
Access to 13 certificate programs, courses and all future releases
Personal Coaching and Career Guidance
Community and live events
Resource and template library

- Game Theory in HR: Applications...
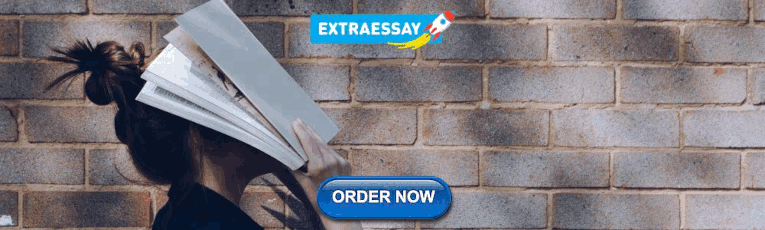
Game Theory in HR: Applications and 3 Case Study Examples

How has HR traditionally mitigated metrics constraints?
- Game theory
- Simulation modeling

The differences between game theory and simulation modeling
Can quickly be incorporated into almost any tactical/one-time day-to-day HR operational problem or project. It is best suited where a key tactical goal is clearly defined. | More intuitive, provides detailed, granular prescriptive insights for identifying and improving all root causes related to any HR process/sub-process problem and provides solutions to manage or eliminate it. |
Needs less data and, in some cases, no data at all. | Very easy to grasp by businesses. |
Can also combine problems relating to multiple sub-processes within the same model/project and give an integrated solution for a project. | Does require a lot of detailed process data and nifty work for intuitive and interactive dashboarding. |
Both are distinct disciplines for HR business analysis, although it may be possible to deploy them together. |

Game theory explained
Can game theory be applied to any out-of-the-box discipline.
- Combinatorial games
- Bayesian game
Solving gender diversity in top leadership via game theory An employee experience platform Humaxa researched gender and racial diversity in leadership in the United States. Based on a study from Maryville University, the split between men and women was 68%/32%. This is typical problem that HR professionals have been trying to address for years. For example, how can we ensure a higher probability of women leaders being promoted to an SVP level? The solution to this is via enabling scenarios in the corporate ladder wherein: 1. Sufficient number of men retire at VP/Director level, thereby increasing the probability of women getting promoted 2. Ensure an attractive voluntary retirement for men at senior management level 3. Lateral hiring of talented women at VP level What is the precise route map and metrics for achieving this? Game theory has answers to such strategic HR problems.
The strategic benefits of game theory for HR
Game theory for hr case studies, case study 1: talent acquisition.
- Location: Conference room, People analytics department, a fortune 500 MNC
- Host: VP People analytics
- Client : VP talent acquisition
HR Game problem statement:
- Need to hire 5 sales direct to head different zones to handle a new line of products about to be launched
- 2 of the hires need to be women
- The average hire’s experience should not be less than 4 years in a senior leadership role.
- Question: Can game theory for HR provide insights to meet the below requirements?
Solution statement :
- You should interview a maximum of 14 male and 8 female candidates who fulfill the prerequisites for the job role of director. There is a 92% chance that you will find the required number of fitments.
- There is an 87% chance that the process will be completed in 84 days and an 80% chance it will be completed in 72 days.
- It is advised not to include any candidate below 2 years of experience in a leadership role. If you do, there is a 90% chance that the average experience in a senior leadership role will be 4 years or above.
How to report this simplistically to HR leadership:
The calculations (non-technical overview).
4 years of high-level recruitment data for director level (region-specific) | |
– Combinatorial and Bayesian game – Game theory for HR is essentially an HR adoption of the mathematical discipline of probability theory. – The approaches used to develop the game theory model were: — Separate binomial probability of men and women, multiplied by the number of applicants — Poisson distribution for the number of days required to complete interviews — Normal distribution and anomaly testing of the selected directors over the last 3 years | |
3 days | |
Alteryx workflow OR Excel Macros OR Python | |
This can be deployed as a one-time analysis (before the start of a project) or as a CEP dashboard running continuously (updating every day) through the lifecycle of the project |
Case study 2: Rewards
- Client: VP Rewards
HR Game Problem Statement :
- Business needs to ensure that exactly 8 directors accept voluntary retirement(VR)
- The average tenure of all the directors accepting VR should be 14 years
- The VR offer can only be given in sequence and after the initial 8 have received the offer. This means that the 9th director can only be given the option to retire voluntarily if 1 out of the 8 directors does not accept the offer.
- The VP Rewards wants to know the minimum number of serving directors that should be given this option to ensure that 8 directors will accept VR.
- Additionally, the VP Rewards wants to know how to ensure that the average tenure will be at least 16 years.
Solution statement:
- To meet the goal of 8 VRs, Rewards will need to give the VR option to 27 Directors to ensure an 99% chance.
- It is not recommended to give the VR to directors with less than 12 years tenure. If so, there is a 95% chance that all the directors accepting the VR program will have an average tenure of at least 14 years.
- The correct sequence in which potential directors must be given the VR can only be analyzed via machine learning, which is beyond the scope of game theory.
How to report this simplistically to HR leadership
6 years high-level VR data of director level (region-specific) | |
– Sequential hypergeometric distribution through looping – Group loops were needed for computation because the VR is executed in phases. | |
7 days | |
Alteryx workflow OR Excel Macros OR Python |
Case study 3: Learning & development
- Client 3: VP Learning & Development (L&D)
Game problem statement :
- L&D is organizing multiple online “remote working leadership training” sessions for senior leaders (such as directors and above) across all 5 global zones.
- L&D must ensure that at least 300 leaders from 5 regions attend one online session.
- VP L&D wants to know how many sessions must be conducted to ensure that 300 or more leaders attend. Also, whether the sessions should be conducted on specific days of the week and how many days can the entire program be completed (a session length will be 4 hours).
- Conduct 12 sessions. 97% chance that 300 or more leaders will attend. Conduct the sessions on Friday afternoon, followed by Wednesday evenings.
- There is an 85% chance that the 12 sessions will be spread over 4 months.
No actual data was available because there was no need for such an exercise in the pre-pandemic period. A small internal experiment was conducted, and the results were magnified to generate soft-simulated data. | |
– Extended Poisson model was employed based on the limited data available. – Reclusion loop was used, and multiple Poisson computations were completed after each simulated session without replacement to arrive at a figure of 12 sessions. – An ANOVA test was conducted on the pre-lunch and post-lunch sessions for all days of the week to find the optimal time slots. | |
7 days | |
Alteryx workflow OR Excel Macros OR Python | |
This can be deployed as a one-time analysis before the start of a project or as a CEP dashboard that runs continuously through the lifecycle of the project. |
Unique challenges:
The methodology used.
- The business case is transformed into game theory
- Historical data was taken ( 3 or 4 years of data )
- Distribution type observed ( this can be done in-house by Minitab statistical software or via taking an obfuscated sample and parsing it via an online tool )
- Probability statistics
- Binomial distribution calculator
- The probabilities are multiplied together for joint probability (as required)
- Compounding reclusive looping has to be done if required (like in the rewards use case) via python or R.
- The probability calculations use linear algebra and factorials. No one needs to do this in practice, as preconfigured statistical tools ( Minitab, Online sites ) are available to calculate this.

Key technical snippets used in the examples
- Some of the above HR-specific computations involve stretching applied probability functions to their limits but are all easily doable by a statistician.
- The above examples represent only 1% of the possibilities of its application in HR. Game theory can be applied to almost every conceivable transactional HR process.
- Most cases only need obfuscated high-level data, which takes care of the need for data confidentiality regulations like the EU GDPR etc. This is a significant advantage when compared to analytics and machine learning approaches.
- Theoretically, these computations can even be executed on excel macros; however, a better idea might be to use platforms such as Alteryx or Python programming.

Why is game theory not extensively used in operational HR?
T he pros and cons of hr gaming theory vs. hr machine learning and ai.
Minimal and only high-level data required | Big data and unobfuscated data required |
Can be computed using a regular PC or a smartphone, with Excel, | Needs a powerful computer or distributed computing |
Formula can be adapted to give immediate answers to any tactical, operational HR problem | Rigid in application possibilities. 90% of machine learning projects in HR are still centered around attrition, succession planning, and workforce optimization |
To conclude
Weekly update.
Stay up-to-date with the latest news, trends, and resources in HR

Carolyn Peer

Raja Sengupta
Related articles.

15 Important Change Management Metrics To Track (In 2024)

11 Important Employee Relations Metrics To Track

Candidate Experience Metrics: How To Measure and Improve Candidate Experience
New articles.

7 Employee Engagement Models To Help Improve Engagement (In 2024)

HRBP Soft Skills: How To Successfully Influence Stakeholders
Subscribe to our weekly newsletter, are you ready for the future of hr.
Learn modern and relevant HR skills, online

- Geopolitics
- Computer Science
- Health Science
- Exercise Science
- Behavioral Science
- Engineering
- Mathematics
Game Theory: Real-Life Examples and Applications

