Generative AI for Educators
As a teacher, we know your time is valuable and student needs are broad. With Generative AI for Educators, you’ll learn how to use generative AI tools to help you save time on everyday tasks, personalize instruction, enhance lessons and activities in creative ways, and more.
- Built by AI experts at Google in collaboration with MIT RAISE
- No previous experience necessary
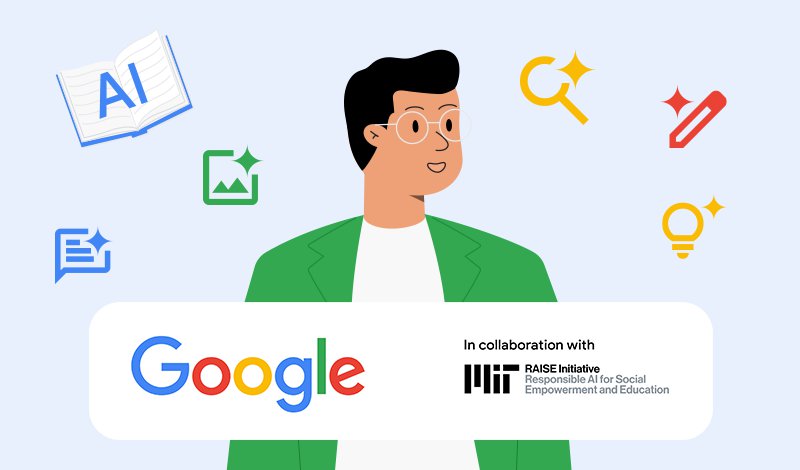
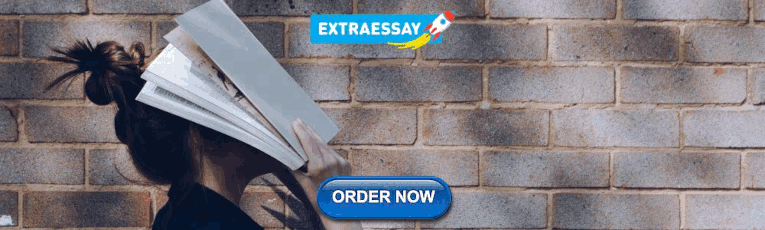
Flexible AI training designed for teachers
This self-paced course fits into a teacher’s busy schedule with flexibility in mind. It offers hands-on, practical experience for teachers across disciplines.
online, self-paced learning
to complete the course
Learn more about the course
Developed by AI experts at Google in collaboration with MIT RAISE , this course will help you bring AI into your practice. You’ll also gain a foundational understanding of AI — you'll learn what it is, the opportunities and limitations of this technology, and how to use it responsibly.
You’ll learn how to use generative AI tools to:
- Save time on everyday tasks like drafting emails and other correspondence
- Personalize instruction for different learning styles and abilities
- Enhance lessons and activities in creative ways
More details
Close details, save time and enhance student learning with ai skills.
When you complete the online course, you’ll gain essential AI skills you can apply to your workflow immediately. By using AI as a helpful collaboration tool, you can work smarter, not harder.

Earn a certificate which you can present to your district for professional development (PD) credit, depending on district and state requirements.

Get hands-on experience using generative AI tools and apply your new skills right away.
Bring this course to your school or district
If you’re a school or district leader, we’ve designed this course to fit into a teacher’s standard school day. The five modules are each thirty minutes or less, allowing teachers to fit the training into professional development or planning periods.
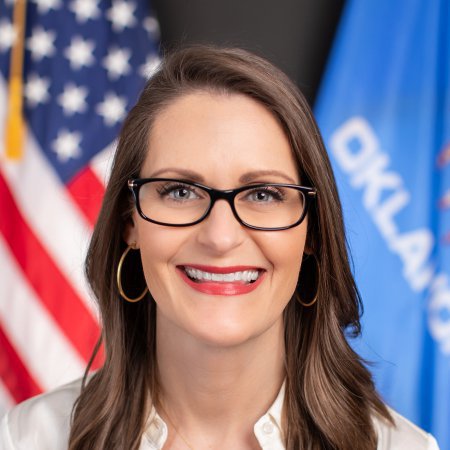
Nellie Tayloe Sanders
Oklahoma Secretary of Education
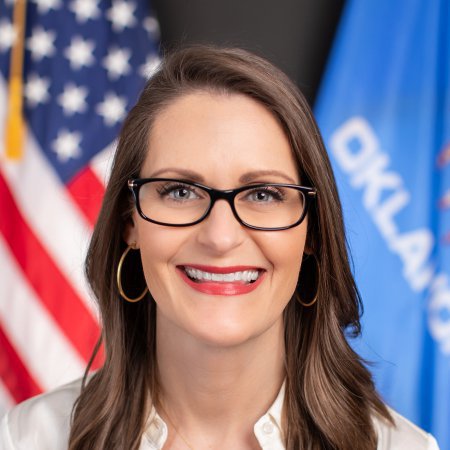
Jose L. Dotres, Superintendent of Schools
Miami-Dade County Public Schools
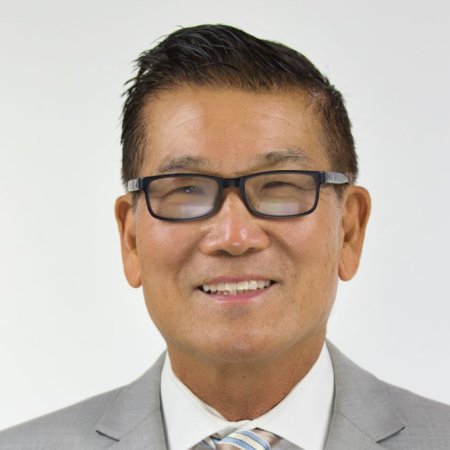
Michael Matsuda, Superintendent of Schools
Anaheim Union High School District
Frequently asked questions
Why enroll in generative ai for educators.
Generative AI for Educators is a two-hour, self-paced course designed to help teachers save time on everyday tasks, personalize instruction to meet student needs, and enhance lessons and activities in creative ways with generative AI tools — no previous experience required. Developed by experts at Google in collaboration with MIT RAISE (Responsible AI for Social Empowerment and Education), this no cost course is built for teachers across disciplines and provides practical, hands-on experience. After completing the course, teachers earn a certificate from the course, which they can present to their district for professional development (PD) credit, depending on district and state requirements.
Who is the Generative AI for Educators course for?
Generative AI for Educators is designed for high school and middle school educators of any subject, with no technical experience required. Any teacher who is interested in using AI to save time and enhance student education could benefit from this course.
How are the skills I learn applicable to my work?
This generative AI course for teachers will provide practical applications that help save time by increasing efficiency, productivity, and creativity. The course includes hands-on experience using generative AI tools to do things like write class correspondence (messages, emails, newsletters), create assessments and provide feedback, differentiate instruction to meet various student needs, and create instructional strategies to make lessons more engaging for students.
What will I get when I finish?
After completing the course, teachers earn a certificate that they can present to their district for professional development (PD) credit, depending on district and state requirements.
How much does Generative AI for Educators cost?
The Generative AI for Educators course is provided at no cost.
Who designed Generative AI for Educators?
Generative AI for Educators was designed by AI experts at Google in collaboration with MIT RAISE (Responsible AI for Social Empowerment and Education).
In what languages is Generative AI for Educators available?
Generative AI for Educators is currently available in English, and we are working to offer this course in additional languages. Please check back here for updates.
Looking to help your middle school students learn AI skills?
Check out Experience AI . Created by Google DeepMind and The Raspberry Pi Foundation, this no cost program provides ready-to-use resources to introduce AI technology in middle school classrooms, including lesson plans, slide decks, worksheets, and videos on AI and machine learning.
What is generative AI?
Generative AI is artificial intelligence that can generate new content, such as text, images, or other media.
Stay up to date on Google Career Certificates
By clicking subscribe, you consent to receive email communication from Grow with Google and its programs. Your information will be used in accordance with Google Privacy Policy and you may opt out at any time by clicking unsubscribe at the bottom of each communication.
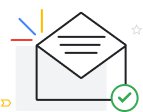
Thanks for subscribing to the Grow with Google newsletter!
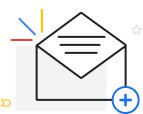
Subscribe to discover even more ways to grow
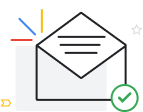
This site uses cookies from Google to deliver its services and to analyze traffic.
for Education
- Google Classroom
- Google Workspace Admin
- Google Cloud
Advancing education with AI
Google is committed to making AI helpful for everyone, in the classroom and beyond.
Bold technology, applied responsibly
AI can never replace the expertise, knowledge or creativity of an educator — but it can be a helpful tool to enhance and enrich teaching and learning experiences. As part of our Responsible AI practices , we use a human-centered design approach. And when it comes to building tools for education, we are especially thoughtful and deliberate.
Elevate educators
AI can help educators boost their creativity and productivity, giving them time back to invest in themselves and their students.
More security with Gmail and ChromeOS
With 99.9% of spam, phishing attempts, and malware blocked by AI-powered detection and zero reported ransomware attacks on any ChromeOS device, we make security our top priority.
More productivity and creativity with Gemini
With Gemini , educators have an AI assistant that can help them save time, get inspired with fresh ideas, and create captivating learning experiences for every student.
More interactivity with YouTube videos in Classroom
Educators can make learning more engaging through video lessons, and save time with AI-suggested questions for YouTube videos in Classroom (coming soon).
Make learning personal for students
AI can help meet students where they are, so they can learn in ways that work for them.
More supportive with practice sets
Practice sets in Google Classroom enables educators to automatically provide their students with real-time feedback and helpful in-the-moment hints if they get stuck.
More accessible with Chromebooks
AI built into Chromebooks provides advanced text-to-speech, dictation, and live and closed captions.
More adaptive with Read Along in Classroom
The Read Along integration with Classroom provides real-time feedback on pronunciation to help build reading skills at a personal pace.
AI training, toolkits and guides for educators
Guardian's Guide to AI
- Explore the guide
Generative AI for Educators
- Explore training
Applied Digital Skills: Discover AI in Daily Life
A Guide to AI in Education
PILOT PROGRAM
AI Track of the Google for Education Pilot Program
- Express interest
Teach AI's AI Guidance for Schools Toolkit
- Explore toolkit
How Google for Education Champions Use AI
- Watch videos
Future of Education Global Research Report
- Explore the findings
Experience AI, Raspberry Pi, and Google DeepMind
Grow with Google: AI and Machine Learning Courses
Google Cloud Skills Boost: Intro to Gen AI Learning
Introduction to Machine Learning
- Explore learning module
EXPLORATION
Google Arts & Culture: Overview of AI
Teaching for tomorrow.
A Google for Education YouTube series, featuring conversations with thought leaders who are shaping the future of education.
- Watch the full playlist
Season 2 Trailer
In season 2 of Teaching for Tomorrow, educational thought leaders talk about the potential of AI to transform teaching and learning, from elevating educators to making learning more inclusive and personal for students.
- Watch video
Interview with David Hardy
David Hardy, CEO of All-365 and Made by Change, explains how digital technology can help make education and learning more inclusive.
Interview with Lisa Nielsen
Lisa Nielsen, Founder of The Innovative Educator, explains how generative AI can help teachers spend more time with students, personalize learning, and connect their classrooms to the world.
How Google is making AI helpful for everyone
Learn more about our company approach to developing and harnessing ai..
- Visit Google AI
Have questions? We’ve got answers
Safety and privacy
How does Google keep a student’s data safe and secure?
Google Workspace for Education is built on our secure, reliable, industry-leading technology infrastructure. Users get the same level of security that Google uses to protect our own services, which are trusted by over a billion users around the world every day. While AI capabilities introduce new ways of interacting with our tools, our overarching privacy policies and practices help keep users and organizations in control of their data. In addition, all core Workspace tools – like Gmail, Google Calendar, and Classroom – meet rigorous local, national, and international compliance standards, including GDPR, FERPA, and COPPA. Chromebooks are designed with multiple layers of security to keep them safe from viruses and malware without any additional software required. Each time a Chromebook powers on, security is checked. And because they can be managed centrally, Chromebooks make it easy for school IT administrators to configure policies and settings, like enabling safe browsing or blocking malicious sites.
Is Google Workspace for Education data used to train Google’s generative AI tools like Gemini and Search?
No. When using Google Workspace for Education Core Services, your customer data is not used to train or improve the underlying generative AI and LLMs that power Gemini, Search, and other systems outside of Google Workspace without permission. And prompts entered when interacting with tools like Gemini are not used without permission beyond the context of that specific user session.
How does Google ensure its AI-enabled technology is safe for kids?
Google takes the safety and security of its users very seriously, especially children. With technology as bold as AI, we believe it is imperative to be responsible from the start. That means designing our AI features and products with age-appropriate experiences and protections that are backed by research. And prior to launching any product, we conduct rigorous testing to ensure that our tools minimize potential harms, and work to ensure that a variety of perspectives are included to identify and mitigate unfair bias.
What is Google’s approach to privacy with AI in education?
Across Google Cloud & Google Workspace, we’ve long shared robust privacy commitments that outline how we protect user data and prioritize privacy. AI doesn’t change these commitments - it actually reaffirms their importance. We are committed to preserving our customers’ privacy with our AI offerings and to supporting their compliance journey. Google Cloud has a long-standing commitment to GDPR compliance, and AI is no different in how we incorporate privacy-by-design and default from the beginning. We engage regularly with customers, regulators, policymakers, and other stakeholders as we evolve our offering to get their feedback for Edu AI offerings which process personal data.
Partnership and resources
Does Google consult with educators and experts when developing AI tools for use in the classroom?
Yes. A big component of being thoughtful with new technology is our commitment to partnering with schools and educators, as well as other education experts (like learning scientists) and organizations, along the way. We don’t just build for educators, we build with them. Through our Customer Advisory Boards and Google for Education Pilot Program, we also work directly with school communities around the world to gather feedback on our products and features before making them widely available. By listening to their perspectives, understanding how they’re using our tools, and addressing their challenges, we can be thoughtful in our product development and implementation. We also roll out new features gradually, ensuring that schools can stay in control of what works best for them.
What resources are being provided by Google to educate teachers on AI?
Teams across Google are actively creating and curating content and tutorials. Here are a few of our favorites, with more on the way: Generative AI for Educators A Guide to AI in Education Grow with Google: AI and machine learning courses Applied Digital Skills: Discover AI in Daily Life Google Cloud Skills Boost: Intro to Gen AI Learning Path Introduction to Machine Learning Google Arts & Culture: overview of AI Experience AI
You're now viewing content for a different region.
For content more relevant to your region, we suggest:
Sign up here for updates, insights, resources, and more.
If you're seeing this message, it means we're having trouble loading external resources on our website.
If you're behind a web filter, please make sure that the domains *.kastatic.org and *.kasandbox.org are unblocked.
To log in and use all the features of Khan Academy, please enable JavaScript in your browser.
AI for education
How will ai shape the future of education, unit 1: getting started with generative ai, unit 2: getting ready to teach with ai, unit 3: lesson plans: ai literacy | common sense education.
Educating in a World of Artificial Intelligence
- Posted February 9, 2023
- By Jill Anderson
- Learning Design and Instruction
- Teachers and Teaching
- Technology and Media

