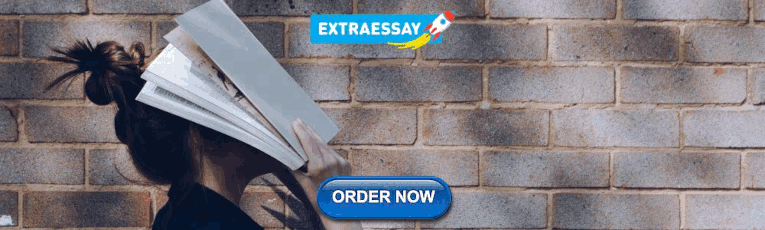
Setting Clear Learning Targets to Guide Instruction for All Students
Research output : Contribution to journal › Article › peer-review
As more states adopt the Common Core State Standards, teachers face new challenges. Teachers must unpack these standards and develop explicit learning targets to make these rigorous standards accessible to their students. This task can be especially challenging for special educators who must balance standards-based education with individualized instruction. This paper describes the value of clarifying learning targets, defines different types of targets, and provides strategies and resources to assist practitioners in unpacking standards to develop learning targets. In addition, the authors suggest how the standards can be used to drive individualized education program planning to maximize learning for students with disabilities and increase the likelihood of student success.
Original language | English (US) |
---|---|
Pages (from-to) | 76-85 |
Number of pages | 10 |
Journal | |
Volume | 50 |
Issue number | 2 |
DOIs | |
State | Published - Nov 8 2014 |
Externally published | Yes |
Bibliographical note
- Common Core State Standards
- learning targets
This output contributes to the following UN Sustainable Development Goals (SDGs)
Publisher link
- 10.1177/1053451214536042
Other files and links
- Link to publication in Scopus
- Link to the citations in Scopus
Fingerprint
- Education Program Social Sciences 100%
- Student Success Social Sciences 100%
- Individualized Instruction Social Sciences 100%
- Core Curriculum Social Sciences 100%
- Explicit Learning Social Sciences 100%
- Students with Disabilities Social Sciences 100%
- Learning Objectives Keyphrases 100%
- Physician Nursing and Health Professions 100%
T1 - Setting Clear Learning Targets to Guide Instruction for All Students
AU - Konrad, Moira
AU - Keesey, Susan
AU - Ressa, Virginia A.
AU - Alexeeff, Maggie
AU - Chan, Paula E.
AU - Peters, Mary T.
N1 - Publisher Copyright: © Hammill Institute on Disabilities 2014.
PY - 2014/11/8
Y1 - 2014/11/8
N2 - As more states adopt the Common Core State Standards, teachers face new challenges. Teachers must unpack these standards and develop explicit learning targets to make these rigorous standards accessible to their students. This task can be especially challenging for special educators who must balance standards-based education with individualized instruction. This paper describes the value of clarifying learning targets, defines different types of targets, and provides strategies and resources to assist practitioners in unpacking standards to develop learning targets. In addition, the authors suggest how the standards can be used to drive individualized education program planning to maximize learning for students with disabilities and increase the likelihood of student success.
AB - As more states adopt the Common Core State Standards, teachers face new challenges. Teachers must unpack these standards and develop explicit learning targets to make these rigorous standards accessible to their students. This task can be especially challenging for special educators who must balance standards-based education with individualized instruction. This paper describes the value of clarifying learning targets, defines different types of targets, and provides strategies and resources to assist practitioners in unpacking standards to develop learning targets. In addition, the authors suggest how the standards can be used to drive individualized education program planning to maximize learning for students with disabilities and increase the likelihood of student success.
KW - Common Core State Standards
KW - IEP goals
KW - learning targets
KW - objectives
KW - planning
UR - http://www.scopus.com/inward/record.url?scp=84908529808&partnerID=8YFLogxK
UR - http://www.scopus.com/inward/citedby.url?scp=84908529808&partnerID=8YFLogxK
U2 - 10.1177/1053451214536042
DO - 10.1177/1053451214536042
M3 - Article
AN - SCOPUS:84908529808
SN - 1053-4512
JO - Intervention in School and Clinic
JF - Intervention in School and Clinic
- Tutorial Review
- Open access
- Published: 24 January 2018
Teaching the science of learning
- Yana Weinstein ORCID: orcid.org/0000-0002-5144-968X 1 ,
- Christopher R. Madan 2 , 3 &
- Megan A. Sumeracki 4
Cognitive Research: Principles and Implications volume 3 , Article number: 2 ( 2018 ) Cite this article
273k Accesses
101 Citations
756 Altmetric
Metrics details
The science of learning has made a considerable contribution to our understanding of effective teaching and learning strategies. However, few instructors outside of the field are privy to this research. In this tutorial review, we focus on six specific cognitive strategies that have received robust support from decades of research: spaced practice, interleaving, retrieval practice, elaboration, concrete examples, and dual coding. We describe the basic research behind each strategy and relevant applied research, present examples of existing and suggested implementation, and make recommendations for further research that would broaden the reach of these strategies.
Significance
Education does not currently adhere to the medical model of evidence-based practice (Roediger, 2013 ). However, over the past few decades, our field has made significant advances in applying cognitive processes to education. From this work, specific recommendations can be made for students to maximize their learning efficiency (Dunlosky, Rawson, Marsh, Nathan, & Willingham, 2013 ; Roediger, Finn, & Weinstein, 2012 ). In particular, a review published 10 years ago identified a limited number of study techniques that have received solid evidence from multiple replications testing their effectiveness in and out of the classroom (Pashler et al., 2007 ). A recent textbook analysis (Pomerance, Greenberg, & Walsh, 2016 ) took the six key learning strategies from this report by Pashler and colleagues, and found that very few teacher-training textbooks cover any of these six principles – and none cover them all, suggesting that these strategies are not systematically making their way into the classroom. This is the case in spite of multiple recent academic (e.g., Dunlosky et al., 2013 ) and general audience (e.g., Dunlosky, 2013 ) publications about these strategies. In this tutorial review, we present the basic science behind each of these six key principles, along with more recent research on their effectiveness in live classrooms, and suggest ideas for pedagogical implementation. The target audience of this review is (a) educators who might be interested in integrating the strategies into their teaching practice, (b) science of learning researchers who are looking for open questions to help determine future research priorities, and (c) researchers in other subfields who are interested in the ways that principles from cognitive psychology have been applied to education.
While the typical teacher may not be exposed to this research during teacher training, a small cohort of teachers intensely interested in cognitive psychology has recently emerged. These teachers are mainly based in the UK, and, anecdotally (e.g., Dennis (2016), personal communication), appear to have taken an interest in the science of learning after reading Make it Stick (Brown, Roediger, & McDaniel, 2014 ; see Clark ( 2016 ) for an enthusiastic review of this book on a teacher’s blog, and “Learning Scientists” ( 2016c ) for a collection). In addition, a grassroots teacher movement has led to the creation of “researchED” – a series of conferences on evidence-based education (researchED, 2013 ). The teachers who form part of this network frequently discuss cognitive psychology techniques and their applications to education on social media (mainly Twitter; e.g., Fordham, 2016 ; Penfound, 2016 ) and on their blogs, such as Evidence Into Practice ( https://evidenceintopractice.wordpress.com/ ), My Learning Journey ( http://reflectionsofmyteaching.blogspot.com/ ), and The Effortful Educator ( https://theeffortfuleducator.com/ ). In general, the teachers who write about these issues pay careful attention to the relevant literature, often citing some of the work described in this review.
These informal writings, while allowing teachers to explore their approach to teaching practice (Luehmann, 2008 ), give us a unique window into the application of the science of learning to the classroom. By examining these blogs, we can not only observe how basic cognitive research is being applied in the classroom by teachers who are reading it, but also how it is being misapplied, and what questions teachers may be posing that have gone unaddressed in the scientific literature. Throughout this review, we illustrate each strategy with examples of how it can be implemented (see Table 1 and Figs. 1 , 2 , 3 , 4 , 5 , 6 and 7 ), as well as with relevant teacher blog posts that reflect on its application, and draw upon this work to pin-point fruitful avenues for further basic and applied research.
Spaced practice schedule for one week. This schedule is designed to represent a typical timetable of a high-school student. The schedule includes four one-hour study sessions, one longer study session on the weekend, and one rest day. Notice that each subject is studied one day after it is covered in school, to create spacing between classes and study sessions. Copyright note: this image was produced by the authors
a Blocked practice and interleaved practice with fraction problems. In the blocked version, students answer four multiplication problems consecutively. In the interleaved version, students answer a multiplication problem followed by a division problem and then an addition problem, before returning to multiplication. For an experiment with a similar setup, see Patel et al. ( 2016 ). Copyright note: this image was produced by the authors. b Illustration of interleaving and spacing. Each color represents a different homework topic. Interleaving involves alternating between topics, rather than blocking. Spacing involves distributing practice over time, rather than massing. Interleaving inherently involves spacing as other tasks naturally “fill” the spaces between interleaved sessions. Copyright note: this image was produced by the authors, adapted from Rohrer ( 2012 )
Concept map illustrating the process and resulting benefits of retrieval practice. Retrieval practice involves the process of withdrawing learned information from long-term memory into working memory, which requires effort. This produces direct benefits via the consolidation of learned information, making it easier to remember later and causing improvements in memory, transfer, and inferences. Retrieval practice also produces indirect benefits of feedback to students and teachers, which in turn can lead to more effective study and teaching practices, with a focus on information that was not accurately retrieved. Copyright note: this figure originally appeared in a blog post by the first and third authors ( http://www.learningscientists.org/blog/2016/4/1-1 )
Illustration of “how” and “why” questions (i.e., elaborative interrogation questions) students might ask while studying the physics of flight. To help figure out how physics explains flight, students might ask themselves the following questions: “How does a plane take off?”; “Why does a plane need an engine?”; “How does the upward force (lift) work?”; “Why do the wings have a curved upper surface and a flat lower surface?”; and “Why is there a downwash behind the wings?”. Copyright note: the image of the plane was downloaded from Pixabay.com and is free to use, modify, and share
Three examples of physics problems that would be categorized differently by novices and experts. The problems in ( a ) and ( c ) look similar on the surface, so novices would group them together into one category. Experts, however, will recognize that the problems in ( b ) and ( c ) both relate to the principle of energy conservation, and so will group those two problems into one category instead. Copyright note: the figure was produced by the authors, based on figures in Chi et al. ( 1981 )
Example of how to enhance learning through use of a visual example. Students might view this visual representation of neural communications with the words provided, or they could draw a similar visual representation themselves. Copyright note: this figure was produced by the authors
Example of word properties associated with visual, verbal, and motor coding for the word “SPOON”. A word can evoke multiple types of representation (“codes” in dual coding theory). Viewing a word will automatically evoke verbal representations related to its component letters and phonemes. Words representing objects (i.e., concrete nouns) will also evoke visual representations, including information about similar objects, component parts of the object, and information about where the object is typically found. In some cases, additional codes can also be evoked, such as motor-related properties of the represented object, where contextual information related to the object’s functional intention and manipulation action may also be processed automatically when reading the word. Copyright note: this figure was produced by the authors and is based on Aylwin ( 1990 ; Fig. 2 ) and Madan and Singhal ( 2012a , Fig. 3 )
Spaced practice
The benefits of spaced (or distributed) practice to learning are arguably one of the strongest contributions that cognitive psychology has made to education (Kang, 2016 ). The effect is simple: the same amount of repeated studying of the same information spaced out over time will lead to greater retention of that information in the long run, compared with repeated studying of the same information for the same amount of time in one study session. The benefits of distributed practice were first empirically demonstrated in the 19 th century. As part of his extensive investigation into his own memory, Ebbinghaus ( 1885/1913 ) found that when he spaced out repetitions across 3 days, he could almost halve the number of repetitions necessary to relearn a series of 12 syllables in one day (Chapter 8). He thus concluded that “a suitable distribution of [repetitions] over a space of time is decidedly more advantageous than the massing of them at a single time” (Section 34). For those who want to read more about Ebbinghaus’s contribution to memory research, Roediger ( 1985 ) provides an excellent summary.
Since then, hundreds of studies have examined spacing effects both in the laboratory and in the classroom (Kang, 2016 ). Spaced practice appears to be particularly useful at large retention intervals: in the meta-analysis by Cepeda, Pashler, Vul, Wixted, and Rohrer ( 2006 ), all studies with a retention interval longer than a month showed a clear benefit of distributed practice. The “new theory of disuse” (Bjork & Bjork, 1992 ) provides a helpful mechanistic explanation for the benefits of spacing to learning. This theory posits that memories have both retrieval strength and storage strength. Whereas retrieval strength is thought to measure the ease with which a memory can be recalled at a given moment, storage strength (which cannot be measured directly) represents the extent to which a memory is truly embedded in the mind. When studying is taking place, both retrieval strength and storage strength receive a boost. However, the extent to which storage strength is boosted depends upon retrieval strength, and the relationship is negative: the greater the current retrieval strength, the smaller the gains in storage strength. Thus, the information learned through “cramming” will be rapidly forgotten due to high retrieval strength and low storage strength (Bjork & Bjork, 2011 ), whereas spacing out learning increases storage strength by allowing retrieval strength to wane before restudy.
Teachers can introduce spacing to their students in two broad ways. One involves creating opportunities to revisit information throughout the semester, or even in future semesters. This does involve some up-front planning, and can be difficult to achieve, given time constraints and the need to cover a set curriculum. However, spacing can be achieved with no great costs if teachers set aside a few minutes per class to review information from previous lessons. The second method involves putting the onus to space on the students themselves. Of course, this would work best with older students – high school and above. Because spacing requires advance planning, it is crucial that the teacher helps students plan their studying. For example, teachers could suggest that students schedule study sessions on days that alternate with the days on which a particular class meets (e.g., schedule review sessions for Tuesday and Thursday when the class meets Monday and Wednesday; see Fig. 1 for a more complete weekly spaced practice schedule). It important to note that the spacing effect refers to information that is repeated multiple times, rather than the idea of studying different material in one long session versus spaced out in small study sessions over time. However, for teachers and particularly for students planning a study schedule, the subtle difference between the two situations (spacing out restudy opportunities, versus spacing out studying of different information over time) may be lost. Future research should address the effects of spacing out studying of different information over time, whether the same considerations apply in this situation as compared to spacing out restudy opportunities, and how important it is for teachers and students to understand the difference between these two types of spaced practice.
It is important to note that students may feel less confident when they space their learning (Bjork, 1999 ) than when they cram. This is because spaced learning is harder – but it is this “desirable difficulty” that helps learning in the long term (Bjork, 1994 ). Students tend to cram for exams rather than space out their learning. One explanation for this is that cramming does “work”, if the goal is only to pass an exam. In order to change students’ minds about how they schedule their studying, it might be important to emphasize the value of retaining information beyond a final exam in one course.
Ideas for how to apply spaced practice in teaching have appeared in numerous teacher blogs (e.g., Fawcett, 2013 ; Kraft, 2015 ; Picciotto, 2009 ). In England in particular, as of 2013, high-school students need to be able to remember content from up to 3 years back on cumulative exams (General Certificate of Secondary Education (GCSE) and A-level exams; see CIFE, 2012 ). A-levels in particular determine what subject students study in university and which programs they are accepted into, and thus shape the path of their academic career. A common approach for dealing with these exams has been to include a “revision” (i.e., studying or cramming) period of a few weeks leading up to the high-stakes cumulative exams. Now, teachers who follow cognitive psychology are advocating a shift of priorities to spacing learning over time across the 3 years, rather than teaching a topic once and then intensely reviewing it weeks before the exam (Cox, 2016a ; Wood, 2017 ). For example, some teachers have suggested using homework assignments as an opportunity for spaced practice by giving students homework on previous topics (Rose, 2014 ). However, questions remain, such as whether spaced practice can ever be effective enough to completely alleviate the need or utility of a cramming period (Cox, 2016b ), and how one can possibly figure out the optimal lag for spacing (Benney, 2016 ; Firth, 2016 ).
There has been considerable research on the question of optimal lag, and much of it is quite complex; two sessions neither too close together (i.e., cramming) nor too far apart are ideal for retention. In a large-scale study, Cepeda, Vul, Rohrer, Wixted, and Pashler ( 2008 ) examined the effects of the gap between study sessions and the interval between study and test across long periods, and found that the optimal gap between study sessions was contingent on the retention interval. Thus, it is not clear how teachers can apply the complex findings on lag to their own classrooms.
A useful avenue of research would be to simplify the research paradigms that are used to study optimal lag, with the goal of creating a flexible, spaced-practice framework that teachers could apply and tailor to their own teaching needs. For example, an Excel macro spreadsheet was recently produced to help teachers plan for lagged lessons (Weinstein-Jones & Weinstein, 2017 ; see Weinstein & Weinstein-Jones ( 2017 ) for a description of the algorithm used in the spreadsheet), and has been used by teachers to plan their lessons (Penfound, 2017 ). However, one teacher who found this tool helpful also wondered whether the more sophisticated plan was any better than his own method of manually selecting poorly understood material from previous classes for later review (Lovell, 2017 ). This direction is being actively explored within personalized online learning environments (Kornell & Finn, 2016 ; Lindsey, Shroyer, Pashler, & Mozer, 2014 ), but teachers in physical classrooms might need less technologically-driven solutions to teach cohorts of students.
It seems teachers would greatly appreciate a set of guidelines for how to implement spacing in the curriculum in the most effective, but also the most efficient manner. While the cognitive field has made great advances in terms of understanding the mechanisms behind spacing, what teachers need more of are concrete evidence-based tools and guidelines for direct implementation in the classroom. These could include more sophisticated and experimentally tested versions of the software described above (Weinstein-Jones & Weinstein, 2017 ), or adaptable templates of spaced curricula. Moreover, researchers need to evaluate the effectiveness of these tools in a real classroom environment, over a semester or academic year, in order to give pedagogically relevant evidence-based recommendations to teachers.
Interleaving
Another scheduling technique that has been shown to increase learning is interleaving. Interleaving occurs when different ideas or problem types are tackled in a sequence, as opposed to the more common method of attempting multiple versions of the same problem in a given study session (known as blocking). Interleaving as a principle can be applied in many different ways. One such way involves interleaving different types of problems during learning, which is particularly applicable to subjects such as math and physics (see Fig. 2 a for an example with fractions, based on a study by Patel, Liu, & Koedinger, 2016 ). For example, in a study with college students, Rohrer and Taylor ( 2007 ) found that shuffling math problems that involved calculating the volume of different shapes resulted in better test performance 1 week later than when students answered multiple problems about the same type of shape in a row. This pattern of results has also been replicated with younger students, for example 7 th grade students learning to solve graph and slope problems (Rohrer, Dedrick, & Stershic, 2015 ). The proposed explanation for the benefit of interleaving is that switching between different problem types allows students to acquire the ability to choose the right method for solving different types of problems rather than learning only the method itself, and not when to apply it.
Do the benefits of interleaving extend beyond problem solving? The answer appears to be yes. Interleaving can be helpful in other situations that require discrimination, such as inductive learning. Kornell and Bjork ( 2008 ) examined the effects of interleaving in a task that might be pertinent to a student of the history of art: the ability to match paintings to their respective painters. Students who studied different painters’ paintings interleaved at study were more successful on a later identification test than were participants who studied the paintings blocked by painter. Birnbaum, Kornell, Bjork, and Bjork ( 2013 ) proposed the discriminative-contrast hypothesis to explain that interleaving enhances learning by allowing the comparison between exemplars of different categories. They found support for this hypothesis in a set of experiments with bird categorization: participants benefited from interleaving and also from spacing, but not when the spacing interrupted side-by-side comparisons of birds from different categories.
Another type of interleaving involves the interleaving of study and test opportunities. This type of interleaving has been applied, once again, to problem solving, whereby students alternate between attempting a problem and viewing a worked example (Trafton & Reiser, 1993 ); this pattern appears to be superior to answering a string of problems in a row, at least with respect to the amount of time it takes to achieve mastery of a procedure (Corbett, Reed, Hoffmann, MacLaren, & Wagner, 2010 ). The benefits of interleaving study and test opportunities – rather than blocking study followed by attempting to answer problems or questions – might arise due to a process known as “test-potentiated learning”. That is, a study opportunity that immediately follows a retrieval attempt may be more fruitful than when that same studying was not preceded by retrieval (Arnold & McDermott, 2013 ).
For problem-based subjects, the interleaving technique is straightforward: simply mix questions on homework and quizzes with previous materials (which takes care of spacing as well); for languages, mix vocabulary themes rather than blocking by theme (Thomson & Mehring, 2016 ). But interleaving as an educational strategy ought to be presented to teachers with some caveats. Research has focused on interleaving material that is somewhat related (e.g., solving different mathematical equations, Rohrer et al., 2015 ), whereas students sometimes ask whether they should interleave material from different subjects – a practice that has not received empirical support (Hausman & Kornell, 2014 ). When advising students how to study independently, teachers should thus proceed with caution. Since it is easy for younger students to confuse this type of unhelpful interleaving with the more helpful interleaving of related information, it may be best for teachers of younger grades to create opportunities for interleaving in homework and quiz assignments rather than putting the onus on the students themselves to make use of the technique. Technology can be very helpful here, with apps such as Quizlet, Memrise, Anki, Synap, Quiz Champ, and many others (see also “Learning Scientists”, 2017 ) that not only allow instructor-created quizzes to be taken by students, but also provide built-in interleaving algorithms so that the burden does not fall on the teacher or the student to carefully plan which items are interleaved when.
An important point to consider is that in educational practice, the distinction between spacing and interleaving can be difficult to delineate. The gap between the scientific and classroom definitions of interleaving is demonstrated by teachers’ own writings about this technique. When they write about interleaving, teachers often extend the term to connote a curriculum that involves returning to topics multiple times throughout the year (e.g., Kirby, 2014 ; see “Learning Scientists” ( 2016a ) for a collection of similar blog posts by several other teachers). The “interleaving” of topics throughout the curriculum produces an effect that is more akin to what cognitive psychologists call “spacing” (see Fig. 2 b for a visual representation of the difference between interleaving and spacing). However, cognitive psychologists have not examined the effects of structuring the curriculum in this way, and open questions remain: does repeatedly circling back to previous topics throughout the semester interrupt the learning of new information? What are some effective techniques for interleaving old and new information within one class? And how does one determine the balance between old and new information?
Retrieval practice
While tests are most often used in educational settings for assessment, a lesser-known benefit of tests is that they actually improve memory of the tested information. If we think of our memories as libraries of information, then it may seem surprising that retrieval (which happens when we take a test) improves memory; however, we know from a century of research that retrieving knowledge actually strengthens it (see Karpicke, Lehman, & Aue, 2014 ). Testing was shown to strengthen memory as early as 100 years ago (Gates, 1917 ), and there has been a surge of research in the last decade on the mnemonic benefits of testing, or retrieval practice . Most of the research on the effectiveness of retrieval practice has been done with college students (see Roediger & Karpicke, 2006 ; Roediger, Putnam, & Smith, 2011 ), but retrieval-based learning has been shown to be effective at producing learning for a wide range of ages, including preschoolers (Fritz, Morris, Nolan, & Singleton, 2007 ), elementary-aged children (e.g., Karpicke, Blunt, & Smith, 2016 ; Karpicke, Blunt, Smith, & Karpicke, 2014 ; Lipko-Speed, Dunlosky, & Rawson, 2014 ; Marsh, Fazio, & Goswick, 2012 ; Ritchie, Della Sala, & McIntosh, 2013 ), middle-school students (e.g., McDaniel, Thomas, Agarwal, McDermott, & Roediger, 2013 ; McDermott, Agarwal, D’Antonio, Roediger, & McDaniel, 2014 ), and high-school students (e.g., McDermott et al., 2014 ). In addition, the effectiveness of retrieval-based learning has been extended beyond simple testing to other activities in which retrieval practice can be integrated, such as concept mapping (Blunt & Karpicke, 2014 ; Karpicke, Blunt, et al., 2014 ; Ritchie et al., 2013 ).
A debate is currently ongoing as to the effectiveness of retrieval practice for more complex materials (Karpicke & Aue, 2015 ; Roelle & Berthold, 2017 ; Van Gog & Sweller, 2015 ). Practicing retrieval has been shown to improve the application of knowledge to new situations (e.g., Butler, 2010 ; Dirkx, Kester, & Kirschner, 2014 ); McDaniel et al., 2013 ; Smith, Blunt, Whiffen, & Karpicke, 2016 ); but see Tran, Rohrer, and Pashler ( 2015 ) and Wooldridge, Bugg, McDaniel, and Liu ( 2014 ), for retrieval practice studies that showed limited or no increased transfer compared to restudy. Retrieval practice effects on higher-order learning may be more sensitive than fact learning to encoding factors, such as the way material is presented during study (Eglington & Kang, 2016 ). In addition, retrieval practice may be more beneficial for higher-order learning if it includes more scaffolding (Fiechter & Benjamin, 2017 ; but see Smith, Blunt, et al., 2016 ) and targeted practice with application questions (Son & Rivas, 2016 ).
How does retrieval practice help memory? Figure 3 illustrates both the direct and indirect benefits of retrieval practice identified by the literature. The act of retrieval itself is thought to strengthen memory (Karpicke, Blunt, et al., 2014 ; Roediger & Karpicke, 2006 ; Smith, Roediger, & Karpicke, 2013 ). For example, Smith et al. ( 2013 ) showed that if students brought information to mind without actually producing it (covert retrieval), they remembered the information just as well as if they overtly produced the retrieved information (overt retrieval). Importantly, both overt and covert retrieval practice improved memory over control groups without retrieval practice, even when feedback was not provided. The fact that bringing information to mind in the absence of feedback or restudy opportunities improves memory leads researchers to conclude that it is the act of retrieval – thinking back to bring information to mind – that improves memory of that information.
The benefit of retrieval practice depends to a certain extent on successful retrieval (see Karpicke, Lehman, et al., 2014 ). For example, in Experiment 4 of Smith et al. ( 2013 ), students successfully retrieved 72% of the information during retrieval practice. Of course, retrieving 72% of the information was compared to a restudy control group, during which students were re-exposed to 100% of the information, creating a bias in favor of the restudy condition. Yet retrieval led to superior memory later compared to the restudy control. However, if retrieval success is extremely low, then it is unlikely to improve memory (e.g., Karpicke, Blunt, et al., 2014 ), particularly in the absence of feedback. On the other hand, if retrieval-based learning situations are constructed in such a way that ensures high levels of success, the act of bringing the information to mind may be undermined, thus making it less beneficial. For example, if a student reads a sentence and then immediately covers the sentence and recites it out loud, they are likely not retrieving the information but rather just keeping the information in their working memory long enough to recite it again (see Smith, Blunt, et al., 2016 for a discussion of this point). Thus, it is important to balance success of retrieval with overall difficulty in retrieving the information (Smith & Karpicke, 2014 ; Weinstein, Nunes, & Karpicke, 2016 ). If initial retrieval success is low, then feedback can help improve the overall benefit of practicing retrieval (Kang, McDermott, & Roediger, 2007 ; Smith & Karpicke, 2014 ). Kornell, Klein, and Rawson ( 2015 ), however, found that it was the retrieval attempt and not the correct production of information that produced the retrieval practice benefit – as long as the correct answer was provided after an unsuccessful attempt, the benefit was the same as for a successful retrieval attempt in this set of studies. From a practical perspective, it would be helpful for teachers to know when retrieval attempts in the absence of success are helpful, and when they are not. There may also be additional reasons beyond retrieval benefits that would push teachers towards retrieval practice activities that produce some success amongst students; for example, teachers may hesitate to give students retrieval practice exercises that are too difficult, as this may negatively affect self-efficacy and confidence.