Game theory is the study of strategic interaction between players in different scenarios. Game theory attempts to explain and predict behavior in strategic situations, such as the stock market, auctions, bargaining, and competition. It is a branch of mathematics that is applicable in many fields, such as economics, business, politics, psychology, and evolutionary biology. Game theory provides a systematic approach to decision making that can be used to identify strategies to maximize rewards or minimize losses. In this article, we will explore game theory and provide some real-world examples of its application.
What is Game Theory?
Game theory is a branch of mathematics used to study strategic decision making. In a game-theoretic setting, two or more players take turns making decisions to maximize their payoff. The game-theoretic solution is usually presented as a combination of strategies that each player should use in the game. The game-theoretic solutions are based on an individual’s decision-making process, their preferences, and the outcomes of the game.
At its core, game theory looks at how players interact when they are trying to make the best decision for themselves. It is based on the underlying assumption that players have rational preferences that they can make use of to maximize their payoff. As such, game theory offers players a way to make optimal decisions under uncertain circumstances.
Examples of Game Theory
Game theory can be applied to a variety of situations, from business strategy to military tactics. Here are some examples of game theory in action:
The Prisoner’s Dilemma
The prisoner’s dilemma is perhaps the most iconic example of game theory. In this situation, two suspects are in custody and are offered a deal. Both suspects can either stay silent or confess to a crime. If one suspect stays silent and the other confesses, then the one who confessed gets a reduced sentence while the other gets a harsh sentence. If both suspects stay silent, they both get a reduced sentence. If both suspects confess, they both get a harsher sentence.
The prisoner’s dilemma illustrates how competing interests can lead to an unfavorable outcome for both players. In this case, the suspects would both be better off if they stayed silent, but since they cannot trust each other they are both likely to confess despite the fact that this will result in a harsher sentence.
The Stag Hunt
The stag hunt is another classic example of game theory. In this situation, two hunters must decide whether to hunt a deer or a rabbit. If both hunters hunt the deer, then they will both get a larger reward. If one hunter hunts the rabbit and the other hunts the deer, then the one who hunted the deer will get the larger reward. If both hunters hunt the rabbit, then they will both get a smaller reward.
The stag hunt illustrates how cooperation can lead to a better outcome for both players. In this case, both hunters would be better off if they cooperated and hunted the deer, but since they cannot trust each other they are both likely to hunt the smaller reward of the rabbit.
The Battle of the Sexes
The battle of the sexes is a famous game theory example that illustrates how different preferences can lead to tension between two players. In this situation, two people have different preferences on what they would like to do on Saturday night, but they must come to a mutual agreement if they are going to enjoy their evening. The two players must decide whether to go to the opera or the football game.
The battle of the sexes illustrates how conflicting interests can lead to an impasse. In this case, both people would be better off if they can come to a mutual agreement, but since they have conflicting preferences they may not be able to do so.
The Greek Gift Dilemma
The Greek gift dilemma is another classic game theory example. In this situation, two people are offered a gift and must decide whether to accept or reject it. If one person accepts the gift and the other rejects it, then the person who accepted the gift gets the reward while the other gets nothing. If both people accept the gift, then they both get a smaller reward. If both people reject the gift, then they both get nothing.
The Greek gift dilemma illustrates how conflicting interests can lead to an undesirable outcome for both players. In this case, both people would be better off if they can
Christophe Garon
February 9, 2023
Comments are closed.
Follow Me On Social
Stay in the loop, recent posts.
- Exploring VHE Gamma-Ray Emission in the Milky Way’s Galactic Centre: Insights from H.E.S.S.
- Exploring Toeplitz Covariance Clustering: A Revolutionary Approach to Multivariate Time Series Analysis
- Understanding Thiol-Ene Photo-Click Hydrogels and Their Impact on Regenerative Medicine
- Unlocking Efficiency with Distribution-Free One-Pass Learning in Online Machine Learning
- Revisiting J. Willard Gibbs: Misreadings in Statistical Mechanics and Their Implications
- Entries feed
- Comments feed
- WordPress.org
© 2024 Christophe Garon — Powered by WordPress
Theme by Anders Noren — Up ↑
Making game theory work for managers
In times of uncertainty, game theory should come to the forefront as a strategic tool, for it offers perspectives on how players might act under various circumstances, as well as other kinds of valuable information for making decisions. Yet many managers are wary of game theory, suspecting that it’s more theoretical than practical. When they do employ this discipline, it’s often misused to provide a single, overly precise answer to complex problems.
Our work on European passenger rail deregulation and other business issues shows that game theory can provide timely guidance to managers as they tackle difficult and, sometimes, unprecedented situations. The key is to use the discipline to develop a range of outcomes based on decisions by reasonable actors and to present the advantages and disadvantages of each option. Our model shifts game theory from a tool that generates a specific answer to a technique for giving informed support to managerial decisions.
Several factors in today’s economic environment should propel game theory to a prominent place in corporate strategy. The global downturn and uncertain recovery, of course, have prompted radical shifts in demand, industrial capacity, and market prices. Some companies, emboldened by the crisis, have tried to steal market share. New global competitors from emerging economies, particularly China and India, are disturbing the established industrial order. They use new technologies and business models and even have novel corporate objectives, often with longer-term horizons for achieving success.
These uncertainties can paralyze corporate decision making or, perhaps worse, compel managers to base their actions on gut feelings and little else. Game theory can revitalize and contribute clear information to decision making—but only if its users choose a set of inputs detailed enough to make the exercise practical and analyze a range of probable scenarios.
Decades old—and misunderstood
Game theory as a management tool has been around for more than 50 years. Today, most university business students are introduced to the idea through the classic “prisoner’s dilemma.” This and similar exercises have instilled the idea that game theory generates a single solution representing the best outcome for reasonable players.
In academic settings, game theory focuses on logically deriving predictions of behavior that are rational for all players and seem likely to occur. It does so by seeking some form of equilibrium, or balance, based on a specific set of assumptions: the prisoners aren’t aware of each other’s actions, can give only one answer, and so on.
But the real world is messier than the neat environment of the prisoner’s dilemma, and game theory loses some traction when faced with practical, dynamically evolving business problems. Companies using this approach often fail to strike the right balance between simplifying a problem to make it manageable and retaining enough complexity to make it relevant. In addition, decision makers often get a single proposed solution without understanding clearly the assumptions that went into its formulation. This problem is especially troublesome because solutions that seek a universal equilibrium among players in a sequence are sensitive to the initial conditions presented and to the assumptions used in deriving an answer.
We have developed a model that addresses these objections. Instead of predicting a single outcome, with all factors balanced, the model first generates a narrow set of strategic options that can be adjusted to account for changes in various assumptions. Instead of solving an individual game, the model automatically involves a sequence of several games, allowing players to adjust their actions after each of them, and finds the best path for different combinations of factors. As one result, it supports executive decisions realistically by presenting managers with the advantages and disadvantages of the strategic options that remain at each stage of the progression. In a second step, the model finds the “best robust option,” considering its upside potential and downside risks under all likely scenarios, assumptions, and sensitivities as time elapses. This approach is different from attempts to look for equilibrium in an artificially simplified world.
Let’s say, for example, that two companies in the global machinery market face an attacker from China planning to open its own multipurpose factory. Depending on myriad assumptions about cost structures, customer demand, market growth, and other factors, the best strategy in one scenario could be for the incumbents to cut prices. In a second scenario, using slightly different assumptions, it could be best to wait until the entrant acts and then to secure the greatest value by reacting appropriately.
Traditional game theory delivers the best answers and equilibriums, which could be completely different for each scenario. Then it tries to predict the most likely scenario. But you can’t analyze uncertainty away, and the traditional approach actually offers management a series of “snapshots,” not a recommendation based on the overall picture. Our model, in contrast, examines how assumptions and actions might change and looks at possible gains and losses for each player in a dynamic world. In the example of the machinery companies, the best robust option could be to leave room for the entrant in a particular niche, where the incumbents are weakest and there’s little risk that the entrant could expand into other segments.
Our model seeks to balance simplicity and relevance by considering a likely set of actions and their effect on important metrics such as demand and profit. Experience and an understanding of the various actors’ sensitivities to different situations guide the analysis. By considering only the most relevant factors, the model manages complexity and, at the same time, creates transparency around important break points for the key drivers. One such break point could be how strongly the market must react to an attacker’s move before an incumbent’s best strategy shifts from coexistence to counterattack.
The best way to understand the model is to examine it in action.
Game theory and European rail
After years of debate and delay, the deregulation of passenger railways in the European Union appears to be gaining momentum. Cross-border passenger service is to be fully open to competition from January 2010. Some member states, including Germany, Italy, Sweden, and the United Kingdom, have taken the initiative and begun opening domestic long-distance passenger rail service to competition, as well.
The experience of other deregulated industries provides rail operators with some lessons, such as the futility of price wars, which generally destroy an industry’s profitability. But the unique characteristics of rail make it exceptionally difficult to predict how competition will alter the playing field. In passenger rail service, for instance, network effects are prevalent, as routes connecting passengers to numerous cities and towns tend to be highly interdependent.
Certainly, new entrants will try to skim off some of the most profitable point-to-point routes. Despite significant upfront capital expenditures, these challengers will probably try to use lower operating costs to undercut the incumbents’ fares. Beyond that, it remains to be seen how and where the attackers will attack and how incumbents will defend themselves.
Besides mutually destructive price wars, what options do the incumbents have? Should they rewrite their schedules to compete with the attackers’ timetables head-to-head? Would it make sense for them to emphasize their superior service or to compete on price by stripping away frills? Should they concede some minor routes to the new entrants in hopes of limiting the damage or fight for every passenger?
To address these questions, the model we developed uses game theory to understand the dynamics of the emerging competition in long-haul passenger rail routes. It breaks down the complex competitive dynamics into a set of sequential games in which an attacker makes a move and an incumbent responds.
From the perspective of the attackers, the range of options available can be distilled into four main choices. The attackers could imitate the incumbents by providing similar or identical service. They could go on the offensive with a more attractive service—for instance, one that is cheaper or more frequent. They could specialize by offering a niche service, probably only at peak hours, that isn’t intended to compete with the incumbents across the schedule. Finally, they could differentiate by providing a clearly distinctive service, such as a low-cost offer focused on leisure travelers, with suitable timetables and less expensive, slower rolling stock.
Likewise, the range of responses available to incumbents on each route under challenge can be broken down to their essence: to ignore the attackers by not reacting at all; to counterattack by contesting the entry through changes in price, frequency of service, and schedules; to coexist by ceding some routes and learning to share them; or to exit a route by stopping service on it.
These initial steps in setting up a game theory model are straightforward. The crucial element is to create a list that is both exhaustive and manageable. But the world is dynamic, and the payoffs for each player depend heavily on the details. Four factors, which must also be included in the rail model, can significantly affect the outcome.
Total changes in demand. What will happen to demand with each move by an attacker and response by an incumbent? When offered a broader, more comprehensive choice of rail links, passengers could change their behavior—for instance, travelling by train instead of car or plane.
Cost differences. New players typically have significantly lower operating costs than incumbents, which, however, generally enjoy economies of scale. But a higher degree of complexity and public-service obligations, such as maintaining uneconomical routes, often negate this advantage.
Network advantages. Incumbents almost always have a network advantage, since attackers rarely replicate an incumbent’s entire system. (Many routes, intrinsically unprofitable by themselves, are valuable only as feeders to the larger network.) Passengers generally prefer seamless connections—a preference that plays to the incumbents’ strengths, especially to and from points beyond the major routes.
Price sensitivity. Attackers typically charge lower fares, and the degree of difference needed for passengers to switch lines or modes of transport (from cars to trains, for instance) is critical to the outcome.
In the common approach to game theory, analysts look at dozens of permutations of actions and reactions, choosing those they feel are consistent and mutually balanced, as well as most likely to occur. Then they make assumptions about these or other factors. The result is a solution, with one particular set of assumptions, derived from all the interests of all the players. The solution could, for instance, be to fight the new entrant tooth and nail on all fronts.
But in looking at the problem, we found several conditions in which the players’ interests could be seen as consistent and mutually balanced. Just as interesting, the results were sensitive to our initial assumptions: in other words, when we slightly modified an assumption about, say, changes in demand, the results would be very different. From this perspective, our model resembles a business simulator, allowing executives to get a clear understanding of the likely evolution of competition under differing conditions. It helps companies to generate the best option as the moves of competitors become clear.
The outcome of the rail analysis
What did the model say about European passenger rail?
Consider, first, one set of conditions. In this scenario, the incumbent operates a fairly large network and has enjoyed monopoly advantages—in particular, relatively high profits. But because of the monopoly legacy, the incumbent suffers from operational inefficiencies and a sizeable cost base. Overall demand is elastic: customers are likely to travel more by rail if service improves and quite likely to accept low-price offers. A new company with a substantially lower cost base considers cherry-picking a few of the more attractive routes by offering improved service.
This model suggests that although the attacker enjoys lower costs and seems to have a favorable starting position, it will probably take only a sliver of market share, and that thanks largely to a general increase in rail use. The incumbent will remain dominant. Seeing the likely outcome of the attacker’s specialized or niche entry, the incumbent’s executives should conclude that a strategy of tolerance would be best. Only a small share of the market is at stake, and the incumbent could lose much more if it engaged in a costly battle for this sliver—for instance, by waging a destructive price war or using other expensive tactics. If the attacker is more aggressive, the incumbent’s best answer would be to fight back with tactics including aggressive price competition, targeted marketing activities, and more frequent and better service on the routes under attack. Note, however, that this would substantially lower profits for both players.
To cover the full range of possibilities, the model can manipulate each variable. Under certain circumstances (if the demand reaction is muted, the incumbent’s cost disadvantage high, and its network advantage small) entrants have the inside track and could probably take control of the market. When circumstances favor the incumbent a little more (because its network advantage is stronger or its cost disadvantage smaller) it will probably have strong incentives to lower prices preemptively to prevent a possible attacker’s entry. If conditions are more ambiguous, the incumbent may have to settle for coexistence, although it can probably retain market leadership. The attacker’s share of the industry’s profits would vary significantly, depending mainly on the incumbent’s network advantage (Exhibit 1).
Three scenarios

When we run the European passenger rail model through an array of different situations, a critical factor appears to be the way demand reacts to liberalization. Will the new offerings seduce travelers to take trains rather than cars or jetliners, or will overall demand remain stagnant, leaving rail companies to battle for an unchanged pool of customers (Exhibit 2)?
The influence of pricing

If the attacker’s entry doesn’t stimulate demand, two operators cannot profitably share most routes: high fixed costs make many of them natural monopolies supporting only a certain level of capacity. A weak incumbent—for instance, one with major cost disadvantages or few network benefits—could be squeezed out by an agile attacker. A strong incumbent could cut fares before the attacker committed itself to any investment, dissuading it from making the challenge. In the end, the competitors will face a winner-takes-all situation, with only one left in the market.
When rail demand can be stimulated, players will probably coexist profitably. But the model suggests that even when the attacker enjoys the best conditions, the incumbent is likely to retain market leadership. Reasonable attackers will have an incentive to enter only on a small scale that the incumbent can usually tolerate. More aggressive moves from either side would trigger ruinous price wars or service expansions, destroying the industry’s overall profitability.
Finally, at each moment, incumbents almost always have one best robust option that conserves much more of their profits than any other course. Quite often, deviating from that option reduces the entire industry’s profits significantly. But unlike a solution based on traditional game theory—a solution optimal only for a single precisely defined future—our model generates an answer that represents the best compromise between risks and opportunities across all likely futures. Unlike the answers suggested by traditional game theory, this one does not require all competitors to behave according to a narrowly defined rational equilibrium at each moment. The transparency of our approach helps executives understand the break points of a strategy: how much reality must differ from its assumptions before a new strategy is needed.
Although we focus here on European passenger rail, our model shows how game theory can be applied to many complex environments and produce results informing many strategic decisions. We’ve applied the model to other problems, with similarly enlightening results. In health care, for example, we examined the dynamics of the commoditization of certain drugs—in particular, after Asian manufacturers offered higher-quality versions of them. We also looked at the strategic options of companies in the chemical industry in the wake of recent overcapacity and reduced demand. Game theory is a powerful framework that enables managers to analyze systematically the ties among interactions between actors in a market and to develop appropriate competitive strategies. But it’s helpful only if executives expect a tool that helps them make informed decisions based on a range of market actions by each player, not a single answer that solves the whole riddle.
Hagen Lindstädt is the head of the Institute for Management at Karlsruhe University, and Jürgen Müller is a principal in McKinsey’s Stockholm office.
Explore a career with us
Related articles.

Getting into your competitor’s head
Prisoner's Dilemma: A Case Study
- This person is not on ResearchGate, or hasn't claimed this research yet.
Abstract and Figures

Discover the world's research
- 25+ million members
- 160+ million publication pages
- 2.3+ billion citations

- Christos H. Papadimitriou

- Robert Axelrod

- Douglas Dion

- Charles A. Holt
- Watson Joel
- Shoham Leyton-Brown Kevin
- Shoham Yoav
- Leyton-Brown Kevin
- Recruit researchers
- Join for free
- Login Email Tip: Most researchers use their institutional email address as their ResearchGate login Password Forgot password? Keep me logged in Log in or Continue with Google Welcome back! Please log in. Email · Hint Tip: Most researchers use their institutional email address as their ResearchGate login Password Forgot password? Keep me logged in Log in or Continue with Google No account? Sign up
- Utility Menu