Senior Researcher Chris Dede isn't overly worried about growing concerns over generative artificial intelligence, like ChatGPT, in education. As a longtime researcher on emerging technologies, he's seen many decades where new technologies promised to upend the field. Instead, Dede says artificial intelligence requires educators to get smarter about how they teach in order to truly take advantage of what AI has to offer.“The trick about AI is that to get it, we need to change what we're educating people for because if you educate people for what AI does well, you're just preparing them to lose to AI. But if you educate them for what AI can't do, then you've got IA [Intelligence Augmentation],” he says. Dede, the associate director of research for the National AI Institute for Adult Learning and Online Education , says AI raises the bar and it has the power to significantly impact learning in powerful ways.
In this episode of the Harvard EdCast, Dede talks about how the field of education needs to evolve and get smarter, in order to work with — not against — artificial intelligence.
ADDITIONAL RESOURCES
- Dede's keynote on Intelligence Augmentation , delivered at an AI and Education conference
- Brief on Intelligence Augmentation, co-authored by Dede for HGSE’s Next Level Lab
Jill Anderson: I'm Jill Anderson. This is the Harvard EdCast.
Chris Dede thinks we need to get smarter about using artificial intelligence and education. He has spent decades exploring emerging learning technologies as a Harvard researcher. The recent explosion of generative AI, like ChatGPT, has been met with mixed reactions in education. Some public school districts have banned it. Some colleges and universities have tweaked their teaching and learning already.
Generative AI raises que
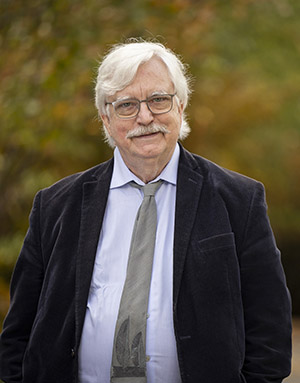
Chris Dede: I've actually been working with AI for more than half a century. Way back when when I was a graduate student, I read the first article on AI in education, which was published in 1970. And the author confidently predicted that we wouldn't need teachers within five or six years because AI was going to do everything. And of course, we still see predictions like that today.
But having lived through nine hype cycles for AI, I'm both impressed by how much it's advanced, but I'm also wary about elaborate claims for it. And there is a lot of excitement now about generative AI is the term that people are using, which includes programs like ChatGPT. It includes things like Dolly that are capable of creating images. It includes really AI on its own doing performances that we previously would have thought were something that people would have to do.
But it's interesting to compare ChatGPT to a search engine. And people don't remember this, but there was a time when-- before search engines when people really struggled to find resources, and there was enormous excitement when search engines came out. And search engines are, in fact, AI. They are based on AI at the back end, coming up with lists of things that hopefully match what you typed in. In fact, the problem with the search engine becomes not trying to find anything, but trying to filter everything to decide what's really useful.
So you can think of ChatGPT as the next step beyond a search engine where instead of getting a list of things and then you decide which might be useful and you examine them, you get an answer that says, this is what I think you want. And that is really more the AI taking charge than it is the AI saying, I can help you. Here's some things that you might look at and decide about. That makes me wary because AI is not at a stage where it really understands what it's saying.
And so it will make up things when it doesn't know them, kind of a not very good student seeing if they can fake out the teacher. And it will provide answers that are not customized to somebody's culture or to somebody's reading level or to somebody's other characteristics. So it's really quite limited.
I know that Harvard has sent some wording out that I've now put into my syllabi about students being welcome to use whatever tools they want. But when they present something as their work, it has to be something that they wrote themselves. It can't be something that somebody else wrote, which is classic plagiarism. It can't be something that Chat AI wrote that they're presenting as their work and so on. I think that what Chat AI does is it raises the bar for human performance.
I know a lot about what people are going through now in terms of job interviews because my older daughter is an HR manager, and my younger daughter just graduated. And she's having a lot of job interviews. And in contrast to earlier times, now, job interviews typically involve a performance.
If you're going to be hired for a marketing position, they'll say bring in a marketing plan when we do our face-to-face interview on this, and we'll evaluate it. Or in her case, in mechanical engineering, they say when you come in, there's this system that you're going to have a chance to debug, and we'll see how well you do it. Those employers are going to type the same thing into Chat AI. And if someone comes in with something that isn't any better than Chat AI, they're not going to get hired because why hire somebody that can't outcompete a free resource?
Jill Anderson: Oh interesting.
Chris Dede: So it raises the bar for human performance in an interesting way.
Jill Anderson: Your research looks at something called intelligence augmentation. I want to know what that means and how that's different from artificial intelligence.
Chris Dede: Intelligence augmentation is really about the opposite of this sort of negative example I was describing where now you've got to outthink Chat AI if you want to get a job. It says, when is the whole more than the sum of the parts? When do a person and AI working together do things that neither one could do as well on their own?
And often, people think, well, yeah, I can see a computer programmer, there might be intelligence augmentation because I know that machines can start to do programming. What they don't realize is that it applies to a wide range of jobs, including mine, as a college professor. So I am the associate director for research in a national AI institute funded by the National Science Foundation on adult learning and online education. And one of the things the Institute is building is AI assistants for college faculty.
So there's question answering assistants to help with student questions, and there's tutoring assistants and library assistants and laboratory assistants. There's even a social assistant that can help students in a large class meet other students who might be good learning partners. So now, as a professor, I'm potentially surrounded by all these assistants who are doing parts of my job, and I can be deskilled by that, which is a bad future. You sort of end up working for the assistant where they say, well, here's a question I can't answer.
So you have to do it. Or you can upskill because the assistant is taking over routine parts of the job. And in turn, you can focus much more deeply on personalization to individual students, on bringing in cultural dimensions and equity dimensions that AI does not understand and cannot possibly help with. The trick about AI is that to get it, we need to change what we're educating people for because if you educate people for what AI does well, you're just preparing them to lose to AI. But if you educate them for what AI can't do, then you've got IA.
Jill Anderson: So that's the goal here. We have to change the way that we're educating young people, even older people at this point. I mean, everybody needs to change the way that they're learning about these things and interacting with them.
Chris Dede: They do. And we're hampered by our system of assessment because the assessments that we use, including Harvard with the GRE and the SAT and so on, those are what AI does well. AI can score really well on psychometric tests. So we're using the wrong measure, if you will. We need to use performance assessments to measure what people can do to get into places like Harvard or higher education in general because that's emphasizing the skills that are going to be really useful for them.
Jill Anderson: You mentioned at the start artificial intelligence isn't really something brand new. This has been around for decades, but we're so slow to adapt and prepare and alter the way that we do things that once it reaches kind of the masses, we're already behind.
Chris Dede: Well, we are. And the other part of it is that we keep putting old wine in new bottles. I mean, this is — if I had to write a headline for the entire history of educational technology, it would be old wine in new bottles. But we don't understand what the new bottle really means.
So let me give you an example of something that I think generative AI could make a big difference, be very powerful, but I'm not seeing it discussed in all the hype about generative AI. And that is evidence-based modeling for local decisions. So let's take climate change.
One of the problems with climate change is that let's say that you're in Des Moines, Iowa, and you read about all this flooding in California. And you say to yourself, well, I'm not next to an ocean. I don't live in California. And I don't see why I should be that worried about this stuff.
Now, no one has done a study, I assume, of flooding in Des Moines, Iowa, in 2050 based on mid-level projections about climate change. But with generative AI, we can estimate that now.
Generative AI can reach out across topographic databases, meteorological databases, and other related databases to come up with here's the parts of Des Moines that are going to go underwater in 2050 and here's how often this is going to happen if these models are correct. That really changes the dialogue about climate change because now you're talking about wait a minute. You mean that park I take my kids to is going to have a foot of water in it? So I think that kind of evidence-based modeling is not something that people are doing with generative AI right now, but it's perfectly feasible. And that's the new wine that we can put in the new bottle.
Jill Anderson: That's really a great way to use that. I mean, and you could even use that in your classroom. Something that you said a long, long time ago was that — and this is paraphrasing — the idea that we often implement new technology, and we make this mistake of focusing on students first rather than teachers. Chris Dede: In December, I gave a keynote at a conference called Empowering Learners for the Age of AU that has been held the last few years. And one of the things I talked about was the shift from teaching to learning. Both are important, but teaching is ultimately sort of pouring knowledge into the minds of learners. And learning is much more open ended, and it's essential for the future because every time you need to learn something new, you can't afford to go back and have another master's degree. You need to be able to do self-directed learning.
And where AI can be helpful with this is that AI can be like an intellectual partner, even when you don't have a teacher that can help you learn in different ways. One of the things that I've been working on with a professor at the Harvard Business School is AI systems that can help you learn negotiation.
Now, the AI can't be the person you're negotiating with. AI is not good at playing human beings — not yet and not for quite a long time, I think. But what AI can do is to create a situation where a human being can play three people at once. So here you are. You're learning how to negotiate a raise.
You go into a virtual conference room. There's three virtual people who are three bosses. There's one simulation specialist behind all three, and you negotiate with them. And then at the end, the system gives you some advice on what you did well and not so well.
And if you have a human mentor, that person gives you advice as well. Ronda Bandy, who was a professor in HGSE until she moved to Hunter College, she and I have published five articles on the work we did for the HGSE's Reach Every Reader Project on using this kind of digital puppeteering to help teachers practice equitable discussion leading. So again, here's something that people aren't talking about where AI on the front end can create rich evocative situations, and AI and machine learning on the back end can find really interesting patterns for improvement.
Jill Anderson: You know, Chris, how hard is it to get there for educators?
Chris Dede: I think, in part, that's what these national AI institutes are about. Our institute, which is really adult learning with a workplace focus, is looking at that part of the spectrum. There's another institute whose focus is middle school and high school and developing AI partners for students where the student and the partner are learning together in a different kind of IA. There's a third Institute that's looking at narrative and storytelling as a powerful form of education and how can AI help with narrative and storytelling.
You can imagine sitting down. Mom and dad aren't around. You've got a storybook like Goldilocks and the Three Bears, and you've got something like Alexa that can listen to what you're reading and respond.
And so you begin, and you say, Goldilocks went out of her house one day and went into the woods and got lost. And Alexa says, why do you think Goldilocks went into the woods? Was she a naughty girl? No. Or was she an adventurous girl, or was she deeply concerned about climate change and wanting to study ecosystems?
I mean, I'm being playful about this, but I think the point is that AI doesn't understand any of the questions that it's asking but it can ask the questions, and then the child can start to think deeper than just regurgitating the story. So there's all sorts of possibilities here that we just have to think of as new wine instead of asking how can AI automate our order thinking about teaching and learning.
Jill Anderson: I've been hearing a lot of concern about writing in particular-- writing papers where young people are actually expressing their own ideas, concerns about plagiarism and cheating, which I would say the latter have long existed as challenges in education, aren't really a new one. Does AI really change this? And how might a higher ed or any educator really look at this differently?
Chris Dede: So I think where AI changes this is it helps us understand the kind of writing that we should be teaching versus the kind of writing that we are teaching. So I remember preparing my children for the SAT, and it used to have something called the essay section. And you had to write this very formal essay that was a certain number of paragraphs, and the topic sentences each had to do this and so on.
Nobody in the world writes those kinds of essays in the real world. They're just like an academic exercise. And of course, AI now can do that beautifully.
But any reporter will tell you that they could never use Chat AI to write their stories because stories is what they write. They write narratives. If you just put in a description, you'll be fired from your reportorial job because no one is interested in descriptions. They want a story.
So giving students a description and teaching them to turn it into a story or teaching them to turn it into something else that has a human and creative dimension for it, how would you write this for a seventh-grader that doesn't have much experience with the world? How would you write this for somebody in Russia building on the foundation of what AI gives you and taking it in ways that only people can? That's where writing should be going.
And of course, good writing teachers will tell you, well, that's nothing new. I've been teaching my students how to write descriptive essays. The people who are most qualified to talk about the limits of AI are the ones who teach what the AI is supposedly doing.
Jill Anderson: So do you have any helpful tips for educators regardless of what level they're working at on where to kind of begin embracing this technology?
Chris Dede: What AI can do well is what's called reckoning, which is calculative prediction. And I've given some examples of that with flooding in Des Moines and other kinds of things. And what people do is practical wisdom, if you will, and it involves culture and ethics and what it's like to be embodied and to have the biological things that are part of human nature and so on.
So when I look at what I'm teaching, I have to ask myself, how much of what I'm teaching is reckoning? So I'm preparing people to lose to AI. And how much of what I'm teaching is practical wisdom?
So for example, we spend a lot of time in vocational technical education and standard academic education teaching people to factor. How do you factor these complex polynomials?
There is no workplace anywhere in the world, even in the most primitive possible conditions, where anybody makes a living by factoring. It's an app. It's an app on a phone. Should you know a little bit about factoring so it's not magic? Sure.
Should you become fluent in factoring? Absolutely not. It's on the wrong side of the equation. So I think just teachers and curriculum developers and assessors and stakeholders in the outcomes of education need to ask themselves, what is being taught now, and which parts of it are shifting over? And how do we include enough about those parts that AI isn't magic? But how do we change the balance of our focus to be more on the practical wisdom side?
Jill Anderson: So final thoughts here — don't be scared but figure out how to use this to your advantage?
Chris Dede: Yeah, don't be scared. AI is not smart. It really isn't. People would be appalled if they knew how little AI understands what it's telling you, especially given how much people seem to be relying on it. But it is capable of taking over parts of what you do that are routine and predictable and, in turn, freeing up the creative and the innovative and the human parts that are really the rewarding part of both work the life.
EdCast: Chris Dede is a senior research fellow at the Harvard Graduate School of Education. He is also a co-principal investigator of the National Artificial Intelligence Institute in adult learning and online education. I'm Jill Anderson. This is the Harvard EdCast produced by the Harvard Graduate School of Education. Thanks for listening. [MUSIC PLAYING]
Subscribe to the Harvard EdCast.

An education podcast that keeps the focus simple: what makes a difference for learners, educators, parents, and communities
Related Articles
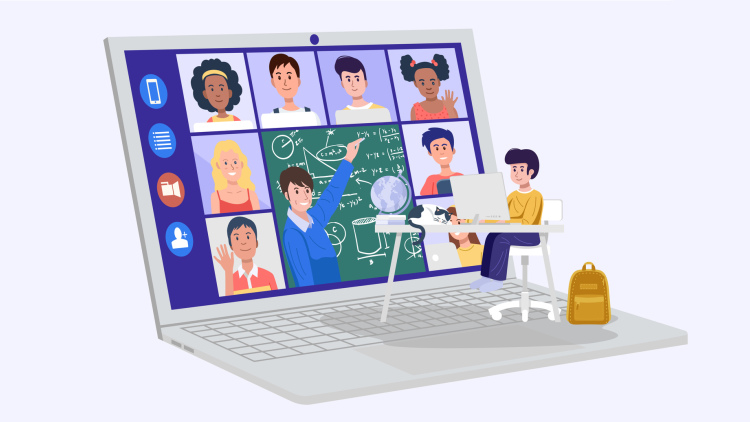
Sal Khan on Innovations in the Classroom
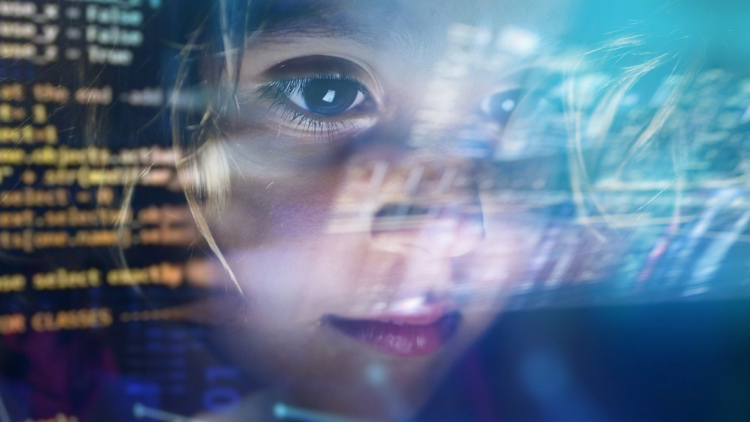
Embracing Artificial Intelligence in the Classroom
Generative AI tools can reflect our failure of imagination and that is when the real learning starts

Learning in Digital Worlds
- Become a Member
- Artificial Intelligence
- Computational Thinking
- Digital Citizenship
- Edtech Selection
- Global Collaborations
- STEAM in Education
- Teacher Preparation
- ISTE Certification
- School Partners
- Career Development
- ISTELive 24
- 2024 ASCD Annual Conference
- Solutions Summit
- Leadership Exchange
- 2024 ASCD Leadership Summit
- Edtech Product Database
- Solutions Network
- Sponsorship & Advertising
- Sponsorship & Advertising
Artificial Intelligence in Education
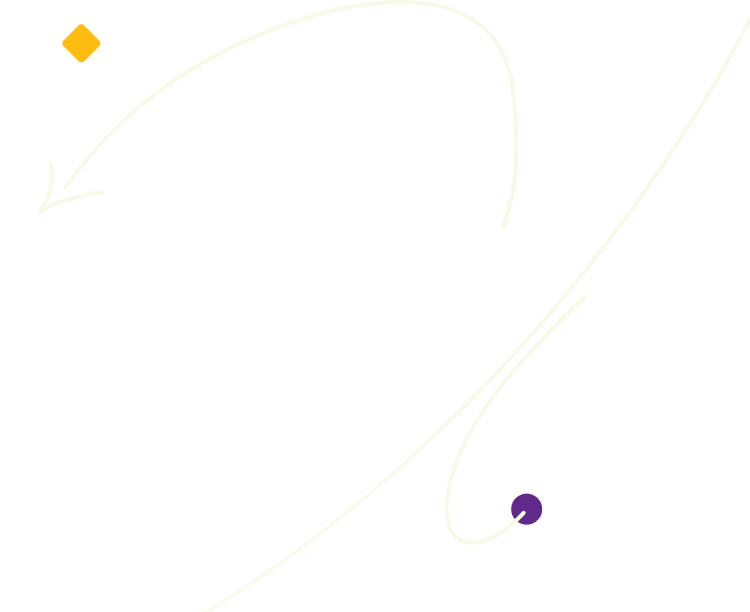
To prepare students to thrive as learners and leaders of the future, educators must become comfortable teaching with and about Artificial Intelligence. Generative AI tools such as ChatGPT , Claude and Midjourney , for example, further the opportunity to rethink and redesign learning. Educators can use these tools to strengthen learning experiences while addressing the ethical considerations of using AI. ISTE is the global leader in supporting schools in thoughtfully, safely and responsibly introducing AI in ways that enhance learning and empower students and teachers.
Interested in learning how to teach AI?
Sign up to learn about ISTE’s AI resources and PD opportunities.

