
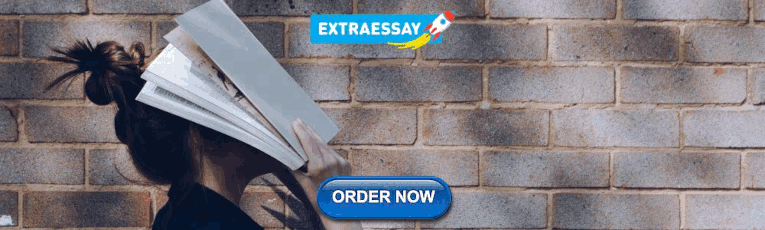
Directional Hypothesis: Definition and 10 Examples
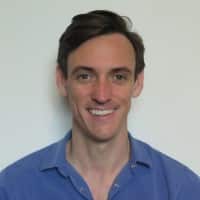
Chris Drew (PhD)
Dr. Chris Drew is the founder of the Helpful Professor. He holds a PhD in education and has published over 20 articles in scholarly journals. He is the former editor of the Journal of Learning Development in Higher Education. [Image Descriptor: Photo of Chris]
Learn about our Editorial Process
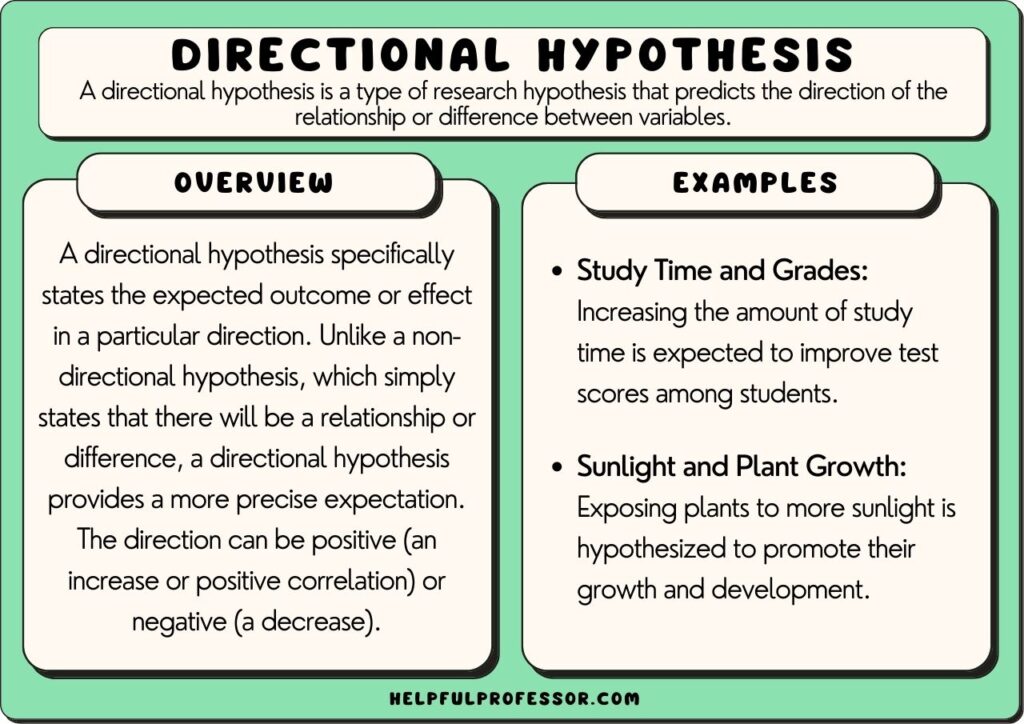
A directional hypothesis refers to a type of hypothesis used in statistical testing that predicts a particular direction of the expected relationship between two variables.
In simpler terms, a directional hypothesis is an educated, specific guess about the direction of an outcome—whether an increase, decrease, or a proclaimed difference in variable sets.
For example, in a study investigating the effects of sleep deprivation on cognitive performance, a directional hypothesis might state that as sleep deprivation (Independent Variable) increases, cognitive performance (Dependent Variable) decreases (Killgore, 2010). Such a hypothesis offers a clear, directional relationship whereby a specific increase or decrease is anticipated.
Global warming provides another notable example of a directional hypothesis. A researcher might hypothesize that as carbon dioxide (CO2) levels increase, global temperatures also increase (Thompson, 2010). In this instance, the hypothesis clearly articulates an upward trend for both variables.
In any given circumstance, it’s imperative that a directional hypothesis is grounded on solid evidence. For instance, the CO2 and global temperature relationship is based on substantial scientific evidence, and not on a random guess or mere speculation (Florides & Christodoulides, 2009).
Directional vs Non-Directional vs Null Hypotheses
A directional hypothesis is generally contrasted to a non-directional hypothesis. Here’s how they compare:
- Directional hypothesis: A directional hypothesis provides a perspective of the expected relationship between variables, predicting the direction of that relationship (either positive, negative, or a specific difference).
- Non-directional hypothesis: A non-directional hypothesis denotes the possibility of a relationship between two variables ( the independent and dependent variables ), although this hypothesis does not venture a prediction as to the direction of this relationship (Ali & Bhaskar, 2016). For example, a non-directional hypothesis might state that there exists a relationship between a person’s diet (independent variable) and their mood (dependent variable), without indicating whether improvement in diet enhances mood positively or negatively. Overall, the choice between a directional or non-directional hypothesis depends on the known or anticipated link between the variables under consideration in research studies.
Another very important type of hypothesis that we need to know about is a null hypothesis :
- Null hypothesis : The null hypothesis stands as a universality—the hypothesis that there is no observed effect in the population under study, meaning there is no association between variables (or that the differences are down to chance). For instance, a null hypothesis could be constructed around the idea that changing diet (independent variable) has no discernible effect on a person’s mood (dependent variable) (Yan & Su, 2016). This proposition is the one that we aim to disprove in an experiment.
While directional and non-directional hypotheses involve some integrated expectations about the outcomes (either distinct direction or a vague relationship), a null hypothesis operates on the premise of negating such relationships or effects.
The null hypotheses is typically proposed to be negated or disproved by statistical tests, paving way for the acceptance of an alternate hypothesis (either directional or non-directional).
Directional Hypothesis Examples
1. exercise and heart health.
Research suggests that as regular physical exercise (independent variable) increases, the risk of heart disease (dependent variable) decreases (Jakicic, Davis, Rogers, King, Marcus, Helsel, Rickman, Wahed, Belle, 2016). In this example, a directional hypothesis anticipates that the more individuals maintain routine workouts, the lesser would be their odds of developing heart-related disorders. This assumption is based on the underlying fact that routine exercise can help reduce harmful cholesterol levels, regulate blood pressure, and bring about overall health benefits. Thus, a direction – a decrease in heart disease – is expected in relation with an increase in exercise.
2. Screen Time and Sleep Quality
Another classic instance of a directional hypothesis can be seen in the relationship between the independent variable, screen time (especially before bed), and the dependent variable, sleep quality. This hypothesis predicts that as screen time before bed increases, sleep quality decreases (Chang, Aeschbach, Duffy, Czeisler, 2015). The reasoning behind this hypothesis is the disruptive effect of artificial light (especially blue light from screens) on melatonin production, a hormone needed to regulate sleep. As individuals spend more time exposed to screens before bed, it is predictably hypothesized that their sleep quality worsens.
3. Job Satisfaction and Employee Turnover
A typical scenario in organizational behavior research posits that as job satisfaction (independent variable) increases, the rate of employee turnover (dependent variable) decreases (Cheng, Jiang, & Riley, 2017). This directional hypothesis emphasizes that an increased level of job satisfaction would lead to a reduced rate of employees leaving the company. The theoretical basis for this hypothesis is that satisfied employees often tend to be more committed to the organization and are less likely to seek employment elsewhere, thus reducing turnover rates.
4. Healthy Eating and Body Weight
Healthy eating, as the independent variable, is commonly thought to influence body weight, the dependent variable, in a positive way. For example, the hypothesis might state that as consumption of healthy foods increases, an individual’s body weight decreases (Framson, Kristal, Schenk, Littman, Zeliadt, & Benitez, 2009). This projection is based on the premise that healthier foods, such as fruits and vegetables, are generally lower in calories than junk food, assisting in weight management.
5. Sun Exposure and Skin Health
The association between sun exposure (independent variable) and skin health (dependent variable) allows for a definitive hypothesis declaring that as sun exposure increases, the risk of skin damage or skin cancer increases (Whiteman, Whiteman, & Green, 2001). The premise aligns with the understanding that overexposure to the sun’s ultraviolet rays can deteriorate skin health, leading to conditions like sunburn or, in extreme cases, skin cancer.
6. Study Hours and Academic Performance
A regularly assessed relationship in academia suggests that as the number of study hours (independent variable) rises, so too does academic performance (dependent variable) (Nonis, Hudson, Logan, Ford, 2013). The hypothesis proposes a positive correlation , with an increase in study time expected to contribute to enhanced academic outcomes.
7. Screen Time and Eye Strain
It’s commonly hypothesized that as screen time (independent variable) increases, the likelihood of experiencing eye strain (dependent variable) also increases (Sheppard & Wolffsohn, 2018). This is based on the idea that prolonged engagement with digital screens—computers, tablets, or mobile phones—can cause discomfort or fatigue in the eyes, attributing to symptoms of eye strain.
8. Physical Activity and Stress Levels
In the sphere of mental health, it’s often proposed that as physical activity (independent variable) increases, levels of stress (dependent variable) decrease (Stonerock, Hoffman, Smith, Blumenthal, 2015). Regular exercise is known to stimulate the production of endorphins, the body’s natural mood elevators, helping to alleviate stress.
9. Water Consumption and Kidney Health
A common health-related hypothesis might predict that as water consumption (independent variable) increases, the risk of kidney stones (dependent variable) decreases (Curhan, Willett, Knight, & Stampfer, 2004). Here, an increase in water intake is inferred to reduce the risk of kidney stones by diluting the substances that lead to stone formation.
10. Traffic Noise and Sleep Quality
In urban planning research, it’s often supposed that as traffic noise (independent variable) increases, sleep quality (dependent variable) decreases (Muzet, 2007). Increased noise levels, particularly during the night, can result in sleep disruptions, thus, leading to poor sleep quality.
11. Sugar Consumption and Dental Health
In the field of dental health, an example might be stating as one’s sugar consumption (independent variable) increases, dental health (dependent variable) decreases (Sheiham, & James, 2014). This stems from the fact that sugar is a major factor in tooth decay, and increased consumption of sugary foods or drinks leads to a decline in dental health due to the high likelihood of cavities.
See 15 More Examples of Hypotheses Here
A directional hypothesis plays a critical role in research, paving the way for specific predicted outcomes based on the relationship between two variables. These hypotheses clearly illuminate the expected direction—the increase or decrease—of an effect. From predicting the impacts of healthy eating on body weight to forecasting the influence of screen time on sleep quality, directional hypotheses allow for targeted and strategic examination of phenomena. In essence, directional hypotheses provide the crucial path for inquiry, shaping the trajectory of research studies and ultimately aiding in the generation of insightful, relevant findings.
Ali, S., & Bhaskar, S. (2016). Basic statistical tools in research and data analysis. Indian Journal of Anaesthesia, 60 (9), 662-669. doi: https://doi.org/10.4103%2F0019-5049.190623
Chang, A. M., Aeschbach, D., Duffy, J. F., & Czeisler, C. A. (2015). Evening use of light-emitting eReaders negatively affects sleep, circadian timing, and next-morning alertness. Proceeding of the National Academy of Sciences, 112 (4), 1232-1237. doi: https://doi.org/10.1073/pnas.1418490112
Cheng, G. H. L., Jiang, D., & Riley, J. H. (2017). Organizational commitment and intrinsic motivation of regular and contractual primary school teachers in China. New Psychology, 19 (3), 316-326. Doi: https://doi.org/10.4103%2F2249-4863.184631
Curhan, G. C., Willett, W. C., Knight, E. L., & Stampfer, M. J. (2004). Dietary factors and the risk of incident kidney stones in younger women: Nurses’ Health Study II. Archives of Internal Medicine, 164 (8), 885–891.
Florides, G. A., & Christodoulides, P. (2009). Global warming and carbon dioxide through sciences. Environment international , 35 (2), 390-401. doi: https://doi.org/10.1016/j.envint.2008.07.007
Framson, C., Kristal, A. R., Schenk, J. M., Littman, A. J., Zeliadt, S., & Benitez, D. (2009). Development and validation of the mindful eating questionnaire. Journal of the American Dietetic Association, 109 (8), 1439-1444. doi: https://doi.org/10.1016/j.jada.2009.05.006
Jakicic, J. M., Davis, K. K., Rogers, R. J., King, W. C., Marcus, M. D., Helsel, D., … & Belle, S. H. (2016). Effect of wearable technology combined with a lifestyle intervention on long-term weight loss: The IDEA randomized clinical trial. JAMA, 316 (11), 1161-1171.
Khan, S., & Iqbal, N. (2013). Study of the relationship between study habits and academic achievement of students: A case of SPSS model. Higher Education Studies, 3 (1), 14-26.
Killgore, W. D. (2010). Effects of sleep deprivation on cognition. Progress in brain research , 185 , 105-129. doi: https://doi.org/10.1016/B978-0-444-53702-7.00007-5
Marczinski, C. A., & Fillmore, M. T. (2014). Dissociative antagonistic effects of caffeine on alcohol-induced impairment of behavioral control. Experimental and Clinical Psychopharmacology, 22 (4), 298–311. doi: https://psycnet.apa.org/doi/10.1037/1064-1297.11.3.228
Muzet, A. (2007). Environmental Noise, Sleep and Health. Sleep Medicine Reviews, 11 (2), 135-142. doi: https://doi.org/10.1016/j.smrv.2006.09.001
Nonis, S. A., Hudson, G. I., Logan, L. B., & Ford, C. W. (2013). Influence of perceived control over time on college students’ stress and stress-related outcomes. Research in Higher Education, 54 (5), 536-552. doi: https://doi.org/10.1023/A:1018753706925
Sheiham, A., & James, W. P. (2014). A new understanding of the relationship between sugars, dental caries and fluoride use: implications for limits on sugars consumption. Public health nutrition, 17 (10), 2176-2184. Doi: https://doi.org/10.1017/S136898001400113X
Sheppard, A. L., & Wolffsohn, J. S. (2018). Digital eye strain: prevalence, measurement and amelioration. BMJ open ophthalmology , 3 (1), e000146. doi: http://dx.doi.org/10.1136/bmjophth-2018-000146
Stonerock, G. L., Hoffman, B. M., Smith, P. J., & Blumenthal, J. A. (2015). Exercise as Treatment for Anxiety: Systematic Review and Analysis. Annals of Behavioral Medicine, 49 (4), 542–556. doi: https://doi.org/10.1007/s12160-014-9685-9
Thompson, L. G. (2010). Climate change: The evidence and our options. The Behavior Analyst , 33 , 153-170. Doi: https://doi.org/10.1007/BF03392211
Whiteman, D. C., Whiteman, C. A., & Green, A. C. (2001). Childhood sun exposure as a risk factor for melanoma: a systematic review of epidemiologic studies. Cancer Causes & Control, 12 (1), 69-82. doi: https://doi.org/10.1023/A:1008980919928
Yan, X., & Su, X. (2009). Linear regression analysis: theory and computing . New Jersey: World Scientific.
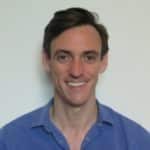
- Chris Drew (PhD) https://helpfulprofessor.com/author/chris-drew-phd-2/ 10 Reasons you’re Perpetually Single
- Chris Drew (PhD) https://helpfulprofessor.com/author/chris-drew-phd-2/ 20 Montessori Toddler Bedrooms (Design Inspiration)
- Chris Drew (PhD) https://helpfulprofessor.com/author/chris-drew-phd-2/ 21 Montessori Homeschool Setups
- Chris Drew (PhD) https://helpfulprofessor.com/author/chris-drew-phd-2/ 101 Hidden Talents Examples
Leave a Comment Cancel Reply
Your email address will not be published. Required fields are marked *

An official website of the United States government
The .gov means it’s official. Federal government websites often end in .gov or .mil. Before sharing sensitive information, make sure you’re on a federal government site.
The site is secure. The https:// ensures that you are connecting to the official website and that any information you provide is encrypted and transmitted securely.
- Publications
- Account settings
Preview improvements coming to the PMC website in October 2024. Learn More or Try it out now .
- Advanced Search
- Journal List
- J Korean Med Sci
- v.37(16); 2022 Apr 25

A Practical Guide to Writing Quantitative and Qualitative Research Questions and Hypotheses in Scholarly Articles
Edward barroga.
1 Department of General Education, Graduate School of Nursing Science, St. Luke’s International University, Tokyo, Japan.
Glafera Janet Matanguihan
2 Department of Biological Sciences, Messiah University, Mechanicsburg, PA, USA.
The development of research questions and the subsequent hypotheses are prerequisites to defining the main research purpose and specific objectives of a study. Consequently, these objectives determine the study design and research outcome. The development of research questions is a process based on knowledge of current trends, cutting-edge studies, and technological advances in the research field. Excellent research questions are focused and require a comprehensive literature search and in-depth understanding of the problem being investigated. Initially, research questions may be written as descriptive questions which could be developed into inferential questions. These questions must be specific and concise to provide a clear foundation for developing hypotheses. Hypotheses are more formal predictions about the research outcomes. These specify the possible results that may or may not be expected regarding the relationship between groups. Thus, research questions and hypotheses clarify the main purpose and specific objectives of the study, which in turn dictate the design of the study, its direction, and outcome. Studies developed from good research questions and hypotheses will have trustworthy outcomes with wide-ranging social and health implications.
INTRODUCTION
Scientific research is usually initiated by posing evidenced-based research questions which are then explicitly restated as hypotheses. 1 , 2 The hypotheses provide directions to guide the study, solutions, explanations, and expected results. 3 , 4 Both research questions and hypotheses are essentially formulated based on conventional theories and real-world processes, which allow the inception of novel studies and the ethical testing of ideas. 5 , 6
It is crucial to have knowledge of both quantitative and qualitative research 2 as both types of research involve writing research questions and hypotheses. 7 However, these crucial elements of research are sometimes overlooked; if not overlooked, then framed without the forethought and meticulous attention it needs. Planning and careful consideration are needed when developing quantitative or qualitative research, particularly when conceptualizing research questions and hypotheses. 4
There is a continuing need to support researchers in the creation of innovative research questions and hypotheses, as well as for journal articles that carefully review these elements. 1 When research questions and hypotheses are not carefully thought of, unethical studies and poor outcomes usually ensue. Carefully formulated research questions and hypotheses define well-founded objectives, which in turn determine the appropriate design, course, and outcome of the study. This article then aims to discuss in detail the various aspects of crafting research questions and hypotheses, with the goal of guiding researchers as they develop their own. Examples from the authors and peer-reviewed scientific articles in the healthcare field are provided to illustrate key points.
DEFINITIONS AND RELATIONSHIP OF RESEARCH QUESTIONS AND HYPOTHESES
A research question is what a study aims to answer after data analysis and interpretation. The answer is written in length in the discussion section of the paper. Thus, the research question gives a preview of the different parts and variables of the study meant to address the problem posed in the research question. 1 An excellent research question clarifies the research writing while facilitating understanding of the research topic, objective, scope, and limitations of the study. 5
On the other hand, a research hypothesis is an educated statement of an expected outcome. This statement is based on background research and current knowledge. 8 , 9 The research hypothesis makes a specific prediction about a new phenomenon 10 or a formal statement on the expected relationship between an independent variable and a dependent variable. 3 , 11 It provides a tentative answer to the research question to be tested or explored. 4
Hypotheses employ reasoning to predict a theory-based outcome. 10 These can also be developed from theories by focusing on components of theories that have not yet been observed. 10 The validity of hypotheses is often based on the testability of the prediction made in a reproducible experiment. 8
Conversely, hypotheses can also be rephrased as research questions. Several hypotheses based on existing theories and knowledge may be needed to answer a research question. Developing ethical research questions and hypotheses creates a research design that has logical relationships among variables. These relationships serve as a solid foundation for the conduct of the study. 4 , 11 Haphazardly constructed research questions can result in poorly formulated hypotheses and improper study designs, leading to unreliable results. Thus, the formulations of relevant research questions and verifiable hypotheses are crucial when beginning research. 12
CHARACTERISTICS OF GOOD RESEARCH QUESTIONS AND HYPOTHESES
Excellent research questions are specific and focused. These integrate collective data and observations to confirm or refute the subsequent hypotheses. Well-constructed hypotheses are based on previous reports and verify the research context. These are realistic, in-depth, sufficiently complex, and reproducible. More importantly, these hypotheses can be addressed and tested. 13
There are several characteristics of well-developed hypotheses. Good hypotheses are 1) empirically testable 7 , 10 , 11 , 13 ; 2) backed by preliminary evidence 9 ; 3) testable by ethical research 7 , 9 ; 4) based on original ideas 9 ; 5) have evidenced-based logical reasoning 10 ; and 6) can be predicted. 11 Good hypotheses can infer ethical and positive implications, indicating the presence of a relationship or effect relevant to the research theme. 7 , 11 These are initially developed from a general theory and branch into specific hypotheses by deductive reasoning. In the absence of a theory to base the hypotheses, inductive reasoning based on specific observations or findings form more general hypotheses. 10
TYPES OF RESEARCH QUESTIONS AND HYPOTHESES
Research questions and hypotheses are developed according to the type of research, which can be broadly classified into quantitative and qualitative research. We provide a summary of the types of research questions and hypotheses under quantitative and qualitative research categories in Table 1 .
Quantitative research questions | Quantitative research hypotheses |
---|---|
Descriptive research questions | Simple hypothesis |
Comparative research questions | Complex hypothesis |
Relationship research questions | Directional hypothesis |
Non-directional hypothesis | |
Associative hypothesis | |
Causal hypothesis | |
Null hypothesis | |
Alternative hypothesis | |
Working hypothesis | |
Statistical hypothesis | |
Logical hypothesis | |
Hypothesis-testing | |
Qualitative research questions | Qualitative research hypotheses |
Contextual research questions | Hypothesis-generating |
Descriptive research questions | |
Evaluation research questions | |
Explanatory research questions | |
Exploratory research questions | |
Generative research questions | |
Ideological research questions | |
Ethnographic research questions | |
Phenomenological research questions | |
Grounded theory questions | |
Qualitative case study questions |
Research questions in quantitative research
In quantitative research, research questions inquire about the relationships among variables being investigated and are usually framed at the start of the study. These are precise and typically linked to the subject population, dependent and independent variables, and research design. 1 Research questions may also attempt to describe the behavior of a population in relation to one or more variables, or describe the characteristics of variables to be measured ( descriptive research questions ). 1 , 5 , 14 These questions may also aim to discover differences between groups within the context of an outcome variable ( comparative research questions ), 1 , 5 , 14 or elucidate trends and interactions among variables ( relationship research questions ). 1 , 5 We provide examples of descriptive, comparative, and relationship research questions in quantitative research in Table 2 .
Quantitative research questions | |
---|---|
Descriptive research question | |
- Measures responses of subjects to variables | |
- Presents variables to measure, analyze, or assess | |
What is the proportion of resident doctors in the hospital who have mastered ultrasonography (response of subjects to a variable) as a diagnostic technique in their clinical training? | |
Comparative research question | |
- Clarifies difference between one group with outcome variable and another group without outcome variable | |
Is there a difference in the reduction of lung metastasis in osteosarcoma patients who received the vitamin D adjunctive therapy (group with outcome variable) compared with osteosarcoma patients who did not receive the vitamin D adjunctive therapy (group without outcome variable)? | |
- Compares the effects of variables | |
How does the vitamin D analogue 22-Oxacalcitriol (variable 1) mimic the antiproliferative activity of 1,25-Dihydroxyvitamin D (variable 2) in osteosarcoma cells? | |
Relationship research question | |
- Defines trends, association, relationships, or interactions between dependent variable and independent variable | |
Is there a relationship between the number of medical student suicide (dependent variable) and the level of medical student stress (independent variable) in Japan during the first wave of the COVID-19 pandemic? |
Hypotheses in quantitative research
In quantitative research, hypotheses predict the expected relationships among variables. 15 Relationships among variables that can be predicted include 1) between a single dependent variable and a single independent variable ( simple hypothesis ) or 2) between two or more independent and dependent variables ( complex hypothesis ). 4 , 11 Hypotheses may also specify the expected direction to be followed and imply an intellectual commitment to a particular outcome ( directional hypothesis ) 4 . On the other hand, hypotheses may not predict the exact direction and are used in the absence of a theory, or when findings contradict previous studies ( non-directional hypothesis ). 4 In addition, hypotheses can 1) define interdependency between variables ( associative hypothesis ), 4 2) propose an effect on the dependent variable from manipulation of the independent variable ( causal hypothesis ), 4 3) state a negative relationship between two variables ( null hypothesis ), 4 , 11 , 15 4) replace the working hypothesis if rejected ( alternative hypothesis ), 15 explain the relationship of phenomena to possibly generate a theory ( working hypothesis ), 11 5) involve quantifiable variables that can be tested statistically ( statistical hypothesis ), 11 6) or express a relationship whose interlinks can be verified logically ( logical hypothesis ). 11 We provide examples of simple, complex, directional, non-directional, associative, causal, null, alternative, working, statistical, and logical hypotheses in quantitative research, as well as the definition of quantitative hypothesis-testing research in Table 3 .
Quantitative research hypotheses | |
---|---|
Simple hypothesis | |
- Predicts relationship between single dependent variable and single independent variable | |
If the dose of the new medication (single independent variable) is high, blood pressure (single dependent variable) is lowered. | |
Complex hypothesis | |
- Foretells relationship between two or more independent and dependent variables | |
The higher the use of anticancer drugs, radiation therapy, and adjunctive agents (3 independent variables), the higher would be the survival rate (1 dependent variable). | |
Directional hypothesis | |
- Identifies study direction based on theory towards particular outcome to clarify relationship between variables | |
Privately funded research projects will have a larger international scope (study direction) than publicly funded research projects. | |
Non-directional hypothesis | |
- Nature of relationship between two variables or exact study direction is not identified | |
- Does not involve a theory | |
Women and men are different in terms of helpfulness. (Exact study direction is not identified) | |
Associative hypothesis | |
- Describes variable interdependency | |
- Change in one variable causes change in another variable | |
A larger number of people vaccinated against COVID-19 in the region (change in independent variable) will reduce the region’s incidence of COVID-19 infection (change in dependent variable). | |
Causal hypothesis | |
- An effect on dependent variable is predicted from manipulation of independent variable | |
A change into a high-fiber diet (independent variable) will reduce the blood sugar level (dependent variable) of the patient. | |
Null hypothesis | |
- A negative statement indicating no relationship or difference between 2 variables | |
There is no significant difference in the severity of pulmonary metastases between the new drug (variable 1) and the current drug (variable 2). | |
Alternative hypothesis | |
- Following a null hypothesis, an alternative hypothesis predicts a relationship between 2 study variables | |
The new drug (variable 1) is better on average in reducing the level of pain from pulmonary metastasis than the current drug (variable 2). | |
Working hypothesis | |
- A hypothesis that is initially accepted for further research to produce a feasible theory | |
Dairy cows fed with concentrates of different formulations will produce different amounts of milk. | |
Statistical hypothesis | |
- Assumption about the value of population parameter or relationship among several population characteristics | |
- Validity tested by a statistical experiment or analysis | |
The mean recovery rate from COVID-19 infection (value of population parameter) is not significantly different between population 1 and population 2. | |
There is a positive correlation between the level of stress at the workplace and the number of suicides (population characteristics) among working people in Japan. | |
Logical hypothesis | |
- Offers or proposes an explanation with limited or no extensive evidence | |
If healthcare workers provide more educational programs about contraception methods, the number of adolescent pregnancies will be less. | |
Hypothesis-testing (Quantitative hypothesis-testing research) | |
- Quantitative research uses deductive reasoning. | |
- This involves the formation of a hypothesis, collection of data in the investigation of the problem, analysis and use of the data from the investigation, and drawing of conclusions to validate or nullify the hypotheses. |
Research questions in qualitative research
Unlike research questions in quantitative research, research questions in qualitative research are usually continuously reviewed and reformulated. The central question and associated subquestions are stated more than the hypotheses. 15 The central question broadly explores a complex set of factors surrounding the central phenomenon, aiming to present the varied perspectives of participants. 15
There are varied goals for which qualitative research questions are developed. These questions can function in several ways, such as to 1) identify and describe existing conditions ( contextual research question s); 2) describe a phenomenon ( descriptive research questions ); 3) assess the effectiveness of existing methods, protocols, theories, or procedures ( evaluation research questions ); 4) examine a phenomenon or analyze the reasons or relationships between subjects or phenomena ( explanatory research questions ); or 5) focus on unknown aspects of a particular topic ( exploratory research questions ). 5 In addition, some qualitative research questions provide new ideas for the development of theories and actions ( generative research questions ) or advance specific ideologies of a position ( ideological research questions ). 1 Other qualitative research questions may build on a body of existing literature and become working guidelines ( ethnographic research questions ). Research questions may also be broadly stated without specific reference to the existing literature or a typology of questions ( phenomenological research questions ), may be directed towards generating a theory of some process ( grounded theory questions ), or may address a description of the case and the emerging themes ( qualitative case study questions ). 15 We provide examples of contextual, descriptive, evaluation, explanatory, exploratory, generative, ideological, ethnographic, phenomenological, grounded theory, and qualitative case study research questions in qualitative research in Table 4 , and the definition of qualitative hypothesis-generating research in Table 5 .
Qualitative research questions | |
---|---|
Contextual research question | |
- Ask the nature of what already exists | |
- Individuals or groups function to further clarify and understand the natural context of real-world problems | |
What are the experiences of nurses working night shifts in healthcare during the COVID-19 pandemic? (natural context of real-world problems) | |
Descriptive research question | |
- Aims to describe a phenomenon | |
What are the different forms of disrespect and abuse (phenomenon) experienced by Tanzanian women when giving birth in healthcare facilities? | |
Evaluation research question | |
- Examines the effectiveness of existing practice or accepted frameworks | |
How effective are decision aids (effectiveness of existing practice) in helping decide whether to give birth at home or in a healthcare facility? | |
Explanatory research question | |
- Clarifies a previously studied phenomenon and explains why it occurs | |
Why is there an increase in teenage pregnancy (phenomenon) in Tanzania? | |
Exploratory research question | |
- Explores areas that have not been fully investigated to have a deeper understanding of the research problem | |
What factors affect the mental health of medical students (areas that have not yet been fully investigated) during the COVID-19 pandemic? | |
Generative research question | |
- Develops an in-depth understanding of people’s behavior by asking ‘how would’ or ‘what if’ to identify problems and find solutions | |
How would the extensive research experience of the behavior of new staff impact the success of the novel drug initiative? | |
Ideological research question | |
- Aims to advance specific ideas or ideologies of a position | |
Are Japanese nurses who volunteer in remote African hospitals able to promote humanized care of patients (specific ideas or ideologies) in the areas of safe patient environment, respect of patient privacy, and provision of accurate information related to health and care? | |
Ethnographic research question | |
- Clarifies peoples’ nature, activities, their interactions, and the outcomes of their actions in specific settings | |
What are the demographic characteristics, rehabilitative treatments, community interactions, and disease outcomes (nature, activities, their interactions, and the outcomes) of people in China who are suffering from pneumoconiosis? | |
Phenomenological research question | |
- Knows more about the phenomena that have impacted an individual | |
What are the lived experiences of parents who have been living with and caring for children with a diagnosis of autism? (phenomena that have impacted an individual) | |
Grounded theory question | |
- Focuses on social processes asking about what happens and how people interact, or uncovering social relationships and behaviors of groups | |
What are the problems that pregnant adolescents face in terms of social and cultural norms (social processes), and how can these be addressed? | |
Qualitative case study question | |
- Assesses a phenomenon using different sources of data to answer “why” and “how” questions | |
- Considers how the phenomenon is influenced by its contextual situation. | |
How does quitting work and assuming the role of a full-time mother (phenomenon assessed) change the lives of women in Japan? |
Qualitative research hypotheses | |
---|---|
Hypothesis-generating (Qualitative hypothesis-generating research) | |
- Qualitative research uses inductive reasoning. | |
- This involves data collection from study participants or the literature regarding a phenomenon of interest, using the collected data to develop a formal hypothesis, and using the formal hypothesis as a framework for testing the hypothesis. | |
- Qualitative exploratory studies explore areas deeper, clarifying subjective experience and allowing formulation of a formal hypothesis potentially testable in a future quantitative approach. |
Qualitative studies usually pose at least one central research question and several subquestions starting with How or What . These research questions use exploratory verbs such as explore or describe . These also focus on one central phenomenon of interest, and may mention the participants and research site. 15
Hypotheses in qualitative research
Hypotheses in qualitative research are stated in the form of a clear statement concerning the problem to be investigated. Unlike in quantitative research where hypotheses are usually developed to be tested, qualitative research can lead to both hypothesis-testing and hypothesis-generating outcomes. 2 When studies require both quantitative and qualitative research questions, this suggests an integrative process between both research methods wherein a single mixed-methods research question can be developed. 1
FRAMEWORKS FOR DEVELOPING RESEARCH QUESTIONS AND HYPOTHESES
Research questions followed by hypotheses should be developed before the start of the study. 1 , 12 , 14 It is crucial to develop feasible research questions on a topic that is interesting to both the researcher and the scientific community. This can be achieved by a meticulous review of previous and current studies to establish a novel topic. Specific areas are subsequently focused on to generate ethical research questions. The relevance of the research questions is evaluated in terms of clarity of the resulting data, specificity of the methodology, objectivity of the outcome, depth of the research, and impact of the study. 1 , 5 These aspects constitute the FINER criteria (i.e., Feasible, Interesting, Novel, Ethical, and Relevant). 1 Clarity and effectiveness are achieved if research questions meet the FINER criteria. In addition to the FINER criteria, Ratan et al. described focus, complexity, novelty, feasibility, and measurability for evaluating the effectiveness of research questions. 14
The PICOT and PEO frameworks are also used when developing research questions. 1 The following elements are addressed in these frameworks, PICOT: P-population/patients/problem, I-intervention or indicator being studied, C-comparison group, O-outcome of interest, and T-timeframe of the study; PEO: P-population being studied, E-exposure to preexisting conditions, and O-outcome of interest. 1 Research questions are also considered good if these meet the “FINERMAPS” framework: Feasible, Interesting, Novel, Ethical, Relevant, Manageable, Appropriate, Potential value/publishable, and Systematic. 14
As we indicated earlier, research questions and hypotheses that are not carefully formulated result in unethical studies or poor outcomes. To illustrate this, we provide some examples of ambiguous research question and hypotheses that result in unclear and weak research objectives in quantitative research ( Table 6 ) 16 and qualitative research ( Table 7 ) 17 , and how to transform these ambiguous research question(s) and hypothesis(es) into clear and good statements.
Variables | Unclear and weak statement (Statement 1) | Clear and good statement (Statement 2) | Points to avoid |
---|---|---|---|
Research question | Which is more effective between smoke moxibustion and smokeless moxibustion? | “Moreover, regarding smoke moxibustion versus smokeless moxibustion, it remains unclear which is more effective, safe, and acceptable to pregnant women, and whether there is any difference in the amount of heat generated.” | 1) Vague and unfocused questions |
2) Closed questions simply answerable by yes or no | |||
3) Questions requiring a simple choice | |||
Hypothesis | The smoke moxibustion group will have higher cephalic presentation. | “Hypothesis 1. The smoke moxibustion stick group (SM group) and smokeless moxibustion stick group (-SLM group) will have higher rates of cephalic presentation after treatment than the control group. | 1) Unverifiable hypotheses |
Hypothesis 2. The SM group and SLM group will have higher rates of cephalic presentation at birth than the control group. | 2) Incompletely stated groups of comparison | ||
Hypothesis 3. There will be no significant differences in the well-being of the mother and child among the three groups in terms of the following outcomes: premature birth, premature rupture of membranes (PROM) at < 37 weeks, Apgar score < 7 at 5 min, umbilical cord blood pH < 7.1, admission to neonatal intensive care unit (NICU), and intrauterine fetal death.” | 3) Insufficiently described variables or outcomes | ||
Research objective | To determine which is more effective between smoke moxibustion and smokeless moxibustion. | “The specific aims of this pilot study were (a) to compare the effects of smoke moxibustion and smokeless moxibustion treatments with the control group as a possible supplement to ECV for converting breech presentation to cephalic presentation and increasing adherence to the newly obtained cephalic position, and (b) to assess the effects of these treatments on the well-being of the mother and child.” | 1) Poor understanding of the research question and hypotheses |
2) Insufficient description of population, variables, or study outcomes |
a These statements were composed for comparison and illustrative purposes only.
b These statements are direct quotes from Higashihara and Horiuchi. 16
Variables | Unclear and weak statement (Statement 1) | Clear and good statement (Statement 2) | Points to avoid |
---|---|---|---|
Research question | Does disrespect and abuse (D&A) occur in childbirth in Tanzania? | How does disrespect and abuse (D&A) occur and what are the types of physical and psychological abuses observed in midwives’ actual care during facility-based childbirth in urban Tanzania? | 1) Ambiguous or oversimplistic questions |
2) Questions unverifiable by data collection and analysis | |||
Hypothesis | Disrespect and abuse (D&A) occur in childbirth in Tanzania. | Hypothesis 1: Several types of physical and psychological abuse by midwives in actual care occur during facility-based childbirth in urban Tanzania. | 1) Statements simply expressing facts |
Hypothesis 2: Weak nursing and midwifery management contribute to the D&A of women during facility-based childbirth in urban Tanzania. | 2) Insufficiently described concepts or variables | ||
Research objective | To describe disrespect and abuse (D&A) in childbirth in Tanzania. | “This study aimed to describe from actual observations the respectful and disrespectful care received by women from midwives during their labor period in two hospitals in urban Tanzania.” | 1) Statements unrelated to the research question and hypotheses |
2) Unattainable or unexplorable objectives |
a This statement is a direct quote from Shimoda et al. 17
The other statements were composed for comparison and illustrative purposes only.
CONSTRUCTING RESEARCH QUESTIONS AND HYPOTHESES
To construct effective research questions and hypotheses, it is very important to 1) clarify the background and 2) identify the research problem at the outset of the research, within a specific timeframe. 9 Then, 3) review or conduct preliminary research to collect all available knowledge about the possible research questions by studying theories and previous studies. 18 Afterwards, 4) construct research questions to investigate the research problem. Identify variables to be accessed from the research questions 4 and make operational definitions of constructs from the research problem and questions. Thereafter, 5) construct specific deductive or inductive predictions in the form of hypotheses. 4 Finally, 6) state the study aims . This general flow for constructing effective research questions and hypotheses prior to conducting research is shown in Fig. 1 .