Using Game Theory in Real Time in the Real World: A Conservation Case Study (Demonstration)
5.15 MB |
Publications: Years
Recent publications.
- Optimization and Planning of Limited Resources for Assisting Non-Profits in Improving Maternal and Child Health
- Case Study: Applying Decision Focused Learning inthe RealWorld
- Decision-Focused Learning without Differentiable Optimization: Learning Locally Optimized Decision Losses
- Combining Machine Learning and Cognitive Models for Adaptive Phishing Training
- Restless and Uncertain: Robust Policies for Restless Bandits via Deep Multi-Agent Reinforcement Learning
- Solving Structured Hierarchical Games Using Differential Backward Induction
- Translating AI to Impact: Uncertainty and Human-Agent Interactions in Multi-Agent Systems for Public Health and Conservation
- Micronutrient Deficiency Prediction via Publicly Available Satellite Data
Thank you for visiting nature.com. You are using a browser version with limited support for CSS. To obtain the best experience, we recommend you use a more up to date browser (or turn off compatibility mode in Internet Explorer). In the meantime, to ensure continued support, we are displaying the site without styles and JavaScript.
- View all journals
- Explore content
- About the journal
- Publish with us
- Sign up for alerts
- Open access
- Published: 03 December 2019
Game theoretical inference of human behavior in social networks
- Nicolò Pagan ORCID: orcid.org/0000-0003-0071-7856 1 &
- Florian Dörfler ORCID: orcid.org/0000-0002-9649-5305 1
Nature Communications volume 10 , Article number: 5507 ( 2019 ) Cite this article
11k Accesses
12 Citations
13 Altmetric
Metrics details
- Decision making
Social networks emerge as a result of actors’ linking decisions. We propose a game-theoretical model of socio-strategic network formation on directed weighted graphs, in which every actors’ benefit is a parametric trade-off between centrality measure, brokerage opportunities, clustering coefficient, and sociological network patterns. We use two different stability definitions to infer individual behavior of homogeneous, rational agents from network structure, and to quantify the impact of cooperation. Our theoretical analysis confirms results known for specific network motifs studied previously in isolation, yet enables us to precisely quantify the trade-offs in the space of user preferences. To deal with complex networks of heterogeneous and irrational actors, we construct a statistical behavior estimation method using Nash equilibrium conditions. We provide evidence that our results are consistent with empirical, historical, and sociological observations on real-world data-sets. Furthermore, our method offers sociological and strategic interpretations of random networks models, such as preferential attachment and small-world networks.
Similar content being viewed by others
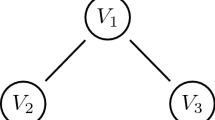
An entropy-based framework to analyze structural power and power alliances in social networks
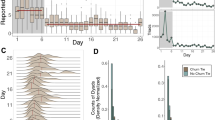
Dynamics of social network emergence explain network evolution
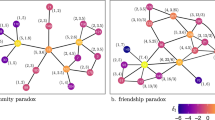
The enmity paradox
Introduction.
There has been a growing interdisciplinary interest in the study of social networks over the past few decades. Especially since the diffusion of on-line platforms, e.g., Twitter or Instagram, there is evidence that both sociological and strategic behavior play an important role 1 . Thus, it became important to understand how networks are formed 2 and especially how networks affect actors’ behavior and vice versa.
Starting from the random graph model proposed by Erdös and Rényi 3 , 4 , the complex networks community developed a number of network formation models driven by sociological observations and supported by empirical evidence. Among them, the small-world network model introduced by Watts and Strogatz 5 shows that the addition of few random ties to a regular lattice (highly locally connected) results into a small diameter network, as in Milgram’s experiment 6 on the six degrees of separation. To explain the emergence of scaling in random networks, Barábasi and Albert proposed the preferential attachment model 7 , in which newborn nodes select their connections proportional to popularity. A broad literature on complex (social) networks and dynamics thereof has grown ever since (see refs. 8 , 9 , 10 ). While such probabilistic models can successfully reproduce the macroscopic statistical structural properties of social networks, they do not offer insights into the sociological microscopic foundations.
One such socio-theoretical and statistical approach was proposed by Snijders 11 with the Stochastic Actor-Oriented Models (SAOM). They consider observed networks as the result of the actors’ linking behavior 12 assuming that social actors can change their outgoing ties. The payoff function that each actor tries to maximize is split into a modeled and a random component, with the former containing statistical parameters that can be estimated from available data through likelihood-based methods 13 . The modeled component is assumed to be a linear combination of effects, e.g., reciprocity, transitivity, or the tendency of having ties at all 13 . Similarly to SAOM, Exponential Random Graph Models (ERGM) 14 study network configurations, which are small subsets of possible network ties (and/or actors’ attributes), e.g., reciprocated ties 15 . Yet, the focus is on ties rather than on actors.
In the economics community, a plausible and widely supported belief is that actors strategically choose their relations to optimize their network positions in an incentive-guided fashion 16 . Similarly to SAOM, strategic network formation models assume that actors aim at maximizing payoff functions that depend on their position in the network and on the topology. The objective is to explain why certain network architectures emerge when actors strive for centrality, while links are costly. The literature on this topic is broad 17 , 18 , 19 , 20 , 21 , 22 , 23 , 24 , 25 , 26 , 27 (see refs. 28 , 29 , 30 , 31 for extensive surveys), yet there is no common agreement on the specific centrality metrics 32 . Among the seminal works on strategic network formation, Bala and Goyal 18 use degree centrality, while the connections model introduced by Jackson and Wolinsky 17 is related to closeness centrality, as shown in ref. 22 . Others 19 , 20 , 21 propose models where actors strive for structural holes, which are missing connections between certain pairs of agents, thus brokerage opportunities. Burt showed that his brokerage constraint measure, defined in ref. 33 , is tightly related to betweenness centrality 34 . According to Coleman 35 , triangulated structures provide cohesive support to the agents. Davis 36 also showed empirically that transitivity, often termed network (or triadic) closure or clustering 15 , 37 , is a prevalent effect in many human social networks as the result of social selection based on, e.g., homophily 38 .
Depending on the choice of payoff function, different models evince a relation between centrality metrics and the stability of specific network architectures. For instance, refs. 20 , 21 show that when actors strive for betwenness centrality, balanced complete bipartite (or more generally multipartite) networks are stable. Conversely, closeness centrality incentives lead to star-like architectures 22 , and complete networks are stable when closed triads are beneficial 23 . The main limitation of these models lies in the isolation of specific centrality metrics and network motifs, which prevents from a comprehensive analysis of the network topology stability with respect to multiple co-existing incentives. Some preliminary attempts in overcoming this limitation can be found in ref. 23 , where the authors compare the approaches based on Coleman and Burt’s theories and experimentally show that the spectrum of stable networks effectively depends on the trade-off between social support and brokerage; and in ref. 24 where betweennes and closeness centralities are simultaneously investigated.
Besides adopting different payoff functions, the literature on strategic network formation exhibits a variety of assumptions: most works consider unweighted and undirected networks, though some variations have been studied (see ref. 25 for a directed version of the connections model, and see ref. 26 for weighted graphs). Yet, while some models rely on agents having perfect information of the network, an active line of research investigates more realistic incomplete information scenarios, e.g., ref. 39 .
In this work, we propose a socio-strategic network formation model whose payoff function is characterized by a parametric combination of a locally assessable Katz centrality 40 and a clustering coefficient. This allows us to represent a wide range of strategic actors’ incentives, from indegree to closeness centrality, or from clustering coefficients to betweenness-type centrality. Moreover, we capture the significant difference between follower and followee using directed networks. Despite having received little attention in the strategic network formation literature, they allow us to emphasize the socio-theoretical interpretation of our payoff function in terms of the effects studied in SAOM.
Our first objective is to theoretically study the relation between homogeneous actors’ behavior and stable network motifs. We do so by means of two different notions of stability: Nash and pairwise-Nash equilibrium. With our parametric model and our analytical proof methods we not only confirm phenomena previously observed in isolation, but we also provide a comprehensive quantitative analysis and we discover transition paradigms in the space of individuals’ preferences.
Secondly, by means of the socio-theoretical interpretation of our payoff function, we propose a statistical method, based on Nash equilibrium conditions. The method enables us to perform individual behavior estimation on real-world networks of heterogeneous and not necessarily rational agents. We validate our predictions on the Medici’s strategic behavior in Renaissance Florence 41 and on the hierarchical network of confiding relationships within an Australian bank 42 .
Third and finally, we show that our behavior estimation method can shed light on a sociological and strategic interpretation of complex random networks models.
Network formation problem setup
Let \({\mathcal{N}}=\left\{1,\ldots ,N\right\}\) be a finite set of actors or agents (with \(N\ge 3\) to avoid trivial cases). Depending on the application, an actor may be a human being, a firm, a country, or some other autonomous entity. Agents are endowed with a payoff function, and they are assumed to be rational, thus they aim at maximizing it. They do so in a myopic fashion, i.e., without anticipating others’ potential reaction. The network relations among these agents are formally represented by weighted and directed graphs \({\mathcal{G}}\) without self-loops, whose nodes are identified with the set \({\mathcal{N}}\) of agents and whose arcs weights \({a}_{ij}\) and \({a}_{ji}\) , in the range between \(0\) and \(1\) , denote the strength of the directed relations among agents \(i\) and \(j\) .
We assume that each agent of the network has control on the weights of her outgoing links, while she cannot affect her incoming links. In other words, she can decide her followees but not her followers. In game-theoretical language, a typical action of agent \(i\) can be expressed by
living in the action space \({\mathcal{A}}={\left[0,1\right]}^{N-1}\) . Conversely, we denote a typical action of all agents but \(i\) as
Payoff function
In a directed network setting, perhaps the simplest measure of centrality is the indegree centrality (e.g., number of followers), which is based on the sum of incoming ties (see Fig. 1 a). Formally, the indegree centrality of agent \(i\) is measured as \({\sum }_{k}{a}_{ki}.\) In the connections model by Jackson and Wolinksy 17 benefits also come from indirect connections, given by \({\delta }^{t}v\) , where \(t\) is the length of the shortest path connecting two agents, \(v\) is a fixed parameter, and \(\delta \in (0,1)\) is a decay factor. Similarly, we use \({\delta }_{i}\in [0,1]\) and define
as a measure of the influence of agent \(i\) in the network. Such a measure extends the indegree centrality definition (which can be recovered by setting \({\delta }_{i}=0\) ) by introducing the contribution of the strength of all weighted paths of length \(2\) and \(3\) which are ending in \(i\) , discounted with factors \({\delta }_{i}\) and \({\delta }_{i}^{2}\) , as shown in Fig. 1 b. This measure can also be viewed as an approximated Katz centrality. In the original definition, Katz 40 considers paths of all lengths, yet in real-world social networks agents have limited information on the network topology (one can think of Linkedin’s 3rd degree of separation). Compared to that, our definition is locally assessable, i.e., it does not require complete information of the entire network, yet it includes most important social networks patterns, such as diads and triads 15 .
