chosen topic. As such, the LDA topics group words that tend to co-occur. From the viewpoint of disease modeling, LDA topics are an attractive data modeling and corpus exploration tool. As illustrative examples, we show the top-20 tokens corresponding to three topics acquired from a corpus of patient notes in Table Table1. 1 . The corpus consists of records of patients with chronic kidney disease.
Topics extracted from our corpus using a plain LDA model
renal | ckd | cr | kidney | appt | lasix | disease | anemia | pth | iv | |
htn | lisinopril | hctz | bp | lipitor | asa | date | amlodipine | ldl | hpl | |
pulm | pulmonary | ct | chest | copd | lung | pfts | sob | cough | pna |
Topic modeling has been leveraged in a wide range of text-based applications, including document classification, summarization and search [ 27 ]. In the clinical domain, Arnold et al. [ 28 ] used LDA for comparing patient notes based on topics. A topic model was learned for different cohorts, with the number of topics derived experimentally based on log-likelihood fit of the created model to a test set. To improve results, only UMLS terms were used as words. More recently, Perotte et al. leveraged topic models in a supervised framework for the task of assigning ICD-9 codes to discharge summaries [ 29 ]. There, the input consisted of the words in the discharge summaries and the hierarchy of ICD-9 codes. Bisgin et al. [ 30 ] applied LDA topic modeling to FDA drug side effects labels, their results demonstrated that the acquired topics properly clustered drugs by safety concerns and therapeutic uses.
As observed for the field of collocation extraction, redundancy mitigation is not mentioned as standard practice in the case of topic modeling.
Impact of corpus characteristics and redundancy on mining techniques
Conventional wisdom is that larger corpora yield better results in text mining. In fact, it is well established empirically that larger datasets yield more accurate models of text processing (see for example, [ 31 - 34 ]). Naturally the corpus must be controlled so that all texts come from a similar domain and genre. Many studies have indeed shown that cross-domain learned corpora yield poor language models [ 35 ]. The field of domain adaptation attempts to compensate for the poor quality of cross-domain data, by adding carefully picked text from other domains [ 36 , 37 ] or other statistical mitigation techniques. In the field of machine translation, for instance, Moore and Lewis [ 38 ] suggested for the task of obtaining an in-domain n-gram model, choosing only a subset of documents from the general corpora based on the domain's n-gram model can improve language model while trained on less data.
In this paper, we address the opposite problem: our original corpus is large, but it does not represent a natural sample of texts because of the way it was constructed. High redundancy and copy-and-paste operations in the notes create a biased sample of the “patient note” genre. From a practical perspective, redundant data in a corpus lead to waste of CPU time in corpus analysis and waste of I/O and storage space especially in long pipelines, where each stage of data processing yields an enriched set of the data.
Downey et al. [ 39 ] suggested a model for unsupervised information extraction which takes redundancy into account when extracting information from the web. They showed that the popular information extraction method, Pointwise Mutual Information (PMI), is less accurate by an order of magnitude compared to a method with redundancy handling. They present a model for unsupervised information extraction which takes redundancy into account when extracting information from the web.
Methods for identifying redundancy in large string-based databases exist in both bioinformatics and plagiarism detection [ 40 - 42 ]. A similar problem has been addressed in the creation of sequence databases for bioinformatics: Holm and Sander [ 43 ] advocated the creation of non-redundant protein sequence databases and suggested that databases limit the level of redundancy. Redundancy avoidance results in smaller size, reduced CPU and improved annotation consistency. Pfam [ 44 ] is a non-redundant protein sequence database manually built using representatives from each protein family. This database is used for construction of Hidden-Markov-Model classifiers widely used in Bioinformatics.
When constructing a corpus of patient notes for statistical purposes, we encounter patients with many records. High redundancy in those documents may skew statistical methods applied to the corpus. This phenomenon also hampers the use of machine learning methods by preventing a good division of the data to non-overlapping test and train sets. In the clinical realm, redundancy of information has been noted and its impact on clinical practice is discussed, but there has not been any work on the impact of redundancy in the EHR from a data mining perspective, nor any solution suggested for how to mitigate the impact of within-patient information redundancy within an EHR-mining framework.
Results and discussion
Quantifying redundancy in a large-scale ehr corpus, word sequence redundancy at the patient level.
The first task we address is to define metrics to measure the level of redundancy in a text corpus. Redundancy across two documents may be measured in different manners: shared words, shared concepts or overlapping word sequences. The most stringent method examines word sequences, and allows for some variation in the sequences (missing or changed words). For example the two sentences: “ Pt developed abd pain and acute cholecystitis ” and “ Pt developed acute abd pain and cholecystitis ” would score 100% identity on shared words but only 73% identity of sequence alignment.
Our EHR corpus can be organized by patient identifier. We can, therefore, quantify the amount of redundancy within a patient record. On average, our corpus contains 14 notes per patient, with standard deviation of 16, minimum of 1 and 167 maximum notes per patient. There are also several note types in the patient record such as imaging reports or admission notes. We expect redundancy to be high across notes of the same patient and low across notes of distinct patients. Furthermore, within a single patient record, we expect heavy redundancy across notes from the same note types. We report redundancy on same patient / similar note type (we focus on the most informative note types: primary provider, follow up and clinical notes; in this analysis we ignore the template-based note types which are redundant by construction).
Within this scope, we observe in our corpus average sequence redundancy ( i.e., the percentage of alignment of two documents) of 29%: that is, on average one third the words of any informative note from a given patient are aligned with a similar sequence of words in another informative note from the same patient. In contrast, the figure drops to an average of 2.9% (with maximum of 8% and standard deviation of 0.6%) when comparing the same note types across two distinct patients.
The results of high redundancy in patient notes are consistent with Wrenn et al. [ 14 ] observations on a similar EHR dataset. The contrast between same-patient and across-patient redundancy, however, is surprising given that the whole corpus is sampled from a population with at least one shared chronic condition. Our interpretation is that the observed redundancy is most likely not due to clinical content but to the process of copy and paste.
Figure Figure1 1 further details the full histogram of redundancy for pairs of same-patient informative notes. The redundancy (percentage of aligned tokens) was computed for the notes of a random sample of 100 patients. For instance, it indicates that 7.6% of the same patient note pairs in the corpus have between 20% and 30% identity.

Distribution of similarity levels across pairs of same-patient informative notes in the corpus.
The detailed distribution supports the distinction into 2 groups of notes: those with heavy repetition (about 37% of the pairs - with similarity between 40% and 100%) and those with no repetition (about 63% of the notes). A possible interpretation is that a group of patient files include many notes and tend to exhibit heavy redundancy while others are shorter with less natural redundancy. The level of overall redundancy is significant and spread over many documents (over a third).
Concept redundancy at the corpus level
Since free-text notes exhibit high level of variability in their language, the redundancy measures may be different when we examine terms normalized against a standard terminology. We now focus on the pre-processed EHR corpus, where named entities are mapped to UMLS Concept Unique Identifiers (CUIs) (Section 4.1.1 describes the automatic mapping method we used). We investigate whether a redundant corpus exhibits a different distribution of concepts than a less redundant one.
We expect that different subsets of the EHR corpus exhibit different levels of redundancy. The All Informative Notes corpus, which contains several notes per patient, but only the ones of types: “primary-provider”, “clinical-note” and “follow-up-note”, is assumed to be highly redundant, since it is homogeneous in style and clinical content. By contrast, The Last Informative Note corpus, which contains only the most recent note per patient, is hypothesized to be the least redundant corpus. The All EHR corpus, which contains all notes of all types, fits between these two extremes, since we expect less redundancy across note types, even for a single patient.
One standard way of characterizing large corpora is to plot the histogram of terms and their raw frequencies in the corpus. According to Zipf’s law, the frequency of a word is inversely proportional to its rank in the frequency table across the corpus, that is, term frequencies follow a power law. Figure Figure2 2 shows the distribution of UMLS concepts (CUI) frequencies in the three corpora with expected decreasing levels of redundancy: the All Informative Notes corpus, the All Notes corpus, and the Last Informative Note Corpus. We observe that the profile in the non-redundant Last Informative Note corpus differs markedly from the ones of the redundant corpora ( All Notes and All Informative Notes ). The non-redundant corpus follows a traditional power law [ 45 ], while the redundant ones exhibit a secondary frequency peak for concepts which appear between 4 and 16 times in the corpus. In the highly-redundant All Informative Notes corpus, the peak is the most pronounced, with more concepts occurring four to eight times in the corpus than once.

Concept-distribution. Distribution of UMLS concept occurrences in corpora with different levels of redundancy. The All Notes ( a ) and All Informative Notes ( b ) corpora have inherent redundancy, while the Last Informative Note ( c ) corpus does not. The shapes of the distributions of concepts differ depending on the presence of redundancy in the corpus.
The difference in shapes of distributions confirms in a qualitative fashion our hypothesis about the three corpora and their varying levels of redundancy. The observed contrast in distribution profiles indicates that more concepts are repeated more often than expected in the redundant corpora, and gives us a first clue that statistical metrics that rely on the regular long-tailed, power-like distributions will show bias when applied on the redundant EHR corpus. A similar pattern is observed at the bi-gram level (a Zipfian distribution for the non-redundant corpus and a non-Zipfian distribution for the redundant corpus).
Impact of redundancy on text mining
We have observed that redundant corpora exhibit different statistical profiles than non-redundant ones, according to their word occurrence distributions. We now investigate whether these differences impact the performance of standard text mining techniques: collocation identification and topic modeling.
We compare the performance of standard algorithms for collocation identification and topic modeling inference on a variety of corpora with different redundancy levels. We introduce synthetic corpora where we can control the level of redundancy. These synthetic corpora are derived from the Wall Street Journal (WSJ) standard corpus. The original WSJ corpus is naturally occurring and does not exhibit the copy and paste redundancy inherent to the EHR corpus. We artificially introduce redundancy by randomly sampling documents and repeating them until a controlled level of redundancy is achieved.
Collocation identification
We expect that in a redundant corpus, the word sequences (n-grams) which are copied often will be over-represented. Our objective is to establish whether the collocation algorithm will detect the same n-grams on a non-redundant corpus or on a version of the same corpus where parts of the documents have been copied.
Two implications of noise are possible. The first is false positive identification, i.e., extracting collocations which are the result of mere chance. The second implication is loss of significant collocations due to noise (or because important collocations are out-ranked by less important ones).
We apply two mutual information collocation identification algorithms (PMI and TMI, see Methods section) to the All Informative Notes corpus (redundant) and to the Last Informative Note corpus (non-redundant). In this scenario, we control for vocabulary: only word types that appear in the smaller corpus ( Last Informative Note ) are considered for collocations. To measure the impact of redundancy on the extracted collocations, for each collocation, we count the number of patients whose notes contain this collocation. A collocation that is supported by evidence from less than three patients is likely to be a false positive signal due to the effect of redundancy ( i.e. , most of the evidence supporting the collocation was created via a copy/paste process).
We observe that the lists of extracted collocations on these two corpora differ markedly (collocations were extracted with a threshold of 0.001 and 0.01 for TMI and PMI respectively). The PMI algorithm identified 15,814 collocations in the All Informative Notes corpus, and 2,527 in the Last Informative Notes corpus. When comparing the collocations extracted from the two corpora, we find that 36% of the collocations identified in the All Informative Notes corpus were supported by 3 patients or less, compared to only 6% in the Last Informative Note corpus. See Table Table2. 2 . For example, a note replicated 5 times signed by “John Doe NP” (Nurse Practitioner) was enough to gain a high PMI of 10.2 for the “Doe NP” bigram (as “Doe” appears only in the presence of “NP”).
Collocations found in redundant and non-redundant corpora
81,928 | 40,774 | |
3,641,031 | 545,231 | |
15,814 | 2,527 | |
0.004 | 0.004 | |
18.2 | 66 | |
36 % | 1 % |
Collocations were extracted using a stringent cutoff of 0.001 PMI.
The second type of error, loss of signal can also be observed. When comparing all collocations using the same TMI cutoff, the All Informative Notes corpus produces 3 times as many collocations as the Last Informative Notes corpus (see Table Table3), 3 ), but we find that only 54% of the collocations found in the non-redundant corpus are represented in the bigger list.
Collocation detection results in the different corpora
5,649/15,814 | 2,082/2,527 | 3,590/6,034 | |
32/18 | 74/66 | 48/37 | |
32%/36% | 1.2%/1% | 6.2%/5.8% |
Another method for selecting the significant collocations is using a top-N cutoff instead of a PMI cutoff. Comparing the top 1,000 collocations with TMI for All Informative Notes and Last Informative Notes, we find a marked difference of 196 collocations.
To control for size, we repeated the same experiment on a standard large-scale corpus, the WSJ dataset, on which collocation identification algorithms have been heavily tested in the past (see Table Table4 4 ).
Comparison of extracted collocations
WSJ-400 | Non-redundant | 214 K / 19 K | 551/565 | 20.2/19.9 |
WSJ-600 | Non-redundant | 309 K / 23.5 K | 943/1,000 | 15.5/15.2 |
WSJ-1300 | Non-redundant | 680 K / 36 K | 1,881/2,518 | 10.8/9.7 |
WSJs5 | Synthetic Redundant | 1.69 M (±42 K)/36 K | 3,035±(63)/17,015±(950) | 7.4±(0.11)/2.8±(0.09) |
Comparison of extracted collocations on synthetic redundant corpora and non-redundant corpora (WSJ – X words / Y distinct words). Collocations were extracted using using True Mutual Information and Pointwise Mutual Information (with cutoffs of 0.001 and 0.01 respectively).
Consider a scenario where a corpus is fed twice or thrice in sequence to PMI (that is, every document occurs exactly twice or thrice), then the list of extracted collocations will be identical to that of the original corpus. This is expected based on the definition of PMI, and we confirm this prediction on WSJx2 and WSJx3 which produce exactly the same list of collocations as WSJ-1300 (WSJx2 is a corpus constructed by doubling every document in WSJ-1300).
We observe a different behavior on WSJs5 (see Table Table4): 4 ): in this corpus, original sentences from WSJ-1300 are sampled between 1 and 5 times in a uniform manner (this process was replicated 10 times to eliminate bias from the random sampling). On this synthetic corpus, we obtain a different list of collocations when using the PMI algorithm: 17,015(±950) instead of 2,737. The growth in number of extracted collocations is expected since WSJs5 is 2.5 times larger than WSJ-1300, but this growth is less than expected when comparing the trend (WSJ-400, WSJ-600, WSJ-1300) with a growth of (565, 1,000 and 2,737) extracted collocations.
On the other hand, the collocations acquired on the redundant WSJs5 corpus have much weaker support than those obtained on WSJ-1300 (they occur on average in 2.8±0.09 instead of 9.6 documents per collocation). The differences we observe in this experiment are caused by the fact that some sentences only are copied, in a variable number of times (some sentences occur once, some twice, and others 5 times). Thus, PMI (which does not simply reflect word frequencies in a corpus, but takes into account global patterns of co-occurrences, since it relies on the probability of seeing terms jointly and terms independently) does not behave similarly when fed with our different corpora.
In the case of this synthetic dataset, the newly acquired collocations are all due to the synthetic copy-paste process and are likely a false positive signal. One may ask, however, whether the fact that the sentences are repeated in EHR corpora reflects on their semantic importance from a clinical standpoint, and therefore, whether the collocations extracted from the full EHR corpus contain more clinically relevant collocations. This hypothesis is rejected by the comparison of the number of “patient-specific” collocations in the redundant corpus and non-redundant one: the collocations acquired on the redundant corpus cannot serve as general reusable terms in the domain, but rather correspond to patient-specific, accidental word co-occurrences such as (first-name last-name) pairs. In other words, the PMI algorithm does not behave as desired because of the observed redundancy. For example, through qualitative inspection of the extracted collocations, we observed that within the top-20 extracted collocations from the full EHR redundant corpus, 17 appear only in a single cluster of redundant documents (a large chain of notes of a single patient copied and pasted). The fact that redundancy never occurs across patients, but within same-patient notes only, seems to create unintended biases in the extracted collocations.
The results on the WSJ and its synthetic variants confirm our results on the EHR corpora: collocations extracted on a redundant corpus differ significantly from those extracted on a corpus of similar size without redundancy. Slightly weaker, though consistent, results were encountered when using an alternative algorithm for collocation identification on the EHR and WSJ corpora (TMI instead of PMI).
Topic modeling
The algorithm for topic modeling that we analyze, LDA, is a complex inference process which captures patterns of word co-occurrences within documents. To investigate the behavior of LDA on corpora with varying levels of redundancy, we rely on two standard evaluation criteria: log-likelihood fit on withheld data and the number of topics required in order to obtain the best fit on the withheld data. The higher the log-likelihood on withheld data, the more successful the topic model is at modeling the document structure of the input corpus. The number of topics is a free parameter of LDA – given two LDA models with the same log-likelihood on withheld data, the one with the lower number of topics has better explanatory power (fewer latent variables or topics are needed to explain the data).
We apply LDA to the same two EHR corpora ( All Informative Notes and Last Informative Note ) as in the collocation identification task, and obtained the results shown in Figure Figure3. 3 . The redundant corpus, though 6.9 times larger, produces the same fit as the non-redundant corpus ( Last Informative Note ).

Model fit as function of number of topics on the EHR corpora.
When applied to the synthetic WSJ corpora, we get a finer picture of the behavior of LDA under various corpora sizes and redundancy levels (Figure (Figure4). 4 ). The WSJ-400, WSJ-600 and WSJ-1300 corpora are non-redundant and have increasing size. We observe that the log-likelihood graphs for them have the same shape, with the larger corpora achieving higher log-likelihood, and the best fits obtained with topic numbers between 100 and 200 (Figure (Figure4a). 4 a). The behavior is different for the redundant corpora. WSJx2, WSJx3, and WSJs5 are all larger in size than WSJ-1300. We therefore would expect them to reach higher log-likelihood, but this does not occur. Instead, their log-likelihood graphs keep increasing as the number of topics increases, all the while remaining consistently inferior to the WSJ-1300 corpus, from which they are derived. The higher the redundancy level (twice, thrice or up-to-five times), the worse the fit.

Model fit as function of number of topics on the WSJ corpora. In ( a ) we compare the effect of size on LDA, bigger corpora yield better fit. In ( b ) we examine the effect of redundancy: the doubled/trebled corpora reduce fit slightly while the noisier WSJs5 performs almost as badly as training on the smaller WSJ-600 corpus.
Furthermore, when comparing WSJx3 and WSJs5 corpora (Figure (Figure4b), 4 b), which have roughly the same size, we note that the more redundant corpus (WSJs5 – 220% non uniform redundancy) has consistently lower fit to withheld data than WSJx3 (200% uniform redundancy). This confirms that redundancy hurts the performance of topic modeling, even when the size of the input corpus is controlled.
Even more striking, when examining the behavior of WSJs5 (with 3,300 documents sampled from 1,300 distinct documents) up to 100 topics, we observe it reaches the same fit as WSJ-600. That is, redundancy “confuses” the LDA algorithm twice: it performs worse than the original WSJ-1300 corpus although it contains the same documents, and the fit is the same as if the algorithm had roughly five times less documents (600 distinct documents from WSJ-600 vs. 1,300 distinct documents or 3,300 documents from WSJs5).
We have seen that for the naturally occurring WSJ corpus training on more data produces better fit to held out data (see Figure Figure4a). 4 a). In contrast, we observe that the redundant All Informative Notes corpus, while 7 times larger than the non-redundant subset, does not increase log-likelihood fit to held out data.
To understand this discrepancy, we examine the topics obtained on the redundant corpora qualitatively. Topics are generated by LDA as ranked lists of words. Once a topic model is applied on a document, we can compute the topic assignment for each word in the document. We observe in the topics learned on the highly redundant corpora that the same word may be assigned to different topics in different copies of the same document. This lack of consistency explains the confusion and consequently low performance achieved by LDA on redundant corpora.
Mitigation strategies for handling redundancy
Given a corpus with inherent redundancy, like the EHR corpus, the basic goal of redundancy mitigation is to choose the largest possible subset of the corpus with an upper bound on the amount of redundancy in that subset.
We compare two mitigation strategies to detect and handle redundancy in a corpus – a baseline relying on document metadata and one based on document content (which is applicable to the common case of anonymized corpora). We focus on the All Informative Notes corpus. The metadata-based baseline produces the Last Informative Note corpus. The content-based mitigation strategy, which relies on fingerprinting, can produce corpora with varying levels of redundancy. We report results for similarity thresholds of 0.20, 0.25 and 0.33. We expect that the lower the similarity threshold, the lower the actual redundancy level of the resulting corpus (in other words, we verify that our fingerprinting redundancy reduction algorithm effectively reduces redundancy).
Descriptive statistics of reduced corpora
Table Table5 5 lists descriptive statistics of the corpora obtained with different methods. The input, Full EHR corpus, is the largest. As expected, the Last Informative Note corpus obtained through our metadata-based baseline is the smallest corpus. While redundancy is reduced, its size is also drastically decreased from the original corpus. As expected, the lower the maximum similarity threshold, the more stringent the criterion to include a document in the corpus, and thus, the smaller the resulting corpus.
Descriptive statistics of the patient notes corpora
All Informative (input) | 8,557 | 6,131,879 | 599,847 |
Last Informative Note (baseline) | 1,247 | 435,387 | 44,145 |
Selective- Fingerprinting maximum similarity 0.33 | 4,524 | 3,614,409 | 337,034 |
Selective-Fingerprinting maximum similarity 0.25 | 3,970 | 3,283,558 | 302,159 |
Selective-Fingerprinting maximum similarity 0.20 | 3,645 | 3,061,854 | 278,644 |
All Informative, input corpus, the corpus obtained by the redundancy reduction baseline ( Last Informative Note ), and the corpora produced by the fingerprinting redundancy reduction strategy at different level.
Computation time for constructing a redundancy-reduced corpus at a given similarity threshold using the selective fingerprinting is 6 minutes (with an Intel Xeon CPU X5570 2.93 GHz).
To confirm that fingerprinting similarity effectively controls the redundancy level of the resulting corpora, we align a random sample of the notes included in the corpus for a sample of patients using different methods and different similarity cutoffs (see Table Table6). 6 ). The average amount of redundancy in removed note pairs is sampled as well. Redundancy is computed in the same way as in Section 2.1.1. We randomly sampled 2,000 same-patient pairs of notes and aligned them using Smith-Waterman alignment.
