
- SUGGESTED TOPICS
- The Magazine
- Newsletters
- Managing Yourself
- Managing Teams
- Work-life Balance
- The Big Idea
- Data & Visuals
- Reading Lists
- Case Selections
- HBR Learning
- Topic Feeds
- Account Settings
- Email Preferences
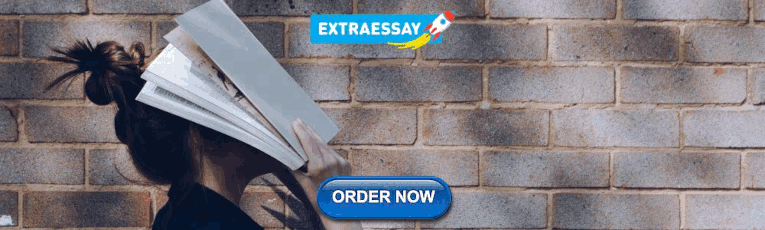
Present Your Data Like a Pro
- Joel Schwartzberg

Demystify the numbers. Your audience will thank you.
While a good presentation has data, data alone doesn’t guarantee a good presentation. It’s all about how that data is presented. The quickest way to confuse your audience is by sharing too many details at once. The only data points you should share are those that significantly support your point — and ideally, one point per chart. To avoid the debacle of sheepishly translating hard-to-see numbers and labels, rehearse your presentation with colleagues sitting as far away as the actual audience would. While you’ve been working with the same chart for weeks or months, your audience will be exposed to it for mere seconds. Give them the best chance of comprehending your data by using simple, clear, and complete language to identify X and Y axes, pie pieces, bars, and other diagrammatic elements. Try to avoid abbreviations that aren’t obvious, and don’t assume labeled components on one slide will be remembered on subsequent slides. Every valuable chart or pie graph has an “Aha!” zone — a number or range of data that reveals something crucial to your point. Make sure you visually highlight the “Aha!” zone, reinforcing the moment by explaining it to your audience.
With so many ways to spin and distort information these days, a presentation needs to do more than simply share great ideas — it needs to support those ideas with credible data. That’s true whether you’re an executive pitching new business clients, a vendor selling her services, or a CEO making a case for change.