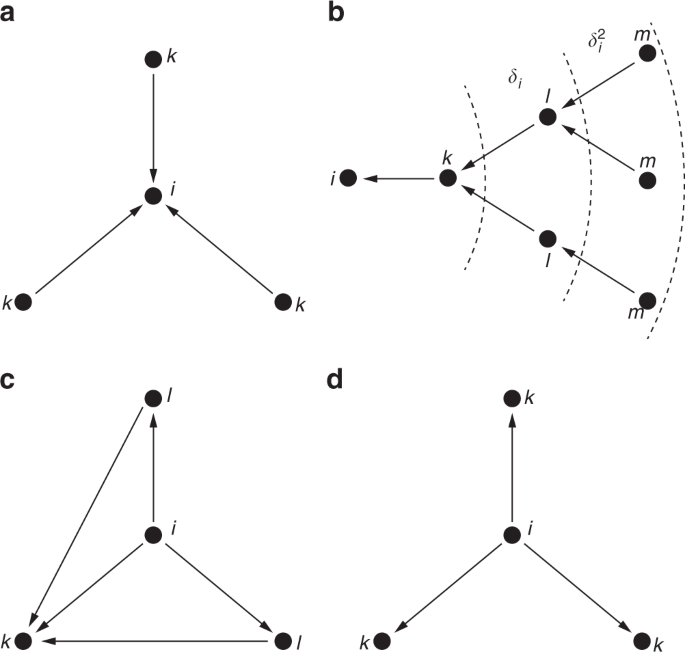
Sketch of the payoff function’s contributions. a The indegree centrality of agent \(i\) is determined by the sum of all the incoming ties. b The influence of agent \(i\) is computed summing up the weighted paths to the node \(i\) , discounted by \(1\) , \({\delta }_{i}\) , \({\delta }_{i}^{2}\) . c The clustering coefficient of agent \(i\) depends on the weighted directed closed triads that surround node \(i\) . d The cost to agent \(i\) corresponds to the outdegree of node \(i\) .
As discussed in the introduction, agents may privilege social support. Formalized in the network settings, agents benefit from being surrounded by closed triads, or in other words when a friend of a friend is a friend 43 . In graph theory, the mean probability that two nodes, which are network neighbors of the same other node, will themselves be neighbors is referred as clustering coefficient 5 . Albeit it might be hard for agents to compute such a probability, they can estimate, for each friend \(k\) , the number of common friends \(l\) . Similarly to the approach in ref. 23 , we define the clustering of agent \(i\) as
as depicted in Fig. 1 c, meaning that the friendship from \(i\) to \(k\) is more valuable the higher the number of common friends \(l\) between \(i\) and \(k\) . Finally, as typically done in the strategic network formation literature, e.g., refs. 17 , 21 , 24 , we model the cost to agent \(i\) as the effort required for agent \(i\) to maintain the link towards the other agents \(k\) , thus proportionally to agent \(i\) ’s outgoing ties \({a}_{ik}\) . The cost, illustrated in Fig. 1 d, results in
Alternatively, as a minor variation of the model, a quadratic cost function as in ref. 23 can be used to model ties with non-constant marginal costs reflecting the fact agents have to divide their attention over all their relationships.
In summary, we assume every agent \(i\in {\mathcal{N}}\) is endowed with a parametric payoff function \({V}_{i}\) which depends on \({a}_{i}\) , the action of agent \(i\) , and on \({{\bf{a}}}_{-i}\) , the action of all other agents:
The individual set of parameters \({P}_{i}=\left\{{\alpha }_{i},{\beta }_{i},{\gamma }_{i},{\delta }_{i}\right\}\) is composed by real numbers, with \({\alpha }_{i}\ge 0\) , \({\gamma }_{i} \,\, > \,\, 0\) , and \({\delta }_{i}\in [0,1]\) , which allow payoff tuning according to the actor’s preferences. For instance, large \({\alpha }_{i}\) and \({\delta }_{i}\) make the influence measure more valuable, while higher values of \({\gamma }_{i}\) increase the cost of maintaining links. Note that \({\beta }_{i}\) can take negative values. In this case, the clustering coefficient \({u}_{i}\) acts as a cost. Drawing inspiration from ref. 23 , this enables us to measure the absence of direct brokerage opportunities and to model a number of contexts in which agents prefer ties with unconnected others, as in Burt’s theory of structural holes 33 . Albeit this cost does not correspond to the original constraint measure constructed by Burt, it preserves the underlying intuition that agents are more constrained by their network if they have many redundant contacts. Hence, negative values of \({\beta }_{i}\) are aligned with Burt’s theory, and thus with betweenness centrality, whereas positive values support transitivity and network closure, according to Coleman’s theory.
Socio-theoretical interpretation
Although our payoff function emerges as a parametric generalization of previous models in the network formation literature, a link to network dynamics models from social science theory, e.g., SAOM, can be established. If we focus on the extended indegree centrality measure \({t}_{i}({{\bf{a}}}_{i},{{\bf{a}}}_{-i},{\delta }_{i})\) , by isolating agent \(i\) ’s contribution we obtain the following expression (the derivation can be found in the proof of Theorem 3 in Supplementary Note 1 )
where \({f}_{i}({{\bf{a}}}_{-i},{\delta }_{i})\) denotes the contribution which does not depend on \(i\) ’s action. In other words, the extended indegree centrality measure includes, among others, reciprocal and three-cycle structures, denoted, respectively, as \({\rm{rec}}({{\bf{a}}}_{i},{{\bf{a}}}_{-i})\) and \({\rm{cycles}}({{\bf{a}}}_{i},{{\bf{a}}}_{-i})\) , and sketched in Fig. 2 a, b.
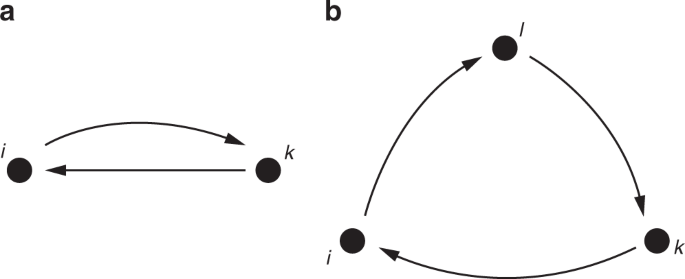
Sketch of reciprocity and cycles. a The reciprocity of agent \(i\) is determined by the sum of the reciprocated ties. b The cycles of agent \(i\) are computed summing up all the length 3 cycles that starts and ends in node \(i\) .
Thus, the payoff function can be conveniently re-written in the following alternative formulation:
where the dependency on basic sociological effects (as in SAOM) or configurations (ERGM) has been highlighted.
In game-theoretic modeling of strategic network formation, two stability notions are typically considered: Nash equilibrium and pairwise stability. The former is based on the idea that agents act purely selfishly: the network is stable when no agent can be better off by unilaterally deviating from her equilibrium strategy. In the latter, a network is stable when no pair of agents can coordinate in order to be both better off. Both notions have been extensively used in strategic network formation analysis. Among the pioneering works, Jackson and Wolinsky 17 and Bala and Goyal 18 used, respectively, pairwise stability and Nash equilibrium.
Following these two classes, we define two types of stability. First, we model purely selfish actors through the Nash equilibrium notion. This is a reasonable approach in many competitive contexts or marketing environments, e.g., when agents strategically retweet or choose their Instagram followees. The formal definition is as follows.
Definition . \({{\mathcal{G}}}^{\star }\) is a Nash equilibrium (NE) if
C 1 . for all agents \(i\) , \({V}_{i}\left({{\bf{a}}}_{i},{{\bf{a}}}_{-i}^{\star }\right)\le {V}_{i}\left({{\bf{a}}}_{i}^{\star },{{\bf{a}}}_{-i}^{\star }\right),\ \forall {{\bf{a}}}_{i}\in {\mathcal{A}}\) .
Note that agents are allowed to play any action in the space \({\mathcal{A}}\) , i.e., to simultaneously change all the outgoing ties.
Second, we propose the pairwise-Nash stability definition, which combines the selfish attitude with the possibility of coordination among agents, and thus is more suitable to model situations where actors are open to cooperation, while also being naturally selfish. Indeed, in many social and economic networks, it is not uncommon to observe cooperation, and previous works (see refs. 44 , 45 ) already made use of pairwise-Nash equilibrium, also referred as Bilateral equilibrium (see ref. 19 ). Practically, agents are allowed to deviate alone or by pairs. Thus, instead of considering every agent singularly, we consider meetings. In a meeting between \(i\) and \(j\) , agent \(i\) can only revise the tie \({a}_{ij}\) , while, simultaneously, \(j\) can modify \({a}_{ji}\) . For every pair of distinct agents \((i,j)\in {\mathcal{N}}\times {\mathcal{N}}\) , let the meeting action be the pair \(\left({a}_{ij},{a}_{ji}\right)\in {\left[0,1\right]}^{2}\) . On the other hand, we denote the action of \(i\) without the link \({a}_{ij}\) as
Further, let all the actions but the meeting pair \(({a}_{ij},{a}_{ji})\) be
Definition . \({{\mathcal{G}}}^{\star }\) is a pairwise-Nash equilibrium (PNE) if
C 2 . for all pairs of agents \((i,j)\) ,
C 3 . for all pairs \((i,j)\) , and for all pairs \(\left({a}_{ij},{a}_{ji}\right)\) in \({\left[0,1\right]}^{2}\) ,
In the above definition, (C2) is the Nash, or selfish, condition which requires that, for every pair \((i,j)\) of agents, neither \(i\) nor \(j\) can be selfishly better off by changing just her individual outgoing tie. (C2) is similar to (C1). However, (C1) implies (C2) but not vice versa, due to the different action spaces; see Remark 2 and Supplementary Fig. 1 in Supplementary Note 1 . On the other hand, (C3) is the cooperative condition, stating that at equilibrium there is no pair of agents who can both be better off by coordinating their actions. Note that (C3) is a Pareto optimality condition (see the Supplementary Note 1 ).
Network motifs analysis
Our results are twofold: in the first analytic part, we initially assume agents being homogeneous ( \({P}_{i}=P,\ \forall i\in {\mathcal{N}}\) ). Thus players’ characteristics other than their connections are neglected, and we present a stability analysis in the space of parameters \(P=\left\{\alpha ,\beta ,\gamma ,\delta \right\}\) , of four prototypical network motifs. For each network topology, we analytically derive necessary and sufficient conditions which guarantee NE and PNE. Analytical tools are discussed in the Methods section and in Supplementary Note 1 , the formal proofs are available in Supplementary Note 2 . In the second part, we consider complex random network models as well as real-world networks, and we assume that actors have heterogeneous (and possibly irrational) behavior: some might be more willing to create close triads to receive social support, whereas others might prefer to build structural bridges in order to get competitive advantages. We then use the Nash stability condition as a quantitative tool to infer the individual preferences \({P}_{i}\) .
In what follows, we first present the equilibrium analysis of the prototypical network motifs depicted in Fig. 3 . The first case is the empty network, i.e., a graph \({{\mathcal{G}}}^{EN}\) of \(N\) nodes such that for every pair \((i,j)\) of agents, \({a}_{ij}={a}_{ji}=0\) . Given this particular topology, the equilibrium condition must guarantee that no links are initiated.
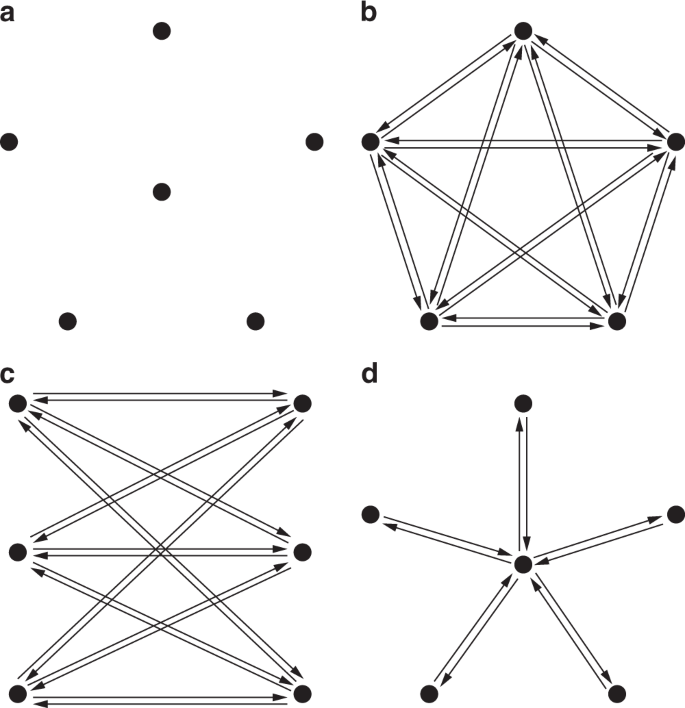
Sketch of the prototypical network motifs studied here. a Empty, b Complete, c Balanced Complete Bipartite, and d Star Network. All links shown have unitary weight.
Theorem . Let \({{\mathcal{G}}}^{EN}\) be an empty network . Then
\({{\mathcal{G}}}^{EN}\) is always a NE ,
\({{\mathcal{G}}}^{EN}\) is a PNE if and only if \(\gamma \ge \alpha \left(1+\delta +{\delta }^{2}\right)\) .
Effectively, when no links are present in the graph, no agent has a selfish incentive to create outgoing ties as she only incurs costs. Thus, the empty network is always a NE. However, when dealing with PNE, cooperation may take place. More precisely, \(\gamma \ge \alpha \left(1+\delta +{\delta }^{2}\right)\) is the necessary (and sufficient) condition for PNE. For otherwise, the cost parameter is cheap enough that agents would initiate reciprocate ties. In summary, in a stable empty network, either agents are non-cooperative, or creating ties is too costly. Figure 4 a shows a comparison of the NE and PNE stability regions of the empty network in the normalized parameter space \(\alpha /\gamma\) versus \(\beta /\gamma\) .
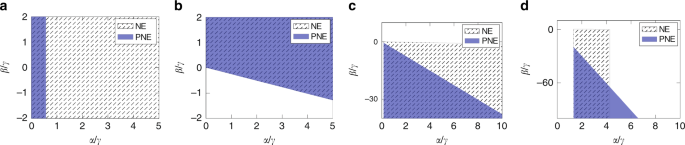
NE and PNE regions of the four network motifs of homogeneous agents. a Empty, b Complete, c Balanced Complete Bipartite, d Star Network. All the plots refer to \(N=50\) agents and \(\delta =0.5\) . Different settings lead to qualitatively similar results.
We then move our analysis to the other extremum: the complete network, i.e., a graph \({{\mathcal{G}}}^{CN}\) of \(N\) nodes such that for every pair \((i,j)\) of distinct agents, \({a}_{ij}={a}_{ji}=1\) . Opposite to the previous case, the equilibrium conditions must guarantee that actors have no incentive on reducing links.
Theorem . Let \({{\mathcal{G}}}^{CN}\) be a complete network . Define
\({{\mathcal{G}}}^{CN}\) is a NE if and only if \(\gamma \le {\bar{\gamma }}_{NE}\) ,
\({{\mathcal{G}}}^{CN}\) is a PNE if and only if \(\gamma \le {\bar{\gamma }}_{PNE}.\)
As one can deduce from the proof (see theorem 8 in Supplementary Note 2 ), both stability conditions require the cost parameter \(\gamma\) to be upper-bounded by the minimal marginal benefit of being linked with everybody else. Concerning the NE, if the ties are too costly ( \({\gamma} \ {> } \ {\bar{\gamma }}_{{\rm{NE}}}\) ), the best action for each agent is to drop all outgoing ties. This transition behavior has dramatic consequences, as it leads to the empty network if agents were simultaneously playing best response. Similar reasoning applies for the PNE, albeit agents cannot drop all their outgoing ties simultaneously.