StretchAI: An AI Coach Just for Educators
ISTE and ASCD are developing the first AI coach specifically for educators. With Stretch AI, educators can get tailored guidance to improve their teaching, from tips on ways to use technology to support learning, to strategies to create more inclusive learning experiences. Answers are based on a carefully validated set of resources and include the citations from source documents used to generate answers. If you are interested in becoming a beta tester for StretchAI, please sign up below.
Leaders' Guide to Artificial Intelligence
School leaders must ensure the use of AI is thoughtful and appropriate, and supports the district’s vision. Download this free guide (or the UK version ) to get the background you need to guide your district in an AI-infused world.
UPDATED! Free Guides for Engaging Students in AI Creation
ISTE and GM have partnered to create Hands-On AI Projects for the Classroom guides to provide educators with a variety of activities to teach students about AI across various grade levels and subject areas. Each guide includes background information for teachers and student-driven project ideas that relate to subject-area standards.
The hands-on activities in the guides range from “unplugged” projects to explore the basic concepts of how AI works to creating chatbots and simple video games with AI, allowing students to work directly with innovative AI technologies and demonstrate their learning.
These updated hands-on guides are available in downloadable PDF format in English, Spanish and Arabic from the list below.
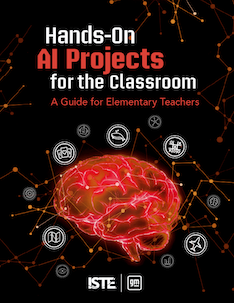
Artificial Intelligence Explorations for Educators unpacks everything educators need to know about bringing AI to the classroom. Sign up for the next course and find out how to earn graduate-level credit for completing the course.
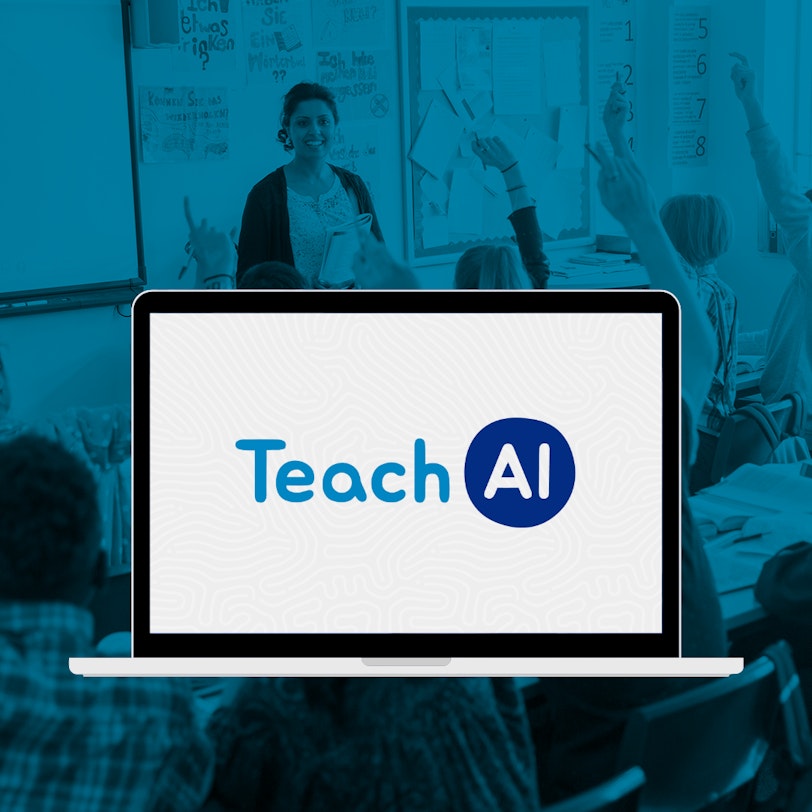
As a co-founder of TeachAI , ISTE provides guidance to support school leaders and policy makers around leveraging AI for learning.
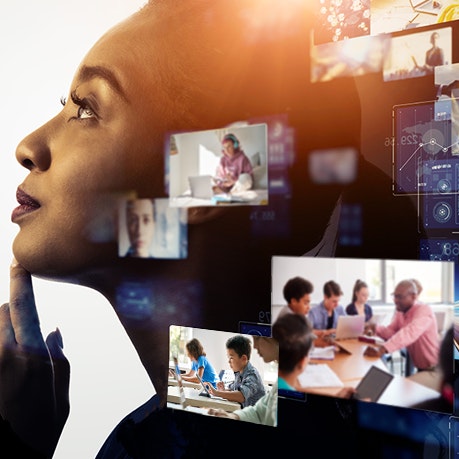
Dive deeper into AI and learn how to navigate ChatGPT in schools with curated resources and tools from ASCD and ISTE.
Join our Educator AI Community on Connect
ISTE+ASCD’s free online community brings together educators from around the world to share ideas and best practices for using artificial intelligence to support learning.
Learn More From These Podcasts, Blog Posts, Case Studies and Websites
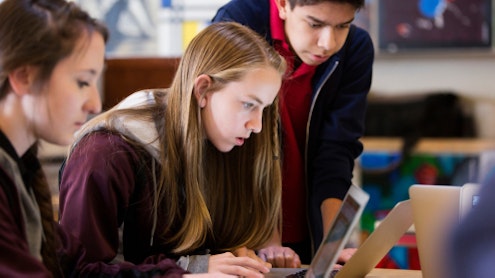
Partners Code.org, ETS, ISTE and Khan Academy offer engaging sessions with renowned experts to demystify AI, explore responsible implementation, address bias, and showcase how AI-powered learning can revolutionize student outcomes
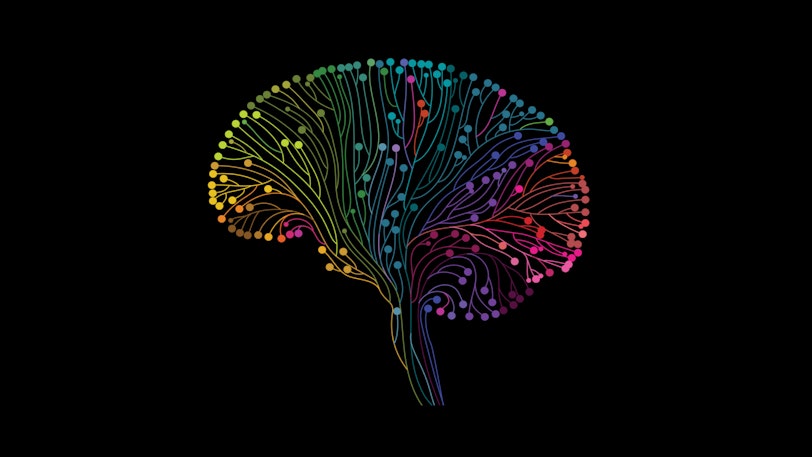
One of the challenges with bias in AI comes down to who has access to these careers in the first place, and that's the area that Tess Posner, CEO of the nonprofit AI4All, is trying to address.
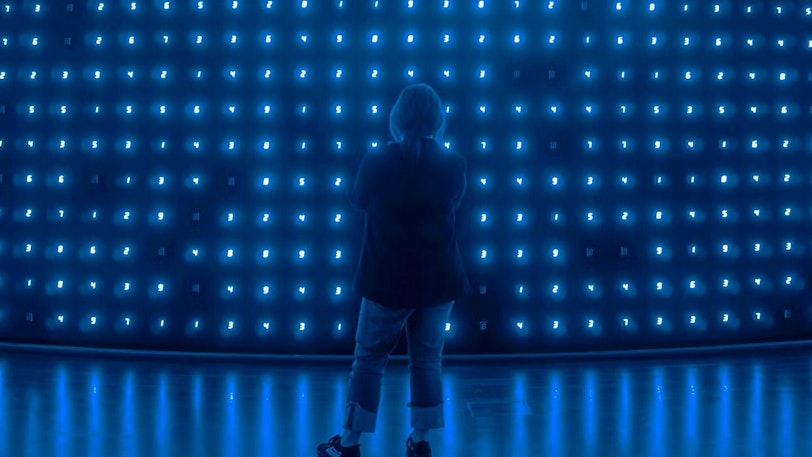
Featuring in-depth interviews with practitioners, guidelines for classroom teachers and a webinar about the importance of AI in education, this site provides K-12 educators with practical tools for integrating AI and computational thinking across their curricula.
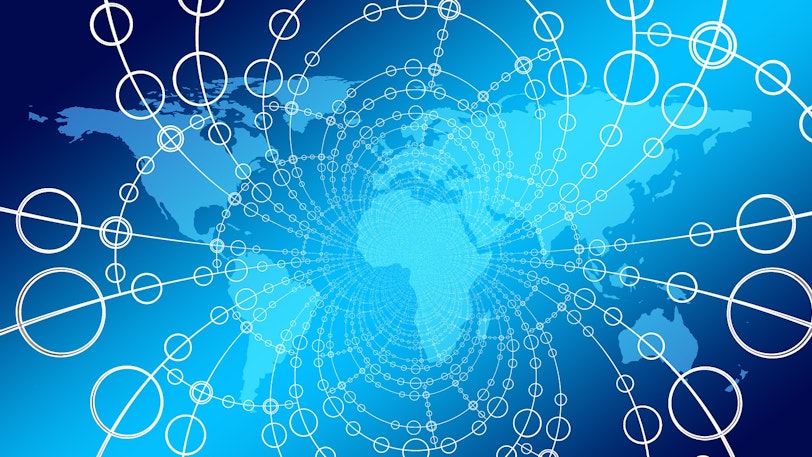
This 15-hour, self-paced introduction to artificial intelligence is designed for students in grades 9-12. Educators and students should create a free account at P-TECH before viewing the course.
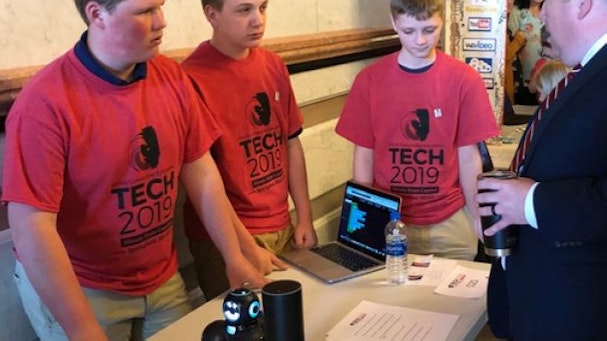
Explore More in the Learning Library
Explore more books, articles, and tools about artificial intelligence in the Learning Library.
- artificial intelligence
This browser is no longer supported.
Upgrade to Microsoft Edge to take advantage of the latest features, security updates, and technical support.
Empower educators to explore the potential of artificial intelligence
Navigate AI in education by looking at essential AI concepts, techniques, and tools, highlighting practical applications. AI can support personalized learning, automate daily tasks, and provide insights for data-driven decision making.
Learning objectives
Upon completion of this module, you'll be able to:
- Describe generative AI in the broader context of AI in terms of how these systems work and what they can do.
- Explain what a large language model (LLM) is and the basics of how it works.
- Use generative and summative capabilities of LLMs (generate text, expand from main points, condense into main points, answer questions based on given text, etc.).
- Summarize potential impacts.
- Explain how AI can be used to improve learning outcomes, reduce educator workload, and increase learner engagement.
- Explain how the use of AI can help learning be more accessible and inclusive.
ISTE Standards for Educators :
Educator - Designer
Educator - Learner
UNESCO Standards for Educators :
Application of Digital skills
Organization and Administration
Understanding ICT in Education
- Introduction min
- Introduction to AI min
- Generative AI min
- Large language models min
- Use AI-powered image generation capabilities effectively min
- AI in education min
- AI tools for educators and accessibility min
- Knowledge check min
- Summary min
Advertisement
Education for AI, not AI for Education: The Role of Education and Ethics in National AI Policy Strategies
- Published: 02 September 2021
- Volume 32 , pages 527–563, ( 2022 )
Cite this article
- Daniel Schiff ORCID: orcid.org/0000-0002-4376-7303 1
12k Accesses
49 Citations
10 Altmetric
Explore all metrics
As of 2021, more than 30 countries have released national artificial intelligence (AI) policy strategies. These documents articulate plans and expectations regarding how AI will impact policy sectors, including education, and typically discuss the social and ethical implications of AI. This article engages in thematic analysis of 24 such national AI policy strategies, reviewing the role of education in global AI policy discourse. It finds that the use of AI in education (AIED) is largely absent from policy conversations, while the instrumental value of education in supporting an AI-ready workforce and training more AI experts is overwhelmingly prioritized. Further, the ethical implications of AIED receive scant attention despite the prominence of AI ethics discussion generally in these documents. This suggests that AIED and its broader policy and ethical implications—good or bad—have failed to reach mainstream awareness and the agendas of key decision-makers, a concern given that effective policy and careful consideration of ethics are inextricably linked, as this article argues. In light of these findings, the article applies a framework of five AI ethics principles to consider ways in which policymakers can better incorporate AIED’s implications. Finally, the article offers recommendations for AIED scholars on strategies for engagement with the policymaking process, and for performing ethics and policy-oriented AIED research to that end, in order to shape policy deliberations on behalf of the public good.
This is a preview of subscription content, log in via an institution to check access.
Access this article
Price includes VAT (Russian Federation)
Instant access to the full article PDF.
Rent this article via DeepDyve
Institutional subscriptions
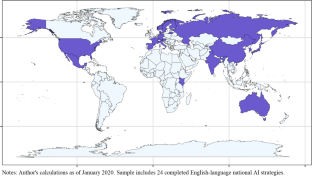
Similar content being viewed by others
Navigating the Implications of AI in Indonesian Education: Tutors, Governance, and Ethical Perspectives
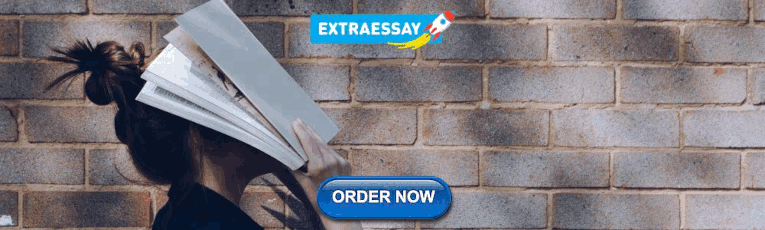
Ethical Guidelines for Artificial Intelligence-Based Learning: A Transnational Study Between China and Finland
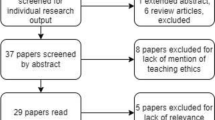
How to teach responsible AI in Higher Education: challenges and opportunities
Availability of data and material.
Not applicable
Code availability
Australia, Canada, Singapore, Denmark, Denmark, Taiwan, France, EU, UK, and South Korea have committed nearly 8 billion with the US contributing or planning to contribute at least 4 billion and China at least 14 billion. These are moving targets and low-end estimates, especially as private investment constitutes an even greater sum.
I do not include documents produced by intergovernmental bodies, such as the United Nations or European Union. While these documents are similar in nature, they are less tied to direct national institutions and strategies, and are therefore less analogous to the other documents. For further details and rationales regarding data collection and inclusion/exclusion criteria, please see Appendix 1 .
Further details about codebook development and iteration are available in Appendix 1 .
For example, Malta’s ( 2019 ) document initially notes that AI for healthcare may be amongst the highest impact projects and “most pressing domestic challenges” worthy of prioritization, but it does not proceed to include any substantive discussion or a subsection on healthcare. In comparison to the document’s discussion of other topics, and in comparison to other countries’ AI policy documents that discuss healthcare in more depth, this relatively more narrow treatment of the topic led to coding it as yellow. Similarly, Russia’s ( 2019 ) brief mention of using AI to “[improve] the quality of education services” does not provide enough detail to be clear about the role of AIED as a potential tool for teaching and learning, and so is considered too ambiguous to code as either green or red.
Any errors are the sole fault of the author.
I nevertheless captured these mentions in the research memo and discuss them at the end of this section.
Note that other policy sectors, like healthcare, also have dedicated agencies and other policy documents, but nevertheless receive more attention than education in AI policy strategies.
See The World Bank Country and Lending Groups classification for classifications by national income.
There are additional explanations to consider as well, though this study does not provide clear evidence to establish these. First, policymakers may simply never have been informed about AIED. AIED has typically been contained within an expert academic domain primarily accessible to computer scientists (Schiff 2021 ). Relatedly, while some AIED applications are beginning to hit mainstream classrooms, relatively few people, adults or children, have experience with them personally. In contrast, general education and its role in the labor market is something that nearly all members of society experience and can relate to, and a traditional focus of policymakers. Barring basic awareness, policymakers may not realize the transformative potential of AIED. If this is accurate, a clear prescription is to significantly increase efforts to inform policymakers about AIED and its ethical implications. This begs the question of whether the AIED community is prepared to do so, something which this article addresses in its Recommendations for AIED Researchers section.
The discussions of Spain, Mexico, Kenya, and India demonstrate how such a link between ethics and policy for AIED might be established even though most countries have not yet identified these connections explicitly.
Note that a similar approach has also been adopted by The Institute for Ethical AI in Education ( 2020 ), which, through a series of workshops and reports, has explored AIED policy by using a different AI ethics framework, the EU’s seven Ethics Guidelines for Trustworthy AI (European Commission 2019 ). This provides further support to the idea of approaching AI governance through an ethical lens.
See Schiff ( 2021 ), The Institute for Ethical AI in Education ( 2020 ), Holmes et al. ( 2021 ), and other articles in this issue for more detailed reviews of AIED ethics.
Acemoglu, D., & Restrepo, P. (2019). The wrong kind of AI? Artificial intelligence and the future of labor demand. Working Paper 25682. National Bureau of Economic Research. https://doi.org/10.3386/w25682 .
Adadi, A., & Berrada, M. (2018). Peeking inside the black-box: A survey on explainable artificial intelligence (XAI). IEEE Access, 6, 52138–52160. https://doi.org/10.1109/ACCESS.2018.2870052 .
Article Google Scholar
Ali, W. (2020). Online and remote learning in higher education institutes: A necessity in light of COVID-19 pandemic. Higher Education Studies, 10 (3), 16–25.
Allen, G. C. (2019). Understanding China’s AI strategy. Center for a New American Security (blog). February 6, 2019. https://www.cnas.org/publications/reports/understanding-chinas-ai-strategy .
Alper, J. and U.S. National Academies of Sciences, Engineering, and Medicine, eds. 2016. Developing a national STEM workforce strategy: A workshop summary . Washington, DC: The National Academies Press.
Amiel, T., & Reeves, T. C. (2008). Design-based research and educational technology: Rethinking technology and the research agenda. Journal of Educational Technology and Society, 11 (4), 29–40.
Google Scholar
Article 19. 2021. “Emotional Entanglement: China’s Emotion Recognition Market and Its Implications for Human Rights.” A19/DIG/2021/001. London, UK: Article 19. https://www.article19.org/wp-content/uploads/2021/01/ER-Tech-China-Report.pdf .
Autor, D. H. (2015). Why are there still so many jobs? the history and future of workplace automation. Journal of Economic Perspectives, 29 (3), 3–30. https://doi.org/10.1257/jep.29.3.3
Bagloee, S. A., Tavana, M., Asadi, M., & Oliver, T. (2016). Autonomous vehicles: Challenges, opportunities, and future implications for transportation policies. Journal of Modern Transportation, 24 (4), 284–303. https://doi.org/10.1007/s40534-016-0117-3
Beauchamp, T. L., & Childress, J. F. (1979). Principles of biomedical ethics . Oxford University Press.
Bellamy, R. K., Dey, K., Hind, M., Hoffman, S. C., Houde, S., Kannan, K., Lohia, P. et al. (2018). AI fairness 360: An extensible toolkit for detecting, understanding, and mitigating unwanted algorithmic bias. [Cs] , October. http://arxiv.org/abs/1810.01943 .
Blockchain & Artificial Intelligence Taskforce, Kenya. 2019. “Kenya: Emerging Digital Technologies for Kenya: Exploration & Analysis.” Nairobi, Kenya: Blockchain & Artificial Intelligence taskforce. https://www.ict.go.ke/blockchain/blockchain.pdf .
Boeglin, J. (2015). The costs of self-driving cars: reconciling freedom and privacy with tort liability in autonomous vehicle regulation. Yale JL & Tech., 17 , 171.
Boin, A. ’t Hart, P., & McConnell, A. (2009). Crisis exploitation: Political and policy impacts of framing contests. Journal of European Public Policy, 16 , 81-106. https://doi.org/10.1080/13501760802453221
Borenstein, J., & Arkin, R. C. (2017). Nudging for good: Robots and the ethical appropriateness of nurturing empathy and charitable behavior. AI & SOCIETY, 32 (4), 499–507.
Bozeman, B. (2002). Public-value failure: When efficient markets may not do. Public Administration Review, 62 (2), 145–161. https://doi.org/10.1111/0033-3352.00165
Bravo-Biosca, A. (2019). Experimental Innovation Policy. Working Paper 26273. National Bureau of Economic Research. https://doi.org/10.3386/w26273 .
British Embassy in Mexico, Oxford Insights, and C Minds. 2018. “Mexico: Towards an AI Strategy in Mexico: Harnessing the AI Revolution.” Mexico City, Mexico: British Embassy in Mexico, Oxford Insights, and C Minds. http://go.wizeline.com/rs/571-SRN-279/images/Towards-an-AI-strategy-in-Mexico.pdf .
Bucchi, M. (2013). Style in science communication. Public Understanding of Science, 22 (8), 904–915. https://doi.org/10.1177/0963662513498202
Buchanan, R. (2020). Through growth to achievement: Examining Edtech as a solution to australia’s declining educational achievement. Policy Futures in Education, 18 , 1026–1043. https://doi.org/10.1177/1478210320910293
Cairney, P., Oliver, K., & Wellstead, A. (2016). To bridge the divide between evidence and policy: reduce ambiguity as much as uncertainty. Public Administration Review, 76 (3), 399–402. https://doi.org/10.1111/puar.12555
Caliskan, A., Bryson, J. J., & Narayanan, A. (2017). Semantics derived automatically from language corpora contain human-like biases. Science, 356 (6334), 183–186. https://doi.org/10.1126/science.aal4230
Castelvecchi, D. (2016). Can we open the black box of AI? Nature News, 538 (7623), 20. https://doi.org/10.1038/538020a
Castleberry, A., & Nolen, A. (2018). Thematic analysis of qualitative research data: Is it as easy as it sounds? Currents in Pharmacy Teaching and Learning, 10 (6), 807–815. https://doi.org/10.1016/j.cptl.2018.03.019
Cath, C., Wachter, S., Mittelstadt, B., Taddeo, M., & Floridi, L. (2018). Artificial Intelligence and the ‘Good Society’: The US, EU, and UK approach. Science and Engineering Ethics, 24 (2), 505–528. https://doi.org/10.1007/s11948-017-9901-7
Chae, Y. (2020). U.S. AI regulation guide: Legislative overview and practical considerations. The Journal of Robotics, Artificial Intelligence and Law, 3 (1), 17–40.
MathSciNet Google Scholar
Char, D. S., Shah, N. H., & Magnus, D. (2018). Implementing machine learning in health care—addressing ethical challenges. The New England Journal of Medicine, 378 (11), 981–983. https://doi.org/10.1056/NEJMp1714229
Chatila, R., & Havens, J. C. (2019). The IEEE global initiative on ethics of autonomous and intelligent systems. In M. I. A. Ferreira, J. S. Sequeira, G. S. Virk, M. O. Tokhi, & E. E. Kadar (Eds.), Robotics and well-being (pp. 11–16). Cham: Springer.
Chapter Google Scholar
Chouldechova, A. (2017). Fair prediction with disparate impact: a study of bias in recidivism prediction instruments. [Cs, Stat] , February. http://arxiv.org/abs/1703.00056 .
Cooper, A. F., & Flemes, D. (2013). Foreign policy strategies of emerging powers in a multipolar world: An introductory review. Third World Quarterly, 34 (6), 943–962. https://doi.org/10.1080/01436597.2013.802501
Cuban, L. (1986). Teachers and machines: The classroom use of technology since 1920 . Teachers College Press.
Daly, A., Hagendorff, T., Li, H., Mann, M., Marda, V., Wagner, B., Wang, W., & Witteborn, S. (2019). Artificial Intelligence, Governance and Ethics: Global Perspectives. SSRN Scholarly Paper ID 3414805. Rochester, NY: Social Science Research Network. https://papers.ssrn.com/abstract=3414805 .
Dawson, D., E. Schleiger, J. Horton, J. McLaughlin, C. Robinson, G. Quezada, J. Scowcroft, & S. Hajkowicz. 2019. “Australia: Artificial Intelligence: Australia’s Ethics Framework.” Canberra, Australia: Data 61, CSIRO.
Dennis, M., Masthoff, J., & Mellish, C. (2016). Adapting progress feedback and emotional support to learner personality. International Journal of Artificial Intelligence in Education, 26 (3), 877–931. https://doi.org/10.1007/s40593-015-0059-7
Department of Public Administration, Italy. 2018. “Italy: Artificial Intelligence at the Service of Citizens.” Rome, Italy: Task Force on Artificial Intelligence of the Agency for Digital Italy and Department for Public Administration, Italy. https://ia.italia.it/assets/whitepaper.pdf .
Dickard, N. (2003). The sustainability challenge: Taking EdTech to the next level . For full text: https://eric.ed.gov/?id=ED477720 .
Dignum, V. (2018). Ethics in artificial intelligence: Introduction to the special issue. Ethics and Information Technology, 20 (1): 1–3 https://doi.org/10.1007/s10676-018-9450-z
Downey, G. L., & Lucena, J. C. (1997). Engineering selves: Hiring in to a contested field of education. In N. Sakellariou & R. Milleron (Eds.), Ethics, politics, and whistleblowing in engineering (pp. 25–43). CRC Press.
Dutton, T. (2018). An overview of national AI strategies. Politics + AI (blog). June 28, 2018. https://medium.com/politics-ai/an-overview-of-national-ai-strategies-2a70ec6edfd .
Dutton, T., Barron, B., & Boskovic, G. (2018). Building an AI world: Report on national and regional AI strategies. Toronto, Canada: CIFAR. https://www.cifar.ca/docs/default-source/ai-society/buildinganaiworld_eng.pdf .
Ely, D. P. (1999). Conditions that facilitate the implementation of educational technology innovations. Educational Technology, 39 (6), 23–27.
European Commission. (2019). Ethical guidelines for trustworthy AI. Brussels, Belgium: European Commission, High-Level Expert Group on Artificial Intelligence (AI HLEG). https://ec.europa.eu/newsroom/dae/document.cfm?doc_id=58477 .
Federal Ministry of Education and Research, Germany. (2018). “Germany: National Strategy for Artificial Intelligence: AI Made in Germany.” Bonn, Germany: Federal Ministry of Education and Research, the Federal Ministry for Economic Affairs and Energy, and Federal Ministry of Labour and Social Affairs, Germany. https://www.ki-strategie-deutschland.de/home.html?file=files/downloads/Nationale_KI-Strategie_engl.pdf .
Fishman, B. J., Penuel, W. R., Allen, A. R., Cheng, B. R., & Sabelli, N. (2013). Design-based implementation research: an emerging model for transforming the relationship of research and practice. p. 21.
Fjeld, J., Achten, N., Hilligoss, H., Nagy, A., & Srikumar, M. (2020). Principled artificial intelligence: Mapping consensus in ethical and rights-based approaches to principles for AI. SSRN Scholarly Paper ID 3518482. Rochester, NY: Berkman Klein Center for Internet & Society. https://papers.ssrn.com/abstract=3518482 .
Floridi, L., & Cowls, J. (2019). A unified framework of five principles for AI in society. Harvard Data Science Review . https://doi.org/10.1162/99608f92.8cd550d1
Frey, C. B., & Osborne, M. A. (2017). The future of employment: how susceptible are jobs to computerisation? Technological Forecasting and Social Change, 114 (January), 254–280. https://doi.org/10.1016/j.techfore.2016.08.019
Friedman, B., Hendry, D. G. & Borning, A. (2017). A survey of value sensitive design methods. Foundations and Trends® in Human–Computer Interaction, 11(2): 63–125. https://doi.org/10.1561/1100000015 .
Future of Life Institute. (2020). AI Policy—United States. Future of Life Institute. 2020. https://futureoflife.org/ai-policy-united-states/ .
Gebru, T., Morgenstern, J., Vecchione, B., Wortman Vaughan, J., Wallach, H., Daumé III, H., & Crawford, K. (2020). Datasheets for Datasets. [Cs] , January. http://arxiv.org/abs/1803.09010 .
Gibert, M., Mondin, C., & Chicoisne, G. (2018). Montréal declaration of responsible AI: 2018 Overview of international recommendations for AI Ethics. University of Montréal. https://www.montrealdeclaration-responsibleai.com/reports-of-montreal-declaration .
Giuliani, D., & Ajadi, S. (2019). 618 Active Tech Hubs: The Backbone of Africa’s Tech Ecosystem. GSMA (blog). July 10, 2019. https://www.gsma.com/mobilefordevelopment/blog/618-active-tech-hubs-the-backbone-of-africas-tech-ecosystem/ .
Graesser, A. C., D’Mello, S. K., & Strain, A. C. (2014). Emotions in advanced learning technologies. In L. Linnenbrink-Garcia & R. Pekrun (Eds.), International handbook of emotions in education (pp. 473–93). New York: Routledge.
Guidotti, R., Monreale, A., Ruggieri, S., Turini, F., Giannotti, F., & Pedreschi, D. (2018). A survey of methods for explaining black box models. ACM Computing Surveys, 51 (5), 1–42. https://doi.org/10.1145/3236009
Guidaudon, V. (2000). European intergration and migration policy. Journal of Common Market Studies, 38 (2), 251–71. https://doi.org/10.1111/1468-5965.00219
Hagendorff, T. (2020). The ethics of AI ethics: An evaluation of guidelines. Minds and Machines . https://doi.org/10.1007/s11023-020-09517-8
Hannafin, R. D., & Savenye, W. C. (1993). Technology in the classroom: The teacher’s new role and resistance to it. Educational Technology, 33 (6), 26–31.
Hao, K. (2019). China has started a grand experiment in AI education. it could reshape how the world learns. MIT Technology Review , August 2, 2019. https://www.technologyreview.com/2019/08/02/131198/china-squirrel-has-started-a-grand-experiment-in-ai-education-it-could-reshape-how-the/ .
Hao, K. (2020). The UK exam debacle reminds us that algorithms can’t fix broken systems. MIT Technology Review , August 20, 2020. https://www.technologyreview.com/2020/08/20/1007502/uk-exam-algorithm-cant-fix-broken-system/ .
Harding, T., & Whitehead, D. (2013). Analysing data in qualitative research. In Z. Schneider & D. Whitehead (Eds.), Nursing and midwifery research: Methods and appraisal for evidence-based practice (4th ed., pp. 127–42). Sydney: Mosby Elsevier.
Harley, J. M., Lajoie, S. P., Frasson, C., & Hall, N. C. (2017). Developing emotion-aware, advanced learning technologies: a taxonomy of approaches and features. International Journal of Artificial Intelligence in Education, 27 (2), 268–297. https://doi.org/10.1007/s40593-016-0126-8
Heyneman, S. P. (2004). International education quality. Economics of Education Review, 23 (4), 441–452. https://doi.org/10.1016/j.econedurev.2003.10.002
Holmes, W., Bektik, D., Whitelock, D., & Woolf, B. P. (2018). Ethics in AIED: Who Cares?. In Artificial Intelligence in Education: 19th International Conference, AIED 2018, London, UK, June 27–30, 2018, Proceedings, Part II , Rosé, C. P., Martínez-Maldonado, R., Hoppe, H. U., Luckin, R., Mavrikis, M., Porayska-Pomsta, K., McLaren, B. and Du Boulay, B (ed) Vol. 10948. Lecture Notes in Computer Science. Cham: Springer International Publishing. https://doi.org/10.1007/978-3-319-93846-2 .
Holmes, W., Porayska-Pomsta, K., Holstein, K., Sutherland, E., Baker, T., Shum, S. B., Santos, O. C., Rodrigo, M. T., Cukurova, M., Bittencourt, I. I., & Koedinger, K. R. (2021). Ethics of AI in education: Towards a community-wide framework. International Journal of Artificial Intelligence in Education . https://doi.org/10.1007/s40593-021-00239-1 .
House of Lords, Select Committee on Artificial Intelligence. (2018). United Kingdom: AI in the UK: Ready, Willing and Able?. London, UK: House of Lords, Select Committee on Artificial Intelligence. https://publications.parliament.uk/pa/ld201719/ldselect/ldai/100/100.pdf .
IEEE. (2020). 7010–2020 - IEEE Recommended Practice for Assessing the Impact of Autonomous and Intelligent Systems on Human Well-Being . S.l.: IEEE. https://ieeexplore.ieee.org/servlet/opac?punumber=9084217 .
Jobin, A., Ienca, M., & Vayena, E. (2019). The global landscape of ai ethics guidelines. Nature Machine Intelligence, 1 (9), 389–399. https://doi.org/10.1038/s42256-019-0088-2
Jonsen, K., & Jehn, K. A. (2009). Using triangulation to validate themes in qualitative studies. Qualitative Research in Organizations and Management: An International Journal, 4 (2), 123–150. https://doi.org/10.1108/17465640910978391
Joshi, Meghna, T. J., & Rangaswamy, N. (2018). Scaling Classroom IT Skill Tutoring: A Case Study from India. In Proceedings of the 2018 CHI Conference on Human Factors in Computing Systems , 630:1–630:12. CHI ’18. New York, NY, USA: ACM. https://doi.org/10.1145/3173574.3174204 .
Kaplan, S. (2008). Framing contests: Strategy making under uncertainty. Organization Science, 19 (5), 729–752. https://doi.org/10.1287/orsc.1070.0340
Katyal, S. K. (2019). Private accountability in the age of artificial intelligence. UCLA Law Review, 66 (1), 54–141.
Kingdon, J. W. (1995). Agendas, alternatives, and public policies (2nd ed.). HarperCollins College Publishers.
Korean Ministry of Science, ICT and Future Planning. (2016). South Korea: Mid-to long-term master plan in preparation for the intelligent information society: Managing the fourth industrial revolution. South Korea: Korean Ministry of Science, ICT and Future Planning (MSIP). http://english.msit.go.kr/cms/english/pl/policies2/__icsFiles/afieldfile/2017/07/20/Master%20Plan%20for%20the%20intelligent%20information%20society.pdf ; https://medium.com/syncedreview/south-korea-aims-high-on-ai-pumps-2-billion-into-r-d-de8e5c0c8ac5 .
Langheinrich, M. (2001). Privacy by design principles of privacy-aware ubiquitous systems. In G. D. Abowd, B. Brumitt, & S. Shafer (Eds.), Ubicomp 2001: Ubiquitous computing (pp. 273–91). Berlin, Heidelberg: Springer.
Lee, J.-W. (2001). Education for technology readiness: Prospects for developing countries. Journal of Human Development, 2 (1), 115–151. https://doi.org/10.1080/14649880120050
Lencucha, R., Kothari, A., & Hamel, N. (2010). Extending collaborations for knowledge translation: Lessons from the community-based participatory research literature. Evidence and Policy: A Journal of Research, Debate and Practice, 6 (1), 61–75. https://doi.org/10.1332/174426410X483006
Lincoln, Y. S., & Guba, E. G. (1985). Naturalistic Inquiry (Vol. 75). Sage.
Lincoln, Y. S., & Guba, E. G. (1986). but is it rigorous? Trustworthiness and authenticity in naturalistic evaluation. New Directions for Program Evaluation, 1986 (30), 73–84. https://doi.org/10.1002/ev.1427
Lord, S. M., Mejia, J. A., Hoople, G., Chen, D., Dalrymple, O., Reddy, E., Przestrzelski, B., & Choi-Fitzpatrick, A. (2018). Creative Curricula for Changemaking Engineers. In 2018 World Engineering Education Forum—Global Engineering Deans Council (WEEF-GEDC) , 1–5. https://doi.org/10.1109/WEEF-GEDC.2018.8629612 .
Lowther, D. L., Inan, F. A., Daniel Strahl, J., & Ross, S. M. (2008). Does technology integration ‘work’ when key barriers are removed? Educational Media International, 45 (3), 195–213. https://doi.org/10.1080/09523980802284317
Mahood, Q., Van Eerd, D., & Irvin, E. (2014). Searching for grey literature for systematic reviews: Challenges and benefits. Research Synthesis Methods, 5 (3), 221–234. https://doi.org/10.1002/jrsm.1106
Malin, J., & Brown, C. (Eds.). (2019). The role of knowledge brokers in education: Connecting the dots between research and practice . Routledge.
Manheim, K. M., & Kaplan, L. (2018). Artificial intelligence: Risks to privacy and democracy. SSRN Scholarly Paper ID 3273016. Rochester, NY: Social Science Research Network. https://papers.ssrn.com/abstract=3273016 .
Marcinkowski, F., Kieslich, K., Starke, C., & Lünich, M. (2020). Implications of AI (Un-)Fairness in Higher Education Admissions: The Effects of Perceived AI (Un-)Fairness on Exit, Voice and Organizational Reputation. In Proceedings of the 2020 Conference on Fairness, Accountability, and Transparency , 122–30. Barcelona Spain: ACM. https://doi.org/10.1145/3351095.3372867 .
McKinsey Global Institute. (2018). Notes From the AI Frontier: Modeling the Impact of AI on the World Economy. McKinsey Global Institute. https://www.mckinsey.com/~/media/McKinsey/Featured%20Insights/Artificial%20Intelligence/Notes%20from%20the%20frontier%20Modeling%20the%20impact%20of%20AI%20on%20the%20world%20economy/MGI-Notes-from-the-AI-frontier-Modeling-the-impact-of-AI-on-the-world-economy-September-2018.ashx .
Mehrabi, N., Morstatter, F., Saxena, F., Lerman, K., & Galstyan, A. (2019). A survey on bias and fairness in machine learning. [Cs] , September. http://arxiv.org/abs/1908.09635 .
Miles, M. B., Huberman, A. M., & Saldaña, J. (2014). Qualitative data analysis: A methods sourcebook (3rd ed.). SAGE Publications Inc.