In addition to the fact that bringing information to mind directly improves memory for that information, engaging in retrieval practice can produce indirect benefits as well (see Roediger et al., 2011 ). For example, research by Weinstein, Gilmore, Szpunar, and McDermott ( 2014 ) demonstrated that when students expected to be tested, the increased test expectancy led to better-quality encoding of new information. Frequent testing can also serve to decrease mind-wandering – that is, thoughts that are unrelated to the material that students are supposed to be studying (Szpunar, Khan, & Schacter, 2013 ).
Practicing retrieval is a powerful way to improve meaningful learning of information, and it is relatively easy to implement in the classroom. For example, requiring students to practice retrieval can be as simple as asking students to put their class materials away and try to write out everything they know about a topic. Retrieval-based learning strategies are also flexible. Instructors can give students practice tests (e.g., short-answer or multiple-choice, see Smith & Karpicke, 2014 ), provide open-ended prompts for the students to recall information (e.g., Smith, Blunt, et al., 2016 ) or ask their students to create concept maps from memory (e.g., Blunt & Karpicke, 2014 ). In one study, Weinstein et al. ( 2016 ) looked at the effectiveness of inserting simple short-answer questions into online learning modules to see whether they improved student performance. Weinstein and colleagues also manipulated the placement of the questions. For some students, the questions were interspersed throughout the module, and for other students the questions were all presented at the end of the module. Initial success on the short-answer questions was higher when the questions were interspersed throughout the module. However, on a later test of learning from that module, the original placement of the questions in the module did not matter for performance. As with spaced practice, where the optimal gap between study sessions is contingent on the retention interval, the optimum difficulty and level of success during retrieval practice may also depend on the retention interval. Both groups of students who answered questions performed better on the delayed test compared to a control group without question opportunities during the module. Thus, the important thing is for instructors to provide opportunities for retrieval practice during learning. Based on previous research, any activity that promotes the successful retrieval of information should improve learning.
Retrieval practice has received a lot of attention in teacher blogs (see “Learning Scientists” ( 2016b ) for a collection). A common theme seems to be an emphasis on low-stakes (Young, 2016 ) and even no-stakes (Cox, 2015 ) testing, the goal of which is to increase learning rather than assess performance. In fact, one well-known charter school in the UK has an official homework policy grounded in retrieval practice: students are to test themselves on subject knowledge for 30 minutes every day in lieu of standard homework (Michaela Community School, 2014 ). The utility of homework, particularly for younger children, is often a hotly debated topic outside of academia (e.g., Shumaker, 2016 ; but see Jones ( 2016 ) for an opposing viewpoint and Cooper ( 1989 ) for the original research the blog posts were based on). Whereas some research shows clear links between homework and academic achievement (Valle et al., 2016 ), other researchers have questioned the effectiveness of homework (Dettmers, Trautwein, & Lüdtke, 2009 ). Perhaps amending homework to involve retrieval practice might make it more effective; this remains an open empirical question.
One final consideration is that of test anxiety. While retrieval practice can be very powerful at improving memory, some research shows that pressure during retrieval can undermine some of the learning benefit. For example, Hinze and Rapp ( 2014 ) manipulated pressure during quizzing to create high-pressure and low-pressure conditions. On the quizzes themselves, students performed equally well. However, those in the high-pressure condition did not perform as well on a criterion test later compared to the low-pressure group. Thus, test anxiety may reduce the learning benefit of retrieval practice. Eliminating all high-pressure tests is probably not possible, but instructors can provide a number of low-stakes retrieval opportunities for students to help increase learning. The use of low-stakes testing can serve to decrease test anxiety (Khanna, 2015 ), and has recently been shown to negate the detrimental impact of stress on learning (Smith, Floerke, & Thomas, 2016 ). This is a particularly important line of inquiry to pursue for future research, because many teachers who are not familiar with the effectiveness of retrieval practice may be put off by the implied pressure of “testing”, which evokes the much maligned high-stakes standardized tests (e.g., McHugh, 2013 ).
Elaboration
Elaboration involves connecting new information to pre-existing knowledge. Anderson ( 1983 , p.285) made the following claim about elaboration: “One of the most potent manipulations that can be performed in terms of increasing a subject’s memory for material is to have the subject elaborate on the to-be-remembered material.” Postman ( 1976 , p. 28) defined elaboration most parsimoniously as “additions to nominal input”, and Hirshman ( 2001 , p. 4369) provided an elaboration on this definition (pun intended!), defining elaboration as “A conscious, intentional process that associates to-be-remembered information with other information in memory.” However, in practice, elaboration could mean many different things. The common thread in all the definitions is that elaboration involves adding features to an existing memory.
One possible instantiation of elaboration is thinking about information on a deeper level. The levels (or “depth”) of processing framework, proposed by Craik and Lockhart ( 1972 ), predicts that information will be remembered better if it is processed more deeply in terms of meaning, rather than shallowly in terms of form. The leves of processing framework has, however, received a number of criticisms (Craik, 2002 ). One major problem with this framework is that it is difficult to measure “depth”. And if we are not able to actually measure depth, then the argument can become circular: is it that something was remembered better because it was studied more deeply, or do we conclude that it must have been studied more deeply because it is remembered better? (See Lockhart & Craik, 1990 , for further discussion of this issue).
Another mechanism by which elaboration can confer a benefit to learning is via improvement in organization (Bellezza, Cheesman, & Reddy, 1977 ; Mandler, 1979 ). By this view, elaboration involves making information more integrated and organized with existing knowledge structures. By connecting and integrating the to-be-learned information with other concepts in memory, students can increase the extent to which the ideas are organized in their minds, and this increased organization presumably facilitates the reconstruction of the past at the time of retrieval.
Elaboration is such a broad term and can include so many different techniques that it is hard to claim that elaboration will always help learning. There is, however, a specific technique under the umbrella of elaboration for which there is relatively strong evidence in terms of effectiveness (Dunlosky et al., 2013 ; Pashler et al., 2007 ). This technique is called elaborative interrogation, and involves students questioning the materials that they are studying (Pressley, McDaniel, Turnure, Wood, & Ahmad, 1987 ). More specifically, students using this technique would ask “how” and “why” questions about the concepts they are studying (see Fig. 4 for an example on the physics of flight). Then, crucially, students would try to answer these questions – either from their materials or, eventually, from memory (McDaniel & Donnelly, 1996 ). The process of figuring out the answer to the questions – with some amount of uncertainty (Overoye & Storm, 2015 ) – can help learning. When using this technique, however, it is important that students check their answers with their materials or with the teacher; when the content generated through elaborative interrogation is poor, it can actually hurt learning (Clinton, Alibali, & Nathan, 2016 ).
Students can also be encouraged to self-explain concepts to themselves while learning (Chi, De Leeuw, Chiu, & LaVancher, 1994 ). This might involve students simply saying out loud what steps they need to perform to solve an equation. Aleven and Koedinger ( 2002 ) conducted two classroom studies in which students were either prompted by a “cognitive tutor” to provide self-explanations during a problem-solving task or not, and found that the self-explanations led to improved performance. According to the authors, this approach could scale well to real classrooms. If possible and relevant, students could even perform actions alongside their self-explanations (Cohen, 1981 ; see also the enactment effect, Hainselin, Picard, Manolli, Vankerkore-Candas, & Bourdin, 2017 ). Instructors can scaffold students in these types of activities by providing self-explanation prompts throughout to-be-learned material (O’Neil et al., 2014 ). Ultimately, the greatest potential benefit of accurate self-explanation or elaboration is that the student will be able to transfer their knowledge to a new situation (Rittle-Johnson, 2006 ).
The technical term “elaborative interrogation” has not made it into the vernacular of educational bloggers (a search on https://educationechochamberuncut.wordpress.com , which consolidates over 3,000 UK-based teacher blogs, yielded zero results for that term). However, a few teachers have blogged about elaboration more generally (e.g., Hobbiss, 2016 ) and deep questioning specifically (e.g., Class Teaching, 2013 ), just without using the specific terminology. This strategy in particular may benefit from a more open dialog between researchers and teachers to facilitate the use of elaborative interrogation in the classroom and to address possible barriers to implementation. In terms of advancing the scientific understanding of elaborative interrogation in a classroom setting, it would be informative to conduct a larger-scale intervention to see whether having students elaborate during reading actually helps their understanding. It would also be useful to know whether the students really need to generate their own elaborative interrogation (“how” and “why”) questions, versus answering questions provided by others. How long should students persist to find the answers? When is the right time to have students engage in this task, given the levels of expertise required to do it well (Clinton et al., 2016 )? Without knowing the answers to these questions, it may be too early for us to instruct teachers to use this technique in their classes. Finally, elaborative interrogation takes a long time. Is this time efficiently spent? Or, would it be better to have the students try to answer a few questions, pool their information as a class, and then move to practicing retrieval of the information?
Concrete examples
Providing supporting information can improve the learning of key ideas and concepts. Specifically, using concrete examples to supplement content that is more conceptual in nature can make the ideas easier to understand and remember. Concrete examples can provide several advantages to the learning process: (a) they can concisely convey information, (b) they can provide students with more concrete information that is easier to remember, and (c) they can take advantage of the superior memorability of pictures relative to words (see “Dual Coding”).
Words that are more concrete are both recognized and recalled better than abstract words (Gorman, 1961 ; e.g., “button” and “bound,” respectively). Furthermore, it has been demonstrated that information that is more concrete and imageable enhances the learning of associations, even with abstract content (Caplan & Madan, 2016 ; Madan, Glaholt, & Caplan, 2010 ; Paivio, 1971 ). Following from this, providing concrete examples during instruction should improve retention of related abstract concepts, rather than the concrete examples alone being remembered better. Concrete examples can be useful both during instruction and during practice problems. Having students actively explain how two examples are similar and encouraging them to extract the underlying structure on their own can also help with transfer. In a laboratory study, Berry ( 1983 ) demonstrated that students performed well when given concrete practice problems, regardless of the use of verbalization (akin to elaborative interrogation), but that verbalization helped students transfer understanding from concrete to abstract problems. One particularly important area of future research is determining how students can best make the link between concrete examples and abstract ideas.
Since abstract concepts are harder to grasp than concrete information (Paivio, Walsh, & Bons, 1994 ), it follows that teachers ought to illustrate abstract ideas with concrete examples. However, care must be taken when selecting the examples. LeFevre and Dixon ( 1986 ) provided students with both concrete examples and abstract instructions and found that when these were inconsistent, students followed the concrete examples rather than the abstract instructions, potentially constraining the application of the abstract concept being taught. Lew, Fukawa-Connelly, Mejí-Ramos, and Weber ( 2016 ) used an interview approach to examine why students may have difficulty understanding a lecture. Responses indicated that some issues were related to understanding the overarching topic rather than the component parts, and to the use of informal colloquialisms that did not clearly follow from the material being taught. Both of these issues could have potentially been addressed through the inclusion of a greater number of relevant concrete examples.
One concern with using concrete examples is that students might only remember the examples – especially if they are particularly memorable, such as fun or gimmicky examples – and will not be able to transfer their understanding from one example to another, or more broadly to the abstract concept. However, there does not seem to be any evidence that fun relevant examples actually hurt learning by harming memory for important information. Instead, fun examples and jokes tend to be more memorable, but this boost in memory for the joke does not seem to come at a cost to memory for the underlying concept (Baldassari & Kelley, 2012 ). However, two important caveats need to be highlighted. First, to the extent that the more memorable content is not relevant to the concepts of interest, learning of the target information can be compromised (Harp & Mayer, 1998 ). Thus, care must be taken to ensure that all examples and gimmicks are, in fact, related to the core concepts that the students need to acquire, and do not contain irrelevant perceptual features (Kaminski & Sloutsky, 2013 ).
The second issue is that novices often notice and remember the surface details of an example rather than the underlying structure. Experts, on the other hand, can extract the underlying structure from examples that have divergent surface features (Chi, Feltovich, & Glaser, 1981 ; see Fig. 5 for an example from physics). Gick and Holyoak ( 1983 ) tried to get students to apply a rule from one problem to another problem that appeared different on the surface, but was structurally similar. They found that providing multiple examples helped with this transfer process compared to only using one example – especially when the examples provided had different surface details. More work is also needed to determine how many examples are sufficient for generalization to occur (and this, of course, will vary with contextual factors and individual differences). Further research on the continuum between concrete/specific examples and more abstract concepts would also be informative. That is, if an example is not concrete enough, it may be too difficult to understand. On the other hand, if the example is too concrete, that could be detrimental to generalization to the more abstract concept (although a diverse set of very concrete examples may be able to help with this). In fact, in a controversial article, Kaminski, Sloutsky, and Heckler ( 2008 ) claimed that abstract examples were more effective than concrete examples. Later rebuttals of this paper contested whether the abstract versus concrete distinction was clearly defined in the original study (see Reed, 2008 , for a collection of letters on the subject). This ideal point along the concrete-abstract continuum might also interact with development.
Finding teacher blog posts on concrete examples proved to be more difficult than for the other strategies in this review. One optimistic possibility is that teachers frequently use concrete examples in their teaching, and thus do not think of this as a specific contribution from cognitive psychology; the one blog post we were able to find that discussed concrete examples suggests that this might be the case (Boulton, 2016 ). The idea of “linking abstract concepts with concrete examples” is also covered in 25% of teacher-training textbooks used in the US, according to the report by Pomerance et al. ( 2016 ); this is the second most frequently covered of the six strategies, after “posing probing questions” (i.e., elaborative interrogation). A useful direction for future research would be to establish how teachers are using concrete examples in their practice, and whether we can make any suggestions for improvement based on research into the science of learning. For example, if two examples are better than one (Bauernschmidt, 2017 ), are additional examples also needed, or are there diminishing returns from providing more examples? And, how can teachers best ensure that concrete examples are consistent with prior knowledge (Reed, 2008 )?
Dual coding
Both the memory literature and folk psychology support the notion of visual examples being beneficial—the adage of “a picture is worth a thousand words” (traced back to an advertising slogan from the 1920s; Meider, 1990 ). Indeed, it is well-understood that more information can be conveyed through a simple illustration than through several paragraphs of text (e.g., Barker & Manji, 1989 ; Mayer & Gallini, 1990 ). Illustrations can be particularly helpful when the described concept involves several parts or steps and is intended for individuals with low prior knowledge (Eitel & Scheiter, 2015 ; Mayer & Gallini, 1990 ). Figure 6 provides a concrete example of this, illustrating how information can flow through neurons and synapses.
In addition to being able to convey information more succinctly, pictures are also more memorable than words (Paivio & Csapo, 1969 , 1973 ). In the memory literature, this is referred to as the picture superiority effect , and dual coding theory was developed in part to explain this effect. Dual coding follows from the notion of text being accompanied by complementary visual information to enhance learning. Paivio ( 1971 , 1986 ) proposed dual coding theory as a mechanistic account for the integration of multiple information “codes” to process information. In this theory, a code corresponds to a modal or otherwise distinct representation of a concept—e.g., “mental images for ‘book’ have visual, tactual, and other perceptual qualities similar to those evoked by the referent objects on which the images are based” (Clark & Paivio, 1991 , p. 152). Aylwin ( 1990 ) provides a clear example of how the word “dog” can evoke verbal, visual, and enactive representations (see Fig. 7 for a similar example for the word “SPOON”, based on Aylwin, 1990 (Fig. 2 ) and Madan & Singhal, 2012a (Fig. 3 )). Codes can also correspond to emotional properties (Clark & Paivio, 1991 ; Paivio, 2013 ). Clark and Paivio ( 1991 ) provide a thorough review of dual coding theory and its relation to education, while Paivio ( 2007 ) provides a comprehensive treatise on dual coding theory. Broadly, dual coding theory suggests that providing multiple representations of the same information enhances learning and memory, and that information that more readily evokes additional representations (through automatic imagery processes) receives a similar benefit.
Paivio and Csapo ( 1973 ) suggest that verbal and imaginal codes have independent and additive effects on memory recall. Using visuals to improve learning and memory has been particularly applied to vocabulary learning (Danan, 1992 ; Sadoski, 2005 ), but has also shown success in other domains such as in health care (Hartland, Biddle, & Fallacaro, 2008 ). To take advantage of dual coding, verbal information should be accompanied by a visual representation when possible. However, while the studies discussed all indicate that the use of multiple representations of information is favorable, it is important to acknowledge that each representation also increases cognitive load and can lead to over-saturation (Mayer & Moreno, 2003 ).
Given that pictures are generally remembered better than words, it is important to ensure that the pictures students are provided with are helpful and relevant to the content they are expected to learn. McNeill, Uttal, Jarvin, and Sternberg ( 2009 ) found that providing visual examples decreased conceptual errors. However, McNeill et al. also found that when students were given visually rich examples, they performed more poorly than students who were not given any visual example, suggesting that the visual details can at times become a distraction and hinder performance. Thus, it is important to consider that images used in teaching are clear and not ambiguous in their meaning (Schwartz, 2007 ).
Further broadening the scope of dual coding theory, Engelkamp and Zimmer ( 1984 ) suggest that motor movements, such as “turning the handle,” can provide an additional motor code that can improve memory, linking studies of motor actions (enactment) with dual coding theory (Clark & Paivio, 1991 ; Engelkamp & Cohen, 1991 ; Madan & Singhal, 2012c ). Indeed, enactment effects appear to primarily occur during learning, rather than during retrieval (Peterson & Mulligan, 2010 ). Along similar lines, Wammes, Meade, and Fernandes ( 2016 ) demonstrated that generating drawings can provide memory benefits beyond what could otherwise be explained by visual imagery, picture superiority, and other memory enhancing effects. Providing convergent evidence, even when overt motor actions are not critical in themselves, words representing functional objects have been shown to enhance later memory (Madan & Singhal, 2012b ; Montefinese, Ambrosini, Fairfield, & Mammarella, 2013 ). This indicates that motoric processes can improve memory similarly to visual imagery, similar to memory differences for concrete vs. abstract words. Further research suggests that automatic motor simulation for functional objects is likely responsible for this memory benefit (Madan, Chen, & Singhal, 2016 ).
When teachers combine visuals and words in their educational practice, however, they may not always be taking advantage of dual coding – at least, not in the optimal manner. For example, a recent discussion on Twitter centered around one teacher’s decision to have 7 th Grade students replace certain words in their science laboratory report with a picture of that word (e.g., the instructions read “using a syringe …” and a picture of a syringe replaced the word; Turner, 2016a ). Other teachers argued that this was not dual coding (Beaven, 2016 ; Williams, 2016 ), because there were no longer two different representations of the information. The first teacher maintained that dual coding was preserved, because this laboratory report with pictures was to be used alongside the original, fully verbal report (Turner, 2016b ). This particular implementation – having students replace individual words with pictures – has not been examined in the cognitive literature, presumably because no benefit would be expected. In any case, we need to be clearer about implementations for dual coding, and more research is needed to clarify how teachers can make use of the benefits conferred by multiple representations and picture superiority.
Critically, dual coding theory is distinct from the notion of “learning styles,” which describe the idea that individuals benefit from instruction that matches their modality preference. While this idea is pervasive and individuals often subjectively feel that they have a preference, evidence indicates that the learning styles theory is not supported by empirical findings (e.g., Kavale, Hirshoren, & Forness, 1998 ; Pashler, McDaniel, Rohrer, & Bjork, 2008 ; Rohrer & Pashler, 2012 ). That is, there is no evidence that instructing students in their preferred learning style leads to an overall improvement in learning (the “meshing” hypothesis). Moreover, learning styles have come to be described as a myth or urban legend within psychology (Coffield, Moseley, Hall, & Ecclestone, 2004 ; Hattie & Yates, 2014 ; Kirschner & van Merriënboer, 2013 ; Kirschner, 2017 ); skepticism about learning styles is a common stance amongst evidence-informed teachers (e.g., Saunders, 2016 ). Providing evidence against the notion of learning styles, Kraemer, Rosenberg, and Thompson-Schill ( 2009 ) found that individuals who scored as “verbalizers” and “visualizers” did not perform any better on experimental trials matching their preference. Instead, it has recently been shown that learning through one’s preferred learning style is associated with elevated subjective judgements of learning, but not objective performance (Knoll, Otani, Skeel, & Van Horn, 2017 ). In contrast to learning styles, dual coding is based on providing additional, complementary forms of information to enhance learning, rather than tailoring instruction to individuals’ preferences.
Genuine educational environments present many opportunities for combining the strategies outlined above. Spacing can be particularly potent for learning if it is combined with retrieval practice. The additive benefits of retrieval practice and spacing can be gained by engaging in retrieval practice multiple times (also known as distributed practice; see Cepeda et al., 2006 ). Interleaving naturally entails spacing if students interleave old and new material. Concrete examples can be both verbal and visual, making use of dual coding. In addition, the strategies of elaboration, concrete examples, and dual coding all work best when used as part of retrieval practice. For example, in the concept-mapping studies mentioned above (Blunt & Karpicke, 2014 ; Karpicke, Blunt, et al., 2014 ), creating concept maps while looking at course materials (e.g., a textbook) was not as effective for later memory as creating concept maps from memory. When practicing elaborative interrogation, students can start off answering the “how” and “why” questions they pose for themselves using class materials, and work their way up to answering them from memory. And when interleaving different problem types, students should be practicing answering them rather than just looking over worked examples.
But while these ideas for strategy combinations have empirical bases, it has not yet been established whether the benefits of the strategies to learning are additive, super-additive, or, in some cases, incompatible. Thus, future research needs to (a) better formalize the definition of each strategy (particularly critical for elaboration and dual coding), (b) identify best practices for implementation in the classroom, (c) delineate the boundary conditions of each strategy, and (d) strategically investigate interactions between the six strategies we outlined in this manuscript.
Aleven, V. A., & Koedinger, K. R. (2002). An effective metacognitive strategy: learning by doing and explaining with a computer-based cognitive tutor. Cognitive Science, 26 , 147–179.
Article Google Scholar
Anderson, J. R. (1983). A spreading activation theory of memory. Journal of Verbal Learning and Verbal Behavior, 22 , 261–295.
Arnold, K. M., & McDermott, K. B. (2013). Test-potentiated learning: distinguishing between direct and indirect effects of tests. Journal of Experimental Psychology: Learning, Memory, and Cognition, 39 , 940–945.
PubMed Google Scholar
Aylwin, S. (1990). Imagery and affect: big questions, little answers. In P. J. Thompson, D. E. Marks, & J. T. E. Richardson (Eds.), Imagery: Current developments . New York: International Library of Psychology.
Google Scholar
Baldassari, M. J., & Kelley, M. (2012). Make’em laugh? The mnemonic effect of humor in a speech. Psi Chi Journal of Psychological Research, 17 , 2–9.
Barker, P. G., & Manji, K. A. (1989). Pictorial dialogue methods. International Journal of Man-Machine Studies, 31 , 323–347.
Bauernschmidt, A. (2017). GUEST POST: two examples are better than one. [Blog post]. The Learning Scientists Blog . Retrieved from http://www.learningscientists.org/blog/2017/5/30-1 . Accessed 25 Dec 2017.
Beaven, T. (2016). @doctorwhy @FurtherEdagogy @doc_kristy Right, I thought the whole point of dual coding was to use TWO codes: pics + words of the SAME info? [Tweet]. Retrieved from https://twitter.com/TitaBeaven/status/807504041341308929 . Accessed 25 Dec 2017.
Bellezza, F. S., Cheesman, F. L., & Reddy, B. G. (1977). Organization and semantic elaboration in free recall. Journal of Experimental Psychology: Human Learning and Memory, 3 , 539–550.
Benney, D. (2016). (Trying to apply) spacing in a content heavy subject [Blog post]. Retrieved from https://mrbenney.wordpress.com/2016/10/16/trying-to-apply-spacing-in-science/ . Accessed 25 Dec 2017.
Berry, D. C. (1983). Metacognitive experience and transfer of logical reasoning. Quarterly Journal of Experimental Psychology, 35A , 39–49.
Birnbaum, M. S., Kornell, N., Bjork, E. L., & Bjork, R. A. (2013). Why interleaving enhances inductive learning: the roles of discrimination and retrieval. Memory & Cognition, 41 , 392–402.
Bjork, R. A. (1999). Assessing our own competence: heuristics and illusions. In D. Gopher & A. Koriat (Eds.), Attention and peformance XVII. Cognitive regulation of performance: Interaction of theory and application (pp. 435–459). Cambridge, MA: MIT Press.
Bjork, R. A. (1994). Memory and metamemory considerations in the training of human beings. In J. Metcalfe & A. Shimamura (Eds.), Metacognition: Knowing about knowing (pp. 185–205). Cambridge, MA: MIT Press.
Bjork, R. A., & Bjork, E. L. (1992). A new theory of disuse and an old theory of stimulus fluctuation. From learning processes to cognitive processes: Essays in honor of William K. Estes, 2 , 35–67.
Bjork, E. L., & Bjork, R. A. (2011). Making things hard on yourself, but in a good way: creating desirable difficulties to enhance learning. Psychology and the real world: Essays illustrating fundamental contributions to society , 56–64.
Blunt, J. R., & Karpicke, J. D. (2014). Learning with retrieval-based concept mapping. Journal of Educational Psychology, 106 , 849–858.
Boulton, K. (2016). What does cognitive overload look like in the humanities? [Blog post]. Retrieved from https://educationechochamberuncut.wordpress.com/2016/03/05/what-does-cognitive-overload-look-like-in-the-humanities-kris-boulton-2/ . Accessed 25 Dec 2017.
Brown, P. C., Roediger, H. L., & McDaniel, M. A. (2014). Make it stick . Cambridge, MA: Harvard University Press.
Book Google Scholar
Butler, A. C. (2010). Repeated testing produces superior transfer of learning relative to repeated studying. Journal of Experimental Psychology: Learning, Memory, and Cognition, 36 , 1118–1133.
Caplan, J. B., & Madan, C. R. (2016). Word-imageability enhances association-memory by recruiting hippocampal activity. Journal of Cognitive Neuroscience, 28 , 1522–1538.
Article PubMed Google Scholar
Cepeda, N. J., Pashler, H., Vul, E., Wixted, J. T., & Rohrer, D. (2006). Distributed practice in verbal recall tasks: a review and quantitative synthesis. Psychological Bulletin, 132 , 354–380.
Cepeda, N. J., Vul, E., Rohrer, D., Wixted, J. T., & Pashler, H. (2008). Spacing effects in learning a temporal ridgeline of optimal retention. Psychological Science, 19 , 1095–1102.
Chi, M. T., De Leeuw, N., Chiu, M. H., & LaVancher, C. (1994). Eliciting self-explanations improves understanding. Cognitive Science, 18 , 439–477.
Chi, M. T., Feltovich, P. J., & Glaser, R. (1981). Categorization and representation of physics problems by experts and novices. Cognitive Science, 5 , 121–152.
CIFE. (2012). No January A level and other changes. Retrieved from http://www.cife.org.uk/cife-general-news/no-january-a-level-and-other-changes/ . Accessed 25 Dec 2017.
Clark, D. (2016). One book on learning that every teacher, lecturer & trainer should read (7 reasons) [Blog post]. Retrieved from http://donaldclarkplanb.blogspot.com/2016/03/one-book-on-learning-that-every-teacher.html . Accessed 25 Dec 2017.
Clark, J. M., & Paivio, A. (1991). Dual coding theory and education. Educational Psychology Review, 3 , 149–210.
Class Teaching. (2013). Deep questioning [Blog post]. Retrieved from https://classteaching.wordpress.com/2013/07/12/deep-questioning/ . Accessed 25 Dec 2017.
Clinton, V., Alibali, M. W., & Nathan, M. J. (2016). Learning about posterior probability: do diagrams and elaborative interrogation help? The Journal of Experimental Education, 84 , 579–599.