Research questions are used more frequently in qualitative research than objectives or hypotheses. 3 These questions seek to discover, understand, explore or describe experiences by asking “What” or “How.” The questions are open-ended to elicit a description rather than to relate variables or compare groups. The questions are continually reviewed, reformulated, and changed during the qualitative study. 3 Research questions are also used more frequently in survey projects than hypotheses in experiments in quantitative research to compare variables and their relationships.
Hypotheses are constructed based on the variables identified and as an if-then statement, following the template, ‘If a specific action is taken, then a certain outcome is expected.’ At this stage, some ideas regarding expectations from the research to be conducted must be drawn. 18 Then, the variables to be manipulated (independent) and influenced (dependent) are defined. 4 Thereafter, the hypothesis is stated and refined, and reproducible data tailored to the hypothesis are identified, collected, and analyzed. 4 The hypotheses must be testable and specific, 18 and should describe the variables and their relationships, the specific group being studied, and the predicted research outcome. 18 Hypotheses construction involves a testable proposition to be deduced from theory, and independent and dependent variables to be separated and measured separately. 3 Therefore, good hypotheses must be based on good research questions constructed at the start of a study or trial. 12
In summary, research questions are constructed after establishing the background of the study. Hypotheses are then developed based on the research questions. Thus, it is crucial to have excellent research questions to generate superior hypotheses. In turn, these would determine the research objectives and the design of the study, and ultimately, the outcome of the research. 12 Algorithms for building research questions and hypotheses are shown in Fig. 2 for quantitative research and in Fig. 3 for qualitative research.

EXAMPLES OF RESEARCH QUESTIONS FROM PUBLISHED ARTICLES
- EXAMPLE 1. Descriptive research question (quantitative research)
- - Presents research variables to be assessed (distinct phenotypes and subphenotypes)
- “BACKGROUND: Since COVID-19 was identified, its clinical and biological heterogeneity has been recognized. Identifying COVID-19 phenotypes might help guide basic, clinical, and translational research efforts.
- RESEARCH QUESTION: Does the clinical spectrum of patients with COVID-19 contain distinct phenotypes and subphenotypes? ” 19
- EXAMPLE 2. Relationship research question (quantitative research)
- - Shows interactions between dependent variable (static postural control) and independent variable (peripheral visual field loss)
- “Background: Integration of visual, vestibular, and proprioceptive sensations contributes to postural control. People with peripheral visual field loss have serious postural instability. However, the directional specificity of postural stability and sensory reweighting caused by gradual peripheral visual field loss remain unclear.
- Research question: What are the effects of peripheral visual field loss on static postural control ?” 20
- EXAMPLE 3. Comparative research question (quantitative research)
- - Clarifies the difference among groups with an outcome variable (patients enrolled in COMPERA with moderate PH or severe PH in COPD) and another group without the outcome variable (patients with idiopathic pulmonary arterial hypertension (IPAH))
- “BACKGROUND: Pulmonary hypertension (PH) in COPD is a poorly investigated clinical condition.
- RESEARCH QUESTION: Which factors determine the outcome of PH in COPD?
- STUDY DESIGN AND METHODS: We analyzed the characteristics and outcome of patients enrolled in the Comparative, Prospective Registry of Newly Initiated Therapies for Pulmonary Hypertension (COMPERA) with moderate or severe PH in COPD as defined during the 6th PH World Symposium who received medical therapy for PH and compared them with patients with idiopathic pulmonary arterial hypertension (IPAH) .” 21
- EXAMPLE 4. Exploratory research question (qualitative research)
- - Explores areas that have not been fully investigated (perspectives of families and children who receive care in clinic-based child obesity treatment) to have a deeper understanding of the research problem
- “Problem: Interventions for children with obesity lead to only modest improvements in BMI and long-term outcomes, and data are limited on the perspectives of families of children with obesity in clinic-based treatment. This scoping review seeks to answer the question: What is known about the perspectives of families and children who receive care in clinic-based child obesity treatment? This review aims to explore the scope of perspectives reported by families of children with obesity who have received individualized outpatient clinic-based obesity treatment.” 22
- EXAMPLE 5. Relationship research question (quantitative research)
- - Defines interactions between dependent variable (use of ankle strategies) and independent variable (changes in muscle tone)
- “Background: To maintain an upright standing posture against external disturbances, the human body mainly employs two types of postural control strategies: “ankle strategy” and “hip strategy.” While it has been reported that the magnitude of the disturbance alters the use of postural control strategies, it has not been elucidated how the level of muscle tone, one of the crucial parameters of bodily function, determines the use of each strategy. We have previously confirmed using forward dynamics simulations of human musculoskeletal models that an increased muscle tone promotes the use of ankle strategies. The objective of the present study was to experimentally evaluate a hypothesis: an increased muscle tone promotes the use of ankle strategies. Research question: Do changes in the muscle tone affect the use of ankle strategies ?” 23
EXAMPLES OF HYPOTHESES IN PUBLISHED ARTICLES
- EXAMPLE 1. Working hypothesis (quantitative research)
- - A hypothesis that is initially accepted for further research to produce a feasible theory
- “As fever may have benefit in shortening the duration of viral illness, it is plausible to hypothesize that the antipyretic efficacy of ibuprofen may be hindering the benefits of a fever response when taken during the early stages of COVID-19 illness .” 24
- “In conclusion, it is plausible to hypothesize that the antipyretic efficacy of ibuprofen may be hindering the benefits of a fever response . The difference in perceived safety of these agents in COVID-19 illness could be related to the more potent efficacy to reduce fever with ibuprofen compared to acetaminophen. Compelling data on the benefit of fever warrant further research and review to determine when to treat or withhold ibuprofen for early stage fever for COVID-19 and other related viral illnesses .” 24
- EXAMPLE 2. Exploratory hypothesis (qualitative research)
- - Explores particular areas deeper to clarify subjective experience and develop a formal hypothesis potentially testable in a future quantitative approach
- “We hypothesized that when thinking about a past experience of help-seeking, a self distancing prompt would cause increased help-seeking intentions and more favorable help-seeking outcome expectations .” 25
- “Conclusion
- Although a priori hypotheses were not supported, further research is warranted as results indicate the potential for using self-distancing approaches to increasing help-seeking among some people with depressive symptomatology.” 25
- EXAMPLE 3. Hypothesis-generating research to establish a framework for hypothesis testing (qualitative research)
- “We hypothesize that compassionate care is beneficial for patients (better outcomes), healthcare systems and payers (lower costs), and healthcare providers (lower burnout). ” 26
- Compassionomics is the branch of knowledge and scientific study of the effects of compassionate healthcare. Our main hypotheses are that compassionate healthcare is beneficial for (1) patients, by improving clinical outcomes, (2) healthcare systems and payers, by supporting financial sustainability, and (3) HCPs, by lowering burnout and promoting resilience and well-being. The purpose of this paper is to establish a scientific framework for testing the hypotheses above . If these hypotheses are confirmed through rigorous research, compassionomics will belong in the science of evidence-based medicine, with major implications for all healthcare domains.” 26
- EXAMPLE 4. Statistical hypothesis (quantitative research)
- - An assumption is made about the relationship among several population characteristics ( gender differences in sociodemographic and clinical characteristics of adults with ADHD ). Validity is tested by statistical experiment or analysis ( chi-square test, Students t-test, and logistic regression analysis)
- “Our research investigated gender differences in sociodemographic and clinical characteristics of adults with ADHD in a Japanese clinical sample. Due to unique Japanese cultural ideals and expectations of women's behavior that are in opposition to ADHD symptoms, we hypothesized that women with ADHD experience more difficulties and present more dysfunctions than men . We tested the following hypotheses: first, women with ADHD have more comorbidities than men with ADHD; second, women with ADHD experience more social hardships than men, such as having less full-time employment and being more likely to be divorced.” 27
- “Statistical Analysis
- ( text omitted ) Between-gender comparisons were made using the chi-squared test for categorical variables and Students t-test for continuous variables…( text omitted ). A logistic regression analysis was performed for employment status, marital status, and comorbidity to evaluate the independent effects of gender on these dependent variables.” 27
EXAMPLES OF HYPOTHESIS AS WRITTEN IN PUBLISHED ARTICLES IN RELATION TO OTHER PARTS
- EXAMPLE 1. Background, hypotheses, and aims are provided
- “Pregnant women need skilled care during pregnancy and childbirth, but that skilled care is often delayed in some countries …( text omitted ). The focused antenatal care (FANC) model of WHO recommends that nurses provide information or counseling to all pregnant women …( text omitted ). Job aids are visual support materials that provide the right kind of information using graphics and words in a simple and yet effective manner. When nurses are not highly trained or have many work details to attend to, these job aids can serve as a content reminder for the nurses and can be used for educating their patients (Jennings, Yebadokpo, Affo, & Agbogbe, 2010) ( text omitted ). Importantly, additional evidence is needed to confirm how job aids can further improve the quality of ANC counseling by health workers in maternal care …( text omitted )” 28
- “ This has led us to hypothesize that the quality of ANC counseling would be better if supported by job aids. Consequently, a better quality of ANC counseling is expected to produce higher levels of awareness concerning the danger signs of pregnancy and a more favorable impression of the caring behavior of nurses .” 28
- “This study aimed to examine the differences in the responses of pregnant women to a job aid-supported intervention during ANC visit in terms of 1) their understanding of the danger signs of pregnancy and 2) their impression of the caring behaviors of nurses to pregnant women in rural Tanzania.” 28
- EXAMPLE 2. Background, hypotheses, and aims are provided
- “We conducted a two-arm randomized controlled trial (RCT) to evaluate and compare changes in salivary cortisol and oxytocin levels of first-time pregnant women between experimental and control groups. The women in the experimental group touched and held an infant for 30 min (experimental intervention protocol), whereas those in the control group watched a DVD movie of an infant (control intervention protocol). The primary outcome was salivary cortisol level and the secondary outcome was salivary oxytocin level.” 29
- “ We hypothesize that at 30 min after touching and holding an infant, the salivary cortisol level will significantly decrease and the salivary oxytocin level will increase in the experimental group compared with the control group .” 29
- EXAMPLE 3. Background, aim, and hypothesis are provided
- “In countries where the maternal mortality ratio remains high, antenatal education to increase Birth Preparedness and Complication Readiness (BPCR) is considered one of the top priorities [1]. BPCR includes birth plans during the antenatal period, such as the birthplace, birth attendant, transportation, health facility for complications, expenses, and birth materials, as well as family coordination to achieve such birth plans. In Tanzania, although increasing, only about half of all pregnant women attend an antenatal clinic more than four times [4]. Moreover, the information provided during antenatal care (ANC) is insufficient. In the resource-poor settings, antenatal group education is a potential approach because of the limited time for individual counseling at antenatal clinics.” 30
- “This study aimed to evaluate an antenatal group education program among pregnant women and their families with respect to birth-preparedness and maternal and infant outcomes in rural villages of Tanzania.” 30
- “ The study hypothesis was if Tanzanian pregnant women and their families received a family-oriented antenatal group education, they would (1) have a higher level of BPCR, (2) attend antenatal clinic four or more times, (3) give birth in a health facility, (4) have less complications of women at birth, and (5) have less complications and deaths of infants than those who did not receive the education .” 30
Research questions and hypotheses are crucial components to any type of research, whether quantitative or qualitative. These questions should be developed at the very beginning of the study. Excellent research questions lead to superior hypotheses, which, like a compass, set the direction of research, and can often determine the successful conduct of the study. Many research studies have floundered because the development of research questions and subsequent hypotheses was not given the thought and meticulous attention needed. The development of research questions and hypotheses is an iterative process based on extensive knowledge of the literature and insightful grasp of the knowledge gap. Focused, concise, and specific research questions provide a strong foundation for constructing hypotheses which serve as formal predictions about the research outcomes. Research questions and hypotheses are crucial elements of research that should not be overlooked. They should be carefully thought of and constructed when planning research. This avoids unethical studies and poor outcomes by defining well-founded objectives that determine the design, course, and outcome of the study.
Disclosure: The authors have no potential conflicts of interest to disclose.
Author Contributions:
- Conceptualization: Barroga E, Matanguihan GJ.
- Methodology: Barroga E, Matanguihan GJ.
- Writing - original draft: Barroga E, Matanguihan GJ.
- Writing - review & editing: Barroga E, Matanguihan GJ.
How to Write a Directional Hypothesis: A Step-by-Step Guide
In research, hypotheses play a crucial role in guiding investigations and making predictions about relationships between variables.
In this blog post, we’ll explore what a directional hypothesis is, why it’s important, and provide a step-by-step guide on how to write one effectively.
What is a Directional Hypothesis?
Examples of directional hypotheses, why to write a directional hypothesis.
Directional hypotheses offer several advantages in research. They provide researchers with a more focused prediction, allowing them to test specific hypotheses rather than exploring all possible relationships between variables.
Step 1: Identify the Variables
Step 2: predict the direction.
Based on your understanding of the relationship between the variables, predict the direction of the effect.
Step 3: Use Clear Language
Write your directional hypothesis using clear and concise language. Avoid technical jargon or terms that may be difficult for readers to understand. Your hypothesis should be easily understood by both researchers and non-experts.
Step 4: Ensure Testability
Step 5: revise and refine.
Writing a directional hypothesis is an essential skill for researchers conducting experiments and investigations.
Whether you’re a researcher or just starting out in the field, mastering the art of writing directional hypotheses will enhance the quality and rigor of your research endeavors.
Related Posts
Types of research questions, 8 types of validity in research | examples, operationalization of variables in research | examples | benefits, 10 types of variables in research: definitions and examples, what is a theoretical framework examples, types of reliability in research | example | ppt, independent vs. dependent variable, 4 research proposal examples | proposal sample, how to write a literature review in research: a step-by-step guide, how to write an abstract for a research paper.
- Resources Home 🏠
- Try SciSpace Copilot
- Search research papers
- Add Copilot Extension
- Try AI Detector
- Try Paraphraser
- Try Citation Generator
- April Papers
- June Papers
- July Papers

The Craft of Writing a Strong Hypothesis

Table of Contents
Writing a hypothesis is one of the essential elements of a scientific research paper. It needs to be to the point, clearly communicating what your research is trying to accomplish. A blurry, drawn-out, or complexly-structured hypothesis can confuse your readers. Or worse, the editor and peer reviewers.
A captivating hypothesis is not too intricate. This blog will take you through the process so that, by the end of it, you have a better idea of how to convey your research paper's intent in just one sentence.
What is a Hypothesis?
The first step in your scientific endeavor, a hypothesis, is a strong, concise statement that forms the basis of your research. It is not the same as a thesis statement , which is a brief summary of your research paper .
The sole purpose of a hypothesis is to predict your paper's findings, data, and conclusion. It comes from a place of curiosity and intuition . When you write a hypothesis, you're essentially making an educated guess based on scientific prejudices and evidence, which is further proven or disproven through the scientific method.
The reason for undertaking research is to observe a specific phenomenon. A hypothesis, therefore, lays out what the said phenomenon is. And it does so through two variables, an independent and dependent variable.
The independent variable is the cause behind the observation, while the dependent variable is the effect of the cause. A good example of this is “mixing red and blue forms purple.” In this hypothesis, mixing red and blue is the independent variable as you're combining the two colors at your own will. The formation of purple is the dependent variable as, in this case, it is conditional to the independent variable.
Different Types of Hypotheses

Types of hypotheses
Some would stand by the notion that there are only two types of hypotheses: a Null hypothesis and an Alternative hypothesis. While that may have some truth to it, it would be better to fully distinguish the most common forms as these terms come up so often, which might leave you out of context.
Apart from Null and Alternative, there are Complex, Simple, Directional, Non-Directional, Statistical, and Associative and casual hypotheses. They don't necessarily have to be exclusive, as one hypothesis can tick many boxes, but knowing the distinctions between them will make it easier for you to construct your own.
1. Null hypothesis
A null hypothesis proposes no relationship between two variables. Denoted by H 0 , it is a negative statement like “Attending physiotherapy sessions does not affect athletes' on-field performance.” Here, the author claims physiotherapy sessions have no effect on on-field performances. Even if there is, it's only a coincidence.
2. Alternative hypothesis
Considered to be the opposite of a null hypothesis, an alternative hypothesis is donated as H1 or Ha. It explicitly states that the dependent variable affects the independent variable. A good alternative hypothesis example is “Attending physiotherapy sessions improves athletes' on-field performance.” or “Water evaporates at 100 °C. ” The alternative hypothesis further branches into directional and non-directional.
- Directional hypothesis: A hypothesis that states the result would be either positive or negative is called directional hypothesis. It accompanies H1 with either the ‘<' or ‘>' sign.
- Non-directional hypothesis: A non-directional hypothesis only claims an effect on the dependent variable. It does not clarify whether the result would be positive or negative. The sign for a non-directional hypothesis is ‘≠.'
3. Simple hypothesis
A simple hypothesis is a statement made to reflect the relation between exactly two variables. One independent and one dependent. Consider the example, “Smoking is a prominent cause of lung cancer." The dependent variable, lung cancer, is dependent on the independent variable, smoking.
4. Complex hypothesis
In contrast to a simple hypothesis, a complex hypothesis implies the relationship between multiple independent and dependent variables. For instance, “Individuals who eat more fruits tend to have higher immunity, lesser cholesterol, and high metabolism.” The independent variable is eating more fruits, while the dependent variables are higher immunity, lesser cholesterol, and high metabolism.
5. Associative and casual hypothesis
Associative and casual hypotheses don't exhibit how many variables there will be. They define the relationship between the variables. In an associative hypothesis, changing any one variable, dependent or independent, affects others. In a casual hypothesis, the independent variable directly affects the dependent.
6. Empirical hypothesis
Also referred to as the working hypothesis, an empirical hypothesis claims a theory's validation via experiments and observation. This way, the statement appears justifiable and different from a wild guess.
Say, the hypothesis is “Women who take iron tablets face a lesser risk of anemia than those who take vitamin B12.” This is an example of an empirical hypothesis where the researcher the statement after assessing a group of women who take iron tablets and charting the findings.
7. Statistical hypothesis
The point of a statistical hypothesis is to test an already existing hypothesis by studying a population sample. Hypothesis like “44% of the Indian population belong in the age group of 22-27.” leverage evidence to prove or disprove a particular statement.
Characteristics of a Good Hypothesis
Writing a hypothesis is essential as it can make or break your research for you. That includes your chances of getting published in a journal. So when you're designing one, keep an eye out for these pointers:
- A research hypothesis has to be simple yet clear to look justifiable enough.
- It has to be testable — your research would be rendered pointless if too far-fetched into reality or limited by technology.
- It has to be precise about the results —what you are trying to do and achieve through it should come out in your hypothesis.
- A research hypothesis should be self-explanatory, leaving no doubt in the reader's mind.
- If you are developing a relational hypothesis, you need to include the variables and establish an appropriate relationship among them.
- A hypothesis must keep and reflect the scope for further investigations and experiments.
Separating a Hypothesis from a Prediction
Outside of academia, hypothesis and prediction are often used interchangeably. In research writing, this is not only confusing but also incorrect. And although a hypothesis and prediction are guesses at their core, there are many differences between them.
A hypothesis is an educated guess or even a testable prediction validated through research. It aims to analyze the gathered evidence and facts to define a relationship between variables and put forth a logical explanation behind the nature of events.
Predictions are assumptions or expected outcomes made without any backing evidence. They are more fictionally inclined regardless of where they originate from.
For this reason, a hypothesis holds much more weight than a prediction. It sticks to the scientific method rather than pure guesswork. "Planets revolve around the Sun." is an example of a hypothesis as it is previous knowledge and observed trends. Additionally, we can test it through the scientific method.
Whereas "COVID-19 will be eradicated by 2030." is a prediction. Even though it results from past trends, we can't prove or disprove it. So, the only way this gets validated is to wait and watch if COVID-19 cases end by 2030.
Finally, How to Write a Hypothesis
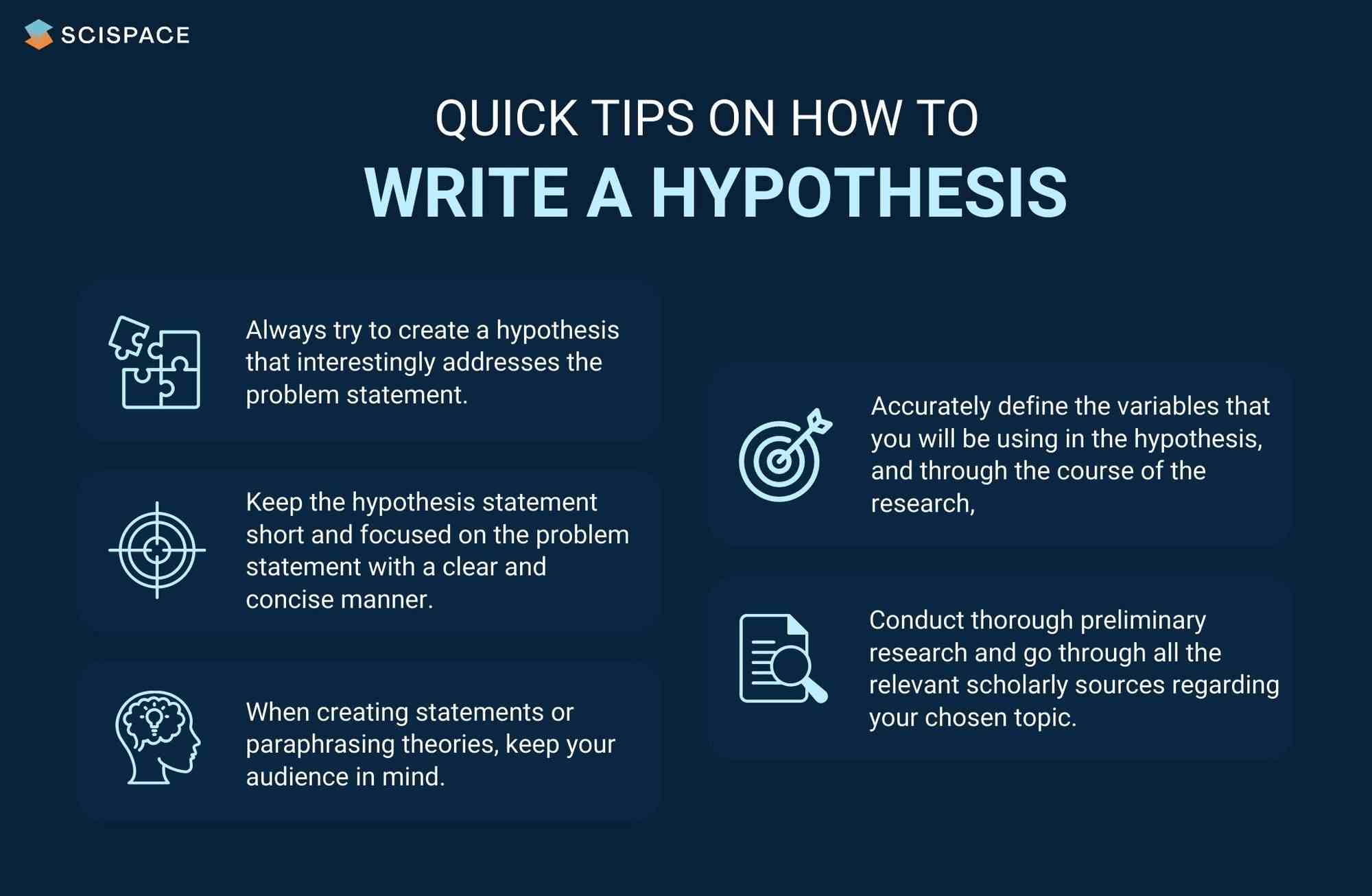
Quick tips on writing a hypothesis
1. Be clear about your research question
A hypothesis should instantly address the research question or the problem statement. To do so, you need to ask a question. Understand the constraints of your undertaken research topic and then formulate a simple and topic-centric problem. Only after that can you develop a hypothesis and further test for evidence.
2. Carry out a recce
Once you have your research's foundation laid out, it would be best to conduct preliminary research. Go through previous theories, academic papers, data, and experiments before you start curating your research hypothesis. It will give you an idea of your hypothesis's viability or originality.
Making use of references from relevant research papers helps draft a good research hypothesis. SciSpace Discover offers a repository of over 270 million research papers to browse through and gain a deeper understanding of related studies on a particular topic. Additionally, you can use SciSpace Copilot , your AI research assistant, for reading any lengthy research paper and getting a more summarized context of it. A hypothesis can be formed after evaluating many such summarized research papers. Copilot also offers explanations for theories and equations, explains paper in simplified version, allows you to highlight any text in the paper or clip math equations and tables and provides a deeper, clear understanding of what is being said. This can improve the hypothesis by helping you identify potential research gaps.
3. Create a 3-dimensional hypothesis
Variables are an essential part of any reasonable hypothesis. So, identify your independent and dependent variable(s) and form a correlation between them. The ideal way to do this is to write the hypothetical assumption in the ‘if-then' form. If you use this form, make sure that you state the predefined relationship between the variables.
In another way, you can choose to present your hypothesis as a comparison between two variables. Here, you must specify the difference you expect to observe in the results.
4. Write the first draft
Now that everything is in place, it's time to write your hypothesis. For starters, create the first draft. In this version, write what you expect to find from your research.
Clearly separate your independent and dependent variables and the link between them. Don't fixate on syntax at this stage. The goal is to ensure your hypothesis addresses the issue.
5. Proof your hypothesis
After preparing the first draft of your hypothesis, you need to inspect it thoroughly. It should tick all the boxes, like being concise, straightforward, relevant, and accurate. Your final hypothesis has to be well-structured as well.
Research projects are an exciting and crucial part of being a scholar. And once you have your research question, you need a great hypothesis to begin conducting research. Thus, knowing how to write a hypothesis is very important.
Now that you have a firmer grasp on what a good hypothesis constitutes, the different kinds there are, and what process to follow, you will find it much easier to write your hypothesis, which ultimately helps your research.
Now it's easier than ever to streamline your research workflow with SciSpace Discover . Its integrated, comprehensive end-to-end platform for research allows scholars to easily discover, write and publish their research and fosters collaboration.
It includes everything you need, including a repository of over 270 million research papers across disciplines, SEO-optimized summaries and public profiles to show your expertise and experience.
If you found these tips on writing a research hypothesis useful, head over to our blog on Statistical Hypothesis Testing to learn about the top researchers, papers, and institutions in this domain.
Frequently Asked Questions (FAQs)
1. what is the definition of hypothesis.
According to the Oxford dictionary, a hypothesis is defined as “An idea or explanation of something that is based on a few known facts, but that has not yet been proved to be true or correct”.
2. What is an example of hypothesis?
The hypothesis is a statement that proposes a relationship between two or more variables. An example: "If we increase the number of new users who join our platform by 25%, then we will see an increase in revenue."
3. What is an example of null hypothesis?
A null hypothesis is a statement that there is no relationship between two variables. The null hypothesis is written as H0. The null hypothesis states that there is no effect. For example, if you're studying whether or not a particular type of exercise increases strength, your null hypothesis will be "there is no difference in strength between people who exercise and people who don't."
4. What are the types of research?
• Fundamental research
• Applied research
• Qualitative research
• Quantitative research
• Mixed research
• Exploratory research
• Longitudinal research
• Cross-sectional research
• Field research
• Laboratory research
• Fixed research
• Flexible research
• Action research
• Policy research
• Classification research
• Comparative research
• Causal research
• Inductive research
• Deductive research
5. How to write a hypothesis?
• Your hypothesis should be able to predict the relationship and outcome.
• Avoid wordiness by keeping it simple and brief.
• Your hypothesis should contain observable and testable outcomes.
• Your hypothesis should be relevant to the research question.
6. What are the 2 types of hypothesis?
• Null hypotheses are used to test the claim that "there is no difference between two groups of data".
• Alternative hypotheses test the claim that "there is a difference between two data groups".
7. Difference between research question and research hypothesis?
A research question is a broad, open-ended question you will try to answer through your research. A hypothesis is a statement based on prior research or theory that you expect to be true due to your study. Example - Research question: What are the factors that influence the adoption of the new technology? Research hypothesis: There is a positive relationship between age, education and income level with the adoption of the new technology.
8. What is plural for hypothesis?
The plural of hypothesis is hypotheses. Here's an example of how it would be used in a statement, "Numerous well-considered hypotheses are presented in this part, and they are supported by tables and figures that are well-illustrated."
9. What is the red queen hypothesis?
The red queen hypothesis in evolutionary biology states that species must constantly evolve to avoid extinction because if they don't, they will be outcompeted by other species that are evolving. Leigh Van Valen first proposed it in 1973; since then, it has been tested and substantiated many times.
10. Who is known as the father of null hypothesis?
The father of the null hypothesis is Sir Ronald Fisher. He published a paper in 1925 that introduced the concept of null hypothesis testing, and he was also the first to use the term itself.
11. When to reject null hypothesis?
You need to find a significant difference between your two populations to reject the null hypothesis. You can determine that by running statistical tests such as an independent sample t-test or a dependent sample t-test. You should reject the null hypothesis if the p-value is less than 0.05.