Redundancy in same patient note pairs
All Informative | 29% | 2,000 |
Selective- Fingerprinting maximum similarity 0.33 | 12.70% | 380 |
Selective-Fingerprinting maximum similarity 0.25 | 9.80% | 305 |
Selective-Fingerprinting maximum similarity 0.2 | 9.30% | 263 |
Amount of redundancy in a random sample of 2,000 same-patient note pairs within the corpora using different similarity thresholds.
To investigate whether the corpora whose redundancy is reduced through our fingerprinting method are robust with respect to text mining methods, we focused on the following corpora: The inherently redundant All Informative Notes corpus, the baseline non-redundant corpus Last Informative Notes , and “ Reduced Redundancy Informative Notes ”, a corpus created by selective fingerprinting with maximum similarity of 25%. The Reduced Redundancy Informative Notes corpus contains 3,970 patient notes, 3.18 as many notes as the Last Informative Notes corpus while having same-patient redundancy of only 9.8% compared to 29% in the All Informative Notes Corpus.
Performance of text mining tasks on reduced corpora
For collocations detection, in Reduced Redundancy Informative Notes , 6,034 collocations were extracted, on average each collocation is supported by 37 distinct patients and collocations supported by 3 patients or less make 6% of the extracted collocation. We see a significant reduction in the number of collocations based on very few patients from 36% to 6% (Table (Table3 3 ).
For topic modeling, Figure Figure5 5 shows the log-likelihood fit on the EHR withheld dataset graphed against the number of topics for the LDA topic modeling for three corpora. We see that the significantly smaller Last Informative Note performs as well as All Informative Notes (8,557 notes vs. 1,247) while Reduced Redundancy Informative Notes (3,970 notes) outperforms both. As we showed in Figure Figure4a, 4 a, we would expect a larger corpus to yield a better fit on the model: All Informative Notes is more than 7 times larger than Last Informative , still it yields the same fit on held out data. This is explained by the non-uniform redundancy of All Informative as shown in Figure Figure4b. 4 b. In contrast, the Reduced Redundancy Informative Notes improves the fit compared to the non-redundant Last Informative Notes in the same manner as WSJ-1300 improves on WSJ-400 (a non-redundant corpus 3 times larger produces a better fit as expected). This healthy behavior strongly indicates that Reduced Redundancy Informative Notes indeed behaved as a non-redundant corpus with respect to the LDA algorithm.

Model fit as function of number of topics. Patient notes corpora, including the “ Reduced Informative ” corpus.
Training and improvement of NLP tools for Medical-Informatics tasks on public available data will continue growing as more EHRs are incorporated into health care givers worldwide. The nature of epidemiological research demands looking at cohorts of patients, such as our kidney patient notes. Such cohort studies require application of text mining and statistical learning methods for: collocation detection (such as PMI and TMI), Topic Modeling with LDA and methods for learning association between conditions, medication and more.
This paper identifies a characteristic of EHR text corpora: their inherent high level of redundancy, caused by the process of cut and paste involved in the creation and editing of patient notes by health providers. We empirically measure this level of redundancy on a large patient note corpus, and verify that such redundancy introduces unwanted bias when applying standard text mining algorithms. Existing text mining algorithms rely on statistical assumptions about the distribution of words and semantic concepts which are not verified on highly redundant corpora. We empirically measure the damage caused by redundancy on the tasks of collocation extraction and topic modeling through a series of controlled experiments. Preliminary qualitative inspection of the results suggests that idiosyncrasies of each patient (where the redundancy occurs) explain the observed bias.
This result indicates the need to examine the effect of redundancy on statistical learning methods before applying any other text mining algorithm to such data. In this paper, we focused on intrinsic, quantitative evaluations to assess the impact of redundancy on two text-mining techniques. Qualitative analysis as well as task-based evaluations are needed to get a full understanding of the role of redundancy in clinical notes on text-mining methods.
We presented a novel corpus subset construction method which efficiently limits the amount of redundancy in the created subset. Our method can produce corpora with different redundancy amounts quickly, without alignment of documents and without any prior knowledge of the documents. We confirmed that the parameter of our Selective Fingerprinting method is a good predictor of document alignment and can be used as the sole method for removing redundancy.
While methods such as our Selective Fingerprinting algorithm that extract a non-redundant / less-redundant subset of the corpus prevent bias, they still lead to lost information of the non-redundant parts of eliminated documents. An alternative route to text mining in the presence of high levels of redundancy consists of keeping all the existing redundant data, but designing redundancy immune statistical learning algorithms. This is a promising route of future research.
EHR corpora
We collected a corpus of patient notes from the clinical data warehouse of the New York-Presbyterian Hospital. The study was approved by the Institutional Review Board (IRB-AAAD9071) and follows HIPAA (Health Insurance Portability and Accountability Act) privacy guidelines. The corpus is homogeneous in its content, as it comprises notes of patients with chronic kidney disease who rely for primary care on one of the institution’s clinic. Each patient record contains different note types, including consult notes from specialists ( e.g., nephrology and cardiology notes), admission notes and discharge summaries, as well as notes from primary providers, which synthesize all of the patient’s problems, medications, assessments and plans.
Notes contain the following metadata: unique patient identifier, date, and note type ( e.g., Primary-Provider). The content of the notes was pre-processed to identify document structure (section boundaries and section headers, lists and paragraph boundaries, and sentence boundaries), shallow syntactic structure (part-of-speech tagging with the GENIA tagger [ 46 ] and phrase chunking with the OpenNLP toolkit [ 47 ], and UMLS concept mentions with our in-house named-entity recognizer HealthTermFinder [ 48 ]). HealthTermFinder identifies named-entities mentions and maps them against semantic concepts in UMLS [ 49 ]. As such, it is possible to map lexical variants ( e.g., “myocardial infarction,” “myocardial infarct,” “MI,” and “heart attack” ) of the same semantic concept to a UMLS CUI (concept unique identifier).
There are 104 different note types in the corpus. Some are template based, such as radiology or lab reports, and others are less structured and contain mostly free text. We identified that note types: “ primary-provider ”, “ clinical-note ” and “ follow-up-note ” contain more information than other note types. Notes of these types were found to contain 37 CUIs on average in comparison to 26 on average for all other note types. We call notes of these 3 types “ Informative Notes ”.
In our experiments, we rely on different variants of the EHR corpus (see Table Table7 7 ):
EHR corpora descriptive statistics
All Notes | 1,604 | 22,564 | 6,131,879 / 138,877 | 599,847 / 7,174 |
All Informative Notes | 1,247 | 8,557 | 2,243,551 / 51,234 | 319,298 / 5,389 |
Last Informative Note | 1,247 | 1,247 | 338,207 / 25,624 | 46,311 / 3,711 |
• The All Notes corpus is our full EHR corpus,
• The All Informative Notes corpus is a subset of All Notes, and contains only the notes of type “ primary-provider ”, “ clinical-note ” and “ follow-up-note ”.
• The Last Informative Note corpus is a subset of All Informative Notes , and contains only the most recent note for each patient.
Synthetic WSJ redundant corpora
We construct synthetic corpora with a controllable level of redundancy to compare the behavior of the text mining methods on various levels of redundancy. The synthetic corpora are based on a sample of the Wall Street Journal corpus, a widely used corpus in the field on Natural Language Processing [ 50 , 51 ]. Table Table8 8 provides descriptive statistics of the different WSJ-based corpora with which we experiment:
Corpora Descriptive statistics
WSJ-400 | 400 | 214 K / 19 K |
WSJ-600 | 600 | 309 K / 23.5 K |
WSJ-1300 | 1,300 | 680 K / 36 K |
WSJx2 | 2,600 | 1.3 M words / 36 K |
WSJx3 | 3,900 | 2.6 M words / 36 K |
WSJs5 | 3,246(±40) | 1.69 M (±42 K) words / 36 K |
Synthetic corpora with various levels of redundancy , for WSJs5 we report averages and standard deviation based on 10 replications.
• The WSJ-1300 corpus contains a random sample of 1,300 documents from the Wall Street Journal corpus,
• The WSJ-400 corpus is a subset of WSJ-1300 of 400 documents,
• The WSJ-600 corpus is a subset of WSJ-1300 of 600 documents,
• The WSJx2 corpus is constructed from WSJ-1300 to simulate redundancy, where each document of WSJ-1300 appears twice in the corpus.
• The WSJx3 corpus is similar to the WSJx2 corpus, except it contains three copies of each document in the WSJ-1300 corpus.
• The WSJs5 corpus is sampled from WSJ-1300 corpus, where each document can appear between one and five times in the corpus, with a uniform probability of 0.2. Note that the WSJs5 corpus has roughly 2.5 times the size of WSJ-1300. The process was repeated 10 times to eliminate bias from the choice of documents repeated.
Quantifying redundancy in the EHR corpus
Metric for assessing redundancy at the patient level.
Given two notes, we computed redundancy for the pair by aligning the two notes. We applied the Smith-Waterman text alignment algorithm, a commonly used string alignment algorithm in bioinformatics [ 52 ]. For each pair, we can then compute the percentage of aligned tokens. Assessing redundancy through alignment is a more appropriate and more stringent method than counting simple token overlap as in a bag-of-word model. High percentage of alignment between two notes indicates not only that tokens are similar across the two notes, but that the sequences of tokens in the notes are also similar.
Metric for assessing redundancy at the corpus level
Given a corpus, a histogram of term frequencies is computed to examine whether the corpus follows Zipf’s law. According to Zipf’s law, terms frequencies have a long tail in their distribution: that is, very few terms occur frequently (typically function words and prominent domain words) while most terms occur only once or twice in the corpus overall. Terms can be either words or semantic concepts.
Mutual information and topic modeling
Collocation identification was carried out on the different corpora using the Ngram Statistics Package [ 53 ], which provides an implementation for collocation detection using True Mutual Information (TMI) and Pointwise Mutual Information (PMI).
We compare LDA topic modeling based on log-likelihood fit to a test set and the number of topics required to obtain the best fit. This is similar to the approach used by Arnold et al . (2010) [ 28 ] and is accepted as a method for comparing LDA performance [ 54 ].
The topic models were learned using the Collapsed Gibbs Sampler provided in Mallet [ 55 ] with the recommended parameters and with hyper-parameter optimization as described in Wallach et al. [ 56 ]. The log-likelihood graphs were computed on withheld datasets. A non-redundant withheld dataset of 233 Informative notes was created for EHR corpus (all the notes from the same patients were removed from the redundant corpora to prevent contamination between corpora and the withheld dataset). For the WSJ corpora, a sample of 400 non-redundant documents was chosen as the withheld set.
Metadata-based baseline
The metadata-based mitigation strategy leverages the note creation date, the note type and the patient identifier information and selects the last available note per patient in the corpus. This baseline ensures the production of a non-redundant corpus, as there is one note per patient only.
Fingerprinting algorithm
Detecting redundancy within the notes of a single patient is feasible using standard alignment methods borrowed from bioinformatics such as: Smith-Waterman [ 52 ], FastA [ 41 ] or Blast2seq [ 40 ]. However, some available EHR corpora are de-identified to protect patient privacy [ 57 ] and notes are not grouped by patients. Aligning all the note pairs in a corpus would be computationally prohibitive, even for optimized techniques (FastA, Blast2Seq).
Approximation techniques to make this problem tractable were developed in bioinformatics to search sequence databases and for plagiarism detection. In both fields, fingerprinting schemes are applied. In BLAST, short substrings are used as fingerprints, whose length is defined by biological significance. These substrings are also used for optimizing the alignment. For plagiarism detection, HaCohen-Kerner et al. [ 42 ] compare two fingerprinting methods: (i) Full fingerprinting – all substrings of length n of a string are used as fingerprints. This means that for a string of length m , m-n+1 fingerprints will be used; and (ii) Selective Fingerprinting – non-overlapping substrings are chosen. This means that for a string of length m , m/n fingerprints will be used.
The parameter n is the granularity of the method, and its choice determines how stringent the comparison is. In order to compare two notes A and B, we compute the number of fingerprints shared by A and B. The level of similarity of B to A is defined as the ratio (number of shared fingerprints) / (number of fingerprints in A).
We use this fingerprinting similarity measure in the following redundancy reduction technique: fingerprints (non-overlapping substrings of length n) are extracted for each document line by line ( i.e. , no fingerprint may span two lines). Documents are added one by one to the new corpus, a document sharing a proportion of fingerprints larger than the cutoff value with a document already in the corpus is not added. See Figure Figure6 6 for pseudo code of this algorithm. This method is a greedy approach similar to the online algorithm described in [ 58 ].

Pseudo Code of greedy controlled redundancy sub-corpus construction algorithm.
An implementation of our algorithm in Python together with all synthetic datasets is available at https://sourceforge.net/projects/corpusredundanc .
a A Python implementation of our algorithm as well as all synthetic datasets are available at https://sourceforge.net/projects/corpusredundanc
Competing interests
The authors declare that they have no competing interests.
Authors’ contributions
RC participated in the study design, carried out the statistical analyses and wrote the paper. ME participated in study design and wrote the paper. NE participated in study design and wrote the paper. All authors read and approved the final manuscript.
Acknowledgements
This work was supported by a National Library of Medicine grant R01 LM010027 (NE). Any opinions, findings, or conclusions are those of the authors, and do not necessarily reflect the views of the funding organization.
- Friedman. A general natural - language text processor for clinical radiology. Jamia - Journal of the American Medical Informatics Association. 1994; 1 (2):161. doi: 10.1136/jamia.1994.95236146. [ PMC free article ] [ PubMed ] [ CrossRef ] [ Google Scholar ]
- Haug P, Koehler S, Lau L, Wang P, Rocha R, Huff S. A natural language understanding system combining syntactic and semantic techniques. Proc Annu Symp Comput Appl Med Care. 1994. pp. 247–251. [ PMC free article ] [ PubMed ]
- Hahn U, Romacker M, Schulz S. MEDSYNDIKATE: a natural language system for the extraction of medical information from finding reports. Int J Med Inform. 2002; 67 (1/3):63–74. [ PubMed ] [ Google Scholar ]
- Kullo IJ, Fan J, Pathak J, Savova GK, Ali Z, Chute CG. Leveraging informatics for genetic studies: use of the electronic medical record to enable a genome-wide association study of peripheral arterial disease. J Am Med Inform Assoc. 2010; 17 (5):568–574. doi: 10.1136/jamia.2010.004366. [ PMC free article ] [ PubMed ] [ CrossRef ] [ Google Scholar ]
- Kho A, Pacheco J, Peissig P, Rasmussen L, Newton K, Weston N, Crane P, Pathak J, Chute C, Bielinski S. Electronic Medical Records for Genetic Research: Results of the eMERGE Consortium. Sci Transl Med. 2011; 3 (79):79re71. [ PMC free article ] [ PubMed ] [ Google Scholar ]
- Kohane IS. Using electronic health records to drive discovery in disease genomics. Nat Rev Genet. 2011; 12 (6):417–428. doi: 10.1038/nrg2999. [ PubMed ] [ CrossRef ] [ Google Scholar ]
- Tatonetti N, Denny J, Murphy S, Fernald G, Krishnan G, Castro V, Yue P, Tsau P, Kohane I, Roden D. et al. Detecting Drug Interactions From Adverse-Event Reports: Interaction Between Paroxetine and Pravastatin Increases Blood Glucose Levels. Clin Pharmacol Ther. 2011; 90 (1):133–142. doi: 10.1038/clpt.2011.83. [ PMC free article ] [ PubMed ] [ CrossRef ] [ Google Scholar ]
- Wang X, Hripcsak G, Markatou M, Friedman C. Active Computerized Pharmacovigilance Using Natural Language Processing, Statistics, and Electronic Health Records: A Feasibility Study. J Am Med Inform Assoc. 2009; 16 (3):328–337. doi: 10.1197/jamia.M3028. [ PMC free article ] [ PubMed ] [ CrossRef ] [ Google Scholar ]
- Hirschtick R. A piece of my mind. Copy-and-paste. . JAMA. 2006; 295 (20):2335–2336. doi: 10.1001/jama.295.20.2335. [ PubMed ] [ CrossRef ] [ Google Scholar ]
- Yackel TR, Embi PJ. Copy-and-paste-and-paste. JAMA. 2006; 296 (19):2315. [ PubMed ] [ Google Scholar ]
- O’Donnell HC, Kaushal R, Barrón Y, Callahan MA, Adelman RD, Siegler EL. Physicians’ Attitudes Towards Copy and Pasting in Electronic Note Writing. J Gen Intern Med. 2009; 24 (1):63–68. doi: 10.1007/s11606-008-0843-2. [ PMC free article ] [ PubMed ] [ CrossRef ] [ Google Scholar ]
- Siegler EL, Adelman R. Copy and Paste: A Remediable Hazard of Electronic Health Records. Am J Med. 2009; 122 (6):495–496. doi: 10.1016/j.amjmed.2009.02.010. [ PubMed ] [ CrossRef ] [ Google Scholar ]
- Markel A. Copy and Paste of Electronic Health Records: A Modern Medical Illness. Am J Med. 2010; 123 (5):e9. doi: 10.1016/j.amjmed.2009.10.012. [ PubMed ] [ CrossRef ] [ Google Scholar ]
- Wrenn JO, Stein DM, Bakken S, Stetson PD. Quantifying clinical narrative redundancy in an electronic health record. J Am Med Inform Assoc. 2010; 17 (1):49. doi: 10.1197/jamia.M3390. [ PMC free article ] [ PubMed ] [ CrossRef ] [ Google Scholar ]
- Zhang R, Pakhomov S, McInnes BT, Melton GB. Evaluating Measures of Redundancy in Clinical Texts. Proc AMIA: 2011; 2011 :1612–1620. [ PMC free article ] [ PubMed ] [ Google Scholar ]
- Lin CY. Rouge: A package for automatic evaluation of summaries. 2004. pp. 74–81. (Text Summarization Branches Out: Proceedings of the ACL-04 Workshop: 2004).
- Altschul SF, Gish W, Miller W, Myers EW, Lipman DJ. Basic local alignment search tool. J Mol Biol. 1990; 215 (3):403–410. [ PubMed ] [ Google Scholar ]
- Manning CD, Schutze H. Foundations of statistical natural language processing. MIT Press, Cambridge MA; 1999. pp. 151–190. [ Google Scholar ]
- Joshi M, Pakhomov S, Pedersen T, Chute CG. A comparative study of supervised learning as applied to acronym expansion in clinical reports. American Medical Informatics Association; 2006. p. 399. (AMIA Annual Symposium Proceedings: 2006). [ PMC free article ] [ PubMed ] [ Google Scholar ]
- Joshi M, Pedersen T, Maclin R. A comparative study of support vector machines applied to the supervised word sense disambiguation problem in the medical domain. 2005. pp. 3449–3468. (Proceedings of the 2nd Indian International Conference on Artificial Intelligence (IICAI’05): 2005).
- Inniss TR, Lee JR, Light M, Grassi MA, Thomas G, Williams AB. Proceedings of the 1st international workshop on Text mining in bioinformatics: 2006. ACM; 2006. Towards applying text mining and natural language processing for biomedical ontology acquisition; pp. 7–14. [ Google Scholar ]
- McInnes BT, Pedersen T, Pakhomov SV. Proceedings of the Workshop on BioNLP 2007: Biological, Translational, and Clinical Language Processing: 2007. Association for Computational Linguistics; 2007. Determining the syntactic structure of medical terms in clinical notes; pp. 9–16. [ Google Scholar ]
- Zhou G, Zhao J, Liu K, Cai L. Exploiting web-derived selectional preference to improve statistical dependency parsing. Proceedings of ACL: 2011; 2011 :1556–1565. [ Google Scholar ]
- Chen HB, Huang HH, Tan CT, Tjiu J, Chen HH. A statistical medical summary translation system. ACM; 2012. pp. 101–110. (Proceedings of the 2nd ACM SIGHIT symposium on International health informatics: 2012). [ Google Scholar ]
- Zeng QT, Crowell J. Semantic classification of consumer health content. MEDNET Retrieved May. 2008; 2006 :19. [ Google Scholar ]
- Jiang Y. A computational semantics system for detecting drug reactions and patient outcomes in personal health messages. University of Illinois at Urbana-Champaign, Urbana-Champaign; 2011. [ Google Scholar ]
- Blei DM, Ng AY, Jordan MI. Latent dirichlet allocation. J Mach Learn Res. 2003; 3 :993–1022. [ Google Scholar ]
- Arnold CW, El-Saden SM, Bui AAT, Taira R. Clinical Case-based Retrieval Using Latent Topic Analysis. American Medical Informatics Association; 2010. p. 26. [ PMC free article ] [ PubMed ] [ Google Scholar ]
- Perotte A, Bartlett N, Elhadad N, Wood F. Hierarchically Supervised Latent Dirichlet Allocation. 2011. (NIPS: 2011).
- Bisgin H, Liu Z, Fang H, Xu X, Tong W. Mining FDA drug labels using an unsupervised learning technique - topic modeling. BMC Bioinforma. 2011; 12 (Suppl 10):S11. doi: 10.1186/1471-2105-12-S10-S11. [ PMC free article ] [ PubMed ] [ CrossRef ] [ Google Scholar ]
- Banko M, Brill E. Mitigating the paucity-of-data problem: Exploring the effect of training corpus size on classifier performance for natural language processing. Association for Computational Linguistics; 2001. pp. 1–5. [ Google Scholar ]
- Kilgarriff A, Grefenstette G. Introduction to the special issue on the web as corpus. Computational linguistics. 2003; 29 (3):333–347. doi: 10.1162/089120103322711569. [ CrossRef ] [ Google Scholar ]
- Atterer M, Sch tze H. The effect of corpus size in combining supervised and unsupervised training for disambiguation. Association for Computational Linguistics; 2006. pp. 25–32. [ Google Scholar ]
- Halevy A, Norvig P, Pereira F. The unreasonable effectiveness of data. Intelligent Systems, IEEE. 2009; 24 (2):8–12. [ Google Scholar ]
- Dredze M, Blitzer J, Talukdar PP, Ganchev K, Graca J, Pereira F. Frustratingly hard domain adaptation for dependency parsing. 2007. pp. 1051–1055.
- Dredze M, Kulesza A, Crammer K. Multi-domain learning by confidence-weighted parameter combination. Mach Learn. 2010; 79 (1):123–149. doi: 10.1007/s10994-009-5148-0. [ CrossRef ] [ Google Scholar ]
- Blitzer J, Dredze M, Pereira F. Biographies, bollywood, boom-boxes and blenders: Domain adaptation for sentiment classification. 2007. p. 440.