Coffield, F., Moseley, D., Hall, E., & Ecclestone, K. (2004). Learning styles and pedagogy in post-16 learning: a systematic and critical review . London: Learning & Skills Research Centre.
Cohen, R. L. (1981). On the generality of some memory laws. Scandinavian Journal of Psychology, 22 , 267–281.
Cooper, H. (1989). Synthesis of research on homework. Educational Leadership, 47 , 85–91.
Corbett, A. T., Reed, S. K., Hoffmann, R., MacLaren, B., & Wagner, A. (2010). Interleaving worked examples and cognitive tutor support for algebraic modeling of problem situations. In Proceedings of the Thirty-Second Annual Meeting of the Cognitive Science Society (pp. 2882–2887).
Cox, D. (2015). No stakes testing – not telling students their results [Blog post]. Retrieved from https://missdcoxblog.wordpress.com/2015/06/06/no-stakes-testing-not-telling-students-their-results/ . Accessed 25 Dec 2017.
Cox, D. (2016a). Ditch revision. Teach it well [Blog post]. Retrieved from https://missdcoxblog.wordpress.com/2016/01/09/ditch-revision-teach-it-well/ . Accessed 25 Dec 2017.
Cox, D. (2016b). ‘They need to remember this in three years time’: spacing & interleaving for the new GCSEs [Blog post]. Retrieved from https://missdcoxblog.wordpress.com/2016/03/25/they-need-to-remember-this-in-three-years-time-spacing-interleaving-for-the-new-gcses/ . Accessed 25 Dec 2017.
Craik, F. I. (2002). Levels of processing: past, present… future? Memory, 10 , 305–318.
Craik, F. I., & Lockhart, R. S. (1972). Levels of processing: a framework for memory research. Journal of Verbal Learning and Verbal Behavior, 11 , 671–684.
Danan, M. (1992). Reversed subtitling and dual coding theory: new directions for foreign language instruction. Language Learning, 42 , 497–527.
Dettmers, S., Trautwein, U., & Lüdtke, O. (2009). The relationship between homework time and achievement is not universal: evidence from multilevel analyses in 40 countries. School Effectiveness and School Improvement, 20 , 375–405.
Dirkx, K. J., Kester, L., & Kirschner, P. A. (2014). The testing effect for learning principles and procedures from texts. The Journal of Educational Research, 107 , 357–364.
Dunlosky, J. (2013). Strengthening the student toolbox: study strategies to boost learning. American Educator, 37 (3), 12–21.
Dunlosky, J., Rawson, K. A., Marsh, E. J., Nathan, M. J., & Willingham, D. T. (2013). Improving students’ learning with effective learning techniques: promising directions from cognitive and educational psychology. Psychological Science in the Public Interest, 14 , 4–58.
Ebbinghaus, H. (1913). Memory (HA Ruger & CE Bussenius, Trans.). New York: Columbia University, Teachers College. (Original work published 1885) . Retrieved from http://psychclassics.yorku.ca/Ebbinghaus/memory8.htm . Accessed 25 Dec 2017.
Eglington, L. G., & Kang, S. H. (2016). Retrieval practice benefits deductive inference. Educational Psychology Review , 1–14.
Eitel, A., & Scheiter, K. (2015). Picture or text first? Explaining sequential effects when learning with pictures and text. Educational Psychology Review, 27 , 153–180.
Engelkamp, J., & Cohen, R. L. (1991). Current issues in memory of action events. Psychological Research, 53 , 175–182.
Engelkamp, J., & Zimmer, H. D. (1984). Motor programme information as a separable memory unit. Psychological Research, 46 , 283–299.
Fawcett, D. (2013). Can I be that little better at……using cognitive science/psychology/neurology to plan learning? [Blog post]. Retrieved from http://reflectionsofmyteaching.blogspot.com/2013/09/can-i-be-that-little-better-atusing.html . Accessed 25 Dec 2017.
Fiechter, J. L., & Benjamin, A. S. (2017). Diminishing-cues retrieval practice: a memory-enhancing technique that works when regular testing doesn’t. Psychonomic Bulletin & Review , 1–9.
Firth, J. (2016). Spacing in teaching practice [Blog post]. Retrieved from http://www.learningscientists.org/blog/2016/4/12-1 . Accessed 25 Dec 2017.
Fordham, M. [mfordhamhistory]. (2016). Is there a meaningful distinction in psychology between ‘thinking’ & ‘critical thinking’? [Tweet]. Retrieved from https://twitter.com/mfordhamhistory/status/809525713623781377 . Accessed 25 Dec 2017.
Fritz, C. O., Morris, P. E., Nolan, D., & Singleton, J. (2007). Expanding retrieval practice: an effective aid to preschool children’s learning. The Quarterly Journal of Experimental Psychology, 60 , 991–1004.
Gates, A. I. (1917). Recitation as a factory in memorizing. Archives of Psychology, 6.
Gick, M. L., & Holyoak, K. J. (1983). Schema induction and analogical transfer. Cognitive Psychology, 15 , 1–38.
Gorman, A. M. (1961). Recognition memory for nouns as a function of abstractedness and frequency. Journal of Experimental Psychology, 61 , 23–39.
Hainselin, M., Picard, L., Manolli, P., Vankerkore-Candas, S., & Bourdin, B. (2017). Hey teacher, don’t leave them kids alone: action is better for memory than reading. Frontiers in Psychology , 8 .
Harp, S. F., & Mayer, R. E. (1998). How seductive details do their damage. Journal of Educational Psychology, 90 , 414–434.
Hartland, W., Biddle, C., & Fallacaro, M. (2008). Audiovisual facilitation of clinical knowledge: A paradigm for dispersed student education based on Paivio’s dual coding theory. AANA Journal, 76 , 194–198.
Hattie, J., & Yates, G. (2014). Visible learning and the science of how we learn . New York: Routledge.
Hausman, H., & Kornell, N. (2014). Mixing topics while studying does not enhance learning. Journal of Applied Research in Memory and Cognition, 3 , 153–160.
Hinze, S. R., & Rapp, D. N. (2014). Retrieval (sometimes) enhances learning: performance pressure reduces the benefits of retrieval practice. Applied Cognitive Psychology, 28 , 597–606.
Hirshman, E. (2001). Elaboration in memory. In N. J. Smelser & P. B. Baltes (Eds.), International encyclopedia of the social & behavioral sciences (pp. 4369–4374). Oxford: Pergamon.
Chapter Google Scholar
Hobbiss, M. (2016). Make it meaningful! Elaboration [Blog post]. Retrieved from https://hobbolog.wordpress.com/2016/06/09/make-it-meaningful-elaboration/ . Accessed 25 Dec 2017.
Jones, F. (2016). Homework – is it really that useless? [Blog post]. Retrieved from http://www.learningscientists.org/blog/2016/4/5-1 . Accessed 25 Dec 2017.
Kaminski, J. A., & Sloutsky, V. M. (2013). Extraneous perceptual information interferes with children’s acquisition of mathematical knowledge. Journal of Educational Psychology, 105 (2), 351–363.
Kaminski, J. A., Sloutsky, V. M., & Heckler, A. F. (2008). The advantage of abstract examples in learning math. Science, 320 , 454–455.
Kang, S. H. (2016). Spaced repetition promotes efficient and effective learning policy implications for instruction. Policy Insights from the Behavioral and Brain Sciences, 3 , 12–19.
Kang, S. H. K., McDermott, K. B., & Roediger, H. L. (2007). Test format and corrective feedback modify the effects of testing on long-term retention. European Journal of Cognitive Psychology, 19 , 528–558.
Karpicke, J. D., & Aue, W. R. (2015). The testing effect is alive and well with complex materials. Educational Psychology Review, 27 , 317–326.
Karpicke, J. D., Blunt, J. R., Smith, M. A., & Karpicke, S. S. (2014). Retrieval-based learning: The need for guided retrieval in elementary school children. Journal of Applied Research in Memory and Cognition, 3 , 198–206.
Karpicke, J. D., Lehman, M., & Aue, W. R. (2014). Retrieval-based learning: an episodic context account. In B. H. Ross (Ed.), Psychology of Learning and Motivation (Vol. 61, pp. 237–284). San Diego, CA: Elsevier Academic Press.
Karpicke, J. D., Blunt, J. R., & Smith, M. A. (2016). Retrieval-based learning: positive effects of retrieval practice in elementary school children. Frontiers in Psychology, 7 .
Kavale, K. A., Hirshoren, A., & Forness, S. R. (1998). Meta-analytic validation of the Dunn and Dunn model of learning-style preferences: a critique of what was Dunn. Learning Disabilities Research & Practice, 13 , 75–80.
Khanna, M. M. (2015). Ungraded pop quizzes: test-enhanced learning without all the anxiety. Teaching of Psychology, 42 , 174–178.
Kirby, J. (2014). One scientific insight for curriculum design [Blog post]. Retrieved from https://pragmaticreform.wordpress.com/2014/05/05/scientificcurriculumdesign/ . Accessed 25 Dec 2017.
Kirschner, P. A. (2017). Stop propagating the learning styles myth. Computers & Education, 106 , 166–171.
Kirschner, P. A., & van Merriënboer, J. J. G. (2013). Do learners really know best? Urban legends in education. Educational Psychologist, 48 , 169–183.
Knoll, A. R., Otani, H., Skeel, R. L., & Van Horn, K. R. (2017). Learning style, judgments of learning, and learning of verbal and visual information. British Journal of Psychology, 108 , 544-563.
Kornell, N., & Bjork, R. A. (2008). Learning concepts and categories is spacing the “enemy of induction”? Psychological Science, 19 , 585–592.
Kornell, N., & Finn, B. (2016). Self-regulated learning: an overview of theory and data. In J. Dunlosky & S. Tauber (Eds.), The Oxford Handbook of Metamemory (pp. 325–340). New York: Oxford University Press.
Kornell, N., Klein, P. J., & Rawson, K. A. (2015). Retrieval attempts enhance learning, but retrieval success (versus failure) does not matter. Journal of Experimental Psychology: Learning, Memory, and Cognition, 41 , 283–294.
Kraemer, D. J. M., Rosenberg, L. M., & Thompson-Schill, S. L. (2009). The neural correlates of visual and verbal cognitive styles. Journal of Neuroscience, 29 , 3792–3798.
Article PubMed PubMed Central Google Scholar
Kraft, N. (2015). Spaced practice and repercussions for teaching. Retrieved from http://nathankraft.blogspot.com/2015/08/spaced-practice-and-repercussions-for.html . Accessed 25 Dec 2017.
Learning Scientists. (2016a). Weekly Digest #3: How teachers implement interleaving in their curriculum [Blog post]. Retrieved from http://www.learningscientists.org/blog/2016/3/28/weekly-digest-3 . Accessed 25 Dec 2017.
Learning Scientists. (2016b). Weekly Digest #13: how teachers implement retrieval in their classrooms [Blog post]. Retrieved from http://www.learningscientists.org/blog/2016/6/5/weekly-digest-13 . Accessed 25 Dec 2017.
Learning Scientists. (2016c). Weekly Digest #40: teachers’ implementation of principles from “Make It Stick” [Blog post]. Retrieved from http://www.learningscientists.org/blog/2016/12/18-1 . Accessed 25 Dec 2017.
Learning Scientists. (2017). Weekly Digest #54: is there an app for that? Studying 2.0 [Blog post]. Retrieved from http://www.learningscientists.org/blog/2017/4/9/weekly-digest-54 . Accessed 25 Dec 2017.
LeFevre, J.-A., & Dixon, P. (1986). Do written instructions need examples? Cognition and Instruction, 3 , 1–30.
Lew, K., Fukawa-Connelly, T., Mejí-Ramos, J. P., & Weber, K. (2016). Lectures in advanced mathematics: Why students might not understand what the mathematics professor is trying to convey. Journal of Research in Mathematics Education, 47 , 162–198.
Lindsey, R. V., Shroyer, J. D., Pashler, H., & Mozer, M. C. (2014). Improving students’ long-term knowledge retention through personalized review. Psychological Science, 25 , 639–647.
Lipko-Speed, A., Dunlosky, J., & Rawson, K. A. (2014). Does testing with feedback help grade-school children learn key concepts in science? Journal of Applied Research in Memory and Cognition, 3 , 171–176.
Lockhart, R. S., & Craik, F. I. (1990). Levels of processing: a retrospective commentary on a framework for memory research. Canadian Journal of Psychology, 44 , 87–112.
Lovell, O. (2017). How do we know what to put on the quiz? [Blog Post]. Retrieved from http://www.ollielovell.com/olliesclassroom/know-put-quiz/ . Accessed 25 Dec 2017.
Luehmann, A. L. (2008). Using blogging in support of teacher professional identity development: a case study. The Journal of the Learning Sciences, 17 , 287–337.
Madan, C. R., Glaholt, M. G., & Caplan, J. B. (2010). The influence of item properties on association-memory. Journal of Memory and Language, 63 , 46–63.
Madan, C. R., & Singhal, A. (2012a). Motor imagery and higher-level cognition: four hurdles before research can sprint forward. Cognitive Processing, 13 , 211–229.
Madan, C. R., & Singhal, A. (2012b). Encoding the world around us: motor-related processing influences verbal memory. Consciousness and Cognition, 21 , 1563–1570.
Madan, C. R., & Singhal, A. (2012c). Using actions to enhance memory: effects of enactment, gestures, and exercise on human memory. Frontiers in Psychology, 3 .
Madan, C. R., Chen, Y. Y., & Singhal, A. (2016). ERPs differentially reflect automatic and deliberate processing of the functional manipulability of objects. Frontiers in Human Neuroscience, 10 .
Mandler, G. (1979). Organization and repetition: organizational principles with special reference to rote learning. In L. G. Nilsson (Ed.), Perspectives on Memory Research (pp. 293–327). New York: Academic Press.
Marsh, E. J., Fazio, L. K., & Goswick, A. E. (2012). Memorial consequences of testing school-aged children. Memory, 20 , 899–906.
Mayer, R. E., & Gallini, J. K. (1990). When is an illustration worth ten thousand words? Journal of Educational Psychology, 82 , 715–726.
Mayer, R. E., & Moreno, R. (2003). Nine ways to reduce cognitive load in multimedia learning. Educational Psychologist, 38 , 43–52.
McDaniel, M. A., & Donnelly, C. M. (1996). Learning with analogy and elaborative interrogation. Journal of Educational Psychology, 88 , 508–519.
McDaniel, M. A., Thomas, R. C., Agarwal, P. K., McDermott, K. B., & Roediger, H. L. (2013). Quizzing in middle-school science: successful transfer performance on classroom exams. Applied Cognitive Psychology, 27 , 360–372.
McDermott, K. B., Agarwal, P. K., D’Antonio, L., Roediger, H. L., & McDaniel, M. A. (2014). Both multiple-choice and short-answer quizzes enhance later exam performance in middle and high school classes. Journal of Experimental Psychology: Applied, 20 , 3–21.
McHugh, A. (2013). High-stakes tests: bad for students, teachers, and education in general [Blog post]. Retrieved from https://teacherbiz.wordpress.com/2013/07/01/high-stakes-tests-bad-for-students-teachers-and-education-in-general/ . Accessed 25 Dec 2017.
McNeill, N. M., Uttal, D. H., Jarvin, L., & Sternberg, R. J. (2009). Should you show me the money? Concrete objects both hurt and help performance on mathematics problems. Learning and Instruction, 19 , 171–184.
Meider, W. (1990). “A picture is worth a thousand words”: from advertising slogan to American proverb. Southern Folklore, 47 , 207–225.
Michaela Community School. (2014). Homework. Retrieved from http://mcsbrent.co.uk/homework-2/ . Accessed 25 Dec 2017.
Montefinese, M., Ambrosini, E., Fairfield, B., & Mammarella, N. (2013). The “subjective” pupil old/new effect: is the truth plain to see? International Journal of Psychophysiology, 89 , 48–56.
O’Neil, H. F., Chung, G. K., Kerr, D., Vendlinski, T. P., Buschang, R. E., & Mayer, R. E. (2014). Adding self-explanation prompts to an educational computer game. Computers In Human Behavior, 30 , 23–28.
Overoye, A. L., & Storm, B. C. (2015). Harnessing the power of uncertainty to enhance learning. Translational Issues in Psychological Science, 1 , 140–148.
Paivio, A. (1971). Imagery and verbal processes . New York: Holt, Rinehart and Winston.
Paivio, A. (1986). Mental representations: a dual coding approach . New York: Oxford University Press.
Paivio, A. (2007). Mind and its evolution: a dual coding theoretical approach . Mahwah: Erlbaum.
Paivio, A. (2013). Dual coding theory, word abstractness, and emotion: a critical review of Kousta et al. (2011). Journal of Experimental Psychology: General, 142 , 282–287.
Paivio, A., & Csapo, K. (1969). Concrete image and verbal memory codes. Journal of Experimental Psychology, 80 , 279–285.
Paivio, A., & Csapo, K. (1973). Picture superiority in free recall: imagery or dual coding? Cognitive Psychology, 5 , 176–206.
Paivio, A., Walsh, M., & Bons, T. (1994). Concreteness effects on memory: when and why? Journal of Experimental Psychology: Learning, Memory, and Cognition, 20 , 1196–1204.
Pashler, H., McDaniel, M., Rohrer, D., & Bjork, R. (2008). Learning styles: concepts and evidence. Psychological Science in the Public Interest, 9 , 105–119.
Pashler, H., Bain, P. M., Bottge, B. A., Graesser, A., Koedinger, K., McDaniel, M., & Metcalfe, J. (2007). Organizing instruction and study to improve student learning. IES practice guide. NCER 2007–2004. National Center for Education Research .
Patel, R., Liu, R., & Koedinger, K. (2016). When to block versus interleave practice? Evidence against teaching fraction addition before fraction multiplication. In Proceedings of the 38th Annual Meeting of the Cognitive Science Society, Philadelphia, PA .
Penfound, B. (2017). Journey to interleaved practice #2 [Blog Post]. Retrieved from https://fullstackcalculus.com/2017/02/03/journey-to-interleaved-practice-2/ . Accessed 25 Dec 2017.
Penfound, B. [BryanPenfound]. (2016). Does blocked practice/learning lessen cognitive load? Does interleaved practice/learning provide productive struggle? [Tweet]. Retrieved from https://twitter.com/BryanPenfound/status/808759362244087808 . Accessed 25 Dec 2017.
Peterson, D. J., & Mulligan, N. W. (2010). Enactment and retrieval. Memory & Cognition, 38 , 233–243.
Picciotto, H. (2009). Lagging homework [Blog post]. Retrieved from http://blog.mathedpage.org/2013/06/lagging-homework.html . Accessed 25 Dec 2017.
Pomerance, L., Greenberg, J., & Walsh, K. (2016). Learning about learning: what every teacher needs to know. Retrieved from http://www.nctq.org/dmsView/Learning_About_Learning_Report . Accessed 25 Dec 2017.
Postman, L. (1976). Methodology of human learning. In W. K. Estes (Ed.), Handbook of learning and cognitive processes (Vol. 3). Hillsdale: Erlbaum.
Pressley, M., McDaniel, M. A., Turnure, J. E., Wood, E., & Ahmad, M. (1987). Generation and precision of elaboration: effects on intentional and incidental learning. Journal of Experimental Psychology: Learning, Memory, and Cognition, 13 , 291–300.
Reed, S. K. (2008). Concrete examples must jibe with experience. Science, 322 , 1632–1633.
researchED. (2013). How it all began. Retrieved from http://www.researched.org.uk/about/our-story/ . Accessed 25 Dec 2017.
Ritchie, S. J., Della Sala, S., & McIntosh, R. D. (2013). Retrieval practice, with or without mind mapping, boosts fact learning in primary school children. PLoS One, 8 (11), e78976.
Rittle-Johnson, B. (2006). Promoting transfer: effects of self-explanation and direct instruction. Child Development, 77 , 1–15.
Roediger, H. L. (1985). Remembering Ebbinghaus. [Retrospective review of the book On Memory , by H. Ebbinghaus]. Contemporary Psychology, 30 , 519–523.
Roediger, H. L. (2013). Applying cognitive psychology to education translational educational science. Psychological Science in the Public Interest, 14 , 1–3.
Roediger, H. L., & Karpicke, J. D. (2006). The power of testing memory: basic research and implications for educational practice. Perspectives on Psychological Science, 1 , 181–210.
Roediger, H. L., Putnam, A. L., & Smith, M. A. (2011). Ten benefits of testing and their applications to educational practice. In J. Mester & B. Ross (Eds.), The psychology of learning and motivation: cognition in education (pp. 1–36). Oxford: Elsevier.
Roediger, H. L., Finn, B., & Weinstein, Y. (2012). Applications of cognitive science to education. In Della Sala, S., & Anderson, M. (Eds.), Neuroscience in education: the good, the bad, and the ugly . Oxford, UK: Oxford University Press.
Roelle, J., & Berthold, K. (2017). Effects of incorporating retrieval into learning tasks: the complexity of the tasks matters. Learning and Instruction, 49 , 142–156.
Rohrer, D. (2012). Interleaving helps students distinguish among similar concepts. Educational Psychology Review, 24(3), 355–367.
Rohrer, D., Dedrick, R. F., & Stershic, S. (2015). Interleaved practice improves mathematics learning. Journal of Educational Psychology, 107 , 900–908.
Rohrer, D., & Pashler, H. (2012). Learning styles: Where’s the evidence? Medical Education, 46 , 34–35.
Rohrer, D., & Taylor, K. (2007). The shuffling of mathematics problems improves learning. Instructional Science, 35 , 481–498.
Rose, N. (2014). Improving the effectiveness of homework [Blog post]. Retrieved from https://evidenceintopractice.wordpress.com/2014/03/20/improving-the-effectiveness-of-homework/ . Accessed 25 Dec 2017.
Sadoski, M. (2005). A dual coding view of vocabulary learning. Reading & Writing Quarterly, 21 , 221–238.
Saunders, K. (2016). It really is time we stopped talking about learning styles [Blog post]. Retrieved from http://martingsaunders.com/2016/10/it-really-is-time-we-stopped-talking-about-learning-styles/ . Accessed 25 Dec 2017.
Schwartz, D. (2007). If a picture is worth a thousand words, why are you reading this essay? Social Psychology Quarterly, 70 , 319–321.
Shumaker, H. (2016). Homework is wrecking our kids: the research is clear, let’s ban elementary homework. Salon. Retrieved from http://www.salon.com/2016/03/05/homework_is_wrecking_our_kids_the_research_is_clear_lets_ban_elementary_homework . Accessed 25 Dec 2017.
Smith, A. M., Floerke, V. A., & Thomas, A. K. (2016). Retrieval practice protects memory against acute stress. Science, 354 , 1046–1048.
Smith, M. A., Blunt, J. R., Whiffen, J. W., & Karpicke, J. D. (2016). Does providing prompts during retrieval practice improve learning? Applied Cognitive Psychology, 30 , 784–802.
Smith, M. A., & Karpicke, J. D. (2014). Retrieval practice with short-answer, multiple-choice, and hybrid formats. Memory, 22 , 784–802.
Smith, M. A., Roediger, H. L., & Karpicke, J. D. (2013). Covert retrieval practice benefits retention as much as overt retrieval practice. Journal of Experimental Psychology: Learning, Memory, and Cognition, 39 , 1712–1725.
Son, J. Y., & Rivas, M. J. (2016). Designing clicker questions to stimulate transfer. Scholarship of Teaching and Learning in Psychology, 2 , 193–207.
Szpunar, K. K., Khan, N. Y., & Schacter, D. L. (2013). Interpolated memory tests reduce mind wandering and improve learning of online lectures. Proceedings of the National Academy of Sciences, 110 , 6313–6317.
Thomson, R., & Mehring, J. (2016). Better vocabulary study strategies for long-term learning. Kwansei Gakuin University Humanities Review, 20 , 133–141.
Trafton, J. G., & Reiser, B. J. (1993). Studying examples and solving problems: contributions to skill acquisition . Technical report, Naval HCI Research Lab, Washington, DC, USA.
Tran, R., Rohrer, D., & Pashler, H. (2015). Retrieval practice: the lack of transfer to deductive inferences. Psychonomic Bulletin & Review, 22 , 135–140.
Turner, K. [doc_kristy]. (2016a). My dual coding (in red) and some y8 work @AceThatTest they really enjoyed practising the technique [Tweet]. Retrieved from https://twitter.com/doc_kristy/status/807220355395977216 . Accessed 25 Dec 2017.
Turner, K. [doc_kristy]. (2016b). @FurtherEdagogy @doctorwhy their work is revision work, they already have the words on a different page, to compliment not replace [Tweet]. Retrieved from https://twitter.com/doc_kristy/status/807360265100599301 . Accessed 25 Dec 2017.
Valle, A., Regueiro, B., Núñez, J. C., Rodríguez, S., Piñeiro, I., & Rosário, P. (2016). Academic goals, student homework engagement, and academic achievement in elementary school. Frontiers in Psychology, 7 .
Van Gog, T., & Sweller, J. (2015). Not new, but nearly forgotten: the testing effect decreases or even disappears as the complexity of learning materials increases. Educational Psychology Review, 27 , 247–264.
Wammes, J. D., Meade, M. E., & Fernandes, M. A. (2016). The drawing effect: evidence for reliable and robust memory benefits in free recall. Quarterly Journal of Experimental Psychology, 69 , 1752–1776.
Weinstein, Y., Gilmore, A. W., Szpunar, K. K., & McDermott, K. B. (2014). The role of test expectancy in the build-up of proactive interference in long-term memory. Journal of Experimental Psychology: Learning, Memory, and Cognition, 40 , 1039–1048.
Weinstein, Y., Nunes, L. D., & Karpicke, J. D. (2016). On the placement of practice questions during study. Journal of Experimental Psychology: Applied, 22 , 72–84.
Weinstein, Y., & Weinstein-Jones, F. (2017). Topic and quiz spacing spreadsheet: a planning tool for teachers [Blog Post]. Retrieved from http://www.learningscientists.org/blog/2017/5/11-1 . Accessed 25 Dec 2017.
Weinstein-Jones, F., & Weinstein, Y. (2017). Topic spacing spreadsheet for teachers [Excel macro]. Zenodo. http://doi.org/10.5281/zenodo.573764 . Accessed 25 Dec 2017.
Williams, D. [FurtherEdagogy]. (2016). @doctorwhy @doc_kristy word accompanying the visual? I’m unclear how removing words benefit? Would a flow chart better suit a scientific exp? [Tweet]. Retrieved from https://twitter.com/FurtherEdagogy/status/807356800509104128 . Accessed 25 Dec 2017.
Wood, B. (2017). And now for something a little bit different….[Blog post]. Retrieved from https://justateacherstandinginfrontofaclass.wordpress.com/2017/04/20/and-now-for-something-a-little-bit-different/ . Accessed 25 Dec 2017.
Wooldridge, C. L., Bugg, J. M., McDaniel, M. A., & Liu, Y. (2014). The testing effect with authentic educational materials: a cautionary note. Journal of Applied Research in Memory and Cognition, 3 , 214–221.
Young, C. (2016). Mini-tests. Retrieved from https://colleenyoung.wordpress.com/revision-activities/mini-tests/ . Accessed 25 Dec 2017.
Download references
Acknowledgements
Not applicable.
YW and MAS were partially supported by a grant from The IDEA Center.
Availability of data and materials
Author information, authors and affiliations.