You might also like

Consensus GPT vs. SciSpace GPT: Choose the Best GPT for Research
Literature Review and Theoretical Framework: Understanding the Differences

Types of Essays in Academic Writing - Quick Guide (2024)
Directional and non-directional hypothesis: A Comprehensive Guide
Karolina Konopka
Customer support manager
Want to talk with us?
In the world of research and statistical analysis, hypotheses play a crucial role in formulating and testing scientific claims. Understanding the differences between directional and non-directional hypothesis is essential for designing sound experiments and drawing accurate conclusions. Whether you’re a student, researcher, or simply curious about the foundations of hypothesis testing, this guide will equip you with the knowledge and tools to navigate this fundamental aspect of scientific inquiry.
Understanding Directional Hypothesis
Understanding directional hypotheses is crucial for conducting hypothesis-driven research, as they guide the selection of appropriate statistical tests and aid in the interpretation of results. By incorporating directional hypotheses, researchers can make more precise predictions, contribute to scientific knowledge, and advance their fields of study.
Definition of directional hypothesis
Directional hypotheses, also known as one-tailed hypotheses, are statements in research that make specific predictions about the direction of a relationship or difference between variables. Unlike non-directional hypotheses, which simply state that there is a relationship or difference without specifying its direction, directional hypotheses provide a focused and precise expectation.
A directional hypothesis predicts either a positive or negative relationship between variables or predicts that one group will perform better than another. It asserts a specific direction of effect or outcome. For example, a directional hypothesis could state that “increased exposure to sunlight will lead to an improvement in mood” or “participants who receive the experimental treatment will exhibit higher levels of cognitive performance compared to the control group.”
Directional hypotheses are formulated based on existing theory, prior research, or logical reasoning, and they guide the researcher’s expectations and analysis. They allow for more targeted predictions and enable researchers to test specific hypotheses using appropriate statistical tests.
The role of directional hypothesis in research
Directional hypotheses also play a significant role in research surveys. Let’s explore their role specifically in the context of survey research:
- Objective-driven surveys : Directional hypotheses help align survey research with specific objectives. By formulating directional hypotheses, researchers can focus on gathering data that directly addresses the predicted relationship or difference between variables of interest.
- Question design and measurement : Directional hypotheses guide the design of survey question types and the selection of appropriate measurement scales. They ensure that the questions are tailored to capture the specific aspects related to the predicted direction, enabling researchers to obtain more targeted and relevant data from survey respondents.
- Data analysis and interpretation : Directional hypotheses assist in data analysis by directing researchers towards appropriate statistical tests and methods. Researchers can analyze the survey data to specifically test the predicted relationship or difference, enhancing the accuracy and reliability of their findings. The results can then be interpreted within the context of the directional hypothesis, providing more meaningful insights.
- Practical implications and decision-making : Directional hypotheses in surveys often have practical implications. When the predicted relationship or difference is confirmed, it informs decision-making processes, program development, or interventions. The survey findings based on directional hypotheses can guide organizations, policymakers, or practitioners in making informed choices to achieve desired outcomes.
- Replication and further research : Directional hypotheses in survey research contribute to the replication and extension of studies. Researchers can replicate the survey with different populations or contexts to assess the generalizability of the predicted relationships. Furthermore, if the directional hypothesis is supported, it encourages further research to explore underlying mechanisms or boundary conditions.
By incorporating directional hypotheses in survey research, researchers can align their objectives, design effective surveys, conduct focused data analysis, and derive practical insights. They provide a framework for organizing survey research and contribute to the accumulation of knowledge in the field.
Examples of research questions for directional hypothesis
Here are some examples of research questions that lend themselves to directional hypotheses:
- Does increased daily exercise lead to a decrease in body weight among sedentary adults?
- Is there a positive relationship between study hours and academic performance among college students?
- Does exposure to violent video games result in an increase in aggressive behavior among adolescents?
- Does the implementation of a mindfulness-based intervention lead to a reduction in stress levels among working professionals?
- Is there a difference in customer satisfaction between Product A and Product B, with Product A expected to have higher satisfaction ratings?
- Does the use of social media influence self-esteem levels, with higher social media usage associated with lower self-esteem?
- Is there a negative relationship between job satisfaction and employee turnover, indicating that lower job satisfaction leads to higher turnover rates?
- Does the administration of a specific medication result in a decrease in symptoms among individuals with a particular medical condition?
- Does increased access to early childhood education lead to improved cognitive development in preschool-aged children?
- Is there a difference in purchase intention between advertisements with celebrity endorsements and advertisements without, with celebrity endorsements expected to have a higher impact?
These research questions generate specific predictions about the direction of the relationship or difference between variables and can be tested using appropriate research methods and statistical analyses.
Definition of non-directional hypothesis
Non-directional hypotheses, also known as two-tailed hypotheses, are statements in research that indicate the presence of a relationship or difference between variables without specifying the direction of the effect. Instead of making predictions about the specific direction of the relationship or difference, non-directional hypotheses simply state that there is an association or distinction between the variables of interest.
Non-directional hypotheses are often used when there is no prior theoretical basis or clear expectation about the direction of the relationship. They leave the possibility open for either a positive or negative relationship, or for both groups to differ in some way without specifying which group will perform better or worse.
Advantages and utility of non-directional hypothesis
Non-directional hypotheses in survey s offer several advantages and utilities, providing flexibility and comprehensive analysis of survey data. Here are some of the key advantages and utilities of using non-directional hypotheses in surveys:
- Exploration of Relationships : Non-directional hypotheses allow researchers to explore and examine relationships between variables without assuming a specific direction. This is particularly useful in surveys where the relationship between variables may not be well-known or there may be conflicting evidence regarding the direction of the effect.
- Flexibility in Question Design : With non-directional hypotheses, survey questions can be designed to measure the relationship between variables without being biased towards a particular outcome. This flexibility allows researchers to collect data and analyze the results more objectively.
- Open to Unexpected Findings : Non-directional hypotheses enable researchers to be open to unexpected or surprising findings in survey data. By not committing to a specific direction of the effect, researchers can identify and explore relationships that may not have been initially anticipated, leading to new insights and discoveries.
- Comprehensive Analysis : Non-directional hypotheses promote comprehensive analysis of survey data by considering the possibility of an effect in either direction. Researchers can assess the magnitude and significance of relationships without limiting their analysis to only one possible outcome.
- S tatistical Validity : Non-directional hypotheses in surveys allow for the use of two-tailed statistical tests, which provide a more conservative and robust assessment of significance. Two-tailed tests consider both positive and negative deviations from the null hypothesis, ensuring accurate and reliable statistical analysis of survey data.
- Exploratory Research : Non-directional hypotheses are particularly useful in exploratory research, where the goal is to gather initial insights and generate hypotheses. Surveys with non-directional hypotheses can help researchers explore various relationships and identify patterns that can guide further research or hypothesis development.
It is worth noting that the choice between directional and non-directional hypotheses in surveys depends on the research objectives, existing knowledge, and the specific variables being investigated. Researchers should carefully consider the advantages and limitations of each approach and select the one that aligns best with their research goals and survey design.
- Share with others
- Twitter Twitter Icon
- LinkedIn LinkedIn Icon
Related posts
Microsurveys: complete guide, recurring surveys: the ultimate guide, close-ended questions: definition, types, and examples, top 10 typeform alternatives and competitors, 15 best google forms alternatives and competitors, top 10 qualtrics alternatives and competitors, get answers today.
- No credit card required
- No time limit on Free plan
You can modify this template in every possible way.
All templates work great on every device.
The Research Hypothesis: Role and Construction
- First Online: 01 January 2012
Cite this chapter
- Phyllis G. Supino EdD 3
6107 Accesses
A hypothesis is a logical construct, interposed between a problem and its solution, which represents a proposed answer to a research question. It gives direction to the investigator’s thinking about the problem and, therefore, facilitates a solution. There are three primary modes of inference by which hypotheses are developed: deduction (reasoning from a general propositions to specific instances), induction (reasoning from specific instances to a general proposition), and abduction (formulation/acceptance on probation of a hypothesis to explain a surprising observation).
A research hypothesis should reflect an inference about variables; be stated as a grammatically complete, declarative sentence; be expressed simply and unambiguously; provide an adequate answer to the research problem; and be testable. Hypotheses can be classified as conceptual versus operational, single versus bi- or multivariable, causal or not causal, mechanistic versus nonmechanistic, and null or alternative. Hypotheses most commonly entail statements about “variables” which, in turn, can be classified according to their level of measurement (scaling characteristics) or according to their role in the hypothesis (independent, dependent, moderator, control, or intervening).
A hypothesis is rendered operational when its broadly (conceptually) stated variables are replaced by operational definitions of those variables. Hypotheses stated in this manner are called operational hypotheses, specific hypotheses, or predictions and facilitate testing.
Wrong hypotheses, rightly worked from, have produced more results than unguided observation
—Augustus De Morgan, 1872[ 1 ]—
This is a preview of subscription content, log in via an institution to check access.
Access this chapter
Subscribe and save.
- Get 10 units per month
- Download Article/Chapter or eBook
- 1 Unit = 1 Article or 1 Chapter
- Cancel anytime
- Available as PDF
- Read on any device
- Instant download
- Own it forever
- Available as EPUB and PDF
- Compact, lightweight edition
- Dispatched in 3 to 5 business days
- Free shipping worldwide - see info
- Durable hardcover edition
Tax calculation will be finalised at checkout
Purchases are for personal use only
Institutional subscriptions
Similar content being viewed by others
The Nature and Logic of Science: Testing Hypotheses
Abductive Research Methods in Psychological Science
Abductive Research Methods in Psychological Science
De Morgan A, De Morgan S. A budget of paradoxes. London: Longmans Green; 1872.
Google Scholar
Leedy Paul D. Practical research. Planning and design. 2nd ed. New York: Macmillan; 1960.
Bernard C. Introduction to the study of experimental medicine. New York: Dover; 1957.
Erren TC. The quest for questions—on the logical force of science. Med Hypotheses. 2004;62:635–40.
Article PubMed Google Scholar
Peirce CS. Collected papers of Charles Sanders Peirce, vol. 7. In: Hartshorne C, Weiss P, editors. Boston: The Belknap Press of Harvard University Press; 1966.
Aristotle. The complete works of Aristotle: the revised Oxford Translation. In: Barnes J, editor. vol. 2. Princeton/New Jersey: Princeton University Press; 1984.
Polit D, Beck CT. Conceptualizing a study to generate evidence for nursing. In: Polit D, Beck CT, editors. Nursing research: generating and assessing evidence for nursing practice. 8th ed. Philadelphia: Wolters Kluwer/Lippincott Williams and Wilkins; 2008. Chapter 4.
Jenicek M, Hitchcock DL. Evidence-based practice. Logic and critical thinking in medicine. Chicago: AMA Press; 2005.
Bacon F. The novum organon or a true guide to the interpretation of nature. A new translation by the Rev G.W. Kitchin. Oxford: The University Press; 1855.
Popper KR. Objective knowledge: an evolutionary approach (revised edition). New York: Oxford University Press; 1979.
Morgan AJ, Parker S. Translational mini-review series on vaccines: the Edward Jenner Museum and the history of vaccination. Clin Exp Immunol. 2007;147:389–94.
Article PubMed CAS Google Scholar
Pead PJ. Benjamin Jesty: new light in the dawn of vaccination. Lancet. 2003;362:2104–9.
Lee JA. The scientific endeavor: a primer on scientific principles and practice. San Francisco: Addison-Wesley Longman; 2000.
Allchin D. Lawson’s shoehorn, or should the philosophy of science be rated, ‘X’? Science and Education. 2003;12:315–29.
Article Google Scholar
Lawson AE. What is the role of induction and deduction in reasoning and scientific inquiry? J Res Sci Teach. 2005;42:716–40.
Peirce CS. Collected papers of Charles Sanders Peirce, vol. 2. In: Hartshorne C, Weiss P, editors. Boston: The Belknap Press of Harvard University Press; 1965.
Bonfantini MA, Proni G. To guess or not to guess? In: Eco U, Sebeok T, editors. The sign of three: Dupin, Holmes, Peirce. Bloomington: Indiana University Press; 1983. Chapter 5.
Peirce CS. Collected papers of Charles Sanders Peirce, vol. 5. In: Hartshorne C, Weiss P, editors. Boston: The Belknap Press of Harvard University Press; 1965.
Flach PA, Kakas AC. Abductive and inductive reasoning: background issues. In: Flach PA, Kakas AC, editors. Abduction and induction. Essays on their relation and integration. The Netherlands: Klewer; 2000. Chapter 1.
Murray JF. Voltaire, Walpole and Pasteur: variations on the theme of discovery. Am J Respir Crit Care Med. 2005;172:423–6.
Danemark B, Ekstrom M, Jakobsen L, Karlsson JC. Methodological implications, generalization, scientific inference, models (Part II) In: explaining society. Critical realism in the social sciences. New York: Routledge; 2002.
Pasteur L. Inaugural lecture as professor and dean of the faculty of sciences. In: Peterson H, editor. A treasury of the world’s greatest speeches. Douai, France: University of Lille 7 Dec 1954.
Swineburne R. Simplicity as evidence for truth. Milwaukee: Marquette University Press; 1997.
Sakar S, editor. Logical empiricism at its peak: Schlick, Carnap and Neurath. New York: Garland; 1996.
Popper K. The logic of scientific discovery. New York: Basic Books; 1959. 1934, trans. 1959.
Caws P. The philosophy of science. Princeton: D. Van Nostrand Company; 1965.
Popper K. Conjectures and refutations. The growth of scientific knowledge. 4th ed. London: Routledge and Keegan Paul; 1972.
Feyerabend PK. Against method, outline of an anarchistic theory of knowledge. London, UK: Verso; 1978.
Smith PG. Popper: conjectures and refutations (Chapter IV). In: Theory and reality: an introduction to the philosophy of science. Chicago: University of Chicago Press; 2003.
Blystone RV, Blodgett K. WWW: the scientific method. CBE Life Sci Educ. 2006;5:7–11.
Kleinbaum DG, Kupper LL, Morgenstern H. Epidemiological research. Principles and quantitative methods. New York: Van Nostrand Reinhold; 1982.
Fortune AE, Reid WJ. Research in social work. 3rd ed. New York: Columbia University Press; 1999.
Kerlinger FN. Foundations of behavioral research. 1st ed. New York: Hold, Reinhart and Winston; 1970.
Hoskins CN, Mariano C. Research in nursing and health. Understanding and using quantitative and qualitative methods. New York: Springer; 2004.
Tuckman BW. Conducting educational research. New York: Harcourt, Brace, Jovanovich; 1972.
Wang C, Chiari PC, Weihrauch D, Krolikowski JG, Warltier DC, Kersten JR, Pratt Jr PF, Pagel PS. Gender-specificity of delayed preconditioning by isoflurane in rabbits: potential role of endothelial nitric oxide synthase. Anesth Analg. 2006;103:274–80.
Beyer ME, Slesak G, Nerz S, Kazmaier S, Hoffmeister HM. Effects of endothelin-1 and IRL 1620 on myocardial contractility and myocardial energy metabolism. J Cardiovasc Pharmacol. 1995;26(Suppl 3):S150–2.
PubMed CAS Google Scholar
Stone J, Sharpe M. Amnesia for childhood in patients with unexplained neurological symptoms. J Neurol Neurosurg Psychiatry. 2002;72:416–7.
Naughton BJ, Moran M, Ghaly Y, Michalakes C. Computer tomography scanning and delirium in elder patients. Acad Emerg Med. 1997;4:1107–10.
Easterbrook PJ, Berlin JA, Gopalan R, Matthews DR. Publication bias in clinical research. Lancet. 1991;337:867–72.
Stern JM, Simes RJ. Publication bias: evidence of delayed publication in a cohort study of clinical research projects. BMJ. 1997;315:640–5.
Stevens SS. On the theory of scales and measurement. Science. 1946;103:677–80.
Knapp TR. Treating ordinal scales as interval scales: an attempt to resolve the controversy. Nurs Res. 1990;39:121–3.
The Cochrane Collaboration. Open Learning Material. www.cochrane-net.org/openlearning/html/mod14-3.htm . Accessed 12 Oct 2009.
MacCorquodale K, Meehl PE. On a distinction between hypothetical constructs and intervening variables. Psychol Rev. 1948;55:95–107.
Baron RM, Kenny DA. The moderator-mediator variable distinction in social psychological research: conceptual, strategic and statistical considerations. J Pers Soc Psychol. 1986;51:1173–82.
Williamson GM, Schultz R. Activity restriction mediates the association between pain and depressed affect: a study of younger and older adult cancer patients. Psychol Aging. 1995;10:369–78.
Song M, Lee EO. Development of a functional capacity model for the elderly. Res Nurs Health. 1998;21:189–98.
MacKinnon DP. Introduction to statistical mediation analysis. New York: Routledge; 2008.
Download references
Author information
Authors and affiliations.
Department of Medicine, College of Medicine, SUNY Downstate Medical Center, 450 Clarkson Avenue, 1199, Brooklyn, NY, 11203, USA
Phyllis G. Supino EdD
You can also search for this author in PubMed Google Scholar
Corresponding author
Correspondence to Phyllis G. Supino EdD .
Editor information
Editors and affiliations.
, Cardiovascular Medicine, SUNY Downstate Medical Center, Clarkson Avenue, box 1199 450, Brooklyn, 11203, USA
Phyllis G. Supino
, Cardiovascualr Medicine, SUNY Downstate Medical Center, Clarkson Avenue 450, Brooklyn, 11203, USA
Jeffrey S. Borer
Rights and permissions
Reprints and permissions
Copyright information
© 2012 Springer Science+Business Media, LLC
About this chapter
Supino, P.G. (2012). The Research Hypothesis: Role and Construction. In: Supino, P., Borer, J. (eds) Principles of Research Methodology. Springer, New York, NY. https://doi.org/10.1007/978-1-4614-3360-6_3
Download citation
DOI : https://doi.org/10.1007/978-1-4614-3360-6_3
Published : 18 April 2012
Publisher Name : Springer, New York, NY
Print ISBN : 978-1-4614-3359-0
Online ISBN : 978-1-4614-3360-6
eBook Packages : Medicine Medicine (R0)
Share this chapter
Anyone you share the following link with will be able to read this content:
Sorry, a shareable link is not currently available for this article.
Provided by the Springer Nature SharedIt content-sharing initiative
- Publish with us
Policies and ethics
- Find a journal
- Track your research
- Privacy Policy

Home » What is a Hypothesis – Types, Examples and Writing Guide
What is a Hypothesis – Types, Examples and Writing Guide
Table of Contents

Definition:
Hypothesis is an educated guess or proposed explanation for a phenomenon, based on some initial observations or data. It is a tentative statement that can be tested and potentially proven or disproven through further investigation and experimentation.
Hypothesis is often used in scientific research to guide the design of experiments and the collection and analysis of data. It is an essential element of the scientific method, as it allows researchers to make predictions about the outcome of their experiments and to test those predictions to determine their accuracy.
Types of Hypothesis
Types of Hypothesis are as follows:
Research Hypothesis
A research hypothesis is a statement that predicts a relationship between variables. It is usually formulated as a specific statement that can be tested through research, and it is often used in scientific research to guide the design of experiments.
Null Hypothesis
The null hypothesis is a statement that assumes there is no significant difference or relationship between variables. It is often used as a starting point for testing the research hypothesis, and if the results of the study reject the null hypothesis, it suggests that there is a significant difference or relationship between variables.
Alternative Hypothesis
An alternative hypothesis is a statement that assumes there is a significant difference or relationship between variables. It is often used as an alternative to the null hypothesis and is tested against the null hypothesis to determine which statement is more accurate.
Directional Hypothesis
A directional hypothesis is a statement that predicts the direction of the relationship between variables. For example, a researcher might predict that increasing the amount of exercise will result in a decrease in body weight.
Non-directional Hypothesis
A non-directional hypothesis is a statement that predicts the relationship between variables but does not specify the direction. For example, a researcher might predict that there is a relationship between the amount of exercise and body weight, but they do not specify whether increasing or decreasing exercise will affect body weight.
Statistical Hypothesis
A statistical hypothesis is a statement that assumes a particular statistical model or distribution for the data. It is often used in statistical analysis to test the significance of a particular result.
Composite Hypothesis
A composite hypothesis is a statement that assumes more than one condition or outcome. It can be divided into several sub-hypotheses, each of which represents a different possible outcome.
Empirical Hypothesis
An empirical hypothesis is a statement that is based on observed phenomena or data. It is often used in scientific research to develop theories or models that explain the observed phenomena.
Simple Hypothesis
A simple hypothesis is a statement that assumes only one outcome or condition. It is often used in scientific research to test a single variable or factor.
Complex Hypothesis
A complex hypothesis is a statement that assumes multiple outcomes or conditions. It is often used in scientific research to test the effects of multiple variables or factors on a particular outcome.
Applications of Hypothesis
Hypotheses are used in various fields to guide research and make predictions about the outcomes of experiments or observations. Here are some examples of how hypotheses are applied in different fields:
- Science : In scientific research, hypotheses are used to test the validity of theories and models that explain natural phenomena. For example, a hypothesis might be formulated to test the effects of a particular variable on a natural system, such as the effects of climate change on an ecosystem.
- Medicine : In medical research, hypotheses are used to test the effectiveness of treatments and therapies for specific conditions. For example, a hypothesis might be formulated to test the effects of a new drug on a particular disease.
- Psychology : In psychology, hypotheses are used to test theories and models of human behavior and cognition. For example, a hypothesis might be formulated to test the effects of a particular stimulus on the brain or behavior.
- Sociology : In sociology, hypotheses are used to test theories and models of social phenomena, such as the effects of social structures or institutions on human behavior. For example, a hypothesis might be formulated to test the effects of income inequality on crime rates.
- Business : In business research, hypotheses are used to test the validity of theories and models that explain business phenomena, such as consumer behavior or market trends. For example, a hypothesis might be formulated to test the effects of a new marketing campaign on consumer buying behavior.
- Engineering : In engineering, hypotheses are used to test the effectiveness of new technologies or designs. For example, a hypothesis might be formulated to test the efficiency of a new solar panel design.
How to write a Hypothesis
Here are the steps to follow when writing a hypothesis:
Identify the Research Question
The first step is to identify the research question that you want to answer through your study. This question should be clear, specific, and focused. It should be something that can be investigated empirically and that has some relevance or significance in the field.
Conduct a Literature Review
Before writing your hypothesis, it’s essential to conduct a thorough literature review to understand what is already known about the topic. This will help you to identify the research gap and formulate a hypothesis that builds on existing knowledge.
Determine the Variables
The next step is to identify the variables involved in the research question. A variable is any characteristic or factor that can vary or change. There are two types of variables: independent and dependent. The independent variable is the one that is manipulated or changed by the researcher, while the dependent variable is the one that is measured or observed as a result of the independent variable.
Formulate the Hypothesis
Based on the research question and the variables involved, you can now formulate your hypothesis. A hypothesis should be a clear and concise statement that predicts the relationship between the variables. It should be testable through empirical research and based on existing theory or evidence.
Write the Null Hypothesis
The null hypothesis is the opposite of the alternative hypothesis, which is the hypothesis that you are testing. The null hypothesis states that there is no significant difference or relationship between the variables. It is important to write the null hypothesis because it allows you to compare your results with what would be expected by chance.
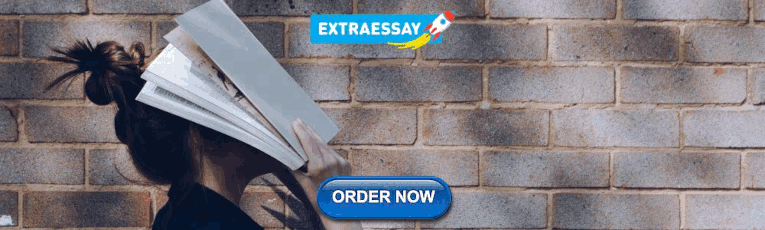
Refine the Hypothesis
After formulating the hypothesis, it’s important to refine it and make it more precise. This may involve clarifying the variables, specifying the direction of the relationship, or making the hypothesis more testable.
Examples of Hypothesis
Here are a few examples of hypotheses in different fields:
- Psychology : “Increased exposure to violent video games leads to increased aggressive behavior in adolescents.”
- Biology : “Higher levels of carbon dioxide in the atmosphere will lead to increased plant growth.”
- Sociology : “Individuals who grow up in households with higher socioeconomic status will have higher levels of education and income as adults.”
- Education : “Implementing a new teaching method will result in higher student achievement scores.”
- Marketing : “Customers who receive a personalized email will be more likely to make a purchase than those who receive a generic email.”
- Physics : “An increase in temperature will cause an increase in the volume of a gas, assuming all other variables remain constant.”
- Medicine : “Consuming a diet high in saturated fats will increase the risk of developing heart disease.”
Purpose of Hypothesis
The purpose of a hypothesis is to provide a testable explanation for an observed phenomenon or a prediction of a future outcome based on existing knowledge or theories. A hypothesis is an essential part of the scientific method and helps to guide the research process by providing a clear focus for investigation. It enables scientists to design experiments or studies to gather evidence and data that can support or refute the proposed explanation or prediction.
The formulation of a hypothesis is based on existing knowledge, observations, and theories, and it should be specific, testable, and falsifiable. A specific hypothesis helps to define the research question, which is important in the research process as it guides the selection of an appropriate research design and methodology. Testability of the hypothesis means that it can be proven or disproven through empirical data collection and analysis. Falsifiability means that the hypothesis should be formulated in such a way that it can be proven wrong if it is incorrect.
In addition to guiding the research process, the testing of hypotheses can lead to new discoveries and advancements in scientific knowledge. When a hypothesis is supported by the data, it can be used to develop new theories or models to explain the observed phenomenon. When a hypothesis is not supported by the data, it can help to refine existing theories or prompt the development of new hypotheses to explain the phenomenon.
When to use Hypothesis
Here are some common situations in which hypotheses are used:
- In scientific research , hypotheses are used to guide the design of experiments and to help researchers make predictions about the outcomes of those experiments.
- In social science research , hypotheses are used to test theories about human behavior, social relationships, and other phenomena.
- I n business , hypotheses can be used to guide decisions about marketing, product development, and other areas. For example, a hypothesis might be that a new product will sell well in a particular market, and this hypothesis can be tested through market research.
Characteristics of Hypothesis
Here are some common characteristics of a hypothesis:
- Testable : A hypothesis must be able to be tested through observation or experimentation. This means that it must be possible to collect data that will either support or refute the hypothesis.
- Falsifiable : A hypothesis must be able to be proven false if it is not supported by the data. If a hypothesis cannot be falsified, then it is not a scientific hypothesis.
- Clear and concise : A hypothesis should be stated in a clear and concise manner so that it can be easily understood and tested.
- Based on existing knowledge : A hypothesis should be based on existing knowledge and research in the field. It should not be based on personal beliefs or opinions.
- Specific : A hypothesis should be specific in terms of the variables being tested and the predicted outcome. This will help to ensure that the research is focused and well-designed.
- Tentative: A hypothesis is a tentative statement or assumption that requires further testing and evidence to be confirmed or refuted. It is not a final conclusion or assertion.
- Relevant : A hypothesis should be relevant to the research question or problem being studied. It should address a gap in knowledge or provide a new perspective on the issue.
Advantages of Hypothesis
Hypotheses have several advantages in scientific research and experimentation:
- Guides research: A hypothesis provides a clear and specific direction for research. It helps to focus the research question, select appropriate methods and variables, and interpret the results.
- Predictive powe r: A hypothesis makes predictions about the outcome of research, which can be tested through experimentation. This allows researchers to evaluate the validity of the hypothesis and make new discoveries.
- Facilitates communication: A hypothesis provides a common language and framework for scientists to communicate with one another about their research. This helps to facilitate the exchange of ideas and promotes collaboration.
- Efficient use of resources: A hypothesis helps researchers to use their time, resources, and funding efficiently by directing them towards specific research questions and methods that are most likely to yield results.
- Provides a basis for further research: A hypothesis that is supported by data provides a basis for further research and exploration. It can lead to new hypotheses, theories, and discoveries.
- Increases objectivity: A hypothesis can help to increase objectivity in research by providing a clear and specific framework for testing and interpreting results. This can reduce bias and increase the reliability of research findings.
Limitations of Hypothesis
Some Limitations of the Hypothesis are as follows:
- Limited to observable phenomena: Hypotheses are limited to observable phenomena and cannot account for unobservable or intangible factors. This means that some research questions may not be amenable to hypothesis testing.
- May be inaccurate or incomplete: Hypotheses are based on existing knowledge and research, which may be incomplete or inaccurate. This can lead to flawed hypotheses and erroneous conclusions.
- May be biased: Hypotheses may be biased by the researcher’s own beliefs, values, or assumptions. This can lead to selective interpretation of data and a lack of objectivity in research.
- Cannot prove causation: A hypothesis can only show a correlation between variables, but it cannot prove causation. This requires further experimentation and analysis.
- Limited to specific contexts: Hypotheses are limited to specific contexts and may not be generalizable to other situations or populations. This means that results may not be applicable in other contexts or may require further testing.
- May be affected by chance : Hypotheses may be affected by chance or random variation, which can obscure or distort the true relationship between variables.
About the author
Muhammad Hassan
Researcher, Academic Writer, Web developer
You may also like

Appendices – Writing Guide, Types and Examples

Research Techniques – Methods, Types and Examples

Dissertation – Format, Example and Template

Data Collection – Methods Types and Examples

Research Report – Example, Writing Guide and...