Ministry of Economic Affairs and Employment, Finland. (2017). Finland: Finland’s Age of Artificial Intelligence Turning Finland into a Leading Country in the Application of Artificial Intelligence. 47/2017. Helsinki, Finland: Ministry of Economic Affairs and Employment. http://julkaisut.valtioneuvosto.fi/bitstream/handle/10024/160391/TEMrap_47_2017_verkkojulkaisu.pdf .
Ministry of Finance and Ministry of Industry, Business and Financial Affairs, Danish Government. (2019). Denmark: National Strategy for Artificial Intelligence. Copenhagen, Denmark: Ministry of Finance and Ministry of Industry, Business and Financial Affairs, Danish Government. https://investindk.com/-/media/invest-in-denmark/files/danish_national_strategy_for_ai2019.ashx?la=en .
Ministry of Science, Innovation and Universities, Spain. (2019). Spanish RDI Strategy in Artificial Intelligence. Madrid, Spain: Ministry of Science, Innovation and Universities. http://www.ciencia.gob.es/stfls/MICINN/Ciencia/Ficheros/Estrategia_Inteligencia_Artificial_EN.PDF .
Ministry of the Economy and Innovation. (2019). Lithuanian Artificial Intelligence Strategy: A Vision of the Future. Vilnius, Lithuania: Ministry of the Economy and Innovation. http://kurklt.lt/wp-content/uploads/2018/09/StrategyIndesignpdf.pdf .
Mitchell, M., Wu, S., Zaldivar, A., Barnes, P., Vasserman, L., Hutchinson, B., Spitzer, E., Raji, I. D. & Gebru, T. (2019). Model cards for model reporting. In Proceedings of the Conference on Fairness, Accountability, and Transparency - FAT* ’19 , 220–29. https://doi.org/10.1145/3287560.3287596 .
Mittelstadt, B. D., Allo, P., Taddeo, M., Wachter, S., & Floridi, L. (2016). The ethics of algorithms: Mapping the debate. Big Data and Society, 3 (2), 205395171667967. https://doi.org/10.1177/2053951716679679
Moher, D., Liberati, A., Tetzlaff, J., Altman, D. G. & The PRISMA Group. (2009). Preferred reporting items for systematic reviews and meta-analyses: The PRISMA statement. PLOS Medicine, 6 (7): e1000097. https://doi.org/10.1371/journal.pmed.1000097
Morganstein, D., & Wasserstein, R. (2014). ASA Statement on value-added models. Statistics and Public Policy, 1 (1), 108–110. https://doi.org/10.1080/2330443X.2014.956906
Morley, J., Floridi, L., Kinsey, L., & Elhalal, A. (2019). From what to how: An initial review of publicly available AI ethics tools, methods and research to translate principles into practices. Science and Engineering Ethics . https://doi.org/10.1007/s11948-019-00165-5
NITI Aayog, India. (2018). India: National Strategy for Artificial Intelligence #AIFORALL. Delhi, India: NITI Aayog. http://niti.gov.in/writereaddata/files/document_publication/NationalStrategy-for-AI-Discussion-Paper.pdf .
Noble, D. F. (1998). Digital diploma mills: The automation of higher education. Science as Culture, 7 (3), 355–368.
Nye, B. D. (2015). Intelligent tutoring systems by and for the developing world: A review of trends and approaches for educational technology in a global context. International Journal of Artificial Intelligence in Education, 25 (2), 177–203. https://doi.org/10.1007/s40593-014-0028-6
Oetzel, M. C., & Spiekermann, S. (2014). A systematic methodology for privacy impact assessments: A design science approach. European Journal of Information Systems, 2 3(2), 126–150. https://doi.org/10.1057/ejis.2013.18 .
Office of the President, Russia. (2019). Russia: National Strategy for the Development of Artificial Intelligence Over the Period Extending up to the Year 2030. Moscow, Russia. http://kremlin.ru/events/president/news/60630 .
Office of the Prime Minister, Malta. (2019). Malta: Towards An AI Strategy. Valletta, Malta: Parliamentary Secretariat for Financial Services, Digital Economy and Innovation, Office of the Prime Minister, Malta. http://malta.ai/wp-content/uploads/2019/04/Draft_Policy_document_-_online_version.pdf .
Oliver, K., Innvar, S., Lorenc, T., Woodman, J., & Thomas, J. (2014). A systematic review of barriers to and facilitators of the use of evidence by policymakers. BMC Health Services Research, 14 (1), 2. https://doi.org/10.1186/1472-6963-14-2
Oswald, M., Grace, J., Urwin, S., & Barnes, G. C. (2018). Algorithmic risk assessment policing models: Lessons from the Durham hart model and ‘experimental’ proportionality. Information and Communications Technology Law, 27 (2), 223–250. https://doi.org/10.1080/13600834.2018.1458455
Parkhurst, J. (2017). The politics of evidence :From evidence -based policy to the good governance of evidence . Taylor & Francis. https://library.oapen.org/handle/20.500.12657/31002 .
Phoenix, J. H., Atkinson, L. G., & Baker, H. (2019). Creating and communicating social research for policymakers in government. Palgrave Communications, 5 (1), 98. https://doi.org/10.1057/s41599-019-0310-1
Pinkwart, N. (2016). Another 25 years of AIED? Challenges and opportunities for intelligent educational technologies of the future. International Journal of Artificial Intelligence in Education, 26 (2), 771–783. https://doi.org/10.1007/s40593-016-0099-7
Porter, M. E. (1996). Competitive advantage, agglomeration economies, and regional policy. International Regional Science Review, 19 (1–2), 85–90. https://doi.org/10.1177/016001769601900208
Qatar Center for Artificial Intelligence. (2019). “Qatar: Blueprint: National Artificial Intelligence Strategy for Qatar.” Ar-Rayyan, Qatar: Qatar: Qatar Center for Artificial Intelligence (QCAI), Qatar Computing Research Institute (QCRI), Hamad Bin Khalifa University. https://qcai.qcri.org/wp-content/uploads/2019/02/National_AI_Strategy_for_Qatar-Blueprint_30Jan2019.pdf .
Radaelli, C. M. (2009). Measuring policy learning: Regulatory impact assessment in Europe. Journal of European Public Policy, 16 (8), 1145–1164. https://doi.org/10.1080/13501760903332647
Raji, I. D., Smart, A., White, R. N., Mitchell, M., Gebru, T., Hutchinson, B., Smith-Loud, J., Theron, D., & Barnes, P. (2020). Closing the AI accountability gap: Defining an end-to-end framework for internal algorithmic auditing. In Proceedings of the 2020 Conference on Fairness, Accountability, and Transparency , 33–44. FAT* ’20. Barcelona, Spain: Association for Computing Machinery. https://doi.org/10.1145/3351095.3372873 .
Reisman, D., Schultz, J., Crawford, K., & Whittaker, M. (2018). Algorithmic impact assessments: A practical framework for public agency accountability. New York, NY: AI Now. https://ainowinstitute.org/aiareport2018.pdf .
Roberts, K., Dowell, A., & Nie, J.-B. (2019). Attempting rigour and replicability in thematic analysis of qualitative research data: A case study of codebook development. BMC Medical Research Methodology, 19 (1), 66. https://doi.org/10.1186/s12874-019-0707-y
Roolaht, T. (2012). The characteristics of small country national innovation systems. In E. G. Carayannis, U. Varblane, & T. Roolaht (Eds.) In novation Systems in Small Catching-Up Economies: New Perspectives on Practice and Policy (pp. 21–37). Springer. https://doi.org/10.1007/978-1-4614-1548-0_2 .
Rothstein, H. R., & Hopewell, S. (2009). Grey literature. The handbook of research synthesis and meta-analysis (pp. 103–25). Russell Sage Foundation.
Schendel, R., & McCowan, T. (2016). Expanding higher education systems in low- and middle-income countries: The challenges of equity and quality. Higher Education, 72 (4), 407–411. https://doi.org/10.1007/s10734-016-0028-6
Schiff, D. (2021). Out of the laboratory and into the classroom: The future of artificial intelligence in education. AI & Society, 36 (1), 331–348. https://doi.org/10.1007/s00146-020-01033-8
Schiff, D., Ayesh, A., Musikanski, L., & Havens, J. C. (2020a). IEEE 7010: A new standard for assessing the well-being implications of artificial intelligence. In 2020 IEEE International Conference on Systems, Man, and Cybernetics (SMC) , 2746–53. https://doi.org/10.1109/SMC42975.2020.9283454 .
Schiff, D., Biddle, J., Borenstein, J., & Laas, K. (2020b). What’s next for AI ethics, policy, and governance? A global overview. In Proceedings of the AAAI/ACM Conference on AI, Ethics, and Society , 153–58. AIES ’20. New York, NY, USA: Association for Computing Machinery. https://doi.org/10.1145/3375627.3375804 .
Schiff, D., Borenstein, J., Laas, K., & Biddle, J. (2021). AI ethics in the public, private, and NGO sectors: A review of a global document collection. IEEE Transactions on Technology and Society, 2 , 31–42. https://doi.org/10.1109/TTS.2021.3052127
Schomberg, R. von. (2013). A vision of responsible research and innovation. In Responsible Innovation (pp. 51–74). Wiley-Blackwell. https://doi.org/10.1002/9781118551424.ch3 .
Scott, J., Lubienski, C., DeBray, E., & Jabbar, H. (2014). The intermediary function in evidence production, promotion, and utilization: The case of educational incentives. In K. S. Finnigan & A. J. Daly (Eds.), Using research evidence in education (pp. 69–89). Cham: Springer.
Selbst, A. D., Boyd, D., Friedler, S. A., Venkatasubramanian, S., & Vertesi, J. (2019). Fairness and abstraction in sociotechnical systems. In Proceedings of the Conference on Fairness, Accountability, and Transparency , 59–68. FAT* ’19. New York, NY, USA: Association for Computing Machinery. https://doi.org/10.1145/3287560.3287598 .
Sin, C. H. (2008). The role of intermediaries in getting evidence into policy and practice: some useful lessons from examining consultancy-client relationships. Evidence and Policy: A Journal of Research, Debate and Practice, 4 (1), 85–103. https://doi.org/10.1332/174426408783477828
Smart Nation and Digital Government Office, Singapore. (2019). Singapore: National Artificial Intelligence Strategy: Advancing Our Smart Nation Journey. Singapore: Smart Nation and Digital Government Office. https://www.smartnation.sg/docs/default-source/default-document-library/national-ai-strategy.pdf .
Stahl, B. C., & Coeckelbergh, M. (2016). Ethics of healthcare robotics: towards responsible research and innovation. Robotics and Autonomous Systems, 86 (December), 152–161. https://doi.org/10.1016/j.robot.2016.08.018
Stern, C., Jordan, Z., & McArthur, A. (2014). Developing the review question and inclusion criteria. AJN the American Journal of Nursing, 114 (4), 53–56. https://doi.org/10.1097/01.NAJ.0000445689.67800.86
Terhart, E. (2013). Teacher resistance against school reform: Reflecting an inconvenient truth. School Leadership and Management, 33 (5), 486–500. https://doi.org/10.1080/13632434.2013.793494
The Institute for Ethical AI in Education. (2020). Interim report: Towards a shared vision of ethical AI in education. The institute for ethical AI in education. https://www.buckingham.ac.uk/wp-content/uploads/2020/02/The-Institute-for-Ethical-AI-in-Educations-Interim-Report-Towards-a-Shared-Vision-of-Ethical-AI-in-Education.pdf .
Thomas, D. R. (2006). A general inductive approach for analyzing qualitative evaluation data. American Journal of Evaluation, 27 (2), 237–246. https://doi.org/10.1177/1098214005283748
Lee, N. T., Resnick, P., & Barton, G. (2019). Algorithmic bias detection and mitigation: best practices and policies to reduce consumer harms. Brookings Institition. https://www.brookings.edu/research/algorithmic-bias-detection-and-mitigation-best-practices-and-policies-to-reduce-consumer-harms/ .
Villani, C., Marc, S., Bonnet, Y., Charly, B., Anne-Charlotte Cornut, François Levin, & Rondepierre, B. (2018). “France: For a Meaningful Artificial Intelligence: Towards a French and European Strategy.” French Parliament. https://www.aiforhumanity.fr/pdfs/MissionVillani_Report_ENG-VF.pdf .
Warschauer, M., & Ames, M. (2010). Can One Laptop per Child save the world's poor?. Journal of International Affairs , 64 (1): 33-51.
West, D. M. (2018). The future of work: Robots, AI, and automation . Brookings Institution Press.
White, M. D., & Marsh, E. E. (2006). Content analysis: A flexible methodology. Library Trends, 55 (1), 22–45. https://doi.org/10.1353/lib.2006.0053
Whittaker, M., Crawford, K., Dobbe, R., Fried, G., Kaziunas, E., Mathur, V., West, S.M., Richardson, R., Schultz, J., & Schwartz, O. (2018). AI Now Report 2018. New York, NY: New York University. https://ainowinstitute.org/AI_Now_2018_Report.pdf .
Williamson, B., & Eynon, R. (2020). Historical threads, missing links, and future directions in AI in education. Learning, Media and Technology, 45 (3), 223–235. https://doi.org/10.1080/17439884.2020.1798995
Winfield, A. (2019). Ethical standards in robotics and AI. Nature Electronics, 2 (2), 46–48. https://doi.org/10.1038/s41928-019-0213-6
Women Leading in AI. (2019). Women leading in AI: 10 Principles of responsible AI. Women Leading in AI. https://womenleadinginai.org/wp-content/uploads/2019/02/WLiAI-Report-2019.pdf .
Woolf, B. P., Ivon, A., David, C., Winslow, B., & Kasia, M. (2010). Affective tutors: Automatic detection of and response to student emotion. In N. Roger, B. Jacqueline, & M. Riichiro (Eds.), Advances in intelligent tutoring systems (pp. 207–27). Berlin, Heidelberg: Springer.
Zarsky, T. Z. (2016). Incompatible: The GDPR in the age of big data. Seton Hall Law Review , 47, 995–1020.
Zeng, Y., Enmeng L., & Cunqing H. (2018). Linking artificial intelligence principles. [Cs] , December. http://arxiv.org/abs/1812.04814 .
Zhang, D., Saurabh, M., Brynjolfsson, E., Etchemendy, J., Ganguli, D., Grosz, B., Lyons, T., Manyika, J., Niebles, J. C., Sellitto, M., Shoham, Y., Clark, J., & Perrault, R. (2021). Artificial Intelligence Index Report 2021. AI Index Steering Committee, Human-Centered AI Institute, Stanford University. https://aiindex.stanford.edu/wp-content/uploads/2021/03/2021-AI-Index-Report_Master.pdf
Zhou, Y., & Danks, D. (2020). Different ‘intelligibility’ for different folks. In Proceedings of the AAAI/ACM Conference on AI, Ethics, and Society , 194–99. AIES ’20. New York, NY, USA: Association for Computing Machinery. https://doi.org/10.1145/3375627.3375810 .
Download references
The author declares no funding.
Author information
Authors and affiliations.
School of Public Policy, Georgia Institute of Technology, Atlanta, GA, USA
Daniel Schiff
You can also search for this author in PubMed Google Scholar
Corresponding author
Correspondence to Daniel Schiff .
Ethics declarations
Conflicts of interest.
The author declares no conflicts of interest or competing interests.
Additional information
Publisher’s note.
Springer Nature remains neutral with regard to jurisdictional claims in published maps and institutional affiliations.
Appendix 1: Methodology
This appendix provides more extensive details surrounding the data collection, screening, coding, and analysis strategy, as well as associated limitations, than is available in the main body of the article.
Data Collection
The data collection process differs from that typically employed in meta-analysis and review papers, such as recommended by the PRISMA Statement (Moher et al. 2009 ), largely because the documents evaluated here are so-called “gray literature” not found in traditional databases (Mahood et al. 2014 ; Rothstein and Hopewell 2009 ). Given the type of documents and substantive focus of the study, the data collection process relied on linkhubs, Google searches, and manual searching of certain documents and countries: e.g., “country name + AI policy strategy.” The search process is connected to ongoing research assessing AI ethics and policy (Schiff et al. 2021 , 2020a , 2020b ). The first author and colleagues have maintained a database of AI policy documents and additional AI ethics documents (available at https://dx.doi.org/10.21227/fcdb-pa48 ). The database was created in Fall 2018 and updated regularly until early 2020. Data collection focused on policy documents benefited especially from lists maintained by the Future of Life Institute ( 2020 ) and Tim Dutton ( 2018 ). These linkhubs contain updates about AI policy developments in dozens of countries, including how far along countries are in development of task forces, funding proposals, and formal AI policy strategy documents.
For each country noted in these linkhubs, I searched for and accessed documents and initiatives mentioned, and performed additional Google searches and manual searches for each country to ensure that the set of national AI policy strategies was as complete as possible. While it is possible that some countries were omitted, perhaps due to lack of language familiarity, the two key sources are invested in tracking national AI policy developments. All such candidate documents were thus captured in the database managed by the first author and colleagues. From this larger database, I extracted only documents produced by public sector organizations (e.g., countries, not corporations or non-governmental organizations). This resulted in 76 public sector AI documents that formed the candidate pool of national AI policy documents.
Screening Process
The screening process involved identifying criteria for types of documents, publication language, the population and phenomena of interest, and time period (Stern et al. 2014 ). The purpose of this study was to assess national AI policy strategies as they relate to education. Therefore, the study applied the following inclusion/exclusion criteria:
Documents needed to be complete and resemble a national AI policy strategy. Some such documents describe themselves as preliminary reports or blueprints, working towards more robust or formalized policy strategies. Nevertheless, many were sufficiently robust so as to be considered as policy strategies. On the other hand, countries that had only announced task forces, funding initiatives, created websites, or otherwise did not have a well-developed document analogous to that of other countries were not included. This ensures countries can be compared fairly and speak to sufficiently detailed items of policy, with a sizable average of 62 pages per document.
Documents needed to be in English, due to the author’s limited language proficiency. However, in a number of cases, governments had produced official English-language translations (e.g., Finland, Italy). While automated translation of non-English documents (e.g., Google Translate) may not be of sufficient quality, there was one unofficial but high-quality translation included in the final sample, of China’s AI policy strategy, performed by the Foundation for Law and International Affairs.
The study also excluded documents produced by inter-governmental organizations, such as the United Nations, the Organization for Economic Cooperation and Development, and the European Union. While these documents are no doubt important, they address a different scope, as they are relatively distant from national-level institutions, funding activities, and other policy activities, such as those involving education policy. This makes these documents less comparable to national-level AI policy strategies.
Finally, in a number of cases, countries produced multiple documents that were potentially relevant to AI policy. Only one document was selected per country. The chosen document was typically the most robust and the most recent, at times an evolution of a previous draft or more preliminary document. Further, some candidate documents were not representative of an overarching national AI policy strategy. For example, documents from Germany addressing autonomous vehicle policy and from Finland addressing work in the age of AI were excluded in preference of Germany’s National Strategy for AI and Finland’s Age of AI. This screening criteria helped to ensure that individual countries were not overrepresented, that information analyzed was not redundant, and that the most robust, high-quality, and comparable policy strategies were selected in each case.
Of the 76 candidate documents, eight did not resemble a complete national policy strategy document, one was not available in English, 13 were inter-governmental, and 30 were excluded in favor of more representative documents. Screening resulted in a final sample of 24 national AI policy strategies.
Codebook Development
After identifying the final sample, the analytical strategy began with the development of a preliminary set of topics, in the form of a codebook (Miles et al. 2014 ; Thomas 2006 ). These topics in the codebook were chosen based on the study’s conceptual scope and framework, the author’s subject matter knowledge, and previous exposure to AI policy strategies. The scope of interest was any discussion of education, construed as broadly as possible, such as youth and adult education, training and re-skilling, investing in educational systems, public education and awareness, the need to develop more AI talent (e.g., computer scientists, engineers), and social and ethical issues related to AI and education.
The initial codebook included 11 categories: Education as Priority Topic, K-12 Education, Post-Secondary Education, Adult Education and Training, General AI Literacy, Training AI Experts, Preparing Workforce, Intelligent Tutoring Systems, Pedagogical Agents and Learning Robots, Predictive Educational Tools, and AI for Healthcare. A best practice in qualitative research is to iterate and refine the codebook through testing on a small subset of the data (Roberts et al. 2019 ). Therefore, I randomly selected five documents—aiming for a meaningfully-sized and somewhat representative subset—and applied the thematic schema to them. This involved reading the documents to determine whether the coding schema could validly and straightforwardly reflect the way education was discussed in the documents, and to identify if the coding schema captured the full range of issues in the documents relevant to the article’s conceptual scope.
Based on this initial test, several categories were modified, removed, and collapsed as follows:
Education as Priority Topic and AI for Healthcare were retained, as they were easy to apply. Either topic might be explicitly noted as a priority topic in a document, for example, if a list of priority policy sectors was mentioned and education was among that list. Alternatively, education/healthcare were coded as priority topics if a significant subsection was dedicated to them, or if there was a similar amount of discussion relative to the length of the document as compared to other documents that did identify education (or healthcare) as an explicit priority.
K-12 Education, Post-Secondary Education, and Adult Education and Training were removed. These categories were originally designed to separate discussion of education by target age/population group. However, the test documents often did not identify the target age/group when discussing AI and education, making this distinction difficult to code accurately. Moreover, these population differences were deemed less relevant for the overall purpose of the article. For example, that documents emphasized the need to develop more AI researchers seemed more pressing to the document authors than whether this development happened in secondary or postsecondary educational institutions.
Training AI Experts and Preparing Workforce for AI were straightforward and were retained.
General AI Literacy was renamed to Public AI Literacy. The former was originally defined to emphasize development of general digital, STEM, and other skills in educational settings. The theme was relabeled and redefined to incorporate AI literacy in both educational (classroom) and ‘public’ settings, because both settings were discussed and justified to pertain to similar policy purposes.
The revised codebook collapsed Intelligent Tutoring Systems and Pedagogical Agents and Learning Robots into Teaching and Learning. Too few documents addressed these issues at the level of detail of individual AIED technologies or tools to allow for reliable identification, as the documents generally employed more abstracted terms and discussions.
The revised codebook also abstracted Predictive Educational Tools into Administrative Tools, as there were several examples of AIED tools mentioned that were better captured by the latter, broader terminology, such as the use of AI for inspection or assigning teachers to schools.
These adjustments resulted in a revised codebook with seven categories (a reasonable number for inductive studies) (Thomas 2006 ), described in the main body. The final coding categories were straightforward to apply to the data and captured relevant concepts within the study’s scope well.
An important note is that, despite an initial attempt to code for discussion of AIED ethics specifically given its importance to this study, discussion of these topics was too rare to justify having as a theme. Most discussion addressing ethics and education was focused on Education for AI purposes, such as training future machine learning experts to develop ethical design skills, rather than addressing ethical implications emanating from AIED. Nevertheless, I captured all mentions of ethics in the context of both Education for AI and AI for Education in my memos, and considered the presence and absence of these topics as part of the interpretive work.
Coding Approach
Next, I applied the codebook to the 24 documents in the sample (approximately 1491 pages total). Each document was read closely and assessed manually along the seven topics using a simple form of content analysis. This consisted of evaluating each document for the presence or absence of each theme (White and Marsh 2006 ), largely a binary exercise, though some documents were coded as borderline cases. In Table 1 , a country is marked as green when a theme was reflected, red when absent, and yellow when the case was sufficiently ambiguous or borderline.
For example, Malta’s (2019) document initially notes that AI for healthcare may be amongst the highest impact projects and “most pressing domestic challenges” worthy of prioritization, but it does not proceed to include any substantive discussion or a subsection on healthcare. In comparison to the document’s discussion of other topics, and in comparison to other countries’ AI policy documents that discuss healthcare in more depth, this relatively more narrow treatment of the topic led to coding it as yellow. Similarly, Russia’s (2019) discussion of using AI to “[improve] the quality of education services” does not provide enough detail to be clear about the role of AIED as a potential tool for teaching and learning, and so is considered to be too ambiguous to code as either green or red.
Analysis Approach
Relevant quotes from the documents were captured in a research memo and organized under the seven categories (Thomas 2006 ) to support higher-order conceptual and thematic interpretation. Additional quotes of interest and minor categories were included here as well, such as any mentions of ethics related to education. From this, I synthesized insights from the frequency and character of these topics, applying a thematic analytic approach (Castleberry and Nolen 2018 ) to identify major findings. This interpretive exercise involves considering second-order meanings or explanations for the patterns identified in the data (Miles et al. 2014 ), including the finding that AIED’s ethical implications are neglected. I present results for each topic in the main article, along with interpretation of key findings within and across topics, to support a broader discussion of the role of education and ethics in AI policy in the subsequent sections.
Limitations
Because the documents were coded by a single researcher, it is not possible to, for example, assess inter-rater reliability. Further, the conceptualization of the study, codebook development, and interpretation were not subject to the perspectives of other researchers or experts outside of the peer review process. However, quantitative measures of reliability are only sometimes considered essential in qualitative research (Castleberry and Nolen 2018 ), and a single coder approach can be appropriate and, in cases, even preferrable (Harding and Whitehead 2013 ). Multiple researchers may not be necessary to provide sufficient consistency and credibility, as single researchers can provide a unitary and consistent perspective, albeit one dependent on that author’s subjective assessments. For example, research using semi-structured interviews with dozens of coding categories and many degrees of detail (e.g., scoring attributes from 1–10) benefit especially from the assessment of interrater reliability, particularly if the codes are challenging to conceptually separate or define. In this study, however, the number of topics is small, the level of detail simple, and the concepts are fairly easy to conceptually separate.
Moreover, in qualitative research, there are common criteria of research rigor used as alternatives to traditional quantitative criteria of validity and reliability. For example, one widely used set of criteria comes from Lincoln and Guba ( 1985 ), who propose credibility as an alternative to internal validity, transferability as an alternative to external validity, dependability as an alternative to reliability, and confirmability as an alternative to objectivity. To satisfy these criteria, the analysis employed several recommended strategies (Lincoln and Guba 1986 ). Within-method triangulation across multiple documents (Jonsen and Jehn 2009 ) and the use of direct quotes as descriptive evidence provide rich support to demonstrate claims, supporting their credibility, dependability, and transferability. Further, because the data are publicly available, as opposed to privately held interview data, for example, they are open to scrutiny and confirmation or disconfirmation. However, in part because of researchers’ individual positions and biases (Castleberry and Nolen 2018 ), it is possible that other researchers would identify different coding categories or identify different salient themes. As such, the single researcher approach is a limitation of this study, discussed in the study’s limitations section. Future research examining the role of education in AI policy would be welcome in assessing the extent to which the findings presented here are indeed credible, dependable, confirmable, and transferable.
Rights and permissions
Reprints and permissions
About this article
Schiff, D. Education for AI, not AI for Education: The Role of Education and Ethics in National AI Policy Strategies. Int J Artif Intell Educ 32 , 527–563 (2022). https://doi.org/10.1007/s40593-021-00270-2
Download citation
Accepted : 26 July 2021
Published : 02 September 2021
Issue Date : September 2022
DOI : https://doi.org/10.1007/s40593-021-00270-2
Share this article
Anyone you share the following link with will be able to read this content:
Sorry, a shareable link is not currently available for this article.
Provided by the Springer Nature SharedIt content-sharing initiative
- Artificial intelligence
- Social implications of technology
- Find a journal
- Publish with us
- Track your research
University of South Florida
Main Navigation