Department of Psychology, University of Massachusetts Lowell, Lowell, MA, USA
Yana Weinstein
Department of Psychology, Boston College, Chestnut Hill, MA, USA
Christopher R. Madan
School of Psychology, University of Nottingham, Nottingham, UK
Department of Psychology, Rhode Island College, Providence, RI, USA
Megan A. Sumeracki
You can also search for this author in PubMed Google Scholar
Contributions
YW took the lead on writing the “Spaced practice”, “Interleaving”, and “Elaboration” sections. CRM took the lead on writing the “Concrete examples” and “Dual coding” sections. MAS took the lead on writing the “Retrieval practice” section. All authors edited each others’ sections. All authors were involved in the conception and writing of the manuscript. All authors gave approval of the final version.
Corresponding author
Correspondence to Yana Weinstein .
Ethics declarations
Ethics approval and consent to participate, consent for publication, competing interests.
YW and MAS run a blog, “The Learning Scientists Blog”, which is cited in the tutorial review. The blog does not make money. Free resources on the strategies described in this tutorial review are provided on the blog. Occasionally, YW and MAS are invited by schools/school districts to present research findings from cognitive psychology applied to education.
Publisher’s Note
Springer Nature remains neutral with regard to jurisdictional claims in published maps and institutional affiliations.
Rights and permissions
Open Access This article is distributed under the terms of the Creative Commons Attribution 4.0 International License ( http://creativecommons.org/licenses/by/4.0/ ), which permits unrestricted use, distribution, and reproduction in any medium, provided you give appropriate credit to the original author(s) and the source, provide a link to the Creative Commons license, and indicate if changes were made.
Reprints and permissions
About this article
Cite this article.
Weinstein, Y., Madan, C.R. & Sumeracki, M.A. Teaching the science of learning. Cogn. Research 3 , 2 (2018). https://doi.org/10.1186/s41235-017-0087-y
Download citation
Received : 20 December 2016
Accepted : 02 December 2017
Published : 24 January 2018
DOI : https://doi.org/10.1186/s41235-017-0087-y
Share this article
Anyone you share the following link with will be able to read this content:
Sorry, a shareable link is not currently available for this article.
Provided by the Springer Nature SharedIt content-sharing initiative
Research Supporting Proficiency-Based Learning: Learning Standards
When educators talk about “proficiency-based learning,” they are referring to a variety of diverse instructional practices—many of which have been used by the world’s best schools and teachers for decades—and to organizational structures that support or facilitate the application of those practices in schools. Proficiency-based learning may take different forms from school to school—there is no universal model or approach—and educators may use some or all of the beliefs and practices of proficiency-based learning identified by the Great Schools Partnership.
On this page, we have provided a selection of statements and references that support and describe one foundational feature of proficiency-based learning systems, Learning Standards . In a few cases, we have also included additional explanation to help readers better understand the statements or the studies from which they were excerpted. The list is not intended to be either comprehensive or authoritative—our goal is merely to give school leaders and educators a brief, accessible introduction to available research.
“Clear learning goals help students learn better (Seidel, Rimmele, & Prenzel, 2005). When students understand exactly what they’re supposed to learn and what their work will look like when they learn it, they’re better able to monitor and adjust their work, select effective strategies, and connect current work to prior learning (Black, Harrison, Lee, Marshall, & Wiliam, 2004; Moss, Brookhart, & Long, 2011). This point has been demonstrated for all age groups, from young children (Higgins, Harris, & Kuehn, 1994) through high school students (Ross & Starling, 2008), and in a variety of subjects—in writing (Andrade, Du, & Mycek, 2010); mathematics (Ross, Hogaboam-Gray, & Rolheiser, 2002); and social studies (Ross & Starling, 2008). The important point here is that students should have clear goals. If the teacher is the only one who understands where learning should be headed, students are flying blind. In all the studies we just cited, students were taught the learning goals and criteria for success, and that’s what made the difference.” —Brookhart, S. M., & Moss, C. M. (2014, October). Learning targets on parade. Educational Leadership , 72 (7), 28–33.
“The most effective teaching and the most meaningful student learning happen when teachers design the right learning target for today’s lesson and use it along with their students to aim for and assess understanding. Our theory grew from continuous research with educators focused on raising student achievement through formative assessment processes (e.g., Brookhart, Moss, & Long, 2009, 2010, 2011; Moss, Brookhart, & Long 2011a, 2011b, 2011c). What we discovered and continue to refine is an understanding of the central role that learning targets play in schools. Learning targets are student-friendly descriptions—via words, pictures, actions, or some combination of the three—of what you intend students to learn or accomplish in a given lesson. When shared meaningfully, they become actual targets that students can see and direct their efforts toward. They also serve as targets for the adults in the school whose responsibility it is to plan, monitor, assess, and improve the quality of learning opportunities to raise the achievement of all students.” —Brookhart, S. M., & Moss, C. M. (2012). Learning targets: Helping students aim for understanding in today’s lesson . Alexandria, VA: Association for Supervision and Curriculum Development.
“Setting objectives and providing feedback work in tandem. Teachers need to identify success criteria for learning objectives so students know when they have achieved those objectives (Hattie & Timperley, 2007). Similarly, feedback should be provided for tasks that are related to the learning objectives; this way, students understand the purpose of the work they are asked to do, build a coherent understanding of a content domain, and develop high levels of skill in a specific domain.” —Dean, C. B., Hubbell, E. R., Pitler, H., & Stone, B. (2012). Classroom instruction that works: Research-based strategies for increasing student achievement . Alexandria, VA: Association for Supervision and Curriculum Development.
“Setting objectives is the process of establishing a direction to guide learning (Pintrich & Schunk, 2002). When teachers communicate objectives for student learning, students can see more easily the connections between what they are doing in class and what they are supposed to learn. They can gauge their starting point in relation to the learning objectives and determine what they need to pay attention to and where they might need help from the teacher or others. This clarity helps decrease anxiety about their ability to succeed. In addition, students build intrinsic motivation when they set personal learning objectives.” —Dean, C. B., Hubbell, E. R., Pitler, H., & Stone, B. (2012). Classroom instruction that works: Research-based strategies for increasing student achievement . Alexandria, VA: Association for Supervision and Curriculum Development.
“Providing specific feedback that helps students know how to improve their performance requires teachers to identify and understand the learning objectives (Stiggins, 2001). If teachers do not understand the learning objectives, it is difficult for them to provide students with information about what good performance or high-quality work looks like…. Effective feedback should also provide information about how close students come to meeting the criterion and details about what they need to do to attain the next level of performance (Shirbagi, 2007; Shute, 2008). Teachers can provide elaboration in the form of worked examples, questions, or prompts—such as ‘What’s this problem all about?’—or as information about the correct answer (Kramarski & Zeichner, 2001; Shute, 2008).” —Dean, C. B., Hubbell, E. R., Pitler, H., & Stone, B. (2012). Classroom instruction that works: Research-based strategies for increasing student achievement . Alexandria, VA: Association for Supervision and Curriculum Development.
“[Learning targets] convey to students the destination for the lesson—what to learn, how deeply to learn it, and exactly how to demonstrate their new learning. In our estimation (Moss & Brookhart, 2009) and that of others (Seidle, Rimmele, & Prenzel, 2005; Stiggins, Arter, Chappuis, & Chappuis, 2009), the intention for the lesson is one of the most important things students should learn. Without a precise description of where they are headed, too many students are ‘flying blind’…. A shared learning target unpacks a ‘lesson-sized’ amount of learning—the precise ‘chunk’ of the particular content students are to master (Leahy, Lyon, Thompson, & Wiliam, 2005). It describes exactly how well we expect them to learn it and how we will ask them to demonstrate that learning…. Instructional objectives are about instruction, derived from content standards, written in teacher language, and used to guide teaching during a lesson or across a series of lessons. They are not designed for students but for the teacher. A shared learning target, on the other hand, frames the lesson from the students’ point of view. A shared learning target helps students grasp the lesson’s purpose—why it is crucial to learn this chunk of information, on this day, and in this way.” —Brookhart, S. M., Long, B. A., & Moss, C. M. (2011, March). Know your learning target. Educational Leadership , 68 (6), 66–69.
“Students who have clear pictures of the learning target and of the criteria for success are likely to also have a sense of what they can and should do to make their work measure up to those criteria and that goal. Clear learning targets direct both teachers and students toward specific goals. Students can meet goals only if they are actually working toward them, and they can’t work toward them until they understand what they are. Once students understand where they are headed, they are more likely to feel that they can be successful, can actually reach the goal. Students’ belief that they can be successful at a particular task or assignment is called self-efficacy (Bandura, 1997). Students who have self-efficacy are more likely to persist in their work and especially more likely to persist in the face of challenge (Pajares, 1996).” —Moss, C. M., & Brookhart, S. M. (2009). Advancing formative assessment in every classroom: A guide for instructional leaders . Alexandria, VA: Association for Supervision and Curriculum Development.
“Although they have different labels ( standards , learning results , expectations , and outcomes ), every state has standards that are determined at the state level. These standards are published and all teachers, parents, and students, should be familiar with them. This is essential because the research shows that ‘it is very difficult for students to achieve a learning goal unless they understand that goal and can assess what they need to do to reach it’ (Black et al., 2003).” —O’Connor, K. (2009, January). Reforming grading practices in secondary schools. Principal’s Research Review , 4 (1), 1–7.
“Arguably the most basic issue a teacher can consider is what he or she will do to establish and communicate learning goals, track student progress, and celebrate success. In effect, this design question includes three distinct but highly related elements: (1) setting and communicating learning goals, (2) tracking student progress, and (3) celebrating success. These elements have a fairly straightforward relationship. Establishing and communicating learning goals are the starting place. After all, for learning to be effective, clear targets in terms of information and skill must be established…. For example, the Lipsey and Wilson (1993) study synthesizes findings from 204 reports. Consider the average effect size of 0.55 from those 204 effect sizes. This means that in the 204 studies they examined, the average score in classes where goal setting was effectively employed was 0.55 standard deviations greater than the average score in classes where goal setting was not employed…. For the Lipsey and Wilson effect size of 0.55, the percentile gain is 21. This means that the average score in classes where goal setting was effectively employed would be 21 percentile points higher than the average score in classes where goal setting was not employed.” —Marzano, R. J., & Brown, J. L. (2007). The art and science of teaching: A comprehensive framework for effective instruction . Alexandria, VA: Association for Supervision and Curriculum Development.
“Equipped with state standards that had been clarified and specified in the district’s written curriculum, teachers in the higher performing schools carefully studied, further detailed and effectively implemented those standards using tools such as curriculum maps, pacing guides, aligned instructional programs and materials, and formative benchmark assessments. Higher performing schools deeply integrated the state standards into their written curriculum, but viewed state standards as the floor for student achievement, not the target. Educators did not see those standards as a digression from the real curriculum, but as the foundation of the curriculum. With a focus on core learning skills, grade-level and vertical teams continually reviewed and revised the curriculum. That curriculum communicated high expectations for all students, not just the academically advanced.” — Dolejs, C. (2006). Report on key practices and policies of consistently higher performing high schools . Washington, DC: National High School Center. (NOTE: Based on an analysis of 74 average and higher performing high schools in 10 states that identified the fundamental teaching and learning practices shared across higher performing high schools.)
← Return to Main Menu
482 Congress Street, Suite 500 Portland, ME 04101 Phone: (207) 773-0505 Fax: (877) 849-7052
©2024 Great Schools Partnership

Setting the Stage for Success: The Power of Learning Targets
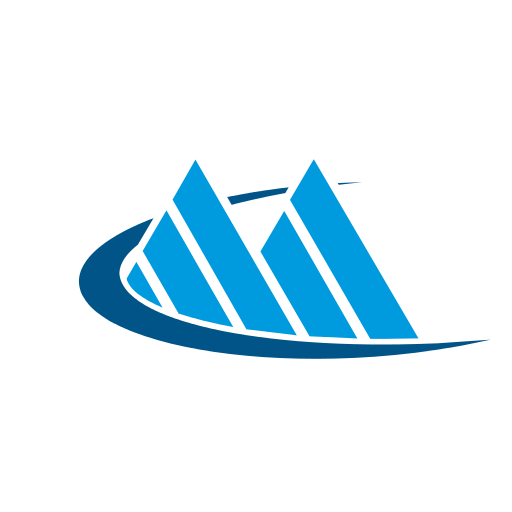
- Classroom Activities/Strategies/Guides
Brian Cagneey, author of The 7 Laws series on personal growth, wrote, “In order to know where you’re headed, you must be aware of your own personal goals.” The same can be said for academic goals. Setting clear goals for learning has huge benefits for both students and teachers.
Learning targets can be used in a variety of settings, including classrooms, schools, and districts. They can be used to guide instruction in all subject areas, and they can be adjusted or revised as needed based on student progress and feedback. When used effectively, learning targets can help create a culture of goal setting and continuous improvement, and can lead to improved student outcomes.
Understanding Learning Targets
Understanding learning targets involves a deep comprehension of what they are, how they are created, and how they can be used to support student learning. Learning targets are specific, measurable statements in student-friendly language describing what students are expected to know, do, or achieve by the end of an activity or unit.
To create effective learning targets, educators typically begin by identifying the key content, skill, or concept students need to achieve. These are then broken down into measurable and achievable learning targets that align with the learning objectives. It is important to note learning objectives are more for the teacher, while the learning targets are for the student. Objectives are often written in a more technical, formal language, whereas learning targets are written using student-friendly language.
Many learning targets are based on Common Core, state, local, or district standards and objectives. For example, if the Common Core standard is “6.RP.3. Use ratio and rate reasoning to solve real-world and mathematical problems, e.g., by reasoning about tables of equivalent ratios, tape diagrams, double number line diagrams, or equations,” the learning target for students might read, “I can explain the relationship between rate, ratio, and percent.” Learners must be able to understand and articulate their own learning goals to be able to reap the benefits.
The Benefits of Using Learning Targets
Using learning targets in the classroom can have numerous benefits for students, teachers, and many others involved in education. Research has shown when students are presented with a learning target at the beginning of a lesson, they benefit greatly from something as simple as a statement of learning or a pre-question to start the lesson.
Improved Student Engagement
Student engagement and student understanding are often two sides of the same coin. When students are engaged with their learning, their retention rates and understanding increase. Depending on how learning targets are written, they can communicate expectations of mental engagement and physical engagement.
For example, Bloom’s Taxonomy includes thought-processing verbs like “define,” “classify,” and “solve” as well as more higher-level learning that involves action verbs like “experiment,” “argue,” and “design.” When a combination of these words are used in learning targets, students are clearly directed about what they need to know and what they need to do to be engaged in learning.
Increased Student Achievement
The ultimate goal of education is student progress and achievement, which can be addressed from the beginning of a lesson. Achievement at the end of a lesson is more obtainable when overtly set at the beginning and routinely monitored and readdressed throughout the lesson.
It can be frustrating for students, just as it is for adults, to be given a task without a clear understanding of what they are trying to achieve. With increased rates of students below grade-level standards in subjects like reading and math, the emphasis on targeted instruction and student achievement is even more important now. Many research-based intervention programs utilize learning targets to build confidence and increase student achievement.
Enhanced Student Ownership of Learning
With improved engagement and an increase in probability for achievement, student learning as a whole becomes a more positive experience. Learning targets provide a sense of clarity and transparency, allowing students to understand the why of a lesson before jumping into the what or the how.
By starting this way, students become empowered to engage in self-assessment and reflective practices, allowing them to monitor their progress and comprehension, rather than relying solely on a teacher’s evaluation at the conclusion of a lesson or unit of study.
Greater School and District Effectiveness
When teachers use learning targets, they can better design and align activities and assessment. Then as classrooms and curriculum are better aligned on a larger scale, schools themselves become more effective. There are many guiding documents and professional development opportunities schools can provide for their educators to learn more about how to better use learning targets in daily teaching.
Daily use of learning targets has the ability to improve educational effectiveness of schools and districts as a whole. This is because school-wide goals and targets are often best incorporated at the classroom level. Teachers and administrators can work together to identify learning targets, ideal methods, and useful resources to achieve those goals.
Improved Communication with Parents
Using daily learning targets has a positive effect not only on students but also on parents and other school leaders, principals, and administrators. While much of the responsibility for a child’s learning feels like it falls on the teacher, there is truly a whole team working toward a child’s academic achievement.
The more a parent can clearly know and understand their child’s learning, the better they can help encourage their student. A clear learning target can also improve communication with other school leaders through things like collaboration with curriculum specialists, horizontal and vertical alignment between grade levels, and district involvement with administrators.
Implementing Learning Targets in the Classroom
Learning Targets by Connie M. Moss and Susan M. Bookhart presents a theory of action for learning targets in the classroom: “The most effective teaching and the most meaningful student learning happen when teachers design the right learning target for today’s lesson and use it along with their students to aim for and assess understanding.” With so much research in favor of learning targets, schools should support educators' efforts through curriculum staff or materials.
Identify the Learning Objectives
Identifying the learning objective is typically the first step for creating an effective learning target. The objective or standard acts as the foundation upon which the learning target is built. Every grade level and every school will have a set of standards they use to guide curriculum development, such as Common Core. Many schools will have some type of curriculum specialist who can support teachers
Once these targets are clearly crafted, they must be clearly communicated to the students. For example, a teacher may verbally express the targets, visually present them on the board, and routinely discuss the targets with students throughout the lesson.
Create a Culture of Goal Setting
Creating a culture of goal setting is a lifelong lesson that will benefit students. By allowing goals to be part of the classroom culture, students are invited to take ownership of their learning. When students know the end goal from the beginning of a lesson, they have a better understanding of what is expected. Therefore, they are better able to mentally process and think critically about how they need to get there.
In 2006, Carol Dweck published, Mindset: The New Psychology of Success: How We Can Learn to Fulfill Our Potential, and it had a huge impact on the education world. Her book helped launch the “growth mindset” concept that found its way into many classrooms. This growth mindset has helped the goal-setting culture by better-fostering a sense of motivation and determination in students as they pursue challenging academic goals.
Incorporate Learning Targets into Lesson Planning
Learning targets can be incorporated into lesson planning in multiple ways. On many lesson plan templates, learning standards and objectives are step one, and identifying learning targets for students are step two. But these learning targets should appear in multiple places as well. Learning targets may appear in the teacher’s copy of the lesson plan, prominently written on a board, on the first or last slide of a presentation of notes, at the top of a student worksheet, and so on.
Monitor Progress Toward Learning Targets
Both teachers and students should be involved in monitoring progress toward learning targets. For example, teachers may create graphs or charts to track student progress on homework or classwork assignments. Likewise, students may do some self-assessment of their own progress toward learning targets with an exit ticket at the end of a lesson.
The exit ticket strategy is a quick, informal assessment for students to complete that not only allows them to reflect on their skills or understanding, but also provides teachers with more data to inform instruction or intervention if necessary.
Adjust Learning Targets as Needed
If learning targets are being regularly monitored, then timely adjustments to those learning targets are also able to take place. Sometimes curriculum or instruction needs modifying or altering, and those changes should be made on more concrete evidence rather than just inferences. Content-focused instruction is crucial, and assessment of learning targets can help ensure curriculum, instructional strategies, and assessment are all aligned.
Assessing Learning Targets
Assessing learning targets is a critical component of the teaching and learning process. It involves not only measuring student progress toward specific learning goals but also using this information to guide instructional decisions. A few key steps teachers can take to effectively assess learning targets include using formative and summative assessments, providing feedback, using data to guide instructional decisions, and involving students in the assessment process.
Use Formative Assessments
Just as learning targets are ongoing expectations for students, formative assessments are ongoing evaluations of those expectations. The purpose of these assessments is to help students and teachers identify areas of strength and growth, monitor progress, and adjust learning or instruction as needed.
Learning targets provide a framework for formative assessments, and teachers can use both to identify areas where students may need additional support during the process rather than come to this realization at the end of a lesson, unit, or year.
Use Summative Tests
Similar to formative assessment, learning targets create a foundation for summative tests as well by defining the specific skills and knowledge students should have learned throughout a lesson, unit, or year. Summative tests provide a way to evaluate how well students have achieved those learning targets.
This is why it is important for assessments to be aligned with the curriculum and standards—to ensure students are not being unintentionally assessed on topics or ideas apart from the original learning goals.
Provide Feedback
It is important to make sure students are receiving regular feedback and sharing assessment results. Sharing the results of assessments, whether formative or summative, help students understand their progress toward achieving the learning goals.
Just as learning targets may be incorporated into each lesson plan or each daily agenda, feedback should also be a regular part of classroom culture. The more students are able to get feedback about the learning targets presented at the beginning, the more likely they will be able to confidently perform on culminating assessments.
Use Data to Guide Instructional Decisions
Learning targets can help everyone in the education world make instructional decisions. Based on the results of learning targets and their assessments, adjustments can be made to learning goals, curriculum, or instruction to better support student learning. Ensuring teachers, curriculum developers, principals, superintendents, school boards, and more have access to this data helps them make instructional decisions on small and larger scales.
Learning targets are more than just a set of goal statements written on the board and glanced over. When implemented and assessed correctly, they have the power to transform student learning. All students, whether on track or behind grade level, can benefit from clear expectations and a classroom culture that focuses on goal setting.
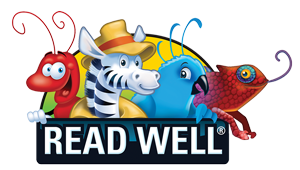
Build Confidence with Flexible, Targeted Instruction (Grades K–3)
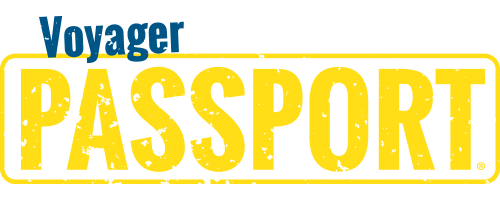
Research-Based Reading Intervention (Grades K–5)

Early Reading Assessments for Elementary
Subscribe to EDVIEW360 to gain access to podcast episodes, webinars and blog posts where top education thought leaders discuss hot topics in the industry.
- Our Mission
Making Learning Targets Clear to Students
When students clearly understand classroom expectations, they’re better able to assess and improve their performance.
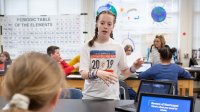
The third-grade classroom is busy. Students are in the middle of making slime, and you can sense the level of engagement across the class. The teacher is working very hard to make sure that students are clear on the goals of learning: to understand the relationships between solids, liquids, and gases. The students can see success criteria posted on the wall and examples of successful work spread across the room. Visiting teachers are engaging in random checks of understanding to ensure that students are clear on expectations.
As part of the school’s improvement process, teachers and principals are visiting classrooms and interviewing students on their understanding of the expectations of learning, the students’ self assessment on their progress, and what next steps they need to take to meet established goals. The team was in the third-grade classroom and filmed student interviews; they discussed the key findings with the teacher after class.
Here are samples of two interviews of students in the same classroom.
Interview 1
Teacher: What are you learning?
Student: We’re learning about slime.
Teacher: How do you know if you are successful?
Student: We don’t want the slime to stick to us.
Interview 2
Teacher: What are you learning?
Student: We’re learning about solids, liquids, and gases.
Student: We can define and relate each phase. Right now we’re learning about solids, liquids, and gases by creating slime.
The teacher is doing great things. The question is whether all students are following the teacher’s lead. Building student clarity is a constant pursuit: We never arrive, but it’s worth our focus.
Clarity Research Snapshot
When students are clear on expectations of learning, they tend to double their rate of learning. Moreover, when students are clear on expectations, they have a better chance of assessing their current performance and using feedback accurately. As John Hattie explains in Visible Learning , self assessment, feedback, and student clarity yield substantial growth in student learning. Yet the implementation of this idea is extremely difficult.
Let’s take a look at some challenges and solutions to implementation.
Challenge 1. Novices don’t really understand rubrics: Experts love rubrics because the bullet points clearly delineate the core expectations that they want students to learn. Novices don’t fully appreciate the bullet points because they simply don’t have a concrete example of what’s written. As such, they scan the tool for items that are familiar to them: activities (such as getting into groups), tasks (such as completing six problems correctly), and contexts (such as exploring bridges). Because of this scanning for the familiar, students begin thinking about group work, assignment completion, and bridges and are therefore less likely to be any clearer on the standards they’re learning about.
To focus students on the actual learning, teachers are encouraged to start with examples of great work that meets the expectations of the teacher. The more students see examples of great work in multiple contexts, the better able students are to use rubrics to evaluate their own work as well as to give, receive, and use feedback. This also makes it easier for them to focus on the core content that the teacher is after.
Challenge 2. Telling people the expectations clearly doesn’t mean the expectations are clear to them: As a school leader, I have found that simply sending a clear message to people doesn’t ensure that they are clear on my message. Almost every message I send must be followed up with clarifying emails, meetings with parents and/or faculty, and a series of communication check-ins. As such, developing clarity isn’t one way. Clarity is interactive and built through multiple engagements.
Co-construction is an interactive process that enables students to build clarity of expectations. Co-construction is the active involvement of building success criteria with students rather than presenting success criteria to students. Here is one example of co-construction:
- Providing students with work samples that illustrate success
- Asking students to identify the parts of the work sample that make it successful (i.e., criteria for success)
- Writing out the criteria for success with students
Challenge 3. The classroom is mostly hidden from teachers, and students give each other inaccurate feedback: As Graham Nuthall tells us in The Hidden Lives of Learners , the majority of the classroom experience is hidden from the teacher’s observation. In the hidden classroom, kids typically give and receive most of the feedback to and from their peers, and most of that feedback is incorrect. To address this, we should consider having students do the following:
- Use the fishbowl protocol to share their feedback, and process the accuracy of the feedback to the expectations of the lesson or unit
- Begin units with discussions on what mastery looks like, and have students evaluate the differences between various levels of mastery
Students’ achievement and attitude improve when they have the tools to own their own learning. As educators, it’s up to us to provide those tools.
Culturally Responsive Assessment in Teaching
Please log in to save materials. Log in
- EPUB 3 Student View
- PDF Student View
- Thin Common Cartridge
- Thin Common Cartridge Student View
- SCORM Package
- SCORM Package Student View
- 1 - Course Objectives
- 2 - Video Glossary of Terms
- 3 - Unit 1: The Importance of Conceptualizing Content Standards/Objectives to Counteract Stereotypes and Incorporate Learner Contributions
- 4 - Making it Clear: What should a student know and be able to do (Content Standards)?
- 5 - Check for Understanding - Formative Assessment
- 6 - Types of Learning Targets
- 7 - Unit 2 Composing and Decomposing Learning Objectives
- 8 - How to Write Learning Objectives Using Revise Bloom's Taxonomy Time: 11 minutes
- 9 - Putting it all together
- 10 - Unit 3: Focus on Diversity of Learners
- 11 - Reflection
- 12 - Help us improve this template! [PLEASE ANSWER THE FOLLOWING QUESTIONS IN THE COMMENTS OF THIS OER]
- View all as one page
Types of Learning Targets
One way to determine if your targets are clear and usable is to determine what kind of learning is being called for. Learning targets are classified into a framework that identifies five kinds of learning targets: knowledge, reasoning, skill, product, and disposition.
Learning Target Types
Knowledge Targets
- Math Example: Recognizes acute, obtuse, and right angles
- ELA Example: Identifies nouns and verbs
- Science Example: Describes how organisms interact with each other to transfer energy and matter in an ecosystem.
Reasoning Targets
- Reasoning targets specify thought processes students are to learn to apply effectively (do well) within a range of subjects; e.g., solve problems, make inferences, draw conclusions, form, and defend judgment.
- Students should develop the ability to apply knowledge in authentic contexts - that is, in contexts that transfer to work and life beyond school. This target requires students to engage in reasoning using their knowledge.
- Reasoning processes can be thought of as falling into one of six overall patterns of reasoning: inference, analysis, comparison, classification, evaluation, and synthesis.