- Moore RC, Lewis W. Intelligent selection of language model training data. Association for Computational Linguistics; 2010. pp. 220–224. [ Google Scholar ]
- Downey D, Etzioni O, Soderland S. Analysis of a probabilistic model of redundancy in unsupervised information extraction. Artif Intell. 2010; 174 (11):726–748. doi: 10.1016/j.artint.2010.04.024. [ CrossRef ] [ Google Scholar ]
- Altschul SF, Madden TL, Schäffer AA, Zhang J, Zhang Z, Miller W, Lipman DJ. Gapped BLAST and PSI-BLAST: a new generation of protein database search programs. Nucleic Acids Res. 1997; 25 (17):3389–3402. doi: 10.1093/nar/25.17.3389. [ PMC free article ] [ PubMed ] [ CrossRef ] [ Google Scholar ]
- Pearson WR. [5] Rapid and sensitive sequence comparison with FASTP and FASTA. Academic Press; 1990. pp. 63–98. (Methods in Enzymology. vol. Volume 183). [ PubMed ] [ Google Scholar ]
- HaCohen-Kerner Y, Tayeb A, Ben-Dror N. Detection of simple plagiarism in computer science papers. Association for Computational Linguistics; 2010. pp. 421–429. [ Google Scholar ]
- Holm L, Sander C. Removing near-neighbour redundancy from large protein sequence collections. Bioinformatics. 1998; 14 (5):423. doi: 10.1093/bioinformatics/14.5.423. [ PubMed ] [ CrossRef ] [ Google Scholar ]
- Bateman A, Birney E, Durbin R, Eddy SR, Howe KL, Sonnhammer ELL. The Pfam protein families database. Nucleic Acids Res. 2000; 28 (1):263. doi: 10.1093/nar/28.1.263. [ PMC free article ] [ PubMed ] [ CrossRef ] [ Google Scholar ]
- Li W. Random texts exhibit Zipf’s-law-like word frequency distribution. Information Theory, IEEE Transactions on. 1992; 38 (6):1842–1845. doi: 10.1109/18.165464. [ CrossRef ] [ Google Scholar ]
- Yoshimasa Tsuruoka YT, Jin-Dong K, Tomoko O, Sophia A, Jun’ichi T. Developing a Robust Part-of-Speech Tagger for Biomedical Text. 2005. (Lecture Notes in Computer Science).
- Baldridge J, Morton T, Bierner G. The opennlp maximum entropy package. 2002. (Technical report, SourceForge).
- Teufel S, Elhadad N. Collection and Linguistic Processing of a Large-scale Corpus of Medical Articles. LREC: 2002; 2002. pp. 1214–1218. [ Google Scholar ]
- Bodenreider O. The unified medical language system (UMLS): integrating biomedical terminology. Nucleic Acids Res. 2004; 32 :D267. doi: 10.1093/nar/gkh061. [ PMC free article ] [ PubMed ] [ CrossRef ] [ Google Scholar ]
- Gildea D. Corpus variation and parser performance. Citeseer; 2001. pp. 167–202. [ Google Scholar ]
- Tsuruoka Y, Tateishi Y, Kim JD, Ohta T, McNaught J, Ananiadou S, Tsujii J. Developing a robust part-of-speech tagger for biomedical text. Advances in informatics. 2005; LNCS 3746 :382–392. [ Google Scholar ]
- Smith TF, Waterman MS, Fitch WM. Comparative biosequence metrics. J Mol Evol. 1981; 18 (1):38–46. doi: 10.1007/BF01733210. [ PubMed ] [ CrossRef ] [ Google Scholar ]
- Banerjee S, Pedersen T. The design, implementation, and use of the ngram statistics package. 2003. pp. 370–381. (Computational Linguistics and Intelligent Text Processing).
- Wallach HM, Murray I, Salakhutdinov R, Mimno D. Evaluation methods for topic models. ACM; 2009. pp. 1105–1112. [ Google Scholar ]
- McCallum AK. Mallet: A machine learning for language toolkit. 2002.
- Wallach H, Mimno D, McCallum A. Rethinking LDA: Why priors matter. Advances in Neural Information Processing Systems. 2009; 22 :1973–1981. [ Google Scholar ]
- Uzuner O. Second i2b2 workshop on natural language processing challenges for clinical records. 2008. p. 1252. [ PubMed ]
- Cormode G, Hadjieleftheriou M. Finding frequent items in data streams. Proceedings of the VLDB Endowment. 2008; 1 (2):1530–1541. [ Google Scholar ]
- Open access
- Published: 15 November 2022
Meta-research evaluating redundancy and use of systematic reviews when planning new studies in health research: a scoping review
- Hans Lund ORCID: orcid.org/0000-0001-6847-8324 1 ,
- Karen A. Robinson ORCID: orcid.org/0000-0003-1021-7820 1 , 2 ,
- Ane Gjerland ORCID: orcid.org/0000-0001-7496-9568 1 ,
- Hanna Nykvist ORCID: orcid.org/0000-0002-1642-2414 1 ,
- Thea Marie Drachen ORCID: orcid.org/0000-0003-4760-5536 3 ,
- Robin Christensen ORCID: orcid.org/0000-0002-6600-0631 4 , 5 ,
- Carsten Bogh Juhl ORCID: orcid.org/0000-0001-8456-5364 6 , 7 ,
- Gro Jamtvedt ORCID: orcid.org/0000-0001-6013-7429 8 ,
- Monica Nortvedt ORCID: orcid.org/0000-0002-1859-6071 9 ,
- Merete Bjerrum ORCID: orcid.org/0000-0001-8249-4021 10 , 11 , 12 ,
- Matt Westmore ORCID: orcid.org/0000-0003-3784-0380 13 ,
- Jennifer Yost ORCID: orcid.org/0000-0002-3170-1956 14 &
- Klara Brunnhuber ORCID: orcid.org/0000-0001-6787-4405 15
on behalf of the Evidence-Based Research Network
Systematic Reviews volume 11 , Article number: 241 ( 2022 ) Cite this article
3660 Accesses
6 Citations
2 Altmetric
Metrics details
Several studies have documented the production of wasteful research, defined as research of no scientific importance and/or not meeting societal needs. We argue that this redundancy in research may to a large degree be due to the lack of a systematic evaluation of the best available evidence and/or of studies assessing societal needs.
The aim of this scoping review is to (A) identify meta-research studies evaluating if redundancy is present within biomedical research, and if so, assessing the prevalence of such redundancy, and (B) to identify meta-research studies evaluating if researchers had been trying to minimise or avoid redundancy.
Eligibility criteria
Meta-research studies (empirical studies) were eligible if they evaluated whether redundancy was present and to what degree; whether health researchers referred to all earlier similar studies when justifying and designing a new study and/or when placing new results in the context of earlier similar trials; and whether health researchers systematically and transparently considered end users’ perspectives when justifying and designing a new study.
Sources of evidence
The initial overall search was conducted in MEDLINE, Embase via Ovid, CINAHL, Web of Science, Social Sciences Citation Index, Arts & Humanities Citation Index, and the Cochrane Methodology Register from inception to June 2015. A 2nd search included MEDLINE and Embase via Ovid and covered January 2015 to 26 May 2021. No publication date or language restrictions were applied.
Charting methods
Charting methods included description of the included studies, bibliometric mapping, and presentation of possible research gaps in the identified meta-research.
We identified 69 meta-research studies. Thirty-four (49%) of these evaluated the prevalence of redundancy and 42 (61%) studies evaluated the prevalence of a systematic and transparent use of earlier similar studies when justifying and designing new studies, and/or when placing new results in context, with seven (10%) studies addressing both aspects. Only one (1%) study assessed if the perspectives of end users had been used to inform the justification and design of a new study. Among the included meta-research studies evaluating whether redundancy was present, only two of nine health domains (medical areas) and only two of 10 research topics (different methodological types) were represented. Similarly, among the included meta-research studies evaluating whether researchers had been trying to minimise or avoid redundancy, only one of nine health domains and only one of 10 research topics were represented.
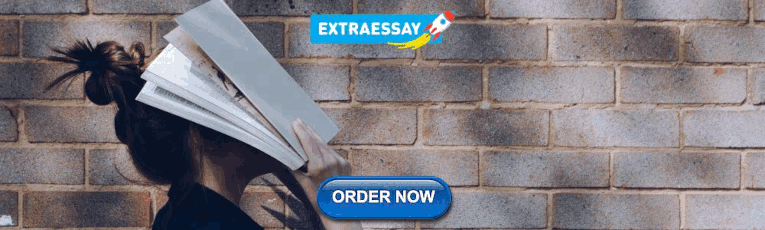
Conclusions that relate to the review questions and objectives
Even with 69 included meta-research studies, there was a lack of information for most health domains and research topics. However, as most included studies were evaluating across different domains, there is a clear indication of a high prevalence of redundancy and a low prevalence of trying to minimise or avoid redundancy. In addition, only one meta-research study evaluated whether the perspectives of end users were used to inform the justification and design of a new study.
Systematic review registration
Protocol registered at Open Science Framework: https://osf.io/3rdua/ (15 June 2021).
Peer Review reports
Introduction
Science is cumulative; every new study should be planned, performed, and interpreted in the context of earlier studies ([ 1 ]; evbres.eu). At least this is how the ideal of science has been described [ 2 , 3 , 4 ]. Whether this ideal was being realised in science was publicly questioned as early as 1884 when Lord Rayleigh stated that “The work which deserves, but I am afraid does not always receive, the most credit is that in which discovery and explanation go hand in hand, in which not only are new facts presented, but their relation to old ones is pointed out” [ 5 ]. The lack of consideration of earlier studies when conducting new studies was analysed in a cumulative meta-analysis in 1992 by Lau et al. [ 6 ] who “found that a consistent, statistically significant reduction in total mortality ... was achieved in 1973, after only eight trials involving 2432 patients had been completed. The results of the 25 subsequent trials, which enrolled an additional 34,542 patients through 1988, had little or no effect on the odds ratio establishing efficacy, but simply narrowed the 95 percent confidence interval”. In the following years, several studies were published indicating that redundant and unnecessary studies have been conducted within different clinical areas such as cardiac diseases [ 7 , 8 ], low back pain [ 9 ], dermatology [ 10 ], lung cancer [ 11 ], and dentistry [ 12 ].
In 2009, Robinson defended her doctoral thesis that showed that authors very rarely consider all earlier studies, but instead referring to only a small fraction of them or none at all [ 13 , 14 ]. As Robinson wrote: “To limit bias, all relevant studies must be identified and considered in a synthesis of existing evidence. While the use of research synthesis to make evidence-informed decisions is now expected in health care, there is also a need for clinical trials to be conducted in a way that is evidence-based. Evidence-based research [emphasised here] is one way to reduce waste in the production and reporting of trials, through the initiation of trials that are needed to address outstanding questions and through the design of new trials in a way that maximises the information gained” [ 13 ]. Shortly after, an international network was established to promote an “Evidence-Based Research” (EBR) approach, that is, “the use of prior research in a systematic and transparent way to inform a new study so that it is answering questions that matter in a valid, efficient and accessible manner” (See: evbres.eu).
In a landmark series published in The Lancet in 2014, a group of researchers presented an overview of possible reasons for waste or inefficiency in biomedical research [ 15 ]. They described five overall areas of concern: (1) research decisions not based on questions relevant to users of research; (2) inappropriate research design, methods, and analysis; (3) inefficient research regulation and management; (4) not fully accessible research information; and (5) biased and not usable research reports. Several of these reasons for waste or inefficient research can be related to a lack of evidence-based research, with researchers addressing low-priority research, not assessing important outcomes, rarely using systematic reviews (SRs) to inform the design of a new study, and new results not being interpreted in the context of the existing evidence base. In this paper, we refer to such failings as questionable research practices (QRPs) [ 16 ]. A QRP does not constitute “research misconduct”, i.e. fabrication or falsification of data and plagiarism, but a failure to align with the principles of scientific integrity.
Even though numerous factors can influence whether researchers perform and publish unnecessary research, in this scoping review we have chosen to focus on meta-research studies evaluating the frequency and characteristics of the following QRPs: (A) authors publishing redundant studies; (B) authors not using the results of a systematic and transparent collection of earlier similar studies when justifying a new study; (C) authors not using the results of a systematic and transparent collection of earlier similar studies when designing a new study; (D) authors not systematically and transparently placing new results in the context of existing evidence; and (E) authors not systematically and transparently using end user’s perspectives to inform the justification of new studies, the design of new studies, or the interpretation of new results.
Our search identified no previous scoping review of meta-research studies evaluating redundancy in biomedical research. As the first scoping review of its kind, our aim was to (A) identify meta-research studies evaluating if redundancy was present, and the prevalence of redundancy within biomedical research, and (B) to identify meta-research studies evaluating if researchers had been trying to minimise or avoid redundancy. It was further our intention to examine the extent, variety, and characteristics of included meta-research studies to identify any research gaps that could be covered by future meta-research.
Protocol and registration
A protocol was registered at Open Science Framework: https://osf.io/3rdua/ (15 June 2021). The reporting of this scoping review follows the PRISMA extension for scoping reviews [ 17 ] (see also Additional File 4 _PRISMA Checklist for Scoping Reviews filled in).
We included meta-research studies evaluating the presence and characteristics of the following QRPs: (A) authors publishing redundant studies; (B) authors not using the results of a systematic and transparent collection of earlier similar studies when justifying a new study; (C) authors not using the results of a systematic and transparent collection of earlier similar studies when designing a new study; (D) authors not systematically and transparently placing new results in the context of existing evidence; and (E) authors not systematically and transparently using end user’s perspectives to inform the justification of new studies, the design of new studies, or the interpretation of new results.
We did not define redundancy ourselves but noted the definitions the study authors were using.
Information sources and search
The initial overall search was conducted in MEDLINE via both PubMed and Ovid, Embase via Ovid, CINAHL via EBSCO, Web of Science (Science Citation Index Expanded (SCI-EXPANDED), Social Sciences Citation Index (SSCI), Arts & Humanities Citation Index (A&HCI), and Cochrane Methodology Register (CMR, Methods Studies) from inception to June 2015. No restrictions on publication date and language were applied.
As there are no standard search terms for meta-research studies (many such studies never used the word “meta-research” or its synonyms) nor for studies evaluating the use of an evidence-based research approach, the first search results were sensitive but lacked precision. A second, more focused search was performed in May 2021 in MEDLINE and Embase via Ovid covering the period from January 2015 to 26 May 2021. To evaluate the recall of the 2nd search strategy, we ran this new search strategy also for the timeframe up to 2015 and found that it would have picked up all studies we had included from our first search. For a more detailed description of the 1st search, see Appendix 1 , and Appendix 2 for the 2nd search.
In addition, we checked reference lists of all included studies and asked experts within the field for any missed relevant literature.
Selection of sources of evidence
The search results were independently screened by two people who resolved disagreements on study selection by consensus and as needed, by discussion with a third reviewer. Full-text screening was performed by four reviewers independently who resolved disagreements on study selection by consensus and discussion.
Data charting process
We developed and pilot tested a data-charting form. Two reviewers independently extracted data, discussed the data, and continuously updated the data charting form in an iterative process.
All studies were categorised according to the following framework that distinguishes between meta-research studies evaluating the presence of the problem, redundant and unnecessary studies, and studies evaluating whether an evidence-based research (EBR) approach had been used (see Table 1 ).
The data-charting form also included information related to the publication (i.e. authors, publication year, country of 1st author, journal, and publishing house), the topic (health domain), data material, overall design, and methods (e.g. sources of data, outcomes), results, and conclusions.
The author names were used to create a bibliometric map in the software VOSViewer (Leiden University’s Centre for Science and Technology Studies (CWTS), VOSviewer version 1.6.17). The map visualised the diversity of published studies, i.e. whether the meta-research identified was published by a small group of authors. The map displays one node/circle for each of the authors included in this review. Larger nodes indicate more relevant articles published by that particular author, and each node is linked with the nodes of its co-authors. On the large map, this linking is illustrated by the nodes being placed close together, so that they form an “island”. Thicker lines between authors mean more papers written together.
Research gaps in meta-research
To identify research gaps within meta-research related to evidence-based research, we (A) counted the number of studies evaluating each of the QRPs listed in the section “Eligibility criteria”; (B) combined a list of research topics with the three main aspects of evidence-based research: justification (Justification) and design (Design) of a new study and the interpretation of the new results in context (Context), as well as measurement of redundancy; (C) combined a list of data materials being used in the meta-research studies with the above-mentioned three aspects of evidence-based research, as well as redundancy; and (D) identified the type of health domains covered by redundancy and the three aspects of evidence-based research.
Selection of evidence sources
We identified a total of 30,592 unique citations and included 69 original meta-research studies in our analysis (see Fig. 1 ; Additional Material 11 ).
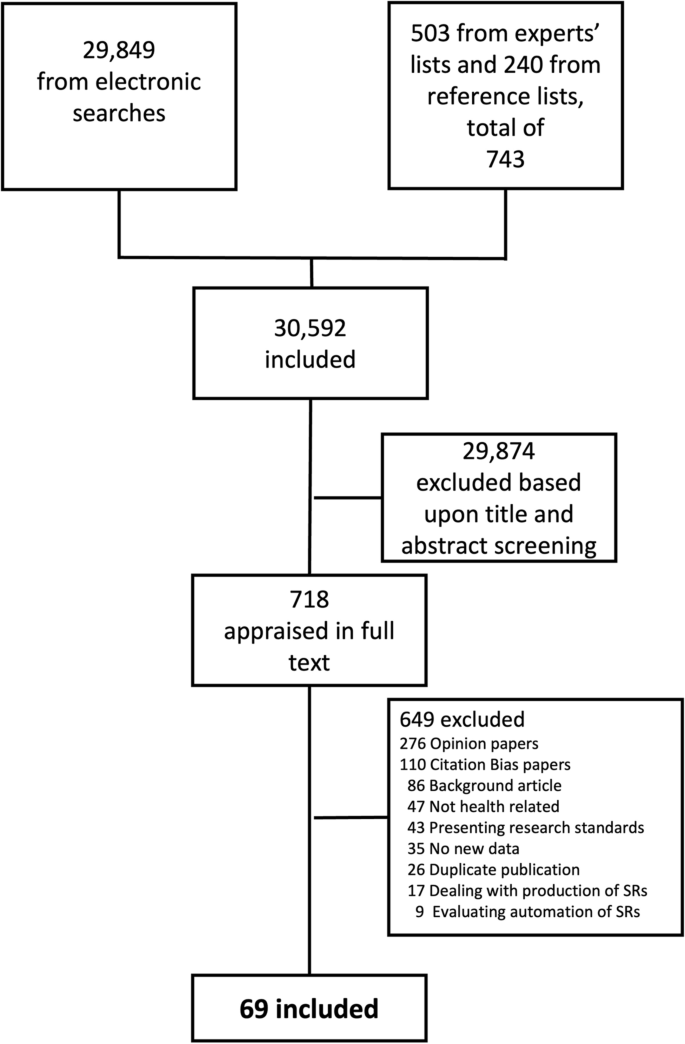
PRISMA flowchart
Characteristics of evidence sources
The specific characteristics of the included studies are presented in Table 2 . The studies were published in the period from 1981 to 2021, with a peak of 10 studies published in 2017 (Supplementary Material 1 ). Only one study was published in the 1980s, while the majority (60 studies; 87%) were published after 2000.
The first authors of the included studies were based at institutions in 13 different countries. Fifty-eight percent of identified studies were published in the UK and USA (20 from each) (see Supplementary Material 2 ).
The included studies evaluated various health domains (Table 5 ), with a large proportion of studies (28 studies, 40.6% of all) cutting across all medical specialties.
A Venn diagram was used to indicate the number of studies investigating if authors were publishing redundant studies, if authors were using an EBR approach to avoid QRPs and any combination hereof (Fig. 2 ).
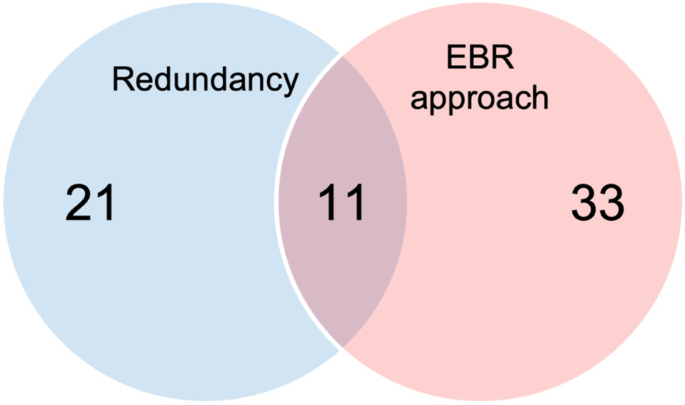
Venn diagram indicating the number of studies that evaluated either redundancy, use of the EBR approach, or both
The information about data material, overall designs, used methods, and metrics, as planned (see Table 1 ), was identified and is listed in Table 3 .
In studies evaluating redundancy, three metrics were used for content analyses (see Table 3 ). The number of overlapping meta-analyses refers to meta-research studies identifying systematic reviews that cover the same topic and hence overlap with each other. One of the included meta-research studies stated that “Systematic reviews often provide a research agenda to guide future research” [ 19 ]; thus, meta-research studies could use this as a metric, i.e. evaluate if authors made any changes to the trial design after publication of the research agenda. The majority of the studies evaluating redundancy used cumulative meta-analyses in one way or another; thus, the overall metric would be a description of cumulative meta-analyses indicating redundancy (see also Supplementary Material 3 ).
A crucial element in using a cumulative meta-analysis is the selection of cut-offs, i.e. the factor determining if a study would be considered to be redundant. A cut-off could also be used without performing a cumulative meta-analysis, but instead stating that “redundant clinical trials were defined as randomized clinical trials that initiated or continued recruiting after 2008” where a clinical guideline was published [ 21 ]. Different criteria were used for cut-off analyses, with four of them using cumulative meta-analysis ( p- value, visual inspection of a forest plot, trial sequential analysis, and failsafe ratio), and the others either extended funnel plot, number of similar trials published after a trial is stopped early for benefit, number of studies published after established “high” certainty of evidence, or the number of studies published after established guidelines had established certainty of evidence (see also Supplementary Material 3 ).