- JS Joel Schwartzberg oversees executive communications for a major national nonprofit, is a professional presentation coach, and is the author of Get to the Point! Sharpen Your Message and Make Your Words Matter and The Language of Leadership: How to Engage and Inspire Your Team . You can find him on LinkedIn and X. TheJoelTruth
Partner Center
Home Blog Design Understanding Data Presentations (Guide + Examples)
Understanding Data Presentations (Guide + Examples)
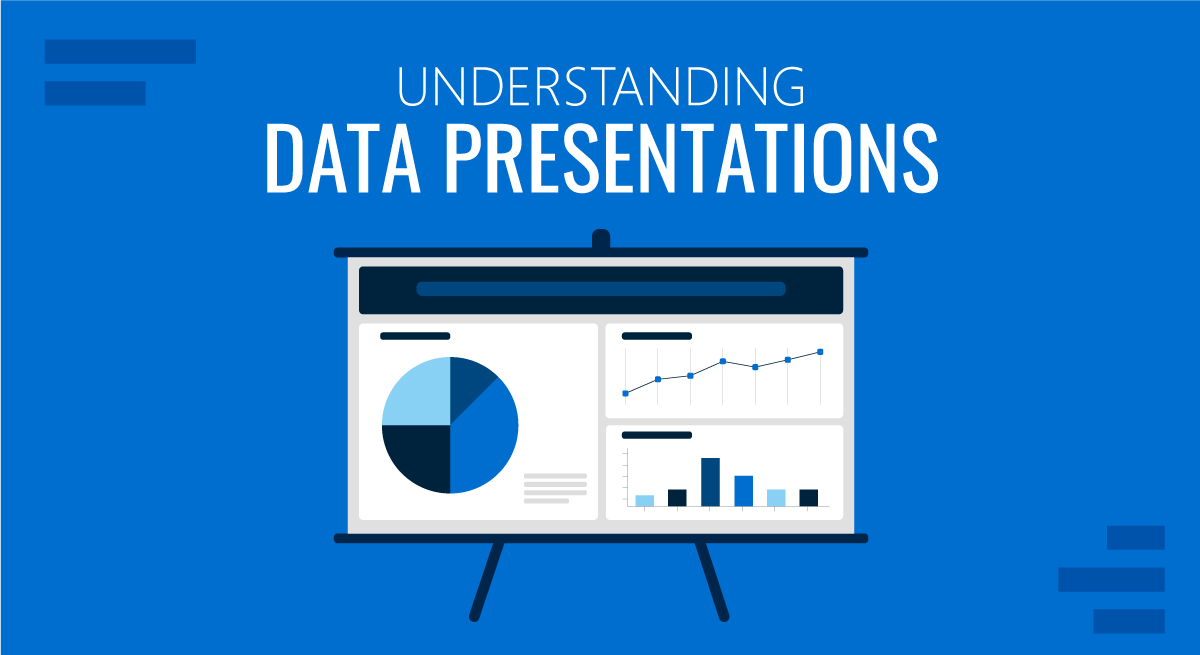
In this age of overwhelming information, the skill to effectively convey data has become extremely valuable. Initiating a discussion on data presentation types involves thoughtful consideration of the nature of your data and the message you aim to convey. Different types of visualizations serve distinct purposes. Whether you’re dealing with how to develop a report or simply trying to communicate complex information, how you present data influences how well your audience understands and engages with it. This extensive guide leads you through the different ways of data presentation.
Table of Contents
What is a Data Presentation?
What should a data presentation include, line graphs, treemap chart, scatter plot, how to choose a data presentation type, recommended data presentation templates, common mistakes done in data presentation.
A data presentation is a slide deck that aims to disclose quantitative information to an audience through the use of visual formats and narrative techniques derived from data analysis, making complex data understandable and actionable. This process requires a series of tools, such as charts, graphs, tables, infographics, dashboards, and so on, supported by concise textual explanations to improve understanding and boost retention rate.
Data presentations require us to cull data in a format that allows the presenter to highlight trends, patterns, and insights so that the audience can act upon the shared information. In a few words, the goal of data presentations is to enable viewers to grasp complicated concepts or trends quickly, facilitating informed decision-making or deeper analysis.
Data presentations go beyond the mere usage of graphical elements. Seasoned presenters encompass visuals with the art of data storytelling , so the speech skillfully connects the points through a narrative that resonates with the audience. Depending on the purpose – inspire, persuade, inform, support decision-making processes, etc. – is the data presentation format that is better suited to help us in this journey.
To nail your upcoming data presentation, ensure to count with the following elements:
- Clear Objectives: Understand the intent of your presentation before selecting the graphical layout and metaphors to make content easier to grasp.
- Engaging introduction: Use a powerful hook from the get-go. For instance, you can ask a big question or present a problem that your data will answer. Take a look at our guide on how to start a presentation for tips & insights.
- Structured Narrative: Your data presentation must tell a coherent story. This means a beginning where you present the context, a middle section in which you present the data, and an ending that uses a call-to-action. Check our guide on presentation structure for further information.
- Visual Elements: These are the charts, graphs, and other elements of visual communication we ought to use to present data. This article will cover one by one the different types of data representation methods we can use, and provide further guidance on choosing between them.
- Insights and Analysis: This is not just showcasing a graph and letting people get an idea about it. A proper data presentation includes the interpretation of that data, the reason why it’s included, and why it matters to your research.
- Conclusion & CTA: Ending your presentation with a call to action is necessary. Whether you intend to wow your audience into acquiring your services, inspire them to change the world, or whatever the purpose of your presentation, there must be a stage in which you convey all that you shared and show the path to staying in touch. Plan ahead whether you want to use a thank-you slide, a video presentation, or which method is apt and tailored to the kind of presentation you deliver.
- Q&A Session: After your speech is concluded, allocate 3-5 minutes for the audience to raise any questions about the information you disclosed. This is an extra chance to establish your authority on the topic. Check our guide on questions and answer sessions in presentations here.
Bar charts are a graphical representation of data using rectangular bars to show quantities or frequencies in an established category. They make it easy for readers to spot patterns or trends. Bar charts can be horizontal or vertical, although the vertical format is commonly known as a column chart. They display categorical, discrete, or continuous variables grouped in class intervals [1] . They include an axis and a set of labeled bars horizontally or vertically. These bars represent the frequencies of variable values or the values themselves. Numbers on the y-axis of a vertical bar chart or the x-axis of a horizontal bar chart are called the scale.
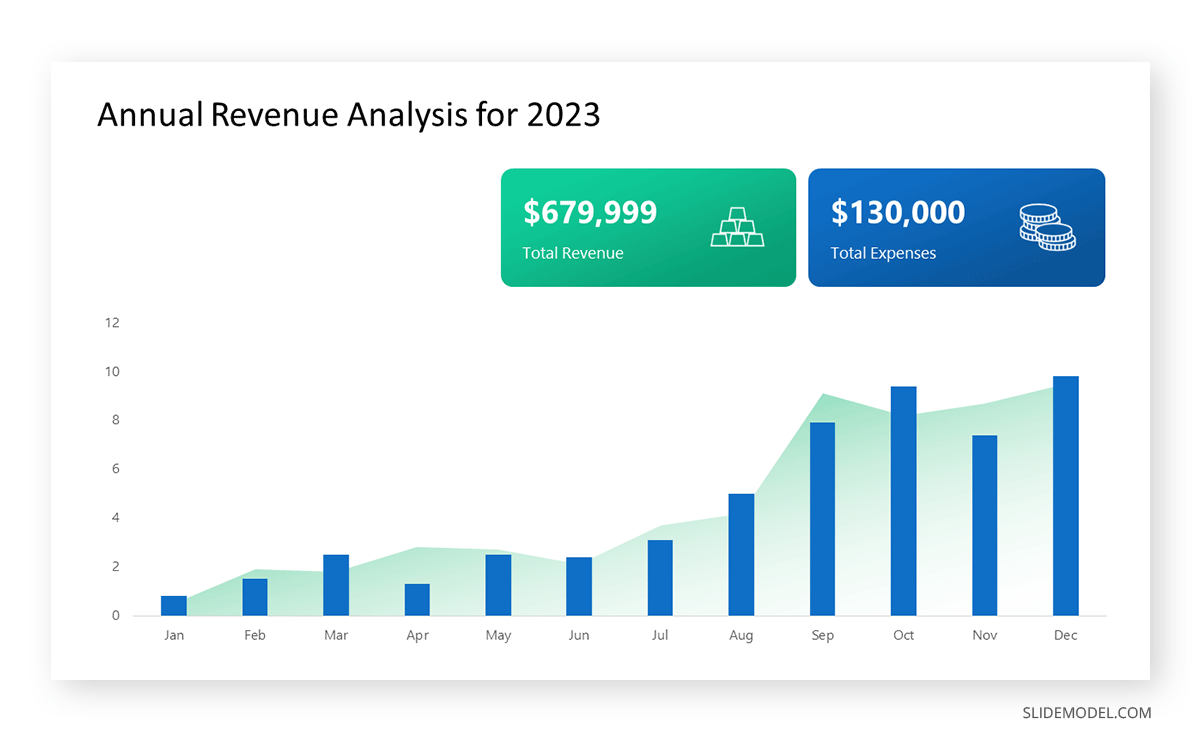
Real-Life Application of Bar Charts
Let’s say a sales manager is presenting sales to their audience. Using a bar chart, he follows these steps.
Step 1: Selecting Data
The first step is to identify the specific data you will present to your audience.
The sales manager has highlighted these products for the presentation.
- Product A: Men’s Shoes
- Product B: Women’s Apparel
- Product C: Electronics
- Product D: Home Decor
Step 2: Choosing Orientation
Opt for a vertical layout for simplicity. Vertical bar charts help compare different categories in case there are not too many categories [1] . They can also help show different trends. A vertical bar chart is used where each bar represents one of the four chosen products. After plotting the data, it is seen that the height of each bar directly represents the sales performance of the respective product.
It is visible that the tallest bar (Electronics – Product C) is showing the highest sales. However, the shorter bars (Women’s Apparel – Product B and Home Decor – Product D) need attention. It indicates areas that require further analysis or strategies for improvement.
Step 3: Colorful Insights
Different colors are used to differentiate each product. It is essential to show a color-coded chart where the audience can distinguish between products.
- Men’s Shoes (Product A): Yellow
- Women’s Apparel (Product B): Orange
- Electronics (Product C): Violet
- Home Decor (Product D): Blue
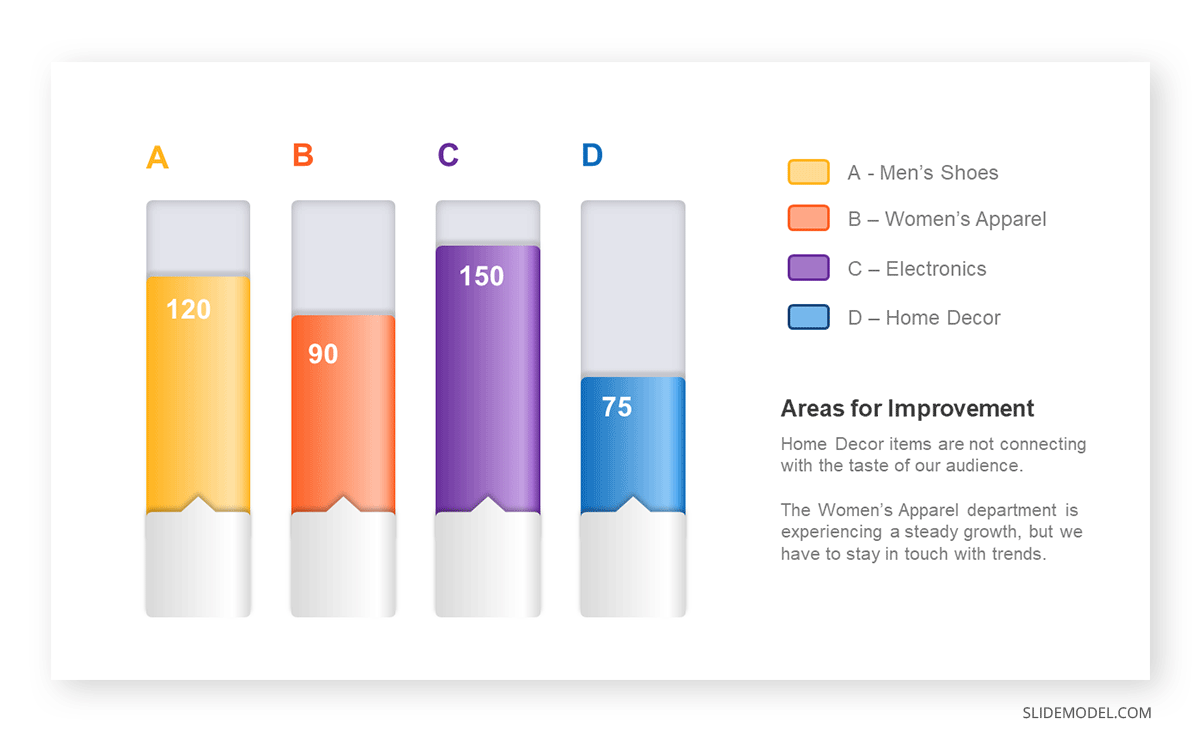
Bar charts are straightforward and easily understandable for presenting data. They are versatile when comparing products or any categorical data [2] . Bar charts adapt seamlessly to retail scenarios. Despite that, bar charts have a few shortcomings. They cannot illustrate data trends over time. Besides, overloading the chart with numerous products can lead to visual clutter, diminishing its effectiveness.
For more information, check our collection of bar chart templates for PowerPoint .
Line graphs help illustrate data trends, progressions, or fluctuations by connecting a series of data points called ‘markers’ with straight line segments. This provides a straightforward representation of how values change [5] . Their versatility makes them invaluable for scenarios requiring a visual understanding of continuous data. In addition, line graphs are also useful for comparing multiple datasets over the same timeline. Using multiple line graphs allows us to compare more than one data set. They simplify complex information so the audience can quickly grasp the ups and downs of values. From tracking stock prices to analyzing experimental results, you can use line graphs to show how data changes over a continuous timeline. They show trends with simplicity and clarity.
Real-life Application of Line Graphs
To understand line graphs thoroughly, we will use a real case. Imagine you’re a financial analyst presenting a tech company’s monthly sales for a licensed product over the past year. Investors want insights into sales behavior by month, how market trends may have influenced sales performance and reception to the new pricing strategy. To present data via a line graph, you will complete these steps.
First, you need to gather the data. In this case, your data will be the sales numbers. For example:
- January: $45,000
- February: $55,000
- March: $45,000
- April: $60,000
- May: $ 70,000
- June: $65,000
- July: $62,000
- August: $68,000
- September: $81,000
- October: $76,000
- November: $87,000
- December: $91,000
After choosing the data, the next step is to select the orientation. Like bar charts, you can use vertical or horizontal line graphs. However, we want to keep this simple, so we will keep the timeline (x-axis) horizontal while the sales numbers (y-axis) vertical.
Step 3: Connecting Trends
After adding the data to your preferred software, you will plot a line graph. In the graph, each month’s sales are represented by data points connected by a line.
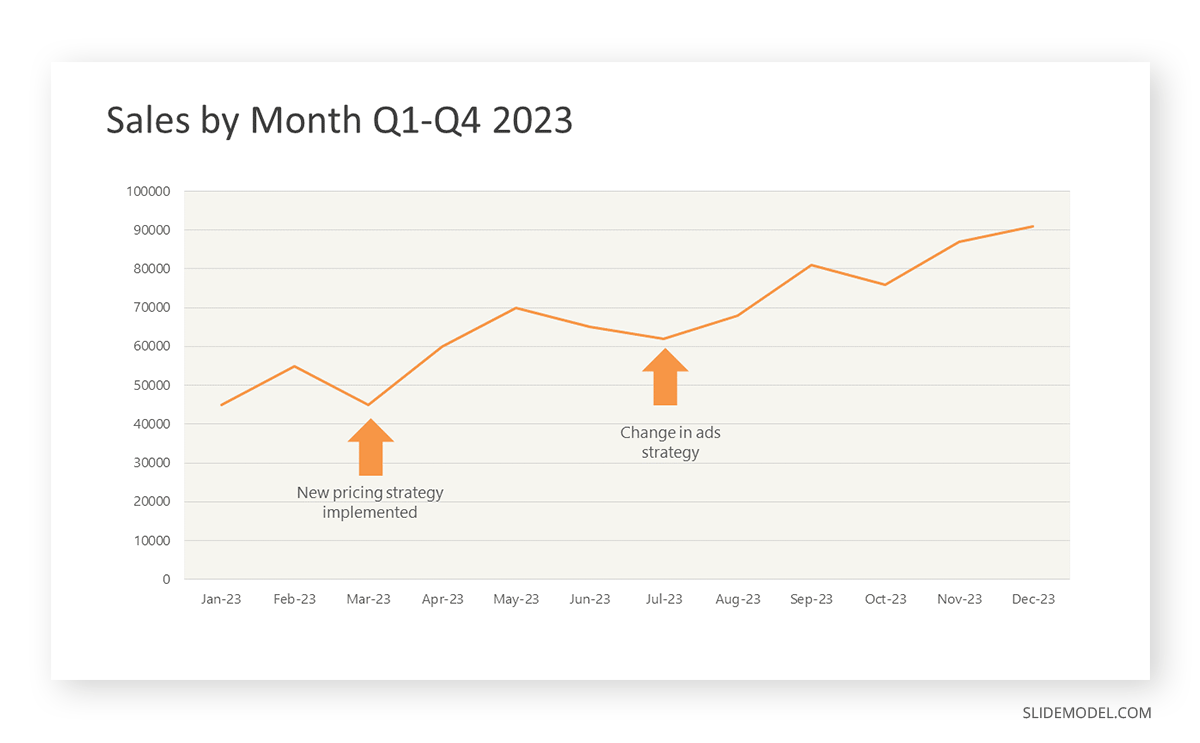
Step 4: Adding Clarity with Color
If there are multiple lines, you can also add colors to highlight each one, making it easier to follow.
Line graphs excel at visually presenting trends over time. These presentation aids identify patterns, like upward or downward trends. However, too many data points can clutter the graph, making it harder to interpret. Line graphs work best with continuous data but are not suitable for categories.
For more information, check our collection of line chart templates for PowerPoint and our article about how to make a presentation graph .
A data dashboard is a visual tool for analyzing information. Different graphs, charts, and tables are consolidated in a layout to showcase the information required to achieve one or more objectives. Dashboards help quickly see Key Performance Indicators (KPIs). You don’t make new visuals in the dashboard; instead, you use it to display visuals you’ve already made in worksheets [3] .
Keeping the number of visuals on a dashboard to three or four is recommended. Adding too many can make it hard to see the main points [4]. Dashboards can be used for business analytics to analyze sales, revenue, and marketing metrics at a time. They are also used in the manufacturing industry, as they allow users to grasp the entire production scenario at the moment while tracking the core KPIs for each line.
Real-Life Application of a Dashboard
Consider a project manager presenting a software development project’s progress to a tech company’s leadership team. He follows the following steps.
Step 1: Defining Key Metrics
To effectively communicate the project’s status, identify key metrics such as completion status, budget, and bug resolution rates. Then, choose measurable metrics aligned with project objectives.
Step 2: Choosing Visualization Widgets
After finalizing the data, presentation aids that align with each metric are selected. For this project, the project manager chooses a progress bar for the completion status and uses bar charts for budget allocation. Likewise, he implements line charts for bug resolution rates.
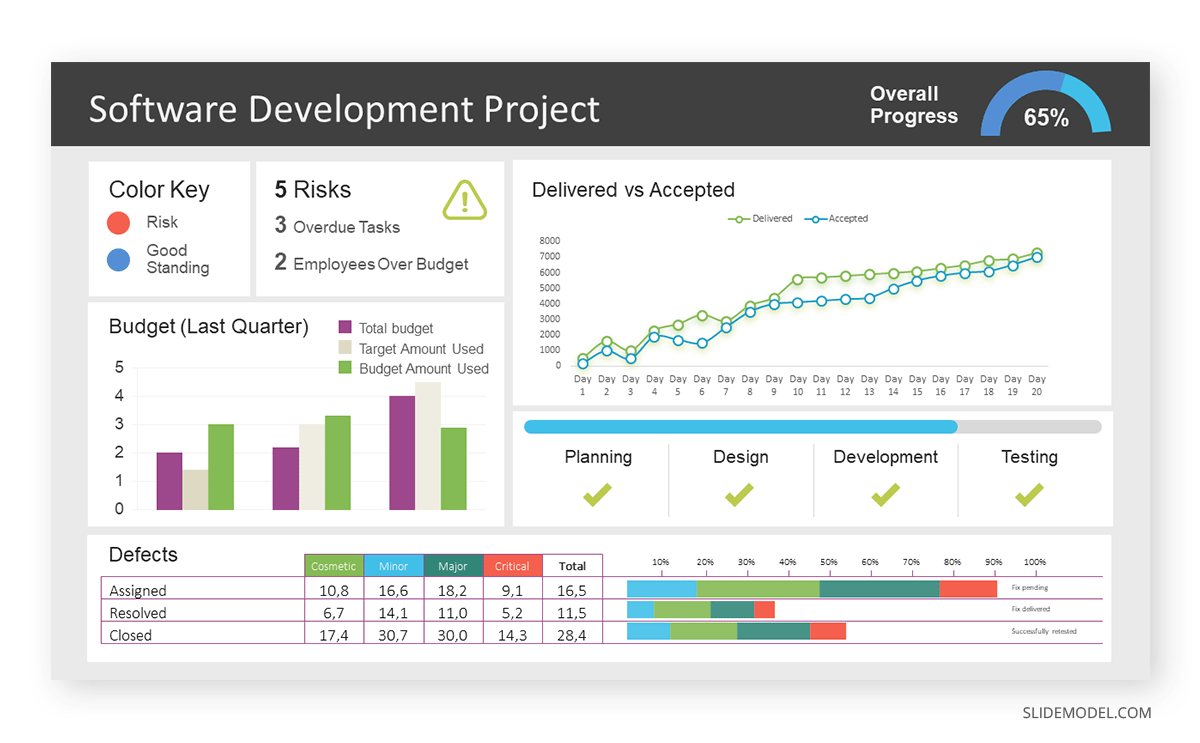
Step 3: Dashboard Layout
Key metrics are prominently placed in the dashboard for easy visibility, and the manager ensures that it appears clean and organized.
Dashboards provide a comprehensive view of key project metrics. Users can interact with data, customize views, and drill down for detailed analysis. However, creating an effective dashboard requires careful planning to avoid clutter. Besides, dashboards rely on the availability and accuracy of underlying data sources.
For more information, check our article on how to design a dashboard presentation , and discover our collection of dashboard PowerPoint templates .
Treemap charts represent hierarchical data structured in a series of nested rectangles [6] . As each branch of the ‘tree’ is given a rectangle, smaller tiles can be seen representing sub-branches, meaning elements on a lower hierarchical level than the parent rectangle. Each one of those rectangular nodes is built by representing an area proportional to the specified data dimension.
Treemaps are useful for visualizing large datasets in compact space. It is easy to identify patterns, such as which categories are dominant. Common applications of the treemap chart are seen in the IT industry, such as resource allocation, disk space management, website analytics, etc. Also, they can be used in multiple industries like healthcare data analysis, market share across different product categories, or even in finance to visualize portfolios.
Real-Life Application of a Treemap Chart
Let’s consider a financial scenario where a financial team wants to represent the budget allocation of a company. There is a hierarchy in the process, so it is helpful to use a treemap chart. In the chart, the top-level rectangle could represent the total budget, and it would be subdivided into smaller rectangles, each denoting a specific department. Further subdivisions within these smaller rectangles might represent individual projects or cost categories.
Step 1: Define Your Data Hierarchy
While presenting data on the budget allocation, start by outlining the hierarchical structure. The sequence will be like the overall budget at the top, followed by departments, projects within each department, and finally, individual cost categories for each project.
- Top-level rectangle: Total Budget
- Second-level rectangles: Departments (Engineering, Marketing, Sales)
- Third-level rectangles: Projects within each department
- Fourth-level rectangles: Cost categories for each project (Personnel, Marketing Expenses, Equipment)
Step 2: Choose a Suitable Tool
It’s time to select a data visualization tool supporting Treemaps. Popular choices include Tableau, Microsoft Power BI, PowerPoint, or even coding with libraries like D3.js. It is vital to ensure that the chosen tool provides customization options for colors, labels, and hierarchical structures.
Here, the team uses PowerPoint for this guide because of its user-friendly interface and robust Treemap capabilities.
Step 3: Make a Treemap Chart with PowerPoint
After opening the PowerPoint presentation, they chose “SmartArt” to form the chart. The SmartArt Graphic window has a “Hierarchy” category on the left. Here, you will see multiple options. You can choose any layout that resembles a Treemap. The “Table Hierarchy” or “Organization Chart” options can be adapted. The team selects the Table Hierarchy as it looks close to a Treemap.
Step 5: Input Your Data
After that, a new window will open with a basic structure. They add the data one by one by clicking on the text boxes. They start with the top-level rectangle, representing the total budget.
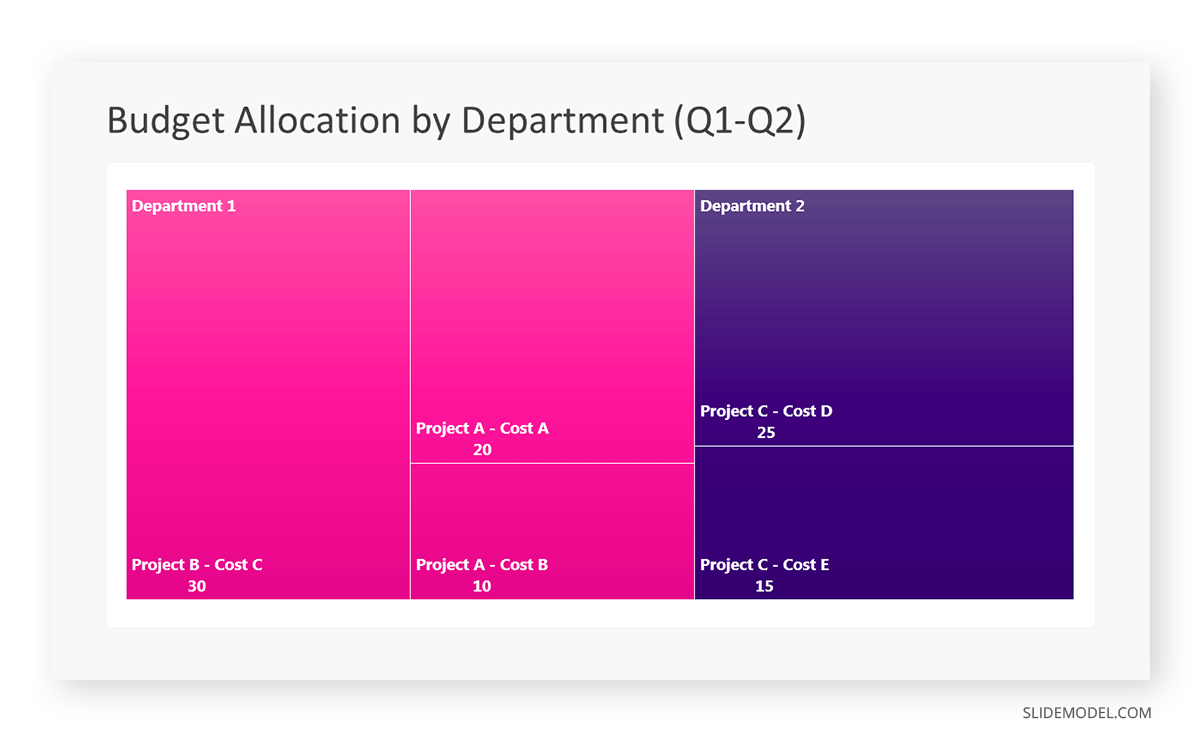
Step 6: Customize the Treemap
By clicking on each shape, they customize its color, size, and label. At the same time, they can adjust the font size, style, and color of labels by using the options in the “Format” tab in PowerPoint. Using different colors for each level enhances the visual difference.
Treemaps excel at illustrating hierarchical structures. These charts make it easy to understand relationships and dependencies. They efficiently use space, compactly displaying a large amount of data, reducing the need for excessive scrolling or navigation. Additionally, using colors enhances the understanding of data by representing different variables or categories.
In some cases, treemaps might become complex, especially with deep hierarchies. It becomes challenging for some users to interpret the chart. At the same time, displaying detailed information within each rectangle might be constrained by space. It potentially limits the amount of data that can be shown clearly. Without proper labeling and color coding, there’s a risk of misinterpretation.
A heatmap is a data visualization tool that uses color coding to represent values across a two-dimensional surface. In these, colors replace numbers to indicate the magnitude of each cell. This color-shaded matrix display is valuable for summarizing and understanding data sets with a glance [7] . The intensity of the color corresponds to the value it represents, making it easy to identify patterns, trends, and variations in the data.
As a tool, heatmaps help businesses analyze website interactions, revealing user behavior patterns and preferences to enhance overall user experience. In addition, companies use heatmaps to assess content engagement, identifying popular sections and areas of improvement for more effective communication. They excel at highlighting patterns and trends in large datasets, making it easy to identify areas of interest.
We can implement heatmaps to express multiple data types, such as numerical values, percentages, or even categorical data. Heatmaps help us easily spot areas with lots of activity, making them helpful in figuring out clusters [8] . When making these maps, it is important to pick colors carefully. The colors need to show the differences between groups or levels of something. And it is good to use colors that people with colorblindness can easily see.
Check our detailed guide on how to create a heatmap here. Also discover our collection of heatmap PowerPoint templates .
Pie charts are circular statistical graphics divided into slices to illustrate numerical proportions. Each slice represents a proportionate part of the whole, making it easy to visualize the contribution of each component to the total.
The size of the pie charts is influenced by the value of data points within each pie. The total of all data points in a pie determines its size. The pie with the highest data points appears as the largest, whereas the others are proportionally smaller. However, you can present all pies of the same size if proportional representation is not required [9] . Sometimes, pie charts are difficult to read, or additional information is required. A variation of this tool can be used instead, known as the donut chart , which has the same structure but a blank center, creating a ring shape. Presenters can add extra information, and the ring shape helps to declutter the graph.
Pie charts are used in business to show percentage distribution, compare relative sizes of categories, or present straightforward data sets where visualizing ratios is essential.
Real-Life Application of Pie Charts
Consider a scenario where you want to represent the distribution of the data. Each slice of the pie chart would represent a different category, and the size of each slice would indicate the percentage of the total portion allocated to that category.
Step 1: Define Your Data Structure
Imagine you are presenting the distribution of a project budget among different expense categories.
- Column A: Expense Categories (Personnel, Equipment, Marketing, Miscellaneous)
- Column B: Budget Amounts ($40,000, $30,000, $20,000, $10,000) Column B represents the values of your categories in Column A.
Step 2: Insert a Pie Chart
Using any of the accessible tools, you can create a pie chart. The most convenient tools for forming a pie chart in a presentation are presentation tools such as PowerPoint or Google Slides. You will notice that the pie chart assigns each expense category a percentage of the total budget by dividing it by the total budget.
For instance:
- Personnel: $40,000 / ($40,000 + $30,000 + $20,000 + $10,000) = 40%
- Equipment: $30,000 / ($40,000 + $30,000 + $20,000 + $10,000) = 30%
- Marketing: $20,000 / ($40,000 + $30,000 + $20,000 + $10,000) = 20%
- Miscellaneous: $10,000 / ($40,000 + $30,000 + $20,000 + $10,000) = 10%
You can make a chart out of this or just pull out the pie chart from the data.
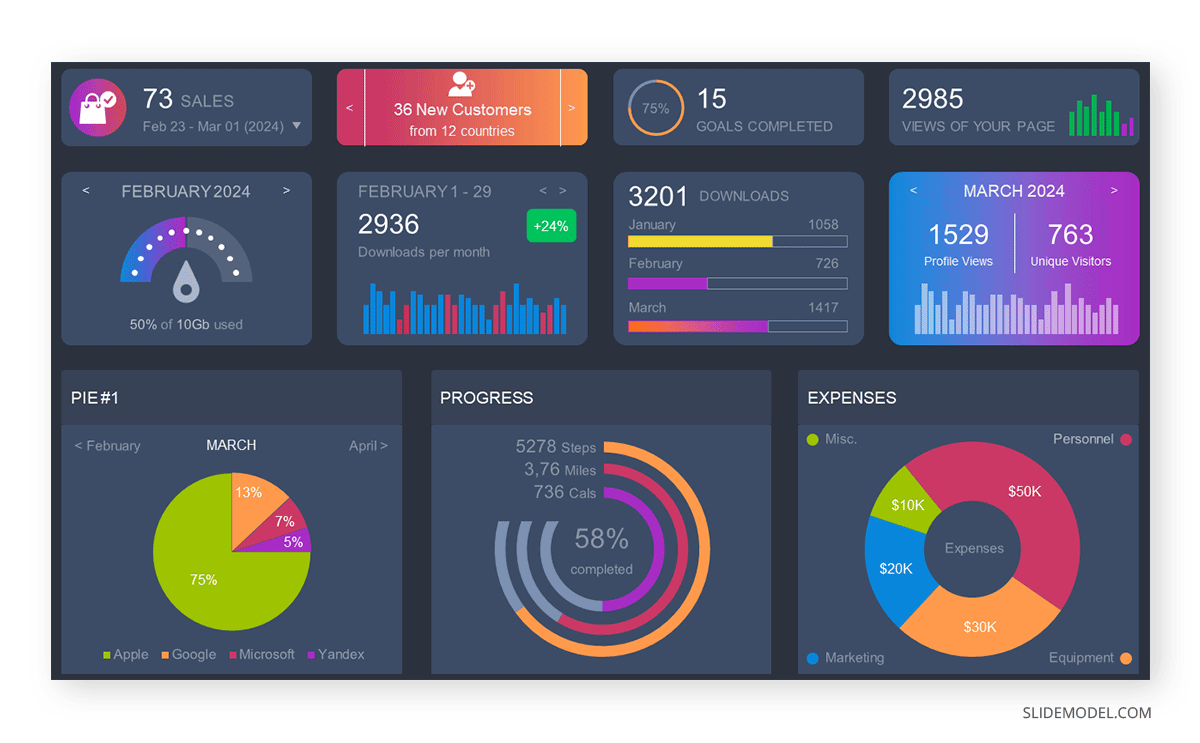
3D pie charts and 3D donut charts are quite popular among the audience. They stand out as visual elements in any presentation slide, so let’s take a look at how our pie chart example would look in 3D pie chart format.
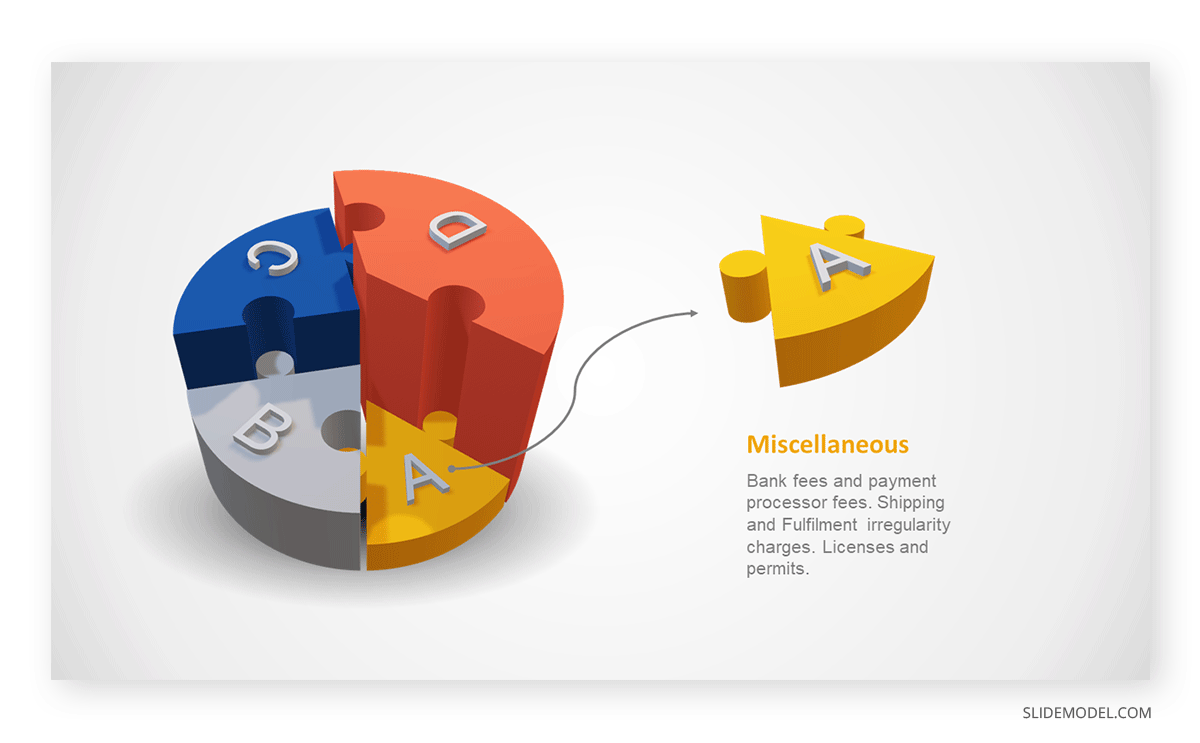
Step 03: Results Interpretation
The pie chart visually illustrates the distribution of the project budget among different expense categories. Personnel constitutes the largest portion at 40%, followed by equipment at 30%, marketing at 20%, and miscellaneous at 10%. This breakdown provides a clear overview of where the project funds are allocated, which helps in informed decision-making and resource management. It is evident that personnel are a significant investment, emphasizing their importance in the overall project budget.
Pie charts provide a straightforward way to represent proportions and percentages. They are easy to understand, even for individuals with limited data analysis experience. These charts work well for small datasets with a limited number of categories.
However, a pie chart can become cluttered and less effective in situations with many categories. Accurate interpretation may be challenging, especially when dealing with slight differences in slice sizes. In addition, these charts are static and do not effectively convey trends over time.
For more information, check our collection of pie chart templates for PowerPoint .
Histograms present the distribution of numerical variables. Unlike a bar chart that records each unique response separately, histograms organize numeric responses into bins and show the frequency of reactions within each bin [10] . The x-axis of a histogram shows the range of values for a numeric variable. At the same time, the y-axis indicates the relative frequencies (percentage of the total counts) for that range of values.
Whenever you want to understand the distribution of your data, check which values are more common, or identify outliers, histograms are your go-to. Think of them as a spotlight on the story your data is telling. A histogram can provide a quick and insightful overview if you’re curious about exam scores, sales figures, or any numerical data distribution.
Real-Life Application of a Histogram
In the histogram data analysis presentation example, imagine an instructor analyzing a class’s grades to identify the most common score range. A histogram could effectively display the distribution. It will show whether most students scored in the average range or if there are significant outliers.
Step 1: Gather Data
He begins by gathering the data. The scores of each student in class are gathered to analyze exam scores.
Names | Score |
---|---|
Alice | 78 |
Bob | 85 |
Clara | 92 |
David | 65 |
Emma | 72 |
Frank | 88 |
Grace | 76 |
Henry | 95 |
Isabel | 81 |
Jack | 70 |
Kate | 60 |
Liam | 89 |
Mia | 75 |
Noah | 84 |
Olivia | 92 |
After arranging the scores in ascending order, bin ranges are set.
Step 2: Define Bins
Bins are like categories that group similar values. Think of them as buckets that organize your data. The presenter decides how wide each bin should be based on the range of the values. For instance, the instructor sets the bin ranges based on score intervals: 60-69, 70-79, 80-89, and 90-100.
Step 3: Count Frequency
Now, he counts how many data points fall into each bin. This step is crucial because it tells you how often specific ranges of values occur. The result is the frequency distribution, showing the occurrences of each group.
Here, the instructor counts the number of students in each category.
- 60-69: 1 student (Kate)
- 70-79: 4 students (David, Emma, Grace, Jack)
- 80-89: 7 students (Alice, Bob, Frank, Isabel, Liam, Mia, Noah)
- 90-100: 3 students (Clara, Henry, Olivia)
Step 4: Create the Histogram
It’s time to turn the data into a visual representation. Draw a bar for each bin on a graph. The width of the bar should correspond to the range of the bin, and the height should correspond to the frequency. To make your histogram understandable, label the X and Y axes.
In this case, the X-axis should represent the bins (e.g., test score ranges), and the Y-axis represents the frequency.
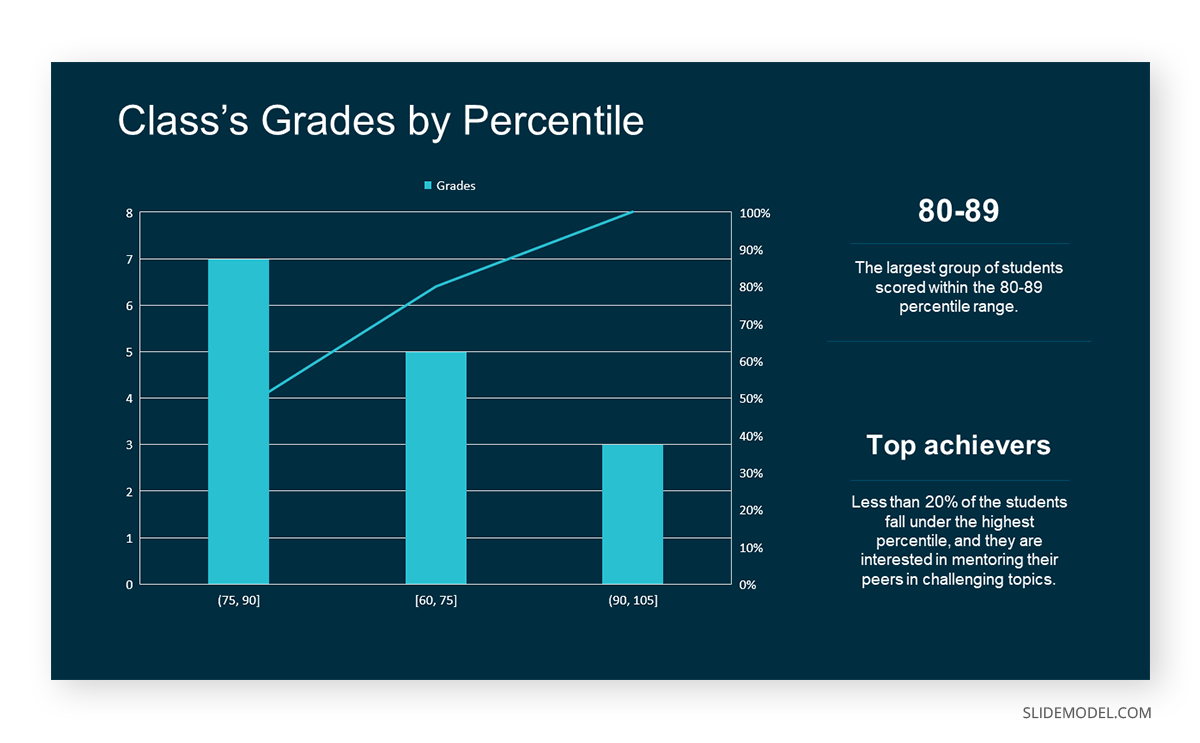
The histogram of the class grades reveals insightful patterns in the distribution. Most students, with seven students, fall within the 80-89 score range. The histogram provides a clear visualization of the class’s performance. It showcases a concentration of grades in the upper-middle range with few outliers at both ends. This analysis helps in understanding the overall academic standing of the class. It also identifies the areas for potential improvement or recognition.
Thus, histograms provide a clear visual representation of data distribution. They are easy to interpret, even for those without a statistical background. They apply to various types of data, including continuous and discrete variables. One weak point is that histograms do not capture detailed patterns in students’ data, with seven compared to other visualization methods.
A scatter plot is a graphical representation of the relationship between two variables. It consists of individual data points on a two-dimensional plane. This plane plots one variable on the x-axis and the other on the y-axis. Each point represents a unique observation. It visualizes patterns, trends, or correlations between the two variables.
Scatter plots are also effective in revealing the strength and direction of relationships. They identify outliers and assess the overall distribution of data points. The points’ dispersion and clustering reflect the relationship’s nature, whether it is positive, negative, or lacks a discernible pattern. In business, scatter plots assess relationships between variables such as marketing cost and sales revenue. They help present data correlations and decision-making.
Real-Life Application of Scatter Plot
A group of scientists is conducting a study on the relationship between daily hours of screen time and sleep quality. After reviewing the data, they managed to create this table to help them build a scatter plot graph:
Participant ID | Daily Hours of Screen Time | Sleep Quality Rating |
---|---|---|
1 | 9 | 3 |
2 | 2 | 8 |
3 | 1 | 9 |
4 | 0 | 10 |
5 | 1 | 9 |
6 | 3 | 7 |
7 | 4 | 7 |
8 | 5 | 6 |
9 | 5 | 6 |
10 | 7 | 3 |
11 | 10 | 1 |
12 | 6 | 5 |
13 | 7 | 3 |
14 | 8 | 2 |
15 | 9 | 2 |
16 | 4 | 7 |
17 | 5 | 6 |
18 | 4 | 7 |
19 | 9 | 2 |
20 | 6 | 4 |
21 | 3 | 7 |
22 | 10 | 1 |
23 | 2 | 8 |
24 | 5 | 6 |
25 | 3 | 7 |
26 | 1 | 9 |
27 | 8 | 2 |
28 | 4 | 6 |
29 | 7 | 3 |
30 | 2 | 8 |
31 | 7 | 4 |
32 | 9 | 2 |
33 | 10 | 1 |
34 | 10 | 1 |
35 | 10 | 1 |
In the provided example, the x-axis represents Daily Hours of Screen Time, and the y-axis represents the Sleep Quality Rating.
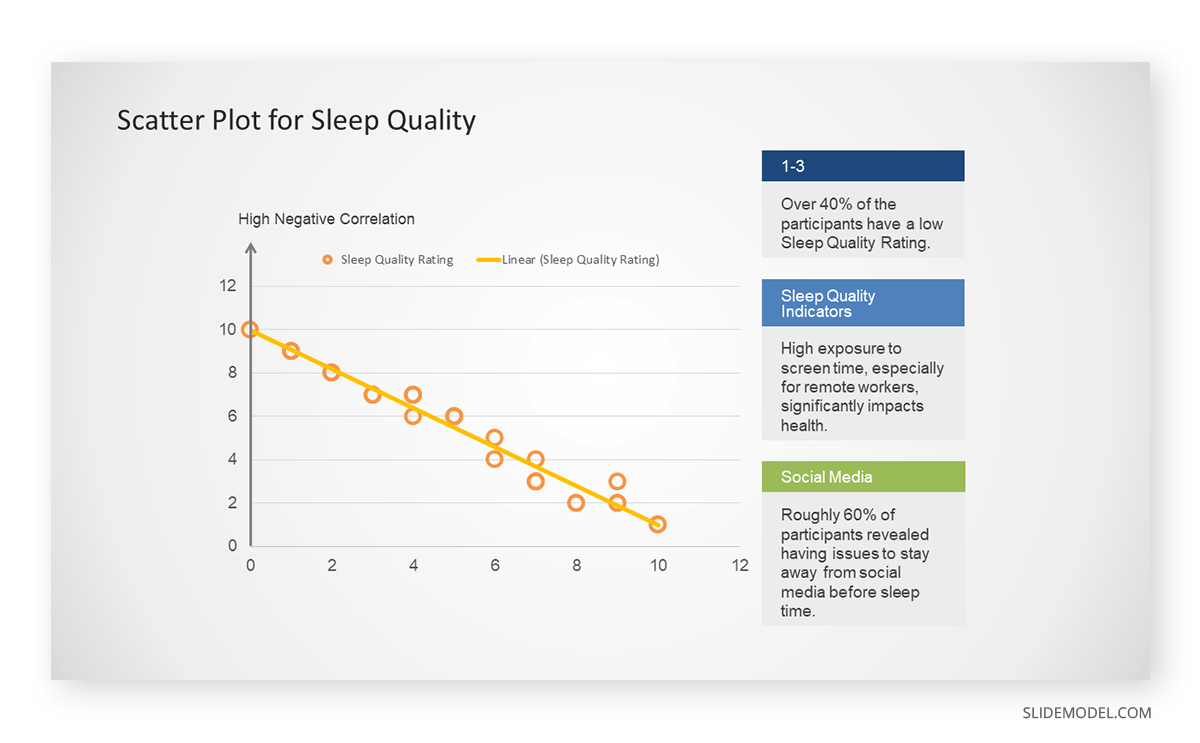
The scientists observe a negative correlation between the amount of screen time and the quality of sleep. This is consistent with their hypothesis that blue light, especially before bedtime, has a significant impact on sleep quality and metabolic processes.
There are a few things to remember when using a scatter plot. Even when a scatter diagram indicates a relationship, it doesn’t mean one variable affects the other. A third factor can influence both variables. The more the plot resembles a straight line, the stronger the relationship is perceived [11] . If it suggests no ties, the observed pattern might be due to random fluctuations in data. When the scatter diagram depicts no correlation, whether the data might be stratified is worth considering.
Choosing the appropriate data presentation type is crucial when making a presentation . Understanding the nature of your data and the message you intend to convey will guide this selection process. For instance, when showcasing quantitative relationships, scatter plots become instrumental in revealing correlations between variables. If the focus is on emphasizing parts of a whole, pie charts offer a concise display of proportions. Histograms, on the other hand, prove valuable for illustrating distributions and frequency patterns.
Bar charts provide a clear visual comparison of different categories. Likewise, line charts excel in showcasing trends over time, while tables are ideal for detailed data examination. Starting a presentation on data presentation types involves evaluating the specific information you want to communicate and selecting the format that aligns with your message. This ensures clarity and resonance with your audience from the beginning of your presentation.
1. Fact Sheet Dashboard for Data Presentation
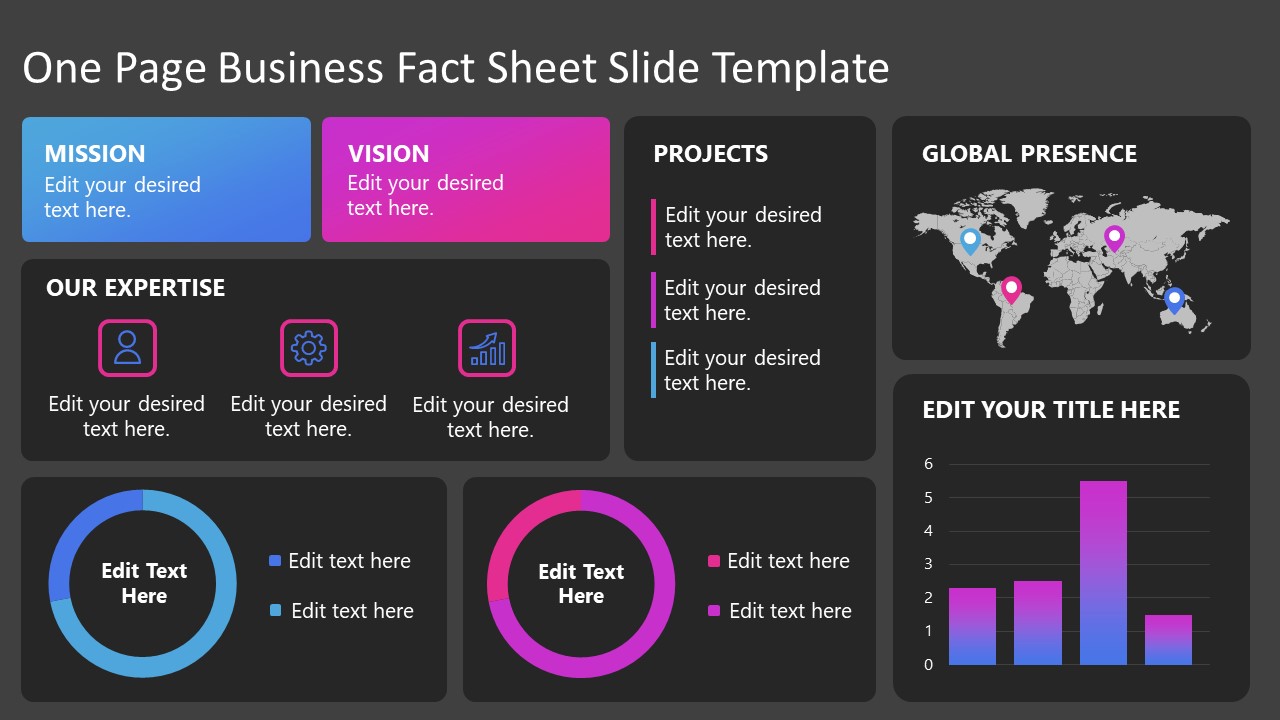
Convey all the data you need to present in this one-pager format, an ideal solution tailored for users looking for presentation aids. Global maps, donut chats, column graphs, and text neatly arranged in a clean layout presented in light and dark themes.
Use This Template
2. 3D Column Chart Infographic PPT Template
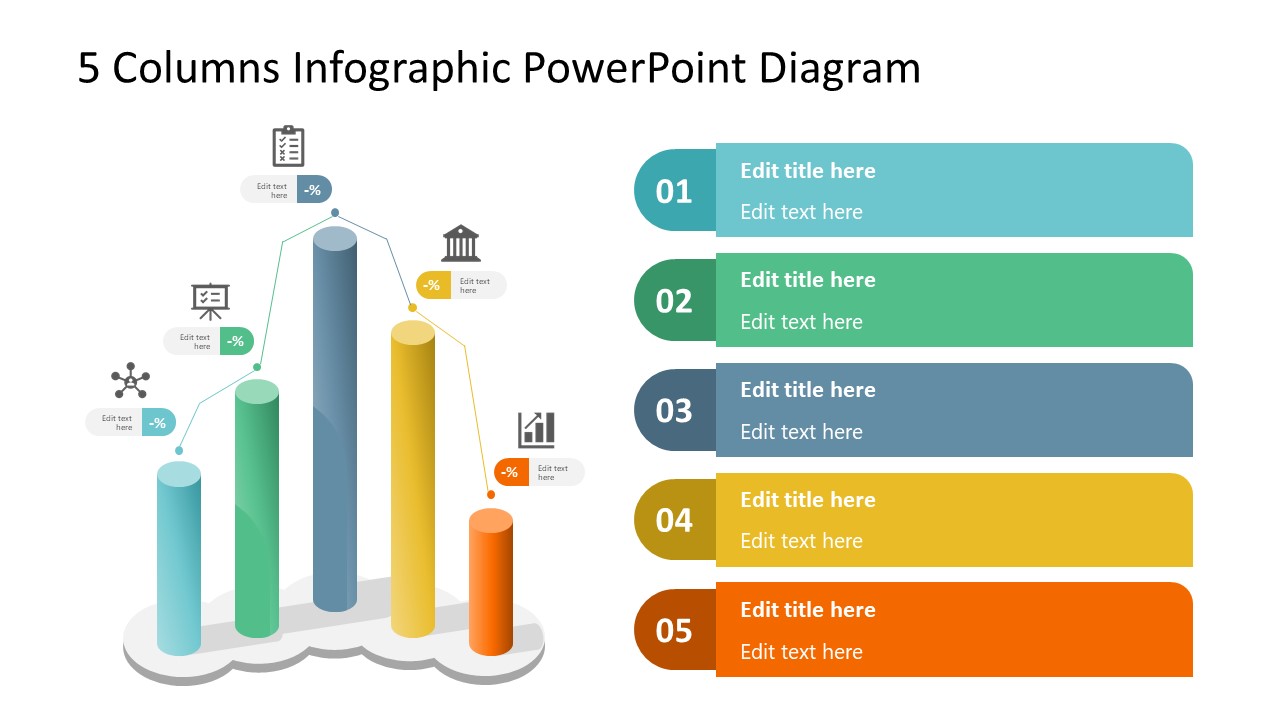
Represent column charts in a highly visual 3D format with this PPT template. A creative way to present data, this template is entirely editable, and we can craft either a one-page infographic or a series of slides explaining what we intend to disclose point by point.
3. Data Circles Infographic PowerPoint Template
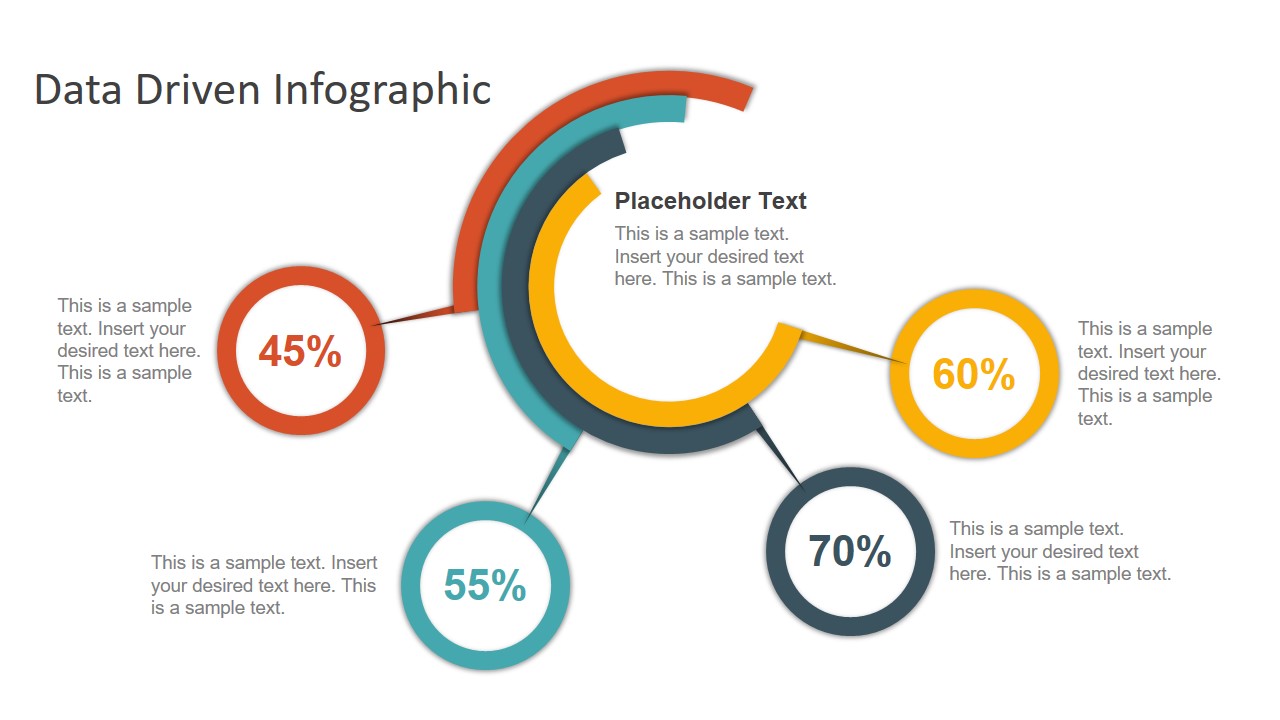
An alternative to the pie chart and donut chart diagrams, this template features a series of curved shapes with bubble callouts as ways of presenting data. Expand the information for each arch in the text placeholder areas.
4. Colorful Metrics Dashboard for Data Presentation
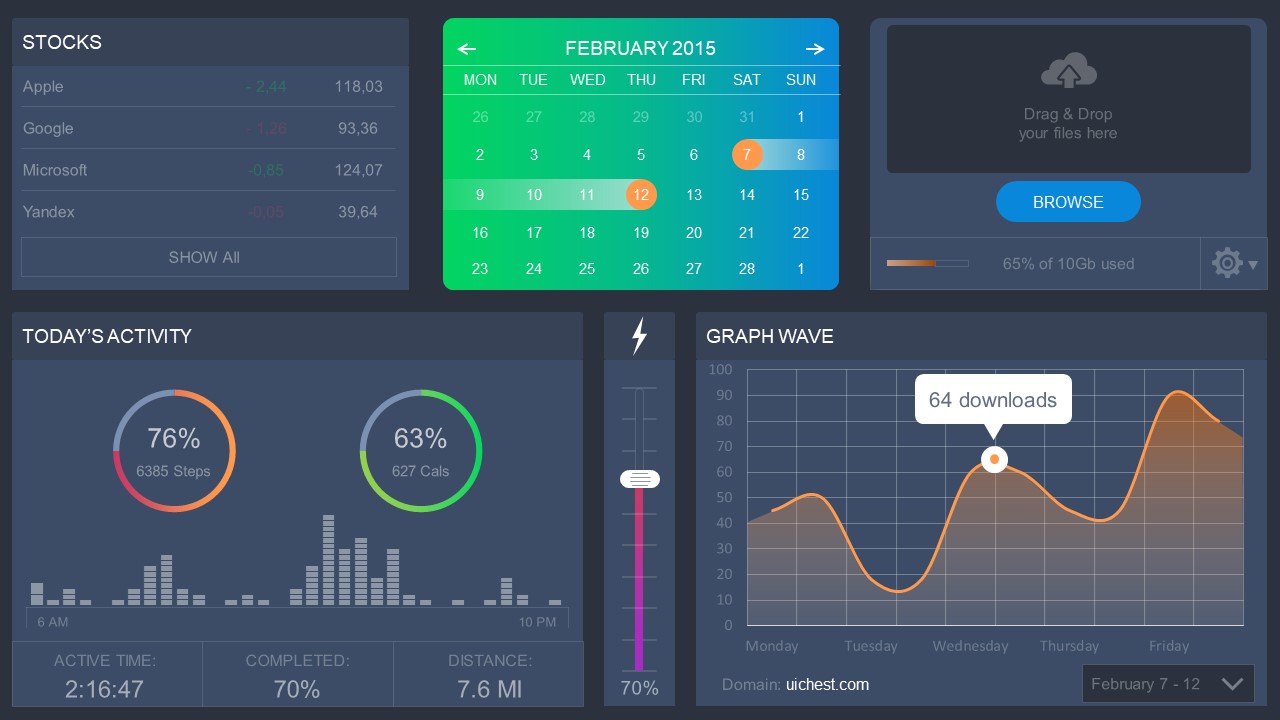
This versatile dashboard template helps us in the presentation of the data by offering several graphs and methods to convert numbers into graphics. Implement it for e-commerce projects, financial projections, project development, and more.
5. Animated Data Presentation Tools for PowerPoint & Google Slides
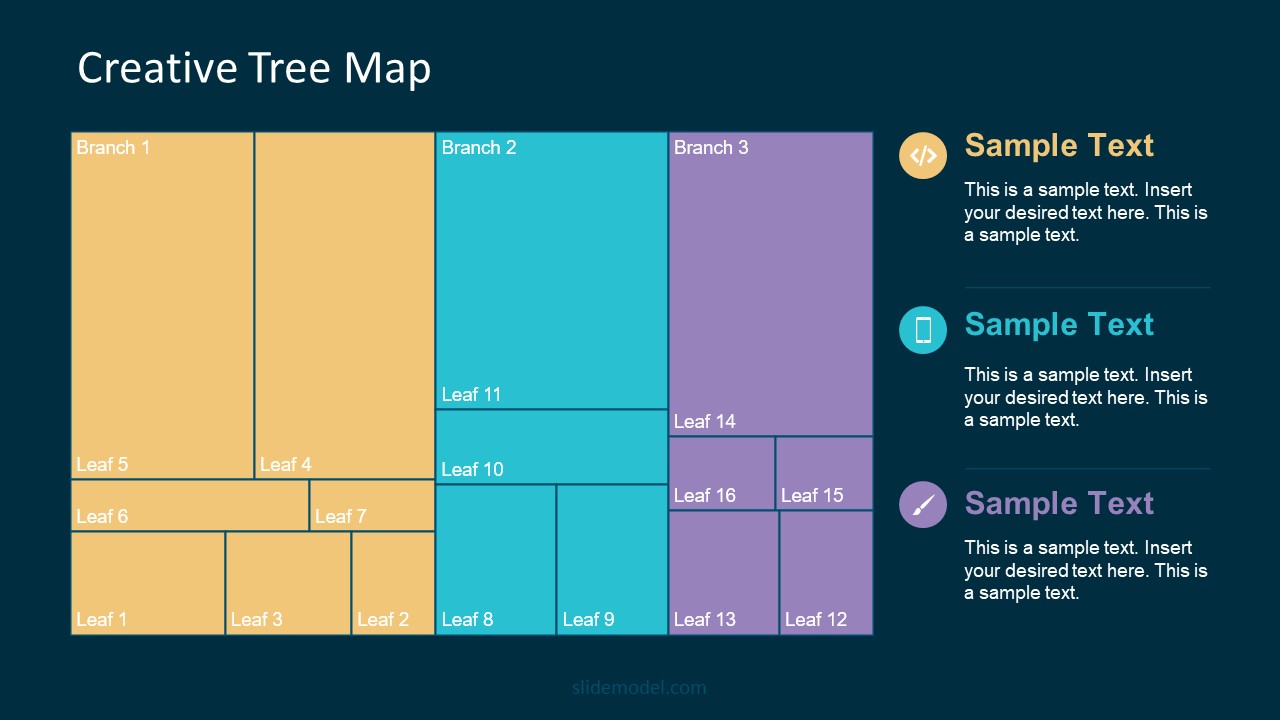
A slide deck filled with most of the tools mentioned in this article, from bar charts, column charts, treemap graphs, pie charts, histogram, etc. Animated effects make each slide look dynamic when sharing data with stakeholders.
6. Statistics Waffle Charts PPT Template for Data Presentations
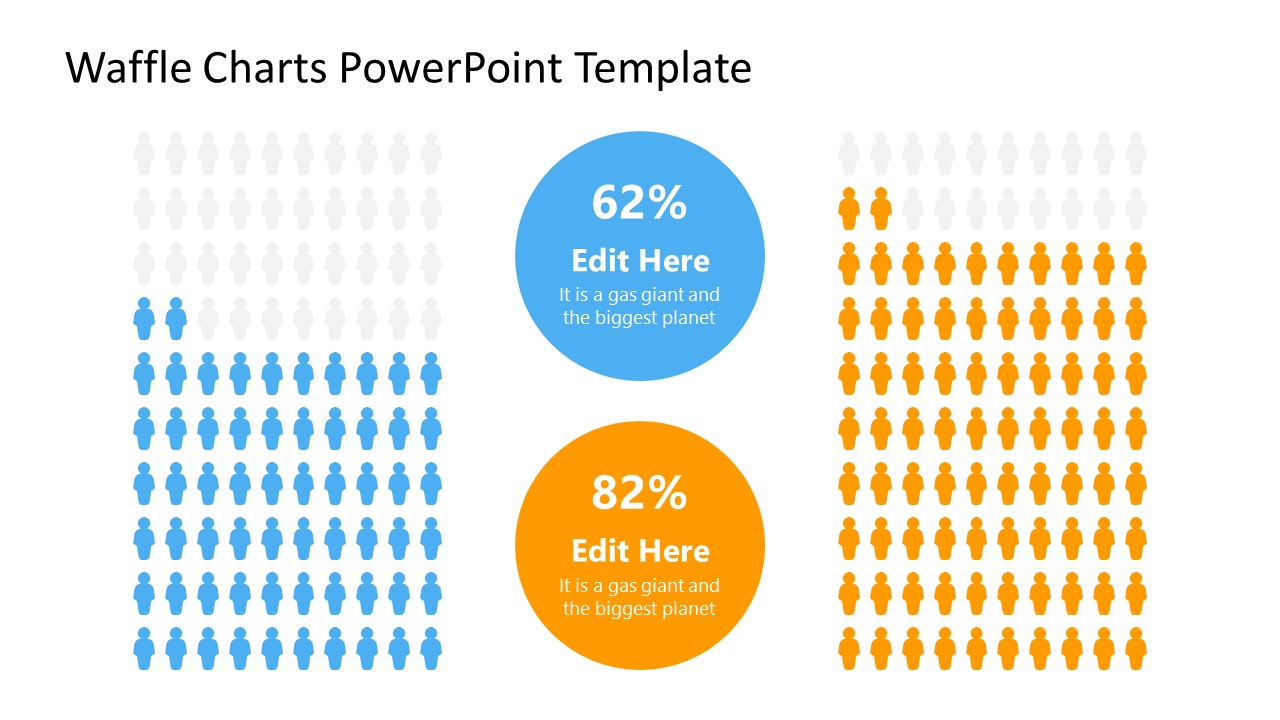
This PPT template helps us how to present data beyond the typical pie chart representation. It is widely used for demographics, so it’s a great fit for marketing teams, data science professionals, HR personnel, and more.
7. Data Presentation Dashboard Template for Google Slides
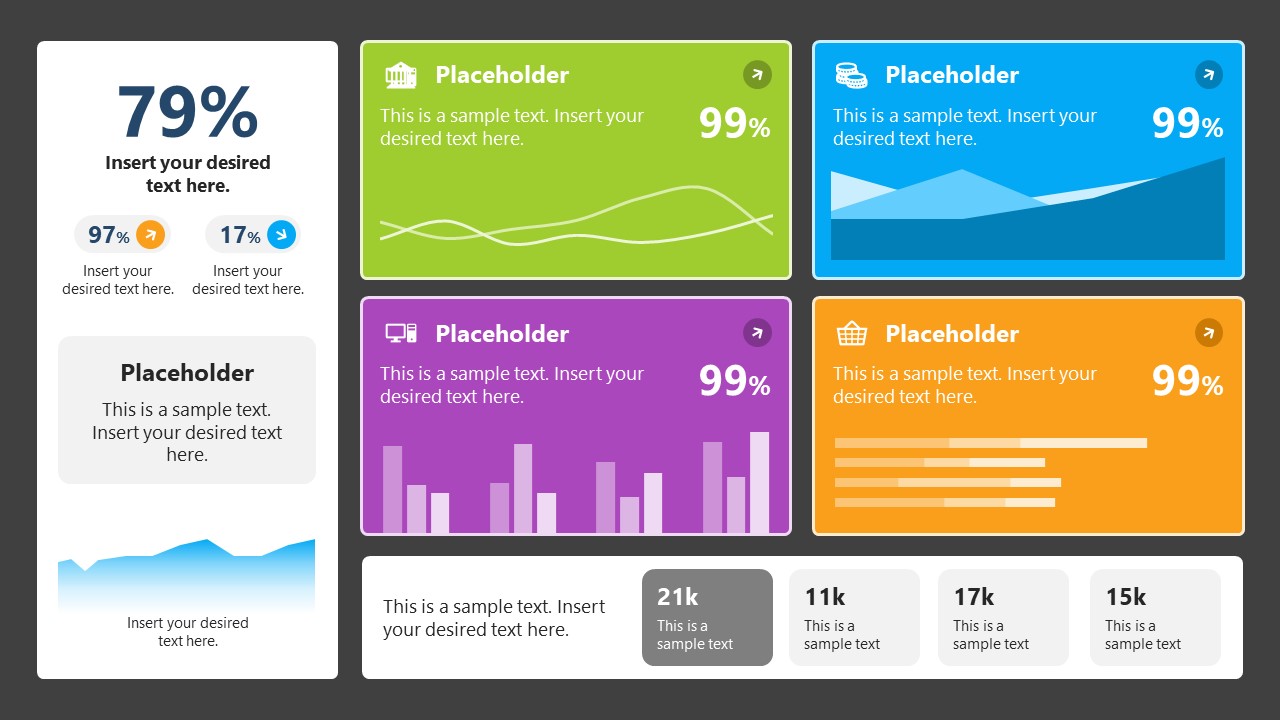
A compendium of tools in dashboard format featuring line graphs, bar charts, column charts, and neatly arranged placeholder text areas.
8. Weather Dashboard for Data Presentation
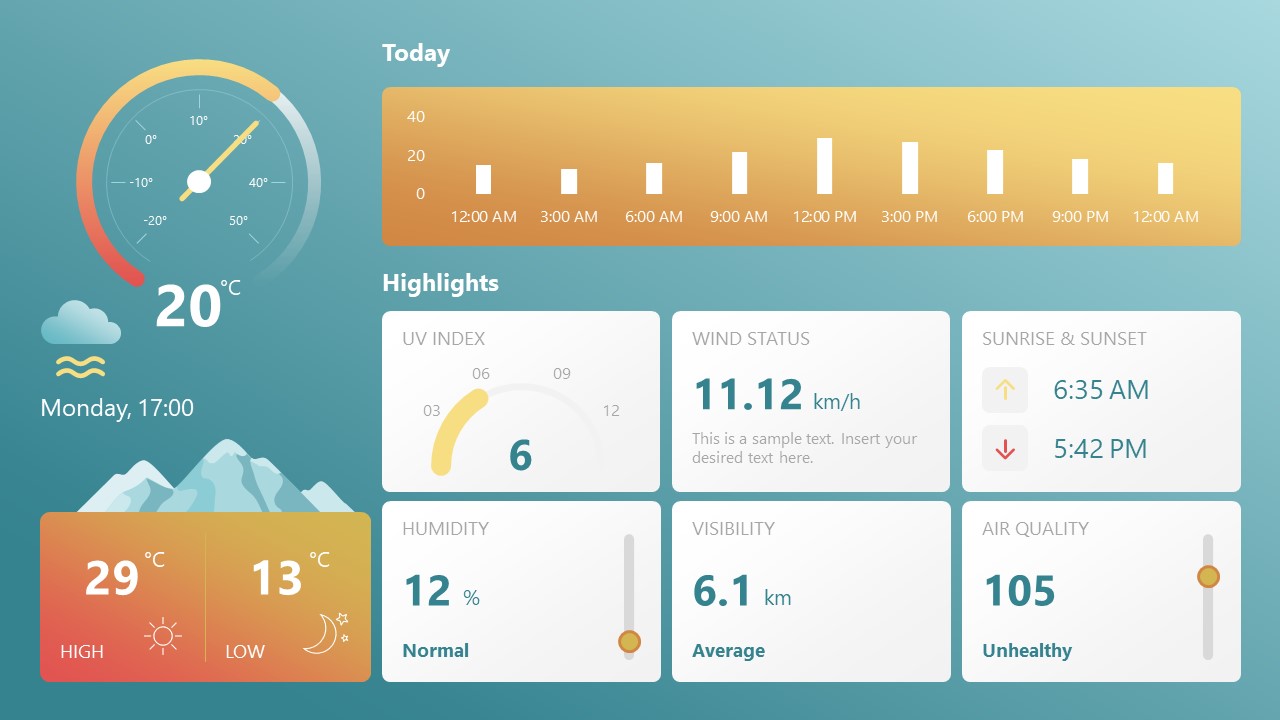
Share weather data for agricultural presentation topics, environmental studies, or any kind of presentation that requires a highly visual layout for weather forecasting on a single day. Two color themes are available.
9. Social Media Marketing Dashboard Data Presentation Template
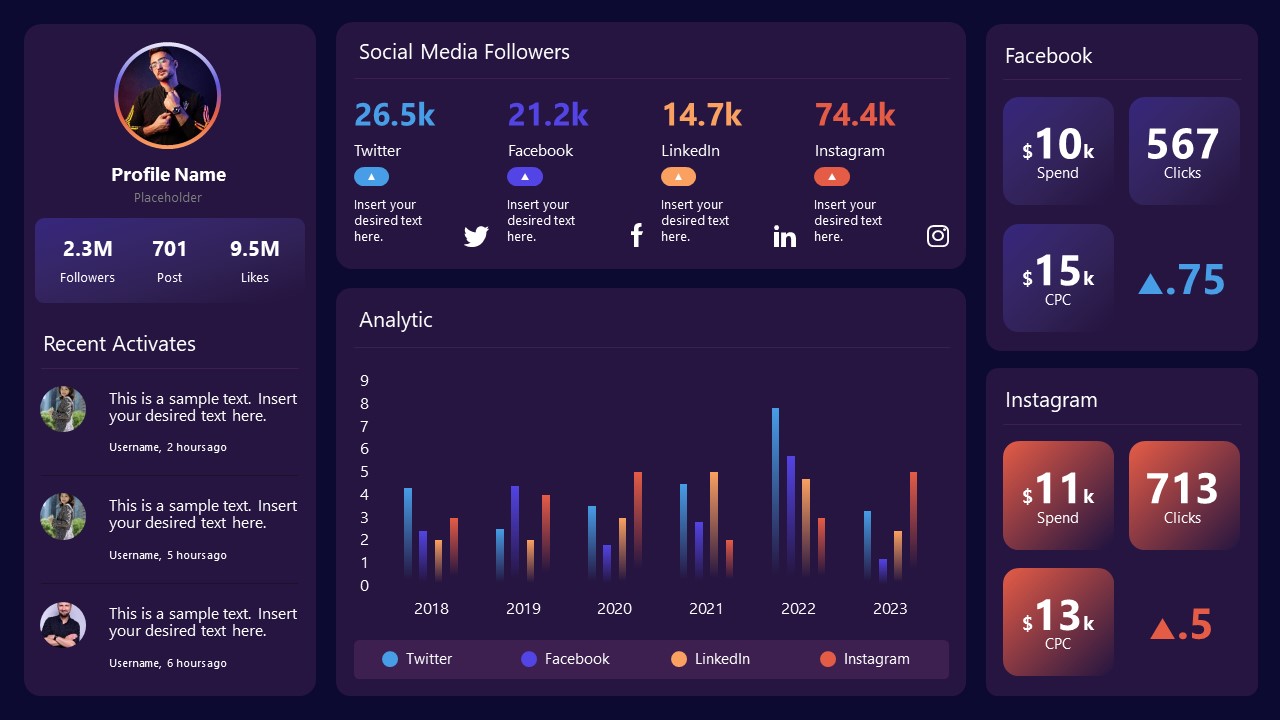
Intended for marketing professionals, this dashboard template for data presentation is a tool for presenting data analytics from social media channels. Two slide layouts featuring line graphs and column charts.
10. Project Management Summary Dashboard Template
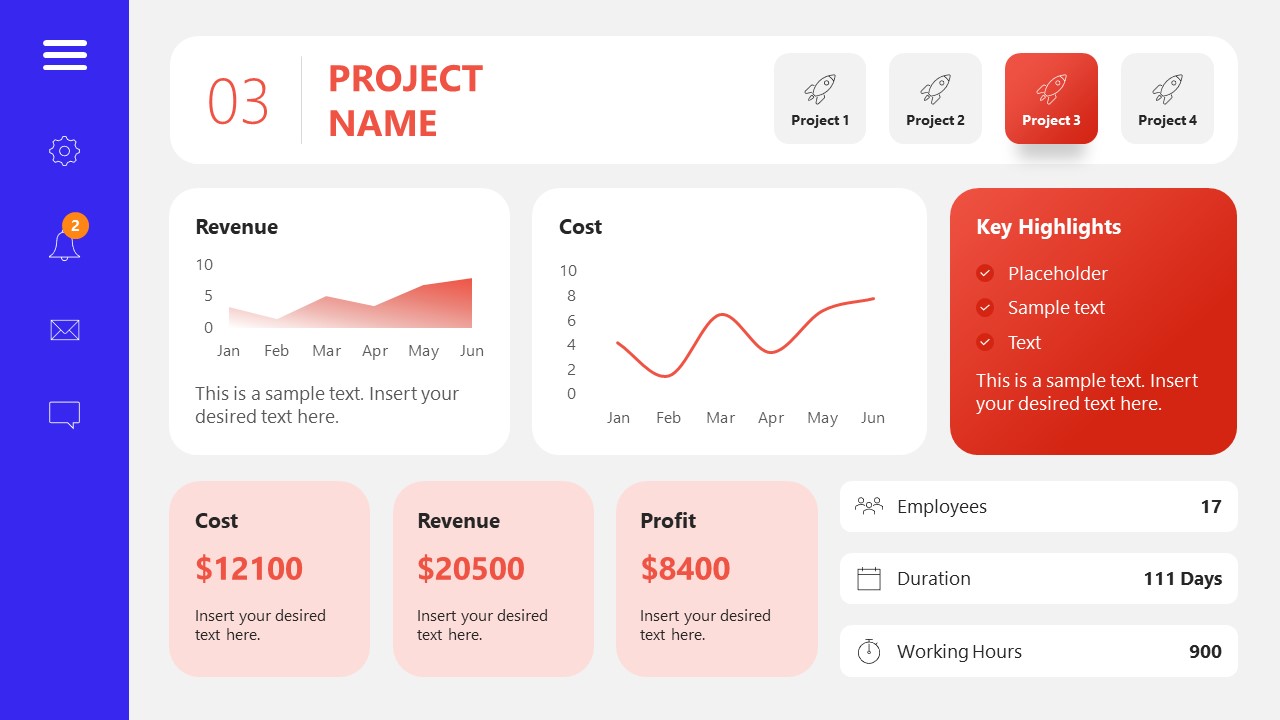
A tool crafted for project managers to deliver highly visual reports on a project’s completion, the profits it delivered for the company, and expenses/time required to execute it. 4 different color layouts are available.
11. Profit & Loss Dashboard for PowerPoint and Google Slides
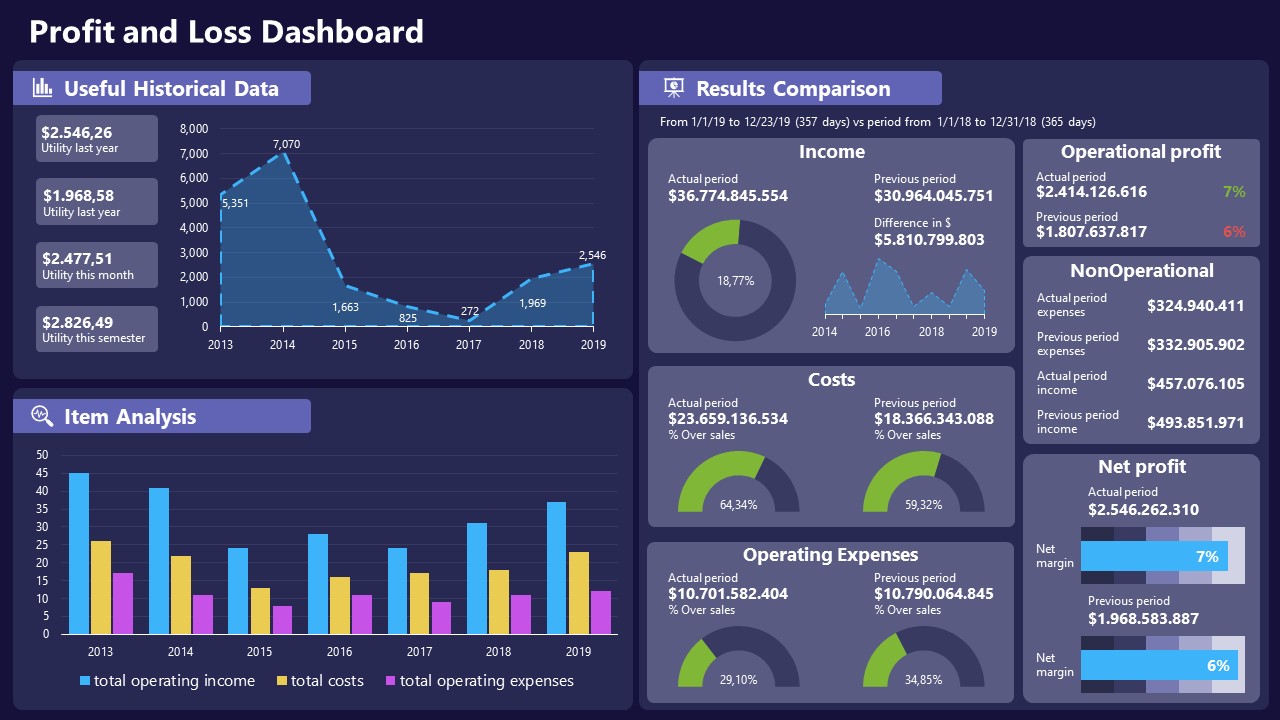
A must-have for finance professionals. This typical profit & loss dashboard includes progress bars, donut charts, column charts, line graphs, and everything that’s required to deliver a comprehensive report about a company’s financial situation.
Overwhelming visuals
One of the mistakes related to using data-presenting methods is including too much data or using overly complex visualizations. They can confuse the audience and dilute the key message.
Inappropriate chart types
Choosing the wrong type of chart for the data at hand can lead to misinterpretation. For example, using a pie chart for data that doesn’t represent parts of a whole is not right.
Lack of context
Failing to provide context or sufficient labeling can make it challenging for the audience to understand the significance of the presented data.
Inconsistency in design
Using inconsistent design elements and color schemes across different visualizations can create confusion and visual disarray.
Failure to provide details
Simply presenting raw data without offering clear insights or takeaways can leave the audience without a meaningful conclusion.
Lack of focus
Not having a clear focus on the key message or main takeaway can result in a presentation that lacks a central theme.
Visual accessibility issues
Overlooking the visual accessibility of charts and graphs can exclude certain audience members who may have difficulty interpreting visual information.
In order to avoid these mistakes in data presentation, presenters can benefit from using presentation templates . These templates provide a structured framework. They ensure consistency, clarity, and an aesthetically pleasing design, enhancing data communication’s overall impact.
Understanding and choosing data presentation types are pivotal in effective communication. Each method serves a unique purpose, so selecting the appropriate one depends on the nature of the data and the message to be conveyed. The diverse array of presentation types offers versatility in visually representing information, from bar charts showing values to pie charts illustrating proportions.
Using the proper method enhances clarity, engages the audience, and ensures that data sets are not just presented but comprehensively understood. By appreciating the strengths and limitations of different presentation types, communicators can tailor their approach to convey information accurately, developing a deeper connection between data and audience understanding.
[1] Government of Canada, S.C. (2021) 5 Data Visualization 5.2 Bar Chart , 5.2 Bar chart . https://www150.statcan.gc.ca/n1/edu/power-pouvoir/ch9/bargraph-diagrammeabarres/5214818-eng.htm
[2] Kosslyn, S.M., 1989. Understanding charts and graphs. Applied cognitive psychology, 3(3), pp.185-225. https://apps.dtic.mil/sti/pdfs/ADA183409.pdf
[3] Creating a Dashboard . https://it.tufts.edu/book/export/html/1870
[4] https://www.goldenwestcollege.edu/research/data-and-more/data-dashboards/index.html
[5] https://www.mit.edu/course/21/21.guide/grf-line.htm
[6] Jadeja, M. and Shah, K., 2015, January. Tree-Map: A Visualization Tool for Large Data. In GSB@ SIGIR (pp. 9-13). https://ceur-ws.org/Vol-1393/gsb15proceedings.pdf#page=15
[7] Heat Maps and Quilt Plots. https://www.publichealth.columbia.edu/research/population-health-methods/heat-maps-and-quilt-plots
[8] EIU QGIS WORKSHOP. https://www.eiu.edu/qgisworkshop/heatmaps.php
[9] About Pie Charts. https://www.mit.edu/~mbarker/formula1/f1help/11-ch-c8.htm
[10] Histograms. https://sites.utexas.edu/sos/guided/descriptive/numericaldd/descriptiven2/histogram/ [11] https://asq.org/quality-resources/scatter-diagram
Like this article? Please share
Data Analysis, Data Science, Data Visualization Filed under Design
Related Articles
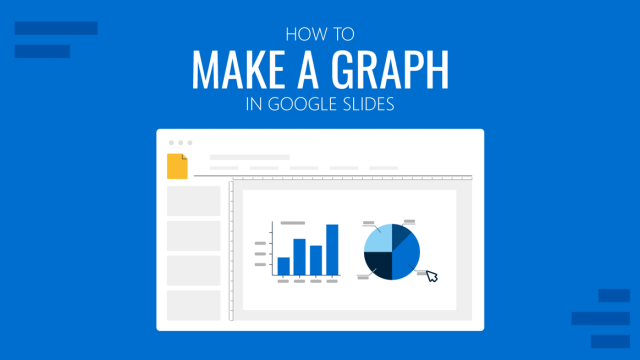
Filed under Google Slides Tutorials • June 3rd, 2024
How To Make a Graph on Google Slides
Creating quality graphics is an essential aspect of designing data presentations. Learn how to make a graph in Google Slides with this guide.
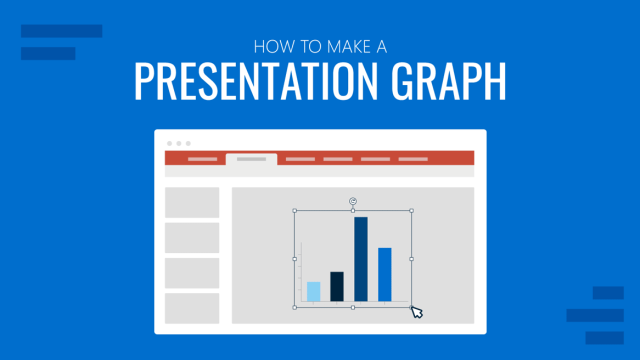
Filed under Design • March 27th, 2024
How to Make a Presentation Graph
Detailed step-by-step instructions to master the art of how to make a presentation graph in PowerPoint and Google Slides. Check it out!
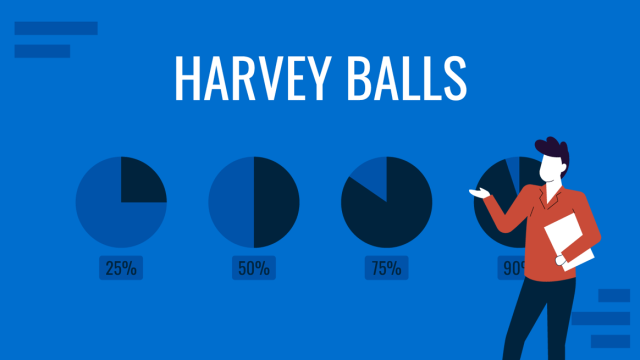
Filed under Presentation Ideas • January 6th, 2024
All About Using Harvey Balls
Among the many tools in the arsenal of the modern presenter, Harvey Balls have a special place. In this article we will tell you all about using Harvey Balls.
Leave a Reply
A Step-by-Step Guide to the Data Analysis Process
Like any scientific discipline, data analysis follows a rigorous step-by-step process. Each stage requires different skills and know-how. To get meaningful insights, though, it’s important to understand the process as a whole. An underlying framework is invaluable for producing results that stand up to scrutiny.
In this post, we’ll explore the main steps in the data analysis process. This will cover how to define your goal, collect data, and carry out an analysis. Where applicable, we’ll also use examples and highlight a few tools to make the journey easier. When you’re done, you’ll have a much better understanding of the basics. This will help you tweak the process to fit your own needs.
Here are the steps we’ll take you through:
- Defining the question
- Collecting the data
- Cleaning the data
- Analyzing the data
- Sharing your results
- Embracing failure
On popular request, we’ve also developed a video based on this article. Scroll further along this article to watch that.