- Together, the six patterns of reasoning represent those most commonly found among taxonomies, content standards documents, and assessments.
- To test reasoning proficiency, the key is to determine "Who is doing the reasoning?" Are the students doing something more than remembering the answers?
Six Patterns of Reasoning
- Inference : Making a reasonable guess based on information or clues
- Analysis : Examining the components or structure of something
- Comparison : Describing similarities and differences between two or more items
- Classification : Sorting things into categories based on certain characteristics
- Evaluation : Expressing and defending an opinion, a point of view, a judgment, or a decision
- Synthesis : Combining discrete elements to create something new
Examples of Reasoning Targets
- Math Reasoning Target - Uses data from a random sample to draw inferences about a population with an unknown characteristic of interest
- ELA Reasoning Target - With prompt and support, describes the relationship between illustrations and the story in which they appear.
- Social Studies Reasoning Target - Compares and contrasts points of view from a historical event
- Science Reasoning Target - Draws conclusions from experiment results
- Health/PE Reasoning Target - Uses criteria to set goals for improving health and fitness practice
- The Arts - Compares purposes of chosen musical examples (Music)
- The Arts - Evaluates the quality of own work to refine it (Visual Arts)
Skill Targets
- Skill targets are those learning targets where a real-time demonstration or physical performance is the heart of learning.
- Subjects such as physical education, fine arts, performing arts, and world languages, have skill development as the core of their discipline.
Examples of Skill Targets
- Math Skill Target - Measures the length of an object twice, using length units of different lengths for the two measurements
- ELA Skill Target - Pronounces, blends, and segments syllables in spoken words
- Social Studies Skill Target - Participates in civic discussions
- Science Skill Target - Uses laboratory equipment safely
- Health/Physical Education Skill Target - Dribbles to keep the ball away from an opponent; passes and receives on the move
- The Arts Skill Target - Integrates voice into character development (Theater)
Product Targets
- Product targets specification for qualities of a good product are the focus.
- Product examples include " creates tables, graphs, scatter plots, and box plots to display data effectively.
- Curricula generally include far fewer product targets than knowledge and reasoning targets.
- Term papers, research reports, and lab reports are product targets when the curriculum guide, calls for students to create them.
- When products are assessed it yields evidence of the intended learning because the creation of the product is the stated learning.
- Does the content standard call for the creation of a product? If so, it's a product target.
- Confusing the activity with the learning target can cause difficulties when classifying product targets.
- If the learning target does not call for the creation of a product, but you want to classify it as a product target, it is possible that you are including the task or activity students will engage in.
- The key question is " What is the intended learning " not "How will students demonstrate it?"
Examples of Product Targets
- Math Product Target - Draws a bar graph to represent a data set with up to four categories.
- ELA Product Target - Writes opinion pieces on topics or texts, supporting a point of view with reasons and information.
- Social Studies Product Target - Creates a timeline to show the order of early explorations and settlements.
- Science Product Target -Makes pictographs to describe observations and draw conclusions.
- Health/PE Product Target - Develops a personal health-related fitness plan.
- The Arts Product Target - Creates drawings demonstrating one- and two-point perspectives (Visual Arts).
Disposition Targets
- Disposition targets reflect attitudes and feelings.
- Disposition targets represent important affective goals we hold for students as byproducts of their educational experience and not assessed for the purpose of grading.
- Although dispositions are nonacademic, they hold students accountable.
Examples of Disposition Targets
- ELA Disposition Target - Look forward to group discussions
- Math Disposition Target - Sees mathematics as important to learn
- Social Studies Disposition Target - Respects individual worth and human dignity
- Science Disposition Target - Seeks opportunities to understand how things work.
- Health/PE Disposition Target - Enjoys playing a sport
- The Arts Disposition Target - Values practice for its own sake.
Home » Teaching Resources » Learning Targets That Motivate Students
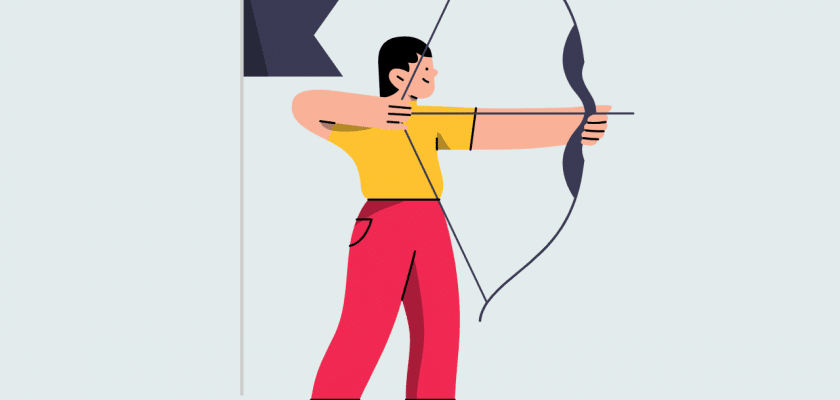
Learning Targets That Motivate Students
Learning targets are the foundation of effective teaching and learning. They give us a clear direction, establish our focus, and provide a means to measure success. This article delves into the world of learning targets, exploring their significance in the classroom, their benefits for both students and teachers, and how to create them effectively.
By the end of today’s lesson, you’ll be able to confidently say, ‘I understand learning targets, and I can create them for my own classroom.’ Let’s get started on this journey of educational improvement.
Table of Contents
What is a learning target, why use learning targets, 6 advantages of enhancing student learning through learning targets, how teachers benefit from learning targets, how to create learning targets, best practices & tips, learning target examples, learning targets faqs.
Learning targets are goals we set for our students. They need to be concrete goals written and worded in a way that’s easy for students to understand. To empower students, clearly defined learning targets should begin with the words, “I can.”
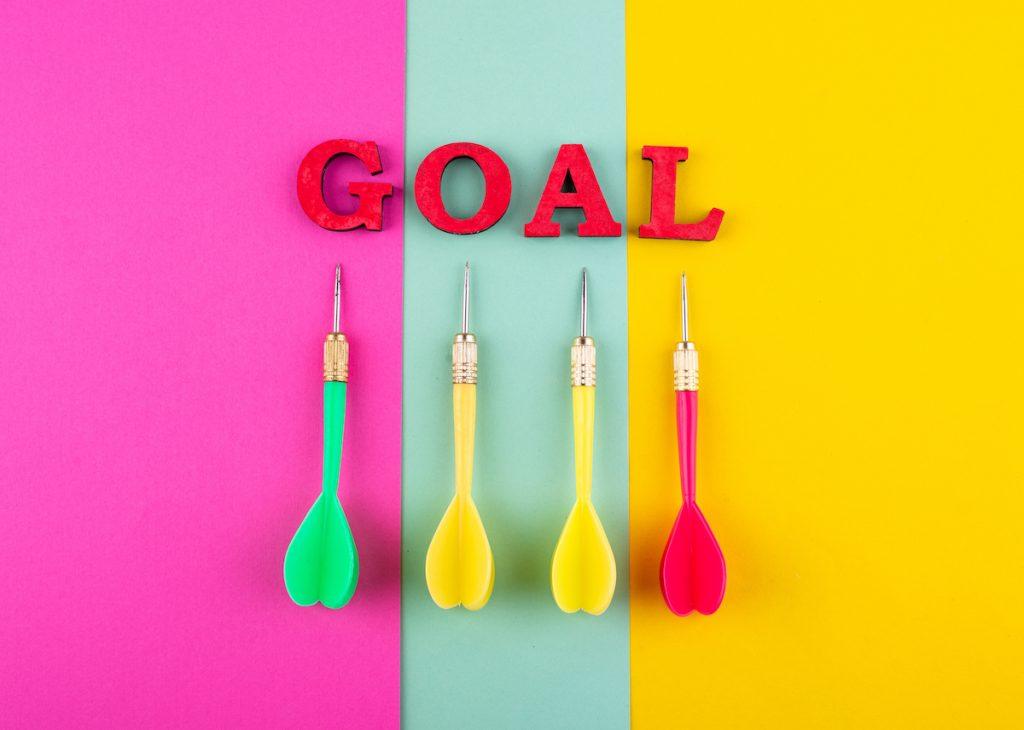
Goal-setting is an important skill to teach kids. In academics, the use of learning targets comes with a variety of benefits. Students can also use goal-setting skills to be successful their whole lives. Let’s go over some of the best reasons to incorporate them into your teaching practice.
1. Increased Achievement
As the saying goes, you don’t know what you don’t know. Understanding exactly what it is you’re supposed to be learning makes it infinitely easier to reach that goal. Learning targets help students grow by informing them exactly what they need to learn.
2. Added Motivation
Just as kids love reaching the next level in a video game, they feel highly motivated to follow through on learning objectives when students understand that there is a clear next step to aim for. A goal helps students become more engaged.
3. Personal Responsibility
Using “I can” statements are verry important reason. They place the responsibility and cognitive process of learning directly on the student. Saying “I can” promotes personal responsibility and confidence.
4. A Boost in Feedback
Knowing what the target is informes a student when they’ve reached it. And if they haven’t, knowing where the target is provides feedback they can use to understand how near or far they are.
5. Improved Self-Reflection Abilities
Putting the student in the driver’s seat of their own education naturally encourages them to be curious about how they’re doing. Knowing the goalposts will get them in the habit of reflecting on their own work and progress.
6. Better Communication
Learning targets are phrases for students to describe their skills succinctly and with confidence. They also help a student prove a skill has been learned. While our use of them in the classroom may be as a motivational statement, consider this: when they grow up to write resumes and go on job interviews, they’ll need this same skill! What a great time to start your students learning how to speak confidently and clearly about your abilities.
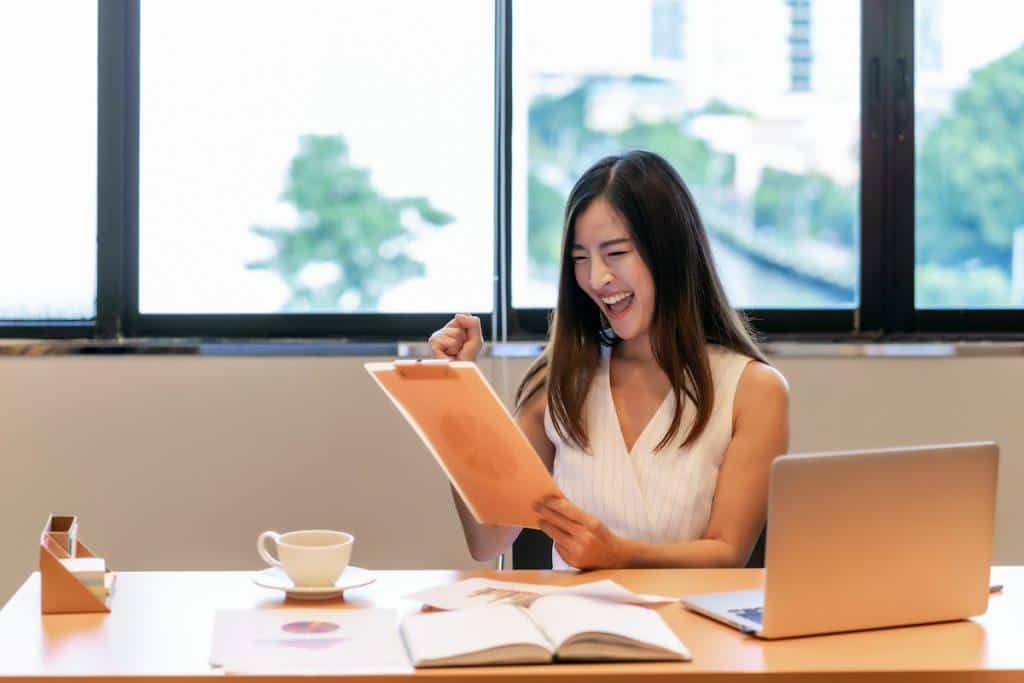
1. Focused Teaching
Clear goal-setting helps teachers focus on the most important areas to teach. When you know exactly what you’re striving for, you’ll be able to cut any fat and hone in on the most relevant teaching material.
2. Improved ability to assess your students
Just as the student will have a better understanding of their progress, so will you. That’s really helpful to you as you assess your own teaching and determine what’s working for you and your small groups of students.
3. Be better able to explain students’ progress
Because you have your own clear understanding of your students’ progress, you’ll have a much easier time communicating it to parents and schools. The learning target already gives you great wording to describe students’ successes and areas for improvement.
4. Career Advancement
Setting your own learning targets will make you a better teacher. A teacher that gets top results in the classroom can use that to advance their career.
Now that you understand the great benefits that come with implementing this strategy into your teaching, let’s go through exactly how to create powerful learning targets.
Learning Target Types.
First, start by identifying which target type is best for your students and goals.
- Knowledge-level : These pertain to factual, procedural, and conceptual knowledge. Ie, knowing things from memory, knowing how to execute a process, and the ability to explain concepts.
- Reasoning-level : Reasoning targets use verbs like “predict, infer, compare, hypothesize, critique, draw conclusions, justify, and evaluate.”
- Skill-level : In this context, “skill” refers solely to physical activities, such as those executed in gym class, the study of foreign languages, or the practice of fine and performing arts.
- Product-level : This is the creation of physical products, such as 3D models, or a piece of writing.
Concrete Learning Objective s
This could be a small goal, or it could be a larger goal that you can also break down into smaller learning targets. You can use national or state standards to guide your choice of age-appropriate goals for your classroom. This Progression of ELA Common Core Standards PDF includes 68 ready-to-view/print pages of goals by grade level for middle school through high school students.
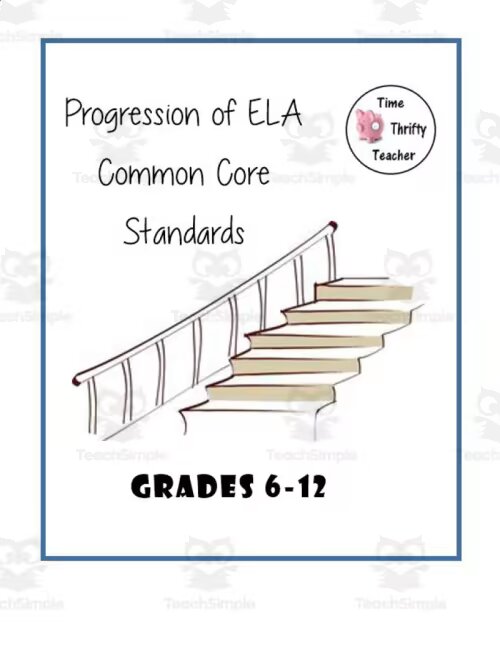
Employ Success Criteria
Learning targets and success criteria go hand-in-hand. Success criteria are the methods used to judge whether a target has been met. When coming up with success criteria, think of verbs you want your student to be able to do. Some examples include define, explain, build, create, or write . Success criteria are what make the learning goal more concrete and measurable. Both you and the student need to know when they’ve hit the bullseye.
State the Goal: Write Learning Targets
State the Goal in a way that’s empowering. Begin with the words “I can,” followed by succinct, easy-to-understand language. The learning target should not include words students don’t already know. They need to be written in student friendly language.
Set a Timeframe
Set a Timeframe for the learning target to be achieved. This might be by the end of class, the end of a project or unit, or the end of the school year .
Alright, you’ve got some great learning targets you want to use with your students, now what?
Make Them Visible
Display your learning targets somewhere that’s easy for students to see.This helps keep those targets fresh in their minds as they work. Some good places to display a learning target is on bulletin boards, whiteboards, and chalkboards. It’s also a great idea to put them on homework assignments, syllabi, and test prep materials.
Bring up the learning targets at least once per lesson. Having an auditory reminder of their daily learning targets and goals by way of you saying them aloud is another incredibly helpful way for students to keep them front of mind.
Student Friendly Language
Make sure the teacher and whole class understands each learning target. The benefits of writing learning targets will go right out the window if your students don’t understand. Encourage your students to ask questions, but also ask them to put the learning targets in their own words.
- Use student-friendly language to word learning targets.
- Phrase with empowering, “I can” statements.
- Incorporate the repetition of learning targets in each lesson.
- Learning targets don’t only have to be academic. You can use them to foster personal development as well. For example, students can work on learning targets like listening skills, public speaking, mood regulation, and beyond.
- Another technique to try is reverse-engineering learning targets. Work through a lesson or a project, and then ask students what they thought the learning target was. This helps develop skills of reflection.
We’ve talked about what you need to do to engage students and craft excellent learning targets, but you’re a teacher, so you know an example always helps! Below are a few learning target examples by subject.
Math Learning Target Examples
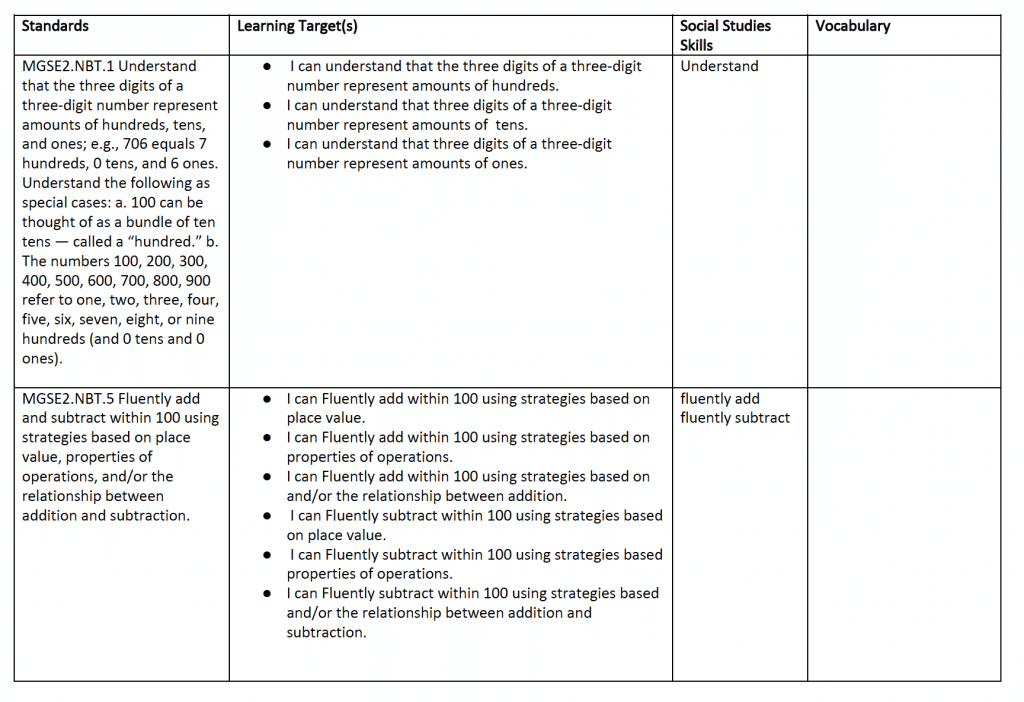
These learning targets are used by Henry County Schools in Georgia for 2nd grade math :
- I can mentally add 10 to a given number 100–900.
- I can explain why addition strategies work using place value.
- I can measure the length of an object by selecting appropriate tools such as rulers.
- I can use subtraction within 100 to solve word problems involving lengths that are given in the same units.
Language Arts Learning Target Examples
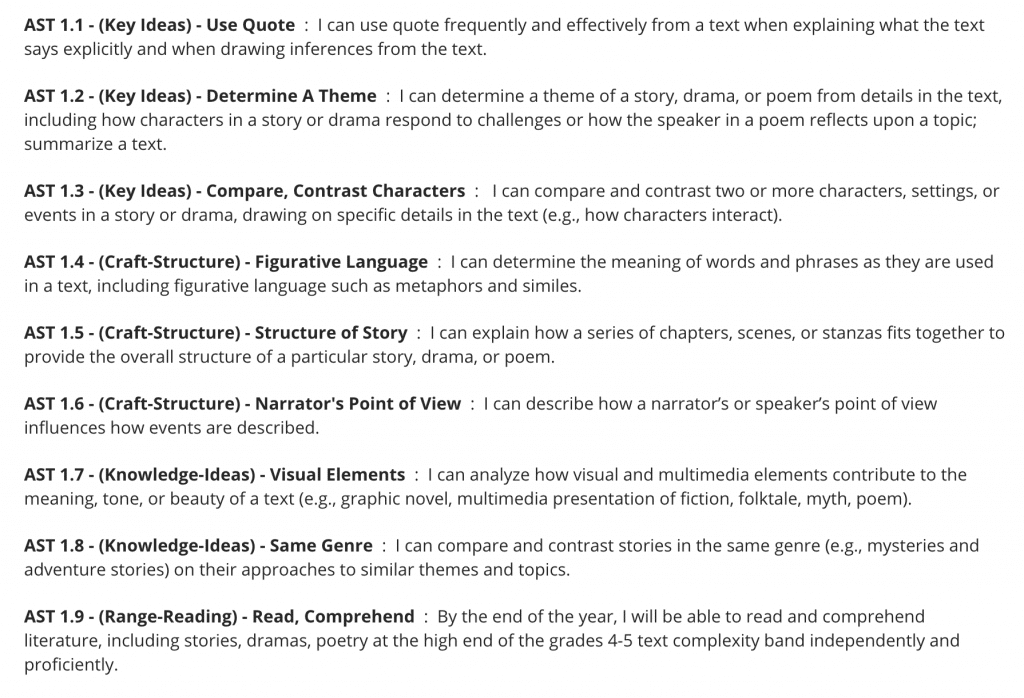
Beaverton School District in Beaverton, OR utilizes these learning targets for 5th-grade language arts :
- I can use quotes frequently and effectively from a text when explaining what the text says explicitly and when drawing inferences from the text .
- I can explain the relationships or interactions between two or more individuals, events, ideas, or concepts in a historical, scientific, or technical text based on specific information in the text.
- I can integrate information from several texts on the same topic in order to write or speak about the subject knowledgeably.
- I can explain the function of conjunctions, prepositions, and interjections in general and their function in particular sentences.
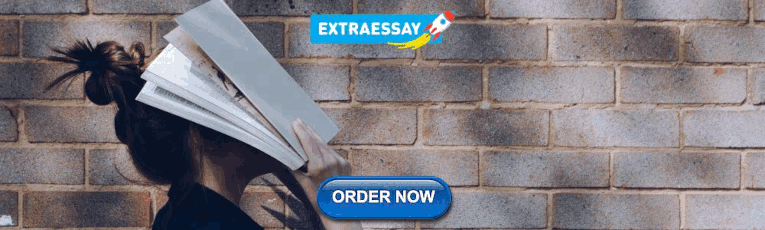
Science Learning Target Examples
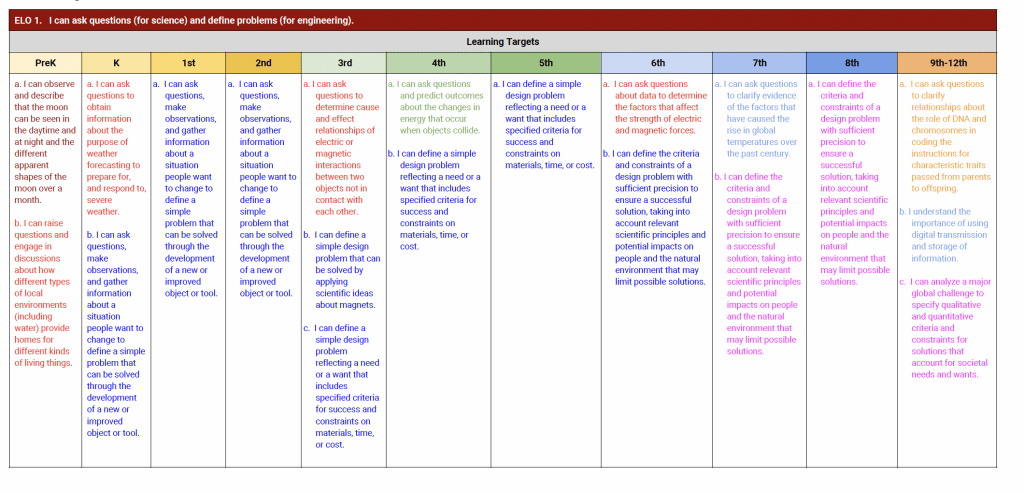
Addison Central School District in Middlebury, VT uses these learning targets for 1st-grade science teachers:
- I can develop a simple sketch, drawing, or physical model to illustrate how the shape of an object helps it function as needed to solve a given problem.
- I can plan and conduct investigations to provide evidence that vibrating materials can make sound and that sound can make materials vibrate.
- I can use materials to design a solution to a human problem by mimicking how plants and/or animals use their external parts to help them survive, grow, and meet their needs.
- I can read texts and use media to determine patterns in the behavior of parents and offspring that help offspring survive.
Social Studies Learning Target Examples
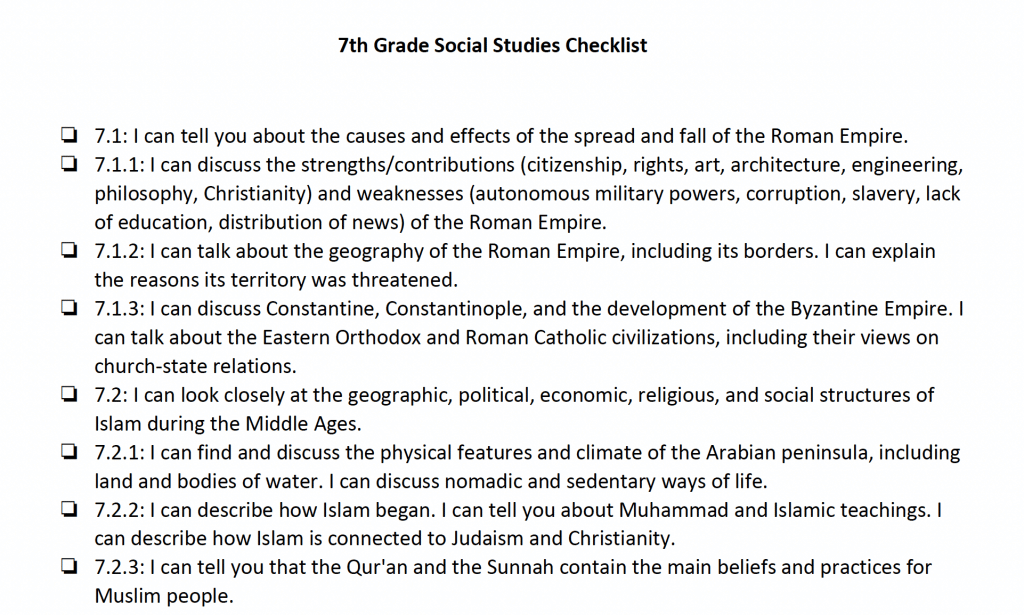
Here are a few examples of 7th Grade Social Studies learning targets program materials employed by Yosemite Valley Charter School in Fresno, CA:
- I can discuss the importance of family, specialized jobs, and local commerce in the growth of West African states and cities.
- I can explain how Christianity spread north of the Alps, and the role that the church and monasteries played after the Roman Empire fell.
- I can tell you about the goods and ideas that were traded among Europe, Africa, Asia, and the Americas during the 15th and 16th centuries. I can talk about the impact of these exchanges on each continent.
- I can discuss how modern-day capitalism first began. I can tell you about different influences such as mercantilism, the cottage industry, the market economy in 17th-century Europe, international trading/marketing patterns, explorers, and map makers.