The number of studies using different cut-off criteria was too low to identify potential differences by cut-off type. For an overview of the data materials, study designs, and study methods used in studies evaluating redundancy and the use of an EBR approach to minimise or avoid redundancy, see Supplementary Materials 7 – 9 .
In studies evaluating the use of an evidence-based research approach to minimise or avoid redundancy, eight different metrics were used to perform a citation analysis (see Table 3 and Supplementary Material 4 ). Furthermore, ten metrics were used to perform a content analysis (see Table 3 and Supplementary Material 4 ).
Only one study performed a survey asking researchers about the use of SRs when justifying and designing new studies (see Supplementary Material 4 ).
The studies evaluating the use of the EBR approach to minimise or avoid redundancy evaluated three different aspects; (A) authors do not use the results of a systematic and transparent collection of earlier similar studies when justifying a new study; (B) authors do not use the results of a systematic and transparent collection of earlier similar studies when designing a new study; and (C) authors do not systematically and transparently place new results in the context of existing evidence. Figure 3 indicates the number of studies evaluating each of these questionable research practices. As only one study evaluated whether authors used the results of a systematic and transparent collection of the new research projects’ end user’s perspectives to inform the justification and design of the new study, it was not included in Fig. 3 .
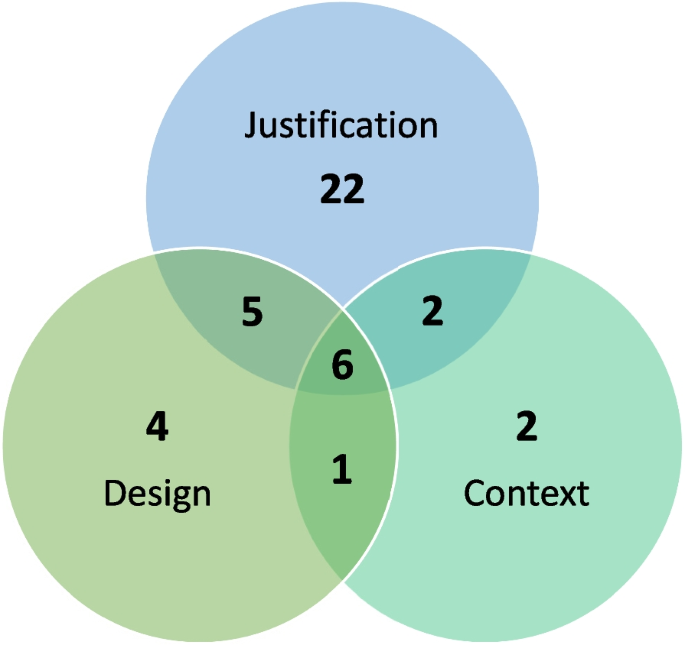
Venn diagram indicating the number of studies that investigated the following questionable research practices; “Justification”—authors do not use the results of a systematic and transparent collection of earlier similar studies when justifying a new study; “Design”—authors do not use the results of a systematic and transparent collection of earlier similar studies when designing a new study; and “Context”—authors do not systematically and transparently place new results in the context of existing evidence, and any combinations thereof. Ten of the studies investigating whether authors use the results of a systematic and transparent collection of earlier similar studies when justifying a new study also evaluated whether authors of a scientific study referred to all earlier similar studies. One of the six studies in the middle section also investigated whether authors used the results of a systematic and transparent collection of the new research projects’ end user’s perspectives to inform the justification and design of the new study
Bibliographic mapping
Bibliographic mapping revealed 41 independent author groups that had conducted the included meta-research studies (see Fig. 4 ). Because of the high number of co-author islands ( n = 41), the total map is quite large. The two smaller maps (see Supplementary Material 5 and 6 ) have zoomed in on the two largest islands in terms of the number of published papers.
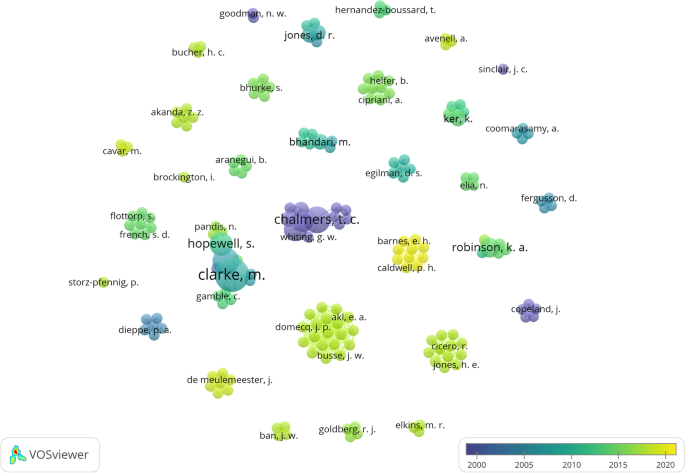
Bibliometric map
This bibliographic mapping of included studies indicates that only a small number of studies were published with the same authors involved. Most studies were conducted by groups of researchers—and some individual researchers—working in isolation from each other (see also Table 2 ). Still, we cannot exclude the possibility that some of these islands may be part of larger collegial groups and that some of the authors from different islands may have co-authored papers on topics not included in this scoping review. Based on the current findings, however, there is no indication that the identified studies were published by a small group of authors and/or research groups.
Research gaps
Tables 4 , 5 , and 6 present the types of research gaps relating to the methods used in the included meta-research studies.
Table 4 presents the number of studies evaluating the four QRPs (see above). Only one study evaluated whether authors used the results of a systematic and transparent collection of end users’ perspectives to inform the justification and design of new studies. Tables 5 and 6 present lists of research topics and health domains combined with the three aspects of evidence-based research: justification (Justification) and design (Design) of a new study and the interpretation of the new results in context (Context), as well as measurement of redundancy. The list of research topics was inspired by Bourne et al. [ 23 ]. The list of health domains was based on Cochrane’s eight Review Group Networks ( Cochrane.org ). Additional tables (see Supplementary Material ) combine lists of the data materials, study designs, and analysis methods used in the meta-research studies with the three aspects of evidence-based research, as well as redundancy.
Fields marked in the tables in light green indicate that the relevant issues had been evaluated by several studies (6 or more), with light red indicating only a few studies (5 or less), and fields marked in red indicate that no studies had been identified. The sum of studies listed in the tables is higher than the total number of included studies because several studies have evaluated more than one questionable research practice as part of the same study.
Conclusions of included studies
We prepared a list of conclusions extracted from the included studies (See Supplementary Material 10 ). Twenty-three studies had concluded that redundancy was present among similar clinical studies, and three studies had identified redundancy among similar SRs. Fifteen studies reported no or poor use of SRs to inform justification of a new study, while six studies showed no or poor use of SRs to inform design, and seven studies demonstrated no or poor use of SRs when placing new results in the context of existing research.
We identified 34 meta-research studies that evaluated whether redundancy existed among similar studies and 42 studies that evaluated whether authors of clinical studies had used a systematic and transparent approach to avoid redundancy in health research, with seven studies addressing both aspects. There is a clear indication of high prevalence of redundancy and low prevalence that researchers had tried to minimise or avoid redundancy from 28 studies evaluating across different medical specialties.
Despite the 69 meta-research studies included in this scoping review, there is a dearth of information for most health domains and research topics. Only a single meta-research study evaluated whether end users were involved in the justification and design of a new study [ 18 ]. Almost all meta-research studies focused on research evaluating the effect of a treatment. Only six studies evaluated research dealing with questions on epidemiology or disease burden, five with disease prevention, and only one with diagnostic issues. This means that a large number of research topics have never been evaluated in relation to the possibility of redundancy in research or whether researchers have used a systematic and transparent approach when justifying and designing new studies and when placing new results in the context of existing evidence, including aetiology, natural history, outcomes, economic evaluations, implementation, and health services and system.
Most meta-research had analysed published papers of original studies (typically treatment evaluation studies), while very few focused on other sorts of research documents such as funding and ethic committee proposals or published protocols. Only four studies explored redundancy in the production of SRs, and none evaluated whether researchers had used a systematic and transparent approach when justifying and designing a new SR, or when placing new results in the context of the existing evidence. Finally, studies applied widely varying methods (cut-off points) and definitions to evaluate whether redundancy was present. A frequency statistic approach was used most often, whereas no study utilised a Bayesian approach.
The seriousness of the problem evaluated in the present scoping review is highlighted in the conclusions of the studies and can be summed up in the following results: Evidence shows that researchers make no or poor use of SRs when justifying and/or designing new studies, or when placing new study results in the context of existing similar research.
Strengths and limitations of this scoping review
Both the long time it took to prepare this scoping review and the large number of hits in the literature search (>30.000 hits) indicate the immense challenges of identifying relevant studies. This is further supported by the high proportion of studies identified via direct contact with experts, reading of reference lists, and additional citation searches. The reasons are at least two-fold. First, it remains difficult to identify research-on-research studies, partly due to the lack of a standardised naming convention for these kind of studies (for example: meta-epidemiology, research on research, meta-research, metascience, and science of science) and the fact that many authors never define their studies as meta-research studies in the first place. Secondly, it is an even greater challenge to identify studies evaluating the specific topics related to the only recently defined evidence-based research concept (i.e. studies identifying redundancy or unnecessary studies, and studies evaluating whether authors are using a systematic and transparent approach when justifying and designing new studies, and when placing new results in the context of the existing evidence). As the presence of our two search strategies indicates, we had to undertake initial searching and screening before we could prepare a sufficiently precise search strategy (see appendix 1 and 2 ).
Thanks to our extensive literature searches, we are assured that this is indeed the first scoping review of meta-research evaluating redundancy in health research and different ways to minimise such redundancy.
We deemed the problem of citation bias as beyond the scope of this scoping review. However, identifying studies that evaluate the reasons why researchers select references in their publications could provide important answers as to the root causes of redundancy and why researchers rarely use a systematic and transparent approach when planning and interpreting new studies. Hence, a further scoping review is in preparation that focuses on studies evaluating citation bias and other biases related to the citing of other publications.
It is also beyond the aim of a scoping review to report the size of reported QRPs, but the fact that a large and very diverse sample of meta-research studies showed that an evidence-based research approach is rarely used indicates that a fundamental problem exists with the way new research is currently planned and interpreted. This is corroborated by the finding that all identified studies consistently reported the same lack of using a systematic and transparent approach when justifying and designing new studies or when placing new findings in the context of the existing body of knowledge, even though these studies had been prepared by a large and diverse group of authors covering many health domains.
As almost all identified studies consistently showed redundancy or poor use of the evidence-based research approach, publication bias cannot be ruled out. It is possible that studies with positive results, i.e. identifying redundancy or bad behaviour, were more likely to have been published. We identified only two studies that did not report a problem: Ker 2015 [ 22 ] and Hoderlein 2017 [ 20 ]. The first of these studies found no reason to assume that the QRP of authors not using the results of a systematic and transparent collection of earlier similar studies when justifying a new study was present as the authors argued that the low quality of earlier studies limited generalisability of results and hence justified yet another study [ 22 ]. It is of note, however, that the number of new studies actually increased after a SR was published, and that, as the authors pointed out, “over half of trials cited at least one of the existing SRs suggests that ignorance of the existing evidence does not fully explain ongoing trial activity” [ 22 ]. The authors also argued that new studies were justified as new patient groups had been added in the clinic to use the treatment. The possibility that authors citing the SR were not utilising it to justify the new study was not considered. To evaluate this aspect, the authors of the meta-research studies would not only need to read each Background or Discussion section but also interpret the sentences related to citing a SR. This would require not only careful text analysis but also interviews with the authors themselves to find out about their reasons for selecting cited references. Based on the results from earlier studies, these reasons can be manyfold, with only a few related to justifying or designing a new study [ 24 , 25 ].
Implications for research practice
This scoping review does not comprehensively evaluate all reasons for redundant research, but our results clearly indicate that researchers hardly ever use a systematic and transparent (“Evidence-Based Research”) approach when planning and interpreting new studies. Even though this explains to a large extent the publication of redundant studies, it is unclear why researchers, who had been trained to be systematic and transparent in everything they do while performing research, are not being similarly systematic and transparent during the planning and interpreting phases of the research process. One reason could be a lack of knowledge about the problem, calling for further education of researchers. Such educational programmes (already taking place in EVBRES (evbres.eu)) should include modules that increase learners’ understanding of the need for systematic reviews and how to use them to inform the justification and design of studies, and when interpreting new results in the context of existing evidence.
Implications for future meta-research
Our analyses showed that only one meta-research study evaluated the inclusion of end users’ perspectives when justifying or designing a new study. Many more studies are needed to evaluate end user involvement in these fundamental research aspects and how end users’ perspectives can be best obtained. Furthermore, only a few studies evaluated redundancy in published SRs, even though some studies have indicated a large increase in the production of SRs over time [ 26 ]. In addition, we have identified at least nine different ways of defining when no further studies are needed (see Supplementary Material 4 ). Most of these definitions have used a frequency statistic approach to determine dichotomy cut-off points. However, as stated in a report from the Cochrane Scientific Committee, SR authors should be discouraged from drawing binary interpretations of effect estimates [ 27 ]. Even with the grading of evidence as a method to avoid this binary approach, there is a need for more precise and reliable methods [ 28 , 29 ].
Additionally, meta-research studies are needed to evaluate how new studies should be justified when applying for ethical approval or funding, or when preparing a study protocol. This would make it easier to evaluate the importance of using a systematic and transparent approach during ethical or funding approval in the interest of both research ethic committees and funding agencies.
Finally, as publication bias could not be ruled out, larger meta-research studies cutting across different health domains are needed to evaluate whether publication bias really exists.
Most of the included meta-research studies analysed the content of published original papers. Even though this can provide a good overview of the situation, the data extracted from original papers rarely explains why researchers have not used an evidence-based research approach. Surveys and qualitative studies are needed to understand the underlying incentives or motivational factors, and the facilitators and barriers behind the lack of a systematic and transparent approach during the justification and design phases of planning a new study.
“These initiatives have mainly emerged from the biomedical sciences and psychology, and there is now an increasing need for initiatives tailored to other research disciplines and cultures.” [ 30 ]. This scoping review has focused solely upon research within health. Considering the characteristics of the described problem (too much redundancy and too little systematicity and transparency while planning new studies and interpreting new results), this problem could exist to a similar level in other research disciplines and faculties, necessitating relevant research within social science, natural science, and the humanities.
Availability of data and materials
The datasets used and/or analysed during the current study are available from the corresponding author on reasonable request.
Robinson KA, Brunnhuber K, Ciliska D, Juhl CB, Christensen R, Lund H, et al. Evidence-Based Research Series-Paper 1: What Evidence-Based Research is and why is it important? J Clin Epidemiol. 2021;129:151–7.
Article PubMed Google Scholar
Wootton D. Experiments. The Invention of Science - a new history of the scientific revolution. New York: HarperCollins Publishers; 2015. p. §4.
Google Scholar
Clarke M, Chalmers I. Discussion sections in reports of controlled trials published in general medical journals: islands in search of continents? JAMA. 1998;280(3):280–2.
Article PubMed CAS Google Scholar
Begg C, Cho M, Eastwood S, Horton R, Moher D, Olkin I, et al. Improving the quality of reporting of randomized controlled trials. The CONSORT statement. JAMA. 1996;276(8):637–9.
Chalmers I, Hedges LV, Cooper H. A brief history of research synthesis. Eval Health Prof. 2002;25(1):12–37.
Lau J, Antman EM, Jimenez-Silva J, Kupelnick B, Mosteller F, Chalmers TC. Cumulative meta-analysis of therapeutic trials for myocardial infarction. N Engl J Med. 1992;327(4):248–54.
Antman EM, Lau J, Kupelnick B, Mosteller F, Chalmers TC. A comparison of results of meta-analyses of randomized control trials and recommendations of clinical experts. Treatments for myocardial infarction. JAMA. 1992;268(2):240–8.
Ban JW, Wallace E, Stevens R, Perera R. Why do authors derive new cardiovascular clinical prediction rules in the presence of existing rules? A mixed methods study. PLoS One. 2017;12(6):e0179102.
Article PubMed PubMed Central Google Scholar
Andrade NS, Flynn JP, Bartanusz V. Twenty-year perspective of randomized controlled trials for surgery of chronic nonspecific low back pain: citation bias and tangential knowledge. Spine J. 2013;13(11):1698–704.
Conde-Taboada A, Aranegui B, Garcia-Doval I, Davila-Seijo P, Gonzalez-Castro U. The use of systematic reviews in clinical trials and narrative reviews in dermatology: is the best evidence being used? Actas Dermosifiliogr. 2014;105(3):295–9.
Crequit P, Trinquart L, Yavchitz A, Ravaud P. Wasted research when systematic reviews fail to provide a complete and up-to-date evidence synthesis: the example of lung cancer. BMC Med. 2016;14(1):8.
Pandis N, Fleming PS, Koletsi D, Hopewell S. The citation of relevant systematic reviews and randomised trials in published reports of trial protocols. Trials. 2016;17(1):581.
Robinson KA. Use of prior research in the justification and interpretation of clinical trials. Baltimore, Maryland: Johns Hopkins University; 2009.
Robinson KA, Goodman SN. A systematic examination of the citation of prior research in reports of randomized, controlled trials. Ann Intern Med. 2011;154(1):50–5.
Macleod MR, Michie S, Roberts I, Dirnagl U, Chalmers I, Ioannidis JP, et al. Biomedical research: increasing value, reducing waste. Lancet. 2014;383(9912):101–4.
Bouter LM, Tijdink J, Axelsen N, Martinson BC, ter Riet G. Ranking major and minor research misbehaviors: results from a survey among participants of four World Conferences on Research Integrity. Res Integr Peer Rev. 2016;1(1):17.
Tricco AC, Lillie E, Zarin W, O'Brien KK, Colquhoun H, Levac D, et al. PRISMA Extension for Scoping Reviews (PRISMA-ScR): Checklist and Explanation. Ann Intern Med. 2018;169(7):467–73.
Fergusson D, Monfaredi Z, Pussegoda K, Garritty C, Lyddiatt A, Shea B, et al. The prevalence of patient engagement in published trials: a systematic review. Res Involve Engage. 2018;4(1):17.
Habre C, Tramer MR, Popping DM, Elia N. Ability of a meta-analysis to prevent redundant research: systematic review of studies on pain from propofol injection. BMJ. 2014;348:g5219.
Hoderlein X, Moseley AM, Elkins MR. Citation of prior research has increased in introduction and discussion sections with time: a survey of clinical trials in physiotherapy. Clin Trials. 2017;14(4):372–80.
Jia Y, Wen J, Qureshi R, Ehrhardt S, Celentano DD, Wei X, et al. Effect of redundant clinical trials from mainland China evaluating statins in patients with coronary artery disease: cross sectional study. BMJ. 2021;372:n48.
Ker K, Roberts I. Exploring redundant research into the effect of tranexamic acid on surgical bleeding: further analysis of a systematic review of randomised controlled trials. BMJ Open. 2015;5(8):e009460.
Bourne AM, Johnston RV, Cyril S, Briggs AM, Clavisi O, Duque G, et al. Scoping review of priority setting of research topics for musculoskeletal conditions. BMJ Open. 2018;8(12):e023962.
Thornley C, Watkinson A, Nicholas D, Volentine R, Jamali HR, Herman E, et al. The role of trust and authority in the citation behaviour of researchers. Inf Res. 2015;20(3):Paper 677.
Herling SF, Jespersen KF, Møller AM. Reflections and practices of citing papers in health care science -a focus group study. Nordisk Sygeplejeforskning. 2021;11(03):235–45.
Article Google Scholar
Ioannidis JP. The Mass Production of Redundant, Misleading, and Conflicted Systematic Reviews and Meta-analyses. Milbank Q. 2016;94(3):485–514.
Schmid C, Chandler J. Should Cochrane apply error-adjustment methods when conducting repeated meta-analyses? : Cochrane; 2018; 2018.
Gartlehner G, Dobrescu A, Evans TS, Bann C, Robinson KA, Reston J, et al. The predictive validity of quality of evidence grades for the stability of effect estimates was low: a meta-epidemiological study. J Clin Epidemiol. 2016;70:52–60.
Mercuri M, Baigrie B, Upshur REG. Going from evidence to recommendations: Can GRADE get us there? J Eval Clin Pract. 2018;24(5):1232–9.
Tijdink JK, Horbach SPJM, Nuijten MB, O’Neill G. Towards a Research Agenda for Promoting Responsible Research Practices. J Empir Res Hum Res Ethics. 2021;16(4):450–60.
Download references
Acknowledgements
This work has been prepared as part of the Evidence-Based Research Network (see: evbres.eu). The Evidence-Based Research Network is an international network that promotes the use of systematic reviews when prioritising, designing, and interpreting research. Evidence-based research is the use of prior research in a systematic and transparent way to inform the new study so that it is answering questions that matter in a valid, efficient, and accessible manner.
The authors thank the Section Evidence-Based Practice, Department for Health and Function, Western Norway University of Applied Sciences, for their generous support of the EBRNetwork. Further, thanks to COST Association for supporting the COST Action “EVBRES” (CA 17117, evbres.eu) and thereby the preparation of this study.
The authors would also like to express their gratitude to those helping with article screening: Marlies Leenaars, Durita Gunnarsson, Gorm Høj Jensen, Line Sjodsholm, Signe Versterre, Linda Baumbach, Karina Johansen, Rune Martens Andersen, and Thomas Aagaard.
Thanks to Gunhild Austrheim, Head of Unit, Library at Western Norway University of Applied Sciences, Norway, for helping with the second search.
This study is part of the European COST Action “EVBRES” (CA 17117). Section for Biostatistics and Evidence-Based Research, the Parker Institute, Bispebjerg and Frederiksberg Hospital (Professor Christensen), are supported by a core grant from the Oak Foundation USA (OCAY-18-774-OFIL).
Author information
Authors and affiliations.