From the comparison between the cost parameter thresholds, we notice that \({\bar{\gamma }}_{{\rm{NE}}}\le {\bar{\gamma }}_{{\rm{PNE}}}\) with strict inequality if \({\beta} \ {> } \ 0\) . This means that, in some cases, the complete network is pairwise-Nash stable, but it is not Nash stable. Such a discrepancy derives from the different action spaces in the stability notions. However, the comparison in Fig. 4 b shows that such a difference is marginally small. Further discussion and a zoomed plot are available in Supplementary Note 2 and Supplementary Fig. 2 . Concerning the impact of cooperation in the PNE, the Pareto condition (C3) is always satisfied whenever the selfish condition (C2) is fulfilled. Thus, cooperation does not restrict the PNE stability region for this network motif.
Finally, Fig. 4 b evinces that higher values of \(\beta\) incentivizes the stability of the complete network. Even when \(\alpha =0\) , \(\beta \ge \frac{\gamma }{N-2}\) is necessary and sufficient to guarantee NE. A similar condition holds for PNE. We emphasize that this result confirms that complete network stability is correlated with high clustering coefficients, as already shown in refs. 23 and 24 . Yet, it reveals that complete networks are stable even if agents strive for betweenness centrality ( \({\beta} \ {<} \ 0\) ), provided that they also have a closeness-type incentive ( \(\alpha /\gamma\) has to be high enough). In other words, it shows that in a stable complete network, ties are relatively cheap and individuals are typically looking for local support, confirming the theory of Coleman 35 , as well as trying to improve their social influence.
Next, we consider an interesting class of topologies: the bipartite networks, which are graphs in which the nodes can be partitioned into two factions such that \({a}_{ij}=0\) when \(i\) and \(j\) belong to the same partition. We first analyze the balanced complete bipartite network, where the two factions are of size \(N/2\) , thus perfectly balanced in the number of nodes, and \({a}_{ij}=1\) , for all pairs \((i,j)\) of nodes belonging to different partitions (complete). Bipartite networks are interesting case studies, as their equilibrium requires two conditions: (i) existing links across the two partitions must not be dropped, (ii) ties within the same partition must not be created.
Theorem . Let \({{\mathcal{G}}}^{BN}\) be a balanced complete bipartite network of \(N\) agents , with \(N\) being even . Define
\({{\mathcal{G}}}^{BN}\) is a NE if and only if \({\underline{\gamma }}_{NE}\le \gamma \le {\bar{\gamma }}_{NE},\)
\({{\mathcal{G}}}^{BN}\) is a PNE if and only if \({\underline{\gamma }}_{PNE}\le \gamma \le {\bar{\gamma }}_{PNE}.\)
According to the theorem, \(\gamma \le {\bar{\gamma }}_{{\rm{NE}}}\) guarantees that agents have no incentive on dropping existing links and \(\gamma \ge {\underline{\gamma }}_{{\rm{NE}}}\) ensures that agents creating links within the same partition will incur sufficiently high cost. Analogous conditions hold for PNE. Figure 4 c shows that, regardless of the stability notion, the second effect emerges to be more relevant as it excludes the upper part of the diagram from the stability region. This confirms that balanced complete bipartite network stability is negatively correlated with the clustering coefficient \(\beta\) , hence positively correlated with betweenness centrality, as previously found in ref. 24 . Thus, we can infer that in a stable balanced complete bipartite network actors are competitive in looking for brokerage opportunities and links are relatively cheap as the network is dense. A comparison between NE and PNE stability regions indicates that the balanced complete bipartite network is less likely to be stable when agents can cooperate. As the proof evinces (see Theorem 9 in Supplementary Note 2 ), actors belonging to the same partition may find it beneficial to establish a mutual tie.
We finally analyze the star network, which can be viewed as an unbalanced complete bipartite network.
Theorem . Let \({{\mathcal{G}}}^{SN}\) be a star network . Define
\({{\mathcal{G}}}^{SN}\) is a NE if and only if \({\underline{\gamma }}_{NE}\le \gamma \le {\bar{\gamma }}_{NE},\)
\({{\mathcal{G}}}^{SN}\) is a PNE if and only if \({\underline{\gamma }}_{PNE}\le \gamma \le {\bar{\gamma }}_{PNE}\) .
The proof evinces that equilibrium requires three conditions: (i) the central node must have no incentive in dropping her ties, (ii) the periphery nodes must not destroy the link to the center of the star, and (iii) must not initiate ties among them. However, whenever (ii) is satisfied, (i) follows, thus it reduces to two conditions. As shown in Fig. 4 d, stability requires a lower bound on \(\alpha /\gamma\) and a linear upper bound on \(\beta /\gamma\) with respect to \(\alpha /\gamma\) , which, respectively, guarantee (ii) and (iii). Moreover, in the NE stability region, an additional upper bound on \(\alpha /\gamma\) prevents from being beneficial for a peripheral agent to drop the link to the central one, while simultaneously connecting to all her similars. Such a threshold is not present in the PNE stability region, as deviations are allowed only by pairs of agents. Further discussion is addressed in Supplementary Note 2 and in Supplementary Figs. 3 and 4 .
Ultimately, we discovered that stability of the star network is correlated with high values of the influence parameter \(\alpha\) , and thus with closeness centrality incentives as in ref. 22 . Yet, our proof suggests that the situation is more complex: we found that observing a stable star network indicates that actors are not interested in local social support and that ties are costly as the star is sparser than the balanced complete bipartite.
Phase diagram comparison
So far we have seen the correlation between different incentives and the stability regions of different network architectures. Now we focus on the co-existence of equilibria in the parameter space of actor preferences. Figure 5 a shows that empty, complete, star and balanced bipartite networks are found to overlap in certain regions of the parameter space. Conversely, Fig. 5 b shows that bipartite and complete networks cannot be simultaneously pairwise-Nash stable, due to cooperation. Similarly, the overlap between the pairwise-Nash stability region of complete and empty networks is now restricted mainly in the proximity of the origin of the phase diagram, i.e., where the cost parameter \(\gamma\) is high. Yet, both plots show that the clustering coefficient \(\beta\) draws a significant separation between complete and bipartite networks.
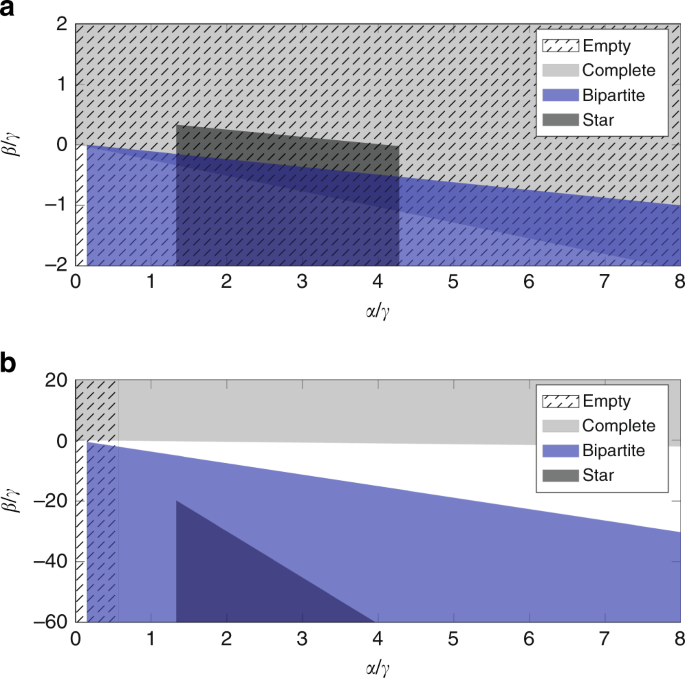
Phase Diagram comparison. a Nash and b Pairwise-Nash equilibrium comparisons of different network motifs of \(N=50\) agents and for \(\delta =0.5\) .
Inference of behavior for complex networks
The theoretical analysis developed so far is elegant and powerful, though restricted to stylized models that appear merely as motifs in empirical networks. In order to be able to analyze complex networks, we need to introduce two elements: (i) agents’ heterogeneity, and (ii) irrationality in the form of an error term. Our goal in this section is to provide the most rational estimate of the heterogeneous individual parameters from an observed state of the network, e.g., a Nash equilibrium. This learning and inference problem can be cast as an inverse optimization problem over candidate objective functions. In econometric and operations research, the common approach is called structural estimation, a method that relies on the existence of a set of necessary (structural) equations for unknown parameters, e.g., first-order optimality conditions for convex problems 46 . Due to the complexity of our structural equations, we developed our own behavior estimation method, borrowing some ideas from ref. 47 . The complete description can be found in the Methods and in Supplementary Note 3 . Below, we summarize the main steps.
Firstly, we use the alternative formulation of the payoff function, introducing a new set of individual parameters
Our choice is motivated by the socio-theoretical interpretation of the influence measure \({t}_{i}\) . We emphasize that the normalization by \({\gamma }_{i}\) is without loss of generality as the equilibria do not change. Moreover, the original set of parameters \({P}_{i}\) can be recovered, though \({\delta }_{i}\) is not necessarily in \([0,1]\) .
Drawing inspiration from the Nikaidô–Hisoda function 48 , we introduce the error function
which takes positive values whenever the preference \({{\boldsymbol{\theta }}}_{i}\) is such that the Nash equilibrium constraint is violated by the action \({{\bf{a}}}_{i}\) of agent \(i\) . Hence, if we consider only the positive contributions \({e}_{i}^{+}({{\bf{a}}}_{i},{{\boldsymbol{\theta }}}_{i}):= \max \left\{0,{e}_{i}({{\bf{a}}}_{i},{{\boldsymbol{\theta }}}_{i})\right\}\) , we can define the average Euclidean distance from the NE conditions as
In our behavior estimation method the goal is to determine the individual set of preferences \(\hat{{{\boldsymbol{\theta }}}_{i}}\) which minimize the distance function \({d}_{i}({{\boldsymbol{\theta }}}_{i})\) . The Minimum NE-Distance problem, which might not have a unique solution, can be solved exactly when there exists a non-empty region \({\Theta }_{i,0}\) in the parameter space where no NE violations occur:
Conversely in case of NE violations (e.g., due to bounded rationality or noisy observations), it can be solved as an Ordinary Least Square (OLS) problem. This allows us to identify an OLS estimate, to unbias it, and to compute confidence intervals.
In the following, we show the applicability of our behavior estimation method to two well-known real-world datasets, as well as two celebrated random networks models.
Medici network
The first example concerns the network of marriage and business connections among Florentine families in the fifteenth century, originally collected by Kent 49 , but first coded by Padgett and Ansell 41 . Unlike more common undirected renditions (see 50 ), we represent this network as a directed multiplex graph consisting of marriage and business ties (see Fig. 6 ), based on the works of refs. 51 and 52 , respectively. A red arc from \(i\) to \(j\) represents a female from family \(i\) married into family \(j\) , whereas blue ties point towards the most prosperous family. We applied our estimation method to three different scenarios: (i) marriage ties network, (ii) business ties network, and (iii) combined network.
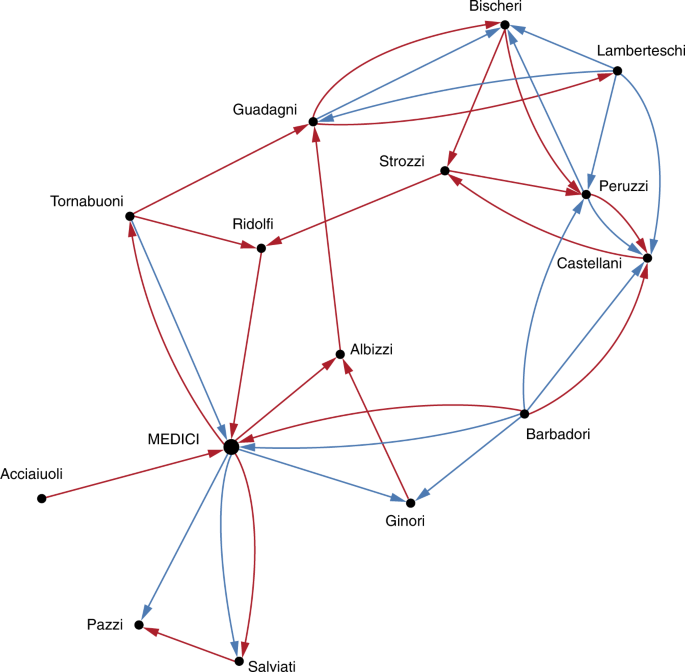
Multiplex graph of marriages (red) and business (blue) relations between 15 Florentine families. The adjacency matrix of the combined network is constructed as the maximum between the adjacency matrices that correspond to the marriage and the business networks.
We focus our analysis on the Medici, who were able to rise in power, even though Florence was previously ruled by an oligarchy of elite families. According to Padgett and Ansell 41 , a key to understanding this lies in the structure of social network relationships. The Medici gradually but surely exploited the structural holes within the oligarchic marriage network, and enabled structural isolation among Medici partisans, deterring them from marrying or having business with the oligarchs. Yet, Padgett and Ansell pointed out that the Medici party was a very centralized system consisted almost entirely of direct ties to the Medici family, and that, within their own party, the Medici did not marry those families with whom they engaged in economic relations, nor did they do business with those whom they married.
In order to understand the structural isolation operated by the Medici family, we compare the outcome of our behavior estimation method in the three different settings (see Table 1 ): the negative values of \({\hat{\theta }}_{3}\) in the isolated settings (marriage or business) testify the broker position of the Medici family in both contexts, also in agreement with the analysis of the brokerage coefficients in the isolated marriage and business networks by Sims and Gilles 51 and Ostrom and Crothers 52 . On the other hand, though, the analysis of the combined network evinces the emergence of the opposite behavior with respect to the parameter \({\hat{\theta }}_{3}\) . Thus, the structural isolation operated on multiplex ties not only guaranteed stability (preventing dissent spreading) but at the same time enhanced social (and political) support (positive \({\hat{\theta }}_{3}\) ) to the Medici family. Furthermore, note that reciprocity cannot emerge in the isolated settings because of the way relations are described in the data. However, the estimate of \({\hat{\theta }}_{1}\) in the combined network confirms the segregation of types of ties with the Medici itself observed by Padgett and Ansell. A low estimate of \({\hat{\theta }}_{2}\) , finally, can be associated with the scarce tendency to cycles which would reduce the segregation of the leaves in the centralized network system of the Medici party.