Ready? Let’s get started with step one.
1. Step one: Defining the question
The first step in any data analysis process is to define your objective. In data analytics jargon, this is sometimes called the ‘problem statement’.
Defining your objective means coming up with a hypothesis and figuring how to test it. Start by asking: What business problem am I trying to solve? While this might sound straightforward, it can be trickier than it seems. For instance, your organization’s senior management might pose an issue, such as: “Why are we losing customers?” It’s possible, though, that this doesn’t get to the core of the problem. A data analyst’s job is to understand the business and its goals in enough depth that they can frame the problem the right way.
Let’s say you work for a fictional company called TopNotch Learning. TopNotch creates custom training software for its clients. While it is excellent at securing new clients, it has much lower repeat business. As such, your question might not be, “Why are we losing customers?” but, “Which factors are negatively impacting the customer experience?” or better yet: “How can we boost customer retention while minimizing costs?”
Now you’ve defined a problem, you need to determine which sources of data will best help you solve it. This is where your business acumen comes in again. For instance, perhaps you’ve noticed that the sales process for new clients is very slick, but that the production team is inefficient. Knowing this, you could hypothesize that the sales process wins lots of new clients, but the subsequent customer experience is lacking. Could this be why customers don’t come back? Which sources of data will help you answer this question?
Tools to help define your objective
Defining your objective is mostly about soft skills, business knowledge, and lateral thinking. But you’ll also need to keep track of business metrics and key performance indicators (KPIs). Monthly reports can allow you to track problem points in the business. Some KPI dashboards come with a fee, like Databox and DashThis . However, you’ll also find open-source software like Grafana , Freeboard , and Dashbuilder . These are great for producing simple dashboards, both at the beginning and the end of the data analysis process.
2. Step two: Collecting the data
Once you’ve established your objective, you’ll need to create a strategy for collecting and aggregating the appropriate data. A key part of this is determining which data you need. This might be quantitative (numeric) data, e.g. sales figures, or qualitative (descriptive) data, such as customer reviews. All data fit into one of three categories: first-party, second-party, and third-party data. Let’s explore each one.
What is first-party data?
First-party data are data that you, or your company, have directly collected from customers. It might come in the form of transactional tracking data or information from your company’s customer relationship management (CRM) system. Whatever its source, first-party data is usually structured and organized in a clear, defined way. Other sources of first-party data might include customer satisfaction surveys, focus groups, interviews, or direct observation.
What is second-party data?
To enrich your analysis, you might want to secure a secondary data source. Second-party data is the first-party data of other organizations. This might be available directly from the company or through a private marketplace. The main benefit of second-party data is that they are usually structured, and although they will be less relevant than first-party data, they also tend to be quite reliable. Examples of second-party data include website, app or social media activity, like online purchase histories, or shipping data.
What is third-party data?
Third-party data is data that has been collected and aggregated from numerous sources by a third-party organization. Often (though not always) third-party data contains a vast amount of unstructured data points (big data). Many organizations collect big data to create industry reports or to conduct market research. The research and advisory firm Gartner is a good real-world example of an organization that collects big data and sells it on to other companies. Open data repositories and government portals are also sources of third-party data .
Tools to help you collect data
Once you’ve devised a data strategy (i.e. you’ve identified which data you need, and how best to go about collecting them) there are many tools you can use to help you. One thing you’ll need, regardless of industry or area of expertise, is a data management platform (DMP). A DMP is a piece of software that allows you to identify and aggregate data from numerous sources, before manipulating them, segmenting them, and so on. There are many DMPs available. Some well-known enterprise DMPs include Salesforce DMP , SAS , and the data integration platform, Xplenty . If you want to play around, you can also try some open-source platforms like Pimcore or D:Swarm .
Want to learn more about what data analytics is and the process a data analyst follows? We cover this topic (and more) in our free introductory short course for beginners. Check out tutorial one: An introduction to data analytics .
3. Step three: Cleaning the data
Once you’ve collected your data, the next step is to get it ready for analysis. This means cleaning, or ‘scrubbing’ it, and is crucial in making sure that you’re working with high-quality data . Key data cleaning tasks include:
- Removing major errors, duplicates, and outliers —all of which are inevitable problems when aggregating data from numerous sources.
- Removing unwanted data points —extracting irrelevant observations that have no bearing on your intended analysis.
- Bringing structure to your data —general ‘housekeeping’, i.e. fixing typos or layout issues, which will help you map and manipulate your data more easily.
- Filling in major gaps —as you’re tidying up, you might notice that important data are missing. Once you’ve identified gaps, you can go about filling them.
A good data analyst will spend around 70-90% of their time cleaning their data. This might sound excessive. But focusing on the wrong data points (or analyzing erroneous data) will severely impact your results. It might even send you back to square one…so don’t rush it! You’ll find a step-by-step guide to data cleaning here . You may be interested in this introductory tutorial to data cleaning, hosted by Dr. Humera Noor Minhas.
Carrying out an exploratory analysis
Another thing many data analysts do (alongside cleaning data) is to carry out an exploratory analysis. This helps identify initial trends and characteristics, and can even refine your hypothesis. Let’s use our fictional learning company as an example again. Carrying out an exploratory analysis, perhaps you notice a correlation between how much TopNotch Learning’s clients pay and how quickly they move on to new suppliers. This might suggest that a low-quality customer experience (the assumption in your initial hypothesis) is actually less of an issue than cost. You might, therefore, take this into account.
Tools to help you clean your data
Cleaning datasets manually—especially large ones—can be daunting. Luckily, there are many tools available to streamline the process. Open-source tools, such as OpenRefine , are excellent for basic data cleaning, as well as high-level exploration. However, free tools offer limited functionality for very large datasets. Python libraries (e.g. Pandas) and some R packages are better suited for heavy data scrubbing. You will, of course, need to be familiar with the languages. Alternatively, enterprise tools are also available. For example, Data Ladder , which is one of the highest-rated data-matching tools in the industry. There are many more. Why not see which free data cleaning tools you can find to play around with?
4. Step four: Analyzing the data
Finally, you’ve cleaned your data. Now comes the fun bit—analyzing it! The type of data analysis you carry out largely depends on what your goal is. But there are many techniques available. Univariate or bivariate analysis, time-series analysis, and regression analysis are just a few you might have heard of. More important than the different types, though, is how you apply them. This depends on what insights you’re hoping to gain. Broadly speaking, all types of data analysis fit into one of the following four categories.
Descriptive analysis
Descriptive analysis identifies what has already happened . It is a common first step that companies carry out before proceeding with deeper explorations. As an example, let’s refer back to our fictional learning provider once more. TopNotch Learning might use descriptive analytics to analyze course completion rates for their customers. Or they might identify how many users access their products during a particular period. Perhaps they’ll use it to measure sales figures over the last five years. While the company might not draw firm conclusions from any of these insights, summarizing and describing the data will help them to determine how to proceed.
Learn more: What is descriptive analytics?
Diagnostic analysis
Diagnostic analytics focuses on understanding why something has happened . It is literally the diagnosis of a problem, just as a doctor uses a patient’s symptoms to diagnose a disease. Remember TopNotch Learning’s business problem? ‘Which factors are negatively impacting the customer experience?’ A diagnostic analysis would help answer this. For instance, it could help the company draw correlations between the issue (struggling to gain repeat business) and factors that might be causing it (e.g. project costs, speed of delivery, customer sector, etc.) Let’s imagine that, using diagnostic analytics, TopNotch realizes its clients in the retail sector are departing at a faster rate than other clients. This might suggest that they’re losing customers because they lack expertise in this sector. And that’s a useful insight!
Predictive analysis
Predictive analysis allows you to identify future trends based on historical data . In business, predictive analysis is commonly used to forecast future growth, for example. But it doesn’t stop there. Predictive analysis has grown increasingly sophisticated in recent years. The speedy evolution of machine learning allows organizations to make surprisingly accurate forecasts. Take the insurance industry. Insurance providers commonly use past data to predict which customer groups are more likely to get into accidents. As a result, they’ll hike up customer insurance premiums for those groups. Likewise, the retail industry often uses transaction data to predict where future trends lie, or to determine seasonal buying habits to inform their strategies. These are just a few simple examples, but the untapped potential of predictive analysis is pretty compelling.
Prescriptive analysis
Prescriptive analysis allows you to make recommendations for the future. This is the final step in the analytics part of the process. It’s also the most complex. This is because it incorporates aspects of all the other analyses we’ve described. A great example of prescriptive analytics is the algorithms that guide Google’s self-driving cars. Every second, these algorithms make countless decisions based on past and present data, ensuring a smooth, safe ride. Prescriptive analytics also helps companies decide on new products or areas of business to invest in.
Learn more: What are the different types of data analysis?
5. Step five: Sharing your results
You’ve finished carrying out your analyses. You have your insights. The final step of the data analytics process is to share these insights with the wider world (or at least with your organization’s stakeholders!) This is more complex than simply sharing the raw results of your work—it involves interpreting the outcomes, and presenting them in a manner that’s digestible for all types of audiences. Since you’ll often present information to decision-makers, it’s very important that the insights you present are 100% clear and unambiguous. For this reason, data analysts commonly use reports, dashboards, and interactive visualizations to support their findings.
How you interpret and present results will often influence the direction of a business. Depending on what you share, your organization might decide to restructure, to launch a high-risk product, or even to close an entire division. That’s why it’s very important to provide all the evidence that you’ve gathered, and not to cherry-pick data. Ensuring that you cover everything in a clear, concise way will prove that your conclusions are scientifically sound and based on the facts. On the flip side, it’s important to highlight any gaps in the data or to flag any insights that might be open to interpretation. Honest communication is the most important part of the process. It will help the business, while also helping you to excel at your job!
Tools for interpreting and sharing your findings
There are tons of data visualization tools available, suited to different experience levels. Popular tools requiring little or no coding skills include Google Charts , Tableau , Datawrapper , and Infogram . If you’re familiar with Python and R, there are also many data visualization libraries and packages available. For instance, check out the Python libraries Plotly , Seaborn , and Matplotlib . Whichever data visualization tools you use, make sure you polish up your presentation skills, too. Remember: Visualization is great, but communication is key!
You can learn more about storytelling with data in this free, hands-on tutorial . We show you how to craft a compelling narrative for a real dataset, resulting in a presentation to share with key stakeholders. This is an excellent insight into what it’s really like to work as a data analyst!
6. Step six: Embrace your failures
The last ‘step’ in the data analytics process is to embrace your failures. The path we’ve described above is more of an iterative process than a one-way street. Data analytics is inherently messy, and the process you follow will be different for every project. For instance, while cleaning data, you might spot patterns that spark a whole new set of questions. This could send you back to step one (to redefine your objective). Equally, an exploratory analysis might highlight a set of data points you’d never considered using before. Or maybe you find that the results of your core analyses are misleading or erroneous. This might be caused by mistakes in the data, or human error earlier in the process.
While these pitfalls can feel like failures, don’t be disheartened if they happen. Data analysis is inherently chaotic, and mistakes occur. What’s important is to hone your ability to spot and rectify errors. If data analytics was straightforward, it might be easier, but it certainly wouldn’t be as interesting. Use the steps we’ve outlined as a framework, stay open-minded, and be creative. If you lose your way, you can refer back to the process to keep yourself on track.
In this post, we’ve covered the main steps of the data analytics process. These core steps can be amended, re-ordered and re-used as you deem fit, but they underpin every data analyst’s work:
- Define the question —What business problem are you trying to solve? Frame it as a question to help you focus on finding a clear answer.
- Collect data —Create a strategy for collecting data. Which data sources are most likely to help you solve your business problem?
- Clean the data —Explore, scrub, tidy, de-dupe, and structure your data as needed. Do whatever you have to! But don’t rush…take your time!
- Analyze the data —Carry out various analyses to obtain insights. Focus on the four types of data analysis: descriptive, diagnostic, predictive, and prescriptive.
- Share your results —How best can you share your insights and recommendations? A combination of visualization tools and communication is key.
- Embrace your mistakes —Mistakes happen. Learn from them. This is what transforms a good data analyst into a great one.
What next? From here, we strongly encourage you to explore the topic on your own. Get creative with the steps in the data analysis process, and see what tools you can find. As long as you stick to the core principles we’ve described, you can create a tailored technique that works for you.
To learn more, check out our free, 5-day data analytics short course . You might also be interested in the following:
- These are the top 9 data analytics tools
- 10 great places to find free datasets for your next project
- How to build a data analytics portfolio
We use essential cookies to make Venngage work. By clicking “Accept All Cookies”, you agree to the storing of cookies on your device to enhance site navigation, analyze site usage, and assist in our marketing efforts.
Manage Cookies
Cookies and similar technologies collect certain information about how you’re using our website. Some of them are essential, and without them you wouldn’t be able to use Venngage. But others are optional, and you get to choose whether we use them or not.
Strictly Necessary Cookies
These cookies are always on, as they’re essential for making Venngage work, and making it safe. Without these cookies, services you’ve asked for can’t be provided.
Show cookie providers
- Google Login
Functionality Cookies
These cookies help us provide enhanced functionality and personalisation, and remember your settings. They may be set by us or by third party providers.
Performance Cookies
These cookies help us analyze how many people are using Venngage, where they come from and how they're using it. If you opt out of these cookies, we can’t get feedback to make Venngage better for you and all our users.
- Google Analytics
Targeting Cookies
These cookies are set by our advertising partners to track your activity and show you relevant Venngage ads on other sites as you browse the internet.
- Google Tag Manager
- Infographics
- Daily Infographics
- Popular Templates
- Accessibility
- Graphic Design
- Graphs and Charts
- Data Visualization
- Human Resources
- Beginner Guides
Blog Data Visualization 10 Data Presentation Examples For Strategic Communication
10 Data Presentation Examples For Strategic Communication
Written by: Krystle Wong Sep 28, 2023
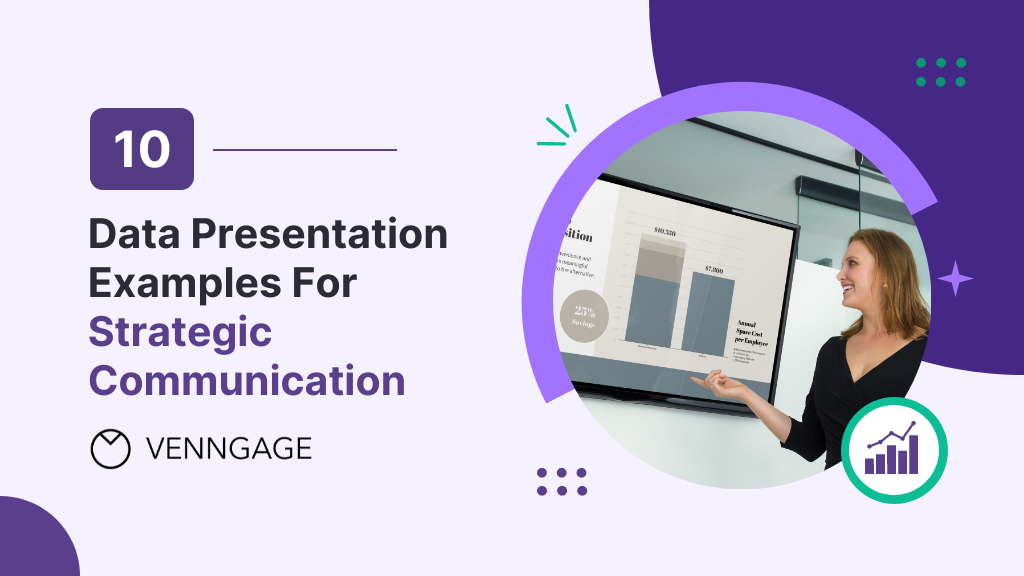
Knowing how to present data is like having a superpower.
Data presentation today is no longer just about numbers on a screen; it’s storytelling with a purpose. It’s about captivating your audience, making complex stuff look simple and inspiring action.
To help turn your data into stories that stick, influence decisions and make an impact, check out Venngage’s free chart maker or follow me on a tour into the world of data storytelling along with data presentation templates that work across different fields, from business boardrooms to the classroom and beyond. Keep scrolling to learn more!
Click to jump ahead:
10 Essential data presentation examples + methods you should know
What should be included in a data presentation, what are some common mistakes to avoid when presenting data, faqs on data presentation examples, transform your message with impactful data storytelling.
Data presentation is a vital skill in today’s information-driven world. Whether you’re in business, academia, or simply want to convey information effectively, knowing the different ways of presenting data is crucial. For impactful data storytelling, consider these essential data presentation methods:
1. Bar graph
Ideal for comparing data across categories or showing trends over time.
Bar graphs, also known as bar charts are workhorses of data presentation. They’re like the Swiss Army knives of visualization methods because they can be used to compare data in different categories or display data changes over time.
In a bar chart, categories are displayed on the x-axis and the corresponding values are represented by the height of the bars on the y-axis.
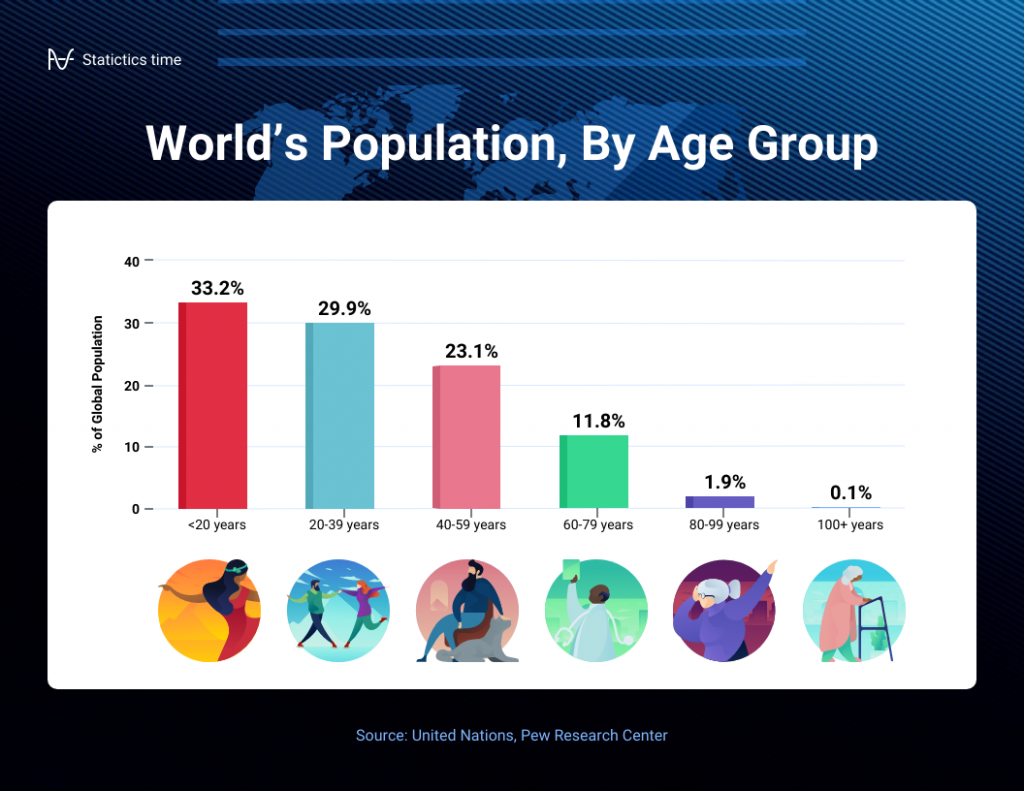
It’s a straightforward and effective way to showcase raw data, making it a staple in business reports, academic presentations and beyond.
Make sure your bar charts are concise with easy-to-read labels. Whether your bars go up or sideways, keep it simple by not overloading with too many categories.
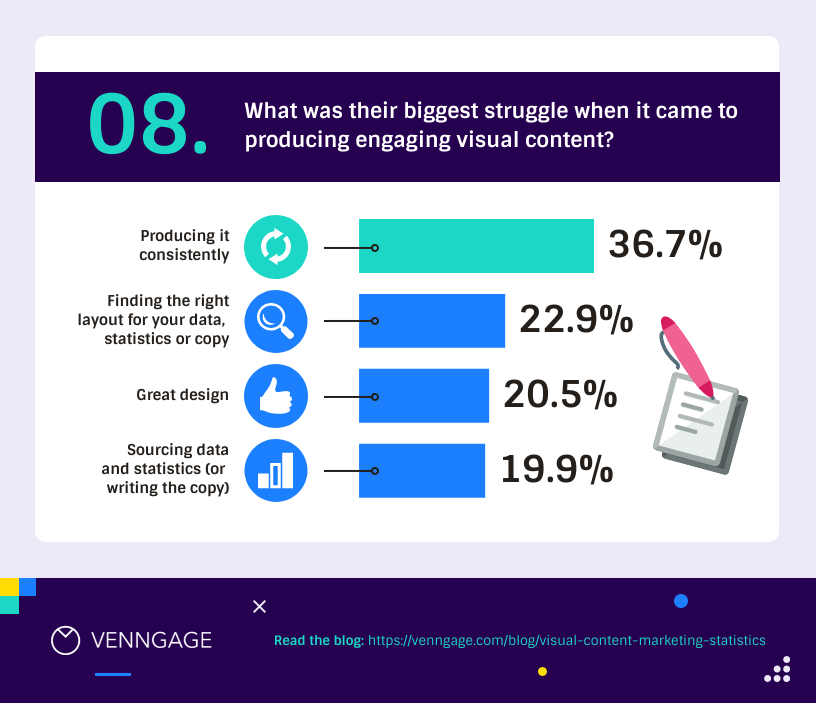
2. Line graph
Great for displaying trends and variations in data points over time or continuous variables.
Line charts or line graphs are your go-to when you want to visualize trends and variations in data sets over time.
One of the best quantitative data presentation examples, they work exceptionally well for showing continuous data, such as sales projections over the last couple of years or supply and demand fluctuations.
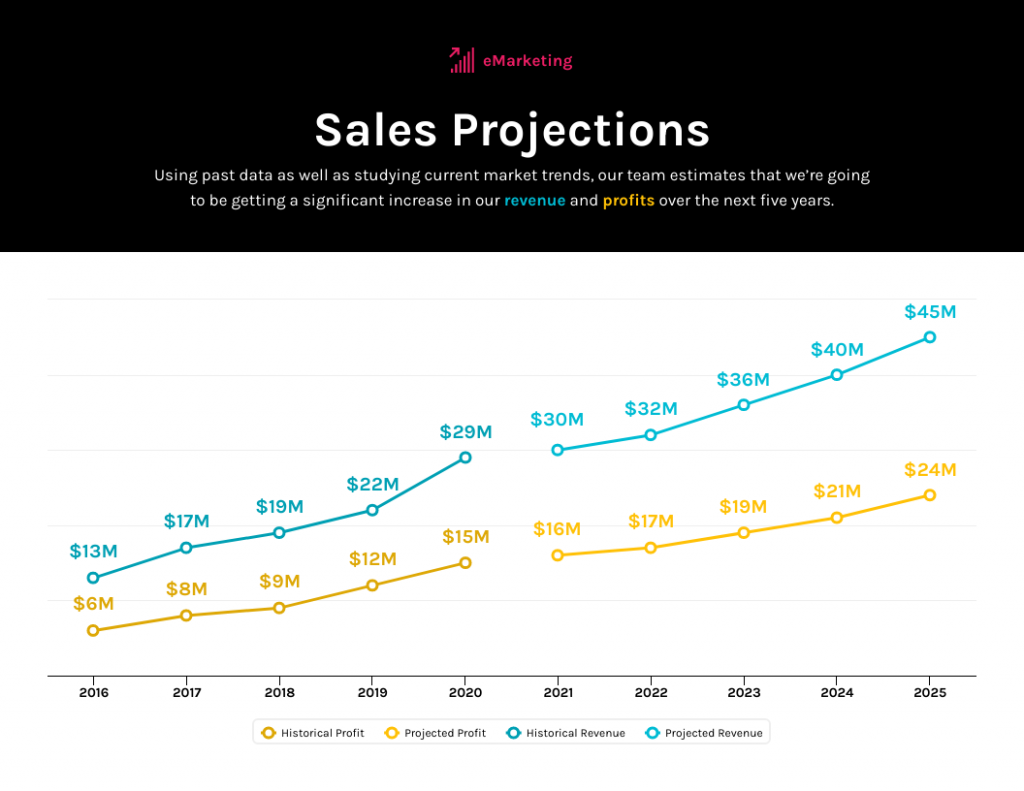
The x-axis represents time or a continuous variable and the y-axis represents the data values. By connecting the data points with lines, you can easily spot trends and fluctuations.
A tip when presenting data with line charts is to minimize the lines and not make it too crowded. Highlight the big changes, put on some labels and give it a catchy title.
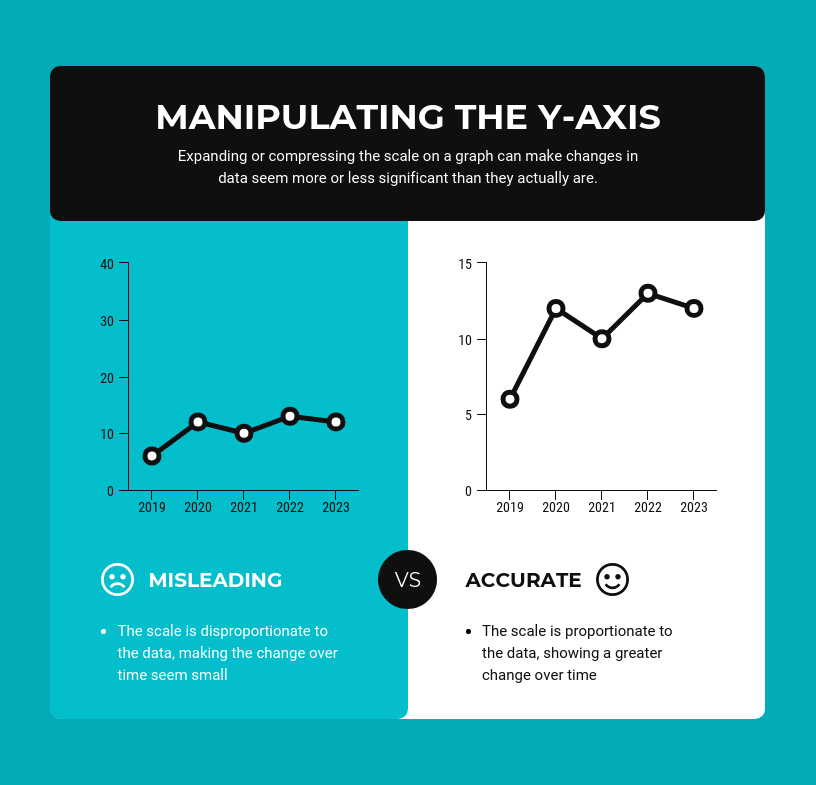
3. Pie chart
Useful for illustrating parts of a whole, such as percentages or proportions.
Pie charts are perfect for showing how a whole is divided into parts. They’re commonly used to represent percentages or proportions and are great for presenting survey results that involve demographic data.
Each “slice” of the pie represents a portion of the whole and the size of each slice corresponds to its share of the total.
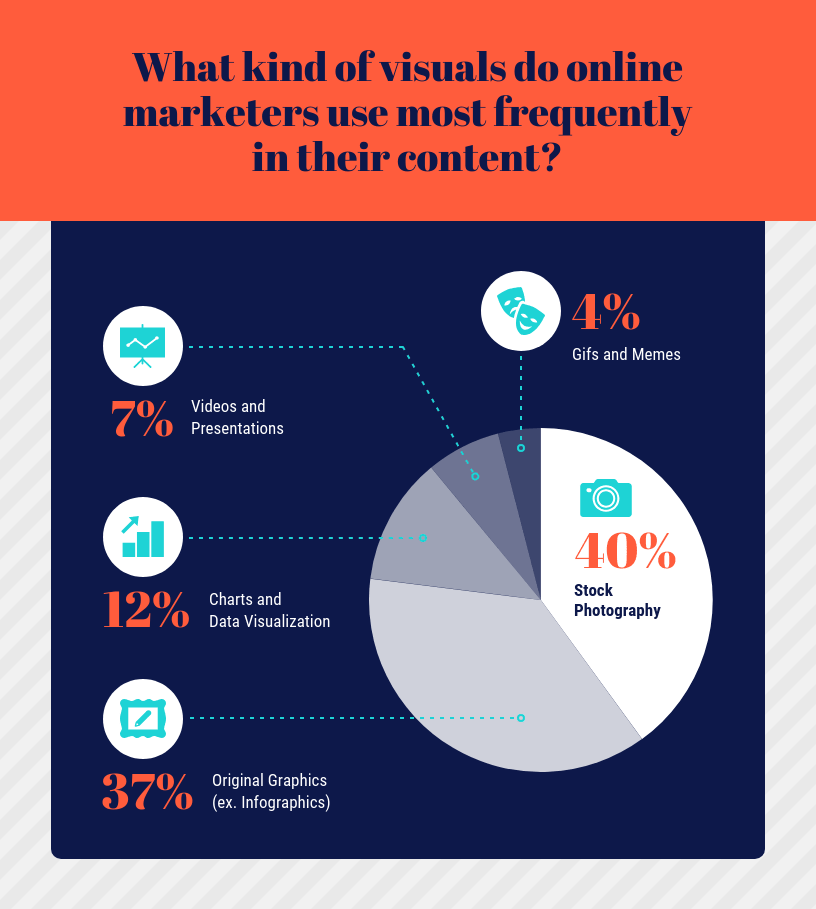
While pie charts are handy for illustrating simple distributions, they can become confusing when dealing with too many categories or when the differences in proportions are subtle.
Don’t get too carried away with slices — label those slices with percentages or values so people know what’s what and consider using a legend for more categories.
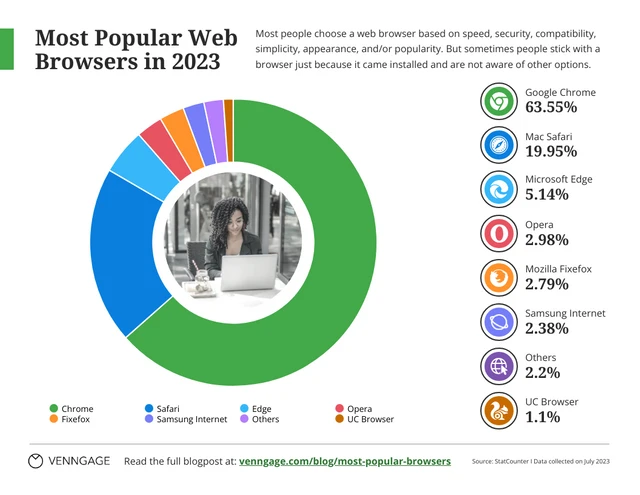
4. Scatter plot
Effective for showing the relationship between two variables and identifying correlations.
Scatter plots are all about exploring relationships between two variables. They’re great for uncovering correlations, trends or patterns in data.
In a scatter plot, every data point appears as a dot on the chart, with one variable marked on the horizontal x-axis and the other on the vertical y-axis.
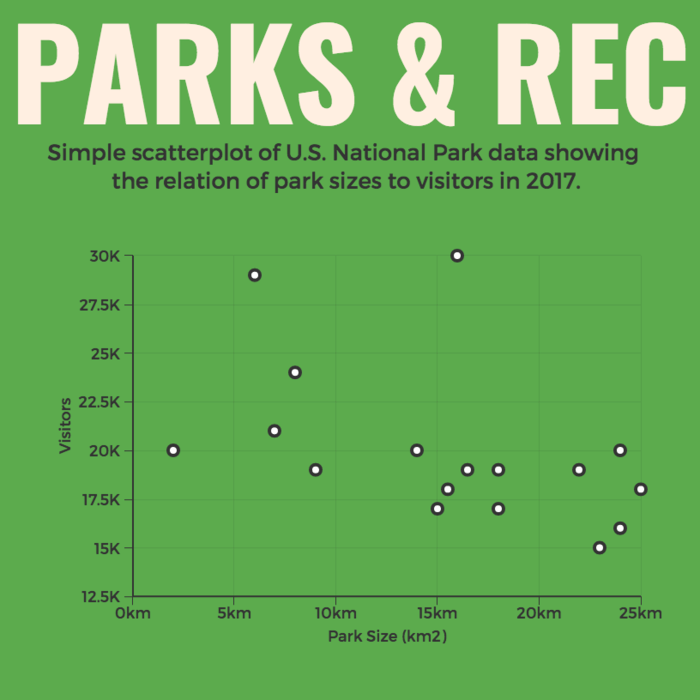
By examining the scatter of points, you can discern the nature of the relationship between the variables, whether it’s positive, negative or no correlation at all.
If you’re using scatter plots to reveal relationships between two variables, be sure to add trendlines or regression analysis when appropriate to clarify patterns. Label data points selectively or provide tooltips for detailed information.
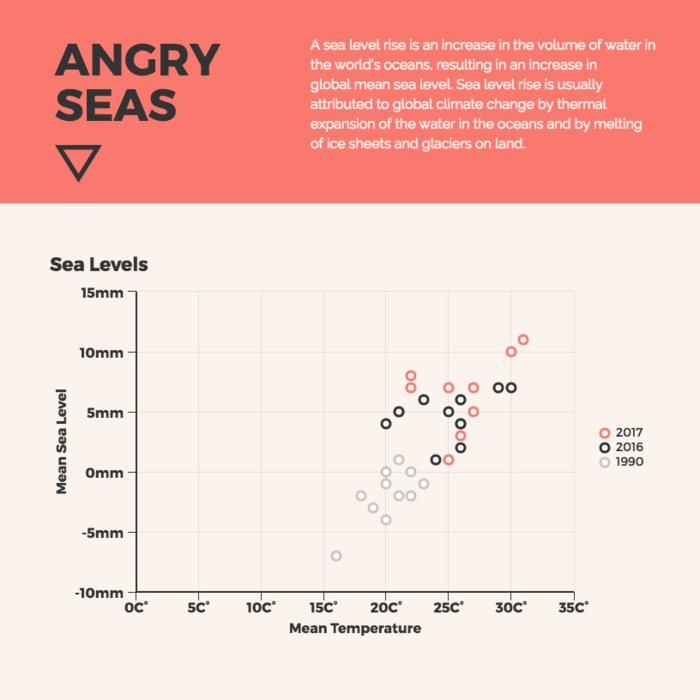
5. Histogram
Best for visualizing the distribution and frequency of a single variable.
Histograms are your choice when you want to understand the distribution and frequency of a single variable.
They divide the data into “bins” or intervals and the height of each bar represents the frequency or count of data points falling into that interval.
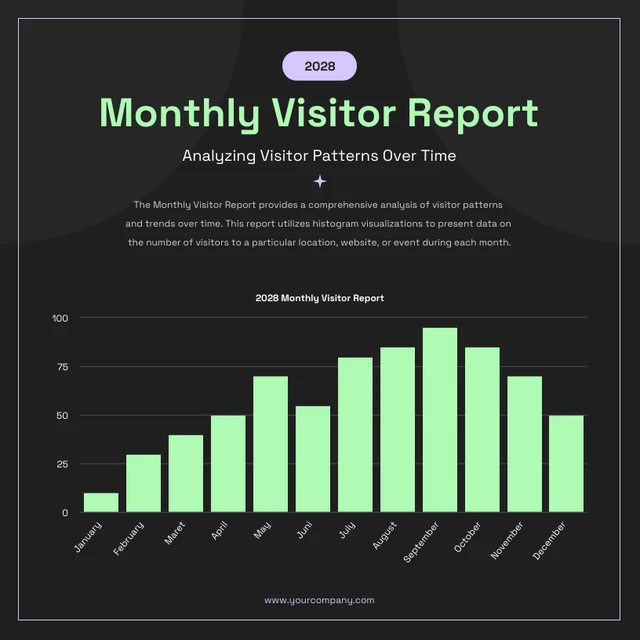
Histograms are excellent for helping to identify trends in data distributions, such as peaks, gaps or skewness.
Here’s something to take note of — ensure that your histogram bins are appropriately sized to capture meaningful data patterns. Using clear axis labels and titles can also help explain the distribution of the data effectively.
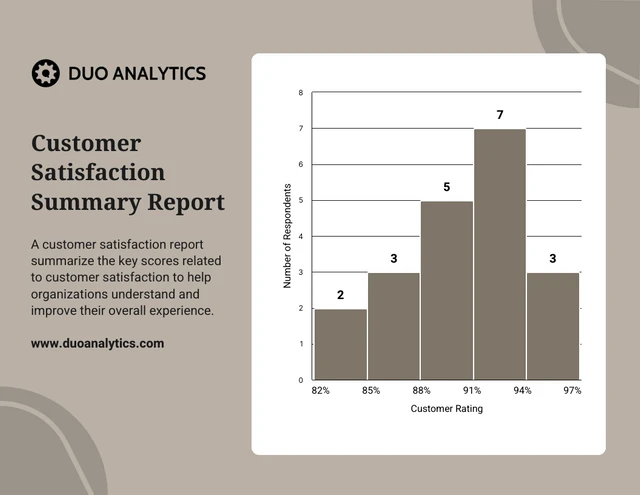
6. Stacked bar chart
Useful for showing how different components contribute to a whole over multiple categories.
Stacked bar charts are a handy choice when you want to illustrate how different components contribute to a whole across multiple categories.
Each bar represents a category and the bars are divided into segments to show the contribution of various components within each category.
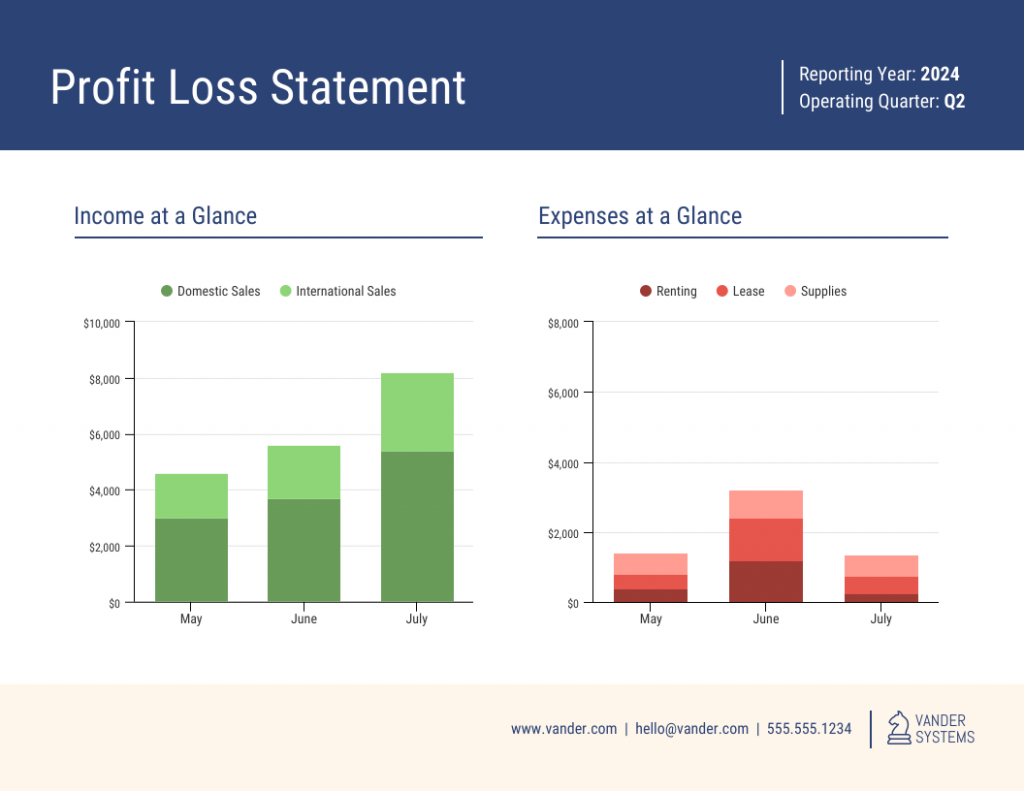
This method is ideal for highlighting both the individual and collective significance of each component, making it a valuable tool for comparative analysis.
Stacked bar charts are like data sandwiches—label each layer so people know what’s what. Keep the order logical and don’t forget the paintbrush for snazzy colors. Here’s a data analysis presentation example on writers’ productivity using stacked bar charts:
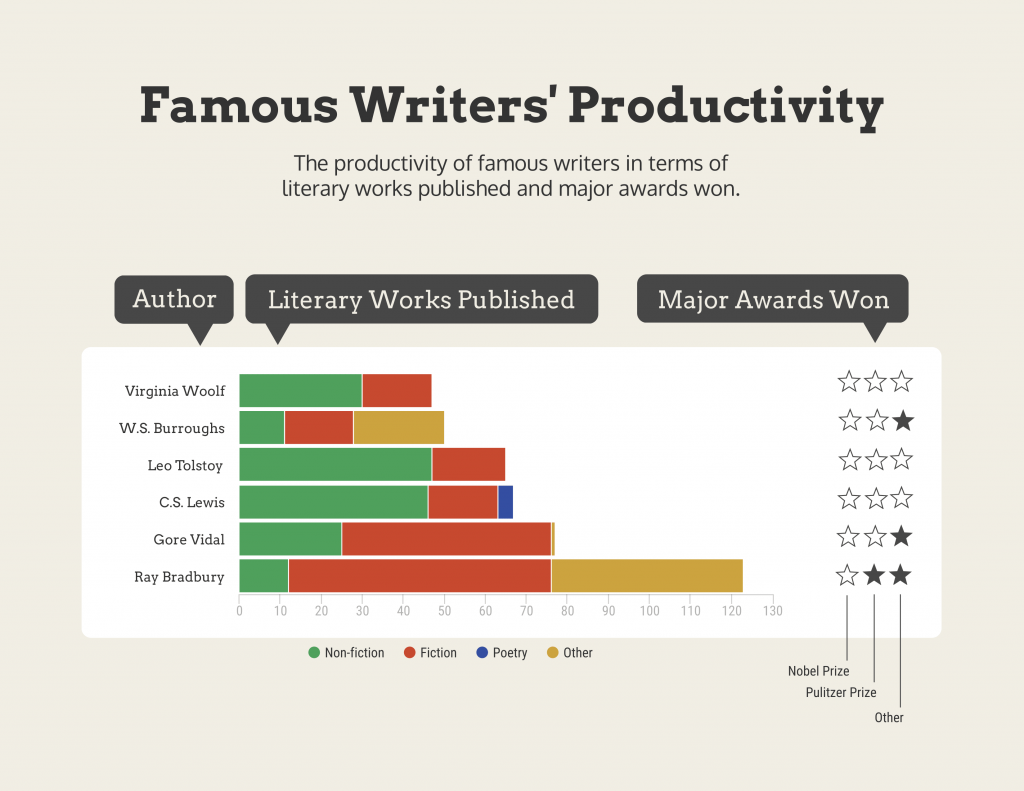
7. Area chart
Similar to line charts but with the area below the lines filled, making them suitable for showing cumulative data.
Area charts are close cousins of line charts but come with a twist.
Imagine plotting the sales of a product over several months. In an area chart, the space between the line and the x-axis is filled, providing a visual representation of the cumulative total.
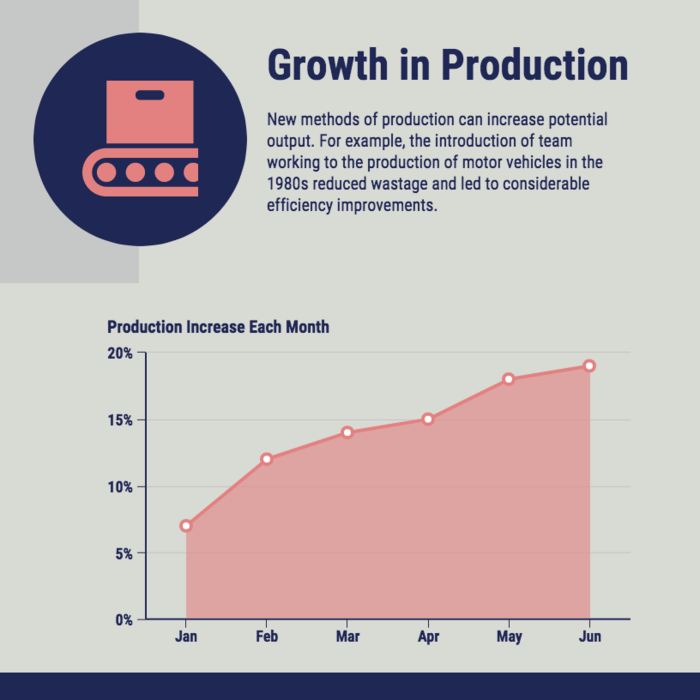
This makes it easy to see how values stack up over time, making area charts a valuable tool for tracking trends in data.
For area charts, use them to visualize cumulative data and trends, but avoid overcrowding the chart. Add labels, especially at significant points and make sure the area under the lines is filled with a visually appealing color gradient.
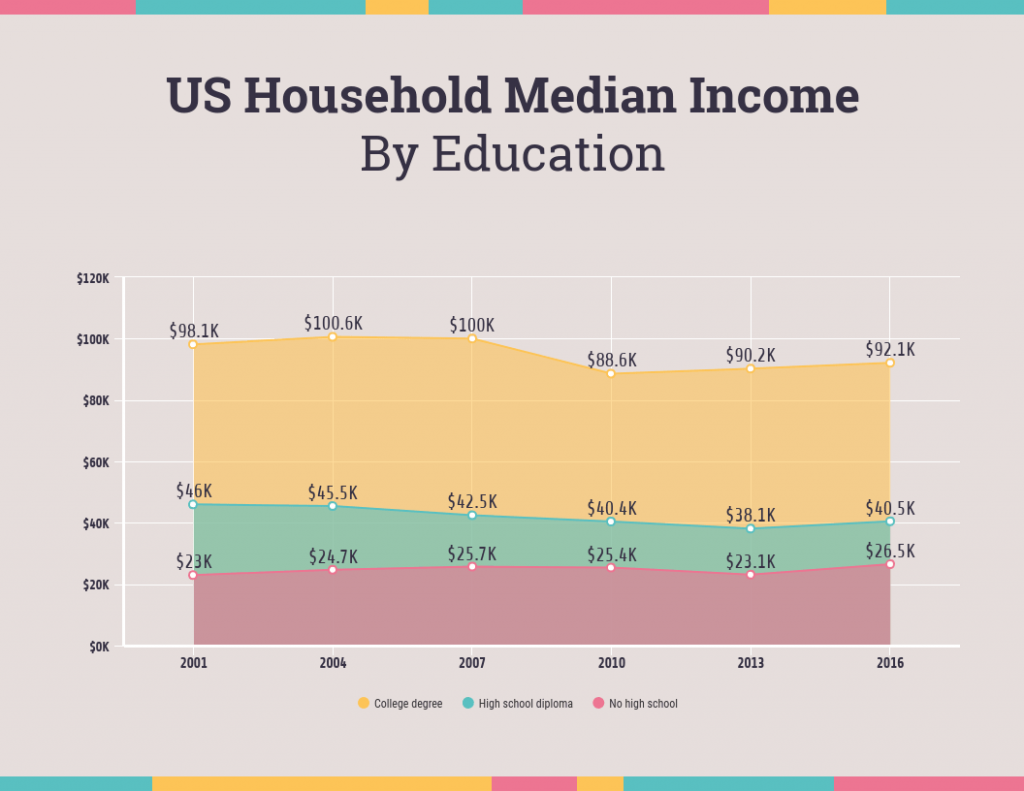
8. Tabular presentation
Presenting data in rows and columns, often used for precise data values and comparisons.
Tabular data presentation is all about clarity and precision. Think of it as presenting numerical data in a structured grid, with rows and columns clearly displaying individual data points.
A table is invaluable for showcasing detailed data, facilitating comparisons and presenting numerical information that needs to be exact. They’re commonly used in reports, spreadsheets and academic papers.
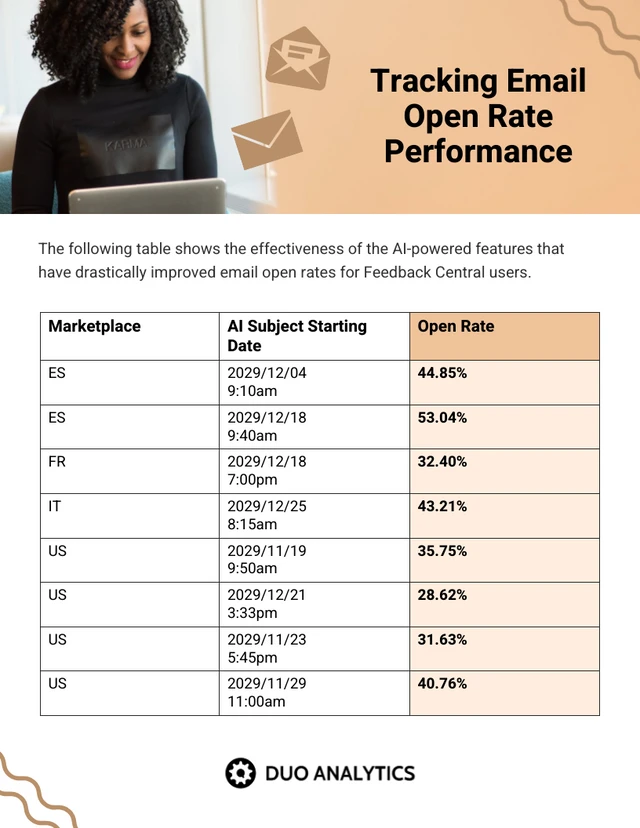
When presenting tabular data, organize it neatly with clear headers and appropriate column widths. Highlight important data points or patterns using shading or font formatting for better readability.
9. Textual data
Utilizing written or descriptive content to explain or complement data, such as annotations or explanatory text.
Textual data presentation may not involve charts or graphs, but it’s one of the most used qualitative data presentation examples.
It involves using written content to provide context, explanations or annotations alongside data visuals. Think of it as the narrative that guides your audience through the data.
Well-crafted textual data can make complex information more accessible and help your audience understand the significance of the numbers and visuals.
Textual data is your chance to tell a story. Break down complex information into bullet points or short paragraphs and use headings to guide the reader’s attention.
10. Pictogram
Using simple icons or images to represent data is especially useful for conveying information in a visually intuitive manner.
Pictograms are all about harnessing the power of images to convey data in an easy-to-understand way.
Instead of using numbers or complex graphs, you use simple icons or images to represent data points.
For instance, you could use a thumbs up emoji to illustrate customer satisfaction levels, where each face represents a different level of satisfaction.
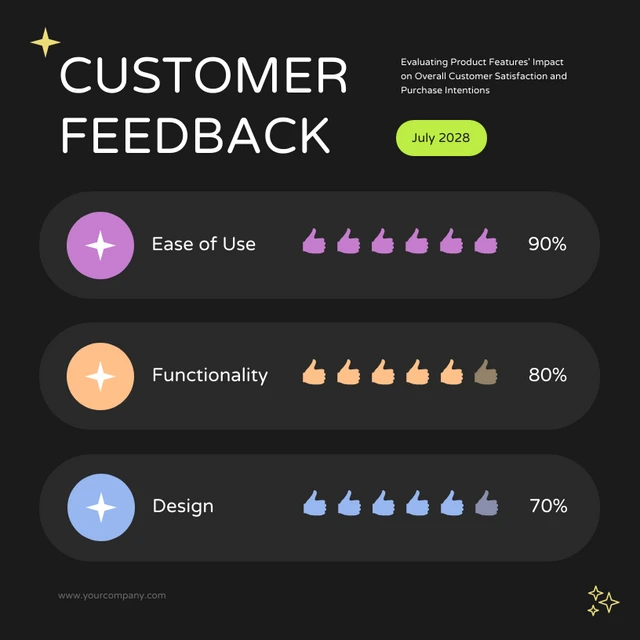
Pictograms are great for conveying data visually, so choose symbols that are easy to interpret and relevant to the data. Use consistent scaling and a legend to explain the symbols’ meanings, ensuring clarity in your presentation.
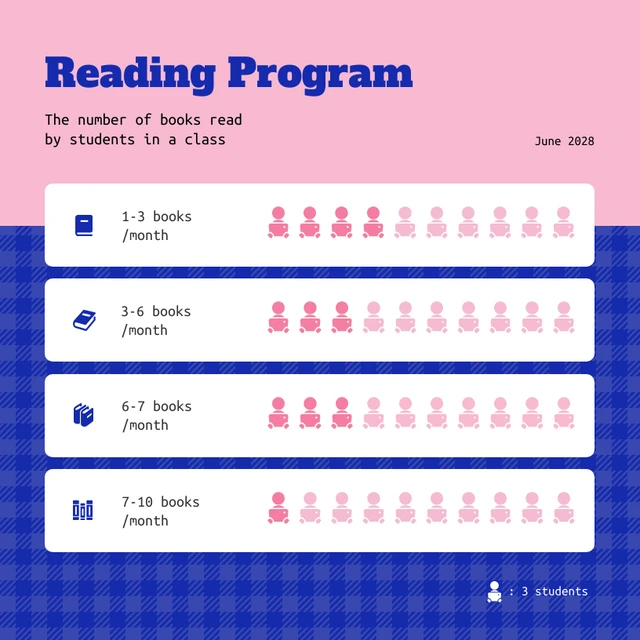
Looking for more data presentation ideas? Use the Venngage graph maker or browse through our gallery of chart templates to pick a template and get started!
A comprehensive data presentation should include several key elements to effectively convey information and insights to your audience. Here’s a list of what should be included in a data presentation:
1. Title and objective
- Begin with a clear and informative title that sets the context for your presentation.
- State the primary objective or purpose of the presentation to provide a clear focus.
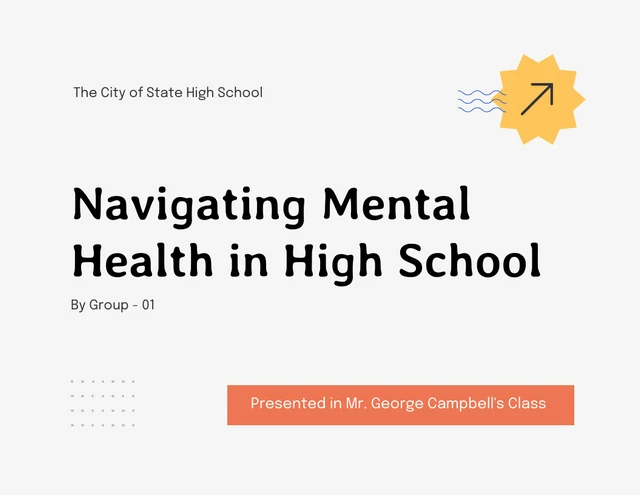
2. Key data points
- Present the most essential data points or findings that align with your objective.
- Use charts, graphical presentations or visuals to illustrate these key points for better comprehension.
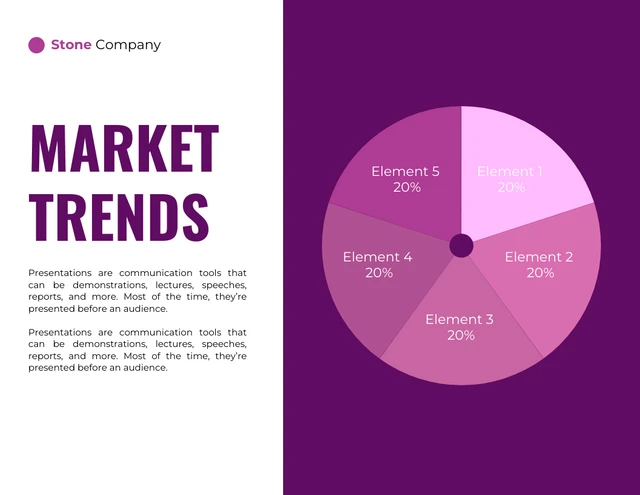
3. Context and significance
- Provide a brief overview of the context in which the data was collected and why it’s significant.
- Explain how the data relates to the larger picture or the problem you’re addressing.
4. Key takeaways
- Summarize the main insights or conclusions that can be drawn from the data.
- Highlight the key takeaways that the audience should remember.
5. Visuals and charts
- Use clear and appropriate visual aids to complement the data.
- Ensure that visuals are easy to understand and support your narrative.
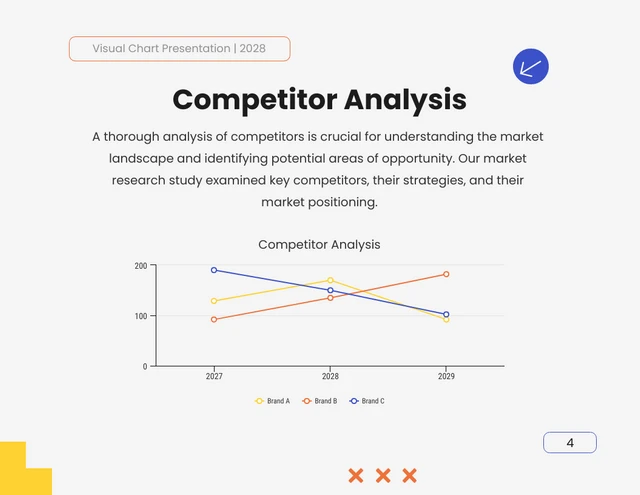
6. Implications or actions
- Discuss the practical implications of the data or any recommended actions.
- If applicable, outline next steps or decisions that should be taken based on the data.
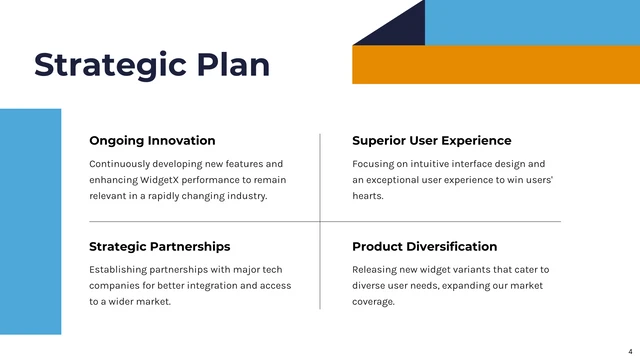
7. Q&A and discussion
- Allocate time for questions and open discussion to engage the audience.
- Address queries and provide additional insights or context as needed.
Presenting data is a crucial skill in various professional fields, from business to academia and beyond. To ensure your data presentations hit the mark, here are some common mistakes that you should steer clear of:
Overloading with data
Presenting too much data at once can overwhelm your audience. Focus on the key points and relevant information to keep the presentation concise and focused. Here are some free data visualization tools you can use to convey data in an engaging and impactful way.
Assuming everyone’s on the same page
It’s easy to assume that your audience understands as much about the topic as you do. But this can lead to either dumbing things down too much or diving into a bunch of jargon that leaves folks scratching their heads. Take a beat to figure out where your audience is coming from and tailor your presentation accordingly.
Misleading visuals
Using misleading visuals, such as distorted scales or inappropriate chart types can distort the data’s meaning. Pick the right data infographics and understandable charts to ensure that your visual representations accurately reflect the data.
Not providing context
Data without context is like a puzzle piece with no picture on it. Without proper context, data may be meaningless or misinterpreted. Explain the background, methodology and significance of the data.
Not citing sources properly
Neglecting to cite sources and provide citations for your data can erode its credibility. Always attribute data to its source and utilize reliable sources for your presentation.
Not telling a story
Avoid simply presenting numbers. If your presentation lacks a clear, engaging story that takes your audience on a journey from the beginning (setting the scene) through the middle (data analysis) to the end (the big insights and recommendations), you’re likely to lose their interest.
Infographics are great for storytelling because they mix cool visuals with short and sweet text to explain complicated stuff in a fun and easy way. Create one with Venngage’s free infographic maker to create a memorable story that your audience will remember.
Ignoring data quality
Presenting data without first checking its quality and accuracy can lead to misinformation. Validate and clean your data before presenting it.
Simplify your visuals
Fancy charts might look cool, but if they confuse people, what’s the point? Go for the simplest visual that gets your message across. Having a dilemma between presenting data with infographics v.s data design? This article on the difference between data design and infographics might help you out.
Missing the emotional connection
Data isn’t just about numbers; it’s about people and real-life situations. Don’t forget to sprinkle in some human touch, whether it’s through relatable stories, examples or showing how the data impacts real lives.
Skipping the actionable insights
At the end of the day, your audience wants to know what they should do with all the data. If you don’t wrap up with clear, actionable insights or recommendations, you’re leaving them hanging. Always finish up with practical takeaways and the next steps.
Can you provide some data presentation examples for business reports?
Business reports often benefit from data presentation through bar charts showing sales trends over time, pie charts displaying market share,or tables presenting financial performance metrics like revenue and profit margins.
What are some creative data presentation examples for academic presentations?
Creative data presentation ideas for academic presentations include using statistical infographics to illustrate research findings and statistical data, incorporating storytelling techniques to engage the audience or utilizing heat maps to visualize data patterns.
What are the key considerations when choosing the right data presentation format?
When choosing a chart format , consider factors like data complexity, audience expertise and the message you want to convey. Options include charts (e.g., bar, line, pie), tables, heat maps, data visualization infographics and interactive dashboards.
Knowing the type of data visualization that best serves your data is just half the battle. Here are some best practices for data visualization to make sure that the final output is optimized.
How can I choose the right data presentation method for my data?
To select the right data presentation method, start by defining your presentation’s purpose and audience. Then, match your data type (e.g., quantitative, qualitative) with suitable visualization techniques (e.g., histograms, word clouds) and choose an appropriate presentation format (e.g., slide deck, report, live demo).
For more presentation ideas , check out this guide on how to make a good presentation or use a presentation software to simplify the process.
How can I make my data presentations more engaging and informative?
To enhance data presentations, use compelling narratives, relatable examples and fun data infographics that simplify complex data. Encourage audience interaction, offer actionable insights and incorporate storytelling elements to engage and inform effectively.
The opening of your presentation holds immense power in setting the stage for your audience. To design a presentation and convey your data in an engaging and informative, try out Venngage’s free presentation maker to pick the right presentation design for your audience and topic.
What is the difference between data visualization and data presentation?
Data presentation typically involves conveying data reports and insights to an audience, often using visuals like charts and graphs. Data visualization , on the other hand, focuses on creating those visual representations of data to facilitate understanding and analysis.
Now that you’ve learned a thing or two about how to use these methods of data presentation to tell a compelling data story , it’s time to take these strategies and make them your own.
But here’s the deal: these aren’t just one-size-fits-all solutions. Remember that each example we’ve uncovered here is not a rigid template but a source of inspiration. It’s all about making your audience go, “Wow, I get it now!”
Think of your data presentations as your canvas – it’s where you paint your story, convey meaningful insights and make real change happen.
So, go forth, present your data with confidence and purpose and watch as your strategic influence grows, one compelling presentation at a time.
Discover popular designs
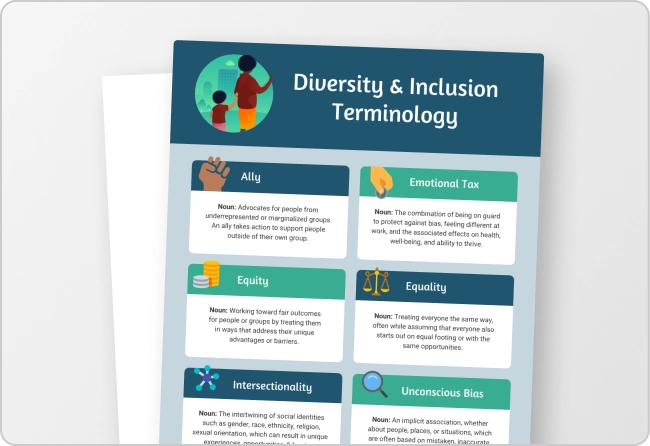
Infographic maker
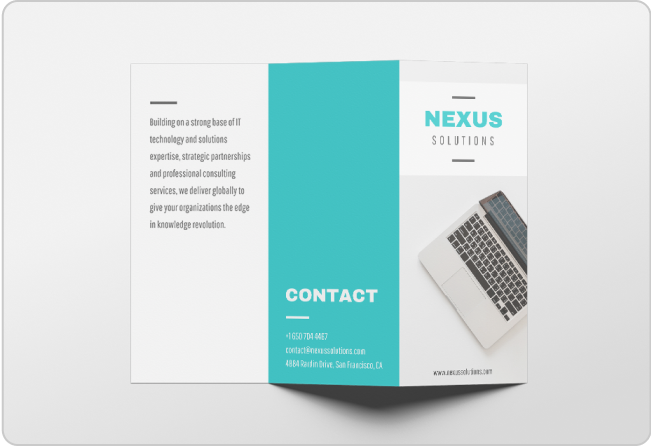
Brochure maker
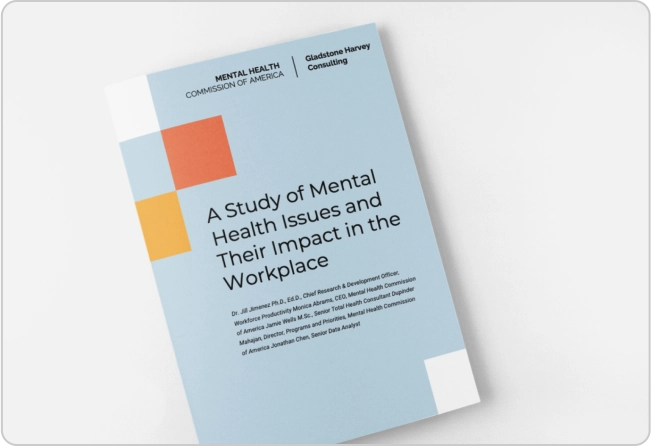
White paper online
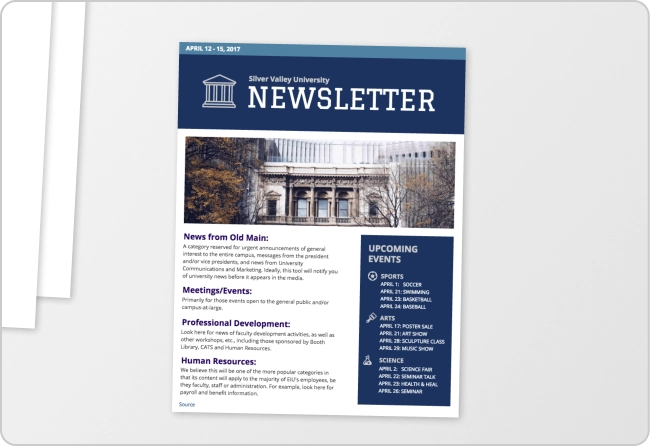
Newsletter creator
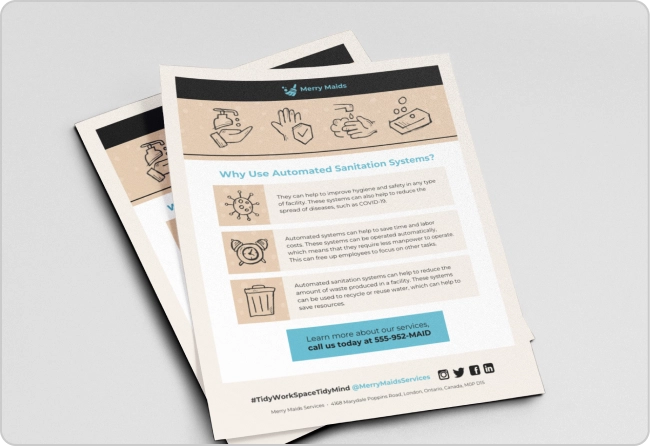
Flyer maker
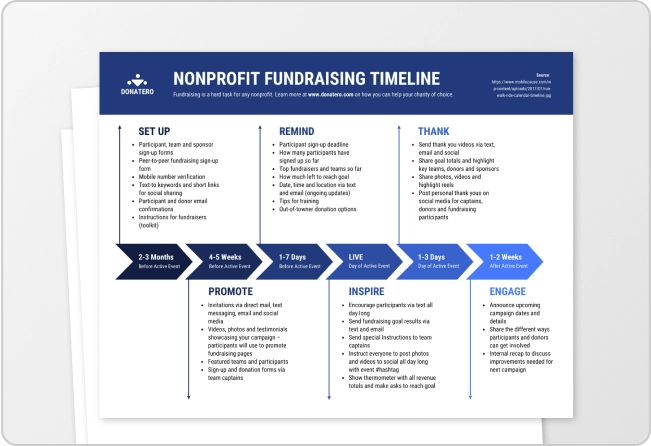
Timeline maker
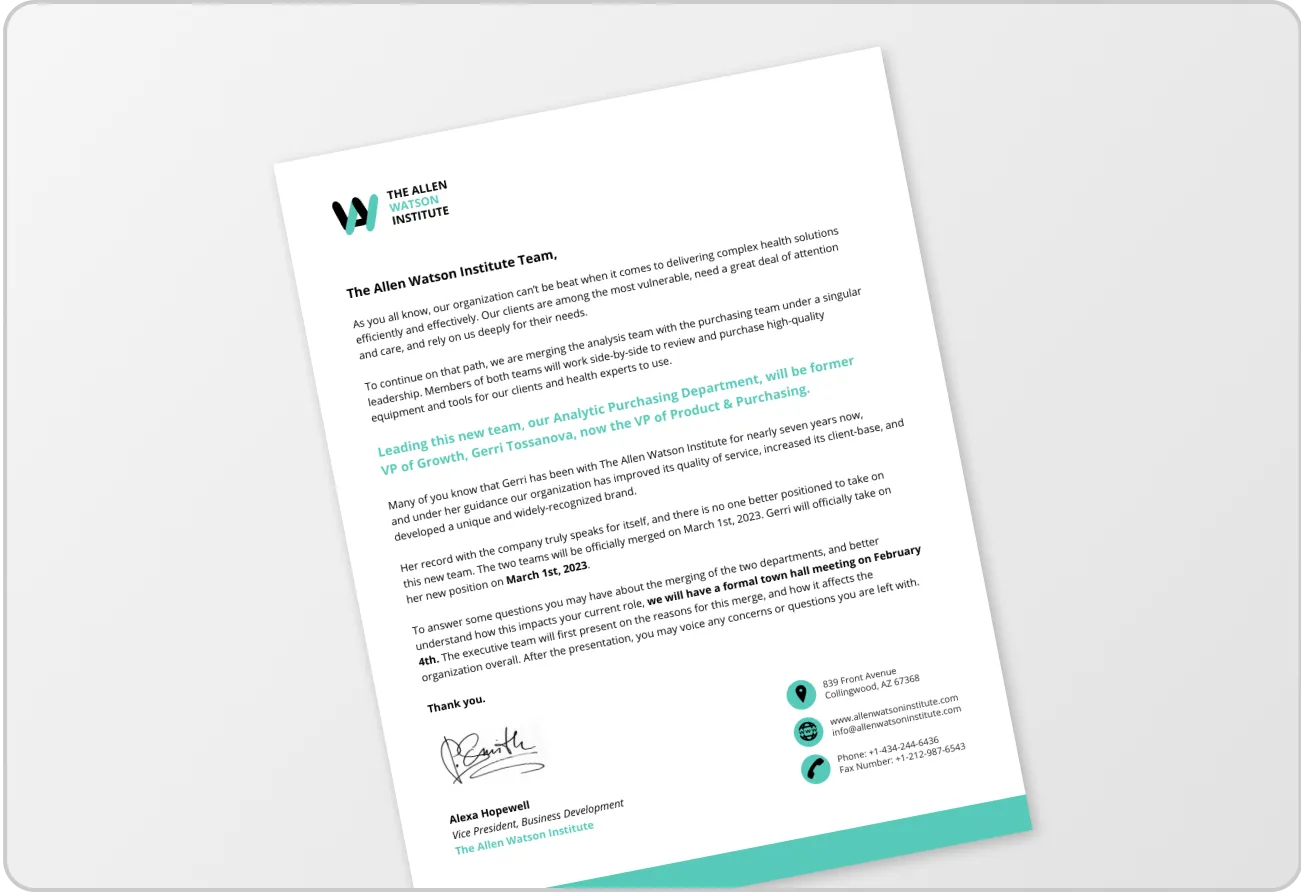
Letterhead maker
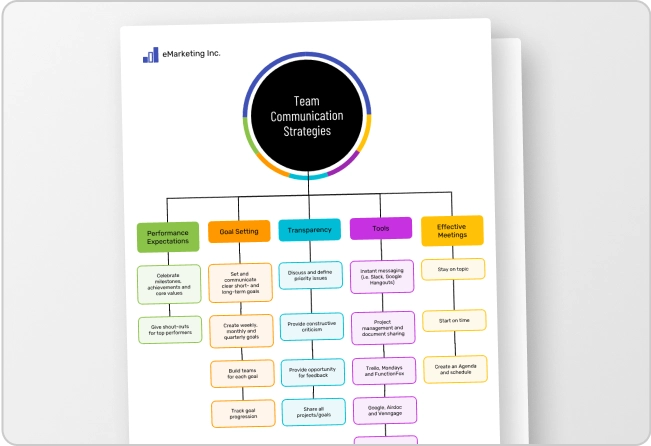
Mind map maker
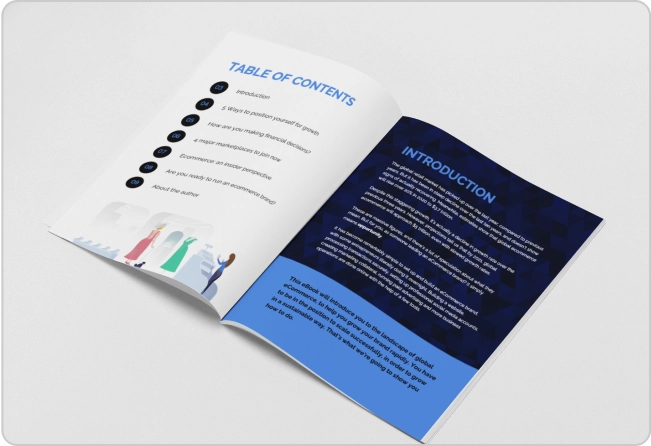
Ebook maker
Mastering the Art of Presenting Data in PowerPoint
Presenting data in PowerPoint is easy. However, making it visually appealing and effective takes more time and effort. It’s not hard to bore your audience with the same old data presentation formats. So, there is one simple golden rule: Make it not boring.
When used correctly, data can add weight, authority, and punch to your message. It should support and highlight your ideas, making a concept come to life. But this begs the question: How to present data in PowerPoint?
After talking to our 200+ expert presentation designers, I compiled information about their best-kept secrets to presenting data in PowerPoint.
Below, I’ll show our designers ' favorite ways to add data visualization for global customers and their expert tips for making your data shine. Read ahead and master the art of data visualization in PowerPoint!