Section Evidence-Based Practice, Department for Health and Function, Western Norway University of Applied Sciences, Inndalsveien 28, P.O.Box 7030, N-5020, Bergen, Norway
Hans Lund, Karen A. Robinson, Ane Gjerland & Hanna Nykvist
Division of General Internal Medicine, Department of Medicine, Johns Hopkins University, Baltimore, MD, USA
Karen A. Robinson
Research and Analysis Department, University Library of Southern Denmark, Odense, Denmark
Thea Marie Drachen
Section for Biostatistics and Evidence-Based Research, the Parker Institute, Bispebjerg and Frederiksberg Hospital, Copenhagen, Denmark
Robin Christensen
Research Unit of Rheumatology, Department of Clinical Research, University of Southern Denmark, Odense University Hospital, Odense, Denmark
Department of Sports Science and Clinical Biomechanics, University of Southern Denmark, Odense, Denmark
Carsten Bogh Juhl
Department of Physiotherapy and Occupational Therapy, Herlev and Gentofte Hospital, Herlev, Denmark
Faculty of Health Sciences, OsloMet, Oslo, Norway
Gro Jamtvedt
Faculty of Health and Social Science, Western Norway University of Applied Sciences, Bergen, Norway
Monica Nortvedt
Research Unit of Nursing and healthcare, Institute of Public Health, Health, Aarhus University, Aarhus, Denmark
Merete Bjerrum
The Centre of Clinical Guidelines, Department of Clinical Medicine, Aalborg University, Aalborg, Denmark
The Danish Centre of Systematic Reviews - A JBI Centre of Excellence, The University of Adelaide, Adelaide, Denmark
Health Research Authority, NHS, London, UK
Matt Westmore
M. Louise Fitzpatrick College of Nursing, Villanova University, Villanova, PA, USA
Jennifer Yost
Clinical Solutions, Elsevier Ltd., 125 London Wall, London, EC2Y 5AS, UK
Klara Brunnhuber
You can also search for this author in PubMed Google Scholar
Contributions
All authors have made substantial contributions to the design of the work, have approved the final submitted version (and any substantially modified version that affected the author's contribution to the study), and have agreed both to be personally accountable for the author’s own contributions and to ensure that questions related to the accuracy or integrity of any part of the work, even ones in which the author was not personally involved, are appropriately investigated, resolved, and the resolution documented in the literature.
Corresponding author
Correspondence to Hans Lund .
Ethics declarations
Ethics approval and consent to participate.
Not applicable.
Consent for publication
Competing interests.
The authors declare that they have no competing interests.
Additional information
Publisher’s note.
Springer Nature remains neutral with regard to jurisdictional claims in published maps and institutional affiliations.
Supplementary Information
Additional file 1: additional material 1..
Figure showing number of studies published per year. Additional Material 2. Figure indicates the number of studies from each country, measured as the country affiliation of 1st authors. Additional Material 3. Metrics used in studies evaluating redundancy. N = Number of studies. The total number is higher than the actual number of studies evaluating redundancy, because most studies have used more than one metric. Additional Material 4. Table presenting the metrics used in studies evaluating the use of the EBR approach to minimise or avoid redundancy. N = Number of studies. The total number is higher than the actual number of studies evaluating the use of the EBR approach because most studies have used more than one metric. Additional Material 5. Bibliographic map, the M. Clarke group. Additional Material 6. Bibliographic map, the T.C. Chalmers group. Additional Material 7. Table listing the data materials used in the included studies. (Note that “Primary studies” include papers using various kinds of studies as data material, including some systematic reviews.) Fields marked in light green indicate several studies (6 or more), those in light red indicate few studies (5 or less), and those marked in red indicate no studies. The sum of studies evaluating redundancy/use of EBR approach is higher than the total number, because several studies have evaluated more than one research question. Also, one included paper evaluating the use of the EBR approach [ 8 ] used both primary studies and researchers as data material and was therefore counted twice in the table. Additional Material 8. Table listing study designs used in the included studies. Fields marked in light green indicate several studies (6 or more), those in light red indicate few studies (5 or less), and those marked in red indicate no studies. The sum of studies evaluating redundancy/use of EBR is higher than the total number, because several studies have evaluated more than one research question. Also, one included paper evaluating the use of the EBR approach [ 8 ] used both cross-sectional and another observational study designs and was therefore counted twice in the table. Additional Material 9. Table listing analysis methods used in the included studies. Fields marked in light green indicate several studies (6 or more), those in light red indicate few studies (5 or less), and those marked in red indicate no studies. Note that many of the included studies used more than one analysis method and investigated more than one research question. For that reason, studies are counted several times in the table, and their sum is much higher than the total number of included papers. Additional Material 10. Table presenting an overview of the different conclusions reported in the included studies. N = number of studies. Additional Material 11. Reference list of included studies.
Additional file 2: Appendix 1.
Search June 2015.
Additional file 3: Appendix 2
. Search May 2021.
Additional file 4:
The filled-in Preferred Reporting Items for Systematic reviews and Meta-Analyses extension for Scoping Reviews (PRISMA-ScR) Checklist.
Rights and permissions
Open Access This article is licensed under a Creative Commons Attribution 4.0 International License, which permits use, sharing, adaptation, distribution and reproduction in any medium or format, as long as you give appropriate credit to the original author(s) and the source, provide a link to the Creative Commons licence, and indicate if changes were made. The images or other third party material in this article are included in the article's Creative Commons licence, unless indicated otherwise in a credit line to the material. If material is not included in the article's Creative Commons licence and your intended use is not permitted by statutory regulation or exceeds the permitted use, you will need to obtain permission directly from the copyright holder. To view a copy of this licence, visit http://creativecommons.org/licenses/by/4.0/ . The Creative Commons Public Domain Dedication waiver ( http://creativecommons.org/publicdomain/zero/1.0/ ) applies to the data made available in this article, unless otherwise stated in a credit line to the data.
Reprints and permissions
About this article
Cite this article.
Lund, H., Robinson, K.A., Gjerland, A. et al. Meta-research evaluating redundancy and use of systematic reviews when planning new studies in health research: a scoping review. Syst Rev 11 , 241 (2022). https://doi.org/10.1186/s13643-022-02096-y
Download citation
Received : 29 April 2022
Accepted : 01 October 2022
Published : 15 November 2022
DOI : https://doi.org/10.1186/s13643-022-02096-y
Share this article
Anyone you share the following link with will be able to read this content:
Sorry, a shareable link is not currently available for this article.
Provided by the Springer Nature SharedIt content-sharing initiative
- Evidence-based research
- Scoping review
- Meta-research
- Research on research
- Systematicity
- Transparency
Systematic Reviews
ISSN: 2046-4053
- Submission enquiries: Access here and click Contact Us
- General enquiries: [email protected]
Thank you for visiting nature.com. You are using a browser version with limited support for CSS. To obtain the best experience, we recommend you use a more up to date browser (or turn off compatibility mode in Internet Explorer). In the meantime, to ensure continued support, we are displaying the site without styles and JavaScript.
- View all journals
- Explore content
- About the journal
- Publish with us
- Sign up for alerts
- Open access
- Published: 10 November 2023
Exploiting redundancy in large materials datasets for efficient machine learning with less data
- Kangming Li ORCID: orcid.org/0000-0003-4471-8527 1 ,
- Daniel Persaud ORCID: orcid.org/0009-0004-9980-2704 1 ,
- Kamal Choudhary ORCID: orcid.org/0000-0001-9737-8074 2 ,
- Brian DeCost ORCID: orcid.org/0000-0002-3459-5888 2 ,
- Michael Greenwood 3 &
- Jason Hattrick-Simpers ORCID: orcid.org/0000-0003-2937-3188 1 , 4 , 5 , 6
Nature Communications volume 14 , Article number: 7283 ( 2023 ) Cite this article
7673 Accesses
16 Citations
65 Altmetric
Metrics details
- Condensed-matter physics
- Materials chemistry
- Scaling laws
- Scientific data
A Publisher Correction to this article was published on 04 January 2024
This article has been updated
Extensive efforts to gather materials data have largely overlooked potential data redundancy. In this study, we present evidence of a significant degree of redundancy across multiple large datasets for various material properties, by revealing that up to 95% of data can be safely removed from machine learning training with little impact on in-distribution prediction performance. The redundant data is related to over-represented material types and does not mitigate the severe performance degradation on out-of-distribution samples. In addition, we show that uncertainty-based active learning algorithms can construct much smaller but equally informative datasets. We discuss the effectiveness of informative data in improving prediction performance and robustness and provide insights into efficient data acquisition and machine learning training. This work challenges the “bigger is better” mentality and calls for attention to the information richness of materials data rather than a narrow emphasis on data volume.
Similar content being viewed by others
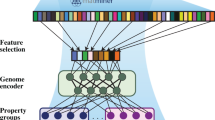
Materials property prediction for limited datasets enabled by feature selection and joint learning with MODNet
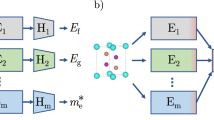
Towards overcoming data scarcity in materials science: unifying models and datasets with a mixture of experts framework
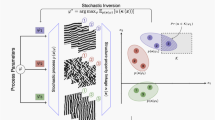
Self-supervised optimization of random material microstructures in the small-data regime
Introduction.
Data is essential to the development and application of machine learning (ML), which has now become a widely adopted tool in materials science 1 , 2 , 3 , 4 , 5 , 6 , 7 , 8 , 9 , 10 , 11 . While data is generally considered to be scarce in various subfields of materials science, there are indications that the era of big data is emerging for certain crucial material properties. For instance, a substantial amount of material data has been produced through high-throughput density functional theory (DFT) calculations 12 , leading to the curation of several large databases with energy and band gap data for millions of crystal structures 13 , 14 , 15 , 16 , 17 . The recently released Open Catalyst datasets contain over 260 million DFT data points for catalyst modeling 18 , 19 . The quantity of available materials data is expected to grow at an accelerated rate, driven by the community’s growing interest in data collection and sharing.
In contrast to the extensive effort to gather ever larger volume of data, information richness of data has so far attracted little attention. Such a discussion is important as it can provide critical feedback to data acquisition strategies adopted in the community. For instance, DFT databases were typically constructed either from exhaustive enumerations over possible chemical combinations and known structural prototypes or from random sub-sampling of such enumerations 14 , 15 , 16 , 17 , 18 , 19 , 20 , but the effectiveness of these strategies in exploring the materials space remains unclear. Furthermore, existing datasets are often used as the starting point for the data acquisition in the next stage. For example, slab structures in Open Catalyst datasets were created based on the bulk materials from Materials Project 18 , 19 . Redundancy in the existing datasets, left unrecognized, may thus be passed on to future datasets, making subsequent data acquisition less efficient.
In addition, examining and eliminating redundancy in existing datasets can improve training efficiency of ML models. Indeed, the large volume of data already presents significant challenges in developing ML models due to the increasingly strong demand for compute power and long training time. For example, over 16,000 GPU days were recently used for analyzing and developing models on the Open Catalyst datasets 21 . Such training budgets are not available to most researchers, hence often limiting model development to smaller datasets or a portion of the available data 22 . On the other hand, recent work on image classification has shown that a small subset of data can be sufficient to train a model with performance comparable to that obtained using the entire dataset 23 , 24 . It has been reported that aggressively filtering training data can even lead to modest performance improvements on natural language tasks, in contrast to the prevailing wisdom of “bigger is better” in this field 25 . To the best of our knowledge, however, there has been no investigation of the presence and degree of data redundancy in materials science. Revealing data redundancy can inform and motivate the community to create smaller benchmark datasets, hence significantly scaling down the training costs and facilitating model development and selection. This may be important in the future if data volume grows much faster than the available training budget, which is a likely scenario, as data volume is proportional to resources available to the entire community, while training budgets are confined to individual research groups.
The examination of data redundancy is also important in other scenarios in materials science. Methods developed for selecting the most informative data can be used as the strong baselines for active learning algorithms, which are increasingly common in ML-driven materials discovery workflows 26 , 27 , 28 , 29 , 30 , 31 , 32 , 33 , 34 . Analysis of information richness can also improve our understanding of the material representation and guide the design of active learning algorithms. In the multi-fidelity data acquisition setting 35 , one can perform high-fidelity measurement only on the informative materials down-selected from the larger but low-fidelity datasets.
In this work, we present a systematic investigation of data redundancy across multiple large material datasets by examining the performance degradation as a function of training set size for traditional descriptor-based models and state-of-the-art neural networks. To identify informative training data, we propose a pruning algorithm and demonstrate that smaller training sets can be used without substantially compromising the ML model performance, highlighting the issue of data redundancy. We also find that selected sets of informative materials transfer well between different ML architectures, but may transfer poorly between substantially different material properties. Finally, we compare uncertainty-based active learning strategies with our pruning algorithm, and discuss the effectiveness of active learning for more efficient high throughput materials discovery and design.
Redundancy evaluation tasks
We investigate data redundancy by examining the performance of ML models. To do so, we use the standard hold-out method for evaluating ML model performance: We create the training set and the hold-out test set from a random split of the given dataset. The training set is used for model training, while the test set is reserved for evaluating the model performance. In the following, we refer to the performance evaluated on this test set as the in-distribution (ID) performance, and this training set as the pool. To reveal data redundancy, we train a ML model on a portion of the pool and check whether its ID performance is comparable to the one resulting from using the entire pool. Since ID performance alone may not be sufficient to prove the redundancy of the remaining unused pool data, we further evaluate the prediction performance on the unused pool data and out-of-distribution (OOD) test data.
Figure 1 illustrates the redundancy evaluation discussed above. We first perform a (90, 10)% random split of the given dataset S 0 to create the pool and the ID test set. To create an OOD test set, we consider new materials included in a more recent version of the database S 1 . Such OOD sets enable the examination of model performance robustness against distribution shifts that may occur when mission-driven research programs focus on new areas of material space 36 . We progressively reduce the training set size from 100% to 5% of the pool via a pruning algorithm (see “Methods”). ML models are trained for each training set size, and their performance is tested on the hold-out ID test data, the unused pool data, and the OOD data, respectively.
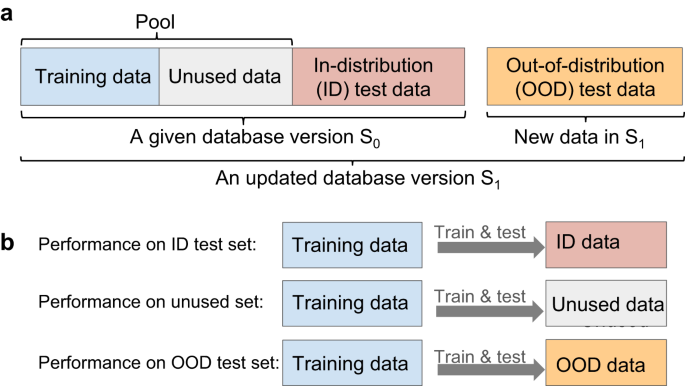
a The dataset splits. b Three Prediction tasks to evaluate model performance and data redundancy.
To ensure a comprehensive and robust assessment of data redundancy, we examine the formation energy, band gap, and bulk modulus data in three widely-used DFT databases, namely JARVIS 15 , Materials Project (MP) 16 , and OQMD 17 . For each database, we consider two release versions to study the OOD performance and to compare the data redundancy between different database versions. The number of entries for these datasets is given in Table 1 .
To ascertain whether data redundancy is model-agnostic, we consider two conventional ML models, namely XGBoost (XGB) 37 and random forests (RF) 38 , and a graph neural network called the Atomistic LIne Graph Neural Network (ALIGNN) 39 . The RF and XGB models are chosen since they are among the most powerful descriptor-based algorithms 40 , whereas ALIGNN is chosen as the representative neural network because of its state-of-the-art performance in the Matbench test suite 41 at the time of writing.
In-distribution performance
We begin by presenting an overview of the ID performance for all the model-property-dataset combinations in Table 2 , where the root mean square errors (RMSE) of the models trained on the entire pool are compared to those obtained with 20% of the pool. For brevity, we refer to the models trained on the entire pool and on the subsets of the pool as the full and reduced models, respectively, but we note that the model specification is the same for both full and reduced models and the terms “reduced” and “full” pertain only to the amount of training data.
For the formation energy prediction, the RMSE of the reduced RF models increase by less than 6% compared to those of the full RF models in all cases. Similarly, the RMSE of the reduced XGB models increase only by 10 to 15% compared to the RMSE of the full XGB models in most datasets, except in OQMD21 where a 3% decrease in the RMSE is observed. The RMSE of the reduced ALIGNN models increase by 15 to 45%, a larger increment than observed for the RF and XGB models. Similar trend is observed for the band gap and bulk modulus prediction, where the RMSE of the reduced models typically increase by no more than 30% compared to those of the full models.
Next, we conduct a detailed analysis for formation energy and band gap properties because of their fundamental importance for a wide range of materials design problems. Figure 2 shows the ID performance as a function of training set size (in percentage of the pool) for the formation energy and band gap prediction in the JARVIS18, MP18, and OQMD14 datasets. Results for other datasets can be found in Supplementary Figs. 1 – 6 .
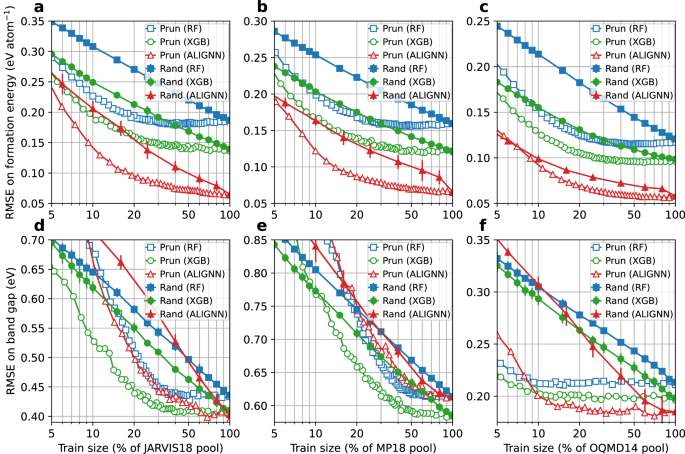
a – c JARVIS18, MP18, and OQMD14 formation energy prediction. d – f JARVIS18, MP18, and OQMD14 band gap prediction. RF random forest, XGB XGBoost, ALIGNN atomistic line graph neural network. The random baseline results to for the XGB and RF (or ALIGNN) models are obtained by averaging over the results of 10 (or 5) random data selections for each training set size, with the error bars denoting the standard deviations. The X axis is in the log scale. Data points are connected by straight lines. Source data are provided as a Source data file .
For the formation energy prediction, the prediction error obtained with the pruned data drops much faster with increasing data size than the one obtained using the randomly selected data. When accounting for more than 5% of the training pool, the pruned datasets lead to better ID performance than the ones from random sampling. In particular, the RF, XGB, and ALIGNN models trained with 20% of the pool selected by the pruning algorithm have the same ID performance as the ones trained with a random selection of around 90%, 70%, and 50%, respectively, of the pool.
A large portion of training data can be removed without significantly hurting the model performance. To demonstrate this, we define a quantitative threshold for the “significance” of the performance degradation as a 10% relative increase in RMSE; data that can be pruned without exceeding this performance degradation threshold are considered redundant. With this definition, only 13% of the JARVIS18 data, and 17% of the MP18 and OQMD data are informative for the RF models. For the XGB models, between 20% and 30% of the data are needed depending on the datasets. For the ALIGNN models, 55%, 40%, and 30% of the JARVIS18, MP18, and OQMD14 data are informative, respectively. While the JARVIS18 dataset may seem to be less redundant for the ALIGNN models, the 10% increase in the RMSE (60 meV atom −1 ) corresponds to an RMSE increase of only 6 meV atom −1 , much smaller than the DFT accuracy of around 100 meV atom −1 with respect to experiments 42 . In fact, training the ALIGNN model on 30% of the JARVIS18 data only leads to a drop of 0.002 in the R 2 test score.
While this work is focused on redundancy which is model and dataset specific, it is still worth commenting on the model performance scaling across models and datasets. When using the random sampling for data selection, we observe a power law scaling for all the models and datasets. For formation energy datasets, switching the models mainly shifts the scaling curve without much change to the slopes. For band gap datasets, switching from RF to XGB models shifts the scaling curve down without changing the slope, whereas switching from tree-based models to ALIGNN leads to a steeper slope and hence better scaling. Compared to training on randomly sampled data, training on informative data as selected by the pruning algorithm can lead to better scaling until reaching saturation when there is no more informative data in the pool. Different datasets exhibit similar scaling behaviors with the slope and saturation point dependent on target property and material space covered by the datasets.
The performance response to the size of band gap data is similar to that observed in the formation energy data. The redundancy issue is also evident in band gap data: a 10% RMSE increase corresponds to training with 25 to 40% of the data in the JARVIS18 and MP18 datasets. Even more strikingly, only 5% (or 10%) of the OQMD14 band gap data are sufficiently informative for the RF and XGB (or ALIGNN) models.
These results demonstrate the feasibility of training on only a small portion of the available data without much performance degradation. We find that this is achieved by skewing the data distribution towards the underrepresented materials. For instance, the distributions of the pruned data are skewed towards materials with large formation energies and band gaps (Fig. 3 ), which are both underrepresented and less accurately predicted materials. These results not only confirm the importance of the data diversity 40 but also highlight the redundancy associated with overrepresented materials.
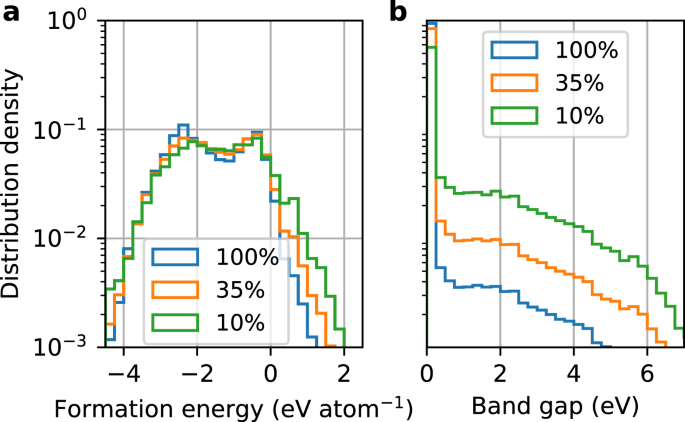
a Formation energy data from the MP18 dataset. b Band gap data from the OQMD14 dataset. The legend indicates the training set size in percentage of the pool. Results for other datasets can be found in Supplementary Figs. 15 and 16 . Source data are provided as a Source data file .
ID performance is not sufficient to prove that the unused data are truly redundant. The effects related to model capability and the test set distribution should also be considered. Indeed, one may argue that the current ML models (in particular, the band gap models) are not advanced enough to learn from the unused data leading to a false sense of the data redundancy. Furthermore, the similar performance of the full and reduced models does not imply a similar performance on a test set following a different distribution. These questions are addressed in the following two sections by discussing the performance on the unused data and on the OOD data.