USF’s Innovative Education helping revolutionize courses through generative AI
- May 15, 2024
Student Success
By Donna Smith , University Communications and Marketing
With artificial intelligence technology rapidly advancing, USF students will soon see some changes in their courses and how they participate in assignments. USF’s Innovative Education has made generative AI part of its broader strategy as it seeks to help faculty integrate it into online courses – serving as a test case for broader course instruction. Offerings include a website loaded with tools and ideas, case studies of how USF faculty have integrated generative AI into their courses and resources on prompt engineering – all of which offer opportunities for USF faculty members eager to optimize their time while equipping their students with essential workforce-ready skills.

Christine Brown
The Innovative Education team created the website to serve as an upskilling resource focused on practical ways to use generative AI for teaching and learning. The site offers strategies for using generative AI to augment course design, as well as ideas for incorporating it into student assignments and projects. It also links to the university’s comprehensive GenAI website that contains guidance on ethical usage, syllabus and course policy recommendations, citations, AI events and more.
“The feedback we've been getting from faculty is that while they've started using generative AI, they're unsure how to integrate it effectively into their course design and teaching,” said Christine Brown, associate vice president of Innovative Education. “So, we’ve provided an easy way for instructors to get a glimpse into what others are doing. We want to inspire faculty to engage with the tech.”
The self-paced workshop, Course Enhancement with Generative AI , provides faculty hands-on experience with generative AI through teaching and learning use cases. The workshop demonstrates how to use generative AI to augment communication, create assessments, rubrics and more. Faculty are asked to put what they learn into practice in their own courses and then share their experiences and insights with peers in the workshop. The course also illustrates how to work it into courses so that students are using it responsibly, not using AI to do the work for them, but to help students think more critically about generative AI so that they become adept with the technology. Through a partnership with the Office of Microcredentials, faculty who complete the workshop earn a badge.

Jenifer Schneider
Jenifer Schneider, Faculty Senate president and literacy studies professor in USF’s College of Education, said Innovative Education, along with the USF Libraries, has been an invaluable source of information.
“Working with them on online course developments and digital learning projects, you learn about new tools and techniques,” Schneider said. “Every time I work with Innovative Education, I learn something new.”
Schneider says she integrates AI into her courses to show her students, who are all teachers seeking graduate degrees, tools to make them more efficient in their work.
“I use it myself to construct modules and interpret data. I can also create discussion questions, summarize research and connect to sources in my research,” Schneider said. “So, I encourage them to use the technology in those same ways. Why would you not leverage a tool that can help you?”
John Licato, assistant professor of computer science and engineering, is also the founding director of the Advancing Machine and Human Reasoning Lab , a cross-disciplinary lab dedicated to studying ways to improve the human reasoning of AI. In collaboration with Innovative Education Studios, Licato recently started a podcast, the AI Bull Ring , which features USF experts, researchers, professors and graduate students who are doing work in the AI field. So far, Licato and his guests have tackled topics such as AI and creativity, AI in journalism and how AI is reshaping education. He says there are so many interesting things happening around AI, but there was a dearth of materials that were accessible for non-experts.
“On the higher end, there are experts in AI who are writing academic papers and talking in highly technical terms, and on the other extreme, there are people out there who are talking about AI, but in some cases, causing harm by spreading misinformation,” Licato said. “We wanted to feature experts in a really accessible discussion on AI.”