Feel free to explore sections to find what's most useful!
How to present data in PowePoint: a step-by-step guide
Creative ways to present data in powerpoint.
- Tips for data visualization
Seeking to optimize your presentations? – 24Slides designers have got you covered!
How you present your data can make or break your presentation. It can make it stand out and stick with your audience, or make it fall flat from the go.
It’s not enough to just copy and paste your data into a presentation slide. Luckily, PowerPoint has many smart data visualization tools! You only need to put in your numbers, and PowerPoint will work it up for you.
Follow these steps, and I guarantee your presentations will level up!
1. Collect your data
First things first, and that is to have all your information ready. Especially for long business presentations, there can be a lot of information to consider when working on your slides. Having it all organized and ready to use will make the whole process much easier to go through.
Consider where your data comes from, whether from research, surveys, or databases. Make sure your data is accurate, up-to-date, and relevant to your presentation topic.
Your goal will be to create clear conclusions based on your data and highlight trends.
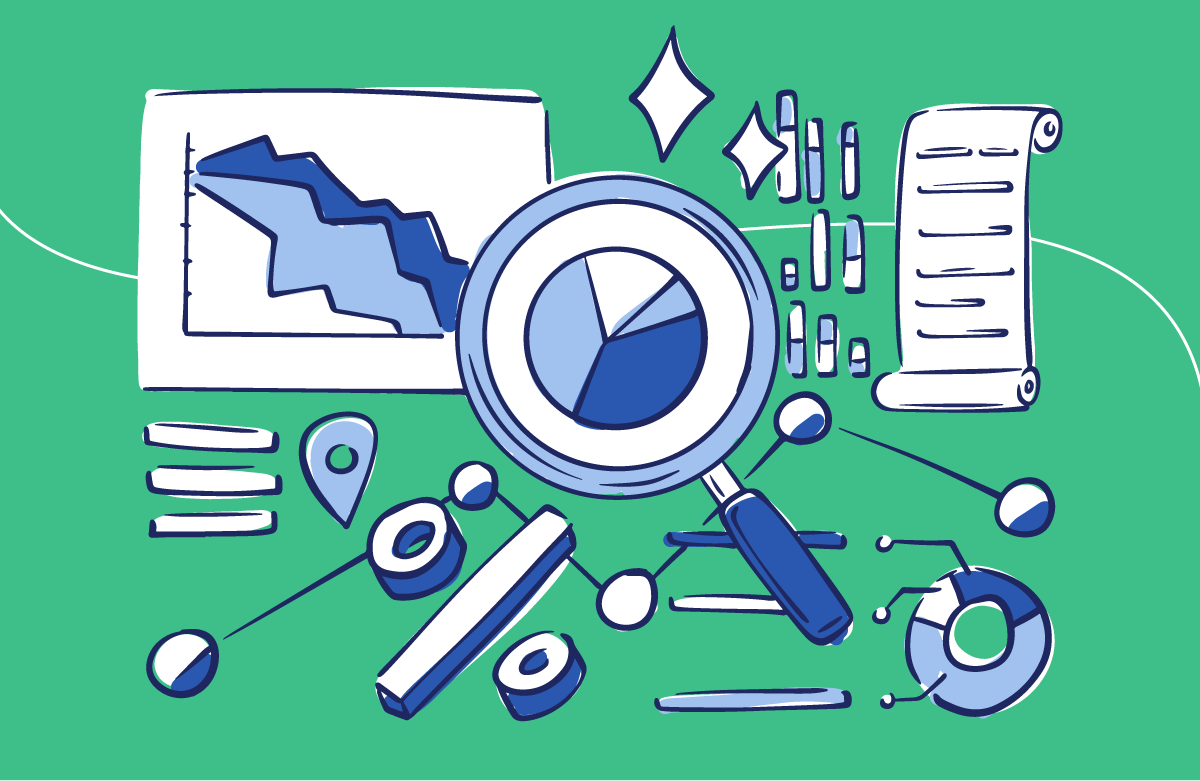
2. Know your audience
Knowing who your audience is and the one thing you want them to get from your data is vital. If you don’t have any idea where to start, you can begin with these key questions:
- What impact do you want your data to make on them?
- Is the subject of your presentation familiar to them?
- Are they fellow sales professionals?
- Are they interested in the relationships in the data you’re presenting?
By answering these, you'll be able to clearly understand the purpose of your data. As a storyteller, you want to capture your audience’s attention.
3. Choose a data visualization option
One key to data visualization in PowerPoint is being aware of your choices and picking the best one for your needs. This depends on the type of data you’re trying to showcase and your story.
When showcasing growth over time, you won’t use a spider chart but a line chart. If you show percentages, a circle graph will probably work better than a timeline. As you can see, knowing how to work with charts, graphs, and tables can level up your presentation.
Later, we’ll review some of the most common tools for data visualization in PowerPoint. This will include what these graphs and charts are best for and how to make the most of each. So read ahead for more information about how to present data in PowerPoint!
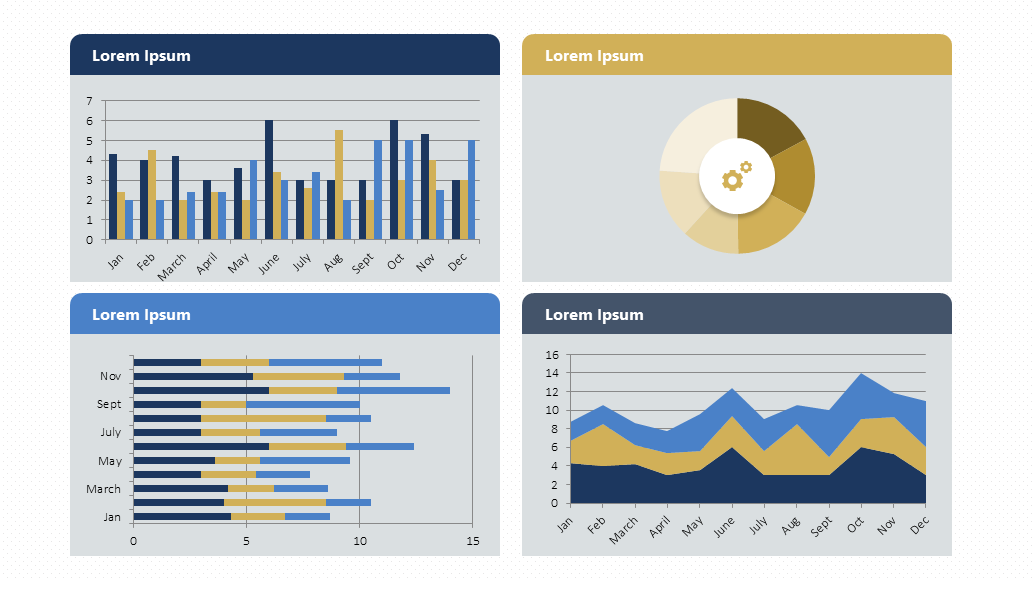
4. Be creative!
PowerPoint can assist with creating graphs and charts, but it's up to you to perfect them. Take into account that PowerPoint has many options. So, don't be afraid to think outside the box when presenting your data.
To enhance your presentation design, try out different color schemes, fonts, and layouts. Add images, icons, and visual elements to highlight your ideas.
If this sounds complicated to you, there's no need to worry. At the end of this article, you’ll find some easy tips for upgrading your data visualization design!
At this point, you might wonder: what is the best way to present data in PowerPoint? Well, let me tell you: it's all about charts. To accomplish a polished presentation, you must use charts instead of words. When visualizing quantitative data, a picture is worth a thousand words.
Based on +10 years of expertise, we've identified key chart types and creative ways to work with them. Let's delve into each one!
Line Charts
Line charts are a classic, which can make them boring. However, if done correctly, they can be striking and effective. But where does their popularity come from? Here's the answer: Line charts work great to show changes over time.
Another critical difference is that line charts are accumulative. For example, you can join them to a column chart to show different data at a glance. They allow data visualization effectively, making it easier to figure out.
To make the most of them, mastering how to work with line charts is essential. But there is good news: you will have a lot of freedom to customize them!
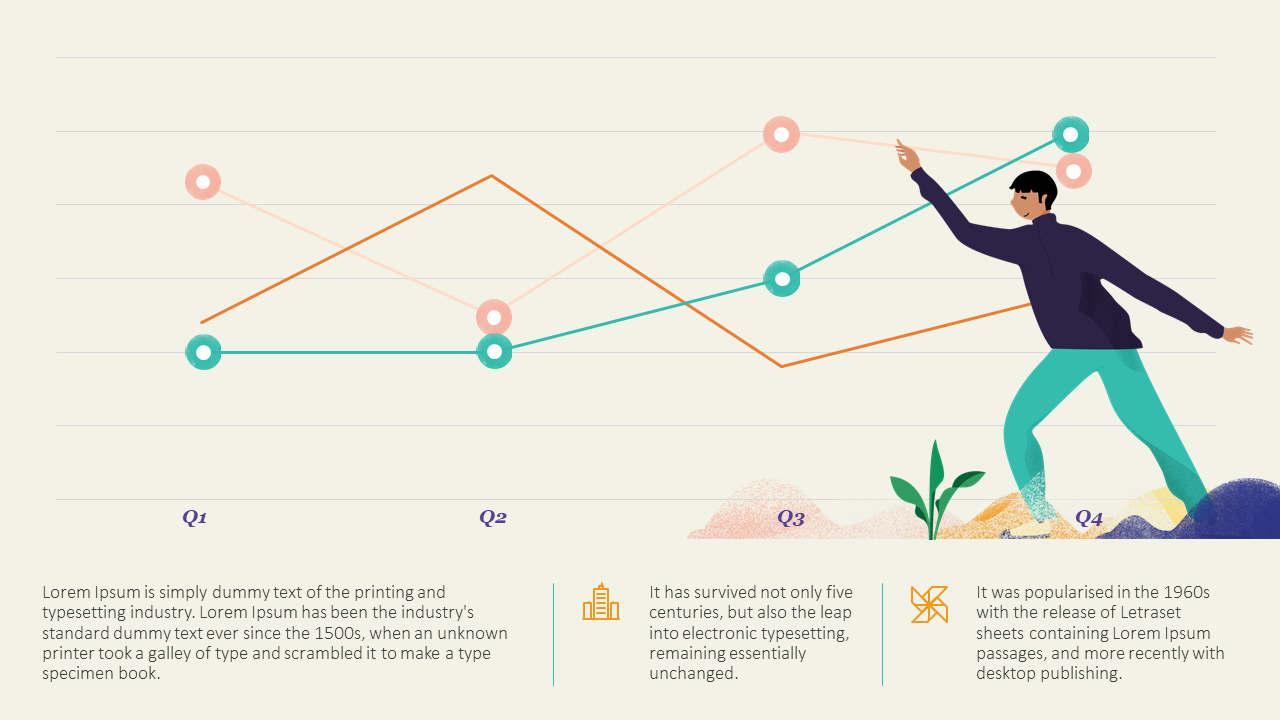
Download our Free Line Chart Template here .
Bar and column charts
Bar and column charts are another classic choice. Again, they are simple and great for comparing different categories. They organize them around two axes: one shows numbers, and the other shows what we want to compare.
But when should you use a bar chart or a column chart? A bar chart is better when comparing different categories and having long labels. A column chart, on the other hand, is better if you have a few categories and want to show changes over time.
You also have the waterfall option, which is perfect for highlighting the difference between gains and losses. It also adds a dynamic touch to your presentation!
Unsure how to implement these charts? Here's how to add a bar or a column chart in PowerPoint.
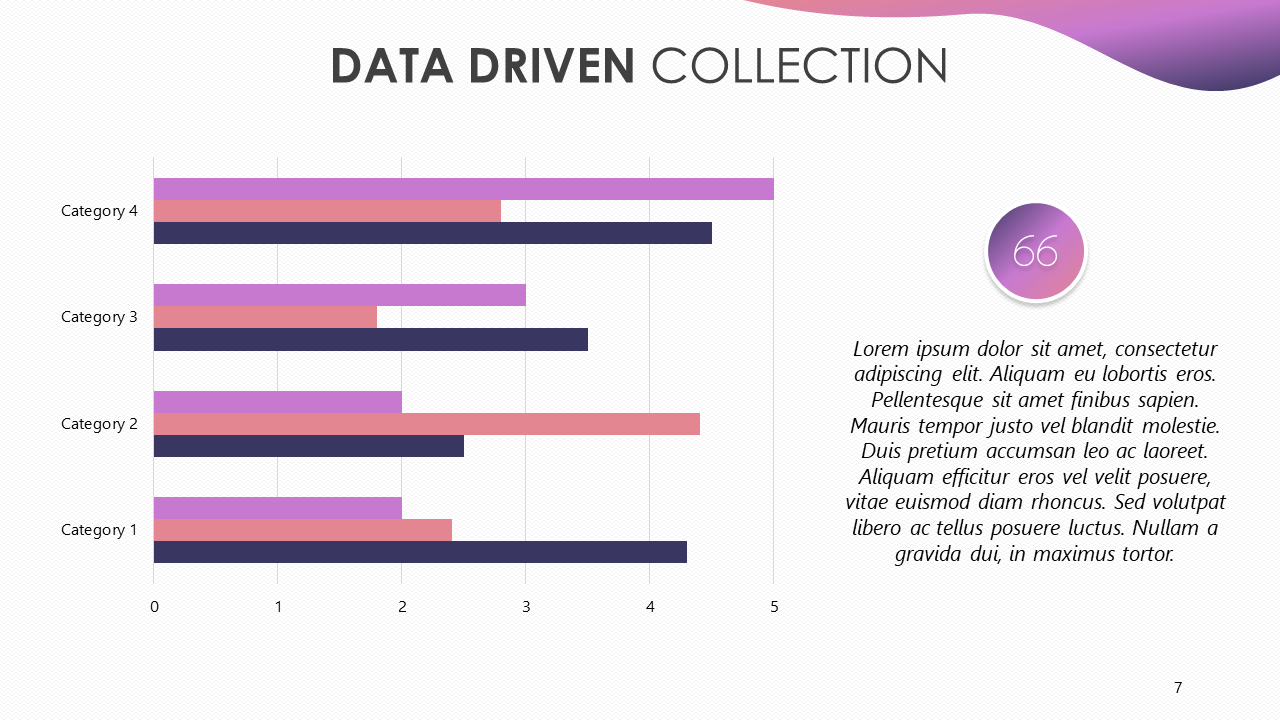
Download our Bar and Column Chart Template here .
Venn diagram
Venn diagrams are definitely something to consider when discussing data visualization—even if its focus is not quantitative data! Venn diagrams are best for showcasing similarities and differences between two (or more) categories or products.
By using overlapping circles, you can quickly and easily see common features between separate ideas. The shared space of the circles shows what is the same between the groups. However, items in the outer parts of each circle show what isn’t a common trait.
They make complex relationships easy to understand. Now, you only need to know how to create a Venn diagram in PowerPoint —quite simple!
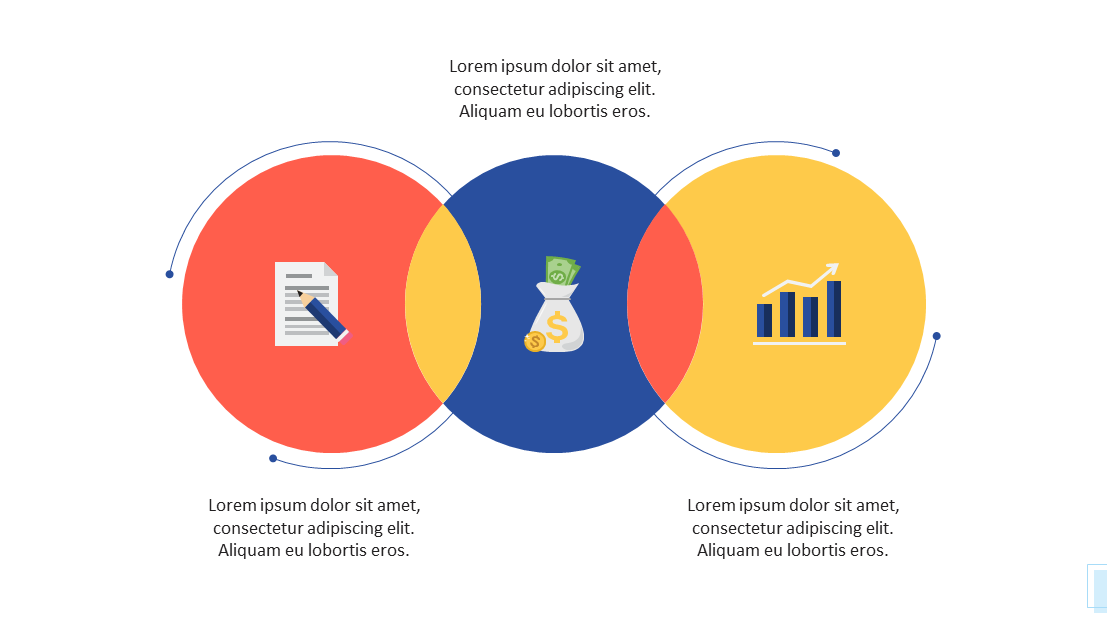
Download our Free Venn Diagram Template here .
Pie charts are a great way to show different percentages of a whole. They immediately identify the largest and smallest values. This means that they are great options for drawing attention to differences between one group and another.
However, many people misuse pie charts by overpacking them. As a rule, keep the chart to six or fewer sections. That way, the data is striking, not confusing. Then, make the pie chart your own with small, individual details and designs.
Once again, the powerful presentation of data is in simplicity.
Are you considering incorporating it into your presentation? Here’s how to easily add a pie chart in PowerPoint.
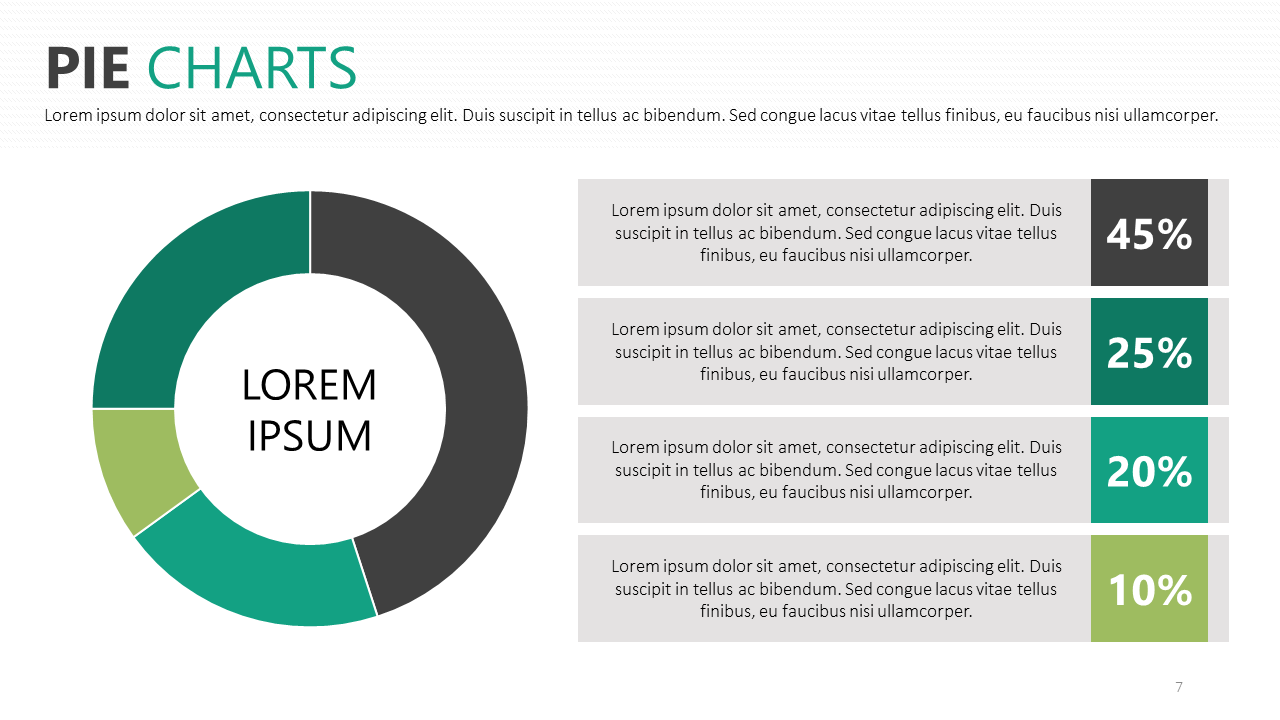
Download our Free Pie Chart Template here .
Bubble Charts
Bubble charts playfully present data in an incredibly visual way. But, what makes them so unique? It's easy: they show different values through varying circle sizes.
Squeezed together, the circles also show a holistic viewpoint. Bigger bubbles catch the eye, while small bubbles illustrate how the data breaks down into smaller values. ¿The result? A presentation of data in a visual form.
It can be one of the most graphic ways to represent the spending distribution. For example, you can instantly see your biggest costs or notice how important finances are getting lost in a sea of bubbles. This quick analysis can be incredibly handy.
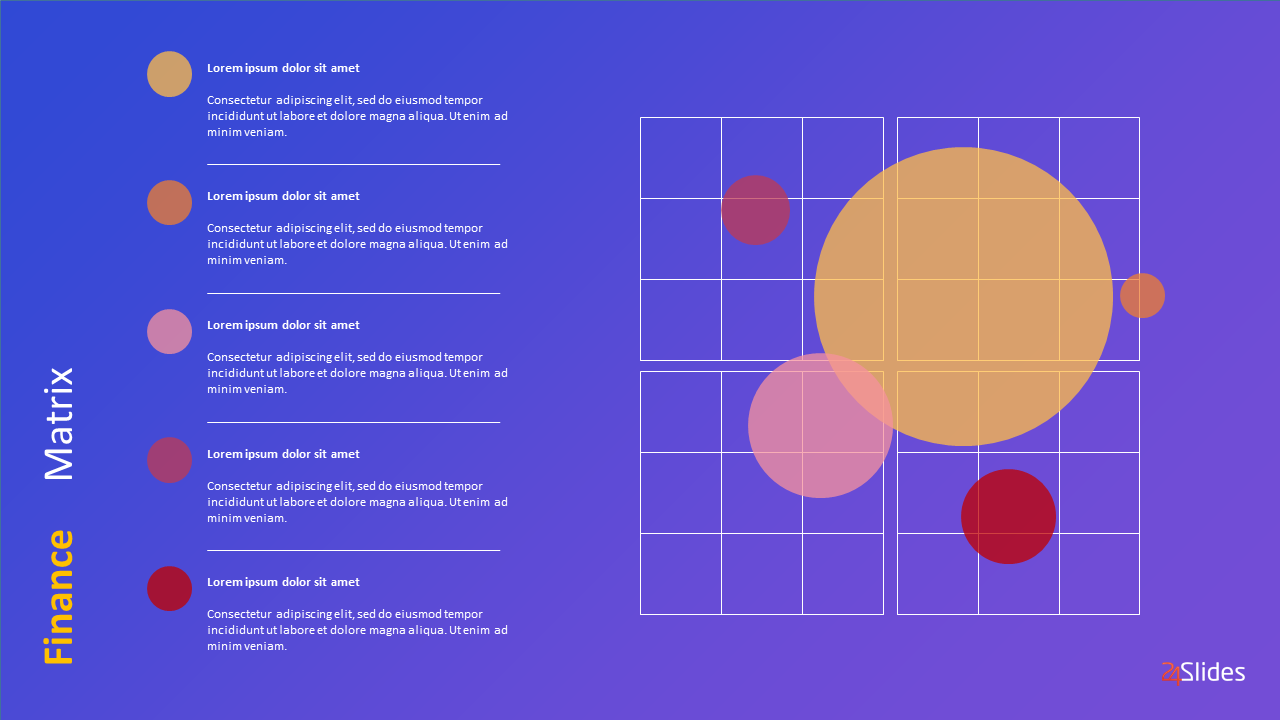
Download our Free Bubble Chart Template here .
Maps are the go-to solution for presenting geographic information . They help put data in a real-world context. You usually take a blank map and use color for the important areas.
Blocks, circles, or shading represent value. Knowing where certain data is can be crucial. A consistent color scheme makes it easy to show how valuable each section is.
They also work great when paired with other forms of data visualization. For example, you can use pie charts to provide information about offices in different cities around the world or bar charts to compare revenue in different locations.
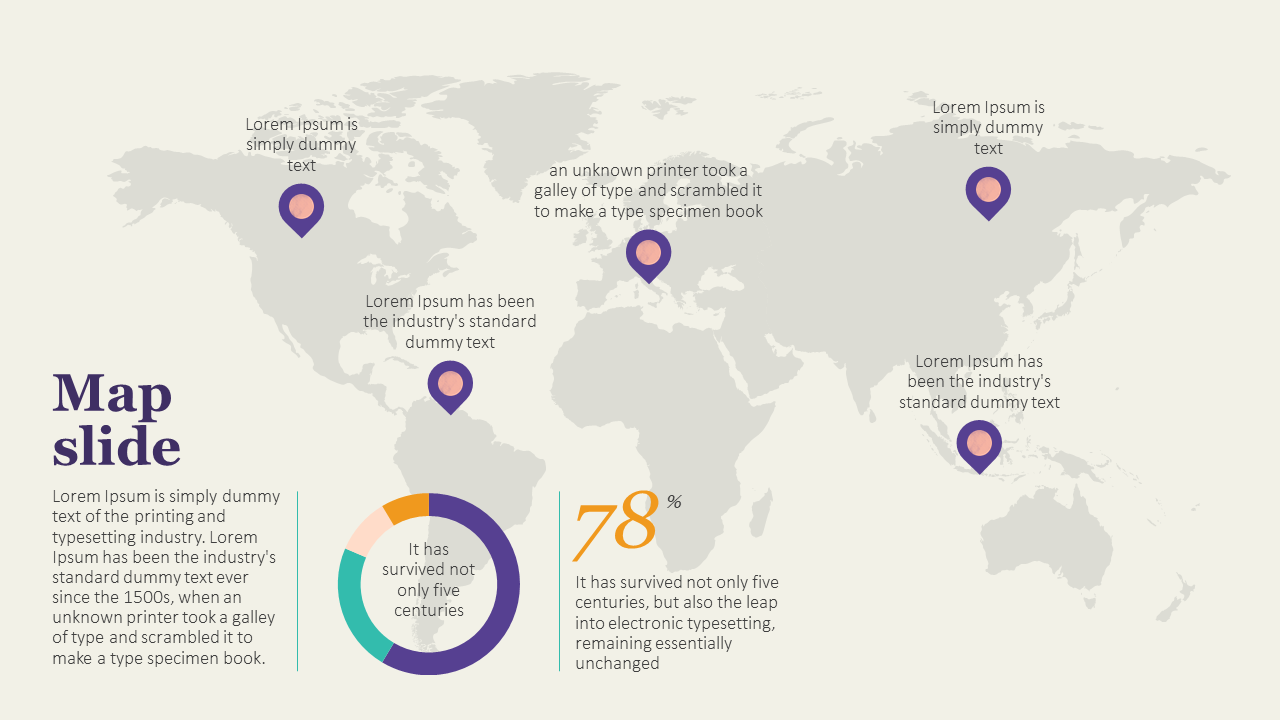
Download our Free World Map Template here .
If you want to display chronological data, you must use a timeline. It’s the most effective and space-efficient way to show time passage.
They make it easy for your audience to understand the sequence of events with clear and concise visuals.
You can use timelines to show your company’s history or significant events that impacted your business. Like maps, you can easily mix them with other types of data visuals. This characteristic allows you to create engaging presentations that tell a comprehensive story.
At this point, it's a matter of understanding how to add a timeline correctly in PowerPoint . Spoiler: it's incredibly easy.
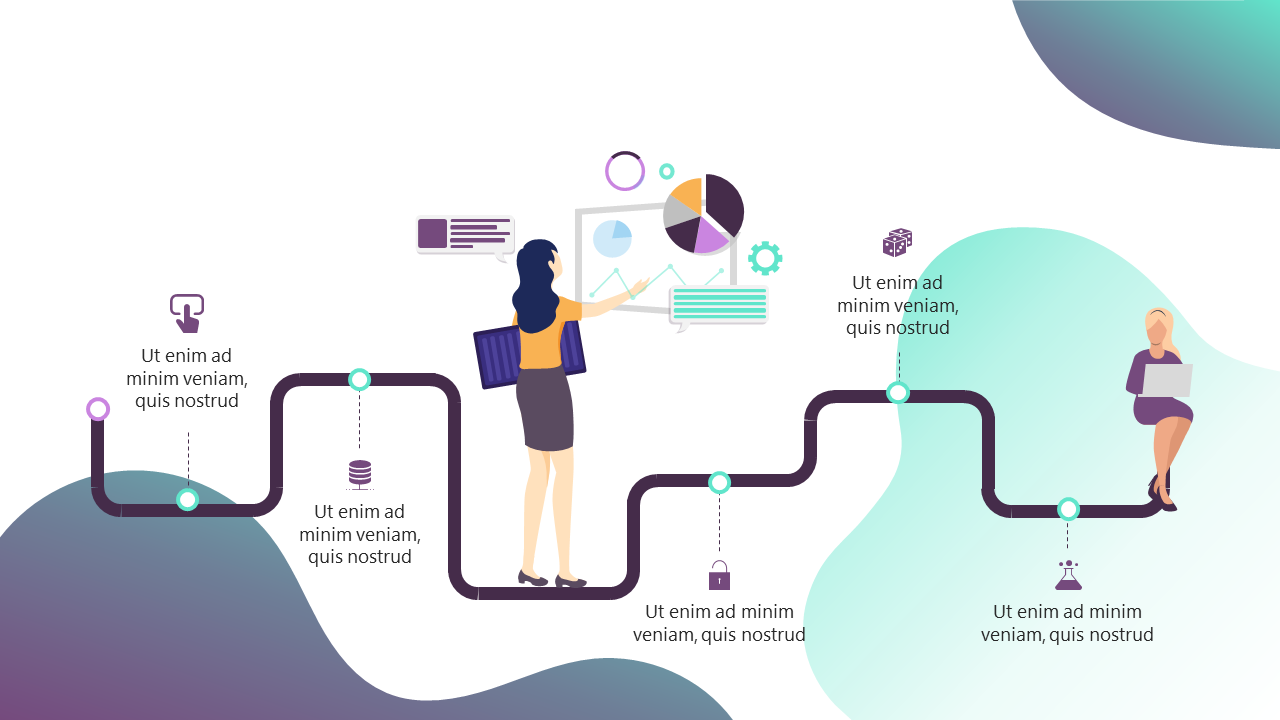
Download our Free Timeline Chart Template here .
Flowcharts, like timelines, represent a succession of events. The main difference is that timelines have determined start and finish points and specific dates. Flowcharts, on the other hand, show the passing from one step to the next.
They are great for showing processes and info that need to be in a specific order. They can also help you communicate cause-and-effect information in a visually engaging way.
Their best feature is that (unlike timelines) they can also be circular, meaning this is a recurrent process. All you need now is to become familiar with creating a flowchart in PowerPoint .
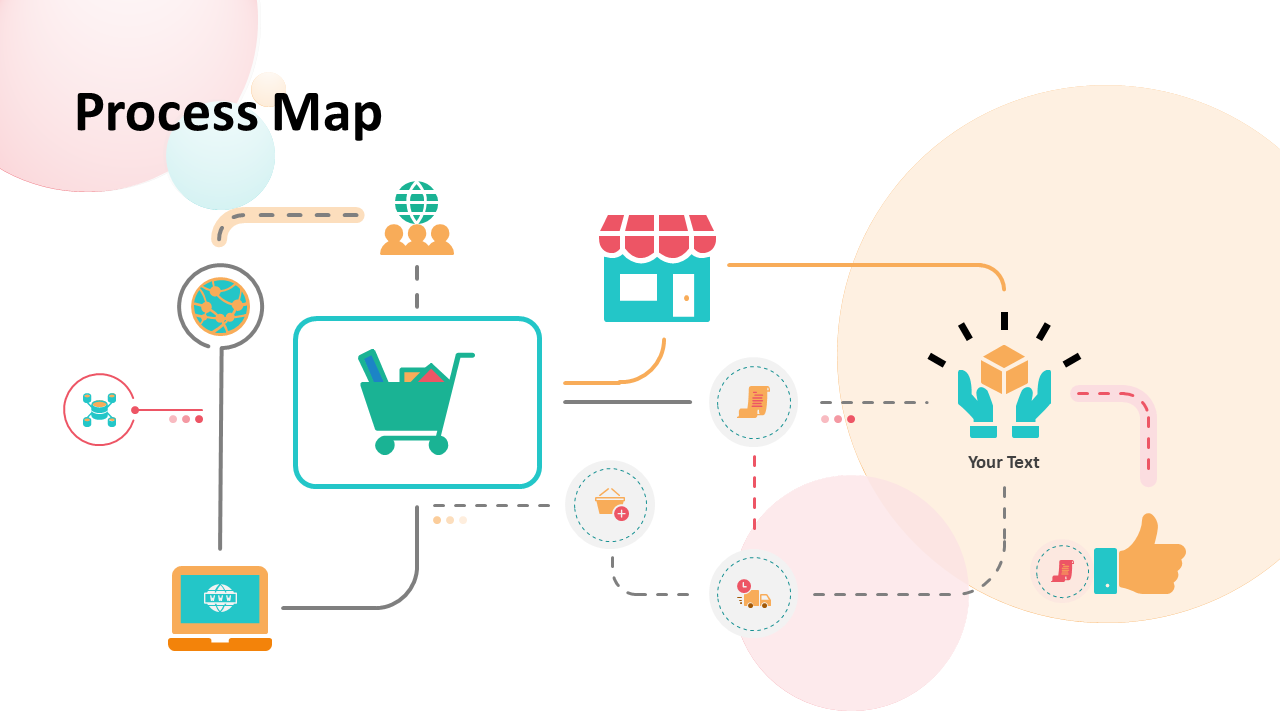
Download our Free Flowchart Template here .
5 Tips for data visualization in PowerPoint
Knowing how to present data in PowerPoint presentations is not hard, but it takes time to master it. After all, practice makes perfect!
I've gathered insights from our 200+ expert designers , and here are the top five tips they suggest for enhancing your data presentations!
1. Keep it simple
Don’t overload your audience with information. Let the data speak for itself. If you write text below a chart, keep it minimalist and highlight the key figures. The important thing in a presentation is displaying data in a clear and digestible way.
Put all the heavy facts and figures in a report, but never on a PowerPoint slide.
You can even avoid charts altogether to keep it as simple as possible. And don't get me wrong. We've already covered that charts are the way to go for presenting data in PowerPoint, but there are a few exceptions.
This begs the question: when shouldn't you use charts in PowerPoint? The answer is quite short. If your data is simple or doesn't add much value to your presentation, you might want to skip using charts.
2. Be original
One of the best ways to make your data impactful is originality. Take time to think about how you could present information uniquely. Think of a whole new concept and play around with it. Even if it’s not yet perfect, people will appreciate the effort to be original.
Experiment with creative ways to present your data, adding storytelling techniques , unique design elements, or interactive features. This approach can make the data more appealing and captivating for your audience.
You can even mix up how to present data in PowerPoint. Instead of just one format, consider using two different types of data presentation on a single slide. For instance, try placing a bar chart on the left and a pie chart showcasing different data on the right.
3. Focus on your brand
Keeping your presentation on-brand can genuinely make you stand out from the crowd! Even if you just focus on your brand’s color scheme, it will make your presentation look more polished and professional.
Have fun experimenting with data visualization tools to ensure they match your company’s products and services. What makes you different from others?
Add your brand's style into your visualization to ensure brand consistency and recognition. Use colors, fonts, and logos aligned with your company's image.
You can even make a presentation that more subtly reflects your brand. Think of what values you want to associate with your company and how you can display these in your presentation design.
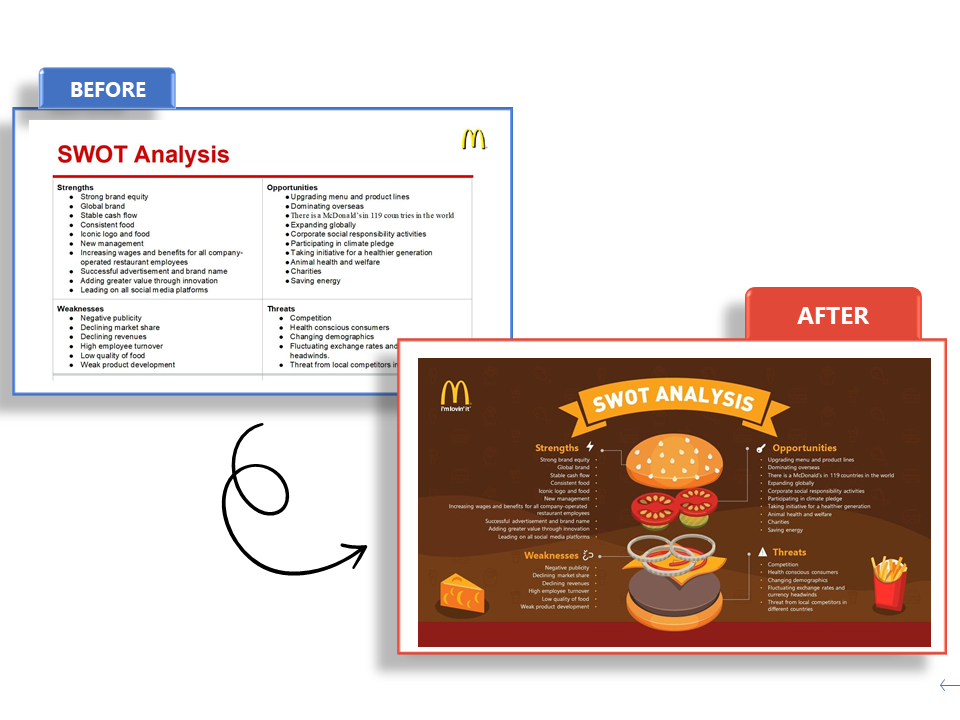
4. Highlight key information
Not distracting your audience nicely brings us to our third point: Highlight key information. Being detailed and informative is important, but grabbing and keeping the audience's attention is crucial.
Presenting numbers in PowerPoint can be difficult, but it doesn’t must be. Make your audience listen to the bigger message of your words, not just the exact details. All the smaller particulars can be confirmed later.
Your listeners don’t want to know the facts and figures to the nearest decimal. They want the whole number, which is easy to spot and understand.
The meaning of the number is more important than its numerical value. Is it high or low? Positive or negative? Good or bad for business? These are the questions to which you want the answers to be clear.
Using colors is an excellent way to work with this. Colors are also a great visual tool to showcase contrast. For example, when you're working on a graph to display your revenue, you can showcase expenses in red and earnings in green. This kind of color-coding will make your data visualization clear from first sight!
5. Use Templates!
Presentation templates can be your best friend when you want to present data effectively in PowerPoint.
They offer pre-designed layouts and styles that can ensure consistency throughout your presentation. Templates allow you to adjust colors, fonts, and layouts to match your branding or personal preferences.
Microsoft Office has its own library of templates, but you can also find some pretty amazing ones online. Take some extra time to search and pick one that truly fits your needs and brand.
¿The good news? Our Templates by 24Slides platform has hundreds of PowerPoint chart templates, all completely free for you to use . You can even download different templates and mix and match slides to make the perfect deck. All are entirely editable, so you can add your own data and forget about design.
If you liked the look of some examples in this article, you might be in luck! Most are part of these, and you can also find them on our Templates platform.
In this article, I've shown why knowing how to present data efficiently in PowerPoint is crucial. Data visualization tools are a must to ensure your message is clear and that it sticks with your audience.
However, achieving results that really stand out could be a huge challenge for beginners. So, If you want to save time and effort on the learning curve of presenting data in PowerPoint, you can always trust professionals!
With 10+ years of experience and more than 200 designers worldwide, we are the world’s largest presentation design company across the globe.
24Slides' professional PowerPoint designers work with businesses worldwide, helping them transform their presentations from ‘okay’ to ‘spectacular.’ With each presentation, we're crafting a powerful tool to captivate audiences and convey messages effectively!
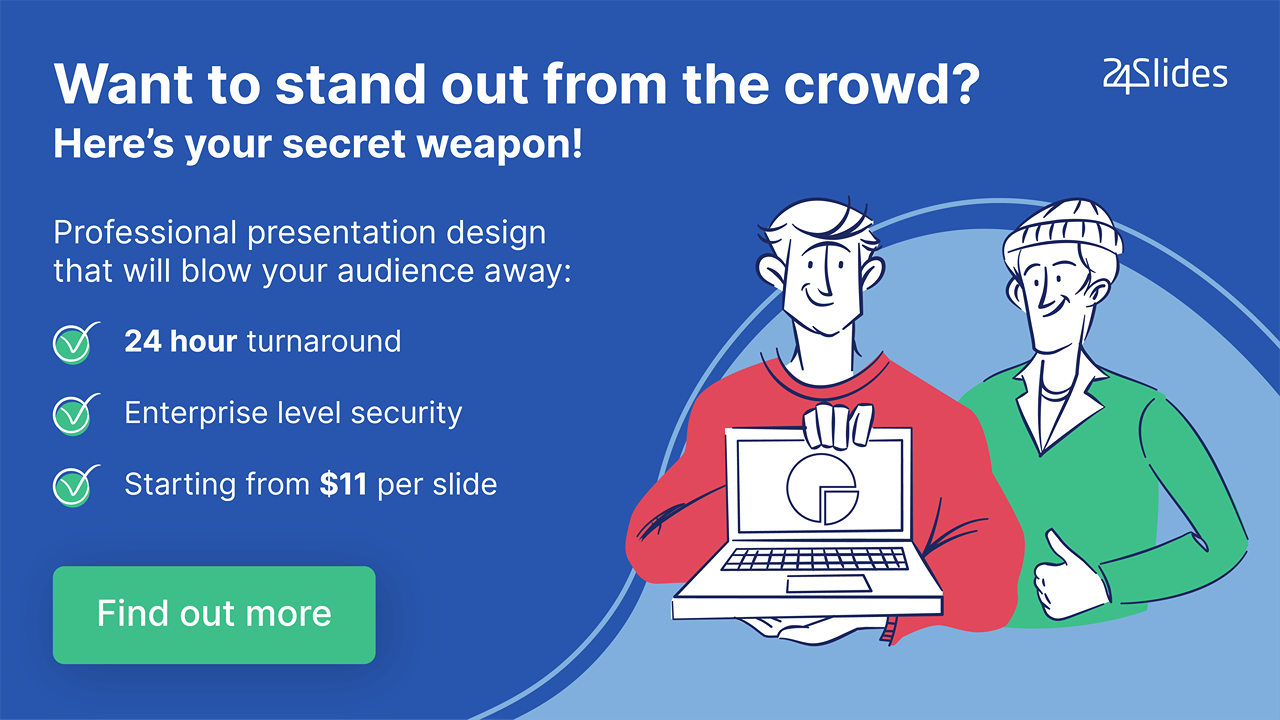
Looking to boost your PowerPoint game? Check out this content:
- PowerPoint 101: The Ultimate Guide for Beginners
- How to Create the Perfect B2B Sales Presentation
- The Ultimate Brand Identity Presentation Guide [FREE PPT Template]
- 7 Essential Storytelling Techniques for your Business Presentation
- The Cost of PowerPoint Presentations: Discover the hidden expenses you might overlook!
Create professional presentations online
Other people also read
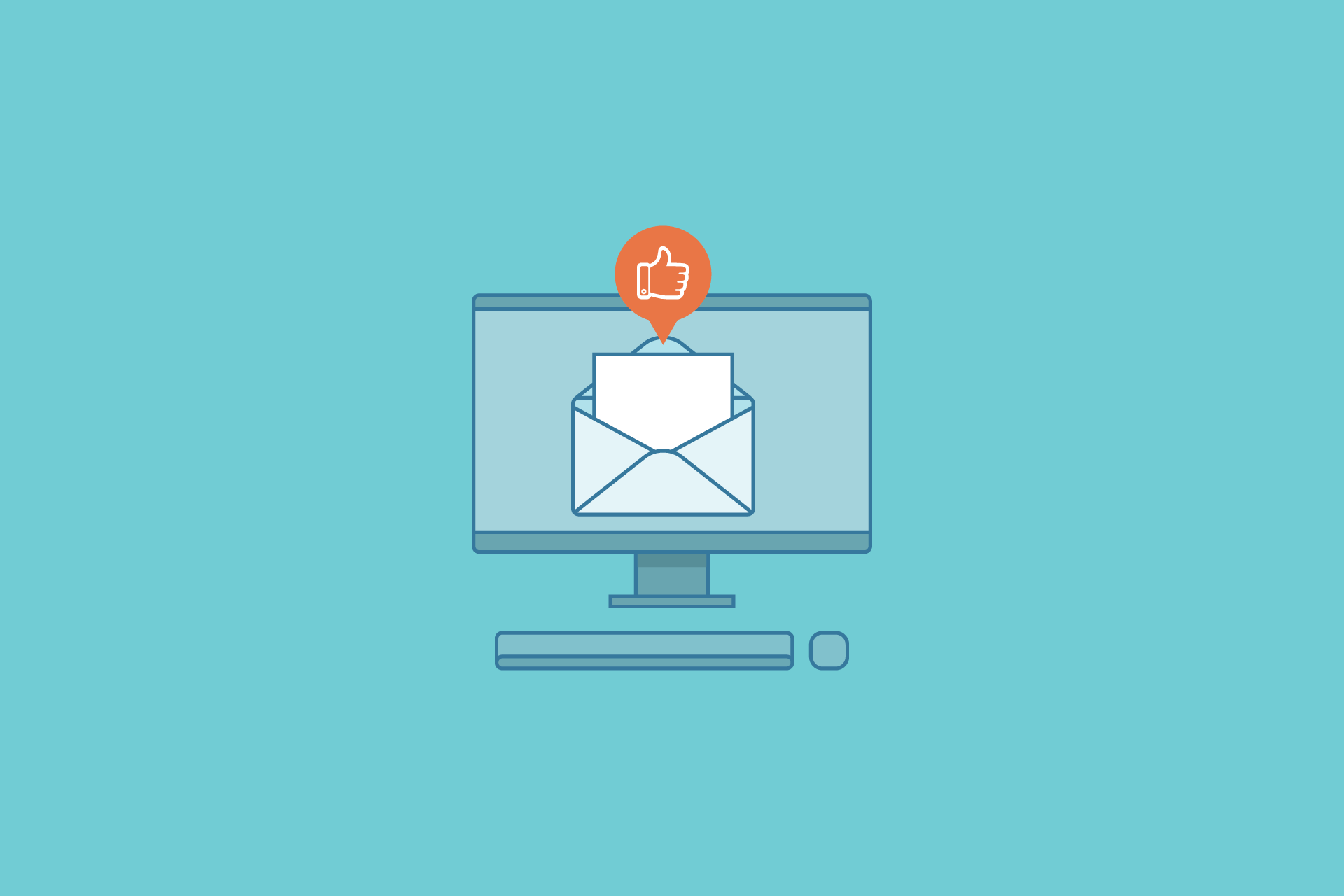
How To Write Effective Emails That Will Improve Your Communi...
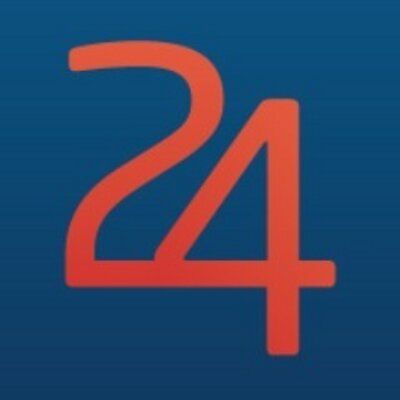
How to Make a Marketing Plan Presentation in PowerPoint
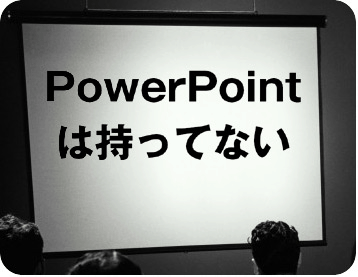
Alternative presentation styles: Takahashi
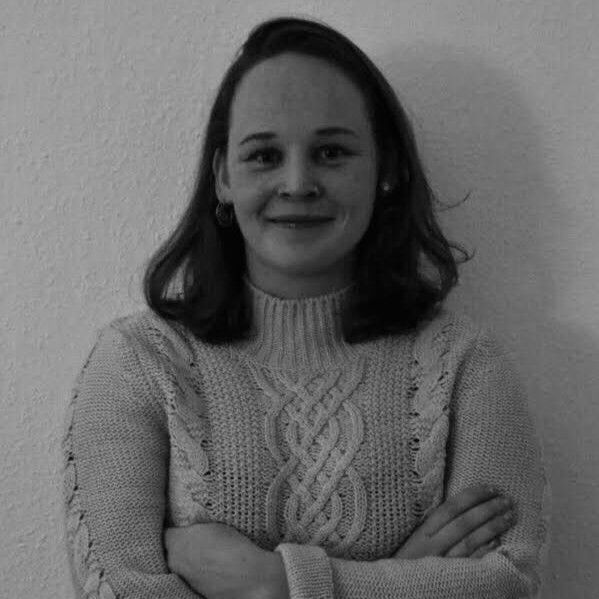
A Guide to Effective Data Presentation
Key objectives of data presentation, charts and graphs for great visuals, storytelling with data, visuals, and text, audiences and data presentation, the main idea in data presentation, storyboarding and data presentation, additional resources, data presentation.
Tools for effective data presentation
Financial analysts are required to present their findings in a neat, clear, and straightforward manner. They spend most of their time working with spreadsheets in MS Excel, building financial models , and crunching numbers. These models and calculations can be pretty extensive and complex and may only be understood by the analyst who created them. Effective data presentation skills are critical for being a world-class financial analyst .
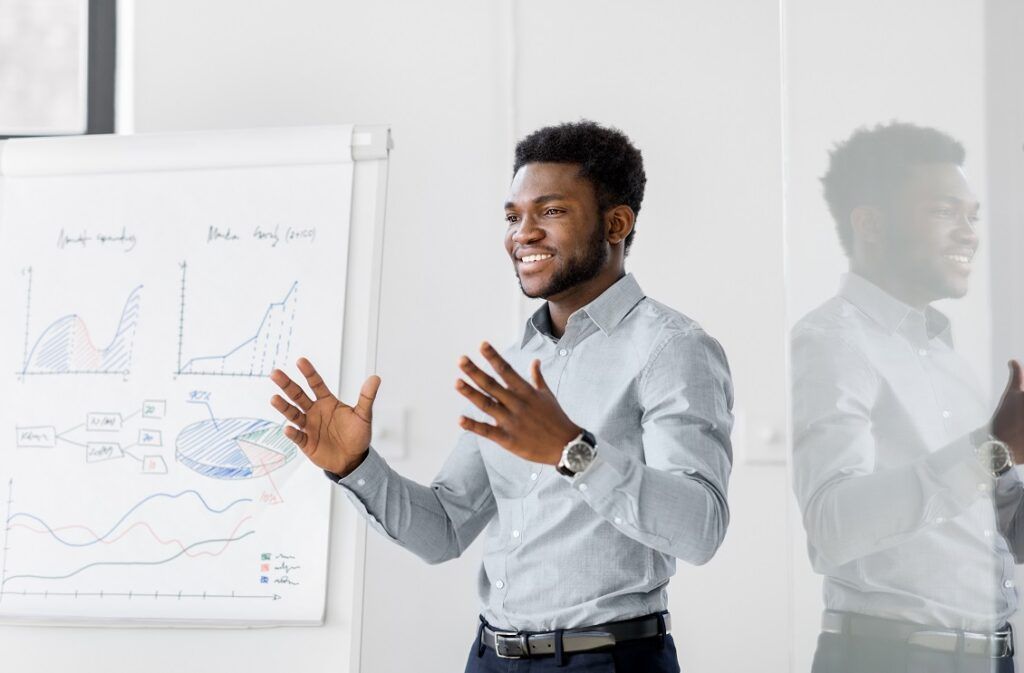
It is the analyst’s job to effectively communicate the output to the target audience, such as the management team or a company’s external investors. This requires focusing on the main points, facts, insights, and recommendations that will prompt the necessary action from the audience.
One challenge is making intricate and elaborate work easy to comprehend through great visuals and dashboards. For example, tables, graphs, and charts are tools that an analyst can use to their advantage to give deeper meaning to a company’s financial information. These tools organize relevant numbers that are rather dull and give life and story to them.
Here are some key objectives to think about when presenting financial analysis:
- Visual communication
- Audience and context
- Charts, graphs, and images
- Focus on important points
- Design principles
- Storytelling
- Persuasiveness
For a breakdown of these objectives, check out Excel Dashboards & Data Visualization course to help you become a world-class financial analyst.
Charts and graphs make any financial analysis readable, easy to follow, and provide great data presentation. They are often included in the financial model’s output, which is essential for the key decision-makers in a company.
The decision-makers comprise executives and managers who usually won’t have enough time to synthesize and interpret data on their own to make sound business decisions. Therefore, it is the job of the analyst to enhance the decision-making process and help guide the executives and managers to create value for the company.
When an analyst uses charts, it is necessary to be aware of what good charts and bad charts look like and how to avoid the latter when telling a story with data.
Examples of Good Charts
As for great visuals, you can quickly see what’s going on with the data presentation, saving you time for deciphering their actual meaning. More importantly, great visuals facilitate business decision-making because their goal is to provide persuasive, clear, and unambiguous numeric communication.
For reference, take a look at the example below that shows a dashboard, which includes a gauge chart for growth rates, a bar chart for the number of orders, an area chart for company revenues, and a line chart for EBITDA margins.
To learn the step-by-step process of creating these essential tools in MS Excel, watch our video course titled “ Excel Dashboard & Data Visualization .” Aside from what is given in the example below, our course will also teach how you can use other tables and charts to make your financial analysis stand out professionally.
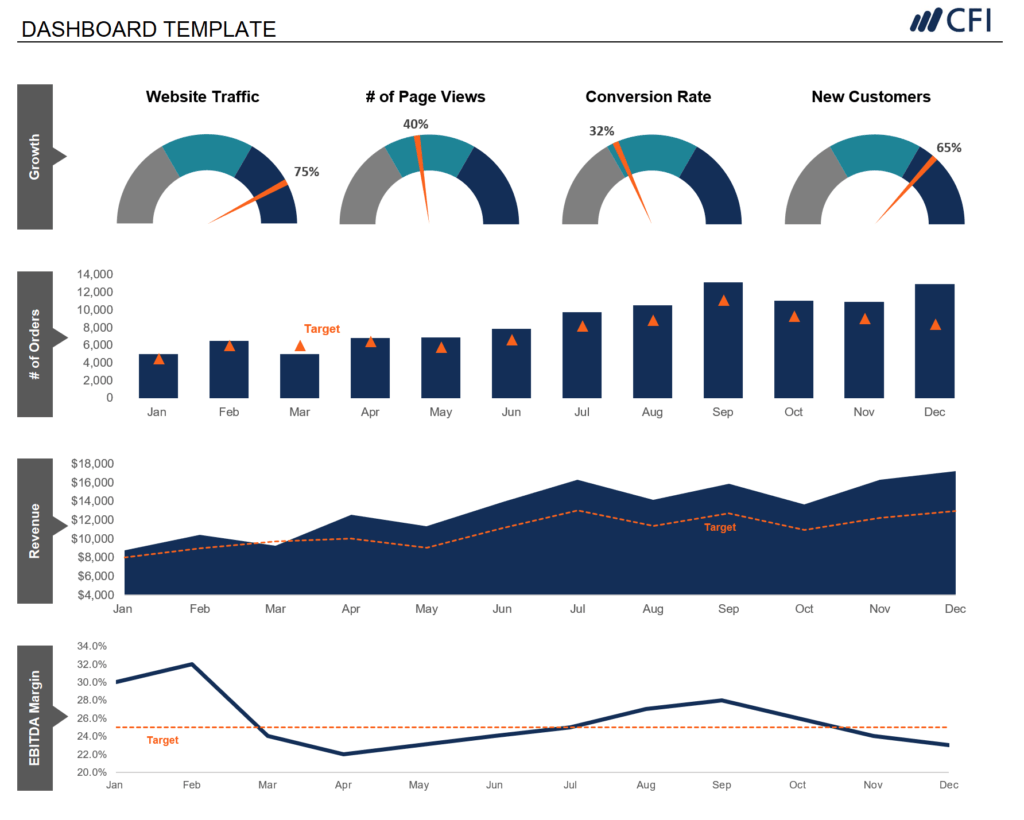
Learn how to build the graph above in our Dashboards Course !
Example of Poorly Crafted Charts
A bad chart, as seen below, will give the reader a difficult time to find the main takeaway of a report or presentation, because it contains too many colors, labels, and legends, and thus, will often look too busy. It also doesn’t help much if a chart, such as a pie chart, is displayed in 3D, as it skews the size and perceived value of the underlying data. A bad chart will be hard to follow and understand.
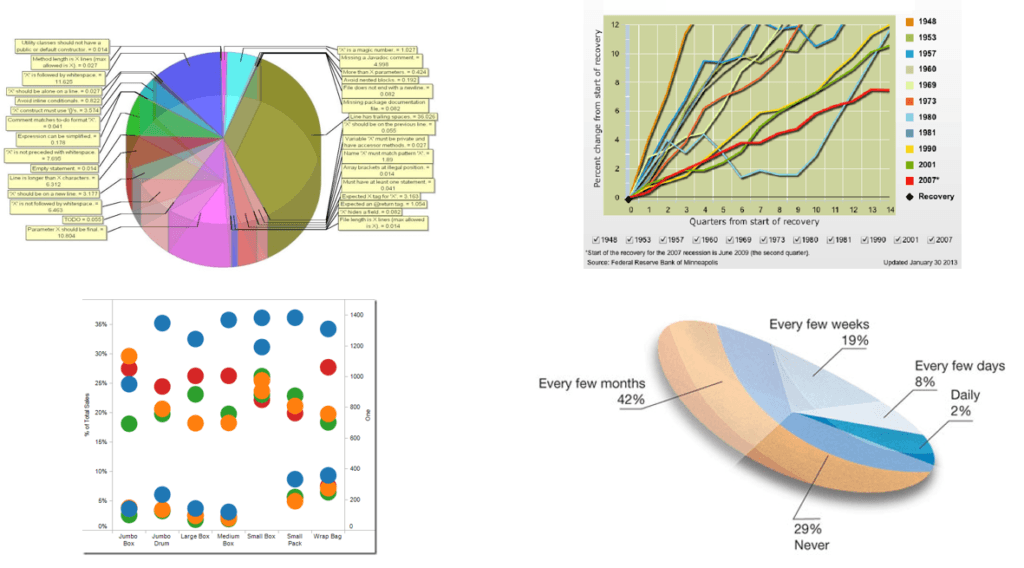
Aside from understanding the meaning of the numbers, a financial analyst must learn to combine numbers and language to craft an effective story. Relying only on data for a presentation may leave your audience finding it difficult to read, interpret, and analyze your data. You must do the work for them, and a good story will be easier to follow. It will help you arrive at the main points faster, rather than just solely presenting your report or live presentation with numbers.
The data can be in the form of revenues, expenses, profits, and cash flow. Simply adding notes, comments, and opinions to each line item will add an extra layer of insight, angle, and a new perspective to the report.
Furthermore, by combining data, visuals, and text, your audience will get a clear understanding of the current situation, past events, and possible conclusions and recommendations that can be made for the future.
The simple diagram below shows the different categories of your audience.
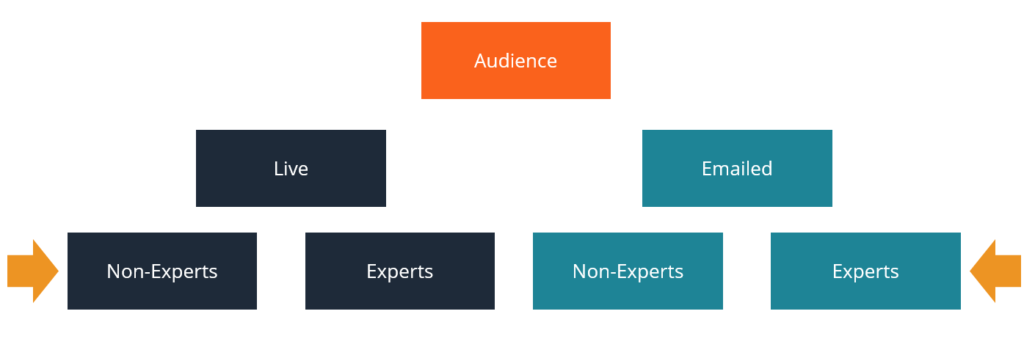
This chart is taken from our course on how to present data .
Internal Audience
An internal audience can either be the executives of the company or any employee who works in that company. For executives, the purpose of communicating a data-filled presentation is to give an update about a certain business activity such as a project or an initiative.
Another important purpose is to facilitate decision-making on managing the company’s operations, growing its core business, acquiring new markets and customers, investing in R&D, and other considerations. Knowing the relevant data and information beforehand will guide the decision-makers in making the right choices that will best position the company toward more success.
External Audience
An external audience can either be the company’s existing clients, where there are projects in progress, or new clients that the company wants to build a relationship with and win new business from. The other external audience is the general public, such as the company’s external shareholders and prospective investors of the company.
When it comes to winning new business, the analyst’s presentation will be more promotional and sales-oriented, whereas a project update will contain more specific information for the client, usually with lots of industry jargon.
Audiences for Live and Emailed Presentation
A live presentation contains more visuals and storytelling to connect more with the audience. It must be more precise and should get to the point faster and avoid long-winded speech or text because of limited time.
In contrast, an emailed presentation is expected to be read, so it will include more text. Just like a document or a book, it will include more detailed information, because its context will not be explained with a voice-over as in a live presentation.
When it comes to details, acronyms, and jargon in the presentation, these things depend on whether your audience are experts or not.
Every great presentation requires a clear “main idea”. It is the core purpose of the presentation and should be addressed clearly. Its significance should be highlighted and should cause the targeted audience to take some action on the matter.
An example of a serious and profound idea is given below.
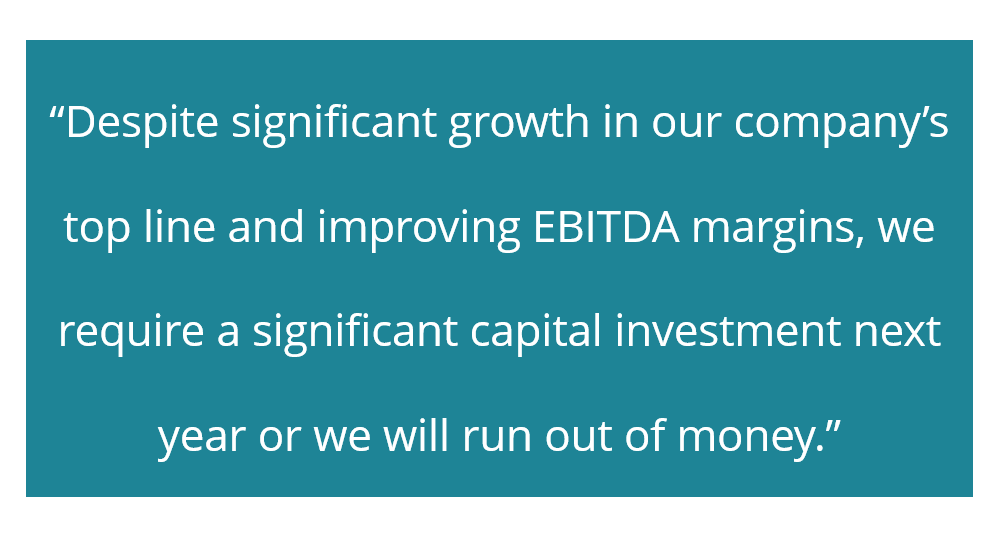
To communicate this big idea, we have to come up with appropriate and effective visual displays to show both the good and bad things surrounding the idea. It should put emphasis and attention on the most important part, which is the critical cash balance and capital investment situation for next year. This is an important component of data presentation.
The storyboarding below is how an analyst would build the presentation based on the big idea. Once the issue or the main idea has been introduced, it will be followed by a demonstration of the positive aspects of the company’s performance, as well as the negative aspects, which are more important and will likely require more attention.
Various ideas will then be suggested to solve the negative issues. However, before choosing the best option, a comparison of the different outcomes of the suggested ideas will be performed. Finally, a recommendation will be made that centers around the optimal choice to address the imminent problem highlighted in the big idea.
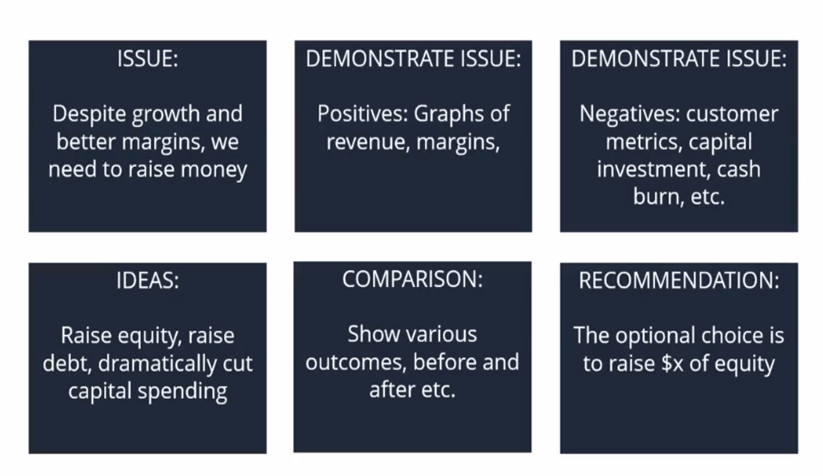
This storyboard is taken from our course on how to present data .
To get to the final point (recommendation), a great deal of analysis has been performed, which includes the charts and graphs discussed earlier, to make the whole presentation easy to follow, convincing, and compelling for your audience.
CFI offers the Business Intelligence & Data Analyst (BIDA)® certification program for those looking to take their careers to the next level. To keep learning and developing your knowledge base, please explore the additional relevant resources below:
- Investment Banking Pitch Books
- Excel Dashboards
- Financial Modeling Guide
- Startup Pitch Book
- See all business intelligence resources
- Share this article
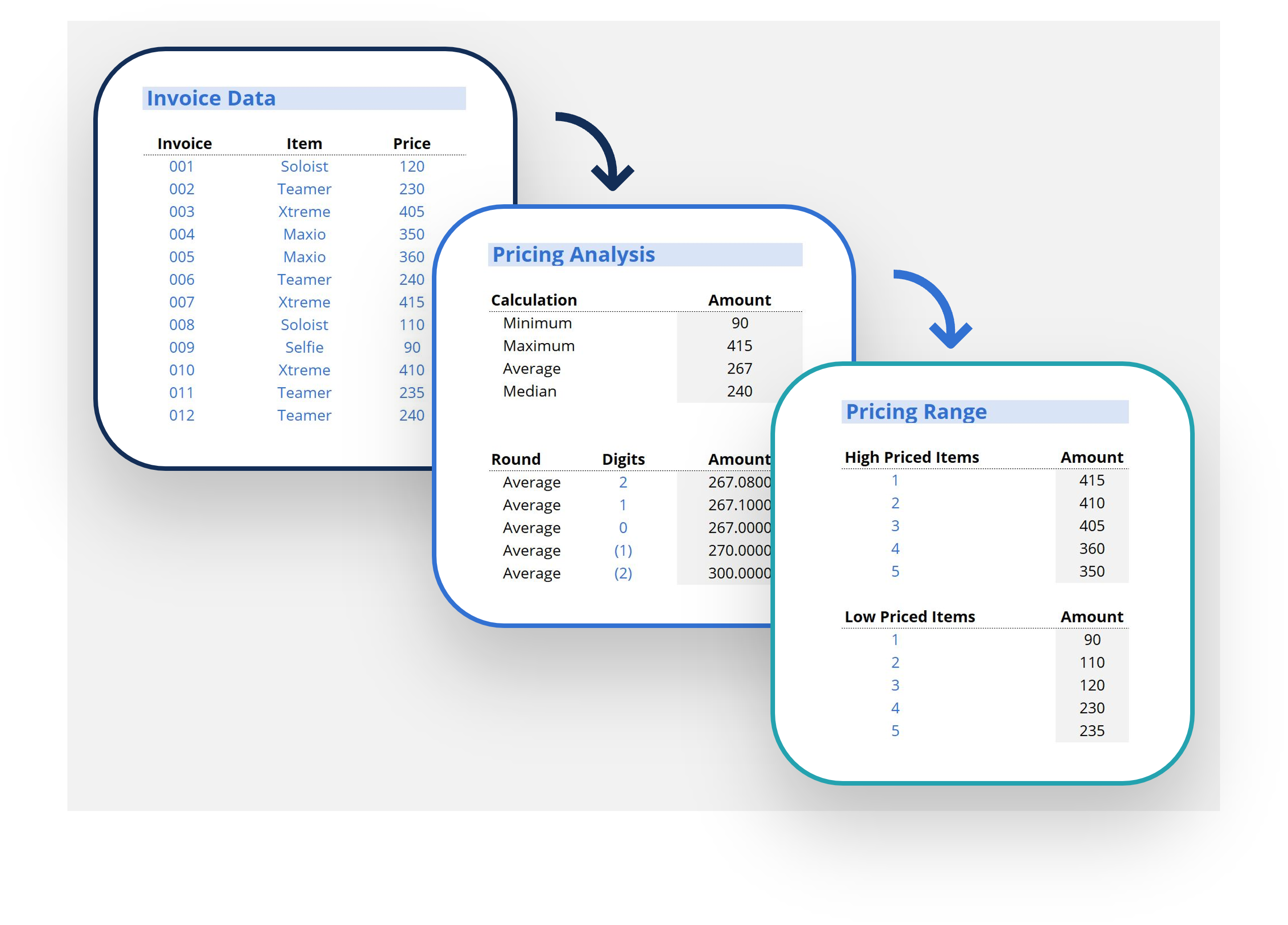
Create a free account to unlock this Template
Access and download collection of free Templates to help power your productivity and performance.
Already have an account? Log in
Supercharge your skills with Premium Templates
Take your learning and productivity to the next level with our Premium Templates.
Upgrading to a paid membership gives you access to our extensive collection of plug-and-play Templates designed to power your performance—as well as CFI's full course catalog and accredited Certification Programs.
Already have a Self-Study or Full-Immersion membership? Log in
Access Exclusive Templates
Gain unlimited access to more than 250 productivity Templates, CFI's full course catalog and accredited Certification Programs, hundreds of resources, expert reviews and support, the chance to work with real-world finance and research tools, and more.
Already have a Full-Immersion membership? Log in