Performance on unused data
Here we further examine the model performance on the unused pool data. Figure 4 shows three representative cases: the JARVIS18 and MP18 formation energy datasets, and the OQMD14 band gap dataset. For the formation energy prediction, the RMSE on the unused data become lower than on the ID RMSE when the training set size is above 5 to 12% of the pool, and is half of the ID RMSE when the training set size is above 30 to 40% of the pool. Similar trend is observed for the band gap prediction with varying thresholds of the performance improvement saturation depending the datasets (Supplementary Figs. 10 – 12) . In particular, the OQMD14 results in Fig. 4 show that the models trained on 10% of the pool can well predict the unused data that account for 90% of the pool, with the associated RMSE much lower than the RMSE on the ID test set. The good prediction on the unused data signifies a lack of new information in these data, confirming that the improvement saturation in the ID performance is caused by the information redundancy in the unused data rather than the incapability of models to learn new information.
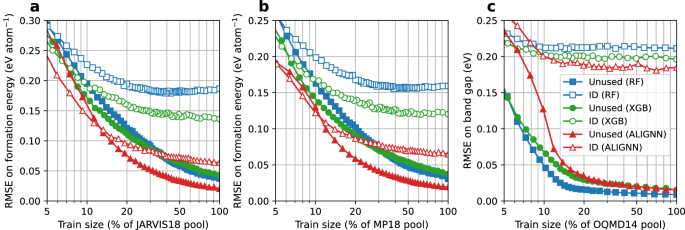
a JARVIS18 formation energy prediction. b MP18 formation energy prediction. c OQMD14 band gap prediction. RF random forest, XGB XGBoost, ALIGNN atomistic line graph neural network. Performance on the ID test set is shown for comparison. Data points are connected by straight lines. Source data are provided as a Source data file .
While the scaling curve for the unused data has a shape similar to the one for the ID test data, the former shows a much steeper slope for the training set sizes below 15% of the pool, and reaches saturation at a slower rate. In addition, it is noted that the ranking of different ML models for their performance on the unused data is not necessarily the same as for the ID test data. For instance, for the JARVIS18 and MP18 formation energy data, the XGB model outperforms the RF model on the ID test set whereas their performance is practically the same on the unused data. Among the models trained on the OQMD14 band gap data, the RF model has the largest RMSE on the ID test set but the lowest error on the unused data.
Out-of-distribution performance
To check whether redundancy in training data also manifests under a distribution shift in test data, we examine the model performance on the OOD test data consisting of the new materials in the latest database versions (JARVIS22, MP21, and OQMD21) using the models trained on the older versions (JARVIS18, MP18, and OQMD14).
First, we find that training on the pruned data can lead to better or similar OOD performance than the randomly sampled data of the same size. We therefore focus here on the OOD performance based on the pruned data shown in Fig. 5 . Overall, the scaling curves for the OOD performance show are similar to those for the ID performance with slightly different slopes and saturation data size, confirming the existence of the data redundancy measured by the OOD performance. Specifically, using 20%, 30%, or 5% to 10% of the JARVIS18, MP18, or OQMD14 data, respectively, can lead to an OOD performance similar to that of the full models, with around 10% RMSE increase.
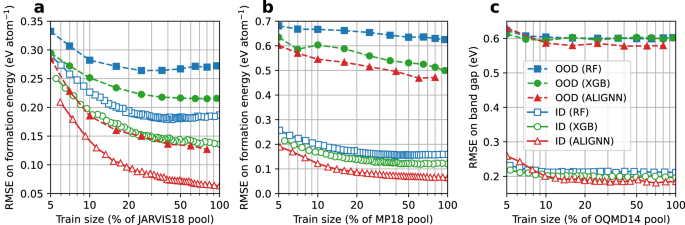
a JARVIS formation energy prediction. b MP formation energy prediction. c OQMD band gap prediction. RF random forest, XGB XGBoost, ALIGNN atomistic line graph neural network. Performance on the ID test set is shown for comparison. Data points are connected by straight lines. The reader interested in the statistical overlaps between the ID and OOD data in the feature space is referred to Supplementary Fig. 24 . Source data are provided as a Source data file .
The performance on OOD data can be severely degraded. Even for the models trained on the entire pool, the increase in the OOD RMSE with respect to the ID RMSE often goes above 200% for the considered databases and can rise up to 640% in the case of the ALIGNN-MP formation energy prediction (Supplementary Table 1) . Therefore, the excellent ID performance obtained with state-of-the-art models and large datasets might be a catastrophically optimistic estimation of the true generalization performance in a realistic materials discovery setting 36 , 40 .
Different databases exhibit a varying degree of performance degradation, which should be correlated with the degree of statistical overlaps between the database versions rather than the quality of the databases. In fact, database updates that induce such performance degradation are desirable because they are indications of new “unknown” observations and can lead to more robust generalization performance. One interesting line of research would be therefore to develop methods to deliberately search for materials where the previous models would fail catastrophically as a path to expand a database.
The strong OOD performance degradation highlights the importance of information richness over data volume. It also raises an interesting question: given a training set A 1 , is it possible to find a smaller training set A 2 such that the A 2 -trained model perform similarly to the A 1 -trained model on an A 1 -favorable test set B 1 (i.e., same distribution as A 1 ) but significantly outperform the A 1 -trained model on an A 1 -unfavorable test set B * (i.e., distribution different from A 1 )? Indeed, we find that training on the heavily pruned MP21 pool ( A 2 ) gives dramatically better prediction performance on the MP21 test data ( B * ) than training on 10 × more data from the MP18 pool ( A 1 ) whereas their performance is similar on the MP18 test set ( B 1 ). The result confirms the idea of finding a training set whose distribution can not only well cover but also significantly extend beyond the original one while still being much smaller in size. The result highlights that information richness and data volume are not necessarily correlated, and the former is much more important for the prediction robustness. By covering more materials within the data distribution, we may better ensure unknown materials are from known distributions (“known unknown”) and avoid unexpected performance degradation (“unknown unknown”), which is particularly important in scenarios such as materials discovery or building universal interatomic potentials 22 , 43 , 44 .
Transferability of pruned material sets
The ID performance results demonstrate that our pruning algorithm effectively identifies informative material sets for a given ML model and material property. A natural follow-up inquiry is the universality, or more specifically, the transferability of these sets between ML architectures and material properties.
We find a reasonable level of transferability of the pruned material set across ML architectures, confirming that data pruned by a given ML architecture remains informative to other ones (Supplementary Figs. 17 – 20) . For example, XGB models trained on RF-pruned data outperform those trained on twice as much randomly selected data for formation energy prediction. Moreover, the XGB model still outperforms an RF model trained on the same pruned data, consistent with our observed performance ranking (XGB > RF). This ensures robustness against information loss with respect to future architecture change: more capable models developed in the future can be expected to extract no less information from the pruned dataset than the current state-of-the-art one, even if the dataset is pruned by the latter. It would therefore be desirable to propose benchmark datasets pruned from existing large databases using current models, which can help accelerate the development of ML models due to the smaller training cost.
In contrast, we find that there is a limited transferability of pruned datasets across different material properties. For instance, the band gap models trained on the pruned formation energy data outperform those trained on randomly sampled data by only by a slight margin (Supplementary Fig. 21) , suggesting little overlap between informative material sets for predicting these two properties. This limited task transferability may be a result of the lack of strong correlation between the formation energy and band gap data, for which the Spearman correlation coefficient is −0.5 in the considered databases. Additionally, the OOD results show that formation energy and band gap models do not necessarily suffer the same degree of performance degradation when tested on new materials despite being trained on the same set of materials (Supplementary Table 1) , indicating learned feature-property relations could differ significantly. These considerations suggest that a fruitful line of future research might explore dataset pruning based on multitask regression models focusing on a diverse set of material properties controlled by different underlying physical phenomena.
Uncertainty-based active learning
In the previous sections we have revealed the data redundancy in the existing large material databases through dataset pruning. How much, then, can we avoid such data redundancy in the first place when constructing the databases? To this end, we consider active learning algorithms that select samples with largest prediction uncertainty (see “Methods”). The first and the second algorithms use the width of the 90% prediction intervals of the RF and XGB models as the uncertainty measure, respectively, whereas the third one is based on the query by committee (QBC), where the uncertainty is taken as the disagreement between the RF and XGB predictions.
Figure 6 shows a comparison of the ID performance of the XGB models trained on the data selected using the active learning algorithm, the pruning algorithm, and the random sampling. The QBC algorithm is found to be the best performing active learning algorithm. For the formation energy prediction across the three databases, 30 to 35% of the pool data selected by the QBC algorithm is enough to achieve the same model performance obtained with 20% of the pool data using the pruning algorithm. Furthermore, the resulting model performance is equivalent to that obtained with 70 to 90% of the pool using the random sampling. As for the band gap prediction, the models trained on the QBC-selected data perform similarly to those trained on the pruned data, or even sometimes outperform the latter when the data volume is below 20% (Supplementary Fig. 23) . In particular, the QBC algorithm can effectively identify 10% of the OQMD14 band gap data as the training data without hurting the model performance (Fig. 6 c). Similar trends are also found for the RF models and for other datasets (Supplementary Fig. 23) .
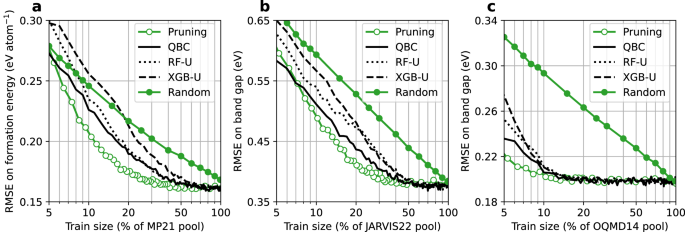
a MP21 formation energy prediction. b JARVIS22 formation energy prediction. c OQMD14 band gap prediction. QBC query by committee, RF-U random forest uncertainty, XGB-U XGBoost uncertainty. The performance obtained using the random sampling and the pruning algorithm is shown for comparison. Data points are connected by straight lines. Source data are provided as a Source data file .
Overall, our results across multiple datasets suggest that it is possible to leverage active learning algorithms to query only 30% of the existing data with a relatively small accuracy loss in the ID prediction. The remaining 70% of the compute may then be used to obtain a larger and more representative material space. Considering the potentially severe performance degradation on OOD samples which are likely to be encountered in material discovery, the gain in the robustness of ML models may be preferred over the incremental gain in the ID performance.
It is worth emphasizing that this work is by no means critical of the curation efforts or significance of these materials datasets. Indeed, many datasets were not originally generated for training ML models; they are the results of long-term project-driven computational campaigns. Some of them were even curated before the widespread use of ML and have played a significant role in fueling the rapid adoption of ML in materials science. On the other hand, the presence and degree of redundancy in a dataset is worth discussing irrespective of the original purpose. Furthermore, ML should be considered not only as a purpose, though it has become a primary use case of these datasets, but also as a statistical means of examining and improving these datasets.
This work is also not to oppose the use of big data, but to advocate a critical assessment of the information richness in data, which has been largely overlooked due to a narrow emphasis on data volume. As materials science transitions towards a big data-driven approach, such evaluations and reflections on current practices and data can offer insights into more efficient data acquisition and sensible resource usage. For instance, conventional high-throughput DFT often relies on enumerations over structural prototypes and chemical combinations. The substantial redundancy revealed in this work suggests these strategies are suboptimal in querying new informative data, whereas uncertainty based active learning can enable a 3× to 10× boost in sampling efficiency. Our scaling results for OOD performance degradation further highlight the importance of information richness over sheer volume for robust predictive models. In this regard, it is preferable to allocate more resources to explore a diverse materials space rather than seeking incremental improvements in prediction accuracy within limited or well-studied regions. This may represent a paradigm shift from systematic high-throughput studies, where we can start with uncertainty based active learning in a much larger design space, and then reconsider the design space by interrogating the model and switching to a property optimization or local interpretable prediction objective.
While the pruning algorithm is proposed here to illustrate data redundancy, such data selection algorithms can have other use cases, e.g., inform the design of active learning algorithms. Indeed, the observation that data redundancy predominantly involves overrepresented materials implies that information entropy might also serve as a promising criterion for data acquisition 40 , 45 . A detailed analysis of pruned material sets may also offer insights into material prototypes and improve understanding of feature-property relationships, including identifying specific groups of redundant materials as well as identifying patterns that explain the poor task transferability of pruned datasets. Finally, the pruning algorithm offers a new funneling strategy for prioritizing materials for high-fidelity measurements. For instance, pruning the existing DFT data obtained with generalized gradient approximation (GGA) functionals can point to the materials to be recomputed with high-fidelity meta-GGA functionals 35 .
We demonstrate that transferability of compact datasets is reasonable across models but is limited across tasks (materials properties). It is discussed in the context of data pruning, but the idea and implication hold for active learning. The limited task transferability indicates that the maximally compact set of materials for property A is not ensured to be the maximally compact set for property B. While this is an interesting observation and invites further investigation, it is not a practical issue for active learning when the measurements of two properties are independent. For example, DFT calculations of band gap and elastic modulus are unrelated, therefore the maximally compact sets of materials can be constructed independently via active learning and need not be the same. For correlated property measurements, however, more careful planning is required. For instance, the calculations of more “expensive” properties such as band gap and elastic modulus would also give the formation energy of the same material since energy is a basic output of any DFT calculations. While the compact datasets for band gap and elastic modulus can still be searched independently without considering formation energy data, the construction of the compact dataset for formation energy should consider the data that can be obtained as by-products from the band gap and elastic modulus calculations.
In conclusion, we investigate data redundancy across multiple material datasets using both conventional ML models and state-of-the-art neural networks. We propose a pruning algorithm to remove uninformative data from the training set, resulting in models that outperform those trained on randomly selected data of the same size. Depending on the dataset and ML architecture, up to 95% of data can be pruned with little degradation in in-distribution performance (defined as <10% increase in RMSE) compared to training on all available data. The removed data, mainly associated with overrepresented material types, are shown to be well predicted by the reduced models trained without them, confirming again the information redundancy. Using new materials in newer database versions as the out-of-distribution test set, we find that 70 to 95% of data can be removed from the training set without exceeding a 10% performance degradation threshold on out-of-distribution data, confirming again that the removed data are redundant and do not lead to improved performance robustness against distribution shift. Transferability analysis shows that the information content of pruned datasets transfers well to different ML architectures but less so between material properties. Finally, we show that the QBC active learning algorithm can achieve an efficiency comparable to the pruning algorithm in terms of finding informative data, hence demonstrating the feasibility of constructing much smaller material databases while still maintaining a high level of information richness. While active learning algorithm may still induce bias in the datasets they generate, we believe that there is an exciting opportunity to optimize high throughput material simulation studies for generalization on a broad array of material property prediction tasks.
Materials datasets
The 2018.06.01 version of Materials Project (MP18), and the 2018.07.07 and 2022.12.12 versions of JARVIS (JARVIS18 and JARVIS22) were retrieved by using JARVIS-tools 15 . The 2021.11.10 version of Materials Project (MP21) was retrieved using the Materials Project API 16 . The OQMD14 and OQMD21 data were retrieved from https://oqmd.org/download .
The JARVIS22, MP21, OQMD21 data were preprocessed as follows. First, entries of materials with a formation energy larger than 5 eV atom −1 were removed. Then, the Voronoi tessellation scheme 46 as implemented in Matminer 47 were used to extract 273 compositional and structural features. The Voronoi tessellation did not work for a very small number of materials and these materials were removed.
For the older versions (JARVIS18, MP18, OQMD14), we did not directly use the structures and label values from the older database. Instead, we use the materials identifiers from the older database to search for the corresponding structures and label values in the newer database. This is to avoid any potential inconsistency caused by the database update.
We considered three ML models here: XGB 37 , RF 38 , and a graph neural network called the Atomistic LIne Graph Neural Network (ALIGNN) 39 . XGB is a gradient-boosted method that builds sequentially a number of decision trees in a way such that each subsequent tree tries to reduce the residuals of the previous one. RF is an ensemble learning method that combines multiple independently built decision trees to improve accuracy and minimize variance. ALIGNN constructs and utilizes graphs of interatomic bonds and bond angles.
We used the RF model as implemented in the scikit-learn 1.2.0 package 48 , and the XGB model as implemented in the XGBoost 1.7.1 package 37 . For the RF model, we used 100 estimators, 30% of the features for the best splitting, and default settings for other hyperparameters. We used a boosted random forest for the XGB model: 4 parallel boosted trees were used; for each tree, we used 1000 estimators, a learning rate of 0.1, an L1 (L2) regularization strength of 0.01 (0.1), and the histogram tree grow method; we set the subsample ratio of training instances to 0.85, the subsample ratio of columns to 0.3 when constructing each tree, and the subsample ratio of columns to 0.5 for each level. The hyperparameter set was kept to be the same in all the model training for the following reasons: First, performing hyperparameter tuning every time when changing the size of the training set would be very computationally expensive. Second, we verified that the model performance using the optimal hyperparameters tuned from the randomized cross-validation search was close to the one using the chosen hyperparameters.
For the ALIGNN model, we used 2 ALIGNN layers, 2 GCN layers, a batch size of 128, and the layer normalization, while keeping other hyperparameters the same as in the original ALIGNN implementation 39 . We trained the ALIGNN model for 50 epochs as we found more epochs did not lead to further performance improvement. We used the same OneCycle learning rate schedule, with 30% of the training budget allocated to linear warmup and 70% to cosine annealing.
Pruning algorithm
We proposed a pruning algorithm that starts with the full training pool and iteratively reduces the training set size. We denote the full training pool as D pool , the training set at the i -th iteration as \({D}_{{{{{{{{\rm{train}}}}}}}}}^{i}\) , the unused set as \({D}_{{{{{{{{\rm{unused}}}}}}}}}^{i}\) ( \(={D}_{{{{{{{{\rm{pool}}}}}}}}}-{D}_{{{{{{{{\rm{train}}}}}}}}}^{i}\) ), and the trained model as M i . At the initial iteration ( i = 0), \({D}_{{{{{{{{\rm{train}}}}}}}}}^{0}={D}_{{{{{{{{\rm{pool}}}}}}}}}\) , and \({D}_{{{{{{{{\rm{unused}}}}}}}}}^{0}\) is empty. At each iteration i > 0, \({D}_{{{{{{{{\rm{train}}}}}}}}}^{i}\) and \({D}_{{{{{{{{\rm{unused}}}}}}}}}^{i}\) are updated as follows: First, a random splitting of \({D}_{{{{{{{{\rm{train}}}}}}}}}^{i-1}\) is performed to obtained two subsets \({D}_{A}^{i}\) (80% of \({D}_{{{{{{{{\rm{train}}}}}}}}}^{i-1}\) ) and \({D}_{B}^{i}\) (20% of \({D}_{{{{{{{{\rm{train}}}}}}}}}^{i-1}\) ). Then, a model \(M{\prime}\) is trained on \({D}_{A}^{i}\) and then tested on \({D}_{B}^{i}\) . The data in \({D}_{B}^{i}\) with lowest prediction errors (denoted as \({D}_{B,{{{{{{\rm{unused}}}}}}}}^{i}\) ) are then removed from the training set. Namely, \({D}_{{{{{{{{\rm{train}}}}}}}}}^{i}={D}_{{{{{{{{\rm{train}}}}}}}}}^{i-1}-{D}_{B,{{{{{{\rm{unused}}}}}}}}^{i}\) , and \({D}_{{{{{{{{\rm{unused}}}}}}}}}^{i}\) = \({D}_{{{{{{{{\rm{unused}}}}}}}}}^{i-1}+{D}_{B,{{{{{{\rm{unused}}}}}}}}^{i}\) . The model M i trained on \({D}_{{{{{{{{\rm{train}}}}}}}}}^{i}\) is then used in the performance evaluation on the ID test set, the unused set \({D}_{{{{{{{{\rm{unused}}}}}}}}}^{i}\) and the OOD test set.
Active learning algorithm
During the active learning process, the training set is initially constructed by randomly sampling 1 to 2% of the pool, and is grown with a batch size of 1 to 2% of the pool by selecting the materials with maximal prediction uncertainty. Three uncertainty measures are used to rank the materials. The first one is based on the uncertainty of the RF model and is calculated as the difference between the 95th and 5th percentile of the tree predictions in the forest. The second one is based on the uncertainty of the XGB model using an instance-based uncertainty estimation for gradient-boosted regression trees developed in ref. 49 . The third one is based on the query by committee, where the uncertainty is taken as the difference between the RF and XGB predictions.
Reporting summary
Further information on research design is available in the Nature Portfolio Reporting Summary linked to this article.
Data availability
The data required and generated by our code are available on Zenodo at https://zenodo.org/record/8200972 . Source data are provided with this paper.
Code availability
The code used in this work is available on GitHub at https://github.com/mathsphy/paper-data-redundancy and a snapshot of the code is provided on Zenodo 50 .
Change history
04 january 2024.
A Correction to this paper has been published: https://doi.org/10.1038/s41467-023-44462-x
Butler, K. T., Davies, D. W., Cartwright, H., Isayev, O. & Walsh, A. Machine learning for molecular and materials science. Nature 559 , 547–555 (2018).
Article ADS CAS PubMed Google Scholar
Vasudevan, R. K. et al. Materials science in the artificial intelligence age: high-throughput library generation, machine learning, and a pathway from correlations to the underpinning physics. MRS Commun. 9 , 821–838 (2019).
Article ADS CAS Google Scholar
Morgan, D. & Jacobs, R. Opportunities and challenges for machine learning in materials science. Annu. Rev. Mater. Res. 50 , 71–103 (2020).
DeCost, B. L. et al. Scientific AI in materials science: a path to a sustainable and scalable paradigm. Mach. Learn.: Sci. Technol. 1 , 033001 (2020).
Google Scholar
Hart, G. L. W., Mueller, T., Toher, C. & Curtarolo, S. Machine learning for alloys. Nat. Rev. Mater. 6 , 730–755 (2021).
Article ADS Google Scholar
Stach, E. et al. Autonomous experimentation systems for materials development: a community perspective. Matter 4 , 2702–2726 (2021).
Article Google Scholar
Choudhary, K. et al. Recent advances and applications of deep learning methods in materials science. npj Comput. Mater. 8 , 59 (2022).