Data Analysis – Process, Methods and Types
psychologyrocks
Hypotheses; directional and non-directional, what is the difference between an experimental and an alternative hypothesis.
Nothing much! If the study is a true experiment then we can call the hypothesis “an experimental hypothesis”, a prediction is made about how the IV causes an effect on the DV. In a study which does not involve the direct manipulation of an IV, i.e. a natural or quasi-experiment or any other quantitative research method (e.g. survey) has been used, then we call it an “alternative hypothesis”, it is the alternative to the null.
Directional hypothesis: A directional (or one-tailed hypothesis) states which way you think the results are going to go, for example in an experimental study we might say…”Participants who have been deprived of sleep for 24 hours will have more cold symptoms the week after exposure to a virus than participants who have not been sleep deprived”; the hypothesis compares the two groups/conditions and states which one will ….have more/less, be quicker/slower, etc.
If we had a correlational study, the directional hypothesis would state whether we expect a positive or a negative correlation, we are stating how the two variables will be related to each other, e.g. there will be a positive correlation between the number of stressful life events experienced in the last year and the number of coughs and colds suffered, whereby the more life events you have suffered the more coughs and cold you will have had”. The directional hypothesis can also state a negative correlation, e.g. the higher the number of face-book friends, the lower the life satisfaction score “
Non-directional hypothesis: A non-directional (or two tailed hypothesis) simply states that there will be a difference between the two groups/conditions but does not say which will be greater/smaller, quicker/slower etc. Using our example above we would say “There will be a difference between the number of cold symptoms experienced in the following week after exposure to a virus for those participants who have been sleep deprived for 24 hours compared with those who have not been sleep deprived for 24 hours.”
When the study is correlational, we simply state that variables will be correlated but do not state whether the relationship will be positive or negative, e.g. there will be a significant correlation between variable A and variable B.
Null hypothesis The null hypothesis states that the alternative or experimental hypothesis is NOT the case, if your experimental hypothesis was directional you would say…
Participants who have been deprived of sleep for 24 hours will NOT have more cold symptoms in the following week after exposure to a virus than participants who have not been sleep deprived and any difference that does arise will be due to chance alone.
or with a directional correlational hypothesis….
There will NOT be a positive correlation between the number of stress life events experienced in the last year and the number of coughs and colds suffered, whereby the more life events you have suffered the more coughs and cold you will have had”
With a non-directional or two tailed hypothesis…
There will be NO difference between the number of cold symptoms experienced in the following week after exposure to a virus for those participants who have been sleep deprived for 24 hours compared with those who have not been sleep deprived for 24 hours.
or for a correlational …
there will be NO correlation between variable A and variable B.
When it comes to conducting an inferential stats test, if you have a directional hypothesis , you must do a one tailed test to find out whether your observed value is significant. If you have a non-directional hypothesis , you must do a two tailed test .
Exam Techniques/Advice
- Remember, a decent hypothesis will contain two variables, in the case of an experimental hypothesis there will be an IV and a DV; in a correlational hypothesis there will be two co-variables
- both variables need to be fully operationalised to score the marks, that is you need to be very clear and specific about what you mean by your IV and your DV; if someone wanted to repeat your study, they should be able to look at your hypothesis and know exactly what to change between the two groups/conditions and exactly what to measure (including any units/explanation of rating scales etc, e.g. “where 1 is low and 7 is high”)
- double check the question, did it ask for a directional or non-directional hypothesis?
- if you were asked for a null hypothesis, make sure you always include the phrase “and any difference/correlation (is your study experimental or correlational?) that does arise will be due to chance alone”
Practice Questions:
- Mr Faraz wants to compare the levels of attendance between his psychology group and those of Mr Simon, who teaches a different psychology group. Which of the following is a suitable directional (one tailed) hypothesis for Mr Faraz’s investigation?
A There will be a difference in the levels of attendance between the two psychology groups.
B Students’ level of attendance will be higher in Mr Faraz’s group than Mr Simon’s group.
C Any difference in the levels of attendance between the two psychology groups is due to chance.
D The level of attendance of the students will depend upon who is teaching the groups.
2. Tracy works for the local council. The council is thinking about reducing the number of people it employs to pick up litter from the street. Tracy has been asked to carry out a study to see if having the streets cleaned at less regular intervals will affect the amount of litter the public will drop. She studies a street to compare how much litter is dropped at two different times, once when it has just been cleaned and once after it has not been cleaned for a month.
Write a fully operationalised non-directional (two-tailed) hypothesis for Tracy’s study. (2)
3. Jamila is conducting a practical investigation to look at gender differences in carrying out visuo-spatial tasks. She decides to give males and females a jigsaw puzzle and will time them to see who completes it the fastest. She uses a random sample of pupils from a local school to get her participants.
(a) Write a fully operationalised directional (one tailed) hypothesis for Jamila’s study. (2) (b) Outline one strength and one weakness of the random sampling method. You may refer to Jamila’s use of this type of sampling in your answer. (4)
4. Which of the following is a non-directional (two tailed) hypothesis?
A There is a difference in driving ability with men being better drivers than women
B Women are better at concentrating on more than one thing at a time than men
C Women spend more time doing the cooking and cleaning than men
D There is a difference in the number of men and women who participate in sports
Revision Activities
writing-hypotheses-revision-sheet
Quizizz link for teachers: https://quizizz.com/admin/quiz/5bf03f51add785001bc5a09e
By Psychstix by Mandy wood
Share this:
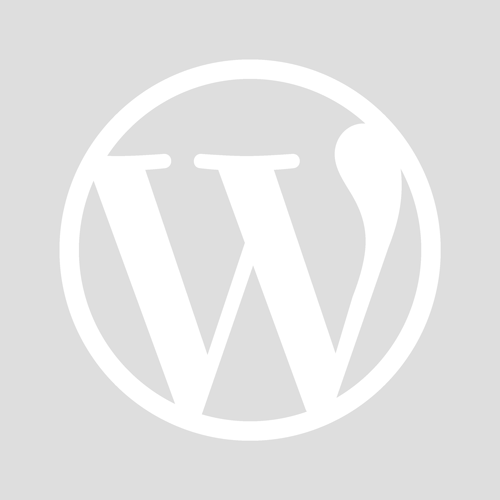
- Already have a WordPress.com account? Log in now.
- Subscribe Subscribed
- Copy shortlink
- Report this content
- View post in Reader
- Manage subscriptions
- Collapse this bar
Aims And Hypotheses, Directional And Non-Directional
March 7, 2021 - paper 2 psychology in context | research methods.
In Psychology, hypotheses are predictions made by the researcher about the outcome of a study. The research can chose to make a specific prediction about what they feel will happen in their research (a directional hypothesis) or they can make a ‘general,’ ‘less specific’ prediction about the outcome of their research (a non-directional hypothesis). The type of prediction that a researcher makes is usually dependent on whether or not any previous research has also investigated their research aim.
Variables Recap:
The independent variable (IV) is the variable that psychologists manipulate/change to see if changing this variable has an effect on the depen dent variable (DV).
The dependent variable (DV) is the variable that the psychologists measures (to see if the IV has had an effect).
Research/Experimental Aim(S):

An aim is a clear and precise statement of the purpose of the study. It is a statement of why a research study is taking place. This should include what is being studied and what the study is trying to achieve. (e.g. “This study aims to investigate the effects of alcohol on reaction times”.
Hypotheses:
This is a testable statement that predicts what the researcher expects to happen in their research. The research study itself is therefore a means of testing whether or not the hypothesis is supported by the findings. If the findings do support the hypothesis then the hypothesis can be retained (i.e., accepted), but if not, then it must be rejected.

(1) Directional Hypothesis: states that the IV will have an effect on the DV and what that effect will be (the direction of results). For example, eating smarties will significantly improve an individual’s dancing ability. When writing a directional hypothesis, it is important that you state exactly how the IV will influence the DV.
(3) A Null Hypothesis: states that the IV will have no significant effect on the DV, for example, ‘eating smarties will have no effect in an individuals dancing ability.’
Research Methods In Psychology
Saul McLeod, PhD
Editor-in-Chief for Simply Psychology
BSc (Hons) Psychology, MRes, PhD, University of Manchester
Saul McLeod, PhD., is a qualified psychology teacher with over 18 years of experience in further and higher education. He has been published in peer-reviewed journals, including the Journal of Clinical Psychology.
Learn about our Editorial Process
Olivia Guy-Evans, MSc
Associate Editor for Simply Psychology
BSc (Hons) Psychology, MSc Psychology of Education
Olivia Guy-Evans is a writer and associate editor for Simply Psychology. She has previously worked in healthcare and educational sectors.
Research methods in psychology are systematic procedures used to observe, describe, predict, and explain behavior and mental processes. They include experiments, surveys, case studies, and naturalistic observations, ensuring data collection is objective and reliable to understand and explain psychological phenomena.
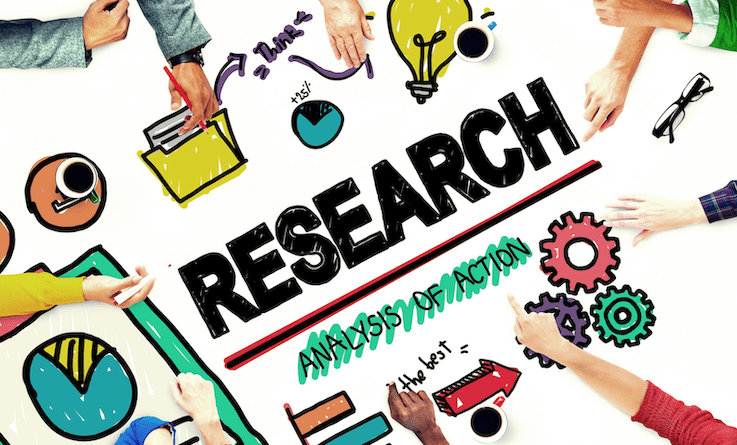
Hypotheses are statements about the prediction of the results, that can be verified or disproved by some investigation.
There are four types of hypotheses :
- Null Hypotheses (H0 ) – these predict that no difference will be found in the results between the conditions. Typically these are written ‘There will be no difference…’
- Alternative Hypotheses (Ha or H1) – these predict that there will be a significant difference in the results between the two conditions. This is also known as the experimental hypothesis.
- One-tailed (directional) hypotheses – these state the specific direction the researcher expects the results to move in, e.g. higher, lower, more, less. In a correlation study, the predicted direction of the correlation can be either positive or negative.
- Two-tailed (non-directional) hypotheses – these state that a difference will be found between the conditions of the independent variable but does not state the direction of a difference or relationship. Typically these are always written ‘There will be a difference ….’
All research has an alternative hypothesis (either a one-tailed or two-tailed) and a corresponding null hypothesis.
Once the research is conducted and results are found, psychologists must accept one hypothesis and reject the other.
So, if a difference is found, the Psychologist would accept the alternative hypothesis and reject the null. The opposite applies if no difference is found.
Sampling techniques
Sampling is the process of selecting a representative group from the population under study.
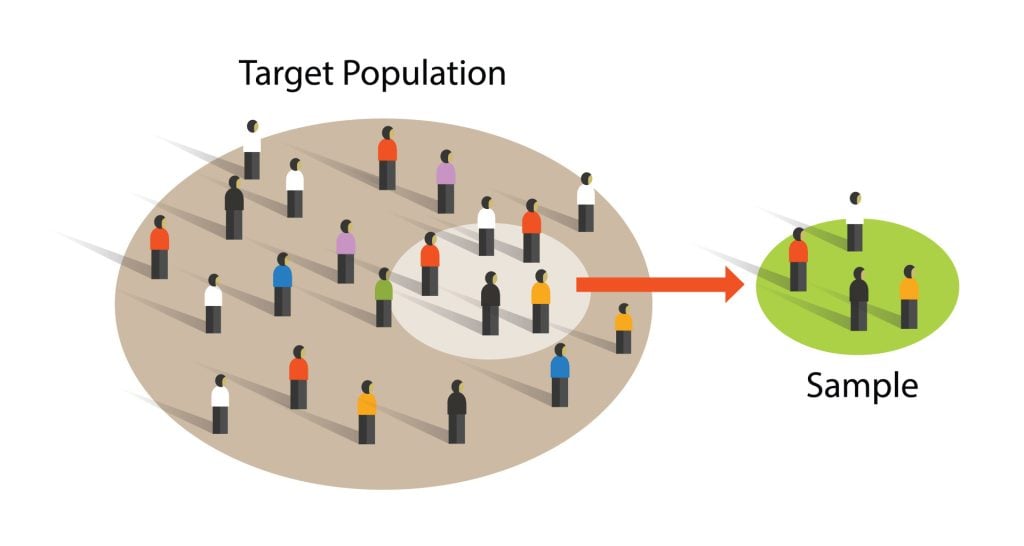
A sample is the participants you select from a target population (the group you are interested in) to make generalizations about.
Representative means the extent to which a sample mirrors a researcher’s target population and reflects its characteristics.
Generalisability means the extent to which their findings can be applied to the larger population of which their sample was a part.
- Volunteer sample : where participants pick themselves through newspaper adverts, noticeboards or online.
- Opportunity sampling : also known as convenience sampling , uses people who are available at the time the study is carried out and willing to take part. It is based on convenience.
- Random sampling : when every person in the target population has an equal chance of being selected. An example of random sampling would be picking names out of a hat.
- Systematic sampling : when a system is used to select participants. Picking every Nth person from all possible participants. N = the number of people in the research population / the number of people needed for the sample.
- Stratified sampling : when you identify the subgroups and select participants in proportion to their occurrences.
- Snowball sampling : when researchers find a few participants, and then ask them to find participants themselves and so on.
- Quota sampling : when researchers will be told to ensure the sample fits certain quotas, for example they might be told to find 90 participants, with 30 of them being unemployed.
Experiments always have an independent and dependent variable .
- The independent variable is the one the experimenter manipulates (the thing that changes between the conditions the participants are placed into). It is assumed to have a direct effect on the dependent variable.
- The dependent variable is the thing being measured, or the results of the experiment.
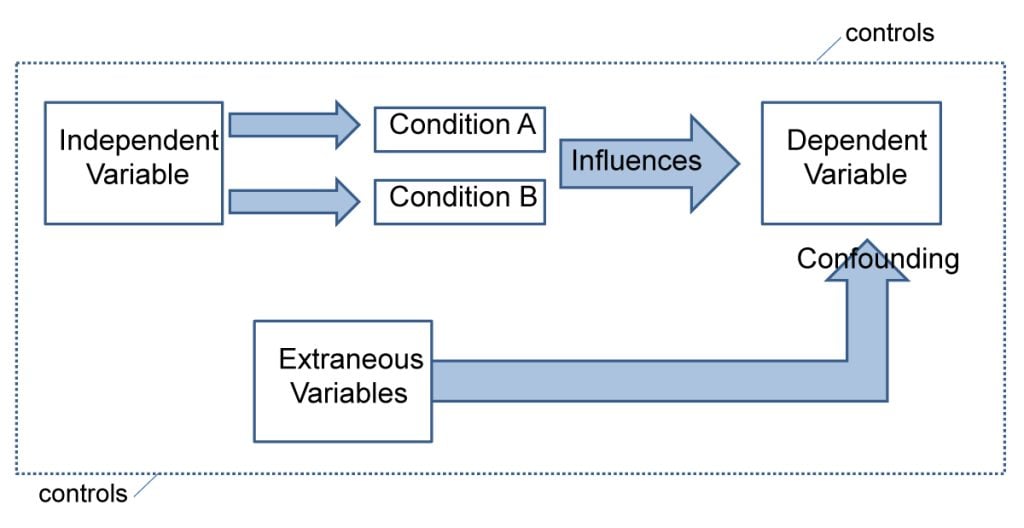
Operationalization of variables means making them measurable/quantifiable. We must use operationalization to ensure that variables are in a form that can be easily tested.
For instance, we can’t really measure ‘happiness’, but we can measure how many times a person smiles within a two-hour period.
By operationalizing variables, we make it easy for someone else to replicate our research. Remember, this is important because we can check if our findings are reliable.
Extraneous variables are all variables which are not independent variable but could affect the results of the experiment.
It can be a natural characteristic of the participant, such as intelligence levels, gender, or age for example, or it could be a situational feature of the environment such as lighting or noise.
Demand characteristics are a type of extraneous variable that occurs if the participants work out the aims of the research study, they may begin to behave in a certain way.
For example, in Milgram’s research , critics argued that participants worked out that the shocks were not real and they administered them as they thought this was what was required of them.
Extraneous variables must be controlled so that they do not affect (confound) the results.
Randomly allocating participants to their conditions or using a matched pairs experimental design can help to reduce participant variables.
Situational variables are controlled by using standardized procedures, ensuring every participant in a given condition is treated in the same way
Experimental Design
Experimental design refers to how participants are allocated to each condition of the independent variable, such as a control or experimental group.
- Independent design ( between-groups design ): each participant is selected for only one group. With the independent design, the most common way of deciding which participants go into which group is by means of randomization.
- Matched participants design : each participant is selected for only one group, but the participants in the two groups are matched for some relevant factor or factors (e.g. ability; sex; age).
- Repeated measures design ( within groups) : each participant appears in both groups, so that there are exactly the same participants in each group.
- The main problem with the repeated measures design is that there may well be order effects. Their experiences during the experiment may change the participants in various ways.
- They may perform better when they appear in the second group because they have gained useful information about the experiment or about the task. On the other hand, they may perform less well on the second occasion because of tiredness or boredom.
- Counterbalancing is the best way of preventing order effects from disrupting the findings of an experiment, and involves ensuring that each condition is equally likely to be used first and second by the participants.
If we wish to compare two groups with respect to a given independent variable, it is essential to make sure that the two groups do not differ in any other important way.
Experimental Methods
All experimental methods involve an iv (independent variable) and dv (dependent variable)..
The researcher decides where the experiment will take place, at what time, with which participants, in what circumstances, using a standardized procedure.
- Field experiments are conducted in the everyday (natural) environment of the participants. The experimenter still manipulates the IV, but in a real-life setting. It may be possible to control extraneous variables, though such control is more difficult than in a lab experiment.
- Natural experiments are when a naturally occurring IV is investigated that isn’t deliberately manipulated, it exists anyway. Participants are not randomly allocated, and the natural event may only occur rarely.
Case studies are in-depth investigations of a person, group, event, or community. It uses information from a range of sources, such as from the person concerned and also from their family and friends.
Many techniques may be used such as interviews, psychological tests, observations and experiments. Case studies are generally longitudinal: in other words, they follow the individual or group over an extended period of time.
Case studies are widely used in psychology and among the best-known ones carried out were by Sigmund Freud . He conducted very detailed investigations into the private lives of his patients in an attempt to both understand and help them overcome their illnesses.
Case studies provide rich qualitative data and have high levels of ecological validity. However, it is difficult to generalize from individual cases as each one has unique characteristics.
Correlational Studies
Correlation means association; it is a measure of the extent to which two variables are related. One of the variables can be regarded as the predictor variable with the other one as the outcome variable.
Correlational studies typically involve obtaining two different measures from a group of participants, and then assessing the degree of association between the measures.
The predictor variable can be seen as occurring before the outcome variable in some sense. It is called the predictor variable, because it forms the basis for predicting the value of the outcome variable.
Relationships between variables can be displayed on a graph or as a numerical score called a correlation coefficient.
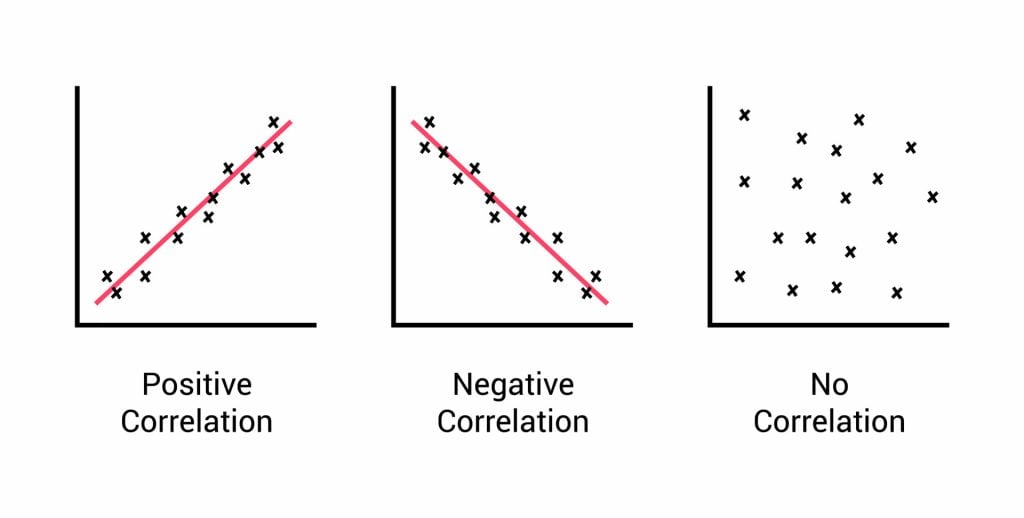
- If an increase in one variable tends to be associated with an increase in the other, then this is known as a positive correlation .
- If an increase in one variable tends to be associated with a decrease in the other, then this is known as a negative correlation .
- A zero correlation occurs when there is no relationship between variables.
After looking at the scattergraph, if we want to be sure that a significant relationship does exist between the two variables, a statistical test of correlation can be conducted, such as Spearman’s rho.
The test will give us a score, called a correlation coefficient . This is a value between 0 and 1, and the closer to 1 the score is, the stronger the relationship between the variables. This value can be both positive e.g. 0.63, or negative -0.63.
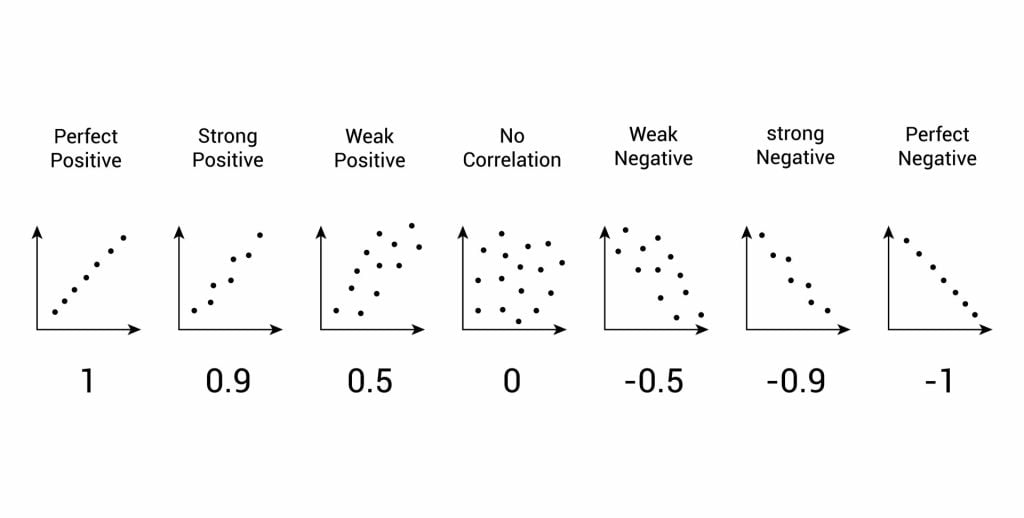
A correlation between variables, however, does not automatically mean that the change in one variable is the cause of the change in the values of the other variable. A correlation only shows if there is a relationship between variables.
Correlation does not always prove causation, as a third variable may be involved.
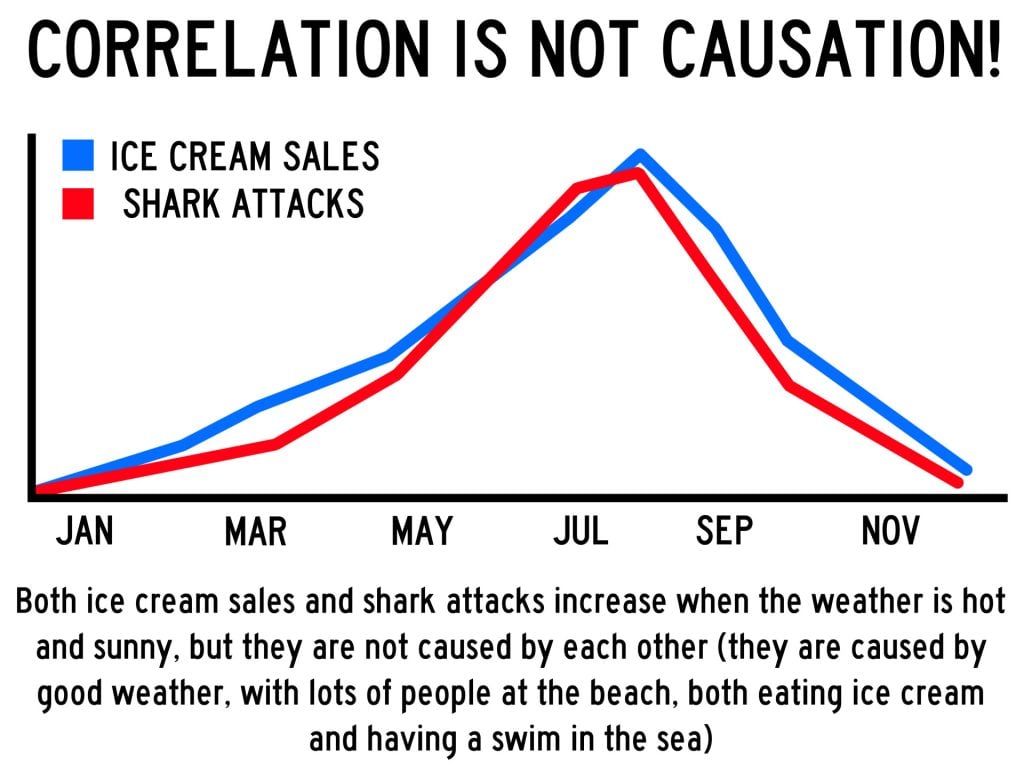
Interview Methods
Interviews are commonly divided into two types: structured and unstructured.
A fixed, predetermined set of questions is put to every participant in the same order and in the same way.
Responses are recorded on a questionnaire, and the researcher presets the order and wording of questions, and sometimes the range of alternative answers.
The interviewer stays within their role and maintains social distance from the interviewee.
There are no set questions, and the participant can raise whatever topics he/she feels are relevant and ask them in their own way. Questions are posed about participants’ answers to the subject
Unstructured interviews are most useful in qualitative research to analyze attitudes and values.
Though they rarely provide a valid basis for generalization, their main advantage is that they enable the researcher to probe social actors’ subjective point of view.
Questionnaire Method
Questionnaires can be thought of as a kind of written interview. They can be carried out face to face, by telephone, or post.
The choice of questions is important because of the need to avoid bias or ambiguity in the questions, ‘leading’ the respondent or causing offense.
- Open questions are designed to encourage a full, meaningful answer using the subject’s own knowledge and feelings. They provide insights into feelings, opinions, and understanding. Example: “How do you feel about that situation?”
- Closed questions can be answered with a simple “yes” or “no” or specific information, limiting the depth of response. They are useful for gathering specific facts or confirming details. Example: “Do you feel anxious in crowds?”
Its other practical advantages are that it is cheaper than face-to-face interviews and can be used to contact many respondents scattered over a wide area relatively quickly.
Observations
There are different types of observation methods :
- Covert observation is where the researcher doesn’t tell the participants they are being observed until after the study is complete. There could be ethical problems or deception and consent with this particular observation method.
- Overt observation is where a researcher tells the participants they are being observed and what they are being observed for.
- Controlled : behavior is observed under controlled laboratory conditions (e.g., Bandura’s Bobo doll study).
- Natural : Here, spontaneous behavior is recorded in a natural setting.
- Participant : Here, the observer has direct contact with the group of people they are observing. The researcher becomes a member of the group they are researching.
- Non-participant (aka “fly on the wall): The researcher does not have direct contact with the people being observed. The observation of participants’ behavior is from a distance
Pilot Study
A pilot study is a small scale preliminary study conducted in order to evaluate the feasibility of the key s teps in a future, full-scale project.
A pilot study is an initial run-through of the procedures to be used in an investigation; it involves selecting a few people and trying out the study on them. It is possible to save time, and in some cases, money, by identifying any flaws in the procedures designed by the researcher.
A pilot study can help the researcher spot any ambiguities (i.e. unusual things) or confusion in the information given to participants or problems with the task devised.
Sometimes the task is too hard, and the researcher may get a floor effect, because none of the participants can score at all or can complete the task – all performances are low.
The opposite effect is a ceiling effect, when the task is so easy that all achieve virtually full marks or top performances and are “hitting the ceiling”.
Research Design
In cross-sectional research , a researcher compares multiple segments of the population at the same time
Sometimes, we want to see how people change over time, as in studies of human development and lifespan. Longitudinal research is a research design in which data-gathering is administered repeatedly over an extended period of time.
In cohort studies , the participants must share a common factor or characteristic such as age, demographic, or occupation. A cohort study is a type of longitudinal study in which researchers monitor and observe a chosen population over an extended period.
Triangulation means using more than one research method to improve the study’s validity.
Reliability
Reliability is a measure of consistency, if a particular measurement is repeated and the same result is obtained then it is described as being reliable.
- Test-retest reliability : assessing the same person on two different occasions which shows the extent to which the test produces the same answers.
- Inter-observer reliability : the extent to which there is an agreement between two or more observers.
Meta-Analysis
Meta-analysis is a statistical procedure used to combine and synthesize findings from multiple independent studies to estimate the average effect size for a particular research question.
Meta-analysis goes beyond traditional narrative reviews by using statistical methods to integrate the results of several studies, leading to a more objective appraisal of the evidence.
This is done by looking through various databases, and then decisions are made about what studies are to be included/excluded.
- Strengths : Increases the conclusions’ validity as they’re based on a wider range.
- Weaknesses : Research designs in studies can vary, so they are not truly comparable.
Peer Review
A researcher submits an article to a journal. The choice of the journal may be determined by the journal’s audience or prestige.
The journal selects two or more appropriate experts (psychologists working in a similar field) to peer review the article without payment. The peer reviewers assess: the methods and designs used, originality of the findings, the validity of the original research findings and its content, structure and language.
Feedback from the reviewer determines whether the article is accepted. The article may be: Accepted as it is, accepted with revisions, sent back to the author to revise and re-submit or rejected without the possibility of submission.
The editor makes the final decision whether to accept or reject the research report based on the reviewers comments/ recommendations.
Peer review is important because it prevent faulty data from entering the public domain, it provides a way of checking the validity of findings and the quality of the methodology and is used to assess the research rating of university departments.
Peer reviews may be an ideal, whereas in practice there are lots of problems. For example, it slows publication down and may prevent unusual, new work being published. Some reviewers might use it as an opportunity to prevent competing researchers from publishing work.
Some people doubt whether peer review can really prevent the publication of fraudulent research.
The advent of the internet means that a lot of research and academic comment is being published without official peer reviews than before, though systems are evolving on the internet where everyone really has a chance to offer their opinions and police the quality of research.
Types of Data
- Quantitative data is numerical data e.g. reaction time or number of mistakes. It represents how much or how long, how many there are of something. A tally of behavioral categories and closed questions in a questionnaire collect quantitative data.
- Qualitative data is virtually any type of information that can be observed and recorded that is not numerical in nature and can be in the form of written or verbal communication. Open questions in questionnaires and accounts from observational studies collect qualitative data.
- Primary data is first-hand data collected for the purpose of the investigation.
- Secondary data is information that has been collected by someone other than the person who is conducting the research e.g. taken from journals, books or articles.
Validity means how well a piece of research actually measures what it sets out to, or how well it reflects the reality it claims to represent.
Validity is whether the observed effect is genuine and represents what is actually out there in the world.
- Concurrent validity is the extent to which a psychological measure relates to an existing similar measure and obtains close results. For example, a new intelligence test compared to an established test.
- Face validity : does the test measure what it’s supposed to measure ‘on the face of it’. This is done by ‘eyeballing’ the measuring or by passing it to an expert to check.
- Ecological validit y is the extent to which findings from a research study can be generalized to other settings / real life.
- Temporal validity is the extent to which findings from a research study can be generalized to other historical times.
Features of Science
- Paradigm – A set of shared assumptions and agreed methods within a scientific discipline.
- Paradigm shift – The result of the scientific revolution: a significant change in the dominant unifying theory within a scientific discipline.
- Objectivity – When all sources of personal bias are minimised so not to distort or influence the research process.
- Empirical method – Scientific approaches that are based on the gathering of evidence through direct observation and experience.
- Replicability – The extent to which scientific procedures and findings can be repeated by other researchers.
- Falsifiability – The principle that a theory cannot be considered scientific unless it admits the possibility of being proved untrue.
Statistical Testing
A significant result is one where there is a low probability that chance factors were responsible for any observed difference, correlation, or association in the variables tested.
If our test is significant, we can reject our null hypothesis and accept our alternative hypothesis.
If our test is not significant, we can accept our null hypothesis and reject our alternative hypothesis. A null hypothesis is a statement of no effect.
In Psychology, we use p < 0.05 (as it strikes a balance between making a type I and II error) but p < 0.01 is used in tests that could cause harm like introducing a new drug.
A type I error is when the null hypothesis is rejected when it should have been accepted (happens when a lenient significance level is used, an error of optimism).
A type II error is when the null hypothesis is accepted when it should have been rejected (happens when a stringent significance level is used, an error of pessimism).
Ethical Issues
- Informed consent is when participants are able to make an informed judgment about whether to take part. It causes them to guess the aims of the study and change their behavior.
- To deal with it, we can gain presumptive consent or ask them to formally indicate their agreement to participate but it may invalidate the purpose of the study and it is not guaranteed that the participants would understand.
- Deception should only be used when it is approved by an ethics committee, as it involves deliberately misleading or withholding information. Participants should be fully debriefed after the study but debriefing can’t turn the clock back.
- All participants should be informed at the beginning that they have the right to withdraw if they ever feel distressed or uncomfortable.
- It causes bias as the ones that stayed are obedient and some may not withdraw as they may have been given incentives or feel like they’re spoiling the study. Researchers can offer the right to withdraw data after participation.
- Participants should all have protection from harm . The researcher should avoid risks greater than those experienced in everyday life and they should stop the study if any harm is suspected. However, the harm may not be apparent at the time of the study.
- Confidentiality concerns the communication of personal information. The researchers should not record any names but use numbers or false names though it may not be possible as it is sometimes possible to work out who the researchers were.