- Certifications

Data Analysis 101: How to Make Your Presentations Practical and Effective
- December 27, 2022
- 54 Comments
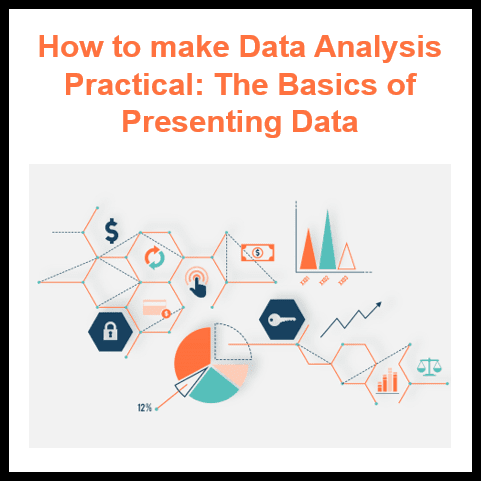
Understanding Importance of Data Analysis
The results of data analysis can give business the vital insights they need to turn in to successful and profitable ventures. It could be the difference between a successful business operation and a business operation that is in trouble.
Data analysis, though one of the most in-demand job roles globally, doesn’t require a degree in statistics or mathematics to do well, and employers from a wide variety of industries are very keen to recruit data analysts.
Businesses hire data analysts in the field of finance, marketing, administration, HR, IT and procurement, to name just a few. Understand the big picture and provide answers. By engaging in data analysis, you can actually delve deep and discover hidden truths that most business people would never be able to do.
What skills you should master to be a data analyst?
While Data Analyst roles are on the rise, there are certain skills that are vital for anyone who wants to become a data analyst . Before the job, a candidate needs to have either a degree in statistics, business or computer science or a related subject, or work experience in these areas.
If you’re interested in becoming a data analyst, you’ll need to know:
- Programming and algorithms
- Data Visualization
- Open-source and cloud technologies
- No coding experience is required.
How much is a data analyst worth? Data analysts earn an average salary of £32,403 per annum, according to jobs site Glassdoor. This pays for a salary, with benefits such as medical insurance and paid leave included in the starting salary. If you think you have the right skills, there are plenty of roles on offer.
What data analysis entails
Data analysis is an analytical process which involves recording and tabulating (recording and entering, entering and tabulating) the quantities of a product, such as numbers of units produced, costs of materials and expenses.
While data analyst can take different forms, for example in databases, in other structures such as spreadsheets, numbers are the main means of data entry. This involves entering and entering the required data in a data analysis system such as Excel.
For example, although a database doesn’t require a data analyst, it can still benefit from data analysis techniques such as binomial testing, ANOVA and Fisher’s exact tests. Where is the data analysis courses in IT? Given the ever-increasing reliance on technology in business, data analysis courses are vital skills.
What are the types of data analysis methods?
- Cluster analysis
The act of grouping a specific set of data in a manner that those elements are more similar to one another than to those in other groups – hence the term ‘cluster.’ Since there is no special target variable while doing clustering, the method is often used to find hidden patterns in the data. The approach is purposely used to offer additional context to a particular trend or dataset.
- Cohort analysis
This type of data analysis method uses historical data to examine and compare a determined segment of users’ behavior, which can then be grouped with others with similar characteristics. By using this data analysis methodology, it’s possible to gain a wealth of insight into consumer needs or a firm understanding of a broader target group.
A dependent variable is an element of a complex system that is assumed to have a single cause, but it’s affected by multiple factors, thus giving researchers an indication as to how a complex system function.
- Regression analysis
The regression analysis is used to predict how the value of a dependent variable changes when one or more independent variables change, stay the same or the dependent variable is not moved. Regression is a sophisticated statistical method that includes mathematical functions that are typically called “segmentation,” “distribution,” and “intercept” functions.
Regression is a type of regression analysis that only contains linear and quadratic functions. You can change the types of factors (or the independent variables) that are selected in regression analysis (it’s typically called “nonlinear regression analysis”) by changing the order in which the models are constructed.To begin, let’s explain how regression analysis works.
Examples in business world
The Oracle Corporation is one of the first multinational companies to adopt this type of analysis method, based on which the company was able to develop predictive modelling systems for marketing purposes.
In a more specific sense, a Regression analysis is a popular type of data analysis used for analyzing the likelihood that a random variable will move up or down a range of parameters in response to a change in a specific control variable.
Companies who use this type of analysis are looking for trends and patterned performance over time. For example, how a company may respond to a rising cost of labor and its effect on its business bottom line, a weather-related issue like an earthquake, a new advertising campaign, or even a surge in customer demand in some areas.
What are basic pointers to consider while presenting data
Recognize that presentation matters.
Too often, analysts make the mistake of presenting information in order to show an abstracted version of it. For instance, say a B2B company has 4 ways to improve their sales funnel:
- More Visually Engaging
- More Easily Transacted
- More Cost Effective
Then, “informative” would mean that a B2B company needs to optimize their sales funnel to each of these to be more “convenient, faster, easier, more visually engaging, or most cost effective.” Sure, it would be nice if they all improved – they would all provide a competitive advantage in some way. But that’s not what the data tells us.
Don’t scare people with numbers
When you’re presenting data, show as many as possible, in as many charts as possible. Then, try to talk through the implications of the data, rather than overwhelming people with an overwhelming amount of data.
Why? Research suggests that when a number is presented in a visual, people become more likely to process it and learn from it. I recommend using video, text, graphs, and pictures to represent your numbers. This creates a more visually appealing data set. The number of followers on Twitter is visually appealing. The number of followers on Facebook is visually appealing. But nobody looks at their Twitter followers. If you don’t know what your numbers mean, how will your audience? That doesn’t mean numbers aren’t important.
Maximize the data pixel ratio
The more data you show to a critical stakeholder, the more likely they are to get lost and distracted from what you’re actually trying to communicate. This is especially important in the case of people in the sales and marketing function.
Do you have a sales person out in the field who is trying to close a deal? It would be a shame if that person got lost in your Excel analytics and lost out on the sale. This problem also occurs on the web.
Consider how web visitors respond to large, colorful charts and graphs. If we’re talking about visualizations that depict web performance, a visual might be helpful. But how often do we see this done? Research shows that people respond better to web-based data in a simplified, less complex format.
Save 3-D for the movies
There are great stories in the universe. This is an oversimplification, but if you look at history, humans only understand stories. We are great storytellers. We develop, through trial and error, our own intuition about the “right” way to tell stories.
One of the most powerful and effective ways to present data is to go beyond the visual to the audible, that is, to tell stories in a way that people can relate to. Everything you hear about computers being a series of numbers is wrong. We visualize numbers in a precise, quantitative way. But the numbers are not a collection of isolated events. To understand them, we need to understand the broader context.
Friends don’t let friends use pie charts
Businesses and analysts have done this since pie charts first appeared on Microsoft Excel sheets. When presenting data, break down your pie chart into its component segments.
As opposed to an equal-sized circle for the average earnings for all the employees, share a pie chart where the percentages for each individual segment are different, with a link to the corresponding chart.
Pair with explanatory text, show their correlation, and make your choice based on your audience, not on whether you want to scare or “educate” them. The majority of audiences will see the same image, regardless of whether it’s presented in a bar chart, bar chart, line chart, or something else.
Choose the appropriate chart
Does the data make logical sense? Check your assumptions against the data. Are the graphs charting only part of the story? Include other variables in the graphs. Avoid using axis labels to mislead. Never rely on axes to infer, “logical” conclusions. Trust your eyes: you know what information your brain can process.
Think of numbers like music — they are pleasing, but not overwhelming. Save 3D for the movies. When everyone is enjoying 4K, 8K, and beyond, it’s hard to envision your audience without the new stuff. I remember the first time I got to see HDTV. At home, I sat behind a chair and kept turning around to watch the TV. But at the theatre, I didn’t need a chair. All I had to do was look up, and see the giant screen, the contrast, and the detail.
Don’t mix chart types for no reason
Excel chart s with colored areas help people focus. Arrows give us scale. Assume your audience doesn’t understand what you’re saying, even if they do. Nobody wants to open a recipe book to learn how to cook soup. Instead, we start with a recipe.
Use a formula to communicate your analysis with as few words as possible. Keep it simple. Resist the urge to over-complicate your presentation. A word cloud is not a word cloud. A bar chart is not a bar chart. If you use a word cloud to illustrate a chart, consider replacing a few words with a gif. A bar chart doesn’t need clouds. And a bar chart doesn’t need clouds. If there’s one thing that’s sure to confuse your audience, it’s bar charts.
Use color with intention
Use color with intention. It’s not about pretty. When it comes to presenting data clearly, “informative” is more important than “beautiful.”
However, visualizations like maps, axes, or snapshots can help visual communication to avoid this pitfall. If you are going to show a few locations on a map, make sure each location has a voice and uses a distinct color. Avoid repeating colors from the map or bottom bar in all the visuals. Be consistent with how you present the data . A pie chart is not very interesting if all it shows is a bunch of varying sizes of the pie.
Data analysis in the workplace, and how it will impact the future of business
Business leaders are taking note of the importance of data analysis skills in their organisation, as it can make an enormous impact on business.
Larger organisations such as Google, Amazon and Facebook employ huge teams of analysts to create their data and statistics. We are already seeing the rise of the next generation of big data analysts – those who can write code that analyses and visualizes the data and report back information to a company to help it improve efficiency and increase revenue.
The increasing need for high-level understanding of data analysis has already led to the role of data analyst becoming available at university level. It is no longer a mandatory business qualification but one that can enhance your CV.
By understanding the importance of each variable, you can improve your business by managing your time and creating more effective systems and processes for running your business. The focus shifts from just providing services to providing value to your customers, creating a better, more intuitive experience for them so they can work with your company for the long-term.
Adopting these small steps will allow you to be more effective in your business and go from being an employee to an entrepreneur.
Share This Post:
54 thoughts on “data analysis 101: how to make your presentations practical and effective”.
Buy Zyvox Online – Special offer: Save up to $498 – buy antibiotics online and get discount for all purchased!
Thanks again for the post.Thanks Again. Cool.
Thanks for great information. What trips can you recommend in 2024? Astro tourism, eco diving, home swapping, train stations are the new food destinations,sports tourism, coolcationing, gig tripping, private group travel?
Enjoyed every bit of your article.Really looking forward to read more. Great.
Really appreciate you sharing this post. Want more.
I am so grateful for your blog. Really Great.
Hey, thanks for the blog post.
Great, thanks for sharing this blog.Thanks Again. Will read on…
Thanks for sharing, this is a fantastic blog.Thanks Again. Great.
Thanks for sharing, this is a fantastic article.Really thank you! Fantastic.
Major thankies for the article post.Thanks Again. Will read on…
Hey, thanks for the blog post. Cool.
A round of applause for your blog post.Really looking forward to read more. Cool.
Appreciate you sharing, great article post. Great.
wow, awesome blog.Much thanks again. Cool.
Say, you got a nice blog.Really thank you! Cool.
Enjoyed every bit of your blog article.Much thanks again. Want more.
A round of applause for your blog post.Much thanks again. Cool.
I’m not sure where you’re getting your info, but good topic. I needs to spend some time learning more or understanding more. Thanks for wonderful info I was looking for this info for my mission.
Im thankful for the blog post.Much thanks again.
A big thank you for your article.Really thank you!
I truly appreciate this article post.Really looking forward to read more. Really Cool.
I really enjoy the article post.Much thanks again.
wow, awesome blog.Thanks Again. Will read on…
Awesome blog post.Much thanks again. Much obliged.
Im thankful for the blog.Much thanks again. Want more.
Thanks a lot for the post.Much thanks again. Want more.
I really liked your article.Really thank you! Really Cool.
A round of applause for your post.Thanks Again. Much obliged.
Say, you got a nice article.Really thank you! Fantastic.
I value the blog article. Really Cool.
A round of applause for your blog article.Really looking forward to read more. Great.
Really appreciate you sharing this blog article. Really Cool.
Really informative article. Really Great.
Fantastic article post.Really looking forward to read more. Really Great.
I really liked your post.Much thanks again. Much obliged.
Beneficial document helps make frequent advance, appreciate it write about, this pile-up connected with expertise is usually to hold finding out, focus is usually the beginning of money.
I really liked your blog article.Much thanks again. Really Great.
I really liked your article post.Really thank you! Fantastic.
Great, thanks for sharing this blog.Thanks Again. Awesome.
Enjoyed every bit of your blog article. Cool.
I really enjoy the blog. Want more.
Im grateful for the blog.Thanks Again. Awesome.
Very neat blog.Thanks Again. Cool.
Muchos Gracias for your blog article.
Im grateful for the post.Really looking forward to read more. Keep writing.
Great, thanks for sharing this post.Really looking forward to read more. Cool.
Thank you ever so for you article post.Really looking forward to read more. Will read on…
Great article post.Much thanks again. Great.
I really like and appreciate your blog post.Thanks Again. Awesome.
Really appreciate you sharing this blog. Really Cool.
Greetings! Incredibly helpful suggestions within this short article! It’s the very little adjustments that make the greatest modifications. Quite a few many thanks for sharing!
Awesome blog.Really looking forward to read more. Much obliged.
Thanks a lot for the blog post.Thanks Again. Much obliged.
Add a Comment Cancel reply
Save my name, email, and website in this browser for the next time I comment.
Get A 5X Raise In Salary
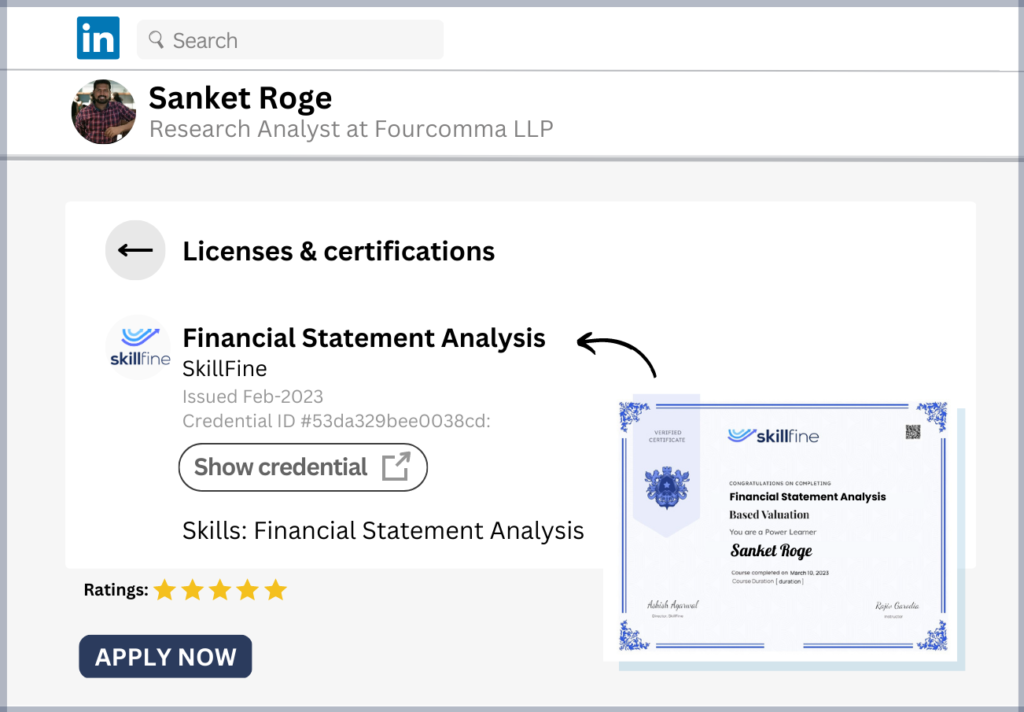
Reset Password
Insert/edit link.
Enter the destination URL
Or link to existing content
- Alternatives
10 Methods of Data Presentation That Really Work in 2024
Leah Nguyen • 15 July, 2024 • 13 min read
Have you ever presented a data report to your boss/coworkers/teachers thinking it was super dope like you’re some cyber hacker living in the Matrix, but all they saw was a pile of static numbers that seemed pointless and didn't make sense to them?
Understanding digits is rigid . Making people from non-analytical backgrounds understand those digits is even more challenging.
How can you clear up those confusing numbers and make your presentation as clear as the day? Let's check out these best ways to present data. 💎
How many type of charts are available to present data? | 7 |
How many charts are there in statistics? | 4, including bar, line, histogram and pie. |
How many types of charts are available in Excel? | 8 |
Who invented charts? | William Playfair |
When were the charts invented? | 18th Century |
More Tips with AhaSlides
- Marketing Presentation
- Survey Result Presentation
- Types of Presentation
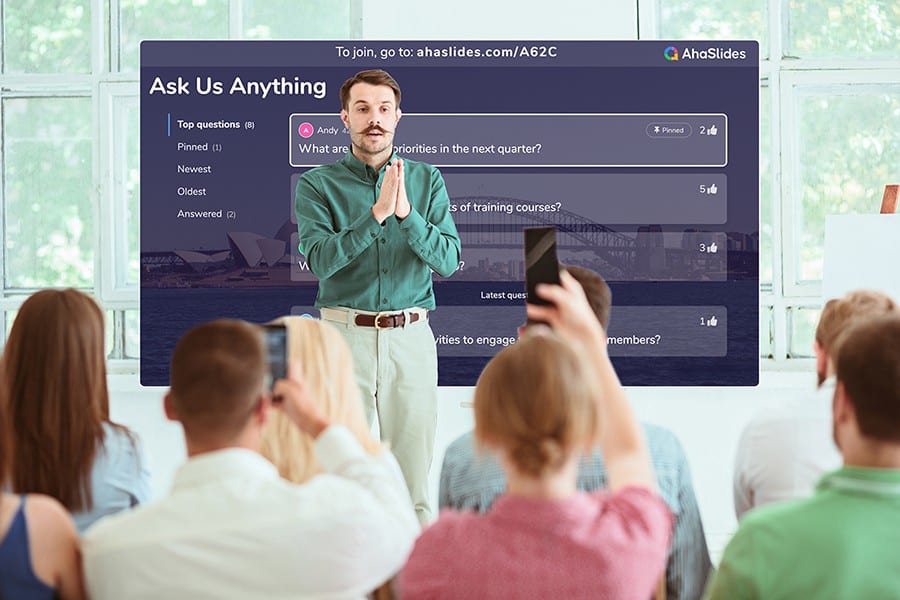
Start in seconds.
Get any of the above examples as templates. Sign up for free and take what you want from the template library!
Data Presentation - What Is It?
The term ’data presentation’ relates to the way you present data in a way that makes even the most clueless person in the room understand.
Some say it’s witchcraft (you’re manipulating the numbers in some ways), but we’ll just say it’s the power of turning dry, hard numbers or digits into a visual showcase that is easy for people to digest.
Presenting data correctly can help your audience understand complicated processes, identify trends, and instantly pinpoint whatever is going on without exhausting their brains.
Good data presentation helps…
- Make informed decisions and arrive at positive outcomes . If you see the sales of your product steadily increase throughout the years, it’s best to keep milking it or start turning it into a bunch of spin-offs (shoutout to Star Wars👀).
- Reduce the time spent processing data . Humans can digest information graphically 60,000 times faster than in the form of text. Grant them the power of skimming through a decade of data in minutes with some extra spicy graphs and charts.
- Communicate the results clearly . Data does not lie. They’re based on factual evidence and therefore if anyone keeps whining that you might be wrong, slap them with some hard data to keep their mouths shut.
- Add to or expand the current research . You can see what areas need improvement, as well as what details often go unnoticed while surfing through those little lines, dots or icons that appear on the data board.
Methods of Data Presentation and Examples
Imagine you have a delicious pepperoni, extra-cheese pizza. You can decide to cut it into the classic 8 triangle slices, the party style 12 square slices, or get creative and abstract on those slices.
There are various ways to cut a pizza and you get the same variety with how you present your data. In this section, we will bring you the 10 ways to slice a pizza - we mean to present your data - that will make your company’s most important asset as clear as day. Let's dive into 10 ways to present data efficiently.
#1 - Tabular
Among various types of data presentation, tabular is the most fundamental method, with data presented in rows and columns. Excel or Google Sheets would qualify for the job. Nothing fancy.
This is an example of a tabular presentation of data on Google Sheets. Each row and column has an attribute (year, region, revenue, etc.), and you can do a custom format to see the change in revenue throughout the year.
When presenting data as text, all you do is write your findings down in paragraphs and bullet points, and that’s it. A piece of cake to you, a tough nut to crack for whoever has to go through all of the reading to get to the point.
- 65% of email users worldwide access their email via a mobile device.
- Emails that are optimised for mobile generate 15% higher click-through rates.
- 56% of brands using emojis in their email subject lines had a higher open rate.
(Source: CustomerThermometer )
All the above quotes present statistical information in textual form. Since not many people like going through a wall of texts, you’ll have to figure out another route when deciding to use this method, such as breaking the data down into short, clear statements, or even as catchy puns if you’ve got the time to think of them.
#3 - Pie chart
A pie chart (or a ‘donut chart’ if you stick a hole in the middle of it) is a circle divided into slices that show the relative sizes of data within a whole. If you’re using it to show percentages, make sure all the slices add up to 100%.
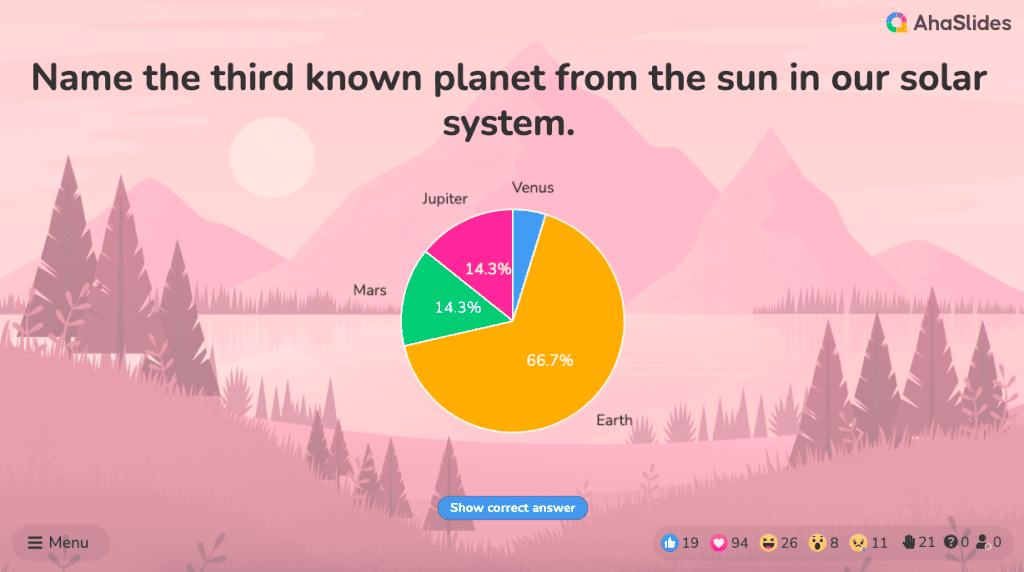
The pie chart is a familiar face at every party and is usually recognised by most people. However, one setback of using this method is our eyes sometimes can’t identify the differences in slices of a circle, and it’s nearly impossible to compare similar slices from two different pie charts, making them the villains in the eyes of data analysts.
#4 - Bar chart
The bar chart is a chart that presents a bunch of items from the same category, usually in the form of rectangular bars that are placed at an equal distance from each other. Their heights or lengths depict the values they represent.
They can be as simple as this:
Or more complex and detailed like this example of data presentation. Contributing to an effective statistic presentation, this one is a grouped bar chart that not only allows you to compare categories but also the groups within them as well.
#5 - Histogram
Similar in appearance to the bar chart but the rectangular bars in histograms don’t often have the gap like their counterparts.
Instead of measuring categories like weather preferences or favourite films as a bar chart does, a histogram only measures things that can be put into numbers.
Teachers can use presentation graphs like a histogram to see which score group most of the students fall into, like in this example above.
#6 - Line graph
Recordings to ways of displaying data, we shouldn't overlook the effectiveness of line graphs. Line graphs are represented by a group of data points joined together by a straight line. There can be one or more lines to compare how several related things change over time.
On a line chart’s horizontal axis, you usually have text labels, dates or years, while the vertical axis usually represents the quantity (e.g.: budget, temperature or percentage).
#7 - Pictogram graph
A pictogram graph uses pictures or icons relating to the main topic to visualise a small dataset. The fun combination of colours and illustrations makes it a frequent use at schools.
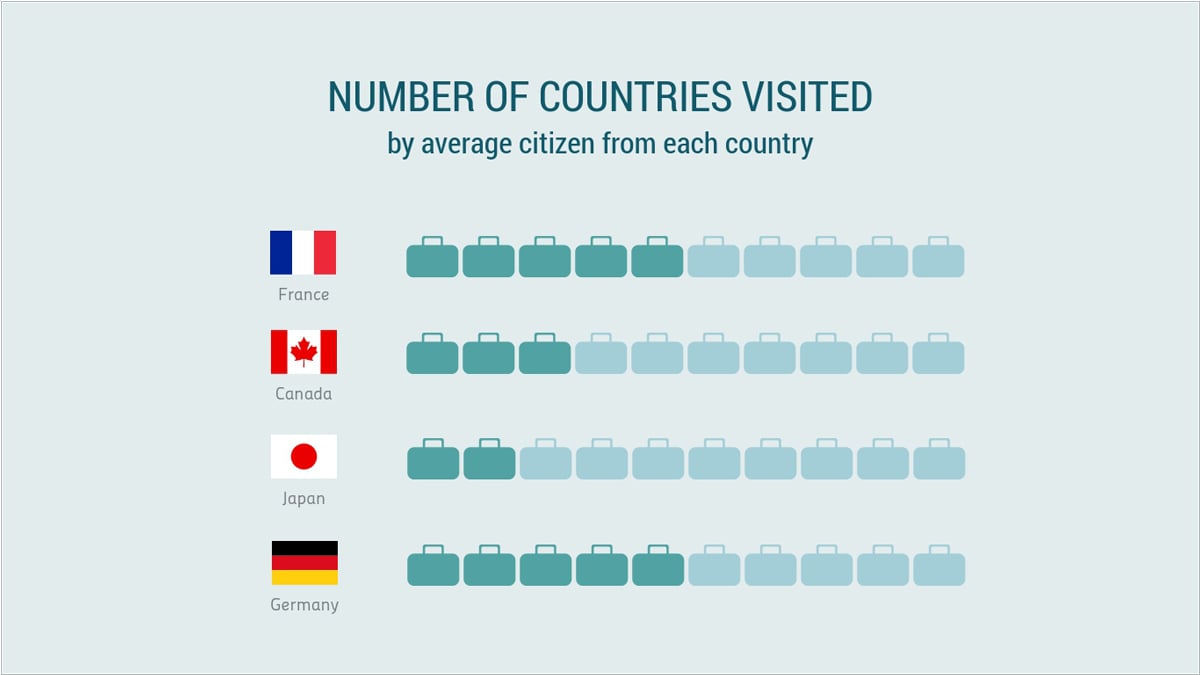
Pictograms are a breath of fresh air if you want to stay away from the monotonous line chart or bar chart for a while. However, they can present a very limited amount of data and sometimes they are only there for displays and do not represent real statistics.
#8 - Radar chart
If presenting five or more variables in the form of a bar chart is too stuffy then you should try using a radar chart, which is one of the most creative ways to present data.
Radar charts show data in terms of how they compare to each other starting from the same point. Some also call them ‘spider charts’ because each aspect combined looks like a spider web.
Radar charts can be a great use for parents who’d like to compare their child’s grades with their peers to lower their self-esteem. You can see that each angular represents a subject with a score value ranging from 0 to 100. Each student’s score across 5 subjects is highlighted in a different colour.
If you think that this method of data presentation somehow feels familiar, then you’ve probably encountered one while playing Pokémon .
#9 - Heat map
A heat map represents data density in colours. The bigger the number, the more colour intensity that data will be represented.
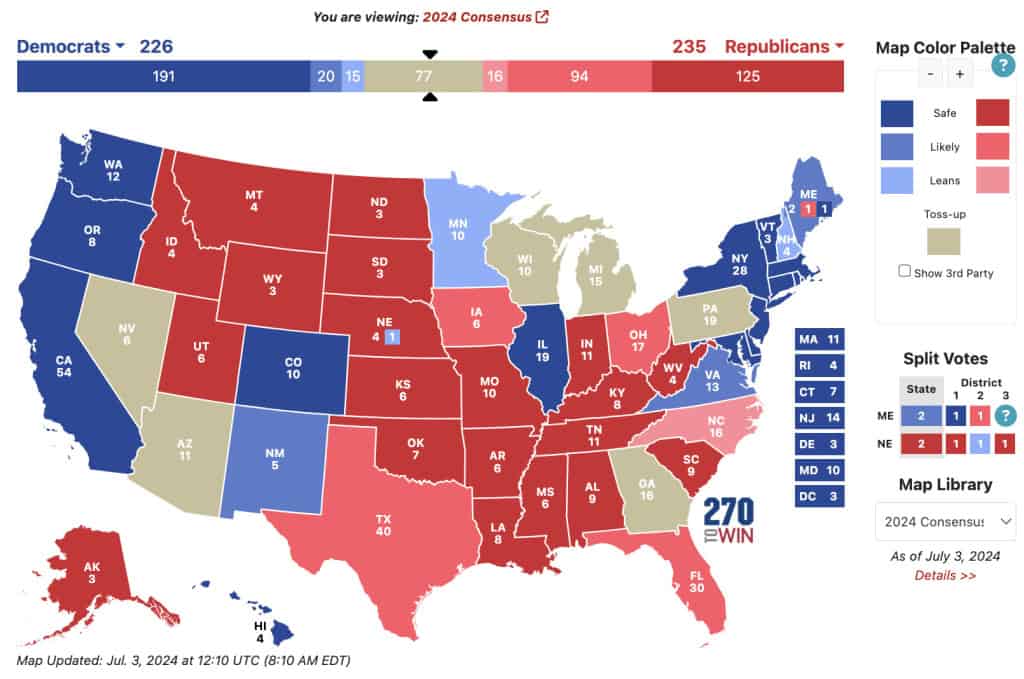
Most US citizens would be familiar with this data presentation method in geography. For elections, many news outlets assign a specific colour code to a state, with blue representing one candidate and red representing the other. The shade of either blue or red in each state shows the strength of the overall vote in that state.
Another great thing you can use a heat map for is to map what visitors to your site click on. The more a particular section is clicked the ‘hotter’ the colour will turn, from blue to bright yellow to red.
#10 - Scatter plot
If you present your data in dots instead of chunky bars, you’ll have a scatter plot.
A scatter plot is a grid with several inputs showing the relationship between two variables. It’s good at collecting seemingly random data and revealing some telling trends.
For example, in this graph, each dot shows the average daily temperature versus the number of beach visitors across several days. You can see that the dots get higher as the temperature increases, so it’s likely that hotter weather leads to more visitors.
5 Data Presentation Mistakes to Avoid
#1 - assume your audience understands what the numbers represent.
You may know all the behind-the-scenes of your data since you’ve worked with them for weeks, but your audience doesn’t.
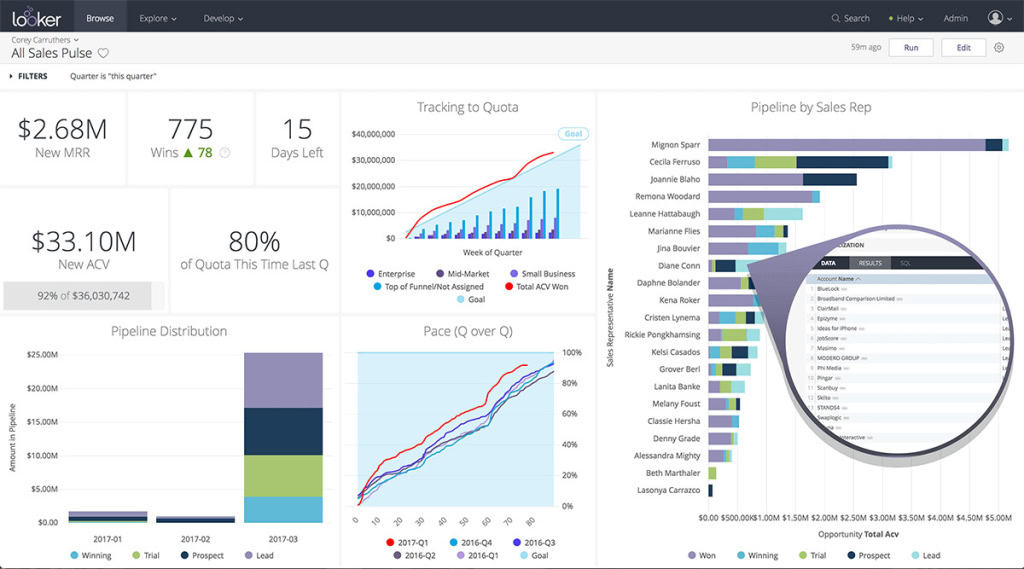
Showing without telling only invites more and more questions from your audience, as they have to constantly make sense of your data, wasting the time of both sides as a result.
While showing your data presentations, you should tell them what the data are about before hitting them with waves of numbers first. You can use interactive activities such as polls , word clouds , online quizzes and Q&A sections , combined with icebreaker games , to assess their understanding of the data and address any confusion beforehand.
#2 - Use the wrong type of chart
Charts such as pie charts must have a total of 100% so if your numbers accumulate to 193% like this example below, you’re definitely doing it wrong.
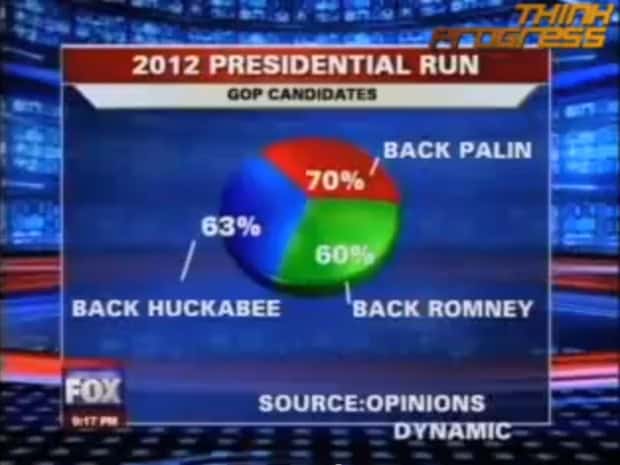
Before making a chart, ask yourself: what do I want to accomplish with my data? Do you want to see the relationship between the data sets, show the up and down trends of your data, or see how segments of one thing make up a whole?
Remember, clarity always comes first. Some data visualisations may look cool, but if they don’t fit your data, steer clear of them.
#3 - Make it 3D
3D is a fascinating graphical presentation example. The third dimension is cool, but full of risks.
Can you see what’s behind those red bars? Because we can’t either. You may think that 3D charts add more depth to the design, but they can create false perceptions as our eyes see 3D objects closer and bigger than they appear, not to mention they cannot be seen from multiple angles.
#4 - Use different types of charts to compare contents in the same category
This is like comparing a fish to a monkey. Your audience won’t be able to identify the differences and make an appropriate correlation between the two data sets.
Next time, stick to one type of data presentation only. Avoid the temptation of trying various data visualisation methods in one go and make your data as accessible as possible.
#5 - Bombard the audience with too much information
The goal of data presentation is to make complex topics much easier to understand, and if you’re bringing too much information to the table, you’re missing the point.
The more information you give, the more time it will take for your audience to process it all. If you want to make your data understandable and give your audience a chance to remember it, keep the information within it to an absolute minimum. You should end your session with open-ended questions to see what your participants really think.
What are the Best Methods of Data Presentation?
Finally, which is the best way to present data?
The answer is…
There is none! Each type of presentation has its own strengths and weaknesses and the one you choose greatly depends on what you’re trying to do.
For example:
- Go for a scatter plot if you’re exploring the relationship between different data values, like seeing whether the sales of ice cream go up because of the temperature or because people are just getting more hungry and greedy each day?
- Go for a line graph if you want to mark a trend over time.
- Go for a heat map if you like some fancy visualisation of the changes in a geographical location, or to see your visitors' behaviour on your website.
- Go for a pie chart (especially in 3D) if you want to be shunned by others because it was never a good idea👇
Frequently Asked Questions
What is a chart presentation.
A chart presentation is a way of presenting data or information using visual aids such as charts, graphs, and diagrams. The purpose of a chart presentation is to make complex information more accessible and understandable for the audience.
When can I use charts for the presentation?
Charts can be used to compare data, show trends over time, highlight patterns, and simplify complex information.
Why should you use charts for presentation?
You should use charts to ensure your contents and visuals look clean, as they are the visual representative, provide clarity, simplicity, comparison, contrast and super time-saving!
What are the 4 graphical methods of presenting data?
Histogram, Smoothed frequency graph, Pie diagram or Pie chart, Cumulative or ogive frequency graph, and Frequency Polygon.
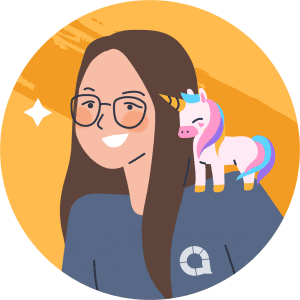
Leah Nguyen
Words that convert, stories that stick. I turn complex ideas into engaging narratives - helping audiences learn, remember, and take action.
Tips to Engage with Polls & Trivia

More from AhaSlides
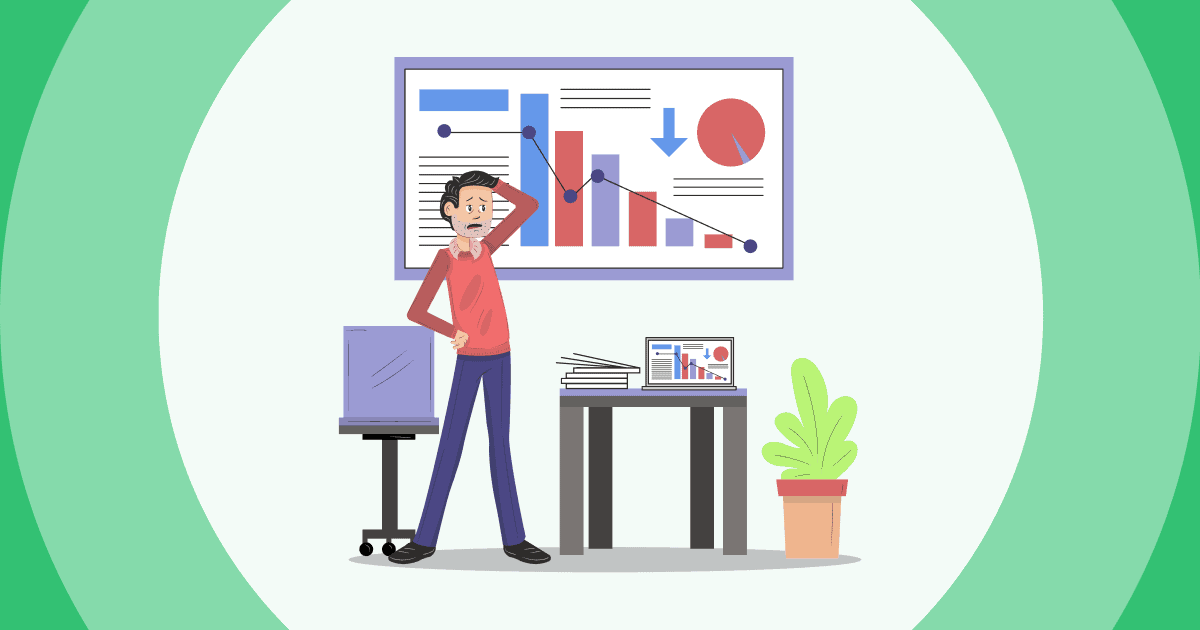

Skills for Learning : Research Skills
Data analysis is an ongoing process that should occur throughout your research project. Suitable data-analysis methods must be selected when you write your research proposal. The nature of your data (i.e. quantitative or qualitative) will be influenced by your research design and purpose. The data will also influence the analysis methods selected.
We run interactive workshops to help you develop skills related to doing research, such as data analysis, writing literature reviews and preparing for dissertations. Find out more on the Skills for Learning Workshops page.
We have online academic skills modules within MyBeckett for all levels of university study. These modules will help your academic development and support your success at LBU. You can work through the modules at your own pace, revisiting them as required. Find out more from our FAQ What academic skills modules are available?
Quantitative data analysis
Broadly speaking, 'statistics' refers to methods, tools and techniques used to collect, organise and interpret data. The goal of statistics is to gain understanding from data. Therefore, you need to know how to:
- Produce data – for example, by handing out a questionnaire or doing an experiment.
- Organise, summarise, present and analyse data.
- Draw valid conclusions from findings.
There are a number of statistical methods you can use to analyse data. Choosing an appropriate statistical method should follow naturally, however, from your research design. Therefore, you should think about data analysis at the early stages of your study design. You may need to consult a statistician for help with this.
Tips for working with statistical data
- Plan so that the data you get has a good chance of successfully tackling the research problem. This will involve reading literature on your subject, as well as on what makes a good study.
- To reach useful conclusions, you need to reduce uncertainties or 'noise'. Thus, you will need a sufficiently large data sample. A large sample will improve precision. However, this must be balanced against the 'costs' (time and money) of collection.
- Consider the logistics. Will there be problems in obtaining sufficient high-quality data? Think about accuracy, trustworthiness and completeness.
- Statistics are based on random samples. Consider whether your sample will be suited to this sort of analysis. Might there be biases to think about?
- How will you deal with missing values (any data that is not recorded for some reason)? These can result from gaps in a record or whole records being missed out.
- When analysing data, start by looking at each variable separately. Conduct initial/exploratory data analysis using graphical displays. Do this before looking at variables in conjunction or anything more complicated. This process can help locate errors in the data and also gives you a 'feel' for the data.
- Look out for patterns of 'missingness'. They are likely to alert you if there’s a problem. If the 'missingness' is not random, then it will have an impact on the results.
- Be vigilant and think through what you are doing at all times. Think critically. Statistics are not just mathematical tricks that a computer sorts out. Rather, analysing statistical data is a process that the human mind must interpret!
Top tips! Try inventing or generating the sort of data you might get and see if you can analyse it. Make sure that your process works before gathering actual data. Think what the output of an analytic procedure will look like before doing it for real.
(Note: it is actually difficult to generate realistic data. There are fraud-detection methods in place to identify data that has been fabricated. So, remember to get rid of your practice data before analysing the real stuff!)
Statistical software packages
Software packages can be used to analyse and present data. The most widely used ones are SPSS and NVivo.
SPSS is a statistical-analysis and data-management package for quantitative data analysis. Click on ‘ How do I install SPSS? ’ to learn how to download SPSS to your personal device. SPSS can perform a wide variety of statistical procedures. Some examples are:
- Data management (i.e. creating subsets of data or transforming data).
- Summarising, describing or presenting data (i.e. mean, median and frequency).
- Looking at the distribution of data (i.e. standard deviation).
- Comparing groups for significant differences using parametric (i.e. t-test) and non-parametric (i.e. Chi-square) tests.
- Identifying significant relationships between variables (i.e. correlation).
NVivo can be used for qualitative data analysis. It is suitable for use with a wide range of methodologies. Click on ‘ How do I access NVivo ’ to learn how to download NVivo to your personal device. NVivo supports grounded theory, survey data, case studies, focus groups, phenomenology, field research and action research.
- Process data such as interview transcripts, literature or media extracts, and historical documents.
- Code data on screen and explore all coding and documents interactively.
- Rearrange, restructure, extend and edit text, coding and coding relationships.
- Search imported text for words, phrases or patterns, and automatically code the results.
Qualitative data analysis
Miles and Huberman (1994) point out that there are diverse approaches to qualitative research and analysis. They suggest, however, that it is possible to identify 'a fairly classic set of analytic moves arranged in sequence'. This involves:
- Affixing codes to a set of field notes drawn from observation or interviews.
- Noting reflections or other remarks in the margins.
- Sorting/sifting through these materials to identify: a) similar phrases, relationships between variables, patterns and themes and b) distinct differences between subgroups and common sequences.
- Isolating these patterns/processes and commonalties/differences. Then, taking them out to the field in the next wave of data collection.
- Highlighting generalisations and relating them to your original research themes.
- Taking the generalisations and analysing them in relation to theoretical perspectives.
(Miles and Huberman, 1994.)
Patterns and generalisations are usually arrived at through a process of analytic induction (see above points 5 and 6). Qualitative analysis rarely involves statistical analysis of relationships between variables. Qualitative analysis aims to gain in-depth understanding of concepts, opinions or experiences.
Presenting information
There are a number of different ways of presenting and communicating information. The particular format you use is dependent upon the type of data generated from the methods you have employed.
Here are some appropriate ways of presenting information for different types of data:
Bar charts: These may be useful for comparing relative sizes. However, they tend to use a large amount of ink to display a relatively small amount of information. Consider a simple line chart as an alternative.
Pie charts: These have the benefit of indicating that the data must add up to 100%. However, they make it difficult for viewers to distinguish relative sizes, especially if two slices have a difference of less than 10%.
Other examples of presenting data in graphical form include line charts and scatter plots .
Qualitative data is more likely to be presented in text form. For example, using quotations from interviews or field diaries.
- Plan ahead, thinking carefully about how you will analyse and present your data.
- Think through possible restrictions to resources you may encounter and plan accordingly.
- Find out about the different IT packages available for analysing your data and select the most appropriate.
- If necessary, allow time to attend an introductory course on a particular computer package. You can book SPSS and NVivo workshops via MyHub .
- Code your data appropriately, assigning conceptual or numerical codes as suitable.
- Organise your data so it can be analysed and presented easily.
- Choose the most suitable way of presenting your information, according to the type of data collected. This will allow your information to be understood and interpreted better.
Primary, secondary and tertiary sources
Information sources are sometimes categorised as primary, secondary or tertiary sources depending on whether or not they are ‘original’ materials or data. For some research projects, you may need to use primary sources as well as secondary or tertiary sources. However the distinction between primary and secondary sources is not always clear and depends on the context. For example, a newspaper article might usually be categorised as a secondary source. But it could also be regarded as a primary source if it were an article giving a first-hand account of a historical event written close to the time it occurred.
- Primary sources
- Secondary sources
- Tertiary sources
- Grey literature
Primary sources are original sources of information that provide first-hand accounts of what is being experienced or researched. They enable you to get as close to the actual event or research as possible. They are useful for getting the most contemporary information about a topic.
Examples include diary entries, newspaper articles, census data, journal articles with original reports of research, letters, email or other correspondence, original manuscripts and archives, interviews, research data and reports, statistics, autobiographies, exhibitions, films, and artists' writings.
Some information will be available on an Open Access basis, freely accessible online. However, many academic sources are paywalled, and you may need to login as a Leeds Beckett student to access them. Where Leeds Beckett does not have access to a source, you can use our Request It! Service .
Secondary sources interpret, evaluate or analyse primary sources. They're useful for providing background information on a topic, or for looking back at an event from a current perspective. The majority of your literature searching will probably be done to find secondary sources on your topic.
Examples include journal articles which review or interpret original findings, popular magazine articles commenting on more serious research, textbooks and biographies.
The term tertiary sources isn't used a great deal. There's overlap between what might be considered a secondary source and a tertiary source. One definition is that a tertiary source brings together secondary sources.
Examples include almanacs, fact books, bibliographies, dictionaries and encyclopaedias, directories, indexes and abstracts. They can be useful for introductory information or an overview of a topic in the early stages of research.
Depending on your subject of study, grey literature may be another source you need to use. Grey literature includes technical or research reports, theses and dissertations, conference papers, government documents, white papers, and so on.
Artificial intelligence tools
Before using any generative artificial intelligence or paraphrasing tools in your assessments, you should check if this is permitted on your course.
If their use is permitted on your course, you must acknowledge any use of generative artificial intelligence tools such as ChatGPT or paraphrasing tools (e.g., Grammarly, Quillbot, etc.), even if you have only used them to generate ideas for your assessments or for proofreading.
- Academic Integrity Module in MyBeckett
- Assignment Calculator
- Building on Feedback
- Disability Advice
- Essay X-ray tool
- International Students' Academic Introduction
- Manchester Academic Phrasebank
- Quote, Unquote
- Skills and Subject Suppor t
- Turnitin Grammar Checker
{{You can add more boxes below for links specific to this page [this note will not appear on user pages] }}
- Research Methods Checklist
- Sampling Checklist
Skills for Learning FAQs

0113 812 1000
- University Disclaimer
- Accessibility
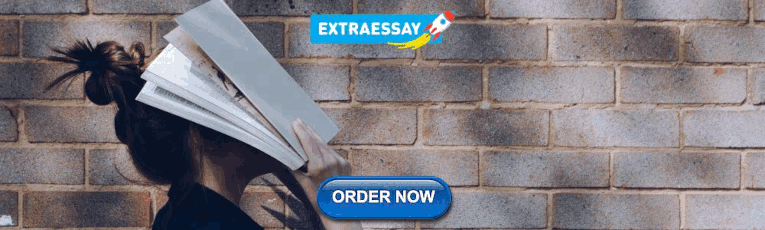
Call Us Today! +91 99907 48956 | [email protected]

It is the simplest form of data Presentation often used in schools or universities to provide a clearer picture to students, who are better able to capture the concepts effectively through a pictorial Presentation of simple data.
2. Column chart

It is a simplified version of the pictorial Presentation which involves the management of a larger amount of data being shared during the presentations and providing suitable clarity to the insights of the data.
3. Pie Charts

Pie charts provide a very descriptive & a 2D depiction of the data pertaining to comparisons or resemblance of data in two separate fields.
4. Bar charts

A bar chart that shows the accumulation of data with cuboid bars with different dimensions & lengths which are directly proportionate to the values they represent. The bars can be placed either vertically or horizontally depending on the data being represented.
5. Histograms
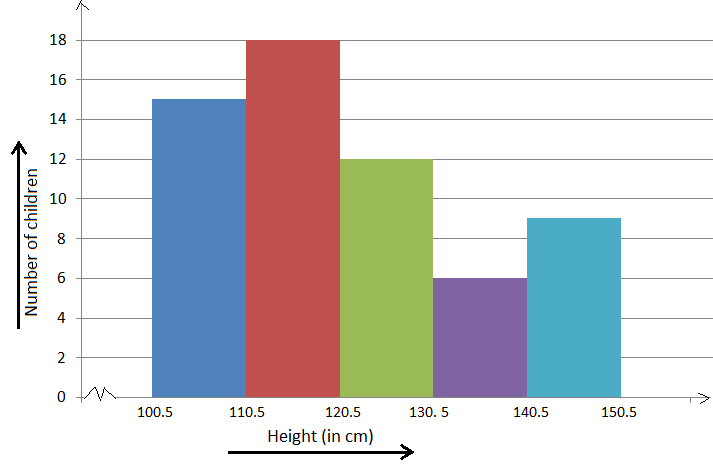
It is a perfect Presentation of the spread of numerical data. The main differentiation that separates data graphs and histograms are the gaps in the data graphs.
6. Box plots

Box plot or Box-plot is a way of representing groups of numerical data through quartiles. Data Presentation is easier with this style of graph dealing with the extraction of data to the minutes of difference.

Map Data graphs help you with data Presentation over an area to display the areas of concern. Map graphs are useful to make an exact depiction of data over a vast case scenario.
All these visual presentations share a common goal of creating meaningful insights and a platform to understand and manage the data in relation to the growth and expansion of one’s in-depth understanding of data & details to plan or execute future decisions or actions.
Importance of Data Presentation
Data Presentation could be both can be a deal maker or deal breaker based on the delivery of the content in the context of visual depiction.
Data Presentation tools are powerful communication tools that can simplify the data by making it easily understandable & readable at the same time while attracting & keeping the interest of its readers and effectively showcase large amounts of complex data in a simplified manner.
If the user can create an insightful presentation of the data in hand with the same sets of facts and figures, then the results promise to be impressive.
There have been situations where the user has had a great amount of data and vision for expansion but the presentation drowned his/her vision.
To impress the higher management and top brass of a firm, effective presentation of data is needed.
Data Presentation helps the clients or the audience to not spend time grasping the concept and the future alternatives of the business and to convince them to invest in the company & turn it profitable both for the investors & the company.
Although data presentation has a lot to offer, the following are some of the major reason behind the essence of an effective presentation:-
- Many consumers or higher authorities are interested in the interpretation of data, not the raw data itself. Therefore, after the analysis of the data, users should represent the data with a visual aspect for better understanding and knowledge.
- The user should not overwhelm the audience with a number of slides of the presentation and inject an ample amount of texts as pictures that will speak for themselves.
- Data presentation often happens in a nutshell with each department showcasing their achievements towards company growth through a graph or a histogram.
- Providing a brief description would help the user to attain attention in a small amount of time while informing the audience about the context of the presentation
- The inclusion of pictures, charts, graphs and tables in the presentation help for better understanding the potential outcomes.
- An effective presentation would allow the organization to determine the difference with the fellow organization and acknowledge its flaws. Comparison of data would assist them in decision making.
Recommended Courses

Data Visualization
Using powerbi &tableau.

Tableau for Data Analysis

MySQL Certification Program

The PowerBI Masterclass
Need help call our support team 7:00 am to 10:00 pm (ist) at (+91 999-074-8956 | 9650-308-956), keep in touch, email: [email protected].
WhatsApp us
What is Data-Driven Analysis? Methods and Examples
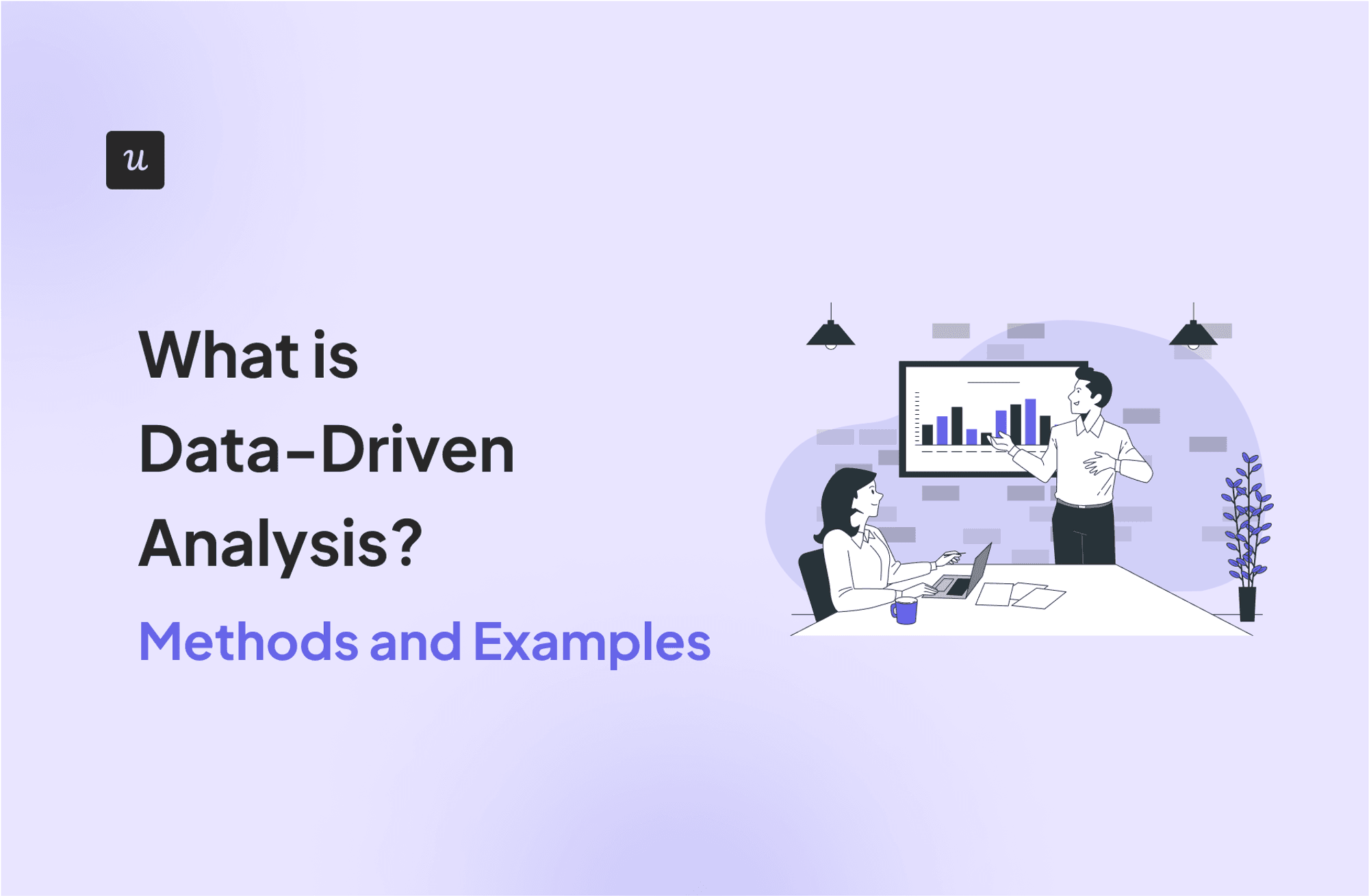
Harnessing the power of data-driven analysis can transform how you make decisions , fine-tune your strategies, and, ultimately, boost your bottom line.
But what exactly does it involve, and how can you use it to your advantage? This article will be your go-to guide, breaking down the concept of data-driven analysis and showing you practical ways to apply it to your specific business needs.
- Data-driven analysis is the process of using data to make strategic decisions or gain insights. It cuts across different business processes — you can implement the principles to create data-driven marketing campaigns, improve your product offerings, optimize customer support , and so on.
- A reliable data-driven approach…
- Helps you make the right decisions .
- Enhances customer understanding.
- Improves accountability and transparency within your organization.
- Examples of data analysis scenarios
- Qualitative data analysis.
- Quantitative data analysis.
- Sentiment analysis.
- How to become a data-driven organization:
- Encourage all teams – from marketing and sales to customer success and product development – to view data as an invaluable asset that can inform their decision-making process.
- Establish a data-driven strategy where small and big data can flow freely and securely across different departments (you can use tools like Userpilot ).
- Leverage no-code analytics tools for reports (that way, your non-technical teams won’t have to rely on professional analysts for predictive analysis and data-driven decision-making).
- Ready to implement a data-driven approach to your processes? Book a demo and see how Userpilot can help you track the different analysis types and deploy contextual in-app solutions.

Try Userpilot and Take Your Product Experience to the Next Level
- 14 Day Trial
- No Credit Card Required
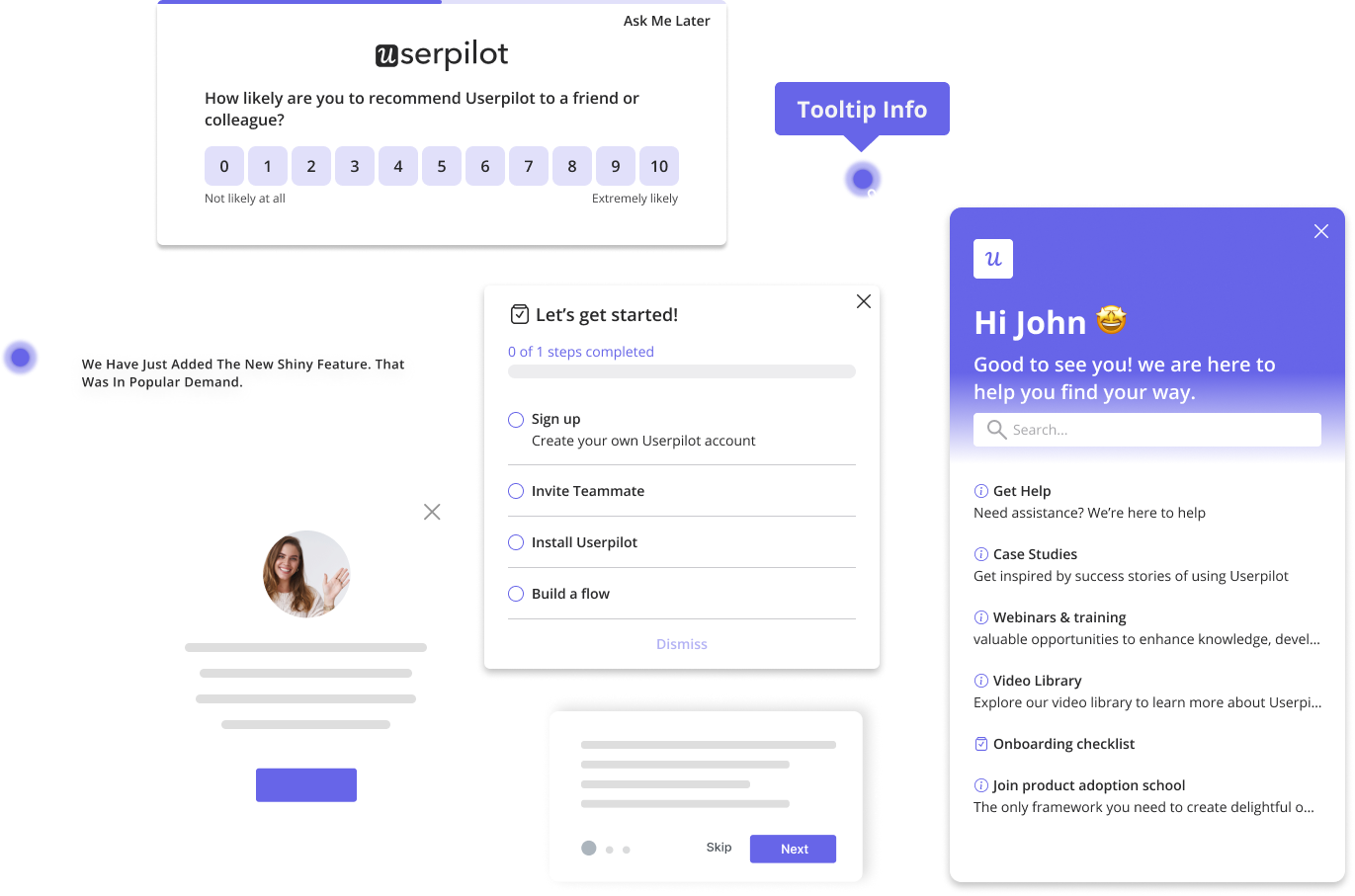
What is data-driven analysis?
Data-driven analysis is the process of using data to make strategic decisions or gain insights. It involves collecting, analyzing, and interpreting data to reveal patterns , trends , and relationships between components.
By leveraging statistical tools and software, data-driven companies are able to make evidence-based decisions, enhancing accuracy and reducing biases.
Why should you take a data-driven approach?
The benefits of data-driven analysis are enormous. Here are a few:
- Informed decision-making : Data-driven analysis provides a factual basis for decision-making, reducing the risk of relying on gut feelings or inaccurate assumptions. By analyzing trends , patterns, and correlations in data, you can identify opportunities, anticipate challenges, and make strategic choices that are more likely to lead to success.
- Enhanced customer understanding : A data-driven strategy gives valuable insights into customer preferences , behaviors , and pain points . This enables you to personalize products, services, and marketing campaigns , tailoring them to meet the specific needs of your target audience.
- Accountability and transparency : A data-driven approach is inherently transparent because the evidence supporting decisions is laid out. This promotes accountability, as it’s easy to track the performance of different strategies and determine who was responsible for each. This transparency can build trust among stakeholders and foster a culture of continuous improvement within the organization.
Examples of data analysis methods
Data analysis methods vary depending on the specific insights you need. Here are a few common examples:
Qualitative data analysis
This method involves analyzing non-numerical data , such as text-based feedback surveys, user interviews, and customer reviews. It often focuses on identifying themes, patterns, and meanings within the data.
Qualitative analysis is best used when you need to uncover the “why” behind user behavior. For example, if you notice a decline in user engagement, you can trigger qualitative surveys and analyze the responses to understand user pain points and note improvement areas.

Quantitative data analysis
This method focuses on numerical data, allowing you to track and measure key performance indicators (KPIs) for your business. You can analyze user engagement metrics , funnel drops , conversion rates, NPS scores, and churn rates to assess your product’s success and identify areas for optimization.
Quantitative analysis can help you make data-driven decisions about pricing , marketing strategies , and product development . For example, you can use funnel analysis to understand where drop-offs occur most in your conversion process and deploy data-driven solutions.

Sentiment analysis
This type of analysis evaluates textual data to determine the sentiment or emotional tone behind it.
Sentiment analysis uses natural language processing and machine learning to classify opinions as positive, negative, or neutral, making it effective for social media monitoring, customer feedback analysis , and brand reputation management.
One valuable application of this analysis is tracking customer sentiment over time. By monitoring the changing emotional tone in user or company accounts, you can quickly identify when a power user is losing interest in your product or, conversely, when a user is becoming more engaged. This valuable insight can help you proactively address potential issues and capitalize on opportunities.
Userpilot’s Profile Analysis feature can help you effortlessly identify sentiment trends for individual and company accounts:

How to build a data-driven culture for your SaaS?
Creating a data-driven culture in your SaaS organization involves several key steps to ensure data is accessible, understandable, and used to inform decision-making. Follow these steps to get started:
Facilitate data collection across departments
Encourage all teams – from marketing and sales to customer success and product development – to view data as an invaluable asset that can inform decision-making. Regularly discuss relevant metrics and tie them to team goals to reinforce this data-driven mindset.
To streamline data collection, leverage no-code and low-code tools like Userpilot to automate event tracking . These tools simplify capturing user actions within your product—such as button clicks, feature usage , and sign-ups—without requiring extensive coding knowledge.
For instance, you can configure Userpilot to track how many users complete your onboarding flow or engage with specific features, providing insights into areas that might require refinement.
Enable data sharing
Data silos are a common obstacle to a truly data-driven culture. To overcome this, it’s imperative you establish a data management system where relevant data can flow freely and securely across different departments.
Centralized analytics dashboards , reports , and data visualization tools play a pivotal role in achieving this. These platforms offer a unified view of key metrics and insights, ensuring everyone has access to the information they need.
However, access alone is not enough. To prevent “data blindness,” where existing data sits unused and insights are missed, prioritize regular communication – share data analysis reports and discuss key findings in cross-departmental meetings. This collaborative, data-driven approach fosters a shared understanding of the company’s performance and facilitates informed decision-making at all levels.

Leverage no-code analytics tools for reports
No-code analytics tools empower individuals with varying technical skills to generate custom analytics reports and dashboards through intuitive interfaces. This removes the dependency on data analysts and accelerates the process of turning raw data into actionable insights .
Userpilot makes it much easier. You can use templates to set up data analytics dashboards that track your most important metrics or build custom dashboards from scratch, only including the metrics you want to see.
For instance, your product team can set a dashboard to monitor adoption rates , while customer success tracks help center visits and onboarding completion – all without writing a single line of code. This ease of use and customization will encourage greater engagement with data across your organization, leading to a more data-driven approach to problem-solving and decision-making.
For comprehensive insights, it’s best to combine different data analysis methods. Qualitative analysis uncovers the motivations behind customer behavior, quantitative analysis illuminates what customers are doing, and sentiment analysis gauges how customers feel about your product or brand.
Ready to get started with data-driven analysis? Book a demo and see how Userpilot can help you track the different analysis types and deploy contextual in-app solutions.
Leave a comment Cancel reply
Save my name, email, and website in this browser for the next time I comment.
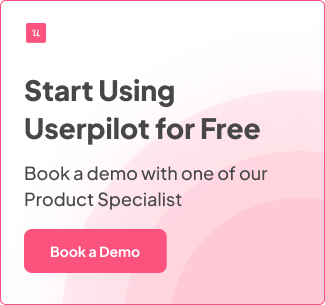
Get The Insights!
The fastest way to learn about Product Growth,Management & Trends.
The coolest way to learn about Product Growth, Management & Trends. Delivered fresh to your inbox, weekly.