Australian bank dataset
The second example deals with a study of structure in a number of branches of a large Australian bank 42 . The unweighted directed network of relationships shown in Fig. 7 is from one particular branch in response to the question “In whom do you feel you would be able to confide if a problem arose that you did not want everyone to know about?”, i.e., the confiding relations, though the same study also analyzed the advice-seeking, close friendship and satisfying interactions relations. From the attributes of the nodes, four different hierarchical levels can be distinguished, i.e., Branch Manager, Deputy Manager, Service Adviser and Teller. Low hierarchical positions occupy the periphery of the network, while high ranked nodes have more incoming connections. From a macroscopic inspection, one can detect the presence of star-like motifs embedded in the network, e.g., around the Branch Manager, and the Service Advisers 1 and 2. However, no other motifs are discernible.
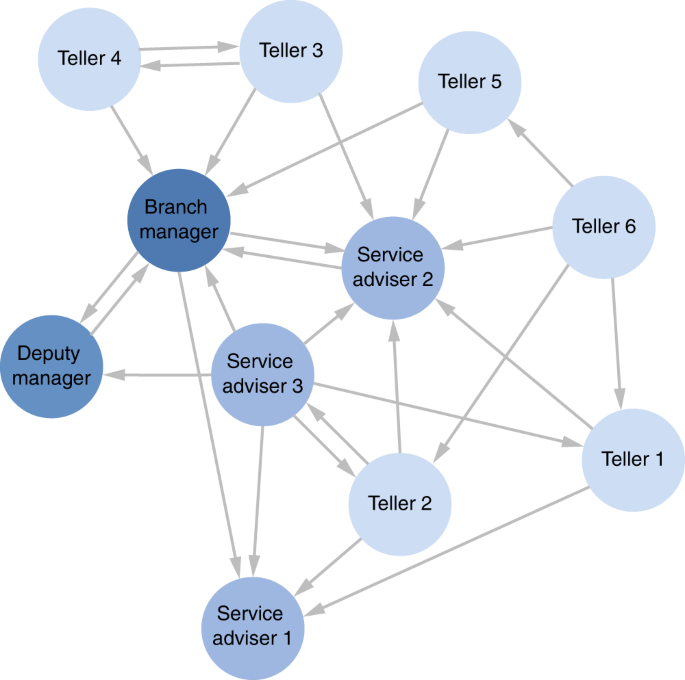
The network of confiding relationship among the 11 agents of the Australian bank dataset 42 . Every link has unitary weight and more important nodes have darker color.
The outcome of our behavior estimation method is shown in Fig. 8 . From the analysis one evinces that more competitive behaviors (negative values of \({\hat{\theta }}_{3}\) ) are typical of high hierarchical positions, e.g., Branch and Deputy manager. Conversely, low-ranking positions are more inclined towards social support (positive \({\hat{\theta }}_{3}\) ), as witnessed by the behavior of tellers 1–6. As observed by Pattison 42 , confiding relations are likely to be more local or restricted in their span, linking individuals from one level in the organization to those in the next. Thus, it is unlikely that high-rank agents exhibit clustering behavior, as there are fewer nodes in the top level of the hierarchical structure.
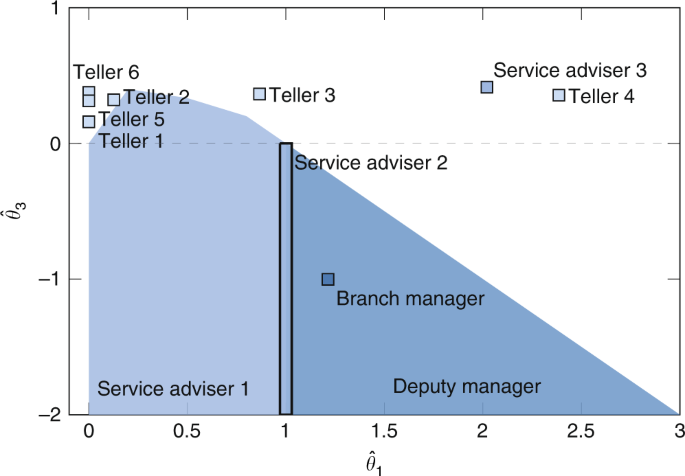
Best estimates \(({\hat{\theta }}_{1},{\hat{\theta }}_{3})\) for the Australian bank dataset 42 . A detailed description of the results and the confidence interval analysis are available in Supplementary Note 4 and Supplementary Table 2 .
The complete analysis reported in Supplementary Note 4 also shows that agents are not particularly inclined towards cyclic structures, in accordance with Davis 36 who showed that cycles are atypical structures in hierarchical networks. Finally, notice that several actors at different hierarchical levels, i.e., Branch and Deputy Managers, Service Adviser 2 and 3, Tellers 3 and 4 exhibit relatively high values of \({\hat{\theta }}_{1}\) , which is symptomatic of higher reciprocity in the relationships. We emphasize that, since relations that span across different hierarchical levels are not common, it is likely for high-rank agents to reciprocate ties, as they have fewer options.
Complex random networks
As discussed in the introduction, a large class of network formation models is based on probabilistic mechanisms. The aim of this section is to use our behavior estimation method to give a sociological and strategic interpretation of them.
The first example concerns the celebrated preferential attachment model introduced by Barabási and Albert 7 . In our analysis, we draw inspiration from ref. 53 to construct a directed version of the model where newborn nodes, introduced over time, receive \({m}_{{\rm{in}}}=2\) incoming edges from existing nodes (selected proportionally to their outdegree) and build \({m}_{{\rm{out}}}=2\) outgoing ties, whose receivers are now selected proportionally to their indegree. The resulting networks exhibit scale-free properties in both in- and outdegree measures (see Supplementary Fig. 6 ).
As we are looking at a growing process, we study the outgoing ties of the newborn agents \(n\) as soon as they are introduced to the network of \(n-1\) agents. More specifically, we consider \(50\) realizations of the Preferential Attachment growing process, and for each of them we focus our analysis on eight newborn agents \(n\) , as shown in Fig. 9 . When the network size \(n\) is still small, newborns tend to reciprocate links and form clusters (red values are symptomatic of high values of \({\hat{\theta }}_{1}\) and \({\hat{\theta }}_{3}\) ). When the network size grows, the behavior of the newborns becomes more and more adverse to reciprocity and clustering. Yet, the tendency of forming reciprocal ties and clusters does not entirely vanish.
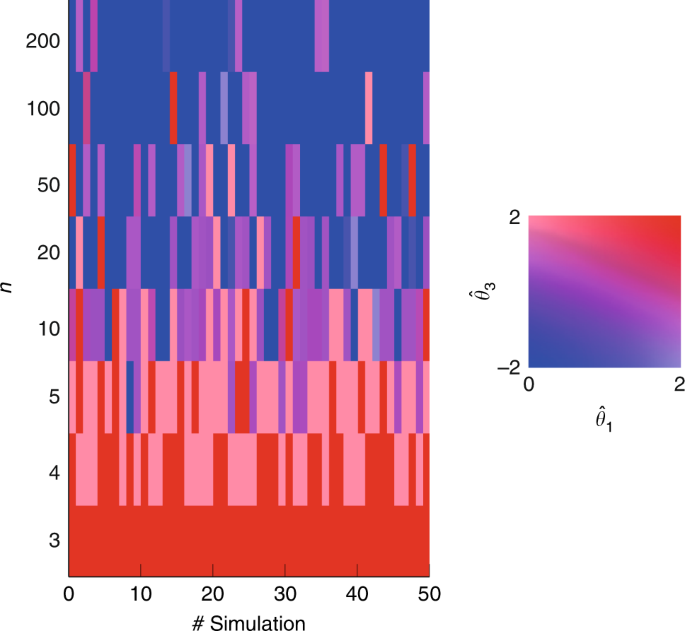
Results of the Preferential Attachment test. For each of the \(50\) simulations, we study the behavior of the newborn agent \(n\) . The left plot shows the minimizer of the distance function through the color map on the right, which associates a color to each pair \(({\hat{\theta }}_{1},{\hat{\theta }}_{3})\) . For illustration and computational purposes, we restrict the parameter space to \(\tilde{\Theta }=[0,2]\times 0\times [-2,2]\) , and the action space of the newborn agent \(n\) to \(\tilde{{\mathcal{A}}}=\{{{\bf{a}}}_{n}\in {\{0,1\}}^{n-1},\,{\text{s.t.}}\,| | {{\bf{a}}}_{n}| {| }_{1}\le {m}_{{{out}}}\}\) . In other words, the newborn agent \(n\) can only choose among all the combinations of (at maximum) \({m}_{out}\) outgoing edges (of weight \(1\) ). Hence, we solve \(({\hat{{\boldsymbol{\theta }}}}_{n,1},{\hat{{\boldsymbol{\theta }}}}_{n,3})\in \arg {\min }_{{{\boldsymbol{\theta }}}_{n}\in \tilde{\Theta }}{(\int _{\tilde{{\mathcal{A}}}}{e}_{n}^{+}{({{\bf{a}}}_{n},{{\boldsymbol{\theta }}}_{n})}^{2}{\rm{d}}{{\bf{a}}}_{n})}^{1/2},\) and we select the estimate with maximum Euclidean norm among the minimizers.
In our second example, we consider the small-world model introduced by Watts and Strogatz 5 , and we adapt it by rewiring only the outgoing edges, as in 54 . In Fig. 10 we collect the heat maps of the average distance function for several networks of fixed size \(N=11\) , different outdegree \(k\) , and rewiring probability \(p\) . We emphasize that when \(p=0\) the network is a regular ring lattice where each node is mutually connected with the \(k\) closest nodes in both directions. In these cases (first row of the figure), the increase of \(k\) coincides with an increase of the local clustering coefficient, which, in turns, corresponds to a change of the estimate of the clustering coefficient \({\hat{\theta }}_{3}\) from the negative to the positive half-space. On the other hand, when \(k=(N-1)/2\) , the ring lattice is a complete network and the rewiring process has no influence. As a matter of fact, the plots of the last column are all equal and they confirm the theoretical results of the complete network (see Fig. 4 b). In the other cases, increasing the rewiring probability \(p\) shifts the estimates of the reciprocity \({\hat{\theta }}_{1}\) towards increasingly smaller values. Such a phenomenon depends on the fact that the rewiring process tends to destroy the initial symmetry of the ties in the ring lattice.
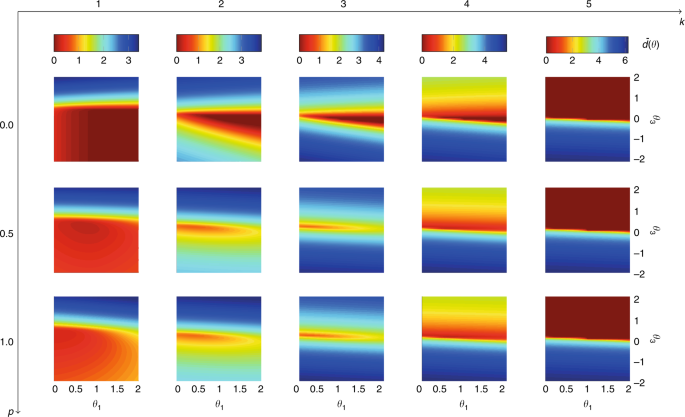
Results of the test on Small-World networks of fixed size \(N=11\) agents, different values of outdegree \(k\) , and of rewiring probability \(p\) . For each pair \((k,p)\) we consider \(n=10\) different realizations and we compute the average distance function \(\tilde{d}({\boldsymbol{\theta }})=\frac{1}{nN}{\sum }_{i=1}^{n}{\sum }_{j=1}^{N}{d}_{i}^{j}(\theta )\) , where \({d}_{i}^{j}({\boldsymbol{\theta }})\) is the distance function of agent \(i\) in the \({j}{\rm{th}}\) realization. For illustration and computational purposes, we restrict the parameter space to \(\tilde{\Theta }=[0,2]\times 0\times [-2,2]\) . The plot shows the heat map of \(\bar{d}\left({\boldsymbol{\theta }}\right)={\mathrm{log}}(\tilde{d}({\boldsymbol{\theta }})+1)\) in the \(({\theta }_{1},{\theta }_{3})\) space, with lower values (thus more likely) associated with the red color. Source data are provided as a Source Data file.
To conclude, the behavior estimation method reveals the existence of a pattern behind random network models, allowing for sociological and strategic interpretations of the probabilistic mechanisms. Further details are available in Supplementary Note 4 .
We proposed a parametric model of strategic network formation where actors can control their followees but not their followers, and they are endowed with a novel payoff function, which is a parametric combination of different incentives: influence, brokerage, closure. In the theoretical analysis, agents are assumed homogeneous, rational, and myopic. We analytically derived stability conditions of several network motifs, considering purely selfish and selfish-cooperative scenarios. We confirmed existing results on the correlation between incentives and stable network architectures, yet revealing new transition paradigms and opening the door for future investigation on cascade effects and robustness of equilibria. Further, our closed-form analytic stability conditions depend, in a parametric fashion, on the trade-offs between different individual incentives, thus providing a way to infer individual tendencies from the observed stable networks. Yet, we quantitatively describe the impact of cooperation on network stability.
We also considered complex networks scenarios with heterogeneous and not necessarily rational actors. Using the Nash equilibrium condition we constructed a statistical behavior estimation method capable to learn the individual user preferences. We applied this method to real-world datasets and random networks models, providing evidence that our results cross-validate empirical, historical, and sociological observations and our method offers sociological and strategic interpretation of random networks mechanisms. We emphasize that our model can be adapted to different descriptions of the payoff function, e.g., considering an extra cost for changing ties, or other individual incentives such as eigenvector centrality, or constraining competitors’ brokerage, as well as to different definitions of equilibrium, e.g., mixed-Nash equilibria or continuous parametric transitions of selfish-cooperative behavior as in 55 .
Theoretical analysis of network motifs
The goal of our theoretical analysis is to derive necessary and sufficient parametric conditions which guarantee NE/PNE. Concerning the NE conditions, we turn the definition into an optimization problem: \({{\mathcal{G}}}^{\star }\) is a Nash equilibrium if, for all agents \(i\) , \({{\bf{a}}}_{i}^{\star }\in \arg {\max }_{{{\bf{a}}}_{i}\in {\mathcal{A}}}{V}_{i}({{\bf{a}}}_{i},{{\bf{a}}}_{-i}^{\star }).\) Then, we use the Variational Inequality (VI) approach (discussed in Supplementary Note 1 ) in order to derive necessary conditions.
Theorem . If \({{\mathcal{G}}}^{\star }\) is a Nash equilibrium then
These conditions are then checked for sufficiency. If not, we restrict them by means of educated counterexamples, and verify again.
Concerning the PNE, we use an analogous VI approach in order to derive necessary conditions for the Nash property (C2). In this case, the necessary conditions are shown to be also sufficient. Finally, concerning the Pareto optimality property (C3), we obtain the necessary conditions by imposing (C3) for appropriate meeting actions. To guarantee sufficiency, we invoke the following result.
Theorem . Let \({{\mathcal{G}}}^{\star }\) be a network .
If (C2) is satisfied for a pair of agents \((i,j)\) and \({a}_{ij}^{\star }={a}_{ji}^{\star }=1\) , then (C3) is satisfied for the same pair ;
if for all pairs \((i,j)\) , the pair \(({a}_{ij}^{\star },{a}_{ji}^{\star })\) satisfies for all pairs \(({a}_{ij},{a}_{ji})\in {\left[0,1\right]}^{2}\) ,
then the Pareto Optimality condition (C3) is satisfied .
Behavior estimation method
For the behavior estimation method, we introduce the NE-distance function \({d}_{i}({{\boldsymbol{\theta }}}_{i})\) , and the goal is to identify the set of parameters \({\hat{{\boldsymbol{\theta }}}}_{i}\) that minimizes it. In order to approach the problem, we first verify that it is well-posed by proving convexity and smoothness of the distance function (the proof can be found in Supplementary Note 3 ). These two properties imply both tractability as well as scalability of algorithmic approaches to the Minimum NE-Distance problem.
Theorem . Let \(f({\bf{x}},{\boldsymbol{\theta }}):{{\mathbb{R}}}^{n}\times {{\mathbb{R}}}^{p}\to {\mathbb{R}}\) be a continuous function of \({\bf{x}}\in {{\mathbb{R}}}^{n}\) and \({\boldsymbol{\theta }}\in {{\mathbb{R}}}^{p}\) . Moreover, assume \(f\) to be linear in \({\boldsymbol{\theta }}\) , and let \({\mathcal{X}}\) be a compact subset of \({{\mathbb{R}}}^{n}\) . Consider the following function:
Then \(F\) is continuously differentiable, and its gradient is