Schleder, G. R., Padilha, A. C., Acosta, C. M., Costa, M. & Fazzio, A. From DFT to machine learning: recent approaches to materials science—a review. J.Phys.: Mater. 2 , 032001 (2019).
CAS Google Scholar
Green, M. L., Maruyama, B. & Schrier, J. Autonomous (AI-driven) materials science. Appl. Phys. Rev. 9 , 030401 (2022).
Kalinin, S. V. et al. Machine learning in scanning transmission electron microscopy. Nat. Rev. Methods Primers 2 , 1–28 (2022).
Krenn, M. et al. On scientific understanding with artificial intelligence. Nat. Rev. Phys. 4 , 761–769 (2022).
Horton, M., Dwaraknath, S. & Persson, K. Promises and perils of computational materials databases. Nat. Comput. Sci. 1 , 3–5 (2021).
Draxl, C. & Scheffler, M. NOMAD: the FAIR concept for big data-driven materials science. MRS Bull. 43 , 676–682 (2018).
Curtarolo, S. et al. AFLOWLIB.ORG: a distributed materials properties repository from high-throughput ab initio calculations. Comput. Mater. Sci. 58 , 227–235 (2012).
Article CAS Google Scholar
Choudhary, K. et al. The joint automated repository for various integrated simulations (JARVIS) for data-driven materials design. npj Comput. Mater. 6 , 173 (2020).
Jain, A. et al. Commentary: The Materials Project: a materials genome approach to accelerating materials innovation. APL Mater. 1 , 011002 (2013).
Saal, J. E., Kirklin, S., Aykol, M., Meredig, B. & Wolverton, C. Materials design and discovery with high-throughput density functional theory: the open quantum materials database (OQMD). Jom 65 , 1501–1509 (2013).
Chanussot, L. et al. Open Catalyst 2020 (OC20) dataset and community challenges. ACS Catal. 11 , 6059–6072 (2021).
Tran, R. et al. The Open Catalyst 2022 (OC22) dataset and challenges for oxide electrocatalysts. ACS Catal . 13 , 3066–3084 (2023).
Shen, J. et al. Reflections on one million compounds in the open quantum materials database (OQMD). J. Phys.: Mater. 5 , 031001 (2022).
Gasteiger, J. et al. GemNet-OC: developing graph neural networks for large and diverse molecular simulation datasets. Transactions on Machine Learning Research (2022).
Choudhary, K. et al. Unified graph neural network force-field for the periodic table: solid state applications. Digit. Discov. 2 , 346–355 (2023).
Yang, S. et al. Dataset pruning: reducing training data by examining generalization influence. In The Eleventh International Conference on Learning Representations (2022).
Sorscher, B., Geirhos, R., Shekhar, S., Ganguli, S. & Morcos, A. Beyond neural scaling laws: beating power law scaling via data pruning. Adv. Neural Inf. Process. Syst. 35 , 19523–19536 (2022).
Geiping, J. & Goldstein, T. Cramming: training a language model on a single GPU in one day. Proceedings of the 40th International Conference on Machine Learning. 202 , 11117–11143 (2022).
Ling, J., Hutchinson, M., Antono, E., Paradiso, S. & Meredig, B. High-dimensional materials and process optimization using data-driven experimental design with well-calibrated uncertainty estimates. Integr. Mater. Manuf. Innov. 6 , 207–217 (2017).
Smith, J. S., Nebgen, B., Lubbers, N., Isayev, O. & Roitberg, A. E. Less is more: sampling chemical space with active learning. J. Chem. Phys. 148 , 241733 (2018).
Article ADS PubMed Google Scholar
Lookman, T., Balachandran, P. V., Xue, D. & Yuan, R. Active learning in materials science with emphasis on adaptive sampling using uncertainties for targeted design. npj Comput. Mater. 5 , 21 (2019).
Jia, X. et al. Anthropogenic biases in chemical reaction data hinder exploratory inorganic synthesis. Nature 573 , 251–255 (2019).
Zhong, M. et al. Accelerated discovery of CO 2 electrocatalysts using active machine learning. Nature 581 , 178–183 (2020).
Kusne, A. G. et al. On-the-fly closed-loop materials discovery via Bayesian active learning. Nat. Commun. 11 , 5966 (2020).
Article ADS CAS PubMed PubMed Central Google Scholar
Rohr, B. et al. Benchmarking the acceleration of materials discovery by sequential learning. Chem. Sci. 11 , 2696–2706 (2020).
Article PubMed PubMed Central Google Scholar
Liang, Q. et al. Benchmarking the performance of Bayesian optimization across multiple experimental materials science domains. npj Comput. Mater. 7 , 188 (2021).
Wang, A., Liang, H., McDannald, A., Takeuchi, I. & Kusne, A. G. Benchmarking active learning strategies for materials optimization and discovery. Oxf. Open Mater. Sci. 2 , itac006 (2022).
Kingsbury, R. S. et al. A flexible and scalable scheme for mixing computed formation energies from different levels of theory. npj Comput. Mater. 8 , 195 (2022).
Li, K., DeCost, B., Choudhary, K., Greenwood, M. & Hattrick-Simpers, J. A critical examination of robustness and generalizability of machine learning prediction of materials properties. npj Comput. Mater. 9 , 55 (2023).
Chen, T. & Guestrin, C. XGBoost. In Proc. 22nd ACM SIGKDD Int. Conf. Knowl. Discov. Data Min . 785–794 (ACM, 2016).
Breiman, L. Random forests. Mach. Learn. 45 , 5–32 (2001).
Choudhary, K. & DeCost, B. Atomistic Line Graph Neural Network for improved materials property predictions. npj Comput. Mater. 7 , 185 (2021).
Zhang, H., Chen, W. W., Rondinelli, J. M. & Chen, W. ET-AL: entropy-targeted active learning for bias mitigation in materials data. Appl. Phys. Rev. 10 , 021403 (2023).
Dunn, A., Wang, Q., Ganose, A., Dopp, D. & Jain, A. Benchmarking materials property prediction methods: the Matbench test set and Automatminer reference algorithm. npj Comput. Mater. 6 , 1–10 (2020).
Kirklin, S. et al. The Open Quantum Materials Database (OQMD): assessing the accuracy of DFT formation energies. npj Comput. Mater. 1 , 15010 (2015).
Takamoto, S. et al. Towards universal neural network potential for material discovery applicable to arbitrary combination of 45 elements. Nat. Commun. 13 , 2991 (2022).
Chen, C. & Ong, S. P. A universal graph deep learning interatomic potential for the periodic table. Nat. Comput. Sci. 2 , 718–728 (2022).
Hennig, P. & Schuler, C. J. Entropy search for information-efficient global optimization. J. Mach. Learn. Res. 13 , 1809–1837 (2012).
Ward, L. et al. Including crystal structure attributes in machine learning models of formation energies via Voronoi tessellations. Phys. Rev. B 96 , 024104 (2017).
Ward, L. et al. Matminer: an open source toolkit for materials data mining. Comput. Mater. Sci. 152 , 60–69 (2018).
Pedregosa, F. et al. Scikit-learn: machine learning in Python. J. Mach. Learn. Res. 12 , 2825–2830 (2011).
MathSciNet Google Scholar
Brophy, J. & Lowd, D. Instance-based uncertainty estimation for gradient-boosted regression trees. In Advances in Neural Information Processing Systems (2022).
Li, K. et al. Exploiting redundancy in large materials datasets for efficient machine learning with less data. Zenodo https://doi.org/10.5281/zenodo.8431636 (2023).
Download references
Acknowledgements
The computations were made on the resources provided by the Calcul Quebec, Westgrid, and Compute Ontario consortia in the Digital Research Alliance of Canada (alliancecan.ca), and the Acceleration Consortium (acceleration.utoronto.ca) at the University of Toronto. We acknowledge funding provided by Natural Resources Canada’s Office of Energy Research and Development (OERD). The research was also, in part, made possible thanks to funding provided to the University of Toronto’s Acceleration Consortium by the Canada First Research Excellence Fund (CFREF-2022-00042). Certain commercial products or company names are identified here to describe our study adequately. Such identification is not intended to imply recommendation or endorsement by the National Institute of Standards and Technology, nor is it intended to imply that the products or names identified are necessarily the best available for the purpose.
Author information
Authors and affiliations.
Department of Materials Science and Engineering, University of Toronto, 27 King’s College Cir, Toronto, ON, Canada
Kangming Li, Daniel Persaud & Jason Hattrick-Simpers
Material Measurement Laboratory, National Institute of Standards and Technology, 100 Bureau Dr, Gaithersburg, MD, USA
Kamal Choudhary & Brian DeCost
Canmet MATERIALS, Natural Resources Canada, 183 Longwood Road south, Hamilton, ON, Canada
Michael Greenwood
Acceleration Consortium, University of Toronto, 27 King’s College Cir, Toronto, ON, Canada
Jason Hattrick-Simpers
Vector Institute for Artificial Intelligence, 661 University Ave, Toronto, ON, Canada
Schwartz Reisman Institute for Technology and Society, 101 College St, Toronto, ON, Canada
You can also search for this author in PubMed Google Scholar
Contributions
K.L. and J.H.-S. conceived and designed the project. K.L. implemented the pruning algorithm. D.P. implemented the active learning algorithms. K.L. performed the ML training, analyzed the results, and drafted the manuscript. J.H.-S. supervised the project. K.L, D.P., K.C, B.D., M.G., and J.H.-S. discussed the results, reviewed and edited the manuscript, and contributed to the manuscript preparation.
Corresponding author
Correspondence to Jason Hattrick-Simpers .
Ethics declarations
Competing interests.
The authors declare no competing interests.
Peer review
Peer review information.
Nature Communications thanks Saulius Gražulis and the other, anonymous, reviewer(s) for their contribution to the peer review of this work. A peer review file is available.
Additional information
Publisher’s note Springer Nature remains neutral with regard to jurisdictional claims in published maps and institutional affiliations.
Supplementary information
Supplementary information, peer review file, reporting summary, source data, rights and permissions.
Open Access This article is licensed under a Creative Commons Attribution 4.0 International License, which permits use, sharing, adaptation, distribution and reproduction in any medium or format, as long as you give appropriate credit to the original author(s) and the source, provide a link to the Creative Commons license, and indicate if changes were made. The images or other third party material in this article are included in the article’s Creative Commons license, unless indicated otherwise in a credit line to the material. If material is not included in the article’s Creative Commons license and your intended use is not permitted by statutory regulation or exceeds the permitted use, you will need to obtain permission directly from the copyright holder. To view a copy of this license, visit http://creativecommons.org/licenses/by/4.0/ .
Reprints and permissions
About this article
Cite this article.
Li, K., Persaud, D., Choudhary, K. et al. Exploiting redundancy in large materials datasets for efficient machine learning with less data. Nat Commun 14 , 7283 (2023). https://doi.org/10.1038/s41467-023-42992-y
Download citation
Received : 30 April 2023
Accepted : 26 October 2023
Published : 10 November 2023
DOI : https://doi.org/10.1038/s41467-023-42992-y
Share this article
Anyone you share the following link with will be able to read this content:
Sorry, a shareable link is not currently available for this article.
Provided by the Springer Nature SharedIt content-sharing initiative
This article is cited by
Structure-based out-of-distribution (ood) materials property prediction: a benchmark study.
- Sadman Sadeed Omee
npj Computational Materials (2024)
Extrapolative prediction of small-data molecular property using quantum mechanics-assisted machine learning
- Hajime Shimakawa
- Akiko Kumada
- Masahiro Sato
JARVIS-Leaderboard: a large scale benchmark of materials design methods
- Kamal Choudhary
- Daniel Wines
- Francesca Tavazza
By submitting a comment you agree to abide by our Terms and Community Guidelines . If you find something abusive or that does not comply with our terms or guidelines please flag it as inappropriate.
Quick links
- Explore articles by subject
- Guide to authors
- Editorial policies
Sign up for the Nature Briefing: AI and Robotics newsletter — what matters in AI and robotics research, free to your inbox weekly.

Microinsurance research: status quo and future research directions
- Published: 19 August 2024
- Volume 49 , pages 417–420, ( 2024 )
Cite this article
- Martin Eling 1 &
Avoid common mistakes on your manuscript.
Eighteen years have passed since Muhammad Yunus was honoured with the Nobel Prize, sparking significant interest in the microfinance and microinsurance sectors. Presently, the economic and social significance of microinsurance, along with its challenges, are acknowledged every day in news outlets and political debates. Over the past decade, the microinsurance sector has seen remarkable growth, particularly in Asian countries, with significant emphasis on serving the low-income population facing life, health and agricultural risks. This expansion is complemented by the development of inclusive insurance programmes, which extend coverage to encompass the burgeoning middle class. These initiatives, ranging from rural schemes to more organised, national programmes with government assistance, play a crucial role in providing vital financial protection to diverse segments of society.
Even as microinsurance gains in importance for both business and society, research in this area is still scarce. Despite some publications, there remains a critical gap in understanding its theoretical benefits, drawbacks, practical effectiveness and the extent of regulation it requires. With this special issue, The Geneva Papers on Risk and Insurance—Issues and Practice continues its tradition of publishing special issues on emerging insurance topics. The journal has a history of dedicating issues to microinsurance (volume 39 (2) in 2014, volume 41 (2) in 2016, volume 44 (3) in 2019 and volume 46 (3) in 2021). As illustrated in Fig. 1 , research on microinsurance has surged over the past two decades, and it is now established as a distinct research domain. The first 10 years saw research output increase by tenfold, from around 100 to over 1000 publications annually. Subsequently, the field has stabilised, with approximately 1500 publications each year. Web of Science peaks in microinsurance publications in 2016 and 2019 coincide with the years this journal released special issues on the subject, highlighting the significant impact these editions have had on scholarly research in the field.
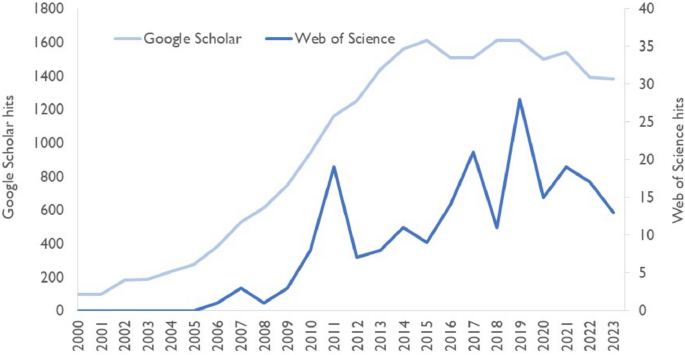
Hits by year for search term microinsurance (as of 4 March 2024)
The journal has published several well-cited articles on the challenges for commercial insurers providing coverage for the low-income market (Churchill 2007 ), the determinants of microinsurance demand (Eling et al. 2014 ) and the barriers to microinsurance adoption (Cole 2015 ). Yet, most articles dealt with narrower (often unique) settings, emphasising the complexity of the topic. Research on microinsurance demonstrates the fundamental problems and need for basic solutions (e.g. obtaining data, data quality, asymmetric information, potential correlations, ‘public good’ character of products, group vs. individual choice, short-term vs. long-term welfare gains, (unexpected) impact of regulation, return on investment, crowd out by public programmes). These special issues of The Geneva Papers therefore serve the industry well by informing on current thinking in the microinsurance space.
This year’s special issue on microinsurance includes three articles selected from nine submissions. The authors have used a range of methodologies from empirical analysis based on surveys to aggregated data. One article also includes a conceptual framework based on Outreville’s insurance demand framework. Two of the three articles deal with the African environment in Ghana, and the other with Turkey. As for the risk categories, one article discusses microinsurance in general (life and non-life), one paper focus on life/health and the other on agricultural risks. We also note that two of the three articles analyse the demand side, and one considers more supply-side frictions.
The first paper, Microinsurance in Ghana: Investigating the impact of Outreville's four-factor framework and firm and product characteristics on adoption , delves into the determinants of microinsurance adoption in Ghana, analysing data from households across six market centres and three regions, alongside data from 14 microinsurance firms and 47 products between 2017 and 2021. The study employs robust probit fixed effects and panel-corrected standard error models, highlighting that income levels, trust in financial institutions, participation in community risk management groups and the national health insurance scheme significantly affect microinsurance adoption. It also notes the influence of firm- and product-specific factors, such as affordability, claims risk, premiums and benefits, alongside the importance of structural, social and economic factors. This comprehensive analysis employs Outreville's four-factor insurance demand framework to categorise the critical factors influencing microinsurance uptake, offering valuable insights for policymakers and practitioners aiming to enhance microinsurance adoption in Ghana.
The second paper, Actuarial premium calculation for beekeeping insurance in Türkiye , explores the modelling of aggregate claims based on hive insurance policy data from 2014 to 2021. Using a collective risk model, the study conducts premium calculations for different geographical regions of Türkiye, identifying Eastern Anatolia as the region with the highest premiums and Central Anatolia the lowest. The research includes cluster analysis to categorise provinces based on claims ratios, revealing significant variations in premium rates. This detailed actuarial analysis aims to provide a foundation for fair and effective premium setting in Türkiye's beekeeping insurance sector, addressing the unique risks and challenges of the industry.
Finally, the third paper, The effect of microinsurance on the financial resilience of low-income households in Ghana: evidence from a propensity score matching analysis , examines how microinsurance enhances the financial resilience of low-income households in Ghana. The study utilises data from households across three regions and employs propensity score matching, tobit and probit instrumental variable techniques. It finds that microinsurance adoption significantly improves financial resilience by increasing income and reducing reliance on precautionary savings. This offers a critical safety net against economic shocks, advocating for the implementation of microinsurance programmes to support financial stability among Ghana’s poor.
One intention of this special issue is to stimulate future research on microinsurance in addition to publishing interesting articles. Indeed, the articles presented in this special issue open several avenues for future research. Firstly, understanding the mechanisms through which microinsurance can further enhance financial resilience in low-income communities, particularly by examining the role of digital technologies and mobile banking in increasing accessibility and reducing costs. This entails a deeper analysis of behavioural factors influencing microinsurance uptake and the impact of financial literacy programmes in the context of social networks. Secondly, there is promising scope for exploring the scalability of beekeeping insurance models to other agricultural sectors, potentially integrating climate risk assessment to develop more comprehensive insurance products. These areas not only offer the potential for significant academic contributions but also hold practical implications for policymakers and practitioners aiming to improve insurance penetration and financial inclusion in developing economies.
In considering this special issue, it is striking that all contributions are empirical and that there is no established theoretical framework to analyse microinsurance. Obviously, one might ask whether a separate theoretical framework for microinsurance is needed or whether microinsurance is just another type of insurance that should be analysed using classical models. The above discussion, however, illustrates the special nature and complex features of microinsurance (e.g. asymmetric information, potential correlations, ‘public good’ character) so that distinct papers that analyse microinsurance from a theoretical point of view might be useful, maybe also with reference to other comparable types of risk.
In addition, considering the growing scale of inclusive insurance, and the overlap of its target population with microinsurance, it is of interest to explore the possibility of partnering the two types of products in certain areas of practice, to learn from the experience of inclusive insurance and to combine forces in the field of financial inclusion.
We feel privileged to be able to benefit from the research of the contributing authors. We hope you will enjoy reading their articles as much as we have enjoyed editing this special issue of The Geneva Papers on Risk and Insurance—Issues and Practice .
Data availability
Not applicable.
Churchill, C. 2007. Insuring the low-income market: Challenges and solutions for commercial insurers. The Geneva Papers on Risk and Insurance—Issues and Practice 32: 401–412.
Article Google Scholar
Cole, S. 2015. Overcoming barriers to microinsurance adoption: Evidence from the field. The Geneva Papers on Risk and Insurance—Issues and Practice 40: 720–740.
Eling, M., S. Pradhan, and J.T. Schmit. 2014. The determinants of microinsurance demand. The Geneva Papers on Risk and Insurance—Issues and Practice 39: 224–263.
Download references
Author information
Authors and affiliations.
Institute of Insurance Economics, University of St.Gallen, Tannenstrasse 19, 9000, St.Gallen, Switzerland
Martin Eling
School of Economics and Institute for Global Health and Development, Peking University, 5, Yiheyuan Road, Haidian District, Beijing, 100871, China
You can also search for this author in PubMed Google Scholar
Corresponding author
Correspondence to Martin Eling .
Additional information
Publisher's note.
Springer Nature remains neutral with regard to jurisdictional claims in published maps and institutional affiliations.
Rights and permissions
Reprints and permissions
About this article
Eling, M., Yao, Y. Microinsurance research: status quo and future research directions. Geneva Pap Risk Insur Issues Pract 49 , 417–420 (2024). https://doi.org/10.1057/s41288-024-00328-x
Download citation
Published : 19 August 2024
Issue Date : July 2024
DOI : https://doi.org/10.1057/s41288-024-00328-x
Share this article
Anyone you share the following link with will be able to read this content:
Sorry, a shareable link is not currently available for this article.
Provided by the Springer Nature SharedIt content-sharing initiative
- Find a journal
- Publish with us
- Track your research
Information
- Author Services
Initiatives
You are accessing a machine-readable page. In order to be human-readable, please install an RSS reader.
All articles published by MDPI are made immediately available worldwide under an open access license. No special permission is required to reuse all or part of the article published by MDPI, including figures and tables. For articles published under an open access Creative Common CC BY license, any part of the article may be reused without permission provided that the original article is clearly cited. For more information, please refer to https://www.mdpi.com/openaccess .
Feature papers represent the most advanced research with significant potential for high impact in the field. A Feature Paper should be a substantial original Article that involves several techniques or approaches, provides an outlook for future research directions and describes possible research applications.
Feature papers are submitted upon individual invitation or recommendation by the scientific editors and must receive positive feedback from the reviewers.
Editor’s Choice articles are based on recommendations by the scientific editors of MDPI journals from around the world. Editors select a small number of articles recently published in the journal that they believe will be particularly interesting to readers, or important in the respective research area. The aim is to provide a snapshot of some of the most exciting work published in the various research areas of the journal.
Original Submission Date Received: .