The fastest way to learn about Product Growth, Management & Trends.
You might also be interested in ...
What is nps & how to increase it + benchmark report 2024.
Aazar Ali Shad
Customer Segmentation Analysis: How-to Guide With Types & Examples
- Privacy Policy

Home » Data Analysis – Process, Methods and Types
Data Analysis – Process, Methods and Types
Table of Contents

Data Analysis
Definition:
Data analysis refers to the process of inspecting, cleaning, transforming, and modeling data with the goal of discovering useful information, drawing conclusions, and supporting decision-making. It involves applying various statistical and computational techniques to interpret and derive insights from large datasets. The ultimate aim of data analysis is to convert raw data into actionable insights that can inform business decisions, scientific research, and other endeavors.
Data Analysis Process
The following are step-by-step guides to the data analysis process:
Define the Problem
The first step in data analysis is to clearly define the problem or question that needs to be answered. This involves identifying the purpose of the analysis, the data required, and the intended outcome.
Collect the Data
The next step is to collect the relevant data from various sources. This may involve collecting data from surveys, databases, or other sources. It is important to ensure that the data collected is accurate, complete, and relevant to the problem being analyzed.
Clean and Organize the Data
Once the data has been collected, it needs to be cleaned and organized. This involves removing any errors or inconsistencies in the data, filling in missing values, and ensuring that the data is in a format that can be easily analyzed.
Analyze the Data
The next step is to analyze the data using various statistical and analytical techniques. This may involve identifying patterns in the data, conducting statistical tests, or using machine learning algorithms to identify trends and insights.
Interpret the Results
After analyzing the data, the next step is to interpret the results. This involves drawing conclusions based on the analysis and identifying any significant findings or trends.
Communicate the Findings
Once the results have been interpreted, they need to be communicated to stakeholders. This may involve creating reports, visualizations, or presentations to effectively communicate the findings and recommendations.
Take Action
The final step in the data analysis process is to take action based on the findings. This may involve implementing new policies or procedures, making strategic decisions, or taking other actions based on the insights gained from the analysis.
Types of Data Analysis
Types of Data Analysis are as follows:
Descriptive Analysis
This type of analysis involves summarizing and describing the main characteristics of a dataset, such as the mean, median, mode, standard deviation, and range.
Inferential Analysis
This type of analysis involves making inferences about a population based on a sample. Inferential analysis can help determine whether a certain relationship or pattern observed in a sample is likely to be present in the entire population.
Diagnostic Analysis
This type of analysis involves identifying and diagnosing problems or issues within a dataset. Diagnostic analysis can help identify outliers, errors, missing data, or other anomalies in the dataset.
Predictive Analysis
This type of analysis involves using statistical models and algorithms to predict future outcomes or trends based on historical data. Predictive analysis can help businesses and organizations make informed decisions about the future.
Prescriptive Analysis
This type of analysis involves recommending a course of action based on the results of previous analyses. Prescriptive analysis can help organizations make data-driven decisions about how to optimize their operations, products, or services.
Exploratory Analysis
This type of analysis involves exploring the relationships and patterns within a dataset to identify new insights and trends. Exploratory analysis is often used in the early stages of research or data analysis to generate hypotheses and identify areas for further investigation.
Data Analysis Methods
Data Analysis Methods are as follows:
Statistical Analysis
This method involves the use of mathematical models and statistical tools to analyze and interpret data. It includes measures of central tendency, correlation analysis, regression analysis, hypothesis testing, and more.
Machine Learning
This method involves the use of algorithms to identify patterns and relationships in data. It includes supervised and unsupervised learning, classification, clustering, and predictive modeling.
Data Mining
This method involves using statistical and machine learning techniques to extract information and insights from large and complex datasets.
Text Analysis
This method involves using natural language processing (NLP) techniques to analyze and interpret text data. It includes sentiment analysis, topic modeling, and entity recognition.
Network Analysis
This method involves analyzing the relationships and connections between entities in a network, such as social networks or computer networks. It includes social network analysis and graph theory.
Time Series Analysis
This method involves analyzing data collected over time to identify patterns and trends. It includes forecasting, decomposition, and smoothing techniques.
Spatial Analysis
This method involves analyzing geographic data to identify spatial patterns and relationships. It includes spatial statistics, spatial regression, and geospatial data visualization.
Data Visualization
This method involves using graphs, charts, and other visual representations to help communicate the findings of the analysis. It includes scatter plots, bar charts, heat maps, and interactive dashboards.
Qualitative Analysis
This method involves analyzing non-numeric data such as interviews, observations, and open-ended survey responses. It includes thematic analysis, content analysis, and grounded theory.
Multi-criteria Decision Analysis
This method involves analyzing multiple criteria and objectives to support decision-making. It includes techniques such as the analytical hierarchy process, TOPSIS, and ELECTRE.
Data Analysis Tools
There are various data analysis tools available that can help with different aspects of data analysis. Below is a list of some commonly used data analysis tools:
- Microsoft Excel: A widely used spreadsheet program that allows for data organization, analysis, and visualization.
- SQL : A programming language used to manage and manipulate relational databases.
- R : An open-source programming language and software environment for statistical computing and graphics.
- Python : A general-purpose programming language that is widely used in data analysis and machine learning.
- Tableau : A data visualization software that allows for interactive and dynamic visualizations of data.
- SAS : A statistical analysis software used for data management, analysis, and reporting.
- SPSS : A statistical analysis software used for data analysis, reporting, and modeling.
- Matlab : A numerical computing software that is widely used in scientific research and engineering.
- RapidMiner : A data science platform that offers a wide range of data analysis and machine learning tools.
Applications of Data Analysis
Data analysis has numerous applications across various fields. Below are some examples of how data analysis is used in different fields:
- Business : Data analysis is used to gain insights into customer behavior, market trends, and financial performance. This includes customer segmentation, sales forecasting, and market research.
- Healthcare : Data analysis is used to identify patterns and trends in patient data, improve patient outcomes, and optimize healthcare operations. This includes clinical decision support, disease surveillance, and healthcare cost analysis.
- Education : Data analysis is used to measure student performance, evaluate teaching effectiveness, and improve educational programs. This includes assessment analytics, learning analytics, and program evaluation.
- Finance : Data analysis is used to monitor and evaluate financial performance, identify risks, and make investment decisions. This includes risk management, portfolio optimization, and fraud detection.
- Government : Data analysis is used to inform policy-making, improve public services, and enhance public safety. This includes crime analysis, disaster response planning, and social welfare program evaluation.
- Sports : Data analysis is used to gain insights into athlete performance, improve team strategy, and enhance fan engagement. This includes player evaluation, scouting analysis, and game strategy optimization.
- Marketing : Data analysis is used to measure the effectiveness of marketing campaigns, understand customer behavior, and develop targeted marketing strategies. This includes customer segmentation, marketing attribution analysis, and social media analytics.
- Environmental science : Data analysis is used to monitor and evaluate environmental conditions, assess the impact of human activities on the environment, and develop environmental policies. This includes climate modeling, ecological forecasting, and pollution monitoring.
When to Use Data Analysis
Data analysis is useful when you need to extract meaningful insights and information from large and complex datasets. It is a crucial step in the decision-making process, as it helps you understand the underlying patterns and relationships within the data, and identify potential areas for improvement or opportunities for growth.
Here are some specific scenarios where data analysis can be particularly helpful:
- Problem-solving : When you encounter a problem or challenge, data analysis can help you identify the root cause and develop effective solutions.
- Optimization : Data analysis can help you optimize processes, products, or services to increase efficiency, reduce costs, and improve overall performance.
- Prediction: Data analysis can help you make predictions about future trends or outcomes, which can inform strategic planning and decision-making.
- Performance evaluation : Data analysis can help you evaluate the performance of a process, product, or service to identify areas for improvement and potential opportunities for growth.
- Risk assessment : Data analysis can help you assess and mitigate risks, whether it is financial, operational, or related to safety.
- Market research : Data analysis can help you understand customer behavior and preferences, identify market trends, and develop effective marketing strategies.
- Quality control: Data analysis can help you ensure product quality and customer satisfaction by identifying and addressing quality issues.
Purpose of Data Analysis
The primary purposes of data analysis can be summarized as follows:
- To gain insights: Data analysis allows you to identify patterns and trends in data, which can provide valuable insights into the underlying factors that influence a particular phenomenon or process.
- To inform decision-making: Data analysis can help you make informed decisions based on the information that is available. By analyzing data, you can identify potential risks, opportunities, and solutions to problems.
- To improve performance: Data analysis can help you optimize processes, products, or services by identifying areas for improvement and potential opportunities for growth.
- To measure progress: Data analysis can help you measure progress towards a specific goal or objective, allowing you to track performance over time and adjust your strategies accordingly.
- To identify new opportunities: Data analysis can help you identify new opportunities for growth and innovation by identifying patterns and trends that may not have been visible before.
Examples of Data Analysis
Some Examples of Data Analysis are as follows:
- Social Media Monitoring: Companies use data analysis to monitor social media activity in real-time to understand their brand reputation, identify potential customer issues, and track competitors. By analyzing social media data, businesses can make informed decisions on product development, marketing strategies, and customer service.
- Financial Trading: Financial traders use data analysis to make real-time decisions about buying and selling stocks, bonds, and other financial instruments. By analyzing real-time market data, traders can identify trends and patterns that help them make informed investment decisions.
- Traffic Monitoring : Cities use data analysis to monitor traffic patterns and make real-time decisions about traffic management. By analyzing data from traffic cameras, sensors, and other sources, cities can identify congestion hotspots and make changes to improve traffic flow.
- Healthcare Monitoring: Healthcare providers use data analysis to monitor patient health in real-time. By analyzing data from wearable devices, electronic health records, and other sources, healthcare providers can identify potential health issues and provide timely interventions.
- Online Advertising: Online advertisers use data analysis to make real-time decisions about advertising campaigns. By analyzing data on user behavior and ad performance, advertisers can make adjustments to their campaigns to improve their effectiveness.
- Sports Analysis : Sports teams use data analysis to make real-time decisions about strategy and player performance. By analyzing data on player movement, ball position, and other variables, coaches can make informed decisions about substitutions, game strategy, and training regimens.
- Energy Management : Energy companies use data analysis to monitor energy consumption in real-time. By analyzing data on energy usage patterns, companies can identify opportunities to reduce energy consumption and improve efficiency.
Characteristics of Data Analysis
Characteristics of Data Analysis are as follows:
- Objective : Data analysis should be objective and based on empirical evidence, rather than subjective assumptions or opinions.
- Systematic : Data analysis should follow a systematic approach, using established methods and procedures for collecting, cleaning, and analyzing data.
- Accurate : Data analysis should produce accurate results, free from errors and bias. Data should be validated and verified to ensure its quality.
- Relevant : Data analysis should be relevant to the research question or problem being addressed. It should focus on the data that is most useful for answering the research question or solving the problem.
- Comprehensive : Data analysis should be comprehensive and consider all relevant factors that may affect the research question or problem.
- Timely : Data analysis should be conducted in a timely manner, so that the results are available when they are needed.
- Reproducible : Data analysis should be reproducible, meaning that other researchers should be able to replicate the analysis using the same data and methods.
- Communicable : Data analysis should be communicated clearly and effectively to stakeholders and other interested parties. The results should be presented in a way that is understandable and useful for decision-making.
Advantages of Data Analysis
Advantages of Data Analysis are as follows:
- Better decision-making: Data analysis helps in making informed decisions based on facts and evidence, rather than intuition or guesswork.
- Improved efficiency: Data analysis can identify inefficiencies and bottlenecks in business processes, allowing organizations to optimize their operations and reduce costs.
- Increased accuracy: Data analysis helps to reduce errors and bias, providing more accurate and reliable information.
- Better customer service: Data analysis can help organizations understand their customers better, allowing them to provide better customer service and improve customer satisfaction.
- Competitive advantage: Data analysis can provide organizations with insights into their competitors, allowing them to identify areas where they can gain a competitive advantage.
- Identification of trends and patterns : Data analysis can identify trends and patterns in data that may not be immediately apparent, helping organizations to make predictions and plan for the future.
- Improved risk management : Data analysis can help organizations identify potential risks and take proactive steps to mitigate them.
- Innovation: Data analysis can inspire innovation and new ideas by revealing new opportunities or previously unknown correlations in data.
Limitations of Data Analysis
- Data quality: The quality of data can impact the accuracy and reliability of analysis results. If data is incomplete, inconsistent, or outdated, the analysis may not provide meaningful insights.
- Limited scope: Data analysis is limited by the scope of the data available. If data is incomplete or does not capture all relevant factors, the analysis may not provide a complete picture.
- Human error : Data analysis is often conducted by humans, and errors can occur in data collection, cleaning, and analysis.
- Cost : Data analysis can be expensive, requiring specialized tools, software, and expertise.
- Time-consuming : Data analysis can be time-consuming, especially when working with large datasets or conducting complex analyses.
- Overreliance on data: Data analysis should be complemented with human intuition and expertise. Overreliance on data can lead to a lack of creativity and innovation.
- Privacy concerns: Data analysis can raise privacy concerns if personal or sensitive information is used without proper consent or security measures.
About the author
Muhammad Hassan
Researcher, Academic Writer, Web developer
You may also like

Evaluating Research – Process, Examples and...

Dissertation vs Thesis – Key Differences

Institutional Review Board – Application Sample...

Research Report – Example, Writing Guide and...

Textual Analysis – Types, Examples and Guide

Discriminant Analysis – Methods, Types and...
- Subscription
21 Data Science Projects for Beginners (with Source Code)
Looking to start a career in data science but lack experience? This is a common challenge. Many aspiring data scientists find themselves in a tricky situation: employers want experienced candidates, but how do you gain experience without a job? The answer lies in building a strong portfolio of data science projects .
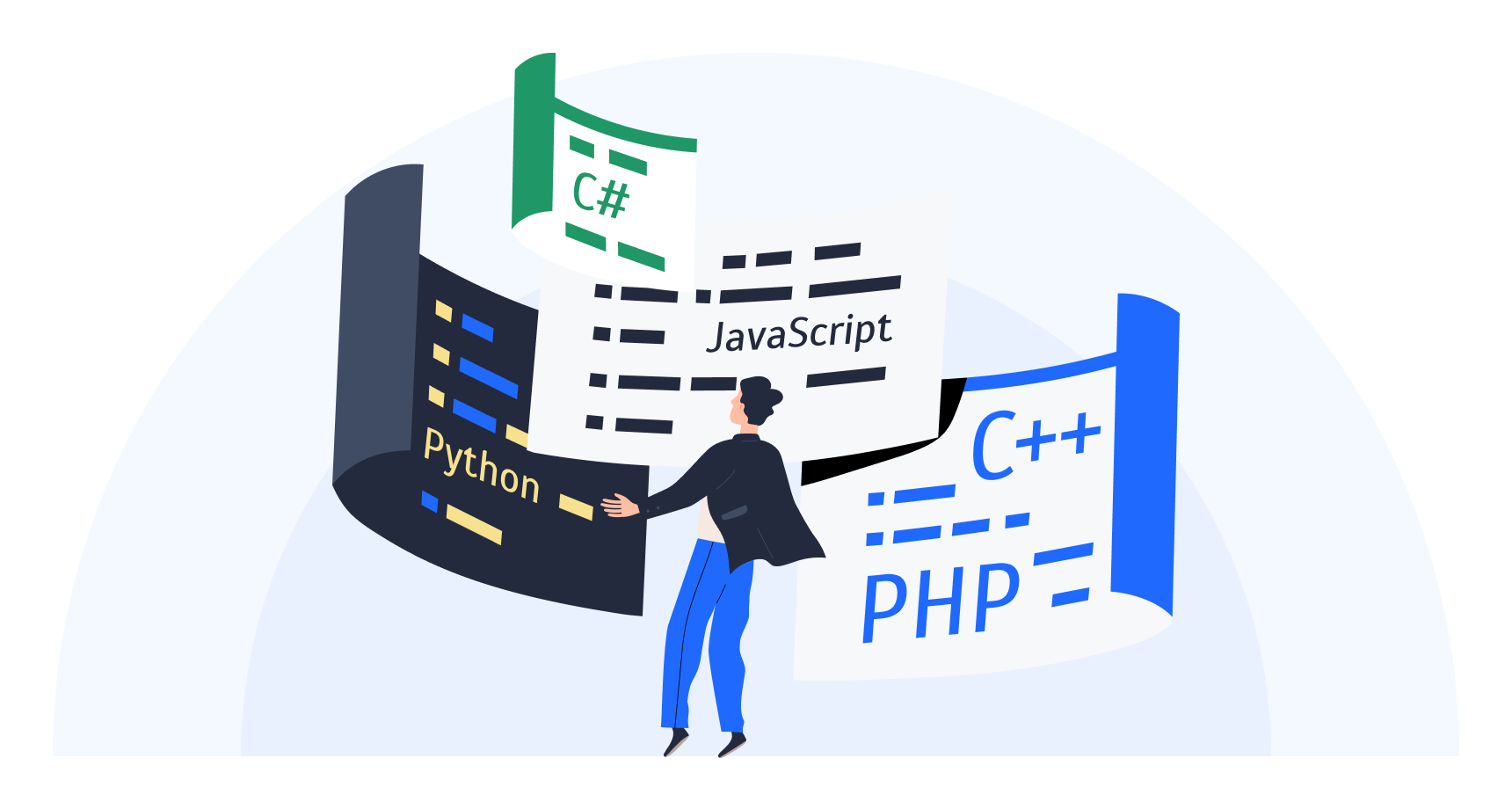
A well-crafted portfolio of data science projects is more than just a collection of your work. It's a powerful tool that:
- Shows your ability to solve real-world problems
- Highlights your technical skills
- Proves you're ready for professional challenges
- Makes up for a lack of formal work experience
By creating various data science projects for your portfolio, you can effectively demonstrate your capabilities to potential employers, even if you don't have any experience . This approach helps bridge the gap between your theoretical knowledge and practical skills.
Why start a data science project?
Simply put, starting a data science project will improve your data science skills and help you start building a solid portfolio of projects. Let's explore how to begin and what tools you'll need.
Steps to start a data science project
- Define your problem : Clearly state what you want to solve .
- Gather and clean your data : Prepare it for analysis.
- Explore your data : Look for patterns and relationships .
Hands-on experience is key to becoming a data scientist. Projects help you:
- Apply what you've learned
- Develop practical skills
- Show your abilities to potential employers
Common tools for building data science projects
To get started, you might want to install:
- Programming languages : Python or R
- Data analysis tools : Jupyter Notebook and SQL
- Version control : Git
- Machine learning and deep learning libraries : Scikit-learn and TensorFlow , respectively, for more advanced data science projects
These tools will help you manage data, analyze it, and keep track of your work.
Overcoming common challenges
New data scientists often struggle with complex datasets and unfamiliar tools. Here's how to address these issues:
- Start small : Begin with simple projects and gradually increase complexity.
- Use online resources : Dataquest offers free guided projects to help you learn.
- Join a community : Online forums and local meetups can provide support and feedback.
Setting up your data science project environment
To make your setup easier :
- Use Anaconda : It includes many necessary tools, like Jupyter Notebook.
- Implement version control: Use Git to track your progress .
Skills to focus on
According to KDnuggets , employers highly value proficiency in SQL, database management, and Python libraries like TensorFlow and Scikit-learn. Including projects that showcase these skills can significantly boost your appeal in the job market.
In this post, we'll explore 21 diverse data science project ideas. These projects are designed to help you build a compelling portfolio, whether you're just starting out or looking to enhance your existing skills. By working on these projects, you'll be better prepared for a successful career in data science.
Choosing the right data science projects for your portfolio
Building a strong data science portfolio is key to showcasing your skills to potential employers. But how do you choose the right projects? Let's break it down.
Balancing personal interests, skills, and market demands
When selecting projects, aim for a mix that :
- Aligns with your interests
- Matches your current skill level
- Highlights in-demand skills
- Projects you're passionate about keep you motivated.
- Those that challenge you help you grow.
- Focusing on sought-after skills makes your portfolio relevant to employers.
For example, if machine learning and data visualization are hot in the job market, including projects that showcase these skills can give you an edge.
A step-by-step approach to selecting data science projects
- Assess your skills : What are you good at? Where can you improve?
- Identify gaps : Look for in-demand skills that interest you but aren't yet in your portfolio.
- Plan your projects : Choose 3-5 substantial projects that cover different stages of the data science workflow. Include everything from data cleaning to applying machine learning models .
- Get feedback and iterate : Regularly ask for input on your projects and make improvements.
Common data science project pitfalls and how to avoid them
Many beginners underestimate the importance of early project stages like data cleaning and exploration. To overcome data science project challeges :
- Spend enough time on data preparation
- Focus on exploratory data analysis to uncover patterns before jumping into modeling
By following these strategies, you'll build a portfolio of data science projects that shows off your range of skills. Each one is an opportunity to sharpen your abilities and demonstrate your potential as a data scientist.
Real learner, real results
Take it from Aleksey Korshuk , who leveraged Dataquest's project-based curriculum to gain practical data science skills and build an impressive portfolio of projects:
The general knowledge that Dataquest provides is easily implemented into your projects and used in practice.
Through hands-on projects, Aleksey gained real-world experience solving complex problems and applying his knowledge effectively. He encourages other learners to stay persistent and make time for consistent learning:
I suggest that everyone set a goal, find friends in communities who share your interests, and work together on cool projects. Don't give up halfway!
Aleksey's journey showcases the power of a project-based approach for anyone looking to build their data skills. By building practical projects and collaborating with others, you can develop in-demand skills and accomplish your goals, just like Aleksey did with Dataquest.
21 Data Science Project Ideas
Excited to dive into a data science project? We've put together a collection of 21 varied projects that are perfect for beginners and apply to real-world scenarios. From analyzing app market data to exploring financial trends, these projects are organized by difficulty level, making it easy for you to choose a project that matches your current skill level while also offering more challenging options to tackle as you progress.
Beginner Data Science Projects
- Profitable App Profiles for the App Store and Google Play Markets
- Exploring Hacker News Posts
- Exploring eBay Car Sales Data
- Finding Heavy Traffic Indicators on I-94
- Storytelling Data Visualization on Exchange Rates
- Clean and Analyze Employee Exit Surveys
- Star Wars Survey
Intermediate Data Science Projects
- Exploring Financial Data using Nasdaq Data Link API
- Popular Data Science Questions
- Investigating Fandango Movie Ratings
- Finding the Best Markets to Advertise In
- Mobile App for Lottery Addiction
- Building a Spam Filter with Naive Bayes
- Winning Jeopardy
Advanced Data Science Projects
- Predicting Heart Disease
- Credit Card Customer Segmentation
- Predicting Insurance Costs
- Classifying Heart Disease
- Predicting Employee Productivity Using Tree Models
- Optimizing Model Prediction
- Predicting Listing Gains in the Indian IPO Market Using TensorFlow
In the following sections, you'll find detailed instructions for each project. We'll cover the tools you'll use and the skills you'll develop. This structured approach will guide you through key data science techniques across various applications.
1. Profitable App Profiles for the App Store and Google Play Markets
Difficulty Level: Beginner
In this beginner-level data science project, you'll step into the role of a data scientist for a company that builds ad-supported mobile apps. Using Python and Jupyter Notebook, you'll analyze real datasets from the Apple App Store and Google Play Store to identify app profiles that attract the most users and generate the highest revenue. By applying data cleaning techniques, conducting exploratory data analysis, and making data-driven recommendations, you'll develop practical skills essential for entry-level data science positions.
Tools and Technologies
- Jupyter Notebook
Prerequisites
To successfully complete this project, you should be comfortable with Python fundamentals such as:
- Variables, data types, lists, and dictionaries
- Writing functions with arguments, return statements, and control flow
- Using conditional logic and loops for data manipulation
- Working with Jupyter Notebook to write, run, and document code
Step-by-Step Instructions
- Open and explore the App Store and Google Play datasets
- Clean the datasets by removing non-English apps and duplicate entries
- Analyze app genres and categories using frequency tables
- Identify app profiles that attract the most users
- Develop data-driven recommendations for the company's next app development project
Expected Outcomes
Upon completing this project, you'll have gained valuable skills and experience, including:
- Cleaning and preparing real-world datasets for analysis using Python
- Conducting exploratory data analysis to identify trends in app markets
- Applying frequency analysis to derive insights from data
- Translating data findings into actionable business recommendations
Relevant Links and Resources
- Example Solution Code
2. Exploring Hacker News Posts
In this beginner-level data science project, you'll analyze a dataset of submissions to Hacker News, a popular technology-focused news aggregator. Using Python and Jupyter Notebook, you'll explore patterns in post creation times, compare engagement levels between different post types, and identify the best times to post for maximum comments. This project will strengthen your skills in data manipulation, analysis, and interpretation, providing valuable experience for aspiring data scientists.
To successfully complete this project, you should be comfortable with Python concepts for data science such as:
- String manipulation and basic text processing
- Working with dates and times using the datetime module
- Using loops to iterate through data collections
- Basic data analysis techniques like calculating averages and sorting
- Creating and manipulating lists and dictionaries
- Load and explore the Hacker News dataset, focusing on post titles and creation times
- Separate and analyze 'Ask HN' and 'Show HN' posts
- Calculate and compare the average number of comments for different post types
- Determine the relationship between post creation time and comment activity
- Identify the optimal times to post for maximum engagement
- Manipulating strings and datetime objects in Python for data analysis
- Calculating and interpreting averages to compare dataset subgroups
- Identifying time-based patterns in user engagement data
- Translating data insights into practical posting strategies
- Original Hacker News Posts dataset on Kaggle
3. Exploring eBay Car Sales Data
In this beginner-level data science project, you'll analyze a dataset of used car listings from eBay Kleinanzeigen, a classifieds section of the German eBay website. Using Python and pandas, you'll clean the data, explore the included listings, and uncover insights about used car prices, popular brands, and the relationships between various car attributes. This project will strengthen your data cleaning and exploratory data analysis skills, providing valuable experience in working with real-world, messy datasets.
To successfully complete this project, you should be comfortable with pandas fundamentals and have experience with:
- Loading and inspecting data using pandas
- Cleaning column names and handling missing data
- Using pandas to filter, sort, and aggregate data
- Creating basic visualizations with pandas
- Handling data type conversions in pandas
- Load the dataset and perform initial data exploration
- Clean column names and convert data types as necessary
- Analyze the distribution of car prices and registration years
- Explore relationships between brand, price, and vehicle type
- Investigate the impact of car age on pricing
- Cleaning and preparing a real-world dataset using pandas
- Performing exploratory data analysis on a large dataset
- Creating data visualizations to communicate findings effectively
- Deriving actionable insights from used car market data
- Original eBay Kleinanzeigen Dataset on Kaggle
4. Finding Heavy Traffic Indicators on I-94
In this beginner-level data science project, you'll analyze a dataset of westbound traffic on the I-94 Interstate highway between Minneapolis and St. Paul, Minnesota. Using Python and popular data visualization libraries, you'll explore traffic volume patterns to identify indicators of heavy traffic. You'll investigate how factors such as time of day, day of the week, weather conditions, and holidays impact traffic volume. This project will enhance your skills in exploratory data analysis and data visualization, providing valuable experience in deriving actionable insights from real-world time series data.
To successfully complete this project, you should be comfortable with data visualization in Python techniques and have experience with:
- Data manipulation and analysis using pandas
- Creating various plot types (line, bar, scatter) with Matplotlib
- Enhancing visualizations using seaborn
- Interpreting time series data and identifying patterns
- Basic statistical concepts like correlation and distribution
- Load and perform initial exploration of the I-94 traffic dataset
- Visualize traffic volume patterns over time using line plots
- Analyze traffic volume distribution by day of the week and time of day
- Investigate the relationship between weather conditions and traffic volume
- Identify and visualize other factors correlated with heavy traffic
- Creating and interpreting complex data visualizations using Matplotlib and seaborn
- Analyzing time series data to uncover temporal patterns and trends
- Using visual exploration techniques to identify correlations in multivariate data
- Communicating data insights effectively through clear, informative plots
- Original Metro Interstate Traffic Volume Data Set
5. Storytelling Data Visualization on Exchange Rates
In this beginner-level data science project, you'll create a storytelling data visualization about Euro exchange rates against the US Dollar. Using Python and Matplotlib, you'll analyze historical exchange rate data from 1999 to 2021, identifying key trends and events that have shaped the Euro-Dollar relationship. You'll apply data visualization principles to clean data, develop a narrative around exchange rate fluctuations, and create an engaging and informative visual story. This project will strengthen your ability to communicate complex financial data insights effectively through visual storytelling.
To successfully complete this project, you should be familiar with storytelling through data visualization techniques and have experience with:
- Creating and customizing plots with Matplotlib
- Applying design principles to enhance data visualizations
- Working with time series data in Python
- Basic understanding of exchange rates and economic indicators
- Load and explore the Euro-Dollar exchange rate dataset
- Clean the data and calculate rolling averages to smooth out fluctuations
- Identify significant trends and events in the exchange rate history
- Develop a narrative that explains key patterns in the data
- Create a polished line plot that tells your exchange rate story
- Crafting a compelling narrative around complex financial data
- Designing clear, informative visualizations that support your story
- Using Matplotlib to create publication-quality line plots with annotations
- Applying color theory and typography to enhance visual communication
- ECB Euro reference exchange rate: US dollar
6. Clean and Analyze Employee Exit Surveys
In this beginner-level data science project, you'll analyze employee exit surveys from the Department of Education, Training and Employment (DETE) and the Technical and Further Education (TAFE) institute in Queensland, Australia. Using Python and pandas, you'll clean messy data, combine datasets, and uncover insights into resignation patterns. You'll investigate factors such as years of service, age groups, and job dissatisfaction to understand why employees leave. This project offers hands-on experience in data cleaning and exploratory analysis, essential skills for aspiring data analysts.
To successfully complete this project, you should be familiar with data cleaning techniques in Python and have experience with:
- Basic pandas operations for data manipulation
- Handling missing data and data type conversions
- Merging and concatenating DataFrames
- Using string methods in pandas for text data cleaning
- Basic data analysis and aggregation techniques
- Load and explore the DETE and TAFE exit survey datasets
- Clean column names and handle missing values in both datasets
- Standardize and combine the "resignation reasons" columns
- Merge the DETE and TAFE datasets for unified analysis
- Analyze resignation reasons and their correlation with employee characteristics
- Applying data cleaning techniques to prepare messy, real-world datasets
- Combining data from multiple sources using pandas merge and concatenate functions
- Creating new categories from existing data to facilitate analysis
- Conducting exploratory data analysis to uncover trends in employee resignations
- DETE Exit Survey Dataset
7. Star Wars Survey
In this beginner-level data science project, you'll analyze survey data about the Star Wars film franchise. Using Python and pandas, you'll clean and explore data collected by FiveThirtyEight to uncover insights about fans' favorite characters, film rankings, and how opinions vary across different demographic groups. You'll practice essential data cleaning techniques like handling missing values and converting data types, while also conducting basic statistical analysis to reveal trends in Star Wars fandom.
To successfully complete this project, you should be familiar with combining, analyzing, and visualizing data while having experience with:
- Converting data types in pandas DataFrames
- Filtering and sorting data
- Basic data aggregation and analysis techniques
- Load the Star Wars survey data and explore its structure
- Analyze the rankings of Star Wars films among respondents
- Explore viewership and character popularity across different demographics
- Investigate the relationship between fan characteristics and their opinions
- Applying data cleaning techniques to prepare survey data for analysis
- Using pandas to explore and manipulate structured data
- Performing basic statistical analysis on categorical and numerical data
- Interpreting survey results to draw meaningful conclusions about fan preferences
- Original Star Wars Survey Data on GitHub
8. Exploring Financial Data using Nasdaq Data Link API
Difficulty Level: Intermediate
In this beginner-friendly data science project, you'll analyze real-world economic data to uncover market trends. Using Python, you'll interact with the Nasdaq Data Link API to retrieve financial datasets, including stock prices and economic indicators. You'll apply data wrangling techniques to clean and structure the data, then use pandas and Matplotlib to analyze and visualize trends in stock performance and economic metrics. This project provides hands-on experience in working with financial APIs and analyzing market data, skills that are highly valuable in data-driven finance roles.
- requests (for API calls)
To successfully complete this project, you should be familiar with working with APIs and web scraping in Python , and have experience with:
- Making HTTP requests and handling responses using the requests library
- Parsing JSON data in Python
- Data manipulation and analysis using pandas DataFrames
- Creating line plots and other basic visualizations with Matplotlib
- Basic understanding of financial terms and concepts
- Set up authentication for the Nasdaq Data Link API
- Retrieve historical stock price data for a chosen company
- Clean and structure the API response data using pandas
- Analyze stock price trends and calculate key statistics
- Fetch and analyze additional economic indicators
- Create visualizations to illustrate relationships between different financial metrics
- Interacting with financial APIs to retrieve real-time and historical market data
- Cleaning and structuring JSON data for analysis using pandas
- Calculating financial metrics such as returns and moving averages
- Creating informative visualizations of stock performance and economic trends
- Nasdaq Data Link API Documentation
9. Popular Data Science Questions
In this beginner-friendly data science project, you'll analyze data from Data Science Stack Exchange to uncover trends in the data science field. You'll identify the most frequently asked questions, popular technologies, and emerging topics. Using SQL and Python, you'll query a database to extract post data, then use pandas to clean and analyze it. You'll visualize trends over time and across different subject areas, gaining insights into the evolving landscape of data science. This project offers hands-on experience in combining SQL, data analysis, and visualization skills to derive actionable insights from a real-world dataset.
To successfully complete this project, you should be familiar with querying databases with SQL and Python and have experience with:
- Writing SQL queries to extract data from relational databases
- Data cleaning and manipulation using pandas DataFrames
- Basic data analysis techniques like grouping and aggregation
- Creating line plots and bar charts with Matplotlib
- Interpreting trends and patterns in data
- Connect to the Data Science Stack Exchange database and explore its structure
- Write SQL queries to extract data on questions, tags, and view counts
- Use pandas to clean the extracted data and prepare it for analysis
- Analyze the distribution of questions across different tags and topics
- Investigate trends in question popularity and topic relevance over time
- Visualize key findings using Matplotlib to illustrate data science trends
- Extracting specific data from a relational database using SQL queries
- Cleaning and preprocessing text data for analysis using pandas
- Identifying trends and patterns in data science topics over time
- Creating meaningful visualizations to communicate insights about the data science field
- Data Science Stack Exchange Data Explorer
10. Investigating Fandango Movie Ratings
In this beginner-friendly data science project, you'll investigate potential bias in Fandango's movie rating system. Following up on a 2015 analysis that found evidence of inflated ratings, you'll compare 2015 and 2016 movie ratings data to determine if Fandango's system has changed. Using Python, you'll perform statistical analysis to compare rating distributions, calculate summary statistics, and visualize changes in rating patterns. This project will strengthen your skills in data manipulation, statistical analysis, and data visualization while addressing a real-world question of rating integrity.
To successfully complete this project, you should be familiar with fundamental statistics concepts and have experience with:
- Data manipulation using pandas (e.g., loading data, filtering, sorting)
- Calculating and interpreting summary statistics in Python
- Creating and customizing plots with matplotlib
- Comparing distributions using statistical methods
- Interpreting results in the context of the research question
- Load the 2015 and 2016 Fandango movie ratings datasets using pandas
- Clean the data and isolate the samples needed for analysis
- Compare the distribution shapes of 2015 and 2016 ratings using kernel density plots
- Calculate and compare summary statistics for both years
- Analyze the frequency of each rating class (e.g., 4.5 stars, 5 stars) for both years
- Determine if there's evidence of a change in Fandango's rating system
- Conducting a comparative analysis of rating distributions using Python
- Applying statistical techniques to investigate potential bias in ratings
- Creating informative visualizations to illustrate changes in rating patterns
- Drawing and communicating data-driven conclusions about rating system integrity
- Original FiveThirtyEight Article on Fandango Ratings
11. Finding the Best Markets to Advertise In
In this beginner-friendly data science project, you'll analyze survey data from freeCodeCamp to determine the best markets for an e-learning company to advertise its programming courses. Using Python and pandas, you'll explore the demographics of new coders, their locations, and their willingness to pay for courses. You'll clean the data, handle outliers, and use frequency analysis to identify countries with the most potential customers. By the end, you'll provide data-driven recommendations on where the company should focus its advertising efforts to maximize its return on investment.
To successfully complete this project, you should have a solid grasp on how to summarize distributions using measures of central tendency, interpret variance using z-scores , and have experience with:
- Filtering and sorting DataFrames
- Handling missing data and outliers
- Calculating summary statistics (mean, median, mode)
- Creating and manipulating new columns based on existing data
- Load the freeCodeCamp 2017 New Coder Survey data
- Identify and handle missing values in the dataset
- Analyze the distribution of participants across different countries
- Calculate the average amount students are willing to pay for courses by country
- Identify and handle outliers in the monthly spending data
- Determine the top countries based on number of potential customers and their spending power
- Cleaning and preprocessing survey data for analysis using pandas
- Applying frequency analysis to identify key markets
- Handling outliers to ensure accurate calculations of spending potential
- Combining multiple factors to make data-driven business recommendations
- freeCodeCamp 2017 New Coder Survey Results
12. Mobile App for Lottery Addiction
In this beginner-friendly data science project, you'll develop the core logic for a mobile app aimed at helping lottery addicts better understand their chances of winning. Using Python, you'll create functions to calculate probabilities for the 6/49 lottery game, including the chances of winning the big prize, any prize, and the expected return on buying a ticket. You'll also compare lottery odds to real-life situations to provide context. This project will strengthen your skills in probability theory, Python programming, and applying mathematical concepts to real-world problems.
To successfully complete this project, you should be familiar with probability fundamentals and have experience with:
- Writing functions in Python with multiple parameters
- Implementing combinatorics calculations (factorials, combinations)
- Working with control structures (if statements, for loops)
- Performing mathematical operations in Python
- Basic set theory and probability concepts
- Implement the factorial and combinations functions for probability calculations
- Create a function to calculate the probability of winning the big prize in a 6/49 lottery
- Develop a function to calculate the probability of winning any prize
- Design a function to compare lottery odds with real-life event probabilities
- Implement a function to calculate the expected return on buying a lottery ticket
- Implementing complex probability calculations using Python functions
- Translating mathematical concepts into practical programming solutions
- Creating user-friendly outputs to effectively communicate probability concepts
- Applying programming skills to address a real-world social issue
13. Building a Spam Filter with Naive Bayes
In this beginner-friendly data science project, you'll build a spam filter using the multinomial Naive Bayes algorithm. Working with the SMS Spam Collection dataset, you'll implement the algorithm from scratch to classify messages as spam or ham (non-spam). You'll calculate word frequencies, prior probabilities, and conditional probabilities to make predictions. This project will deepen your understanding of probabilistic machine learning algorithms, text classification, and the practical application of Bayesian methods in natural language processing.
To successfully complete this project, you should be familiar with conditional probability and have experience with:
- Python programming, including working with dictionaries and lists
- Understand probability concepts like conditional probability and Bayes' theorem
- Text processing techniques (tokenization, lowercasing)
- Pandas for data manipulation
- Understanding of the Naive Bayes algorithm and its assumptions
- Load and explore the SMS Spam Collection dataset
- Preprocess the text data by tokenizing and cleaning the messages
- Calculate the prior probabilities for spam and ham messages
- Compute word frequencies and conditional probabilities
- Implement the Naive Bayes algorithm to classify messages
- Test the model and evaluate its accuracy on unseen data
- Implementing the multinomial Naive Bayes algorithm from scratch
- Applying Bayesian probability calculations in a real-world context
- Preprocessing text data for machine learning applications
- Evaluating a text classification model's performance
- SMS Spam Collection Dataset
14. Winning Jeopardy
In this beginner-friendly data science project, you'll analyze a dataset of Jeopardy questions to uncover patterns that could give you an edge in the game. Using Python and pandas, you'll explore over 200,000 Jeopardy questions and answers, focusing on identifying terms that appear more often in high-value questions. You'll apply text processing techniques, use the chi-squared test to validate your findings, and develop strategies for maximizing your chances of winning. This project will strengthen your data manipulation skills and introduce you to practical applications of natural language processing and statistical testing.
To successfully complete this project, you should be familiar with intermediate statistics concepts like significance and hypothesis testing with experience in:
- String operations and basic regular expressions in Python
- Implementing the chi-squared test for statistical analysis
- Working with CSV files and handling data type conversions
- Basic natural language processing concepts (e.g., tokenization)
- Load the Jeopardy dataset and perform initial data exploration
- Clean and preprocess the data, including normalizing text and converting dollar values
- Implement a function to find the number of times a term appears in questions
- Create a function to compare the frequency of terms in low-value vs. high-value questions
- Apply the chi-squared test to determine if certain terms are statistically significant
- Analyze the results to develop strategies for Jeopardy success
- Processing and analyzing large text datasets using pandas
- Applying statistical tests to validate hypotheses in data analysis
- Implementing custom functions for text analysis and frequency comparisons
- Deriving actionable insights from complex datasets to inform game strategy
- J! Archive - Fan-created archive of Jeopardy! games and players
15. Predicting Heart Disease
Difficulty Level: Advanced
In this challenging but guided data science project, you'll build a K-Nearest Neighbors (KNN) classifier to predict the risk of heart disease. Using a dataset from the UCI Machine Learning Repository, you'll work with patient features such as age, sex, chest pain type, and cholesterol levels to classify patients as having a high or low risk of heart disease. You'll explore the impact of different features on the prediction, optimize the model's performance, and interpret the results to identify key risk factors. This project will strengthen your skills in data preprocessing, exploratory data analysis, and implementing classification algorithms for healthcare applications.
- scikit-learn
To successfully complete this project, you should be familiar with supervised machine learning in Python and have experience with:
- Implementing machine learning workflows with scikit-learn
- Understanding and interpreting classification metrics (accuracy, precision, recall)
- Feature scaling and preprocessing techniques
- Basic data visualization with Matplotlib
- Load and explore the heart disease dataset from the UCI Machine Learning Repository
- Preprocess the data, including handling missing values and scaling features
- Split the data into training and testing sets
- Implement a KNN classifier and evaluate its initial performance
- Optimize the model by tuning the number of neighbors (k)
- Analyze feature importance and their impact on heart disease prediction
- Interpret the results and summarize key findings for healthcare professionals
- Implementing and optimizing a KNN classifier for medical diagnosis
- Evaluating model performance using various metrics in a healthcare context
- Analyzing feature importance in predicting heart disease risk
- Translating machine learning results into actionable healthcare insights
- UCI Machine Learning Repository: Heart Disease Dataset
16. Credit Card Customer Segmentation
In this challenging but guided data science project, you'll perform customer segmentation for a credit card company using unsupervised learning techniques. You'll analyze customer attributes such as credit limit, purchases, cash advances, and payment behaviors to identify distinct groups of credit card users. Using the K-means clustering algorithm, you'll segment customers based on their spending habits and credit usage patterns. This project will strengthen your skills in data preprocessing, exploratory data analysis, and applying machine learning for deriving actionable business insights in the financial sector.
To successfully complete this project, you should be familiar with unsupervised machine learning in Python and have experience with:
- Implementing K-means clustering with scikit-learn
- Feature scaling and dimensionality reduction techniques
- Creating scatter plots and pair plots with Matplotlib and seaborn
- Interpreting clustering results in a business context
- Load and explore the credit card customer dataset
- Perform exploratory data analysis to understand relationships between customer attributes
- Apply principal component analysis (PCA) for dimensionality reduction
- Implement K-means clustering on the transformed data
- Visualize the clusters using scatter plots of the principal components
- Analyze cluster characteristics to develop customer profiles
- Propose targeted strategies for each customer segment
- Applying K-means clustering to segment customers in the financial sector
- Using PCA for dimensionality reduction in high-dimensional datasets
- Interpreting clustering results to derive meaningful customer profiles
- Translating data-driven insights into actionable marketing strategies
- Credit Card Dataset for Clustering on Kaggle
17. Predicting Insurance Costs
In this challenging but guided data science project, you'll predict patient medical insurance costs using linear regression. Working with a dataset containing features such as age, BMI, number of children, smoking status, and region, you'll develop a model to estimate insurance charges. You'll explore the relationships between these factors and insurance costs, handle categorical variables, and interpret the model's coefficients to understand the impact of each feature. This project will strengthen your skills in regression analysis, feature engineering, and deriving actionable insights in the healthcare insurance domain.
To successfully complete this project, you should be familiar with linear regression modeling in Python and have experience with:
- Implementing linear regression models with scikit-learn
- Handling categorical variables (e.g., one-hot encoding)
- Evaluating regression models using metrics like R-squared and RMSE
- Creating scatter plots and correlation heatmaps with seaborn
- Load and explore the insurance cost dataset
- Perform data preprocessing, including handling categorical variables
- Conduct exploratory data analysis to visualize relationships between features and insurance costs
- Create training/testing sets to build and train a linear regression model using scikit-learn
- Make predictions on the test set and evaluate the model's performance
- Visualize the actual vs. predicted values and residuals
- Implementing end-to-end linear regression analysis for cost prediction
- Handling categorical variables in regression models
- Interpreting regression coefficients to derive business insights
- Evaluating model performance and understanding its limitations in healthcare cost prediction
- Medical Cost Personal Datasets on Kaggle
18. Classifying Heart Disease
In this challenging but guided data science project, you'll work with the Cleveland Clinic Foundation heart disease dataset to develop a logistic regression model for predicting heart disease. You'll analyze features such as age, sex, chest pain type, blood pressure, and cholesterol levels to classify patients as having or not having heart disease. Through this project, you'll gain hands-on experience in data preprocessing, model building, and interpretation of results in a medical context, strengthening your skills in classification techniques and feature analysis.
To successfully complete this project, you should be familiar with logistic regression modeling in Python and have experience with:
- Implementing logistic regression models with scikit-learn
- Evaluating classification models using metrics like accuracy, precision, and recall
- Interpreting model coefficients and odds ratios
- Creating confusion matrices and ROC curves with seaborn and Matplotlib
- Load and explore the Cleveland Clinic Foundation heart disease dataset
- Perform data preprocessing, including handling missing values and encoding categorical variables
- Conduct exploratory data analysis to visualize relationships between features and heart disease presence
- Create training/testing sets to build and train a logistic regression model using scikit-learn
- Visualize the ROC curve and calculate the AUC score
- Summarize findings and discuss the model's potential use in medical diagnosis
- Implementing end-to-end logistic regression analysis for medical diagnosis
- Interpreting odds ratios to understand risk factors for heart disease
- Evaluating classification model performance using various metrics
- Communicating the potential and limitations of machine learning in healthcare
19. Predicting Employee Productivity Using Tree Models
In this challenging but guided data science project, you'll analyze employee productivity in a garment factory using tree-based models. You'll work with a dataset containing factors such as team, targeted productivity, style changes, and working hours to predict actual productivity. By implementing both decision trees and random forests, you'll compare their performance and interpret the results to provide actionable insights for improving workforce efficiency. This project will strengthen your skills in tree-based modeling, feature importance analysis, and applying machine learning to solve real-world business problems in manufacturing.
To successfully complete this project, you should be familiar with decision trees and random forest modeling and have experience with:
- Implementing decision trees and random forests with scikit-learn
- Evaluating regression models using metrics like MSE and R-squared
- Interpreting feature importance in tree-based models
- Creating visualizations of tree structures and feature importance with Matplotlib
- Load and explore the employee productivity dataset
- Perform data preprocessing, including handling categorical variables and scaling numerical features
- Create training/testing sets to build and train a decision tree regressor using scikit-learn
- Visualize the decision tree structure and interpret the rules
- Implement a random forest regressor and compare its performance to the decision tree
- Analyze feature importance to identify key factors affecting productivity
- Fine-tune the random forest model using grid search
- Summarize findings and provide recommendations for improving employee productivity
- Implementing and comparing decision trees and random forests for regression tasks
- Interpreting tree structures to understand decision-making processes in productivity prediction
- Analyzing feature importance to identify key drivers of employee productivity
- Applying hyperparameter tuning techniques to optimize model performance
- UCI Machine Learning Repository: Garment Employee Productivity Dataset
20. Optimizing Model Prediction
In this challenging but guided data science project, you'll work on predicting the extent of damage caused by forest fires using the UCI Machine Learning Repository's Forest Fires dataset. You'll analyze features such as temperature, relative humidity, wind speed, and various fire weather indices to estimate the burned area. Using Python and scikit-learn, you'll apply advanced regression techniques, including feature engineering, cross-validation, and regularization, to build and optimize linear regression models. This project will strengthen your skills in model selection, hyperparameter tuning, and interpreting complex model results in an environmental context.
To successfully complete this project, you should be familiar with optimizing machine learning models and have experience with:
- Implementing and evaluating linear regression models using scikit-learn
- Applying cross-validation techniques to assess model performance
- Understanding and implementing regularization methods (Ridge, Lasso)
- Performing hyperparameter tuning using grid search
- Interpreting model coefficients and performance metrics
- Load and explore the Forest Fires dataset, understanding the features and target variable
- Preprocess the data, handling any missing values and encoding categorical variables
- Perform feature engineering, creating interaction terms and polynomial features
- Implement a baseline linear regression model and evaluate its performance
- Apply k-fold cross-validation to get a more robust estimate of model performance
- Implement Ridge and Lasso regression models to address overfitting
- Use grid search with cross-validation to optimize regularization hyperparameters
- Compare the performance of different models using appropriate metrics (e.g., RMSE, R-squared)
- Interpret the final model, identifying the most important features for predicting fire damage
- Visualize the results and discuss the model's limitations and potential improvements
- Implementing advanced regression techniques to optimize model performance
- Applying cross-validation and regularization to prevent overfitting
- Conducting hyperparameter tuning to find the best model configuration
- Interpreting complex model results in the context of environmental science
- UCI Machine Learning Repository: Forest Fires Dataset
21. Predicting Listing Gains in the Indian IPO Market Using TensorFlow
In this challenging but guided data science project, you'll develop a deep learning model using TensorFlow to predict listing gains in the Indian Initial Public Offering (IPO) market. You'll analyze historical IPO data, including features such as issue price, issue size, subscription rates, and market conditions, to forecast the percentage increase in share price on the day of listing. By implementing a neural network classifier, you'll categorize IPOs into different ranges of listing gains. This project will strengthen your skills in deep learning, financial data analysis, and using TensorFlow for real-world predictive modeling tasks in the finance sector.
To successfully complete this project, you should be familiar with deep learning in TensorFlow and have experience with:
- Building and training neural networks using TensorFlow and Keras
- Preprocessing financial data for machine learning tasks
- Implementing classification models and interpreting their results
- Evaluating model performance using metrics like accuracy and confusion matrices
- Basic understanding of IPOs and stock market dynamics
- Load and explore the Indian IPO dataset using pandas
- Preprocess the data, including handling missing values and encoding categorical variables
- Engineer features relevant to IPO performance prediction
- Split the data into training/testing sets then design a neural network architecture using Keras
- Compile and train the model on the training data
- Evaluate the model's performance on the test set
- Fine-tune the model by adjusting hyperparameters and network architecture
- Analyze feature importance using the trained model
- Visualize the results and interpret the model's predictions in the context of IPO investing
- Implementing deep learning models for financial market prediction using TensorFlow
- Preprocessing and engineering features for IPO performance analysis
- Evaluating and interpreting classification results in the context of IPO investments
- Applying deep learning techniques to solve real-world financial forecasting problems
- Securities and Exchange Board of India (SEBI) IPO Statistics
How to Prepare for a Data Science Job
Landing a data science job requires strategic preparation. Here's what you need to know to stand out in this competitive field:
- Research job postings to understand employer expectations
- Develop relevant skills through structured learning
- Build a portfolio of hands-on projects
- Prepare for interviews and optimize your resume
- Commit to continuous learning
Research Job Postings
Start by understanding what employers are looking for. Check out data science job listings on these platforms:
Steps to Get Job-Ready
Focus on these key areas:
- Skill Development: Enhance your programming, data analysis, and machine learning skills. Consider a structured program like Dataquest's Data Scientist in Python path .
- Hands-On Projects: Apply your skills to real projects. This builds your portfolio of data science projects and demonstrates your abilities to potential employers.
- Put Your Portfolio Online: Showcase your projects online. GitHub is an excellent platform for hosting and sharing your work.
Pick Your Top 3 Data Science Projects
Your projects are concrete evidence of your skills. In applications and interviews, highlight your top 3 data science projects that demonstrate:
- Critical thinking
- Technical proficiency
- Problem-solving abilities
We have a ton of great tips on how to create a project portfolio for data science job applications .
Resume and Interview Preparation
Your resume should clearly outline your project experiences and skills . When getting ready for data science interviews , be prepared to discuss your projects in great detail. Practice explaining your work concisely and clearly.
Job Preparation Advice
Preparing for a data science job can be daunting. If you're feeling overwhelmed:
- Remember that everyone starts somewhere
- Connect with mentors for guidance
- Join the Dataquest community for support and feedback on your data science projects
Continuous Learning
Data science is an evolving field. To stay relevant:
- Keep up with industry trends
- Stay curious and open to new technologies
- Look for ways to apply your skills to real-world problems
Preparing for a data science job involves understanding employer expectations, building relevant skills, creating a strong portfolio, refining your resume, preparing for interviews, addressing challenges, and committing to ongoing learning. With dedication and the right approach, you can position yourself for success in this dynamic field.
Data science projects are key to developing your skills and advancing your data science career. Here's why they matter:
- They provide hands-on experience with real-world problems
- They help you build a portfolio to showcase your abilities
- They boost your confidence in handling complex data challenges
In this post, we've explored 21 beginner-friendly data science project ideas ranging from easier to harder. These projects go beyond just technical skills. They're designed to give you practical experience in solving real-world data problems – a crucial asset for any data science professional.
We encourage you to start with any of these beginner data science projects that interests you. Each one is structured to help you apply your skills to realistic scenarios, preparing you for professional data challenges. While some of these projects use SQL, you'll want to check out our post on 10 Exciting SQL Project Ideas for Beginners for dedicated SQL project ideas to add to your data science portfolio of projects.
Hands-on projects are valuable whether you're new to the field or looking to advance your career. Start building your project portfolio today by selecting from the diverse range of ideas we've shared. It's an important step towards achieving your data science career goals.
More learning resources
Applying to business analyst jobs, part 1: the application, 8 tableau jobs that are in-demand in 2024.
Learn data skills 10x faster
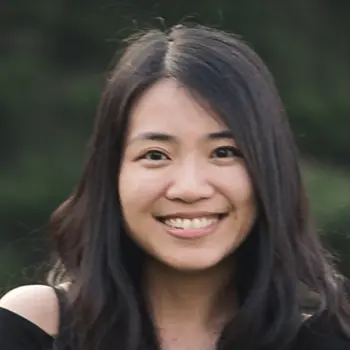
Join 1M+ learners
Enroll for free
- Data Analyst (Python)
- Gen AI (Python)
- Business Analyst (Power BI)
- Business Analyst (Tableau)
- Machine Learning
- Data Analyst (R)