Educational resources and simple solutions for your research journey
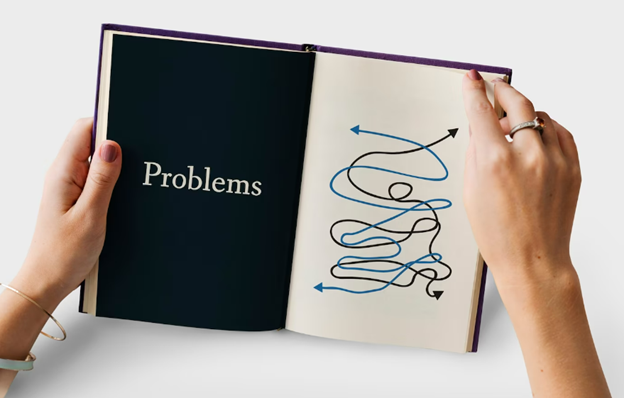
What is a Research Hypothesis: How to Write it, Types, and Examples
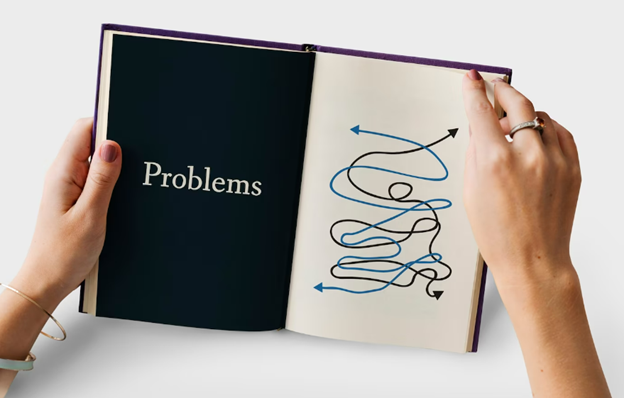
Any research begins with a research question and a research hypothesis . A research question alone may not suffice to design the experiment(s) needed to answer it. A hypothesis is central to the scientific method. But what is a hypothesis ? A hypothesis is a testable statement that proposes a possible explanation to a phenomenon, and it may include a prediction. Next, you may ask what is a research hypothesis ? Simply put, a research hypothesis is a prediction or educated guess about the relationship between the variables that you want to investigate.
It is important to be thorough when developing your research hypothesis. Shortcomings in the framing of a hypothesis can affect the study design and the results. A better understanding of the research hypothesis definition and characteristics of a good hypothesis will make it easier for you to develop your own hypothesis for your research. Let’s dive in to know more about the types of research hypothesis , how to write a research hypothesis , and some research hypothesis examples .
Table of Contents
What is a hypothesis ?
A hypothesis is based on the existing body of knowledge in a study area. Framed before the data are collected, a hypothesis states the tentative relationship between independent and dependent variables, along with a prediction of the outcome.
What is a research hypothesis ?
Young researchers starting out their journey are usually brimming with questions like “ What is a hypothesis ?” “ What is a research hypothesis ?” “How can I write a good research hypothesis ?”
A research hypothesis is a statement that proposes a possible explanation for an observable phenomenon or pattern. It guides the direction of a study and predicts the outcome of the investigation. A research hypothesis is testable, i.e., it can be supported or disproven through experimentation or observation.

Characteristics of a good hypothesis
Here are the characteristics of a good hypothesis :
- Clearly formulated and free of language errors and ambiguity
- Concise and not unnecessarily verbose
- Has clearly defined variables
- Testable and stated in a way that allows for it to be disproven
- Can be tested using a research design that is feasible, ethical, and practical
- Specific and relevant to the research problem
- Rooted in a thorough literature search
- Can generate new knowledge or understanding.
How to create an effective research hypothesis
A study begins with the formulation of a research question. A researcher then performs background research. This background information forms the basis for building a good research hypothesis . The researcher then performs experiments, collects, and analyzes the data, interprets the findings, and ultimately, determines if the findings support or negate the original hypothesis.
Let’s look at each step for creating an effective, testable, and good research hypothesis :
- Identify a research problem or question: Start by identifying a specific research problem.
- Review the literature: Conduct an in-depth review of the existing literature related to the research problem to grasp the current knowledge and gaps in the field.
- Formulate a clear and testable hypothesis : Based on the research question, use existing knowledge to form a clear and testable hypothesis . The hypothesis should state a predicted relationship between two or more variables that can be measured and manipulated. Improve the original draft till it is clear and meaningful.
- State the null hypothesis: The null hypothesis is a statement that there is no relationship between the variables you are studying.
- Define the population and sample: Clearly define the population you are studying and the sample you will be using for your research.
- Select appropriate methods for testing the hypothesis: Select appropriate research methods, such as experiments, surveys, or observational studies, which will allow you to test your research hypothesis .
Remember that creating a research hypothesis is an iterative process, i.e., you might have to revise it based on the data you collect. You may need to test and reject several hypotheses before answering the research problem.
How to write a research hypothesis
When you start writing a research hypothesis , you use an “if–then” statement format, which states the predicted relationship between two or more variables. Clearly identify the independent variables (the variables being changed) and the dependent variables (the variables being measured), as well as the population you are studying. Review and revise your hypothesis as needed.
An example of a research hypothesis in this format is as follows:
“ If [athletes] follow [cold water showers daily], then their [endurance] increases.”
Population: athletes
Independent variable: daily cold water showers
Dependent variable: endurance
You may have understood the characteristics of a good hypothesis . But note that a research hypothesis is not always confirmed; a researcher should be prepared to accept or reject the hypothesis based on the study findings.
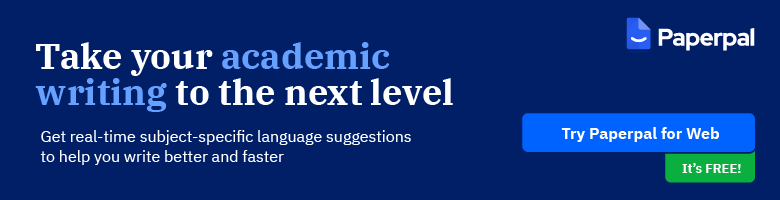
Research hypothesis checklist
Following from above, here is a 10-point checklist for a good research hypothesis :
- Testable: A research hypothesis should be able to be tested via experimentation or observation.
- Specific: A research hypothesis should clearly state the relationship between the variables being studied.
- Based on prior research: A research hypothesis should be based on existing knowledge and previous research in the field.
- Falsifiable: A research hypothesis should be able to be disproven through testing.
- Clear and concise: A research hypothesis should be stated in a clear and concise manner.
- Logical: A research hypothesis should be logical and consistent with current understanding of the subject.
- Relevant: A research hypothesis should be relevant to the research question and objectives.
- Feasible: A research hypothesis should be feasible to test within the scope of the study.
- Reflects the population: A research hypothesis should consider the population or sample being studied.
- Uncomplicated: A good research hypothesis is written in a way that is easy for the target audience to understand.
By following this research hypothesis checklist , you will be able to create a research hypothesis that is strong, well-constructed, and more likely to yield meaningful results.
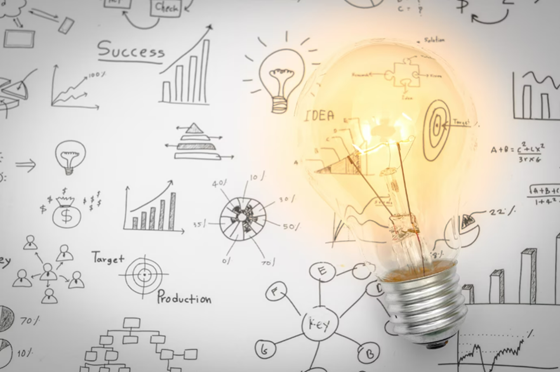
Types of research hypothesis
Different types of research hypothesis are used in scientific research:
1. Null hypothesis:
A null hypothesis states that there is no change in the dependent variable due to changes to the independent variable. This means that the results are due to chance and are not significant. A null hypothesis is denoted as H0 and is stated as the opposite of what the alternative hypothesis states.
Example: “ The newly identified virus is not zoonotic .”
2. Alternative hypothesis:
This states that there is a significant difference or relationship between the variables being studied. It is denoted as H1 or Ha and is usually accepted or rejected in favor of the null hypothesis.
Example: “ The newly identified virus is zoonotic .”
3. Directional hypothesis :
This specifies the direction of the relationship or difference between variables; therefore, it tends to use terms like increase, decrease, positive, negative, more, or less.
Example: “ The inclusion of intervention X decreases infant mortality compared to the original treatment .”
4. Non-directional hypothesis:
While it does not predict the exact direction or nature of the relationship between the two variables, a non-directional hypothesis states the existence of a relationship or difference between variables but not the direction, nature, or magnitude of the relationship. A non-directional hypothesis may be used when there is no underlying theory or when findings contradict previous research.
Example, “ Cats and dogs differ in the amount of affection they express .”
5. Simple hypothesis :
A simple hypothesis only predicts the relationship between one independent and another independent variable.
Example: “ Applying sunscreen every day slows skin aging .”
6 . Complex hypothesis :
A complex hypothesis states the relationship or difference between two or more independent and dependent variables.
Example: “ Applying sunscreen every day slows skin aging, reduces sun burn, and reduces the chances of skin cancer .” (Here, the three dependent variables are slowing skin aging, reducing sun burn, and reducing the chances of skin cancer.)
7. Associative hypothesis:
An associative hypothesis states that a change in one variable results in the change of the other variable. The associative hypothesis defines interdependency between variables.
Example: “ There is a positive association between physical activity levels and overall health .”
8 . Causal hypothesis:
A causal hypothesis proposes a cause-and-effect interaction between variables.
Example: “ Long-term alcohol use causes liver damage .”
Note that some of the types of research hypothesis mentioned above might overlap. The types of hypothesis chosen will depend on the research question and the objective of the study.
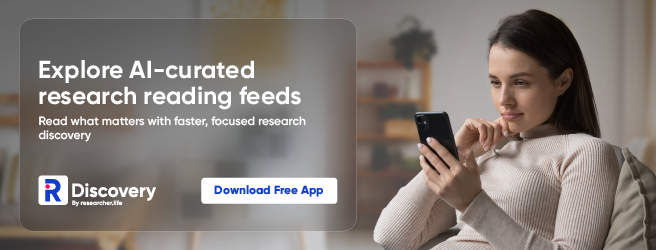
Research hypothesis examples
Here are some good research hypothesis examples :
“The use of a specific type of therapy will lead to a reduction in symptoms of depression in individuals with a history of major depressive disorder.”
“Providing educational interventions on healthy eating habits will result in weight loss in overweight individuals.”
“Plants that are exposed to certain types of music will grow taller than those that are not exposed to music.”
“The use of the plant growth regulator X will lead to an increase in the number of flowers produced by plants.”
Characteristics that make a research hypothesis weak are unclear variables, unoriginality, being too general or too vague, and being untestable. A weak hypothesis leads to weak research and improper methods.
Some bad research hypothesis examples (and the reasons why they are “bad”) are as follows:
“This study will show that treatment X is better than any other treatment . ” (This statement is not testable, too broad, and does not consider other treatments that may be effective.)
“This study will prove that this type of therapy is effective for all mental disorders . ” (This statement is too broad and not testable as mental disorders are complex and different disorders may respond differently to different types of therapy.)
“Plants can communicate with each other through telepathy . ” (This statement is not testable and lacks a scientific basis.)
Importance of testable hypothesis
If a research hypothesis is not testable, the results will not prove or disprove anything meaningful. The conclusions will be vague at best. A testable hypothesis helps a researcher focus on the study outcome and understand the implication of the question and the different variables involved. A testable hypothesis helps a researcher make precise predictions based on prior research.
To be considered testable, there must be a way to prove that the hypothesis is true or false; further, the results of the hypothesis must be reproducible.
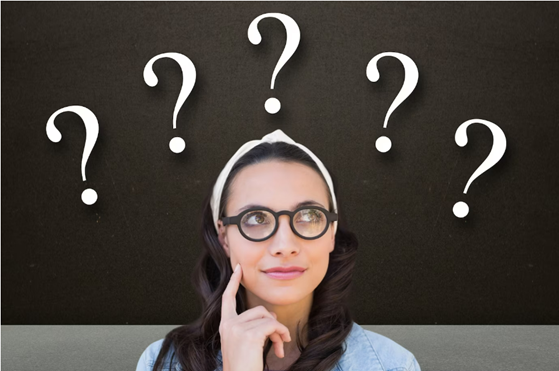
Frequently Asked Questions (FAQs) on research hypothesis
1. What is the difference between research question and research hypothesis ?
A research question defines the problem and helps outline the study objective(s). It is an open-ended statement that is exploratory or probing in nature. Therefore, it does not make predictions or assumptions. It helps a researcher identify what information to collect. A research hypothesis , however, is a specific, testable prediction about the relationship between variables. Accordingly, it guides the study design and data analysis approach.
2. When to reject null hypothesis ?
A null hypothesis should be rejected when the evidence from a statistical test shows that it is unlikely to be true. This happens when the test statistic (e.g., p -value) is less than the defined significance level (e.g., 0.05). Rejecting the null hypothesis does not necessarily mean that the alternative hypothesis is true; it simply means that the evidence found is not compatible with the null hypothesis.
3. How can I be sure my hypothesis is testable?
A testable hypothesis should be specific and measurable, and it should state a clear relationship between variables that can be tested with data. To ensure that your hypothesis is testable, consider the following:
- Clearly define the key variables in your hypothesis. You should be able to measure and manipulate these variables in a way that allows you to test the hypothesis.
- The hypothesis should predict a specific outcome or relationship between variables that can be measured or quantified.
- You should be able to collect the necessary data within the constraints of your study.
- It should be possible for other researchers to replicate your study, using the same methods and variables.
- Your hypothesis should be testable by using appropriate statistical analysis techniques, so you can draw conclusions, and make inferences about the population from the sample data.
- The hypothesis should be able to be disproven or rejected through the collection of data.
4. How do I revise my research hypothesis if my data does not support it?
If your data does not support your research hypothesis , you will need to revise it or develop a new one. You should examine your data carefully and identify any patterns or anomalies, re-examine your research question, and/or revisit your theory to look for any alternative explanations for your results. Based on your review of the data, literature, and theories, modify your research hypothesis to better align it with the results you obtained. Use your revised hypothesis to guide your research design and data collection. It is important to remain objective throughout the process.
5. I am performing exploratory research. Do I need to formulate a research hypothesis?
As opposed to “confirmatory” research, where a researcher has some idea about the relationship between the variables under investigation, exploratory research (or hypothesis-generating research) looks into a completely new topic about which limited information is available. Therefore, the researcher will not have any prior hypotheses. In such cases, a researcher will need to develop a post-hoc hypothesis. A post-hoc research hypothesis is generated after these results are known.
6. How is a research hypothesis different from a research question?
A research question is an inquiry about a specific topic or phenomenon, typically expressed as a question. It seeks to explore and understand a particular aspect of the research subject. In contrast, a research hypothesis is a specific statement or prediction that suggests an expected relationship between variables. It is formulated based on existing knowledge or theories and guides the research design and data analysis.
7. Can a research hypothesis change during the research process?
Yes, research hypotheses can change during the research process. As researchers collect and analyze data, new insights and information may emerge that require modification or refinement of the initial hypotheses. This can be due to unexpected findings, limitations in the original hypotheses, or the need to explore additional dimensions of the research topic. Flexibility is crucial in research, allowing for adaptation and adjustment of hypotheses to align with the evolving understanding of the subject matter.
8. How many hypotheses should be included in a research study?
The number of research hypotheses in a research study varies depending on the nature and scope of the research. It is not necessary to have multiple hypotheses in every study. Some studies may have only one primary hypothesis, while others may have several related hypotheses. The number of hypotheses should be determined based on the research objectives, research questions, and the complexity of the research topic. It is important to ensure that the hypotheses are focused, testable, and directly related to the research aims.
9. Can research hypotheses be used in qualitative research?
Yes, research hypotheses can be used in qualitative research, although they are more commonly associated with quantitative research. In qualitative research, hypotheses may be formulated as tentative or exploratory statements that guide the investigation. Instead of testing hypotheses through statistical analysis, qualitative researchers may use the hypotheses to guide data collection and analysis, seeking to uncover patterns, themes, or relationships within the qualitative data. The emphasis in qualitative research is often on generating insights and understanding rather than confirming or rejecting specific research hypotheses through statistical testing.
Editage All Access is a subscription-based platform that unifies the best AI tools and services designed to speed up, simplify, and streamline every step of a researcher’s journey. The Editage All Access Pack is a one-of-a-kind subscription that unlocks full access to an AI writing assistant, literature recommender, journal finder, scientific illustration tool, and exclusive discounts on professional publication services from Editage.
Based on 22+ years of experience in academia, Editage All Access empowers researchers to put their best research forward and move closer to success. Explore our top AI Tools pack, AI Tools + Publication Services pack, or Build Your Own Plan. Find everything a researcher needs to succeed, all in one place – Get All Access now starting at just $14 a month !
Related Posts

Back to School – Lock-in All Access Pack for a Year at the Best Price

Journal Turnaround Time: Researcher.Life and Scholarly Intelligence Join Hands to Empower Researchers with Publication Time Insights
- Dissertation Proofreading and Editing
- Dissertation Service
- Dissertation Proposal Service
- Dissertation Chapter
- Dissertation Topic and Outline
- Statistical Analysis Services
- Model Answers and Exam Notes
- Dissertation Samples
- Essay Writing Service
- Assignment Service
- Report Service
- Coursework Service
- Literature Review Service
- Reflective Report Service
- Presentation Service
- Poster Service
- Criminal Psychology Dissertation Topics | List of Trending Ideas With Research Aims
- Cognitive Psychology Dissertation Topics | 10 Top Ideas For Research in 2024
- Social Psychology Dissertation Topics | 10 Latest Research Ideas
- Top 10 Clinical Psychology Dissertation Topics with Research Aims
- Educational Psychology Dissertation Topics | 10 Interesting Ideas For Research
- Customer Service Dissertation Topics | List of Latest Ideas For Students
- 15 Interesting Music Dissertation Topics
- Business Intelligence Dissertation Topics | List of Top Ideas With Research Aims
- Physical Education Dissertation Topics | 15 Interesting Title Examples
- 15 Top Forensic Science Dissertation Topics with Research Aims
- Islamic Finance Dissertation Topics | List of 15 Top Ideas With Research Aims
- Dissertation Examples
- Dissertation Proposal Examples
- Essay Examples
- Report Examples
- Coursework Examples
- Assignment Examples
- Literature Review Examples
- Dissertation Topic and Outline Examples
- Dissertation Chapter Examples
- Dissertation Help
- Dissertation Topics
- Academic Library
- Assignment Plagiarism Checker
- Coursework Plagiarism Checke
- Dissertation Plagiarism Checker
- Thesis Plagiarism Checker
- Report Plagiarism Checke
- Plagiarism Remover Service
- Plagiarism Checker Free Service
- Turnitin Plagiarism Checker Free Service
- Free Plagiarism Checker for Students
- Difference Between Paraphrasing & Plagiarism
- Free Similarity Checker
- How Plagiarism Checkers Work?
- How to Cite Sources to Avoid Plagiarism?
- Free Topics
- Get a Free Quote
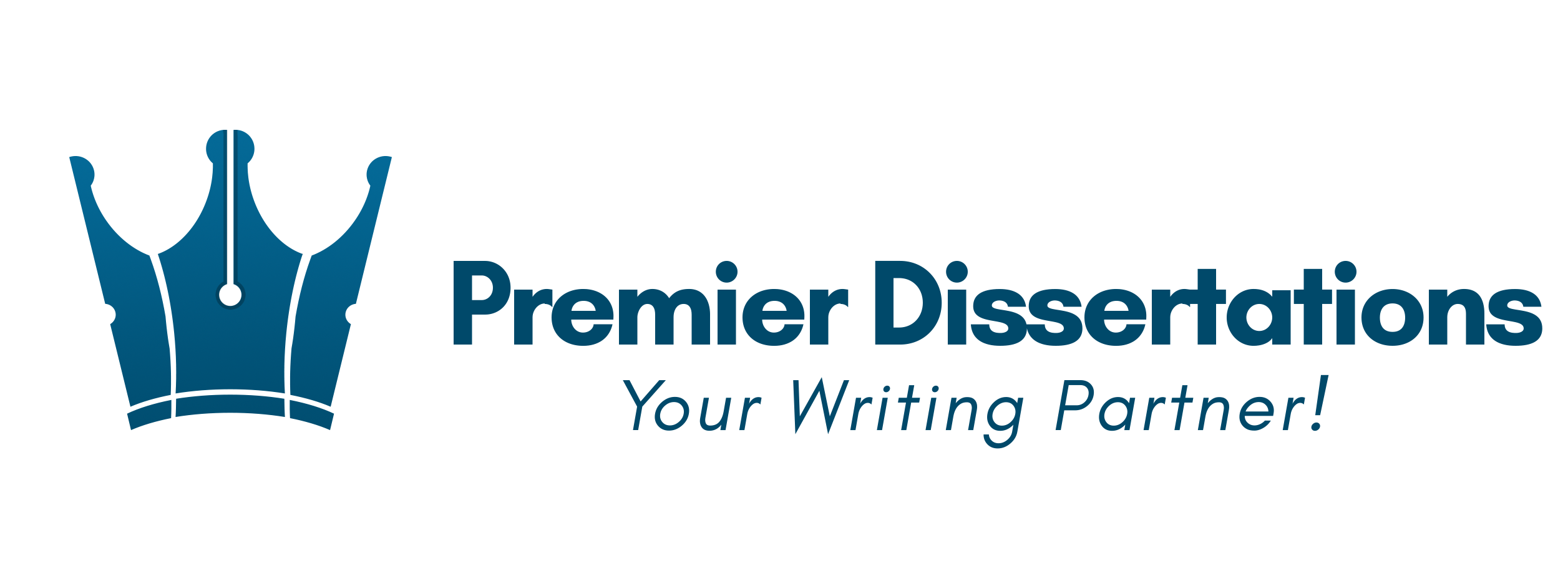
- Report Generating Service
- Model Answers and Exam Notes Writing
- Reflective or Personal Report Writing
- Poster Writing
- Literature Review Writing
- Premier Sample Dissertations
- Course Work
- Cognitive Psychology Dissertation Topics
- Physical Education Dissertation Topics
- 15 Top Forensic Science Dissertation Topics
- Top 10 Clinical Psychology Dissertation Topics
- Islamic Finance Dissertation Topics
- Social Psychology Dissertation Topics
- Educational Psychology Dissertation Topics
- Business Intelligence Dissertation Topics
- Customer Service Dissertation Topics
- Criminal Psychology Dissertation Topics

- Literature Review Example
- Report Example
- Assignment Example
- Coursework Example

- Coursework Plagiarism Checker
- Turnitin Plagiarism Checker
- Paraphrasing and Plagiarism
- Best Dissertation Plagiarism Checker
- Report Plagiarism Checker
- Similarity Checker
- Plagiarism Checker Free
- FREE Topics
Get an experienced writer start working
Review our examples before placing an order, learn how to draft academic papers, research hypotheses: directional vs. non-directional hypotheses.
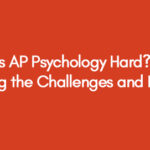
Is AP Psychology Hard? Exploring the Challenges and Rewards
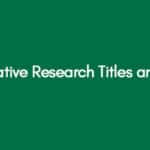
280+ Quantitative Research Titles and Topics
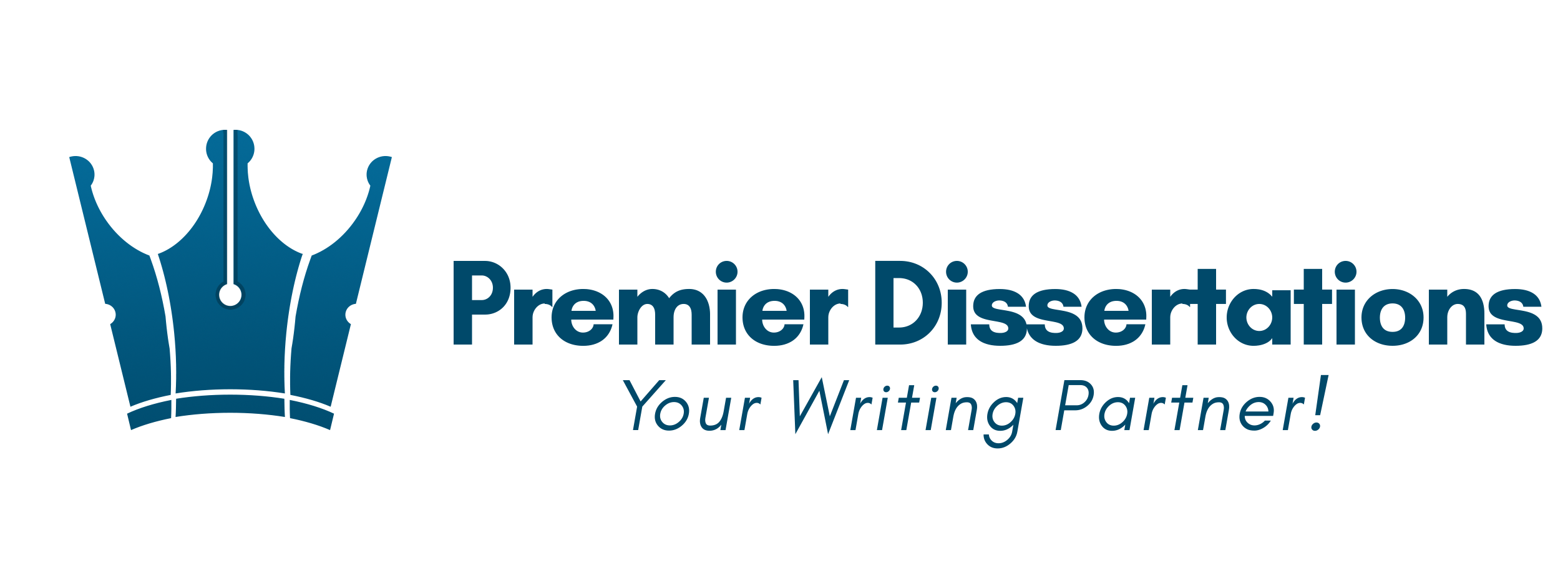
- Dissertation
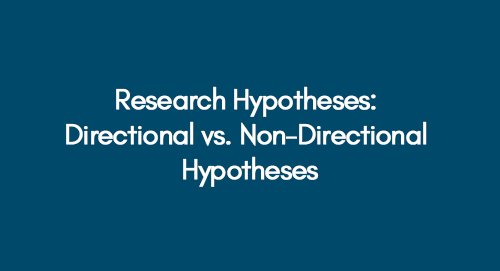
A research hypothesis is a statement that predicts or expects a relationship between variables, and it is tested through research. To create a hypothesis, researchers often review existing literature on the topic. This hypothesis is based on theories, observations, or empirical evidence. It guides the research process, including experiment design, data collection, and analysis. Ultimately, the hypothesis aims to predict the outcome of the study.
What is a Hypothesis in a Dissertation?
This article compares directional and non-directional hypotheses and provides guidelines for writing an effective hypothesis in research. The study explores the differences in predictions and research design implications between the two hypotheses.
3-Step Dissertation Process!