John Licato records a podcast.
Innovative Education’s support for faculty and generative AI is one piece of a broader USF strategy to provide generative AI opportunities to ethically and effectively advance USF’s mission. Experts from the USF colleges, Libraries, Center for Innovative Teaching and Learning, Information Technology, USF Health and Innovative Education are all working in concert to maximize the benefits of generative AI in ensuring student success.
“There’s no going backwards – we have to move forward,” Brown said. We must ensure that both our faculty and students are proficient in using generative AI. Our objective at USF is to prepare students to be successful in the workforce and in life, and in order to do this, they have to know how to use this technology responsibly and ethically.”
Return to article listing
Donna Smith , Innovative Education
News Archive
Learn more about USF's journey to Preeminence by viewing Newsroom articles from past years.
USF in the News
Smithsonian magazine: journey into the fiery depths of earth’s youngest caves.
May 16, 2024
Fortune: Bird flu FAQ - Everything you need to know about the H5N1 outbreak that’s spread to dairy cows in 9 states
May 9, 2024
Bay News 9: USF set to launch AI and cybersecurity college
May 5, 2024
Tampa Bay Business Journal: USF ranked in the top 50 for patents worldwide
April 29, 2024
More USF in the News
Featured Topics
Featured series.
A series of random questions answered by Harvard experts.
Explore the Gazette
Read the latest.
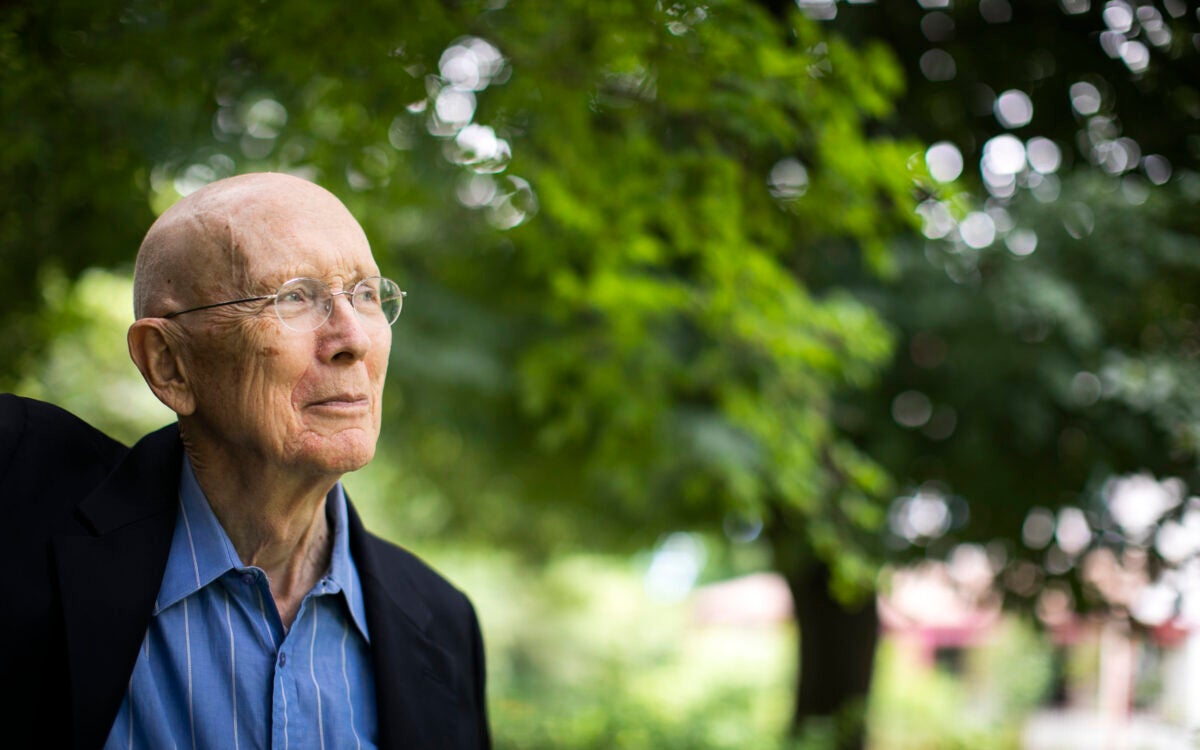
‘The scientist is not in the business of following instructions.’
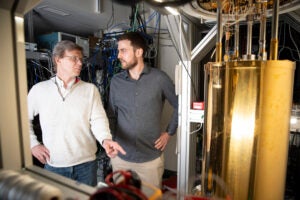
Glimpse of next-generation internet
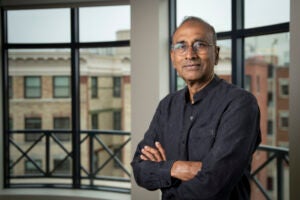
Science is making anti-aging progress. But do we want to live forever?
What is ‘original scholarship’ in the age of ai.
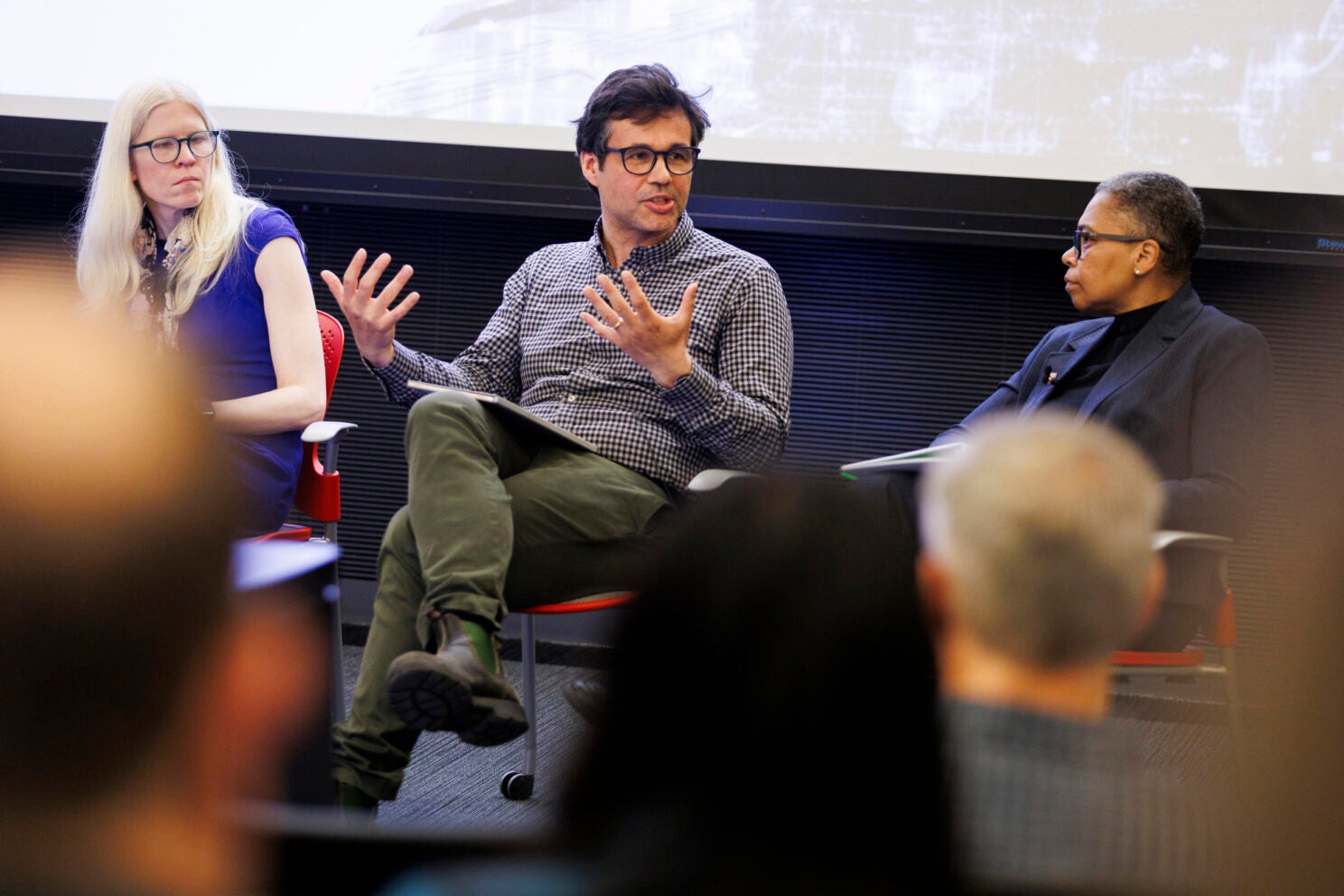
Melissa Dell (from left), Alex Csiszar, and Latanya Sweeney.
Photos by Stephanie Mitchell/Harvard Staff Photographer
Anne J. Manning
Harvard Staff Writer
Symposium considers how technology is changing academia
While moderating a talk on artificial intelligence last week, Latanya Sweeney posed a thought experiment. Picture three to five years from now. AI companies are continuing to scrape the internet for data to feed their large language models. But unlike today’s internet, which is largely human-generated content, most of that future internet’s content has been generated by … large language models.
The scenario is not farfetched considering the explosive growth of generative AI in the last two years, suggested the Faculty of Arts and Sciences and Harvard Kennedy School professor.
Sweeney’s panel was part of a daylong symposium on AI hosted by the FAS last week that considered questions such as: How are generative AI technologies such as ChatGPT disrupting what it means to own one’s work? How can AI be leveraged thoughtfully while maintaining academic and research integrity? Just how good are these large language model-based programs going to get? (Very, very good.)
“Here at the FAS, we’re in a unique position to explore questions and challenges that come from this new technology,” said Hopi Hoekstra , Edgerley Family Dean of the Faculty of Arts and Sciences, during her opening remarks. “Our community is full of brilliant thinkers, curious researchers, and knowledgeable scholars, all able to lend their variety of expertise to tackling the big questions in AI, from ethics to societal implications.”
In an all-student panel, philosophy and math concentrator Chinmay Deshpande ’24 compared the present moment to the advent of the internet, and how that revolutionary technology forced academic institutions to rethink how to test knowledge. “Regardless of what we think AI will look like down the line, I think it’s clear it’s starting to have an impact that’s qualitatively similar to the impact of the internet,” Deshpande said. “And thinking about pedagogy, we should think about AI along somewhat similar lines.”
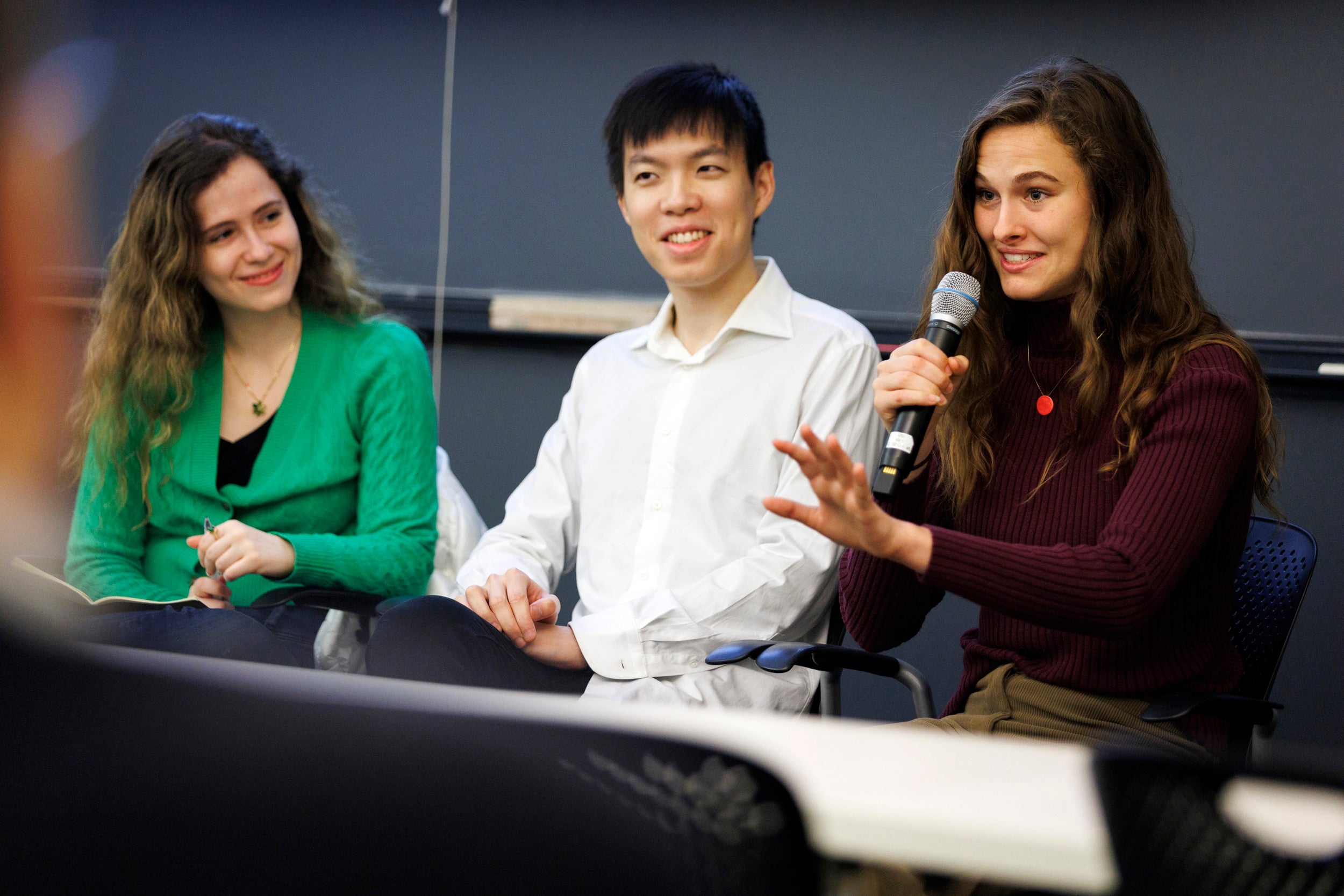
Computer science concentrator and master’s degree student Naomi Bashkansky ’25, who is exploring AI safety issues with fellow students, urged Harvard to provide thought leadership on the implications of an AI-saturated world, in part by offering courses that integrate the basics of large language models into subjects like biology or writing.
Harvard Law School student Kevin Wei agreed.
“We’re not grappling sufficiently with the way the world will change, and especially the way the economy and labor market will change, with the rise of generative AI systems,” Wei said. “Anything Harvard can do to take a leading role in doing that … in discussions with government, academia, and civil society … I would like to see a much larger role for the University.”
The day opened with a panel on original scholarship, co-sponsored by the Mahindra Humanities Center and the Edmond & Lily Safra Center for Ethics . Panelists explored ethics of authorship in the age of instant access to information and blurred lines of citation and copyright, and how those considerations vary between disciplines.
David Joselit , the Arthur Kingsley Professor of Art, Film, and Visual Studies, said challenges wrought by AI have precedent in the history of art; the idea of “authorship” has been undermined in the modern era because artists have often focused on the idea as what counts as the artwork, rather than its physical execution. “It seems to me that AI is a mechanization of that kind of distribution of authorship,” Joselit said. He posed the idea that AI should be understood “as its own genre, not exclusively as a tool.”
Another symposium topic included a review of Harvard Library’s law, information policy, and AI survey research revealing how students are using AI for academic work. Administrators from across the FAS also shared examples of how they are experimenting with AI tools to enhance their productivity. Panelists from the Bok Center shared how AI has been used in teaching this year, and Harvard University Information Technology gave insight into tools it is building to support instructors.
Throughout the ground floor of the Northwest Building, where the symposium took place, was a poster fair keying off final projects from Sweeney’s course “Tech Science to Save the World,” in which students explored how scientific experimentation and technology can be used to solve real-world problems. Among the posters: “Viral or Volatile? TikTok and Democracy,” and “Campaign Ads in the Age of AI: Can Voters Tell the Difference?”
Students from the inaugural General Education class “ Rise of the Machines? ” capped the day, sharing final projects illustrating current and future aspects of generative AI.
Share this article
You might like.
George Whitesides became a giant of chemistry by keeping it simple
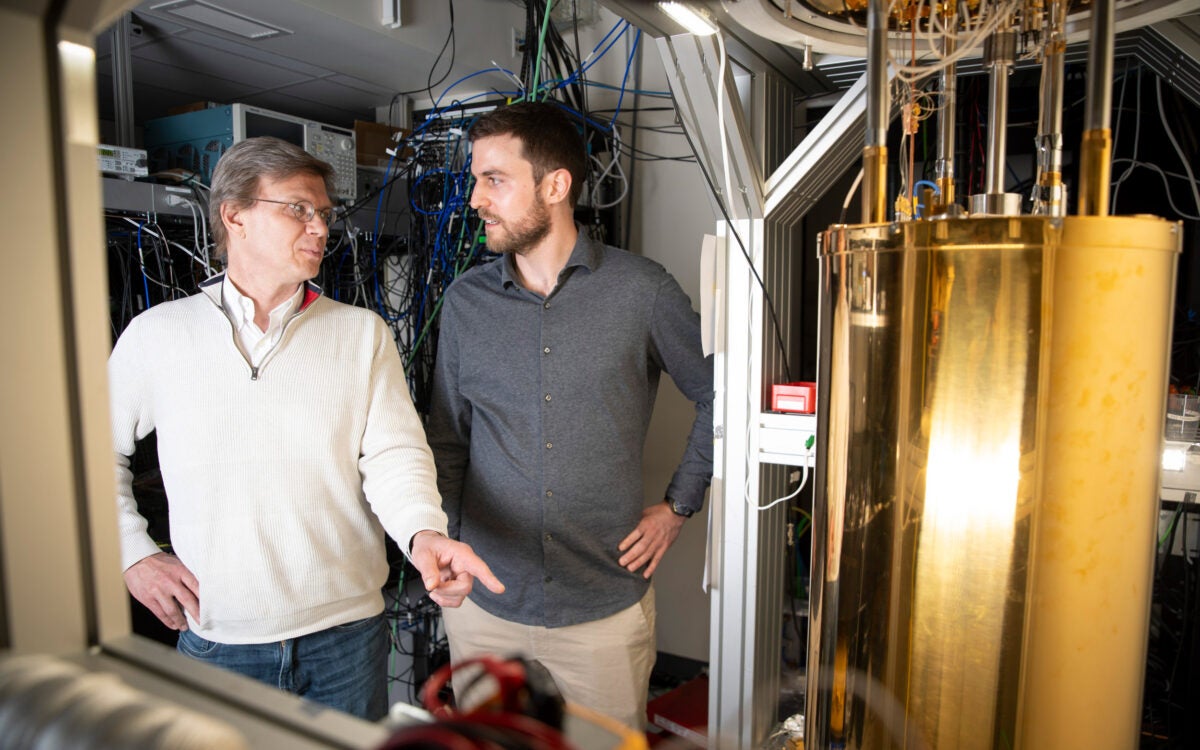
Physicists demo first metro-area quantum computer network in Boston
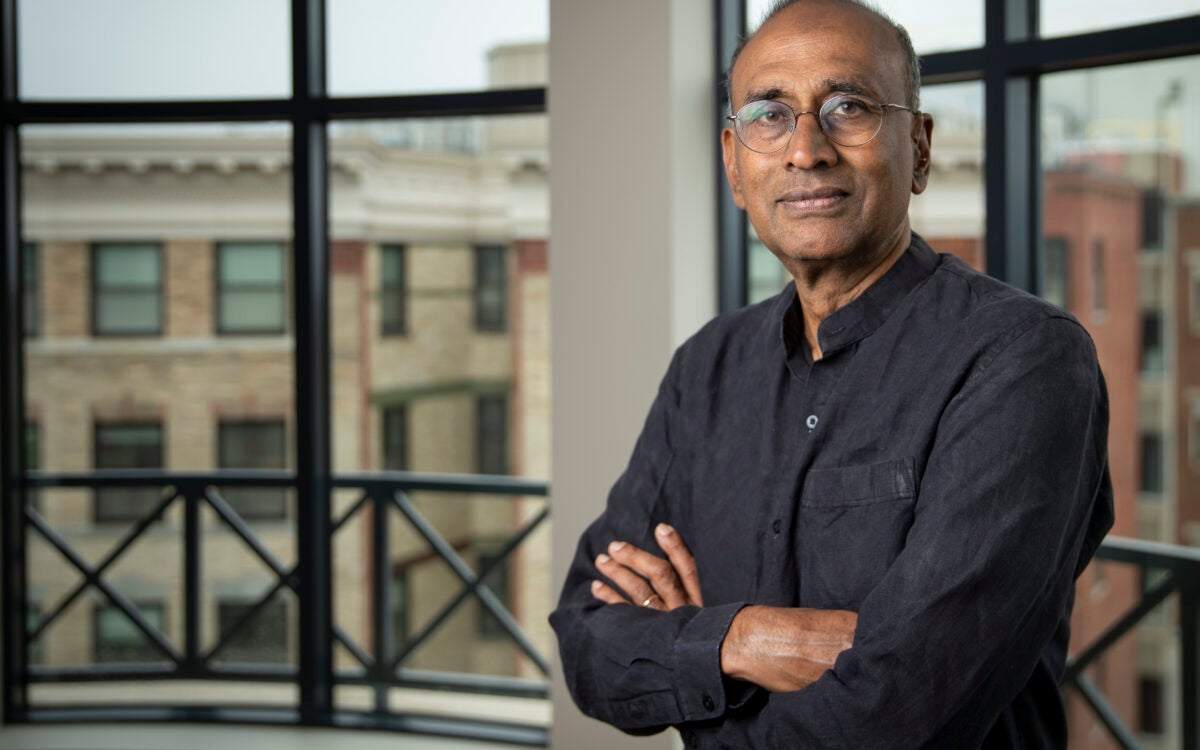
Nobel laureate details new book, which surveys research, touches on larger philosophical questions
Epic science inside a cubic millimeter of brain
Researchers publish largest-ever dataset of neural connections
How far has COVID set back students?
An economist, a policy expert, and a teacher explain why learning losses are worse than many parents realize
Pop star on one continent, college student on another
Model and musician Kazuma Mitchell managed to (mostly) avoid the spotlight while at Harvard
Center for Security and Emerging Technology
Riding the ai wave: what’s happening in k-12 education.
Ali Crawford
Over the past year, artificial intelligence has quickly become a focal point in K-12 education. This blog post describes new and existing K-12 AI education efforts so that U.S. policymakers and other decision-makers may better understand what’s happening in practice.
What’s Happening in Practice?
Last year, artificial intelligence became a focal point in K-12 education. Now more than ever, there is significant interest in understanding not only the landscape of K-12 AI education, but also progress in related subjects like computer science or science, technology, engineering, and mathematics more broadly. Previously, the National Security Commission on AI’s (NSCAI) Final Report stated that investing in AI and STEM education was critical to future U.S. technological competitiveness and national security. However, the education space is quickly becoming saturated with guidance, curricula, materials, and opinions on what AI education is and how to teach about and with AI. To help make sense of the AI education landscape, this blog post describes new and existing K-12 AI education efforts so that U.S. policymakers and other decision-makers may better understand what is happening in practice. These insights and observations are drawn from a deep exploration of K-12 AI education over the last year, including discussions with stakeholders, conference attendances, and literature reviews.
Because the U.S. education system is largely decentralized, progress in K-12 AI education is being driven by local school districts and state departments of education, with input from non-profits and industry partners. In practice, this means that the design, approach, and implementation of K-12 AI education vary across states and school districts. Many factors influence the decisions made at the local level, such as the novelty of AI as a subject in K-12 curricula, varying definitions of what constitutes AI education, access to funding and human capital, and general variance in computer science and technology education progress across the country. For those concerned about the AI digital divide, this creates a sense of unbalance in the education system, where schools that can implement AI curricula are ahead and schools that are unable to are behind.
Our findings include:
Finding 1: High schools are adopting and implementing AI career technical education (CTE) programs. Over the last year, we identified at least 19 high schools across Maryland , Georgia , California , and Florida that have either already implemented or are preparing to implement an AI-specific CTE program. All of the 19 high schools are public schools, and at least eight are magnet schools. The school districts in Maryland, Georgia, and California each developed AI-specific CTE programs and implemented them within individual schools. The Florida Department of Education, in partnership with the University of Florida, developed an AI-specific CTE program at the state level, which 13 Florida high schools either have or are planning to adopt.
Once known as vocational training, CTE programs are optional and supplemental methods of instruction that are designed to prepare students with the technical knowledge, skills, and abilities related to specific occupations and career clusters. CTE programs span nearly every major industry, from healthcare and cosmetology to manufacturing and computer science, and are increasingly seen as an opportunity to strengthen the connection and coherence among K-12 education, postsecondary education, and workforce development efforts. Programs can start as early as middle school but are more common at the high school and technical college levels, thus allowing students to explore future career fields, earn college credits, or obtain industry-recognized certifications. Well-designed pathways may also enable students to gain an early start in their field and shorten the time required to enter the workforce. Almost all public school districts across the United States offer a variety of CTE programs, with approximately 7.5 million secondary students presently enrolled across disciplines.
The new Florida AI CTE pathway is an example of one such program and focuses on technical skill proficiency and competency-based applied learning of AI. There are four classes in this CTE pathway: (1) AI in the World, where students explore the role of data and ethics in AI applications and how AI agents make decisions; (2) Applications of AI, designed to deepen understanding of AI applications and how to build AI models; (3) Procedural Programming, which continues the study of computer programming concepts with a focus on the creation of software applications; and (4) Foundations of Machine Learning, designed to provide students with core foundational knowledge to deepen understanding of machine learning (ML) practices and applications.
Another example is an AI CTE program out of Georgia . In 2022, the district opened an AI-themed high school and created a three-course AI CTE pathway that incorporates AI “literacy” and “core” AI skills into the curriculum. The first course in the CTE pathway, Foundations of AI, introduces students to programming, data science, math, and ethical reasoning skills. The second, AI Concepts, teaches students about the history of AI, current AI research, and the societal and ethical impacts of AI. The third course, AI Applications, teaches students to design and test AI-powered solutions. While this pathway is for students who want to dive deeper into AI-focused studies, an elementary and middle school feeder program has started piloting AI-ready learning embedded courses to develop the necessary skills for deeper student engagement at the high school level.
Finding 2: Nonprofit organizations, academic institutions, and industry fill critical gaps in the educational ecosystem by designing and delivering content and curricula, providing teacher training programs, and emphasizing foundational skill-building. These entities also assist schools, districts, and states with developing curricula and educational frameworks in the absence of state and federal policy. In May 2018, the Association for the Advancement of Artificial Intelligence and the Computer Science Teachers Association launched the AI for K-12 working group (AI4K12) to develop some of the first AI teaching guidelines for K–12 schools. AI4K12 introduced “Five Big Ideas” in AI as its core framework: (1) Perception, (2) Representation and Reasoning, (3) Learning, (4) Natural Interactions, and (5) Societal Impact. The guidelines outline key learning objectives for students across different grade bands and can assist standards writers and curricula developers with incorporating essential knowledge and skills related to AI concepts. The framework is also recognized by the United Nations Educational, Scientific, and Cultural Organization (UNESCO) as one of the few existing AI education frameworks.
There are nonprofits leading efforts to develop readily-adoptable AI education curricula and tools for K-12 classroom educators. One example is the MIT Media Lab, a research laboratory at the Massachusetts Institute of Technology, which developed the DAILy curriculum for middle school students to explore AI concepts and applications using hands-on and computer-based activities. The curriculum includes four units: (1) What is AI? (2) Supervised Machine Learning, (3) Generative Adversarial Networks, and (4) AI + My Future. Each unit includes slides for lessons, teacher scripts, and interactive classroom activities. The curriculum was designed to to scale programming and training for teachers which can be barriers to adoption.
The AI Education Project, another nonprofit organization, promotes general AI literacy by providing AI education to all students through interdisciplinary approaches. The organization developed two classroom-ready courses . The first course, Introduction to AI, uses project-based learning to teach students in 9-12 grade how to build, test, and refine an AI application without coding. The course is designed for computer science, STEM, or CTE teachers. The second course, AI Snapshots, is designed to facilitate a basic understanding of AI and its connections with math, science, English, and social studies beginning in middle school, and is designed for all teachers regardless of discipline.
In addition, nonprofit organizations are emerging to provide schools and policymakers with policy and implementation guidance on AI education. One example is TeachAI , an effort led by Code.org, the Educational Testing Service, the International Society for Technology in Education, and Khan Academy, that highlights both the potential benefits (e.g., assessments, personalized learning, operational efficiency) and harms (e.g., plagiarism, overreliance, perpetuating bias) of AI in the classroom.
Certain federal STEM education initiatives support the critical work of nonprofit and educational leaders in AI K-12 education. One such program is the National Science Foundation’s Innovative Technology Experiences for Students and Teachers (ITEST) which supports research on increasing student interest in careers in STEM from preschool to high school. Recent awards totaling over $13 million support multiple projects aimed specifically at AI education initiatives such as:
- Introducing AI concepts to middle school students and teachers through summer camps, workshops, and school-based programs in rural communities where students often lack access to advanced STEM educational opportunities.
- Developing AI literacy through a research-informed educational ecosystem for after-school and summer programs where functional AI-enabled solutions to problems are presented in fictional stories that the students read in English-language arts and summer reading programs.
- Teaching fundamental machine learning concepts to middle school students by using ML models to classify shark teeth by their shape and function and address educational disparities in STEM to encourage students from underrepresented groups to consider STEM career pathways.
Finding 3: Conceptions of AI literacy can be broad. Defining AI literacy involves finding the right balance between theoretical knowledge and practical skills, as well as determining the necessary depth in each area. A key question is whether the understanding of AI concepts is adequate for achieving literacy, or if students must also possess technical abilities, like coding, to develop and work with AI models.
Our review of existing AI education literature reveals a trend toward definitions that do not mandate hard technical skills. Long and Magerko aggregated emerging themes and trends in the field of AI education by constructing 17 competencies that focus on building knowledge of AI concepts. They define “AI literacy” as “a set of competencies that enables individuals to critically evaluate AI technologies, communicate and collaborate effectively with AI; and use AI as a tool online, at home, and in the workplace.” This framework of AI literacy complements AI4K12’s pioneering work on the “Five Big Ideas,” both emphasizing reflective and evaluative skills over hard technical skills. A proposal for an elementary school AI curriculum incorporates both frameworks, demonstrating their potential for AI education in practice.
However, efforts to build an AI workforce often concentrate on strategies to build and maintain a workforce that is prepared to design, build, and test AI systems, software, or products. The necessary education and training needed will differ from workers with more generalist backgrounds or the average consumer. Therefore, we think the right approach might depend on the educational goal, which requires a necessary distinction between “AI in education” and “AI education.”
AI as an educational or classroom tool considers the use of AI-enabled tools to advance and improve educational practices for effective teaching and learning. This can take many forms , such as classroom management, individualized performance tracking, or other comprehensive classroom analytics that can serve as metrics of literacy in the effective use of AI tools in the classroom. Using AI-enabled tools can foster AI literacy to enable individuals to not only evaluate AI technologies but also to use AI as a tool .
AI education is concerned with actual educational policy and competencies for understanding technical AI concepts, foundations of AI technologies, how AI uses and perceives data, and how to use or build an AI tool. This also includes how concepts of AI are taught in the classroom through examining different agendas, pedagogical approaches, and definitions of what specific lessons and skill-building constitutes “foundational AI.”
Without technical requirements, the core concepts of AI literacy can be captured by, or integrated with, established instructional content in digital literacy and computer science standards across states. Common themes include computer literacy, digital citizenship, and computational thinking. Familiarity with the power and limitations of computation and communication technology is an important facet of STEM literacy, but computational literacy and computational thinking are not just limited to the STEM disciplines and are considered universally beneficial across all disciplines in K-12. States and schools already incorporate digital literacy and computational thinking into state standards and curricula. The motivation for including this type of instruction is that it will enable students to develop skills to deconstruct problems, recognize patterns, and think critically about solutions.
As more states adopt computer science standards in their K-12 curricula, there might be a concurrent rise in either intentionally or unintentionally adopting components of AI literacy for students. However, states may vary in their approaches. For instance, California positions computer science as a standalone standard , on par with subjects like math and science, while other states fold it into a wider curriculum. Decisions on whether to introduce AI literacy as a new, independent standard or to integrate it within existing standards will influence resource allocation and the focus areas for teacher training.
Concluding Considerations
Schools are adopting diverse educational tools and methods, turning to options like CTE programs to address the growing demand for AI education. Non-profit organizations, academic institutions, and industry players are supporting these efforts by developing AI curricula and educational tools to help teachers and schools become better equipped. While the necessity of AI education is widely recognized, the key competencies needed to achieve AI literacy have yet to be established. What is evident, though, is a strong commitment from states and educators to reshape K-12 education.
Commonalities in schools offering standalone AI programming. Schools offering AI programming have existing computer science, data science, cloud computing, or cybersecurity CTE programs. This suggests that schools or states with existing computer science and technology education infrastructure may be more capable of adopting AI education standards or programming. It also suggests that these schools already have faculty that are prepared and able to teach separate AI classes. Therefore, the marginal cost of adding a separate curriculum is likely significantly lower for these schools.
While there is no singular approach to AI education, many share common ground. Efforts to integrate or adopt computer science curricula are one way schools can introduce new technical topics or integrate them into existing ones, develop standards or assessments, and understand the required support for classrooms and educators. Nearly 60% of U.S. high schools offer at least one computer science class and 30 states require that their schools offer computer science. Disparities in access persist in rural regions, urban areas, and low-income communities. This is not to suggest that progress in equitable computer science education across the United States has been slow or stagnant. Instead, it is meant to highlight that a percentage of U.S. schools might not be prepared to begin thinking about AI education.
Schools that do not have structured AI coursework may not be falling too far behind. In its final report, the NSCAI urges the nation to prioritize investments in STEM education based on its assessment that the U.S. education system is not producing sufficient AI talent to meet U.S. demand. This is a national challenge, but education systems can fill this gap even without separate AI classes or educators or specific CTE pathways. For example, it is likely that AI concepts are taught either intentionally or unintentionally within standard computer science, mathematics, and other course work. This is why educational goals are important when considering what constitutes AI literacy—general STEM foundations, such as basic statistics or computer science, can still prepare students for future exploration of AI as a discipline or career.
Teacher shortages are an often-overlooked challenge. Computer science, mathematics, and science teachers are most likely the educators who are more prepared to teach elements of AI education with minimal upskilling required. In some cases, one teacher may act as all three. For the 2023-2024 school year alone, 32 states report some form of a teacher shortage in the area of mathematics and science for at least one school district within the state. Seven states report teacher shortages specifically in computer science. A survey of 1,200 school and district officials by Frontline Education suggests that the top reasons for teacher shortages are: (1) a lack of fully qualified applicants; (2) salary and/or benefits are lacking compared to other careers; and (3) fewer new education school graduates. Even the NSCAI report and others acknowledge that recruiting high-quality K-12 teachers with STEM experience and proficiency is difficult.
Recognizing the need for increased resource allocation in teacher training, states have made positive strides. 2023 saw the largest increase in high schools offering computer science courses since 2018. Thirty-four states have adopted or updated policies to establish computer science as foundational, supported by an allocation of over $120 million in 25 state budgets for computer science education. These funds include increased professional learning opportunities to prepare teachers with the necessary skills to teach computer science effectively. For example, in Colorado , the state-funded Computer Science Teacher Education Grant (CSEd) Program is designed to train teachers in computer science education. Professional learning, upskilling, and certification opportunities are crucial not just for teaching computer science, but also for enabling more teachers with the skills and toolkits to integrate AI education into K-12 classrooms.
Ali Crawford is a research analyst at CSET, where she works on the CyberAI Project. She also serves in an uncompensated capacity on the advisory board of TeachAI, where she represents CSET.
Cherry Wu is a research assistant at CSET, where she works on the CyberAI Project. She is also a current master’s student at Georgetown University’s Walsh School of Foreign Service.
Related Content
Highlights from the national cyber workforce and education strategy.
The much-anticipated National Cyber Workforce and Education Strategy (NCWES) provides a comprehensive set of strategic objectives for training and producing more cyber talent by prioritizing and encouraging the development of more localized cyber ecosystems that… Read More
Levers for Improving Diversity in Computer Science
Universities can build more inclusive computer science programs by addressing the reasons that students may be deterred from pursuing the field. This blog post explores some of those reasons, features of CS education that cause… Read More
Building the Cybersecurity Workforce Pipeline
Creating adequate talent pipelines for the cybersecurity workforce is an ongoing priority for the federal government. Understanding the effectiveness of current education initiatives will help policymakers make informed decisions. This report analyzes the National Centers… Read More
U.S. High School Cybersecurity Competitions
In the current cyber-threat environment, a well-educated workforce is critical to U.S. national security. Today, however, nearly six hundred thousand cybersecurity positions remain unfilled across the public and private sectors. This report explores high school… Read More
This website uses cookies.
Privacy overview.
The Pros and Cons of AI in Special Education