Data Analysis in Research
Ai generator.
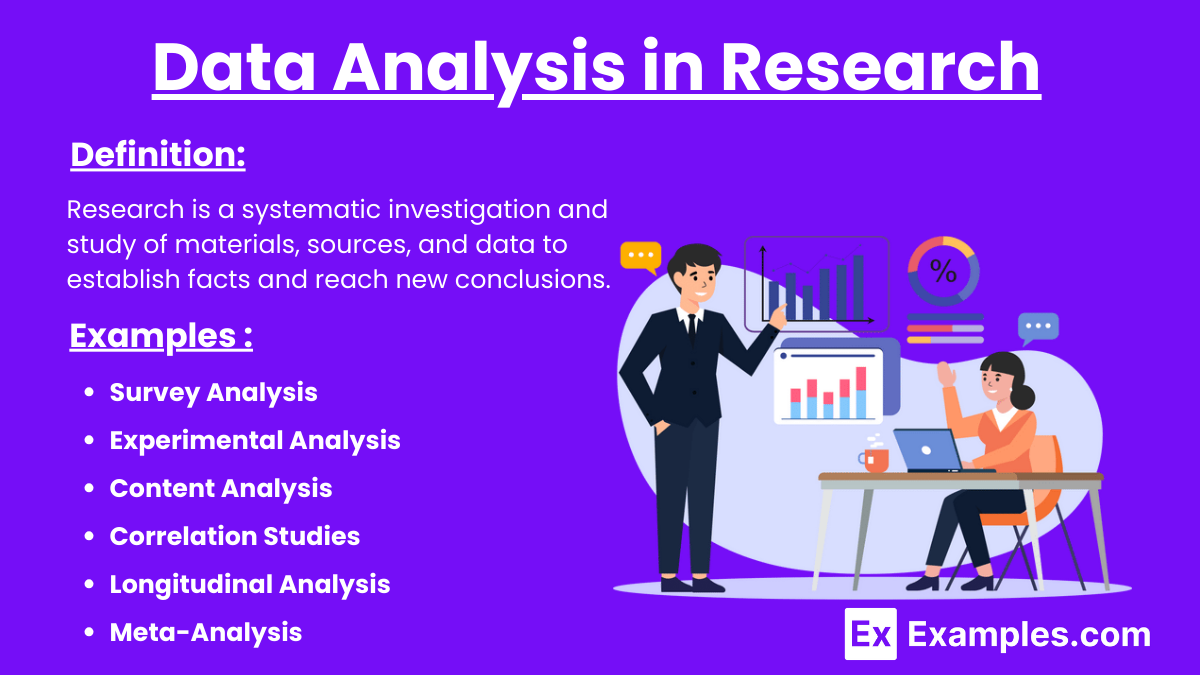
Data analysis in research involves systematically applying statistical and logical techniques to describe, illustrate, condense, and evaluate data. It is a crucial step that enables researchers to identify patterns, relationships, and trends within the data, transforming raw information into valuable insights. Through methods such as descriptive statistics, inferential statistics, and qualitative analysis, researchers can interpret their findings, draw conclusions, and support decision-making processes. An effective data analysis plan and robust methodology ensure the accuracy and reliability of research outcomes, ultimately contributing to the advancement of knowledge across various fields.
What is Data Analysis in Research?
Data analysis in research involves using statistical and logical techniques to describe, summarize, and compare collected data. This includes inspecting, cleaning, transforming, and modeling data to find useful information and support decision-making. Quantitative data provides measurable insights, and a solid research design ensures accuracy and reliability. This process helps validate hypotheses, identify patterns, and make informed conclusions, making it a crucial step in the scientific method.
Examples of Data analysis in Research
- Survey Analysis : Researchers collect survey responses from a sample population to gauge opinions, behaviors, or characteristics. Using descriptive statistics, they summarize the data through means, medians, and modes, and then inferential statistics to generalize findings to a larger population.
- Experimental Analysis : In scientific experiments, researchers manipulate one or more variables to observe the effect on a dependent variable. Data is analyzed using methods such as ANOVA or regression analysis to determine if changes in the independent variable(s) significantly affect the dependent variable.
- Content Analysis : Qualitative research often involves analyzing textual data, such as interview transcripts or open-ended survey responses. Researchers code the data to identify recurring themes, patterns, and categories, providing a deeper understanding of the subject matter.
- Correlation Studies : Researchers explore the relationship between two or more variables using correlation coefficients. For example, a study might examine the correlation between hours of study and academic performance to identify if there is a significant positive relationship.
- Longitudinal Analysis : This type of analysis involves collecting data from the same subjects over a period of time. Researchers analyze this data to observe changes and developments, such as studying the long-term effects of a specific educational intervention on student achievement.
- Meta-Analysis : By combining data from multiple studies, researchers perform a meta-analysis to increase the overall sample size and enhance the reliability of findings. This method helps in synthesizing research results to draw broader conclusions about a particular topic or intervention.
Data analysis in Qualitative Research
Data analysis in qualitative research involves systematically examining non-numeric data, such as interviews, observations, and textual materials, to identify patterns, themes, and meanings. Here are some key steps and methods used in qualitative data analysis:
- Coding : Researchers categorize the data by assigning labels or codes to specific segments of the text. These codes represent themes or concepts relevant to the research question.
- Thematic Analysis : This method involves identifying and analyzing patterns or themes within the data. Researchers review coded data to find recurring topics and construct a coherent narrative around these themes.
- Content Analysis : A systematic approach to categorize verbal or behavioral data to classify, summarize, and tabulate the data. This method often involves counting the frequency of specific words or phrases.
- Narrative Analysis : Researchers focus on the stories and experiences shared by participants, analyzing the structure, content, and context of the narratives to understand how individuals make sense of their experiences.
- Grounded Theory : This method involves generating a theory based on the data collected. Researchers collect and analyze data simultaneously, continually refining and adjusting their theoretical framework as new data emerges.
- Discourse Analysis : Examining language use and communication patterns within the data, researchers analyze how language constructs social realities and power relationships.
- Case Study Analysis : An in-depth analysis of a single case or multiple cases, exploring the complexities and unique aspects of each case to gain a deeper understanding of the phenomenon under study.
Data analysis in Quantitative Research
Data analysis in quantitative research involves the systematic application of statistical techniques to numerical data to identify patterns, relationships, and trends. Here are some common methods used in quantitative data analysis:
- Descriptive Statistics : This includes measures such as mean, median, mode, standard deviation, and range, which summarize and describe the main features of a data set.
- Inferential Statistics : Techniques like t-tests, chi-square tests, and ANOVA (Analysis of Variance) are used to make inferences or generalizations about a population based on a sample.
- Regression Analysis : This method examines the relationship between dependent and independent variables. Simple linear regression analyzes the relationship between two variables, while multiple regression examines the relationship between one dependent variable and several independent variables.
- Correlation Analysis : Researchers use correlation coefficients to measure the strength and direction of the relationship between two variables.
- Factor Analysis : This technique is used to identify underlying relationships between variables by grouping them into factors based on their correlations.
- Cluster Analysis : A method used to group a set of objects or cases into clusters, where objects in the same cluster are more similar to each other than to those in other clusters.
- Hypothesis Testing : This involves testing an assumption or hypothesis about a population parameter. Common tests include z-tests, t-tests, and chi-square tests, which help determine if there is enough evidence to reject the null hypothesis.
- Time Series Analysis : This method analyzes data points collected or recorded at specific time intervals to identify trends, cycles, and seasonal variations.
- Multivariate Analysis : Techniques like MANOVA (Multivariate Analysis of Variance) and PCA (Principal Component Analysis) are used to analyze data that involves multiple variables to understand their effect and relationships.
- Structural Equation Modeling (SEM) : A multivariate statistical analysis technique that is used to analyze structural relationships. This method is a combination of factor analysis and multiple regression analysis and is used to analyze the structural relationship between measured variables and latent constructs.
Data analysis in Research Methodology
Data analysis in research methodology involves the process of systematically applying statistical and logical techniques to describe, condense, recap, and evaluate data. Here are the key components and methods involved:
- Data Preparation : This step includes collecting, cleaning, and organizing raw data. Researchers ensure data quality by handling missing values, removing duplicates, and correcting errors.
- Descriptive Analysis : Researchers use descriptive statistics to summarize the basic features of the data. This includes measures such as mean, median, mode, standard deviation, and graphical representations like histograms and pie charts.
- Inferential Analysis : This involves using statistical tests to make inferences about the population from which the sample was drawn. Common techniques include t-tests, chi-square tests, ANOVA, and regression analysis.
- Qualitative Data Analysis : For non-numeric data, researchers employ methods like coding, thematic analysis, content analysis, narrative analysis, and discourse analysis to identify patterns and themes.
- Quantitative Data Analysis : For numeric data, researchers apply statistical methods such as correlation, regression, factor analysis, cluster analysis, and time series analysis to identify relationships and trends.
- Hypothesis Testing : Researchers test hypotheses using statistical methods to determine whether there is enough evidence to reject the null hypothesis. This involves calculating p-values and confidence intervals.
- Data Interpretation : This step involves interpreting the results of the data analysis. Researchers draw conclusions based on the statistical findings and relate them back to the research questions and objectives.
- Validation and Reliability : Ensuring the validity and reliability of the analysis is crucial. Researchers check for consistency in the results and use methods like cross-validation and reliability testing to confirm their findings.
- Visualization : Effective data visualization techniques, such as charts, graphs, and plots, are used to present the data in a clear and understandable manner, aiding in the interpretation and communication of results.
- Reporting : The final step involves reporting the results in a structured format, often including an introduction, methodology, results, discussion, and conclusion. This report should clearly convey the findings and their implications for the research question.
Types of Data analysis in Research
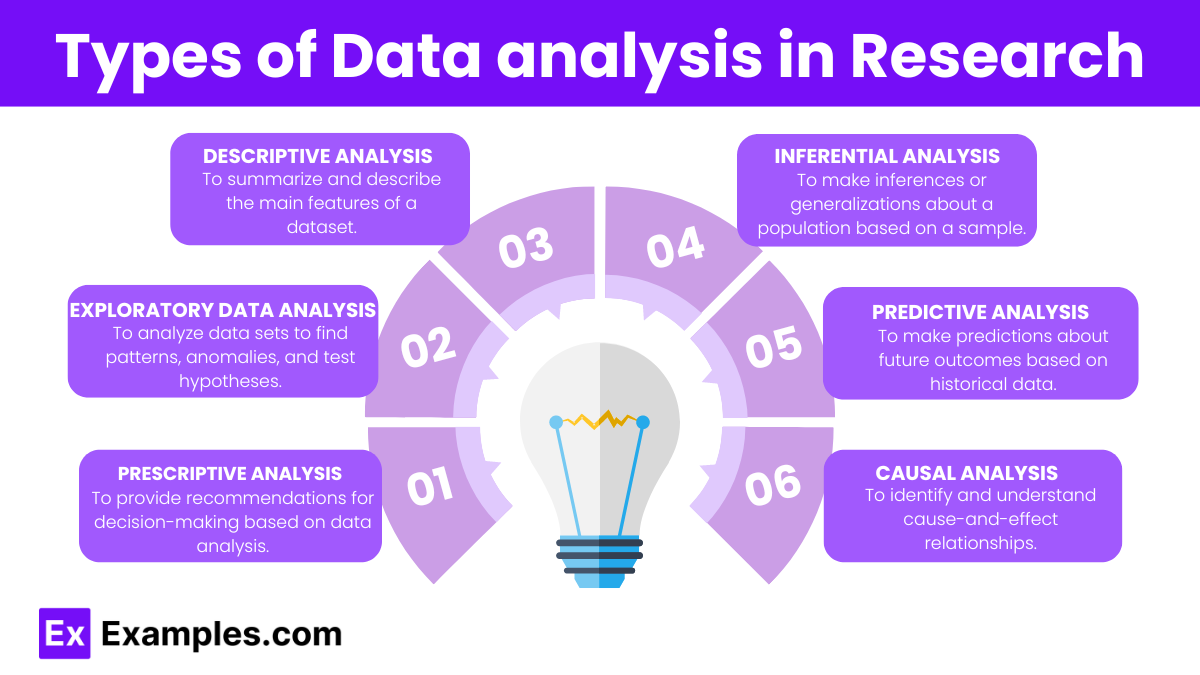
- Purpose : To summarize and describe the main features of a dataset.
- Methods : Mean, median, mode, standard deviation, frequency distributions, and graphical representations like histograms and pie charts.
- Example : Calculating the average test scores of students in a class.
- Purpose : To make inferences or generalizations about a population based on a sample.
- Methods : T-tests, chi-square tests, ANOVA (Analysis of Variance), regression analysis, and confidence intervals.
- Example : Testing whether a new teaching method significantly affects student performance compared to a traditional method.
- Purpose : To analyze data sets to find patterns, anomalies, and test hypotheses.
- Methods : Visualization techniques like box plots, scatter plots, and heat maps; summary statistics.
- Example : Visualizing the relationship between hours of study and exam scores using a scatter plot.
- Purpose : To make predictions about future outcomes based on historical data.
- Methods : Regression analysis, machine learning algorithms (e.g., decision trees, neural networks), and time series analysis.
- Example : Predicting student graduation rates based on their academic performance and demographic data.
- Purpose : To provide recommendations for decision-making based on data analysis.
- Methods : Optimization algorithms, simulation, and decision analysis.
- Example : Suggesting the best course of action for improving student retention rates based on various predictive factors.
- Purpose : To identify and understand cause-and-effect relationships.
- Methods : Controlled experiments, regression analysis, path analysis, and structural equation modeling (SEM).
- Example : Determining the impact of a specific intervention, like a new curriculum, on student learning outcomes.
- Purpose : To understand the specific mechanisms through which variables affect one another.
- Methods : Detailed modeling and simulation, often used in scientific research to understand biological or physical processes.
- Example : Studying how a specific drug interacts with biological pathways to affect patient health.
How to write Data analysis in Research
Data analysis is crucial for interpreting collected data and drawing meaningful conclusions. Follow these steps to write an effective data analysis section in your research.
1. Prepare Your Data
Ensure your data is clean and organized:
- Remove duplicates and irrelevant data.
- Check for errors and correct them.
- Categorize data if necessary.
2. Choose the Right Analysis Method
Select a method that fits your data type and research question:
- Quantitative Data : Use statistical analysis such as t-tests, ANOVA, regression analysis.
- Qualitative Data : Use thematic analysis, content analysis, or narrative analysis.
3. Describe Your Analytical Techniques
Clearly explain the methods you used:
- Software and Tools : Mention any software (e.g., SPSS, NVivo) used.
- Statistical Tests : Detail the statistical tests applied, such as chi-square tests or correlation analysis.
- Qualitative Techniques : Describe coding and theme identification processes.
4. Present Your Findings
Organize your findings logically:
- Use Tables and Figures : Display data in tables, graphs, and charts for clarity.
- Summarize Key Results : Highlight the most significant findings.
- Include Relevant Statistics : Report p-values, confidence intervals, means, and standard deviations.
5. Interpret the Results
Explain what your findings mean in the context of your research:
- Compare with Hypotheses : State whether the results support your hypotheses.
- Relate to Literature : Compare your results with previous studies.
- Discuss Implications : Explain the significance of your findings.
6. Discuss Limitations
Acknowledge any limitations in your data or analysis:
- Sample Size : Note if the sample size was small.
- Biases : Mention any potential biases in data collection.
- External Factors : Discuss any factors that might have influenced the results.
7. Conclude with a Summary
Wrap up your data analysis section:
- Restate Key Findings : Briefly summarize the main results.
- Future Research : Suggest areas for further investigation.
Importance of Data analysis in Research
Data analysis is a fundamental component of the research process. Here are five key points highlighting its importance:
- Enhances Accuracy and Reliability Data analysis ensures that research findings are accurate and reliable. By using statistical techniques, researchers can minimize errors and biases, ensuring that the results are dependable.
- Facilitates Informed Decision-Making Through data analysis, researchers can make informed decisions based on empirical evidence. This is crucial in fields like healthcare, business, and social sciences, where decisions impact policies, strategies, and outcomes.
- Identifies Trends and Patterns Analyzing data helps researchers uncover trends and patterns that might not be immediately visible. These insights can lead to new hypotheses and areas of study, advancing knowledge in the field.
- Supports Hypothesis Testing Data analysis is vital for testing hypotheses. Researchers can use statistical methods to determine whether their hypotheses are supported or refuted, which is essential for validating theories and advancing scientific understanding.
- Provides a Basis for Predictions By analyzing current and historical data, researchers can develop models that predict future outcomes. This predictive capability is valuable in numerous fields, including economics, climate science, and public health.
FAQ’s
What is the difference between qualitative and quantitative data analysis.
Qualitative analysis focuses on non-numerical data to understand concepts, while quantitative analysis deals with numerical data to identify patterns and relationships.
What is descriptive statistics?
Descriptive statistics summarize and describe the features of a data set, including measures like mean, median, mode, and standard deviation.
What is inferential statistics?
Inferential statistics use sample data to make generalizations about a larger population, often through hypothesis testing and confidence intervals.
What is regression analysis?
Regression analysis examines the relationship between dependent and independent variables, helping to predict outcomes and understand variable impacts.
What is the role of software in data analysis?
Software like SPSS, R, and Excel facilitate data analysis by providing tools for statistical calculations, visualization, and data management.
What are data visualization techniques?
Data visualization techniques include charts, graphs, and maps, which help in presenting data insights clearly and effectively.
What is data cleaning?
Data cleaning involves removing errors, inconsistencies, and missing values from a data set to ensure accuracy and reliability in analysis.
What is the significance of sample size in data analysis?
Sample size affects the accuracy and generalizability of results; larger samples generally provide more reliable insights.
How does correlation differ from causation?
Correlation indicates a relationship between variables, while causation implies one variable directly affects the other.
What are the ethical considerations in data analysis?
Ethical considerations include ensuring data privacy, obtaining informed consent, and avoiding data manipulation or misrepresentation.
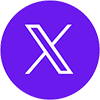
Text prompt
- Instructive
- Professional
10 Examples of Public speaking
20 Examples of Gas lighting
- Sales Questions and Answers
How do I use the Data Visualization Configuration tool?
The Data Visualization Configuration tool helps you visualize Oracle Transactional Business Intelligence (OTBI) reports and saved searches. Using this tool, you can create different visualizations, including bar charts, scatter charts, and more.
Create an OTBI Analysis Report or a Saved Search
Create detailed reports and interactions, design the presentation of your data.
This section covers:
Creating an OTBI analysis report
Creating a Saved Search
For this example, we'll create an OTBI analysis report on Customer Overview in CRM:
- Navigate to Navigator > Tools > Reports and Analytics > Browse Catalog .
- Create a new analysis by navigating to Create > Analysis > CRM-Sales-Customer Overview . You can create the analysis based on any existing areas.
- Select the required details in the relevant Subject Area . Tailor the analysis report by defining report details such as Customer Name, Customer Row ID, and Opportunity ID.
- Select the required details in the Subject Area . You can also add pipeline facts such as Number of Customers and Number of Opportunities.
- Optionally, you can set your styling preferences. Switch to the Results tab and select the Content Paging option.
- Select Enable Alternate Styling and Repeat in Each Row options.
- Once you're satisfied with the report, click Save .
You can create a custom saved search based on existing searches and modify filters according to your requirements. For this example, we’ll create a saved search on demos, based on the My Open Opportunities existing search.
- Navigate to Navigator > Workspace .
- Select Opportunities from the drop-down and select My Open Opportunities .
- Search for demo in the search box.
- Optionally, you can select Set as my default saved search .
- You can define who can access this saved search from the listed access groups. For this example, select Everyone from the drop-down.
- Click Create .
To create an interaction with the visualization, you need to create a custom detailed report and link it to the interaction.
Creating a Detailed Report
- Edit the created analysis report.
- Switch to Criteria tab.
- You can tailor the displayed information. For this example, add Employee Name from the Subject Area options.
- Save this report in the same path as the created OTBI analysis report.
Creating an Interaction
- Click Settings in the Customer Name column, and then select Column Properties .
- Select the Interaction tab.
- Select Action Links in the Primary Interaction area.
- Click Add Action Link .
- Click Create New Action on the New Action Link window.
- Select the Navigate to BI Content option.
- Navigate to the location where the detailed report is stored, and then select it.
- Select Options and ensure that Open in New Window option is selected.
- Select the Do not display in a popup if only one action link is available at runtime option.
- Save the report.
This topic covers:
Creating the visualization from the OTBI analysis
Creating the visualization from the Adaptive Search
Editing or Deleting the Visual Configuration
With the OTBI analysis report and the detailed report, you can now create the Visualization configuration.
- Navigate to Visualization Configuration .
- Add a new configuration by navigating to Navigator > Configurations > Application Composer > Visual Configuration > Add .
- Select the source type as OTBI Analysis .
- Search for and select the created OTBI analysis report.
- You can choose from Visualization types such as Bar chart, Combo chart and more. For this example, select Bar Chart .
- You can change the type of data visualized on the axes, the chart orientation, and layout. For this example, select Name on X-axis and categorize it by Opportunity ID .
- Select the type of orientation for the visualization from the Vertical and Horizontal options.
- You can filter the information based on different column parameters. For example, you can select the Owner parameter and select the Owner’s name .
- Create an interaction by searching for and adding the detailed report.
- Create the visualization.
- Once you’re satisfied with the visualization created, you can set the status as Active .
- You can now interact with the bar chart.
- Select the source type as Adaptive Search .
- Navigate and select the created saved search.
- Configure the visualization as required.
- You can now interact with the visualization to view the detailed visualization insights.
When the visualization is active, Source Type, Visualization, and Source cannot be changed. To make these changes, do the following:
- From the Status drop-down list, select Inactive .
- Click Update .
- From the Status drop-down list, select Draft .
- You can now edit or delete the visualization.
- Click Delete on the configuration draft page.
- Click Delete from the configuration list.
Graduate College
2024 poster presentations, wednesday, july 24 @ iowa memorial union, main lounge.
PRESENTATION SESSION I (1:00 - 1:45 p.m.) | ||||
---|---|---|---|---|
Student Name | Poster Title | Program | Faculty Mentor | Poster Number |
Annie Paik & Seojin Pyoun | Specifying the biochemical properties of the tardigrade damage suppressor (Dsup) protein | Biochemistry Summer Undergraduate Research Fellowship (BSURF) | Todd Washington | 1 |
Aaron Smith | Siritnol’s Cytotoxic Effects in Diffuse Intrinsic Pontine Glioma (DIPG) | Cancer Research Opportunities at Iowa (CROI) | Michelle Howard | 2 |
Abdul Quraishi | Comparative Analysis of Water Quality: University of Iowa Water, Iowa City Water, and River Water | BEST Summer Training Program | Darrin Thompson | 3 |
Adam Benmoussa | The Crystallization and Structure of Human Alternative Replication Protein A | OUR/ICRU Research Fellow | Maria Spies | 4 |
Adam Sayyed | Evaluating The Performance Of LLAMA3 Model | Summer Research Opportunities Program (SROP) | Bijaya Adhikari | 5 |
Adriana M. Rosario-Reyes | Optimizing Bacterial Ghost Production and Loading Ciprofloxacin in Strains for Enhanced Drug Delivery | Summer Research Opportunities Program (SROP) | Chris Vidmar | 6 |
Adriana Novello | Transforming Trauma: Insights from Formerly Incarcerated Survivors on Advocating for Criminal Justice Reform in Gender-Based Violence | Summer Research Opportunities Program (SROP) | Amber Joy Powell | 7 |
Afuosino Oiboh | Examining the relationship between preschoolers' density of African American English usage and ratings of language ability in different language sampling environments | Summer Research Opportunities Program (SROP) | Philip Combiths | 8 |
Aimee Rodriguez | Loss of GPx2 promotes migration of colon cancer cells | Summer Undergraduate MSTP Research Program (SUMR) | Sarah Short | 9 |
Ajwad Iqbal | Role of Complement Cascade in Juvenile Onset Huntington's Disease | BEST Summer Training Program | Peg Nopoulos | 10 |
Alejandra Vilca Landa | Effectiveness of Ascorbate + Ferumoxytol treatment in the reduction of Non-Small cancer lung cell growth | Radiation Biology & Physics Undergraduate Research Diversity Program | Douglas Spitz & Melissa Fath | 11 |
Alex Valdez | Localization of Essential Proteins Potentially Involved in Cell Division in | Microbiology | Craig Ellermeier | 12 |
Aliyah Basham | Metabolic Effects of Cachexia-related Cytokines | Cancer Research Opportunities at Iowa (CROI) | Erin Talbert | 13 |
Allie Marmaras | Sensitizing Keap1 Mutant NSCLC to Auranofin and Ascorbate through Prolyl Hydroxylase Inhibitors | Radiation Biology & Physics Undergraduate Research Diversity Program | Douglas Spitz & Melissa Fath | 14 |
Alyson Honeyman | Optimizing the therapeutic index in pediatric brain cancers | Radiation Biology & Physics Undergraduate Research Diversity Program | Michelle Howard | 15 |
Amrita Gajmer | Effects of Placental Igf-1 Expression on Stereotypical Behaviors and Striatum Structure in Mice. | BEST Summer Training Program | Dr. Stevens / Annemarie J. Carver | 16 |
Analise Pugh | "American Woman": How do Corporate Sponsors Frame the USWNT? | Summer Research Opportunities Program (SROP) | Thomas Oates | 17 |
Angie Li | Investigating the Role of Iron in Growth in the Presence and Absence of Lactoferrin | BEST Summer Training Program | Jennifer Bermick | 18 |
Annika Ellis | Exploring Verbal Interaction during Mealtime Care: An Overview of Verbal Coding for the OPTIMAL Study | OUR/ICRU Research Fellow | Wen Liu | 19 |
Arissa Khan | HALCYON: Differential Energy Measurements of Cosmic Rays as a Function of Altitude | Edge of Space Program | Allison Jaynes | 20 |
Ashlyn Frahm | Livestock-associated Infections in Cystic Fibrosis | Interdisciplinary Evolutionary Biology | Anthony Fischer | 21 |
Avery Babcock | Protective Role of a Macrophage Lysosomal Reductase in Atherogenesis | Biomedical Scholars Summer Undergraduate Research Program (BSSURP) | Ling Yang | 22 |
Ben Otoadese | Categorizing What Healthcare Professionals Touch During Their Processes of Care | Summer Undergraduate MSTP Research Program (SUMR) | Loreen Herwaldt | 23 |
Berkley Barnett | The Integration of Israeli Food into American Jewish Identity | OUR/ICRU Research Fellow | Ari Ariel | 24 |
Brianna Blaine | The Effect of Extracellular Vesicles from Diseased Pregnancies on Human Cortical Organoids | INI Summer Scholars | Serena Banu Gumusoglu | 25 |
Brittany Ramirez | Effects of early life stress on gene expression in the medial prefrontal cortex: Connections with neuropsychiatric disorders and the maternal brain | Biomedical Scholars Summer Undergraduate Research Program (BSSURP) | Hanna Stevens & Rainbo Hultman | 26 |
Bryanna Shao | Effect of mitochondrial-targeted drug mitoquinone on mouse stress vulnerability network | Summer Undergraduate MSTP Research Program (SUMR) | Rainbo Hultman | 27 |
Carrington Mattis | Wartime Restrictions: The Changing Landscape for NGOs in Conflict Zones | Summer Research Opportunities Program (SROP) | Brian Lai | 28 |
Carson Page | Elucidating functions of ICP22 in HSV-1 by analysis of point mutant phenotypes | Microbiology | Richard Roller | 29 |
Cassandra Garcia | Investigating the Methyltransferases Responsible for Hypermethylation Under Hypoxia | Cancer Research Opportunities at Iowa (CROI) | Adam Mailloux | 30 |
Cindy Lara | The Role of Socioeconomic Status in the Development of Children's Externalizing Problems: Examining Parental Acceptance as a Potential Moderator | Summer Research Opportunities Program (SROP) | Isaac T. Petersen | 31 |
Colin Houts | Fluorescent Hydrogels for Implant Infection Control | OUR/ICRU Research Fellow | Eric Nuxoll | 32 |
Connor McMillin | Studying Dark Matter Through Black Hole Geodesics | Non-UI Student, No Program | Vincent Rodgers | 33 |
Danielle Nagaito | Fetal growth restriction is not an indicator of a baby being small for gestational age | BEST Summer Training Program | Mark Santillan | 34 |
Darby Forsyth | Impact of Simulated Heatwave on Pregnant Mice: Insights into Pulmonary Metabolic Function | OUR/ICRU Research Fellow | Laura Dean | 35 |
Dean Omar | Acute Change in Inflammatory Response By Coating Endotracheal Tubes | BEST Summer Training Program | Marlan Hansen | 36 |
Elizabeth Quam | Biochemical Insights into Type IV Pili-Mediated Motility: A Study on Protein-Protein Interactions | Biochemistry Summer Undergraduate Research Fellowship (BSURF) | Ernesto Fuentes | 37 |
Elizabeth Theeler | Examining the Impact of Early Adolescent Bicycle Safety Education on Youth Riding Behavior | OUR/ICRU Research Fellow | Ryan Dusil | 38 |
Ella Sherlock | Clusters of continuous glucose, sleep monitoring, and pain: Identifying phenotypes as a step towards dementia reduction | OUR/ICRU Research Fellow | Alison Anderson | 39 |
Ellie Gardner | Characterization of misexpression in knockdown embryos | Biomedical Scholars Summer Undergraduate Research Program (BSSURP) | Douglas Houston | 40 |
Emma Demuth | Assessing Depression and Anxiety in Participants with Chiari Malformation Before and After Surgery. | OUR/ICRU Research Fellow | Daniel Tranel | 41 |
Erik Sillaste | The Role of Satellite Cell-Derived FGF21 on Skeletal Muscle in Duchenne Muscular Dystrophy | Summer Undergraduate MSTP Research Program (SUMR) | Hongshuai Li | 42 |
Eryka Appiah-Amaning | Disrupting redox regulation in rare pediatric brain tumor | Cancer Research Opportunities at Iowa (CROI) | Michelle Howard | 43 |
Evan Larson | Determining the Correlation Between Polymorphic HLA Class II Genes and the Gut Microbiome in Multiple Sclerosis Patients | Biomedical Scholars Summer Undergraduate Research Program (BSSURP) | Ashutosh Mangalam | 44 |
Fabiola Castaneda-Santiago | How does proximity to old growth forests affect tree diversity in young forests? | Interdisciplinary Evolutionary Biology | Andrew Forbes | 45 |
Faith Fairbairn | Placental overexpression alters cell cycle transition from G1 to S phase in the mouse ganglionic eminence | Biomedical Scholars Summer Undergraduate Research Program (BSSURP) | Hanna Stevens | 46 |
Grace Peil | GPx1 Attenuates Migration and EMT in Colon Cancer Cells | Cancer Research Opportunities at Iowa (CROI) | Sarah Short | 47 |
Grayson Bonilla | Synergy Observed: PARP Inhibitor Combined with Wee1 or HDAC Inhibitors in Serous Endometrial Cancer | Cancer Research Opportunities at Iowa (CROI) | Shujie Yang | 48 |
Hiruni Sumanasiri | Preclinical Models of Gynecologic Cancer from Diverse Populations | Cancer Research Opportunities at Iowa (CROI) | Kristi Thiel | 49 |
Ian Chan | Using Historical Land Patterns to Predict the Spread of Periodical Cicadas Across Johnson County | Interdisciplinary Evolutionary Biology | Andrew Forbes | 50 |
Ian Holtz-Hazeltine | Evaluating the importance of Cathepsin L during Filovirus Glycoprotein mediated entry | Microbiology | Wendy Maury | 51 |
Jake Gioffredi | Mapping a direct DRN-PBel connection: a central piece in the hypercapnic arousal circuit with implications in SUDEP | Biomedical Scholars Summer Undergraduate Research Program (BSSURP) | Gordon Buchanan | 52 |
James Neal | Effects of Mild Traumatic Brain Injury on Basic Cognitive Processing in Mice | Biomedical Scholars Summer Undergraduate Research Program (BSSURP) | Kumar Narayanan | 53 |
Jan Bilbao Del Valle | Using CRISPRi to study essential DNA repair genes in Bacteroides spp. | Microbiology | Dustin Bosch | 54 |
Jessica Miller | Polyphosphate Dynamics and Cellular Responses to Phosphate Starvation in Related Yeast Species | OUR/ICRU Research Fellow | Bin He | 55 |
Johanna Kopelman | The Power of a Hot Lunch: School Lunch Programs, Rural Electrification, and "Modern" Education in 1930s-1940s Iowa | Summer Research Opportunities Program (SROP) | Ashley Howard | 56 |
Lonni Garcia | "Who Would Believe Me?" How Incarcerated Boys Describe Sexual Harassment & Abuse by Female Staff | Summer Research Opportunities Program (SROP) | Amber Joy Powell | 57 |
Makenzie Kennedy | Affective Prosody Perception and Psychopathology | Summer Research Opportunities Program (SROP) | Dorit Kliemann | 58 |
Natalee Kohl | Investigating Histone Modifications in Memory Consolidation via Immunofluorescence | Summer Research Opportunities Program (SROP) | Snehajyoti Chatterjee | 59 |
Taylor Stalnaker | On the tip of the tongue: Left anterior insula damage is associated with category-specific performance deficits in fruit/vegetable naming and fluency | Summer Research Opportunities Program (SROP) | Daniel Tranel | 60 |
PRESENTATION SESSION II (1:50 - 2:35 p.m.) | ||||
---|---|---|---|---|
Student Name | Poster Title | Program | Faculty Mentor | Poster Number |
Anya Nanjappa & Anika Jain | Bridging Impulse Control and Academic Success: The Impact of Teacher and Parent Connections | UI Student, No Program | Isaac T. Petersen | 1 |
Andrew Nguyen | Beam Collimation and Electron Scattering Accessories for FLEX-9 LINAC Delivering Ultra-High Dose Rate Radiotherapy | Radiation Biology & Physics Undergraduate Research Diversity Program | Ryan Flynn | 2 |
Caiden Atienza | Dosimetric Analysis of MR-guided Adaptive Radiotherapy in Head and Neck Cancer | Radiation Biology & Physics Undergraduate Research Diversity Program | Daniel Hyer | 3 |
Cameron Moore | Investigating the Role of the Mitochondrial Calcium Uniporter (MCU) in Mouse Model of Frontotemporal Dementia (FTD) | UI Student, No Program | Yuriy Usachev | 4 |
Elizabeth Castillo | Cross-cultural adaptation and validation of Achilles tendinopathy surveys for Spanish speaking populations | BEST Summer Training Program | Ruth Chimenti | 5 |
Elizabeth Ramirez | Lung Texture Comparison between Computed Tomography and Magnetic Resonance Imaging | BEST Summer Training Program | Dr. Sean Fain; Marrissa McIntosh | 6 |
Ethan Hahlbeck | Assessing the stability of pancreatic lipase and its inhibition by ANGPTL4 | UI Student, No Program | Brandon Davies | 7 |
Evelyn Vega | Building Instrumentation to Measure Height as a Proxy for Biodiversity | Edge of Space Program | Susan Meerdink | 8 |
Gabriel Bulacan | Activation of Natural Killer Cells using JC virus-Positive Plasma | Summer Undergraduate MSTP Research Program (SUMR) | C. Sabrina Tan | 9 |
Gabrielle Bingener | Moral Injury in Mandated Reporting of Prenatal Substance Use | Summer Undergraduate MSTP Research Program (SUMR) | Nichole Nidey | 10 |
Hadrien Lehman | Immunologic and Chest Imaging Abnormalities in Post-Acute Sequelae of COVID-19 | Summer Undergraduate MSTP Research Program (SUMR) | Josalyn Cho | 11 |
Jackie Chitty | Biodiversity of oak gall-associated | Interdisciplinary Evolutionary Biology | Andrew Forbes | 12 |
Jackson Kilburg | Complex formation of coexpressed mutant ANGPTL3 and ANGPTL8 and its ability to inhibit Lipoprotein Lipase | Biochemistry Summer Undergraduate Research Fellowship (BSURF) | Brandon Davies | 13 |
Jayvier Plaza Hernández | Investigating the material state dynamics of Ribonucleoprotein-based membraneless organelles in | Interdisciplinary Evolutionary Biology | Bryan Phillips | 14 |
Johanna "JoJo" Peplinski | Synovia Treated with Carbon Monoxide Demonstrate Decreased Indications of Protein Oxidation | Biomedical Scholars Summer Undergraduate Research Program (BSSURP) | Mitchell C. Coleman | 15 |
Jolcey Santana | Investigating The Contribution of a Unique Immune Cell Population in Inhibiting Malaria | Microbiology | Noah Butler | 16 |
Jordan Mimms | Flattening and Broadening the Flex-9 electron beam for FLASH Studies | Radiation Biology & Physics Undergraduate Research Diversity Program | Joel St-Aubin | 17 |
Jose Suastes | Identifying Influenza Virus Mutations Required for Diverse Host Glycan Receptor Utilization | Microbiology | Balaji Manicassamy | 18 |
Julia Mullane | Identifying Disorder-Relevant Behavioral Differences in Mice with Nonsense Mutations in SCN2A | Biomedical Scholars Summer Undergraduate Research Program (BSSURP) | Aislinn Williams | 19 |
Kaden Bex | Prostaglandin regulation of the nucleoskeleton promotes collective cell migration | Cancer Research Opportunities at Iowa (CROI) | Tina Tootle | 20 |
Kaho Hoshi | Evaluating the role of IK-001, a potent small molecule TEAD inhibitor, as an anti-metastatic agent | Non-UI Student, No Program | Michael Henry | 21 |
Kailey Hogaboom | ISGylation restricts the growth of Serovar D during infection | Microbiology | Mary Weber | 22 |
Kamilla Jacobo | Identifying What Language Persons with Lived Experience of Substance Use Prefer | BEST Summer Training Program | Nichole Nidey | 23 |
Kate Beeman | Locating Midwest Drinking Water Kiosks: A New Dataset for Understanding the Equity of Drinking Water | OUR/ICRU Research Fellow | Samantha Zuhlke | 24 |
Katherine Salmon | Elton John and the Grand Canyon: Measuring Proper Name Retrieval in Cognitively Healthy Individuals | Biomedical Scholars Summer Undergraduate Research Program (BSSURP) | Daniel Tranel | 25 |
Katie Piaszynski | Centriole targeting of inactive Polo kinase drives loss of Drosophila germline stem cells | OUR/ICRU Research Fellow | Pamela Geyer | 26 |
Kayla Vital | What Are The Types of Physical Activity or Exercise Interventions To Improve Quality of Life In Ovarian Cancer Survivors? | Cancer Research Opportunities at Iowa (CROI) | Jessica Gorzelitz | 27 |
Kaylee Serrno | Using Cellphone Signal Strength to Study Corn Plant Drinking Habits. | UI Student, No Program | Anton Kruger & Brian Hornbuckle | 28 |
Kelsey Martin | Evolution of Potential Pioneer Factor Ability Between Orthologous Transcription Factors | Biomedical Scholars Summer Undergraduate Research Program (BSSURP) | Bin He | 29 |
Kennedy Tutak | Genetic and Epigenetic Analysis in Schizophrenia from Human Blood | Biomedical Scholars Summer Undergraduate Research Program (BSSURP) | Marie Gaine | 30 |
Kevin Guo | Preservation of Catalase Activity in Chronic Infections Indicates Continued Exposure to Reactive Oxygen Species | Summer Undergraduate MSTP Research Program (SUMR) | Anthony Fischer | 31 |
Kevin Lu | Manipulation of Thiol Metabolism During Carbon Monoxide Treatment for Cartilage Injury Reveals Zone-specific Responses | Biomedical Scholars Summer Undergraduate Research Program (BSSURP) | Mitchell Coleman | 32 |
KJ Foster-Middleton | Validation of ThermoMPNN as a Tool for Predicting Mutational Free Energy Difference in Genetic Disease | OUR/ICRU Research Fellow | Michael Schnieders | 33 |
Kyle Westhoff | Effects of radiation on cerebral microvessels and mitochondrial activity | OUR/ICRU Research Fellow | Isabella Grumbach | 34 |
Lake Palmeri | Sex Determination in New Zealand Mud Snails: Identifying Genes & Chromosomal Regions with Sex-limited Features | Interdisciplinary Evolutionary Biology | John Logsdon | 35 |
Larissa Cooper | interacts with host cell centrosomes using type III secretion system effectors | Microbiology | Mary Weber | 36 |
Lia Scharnau | RNA processing body biocondensate formation in and | Biomedical Scholars Summer Undergraduate Research Program (BSSURP) | Jan Fassler | 37 |
Lillian Boge | Effect of carbon monoxide entrapping materials on the prevention of intra-abdominal adhesion | Cancer Research Opportunities at Iowa (CROI) | Jianling Bi | 38 |
Lydia Watkins | Auditory Cortex Role in Long-Term Fear Memory with Complex Sounds | INI Summer Scholars | Isabel Muzzio | 39 |
Makenna Eadie | Evaluating the Effect of FOXM1 Overexpression in Malignant Peripheral Nerve Sheath Tumors (MPNSTs) | Cancer Research Opportunities at Iowa (CROI) | Dawn Quelle | 40 |
Mamadu Diallo | The Use of Rotarod Training to Study Stereotyped Behaviors | BEST Summer Training Program | Ted Abel | 41 |
Marielena Chacon-Pacheco | Non-Invasive Detection of Cancer Biomarkers: A Metallomic Approach Using Toenail Samples | Cancer Research Opportunities at Iowa (CROI) | Jong Sung Kim | 42 |
Max Halbach | Murder on Lake Pepin: Contested Constructions of Justice in the Western Great Lakes, 1824-1829 | OUR/ICRU Research Fellow | Stephen Warren | 43 |
Nate Koester | Quantitative Analysis of PCB 11 (3,3’-dichlorobiphenyl) and Hydroxylated Metabolites in the Brain of P21 Wildtype Maternal Mice | OUR/ICRU Research Fellow | Crystal Roach | 44 |
Nathan Barlow | Feasibility of Machine Learning Algorithms to Predict Aortic Stiffness from Radial Pulse Waveforms | OUR/ICRU Research Fellow | Matthew K. Armstrong | 45 |
Nathan Steimel | Impact of PRC2 loss on MPNST sensitivity to MEK and CDK4/6 inhibition | Cancer Research Opportunities at Iowa (CROI) | Rebecca Dodd | 46 |
Nina Sandoval | Studying Signaling Properties of Human Disease Associated TRAF3 Mutations in Cell Line Models | Cancer Research Opportunities at Iowa (CROI) | Bruce Hostager | 47 |
Omar Aristizabal | VIRAL PATHOGENESIS IN THE BRAIN OF RHESUS MACAQUES DURING ACUTE SIV INFECTION TREATED WITH A BI-SPECIFIC ANTIBODY | UI Student, No Program | C. Sabrina Tan | 48 |
Parker Sternhagen | Utility Task Vehicle Crashes and Injuries in Iowa | OUR/ICRU Research Fellow | Charles Jennissen | 49 |
Puja Mekala | LEARNING AND APPLYING RESEARCH METHODS TO SCORE ACTIGRAPHY DATA IN THE PERINATAL PERIOD | BEST Summer Training Program | Kara Whitaker | 50 |
Raha Goodarzi | Effects of Marbach-Schaaf Neurodevelopmental Syndrome (MASNS) on Protein Kinase A (PKA) | BEST Summer Training Program | Stefan Strack | 51 |
Rowan Boulter | Uncovering regulatory mechanisms of autoimmunity through examination of PD-1 inhibitor-induced Bullous pemphigoid. | OUR/ICRU Research Fellow | Kelly Messingham | 52 |
Ruben Delatorre | How Convective Storms Impact Biological Atmospheric Particles | OUR/ICRU Research Fellow | Elizabeth Stone | 53 |
Samantha Anema | Investigating the effect of chronic sleep restriction on tau pathology | Biomedical Scholars Summer Undergraduate Research Program (BSSURP) | Ted Abel | 54 |
Stella Mesch | Examining child sleep problems as a mediator of the association between parent and child internalizing psychopathology | OUR/ICRU Research Fellow | Isaac T. Petersen | 55 |
Sydney Sands | To Explore or to Exploit | OUR/ICRU Research Fellow | Leyre Castro | 56 |
Tina Hong | ADH5 maintains sympathetic innervation and activity in brown adipose tissue | Non-UI Student, No Program | Ling Yang | 57 |
Trinity Janecek | Efficacy testing of novel antifungals against pathogenic mold species to identify candidates for preclinical development | OUR/ICRU Research Fellow | Sarah Beattie | 58 |
Tyler Draayer | Relaxed selection in sperm genes in Camelids: evidence for rapid gene evolution and pseudogenes | OUR/ICRU Research Fellow | John Logsdon Jr. | 59 |
Yihan Shen | Studying Dark Matter Through Black Hole Geodesics | Non-UI Student, No Program | Vincent Rodgers | 60 |
PRESENTATION SESSION III (2:40 - 3:25 p.m.) | ||||
---|---|---|---|---|
Student Name | Poster Title | Program | Faculty Mentor | Poster Number |
Arianna Escandon & Waad Abdella | Quantifying natural variation in algae elemental composition: testing a hypothesis linking ploidy and nutrient availability | Iowa Sciences Academy (ISA) | Briante Najev | 1 |
Seren Castellano & Zoe Marriner | Investigating the Impact of Genetic Background on Evolutionary Potential | Interdisciplinary Evolutionary Biology | Bin He | 2 |
Adriana Castellano | Putting Snails to Sleep for Science: Refining Anesthesia Techniques for Regeneration Research | Iowa Sciences Academy (ISA) | Maurine Neiman | 3 |
Ainsley Rothgeb | Grooming Microstructure in Rats with Lesions of the Lateral Cerebellar Nucleus | Iowa Sciences Academy (ISA) | Krystal Parker | 4 |
Ana Barroso | Regulation and Expression of an Insulin Secretion-Enhancing Micropeptide in CFRD | Iowa Sciences Academy (ISA) | Tate Neff | 5 |
Andrea Medina | Neurophysiological Correlates of Visual Perspective Taking in Theory of Mind: A Literature Review | Iowa Sciences Academy (ISA) | Amanda McCleery | 6 |
Avery Wilson | DNA-RNA hybrids in the rDNA as a source of genomic instability in the germline | Iowa Sciences Academy (ISA) | Sarit Smolikove | 7 |
Bailey Baumert | Brf1-dependent antiviral activity during gammaherpesvirus infection | Microbiology,OUR/ICRU Research Fellow | Jessica Tucker | 8 |
Bhoomika Shettigar | Relations Between Green Space Access and Children’s Cognitive Performance | Iowa Sciences Academy (ISA) | Ece Demir-Lira | 9 |
Caitlin Messingham | Acute malaria reduces the antibody response to the Ebolavirus vaccine | Microbiology,UI Student, No Program | Wendy Maury | 10 |
Elizabeth Barroso | The Role of GSNOR in Bone Marrow Mesenchymal Stem Cell Differentiation | Iowa Sciences Academy (ISA) | Ling Yang | 11 |
Ellie Wojcikowski | Heart Rate Variability (HRV) Along the Schizotypal Spectrum: A Literature Review | OUR/ICRU Research Fellow | Amanda McCleery | 12 |
Jasmyn Hoeger | Translational Control of Cardiac Gene Expression through Alternative Open Reading Frames | Iowa Sciences Academy (ISA) | Ryan Boudreau | 13 |
Jessie Newbanks | Effects of the antioxidant N-acetylcysteine on innate immune response-driven seizures in a fly epilepsy model | Iowa Sciences Academy (ISA) | John Manak | 14 |
Krishna Bharadwaj | phylogenetic TBR1 gene tree for primates | Iowa Sciences Academy (ISA) | Andrew Kitchen | 15 |
Kya Foxx | Exploring the binding properties of KRT32/KRT82 to understand disruption of hair shaft anchoring in Loose Anagen Hair Syndrome | Summer Undergraduate MSTP Research Program (SUMR) | Hatem El-Shanti | 16 |
Kyle Balk | Carbon Monoxide Gas Entrapping Materials Prevent Abdominal Adhesions in a Mouse Model | Radiation Biology & Physics Undergraduate Research Diversity Program | James Byrne | 17 |
Lee Flores | Automated Behavioral Training for Teacher-Student Interactions: Leveraging Large Language Models to Dynamically Generate Assessment and Feedback | Iowa Sciences Academy (ISA) | Tyler Bell | 18 |
Lily Schaefer | Effects of Salt and Pathway Inhibitors on Trachea Epithelial Cell Differentiation | Iowa Sciences Academy (ISA) | Tina Zhang | 19 |
Luke Halligan | Deciphering Alternative Splicing of Sodium Glucose Cotransporter 1 (SGLT1) in Cardiomyocytes | Biomedical Scholars Summer Undergraduate Research Program (BSSURP) | Ferhaan Ahmad | 20 |
Luke Hawkins | Enhancing Feedback-Informed Group Therapy Using Machine Learning | Iowa Sciences Academy (ISA) | Martin Kivlighan | 21 |
Lydia Karr | Comparing and contrasting current methods used to assign behavior states to human infants aged 0-6 months | Iowa Sciences Academy (ISA) | Greta Sokoloff & Mark Blumberg | 22 |
Mark Schultz | Optimization of NPC1 over-expression to mimic endogenous proteostasis | Biomedical Scholars Summer Undergraduate Research Program (BSSURP) | Mark Schultz | 23 |
Matthew Lopez | Exploring the role of sRNAs in modulating CsrA activity in | Microbiology | Michael Gebhardt | 24 |
Max Casini | The Effects of Stimulant Medication on the Social Behavior of Children With Attention-Deficit/Hyperactivity Disorder (ADHD) | Iowa Sciences Academy (ISA) | Matthew O'Brien | 25 |
Mostafa Telfah | Analyzing motor cortex neural activity in Parkinson's disease patients and healthy participants performing an interval timing task | INI Summer Scholars | Rodica Curtu | 26 |
Nabil Othman | Puff and Run: How PPL2 Dopaminergic Neurons Regulate Arousal States in | Summer Undergraduate MSTP Research Program (SUMR) | Seth Tomchik | 27 |
Natalie Brown | Project Gaea | Edge of Space Program | Susan Meerdink | 28 |
Natalie Kehrli | Tracking Physical Activity in Severe Mental Illness: Insights From Pedometry-Based Studies | Iowa Sciences Academy (ISA) | Amanda McCleery | 29 |
Nathan Schwartz | Mechanisms of cell death in mouse bone marrow derived macrophages following ionizing radiation | Biomedical Scholars Summer Undergraduate Research Program (BSSURP) | Prajwal Gurung | 30 |
Nikhil Anand | Progestin treatment of endometrial cancer: Using CRISPR to determine the role of non-canonical steroid-hormone receptors. | Biomedical Scholars Summer Undergraduate Research Program (BSSURP) | Miles Pufall | 31 |
Njenga Kamau | Evaluating the Utility of Iron Oxide Nanoparticles for Pre-Clinical Radiation Dose Estimation | Radiation Biology & Physics Undergraduate Research Diversity Program | Michael Petronek | 32 |
Noah Sanders | Expanding Dataset's Diversity and Inclusion for Lung Cancer Prediction Using Repeatable Semi-automated Segmentations of Pulmonary Nodules | Cancer Research Opportunities at Iowa (CROI) | Jessica Sieren | 33 |
Nohshin Nafisa | Simulation of Rationally Designed Anisotropic and Auxetic Hydrogel Patches to Predict Property Materials | Summer Undergraduate MSTP Research Program (SUMR) | Shaoping Xiao | 34 |
Olivia Frary | Sex Determination in New Zealand Mud Snails: Identifying Genes & Chromosomal Regions with Sex-limited Features | Interdisciplinary Evolutionary Biology | John Logsdon | 35 |
Paige Wiebke | A new mouse model for the vision disorder Retinitis Pigmentosa | UI Student, No Program | Lori Wallrath | 36 |
Pasi Jouhikainen | Continuous Rating of Basic Emotional Dimensions | UI Student, No Program | Dorit Kliemann | 37 |
Paul Roscoe | Characterizing Human RDM1: A Novel Anticancer Target | Biochemistry Summer Undergraduate Research Fellowship (BSURF) | Maria Spies | 38 |
Rachel Brown | Comparing Demographics and Cardiovascular Disease Risk Factors Between Endometrial Cancer Survivors and Healthy Postmenopausal Women | Cancer Research Opportunities at Iowa (CROI) | Jessica Gorzelitz | 39 |
Riaz Meah | New Insights into Popular Cancer Drugs: Visualizing PARP1 Activity and Inhibition on DNA G-Quadruplexes | Cancer Research Opportunities at Iowa (CROI) | Maria Spies | 40 |
Ryan Kiddle | Determinants of Colorectal Cancer Metastasis Colonization by Commensal and Pathobiont Bacteria | Cancer Research Opportunities at Iowa (CROI) | Dustin Bosch | 41 |
Rylie Elbert | The Impact of RNA Polymerase III Transcription on MHV68 Spread | Microbiology | Jessica Tucker | 42 |
Samantha Mock | Beneficial Suppresses Tumorigenic Effects of Pathobiont in an in vitro Colonic Epithelial Cancer Cell Model | Cancer Research Opportunities at Iowa (CROI) | Ashutosh Mangalam | 43 |
Sana Nadeem | Impact of Long-Term SSRI Use and Pregnancy on Mood and Cognition in Mice with and without Preeclampsia | BEST Summer Training Program | Serena Banu Gumusoglu | 44 |
Sara Lastine | Archetypal Analysis for Categorizing Poetry Lines Based on Psychological Trait Symptoms | BEST Summer Training Program | Jacobs Michaelson | 45 |
Sarah Sheitelman | Adipokine Changes in Women with Pregnancy Complications Affect Microvessel Function | BEST Summer Training Program | Anna Stanhewicz | 46 |
Semaj Willis | Evaluation of Title V Community-Based Doula Project for African American Families | BEST Summer Training Program | DeShauna Jones | 47 |
Shanmukh Boggarapu | Placental Igf-1 Overexpression Alters Striatal Development Embryonically and Postnatally in Mice | Non-UI Student, No Program | Hanna Stevens | 48 |
Sophia Heim | Accelerating Knowledge Discovery: Harnessing the Power of Artificial Intelligence for Systematic Reviews | UI Student, No Program | Hans-Joachim Lehmler | 49 |
Sorayya Nazari | Impact of Fibroblast Growth Factor Receptor 1 on Breast Cancer | Cancer Research Opportunities at Iowa (CROI) | Michael K Wendt | 50 |
Spencer Yates | Profiling the Immune Antagonistic Properties of MERS-CoV ORF5 Protein | Microbiology | Stanley Perlman | 51 |
Sunny Fieser | Methodology for Studying Alzheimer's Disease in Cultured Neurons | Iowa Sciences Academy (ISA) | Marco Hefti | 52 |
Taylar Simmons | Optimization of NPC1 over-expression to mimic endogenous proteostasis | Biomedical Scholars Summer Undergraduate Research Program (BSSURP) | Mark Schultz | 53 |
Thomas Hart | Anxiety Symptom Severity Is Associated with Increased Arterial Blood Pressure and Arterial Blood Pressure Variability in Apparently Healthy Young Adults. | BEST Summer Training Program,OUR/ICRU Research Fellow | Nathaniel Jenkins | 54 |
Vinny Chloros | Snail Parents & Nutrition: Are Reproductive Snails Depositing Their Bodily Phosphorous to Their Offspring? | Interdisciplinary Evolutionary Biology | Maurine Neiman | 55 |
Viviana Ramirez | Exploring Sleep Patterns and Cognitive Performance in Older Adults: Regularity, Midpoint, and Sleep Tracking Apps | OUR/ICRU Research Fellow | Chooza Moon | 56 |
Xandra McGlasson | Can Your Car Be a Cardiac Monitor? | OUR/ICRU Research Fellow | Deema Totah | 57 |
Yiping Geng | The Role of Myeloid-Derived Mononuclear Phagocytes in Early Ebola Virus Infection. | Biomedical Scholars Summer Undergraduate Research Program (BSSURP) | Wendy Maury | 58 |
Zachary Darr | SMAD7 to the rescue: Curing Emery-Dreifuss muscular dystrophy | OUR/ICRU Research Fellow | Lori Wallrath | 59 |
Zachary Minthorn | Does genome architecture influence susceptibility to nutrient limitation? | Interdisciplinary Evolutionary Biology | Maurine Neiman | 60 |
Thank you for visiting nature.com. You are using a browser version with limited support for CSS. To obtain the best experience, we recommend you use a more up to date browser (or turn off compatibility mode in Internet Explorer). In the meantime, to ensure continued support, we are displaying the site without styles and JavaScript.
- View all journals
- Explore content
- About the journal
- Publish with us
- Sign up for alerts
- Open access
- Published: 19 July 2024
Mutations in glioblastoma proteins do not disrupt epitope presentation and recognition, maintaining a specific CD8 T cell immune response potential
- Renata Fioravanti Tarabini 1 ,
- Gustavo Fioravanti Vieira 3 , 4 ,
- Maurício Menegatti Rigo 2 nAff5 &
- Ana Paula Duarte de Souza 1
Scientific Reports volume 14 , Article number: 16721 ( 2024 ) Cite this article
Metrics details
- Computational biology and bioinformatics
Antigen-specific cytotoxic CD8 T cells are extremely effective in controlling tumor growth and have been the focus of immunotherapy approaches. We leverage in silico tools to investigate whether the occurrence of mutations in proteins previously described as immunogenic and highly expressed by glioblastoma multiforme (GBM), such as Epidermal Growth Factor Receptor (EGFR), Isocitrate Dehydrogenase 1 (IDH1), Phosphatase and Tensin homolog (PTEN) and Tumor Protein 53 (TP53), may be contributing to the differential presentation of immunogenic epitopes. We recovered Class I MHC binding information from wild-type and mutated proteins using the Immune Epitope Database (IEDB). After that, we built peptide-MHC (pMHC-I) models in HLA-arena, followed by hierarchical clustering analysis based on electrostatic surface features from each complex. We identified point mutations that are determinants for the presentation of a set of peptides from TP53 protein. We point to structural features in the pMHC-I complexes of wild-type and mutated peptides, which may play a role in the recognition of CD8 T cells. To further explore these features, we performed 100 ns molecular dynamics simulations for the peptide pairs (wt/mut) selected. In pursuit of novel therapeutic targets for GBM treatment, we selected peptides where our predictive results indicated that mutations would not disrupt epitope presentation, thereby maintaining a specific CD8 T cell immune response. These peptides hold potential for future GBM interventions, including peptide-based or mRNA vaccine development applications.
Introduction
Glioblastoma multiforme (GBM) is the most common and aggressive form of tumor of the central nervous system (CNS) with poor prognosis and high levels of morbidity and mortality 1 . GBM incidence in adults is 3.7 per 100,000 person-years 2 , and only 2.2% of patients are estimated to survive three years or more after diagnosis 3 . In children, GBM accounts for approximately 8–12% of all primary CNS tumors, and about 25 percent of children with this tumor live for five years or more 4 . Unfortunately, therapeutic options for these tumors are still minimal.
GBM is characterized by intra- and intertumoral heterogeneity, highly invasive cellular properties, and an immunosuppressive microenvironment that promotes GBM growth through complex interactions 5 . Patients with GBM considered long-term survivors have more significant infiltration of CD8 T cells than short-term survivors, positively correlating CD8 T cells with a better survival rate 6 . Based on this knowledge, immunotherapy emerges as a promising therapeutic approach 7 .
T-cell-mediated immunotherapy has shown promise in clinical trials for cancer. The effectiveness of immunotherapy based on the T cell response depends on the stimulatory context and the adequate choice of tumor antigen to be used, more precisely on the T cell epitopes contained in these tumor proteins 8 . Peptides stably bound to MHC-I, also called epitopes, will be presented on the cell's surface for later recognition by the T-cell receptor (TCR). CD8 T cells that recognize these epitopes exert direct effector functions, producing inflammatory or regulatory cytokines and promoting cytotoxicity. Also, CD8 T cell response can generate long-term memory populations allowing the host to respond rapidly to subsequent encounters of the same epitope 9 .
There are several immunogenic CD8 T cell epitopes described for GBM, especially in the highly expressed proteins, such as Epidermal Growth Factor Receptor (EGFR), Isocitrate Dehydrogenase 1 (IDH1), Phosphatase and Tensin homolog (PTEN), and Tumor Protein 53 (TP53). However, tumor progression occurs regardless of the presence of T cell-mediated response, showing the high capacity of this type of tumor to escape from the immune surveillance mechanisms 10 and induce immunosuppression. For instance, GBM can create a highly immunosuppressive microenvironment characterized by the presence of cells like regulatory T cells (Tregs), myeloid-derived suppressor cells (MDSCs), and M2-polarized macrophages 11 . The expression of specific checkpoint molecules (e.g., PD-L1) can also lead to T cell exhaustion and inhibition of T cell cytotoxic function 12 . Another important aspect is that the tumor proteins constantly mutate, which may affect the recognition of immunogenic epitopes 13 , 14 .
In this study, we focused on the occurrence of mutations in proteins previously described as immunogenic and highly expressed by GBM, such as TP53, PTEN, EGFR, and IDH1. We investigated the role of prominent mutations on the disappearance or differential presentation of immunogenic epitopes. We used the combined MHC-I intracellular pathway prediction tools from the Immune Epitope Database (IEDB) 15 , 16 , alongside machine learning (ML) methods for pMHC-I immunogenicity prediction 17 . Furthermore, we leverage HLA-Arena 18 to create and execute workflows for structural modeling, analysis, and visualization of pMHC-I complexes. We identified six GBM peptides from TP53 protein whose presentation remains unaffected by mutations, as consistently predicted by in silico tools. To further validate these findings, we performed 100 ns of molecular dynamics simulation on these six peptide pairs (wt/mut). The simulation results supported the stability and binding potential of these peptides, reinforcing their promise as targets for the development of immunotherapeutic strategies in GBM treatment, potentially paving the way for more effective clinical interventions.
Immunogenic epitopes containing missense mutations can still be generated through MHC-I pathway
We looked for missense mutations on the TCGA-GDC cancer database, and we found that the EGFR, IDH1, PTEN, and TP53 proteins were the most frequently mutated proteins in GBM. A total of 49, 5, 57, and 64 missense mutations were uncovered for EGFR, IDH1, PTEN, and TP53, respectively (Supplementary Table S1 ). From this list of mutations, 4 occurred in immunogenic epitopes of EGFR, 1 in IDH1, 2 in PTEN, and 22 in TP53 (Supplementary Table S2 ). Immunogenic epitopes were recovered from IEDB.
Next, we used the combined predictors of proteasomal processing, TAP transport, and MHC binding to score and identify the probability of presentation of the mutated epitopes in selected proteins. The total score for wild-type and mutated epitopes and their respective upstream/downstream epitopes were compared (Supplementary Figs. S1 and S2 ). Our analysis revealed no significant differences in the mean total scores of mutated epitopes compared to their respective wild-type counterparts. This suggests that the missense mutations do not impact the presentation of these epitopes. However, when directly comparing the pair of wild-type and mutated epitopes we found that in 39 pairs, the wild-type and mutated epitope total scores were below the mean total score, and the difference in the total score between the wild-type and the mutated epitope was higher than 0.5 (arbitrary value). Because this result suggests that the mutation might influence the peptide generation in the processing pathway, these 39 pairs were not considered for downstream analysis. We selected the 83 remaining pairs for further structural analysis.
Structural analysis of MHCs in the context of wild-type and mutated epitopes
As explained in the Materials and Methods section, we kept the same MHCflurry cutoff value for both wild-type and mutant epitopes. Because HLA-Arena models only pMHC-I complexes that pass through MHCflurry cutoff, there were cases where the pair (wild-type/mutant) was not modeled (Supplementary Table S3 ). In our case, 24 pairs out of 83 were modeled in the context of different HLA molecules (Table 1 ) and used for downstream analyses.
To evaluate the similarity of the TCR-interacting surface of the remaining 24 pMHC-I complex pairs, we performed a hierarchical clustering analysis (HCA) of the electrostatic potential collected from 46 regions of interest (Supplementary Fig. S3 ). The use of HCA for selection of similar pMHC-I pairs based on electrostatic features was validated elsewhere 19 , 20 , 21 . Figure 1 A shows the HCA for TP53-derived peptides, while Fig. 1 B illustrates the image similarity between two distinct peptide-MHC class I (pMHC-I) pairs (wild-type/mutant). Although qualitative differences and similarities in the pMHC-I complexes can be observed, the HCA offers a quantitative approach to emphasize these characteristics more distinctly, at the same time allowing the clustering of similar pairs. For TP53 epitopes, 8 pairs of pMHC complexes were clustered together.
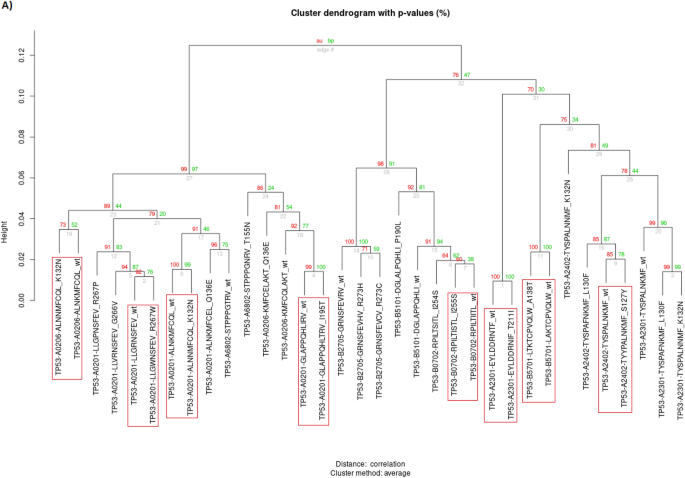
HCA of the electrostatic potential and exemplification of their electrostatic and structural similarity. ( A ) Dendrogram of the TP53 protein demonstrating the pairs of pMHC complexes, the red rectangles indicate the pairs preserving structural characteristics. ( B ) Top view of pMHC complexes highlighting the electrostatic similarity between wild-type and mutated epitopes from TP53 protein (red, white, and blue represent negative, neutral, and positive charges, respectively). AU, Approximately Unbiased; BP, Bootstrap Probability.
The HCA results for IDH1 proteins showed a different pattern, positioning the wild-type epitope on a distant branch compared to the mutated epitopes. Since we are interested in the pair of epitopes where the wild-type is structurally similar to the mutated counterpart, we did not pursue additional analysis on IDH1 epitopes (Fig. 2 ).
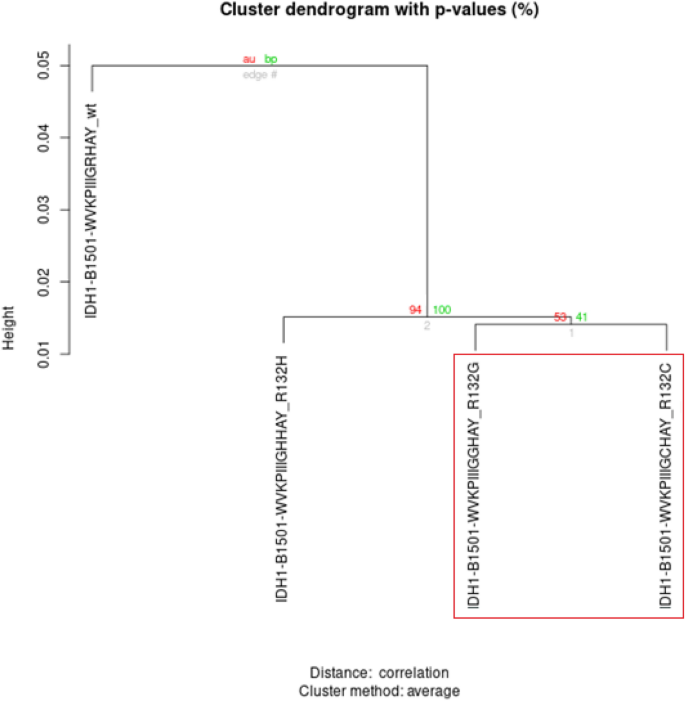
Dendrogram illustrating the HCA of the IDH1 protein, focusing on four pMHC complexes: WVKPIIIGRHAY-WT, WVKPIIIGHHAY-R132H, WVKPIIIGGHAY-R132G, and WVKPIIIGCHAY-R132C. The analysis reveals distinct clustering, with the mutated pMHC complexes R132G and R132C forming one cluster, followed by the appearance of R132H. The wild-type (WT) complex is positioned as the most distant group, highlighting the significant variance from the mutated forms.
Filtering targeted epitopes for GBM therapy
To pursue new targets for GBM therapy, we selected only the epitope pairs that presented better-predicted results in all analyses (i.e., sequence-based prediction and HCA–based structural analysis). The rationale behind this approach is to select epitopes where the described mutation has a lower chance of affecting the CD8 T cell-specific immune response. In total, six TP53 epitopes stand out (Table 2 ). Since immunogenicity is crucial, we submitted each pMHC-I to an immunogenicity prediction tool (TLImm) 17 . We observed that the scores were similar to or even better (3 out of 6 cases) than the well-known immunogenic wild-type peptide.
To further explore structural features in a dynamic environment, we decided to run 100 ns molecular dynamics simulation for all 12 pMHC-I complexes selected from Table 2 . We extracted the main quantitative features from each simulation, such as Root Mean Square Deviation (RMSD), Root Mean Square Fluctuation (RMSF), Radius of Gyration (RoG), and mean contacts between the epitope and the MHC-I (Table 3 ).
One of the measures reflecting pMHC-I stability is the RMSD. In most cases, the average RMSD for both the protein and epitope was lower for the mutated peptide (Table 3 ). The effect size of this difference, quantified using Cohen’s d descriptor, ranged from medium to huge (see details in the Methods section). In all simulations, the RMSD reached a plateau before the 100 ns mark, indicating that the simulation time was appropriate (Fig. 3 ). Interestingly, the density plot analysis of the protein RMSD revealed primarily single peaks, except for the HLA-A*02:01-LLGWNSFEV complex, which displayed two distinct peaks. When we analyzed the epitope RMSD we could observe that more pMHC-I complexes showed multiple peaks, likely because the calculation involved only 9 to 10 residues (Supplementary Fig. S4 ). Nonetheless, the epitope RMSD remained stable throughout the simulation in all cases.
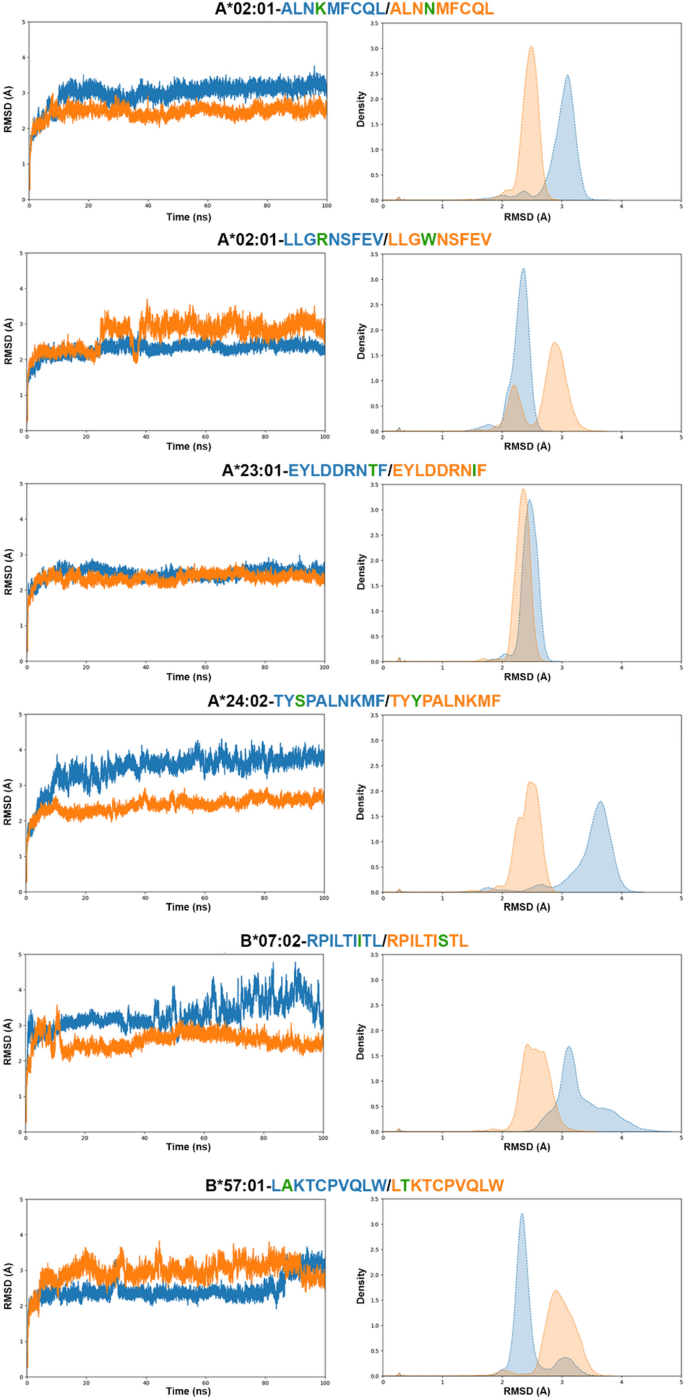
Protein RMSD (in Å) for 6 selected pMHC-I pairs. The graphs on the left show the RMSD along time (in nanoseconds), while the graphs on the right shows the RMSD density plot for the whole simulation. Wild-type peptides are colored in blue, while the mutated counterpart is colored in orange. The mutated residue is shown in green.
We were also interested in evaluating the free energy surface (FES) landscape for each of the pairs analyzed (Figs. 4 and 5 ). The FES can be used to understand the stability and conformational changes of molecular systems because it is derived from RMSD and RoG values. Complexes HLA-A*02:01-ALNKMFCQL/ALNNMFCQL and HLA-A*23:01-EYLDDRNTF/EYLDDRNIF show a slightly different distribution of energy minima, but FES plots support the hypothesis that the mutation in these cases does not drastically destabilize the peptide-MHC complex. Other complexes show a more complex scenario, indicating that the mutation can introduce alternative conformations (e.g., HLA-A*02:01-LLGRNSFEV/LLGWNSFEV, which aligns with the protein RMSD plots) or additional flexibility (e.g., HLA-B*07:02-ALNKMFCQL/ALNNMFCQL, consistent with the epitope RMSF values in Table 3 ). Still, and supported by RMSD analysis, the mutation was not sufficient to destabilize any of the pMHC complexes analyzed.
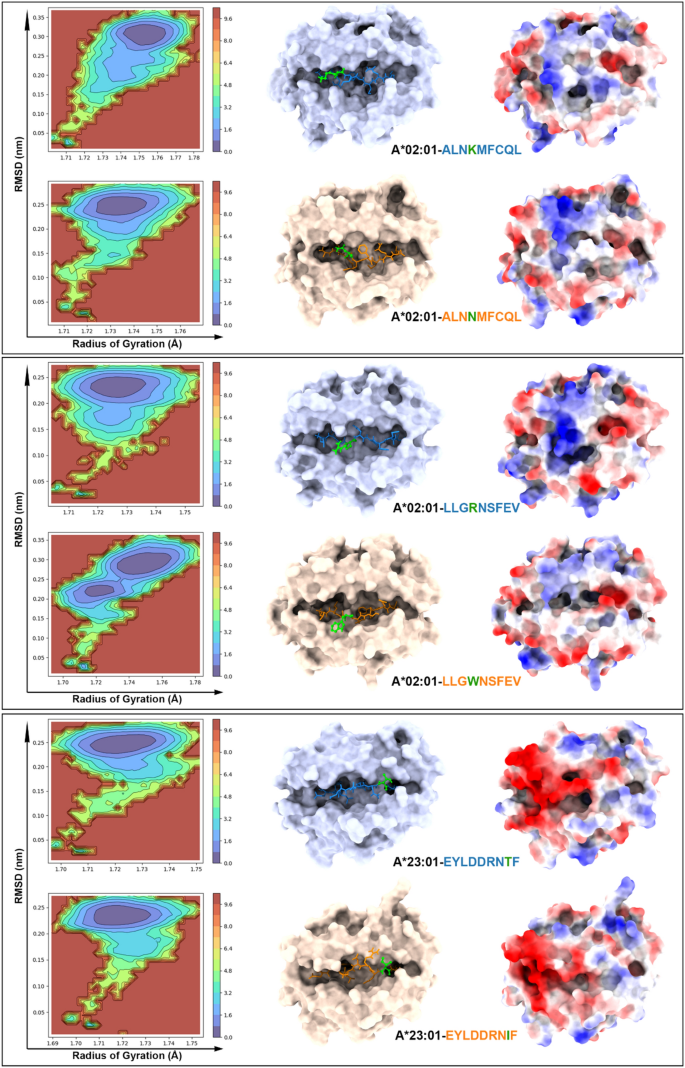
Free energy surface (FES) landscape (left) and lowest energy structures retrieved from simulation (right) for complexes HLA-A*02:01-ALNKMFCQL/ALNNMFCQL, HLA-A*02:01-LLGRNSFEV/LLGWNSFEV, and HLA-A*23:01-EYLDDRNTF/EYLDDRNIF. The FES plots depict the stability and conformational changes of the peptide-MHC complexes. In the middle, the MHC structures are shown with the peptide atoms highlighted, where the mutated residues are marked in green. On the right, the electrostatic potential surface of the peptide-MHC (pMHC-I) complex is displayed, illustrating the potential impact of mutations on the complex's surface properties.
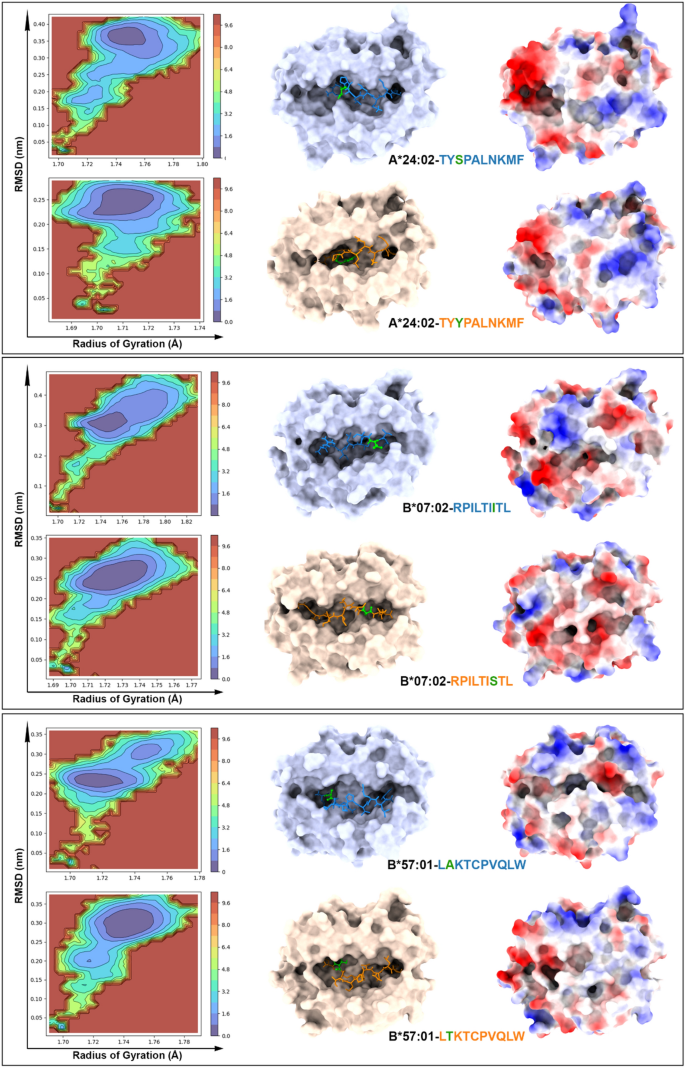
Free energy surface (FES) landscape (left) and lowest energy structures retrieved from simulation (right) for complexes HLA-A*24:02-TYSPALNKMF/TYYPALNKMF, HLA-B*07:02-RPILTIITL/RPILTISTL, and HLA-B*57:01-LAKTCPVQLW/LTKTCPVQLW. The FES plots depict the stability and conformational changes of the peptide-MHC complexes. In the middle, the MHC structures are shown with the peptide atoms highlighted, where the mutated residues are marked in green. On the right, the electrostatic potential surface of the peptide-MHC (pMHC-I) complex is displayed, illustrating the potential impact of mutations on the complex's surface properties.
We sought to determine if the electrostatic potential would change when comparing the modeled pMHC-I models against the lowest energy structures generated during the molecular dynamics (MD) simulation (Supplementary Fig. S5 ), and we also compared the lowest energy structures to the wild-type/mutated pairs. We observed primarily topographical modifications, along with differences in the distribution of charges around the TCR-interacting surface for some of the pMHC-I complexes. For instance, in the HLA-A*02:01-LLGRNSFEV/LLGWNSFEV complex, the substitution of Arginine with Tryptophan resulted in a loss of positive charges (Fig. 4 ). In the HLA-B*07:02-RPILTIITL/RPILTISTL complex, despite the modification occurring at the C-terminus of the epitope, a new positive charge emerged at the N-terminal region near residue 2 (Fig. 5 ). Additionally, the HLA-B*57:01-LAKTCPVQLW/LTKTCPVQLW complex exhibited significant topographical changes, likely due to the need to accommodate the more hydrophilic Threonine residue in the 10-mer peptide (Fig. 5 ). This adaptation resulted in a pronounced negative charge at the N-terminal part of the epitope and a gradual loss of the negative charge at the C-terminus.
Finally, we aimed to gain a better understanding of the contact map during the simulation between the peptide (wild-type/mutant) and the respective MHC-I (Fig. 6 ). We focused on contacts within the range of 0.4 nm (4 Å), a distance that captures key interactions such as hydrogen bonds, van der Waals interactions, hydrophobic contacts, and potential salt bridges. These interactions are crucial for the stability and specificity of the peptide-MHC-I complex and can provide insights into the effects of mutations on peptide binding and presentation.
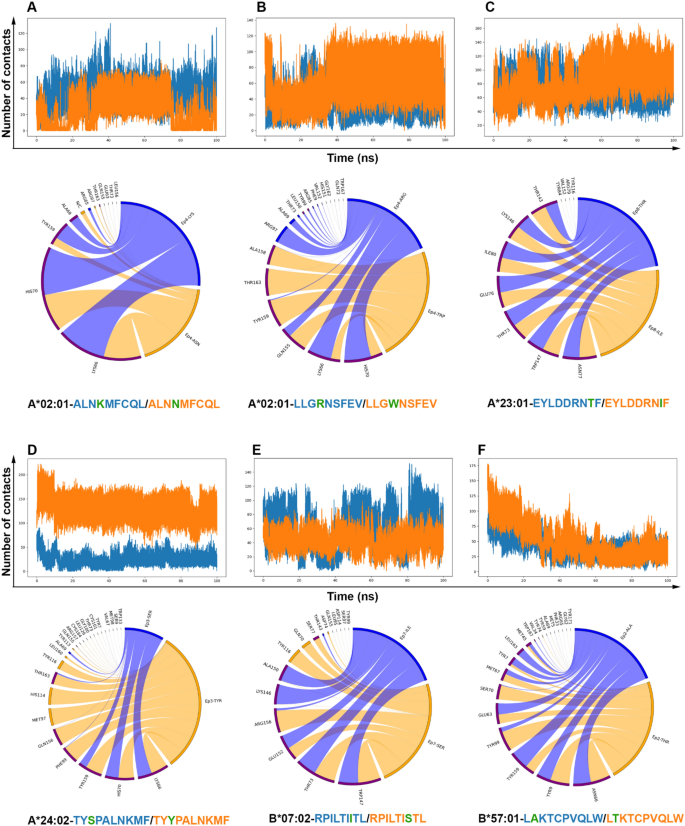
Contacts performed in the range of 0.4 nm between peptide and MHC-I. The line graphs represent the number of contacts performed along the simulation (wild-type peptide in blue and mutated peptide in orange). The circular alluvial plots show the residues interacting with the wt/mutated residue. The links are in blue (wt residue) or orange (mut residue). In each sector, the color blue, orange, or purple represent the MHC residues that are being contacted by the wt residue, by the mut residue or by both, respectively.
We observed that the contacts tended to increase or remain stable in most of the pMHC-I complexes, with the exceptions illustrated in Fig. 6 A,F. It is clear that most of the time, the same MHC-I residues were contacted by both wild-type and mutated residues throughout the simulation. However, in some specific cases, like the HLA-A*02:01-LLGRNSFEV/LLGWNSFEV complex (Fig. 6 B), MHC-I residues were mainly contacted by the mut residue. One particular noteworthy case is the HLA-A*24:02-TYSPALNKMF/TYYPALNKMF complex (Fig. 6 D). In this case, the number of contacts between the mutated epitope and the MHC-I was five times greater than those of the wild-type peptide. This significant increase suggests that the mutation greatly enhances the interaction between the epitope and the MHC-I. Additionally, some key contacts were unique to the mutated epitope and MHC-I, involving residues such as HIS114 and MET97, which were not observed in the wild-type interactions. These unique contacts may positively contribute to binding affinity and specificity observed in the mutated complex.
MHC-II binding analysis of the selected epitopes
Because of the importance of CD4 T cells in helping CD8 T cells and exerting antitumor immune response 22 , we used a sequence-based MHC-II peptide predictor ( https://services.healthtech.dtu.dk/services/NetMHCII-2.3/ ) to rank the binding capacity of the epitopes selected on Table 3 . As a result, we obtained 9 epitopes (wild-type and mutated) that showed strong binding affinity to the alleles described for MHC-II (Table 3 ). Alleles were selected according to epitope prediction based on HLA class II binding in the human population 23 . These data indicate that the selected epitopes can also bind MHC-II inducing a CD4 T cell response.
In the present study we performed a computational analysis to highlight the role of mutations in different GBM-related proteins and the peptide presentation in the context of MHC-I receptors. We showed that despite the presence of mutation in these peptides, pMHC-I structural similarity may still elicit the same CD8 T cell response. This conclusion is supported by molecular dynamics experiments.
First, we selected 83 mutant and wild-type peptide pairs based on MHC-I pathway prediction tools results, indicating that MHC-I presentation of mutated epitopes on selected proteins may be unaffected. After adjusting MHC-I binding cutoffs, 24 from 83 pMHC-I complex pairs were modeled. Applying the hierarchical cluster analysis on TCR-interacting surface features of each pMHC-I complex, epitopes from TP53 protein presented better clustering. Finally, we selected 6 pairs of putative epitopes based on the results of all the computational tools used. Our data suggest that these selected putative epitopes can be future targets for the development of new therapies against GBM, given that the mutation that will occur in the epitope might not affect the CD8 T cell response. Also, four of these pairs can also bind the MHC-II and feasibly contribute to the CD4 T cell response.
To determine if the observed differences in RMSD, and consequently stability, were significant for cases where the mutated epitope exhibited lower RMSD values, we employed both t-test statistics and Cohen’s d descriptor. The t-test results were significant (p < 0.001) in all cases, confirming the statistical significance of the differences. Furthermore, the effect sizes, as measured by Cohen’s d, were significant across all cases. These findings suggest that the mutated epitopes have a high likelihood of remaining stable on the cell surface when bound to MHC-I. Note that the proteins EGFR, IDH1 and PTEN, despite being highly expressed in GBM and with a high frequency of mutations, did not show a good overall result. This may be related to the number of mutations that are found in the immunogenic peptides. The majority of mutations in immunogenic epitopes occurred on TP53, 22 in total. It is not surprising that the TP53 protein is highlighted, since it is one of the most commonly dysregulated proteins in cancer, in 84% of GBM according to the TCGA and in up to 94.1% of GBM cell lines 24 . Also, TP53 is a well-known antigen recognized by antitumor immune response 25 . For example, Kim et al . , sequenced the entire exome in 163 patients with solid metastatic cancers, identified 78 who had missense mutations in TP53 and, through immunological screening, identified 21 unique T cell reactivity 26 . Certain mutations in this protein give rise to tumor-specific amino acid sequences that can provide T cell targets in the context of MHC-I 27 , although some mutations can lead to the impairment of already immunogenic epitopes.
Peptide-based vaccines against GBM have been already studied using different antigen targets 28 . There was a clinical trial of a personalized vaccine using four different peptides in relapsed GBM patients, but the trial did not meet the primary or secondary outcomes 29 . Although these results were not satisfactory, more targets for peptide vaccines are worth exploring 30 .A strategy to increase the binding to the MHC and improve peptide vaccines is the modification of antigenic peptide's primary anchors 31 . Borbulevych et al . demonstrated that the greater immunogenicity of the peptide is due to the greater stability of the pMHC complex, validating the anchor fixation approach to generate therapeutic candidate vaccines 32 . Here, for instance, we show a pMHC-I complex where we changed a key anchor position (B*57:01, position 2), from Alanine to Threonine, but this did not seem to affect the total number of contacts between peptide and MHC-I. Other studies have shown that modified TCRs can be used to produce T lymphocyte populations with the high specificity for use in antigen-specific T cell therapy 33 . Here, we demonstrate how different mutations influence the topography and electrostatic potential of the TCR-interacting surface features. This type of study provides insights into the structural characteristics of pMHC-I, which are crucial for advancing TCR engineering and enhancing our understanding of TCR-pMHC-I interactions. Mutations in the peptide-binding pockets of HLA-A2.1 also have significant effects on CD8 T cell recognition 34 .
One of the limitations of our study is the lack of immunogenicity evaluation of the selected mutated peptides using in vitro and in vivo assays for MHC-I binding and T-cell stimulation. Despite other studies have confirmed that in silico structural analysis can confirm immunogenicity of the peptides 20 , 21 , 35 , we also ran an immunogenicity predictor based on transfer learning called TLImm. The scores obtained show similar or, for some cases, better scores when comparing the mutated peptides to the wild-type, immunogenic ones.
In conclusion, our findings suggest that the complex presenting the mutated peptide TYYPALNKMF, when bound to HLA-A*24:02, exhibits the most promising characteristics for use as a GBM peptide target. Notably, the protein and epitope RMSD values for the mutant complex were significantly lower compared to those of the wild-type. Additionally, the FES plots and the electrostatic potential at the TCR-interacting surface were remarkably similar between the wild-type and mutated complexes. Finally, the mutated epitope demonstrated more than a fivefold increase in the number of contacts relative to the wild-type. These attributes underscore the potential of the TYYPALNKMF peptide as a viable target for GBM immunotherapy.
In summary, we report that through predictive analyses, we were able to discern mutations capable of influencing, or not, the immune response, thereby allowing the targeting of mutations that elicited a response for use in immunotherapeutic approaches. Based on the information outlined in this study, we propose potential targets for glioblastoma multiforme (GBM) therapies, all derived from the TP53 protein.
Materials and methods
Search for mutations in highly expressed glioma proteins and potentially immunogenic epitopes.
We first accessed the TCGA Database ( https://cancergenome.nih.gov/ ), which provides the main genomic alterations in different types of cancer obtained from patient samples, to search for genes in the GDC (Genomic Data Commons) portal. We aimed at genes that have a high frequency of missense mutations in GBM ( https://portal.gdc.cancer.gov/ ): EGFR , IDH1 , PTEN , and TP53 . We used the IEDB Database to search for immunogenic epitopes present in these proteins, using the search filters “T Cell assay”, “class I MHC restriction”, “human host”, and “antigen”, in this way we selected only the epitopes capable of generating a T-cell response.
Download the protein sequences
The sequences of Homo sapiens EGFR (UniProt ID: P00533), IDH1 (UniProt ID: O75874), PTEN (UniProt ID: P60484), and TP53 (UniProt ID: P04637) proteins were obtained from the UniProtKB database ( http://www.uniprot.org/ ) in the FASTA format. We mutated the sequences manually according to the mutations found in the GDC 28 , 36 , 37 , 38 , 39 , 40 , 41 , 42 , 43 , 44 .
Class I MHC antigen processing analysis
We next used the combined prediction tool “Proteasomal cleavage/TAP transport/MHC class I combined predictor” available on the IEDB platform. The FASTA sequences of wild-type and mutated proteins were used as input. As output, we got a list of peptides that could be presented at the cell surface with their respective results for Total Score (data not shown). From this list, we searched for the wild-type epitopes and the mutated epitopes previously selected from GDC. In addition to these, we also searched for epitopes downstream and upstream including the mutated amino acid position, thus covering all peptides containing that mutation. In this work, we focus on total score results because it combines proteasomal cleavage, TAP transport, and MHC binding predictions. We used the GraphPad Prism 9.4.0 software (GraphPad Software, Inc., San Diego, CA) to compare the total score mean from wild-type and mutated epitopes found for the same MHC-I allele as well as their upstream and downstream epitopes and perform pairwise statistical analysis (t-test; p < 0.05). To select the epitope pairs (wild-type and mutated) for further analysis, we defined the following rules:
the described wild-type and corresponding mutated epitope should be above the average total score;
the difference in the total score between the wild-type and the mutated epitope is not higher than 0.5 (arbitrary value).
To analyze the immunogenicity probabilities, we used a transfer learning-based prediction tool for peptide immunogenicity (TLImm) (available at: https://github.com/KavrakiLab/TL-MHC/tree/master/TLImm ). This tool leverages comprehensive datasets encompassing binding affinity and mass spectrometry (MS).
Modeling of pMHC-I
We first used the MHCflurry tool 45 , included in the HLA-Arena, which based on the chosen cutoff, generates the predictions (represents the affinity to MHC-I binding) relative to each wild-type epitope previously selected. Then we set the HLA-Arena with this cutoff value and used the virtual screening notebook with default options to construct the pMHC-I complexes for each pair of wild-type and mutated epitopes for the corresponding MHC-I allele. We submitted peptides for HLA-Arena analysis with different lengths, since MHCflurry allows us to analyze peptides of up to 15 amino acids in length.
Generation and visualization of pMHC-I electrostatic potential
We used the PyMOL program 46 to generate a visual representation of the electrostatic potential of the TCR-interacting surface of each pMHC-I complex obtained from HLA-Arena. We aligned the pMHCs and obtained the RMSD values before imaging the electrostatic surface of each complex. We generated an in-house plugin (“histogram2csv'' available at https://github.com/LAD-PUCRS/Arena_SARS-BCG ) to extract data regarding the mean and standard deviation of the RGB values of each image in 46 regions of interest (Supporting Information, Fig. S5 ) as described in 19 . These values were used for further hierarchical clustering analysis with the 'pvclust' package 47 from R software ( https://www.r-project.org/ ) 48 . Pvclust is designed to evaluate the uncertainty associated with hierarchical cluster analysis. Pvclust provides two types of p-values: AU (Approximately Unbiased) p-value and BP (Bootstrap Probability) value. The AU p-value, computed through multiscale bootstrap resampling, offers a more precise approximation to an unbiased p-value than the BP value, which is calculated using normal bootstrap resampling 47 . The input data are color histogram (RGB) values for each pMHC-I complex, regarding the information on charge distribution and surface structure. We followed the steps described at “ https://github.com/LAD-PUCRS/Arena_SARS-BCG ” to generate the dendrogram from the hierarchical analysis. These dendrograms depict pMHC-I electrostatic similarity based on RGB values. For the generation of electrostatic potential for the pMHC-I complexes derived from the molecular dynamics simulation we used ChimeraX v1.7 49 with default parameters.
The summary of the methods is schematized on the flowchart (Fig. 7 ).
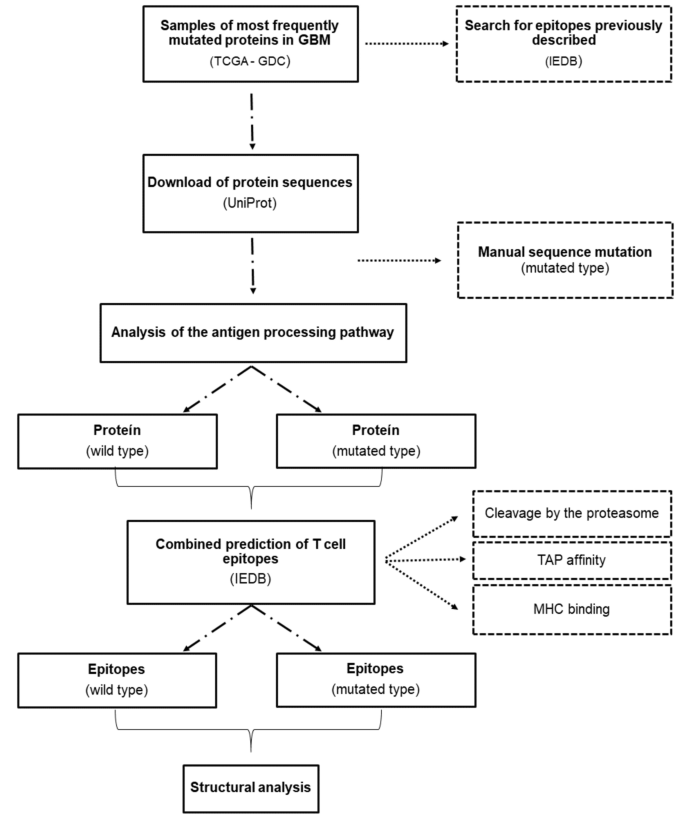
Flowchart with all the steps from data acquisition to processing and structural analysis of MHC-I complexes.
Molecular dynamics simulations
Energy minimization, equilibration, and production.
Input structures for molecular dynamics (MD) simulation were pre-processed with the PDB2PQR webserver 50 , fixed as needed and protonated at pH 7.0 using PROPKA algorithm 51 . To improve performance, the HLA receptor was truncated at residue 182, as previously described in Abella et al., 2020 52 . This resulted in a system composed by the domains alpha-1 and alpha-2 of the HLA and the bound peptide. The molecular dynamics (MD) simulations were performed with GROMACS 2024.2, CHARMM36 force field, and TIP3 water model. The simulation box was defined with a minimum distance of 1.5 nm (15 Å) between the protein and the box edge. The system was then solvated, and ions were added to neutralize the system and achieve a physiological ion concentration of 0.15 M NaCl. The algorithms v-rescale (tau-t = 0.1 ps) and Parrinello-Rahman (tau-p = 2 ps) were used for temperature and pressure coupling, respectively. A cutoff value of 1.2 nm was used for both the van der Waals and Coulomb interactions, with Fast Particle Mesh Ewald electrostatics (PME). The production stage of each MD simulation was preceded by three steps of energy minimization (EM) and eight steps of equilibration (EQ), as previously described 53 . Briefly, energy minimization (EM) was performed using the steepest-descent algorithm with position restraints applied to all heavy atoms of the amino acids, set at 5000 kJ mol −1 nm −2 . The second EM step employed the same algorithm but removed the restraints. In the third EM step, the conjugate-gradient algorithm was used without any restraints to further relax the protein structure. The equilibration phase begins at a temperature of 310 K, maintained for 300 ps, with position restraints applied to the protein heavy atoms (5000 kJ mol −1 nm −2 ). This step allows solvation layers to form without disturbing the HLA-I folding. Subsequently, the temperature is reduced to 280 K, and the position restraints are gradually decreased. The temperature is then progressively increased to 300 K. These equilibration steps constitute the first 500 ps of each MD simulation. During the production stage, the temperature is kept constant at 300 K. Each pMHC-I complex was simulated for 100 ns.
The raw trajectory files were post-processed using GROMACS tools (e.g., gmx trjconv) to perform rotational and translational alignment of sampled conformations, correct for the effects of periodic boundary conditions, and remove water molecules. The RMSD, RMSF, and Radius of Gyration were computed using the programs gmx rms, gmx rmsf, and gmx gyrate, respectively. For each pair, we performed a t-test to determine if there was a difference between WT and mutant RMSD values. A large absolute value indicates a large difference between the group means, while the sign (positive or negative) indicates the direction of the difference. In all cases (6 pairs analyzed), the RMSD frequency was statistically different between pairs. To evaluate the effect size, we computed Cohen’s d value for each pair (WT vs mutated), which is often used in the context of t-tests. As suggested by Cohen and expanded by Sawilowsky 54 , 55 , the effect size can be described as very small (d = 0.01), small (d = 0.20), medium (d = 0.50), large (d = 0.80), very large (d = 1.20), and huge (d = 2.0). The contact analysis was performed using the program gmx mindist, with a cutoff of 0.4 nm. Plots were generated using python in-house scripts and ggplot. The free energy surface (FES) was computed using the fes.py script, developed by Birgit Strodell from the Multiscale Modelling Group ( http://www.strodel.info/ ) and optimized by Cristóvão Freitas Iglesias Junior ( http://lmdm.biof.ufrj.br/ ). We further adapted the script to a new Python language (Python 3) and included new code to retrieve the lowest energy structure.
Data availability
The datasets generated during and/or analyzed during the current study are available from the corresponding author on reasonable request.
Barbaro, M. et al. Causes of death and end-of-life care in patients with intracranial high-grade gliomas: A retrospective observational study. Neurology 98 , 1 (2022).
Google Scholar
Poon, M. T. C., Sudlow, C. L. M., Figueroa, J. D. & Brennan, P. M. Longer-term (≥ 2 years) survival in patients with glioblastoma in population-based studies pre- and post-2005: a systematic review and meta-analysis. Sci. Rep. 10 , 1 (2020).
Smoll, N. R., Schaller, K. & Gautschi, O. P. Long-term survival of patients with glioblastoma multiforme (GBM). J. Clin. Neurosci. 20 , 670 (2013).
PubMed Google Scholar
El-Ayadi, M. et al. High-grade glioma in very young children: A rare and particular patient population. Oncotarget 8 , 64564. https://doi.org/10.18632/oncotarget.18478 (2017).
Perrin, S. L. et al. Glioblastoma heterogeneity and the tumour microenvironment: Implications for preclinical research and development of new treatments. Biochem. Soc. Trans. 47 , 625. https://doi.org/10.1042/BST20180444 (2019).
CAS PubMed Google Scholar
Ueda, R. et al. Dicer-regulated microRNAs 222 and 339 promote resistance of cancer cells to cytotoxic T-lymphocytes by down-regulation of ICAM-1. Proc. Natl. Acad. Sci. U. S. A. 106 , 10746 (2009).
ADS CAS PubMed PubMed Central Google Scholar
Chheda, Z. S. et al. Novel and shared neoantigen derived from histone 3 variant H3.3K27M mutation for glioma T cell therapy. J. Exp. Med. 215 , 141 (2018).
CAS PubMed PubMed Central Google Scholar
Samaha, H. et al. A homing system targets therapeutic T cells to brain cancer. Nature 561 , 331 (2018).
Peters, B., Nielsen, M. & Sette, A. T cell epitope predictions. Annu. Rev. Immunol. 38 , 123 (2020).
Kim, S. K. & Cho, S. W. The evasion mechanisms of cancer immunity and drug intervention in the tumor microenvironment. Front. Pharmacol. 13 , 1–16 (2022).
Zhang, X. et al. The immunosuppressive microenvironment and immunotherapy in human glioblastoma. Front. Immunol. 13 , 1003651. https://doi.org/10.3389/fimmu.2022.1003651 (2022).
Nduom, E. K. et al. PD-L1 expression and prognostic impact in glioblastoma. Neuro Oncol. 18 (2), 195–205. https://doi.org/10.1093/neuonc/nov172 (2016).
Kim, K. et al. Predicting clinical benefit of immunotherapy by antigenic or functional mutations affecting tumour immunogenicity. Nat. Commun. 11 , 1 (2020).
ADS Google Scholar
Hutchison, S. & Pritchard, A. L. Identifying neoantigens for use in immunotherapy. Mammalian Genome 29 , 714. https://doi.org/10.1007/s00335-018-9771-6 (2018).
Zhang, Q. et al. Immune epitope database analysis resource (IEDB-AR). Nucleic Acids Res. 36 , 513 (2008).
Kim, Y., Sette, A. & Peters, B. Applications for T-cell epitope queries and tools in the immune epitope database and analysis resource. J. Immunol. Methods 374 , 513 (2011).
Fasoulis, R., Menegatti, M., Amaral, D., Paliouras, G. & Kavraki, L. E. ImmunoInformatics Transfer learning improves pMHC kinetic stability and immunogenicity predictions. ImmunoInformatics 13 , 100030 (2024).
Antunes, D. A. et al. HLA-Arena: A customizable environment for the structural modeling and analysis of peptide-HLA complexes for cancer immunotherapy. JCO Clin. Cancer Inf. https://doi.org/10.1200/cci.19.00123 (2020).
Tarabini, R. F. et al. Large-scale structure-based screening of potential T cell cross-reactivities involving peptide-targets from BCG vaccine and SARS-CoV-2. Front. Immunol. 12 , 1 (2022).
Mendes, M. F. A., Antunes, D. A., Rigo, M. M., Sinigaglia, M. & Vieira, G. F. Improved structural method for T-cell cross-reactivity prediction. Mol. Immunol. 67 , 303 (2015).
Antunes, D. A. et al. Interpreting T-cell cross-reactivity through structure: Implications for TCR-based cancer immunotherapy. Front. Immunol. 8 , 1210 (2017).
PubMed PubMed Central Google Scholar
Wu, K. & Fong, L. CD4+ T cells help myeloid-mediated killing of immune-evasive tumors. Trends Cancer 9 , 777. https://doi.org/10.1016/j.trecan.2023.07.013 (2023).
Paul, S. et al. Development and validation of a broad scheme for prediction of HLA class II restricted T cell epitopes. J. Immunol. Methods 422 , 28 (2015).
Zhang, Y. et al. The p53 pathway in glioblastoma. Cancers 10 , 297. https://doi.org/10.3390/cancers10090297 (2018).
Hsiue, E. H. C. et al. Targeting a neoantigen derived from a common TP53 mutation. Science (80-. ). 371 , 6533 (2021).
Kim, S. P. et al. Adoptive cellular therapy with autologous tumor-infiltrating lymphocytes and T-cell receptor–engineered T cells targeting common p53 neoantigens in human solid tumors. Cancer Immunol. Res. OF1–OF15. https://doi.org/10.1158/2326-6066.cir-22-0040 (2022).
Yanuck, M. et al. A mutant p53 tumor suppressor protein is a target for peptide-induced CD8+ cytotoxic T-cells. Cancer Res. 53 , 1 (1993).
Langdon, C. G. Nuclear PTEN’s functions in suppressing tumorigenesis: Implications for rare cancers. Biomolecules 13 , 259. https://doi.org/10.3390/biom13020259 (2023).
Narita, Y. et al. A randomized, double-blind, phase III trial of personalized peptide vaccination for recurrent glioblastoma. Neuro. Oncol. 21 , 348 (2019).
Yang, T. et al. Peptide vaccine against glioblastoma: from bench to bedside. Holist. Integr. Oncol. 1 , 1 (2022).
CAS Google Scholar
Gad, M. et al. MUC1-derived glycopeptide libraries with improved MHC anchors are strong antigens and prime mouse T cells for proliferative responses to lysates of human breast cancer tissue. Eur. J. Immunol. 33 , 1624. https://doi.org/10.1002/eji.200323698 (2003).
Borbulevych, O. Y., Baxter, T. K., Yu, Z., Restifo, N. P. & Baker, B. M. Increased immunogenicity of an anchor-modified tumor-associated antigen is due to the enhanced stability of the peptide/MHC complex: Implications for vaccine design. J. Immunol. 174 , 4812 (2005).
Tendeiro Rego, R., Morris, E. C. & Lowdell, M. W. T-cell receptor gene-modified cells: Past promises, present methodologies and future challenges. Cytotherapy 21 , 1 (2019).
Matsui, M., Moots, R. J., McMichael, A. J. & Frelinger, J. A. Significance of the six peptide-binding pockets of HLA-A21 in influenza a matrix peptide-specific cytotoxic T-lymphocyte reactivity. Hum. Immunol. 41 , 160 (1994).
Soon, C. F. et al. Hepatitis E Virus (HEV)-specific T cell receptor cross-recognition: Implications for immunotherapy. Front. Immunol. 10 , 1–14 (2019).
Cao, M. et al. A three-component multi-b-value diffusion-weighted imaging might be a useful biomarker for detecting microstructural features in gliomas with differences in malignancy and IDH-1 mutation status. Eur. Radiol. https://doi.org/10.1007/s00330-022-09212-5 (2022).
Yan, H. et al. IDH1 and IDH2 Mutations in Gliomas. N. Engl. J. Med. 360 , 765 (2009).
Guo, G., Narayan, R., Horton, L., Patel, T. & Habib, A. The Role of EGFR-Met Interactions in the Pathogenesis of Glioblastoma and Resistance to Treatment. Curr. Cancer Drug Targets 17 , 297 (2017).
Richardson, T. E. et al. Rapid progression to glioblastoma in a subset of IDH-mutated astrocytomas: A genome-wide analysis. J. Neurooncol. 133 , 183 (2017).
Vidotto, T. et al. Pan-genomic analysis shows hemizygous PTEN loss tumors are associated with immune evasion and poor outcome. bioRxiv 2022.09.16.508308. https://doi.org/10.1038/s41598-023-31759-6 (2022).
Wang, H., Guo, M., Wei, H. & Chen, Y. Targeting p53 pathways: Mechanisms, structures, and advances in therapy. Signal Transduct. Target. Ther. 8 , 1. https://doi.org/10.1038/s41392-023-01347-1 (2023).
Spino, M. et al. Cell surface notch ligand dll3 is a therapeutic target in isocitrate dehydrogenase–mutant glioma. Clin. Cancer Res. 25 , 1261 (2019).
Le Rhun, E. et al. Molecular targeted therapy of glioblastoma. Cancer Treatment Rev. 80 , 1018. https://doi.org/10.1016/j.ctrv.2019.101896 (2019).
England, B., Huang, T. & Karsy, M. Current understanding of the role and targeting of tumor suppressor p53 in glioblastoma multiforme. Tumor Biol. 34 , 2063. https://doi.org/10.1007/s13277-013-0871-3 (2013).
O’Donnell, T. J. et al. MHCflurry: Open-source class I MHC binding affinity prediction. Cell Syst. 7 , 2174 (2018).
Yuan, S., Chan, H. C. S. & Hu, Z. Using PyMOL as a platform for computational drug design. Wiley Interdiscip. Rev. Comput. Mol. Sci. 7 , 2. https://doi.org/10.1002/wcms.1298 (2017).
Suzuki, R. & Shimodaira, H. Pvclust: An R package for assessing the uncertainty in hierarchical clustering. Bioinformatics 22 , 1540 (2006).
Ihaka, R. & Gentleman, R. R: A language for data analysis and graphics. J. Comput. Graph. Stat. 5 , 299 (1996).
Meng, E. C. et al. UCSF ChimeraX: Tools for structure building and analysis. Protein Sci. 32 (11), e4792. https://doi.org/10.1002/pro.4792 (2023).
Dolinsky, T. J., Nielsen, J. E., McCammon, J. A. & Baker, N. A. PDB2PQR: An automated pipeline for the setup of Poisson-Boltzmann electrostatics calculations. Nucleic Acids Res. 32 (Web Server), W665–W667. https://doi.org/10.1093/nar/gkh381 (2004).
Olsson, M. H., Søndergaard, C. R., Rostkowski, M. & Jensen, J. H. PROPKA3: Consistent Treatment of Internal and Surface Residues in Empirical pKa Predictions. J. Chem. Theory Comput. 7 (2), 525–537. https://doi.org/10.1021/ct100578z (2011).
Abella, J. R. et al. Markov state modeling reveals alternative unbinding pathways for peptide–MHC complexes. Proc. Natl. Acad. Sci. U. S. A. 117 (48), 30610–30618. https://doi.org/10.1073/pnas.2007246117 (2020).
Jackson, K. R. et al. Charge-based interactions through peptide position 4 drive diversity of antigen presentation by human leukocyte antigen class I molecules. PNAS Nexus. 1 (3), pgac124. https://doi.org/10.1093/pnasnexus/pgac124 (2022).
Cohen, J. Statistical Power Analysis for the Behavioral Sciences (Routledge, New York, 1998).
Sawilowsky, S. New effect size rules of thumb. J. Mod. Appl. Stat. Methods 8 (2), 597–599. https://doi.org/10.22237/jmasm/1257035100 (2009).
MathSciNet Google Scholar
Download references
Acknowledgements
This study was financed in part by the Coordenação de Aperfeiçoamento de Pessoal de Nível Superior—Brasil (CAPES)—Finance Code 001. MMR was supported by a CPRIT fellowship RP170593.
Author information
Maurício Menegatti Rigo
Present address: Center for Discovery and Innovation, Hackensack Meridian Health, Nutley, NJ, USA
Authors and Affiliations
Laboratory of Clinical and Experimental Immunology, Infant Center, School of Health Science, Pontifical Catholic University of Rio Grande do Sul (PUCRS), Porto Alegre, Brazil
Renata Fioravanti Tarabini & Ana Paula Duarte de Souza
Kavraki Lab, Department of Computer Science, Rice University, Houston, TX, USA
Post-Graduation Program in Genetics and Molecular Biology, Universidade Federal do Rio Grande do Sul, Porto Alegre, Brazil
Gustavo Fioravanti Vieira
Post-Graduation Program in Health and Human Development, Universidade La Salle, Canoas, Brazil
You can also search for this author in PubMed Google Scholar
Contributions
All authors contributed to the study conception and design. All authors read and approved the final manuscript.
Corresponding authors
Correspondence to Maurício Menegatti Rigo or Ana Paula Duarte de Souza .
Ethics declarations
Competing interests.
The authors declare no competing interests.
Additional information
Publisher's note.
Springer Nature remains neutral with regard to jurisdictional claims in published maps and institutional affiliations.
Supplementary Information
Supplementary information., rights and permissions.
Open Access This article is licensed under a Creative Commons Attribution 4.0 International License, which permits use, sharing, adaptation, distribution and reproduction in any medium or format, as long as you give appropriate credit to the original author(s) and the source, provide a link to the Creative Commons licence, and indicate if changes were made. The images or other third party material in this article are included in the article's Creative Commons licence, unless indicated otherwise in a credit line to the material. If material is not included in the article's Creative Commons licence and your intended use is not permitted by statutory regulation or exceeds the permitted use, you will need to obtain permission directly from the copyright holder. To view a copy of this licence, visit http://creativecommons.org/licenses/by/4.0/ .
Reprints and permissions
About this article
Cite this article.
Tarabini, R.F., Fioravanti Vieira, G., Rigo, M.M. et al. Mutations in glioblastoma proteins do not disrupt epitope presentation and recognition, maintaining a specific CD8 T cell immune response potential. Sci Rep 14 , 16721 (2024). https://doi.org/10.1038/s41598-024-67099-2
Download citation
Received : 28 January 2024
Accepted : 08 July 2024
Published : 19 July 2024
DOI : https://doi.org/10.1038/s41598-024-67099-2
Share this article
Anyone you share the following link with will be able to read this content:
Sorry, a shareable link is not currently available for this article.
Provided by the Springer Nature SharedIt content-sharing initiative
By submitting a comment you agree to abide by our Terms and Community Guidelines . If you find something abusive or that does not comply with our terms or guidelines please flag it as inappropriate.
Quick links
- Explore articles by subject
- Guide to authors
- Editorial policies
Sign up for the Nature Briefing: Cancer newsletter — what matters in cancer research, free to your inbox weekly.