You can also try out ready-to-print and display learning targets, such as this PDF of ELA Learning Targets & “I Can” Statements for 8th Grade
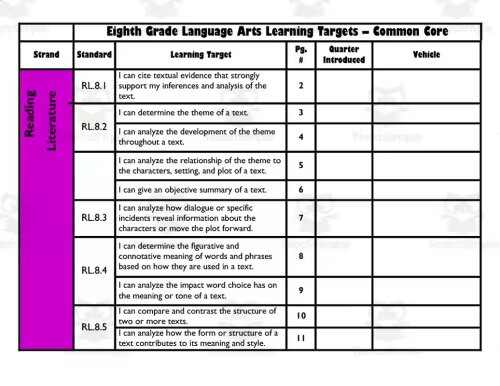
Below are a few example used by the special education program of Jordan School District in West Jordan, UT: I can identify emotions, thoughts, or triggers associated with my depression and/or anxiety in order to develop and utilize coping strategies I can identify and demonstrate at least 5 emotional regulation skills that are effective for me. I can determine healthy ways to accept, manage, and adapt to changes in relationships. I can identify and demonstrate appropriate social skills with classroom peers.
Unlike learning targets, objectives are written from the teacher’s point of view and address the educator’s goals. These are often worded as “we will” statements.
Learning targets are all for naught if they aren’t reflected in learning activities and assessments. Imagine teaching students about the French Revolution, and then giving them a quiz on the Pythagorean Theorem. Use your learning targets to inform each step of your teaching.
Share Article:
Download unlimited teaching resources, join free today.
Laurie H. was a fulltime teacher for over a decade and now enjoys using her experience in education for writing.
We have a lot of interesting articles and educational resources from a wide variety of authors and teaching professionals.
An Unbiased Review Of Teachers Pay Teachers
50 teacher memes that will make you laugh.
Last Updated on July 18, 2023 by Teach Simple
- Data Science
- Data Analysis
- Data Visualization
- Machine Learning
- Deep Learning
- Computer Vision
- Artificial Intelligence
- AI ML DS Interview Series
- AI ML DS Projects series
- Data Engineering
- Web Scrapping
10 Must Read Machine Learning Research Papers
Machine learning is a rapidly evolving field with research papers often serving as the foundation for discoveries and advancements. For anyone keen to delve into the theoretical and practical aspects of machine learning, the following ten research papers are essential reads. They cover foundational concepts, groundbreaking techniques, and key advancements in the field.
Table of Content
1. “A Few Useful Things to Know About Machine Learning” by Pedro Domingos
2. “imagenet classification with deep convolutional neural networks” by alex krizhevsky, ilya sutskever, and geoffrey e. hinton, 3. “playing atari with deep reinforcement learning” by volodymyr mnih et al., 4. “sequence to sequence learning with neural networks” by ilya sutskever, oriol vinyals, and quoc v. le, 5. “attention is all you need” by ashish vaswani et al., 6. “generative adversarial nets” by ian goodfellow et al., 7. “bert: pre-training of deep bidirectional transformers for language understanding” by jacob devlin et al., 8. “deep residual learning for image recognition” by kaiming he et al., 9. “a survey on deep learning in medical image analysis” by geert litjens et al., 10. “alphago: mastering the game of go with deep neural networks and tree search” by silver et al..
This article highlights 10 must-read machine learning research papers that have significantly contributed to the development and understanding of machine learning. Whether you’re a beginner or an experienced practitioner, these papers provide invaluable insights that will help you grasp the complexities of machine learning and its potential to transform industries.
Summary : Pedro Domingos provides a comprehensive overview of essential machine learning concepts and common pitfalls. This paper is a great starting point for understanding the broader landscape of machine learning.
Key Contributions:
- Distills core principles and practical advice.
- Discusses overfitting, feature engineering, and model selection.
- Offers insights into the trade-offs between different machine learning algorithms.
Access: Read the Paper
Summary : Often referred to as the “AlexNet” paper, this work introduced a deep convolutional neural network that significantly improved image classification benchmarks, marking a turning point in computer vision.
- Demonstrated the power of deep learning for image classification.
- Introduced techniques like dropout and ReLU activations.
- Showed the importance of large-scale datasets and GPU acceleration.
Summary : This paper from DeepMind presents the use of deep Q-networks (DQN) to play Atari games . It was a seminal work in applying deep learning to reinforcement learning.
- Introduced the concept of using deep learning for Q-learning.
- Showcased the ability of DQNs to learn complex behaviors from raw pixel data.
- Paved the way for further research in reinforcement learning.
Summary : This paper introduced the sequence-to-sequence (seq2seq) learning framework , which has become fundamental for tasks such as machine translation and text summarization.
- Proposed an encoder-decoder architecture for sequence tasks.
- Demonstrated effective training of neural networks for sequence modeling.
- Laid the groundwork for subsequent advancements in natural language processing.
Summary : This paper introduces the Transformer model, which relies solely on attention mechanisms, discarding recurrent layers used in previous models. It has become the backbone of many modern NLP systems.
- Proposed the Transformer architecture, which uses self-attention to capture dependencies.
- Demonstrated improvements in training efficiency and performance over RNN-based models.
- Led to the development of models like BERT, GPT, and others.
Summary : Ian Goodfellow and his colleagues introduced Generative Adversarial Networks (GANs) , a revolutionary framework for generating realistic data through adversarial training.
- Proposed a novel approach where two neural networks compete against each other.
- Enabled the generation of high-quality images, text, and other data types.
- Spurred a plethora of research on GAN variations and applications.
Summary : BERT (Bidirectional Encoder Representations from Transformers) introduced a new way of pre-training language models, significantly improving performance on various NLP benchmarks.
- Proposed bidirectional training of transformers to capture context from both directions.
- Achieved state-of-the-art results on several NLP tasks.
- Set the stage for subsequent models like RoBERTa, ALBERT, and DistilBERT.
Summary : This paper introduces Residual Networks (ResNets), which utilize residual learning to train very deep neural networks effectively.
- Addressed the issue of vanishing gradients in very deep networks.
- Demonstrated that extremely deep networks can be trained successfully.
- Improved performance on image classification tasks and influenced subsequent network architectures.
Summary : This survey provides a comprehensive review of deep learning techniques applied to medical image analysis, summarizing the state of the art in this specialized field.
- Reviewed various deep learning methods used in medical imaging.
- Discussed challenges and future directions in the field.
- Provided insights into applications such as disease detection and image segmentation.
Summary : This paper describes AlphaGo, the first AI to defeat a world champion in the game of Go, using a combination of deep neural networks and Monte Carlo tree search.
- Demonstrated the effectiveness of combining deep learning with traditional search techniques.
- Achieved a major milestone in AI by mastering a complex game.
- Influenced research in AI and its application to other complex decision-making problems.
These ten research papers cover a broad spectrum of machine learning advancements, from foundational concepts to cutting-edge techniques. They provide valuable insights into the development and application of machine learning technologies, making them essential reads for anyone looking to deepen their understanding of the field. By exploring these papers, you can gain a comprehensive view of how machine learning has evolved and where it might be heading in the future.
10 Must Read Machine Learning Research Papers – FAQ’s
What are large language models (llms) and why are they important.
Large Language Models (LLMs) are advanced AI systems designed to understand and generate human language. They are built using deep learning techniques, particularly transformer architectures. LLMs are important because they enable applications such as text generation, translation, and sentiment analysis, significantly advancing the field of natural language processing (NLP).
Why should I read “A Few Useful Things to Know About Machine Learning” by Pedro Domingos?
Pedro Domingos’ paper provides a broad overview of key machine learning concepts, common challenges, and practical advice. It’s an excellent resource for both beginners and experienced practitioners to understand the underlying principles of machine learning and avoid common pitfalls.
What impact did “ImageNet Classification with Deep Convolutional Neural Networks” have on the field?
The “AlexNet” paper revolutionized image classification by demonstrating the effectiveness of deep convolutional neural networks. It significantly improved benchmark results on ImageNet and introduced techniques like dropout and ReLU activations, which are now standard in deep learning.
Please Login to comment...
Similar reads.
- AI-ML-DS Blogs
- California Lawmakers Pass Bill to Limit AI Replicas
- Best 10 IPTV Service Providers in Germany
- Python 3.13 Releases | Enhanced REPL for Developers
- IPTV Anbieter in Deutschland - Top IPTV Anbieter Abonnements
- Content Improvement League 2024: From Good To A Great Article
Improve your Coding Skills with Practice
What kind of Experience do you want to share?
Thank you for visiting nature.com. You are using a browser version with limited support for CSS. To obtain the best experience, we recommend you use a more up to date browser (or turn off compatibility mode in Internet Explorer). In the meantime, to ensure continued support, we are displaying the site without styles and JavaScript.
- View all journals
- Explore content
- About the journal
- Publish with us
- Sign up for alerts
- Open access
- Published: 22 July 2024
Neural general circulation models for weather and climate
- Dmitrii Kochkov ORCID: orcid.org/0000-0003-3846-4911 1 na1 ,
- Janni Yuval ORCID: orcid.org/0000-0001-7519-0118 1 na1 ,
- Ian Langmore 1 na1 ,
- Peter Norgaard 1 na1 ,
- Jamie Smith 1 na1 ,
- Griffin Mooers 1 ,
- Milan Klöwer 2 ,
- James Lottes 1 ,
- Stephan Rasp 1 ,
- Peter Düben ORCID: orcid.org/0000-0002-4610-3326 3 ,
- Sam Hatfield 3 ,
- Peter Battaglia 4 ,
- Alvaro Sanchez-Gonzalez 4 ,
- Matthew Willson ORCID: orcid.org/0000-0002-8730-1927 4 ,
- Michael P. Brenner 1 , 5 &
- Stephan Hoyer ORCID: orcid.org/0000-0002-5207-0380 1 na1
Nature volume 632 , pages 1060–1066 ( 2024 ) Cite this article
56k Accesses
3 Citations
678 Altmetric
Metrics details
- Atmospheric dynamics
- Climate and Earth system modelling
- Computational science
General circulation models (GCMs) are the foundation of weather and climate prediction 1 , 2 . GCMs are physics-based simulators that combine a numerical solver for large-scale dynamics with tuned representations for small-scale processes such as cloud formation. Recently, machine-learning models trained on reanalysis data have achieved comparable or better skill than GCMs for deterministic weather forecasting 3 , 4 . However, these models have not demonstrated improved ensemble forecasts, or shown sufficient stability for long-term weather and climate simulations. Here we present a GCM that combines a differentiable solver for atmospheric dynamics with machine-learning components and show that it can generate forecasts of deterministic weather, ensemble weather and climate on par with the best machine-learning and physics-based methods. NeuralGCM is competitive with machine-learning models for one- to ten-day forecasts, and with the European Centre for Medium-Range Weather Forecasts ensemble prediction for one- to fifteen-day forecasts. With prescribed sea surface temperature, NeuralGCM can accurately track climate metrics for multiple decades, and climate forecasts with 140-kilometre resolution show emergent phenomena such as realistic frequency and trajectories of tropical cyclones. For both weather and climate, our approach offers orders of magnitude computational savings over conventional GCMs, although our model does not extrapolate to substantially different future climates. Our results show that end-to-end deep learning is compatible with tasks performed by conventional GCMs and can enhance the large-scale physical simulations that are essential for understanding and predicting the Earth system.
Similar content being viewed by others
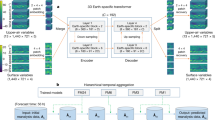
Accurate medium-range global weather forecasting with 3D neural networks
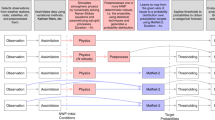
Deep learning for twelve hour precipitation forecasts
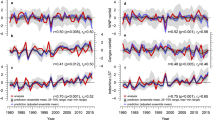
Skilful predictions of the Asian summer monsoon one year ahead
Solving the equations for Earth’s atmosphere with general circulation models (GCMs) is the basis of weather and climate prediction 1 , 2 . Over the past 70 years, GCMs have been steadily improved with better numerical methods and more detailed physical models, while exploiting faster computers to run at higher resolution. Inside GCMs, the unresolved physical processes such as clouds, radiation and precipitation are represented by semi-empirical parameterizations. Tuning GCMs to match historical data remains a manual process 5 , and GCMs retain many persistent errors and biases 6 , 7 , 8 . The difficulty of reducing uncertainty in long-term climate projections 9 and estimating distributions of extreme weather events 10 presents major challenges for climate mitigation and adaptation 11 .
Recent advances in machine learning have presented an alternative for weather forecasting 3 , 4 , 12 , 13 . These models rely solely on machine-learning techniques, using roughly 40 years of historical data from the European Center for Medium-Range Weather Forecasts (ECMWF) reanalysis v5 (ERA5) 14 for model training and forecast initialization. Machine-learning methods have been remarkably successful, demonstrating state-of-the-art deterministic forecasts for 1- to 10-day weather prediction at a fraction of the computational cost of traditional models 3 , 4 . Machine-learning atmospheric models also require considerably less code, for example GraphCast 3 has 5,417 lines versus 376,578 lines for the National Oceanic and Atmospheric Administration’s FV3 atmospheric model 15 (see Supplementary Information section A for details).
Nevertheless, machine-learning approaches have noteworthy limitations compared with GCMs. Existing machine-learning models have focused on deterministic prediction, and surpass deterministic numerical weather prediction in terms of the aggregate metrics for which they are trained 3 , 4 . However, they do not produce calibrated uncertainty estimates 4 , which is essential for useful weather forecasts 1 . Deterministic machine-learning models using a mean-squared-error loss are rewarded for averaging over uncertainty, producing unrealistically blurry predictions when optimized for multi-day forecasts 3 , 13 . Unlike physical models, machine-learning models misrepresent derived (diagnostic) variables such as geostrophic wind 16 . Furthermore, although there has been some success in using machine-learning approaches on longer timescales 17 , 18 , these models have not demonstrated the ability to outperform existing GCMs.
Hybrid models that combine GCMs with machine learning are appealing because they build on the interpretability, extensibility and successful track record of traditional atmospheric models 19 , 20 . In the hybrid model approach, a machine-learning component replaces or corrects the traditional physical parameterizations of a GCM. Until now, the machine-learning component in such models has been trained ‘offline’, by learning parameterizations independently of their interaction with dynamics. These components are then inserted into an existing GCM. The lack of coupling between machine-learning components and the governing equations during training potentially causes serious problems, such as instability and climate drift 21 . So far, hybrid models have mostly been limited to idealized scenarios such as aquaplanets 22 , 23 . Under realistic conditions, machine-learning corrections have reduced some biases of very coarse GCMs 24 , 25 , 26 , but performance remains considerably worse than state-of-the-art models.
Here we present NeuralGCM, a fully differentiable hybrid GCM of Earth’s atmosphere. NeuralGCM is trained on forecasting up to 5-day weather trajectories sampled from ERA5. Differentiability enables end-to-end ‘online training’ 27 , with machine-learning components optimized in the context of interactions with the governing equations for large-scale dynamics, which we find enables accurate and stable forecasts. NeuralGCM produces physically consistent forecasts with accuracy comparable to best-in-class models across a range of timescales, from 1- to 15-day weather to decadal climate prediction.
Neural GCMs
A schematic of NeuralGCM is shown in Fig. 1 . The two key components of NeuralGCM are a differentiable dynamical core for solving the discretized governing dynamical equations and a learned physics module that parameterizes physical processes with a neural network, described in full detail in Methods , Supplementary Information sections B and C , and Supplementary Table 1 . The dynamical core simulates large-scale fluid motion and thermodynamics under the influence of gravity and the Coriolis force. The learned physics module (Supplementary Fig. 1 ) predicts the effect of unresolved processes, such as cloud formation, radiative transport, precipitation and subgrid-scale dynamics, on the simulated fields using a neural network.
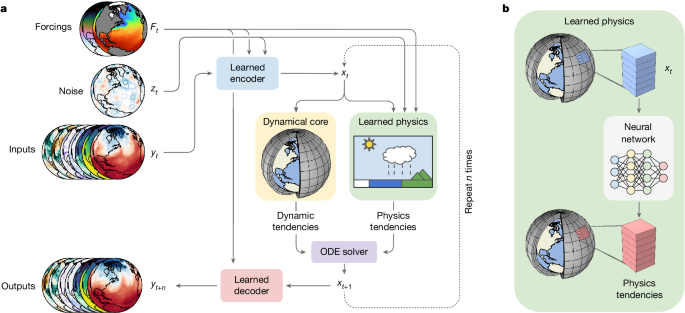
a , Overall model structure, showing how forcings F t , noise z t (for stochastic models) and inputs y t are encoded into the model state x t . The model state is fed into the dynamical core, and alongside forcings and noise into the learned physics module. This produces tendencies (rates of change) used by an implicit–explicit ordinary differential equation (ODE) solver to advance the state in time. The new model state x t +1 can then be fed back into another time step, or decoded into model predictions. b , The learned physics module, which feeds data for individual columns of the atmosphere into a neural network used to produce physics tendencies in that vertical column.
The differentiable dynamical core in NeuralGCM allows an end-to-end training approach, whereby we advance the model multiple time steps before employing stochastic gradient descent to minimize discrepancies between model predictions and reanalysis (Supplementary Information section G.2 ). We gradually increase the rollout length from 6 hours to 5 days (Supplementary Information section G and Supplementary Table 5 ), which we found to be critical because our models are not accurate for multi-day prediction or stable for long rollouts early in training (Supplementary Information section H.6.2 and Supplementary Fig. 23 ). The extended back-propagation through hundreds of simulation steps enables our neural networks to take into account interactions between the learned physics and the dynamical core. We train deterministic and stochastic NeuralGCM models, each of which uses a distinct training protocol, described in full detail in Methods and Supplementary Table 4 .
We train a range of NeuralGCM models at horizontal resolutions with grid spacing of 2.8°, 1.4° and 0.7° (Supplementary Fig. 7 ). We evaluate the performance of NeuralGCM at a range of timescales appropriate for weather forecasting and climate simulation. For weather, we compare against the best-in-class conventional physics-based weather models, ECMWF’s high-resolution model (ECMWF-HRES) and ensemble prediction system (ECMWF-ENS), and two of the recent machine-learning-based approaches, GraphCast 3 and Pangu 4 . For climate, we compare against a global cloud-resolving model and Atmospheric Model Intercomparison Project (AMIP) runs.
Medium-range weather forecasting
Our evaluation set-up focuses on quantifying accuracy and physical consistency, following WeatherBench2 12 . We regrid all forecasts to a 1.5° grid using conservative regridding, and average over all 732 forecasts made at noon and midnight UTC in the year 2020, which was held-out from training data for all machine-learning models. NeuralGCM, GraphCast and Pangu compare with ERA5 as the ground truth, whereas ECMWF-ENS and ECMWF-HRES compare with the ECMWF operational analysis (that is, HRES at 0-hour lead time), to avoid penalizing the operational forecasts for different biases than ERA5.
Model accuracy
We use ECMWF’s ensemble (ENS) model as a reference baseline as it achieves the best performance across the majority of lead times 12 . We assess accuracy using (1) root-mean-squared error (RMSE), (2) root-mean-squared bias (RMSB), (3) continuous ranked probability score (CRPS) and (4) spread-skill ratio, with the results shown in Fig. 2 . We provide more in-depth evaluations including scorecards, metrics for additional variables and levels and maps in Extended Data Figs. 1 and 2 , Supplementary Information section H and Supplementary Figs. 9 – 22 .
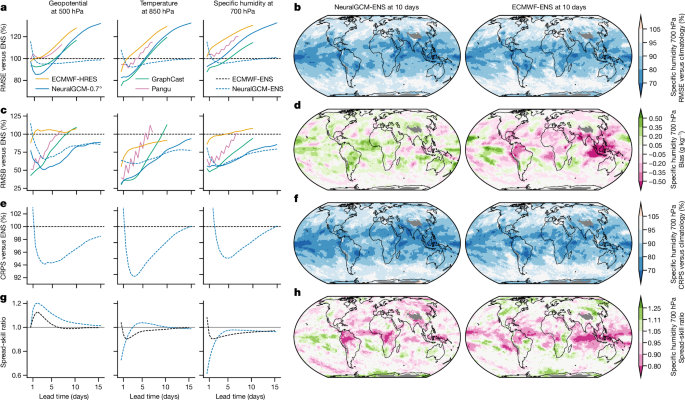
a , c , RMSE ( a ) and RMSB ( c ) for ECMWF-ENS, ECMWF-HRES, NeuralGCM-0.7°, NeuralGCM-ENS, GraphCast 3 and Pangu 4 on headline WeatherBench2 variables, as a percentage of the error of ECMWF-ENS. Deterministic and stochastic models are shown in solid and dashed lines respectively. e , g , CRPS relative to ECMWF-ENS ( e ) and spread-skill ratio for the ENS and NeuralGCM-ENS models ( g ). b , d , f , h , Spatial distributions of RMSE ( b ), bias ( d ), CRPS ( f ) and spread-skill ratio ( h ) for NeuralGCM-ENS and ECMWF-ENS models for 10-day forecasts of specific humidity at 700 hPa. Spatial plots of RMSE and CRPS show skill relative to a probabilistic climatology 12 with an ensemble member for each of the years 1990–2019. The grey areas indicate regions where climatological surface pressure on average is below 700 hPa.
Deterministic models that produce a single weather forecast for given initial conditions can be compared effectively using RMSE skill at short lead times. For the first 1–3 days, depending on the atmospheric variable, RMSE is minimized by forecasts that accurately track the evolution of weather patterns. At this timescale we find that NeuralGCM-0.7° and GraphCast achieve best results, with slight variations across different variables (Fig. 2a ). At longer lead times, RMSE rapidly increases owing to chaotic divergence of nearby weather trajectories, making RMSE less informative for deterministic models. RMSB calculates persistent errors over time, which provides an indication of how models would perform at much longer lead times. Here NeuralGCM models also compare favourably against previous approaches (Fig. 2c ), with notably much less bias for specific humidity in the tropics (Fig. 2d ).
Ensembles are essential for capturing intrinsic uncertainty of weather forecasts, especially at longer lead times. Beyond about 7 days, the ensemble means of ECMWF-ENS and NeuralGCM-ENS forecasts have considerably lower RMSE than the deterministic models, indicating that these models better capture the average of possible weather. A better metric for ensemble models is CRPS, which is a proper scoring rule that is sensitive to full marginal probability distributions 28 . Our stochastic model (NeuralGCM-ENS) running at 1.4° resolution has lower error compared with ECMWF-ENS across almost all variables, lead times and vertical levels for ensemble-mean RMSE, RSMB and CRPS (Fig. 2a,c,e and Supplementary Information section H ), with similar spatial patterns of skill (Fig. 2b,f ). Like ECMWF-ENS, NeuralGCM-ENS has a spread-skill ratio of approximately one (Fig. 2d ), which is a necessary condition for calibrated forecasts 29 .
An important characteristic of forecasts is their resemblance to realistic weather patterns. Figure 3 shows a case study that illustrates the performance of NeuralGCM on three types of important weather phenomenon: tropical cyclones, atmospheric rivers and the Intertropical Convergence Zone. Figure 3a shows that all the machine-learning models make significantly blurrier forecasts than the source data ERA5 and physics-based ECMWF-HRES forecast, but NeuralCGM-0.7° outperforms the pure machine-learning models, despite its coarser resolution (0.7° versus 0.25° for GraphCast and Pangu). Blurry forecasts correspond to physically inconsistent atmospheric conditions and misrepresent extreme weather. Similar trends hold for other derived variables of meteorological interest (Supplementary Information section H.2 ). Ensemble-mean predictions, from both NeuralGCM and ECMWF, are closer to ERA5 in an average sense, and thus are inherently smooth at long lead times. In contrast, as shown in Fig. 3 and in Supplementary Information section H.3 , individual realizations from the ECMWF and NeuralGCM ensembles remain sharp, even at long lead times. Like ECMWF-ENS, NeuralGCM-ENS produces a statistically representative range of future weather scenarios for each weather phenomenon, despite its eight-times-coarser resolution.
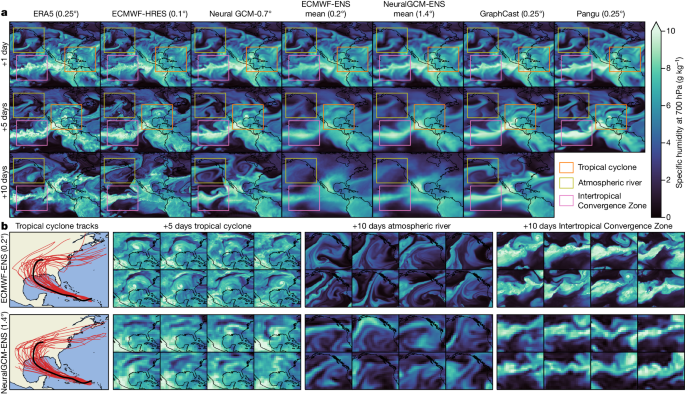
All forecasts are initialized at 2020-08-22T12z, chosen to highlight Hurricane Laura, the most damaging Atlantic hurricane of 2020. a , Specific humidity at 700 hPa for 1-day, 5-day and 10-day forecasts over North America and the Northeast Pacific Ocean from ERA5 14 , ECMWF-HRES, NeuralGCM-0.7°, ECMWF-ENS (mean), NeuralGCM-ENS (mean), GraphCast 3 and Pangu 4 . b , Forecasts from individual ensemble members from ECMWF-ENS and NeuralGCM-ENS over regions of interest, including predicted tracks of Hurricane Laura from each of the 50 ensemble members (Supplementary Information section I.2 ). The track from ERA5 is plotted in black.
We can quantify the blurriness of different forecast models via their power spectra. Supplementary Figs. 17 and 18 show that the power spectra of NeuralCGM-0.7° is consistently closer to ERA5 than the other machine-learning forecast methods, but is still blurrier than ECMWF’s physical forecasts. The spectra of NeuralGCM forecasts is also roughly constant over the forecast period, in stark contrast to GraphCast, which worsens with lead time. The spectrum of NeuralGCM becomes more accurate with increased resolution (Supplementary Fig. 22 ), which suggests the potential for further improvements of NeuralGCM models trained at higher resolutions.
Water budget
In NeuralGCM, advection is handled by the dynamical core, while the machine-learning parameterization models local processes within vertical columns of the atmosphere. Thus, unlike pure machine-learning methods, local sources and sinks can be isolated from tendencies owing to horizontal transport and other resolved dynamics (Supplementary Fig. 3 ). This makes our results more interpretable and facilitates the diagnosis of the water budget. Specifically, we diagnose precipitation minus evaporation (Supplementary Information section H.5 ) rather than directly predicting these as in machine-learning-based approaches 3 . For short weather forecasts, the mean of precipitation minus evaporation has a realistic spatial distribution that is very close to ERA5 data (Extended Data Fig. 4c–e ). The precipitation-minus-evaporation rate distribution of NeuralGCM-0.7° closely matches the ERA5 distribution in the extratropics (Extended Data Fig. 4b ), although it underestimates extreme events in the tropics (Extended Data Fig. 4a ). It is noted that the current version of NeuralGCM directly predicts tendencies for an atmospheric column, and thus cannot distinguish between precipitation and evaporation.
Geostrophic wind balance
We examined the extent to which NeuralGCM, GraphCast and ECMWF-HRES capture the geostrophic wind balance, the near-equilibrium between the dominant forces that drive large-scale dynamics in the mid-latitudes 30 . A recent study 16 highlighted that Pangu misrepresents the vertical structure of the geostrophic and ageostrophic winds and noted a deterioration at longer lead times. Similarly, we observe that GraphCast shows an error that worsens with lead time. In contrast, NeuralGCM more accurately depicts the vertical structure of the geostrophic and ageostrophic winds, as well as their ratio, compared with GraphCast across various rollouts, when compared against ERA5 data (Extended Data Fig. 3 ). However, ECMWF-HRES still shows a slightly closer alignment to ERA5 data than NeuralGCM does. Within NeuralGCM, the representation of the geostrophic wind’s vertical structure only slightly degrades in the initial few days, showing no noticeable changes thereafter, particularly beyond day 5.