- Active Journals
- Find a Journal
- Proceedings Series
- For Authors
- For Reviewers
- For Editors
- For Librarians
- For Publishers
- For Societies
- For Conference Organizers
- Open Access Policy
- Institutional Open Access Program
- Special Issues Guidelines
- Editorial Process
- Research and Publication Ethics
- Article Processing Charges
- Testimonials
- Preprints.org
- SciProfiles
- Encyclopedia
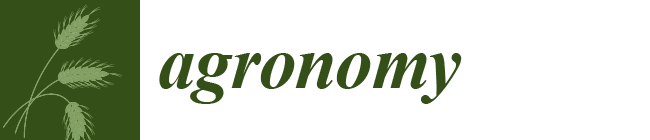
Article Menu
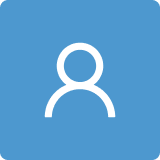
- Subscribe SciFeed
- Recommended Articles
- Google Scholar
- on Google Scholar
- Table of Contents
Find support for a specific problem in the support section of our website.
Please let us know what you think of our products and services.
Visit our dedicated information section to learn more about MDPI.
JSmol Viewer
Research on acoustic signal identification mechanism and denoising methods of combine harvesting loss.
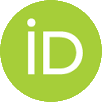
1. Introduction
2. materials and methods, 2.1. impact simulation between plate and throws, 2.2. signal acquisition and analysis, 2.2.1. design of the signal acquisition circuit, 2.2.2. acoustic monitoring test bench, 2.2.3. acoustic feature analysis, 2.3. research on loss detection methods, 2.3.1. signal denoising method, 2.3.2. recognition and counting method of grain signals, 4. discussion, 5. conclusions, author contributions, data availability statement, conflicts of interest.
- Gao, L.; Xu, S.; Li, Z.; Cheng, S.; Yu, W.; Zhang, Y.; Wu, C. Main grain crop postharvest losses and its reducing potential in China. Trans. Chin. Soc. Agric. Eng. (Trans. CSAE) 2016 , 32 , 1–11. [ Google Scholar ]
- Liu, J.G.; Lundqvist, J.; Weinberg, J.; Gustafsson, J. Food Losses and Waste in China and Their Implication for Water and Land. Environ. Sci. Technol. 2013 , 47 , 10137–10144. [ Google Scholar ] [ CrossRef ]
- Nie, X.; Sun, Y.; Chen, X.; Wang, J. Based on DSP and Winform, the software research of online monitoring system of grain loss rate and the construction of cloud platform are studied. In Proceedings of the 2020 ASABE Annual International Meeting, Virtual, 13–15 July 2020. [ Google Scholar ] [ CrossRef ]
- Liang, Z.; Wada, M. Development of cleaning systems for combine harvesters: A review. Biosyst. Eng. 2023 , 236 , 79–102. [ Google Scholar ] [ CrossRef ]
- Jie, Z.; Liu, H.; Hou, F. Research advances and prospects of combine on precision agriculture in China. Trans. Chin. Soc. Agric. Eng. 2005 , 21 , 179–182. [ Google Scholar ]
- Zhuo, W.; Dong, C.; Xiaoping, B.; Hechun, H. Improvement and Experiment of Cleaning Loss Rate Monitoring Device for Corn Combine Harvester. Trans. Chin. Soc. Agric. Mach. 2018 , 49 , 100–108. [ Google Scholar ] [ CrossRef ]
- Wayne, M.; Thomas, G.; Robert, J.; James, N. Unthreshed Head Grain Loss Monitor. U.S. Patent US4825146A, 25 April 1989. Available online: https://patents.google.com/patent/US4825146A/en (accessed on 14 August 2024).
- Richard, K. Absolute Grain Loss Monitor. U.S. Patent US4360998A, 30 November 1982. Available online: https://patents.google.com/patent/US4360998A/en (accessed on 14 August 2024).
- Jahari, M.; Yamamoto, K.; Miyamoto, M.; Kondo, N.; Ogawa, Y.; Suzuki, T.; Ahmad, U. Double lighting machine vision system to monitor harvested paddy grain quality during head-feeding combine harvester operation. Machines 2015 , 3 , 352–363. [ Google Scholar ] [ CrossRef ]
- Chen, J.; Lian, Y.; Li, Y. Real-time grain impurity sensing for rice combine harvesters using image processing and decision-tree algorithm. Comput. Electron. Agric. 2020 , 175 , 105591. [ Google Scholar ] [ CrossRef ]
- Gao, J.; Zhang, G.; Yu, L.; Li, Y. Chaos detection of grain impact at combine cleaning loss sensor. Trans. Chin. Soc. Agric. Eng. (Trans. CSAE) 2011 , 27 , 22–27. [ Google Scholar ] [ CrossRef ]
- Liang, Z. Selecting the proper material for a grain loss sensor based on DEM simulation and structure optimization to improve monitoring ability. Precis. Agric. 2021 , 22 , 1120–1133. [ Google Scholar ] [ CrossRef ]
- Liang, Z.; Li, Y.; Baerdemaeker, J.; Xu, L.; Saeys, W. Development and testing of a multi-duct cleaning device for tangential-longitudinal flow rice combine harvesters. Biosyst. Eng. 2019 , 182 , 95–106. [ Google Scholar ] [ CrossRef ]
- Sun, Y.; Yu, Y.; Tian, H.; Qian, X.; Wang, J. Design and modeling of grain impact sensor utilizing two crossed polyvinylidene fluoride films. In Proceedings of the 2017 ASABE Annual International Meeting, Spokane, DC, USA, 16–19 July 2017. [ Google Scholar ] [ CrossRef ]
- Xu, L.; Wei, C.; Liang, Z.; Chai, X.; Li, Y.; Liu, Q. Development of rapeseed cleaning loss monitoring system and experiments in a combine harvester. Biosyst. Eng. 2019 , 178 , 118–130. [ Google Scholar ] [ CrossRef ]
- Jin, M.; Zhao, Z.; Chen, S.; Chen, J. Improved piezoelectric grain cleaning loss sensor based on adaptive neuro-fuzzy inference system. Precis. Agric. 2022 , 23 , 1174–1188. [ Google Scholar ] [ CrossRef ]
- Wu, Y.H.; Li, X.Y.; Mao, E.R.; Du, Y.F.; Yang, F. Design and development of monitoring device for corn grain cleaning loss based on piezoelectric effect. Comput. Electron. Agric. 2020 , 179 , 11. [ Google Scholar ] [ CrossRef ]
- Baydar, N.; Ball, A. Detection of gear failures via vibration and acoustic signals using wavelet transform. Mech. Syst. Signal Process. 2003 , 17 , 787–804. [ Google Scholar ] [ CrossRef ]
- Park, S.W.; Lee, S.K. Development of a new sound metric for impact sound in a passenger car using the wavelet transform. Int. J. Automot. Technol. 2010 , 11 , 809–818. [ Google Scholar ] [ CrossRef ]
- Loss, L.H. Experiments with Acoustic Sensors for Grain Loss Measuring on Combines ; SAE International: Warrendale, PA, USA, 1992. [ Google Scholar ] [ CrossRef ]
- Batcheller, B.D.; Gelinske, J.N.; Nystuen, P.A.; Reich, A.A. System and Method for Determining Material Yield and/or Loss from a Harvesting Machine Using Acoustic Sensors. U.S. Patent US9474208B2, 25 October 2016. Available online: https://patents.google.com/patent/US9474208B2/en (accessed on 14 August 2024).
- Li, Q.; Jones, N. Shear and adiabatic shear failures in an impulsively loaded fully clamped beam. Int. J. Impact Eng. 1999 , 22 , 589–607. [ Google Scholar ] [ CrossRef ]
- Benson, D.J.; Stander, N.; Jensen, M.R.; Craig, K.J. On the application of LS-OPT to identify non-linear material models in LS-DYNA. In Proceedings of the 7th International LS-DYNA Users Conference, Dearborn, MI, USA, 6–8 May 2002; Available online: https://www.dynalook.com/conferences/international-conf-2002/Session_16-4.pdf (accessed on 14 August 2024).
- Carney, K.; Pereira, J.M.; Revilock, D.; Matheny, P. Jet engine fan blade containment using an alternate geometry. Int. J. Impact Eng. 2009 , 36 , 720–728. [ Google Scholar ] [ CrossRef ]
- Jorgensen, K.; Swan, V. Modeling of armour-piercing projectile perforation of thick aluminium plates. In Proceedings of the 13th International LS-DYNA Users Conference, Detroit, MI, USA, 8–10 June 2014. [ Google Scholar ]
- Ma, X.; Lei, D.; Zhao, S.; Zhao, Z. Study on the Mechanical-Rheological Properties of Soybean and Wheat Grain Grown in Northeast China. Trans. CSAE 1999 , 3 , 70–75. [ Google Scholar ]
- Liang, L.; Guo, Y. Relationship between stalk biomechanical properties and morphological traits of wheat at different growth stages. Trans. CSAE 2008 , 8 , 131–134. [ Google Scholar ]
- Liang, Z.; Li, Y.; Zhao, Z. Monitoring method and sensor for grain separation loss on axial flow combine harvester. Trans. Chin. Soc. Agric. Eng. (Trans. CSAE) 2014 , 30 , 18–26. [ Google Scholar ] [ CrossRef ]
- Feldman, M. Hilbert transform in vibration analysis. Mech. Syst. Signal Process. 2011 , 25 , 735–802. [ Google Scholar ] [ CrossRef ]
- Shen, J.; Zhao, S.; Chen, J. The Processing Way of Pipeline Vibration Signal based on Wavelet Transform. In Proceedings of the International Conference on Advanced Design and Manufacturing Engineering (ADME 2011), Guangzhou, China, 16–18 September 2011. [ Google Scholar ] [ CrossRef ]
- Huang, N.E.; Shen, Z.; Long, S.R.; Wu, M.C.; Shih, H.H.; Zheng, Q.; Liu, H.H. The empirical mode decomposition and the Hilbert spectrum for nonlinear and non-stationary time series analysis. Proc. R. Soc. Lond. Ser. A Math. Phys. Eng. Sci. 1998 , 454 , 903–995. [ Google Scholar ] [ CrossRef ]
- Boudraa, A.O.; Cexus, J.C. EMD-based signal filtering. IEEE Trans. Instrum. Meas. 2007 , 56 , 2196–2202. [ Google Scholar ] [ CrossRef ]
- Du, Y.; Zhang, L.; Mao, E.; Li, X.; Wang, H. Design and experiment of corn combine harvester grain loss monitoring sensor based on EMD. Trans. Chin. Soc. Agric. Mach. 2022 , 53 , 158–165. [ Google Scholar ] [ CrossRef ]
- Li, Y.; Chen, T.; Xiaopeng, W.; Kunpeng, Y.; Chao, Z. Theoretical analysis and numerical simulation for impact noise due to impact of two cylinders. J. Vib. Shock 2014 , 33 , 162–166+173. [ Google Scholar ] [ CrossRef ]
- Sasaoka, N.; Hamahashi, N.; Itoh, Y. Speech Enhancement with Impact Noise Activity Detection Based on the Kurtosis of an Instantaneous Power Spectrum. Ieice Trans. Fundam. Electron. Commun. Comput. Sci. 2017 , E100A , 1942–1950. [ Google Scholar ] [ CrossRef ]
- Jiao, Y.; Shi, H.; Wang, X. Lifting Wavelet Denoising Algorithm For Acoustic Emission Signal. In Proceedings of the International Conference on Robots & Intelligent System (ICRIS), Zhangjiajie, China, 27–28 August 2016. [ Google Scholar ] [ CrossRef ]
- Shi, Y.; Zhang, J.; Jiao, J.; Zhao, R.; Cao, H. Calibration Analysis of High-G MEMS Accelerometer Sensor Based on Wavelet and Wavelet Packet Denoising. Sensors 2021 , 21 , 1231. [ Google Scholar ] [ CrossRef ] [ PubMed ]
- Wang, Z.; Liu, X.; Liu, X. Wavelet and Empirical Mode Decomposition Denoising for GLAS Full Waveform Data. Laser Optoelectron. Prog. 2021 , 58 , 364–371. [ Google Scholar ]
- Liang, Z.; Li, Y.; Zhao, Z.; Xu, L.; Zhao, Z. Sensor for monitoring rice grain sieve losses in combine harvesters. Biosyst. Eng. 2016 , 147 , 51–66. [ Google Scholar ] [ CrossRef ]
Click here to enlarge figure
Material Properties | Grain | Stem | Plate | Air |
---|---|---|---|---|
Density/kg·m | 760 | 160 | 7850 | 1.3 |
Young’s modulus/MPa | 464 | 410 | 2.1 × 10 | |
Poisson’s ratio | 0.40 | 0.35 | 0.30 | |
Pressure cutoff/Pa | −1 × 10 | |||
Viscosity coefficient/N·s·m | 2 × 10 | |||
Initial internal energy/Pa | 2.5 × 10 |
Parameter value | 0.56 | 0.02 | |
Parameter value | 3 | 4 | 2 |
Parameter value | −1 | 1 | −1 |
Step | Intrinsic Modes of the Grain Model (Hz) | Intrinsic Modes of the Stem Model (Hz) |
---|---|---|
1 | 0 | 0 |
2 | 3.2584 × 10 | 0 |
3 | 7.6664 × 10 | 0 |
4 | 1.4152 × 10 | 5.2634 × 10 |
5 | 1.5645 × 10 | 5.7461 × 10 |
6 | 2.1082 × 10 | 6.6704 × 10 |
7 | 37,750 | 4784.1 |
8 | 37,837 | 12,009 |
9 | 44,106 | 13,063 |
10 | 46,978 | 21,041 |
11 | 46,985 | 21,082 |
12 | 51,154 | 24,800 |
Group | Experimental Time (Sec) | Detected Grain Loss | Actual Grain Loss | Detection Error (%) |
---|---|---|---|---|
1 | 45 | 1636 | 1695 | −3.4 |
2 | 43 | 1623 | 1772 | −8.4 |
3 | 42 | 1330 | 1233 | +7.9 |
4 | 46 | 1130 | 1079 | +4.8 |
5 | 47 | 1404 | 1464 | −4.1 |
6 | 42 | 1250 | 1156 | +8.1 |
Average | 44 | 1396 | 1400 | 6.1 |
The statements, opinions and data contained in all publications are solely those of the individual author(s) and contributor(s) and not of MDPI and/or the editor(s). MDPI and/or the editor(s) disclaim responsibility for any injury to people or property resulting from any ideas, methods, instructions or products referred to in the content. |
Share and Cite
Shen, Y.; Gao, J.; Jin, Z. Research on Acoustic Signal Identification Mechanism and Denoising Methods of Combine Harvesting Loss. Agronomy 2024 , 14 , 1816. https://doi.org/10.3390/agronomy14081816
Shen Y, Gao J, Jin Z. Research on Acoustic Signal Identification Mechanism and Denoising Methods of Combine Harvesting Loss. Agronomy . 2024; 14(8):1816. https://doi.org/10.3390/agronomy14081816
Shen, Yuhao, Jianmin Gao, and Zhipeng Jin. 2024. "Research on Acoustic Signal Identification Mechanism and Denoising Methods of Combine Harvesting Loss" Agronomy 14, no. 8: 1816. https://doi.org/10.3390/agronomy14081816
Article Metrics
Article access statistics, further information, mdpi initiatives, follow mdpi.
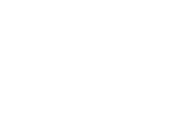
Subscribe to receive issue release notifications and newsletters from MDPI journals
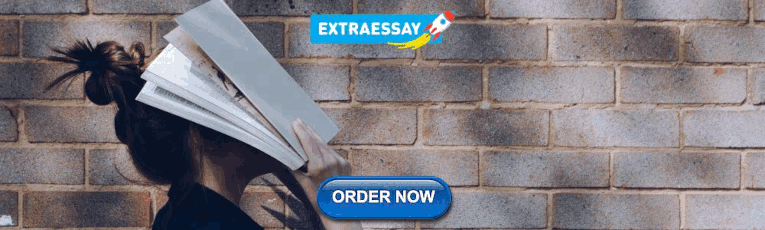
COMMENTS
View a PDF of the paper titled Information Redundancy and Biases in Public Document Information Extraction Benchmarks, by Seif Laatiri and 5 other authors. Advances in the Visually-rich Document Understanding (VrDU) field and particularly the Key-Information Extraction (KIE) task are marked with the emergence of efficient Transformer-based ...
Our research highlighted that KIE standard benchmarks such as SROIE and FUNSD contain significant similarity between training and testing documents and can be adjusted to better evaluate the generalization of models. In this work, we designed experiments to quantify the information redundancy in public benchmarks, revealing a 75% template ...
Find the latest published documents for information redundancy, Related hot topics, top authors, the most cited documents, and related journals ... these operations lead to information redundancy and confusion between crowd and background information. In this paper, we propose a multi-scale guided attention network (MGANet) to solve the above ...
In the paper, they decompose the interaction into unique, redundant, and synergistic information, as recently proposed by Williams and Beer (2010, 2011; see those papers for intuitive partial information diagrams). GK2017a develop a new approach to apportion between redundancy (two sources provide overlapping information) and synergy (the total ...
Information redundancy refers to the implementation of fault-tolerance in computer systems by using mechanisms such as error-detecting and -correcting codes, data replication techniques, and algorithm-based fault-tolerance. AI generated definition based on: Fault-Tolerant Systems (Second Edition), 2021. About this page.
For reciprocal ties, the followees' information redundancy is 0.43 on average, whereas the average information redundancy is 0.33 for nonreciprocal ties, χ 2 (1, N = 1,613,733) = 50,676, p < .001. The Spearman's rank correlation between the number of followees and information redundancy is 0.17 (p < .001). This means that high information ...
It remains controversial whether community structures in social networks are beneficial or not for information diffusion. This study examined the relationships among four core concepts in social network analysis—network redundancy, information redundancy, ego-alter similarity, and tie strength—and their impacts on information diffusion.
Don't use the same pronoun to reference more than one antecedent (e.g. " They asked whether they were ready for them") Avoid repetition of particular sounds or words (e.g. " Several shelves sheltered similar sets of shells ") Avoid redundancies (e.g " In the year 2019 " instead of " in 2019 ") Don't state the obvious (e.g.
In this paper, we are concerned with effectively identifying and reducing redundant information in search results. In particular, we describe how we automatically detect content that is lexically ...
Stay informed on the latest trending ML papers with code, research developments, libraries, methods, and datasets. Read previous issues. Subscribe. Join the community ... In this work, we designed experiments to quantify the information redundancy in public benchmarks, revealing a 75% template replication in SROIE official test set and 16% in ...
Abstract. Information redundancy is one of the key mechanisms by which to implement fault-tolerance. This chapter starts with error-detecting and -correcting codes. It then looks at one important ...
This paper reviews empirical research on the redundancy effect (63 studies) and classifies two types of redundancy: (1) content redundancy, and (2) working memory channel redundancy. ... If redundant information is added to learning material that contains high element interactivity, it is more likely to be detrimental because it adds extraneous ...
Abstract. This paper explores the relationship between the uncertainty of information (UoI) and information entropy as applied to multiple-source data fusion (MSDF). Many MSDF methods maximize system-wide entropy by minimizing source-data redundancy. However, the potential for uncertainty in the system provides a role for redundancy to confirm ...
Redundant means unnecessary because it is more than is needed [ 1 ]. For systematic reviews, it has been stated that the extent of their redundancy has reached "epidemic proportions" [ 2 ]. However, it was also emphasized that not all duplication is bad, that replication in research is essential, and that it can help discover unfortunate ...
In both cases, there is redundant information that the learner has to process. ... To contribute to conceptual clarity in redundancy research, in this paper, we want to compare these different types experimentally and investigate possible main and interaction effects. Therefore, we propose two distinct alternative classifications of redundancy ...
The most common form of information redundancy is coding, which adds check bits to the data, allowing us to verify the correctness of the data before using it and, in some cases, even allowing the correction of the erroneous data bits. Several commonly used error-detecting and error-correcting codes are discussed in Section 3.1.
We focus on copy-and-paste redundancy: clinicians typically copy and paste information from previous notes when documenting a current patient encounter. Thus, within a longitudinal patient record, one expects to observe heavy redundancy. In this paper, we ask three research questions: (i) How can redundancy be quantified in large-scale text ...
Several studies have documented the production of wasteful research, defined as research of no scientific importance and/or not meeting societal needs. We argue that this redundancy in research may to a large degree be due to the lack of a systematic evaluation of the best available evidence and/or of studies assessing societal needs. The aim of this scoping review is to (A) identify meta ...
In describing the redundancy of raw data, the rate of a source of information is the average entropy per symbol. For memoryless sources, this is merely the entropy of each symbol, while, in the most general case of a stochastic process, it is = (,, …), in the limit, as n goes to infinity, of the joint entropy of the first n symbols divided by n.It is common in information theory to speak of ...
Extensive efforts to gather materials data have largely overlooked potential data redundancy. In this study, we present evidence of a significant degree of redundancy across multiple large ...
Explore the latest full-text research PDFs, articles, conference papers, preprints and more on REDUNDANCY. Find methods information, sources, references or conduct a literature review on REDUNDANCY
We then propose a taxonomy of theoretical contributions typically observed in Information Systems Research (ISR). Based on this taxonomy of contributions, the typical critiques observed in empirical Econ-IS papers, and a set of published papers, we provide some broad guidelines for how authors may craft an effective theoretical contribution for ...
Fault-Tolerant Systems. By C. Mani Krishna. Chapter 3: Information Redundancy. Errors in data may occur when the data are being transferred from one unit to another, from one system to another, or even while the data are stored in a memory unit. To tolerate such errors, we introduce redundancy into the data: this is called information redundancy.
The theme of RAILS 2023 was It Takes a Village: Transforming Information & Library Studies Research and Practice through Partnerships and Co-Design to explore how researchers and practitioners in the library and information field are working collaboratively with each other and with communities to try to move beyond a researcher and ...
As illustrated in Fig. 1, research on microinsurance has surged over the past two decades, and it is now established as a distinct research domain. The first 10 years saw research output increase by tenfold, from around 100 to over 1000 publications annually. Subsequently, the field has stabilised, with approximately 1500 publications each year.
Eukaryotic phytoplankton play a major role in the circulation of material and energy in a lake's ecosystem. The acquisition of information on the eukaryotic phytoplankton community is extremely significant for handling and regulating the ecosystems of lakes. In this study, samples were collected from the western half of Chaohu Lake in the summer and winter periods. Analyses revealed that the ...
Sensors are very import parts of the IoT (Internet of Things). To detect the cleaning loss of combine harvesters during operation, a new detection method based on acoustic signal was proposed. The simulation models of the impact among grain, stem and plate were established by using the ALE algorithm, and the vibration excitation characteristics of the grain and stem were investigated; the ...