Get 3+ Topics

Dissertation Proposal

Get Final Dissertation
Types of hypothesis.
There are two main types of hypotheses in research:
Null Hypothesis (H0)
The null hypothesis is the default assumption in statistical analysis that there is no significant relationship or effect between the variables being studied. It suggests that any observed differences or relationships are due to chance.
Alternative Hypothesis (Ha or H1)
The alternative hypothesis proposes a significant relationship or effect between variables, contradicting the null hypothesis. It reflects the researcher's expectations based on existing theories or observations.
What is Directional Hypotheses?
A directional hypothesis is a type of hypothesis that is used to predict a specific change or outcome in a research study. It is typically used when researchers have a clear idea of the direction in which they expect their results to go, either an increase or decrease, and want to test this prediction. By making a directional hypothesis, researchers can focus their research efforts and design studies that are more likely to uncover meaningful results. In essence, a directional hypothesis is a statement that predicts the direction of the change that is expected to occur between two groups or variables that are being investigated.
Examples of Directional Hypothesis
Example 1: Online versus Traditional Classroom Learning
For instance, consider a study comparing the average study time of college students in online courses versus those in traditional classroom settings. Drawing on prior research indicating that online learning might lead to reduced engagement, a potential directional hypothesis could be: "Students enrolled in online classes will spend fewer weekly study hours than those in traditional classrooms."
In this scenario, our hypothesis presents a clear expectation—that the average number of weekly study hours among online learners will be lower than that of traditional learners. If the actual findings reveal no significant difference or even higher study times among online learners, then our hypothesis would be refuted.
Example 2: Carbon Dioxide Levels and Global Warming
A directional hypothesis in this scenario would propose a specific change in direction between these two variables. For instance, a directional hypothesis might state that as carbon dioxide levels increase, global temperatures will also rise. This hypothesis suggests a causal relationship between the increase in CO2 levels and the phenomenon of global warming, indicating a direction of change in global temperatures corresponding to changes in CO2 levels.
What is a Non-Directional Hypotheses?
In scientific research, a non-directional hypothesis, or null hypothesis, is a statement that suggests the absence of a relationship or difference between the variables being studied. This type of hypothesis is used to test the validity of a research question by assuming that there is no significant effect or relationship between the variables under investigation. The null hypothesis is typically tested against an alternative hypothesis, which proposes that there is a significant effect or relationship between the variables. If the null hypothesis is rejected, it means that there is enough evidence to suggest that the alternative hypothesis is true, and the variables are indeed related or different from each other.
Non-Directional Hypothesis Example
Example: Is there a difference in anxiety levels between students who receive traditional classroom instruction and those who participate in online learning?
In this non-directional hypothesis, researchers are interested in understanding if there's a disparity in anxiety levels between students who are taught in traditional classrooms versus those who learn online. The non-directional hypothesis posits that there won't be any notable variance in anxiety levels between the two groups. This means that the researchers are not predicting whether one group will have higher or lower anxiety levels; rather, they are exploring if there's any difference at all.
Testimonials
Very satisfied students
This is our reason for working. We want to make all students happy, every day. Review us on Sitejabber
Directional vs. Non-Directional Hypotheses in Research
Both directional and non directional hypothesis have their place in research, and choosing the appropriate type depends on the research question being investigated. Researchers can use directional or non-directional hypotheses in their studies, depending on their specific expectations about the relationship between variables. A directional hypothesis predicts a specific direction of change, while a non-directional hypothesis predicts that there will be a difference between groups or conditions without specifying the direction of that difference. It's important to understand the difference between these types of hypotheses to conduct rigorous and insightful research. Directional hypotheses are useful when researchers want to test a specific expectation about the relationship between variables, while non-directional hypotheses are more appropriate when researchers simply want to test if there is any difference between groups or conditions.
How to Write an Effective Hypothesis in Research?
Writing an effective hypothesis involves several key steps to ensure clarity, testability, and relevance to the research question. Here's a guide on how to write an effective hypothesis:
- Identify the Research Question: Start by clearly defining the research question or problem you want to investigate. Your hypothesis should directly address this question.
- State the Null Hypothesis: The null hypothesis (H0) is a statement that there is no relationship or effect between the variables being studied. It serves as the default assumption and is typically stated as the absence of an effect or difference.
- Formulate the Alternative Hypothesis: The alternative hypothesis (H1 or Ha) is the statement that contradicts the null hypothesis and suggests that there is a relationship or effect between the variables. It reflects what you expect to find in your research.
- Make it Testable: Your hypothesis should be testable through empirical observation or experimentation. This means that there must be a way to collect data or evidence to support or refute the hypothesis.
- Be Specific and Clear: Clearly state the variables involved and the expected relationship between them. Avoid vague or ambiguous language to ensure that your hypothesis is easy to understand and interpret.
- Use Quantifiable Terms: Whenever possible, use quantifiable terms or measurable variables in your hypothesis. This makes it easier to collect data and analyze results objectively.
- Consider the Scope: Ensure that your hypothesis is focused and specific to the research hypothesis at hand. Avoid making broad generalizations that are difficult to test or validate.
- Revise and Refine: Once you've drafted your hypothesis, review it carefully to ensure accuracy and coherence. Revise as needed to clarify any ambiguities or inconsistencies.
Need Help with Academic Writing? Get a Response within 24 Hours!
How Does It Work ?
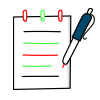
Fill the Form
Please fill the free topic form and share your requirements
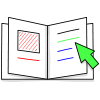
Writer Starts Working
The writer starts to find a topic for you (based on your requirements)
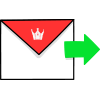
3+ Topics Emailed!
The writer shared custom topics with you within 24 hours
In conclusion, directional hypotheses predict whether variables will increase or decrease, providing a definite expectation about the direction of the relationship under investigation. Non-directional hypotheses, on the other hand, only claim that there is a difference between variables without specifying the direction of the change, leaving it open to any possibility. Both types of hypotheses play an important role in guiding research investigations and developing testable predictions.
Get 3+ Free Dissertation Topics within 24 hours?
Your Number
Academic Level Select Academic Level Undergraduate Masters PhD
Area of Research
Editor Arsalan
Related posts.
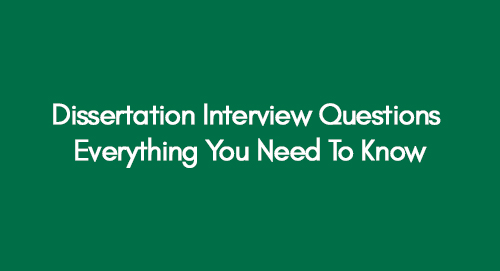
Dissertation Interview Questions | Everything You Need To Know
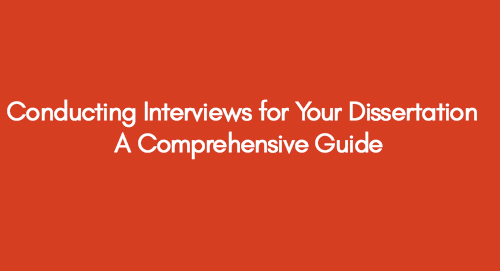
Conducting Interviews for Your Dissertation | A Comprehensive Guide
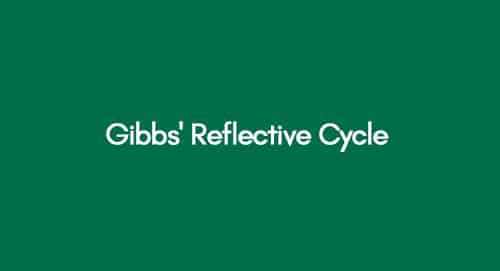
What is Gibbs’ Reflective Cycle and How Can It Benefit You? | Applications and Example
Comments are closed.
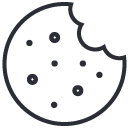
Thank you for visiting nature.com. You are using a browser version with limited support for CSS. To obtain the best experience, we recommend you use a more up to date browser (or turn off compatibility mode in Internet Explorer). In the meantime, to ensure continued support, we are displaying the site without styles and JavaScript.
- View all journals
- Explore content
- About the journal
- Publish with us
- Sign up for alerts
- Open access
- Published: 02 September 2024
Green spaces provide substantial but unequal urban cooling globally
- Yuxiang Li 1 ,
- Jens-Christian Svenning ORCID: orcid.org/0000-0002-3415-0862 2 ,
- Weiqi Zhou ORCID: orcid.org/0000-0001-7323-4906 3 , 4 , 5 ,
- Kai Zhu ORCID: orcid.org/0000-0003-1587-3317 6 ,
- Jesse F. Abrams ORCID: orcid.org/0000-0003-0411-8519 7 ,
- Timothy M. Lenton ORCID: orcid.org/0000-0002-6725-7498 7 ,
- William J. Ripple 8 ,
- Zhaowu Yu ORCID: orcid.org/0000-0003-4576-4541 9 ,
- Shuqing N. Teng 1 ,
- Robert R. Dunn 10 &
- Chi Xu ORCID: orcid.org/0000-0002-1841-9032 1
Nature Communications volume 15 , Article number: 7108 ( 2024 ) Cite this article
Metrics details
- Climate-change mitigation
- Urban ecology
Climate warming disproportionately impacts countries in the Global South by increasing extreme heat exposure. However, geographic disparities in adaptation capacity are unclear. Here, we assess global inequality in green spaces, which urban residents critically rely on to mitigate outdoor heat stress. We use remote sensing data to quantify daytime cooling by urban greenery in the warm seasons across the ~500 largest cities globally. We show a striking contrast, with Global South cities having ~70% of the cooling capacity of cities in the Global North (2.5 ± 1.0 °C vs. 3.6 ± 1.7 °C). A similar gap occurs for the cooling adaptation benefits received by an average resident in these cities (2.2 ± 0.9 °C vs. 3.4 ± 1.7 °C). This cooling adaptation inequality is due to discrepancies in green space quantity and quality between cities in the Global North and South, shaped by socioeconomic and natural factors. Our analyses further suggest a vast potential for enhancing cooling adaptation while reducing global inequality.
Introduction
Heat extremes are projected to be substantially intensified by global warming 1 , 2 , imposing a major threat to human mortality and morbidity in the coming decades 3 , 4 , 5 , 6 . This threat is particularly concerning as a majority of people now live in cities 7 , including those cities suffering some of the hottest climate extremes. Cities face two forms of warming: warming due to climate change and warming due to the urban heat island effect 8 , 9 , 10 . These two forms of warming have the potential to be additive, or even multiplicative. Climate change in itself is projected to result in rising maximum temperatures above 50 °C for a considerable fraction of the world if 2 °C global warming is exceeded 2 ; the urban heat island effect will cause up to >10 °C additional (surface) warming 11 . Exposures to temperatures above 35 °C with high humidity or above 40 °C with low humidity can lead to lethal heat stress for humans 12 . Even before such lethal temperatures are reached, worker productivity 13 and general health and well-being 14 can suffer. Heat extremes are especially risky for people living in the Global South 15 , 16 due to warmer climates at low latitudes. Climate models project that the lethal temperature thresholds will be exceeded with increasing frequencies and durations, and such extreme conditions will be concentrated in low-latitude regions 17 , 18 , 19 . These low-latitude regions overlap with the major parts of the Global South where population densities are already high and where population growth rates are also high. Consequently, the number of people exposed to extreme heat will likely increase even further, all things being equal 16 , 20 . That population growth will be accompanied by expanded urbanization and intensified urban heat island effects 21 , 22 , potentially exacerbating future Global North-Global South heat stress exposure inequalities.
Fortunately, we know that heat stress can be buffered, in part, by urban vegetation 23 . Urban green spaces, and especially urban forests, have proven an effective means through which to ameliorate heat stress through shading 24 , 25 and transpirational cooling 26 , 27 . The buffering effect of urban green spaces is influenced by their area (relative to the area of the city) and their spatial configuration 28 . In this context, green spaces become a kind of infrastructure that can and should be actively managed. At broad spatial scales, the effect of this urban green infrastructure is also mediated by differences among regions, whether in their background climate 29 , composition of green spaces 30 , or other factors 31 , 32 , 33 , 34 . The geographic patterns of the buffering effects of green spaces, whether due to geographic patterns in their areal extent or region-specific effects, have so far been poorly characterized.
On their own, the effects of climate change and urban heat islands on human health are likely to become severe. However, these effects will become even worse if they fall disproportionately in cities or countries with less economic ability to invest in green space 35 or in other forms of cooling 36 , 37 . A number of studies have now documented the so-called ‘luxury effect,’ wherein lower-income parts of cities tend to have less green space and, as a result, reduced biodiversity 38 , 39 . Where the luxury effect exists, green space and its benefits become, in essence, a luxury good 40 . If the luxury effect holds among cities, and lower-income cities also have smaller green spaces, the Global South may have the least potential to mitigate the combined effects of climate warming and urban heat islands, leading to exacerbated and rising inequalities in heat exposure 41 .
Here, we assess the global inequalities in the cooling capability of existing urban green infrastructure across urban areas worldwide. To this end, we use remotely sensed data to quantify three key variables, i.e., (1) cooling efficiency, (2) cooling capacity, and (3) cooling benefit of existing urban green infrastructure for ~500 major cities across the world. Urban green infrastructure and temperature are generally negatively and relatively linearly correlated at landscape scales, i.e., higher quantities of urban green infrastructure yield lower temperatures 42 , 43 . Cooling efficiency is widely used as a measure of the extent to which a given proportional increase in the area of urban green infrastructure leads to a decrease in temperature, i.e., the slope of the urban green infrastructure-temperature relationship 42 , 44 , 45 (see Methods for details). This simple metric allows quantifying the quality of urban green infrastructure in terms of ameliorating the urban heat island effect. Meanwhile, the extent to which existing urban green infrastructure cools down an entire city’s surface temperatures (compared to the non-vegetated built-up areas) is referred to as cooling capacity. Hence, cooling capacity is a function of the total quantity of urban green infrastructure and its cooling efficiency (see Methods).
As a third step, we account for the spatial distributions of urban green infrastructure and populations to quantify the benefit of cooling mitigation received by an average urban inhabitant in each city given their location. This cooling benefit is a more direct measure of the cooling realized by people, after accounting for the within-city geography of urban green infrastructure and population density. We focus on cooling capacity and cooling benefit as the measures of the cooling capability of individual cities for assessing their global inequalities. We are particularly interested in linking cooling adaptation inequality with income inequality 40 , 46 . While this can be achieved using existing income metrics for country classifications 47 , here we use the traditional Global North/South classification due to its historical ties to geography which is influential in climate research.
Results and discussion
Our analyses indicate that existing green infrastructure of an average city has a capability of cooling down surface temperatures by ~3 °C during warm seasons. However, a concerning disparity is evident; on average Global South cities have only two-thirds the cooling capacity and cooling benefit compared to Global North cities. This inequality is attributable to the differences in both quantity and quality of existing urban green infrastructure among cities. Importantly, we find that there exists considerable potential for many cities to enhance the cooling capability of their green infrastructure; achieving this potential could dramatically reduce global inequalities in adaptation to outdoor heat stress.
Quantifying cooling inequality
Our analyses showed that both the quantity and quality of the existing urban green infrastructure vary greatly among the world’s ~500 most populated cities (see Methods for details, and Fig. 1 for examples). The quantity of urban green infrastructure measured based on remotely sensed indicators of spectral greenness (Normalized Difference Vegetation Index, NDVI, see Methods) had a coefficient of variation (CV) of 35%. Similarly, the quality of urban green infrastructure in terms of cooling efficiency (daytime land surface temperatures during peak summer) had a CV of 37% (Supplementary Figs. 1 , 2 ). The global mean value of cooling capacity is 2.9 °C; existing urban green infrastructure ameliorates warm-season heat stress by 2.9 °C of surface temperature in an average city. In truth, however, the variation in cooling capacity was great (global CV in cooling capacity as large as ~50%), such that few cities were average. This variation is strongly geographically structured. Cities closer to the equator - tropical and subtropical cities - tend to have relatively weak cooling capacities (Fig. 2a, b ). As Global South countries are predominantly located at low latitudes, this pattern leads to a situation in which Global South cities, which tend to be hotter and relatively lower-income, have, on average, approximately two-thirds the cooling capacity of the Global North cities (2.5 ± 1.0 vs. 3.6 ± 1.7°C, Wilcoxon test, p = 2.7e-12; Fig. 2c ). The cities that most need to rely on green infrastructure are, at present, those that are least able to do so.
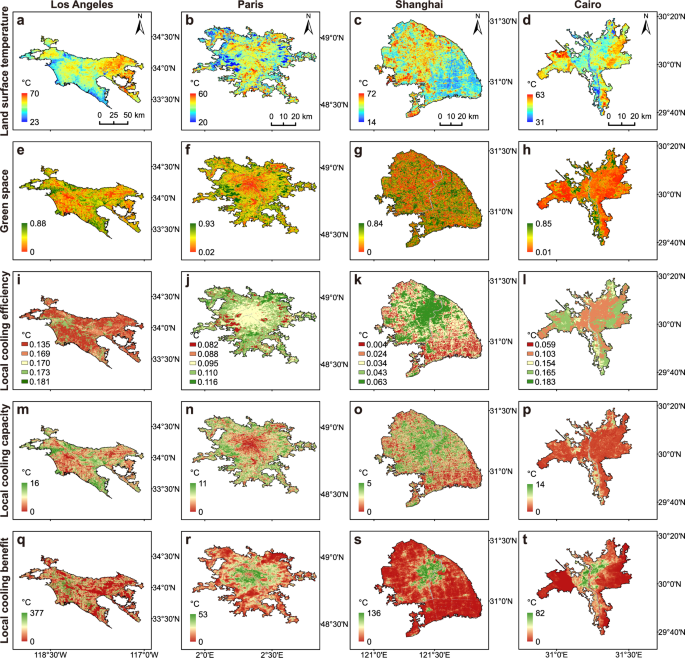
a , e , i , m , q Los Angeles, US. b , f , j , n , r Paris, France. c , g , k , o , s Shanghai, China. d , h , l , p , t Cairo, Egypt. Local cooling efficiency is calculated for different local climate zone types to account for within-city heterogeneity. In densely populated parts of cities, local cooling capacity tends to be lower due to reduced green space area, whereas local cooling benefit (local cooling capacity multiplied by a weight term of local population density relative to city mean) tends to be higher as more urban residents can receive cooling amelioration.
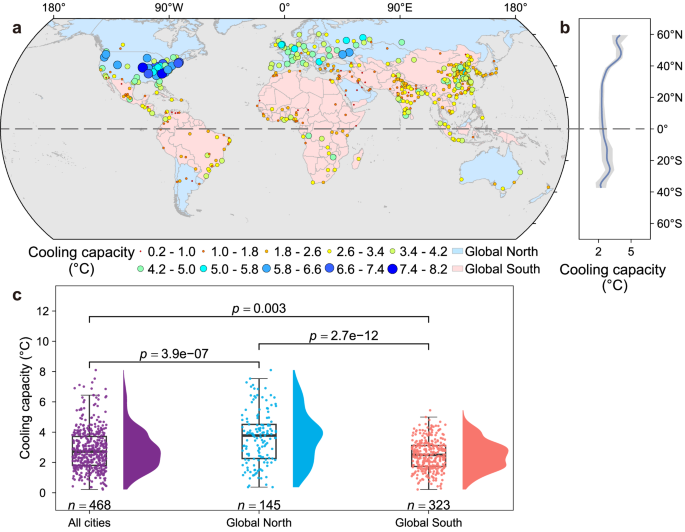
a Global distribution of cooling capacity for the 468 major urbanized areas. b Latitudinal pattern of cooling capacity. c Cooling capacity difference between the Global North and South cities. The cooling capacity offered by urban green infrastructure evinces a latitudinal pattern wherein lower-latitude cities have weaker cooling capacity ( b , cubic-spline fitting of cooling capacity with 95% confidence interval is shown), representing a significant inequality between Global North and South countries: city-level cooling capacity for Global North cities are about 1.5-fold higher than in Global South cities ( c ). Data are presented as box plots, where median values (center black lines), 25th percentiles (box lower bounds), 75th percentiles (box upper bounds), whiskers extending to 1.5-fold of the interquartile range (IQR), and outliers are shown. The tails of the cooling capacity distributions are truncated at zero as all cities have positive values of cooling capacity. Notice that no cities in the Global South have a cooling capacity greater than 5.5 °C ( c ). This is because no cities in the Global South have proportional green space areas as great as those seen in the Global North (see also Fig. 4b ). A similar pattern is found for cooling benefit (Supplementary Fig. 3 ). The two-sided non-parametric Wilcoxon test was used for statistical comparisons.
When we account for the locations of urban green infrastructure relative to humans within cities, the cooling benefit of urban green infrastructure realized by an average urban resident generally becomes slightly lower than suggested by cooling capacity (see Methods; Supplementary Fig. 3 ). Urban residents tend to be densest in the parts of cities with less green infrastructure. As a result, the average urban resident experiences less cooling amelioration than expected. However, this heterogeneity has only a minor effect on global-scale inequality. As a result, the geographic trends in cooling capacity and cooling benefit are similar: mean cooling benefit for an average urban resident also presents a 1.5-fold gap between Global South and North cities (2.2 ± 0.9 vs. 3.4 ± 1.7 °C, Wilcoxon test, p = 3.2e-13; Supplementary Fig. 3c ). Urban green infrastructure is a public good that has the potential to help even the most marginalized populations stay cool; unfortunately, this public benefit is least available in the Global South. When walking outdoors, the average person in an average Global South city receives only two-thirds the cooling amelioration from urban green infrastructure experienced by a person in an average Global North city. The high cooling amelioration capacity and benefit of the Global North cities is heavily influenced by North America (specifically, Canada and the US), which have both the highest cooling efficiency and the largest area of green infrastructure, followed by Europe (Supplementary Fig. 4 ).
One way to illustrate the global inequality of cooling capacity or benefit is to separately look at the cities that are most and least effective in ameliorating outdoor heat stress. Our results showed that ~85% of the 50 most effective cities (with highest cooling capacity or cooling benefit) are located in the Global North, while ~80% of the 50 least effective are Global South cities (Fig. 3 , Supplementary Fig. 5 ). This is true without taking into account the differences in the background temperatures and climate warming of these cities, which will exacerbate the effects on human health; cities in the Global South are likely to be closer to the limits of human thermal comfort and even, increasingly, the limits of the temperatures and humidities (wet-bulb temperatures) at which humans can safely work or even walk, such that the ineffectiveness of green spaces in those cities in cooling will lead to greater negative effects on human health 48 , work 14 , and gross domestic product (GDP) 49 . In addition, Global South cities commonly have higher population densities (Fig. 3 , Supplementary Fig. 5 ) and are projected to have faster population growth 50 . This situation will plausibly intensify the urban heat island effect because of the need of those populations for housing (and hence tensions between the need for buildings and the need for green spaces). It will also increase the number of people exposed to extreme urban heat island effects. Therefore, it is critical to increase cooling benefit via expanding urban green spaces, so that more people can receive the cooling mitigation from a given new neighboring green space if they live closer to each other. Doing so will require policies that incentivize urban green spaces as well as architectural innovations that make innovations such as plant-covered buildings easier and cheaper to implement.
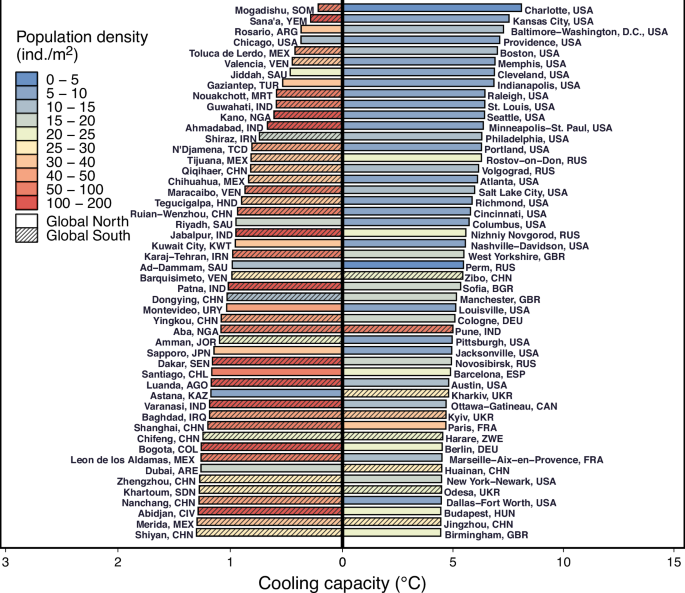
The axes on the right are an order of magnitude greater than those on the left, such that the cooling capacity of Charlotte in the United States is about 37-fold greater than that of Mogadishu (Somalia) and 29-fold greater than that of Sana’a (Yemen). The cities presenting lowest cooling capacities are most associated with Global South cities at higher population densities.
Of course, cities differ even within the Global North or within the Global South. For example, some Global South cities have high green space areas (or relatively high cooling efficiency in combination with moderate green space areas) and hence high cooling capacity. These cities, such as Pune (India), will be important to study in more detail, to shed light on the mechanistic details of their cooling abilities as well as the sociopolitical and other factors that facilitated their high green area coverage and cooling capabilities (Supplementary Figs. 6 , 7 ).
We conducted our primary analyses using a spatial grain of 100-m grid cells and Landsat NDVI data for quantifying spectral greenness. Our results, however, were robust at the coarser spatial grain of 1 km. We find a slightly larger global cooling inequality (~2-fold gap between Global South and North cities) at the 1-km grain using MODIS data (see Methods and Supplementary Fig. 17 ). MODIS data have been frequently used for quantifying urban heat island effects and cooling mitigation 44 , 45 , 51 . Our results reinforce its robustness for comparing urban thermal environments between cities across broad scales.
Influencing factors
The global inequality of cooling amelioration could have a number of proximate causes. To understand their relative influence, we first separately examined the effects of quality (cooling efficiency) and quantity (NDVI as a proxy indicator of urban green space area) of urban green infrastructure. The simplest null model is one in which cooling capacity (at the city scale) and cooling benefit (at the human scale) are driven primarily by the proportional area in a city dedicated to green spaces. Indeed, we found that both cooling capacity and cooling benefit were strongly correlated with urban green space area (Fig. 4 , Supplementary Fig. 8 ). This finding is useful with regards to practical interventions. In general, cities that invest in saving or restoring more green spaces will receive more cooling benefits from those green spaces. By contrast, differences among cities in cooling efficiency played a more minor role in determining the cooling capacity and benefit of cities (Fig. 4 , Supplementary Fig. 8 ).
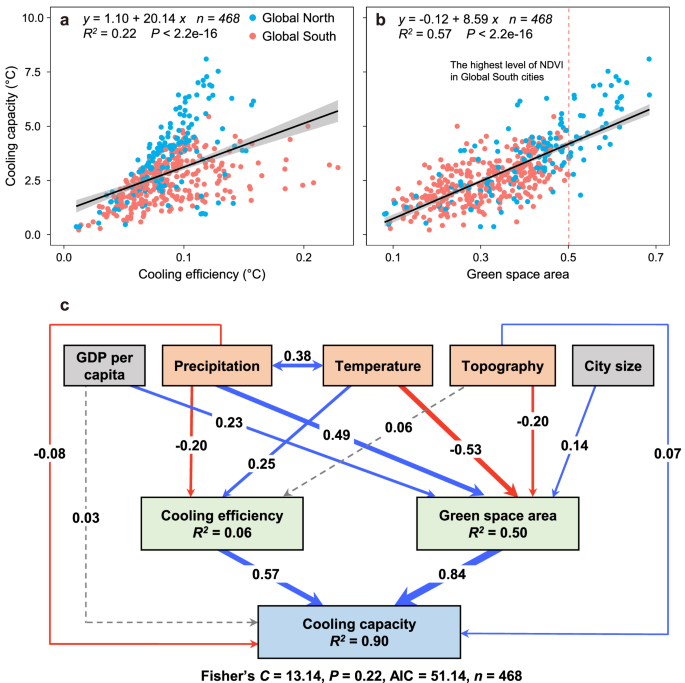
a Relationship between cooling efficiency and cooling capacity. b Relationship between green space area (measured by mean Landsat NDVI in the hottest month of 2018) and cooling capacity. Note that the highest level of urban green space area in the Global South cities is much lower than that in the Global North (dashed line in b ). Gray bands indicate 95% confidence intervals. Two-sided t-tests were conducted. c A piecewise structural equation model based on assumed direct and indirect (through influencing cooling efficiency and urban green space area) effects of essential natural and socioeconomic factors on cooling capacity. Mean annual temperature and precipitation, and topographic variation (elevation range) are selected to represent basic background natural conditions; GDP per capita is selected to represent basic socioeconomic conditions. The spatial extent of built-up areas is included to correct for city size. A bi-directional relationship (correlation) is fitted between mean annual temperature and precipitation. Red and blue solid arrows indicate significantly negative and positive coefficients with p ≤ 0.05, respectively. Gray dashed arrows indicate p > 0.05. The arrow width illustrates the effect size. Similar relationships are found for cooling benefits realized by an average urban resident (see Supplementary Fig. 8 ).
A further question is what shapes the quality and quantity of urban green infrastructure (which in turn are driving cooling capacity)? Many inter-correlated factors are possibly operating at multiple scales, making it difficult to disentangle their effects, especially since experiment-based causal inference is usually not feasible for large-scale urban systems. From a macroscopic perspective, we test the simple hypothesis that the background natural and socioeconomic conditions of cities jointly affect their cooling capacity and benefit in both direct and indirect ways. To this end, we constructed a minimal structural equation model including only the most essential variables reflecting background climate (mean annual temperature and precipitation), topographic variation (elevation range), as well as gross domestic product (GDP) per capita and city area (see Methods; Fig. 4c ).
We found that the quantity of green spaces in a city (again, in proportion to its size) was positively correlated with GDP per capita and city area; wealthier cities have more green spaces. It is well known that wealth and green spaces are positively correlated within cities (the luxury effect) 40 , 46 ; our analysis shows that a similar luxury effect occurs among them at a global scale. In addition, larger cities often have proportionally more green spaces, an effect that may be due to the tendency for large cities (particularly in the US and Canada) to have lower population densities. Cities that were hotter and had more topographic variation tended to have fewer green spaces and those that were more humid tended to have more green spaces. Given that temperature and humidity are highly correlated with the geography of the Global South and Global North, it is difficult to know whether these effects are due to the direct effects of temperature and precipitation, for example, on the growth rate of vegetation and hence the transition of abandoned lots into green spaces, or are associated with historical, cultural and political differences that via various mechanisms correlate to climate. Our structural equation model explained only a small fraction of variation among cities in their cooling efficiency, which is to say the quality of their green space. Cooling efficiency was modestly influenced by background temperature and precipitation—the warmer a city, the greater the cooling efficiency in that city; conversely, the more humid a city the less the cooling efficiency of that city.
Our analyses suggested that the lower cooling adaptation capabilities of Global South cities can be explained by their lower quantity of green infrastructure and, to a much lesser extent, their weaker cooling efficiency (quality; Supplementary Fig. 2 ). These patterns appear to be in part structured by GDP, but are also associated with climatic conditions 39 , and other factors. A key question, unresolved by our work, is whether the climatic correlates of the size of green spaces in cities are due to the effects of climate per se or if they, instead, reflect correlates between contemporary climate and the social, cultural, and political histories of cities in the Global South 52 . Since urban planning has much inertia, especially in big cities, those choices might be correlated with climate because of the climatic correlates of political histories. It is also possible that these dynamics relate, in part, to the ways in which climate influences vegetation structure. However, this seems less likely given that under non-urban conditions vegetation cover (and hence cooling capacity) is normally positively correlated with mean annual temperature across the globe, opposite to our observed negative relationships for urban systems (Supplementary Fig. 9g ). Still, it is possible that increased temperatures in cities due to the urban heat island effects may lead to temperature-vegetation cover-cooling capacity relationships that differ from those in natural environments 53 , 54 . Indeed, a recent study found that climate warming will put urban forests at risk, and the risk is disproportionately higher in the Global South 55 .
Our model serves as a starting point for unraveling the mechanisms underlying global cooling inequality. We cannot rule out the possibility that other unconsidered factors correlated with the studied variables play important roles. We invite systematic studies incorporating detailed sociocultural and ecological variables to address this question across scales.
Potential of enhancing cooling and reducing inequality
Can we reduce the inequality in cooling capacity and benefits that we have discovered among the world’s largest cities? Nuanced assessments of the potential to improve cooling mitigation require comprehensive considerations of socioeconomic, cultural, and technological aspects of urban management and policy. It is likely that cities differ greatly in their capacity to implement cooling through green infrastructure, whether as a function of culture, governance, policy or some mix thereof. However, any practical attempts to achieve greater cooling will occur in the context of the realities of climate and existing land use. To understand these realities, we modeled the maximum additional cooling capacity that is possible in cities, given existing constraints. We assume that this capacity depends on the quality (cooling efficiency) and quantity of urban green infrastructure. Our approach provides a straightforward metric of the cooling that could be achieved if all parts of a city’s green infrastructure were to be enhanced systematically.
The positive outlook is that our analyses suggest a considerable potential of improving cooling capacity by optimizing urban green infrastructure. An obvious way is through increases in urban green infrastructure quantity. We employ an approach in which we consider each local climate zone 56 to have a maximum NDVI and cooling efficiency (see Methods). For a given local climate zone, the city with the largest NDVI values or cooling efficiency sets the regional upper bounds for urban green infrastructure quantities or quality that can be achieved. Notably, these maxima are below the maxima for forests or other non-urban spaces for the simple reason that, as currently imagined, cities must contain gray (non-green) spaces in the form of roads and buildings. In this context, we conduct a thought experiment. What if we could systematically increase NDVI of all grid cells in each city, per local climate zone type, to a level corresponding to the median NDVI of grid cells in that upper bound city while keeping cooling efficiency unchanged (see Methods). If we were able to achieve this goal, the cooling capacity of cities would increase by ~2.4 °C worldwide. The increase would be even greater, ~3.8°C, if the 90th percentile (within the reference maximum city) was reached (Fig. 5a ). The potential for cooling benefit to the average urban resident is similar to that of cooling capacity (Supplementary Fig. 10a ). There is also potential to reduce urban temperatures if we can enhance cooling efficiency. However, the benefits of increases in cooling efficiency are modest (~1.5 °C increases at the 90th percentile of regional upper bounds) when holding urban green infrastructure quantity constant. In theory, if we could maximize both quantity and cooling efficiency of urban green infrastructure (to 90th percentiles of their regional upper bounds respectively), we would yield increases in cooling capacity and benefit up to ~10 °C, much higher than enhancing green space area or cooling efficiency alone (Fig. 5a , Supplementary Fig. 10a ). Notably, such co-maximization of green space area and cooling efficiency would substantially reduce global inequality to Gini <0.1 (Fig. 5b , Supplementary Fig. 10b ). Our analyses thus provide an important suggestion that enhancing both green space quantity and quality can yield a synergistic effect leading to much larger gains than any single aspect alone.
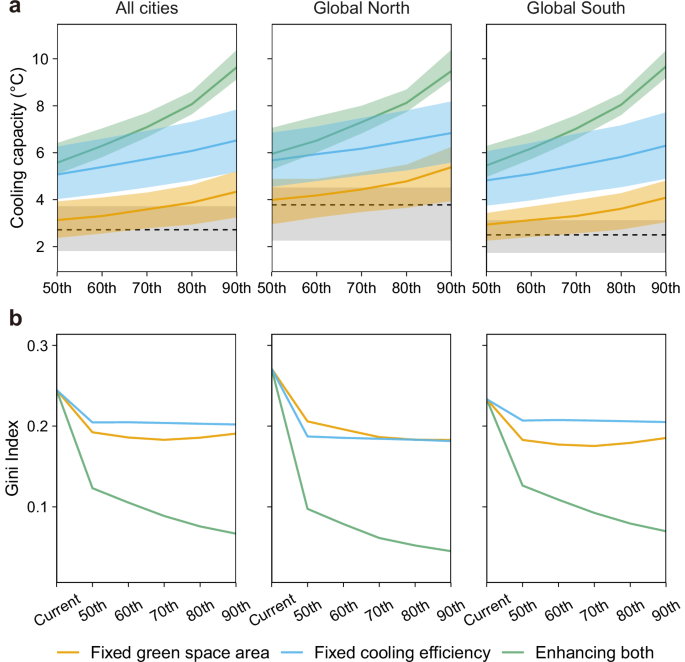
a The potential of enhancing cooling capacity via either enhancing urban green infrastructure quality (i.e., cooling efficiency) while holding quantity (i.e., green space area) fixed (yellow), or enhancing quantity while holding quality fixed (blue) is much lower than that of enhancing both quantity and quality (green). The x-axis indicates the targets of enhancing urban green infrastructure quantity and/or quality relative to the 50–90th percentiles of NDVI or cooling efficiency, see Methods). The dashed horizontal lines indicate the median cooling capacity of current cities. Data are presented as median values with the colored bands corresponding to 25–75th percentiles. b The potential of reducing cooling capacity inequality is also higher when enhancing both urban green infrastructure quantity and quality. The Gini index weighted by population density is used to measure inequality. Similar results were found for cooling benefit (Supplementary Fig. 10 ).
Different estimates of cooling capacity potential may be reached based on varying estimates and assumptions regarding the maximum possible quantity and quality of urban green infrastructure. There is no single, simple way to make these estimates, especially considering the huge between-city differences in society, culture, and structure across the globe. Our example case (above) begins from the upper bound city’s median NDVI, taking into account different local climate zone types and background climate regions (regional upper bounds). This is based on the assumption that for cities within the same climate regions, their average green space quantity may serve as an attainable target. Still, urban planning is often made at the level of individual cities, often only implemented to a limited extent and made with limited consideration of cities in other regions and countries. A potentially more realistic reference may be taken from the existing green infrastructure (again, per local climate zone type) within each particular city itself (see Methods): if a city’s sparsely vegetated areas was systematically elevated to the levels of 50–90th percentiles of NDVI within their corresponding local climate zones within the city, cooling capacity would still increase, but only by 0.5–1.5 °C and with only slightly reduced inequalities among cities (Supplementary Fig. 11 ). This highlights that ambitious policies, inspired by the greener cities worldwide, are necessary to realize the large cooling potential in urban green infrastructure.
In summary, our results demonstrate clear inequality in the extent to which urban green infrastructure cools cities and their denizens between the Global North and South. Much attention has been paid to the global inequality of indoor heat adaptation arising from the inequality of resources (e.g., less affordable air conditioning and more frequent power shortages in the Global South) 36 , 57 , 58 , 59 . Our results suggest that the inequality in outdoor adaptation is particularly concerning, especially as urban populations in the Global South are growing rapidly and are likely to face the most severe future temperature extremes 60 .
Previous studies have been focusing on characterizing urban heat island effects, urban vegetation patterns, resident exposure, and cooling effects in particular cities 26 , 28 , 34 , 61 , regions 22 , 25 , 62 , or continents 32 , 44 , 63 . Recent studies start looking at global patterns with respect to cooling efficiency or green space exposure 35 , 45 , 64 , 65 . Our approach is one drawn from the fields of large-scale ecology and macroecology. This approach is complementary to and, indeed, can, in the future, be combined with (1) mechanism driven biophysical models 66 , 67 to predict the influence of the composition and climate of green spaces on their cooling efficiency, (2) social theory aimed at understanding the factors that govern the amount of green space in cities as well as the disparity among cities 68 , (3) economic models of the effects of policy changes on the amount of greenspace and even (4) artist-driven projects that seek to understand the ways in which we might reimagine future cities 69 . Our simple explanatory model is, ultimately, one lens on a complex, global phenomenon.
Our results convey some positive outlook in that there is considerable potential to strengthen the cooling capability of cities and to reduce inequalities in cooling capacities at the same time. Realizing this nature-based solution, however, will be challenging. First, enhancing urban green infrastructure requires massive investments, which are more difficult to achieve in Global South cities. Second, it also requires smart planning strategies and advanced urban design and greening technologies 37 , 70 , 71 , 72 . Spatial planning of urban green spaces needs to consider not only the cooling amelioration effect, but also their multifunctional aspects that involve multiple ecosystem services, mental health benefits, accessibility, and security 73 . In theory, a city can maximize its cooling while also maximizing density through the combination of high-density living, ground-level green spaces, and vertical and rooftop gardens (or even forests). In practice, the current cities with the most green spaces tend to be lower-density cities 74 (Supplementary Fig. 12 ). Still, innovation and implementation of new technologies that allow green spaces and high-density living to be combined have the potential to reduce or disconnect the negative relationship between green space area and population density 71 , 75 . However, this development has yet to be realized. Another dimension of green spaces that deserves more attention is the geography of green spaces relative to where people are concentrated within cities. A critical question is how best should we distribute green spaces within cities to maximize cooling efficiency 76 and minimize within-city cooling inequality towards social equity 77 ? Last but not least, it is crucial to design and manage urban green spaces to be as resilient as possible to future climate stress 78 . For many cities, green infrastructure is likely to remain the primary means people will have to rely on to mitigate the escalating urban outdoor heat stress in the coming decades 79 .