Generalizing to unseen data
Physically consistent weather models should still perform well for weather conditions for which they were not trained. We expect that NeuralGCM may generalize better than machine-learning-only atmospheric models, because NeuralGCM employs neural networks that act locally in space, on individual vertical columns of the atmosphere. To explore this hypothesis, we compare versions of NeuralCGM-0.7° and GraphCast trained to 2017 on 5 years of weather forecasts beyond the training period (2018–2022) in Supplementary Fig. 36 . Unlike GraphCast, NeuralGCM does not show a clear trend of increasing error when initialized further into the future from the training data. To extend this test beyond 5 years, we trained a NeuralGCM-2.8° model using only data before 2000, and tested its skill for over 21 unseen years (Supplementary Fig. 35 ).
Climate simulations
Although our deterministic NeuralGCM models are trained to predict weather up to 3 days ahead, they are generally capable of simulating the atmosphere far beyond medium-range weather timescales. For extended climate simulations, we prescribe historical sea surface temperature (SST) and sea-ice concentration. These simulations feature many emergent phenomena of the atmosphere on timescales from months to decades.
For climate simulations with NeuralGCM, we use 2.8° and 1.4° deterministic models, which are relatively inexpensive to train (Supplementary Information section G.7 ) and allow us to explore a larger parameter space to find stable models. Previous studies found that running extended simulations with hybrid models is challenging due to numerical instabilities and climate drift 21 . To quantify stability in our selected models, we run multiple initial conditions and report how many of them finish without instability.
Seasonal cycle and emergent phenomena
To assess the capability of NeuralGCM to simulate various aspects of the seasonal cycle, we run 2-year simulations with NeuralGCM-1.4°. for 37 different initial conditions spaced every 10 days for the year 2019. Out of these 37 initial conditions, 35 successfully complete the full 2 years without instability; for case studies of instability, see Supplementary Information section H.7 , and Supplementary Figs. 26 and 27 . We compare results from NeuralGCM-1.4° for 2020 with ERA5 data and with outputs from the X-SHiELD global cloud-resolving model, which is coupled to an ocean model nudged towards reanalysis 31 . This X-SHiELD run has been used as a target for training machine-learning climate models 24 . For comparison, we evaluate models after regridding predictions to 1.4° resolution. This comparison slightly favours NeuralGCM because NeuralGCM was tuned to match ERA5, but the discrepancy between ERA5 and the actual atmosphere is small relative to model error.
Figure 4a shows the temporal variation of the global mean temperature to 2020, as captured by 35 simulations from NeuralGCM, in comparison with the ERA5 reanalysis and standard climatology benchmarks. The seasonality and variability of the global mean temperature from NeuralGCM are quantitatively similar to those observed in ERA5. The ensemble-mean temperature RMSE for NeuralGCM stands at 0.16 K when benchmarked against ERA5, which is a significant improvement over the climatology’s RMSE of 0.45 K. We find that NeuralGCM accurately simulates the seasonal cycle, as evidenced by metrics such as the annual cycle of the global precipitable water (Supplementary Fig. 30a ) and global total kinetic energy (Supplementary Fig. 30b ). Furthermore, the model captures essential atmospheric dynamics, including the Hadley circulation and the zonal-mean zonal wind (Supplementary Fig. 28 ), as well as the spatial patterns of eddy kinetic energy in different seasons (Supplementary Fig. 31 ), and the distinctive seasonal behaviours of monsoon circulation (Supplementary Fig. 29 ; additional details are provided in Supplementary Information section I.1 ).
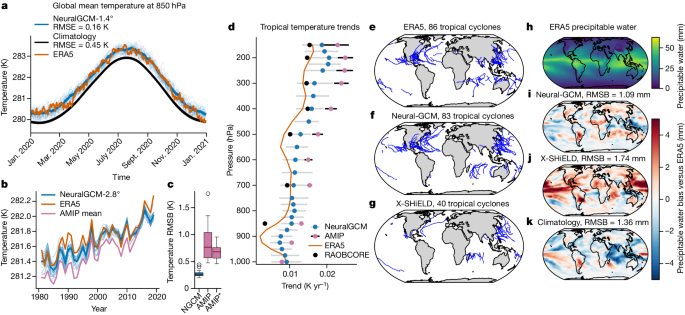
a , Global mean temperature for ERA5 14 (orange), 1990–2019 climatology (black) and NeuralGCM-1.4° (blue) for 2020 using 35 simulations initialized every 10 days during 2019 (thick line, ensemble mean; thin lines, different initial conditions). b , Yearly global mean temperature for ERA5 (orange), mean over 22 CMIP6 AMIP experiments 34 (violet; model details are in Supplementary Information section I.3 ) and NeuralGCM-2.8° for 22 AMIP-like simulations with prescribed SST initialized every 10 days during 1980 (thick line, ensemble mean; thin lines, different initial conditions). c , The RMSB of the 850-hPa temperature averaged between 1981 and 2014 for 22 NeuralGCM-2.8° AMIP runs (labelled NGCM), 22 CMIP6 AMIP experiments (labelled AMIP) and debiased 22 CMIP6 AMIP experiments (labelled AMIP*; bias was removed by removing the 850-hPa global temperature bias). In the box plots, the red line represents the median. The box delineates the first to third quartiles; the whiskers extend to 1.5 times the interquartile range (Q1 − 1.5IQR and Q3 + 1.5IQR), and outliers are shown as individual dots. d , Vertical profiles of tropical (20° S–20° N) temperature trends for 1981–2014. Orange, ERA5; black dots, Radiosonde Observation Correction using Reanalyses (RAOBCORE) 41 ; blue dots, mean trends for NeuralGCM; purple dots, mean trends from CMIP6 AMIP runs (grey and black whiskers, 25th and 75th percentiles for NeuralGCM and CMIP6 AMIP runs, respectively). e – g , Tropical cyclone tracks for ERA5 ( e ), NeuralGCM-1.4° ( f ) and X-SHiELD 31 ( g ). h – k , Mean precipitable water for ERA5 ( h ) and the precipitable water bias in NeuralGCM-1.4° ( i ), initialized 90 days before mid-January 2020 similarly to X-SHiELD, X-SHiELD ( j ) and climatology ( k ; averaged between 1990 and 2019). In d – i , quantities are calculated between mid-January 2020 and mid-January 2021 and all models were regridded to a 256 × 128 Gaussian grid before computation and tracking.
Next, we compare the annual biases of a single NeuralGCM realization with a single realization of X-SHiELD (the only one available), both initiated in mid-October 2019. We consider 19 January 2020 to 17 January 2021, the time frame for which X-SHiELD data are available. Global cloud-resolving models, such as X-SHiELD, are considered state of the art, especially for simulating the hydrological cycle, owing to their resolution being capable of resolving deep convection 32 . The annual bias in precipitable water for NeuralGCM (RMSE of 1.09 mm) is substantially smaller than the biases of both X-SHiELD (RMSE of 1.74 mm) and climatology (RMSE of 1.36 mm; Fig. 4i–k ). Moreover, NeuralGCM shows a lower temperature bias in the upper and lower troposphere than X-SHiELD (Extended Data Fig. 6 ). We also indirectly compare precipitation bias in X-SHiELD with precipitation-minus-evaporation bias in NeuralGCM-1.4°, which shows slightly larger bias and grid-scale artefacts for NeuralGCM (Extended Data Fig. 5 ).
Finally, to assess the capability of NeuralGCM to generate tropical cyclones in an annual model integration, we use the tropical cyclone tracker TempestExtremes 33 , as described in Supplementary Information section I.2 , Supplementary Fig. 34 and Supplementary Table 6 . Figure 4e–g shows that NeuralGCM, even at a coarse resolution of 1.4°, produces realistic trajectories and counts of tropical cyclone (83 versus 86 in ERA5 for the corresponding period), whereas X-SHiELD, when regridded to 1.4° resolution, substantially underestimates the tropical cyclone count (40). Additional statistical analyses of tropical cyclones can be found in Extended Data Figs. 7 and 8 .
Decadal simulations
To assess the capability of NeuralGCM to simulate historical temperature trends, we conduct AMIP-like simulations over a duration of 40 years with NeuralGCM-2.8°. Out of 37 different runs with initial conditions spaced every 10 days during the year 1980, 22 simulations were stable for the entire 40-year period, and our analysis focuses on these results. We compare with 22 simulations run with prescribed SST from the Coupled Model Intercomparison Project Phase 6 (CMIP6) 34 , listed in Supplementary Information section I.3 .
We find that all 40-year simulations of NeuralGCM, as well as the mean of the 22 AMIP runs, accurately capture the global warming trends observed in ERA5 data (Fig. 4b ). There is a strong correlation in the year-to-year temperature trends with ERA5 data, suggesting that NeuralGCM effectively captures the impact of SST forcing on climate. When comparing spatial biases averaged over 1981–2014, we find that all 22 NeuralGCM-2.8° runs have smaller bias than the CMIP6 AMIP runs, and this result remains even when removing the global temperature bias in CMIP6 AMIP runs (Fig. 4c and Supplementary Figs. 32 and 33 ).
Next, we investigated the vertical structure of tropical warming trends, which climate models tend to overestimate in the upper troposphere 35 . As shown in Fig. 4d , the trends, calculated by linear regression, of NeuralGCM are closer to ERA5 than those of AMIP runs. In particular, the bias in the upper troposphere is reduced. However, NeuralGCM does show a wider spread in its predictions than the AMIP runs, even at levels near the surface where temperatures are typically more constrained by prescribed SST.
Lastly, we evaluated NeuralGCM’s capability to generalize to unseen warmer climates by conducting AMIP simulations with increased SST (Supplementary Information section I.4.2 ). We find that NeuralGCM shows some of the robust features of climate warming response to modest SST increases (+1 K and +2 K); however, for more substantial SST increases (+4 K), NeuralGCM’s response diverges from expectations (Supplementary Fig. 37 ). In addition, AMIP simulations with increased SST show climate drift, underscoring NeuralGCM’s limitations in this context (Supplementary Fig. 38 ).
NeuralGCM is a differentiable hybrid atmospheric model that combines the strengths of traditional GCMs with machine learning for weather forecasting and climate simulation. To our knowledge, NeuralGCM is the first machine-learning-based model to make accurate ensemble weather forecasts, with better CRPS than state-of-the-art physics-based models. It is also, to our knowledge, the first hybrid model that achieves comparable spatial bias to global cloud-resolving models, can simulate realistic tropical cyclone tracks and can run AMIP-like simulations with realistic historical temperature trends. Overall, NeuralGCM demonstrates that incorporating machine learning is a viable alternative to building increasingly detailed physical models 32 for improving GCMs.
Compared with traditional GCMs with similar skill, NeuralGCM is computationally efficient and low complexity. NeuralGCM runs at 8- to 40-times-coarser horizontal resolution than ECMWF’s Integrated Forecasting System and global cloud-resolving models, which enables 3 to 5 orders of magnitude savings in computational resources. For example, NeuralGCM-1.4° simulates 70,000 simulation days in 24 hours using a single tensor-processing-unit versus 19 simulated days on 13,824 central-processing-unit cores with X-SHiELD (Extended Data Table 1 ). This can be leveraged for previously impractical tasks such as large ensemble forecasting. NeuralGCM’s dynamical core uses global spectral methods 36 , and learned physics is parameterized with fully connected neural networks acting on single vertical columns. Substantial headroom exists to pursue higher accuracy using advanced numerical methods and machine-learning architectures.
Our results provide strong evidence for the disputed hypothesis 37 , 38 , 39 that learning to predict short-term weather is an effective way to tune parameterizations for climate. NeuralGCM models trained on 72-hour forecasts are capable of realistic multi-year simulation. When provided with historical SSTs, they capture essential atmospheric dynamics such as seasonal circulation, monsoons and tropical cyclones. However, we will probably need alternative training strategies 38 , 39 to learn important processes for climate with subtle impacts on weather timescales, such as a cloud feedback.
The NeuralGCM approach is compatible with incorporating either more physics or more machine learning, as required for operational weather forecasts and climate simulations. For weather forecasting, we expect that end-to-end learning 40 with observational data will allow for better and more relevant predictions, including key variables such as precipitation. Such models could include neural networks acting as corrections to traditional data assimilation and model diagnostics. For climate projection, NeuralGCM will need to be reformulated to enable coupling with other Earth-system components (for example, ocean and land), and integrating data on the atmospheric chemical composition (for example, greenhouse gases and aerosols). There are also research challenges common to current machine-learning-based climate models 19 , including the capability to simulate unprecedented climates (that is, generalization), adhering to physical constraints, and resolving numerical instabilities and climate drift. NeuralGCM’s flexibility to incorporate physics-based models (for example, radiation) offers a promising avenue to address these challenges.
Models based on physical laws and empirical relationships are ubiquitous in science. We believe the differentiable hybrid modelling approach of NeuralGCM has the potential to transform simulation for a wide range of applications, such as materials discovery, protein folding and multiphysics engineering design.
Differentiable atmospheric model
NeuralGCM combines components of the numerical solver and flexible neural network parameterizations. Simulation in time is carried out in a coordinate system suitable for solving the dynamical equations of the atmosphere, describing large-scale fluid motion and thermodynamics under the influence of gravity and the Coriolis force.
Our differentiable dynamical core is implemented in JAX, a library for high-performance code in Python that supports automatic differentiation 42 . The dynamical core solves the hydrostatic primitive equations with moisture, using a horizontal pseudo-spectral discretization and vertical sigma coordinates 36 , 43 . We evolve seven prognostic variables: vorticity and divergence of horizontal wind, temperature, surface pressure, and three water species (specific humidity, and specific ice and liquid cloud water content).
Our learned physics module uses the single-column approach of GCMs 2 , whereby information from only a single atmospheric column is used to predict the impact of unresolved processes occurring within that column. These effects are predicted using a fully connected neural network with residual connections, with weights shared across all atmospheric columns (Supplementary Information section C.4 ).
The inputs to the neural network include the prognostic variables in the atmospheric column, total incident solar radiation, sea-ice concentration and SST (Supplementary Information section C.1 ). We also provide horizontal gradients of the prognostic variables, which we found improves performance 44 . All inputs are standardized to have zero mean and unit variance using statistics precomputed during model initialization. The outputs are the prognostic variable tendencies scaled by the fixed unconditional standard deviation of the target field (Supplementary Information section C.5 ).
To interface between ERA5 14 data stored in pressure coordinates and the sigma coordinate system of our dynamical core, we introduce encoder and decoder components (Supplementary Information section D ). These components perform linear interpolation between pressure levels and sigma coordinate levels. We additionally introduce learned corrections to both encoder and decoder steps (Supplementary Figs. 4–6 ), using the same column-based neural network architecture as the learned physics module. Importantly, the encoder enables us to eliminate the gravity waves from initialization shock 45 , which otherwise contaminate forecasts.
Figure 1a shows the sequence of steps that NeuralGCM takes to make a forecast. First, it encodes ERA5 data at t = t 0 on pressure levels to initial conditions on sigma coordinates. To perform a time step, the dynamical core and learned physics (Fig. 1b ) then compute tendencies, which are integrated in time using an implicit–explicit ordinary differential equation solver 46 (Supplementary Information section E and Supplementary Table 2 ). This is repeated to advance the model from t = t 0 to t = t final . Finally, the decoder converts predictions back to pressure levels.
The time-step size of the ODE solver (Supplementary Table 3 ) is limited by the Courant–Friedrichs–Lewy condition on dynamics, and can be small relative to the timescale of atmospheric change. Evaluating learned physics is approximately 1.5 times as expensive as a time step of the dynamical core. Accordingly, following the typical practice for GCMs, we hold learned physics tendencies constant for multiple ODE time steps to reduce computational expense, typically corresponding to 30 minutes of simulation time.
Deterministic and stochastic models
We train deterministic NeuralGCM models using a combination of three loss functions (Supplementary Information section G.4 ) to encourage accuracy and sharpness while penalizing bias. During the main training phase, all losses are defined in a spherical harmonics basis. We use a standard mean squared error loss for prompting accuracy, modified to progressively filter out contributions from higher total wavenumbers at longer lead times (Supplementary Fig. 8 ). This filtering approach tackles the ‘double penalty problem’ 47 as it prevents the model from being penalized for predicting high-wavenumber features in incorrect locations at later times, especially beyond the predictability horizon. A second loss term encourages the spectrum to match the training data using squared loss on the total wavenumber spectrum of prognostic variables. These first two losses are evaluated on both sigma and pressure levels. Finally, a third loss term discourages bias by adding mean squared error on the batch-averaged mean amplitude of each spherical harmonic coefficient. For analysis of the impact that various loss functions have, refer to Supplementary Information section H.6.1 , and Supplementary Figs. 23 and 24 . The combined action of the three training losses allow the resulting models trained on 3-day rollouts to remain stable during years-to-decades-long climate simulations. Before final evaluations, we perform additional fine-tuning of just the decoder component on short rollouts of 24 hours (Supplementary Information section G.5 ).
Stochastic NeuralGCM models incorporate inherent randomness in the form of additional random fields passed as inputs to neural network components. Our stochastic loss is based on the CRPS 28 , 48 , 49 . CRPS consists of mean absolute error that encourages accuracy, balanced by a similar term that encourages ensemble spread. For each variable we use a sum of CRPS in grid space and CRPS in the spherical harmonic basis below a maximum cut-off wavenumber (Supplementary Information section G.6 ). We compute CRPS on rollout lengths from 6 hours to 5 days. As illustrated in Fig. 1 , we inject noise to the learned encoder and the learned physics module by sampling from Gaussian random fields with learned spatial and temporal correlation (Supplementary Information section C.2 and Supplementary Fig. 2 ). For training, we generate two ensemble members per forecast, which suffices for an unbiased estimate of CRPS.
Data availability
For training and evaluating the NeuralGCM models, we used the publicly available ERA5 dataset 14 , originally downloaded from https://cds.climate.copernicus.eu/ and available via Google Cloud Storage in Zarr format at gs://gcp-public-data-arco-era5/ar/full_37-1h-0p25deg-chunk-1.zarr-v3. To compare NeuralGCM with operational and data-driven weather models, we used forecast datasets distributed as part of WeatherBench2 12 at https://weatherbench2.readthedocs.io/en/latest/data-guide.html , to which we have added NeuralGCM forecasts for 2020. To compare NeuralGCM with atmospheric models in climate settings, we used CMIP6 data available at https://catalog.pangeo.io/browse/master/climate/ , as well as X-SHiELD 24 outputs available on Google Cloud storage in a ‘requester pays’ bucket at gs://ai2cm-public-requester-pays/C3072-to-C384-res-diagnostics. The Radiosonde Observation Correction using Reanalyses (RAOBCORE) V1.9 that was used as reference tropical temperature trends was downloaded from https://webdata.wolke.img.univie.ac.at/haimberger/v1.9/ . Base maps use freely available data from https://www.naturalearthdata.com/downloads/ .
Code availability
The NeuralGCM code base is separated into two open source projects: Dinosaur and NeuralGCM, both publicly available on GitHub at https://github.com/google-research/dinosaur (ref. 50 ) and https://github.com/google-research/neuralgcm (ref. 51 ). The Dinosaur package implements a differentiable dynamical core used by NeuralGCM, whereas the NeuralGCM package provides machine-learning models and checkpoints of trained models. Evaluation code for NeuralGCM weather forecasts is included in WeatherBench2 12 , available at https://github.com/google-research/weatherbench2 (ref. 52 ).
Bauer, P., Thorpe, A. & Brunet, G. The quiet revolution of numerical weather prediction. Nature 525 , 47–55 (2015).
Article ADS CAS PubMed Google Scholar
Balaji, V. et al. Are general circulation models obsolete? Proc. Natl Acad. Sci. USA 119 , e2202075119 (2022).
Article CAS PubMed PubMed Central Google Scholar
Lam, R. et al. Learning skillful medium-range global weather forecasting. Science 382 , 1416–1421 (2023).
Article ADS MathSciNet CAS PubMed Google Scholar
Bi, K. et al. Accurate medium-range global weather forecasting with 3D neural networks. Nature 619 , 533–538 (2023).
Article ADS CAS PubMed PubMed Central Google Scholar
Hourdin, F. et al. The art and science of climate model tuning. Bull. Am. Meteorol. Soc. 98 , 589–602 (2017).
Article ADS Google Scholar
Bony, S. & Dufresne, J.-L. Marine boundary layer clouds at the heart of tropical cloud feedback uncertainties in climate models. Geophys. Res. Lett. 32 , L20806 (2005).
Webb, M. J., Lambert, F. H. & Gregory, J. M. Origins of differences in climate sensitivity, forcing and feedback in climate models. Clim. Dyn. 40 , 677–707 (2013).
Article Google Scholar
Sherwood, S. C., Bony, S. & Dufresne, J.-L. Spread in model climate sensitivity traced to atmospheric convective mixing. Nature 505 , 37–42 (2014).
Article ADS PubMed Google Scholar
Palmer, T. & Stevens, B. The scientific challenge of understanding and estimating climate change. Proc. Natl Acad. Sci. USA 116 , 24390–24395 (2019).
Fischer, E. M., Beyerle, U. & Knutti, R. Robust spatially aggregated projections of climate extremes. Nat. Clim. Change 3 , 1033–1038 (2013).
Field, C. B. Managing the Risks of Extreme Events and Disasters to Advance Climate Change Adaptation: Special Report of the Intergovernmental Panel on Climate Change (Cambridge Univ. Press, 2012).
Rasp, S. et al. WeatherBench 2: A benchmark for the next generation of data-driven global weather models. J. Adv. Model. Earth Syst. 16 , e2023MS004019 (2024).
Keisler, R. Forecasting global weather with graph neural networks. Preprint at https://arxiv.org/abs/2202.07575 (2022).
Hersbach, H. et al. The ERA5 global reanalysis. Q. J. R. Meteorol. Soc. 146 , 1999–2049 (2020).
Zhou, L. et al. Toward convective-scale prediction within the next generation global prediction system. Bull. Am. Meteorol. Soc. 100 , 1225–1243 (2019).
Bonavita, M. On some limitations of current machine learning weather prediction models. Geophys. Res. Lett. 51 , e2023GL107377 (2024).
Weyn, J. A., Durran, D. R. & Caruana, R. Improving data-driven global weather prediction using deep convolutional neural networks on a cubed sphere. J. Adv. Model. Earth Syst. 12 , e2020MS002109 (2020).
Watt-Meyer, O. et al. ACE: a fast, skillful learned global atmospheric model for climate prediction. Preprint at https://arxiv.org/abs/2310.02074 (2023).
Bretherton, C. S. Old dog, new trick: reservoir computing advances machine learning for climate modeling. Geophys. Res. Lett. 50 , e2023GL104174 (2023).
Reichstein, M. et al. Deep learning and process understanding for data-driven Earth system science. Nature 566 , 195–204 (2019).
Brenowitz, N. D. & Bretherton, C. S. Spatially extended tests of a neural network parametrization trained by coarse-graining. J. Adv. Model. Earth Syst. 11 , 2728–2744 (2019).
Rasp, S., Pritchard, M. S. & Gentine, P. Deep learning to represent subgrid processes in climate models. Proc. Natl Acad. Sci. USA 115 , 9684–9689 (2018).
Yuval, J. & O’Gorman, P. A. Stable machine-learning parameterization of subgrid processes for climate modeling at a range of resolutions. Nat. Commun. 11 , 3295 (2020).
Kwa, A. et al. Machine-learned climate model corrections from a global storm-resolving model: performance across the annual cycle. J. Adv. Model. Earth Syst. 15 , e2022MS003400 (2023).
Arcomano, T., Szunyogh, I., Wikner, A., Hunt, B. R. & Ott, E. A hybrid atmospheric model incorporating machine learning can capture dynamical processes not captured by its physics-based component. Geophys. Res. Lett. 50 , e2022GL102649 (2023).
Han, Y., Zhang, G. J. & Wang, Y. An ensemble of neural networks for moist physics processes, its generalizability and stable integration. J. Adv. Model. Earth Syst. 15 , e2022MS003508 (2023).
Gelbrecht, M., White, A., Bathiany, S. & Boers, N. Differentiable programming for Earth system modeling. Geosci. Model Dev. 16 , 3123–3135 (2023).
Gneiting, T. & Raftery, A. E. Strictly proper scoring rules, prediction, and estimation. J. Am. Stat. Assoc. 102 , 359–378 (2007).
Article MathSciNet CAS Google Scholar
Fortin, V., Abaza, M., Anctil, F. & Turcotte, R. Why should ensemble spread match the RMSE of the ensemble mean? J. Hydrometeorol. 15 , 1708–1713 (2014).
Holton, J. R. An introduction to Dynamic Meteorology 5th edn (Elsevier, 2004).
Cheng, K.-Y. et al. Impact of warmer sea surface temperature on the global pattern of intense convection: insights from a global storm resolving model. Geophys. Res. Lett. 49 , e2022GL099796 (2022).
Stevens, B. et al. DYAMOND: the dynamics of the atmospheric general circulation modeled on non-hydrostatic domains. Prog. Earth Planet. Sci. 6 , 61 (2019).
Ullrich, P. A. et al. TempestExtremes v2.1: a community framework for feature detection, tracking, and analysis in large datasets. Geosc. Model Dev. 14 , 5023–5048 (2021).
Eyring, V. et al. Overview of the Coupled Model Intercomparison Project Phase 6 (CMIP6) experimental design and organization. Geosci. Model Dev. 9 , 1937–1958 (2016).
Mitchell, D. M., Lo, Y. E., Seviour, W. J., Haimberger, L. & Polvani, L. M. The vertical profile of recent tropical temperature trends: persistent model biases in the context of internal variability. Environ. Res. Lett. 15 , 1040b4 (2020).
Bourke, W. A multi-level spectral model. I. Formulation and hemispheric integrations. Mon. Weather Rev. 102 , 687–701 (1974).
Ruiz, J. J., Pulido, M. & Miyoshi, T. Estimating model parameters with ensemble-based data assimilation: a review. J. Meteorol. Soc. Jpn Ser. II 91 , 79–99 (2013).
Schneider, T., Lan, S., Stuart, A. & Teixeira, J. Earth system modeling 2.0: a blueprint for models that learn from observations and targeted high-resolution simulations. Geophys. Res. Lett. 44 , 12–396 (2017).
Schneider, T., Leung, L. R. & Wills, R. C. J. Opinion: Optimizing climate models with process knowledge, resolution, and artificial intelligence. Atmos. Chem. Phys. 24 , 7041–7062 (2024).
Sutskever, I., Vinyals, O. & Le, Q. V. Sequence to sequence learning with neural networks. Adv. Neural Inf. Process. Syst. 27 , 3104–3112 (2014).
Haimberger, L., Tavolato, C. & Sperka, S. Toward elimination of the warm bias in historic radiosonde temperature records—some new results from a comprehensive intercomparison of upper-air data. J. Clim. 21 , 4587–4606 (2008).
Bradbury, J. et al. JAX: composable transformations of Python+NumPy programs. GitHub http://github.com/google/jax (2018).
Durran, D. R. Numerical Methods for Fluid Dynamics: With Applications to Geophysics Vol. 32, 2nd edn (Springer, 2010).
Wang, P., Yuval, J. & O’Gorman, P. A. Non-local parameterization of atmospheric subgrid processes with neural networks. J. Adv. Model. Earth Syst. 14 , e2022MS002984 (2022).
Daley, R. Normal mode initialization. Rev. Geophys. 19 , 450–468 (1981).