Moreover, F is a convex function .
Then, we distinguish two cases. In the first case there are no violations, and the minimum of the distance function is \(0\) . In this case, the set of minimizers is a convex polyhedron that can be described by a finite number of inequalities:
where \({e}_{i}({{\bf{a}}}_{i},{{\boldsymbol{\theta }}}_{i})={V}_{i}\left({{\bf{a}}}_{i},{{\bf{a}}}_{-i}^{\star },{P}_{i}\right)-{V}_{i}\left({{\bf{a}}}_{i}^{\star },{{\bf{a}}}_{-i}^{\star },{P}_{i}\right)\) and \({{\mathcal{A}}}_{\left\{0,1\right\}}={\left\{0,1\right\}}^{N-1}\) , i.e., the inequalities need to be evaluated only at the vertices of the action space. We emphasize that, for all \({{\boldsymbol{\theta }}}_{i}\in {\Theta }_{i,0}\) , the NE conditions corresponding to agent \(i\) are full-filled.
In the second case, when NE violations occur, we consider a discrete version of the Minimum NE-Distance problem by approximating the integral over the action space with a finite sum over a regular grid. The discrete problem inherits smoothness and convexity, so it is possible to use a projected gradient method to find a solution. Moreover, the discrete problem can be viewed as an Ordinary Least Square regression problem, with the exception that error terms are non-negative, thus the bias of the estimates is non-zero and errors cannot be modeled as normally distributed. Nonetheless, we are able to build confidence intervals of the parameters. The detailed method is described in Supplementary Note 3 .
Reporting summary
Further information on research design is available in the Nature Research Reporting Summary linked to this article.
Data availability
The datasets as well as the source data files discussed are available, respectively, at the following public repositories 56 , 57 . A reporting summary for this article is available as a Supplementary Information file.
Code availability
The code that performs the behavior estimation method as well as the tests of the datasets and of the random network models are available at the following public repository 58 .
Tuten, T. L. and Solomon, M. R. Social Media Marketing (Sage, 2017).
Jackson, M. O. In Group Formation in Economics: Networks, Clubs, and Coalitions (eds Demange, G. & Wooders, M.) 11–49 (Cambridge, 2005).
Erdös, P. & Rényi, A. On random graphs, i. Publ. Math. (Debrecen) 6 , 290–297 (1959).
MathSciNet MATH Google Scholar
Erdös, P. & Rényi, A. On the evolution of random graphs. Publ. Math. Inst. Hung. Acad. Sci 5 , 17–60 (1960).
Watts, D. J. & Strogatz, S. H. Collective dynamics of ‘small-world’ networks. Nature 393 , 440 (1998).
Article ADS CAS Google Scholar
Milgram, S. The small world problem. Psychology today 2 , 60–67 (1967).
Google Scholar
Barabási, A.-L. & Albert, R. Emergence of scaling in random networks. Science 286 , 509–512 (1999).
Article ADS MathSciNet Google Scholar
Caldarelli, G. In Large Scale Structure and Dynamics of Complex Networks: From Information Technology to Finance and Natural Science 2 (World Scientific, 2007).
Latora, V., Nicosia, V., & Russo, G. Complex Networks: Principles, Methods and Applications (Cambridge University Press, 2017).
Castellano, C., Fortunato, S. & Loreto, V. Statistical physics of social dynamics. Rev. Mod. Phys. 81 , 591 (2009).
Article ADS Google Scholar
Snijders, T. A. Stochastic actor-oriented models for network change. J. Math. Sociol. 21 , 149–172 (1996).
Article Google Scholar
Snijders, T. A. The statistical evaluation of social network dynamics. Sociol. Methodol. 31 , 361–395 (2001).
Snijders, T. A., Van de Bunt, G. G. & Steglich, C. E. Introduction to stochastic actor-based models for network dynamics. Social Networks 32 , 44–60 (2010).
Lusher, D., Koskinen, J., & Robins, G. Exponential Random Graph Models for Social Networks: Theory, methods, and Applications (Cambridge University Press, 2013).
Robins, G., Pattison, P., Kalish, Y. & Lusher, D. An introduction to exponential random graph (p*) models for social networks. Social Networks 29 , 173–191 (2007).
Flap, H. & Völker, B. Creation and Returns of Social Capital (Routledge, 2004).
Jackson, M. O. & Wolinsky, A. A strategic model of social and economic networks. J. Econom. Theory 71 , 44–74 (1996).
Article MathSciNet Google Scholar
Bala, V. & Goyal, S. A noncooperative model of network formation. Econometrica 68 , 1181–1229 (2000).
Goyal, S. & Vega-Redondo, F. Structural holes in social networks. J. Econom. Theory 137 (nov), 460–492 (2007).
Buskens, V. & van de Rijt, A. Dynamics of networks if everyone strives for structural holes. Am. J. Sociol. 114 , 371–407 (2008).
Kleinberg, J., Suri, S., Tardos, É., & Wexler, T. Strategic network formation with structural holes. In Proc. 9th ACM Conference on Electronic Commerce 284–293 (ACM, 2008).
Buechel, B. In Networks, Topology and Dynamics. Springer Lecture Notes in Economic and Mathematical Systems Vol. 613 , 95–109 (Springer, 2008).
Burger, M. J. & Buskens, V. Social context and network formation: an experimental study. Social Networks 31 , 63–75 (2009).
Buechel, B. & Buskens, V. The dynamics of closeness and betweenness. J. Math. Sociol. 37 (), 159–191 (2013).
Dutta, B. & Jackson, M. O. The stability and efficiency of directed communication networks. Rev. Econom. Des. 5 , 251–272 (2000).
Bloch, F. & Dutta, B. Communication networks with endogenous link strength. Games Econom. Behavior 66 , 39–56 (2009).
Zenou, Y. In International Encyclopedia of the Social & Behavioral Sciences 2nd edn (ed. Wright, J. D.) 572–581 (Elsevier, Oxford, 2015).
Doreian, P. & Stokman, F. N. Evolution of Social Networks 1 (Psychology Press, 1997).
Snijders, T. A. & Doreian, P. Introduction to the special issue on network dynamics. Social Networks 32 , 1–3 (2010).
Jackson, M. O. Social and Economic Networks (Princeton University Press, 2010).
Goyal, S. Connections: An Introduction to the Economics of Networks . (Princeton University Press, 2012).
Freeman, L. C. Centrality in social networks conceptual clarification. Social Networks 1 , 215–239 (1978).
Burt, R. S. Structural Hole (Harvard Business School Press, Cambridge, MA, 1992).
Burt, R. S. Bridge decay. Social Networks 24 , 333–363 (2002).
Coleman, J. Foundations of Social Theory (Belknap, Cambridge, MA, 1990).
Davis, J. A. Clustering and hierarchy in interpersonal relations: testing two graph theoretical models on 742 sociomatrices. Am. Sociol. Rev. 35 , 843–851 (1970).
Cartwright, D. & Harary, F. Structural balance: a generalization of heider’s theory. Psychol. Rev. 63 , 277 (1956).
Article CAS Google Scholar
McPherson, M., Smith-Lovin, L. & Cook, J. M. Birds of a feather: homophily in social networks. Annu. Rev. Soc. 27 , 415–444 (2001).
Song, Y. & Schaar, M. Dynamic network formation with incomplete information. Econom. Theory 59 , 301–331 (2015).
Katz, L. A new status index derived from sociometric analysis. Psychometrika 18 , 39–43 (1953).
Padgett, J. F. & Ansell, C. K. Robust action and the rise of the medici, 1400-1434. Am. J. Sociol. 98 , 1259–1319 (1993).
Pattison, P., Wasserman, S., Robins, G. & Kanfer, A. M. Statistical evaluation of algebraic constraints for social networks. J. Math. Psychology 44 , 536–568 (2000).
Article MathSciNet CAS Google Scholar
Heider, F. Attitudes and cognitive organization. J. Psychol. 21 , 107–112 (1946).
Bloch, F. & Jackson, M. O. Definitions of equilibrium in network formation games. Int. J. Game Theory 34 , 305–318 (2006).
Calvó-Armengol, A. & Ilkiliç, R. Pairwise-stability and Nash equilibria in network formation. Int. J. Game Theory 38 , 51–79 (2009).
Allon, G., Federgruen, A. & Pierson, M. How much is a reduction of your customers’ wait worth? an empirical study of the fast-food drive-thru industry based on structural estimation methods. Manuf. Service Oper. Manage. 13 , 489–507 (2011).
Bertsimas, D., Gupta, V. & Paschalidis, I. C. Data-driven estimation in equilibrium using inverse optimization. Math. Prog. 153 , 595–633 (2015).
Nikaidô, H. et al. Note on non-cooperative convex games. Pac. J. Math. 5 (), 807–815 (1955).
Kent, D. V. The Rise of the Medici: Faction in Florence (pp. 1426–1434. Oxford University Press, USA, 1978).
Borgatti, S. P., Everett, M. G., & Freeman, L. C. In Encyclopedia of Social Network Analysis and Mining 2261–2267 (Springer, 2014).
Sims, O. & Gilles, R. Critical nodes in directed networks. Preprint at http://arXiv.org/abs/arXiv:1401.0655 (2014).
Ostrom, E. & Crothers, C. In Institutional Analysis and Development: Elements of the Framework in Historical Perspective (ed. Crothers, C.) 261–288 ( Encyclopedia of Life Support Systems (EOLSS) , 2010).
Bollobás, B., Borgs, C., Chayes, J., & Riordan, O. Directed scale-free graphs. In Proc. 14th Annual ACM-SIAM Symposium on Discrete algorithms 132–139 (Society for Industrial and Applied Mathematics, 2003).
Sánchez, A. D., López, J. M. & Rodríguez, M. A. Nonequilibrium phase transitions in directed small-world networks. Phys. Rev. Lett. 88 (Jan), 048701 (2002).
Grauwin, S., Bertin, E., Lemoy, R. & Jensen, P. Competition between collective and individual dynamics. Proc. Natl Acad. Sci. 106 , 20622–20626 (2009).
Pagan, N. & Dörfler, F. Game theoretical inference of human behavior in social networks (data sets). https://doi.org/10.3929/ethz-b-000360648 (2019).
Pagan, N. & Dörfler, F. Game theoretical inference of human behavior in social networks (source data). https://doi.org/10.3929/ethz-b-000360651 (2019).
Pagan, N. & Dörfler, F. Game theoretical inference of human behavior in social networks (code). https://doi.org/10.3929/ethz-b-000360647 (2019).
Download references
Acknowledgements
The authors thank Prof. C. Stadtfeld for the interesting discussions on the individual incentives, and M. Gallana for his contribution on the statistical interpretation of the behavior estimation method. They also gratefully acknowledge financial support from ETH Zürich.
Author information
Authors and affiliations.
Automatic Control Laboratory, ETH Zürich, Physikstrasse 3, 8092, Zürich, Switzerland
Nicolò Pagan & Florian Dörfler
You can also search for this author in PubMed Google Scholar
Contributions
N.P. and F.D. designed research, N.P. performed research, N.P. wrote this manuscript, and F.D. edited this manuscript.
Corresponding author
Correspondence to Nicolò Pagan .
Ethics declarations
Competing interests.
The authors declare no conflict of interest.
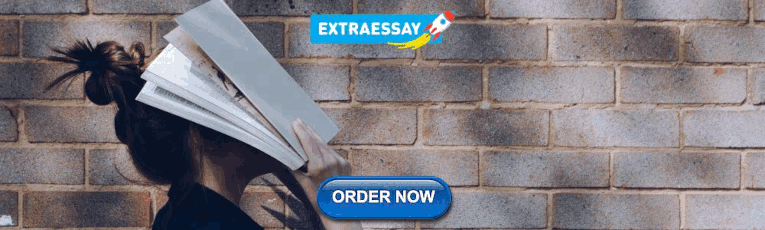
Additional information
Peer review information Nature Communications thanks Christoph Stadtfeld and the other, anonymous, reviewer(s) for their contribution to the peer review of this work. Peer reviewer reports are available.
Publisher’s note Springer Nature remains neutral with regard to jurisdictional claims in published maps and institutional affiliations.
Supplementary information
Supplementary information, peer review file, reporting summary, rights and permissions.
Open Access This article is licensed under a Creative Commons Attribution 4.0 International License, which permits use, sharing, adaptation, distribution and reproduction in any medium or format, as long as you give appropriate credit to the original author(s) and the source, provide a link to the Creative Commons license, and indicate if changes were made. The images or other third party material in this article are included in the article’s Creative Commons license, unless indicated otherwise in a credit line to the material. If material is not included in the article’s Creative Commons license and your intended use is not permitted by statutory regulation or exceeds the permitted use, you will need to obtain permission directly from the copyright holder. To view a copy of this license, visit http://creativecommons.org/licenses/by/4.0/ .
Reprints and permissions
About this article
Cite this article.
Pagan, N., Dörfler, F. Game theoretical inference of human behavior in social networks. Nat Commun 10 , 5507 (2019). https://doi.org/10.1038/s41467-019-13148-8
Download citation
Received : 23 November 2018
Accepted : 22 October 2019
Published : 03 December 2019
DOI : https://doi.org/10.1038/s41467-019-13148-8
Share this article
Anyone you share the following link with will be able to read this content:
Sorry, a shareable link is not currently available for this article.
Provided by the Springer Nature SharedIt content-sharing initiative
This article is cited by
A meritocratic network formation model for the rise of social media influencers.
- Nicolò Pagan
- Florian Dörfler
Nature Communications (2021)
By submitting a comment you agree to abide by our Terms and Community Guidelines . If you find something abusive or that does not comply with our terms or guidelines please flag it as inappropriate.
Quick links
- Explore articles by subject
- Guide to authors
- Editorial policies
Sign up for the Nature Briefing newsletter — what matters in science, free to your inbox daily.