- Share article
Special education teachers fill out mountains of paperwork, customize lessons for students with a wide range of learning differences, and attend hours of bureaucratic meetings.
It’s easy to see why it would be tempting to outsource parts of that job to a robot.
While there may never be a special educator version of “Star Wars”’ protocol droid C-3PO, generative artificial tools—including ChatGPT and others developed with the large language models created by its founder, Open AI—can help special education teachers perform parts of their job more efficiently, allowing them to spend more time with their students, experts and educators say.
But those shortcuts come with plenty of cautions, they add.
Teachers need to review artificial intelligence’s suggestions carefully to ensure that they are right for specific students. Student data—including diagnoses of learning differences or cognitive disorders—need to be kept private.
Even special educators who have embraced the technology urge to proceed with care.
“I’m concerned about how AI is being presented right now to educators, that it’s this magical tool,” said Julie Tarasi, who teaches special education at Lakeview Middle School in the Park Hill school district near Kansas City, Mo. She recently completed a course in AI sponsored by the International Society for Technology in Education. “And I don’t think that the AI literacy aspect of it is necessarily being [shared] to the magnitude that it should be with teachers.”
Park Hill is cautiously experimenting with AI’s potential as a paperwork partner for educators and an assistive technology for some students in special education.
The district is on the vanguard. Only about 1 in 6 principals and district leaders—16 percent—said their schools or districts were piloting AI tools or using them in a limited manner with students in special education, according to a nationally representative EdWeek Research Center survey conducted in March and April.
AI tools may work best for teachers who already have a deep understanding of what works for students in special education, and of the tech itself, said Amanda Morin, a member of the advisory board for the learner-variability project at Digital Promise, a nonprofit organization that works on equity and technology issues in schools.
“If you feel really confident in your special education knowledge and experience and you have explored AI [in depth], I think those two can combine in a way that can really accelerate the way you serve students,” Morin said.
But “if you are a novice at either, it’s not going to serve your students well because you don’t know what you don’t know yet,” she added. “You may not even know if the tool is giving you a good answer.”
Here are some of the areas where Park Hill educators and other school and district leaders see AI’s promise for special education—and what caveats to look out for:
Promise: Reducing the paperwork burden.
Some special education teachers spend as many as eight hours a week writing student-behavior plans, progress reports, and other documentation.
“Inevitably, we’re gonna get stuck, we’re gonna struggle to word things,” Tarasi said. AI can be great for busting through writer’s block or finding a clearer, more objective way to describe a student’s behavior, she said.
What’s more, tools such as Magic School—an AI platform created for K-12 education—can help special education teachers craft the student learning goals that must be included in an individualized education program, or IEP.
“I can say ‘I need a reading goal to teach vowels and consonants to a student,’ and it will generate a goal,” said Tara Bachmann, Park Hill’s assistive-technology facilitator. “You can put the criteria you want in, but it makes it measurable, then my teachers can go in and insert the specifics about the student” without involving AI, Bachmann said.
These workarounds can cut the process of writing an IEP by up to 30 minutes, Bachmann said—giving teachers more time with students.
AI can also come to the rescue when a teacher needs to craft a polite, professional email to a parent after a stress-inducing encounter with their child.
Some Park Hill special education teachers use “Goblin,” a free tool aimed at helping neurodivergent people organize tasks, to take the “spice” out of those messages, Tarasi said.
A teacher could write “the most emotionally charged email. Then you hit a button called ‘formalize.’ And it makes it like incredibly professional,” Bachmann said. “Our teachers like it because they have a way to release the emotion but still communicate the message to the families.”
Caveat: Don’t share personally identifiable student information. Don’t blindly embrace AI’s suggestions.
Teachers must be extremely careful about privacy issues when using AI tools to write documents—from IEPs to emails—that contain sensitive student information, Tarasi said.
“If you wouldn’t put it on a billboard outside of the school, you should not be putting it into any sort of AI,” Tarasi said. “There’s no sense of guaranteed privacy.”
Tarasi advises her colleagues to “absolutely not put in names” when using generative AI to craft documents, she said. While including students’ approximate grade level may be OK in certain circumstances, inputting their exact age or mentioning a unique diagnosis is a no-no.
To be sure, if the information teachers put into AI is too vague, educators might not get accurate suggestions for their reports. That requires a balance.
“You need to be specific without being, without being pinpoint,” Tarasi said.
Caveat: AI works best for teachers who already understand special education
Another caution: Although AI tools can help teachers craft a report or customize a general education lesson for students in special education, teachers need to already have a deep understanding of their students to know whether to adopt its recommendations.
Relying solely on AI tools for lesson planning or writing reports “takes the individualized out of individualized education,” Morin said. “Because what [the technology] is doing is spitting out things that come up a lot” as opposed to carefully considering what’s best for a specific student, like a good teacher can.
Educators can tweak their prompts—the questions they ask AI—to get better, more specific advice, she added.
“A seasoned special educator would be able to say ‘So I have a student with ADHD, and they’re fidgety’ and get more individualized recommendations,” Morin said.
Promise: Making lessons more accessible.
Ensuring students in special education master the same course content as their peers can require teachers to spend hours simplifying the language of a text to an appropriate reading level.
Generative AI tools can accomplish that same task—often called “leveling a text"—in just minutes, said Josh Clark, the leader of the Landmark School , a private school in Massachusetts serving children with dyslexia and other language-based learning differences.
“If you have a class of 30 kids in 9th grade, and they’re all reading about photosynthesis, then for one particular child, you can customize [the] reading level without calling them out and without anybody else knowing and without you, the teacher, spending hours,” Clark said. “I think that’s a super powerful way of allowing kids to access information they may not be able to otherwise.”
Similarly, in Park Hill, Bachmann has used Canva—a design tool with a version specifically geared toward K-12 schools and therefore age-appropriate for many students—to help a student with cerebral palsy create the same kind of black-and-white art his classmates were making.
Kristen Ponce, the district’s speech and language pathologist, has used Canva to provide visuals for students in special education as they work to be more specific in their communication.
Case-in-point: One of Ponce’s students loves to learn about animals, but he has a very clear idea of what he’s looking for, she said. If the student just says “bear,” Canva will pull up a picture of, for instance, a brown grizzly. But the student may have been thinking of a polar bear.
That gives Ponce the opportunity to tell him, “We need to use more words to explain what you’re trying to say here,” she said. “We were able to move from ‘bear’ to ‘white bear on ice.’”
Caveat: It’s not always appropriate to use AI as an accessibility tool.
Not every AI tool can be used with every student. For instance, there are age restrictions for tools like ChatGPT, which isn’t for children under 13 or those under 18 without parent permission, Bachmann said. (ChatGPT does not independently verify a user’s age.)
“I caution my staff about introducing it to children who are too young and remembering that and that we try to focus on what therapists and teachers can do collectively to make life easier for [students],” she said.
“Accessibility is great,” she said. But when a teacher is thinking about “unleashing a child freely on AI, there is caution to it.”
Promise: Using AI tools to help students in special education communicate.
Park Hill is just beginning to use AI tools to help students in special education express their ideas.
One recent example: A student with a traumatic brain injury that affected her language abilities made thank you cards for several of her teachers using Canva.
“She was able to generate personal messages to people like the school nurses,” Bachmann said. “To her physical therapist who has taken her to all kinds of events outside in the community. She said, ‘You are my favorite therapist.’ She got very personal.”
There may be similar opportunities for AI to help students in special education write more effectively.
Some students with learning and thinking differences have trouble organizing their thoughts or getting their point across.
“When we ask a child to write, we’re actually asking them to do a whole lot of tasks at once,” Clark said. Aspects of writing that might seem relatively simple to a traditional learner—word retrieval, grammar, punctuation, spelling—can be a real roadblock for some students in special education, he said.
“It’s a huge distraction,” Clark said. The student may “have great ideas, but they have difficulty coming through.”
Caveat: Students may miss out on the critical-thinking skills writing builds.
Having students with language-processing differences use AI tools to better express themselves holds potential, but if it is not done carefully, students may miss developing key skills, said Digital Promise’s Morin.
AI “can be a really positive adaptive tool, but I think you have to be really structured about how you’re doing it,” she said.
ChatGPT or a similar tool may be able to help a student with dyslexia or a similar learning difference “create better writing, which I think is different than writing better,” Morin said.
Since it’s likely that students will be able to use those tools in the professional world, it makes sense that they begin using them in school, she said.
But the tools available now may not adequately explain the rationale behind the changes they make to a student’s work or help students express themselves more clearly in the future.
“The process is just as important as the outcome, especially with kids who learn differently, right?” Morin said. “Your process matters.”
Clark agreed on the need for moving cautiously. His own school is trying what he described as “isolated experiments” in using AI to help students with language-processing differences express themselves better.
The school is concentrating, for now, on older students preparing to enter college. Presumably, many will be able to use AI to complete some postsecondary assignments. “How do we make sure it’s an equal playing field?” Clark said.
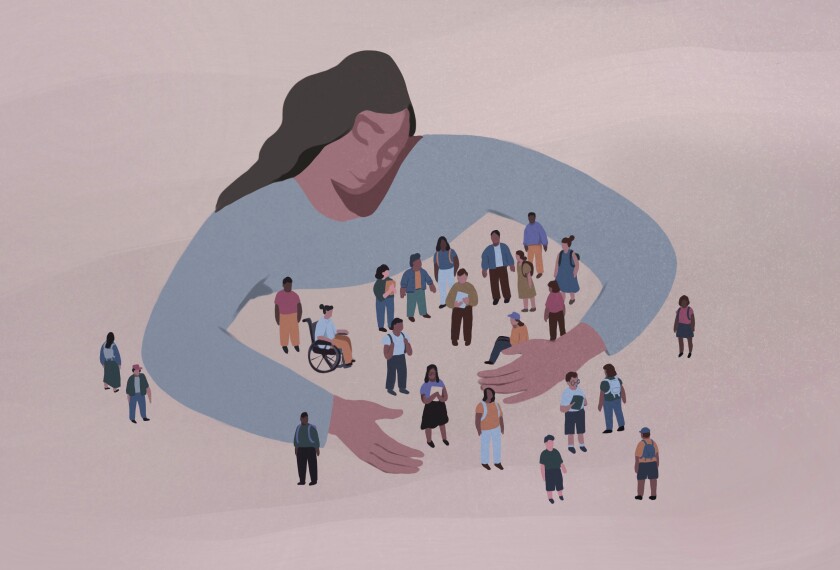
Sign Up for EdWeek Update
Edweek top school jobs.