We used the world population data from the World’s Cities in 2018 Data Booklet 80 to select 502 major cities with population over 1 million people (see Supplementary Data 1 for the complete list of the studied cities). Cities are divided into the Global North and Global South based on the Human Development Index (HDI) from the Human Development Report 2019 81 . For each selected city, we used the 2018 Global Artificial Impervious Area (GAIA) data at 30 m resolution 82 to determine its geographic extent. The derived urban boundary polygons thus encompass a majority of the built-up areas and urban residents. In using this approach, rather than urban administrative boundaries, we can focus on the relatively densely populated areas where cooling mitigation is most needed, and exclude areas dominated by (semi) natural landscapes that may bias the subsequent quantifications of the cooling effect. Our analyses on the cooling effect were conducted at the 100 m spatial resolution using Landsat data and WorldPop Global Project Population Data of 2018 83 . In order to test for the robustness of the results to coarser spatial scales, we also repeated the analyses at 1 km resolution using MODIS data, which have been extensively used for quantifying urban heat island effects and cooling mitigation 44 , 45 , 51 . We discarded the five cities with sizes <30 km 2 as they were too small for us to estimate their cooling efficiency based on linear regression (see section below for details). We combined closely located cities that form contiguous urban areas or urban agglomerations, if their urban boundary polygons from GAIA merged (e.g., Phoenix and Mesa in the United States were combined). Our approach yielded 468 polygons, each representing a major urbanized area that were the basis for all subsequent analyses. Because large water bodies can exert substantial and confounding cooling effects, we excluded permanent water bodies including lakes, reservoirs, rivers, and oceans using the Copernicus Global Land Service (CGLS) Land Cover data for 2018 at 10 m resolution 84 .
Quantifying the cooling effect
As a first step, we calculated cooling efficiency for each studied city within the GAIA-derived urban boundary. Cooling efficiency quantifies the extent to which a given area of green spaces in a city can reduce temperatures. It is a measure of the effectiveness (quality) of urban green spaces in terms of heat amelioration. Cooling efficiency is typically measured by calculating the slope of the relationship between remotely-sensed land surface temperature (LST) and vegetation cover through ordinary least square regression 42 , 44 , 45 . It is known that cooling efficiency varies between cities. Influencing factors might include background climate 29 , species composition 30 , 85 , landscape configuration 28 , topography 86 , proximity to large water bodies 33 , 87 , urban morphology 88 , and city management practices 31 . However, the mechanism underlying the global pattern of cooling efficiency remains unclear.
We used Landsat satellite data provided by the United States Geological Survey (USGS) to calculate the cooling efficiency of each studied city. We used the cloud-free Landsat 8 Level 2 LST and NDVI data. For each city we calculated the mean LST in each month of 2018 to identify the hottest month, and then derived the hottest month LST; we used the cloud-free Landsat 8 data to calculate the mean NDVI for the hottest month correspondingly.
We quantified cooling efficiency for different local climate zones 56 separately for each city, to account for within-city variability of thermal environments. To this end, we used the Copernicus Global Land Service data (CGLS) 84 and Global Human Settlement Layers (GHSL) Built-up height data 89 of 2018 at the 100 m resolution to identify five types of local climate zones: non-tree vegetation (shrubs, herbaceous vegetation, and cultivated vegetation according to the CGLS classification system), low-rise buildings (built up and bare according to the CGLS classification system, with building heights ≤10 m according to the GHSL data), medium-high-rise buildings (built up and bare areas with building heights >10 m), open tree cover (open forest with tree cover 15–70% according to the CGLS system), and closed tree cover (closed forest with tree cover >70%).
For each local climate zone type in each city, we constructed a regression model with NDVI as the predictor variable and LST as the response variable (using the ordinary least square method). We took into account the potential confounding factors including topographic elevation (derived from MERIT DEM dataset 90 ), building height (derived from the GHSL dataset 89 ), and distance to water bodies (derived from the GSHHG dataset 91 ), the model thus became: LST ~ NDVI + topography + building height + distance to water. Cooling efficiency was calculated as the absolute value of the regression coefficient of NDVI, after correcting for those confounding factors. To account for the multi-collinearity issue, we conducted variable selection based on the variance inflation factor (VIF) to achieve VIF < 5. Before the analysis, we discarded low-quality Landsat pixels, and filtered out the pixels with NDVI < 0 (normally less than 1% in a single city). Cooling efficiency is known to be influenced by within-city heterogeneity 92 , 93 , and, as a result, might sometimes better fit non-linear relationships at local scales 65 , 76 . However, our central aim is to assess global cooling inequality based on generalized relationships that fit the majority of global cities. Previous studies have shown that linear relationships can do this job 42 , 44 , 45 , therefore, here we used linear models to assess cooling efficiency.
As a second step, we calculated the cooling capacity of each city. Cooling capacity is a positive function of the magnitude of cooling efficiency and the proportional area of green spaces in a city and is calculated based on NDVI and the derived cooling efficiency (Eq. 1 , Supplementary Fig. 13 ):
where CC lcz and CE lcz are the cooling capacity and cooling efficiency for a given local climate zone type in a city, respectively; NDVI i is the mean NDVI for 100-m grid cell i ; NDVI min is the minimum NDVI across the city; and n is the total number of grid cells within the local climate zone. Local cooling capacity for each grid cell i (Fig. 1 , Supplementary Fig. 7 ) can be derived in this way as well (Supplementary Fig. 13 ). For a particular city, cooling capacity may be dependent on the spatial configuration of its land use/cover 28 , 94 , but here we condensed cooling capacity to city average (Eq. 2 ), thus did not take into account these local-scale factors.
where CC is the average cooling capacity of a city; n lcz is the number of grid cells of the local climate zone; m is the total number of grid cells within the whole city.
As a third step, we calculated the cooling benefit realized by an average urban resident (cooling benefit in short) in each city. Cooling benefit depends not only on the cooling capacity of a city, but also on where people live within a city relative to greener or grayer areas of the city. For example, cooling benefits in a city might be low even if the cooling capacity is high if the green parts and the dense-population parts of a city are inversely correlated. Here, we are calculating these averages while aware that in any particular city the exposure of a particular person will depend on the distribution of green spaces in a city, and the occupation, movement trajectories of a person, etc. On the scale of a city, we calculated cooling benefit following a previous study 35 , that is, simply adding a weight term of population size per 100-m grid cell into cooling capacity in Eq. ( 1 ):
Where CB lcz is the cooling benefit of a given local climate zone type in a specific city, pop i is the number of people within grid cell i , \(\overline{{pop}}\) is the mean population of the city.
Where CB is the average cooling benefit of a city. The population data were obtained from the 100-m resolution WorldPop Global Project Population Data of 2018 83 . Local cooling benefit for a given grid cell i can be calculated in a similar way, i.e., local cooling capacity multiplied by a weight term of local population density relative to mean population density. Local cooling benefits were mapped for example cities for the purpose of illustrating the effect of population spatial distribution (Fig. 1 , Supplementary Fig. 7 ), but their patterns were not examined here.
Based on the aforementioned three key variables quantified at 100 m grid cells, we conducted multivariate analyses to examine if and to what extent cooling efficiency and cooling benefit are shaped by essential natural and socioeconomic factors, including background climate (mean annual temperature from ECMWF ERA5 dataset 95 and precipitation from TerraClimate dataset 96 ), topography (elevation range 90 ), and GDP per capita 97 , with city size (geographic extent) corrected for. We did not include humidity because it is strongly correlated with temperature and precipitation, causing serious multi-collinearity problems. We used piecewise structural equation modeling to test the direct effects of these factors and indirect effects via influencing cooling efficiency and vegetation cover (Fig. 4c , Supplementary Fig. 8c ). To account for the potential influence of spatial autocorrelation, we used spatially autoregressive models (SAR) to test for the robustness of the observed effects of natural and socioeconomic factors on cooling capacity and benefit (Supplementary Fig. 14 ).
Testing for robustness
We conducted the following additional analyses to test for robustness. We obtained consistent results from these robustness analyses.
(1) We looked at the mean hottest-month LST and NDVI within 3 years (2017-2019) to check the consistency between the results based on relatively short (1 year) vs. long (3-year average) time periods (Supplementary Fig. 15 ).
(2) We carried out the approach at a coarser spatial scale of 1 km, using MODIS-derived NDVI and LST, as well as the population data 83 in the hottest month of 2018. In line with our finer-scale analysis of Landsat data, we selected the hottest month and excluded low-quality grids affected by cloud cover and water bodies 98 (water cover > 20% in 1 × 1 km 2 grid cells) of MODIS LST, and calculated the mean NDVI for the hottest month. We ultimately obtained 441 cities (or urban agglomerations) for analysis. At the 1 km resolution, some local climate zone types would yield insufficient samples for constructing cooling efficiency models. Therefore, instead of identifying local climate zone explicitly, we took an indirect approach to account for local climate confounding factors, that is, we constructed a multiple regression model for a whole city incorporating the hottest-month local temperature 95 , precipitation 96 , and humidity (based on NASA FLDAS dataset 99 ), albedo (derived from the MODIS MCD43A3 product 100 ), aerosol loading (derived from the MODIS MCD19A2 product 101 ), wind speed (based on TerraClimate dataset 96 ), topography elevation 90 , distance to water 91 , urban morphology (building height 102 ), and human activity intensity (VIIRS nighttime light data as a proxy indicator 103 ). We used the absolute value of the linear regression coefficient of NDVI as the cooling efficiency of the whole city (model: LST ~ NDVI + temperature + precipitation + humidity + distance to water + topography + building height + albedo + aerosol + wind speed + nighttime light), and calculated cooling capacity and cooling benefit based on the same method. Variable selection was conducted using the criterion of VIF < 5.
Our results indicated that MODIS-based cooling capacity and cooling benefit are significantly correlated with the Landsat-based counterparts (Supplementary Fig. 16 ); importantly, the gap between the Global South and North cities is around two-fold, close to the result from the Landsat-based result (Supplementary Fig. 17 ).
(3) For the calculation of cooling benefit, we considered different spatial scales of human accessibility to green spaces: assuming the population in each 100 × 100 m 2 grid cell could access to green spaces within neighborhoods of certain extents, we calculated cooling benefit by replacing NDVI i in Eq. ( 3 ) with mean NDVI within the 300 × 300 m 2 and 500 × 500 m 2 extents centered at the focal grid cell (Supplementary Fig. 18 ).
(4) Considering cities may vary in minimum NDVI, we assessed if this variation could affect resulting cooling capacity patterns. To this end, we calculated the cooling capacity for each studied city using NDVI = 0 as the reference (i.e., using NDVI = 0 instead of minimum NDVI in Supplementary Fig. 13b ), and correlated it with that using minimum NDVI as the reference (Supplementary Fig. 19 ).
Quantifying between-city inequality
Inequalities in access to the benefits of green spaces in cities exist within cities, as is increasingly well-documented 104 . Here, we focus instead on the inequalities among cities. We used the Gini coefficient to measure the inequality in cooling capacity and cooling benefit between all studied cities across the globe as well as between Global North or South cities. We calculated Gini using the population-density weighted method (Fig. 5b ), as well as the unweighted and population-size weighted methods (Supplementary Fig. 20 ).
Estimating the potential for more effective and equal cooling amelioration
We estimated the potential of enhancing cooling amelioration based on the assumptions that urban green space quality (cooling efficiency) and quantity (NDVI) can be increased to different levels, and that relative spatial distributions of green spaces and population can be idealized (so that their spatial matches can maximize cooling benefit). We assumed that macro-climate conditions act as the constraints of vegetation cover and cooling efficiency. We calculated the 50th, 60th, 70th, 80th, and 90th percentiles of NDVI within each type of local climate zone of each city. For a given local climate zone type, we obtained the city with the highest NDVI per percentile value as the regional upper bounds of urban green infrastructure quantity. The regional upper bounds of cooling efficiency are derived in a similar way. For each local climate zone in a city, we generated a potential NDVI distribution where all grid cells reach the regional upper bound values for the 50th, 60th, 70th, 80th, or 90th percentile of urban green space quantity or quality, respectively. NDVI values below these percentiles were increased, whereas those above these percentiles remained unchanged. The potential estimates are essentially dependent on the references, i.e., the optimal cooling efficiency and NDVI that a given city can reach. However, such references are obviously difficult to determine, because complex natural and socioeconomic conditions could play important roles in determining those cooling optima, and the dominant factors are unknown at a global scale. We employed the simplifying assumption that background climate could act as an essential constraint according to our results. We therefore used the Köppen climate classification system 105 to determine the reference separately in each climate region (tropical, arid, temperate, and continental climate regions were involved for all studied cities).
We calculated potential cooling capacity and cooling benefit based on these potential NDVI maps (Fixed cooling efficiency in Fig. 5 ). We then calculated the potentials if cooling efficiency of each city can be enhanced to 50–90th percentile across all urban local climate zones within the corresponding biogeographic region (Fixed green space area in Fig. 5 ). We also calculated the potentials if both NDVI and cooling efficiency were enhanced (Enhancing both in Fig. 5) to a certain corresponding level (i.e., i th percentile NDVI + i th percentile cooling efficiency). We examined if there are additional effects of idealizing relative spatial distributions of urban green spaces and humans on cooling benefits. To this end, the pixel values of NDVI or population amount remained unchanged, but their one-to-one correspondences were based on their ranking: the largest population corresponds to the highest NDVI, and so forth. Under each scenario, we calculated cooling capacity and cooling benefit for each city, and the between-city inequality was measured by the Gini coefficient.
We used the Google Earth Engine to process the spatial data. The statistical analyses were conducted using R v4.3.3 106 , with car v3.1-2 107 , piecewiseSEM v2.1.2 108 , and ineq v0.2-13 109 packages. The global maps of cooling were created using the ArcGIS v10.3 software.
Reporting summary
Further information on research design is available in the Nature Portfolio Reporting Summary linked to this article.
Data availability
City population statistics data is collected from the Population Division of the Department of Economic and Social Affairs of the United Nations ( https://www.un.org/development/desa/pd/content/worlds-cities-2018-data-booklet ). Global North-South division is based on Human Development Report 2019 which from United Nations Development Programme ( https://hdr.undp.org/content/human-development-report-2019 ). Global urban boundaries from GAIA data are available from Star Cloud Data Service Platform ( https://data-starcloud.pcl.ac.cn/resource/14 ) . Global water data is derived from 2018 Copernicus Global Land Service (CGLS 100-m) data ( https://developers.google.com/earth-engine/datasets/catalog/COPERNICUS_Landcover_100m_Proba-V-C3_Global ), European Space Agency (ESA) WorldCover 10 m 2020 product ( https://developers.google.com/earth-engine/datasets/catalog/ESA_WorldCover_v100 ), and GSHHG (A Global Self-consistent, Hierarchical, High-resolution Geography Database) at https://www.soest.hawaii.edu/pwessel/gshhg/ . Landsat 8 LST and NDVI data with 30 m resolution are available at https://developers.google.com/earth-engine/datasets/catalog/LANDSAT_LC08_C02_T1_L2 . Land surface temperature (LST) data with 1 km from MODIS Aqua product (MYD11A1) is available at https://developers.google.com/earth-engine/datasets/catalog/MODIS_061_MYD11A1 . NDVI (1 km) dataset from MYD13A2 is available at https://developers.google.com/earth-engine/datasets/catalog/MODIS_061_MYD13A2 . Population data (100 m) is derived from WorldPop ( https://developers.google.com/earth-engine/datasets/catalog/WorldPop_GP_100m_pop ). Local climate zones are also based on 2018 CGLS data ( https://developers.google.com/earth-engine/datasets/catalog/COPERNICUS_Landcover_100m_Proba-V-C3_Global ), and built-up height data is available from Global Human Settlement Layers (GHSL, 100 m) ( https://developers.google.com/earth-engine/datasets/catalog/JRC_GHSL_P2023A_GHS_BUILT_H ). Temperature data is calculated from ERA5-Land Monthly Aggregated dataset ( https://developers.google.com/earth-engine/datasets/catalog/ECMWF_ERA5_LAND_MONTHLY_AGGR ). Precipitation and wind data are calculated from TerraClimate (Monthly Climate and Climatic Water Balance for Global Terrestrial Surfaces, University of Idaho) ( https://developers.google.com/earth-engine/datasets/catalog/IDAHO_EPSCOR_TERRACLIMATE ). Humidity data is calculated from Famine Early Warning Systems Network (FEWS NET) Land Data Assimilation System ( https://developers.google.com/earth-engine/datasets/catalog/NASA_FLDAS_NOAH01_C_GL_M_V001 ). Topography data from MERIT DEM (Multi-Error-Removed Improved-Terrain DEM) product is available at https://developers.google.com/earth-engine/datasets/catalog/MERIT_DEM_v1_0_3 . GDP from Gross Domestic Product and Human Development Index dataset is available at https://doi.org/10.5061/dryad.dk1j0 . VIIRS nighttime light data is available at https://developers.google.com/earth-engine/datasets/catalog/NOAA_VIIRS_DNB_MONTHLY_V1_VCMSLCFG . City building volume data from Global 3D Building Structure (1 km) is available at https://doi.org/10.34894/4QAGYL . Albedo data is derived from the MODIS MCD43A3 product ( https://developers.google.com/earth-engine/datasets/catalog/MODIS_061_MCD43A3 ), and aerosol data is derived from the MODIS MCD19A2 product ( https://developers.google.com/earth-engine/datasets/catalog/MODIS_061_MCD19A2_GRANULES ). All data used for generating the results are publicly available at https://doi.org/10.6084/m9.figshare.26340592.v1 .
Code availability
The codes used for data collection and analyses are publicly available at https://doi.org/10.6084/m9.figshare.26340592.v1 .
Dosio, A., Mentaschi, L., Fischer, E. M. & Wyser, K. Extreme heat waves under 1.5 °C and 2 °C global warming. Environ. Res. Lett. 13 , 054006 (2018).
Article ADS Google Scholar
Suarez-Gutierrez, L., Müller, W. A., Li, C. & Marotzke, J. Hotspots of extreme heat under global warming. Clim. Dyn. 55 , 429–447 (2020).
Article Google Scholar
Guo, Y. et al. Global variation in the effects of ambient temperature on mortality: a systematic evaluation. Epidemiology 25 , 781–789 (2014).
Article PubMed PubMed Central Google Scholar
Mora, C. et al. Global risk of deadly heat. Nat. Clim. Chang. 7 , 501–506 (2017).
Ebi, K. L. et al. Hot weather and heat extremes: health risks. Lancet 398 , 698–708 (2021).
Article PubMed Google Scholar
Lüthi, S. et al. Rapid increase in the risk of heat-related mortality. Nat. Commun. 14 , 4894 (2023).
Article ADS PubMed PubMed Central Google Scholar
United Nations Department of Economic Social Affairs, Population Division. in World Population Prospects 2022: Summary of Results (United Nations Fund for Population Activities, 2022).
Sachindra, D., Ng, A., Muthukumaran, S. & Perera, B. Impact of climate change on urban heat island effect and extreme temperatures: a case‐study. Q. J. R. Meteorol. Soc. 142 , 172–186 (2016).
Guo, L. et al. Evaluating contributions of urbanization and global climate change to urban land surface temperature change: a case study in Lagos, Nigeria. Sci. Rep. 12 , 14168 (2022).
Article ADS CAS PubMed PubMed Central Google Scholar
Liu, Z. et al. Surface warming in global cities is substantially more rapid than in rural background areas. Commun. Earth Environ. 3 , 219 (2022).
Mentaschi, L. et al. Global long-term mapping of surface temperature shows intensified intra-city urban heat island extremes. Glob. Environ. Change 72 , 102441 (2022).
Asseng, S., Spänkuch, D., Hernandez-Ochoa, I. M. & Laporta, J. The upper temperature thresholds of life. Lancet Planet. Health 5 , e378–e385 (2021).
Zander, K. K., Botzen, W. J., Oppermann, E., Kjellstrom, T. & Garnett, S. T. Heat stress causes substantial labour productivity loss in Australia. Nat. Clim. Chang. 5 , 647–651 (2015).
Flouris, A. D. et al. Workers’ health and productivity under occupational heat strain: a systematic review and meta-analysis. Lancet Planet. Health 2 , e521–e531 (2018).
Xu, C., Kohler, T. A., Lenton, T. M., Svenning, J.-C. & Scheffer, M. Future of the human climate niche. Proc. Natl Acad. Sci. USA 117 , 11350–11355 (2020).
Lenton, T. M. et al. Quantifying the human cost of global warming. Nat. Sustain. 6 , 1237–1247 (2023).
Harrington, L. J. et al. Poorest countries experience earlier anthropogenic emergence of daily temperature extremes. Environ. Res. Lett. 11 , 055007 (2016).
Bathiany, S., Dakos, V., Scheffer, M. & Lenton, T. M. Climate models predict increasing temperature variability in poor countries. Sci. Adv. 4 , eaar5809 (2018).
Alizadeh, M. R. et al. Increasing heat‐stress inequality in a warming climate. Earth Future 10 , e2021EF002488 (2022).
Tuholske, C. et al. Global urban population exposure to extreme heat. Proc. Natl Acad. Sci. USA 118 , e2024792118 (2021).
Article CAS PubMed PubMed Central Google Scholar
Manoli, G. et al. Magnitude of urban heat islands largely explained by climate and population. Nature 573 , 55–60 (2019).
Article ADS CAS PubMed Google Scholar
Wang, J. et al. Anthropogenic emissions and urbanization increase risk of compound hot extremes in cities. Nat. Clim. Chang. 11 , 1084–1089 (2021).
Article ADS CAS Google Scholar
Bowler, D. E., Buyung-Ali, L., Knight, T. M. & Pullin, A. S. Urban greening to cool towns and cities: a systematic review of the empirical evidence. Landsc. Urban Plan. 97 , 147–155 (2010).
Armson, D., Stringer, P. & Ennos, A. The effect of tree shade and grass on surface and globe temperatures in an urban area. Urban For. Urban Green. 11 , 245–255 (2012).
Wang, C., Wang, Z. H. & Yang, J. Cooling effect of urban trees on the built environment of contiguous United States. Earth Future 6 , 1066–1081 (2018).
Pataki, D. E., McCarthy, H. R., Litvak, E. & Pincetl, S. Transpiration of urban forests in the Los Angeles metropolitan area. Ecol. Appl. 21 , 661–677 (2011).
Konarska, J. et al. Transpiration of urban trees and its cooling effect in a high latitude city. Int. J. Biometeorol. 60 , 159–172 (2016).
Article ADS PubMed Google Scholar
Li, X., Zhou, W., Ouyang, Z., Xu, W. & Zheng, H. Spatial pattern of greenspace affects land surface temperature: evidence from the heavily urbanized Beijing metropolitan area, China. Landsc. Ecol. 27 , 887–898 (2012).
Yu, Z., Xu, S., Zhang, Y., Jørgensen, G. & Vejre, H. Strong contributions of local background climate to the cooling effect of urban green vegetation. Sci. Rep. 8 , 6798 (2018).
Richards, D. R., Fung, T. K., Belcher, R. & Edwards, P. J. Differential air temperature cooling performance of urban vegetation types in the tropics. Urban For. Urban Green. 50 , 126651 (2020).
Winbourne, J. B. et al. Tree transpiration and urban temperatures: current understanding, implications, and future research directions. BioScience 70 , 576–588 (2020).
Schwaab, J. et al. The role of urban trees in reducing land surface temperatures in European cities. Nat. Commun. 12 , 6763 (2021).
Vo, T. T. & Hu, L. Diurnal evolution of urban tree temperature at a city scale. Sci. Rep. 11 , 10491 (2021).
Wang, J. et al. Comparing relationships between urban heat exposure, ecological structure, and socio-economic patterns in Beijing and New York City. Landsc. Urban Plan. 235 , 104750 (2023).
Chen, B. et al. Contrasting inequality in human exposure to greenspace between cities of Global North and Global South. Nat. Commun. 13 , 4636 (2022).
Pavanello, F. et al. Air-conditioning and the adaptation cooling deficit in emerging economies. Nat. Commun. 12 , 6460 (2021).
Turner, V. K., Middel, A. & Vanos, J. K. Shade is an essential solution for hotter cities. Nature 619 , 694–697 (2023).
Hope, D. et al. Socioeconomics drive urban plant diversity. Proc. Natl Acad. Sci. USA 100 , 8788–8792 (2003).
Leong, M., Dunn, R. R. & Trautwein, M. D. Biodiversity and socioeconomics in the city: a review of the luxury effect. Biol. Lett. 14 , 20180082 (2018).
Schwarz, K. et al. Trees grow on money: urban tree canopy cover and environmental justice. PloS ONE 10 , e0122051 (2015).
Chakraborty, T., Hsu, A., Manya, D. & Sheriff, G. Disproportionately higher exposure to urban heat in lower-income neighborhoods: a multi-city perspective. Environ. Res. Lett. 14 , 105003 (2019).
Wang, J. et al. Significant effects of ecological context on urban trees’ cooling efficiency. ISPRS J. Photogramm. Remote Sens. 159 , 78–89 (2020).
Marando, F. et al. Urban heat island mitigation by green infrastructure in European Functional Urban Areas. Sust. Cities Soc. 77 , 103564 (2022).
Cheng, X., Peng, J., Dong, J., Liu, Y. & Wang, Y. Non-linear effects of meteorological variables on cooling efficiency of African urban trees. Environ. Int. 169 , 107489 (2022).
Yang, Q. et al. Global assessment of urban trees’ cooling efficiency based on satellite observations. Environ. Res. Lett. 17 , 034029 (2022).
Yin, Y., He, L., Wennberg, P. O. & Frankenberg, C. Unequal exposure to heatwaves in Los Angeles: Impact of uneven green spaces. Sci. Adv. 9 , eade8501 (2023).
Fantom N., Serajuddin U. The World Bank’s Classification of Countries by Income (The World Bank, 2016).
Iungman, T. et al. Cooling cities through urban green infrastructure: a health impact assessment of European cities. Lancet 401 , 577–589 (2023).
He, C. et al. The inequality labor loss risk from future urban warming and adaptation strategies. Nat. Commun. 13 , 3847 (2022).
Kii, M. Projecting future populations of urban agglomerations around the world and through the 21st century. npj Urban Sustain 1 , 10 (2021).
Paschalis, A., Chakraborty, T., Fatichi, S., Meili, N. & Manoli, G. Urban forests as main regulator of the evaporative cooling effect in cities. AGU Adv. 2 , e2020AV000303 (2021).
Hunte, N., Roopsind, A., Ansari, A. A. & Caughlin, T. T. Colonial history impacts urban tree species distribution in a tropical city. Urban For. Urban Green. 41 , 313–322 (2019).
Kabano, P., Harris, A. & Lindley, S. Sensitivity of canopy phenology to local urban environmental characteristics in a tropical city. Ecosystems 24 , 1110–1124 (2021).
Frank, S. D. & Backe, K. M. Effects of urban heat islands on temperate forest trees and arthropods. Curr. Rep. 9 , 48–57 (2023).
Esperon-Rodriguez, M. et al. Climate change increases global risk to urban forests. Nat. Clim. Chang. 12 , 950–955 (2022).
Stewart, I. D. & Oke, T. R. Local climate zones for urban temperature studies. Bull. Am. Meteorol. Soc. 93 , 1879–1900 (2012).
Biardeau, L. T., Davis, L. W., Gertler, P. & Wolfram, C. Heat exposure and global air conditioning. Nat. Sustain. 3 , 25–28 (2020).
Davis, L., Gertler, P., Jarvis, S. & Wolfram, C. Air conditioning and global inequality. Glob. Environ. Change 69 , 102299 (2021).
Colelli, F. P., Wing, I. S. & Cian, E. D. Air-conditioning adoption and electricity demand highlight climate change mitigation–adaptation tradeoffs. Sci. Rep. 13 , 4413 (2023).
Sun, L., Chen, J., Li, Q. & Huang, D. Dramatic uneven urbanization of large cities throughout the world in recent decades. Nat. Commun. 11 , 5366 (2020).
Liu, D., Kwan, M.-P. & Kan, Z. Analysis of urban green space accessibility and distribution inequity in the City of Chicago. Urban For. Urban Green. 59 , 127029 (2021).
Hsu, A., Sheriff, G., Chakraborty, T. & Manya, D. Disproportionate exposure to urban heat island intensity across major US cities. Nat. Commun. 12 , 2721 (2021).
Zhao, L., Lee, X., Smith, R. B. & Oleson, K. Strong contributions of local background climate to urban heat islands. Nature 511 , 216–219 (2014).
Wu, S., Chen, B., Webster, C., Xu, B. & Gong, P. Improved human greenspace exposure equality during 21st century urbanization. Nat. Commun. 14 , 6460 (2023).
Zhao, J., Zhao, X., Wu, D., Meili, N. & Fatichi, S. Satellite-based evidence highlights a considerable increase of urban tree cooling benefits from 2000 to 2015. Glob. Chang. Biol. 29 , 3085–3097 (2023).
Article CAS PubMed Google Scholar
Nice, K. A., Coutts, A. M. & Tapper, N. J. Development of the VTUF-3D v1. 0 urban micro-climate model to support assessment of urban vegetation influences on human thermal comfort. Urban Clim. 24 , 1052–1076 (2018).
Meili, N. et al. An urban ecohydrological model to quantify the effect of vegetation on urban climate and hydrology (UT&C v1. 0). Geosci. Model Dev. 13 , 335–362 (2020).
Nesbitt, L., Meitner, M. J., Sheppard, S. R. & Girling, C. The dimensions of urban green equity: a framework for analysis. Urban For. Urban Green. 34 , 240–248 (2018).
Hedblom, M., Prévot, A.-C. & Grégoire, A. Science fiction blockbuster movies—a problem or a path to urban greenery? Urban For. Urban Green. 74 , 127661 (2022).
Norton, B. A. et al. Planning for cooler cities: a framework to prioritise green infrastructure to mitigate high temperatures in urban landscapes. Landsc. Urban Plan 134 , 127–138 (2015).
Medl, A., Stangl, R. & Florineth, F. Vertical greening systems—a review on recent technologies and research advancement. Build. Environ. 125 , 227–239 (2017).
Chen, B., Lin, C., Gong, P. & An, J. Optimize urban shade using digital twins of cities. Nature 622 , 242–242 (2023).
Pamukcu-Albers, P. et al. Building green infrastructure to enhance urban resilience to climate change and pandemics. Landsc. Ecol. 36 , 665–673 (2021).
Haaland, C. & van Den Bosch, C. K. Challenges and strategies for urban green-space planning in cities undergoing densification: a review. Urban For. Urban Green. 14 , 760–771 (2015).
Shafique, M., Kim, R. & Rafiq, M. Green roof benefits, opportunities and challenges—a review. Renew. Sust. Energ. Rev. 90 , 757–773 (2018).
Wang, J., Zhou, W. & Jiao, M. Location matters: planting urban trees in the right places improves cooling. Front. Ecol. Environ. 20 , 147–151 (2022).
Lan, T., Liu, Y., Huang, G., Corcoran, J. & Peng, J. Urban green space and cooling services: opposing changes of integrated accessibility and social equity along with urbanization. Sust. Cities Soc. 84 , 104005 (2022).
Wood, S. & Dupras, J. Increasing functional diversity of the urban canopy for climate resilience: Potential tradeoffs with ecosystem services? Urban For. Urban Green. 58 , 126972 (2021).
Wong, N. H., Tan, C. L., Kolokotsa, D. D. & Takebayashi, H. Greenery as a mitigation and adaptation strategy to urban heat. Nat. Rev. Earth Environ. 2 , 166–181 (2021).
United Nations. Department of economic and social affairs, population division. in The World’s Cities in 2018—Data Booklet (UN, 2018).
United Nations Development Programme (UNDP). Human Development Report 2019: Beyond Income, Beyond Averages, Beyond Today: Inequalities in Human Development in the 21st Century (United Nations Development Programme (UNDP), 2019)
Li, X. et al. Mapping global urban boundaries from the global artificial impervious area (GAIA) data. Environ. Res. Lett. 15 , 094044 (2020).
Stevens, F. R., Gaughan, A. E., Linard, C. & Tatem, A. J. Disaggregating census data for population mapping using random forests with remotely-sensed and ancillary data. PloS ONE 10 , e0107042 (2015).
Buchhorn, M. et al. Copernicus global land cover layers—collection 2. Remote Sens 12 , 1044 (2020).
Gillerot, L. et al. Forest structure and composition alleviate human thermal stress. Glob. Change Biol. 28 , 7340–7352 (2022).
Article CAS Google Scholar
Hamada, S., Tanaka, T. & Ohta, T. Impacts of land use and topography on the cooling effect of green areas on surrounding urban areas. Urban For. Urban Green. 12 , 426–434 (2013).
Sun, X. et al. Quantifying landscape-metrics impacts on urban green-spaces and water-bodies cooling effect: the study of Nanjing, China. Urban For . Urban Green. 55 , 126838 (2020).
Zhang, Q., Zhou, D., Xu, D. & Rogora, A. Correlation between cooling effect of green space and surrounding urban spatial form: Evidence from 36 urban green spaces. Build. Environ. 222 , 109375 (2022).
Pesaresi, M., Politis, P. GHS-BUILT-H R2023A - GHS building height, derived from AW3D30, SRTM30, and Sentinel2 composite (2018) . European Commission, Joint Research Centre (JRC) https://doi.org/10.2905/85005901-3A49-48DD-9D19-6261354F56FE (2023).
Yamazaki, D. et al. A high‐accuracy map of global terrain elevations. Geophys. Res. Lett. 44 , 5844–5853 (2017).
Wessel, P. & Smith, W. H. A global, self‐consistent, hierarchical, high‐resolution shoreline database. J. Geophys. Res. Solid Earth 101 , 8741–8743 (1996).
Ren et al. climatic map studies: a review. Int. J. Climatol. 31 , 2213–2233 (2011).
Zhou, X. et al. Evaluation of urban heat islands using local climate zones and the influence of sea-land breeze. Sust. Cities Soc. 55 , 102060 (2020).
Zhou, W., Huang, G. & Cadenasso, M. L. Does spatial configuration matter? Understanding the effects of land cover pattern on land surface temperature in urban landscapes. Landsc. Urban Plan 102 , 54–63 (2011).
Muñoz Sabater, J. ERA5-Land monthly averaged data from 1981 to present . Copernicus Climate Change Service (C3S) Climate Data Store (CDS) https://doi.org/10.24381/cds.68d2bb30 (2019).
Abatzoglou, J. T., Dobrowski, S. Z., Parks, S. A. & Hegewisch, K. C. TerraClimate, a high-resolution global dataset of monthly climate and climatic water balance from 1958–2015. Sci. Data 5 , 1–12 (2018).
Kummu, M., Taka, M. & Guillaume, J. H. Gridded global datasets for gross domestic product and Human Development Index over 1990–2015. Sci. Data 5 , 1–15 (2018).
Zanaga, D. et al. ESA WorldCover 10 m 2020 v100. https://doi.org/10.5281/zenodo.5571936 (2021).
McNally, A. et al. A land data assimilation system for sub-Saharan Africa food and water security applications. Sci. Data 4 , 1–19 (2017).
Schaaf C., & Wang Z. MODIS/Terra+Aqua BRDF/Albedo Daily L3 Global - 500m V061 . NASA EOSDIS Land Processes Distributed Active Archive Center. https://doi.org/10.5067/MODIS/MCD43A3.061 (2021).
Lyapustin A., & Wang Y. MODIS/Terra+Aqua Land Aerosol Optical Depth Daily L2G Global 1km SIN Grid V061 . NASA EOSDIS Land Processes Distributed Active Archive Center. https://doi.org/10.5067/MODIS/MCD19A2.061 (2022).
Li, M., Wang, Y., Rosier, J. F., Verburg, P. H. & Vliet, J. V. Global maps of 3D built-up patterns for urban morphological analysis. Int. J. Appl. Earth Obs. Geoinf. 114 , 103048 (2022).
Google Scholar
Elvidge, C. D., Baugh, K., Zhizhin, M., Hsu, F. C. & Ghosh, T. VIIRS night-time lights. Int. J. Remote Sens. 38 , 5860–5879 (2017).
Zhou, W. et al. Urban tree canopy has greater cooling effects in socially vulnerable communities in the US. One Earth 4 , 1764–1775 (2021).
Beck, H. E. et al. Present and future Köppen-Geiger climate classification maps at 1-km resolution. Sci. Data 5 , 1–12 (2018).
R. Core Team. R: A Language and Environment for Statistical Computing . R Foundation for Statistical Computing, Vienna, Austria. https://www.R-project.org/ (2023).
Fox J., & Weisberg S. An R Companion to Applied Regression 3rd edn (Sage, 2019). https://socialsciences.mcmaster.ca/jfox/Books/Companion/ .
Lefcheck, J. S. piecewiseSEM: Piecewise structural equation modelling in r for ecology, evolution, and systematics. Methods Ecol. Evol. 7 , 573–579 (2016).
Zeileis, A. _ineq: Measuring Inequality, Concentration, and Poverty_ . R package version 0.2-13. https://CRAN.R-project.org/package=ineq (2014).
Download references
Acknowledgements
We thank all the data providers. We thank Marten Scheffer for valuable discussion. C.X. is supported by the National Natural Science Foundation of China (Grant No. 32061143014). J.-C.S. was supported by Center for Ecological Dynamics in a Novel Biosphere (ECONOVO), funded by Danish National Research Foundation (grant DNRF173), and his VILLUM Investigator project “Biodiversity Dynamics in a Changing World”, funded by VILLUM FONDEN (grant 16549). W.Z. was supported by the National Science Foundation of China through Grant No. 42225104. T.M.L. and J.F.A. are supported by the Open Society Foundations (OR2021-82956). W.J.R. is supported by the funding received from Roger Worthington.
Author information
Authors and affiliations.
School of Life Sciences, Nanjing University, Nanjing, China
Yuxiang Li, Shuqing N. Teng & Chi Xu
Center for Ecological Dynamics in a Novel Biosphere (ECONOVO), Department of Biology, Aarhus University, Aarhus, Denmark
Jens-Christian Svenning
State Key Laboratory of Urban and Regional Ecology, Research Center for Eco-Environmental Sciences, Chinese Academy of Sciences, Beijing, China
University of Chinese Academy of Sciences, Beijing, China
Beijing Urban Ecosystem Research Station, Research Center for Eco-Environmental Sciences, Chinese Academy of Sciences, Beijing, China
School for Environment and Sustainability, University of Michigan, Ann Arbor, MI, USA
Global Systems Institute, University of Exeter, Exeter, UK
Jesse F. Abrams & Timothy M. Lenton
Department of Forest Ecosystems and Society, Oregon State University, Corvallis, OR, USA
William J. Ripple
Department of Environmental Science and Engineering, Fudan University, Shanghai, China
Department of Applied Ecology, North Carolina State University, Raleigh, NC, USA
Robert R. Dunn
You can also search for this author in PubMed Google Scholar
Contributions
Y.L., S.N.T., R.R.D., and C.X. designed the study. Y.L. collected the data, generated the code, performed the analyses, and produced the figures with inputs from J.-C.S., W.Z., K.Z., J.F.A., T.M.L., W.J.R., Z.Y., S.N.T., R.R.D. and C.X. Y.L., S.N.T., R.R.D. and C.X. wrote the first draft with inputs from J.-C.S., W.Z., K.Z., J.F.A., T.M.L., W.J.R., and Z.Y. All coauthors interpreted the results and revised the manuscript.
Corresponding authors
Correspondence to Shuqing N. Teng , Robert R. Dunn or Chi Xu .
Ethics declarations
Competing interests.
The authors declare no competing interests.
Peer review
Peer review information.
Nature Communications thanks Chris Webster and the other, anonymous, reviewer(s) for their contribution to the peer review of this work. A peer review file is available.
Additional information
Publisher’s note Springer Nature remains neutral with regard to jurisdictional claims in published maps and institutional affiliations.
Supplementary information
Supplementary information, peer review file, description of additional supplementary files, supplementary data 1, reporting summary, rights and permissions.
Open Access This article is licensed under a Creative Commons Attribution-NonCommercial-NoDerivatives 4.0 International License, which permits any non-commercial use, sharing, distribution and reproduction in any medium or format, as long as you give appropriate credit to the original author(s) and the source, provide a link to the Creative Commons licence, and indicate if you modified the licensed material. You do not have permission under this licence to share adapted material derived from this article or parts of it. The images or other third party material in this article are included in the article’s Creative Commons licence, unless indicated otherwise in a credit line to the material. If material is not included in the article’s Creative Commons licence and your intended use is not permitted by statutory regulation or exceeds the permitted use, you will need to obtain permission directly from the copyright holder. To view a copy of this licence, visit http://creativecommons.org/licenses/by-nc-nd/4.0/ .
Reprints and permissions
About this article
Cite this article.
Li, Y., Svenning, JC., Zhou, W. et al. Green spaces provide substantial but unequal urban cooling globally. Nat Commun 15 , 7108 (2024). https://doi.org/10.1038/s41467-024-51355-0
Download citation
Received : 06 December 2023
Accepted : 05 August 2024
Published : 02 September 2024
DOI : https://doi.org/10.1038/s41467-024-51355-0
Share this article
Anyone you share the following link with will be able to read this content:
Sorry, a shareable link is not currently available for this article.
Provided by the Springer Nature SharedIt content-sharing initiative
By submitting a comment you agree to abide by our Terms and Community Guidelines . If you find something abusive or that does not comply with our terms or guidelines please flag it as inappropriate.
Quick links
- Explore articles by subject
- Guide to authors
- Editorial policies
Sign up for the Nature Briefing newsletter — what matters in science, free to your inbox daily.