Monitoring and Evaluation Officer
The British Academy
- Closing: 12:00pm, 8th Aug 2024 BST
Job Description
The British Academy – the UK’s national body for the humanities and social sciences - is seeking a Monitoring & Evaluation officer to join its newly established Monitoring & Evaluation team based in the Research Directorate, providing key support in the delivery of the Academy’s strategy.
The Academy recently released its strategy for 2023-2027. Our three strategic priorities for this period are:
Strengthening and championing the humanities and social sciences
Mobilising our disciplines for the benefit of everyone
Opening up the Academy
To support the success of these strategic priorities the Academy established a new Monitoring & Evaluation team in 2023. The Academy recognises the growing importance of capturing, utilising, and visualising data effectively to ensure we can strongly advocate for SHAPE through appropriate monitoring and evaluation of the work we do and support.
You will play a key role in the Monitoring and Evaluation team in supporting the development and implementation of a modern, streamlined approach to the way in which we monitor and evaluate our work, particularly with respect to the International Science Partnerships Fund. We are looking for dynamic individuals, either with experience in monitoring and evaluation, or transferable skills from other roles, who are keen to be part of a new team at the start of a new chapter at the Academy.
ROLE PURPOSE / OVERVIEW
The Monitoring & Evaluation Officer will be part of the Monitoring & Evaluation team, reporting to the Senior Data Analyst.
You will play a key role in advising on and delivering the monitoring and evaluation requirements of the Academy for their portfolio of funding under the International Science Partnerships Fund (ISPF). This includes developing streamlined data collection tools that minimise the burden on award holders and Academy staff whilst meeting stringent reporting requirements.
Other responsibilities include the analysis of data and statistics and their visual representation in reports and presentations for internal and external audiences including funders, as well as identifying how reporting requirements or strategies developed for ISPF might support other British Academy teams and wider Monitoring and Evaluation requirements.
The role is positioned within the Monitoring & Evaluation team of the British Academy’s Research Directorate and works closely with colleagues in the International team as well members of the Policy Directorate and staff located in the Development and Communications Directorate.
OFFICE LOCATION - 10-11 Carlton House Terrace, St James Park, London, SW1Y 5AH
KEY RESPONSIBILITIES
1. Develop streamlined data collection tools for ISPF monitoring and evaluation that minimises the burden on award holders and Academy staff whilst still meeting stringent reporting requirements.
Work with the Senior Data Analyst in determining the viability of existing data management systems to fulfil the requirements of ISPF reporting.
Develop appropriate methodologies and templates on British Academy information management systems to ensure the timely and accurate collection of data within an appropriate format for ISPF reporting purposes.
In collaboration with colleagues in the International team, continually identify, review and implement efficiencies within existing reporting processes and platforms.
Monitor and report on data quality, liaising with data owners within the Academy to ensure the accurate capture of required information.
2. Produce high-quality analysis of the Academy’s ISPF research funding portfolio.
Extract data from a variety of sources, both internal and external, to produce accurate and insightful quantitative and qualitative analysis on applications and awards for ISPF schemes.
Produce reports, infographics and visualisations as required for internal British Academy Committees, DSIT ISPF teams and their appointed evaluation consultants, producing work that engages and informs decision makers at all levels.
Where appropriate, utilise data visualisation software to build reporting tools that allow for the regular monitoring of ISPF performance.
Quality assure all data analysis before publication to ensure a consistently high standard of delivery.
3. Identify and communicate any reporting strategies developed for ISPF that might support other British Academy teams and wider Monitoring and Evaluation requirements.
Develop good working relationships with colleagues based in other Directorates to explore and identify what additional support they might require for their monitoring and evaluation requirements.
Seek out innovative approaches to data analysis and presentation to improve reporting to internal and external partners.
Work with the Senior Data Analyst to identify how lessons learned from the ISPF reporting and analysis can be cascaded to wider monitoring and evaluation work throughout the British Academy.
KEY STAKEHOLDERS
Head of Monitoring & Evaluation
Head of International Funding
Head of Global Strategy, Policy & Engagement
Senior Monitoring and Evaluation Lead
Department for Science, Innovation and Technology
External evaluation agencies/companies
University and IRO research funding offices
Other relevant funders in the HEI landscape
ROLE REQUIREMENTS
QUALIFICATIONS
WORKING RELATIONSHIPS
Works and communicates effectively
Is proactive in developing and maintaining effective working relationships with others internally and externally
Demonstrates a positive attitude towards helping others by providing appropriate support, using initiative, and not waiting to be asked
Adopts a communication style appropriate to the situation and the audience, understanding that communication is two-way
Seeks and responds to feedback from others, and does not take constructive criticism personally
Values others’ input and expertise, treating everyone with respect
Shares all relevant or useful information and shares knowledge and skills with others
Is sensitive to different working styles and views, adapting where appropriate
Is willing and able to compromise and negotiate, including to resolve possible conflicts • Handles difficult situations with sensitivity & respect
Communicates effectively with staff across departments
Promotes a positive impression of the Academy both internally and externally
Encourages commitment & positivity in self and others
KNOWLEDGE AND SKILLS
Demonstrates and develops appropriate knowledge and skills
Experience of collecting, quality assuring and managing data to a high degree of quality within information systems and databases as required
Experience in analysing both quantitative and qualitative data to produce reports and presentations for both internal and external audiences
Strong organisational and project management skills with the ability to manage multiple projects simultaneously.
Experience of working independently with strong attention to detail.
Understanding of the research funding landscape with knowledge of Humanities and Social Sciences, or willingness to learn proactively in post
Knowledge of monitoring and evaluation methodologies both qualitative and quantitative, or willingness to learn proactively in post
High level of literacy and numeracy.
Excellent IT skills, specifically Excel, and the capability to learn new IT skills readily.
Data Visualisation experience, ideally using packages such as Microsoft Power BI, or capability to expand data presentation skills with these or similar packages
Excellent analytical and problem-solving skills
Excellent interpersonal and communication skills
PROACTIVITY AND PLANNING
Manages time and resources effectively in order to achieve own and organisational objectives.
Prioritises tasks to meet deadlines
Displays resilience in coping with workload
Sets clear and structured objectives
Keeps relevant colleagues informed and seeks input
Evaluates and manages risks effectively
Takes ownership of tasks, including delegating effectively where appropriate
Monitors progress effectively and adapts plans where possible
Evaluates and reflects on plans & delivery and applies learning to future planning
Offers appropriate challenge to potential waste or inefficiency in using resources.
Makes suggestions for improving the way things are done
Is receptive to new ideas and change, adapting own ways of working as appropriate
PROBLEM SOLVING AND DECISION MAKING
Ensures good decisions are made through a rational and methodical approach
Identifies problems and opportunities and acts on them
Has a pro-active, can do, attitude, taking personal responsibility for correcting problems or resolving issues
Focuses on solutions and manages pressure
Anticipates obstacles and thinks ahead
Copes with the unexpected
Sets the right balance between referring decisions to the appropriate level and taking things forward without referral
Takes a flexible approach to their work, happy to take on different responsibilities in the interests of the Academy
Is aware of own strengths and weaknesses, and prepared to admit own mistakes
Seeks and uses opportunities to learn and develop, and is quick in picking up new tasks
Encourages creativity and innovation, accepting that mistakes sometimes happen
Gives a clear sense of direction while inspiring others with vision and actions
Is happy to support other team members during busy periods, whilst not compromising your own workload.
Treats other staff members and stakeholders with respect and honesty.
Sets the right balance between referring decisions to the appropriate level and taking things forward without referral.
Takes ownership of their responsibilities
Willing to travel on British Academy business.
Able to be flexible about working hours on occasion
WORKPLACE VALUES
The Academy’s workplace values and supporting text are set out below. We share these core values with each other. They guide how we work together and with others. We demonstrate them through our behaviours.
COLLABORATION- We consistently work together to a common purpose, supporting each other, communicating openly and sharing knowledge. We are flexible and adaptable, receiving feedback constructively
CREATIVITY - We bring imagination and curiosity to our work, valuing learning, diverse thinking, and innovation. We are open-minded, receptive, and responsive, taking account of alternative perspectives
EMPATHY-We are understanding, sensitive, compassionate, and considerate, mindful of our own wellbeing alongside that of others
EXCELLENCE - We always do our best, without striving to be perfect, applying our expertise and experience to develop and grow
INTEGRITY - We are open and honest with each other, accepting personal responsibility and demonstrating loyalty. We are reliable and dependable, having belief that we will be transparent and clear
RESILIENCE - We are confident, bold, and tenacious, knowing when to listen as well as when to speak up. We respond positively to challenges and overcome problems
RESPECT - We are inclusive in our regard for each other, showing courtesy and appreciation. We treat people fairly and equally, welcoming everyone’s contribution and difference
APPLICATION PROCESS
We have a two-step approach to hiring, beginning with submitting an application through the Applied platform. This will be followed by a panel interview and may involve a task component.
Please note that you may be asked to reference any literature that you may refer to, as applications that are highly plagiarised and not your own work. e.g. ChatGPT, will be declined.
The deadline for applications is 12:00 noon 8 August 2024, however, we may close this role sooner if we receive a high volume of applications . In these circumstances we will give those who have shown interest 24 hours notice of the change of deadline.
Interviews for this role are currently scheduled for week commencing 2 September 2024 but this may be subject to change.
Know someone great for this?
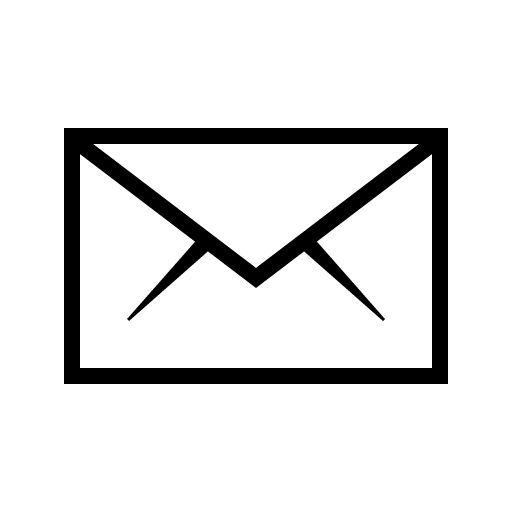
Removing bias from the hiring process
Start your de-biased application
- Your application will be anonymously reviewed by our hiring team to ensure fairness
- You won't need a CV to apply to this job
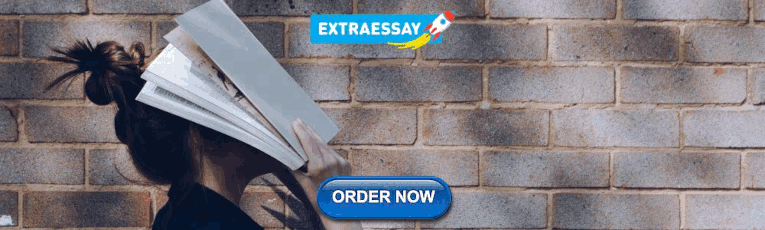
IMAGES
VIDEO
COMMENTS
Present Your Data Like a Pro. Summary. While a good presentation has data, data alone doesn't guarantee a good presentation. It's all about how that data is presented. The quickest way to ...
Step 1: Define Your Data Hierarchy. While presenting data on the budget allocation, start by outlining the hierarchical structure. The sequence will be like the overall budget at the top, followed by departments, projects within each department, and finally, individual cost categories for each project. Example:
1. Step one: Defining the question. The first step in any data analysis process is to define your objective. In data analytics jargon, this is sometimes called the 'problem statement'. Defining your objective means coming up with a hypothesis and figuring how to test it.
8. Tabular presentation. Presenting data in rows and columns, often used for precise data values and comparisons. Tabular data presentation is all about clarity and precision. Think of it as presenting numerical data in a structured grid, with rows and columns clearly displaying individual data points.
Make sure your data is accurate, up-to-date, and relevant to your presentation topic. Your goal will be to create clear conclusions based on your data and highlight trends. 2. Know your audience. Knowing who your audience is and the one thing you want them to get from your data is vital.
It's time to put all your data, and data visualizations into your presentation and learn the skill to present your findings like a pro.0:00 Putting It All To...
Key Objectives of Data Presentation. Here are some key objectives to think about when presenting financial analysis: Visual communication. Audience and context. Charts, graphs, and images. Focus on important points. Design principles. Storytelling. Persuasiveness.
10. Go beyond charts. A data presentation doesn't have to be all data all the time. Find the key messages and express them succinctly in text. Look for examples that underscore those messages. And incorporate images and other visuals that create emotional connections. 11.
Use a formula to communicate your analysis with as few words as possible. Keep it simple. Resist the urge to over-complicate your presentation. A word cloud is not a word cloud. A bar chart is not a bar chart. If you use a word cloud to illustrate a chart, consider replacing a few words with a gif.
Among various types of data presentation, tabular is the most fundamental method, with data presented in rows and columns. Excel or Google Sheets would qualify for the job. Nothing fancy. This is an example of a tabular presentation of data on Google Sheets.
Here are 10 data presentation tips to effectively communicate with executives, senior managers, marketing managers, and other stakeholders. 1. Choose a Communication Style. Every data professional has a different way of presenting data to their audience. Some people like to tell stories with data, illustrating solutions to existing and ...
Data presentation is a process of comparing two or more data sets with visual aids, such as graphs. Using a graph, you can represent how the information relates to other data. This process follows data analysis and helps organise information by visualising and putting it into a more readable format. This process is useful in nearly every ...
Overview. Data analysis is an ongoing process that should occur throughout your research project. Suitable data-analysis methods must be selected when you write your research proposal. The nature of your data (i.e. quantitative or qualitative) will be influenced by your research design and purpose. The data will also influence the analysis ...
DATA PRESENTATION, ANALYSIS AND INTERPRETATION. 4.0 Introduction. This chapter is concerned with data pres entation, of the findings obtained through the study. The. findings are presented in ...
Data Analysis and Data Presentation have a practical implementation in every possible field. It can range from academic studies, commercial, industrial and marketing activities to professional practices. In its raw form, data can be extremely complicated to decipher and in order to extract meaningful insights from the data, data analysis is an important step towards breaking down data into ...
analysis to use on a set of data and the relevant forms of pictorial presentation or data display. The decision is based on the scale of measurement of the data. These scales are nominal, ordinal and numerical. Nominal scale A nominal scale is where: the data can be classified into a non-numerical or named categories, and
What is data-driven analysis? Data-driven analysis is the process of using data to make strategic decisions or gain insights. It involves collecting, analyzing, and interpreting data to reveal patterns, trends, and relationships between components.. By leveraging statistical tools and software, data-driven companies are able to make evidence-based decisions, enhancing accuracy and reducing biases.
Data Analysis. Definition: Data analysis refers to the process of inspecting, cleaning, transforming, and modeling data with the goal of discovering useful information, drawing conclusions, and supporting decision-making. It involves applying various statistical and computational techniques to interpret and derive insights from large datasets.
Master the art of analysing and interpreting data for your dissertation with our comprehensive guide. Learn essential techniques for quantitative and qualitative analysis, data preparation, and effective presentation to enhance the credibility and impact of your research.
Gather and clean your data: Prepare it for analysis. Explore your data: Look for patterns and relationships. Hands-on experience is key to becoming a data scientist. Projects help you: Apply what you've learned; Develop practical skills; Show your abilities to potential employers; Common tools for building data science projects
How to write Data analysis in Research. Data analysis is crucial for interpreting collected data and drawing meaningful conclusions. Follow these steps to write an effective data analysis section in your research. 1. Prepare Your Data. Ensure your data is clean and organized: Remove duplicates and irrelevant data. Check for errors and correct them.
Design the Presentation of your Data; Create an OTBI Analysis Report or a Saved Search. This section covers: Creating an OTBI analysis report; Creating a Saved Search; Creating an OTBI analysis report. For this example, we'll create an OTBI analysis report on Customer Overview in CRM:
PRESENTATION SESSION III (2:40 - 3:25 p.m.) Student Name Poster Title Program Faculty Mentor Poster Number; Arianna Escandon & Waad Abdella: Quantifying natural variation in algae elemental composition: testing a hypothesis linking ploidy and nutrient availability
In the present study we performed a computational analysis to highlight the role of mutations in different GBM-related proteins and the peptide presentation in the context of MHC-I receptors.
Seek out innovative approaches to data analysis and presentation to improve reporting to internal and external partners. Work with the Senior Data Analyst to identify how lessons learned from the ISPF reporting and analysis can be cascaded to wider monitoring and evaluation work throughout the British Academy.