Sign Up & Sign In


NEWS: IITE and partners in action

TeensLIVE Armenia Empowers Youth Through Interactive Training Sessions

UNESCO IITE took part in the Qatar National Consultation on Media and Information Literacy
Unesco iite and kaktus media launched a digital encyclopedia for parents in kyrgyzstan, an adolescent in the city–2024: conference roundtable on choices adolescents make.

UNESCO IITE Celebrates Inaugural International Day of Digital Learning
Announcements, call for an individual consultant to carry out the best practice analysis and research in “improving teachers’ digital technology competencies, including intelligent educational literacy”, individual consultancy: review of digital technologies and tools used for assessment and feedback in higher education (deadline extended).

Teaching in the 21st Century Teacher Competition 2.0 (Extended)
Call for an individual consultant to carry out the research and best practice analysis in “digital inclusion and e-learning for the elderly” (extended), vacancy: consultant – developing an online course for tvet educators on creativity.

Official launch of the platform “E-Library for teachers”

A chatbot for teenagers about puberty, relationships, and health launched in Kyrgyzstan

Combat COVID-19: Keep learning. Together we are on the move!
Unesco education news.

UNESCO Prize for Girls’ and Women’s Education now open for 2024 nominations

Global report on teachers: What you need to know

Fostering a Culture of Lifelong Learning in the Digital Era
- Newsletters
IE 11 Not Supported
Opinion: what is higher ed’s role in providing ai job skills, in addition to programming and technical skills, the next generation of ai developers may also need training in subjects traditionally aligned with liberal-arts education, such as ethics, problem-solving and communication..

WHAT AI WILL DO — AND WHAT IT STILL CAN’T
Critical skills for working in ai, according to ai, ai replacing jobs in higher ed.

More From Forbes
Formation upskills displaced tech workers with ai-driven education.
- Share to Facebook
- Share to Twitter
- Share to Linkedin
Dynamic cohorts and content keep students on the optimal flightpath.
With new waves of technology constantly emerging and nearly 250,000 tech workers displaced, the need for upskilling and retooling is pervasive. Since at least the 1960s , tech companies and universities have been exploring ways of using technology to allow the latter to serve the former, a need driven by the fact that the skills engineers require as undergraduates need to be continually refreshed if they are to meet the demands of a fast-changing tech world. As rates of technological change continue to increase, and with the emergence of technologies like blockchain, large language models, generative pre-trained transformers, and other types of AI, not only is the education one receives when at college quickly outdated, but the education provided by colleges may not be the best way to learn what companies are looking for. Something faster and more relevant is needed.
Against this backdrop, Formation has created an adaptable learning system that harnesses AI to solve this problem for software engineers and other technical workers looking to transition from mid-level positions to senior ones. Through a dynamic approach to constructing courses and cohorts that locks the outcome while varying the time, Formation has shown the potential to deliver life-changing outcomes, with students seeing significant returns on their educational investment .
Individual Differences And Individualized Approaches
The biggest challenge of teaching any group of students is the fact no matter how similar they may be, they will inevitably need different things at different times if their educational trajectory is to be optimal.
When I was directing the Education Program for Gifted Youth at Stanford University, we would often confront the following scenario. A group of parents would find themselves at a school, perhaps at some sporting event, and would begin to share frustrations about the pace of their children's mathematics course. The frustration would inevitably be that they spend too much time reviewing things their kid already knows. Collectively, they would wish they could have a teacher ready to quickly go through the easier material and slowly go through the more difficult. What the parents would inevitably fail to recognize, but what the data clearly showed, is that what one student finds hard, another will find easy, and this will remain true no matter how rarefied the population is. Time and again, the conclusion was clear: individual differences are robust and multidimensional.
Consider, then, that if even a subject as stable as elementary school mathematics was hard to optimize in a classroom, how much harder must it be working with a constantly evolving curriculum where students come to the class with radically diverse backgrounds and knowledge?
Google Chrome Gets Third Emergency Update In A Week As Attacks Continue
Japanese fans are puzzled that yasuke is in assassin s creed shadows, forbes releases 2024 30 under 30 asia list.
To optimize a class for such a group of students, at least three things must be done:
- Understand what students already know so that time is not wasted reteaching
- Understand the destination students are after
- Understand how, given where they are, what the next thing they need to learn is to be on the optimal path toward that destination.
This is the problem that Formation is addressing with its dynamic learning system.
Dynamic Cohorts And Adaptive Learning
Formation takes a dynamic approach to solving the problem of effective cohort formation and determining targeted content delivery. Each week, their adaptive learning system dynamically assembles cohorts based on a set of proprietary parameters, including the goals of students and their current velocity along their learning trajectories. Each cohort will meet as a class to work with a mentor on that week’s topic. At the end of the week, students are assessed, the cohorts are refreshed, and new classes are assembled. This approach ensures that students remain in their zone of proximal development and that no one moves forward without first attaining the knowledge needed to take the next step.
Sophie Novati, CEO and co-founder of Formation, sees this style of adaptive learning as so clearly superior that she envisions a future where the idea that once upon a time, you could get a class schedule on Day 1, delineating what would happen in each future class session, will seem preposterous. No matter who is learning, it is inevitable that they will be different from their peers in their starting points, their trajectories, and their ultimate destination. Rather than chain students together in the expectation they will advance in lockstep with their classrooms, Formation sets each person free to reach their end goal as soon as possible while ensuring that they have learned what they set out to learn when they arrive.
With their adaptive learning environment, Formation can assure students that given where they are and whether they want to go, Formation will ensure they are on the shortest path from A to B. From a student's point of view, they can trust that Formation will see them through to the end, no matter how long it takes. Formation is so confident that it will deliver optimal results that it offers unlimited access memberships that provide continuing training until the student is placed. These can be paid for in several ways, ranging from all-at-once deferred loan payments to income-based repayments.
In the end, as a company, Formation is committed to getting students where they want to be. As regards how long that might take, the simple answer is “as soon as possible.”
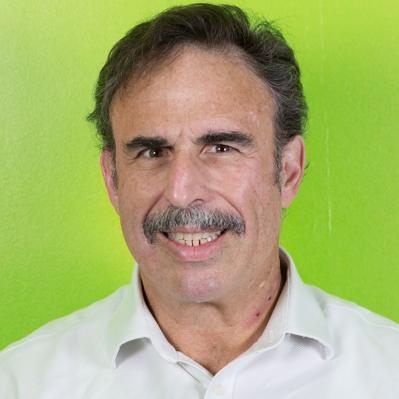
- Editorial Standards
- Reprints & Permissions
Join The Conversation
One Community. Many Voices. Create a free account to share your thoughts.
Forbes Community Guidelines
Our community is about connecting people through open and thoughtful conversations. We want our readers to share their views and exchange ideas and facts in a safe space.
In order to do so, please follow the posting rules in our site's Terms of Service. We've summarized some of those key rules below. Simply put, keep it civil.
Your post will be rejected if we notice that it seems to contain:
- False or intentionally out-of-context or misleading information
- Insults, profanity, incoherent, obscene or inflammatory language or threats of any kind
- Attacks on the identity of other commenters or the article's author
- Content that otherwise violates our site's terms.
User accounts will be blocked if we notice or believe that users are engaged in:
- Continuous attempts to re-post comments that have been previously moderated/rejected
- Racist, sexist, homophobic or other discriminatory comments
- Attempts or tactics that put the site security at risk
- Actions that otherwise violate our site's terms.
So, how can you be a power user?
- Stay on topic and share your insights
- Feel free to be clear and thoughtful to get your point across
- ‘Like’ or ‘Dislike’ to show your point of view.
- Protect your community.
- Use the report tool to alert us when someone breaks the rules.
Thanks for reading our community guidelines. Please read the full list of posting rules found in our site's Terms of Service.
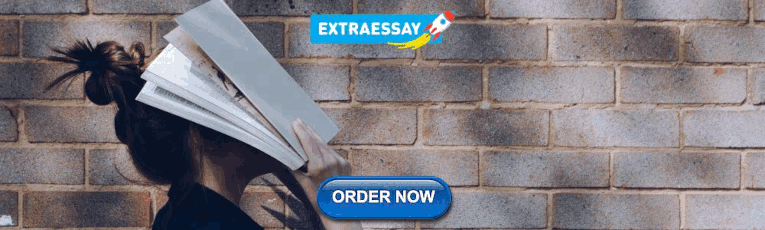
IMAGES
VIDEO
COMMENTS
Generative AI for Educators is a two-hour, self-paced course designed to help teachers save time on everyday tasks, personalize instruction to meet student needs, and enhance lessons and activities in creative ways with generative AI tools — no previous experience required. Developed by experts at Google in collaboration with MIT RAISE ...
This course is designed by teachers, for teachers, and will bridge the gap between commonly held beliefs about AI, and what it really is. AI can be embedded into all areas of the school curriculum and this course will show you how. This course will appeal to teachers who want to increase their general understanding of AI, including why it is ...
There are 4 modules in this course. Discover the foundations of generative AI in our dynamic course. Gain a comprehensive grasp of generative AI basics, including definitions, prompt engineering, ethical considerations, and best practices. This engaging, discussion-focused course empowers learners to explore generative AI through hands-on ...
Here are a few of our favorites, with more on the way: Generative AI for Educators A Guide to AI in Education Grow with Google: AI and machine learning courses Applied Digital Skills: Discover AI in Daily Life Google Cloud Skills Boost: Intro to Gen AI Learning Path Introduction to Machine Learning Google Arts & Culture: overview of AI ...
AI chatbots & friendship. Khan Academy believes that AI and Large Language Models hold the potential to transform education. By providing teachers and students resources and tools to design joyful, personalized, interactive, engaging learning experiences that drive deep learning in the months and years ahead, we hope to become a trusted partner ...
At the recent AI+Education Summit, Stanford researchers, students, and industry leaders discussed both the potential of AI to transform education for the better and the risks at play. ... Several themes emerged over the course of the day on AI's potential, as well as its significant risks. First, a look at AI's potential: 1. Enhancing ...
The 2023 AI Index Report from the Stanford Institute for Human-Centered AI has documented notable acceleration of investment in AI as well as an increase of research on ethics, including issues of fairness and transparency.2 Of course, research on topics like ethics is increasing because problems are observed.
This is the Harvard EdCast. Chris Dede thinks we need to get smarter about using artificial intelligence and education. He has spent decades exploring emerging learning technologies as a Harvard researcher. The recent explosion of generative AI, like ChatGPT, has been met with mixed reactions in education.
Advances in artificial intelligence (AI) could transform education systems and make them more equitable. It can accelerate the long overdue transformation of education systems towards inclusive learning that will prepare young people to thrive and shape a better future.; At the same time, teachers can use these technologies to enhance their teaching practice and professional experience.
Artificial Intelligence (AI) has the potential to address some of the biggest challenges in education today, innovate teaching and learning practices, and accelerate progress towards SDG 4. However, rapid technological developments inevitably bring multiple risks and challenges, which have so far outpaced policy debates and regulatory frameworks.
With increasing interest in AI and education, the Education 4.0 Alliancesought to understand the current state and future promises of the technology for education. The latest report - Shaping the Future of Learning: The Role of AI in Education 4.0- shows four key promises that have emerged for AI to enable Education 4.0: 1.
ISTE and ASCD are developing the first AI coach specifically for educators. With Stretch AI, educators can get tailored guidance to improve their teaching, from tips on ways to use technology to support learning, to strategies to create more inclusive learning experiences. Answers are based on a carefully validated set of resources and include ...
Learn about the latest updates and availability for Microsoft Copilot in education. Smart learning: AI resources every educator should know. Enhance AI literacy with 11 resources from Microsoft Education useful for educators, parents/guardians, and curious learners. Unlocking productivity and personalizing learning with AI.
This module is part of these learning paths. AI for educators. Skill up with the latest in AI, Microsoft 365, Windows, and security in education. Explore essential AI concepts, techniques, and tools that can support personalized learning, automate daily tasks, and provide insights for education.
Description. This course is designed for educators who are interested in understanding the basics of Artificial Intelligence (AI) in Education. The course will provide an overview of AI in education and help you gain an understanding of AI technologies in the educational setting. You will learn about the current usage of AI in classrooms and ...
Credit: AI4K12 (CC BY NC SA 4.0) In fact, in 2019, an NSF-funded project led to the development of national guidelines for teaching and learning about AI in K-12 school settings. Prior to this, there were few to no curriculums in the U.S. dedicated to teaching young, pre-college students about the fundamental knowledge and skills related to AI.
Having teachers train an AI system to grade yielded marginal improvement. Now, they're making progress by having AI systems apply learning from past exams to grade new ones. They'll test the system more fully this year. On the training front, Piech is overseeing efforts to offer AI-based teacher puzzles and feedback to improve teaching skills.
As of 2021, more than 30 countries have released national artificial intelligence (AI) policy strategies. These documents articulate plans and expectations regarding how AI will impact policy sectors, including education, and typically discuss the social and ethical implications of AI. This article engages in thematic analysis of 24 such national AI policy strategies, reviewing the role of ...
Artificial intelligence tools such as ChatGPT, Gemini, Copilot and many others are forcing educators to rethink assignments, activities, courses, and questions. ... This course was also awarded the 2024 Outstanding Program: Noncredit Award by the UCPEA, the online and professional education association, recognizing the course for its high quality.
With artificial intelligence technology rapidly advancing, USF students will soon see some changes in their courses and how they participate in assignments. USF's Innovative Education has made generative AI part of its broader strategy as it seeks to help faculty integrate it into online courses - serving as a test case for broader course ...
Computer science concentrator and master's degree student Naomi Bashkansky '25, who is exploring AI safety issues with fellow students, urged Harvard to provide thought leadership on the implications of an AI-saturated world, in part by offering courses that integrate the basics of large language models into subjects like biology or writing.
Because the U.S. education system is largely decentralized, progress in K-12 AI education is being driven by local school districts and state departments of education, with input from non-profits and industry partners. In practice, this means that the design, approach, and implementation of K-12 AI education vary across states and school districts.
AI tools may work best for teachers who already have a deep understanding of what works for students in special education, and of the tech itself, said Amanda Morin, a member of the advisory board ...
This year, the competition will focus on promoting green education, the use of generative AI, the development of creativity, as well as gamification and game-based learning topics. 14 May 2024 Call for an individual consultant to carry out the Research and Best Practice Analysis in "Digital inclusion and e-learning for the elderly" (Extended)
The materials in the course are based on studies carried out for the UNESCO Institute of IT in Education, Moscow. Learning Outcomes: to be aware of the range of reasons for using ICT to critique the strategies for developing ICT over time to analyse the strengths and weakness of different decision-making mechanisms to become familiar with a ...
While higher education plays a key role in educating and training students for prospective careers in AI, ironically, traditional jobs within the education environment could be changing at the ...
Despite challenging times, artificial intelligence (AI) has become part of MTUCI's toolbox to protect public health as the university enters "the new normal" of hybrid learning. ... offering training at all levels of higher education, from bachelor to doctoral degrees. MTUCI has been actively involved with the International ...
An AI bootcamp is an intensive, accelerated training program that explores AI fundamentals, concepts and techniques and equips learners with career-ready skills. AI bootcamps focus on practical ...
Formation's adaptable learning system harnesseses AI to dynamically create courses and cohorts allowing engineers to upskill in pursuit of career advancement.
New University of Nevada online courses aim to teach future educators about AI limitations through competition. Amid the swirl of concern about generative artificial intelligence in the classroom, a Nevada university is trying a different tactic by having students compete against ChatGPT in writing assignments. Students in two courses at the University of Nevada, Reno, are going head-to-head ...