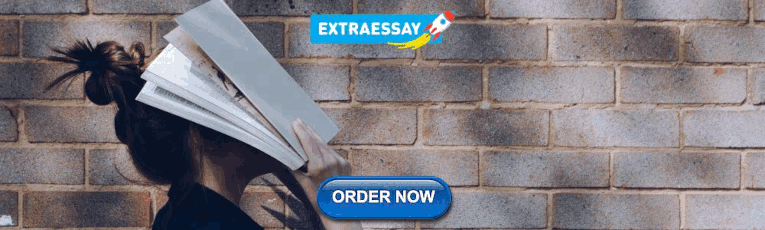
IMAGES
VIDEO
COMMENTS
A hypothesis test can either contain a directional hypothesis or a non-directional hypothesis: Directional hypothesis: The alternative hypothesis contains the less than ("<") or greater than (">") sign. This indicates that we're testing whether or not there is a positive or negative effect. Non-directional hypothesis: The alternative ...
Directional Hypothesis Examples. 1. Exercise and Heart Health. Research suggests that as regular physical exercise (independent variable) increases, the risk of heart disease (dependent variable) decreases (Jakicic, Davis, Rogers, King, Marcus, Helsel, Rickman, Wahed, Belle, 2016). In this example, a directional hypothesis anticipates that the ...
A directional hypothesis is a prediction made by a researcher regarding a positive or negative change, relationship, or difference between two variables of a population. This prediction is typically based on past research, accepted theory, extensive experience, or literature on the topic. Key words that distinguish a directional hypothesis are ...
INTRODUCTION. Scientific research is usually initiated by posing evidenced-based research questions which are then explicitly restated as hypotheses.1,2 The hypotheses provide directions to guide the study, solutions, explanations, and expected results.3,4 Both research questions and hypotheses are essentially formulated based on conventional theories and real-world processes, which allow the ...
Step 3: Use Clear Language. Write your directional hypothesis using clear and concise language. Avoid technical jargon or terms that may be difficult for readers to understand. Your hypothesis should be easily understood by both researchers and non-experts.
Non-directional hypothesis: A non-directional hypothesis only claims an effect on the dependent variable. It does not clarify whether the result would be positive or negative. The sign for a non-directional hypothesis is '≠.' 3. Simple hypothesis. A simple hypothesis is a statement made to reflect the relation between exactly two variables.
Directional hypotheses, also known as one-tailed hypotheses, are statements in research that make specific predictions about the direction of a relationship or difference between variables. Unlike non-directional hypotheses, which simply state that there is a relationship or difference without specifying its direction, directional hypotheses ...
A research hypothesis, in its plural form "hypotheses," is a specific, testable prediction about the anticipated results of a study, established at its outset. It is a key component of the scientific method. Hypotheses connect theory to data and guide the research process towards expanding scientific understanding.
A hypothesis (from the Greek, foundation) is a logical construct, interposed between a problem and its solution, which represents a proposed answer to a research question. It gives direction to the investigator's thinking about the problem and, therefore, facilitates a solution. Unlike facts and assumptions (presumed true and, therefore, not ...
Whether a directional or non-directional hypothesis is chosen depends on knowledge from previous research. If the findings of previous research suggest the direction of the findings use a directional hypothesis. When there is little or no research or the findings are ambiguous, it is best to use a non-directional hypothesis.
Definition: Hypothesis is an educated guess or proposed explanation for a phenomenon, based on some initial observations or data. It is a tentative statement that can be tested and potentially proven or disproven through further investigation and experimentation. Hypothesis is often used in scientific research to guide the design of experiments ...
The directional hypothesis can also state a negative correlation, e.g. the higher the number of face-book friends, the lower the life satisfaction score ". Non-directional hypothesis: A non-directional (or two tailed hypothesis) simply states that there will be a difference between the two groups/conditions but does not say which will be ...
Three Different Hypotheses: (1) Directional Hypothesis: states that the IV will have an effect on the DV and what that effect will be (the direction of results). For example, eating smarties will significantly improve an individual's dancing ability. When writing a directional hypothesis, it is important that you state exactly how the IV will ...
study) Describe the experiences (e.g., phenomenology) Report the stories (e.g., narrative research) Use these more exploratory verbs that are nondirectional rather than directional words that suggest quantitative research, such as "affect," "influence," "impact," "determine," "cause," and "relate.".
Abstract. Statistical hypothesis testing is common in research, but a conventional understanding sometimes leads to mistaken application and misinterpretation. The logic of hypothesis testing presented in this article provides for a clearer understanding, application, and interpretation. Key conclusions are that (a) the magnitude of an estimate ...
Hypotheses: directional or non-directional? 6.2 166-167 Chapter 6: Research methods Experimental method Your task: Decide whether the following general hypotheses are directional or non-directional. 1. Alcohol affects reaction time. 2. Men who have beards are perceived as older than clean-shaven men. 3. The quality of beer affects bar takings. 4.
Research methods in psychology are systematic procedures used to observe, describe, predict, and explain behavior and mental processes. ... This is also known as the experimental hypothesis. One-tailed (directional) hypotheses - these state the specific direction the researcher expects the results to move in, e.g. higher, lower, more, less ...
It seeks to explore and understand a particular aspect of the research subject. In contrast, a research hypothesis is a specific statement or prediction that suggests an expected relationship between variables. It is formulated based on existing knowledge or theories and guides the research design and data analysis. 7.
Whether a directional or non-directional hypothesis is chosen depends on knowledge from previous research. If the findings of previous research suggest the direction of the findings use directional hypothesis. When there is little or no research or the findings are ambiguous, it is best to use a non-directional hypothesis. IV and DV Identification
There are seven different types of research hypotheses. Simple Hypothesis. A simple hypothesis predicts the relationship between a single dependent variable and a single independent variable. Complex Hypothesis. A complex hypothesis predicts the relationship between two or more independent and dependent variables. Directional Hypothesis.
A directional hypothesis predicts a specific direction of change, while a non-directional hypothesis predicts that there will be a difference between groups or conditions without specifying the direction of that difference. It's important to understand the difference between these types of hypotheses to conduct rigorous and insightful research ...
A directional hypothesis is when a prediction is made about the specific effects of an experimental variable or treatment. ... Research Methods in Psychology for Teachers: Professional Development ...
A bi-directional relationship (correlation) is fitted between mean annual temperature and precipitation. Red and blue solid arrows indicate significantly negative and positive coefficients with p ...