Whitaker, J. S. & Kar, S. K. Implicit–explicit Runge–Kutta methods for fast–slow wave problems. Mon. Weather Rev. 141 , 3426–3434 (2013).
Gilleland, E., Ahijevych, D., Brown, B. G., Casati, B. & Ebert, E. E. Intercomparison of spatial forecast verification methods. Weather Forecast. 24 , 1416–1430 (2009).
Rasp, S. & Lerch, S. Neural networks for postprocessing ensemble weather forecasts. Month. Weather Rev. 146 , 3885–3900 (2018).
Pacchiardi, L., Adewoyin, R., Dueben, P. & Dutta, R. Probabilistic forecasting with generative networks via scoring rule minimization. J. Mach. Learn. Res. 25 , 1–64 (2024).
Smith, J. A., Kochkov, D., Norgaard, P., Yuval, J. & Hoyer, S. google-research/dinosaur: 1.0.0. Zenodo https://doi.org/10.5281/zenodo.11376145 (2024).
Kochkov, D. et al. google-research/neuralgcm: 1.0.0. Zenodo https://doi.org/10.5281/zenodo.11376143 (2024).
Rasp, S. et al. google-research/weatherbench2: v0.2.0. Zenodo https://doi.org/10.5281/zenodo.11376271 (2023).
Download references
Acknowledgements
We thank A. Kwa, A. Merose and K. Shah for assistance with data acquisition and handling; L. Zepeda-Núñez for feedback on the paper; and J. Anderson, C. Van Arsdale, R. Chemke, G. Dresdner, J. Gilmer, J. Hickey, N. Lutsko, G. Nearing, A. Paszke, J. Platt, S. Ponda, M. Pritchard, D. Rothenberg, F. Sha, T. Schneider and O. Voicu for discussions.
Author information
These authors contributed equally: Dmitrii Kochkov, Janni Yuval, Ian Langmore, Peter Norgaard, Jamie Smith, Stephan Hoyer
Authors and Affiliations
Google Research, Mountain View, CA, USA
Dmitrii Kochkov, Janni Yuval, Ian Langmore, Peter Norgaard, Jamie Smith, Griffin Mooers, James Lottes, Stephan Rasp, Michael P. Brenner & Stephan Hoyer
Earth, Atmospheric and Planetary Sciences, Massachusetts Institute of Technology, Cambridge, MA, USA
Milan Klöwer
European Centre for Medium-Range Weather Forecasts, Reading, UK
Peter Düben & Sam Hatfield
Google DeepMind, London, UK
Peter Battaglia, Alvaro Sanchez-Gonzalez & Matthew Willson
School of Engineering and Applied Sciences, Harvard University, Cambridge, MA, USA
Michael P. Brenner
You can also search for this author in PubMed Google Scholar
Contributions
D.K., J.Y., I.L., P.N., J.S. and S. Hoyer contributed equally to this work. D.K., J.Y., I.L., P.N., J.S., G.M., J.L. and S. Hoyer wrote the code. D.K., J.Y., I.L., P.N., G.M. and S. Hoyer trained models and analysed the data. M.P.B. and S. Hoyer managed and oversaw the research project. M.K., S.R., P.D., S. Hatfield, P.B. and M.P.B. contributed technical advice and ideas. M.W. ran experiments with GraphCast for comparison with NeuralGCM. A.S.-G. assisted with data preparation. D.K., J.Y., I.L., P.N. and S. Hoyer wrote the paper. All authors gave feedback and contributed to editing the paper.
Corresponding authors
Correspondence to Dmitrii Kochkov , Janni Yuval or Stephan Hoyer .
Ethics declarations
Competing interests.
D.K., J.Y., I.L., P.N., J.S., J.L., S.R., P.B., A.S.-G., M.W., M.P.B. and S. Hoyer are employees of Google. S. Hoyer, D.K., I.L., J.Y., G.M., P.N., J.S. and M.B. have filed international patent application PCT/US2023/035420 in the name of Google LLC, currently pending, relating to neural general circulation models.
Peer review
Peer review information.
Nature thanks Karthik Kashinath and the other, anonymous, reviewer(s) for their contribution to the peer review of this work. Peer reviewer reports are available.
Additional information
Publisher’s note Springer Nature remains neutral with regard to jurisdictional claims in published maps and institutional affiliations.
Extended data figures and tables
Extended data fig. 1 maps of bias for neuralgcm-ens and ecmwf-ens forecasts..
Bias is averaged over all forecasts initialized in 2020.
Extended Data Fig. 2 Maps of spread-skill ratio for NeuralGCM-ENS and ECMWF-ENS forecasts.
Spread-skill ratio is averaged over all forecasts initialized in 2020.
Extended Data Fig. 3 Geostrophic balance in NeuralGCM, GraphCast 3 and ECMWF-HRES.
Vertical profiles of the extratropical intensity (averaged between latitude 30°–70° in both hemispheres) and over all forecasts initialized in 2020 of (a,d,g) geostrophic wind, (b,e,h) ageostrophic wind and (c,f,i) the ratio of the intensity of ageostrophic wind over geostrophic wind for ERA5 (black continuous line in all panels), (a,b,c) NeuralGCM-0.7°, (d,e,f) GraphCast and (g,h,i) ECMWF-HRES at lead times of 1 day, 5 days and 10 days.
Extended Data Fig. 4 Precipitation minus evaporation calculated from the third day of weather forecasts.
(a) Tropical (latitudes −20° to 20°) precipitation minus evaporation (P minus E) rate distribution, (b) Extratropical (latitudes 30° to 70° in both hemispheres) P minus E, (c) mean P minus E for 2020 ERA5 14 and (d) NeuralGCM-0.7° (calculated from the third day of forecasts and averaged over all forecasts initialized in 2020), (e) the bias between NeuralGCM-0.7° and ERA5, (f-g) Snapshot of daily precipitation minus evaporation for 2020-01-04 for (f) NeuralGCM-0.7° (forecast initialized on 2020-01-02) and (g) ERA5.
Extended Data Fig. 5 Indirect comparison between precipitation bias in X-SHiELD and precipitation minus evaporation bias in NeuralGCM-1.4°.
Mean precipitation calculated between 2020-01-19 and 2021-01-17 for (a) ERA5 14 (c) X-SHiELD 31 and the biases in (e) X-SHiELD and (g) climatology (ERA5 data averaged over 1990-2019). Mean precipitation minus evaporation calculated between 2020-01-19 and 2021-01-17 for (b) ERA5 (d) NeuralGCM-1.4° (initialized in October 18th 2019) and the biases in (f) NeuralGCM-1.4° and (h) climatology (data averaged over 1990–2019).
Extended Data Fig. 6 Yearly temperature bias for NeuralGCM and X-SHiELD 31 .
Mean temperature between 2020-01-19 to 2020-01-17 for (a) ERA5 at 200hPa and (b) 850hPa. (c,d) the bias in the temperature for NeuralGCM-1.4°, (e,f) the bias in X-SHiELD and (g,h) the bias in climatology (calculated from 1990–2019). NeuralGCM-1.4° was initialized in 18th of October (similar to X-SHiELD).
Extended Data Fig. 7 Tropical Cyclone densities and annual regional counts.
(a) Tropical Cyclone (TC) density from ERA5 14 data spanning 1987–2020. (b) TC density from NeuralGCM-1.4° for 2020, generated using 34 different initial conditions all initialized in 2019. (c) Box plot depicting the annual number of TCs across different regions, based on ERA5 data (1987–2020), NeuralGCM-1.4° for 2020 (34 initial conditions), and orange markers show ERA5 for 2020. In the box plots, the red line represents the median; the box delineates the first to third quartiles; the whiskers extend to 1.5 times the interquartile range (Q1 − 1.5IQR and Q3 + 1.5IQR), and outliers are shown as individual dots. Each year is defined from January 19th to January 17th of the following year, aligning with data availability from X-SHiELD. For NeuralGCM simulations, the 3 initial conditions starting in January 2019 exclude data for January 17th, 2021, as these runs spanned only two years.
Extended Data Fig. 8 Tropical Cyclone maximum wind distribution in NeuralGCM vs. ERA5 14 .
Number of Tropical Cyclones (TCs) as a function of maximum wind speed at 850hPa across different regions, based on ERA5 data (1987–2020; in orange), and NeuralGCM-1.4° for 2020 (34 initial conditions; in blue). Each year is defined from January 19th to January 17th of the following year, aligning with data availability from X-SHiELD. For NeuralGCM simulations, the 3 initial conditions starting in January 2019 exclude data for January 17th, 2021, as these runs spanned only two years.
Supplementary information
Supplementary information.
Supplementary Information (38 figures, 6 tables): (A) Lines of code in atmospheric models; (B) Dynamical core of NeuralGCM; (C) Learned physics of NeuralGCM; (D) Encoder and decoder of NeuralGCM; (E) Time integration; (F) Evaluation metrics; (G) Training; (H) Additional weather evaluations; (I) Additional climate evaluations.
Peer Review File
Rights and permissions.
Open Access This article is licensed under a Creative Commons Attribution 4.0 International License, which permits use, sharing, adaptation, distribution and reproduction in any medium or format, as long as you give appropriate credit to the original author(s) and the source, provide a link to the Creative Commons licence, and indicate if changes were made. The images or other third party material in this article are included in the article’s Creative Commons licence, unless indicated otherwise in a credit line to the material. If material is not included in the article’s Creative Commons licence and your intended use is not permitted by statutory regulation or exceeds the permitted use, you will need to obtain permission directly from the copyright holder. To view a copy of this licence, visit http://creativecommons.org/licenses/by/4.0/ .
Reprints and permissions
About this article
Cite this article.
Kochkov, D., Yuval, J., Langmore, I. et al. Neural general circulation models for weather and climate. Nature 632 , 1060–1066 (2024). https://doi.org/10.1038/s41586-024-07744-y
Download citation
Received : 13 November 2023
Accepted : 15 June 2024
Published : 22 July 2024
Issue Date : 29 August 2024
DOI : https://doi.org/10.1038/s41586-024-07744-y
Share this article
Anyone you share the following link with will be able to read this content:
Sorry, a shareable link is not currently available for this article.
Provided by the Springer Nature SharedIt content-sharing initiative
This article is cited by
Google ai predicts long-term climate trends and weather — in minutes.
- Helena Kudiabor
Nature (2024)
Weather and climate predicted accurately — without using a supercomputer
- Oliver Watt-Meyer
By submitting a comment you agree to abide by our Terms and Community Guidelines . If you find something abusive or that does not comply with our terms or guidelines please flag it as inappropriate.
Quick links
- Explore articles by subject
- Guide to authors
- Editorial policies
Sign up for the Nature Briefing: AI and Robotics newsletter — what matters in AI and robotics research, free to your inbox weekly.

An Effective Smart Water Quality Monitoring and Management System Using IoT and Machine Learning
- Original Research
- Published: 31 August 2024
- Volume 5 , article number 846 , ( 2024 )
Cite this article
- Shanvendra Rai 1 ,
- Dhanasree S. Poduval 1 ,
- Utkarsh Anand 1 ,
- Vishnu Verma 1 &
- Subhasish Banerjee ORCID: orcid.org/0000-0003-1920-1913 1
Water is a fundamental and essential requirement for human existence, as nearly 70% of our body is constituted with water. Consumption of deteriorated water quality can lead to the cause of various life-threatening diseases such as Cholera, typhoid, etc. Annually, an estimated 3.4 million individuals die from drinking polluted water. Despite numerous technological advancements, traditional methods continue to be employed for monitoring water quality. These methods are very inefficient as they are quite time-consuming, expensive, and cannot provide real-time information for monitoring water quality. Therefore, this article suggested a model designed on the Internet of Things (IoT) that addresses the existing underlying water quality issues and could replace the conventional way of water monitoring systems. To check the water quality parameters, several sensors (SNs) have been used to collect the real-time data and transfer further for analysis purposes via a range of machine learning techniques, including XGBoost, random forest, AdaBoost, and decision tree. These methods exhibit robust performance in terms of accuracy, precision, recall, and f1 score. Through the combination of the IoT and ML, the proposed real-time water quality monitoring (WQM) system offers continuous monitoring, analysis, and prediction of water quality parameters. The integration of these technologies and outcomes of experimental works prove that the proposed model can help to safeguard the availability of potable and clean water resources for present and future generations.
This is a preview of subscription content, log in via an institution to check access.
Access this article
Subscribe and save.
- Get 10 units per month
- Download Article/Chapter or eBook
- 1 Unit = 1 Article or 1 Chapter
- Cancel anytime
Price includes VAT (Russian Federation)
Instant access to the full article PDF.
Rent this article via DeepDyve
Institutional subscriptions
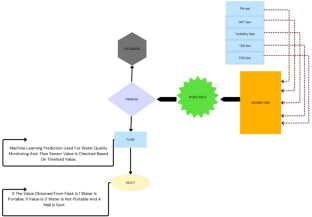
Explore related subjects
- Artificial Intelligence
Data Availability
The data and material that support the findings of this study are available from the corresponding author, Subhasish Banerjee, upon reasonable request.
Benedict S. Serverless blockchain-enabled architecture for iot societal applications. IEEE Trans Comput Social Syst. 2020;7(5):1146–58.
Article Google Scholar
Curry E, Hasan S, Kouroupetroglou C, Fabritius W, ul Hassan U, Derguech W. Internet of things enhanced user experience for smart water and energy management. IEEE Internet Comput. 2018;22(1):18–28.
Deep B, Mathur I, Joshi N. Coalescing IoT and Wi-Fi technologies for an optimized approach in urban route planning. Environ Sci Pollut Res. 2020;27:34434–41.
Demissie H, Lu S, Jiao R, Liu L, Xiang Y, Ritigala T, Wang D. Advances in micro interfacial phenomena of adsorptive micellar flocculation: principles and application for water treatment. Water Res. 2021;202:117414.
Li M, He P, Zhao L. Dynamic load balancing applying water-filling approach in smart grid systems. IEEE Internet Things J. 2017;4(1):247–57.
Di Luccio D, Riccio A, Galletti A, Laccetti G, Lapegna M, Marcellino L, Montella R. Coastal Marine data crowdsourcing using the internet of floating things: improving the results of a water quality model. IEEE Access. 2020;8:101209–23.
Wang D, Xiang H. Composite control of post-chlorine dosage during drinking water treatment. IEEE Access. 2019;7:27893–8.
Ajith JB, Manimegalai R, Ilayaraja V. (2020, February). An IoT based smart water quality monitoring system using cloud. In 2020 International conference on emerging trends in information technology and engineering (ic-ETITE) (pp. 1–7). IEEE.
Anuradha T, Bhakti CR, Pooja D. IoT based low cost system for monitoring of water quality in real time. Int Res J Eng Technol (IRJET). 2018;5(5):60–72.
Google Scholar
Sengupta B, Sawant S, Dhanawade M, Bhosale S. Water quality monitoring using IoT. Int Res J Eng Technol. 2019;6(6):695–701.
Geetha S, Gouthami SJSW. Internet of things enabled real time water quality monitoring system. Smart Water. 2016;2(1):1–19.
Hamid SA, Rahim AMA, Fadhlullah SY, Abdullah S, Muhammad Z, Leh NAM. (2020, August). IoT based water quality monitoring system and evaluation. In 2020 10th IEEE International Conference on Control System, Computing and Engineering (ICCSCE) (pp. 102–106). IEEE.
Kumar MJV, Samalla K. Design and Development of water quality monitoring system in IoT. Int J Recent Technol Eng (IJRTE). 2019;7(5S3):2277–3878.
Mukta M, Islam S, Barman SD, Reza AW, Khan MSH. (2019, February). IoT based smart water quality monitoring system. In 2019 IEEE 4th International Conference on Computer and Communication Systems (ICCCS) (pp. 669–673). IEEE.
Pasika S, Gandla ST. Smart water quality monitoring system with cost-effective using IoT. Heliyon. 2020;6(7):e04096.
Konde S, Deosarkar S. IoT based water quality monitoring system, in Proceedings (ICCIP), 2(3), pp 202, 2020.
Sugapriyaa T, Rakshaya S, Ramyadevi K, Ramya M, Rashmi PG. Smart water quality monitoring system for real-time applications. Int J Pure Appl Math. 2018;118(7):1363–9.
Priya SK, Shenbagalakshmi G, Revathi T. (2018, April). IoT based automation of real time in-pipe contamination detection system in drinking water. In 2018 International conference on communication and signal processing (ICCSP) (pp. 1014–1018). IEEE.
Moparthi NR, Mukesh C, Sagar PV. (2018, February). Water quality monitoring system using IoT. In 2018 Fourth international conference on advances in electriclectronics, information, communication and bio-informatics (AEEICB) (pp. 1–5). IEEE.
Olatinwo SO, Joubert TH. Enabling communication networks for water quality monitoring applications: a survey. IEEE Access. 2019;7:100332–62.
Serra H, Bastos I, de Melo JL, Oliveira JP, Paulino N, Nefzaoui E, Bourouina T. A 0.9-V analog-to-digital acquisition channel for an IoT water management sensor node. IEEE Trans Circuits Syst II Express Briefs. 2019;66(10):1678–82.
AlMetwally SAH, Hassan MK, Mourad MH. Real time internet of things (IoT) based water quality management system. Procedia CIRP. 2020;91:478–85.
Huan J, Li H, Wu F, Cao W. Design of water quality monitoring system for aquaculture ponds based on NB-IoT. Aquacult Eng. 2020;90:102088.
Di Luccio, D., Riccio, A., Galletti, A., Laccetti, G., Lapegna, M., Marcellino, L., ... & Montella, R. (2020). Coastal marine data crowdsourcing using the Internet of Floating Things: Improving the results of a water quality model. IEEE Access, 8, 101209-101223.
Lakshmikantha V, Hiriyannagowda A, Manjunath A, Patted A, Basavaiah J, Anthony AA. IoT based smart water quality monitoring system. Global Transitions Proc. 2021;2(2):181–6.
Roy SK, Misra S, Raghuwanshi NS, Das SK. AgriSens: IoT-based dynamic irrigation scheduling system for water management of irrigated crops. IEEE Internet Things J. 2020;8(6):5023–30.
Bhardwaj A, Dagar V, Khan MO, Aggarwal A, Alvarado R, Kumar M, Proshad R. Smart IoT and machine learning-based framework for water quality assessment and device component monitoring. Environ Sci Pollut Res. 2022;29(30):46018–36.
Adeleke IA, Nwulu NI, Ogbolumani OA. A hybrid machine learning and embedded IoT-based water quality monitoring system. Internet Things. 2023;22:100774.
Jéquier E, Constant F. Water as an essential nutrient: the physiological basis of hydration. Eur J Clin Nutr. 2010;64(2):115–23.
Source/Link. https://www.kaggle.com/datasets/gulabsah23/water-quality-dataset
Download references
This research did not receive any specific funding and it is carried out as part of the employment and higher degree of the authors.
Author information
Authors and affiliations.
Department of Computer Science & Engineering, National Institute of Technology Arunachal Pradesh, Itanagar, Papumpare, Jote, Arunachal Pradesh, 791113, India
Shanvendra Rai, Dhanasree S. Poduval, Utkarsh Anand, Vishnu Verma & Subhasish Banerjee
You can also search for this author in PubMed Google Scholar
Corresponding author
Correspondence to Subhasish Banerjee .
Ethics declarations
Conflict of interest.
The authors declare that they have no conflict of interest.
Ethical Approval
This article does not contain any studies with human participants or animals performed by any of the authors.
Additional information
Publisher’s note.
Springer Nature remains neutral with regard to jurisdictional claims in published maps and institutional affiliations.
Electronic Supplementary Material
Below is the link to the electronic supplementary material.
Supplementary Material 1
Rights and permissions.
Springer Nature or its licensor (e.g. a society or other partner) holds exclusive rights to this article under a publishing agreement with the author(s) or other rightsholder(s); author self-archiving of the accepted manuscript version of this article is solely governed by the terms of such publishing agreement and applicable law.
Reprints and permissions
About this article
Rai, S., Poduval, D.S., Anand, U. et al. An Effective Smart Water Quality Monitoring and Management System Using IoT and Machine Learning. SN COMPUT. SCI. 5 , 846 (2024). https://doi.org/10.1007/s42979-024-03208-2
Download citation
Received : 13 February 2024
Accepted : 05 August 2024
Published : 31 August 2024
DOI : https://doi.org/10.1007/s42979-024-03208-2
Share this article
Anyone you share the following link with will be able to read this content:
Sorry, a shareable link is not currently available for this article.
Provided by the Springer Nature SharedIt content-sharing initiative
- Microprocessor
- Turbidity sensor
- Machine learning
- Find a journal
- Publish with us
- Track your research
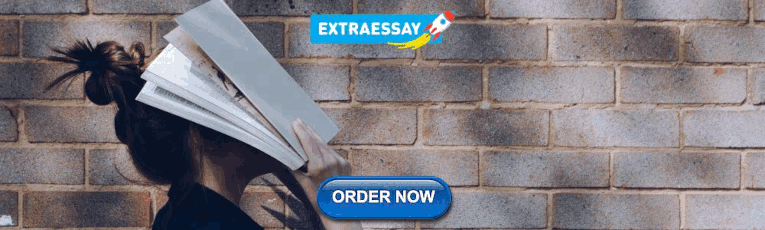
IMAGES
VIDEO
COMMENTS
This task can be especially challenging for special educators who must balance standards-based education with individualized instruction. This paper describes the value of clarifying learning targets, defines different types of targets, and provides strategies and resources to assist practitioners in unpacking standards to develop learning targets.
This paper describes the value of clarifying learning targets, defines different types of targets, and provides strategies and resources to assist practitioners in unpacking standards to develop learning targets. ... Dive into the research topics of 'Setting Clear Learning Targets to Guide Instruction for All Students'. Together they form a ...
"Success criteria make the learning target, or 'it,' visible for both teachers and students by . describing what learners must know and be able to do that would demonstrate that they . have met the learning intentions for the day" (Almarode et al, 2021, Ch. 1). To illustrate the . potential of success criteria, consider the research on
The sheer number of targets set in these seven schools over 4 years underscores the magnitude of the SLO system undertaking: In Table 4, we show that between about 8,000 and 9,000 individual student learning targets were set per test and subject from 2011-2012 through 2014-2015, for a total of approximately 34,000 target scores.
earning targets in your classroom and school. It's only through collab-orative and evidence-based decision making that you will advance a learning target theory of actio. r Self-Assessment Targets and Look-Fors GuideTarget 1: Each time I plan a lesson, I begin by defining the learning target that my students a.
Student Goal Setting. The act of goal setting is a desired competency area for students associated with the "learning-to-learn" skills students need to engage in deeper learning (William and Flora Hewlett Foundation, 2013). The act of goal setting, therefore, is a practice that educators can use to help fuel students' learning-to-learn ...
The science of learning has made a considerable contribution to our understanding of effective teaching and learning strategies. However, few instructors outside of the field are privy to this research. In this tutorial review, we focus on six specific cognitive strategies that have received robust support from decades of research: spaced practice, interleaving, retrieval practice, elaboration ...
Well-defined learning objectives outline the desired outcome for learners, which will help specify the instructional method. For example, if we want the learners to demonstrate correct intubation procedure in a normal adult 100% of the time, we need the instructional method to involve some sort of hands-on experience so that learners can ...
A shared learning target, on the other hand, frames the lesson from the students' point of view. A shared learning target helps students grasp the lesson's purpose—why it is crucial to learn this chunk of information, on this day, and in this way." —Brookhart, S. M., Long, B. A., & Moss, C. M. (2011, March). Know your learning target.
The paper engages with target setting, one of the government's key priorities, from the standpoint, not of teachers, policy makers, parents or academics, but rather from the perspective of the ...
With so much research in favor of learning targets, schools should support educators' efforts through curriculum staff or materials. Identify the Learning Objectives. Identifying the learning objective is typically the first step for creating an effective learning target. The objective or standard acts as the foundation upon which the learning ...
Crawford C. C. (1925a). The correlation between college lecture notes and quiz papers. Journal of Educational Research, 12, 282-291. Crossref. Google Scholar. Crawford C. C. (1925b). Some experimental studies of the results of college note-taking. ... Distributed practice and procedural memory consolidation in musicians' skill learning ...
Learning Targets, Student Look‐Fors, Performance of Understanding, Formative Learning Cycle 1. Learning Targets • If students are not using it (aiming for understanding of important concepts and becoming more proficient in targeted skills) they are not engaged in the
The effectiveness of research-based learning. Conducting one's own research project involves various cognitive, behavioural, and affective experiences (Lopatto, Citation 2009, 29), which in turn lead to a wide range of benefits associated with RBL. RBL is associated with long-term societal benefits because it can foster scientific careers: Students participating in RBL reported a greater ...
Bloom's taxonomy. Knowledge is the foundational cognitive skill and refers to the retention of specific, discrete pieces of information like facts and definitions or methodology, such as the sequence of events in a step-by-step process. Knowledge can be assessed by straightforward means, for example, multiple choice or short-answer questions ...
Introduction2 Purpose and Vision: Defining a Compelling Reason to Innovate 4 Learning Model: Clarifying How Learning and Teaching Will Change 10 Alignment: Ensuring Culture and Structures Support Mastery Learning 15 Sustainability: Building Capacity to Sustain Change Over Time 21 Concluding Comments 24 This paper is the third in a series of papers that highlight the work of the Mastery Transcript
Abstract. Learning objectives (LOs) are used to communicate the purpose of instruction. Done well, they convey the expectations that the instructor—and by extension, the academic field—has in terms of what students should know and be able to do after completing a course of study. As a result, they help students better understand course ...
Here is one example of co-construction: Providing students with work samples that illustrate success. Asking students to identify the parts of the work sample that make it successful (i.e., criteria for success) Writing out the criteria for success with students. Challenge 3.
Putting the standard on the board. Having the students break down the nouns and verbs. Writing the essential questions on the board. Writing the learning targets on the board. Stating the learning targets and standard. As leaders, we are doing the following: Conducting focus walks to see if they are posted.
Learning outcome examples adapted from, Nelson Baker at Georgia Tech: [email protected]. How Bloom's works with Quality Matters. For a course to meet the Quality Matters standards it must have learning outcomes that are measurable. Using a verb table like the one above will help you avoid verbs that cannot be quantified, like: understand, learn, appreciate, or enjoy.
Term papers, research reports, and lab reports are product targets when the curriculum guide, calls for students to create them. When products are assessed it yields evidence of the intended learning because the creation of the product is the stated learning.
3. Be better able to explain students' progress. Because you have your own clear understanding of your students' progress, you'll have a much easier time communicating it to parents and schools. The learning target already gives you great wording to describe students' successes and areas for improvement. 4.
This paper addresses the challenge of active tracking of space non-cooperative targets, a critical task in various aerospace applications. Traditional active tracking algorithms often require extensive data and suffer from limited generalization ability, making them inefficient for tracking targets with diverse characteristics. To overcome these limitations, we propose an end-to-end active ...
Paved the way for further research in reinforcement learning. Access: Read the Paper . 4. "Sequence to Sequence Learning with Neural Networks" by Ilya Sutskever, Oriol Vinyals, and Quoc V. Le . Summary: This paper introduced the sequence-to-sequence (seq2seq) learning framework, which has become fundamental for tasks such as machine ...
A hybrid model that combines a differentiable solver for atmospheric dynamics with machine-learning components is capable of weather forecasts and climate simulations on par with the best ...
Water is a fundamental and essential requirement for human existence, as nearly 70% of our body is constituted with water. Consumption of deteriorated water quality can lead to the cause of various life-threatening diseases such as Cholera, typhoid, etc. Annually, an estimated 3.4 million individuals die from drinking polluted water. Despite numerous technological advancements, traditional ...