8 Game Theory Examples in Real Life
When we hear the word ‘game,’ we usually start thinking of some fun and amazing activities that one plays, but the ‘game theory’ is the study of the mathematical and scientific model of strategic decision making, which focuses on analyzing the various cost and benefits involved in any situation (game) and trying to make the best possible solution that offers the maximum benefit and minimum or zero lose. The economist Oskar Morgenstern and the mathematician John Neumann first formulated the game theory in 1940, and another mathematician John Nash further advanced their work and modernised the game theory. The game theory comes into play whenever the person tries to make any decision by understanding the several rules of the game, wherein each of the players receives the payoff according to the effectiveness of his/her decision. Nearly 12 professional economists and researchers have been bestowed with the Noble Prize in Economics for contributing to the advancement of the game theory. The game theory finds its applications in several areas, which include finance, business, psychology, political science, social sciences, computer science, mathematics, and philosophy. An understanding of the game theory can help the person in making a clear analysis of the problem, and finding the best possible solution to any given problem. Here in this article, we’ll learn about the game theory and the various real-life examples of the game theory.
Understanding the Game Theory
Let us first understand the few main terms described in the game theory.
Game: It refers to certain events or circumstances, wherein the outcome is dependent upon the actions or the decisions of the players involved.
Players: It refers to the people that make the strategic decisions in the game.
Strategy: It refers to the set of actions taken by the player to deal with the various circumstances that may arise in the game.
Payoff: It refers to the payout that the player gets if he/she arrives at any specific outcome. The payout could be money or other profitable or beneficial items.
Information set: It is the pieces of information that are given at any specific time during the game. The information set is generally applied in case the game consists of sequential components.
The Nash Equilibrium: Nash equilibrium is an important concept in game theory. It is named after its pioneer John Nash; he received the Noble prize for his contribution to game theory in 1994. Nash equilibrium refers to a state where the players reached the outcome, and they can not make any further changes by making independent decisions to rise their payoffs.
The game theory focuses on formulating a model of decision-making by identifying the players’ preferences, and possible strategies. The game theory proposes that the outcome of a game is influenced by the actions and decisions of all the players involved in the game, and each player thinks rationally to get the maximum payoff. A person can utilize the game theory to find an effective solution in any situation involving two or more players, wherein he/she is aware of the predictable consequences and payoffs. The game theory is based on the concept that the payoff of the one player is dependent upon the strategies or the decisions of the other player/players.
Five Types of Games in Game Theory
1. cooperative and non-cooperative games.
There are several types of games in the game theory, however, the two commonly known types of game theory are cooperative and non-cooperative games. In cooperative games, the players have to follow a specific strategy by negotiating with the other players. The problems are solved by following the agreement based on the mutual discussion among the players. The cooperative game theory focuses on the interaction of the cooperative groups and coalitions when the players are only aware of the payoffs. The cooperative game theory also questions how do the groups formulate and how the payoffs are distributed among the players. Let us understand this type through an example, suppose company X which manufactures alcohol has to spend a huge amount on the marketing of its product, hence the management decides to reduce the high ad-expenditure. But, the problem is that there is not any surety that the other companies who produce the same product will also do the same and spend less on marketing. Hence, a dillemma is created among the cigarette manufacturing companies that whether they should lower their budget on marketing or not because if one company does not follow the agreement and spends more on marketing then that company may get the profit while others who follow the agreement are likely to face the losses. However, if the authorities or the government passes a rule that restricts cigarette advertisement, it would be helpful for company A in reducing its advertisement budget. Here, even though the government may take the decision for the health of the people but it worked as cooperation for company A, hence it is an example of a cooperative game. Contrary to cooperative games, in non-cooperative games, the player focuses only on their profits and makes the strategies for their own maximum profit. Non-cooperative theory deals with how do the players think rationally and deal with the other players to achieve their targets. Rock-paper-scissors are a prominent example of a non-cooperative game. It is a strategic game, wherein the outcome is based on the combination of various sets of choices.
2. Normal Form and Extensive Form Game
When the description of the game, i.e., the strategies of the players and the payoffs are represented in the tabular or matrix form, it is called normal form games. These types of games help to find the Nash equilibrium and the strategies that can dominate the other players. The matrix in the normal game form represents the type of strategies used by the players and the possible outcomes associated with those strategies. While in the case of the extensive game forms, the description of the game is given in the form of a decision tree. In the case of the extensive game, the naturally occurring event in the game can be easily represented. The representation forms a tree-like structure, and the player’s names are defined on the nodes. Also, the possible actions and the payoffs of the players are also represented in the structure. To understand the extensive form of games, let us consider an example, suppose company A is an older organization with around a decade of experience, and company B is in the initial phase and wants to enter the market. Company B has two strategies, the first is that enter the market and face a strong challenge from the existing company B, and the second option is that avoid the chance to earn more profit by not entering the new market. Company A also has two strategies, i.e., either to face the competition from company A and risk its existence or help organization B in entering the new market and both companies cooperate with each other. The figure below represents that company B takes the first step and this step is later followed by company A. The payoff of company B will be zero if it does not enter the market, but if the company decides to enter the market the payoff would depend upon the situation of the market which is controlled by company A. If both the companies decide to compete with each other, both of them are likely to face a loss. But, if company A cooperates with company B, then both the companies can make an equal profit. Hence, the best suitable strategy, in this case, would be that company B enters the market and company A helps company B.
3. Symmetric and Asymmetric Games
The main feature of symmetric games is that all the players in these games adopt the same strategies. This is usually applicable in the short-duration games because in the long-duration games the players get a more number of options. In symmetric games, the decisions do not depend upon the player, in fact, it is based on the type of strategies used. The decisions in the symmetric games remain the same even if the players are interchanged in the game. The prisoner’s dilemma is a prominent example of the symmetric game. This example is discussed further in this article. In the case of asymmetric games, the decisions depend upon the players. In these games, if a particular strategy provides benefits to one player, it does not assure that the other player will also get equal benefits. A prominent example of asymmetric games is the decision of the company to enter the new market.
4. Simultaneous Games and Sequential Games
Those games in which the two or more players simultaneously play the move (adopt strategies) are known as simultaneous games. In simultaneous games, the players are not aware of the moves of the other players, while in the case of sequential games the players know about the moves of the other players if they already have applied any particular strategy. However, the players do not have a deep understanding of the type of strategies that may be used by the other players, for example, the player has the knowledge that the other player may not use only a single strategy, but he/she does not know that how many strategies the other player may adopt. We can represent the sequential games in the extensive form, and the simultaneous games in the normal form. The application of the simultaneous move game can be understood through the following example.
Suppose company A and company B both want to outsource their marketing budget. However, the companies have a fear that if they outsource their marketing budget, the other companies that are spending huge on marketing will gain more profit. Hence companies A and B have two strategies, i.e., either to outsource their marketing budget or not. In the following table, it can be observed that companies A and B are not aware of each other’s strategies. Both the companies operate on the assumption that the other company will choose the best strategy for itself, hence both the companies will choose the strategy that benefits themselves.
This example can also be used to represent the sequential move games. Suppose company A makes the first move and decides either to outsource its marketing strategies or not (as shown in the image below). It can be seen in the image below that the first strategy was decided by company A, and the decision of company B is based on the decision of company A. However, the outcome of the game is dependent upon the strategy of company B. In this example, the second player (company Y) has the knowledge of the strategy of the first player (company X).
5. Constant Sum, Zero-sum and Non-Zero-Sum Games
In the constant sum game, even if the players have received different outcomes, the sum of all the outcomes will always remain constant. In the zero-sum game, the net of all the player’s outcomes is zero. In a zero-sum game, the available resources can not be affected by the strategies of the different players. Also, the important feature of this game is that the gains and losses are proportional, i.e., the loss of one player is equal to the gains of the other player. On contrary to this, in the non-zero games, the net of all the outcomes of the players is not zero. The non-zero-sum games can be converted to zero-sum games if we add an extra player (dummy player). The dummy player’s losses are proportional to the net earnings of the other players. The main examples of zero-sum games are gambling and chess, in these games clearly if one player losses the other player wins, i.e., the loss of one player is the gain of the other player. Also, ‘matching coin’ is a most used type of zero-sum game. It consists of two players, says player A and player B. In this game, each player simultaneously puts the coins on the table, and the payoff is dependent upon whether the coins match or not. If both the coins show head or tails, then player A will win the game and gets the coin of player B. If the coins do not match, player B will win the game and he/she will keep the coin of player A.
Examples of Game Theory Strategies
Game theory is used to analyse the number of games. Let us understand the analyses of some games through the following examples,
1. Prisoner’s Dilemma
The prisoner’s dilemma is one of the most popular examples of game theory. Let us suppose two people say Prisoner-A and Prisoner-B are arrested for committing a crime, however, the prosecutor still needs a shred of proper evidence to decide their conviction. To get the confession from the prisoners they question the two in the different rooms. Both the prisoners are separated and there are not any means of communication between them. The officials proposed some deals to both the prisoners. According to the deals, if both the prisoners confess the crime, each of the prisoners will receive a prison sentence of five years. If prisoner-A confesses the crime, but prisoner B denies it, then Prisoner A will receive a three years prison sentence, and prisoner B will be freed. If prisoner B confesses, but prisoner A denies, then prisoner B will get the three years prison sentence, and prisoner A will be freed from the prison sentence. If both the prisoners do not confess, they both will receive two years of prison confession. Now, from all of the given deals, the most favourable one is that neither of the prisoners confesses as they are receiving the lesser punishment (two years of imprisonment) in this case only. However, the problem is that the prisoners do not know the strategies each other, they are not certain whether the other prisoner will confess or not. According to the Nash equilibrium, the prisoners are more likely to choose the deal that is best for their own but is worst for both of them collectively, i.e., the expression ‘tit for tat’ is considered the best solution as per the game theory. The concept of tit-for-tat was presented by an American mathematical psychologist, Anatol Rapoport, who stated that the players are likely to not cooperate if provoked, while they are likely to cooperate if unprovoked.
2. Deadlock
The deadlock is a social dilemma similar to the example of the prisoner’s dilemma in which the players either cooperate with each other or do not cooperate (defect). In a deadlock, if organization X and organization Y both cooperate, then both the organization will receive the payoff of 1, and if they both do not cooperate, they will receive the payoff of 2. If organization X cooperates and organization Y defects, the organization will get the payoff of zero, while organization Y will get the payoff of 3. Unlike prisoner’s dilemma, in case of deadlock, if both the organizations do not cooperate is also an effective strategy. Let us understand the deadlock through an example. Suppose the two powerful nations are trying to settle for an agreement that manufacturing nuclear weapons should be prohibited. In this case, cooperation means that both nations stick to the agreement and do not manufacture nuclear weapons, while the defections imply that either one or both the nations secretly break the agreement and manufacture the nuclear weapons. Unfortunately, in this case, the dominant outcome for either of the nation would be secretly breaking the agreement and manufacturing nuclear weapons because it will give that nation a benefit over the other nation in case the war occurs. The other effective option is that if both the nation do not cooperate and keep on manufacturing nuclear weapons. The following image represents the example of a deadlock between organization X and organization Y.
3. Cournot Competition
This game is named after its inventor Augustin Cournot, a French mathematician who presented this model in 1838. Its concept is similar to the prisoner’s dilemma. The Cournot model is utilized in explaining the duopoly, i.e., the competition between the two main organizations in a market. For example, suppose the company X and Y both manufacture similar products and they can manufacture the product in large and small quantities. If both the companies corporate with each other and decide to manufacture the product in lower quantity, then the limited supply of that product in the market will result in a rise in the price of that product, hence both the companies will get the benefit. However, if both the companies revoke and decide to defeat each other and produce the product of higher quality, it will result in a low price of that product and both the companies will face the drawbacks. Also, if one company cooperates and produces the product in lower quantity while the other company do not, i.e., produce the product in high quantity, then the former company will suffer the loss, while the latter will earn the maximum profit. The payoff matrix of company X and company Y is shown in the image-A below (profits are represented in millions of dollars).
4. Dictator Game
In this game, player X has to decide the division of the payoff with the player Y, who has zero contribution to the decision of player X. According to the results revealed by different experiments, it was found that in this scenario, 50 per cent of people keep all the payoff to themselves, 45 per cent of people give a small share of the payoff to the other, while only 5 per cent of people split the payoff equally. The dictator game is almost similar to the ultimatum game; in the ultimatum game, a certain amount of cash is given to player X, part of which he/she has to give to the player Y, who has to either accept or reject the given amount. If player Y rejects the amount offered, then both the players will get nothing.
5. Coordination Game
In coordination games, the players get the maximum benefits if they choose the same strategy. For example, suppose the two giant tech companies are in the dilemma of whether to introduce new technology in the computers that would make them earn huge or advance the old technology that would make them earn only a few. The problem is if only one company decides to introduce the new technology in computers the customers would find it difficult to adapt to the new technology and prefers to buy the systems with the technology that the customers are familiar to use, hence as a result the company will face losses. On the other hand, if both the companies decide to introduce the new technology, the customers will be left with no other option than to buy the computers with the new technology. Hence, in this case, both the companies will earn more and gets a profit.
6. The Centipede Game
In the centipede game, the two players get the alternative turns to get the larger share of the money stash, which is increasing slowly. It is a sequential game as players do not make the move simultaneously, in fact, they make the moves one after the other, hence they are aware of the strategy of the player who made the move before them. The game ends the moment when the players get the stash, where one player gets the larger portion and the other gets the smaller portion. For example, suppose player X starts the game and has to either ‘take’ or ‘pass’ the stash, which amounts to two dollars. If player X takes the stash, then both player X and player Y will get one dollar each, but if player X pass the stash, now, player Y has to decide whether to pass or take the stash. If player Y takes this stash, he will get the three dollars (previous 2 dollars + 1 dollar), while player X will get the zero dollars. However, if player Y passes, player A gets the chance to decide. In case both the players always choose to pass the stash, they both will receive the payoff of 100 dollars at the last. The catch here is that the players will get the maximum payoff if both the players keep on passing until the last of the game. But, as predicted by the Nash equilibrium, if any of the players disrupt the game and choose to ‘take the stash’ the players are likely to get the lowest payoff of one dollar. However, some experimental studies have revealed that this type of rational behaviour, which is anticipated by the game theory, is rarely observed in real life.
7. Volunteer’s Dilemma
In the case of the volunteer’s dilemma, someone has to take the responsibility for the benefit of a large number of people. According to the game theory, the worst outcome would be, if nobody dares to volunteer. For example, suppose some junior members of the recruitment agency assigned by the government to hire the people for various state-level vacancies are frauds and are responsible for the malfunctioning the recruitment drives. The higher authorities are unaware of this malfunctioning. Some other members who know about this fraud are hesitant to tell the higher authorities about this fraud as they are scared to lose their job or be called whistleblowers by the members involved in the fraud. But, if nobody dares to volunteer the fraud will result in a large disruption in the state.
Real-Life Game Theory Examples
Every person uses game theory in his/her everyday life whether consciously or unconsciously. Our everyday decisions of doing a particular task first and the other tasks later are based on the concept of the game theory. Let us discuss some real-life examples where the concepts of game theory are being utilized.
1. Pricing Decision
Game theory helps in determining the strategies of the consumer and the retailer price. Retailers attract the market by luring them with attractive sales on particular goods or services to increase their sales. You must be aware of that off-season sale, when the vendors offer the product at an amazing price, well this is one of the strategies of the game theory. The two main players in this game of pricing decisions are the retailers and the consumers. The customers want the goods at the best prices, and the retailer makes use of the best pricing tactic.
2. Collective Bargaining
One of the best examples of game theory is negotiation or collective bargaining. For example, in case of a strike by the employees to raise their wages, the union and management negotiate with the protestors and prefer to increase the wages because as per the game theory this seems to be the best possible solution to handle the situation as this ensures the benefits of both the workers and the management. Other applications include negotiations with the suppliers and negotiation for compensation or incentives by the workers to the management or the supplier.
3. New Product Decision
The understanding of Game theory helps businesses to decide whether they should launch a new product or not. Game theory helps them in understanding the moves of the competitor that may launch a similar product, and also the strategies they may apply in defence. The game theory can provide a rough analysis of the success and the failure of the new launch, hence businessmen could take the best decision with the help of game theory.
4. In Negotiating Salary
Asking for raise in salary is a complex task, it gets even more complex if more than one person is involved in the negotiation. The game theory helps in better negotiation with the manager. One should be aware of the kind of services he/she provides and the worth of those services in the company. One can not simply go and ask for a raise just because he/she wants a higher salary. According to the game theory, you must be well known about the person with whom you are making the salary negotiations and be prepared for the potential responses and the counter-arguments. If you know how to effectively respond to the person with whom you are negotiating about the salary, then chances are high that you will get the raise, considering you have the skill and values that add worth to the organization.
5. While Buying Car
Game theory can help you to buy the car at a much cheaper price than the amount it will cost you otherwise. The first step of car buying is looking for the dealers near your area that sells the car you want. Now, rather than simply visiting the dealers and bargaining with the salesperson, the better step is to call each dealer and mention to them that you are looking for a particular car and you will buy it from the dealer that will give the best offer. The dealer may deny negotiating about the price on the mobile, but you should respond confidently that you are only available for the negotiations over the phone and will consider buying if the offer is good. The dealer will then try to give the best offer to seal the deal. Hence, by using the strategies of the game theory you can buy the car at much lower prices.
6. Real Estate
You may have never noticed before, but the game theory finds its applications in real estate. Most of the real estate negotiations are already understood by the agents which makes it easier for sealing a deal. But, the situation is entirely different in the multi offers deals. You will only have the three options if the bid you have submitted is announced as a multi-offer situation by the real estate agent. These three options are
- Keeping the original offer
- Withdrawing the current offer
- raises the offer price
To win the bid the person have to overbid the other bidder. If the game theory is followed, the person should bid the number he/she has calculated to win the bid according to his/her budget, rather than increasing the price in bidding over the budget. In this case, even if you lose the bid, you still had made an effective decision because bidding at a price that is not in your budget is an incorrect decision that may cause a burden on you.
7. Rise and Fall of Bitcoin
Sometimes the decisions of the people in doing a certain task are not only based on their own perspective but it is influenced by the decisions of the majority of the population. Stock trading and crypto trading are prominent examples of decisions dependent upon other people and utilization of game theory. Suppose you want to buy a bitcoin. What would be your first step? Of course, you will look at the chart of the recent fluctuation to decide whether to buy the bitcoin or not. You see that a large number of people are buying the bitcoin and they are gaining profit and you also buy the bitcoin. Now, suppose a well known financial expert posts a tweet that questions the stability of the bitcoin. Like another majority of people, you also think of selling the bitcoin as an expert has criticized it, and the value of the bitcoin gets lower. Hence, in this case, the game theory results in the rise and fall of bitcoin.
8. During Auction
Before putting any of your assets in the auction, deciding the type of auction that would be suitable for better gain is an important step. There are a number of different methods in which an auction can be conducted; the ‘second prize auction’ recommended by Canadian-American economics professor William Vickery is one of the best ways of conducting an auction. In this method, the members bid for the asset by offering the highest amount, however, the person who bid the highest amount is not required to give the highest bid price, instead, he/she has to pay the second-highest bid price. This strategy makes the participants bid for the higher amount as they will think that they are saving money as they have to pay the second-highest bid price and not the highest bid price.
Related Posts
Examples of Ray in Real Life
6 Hypothesis Examples in Psychology
Examples of Compound Interest in Real Life
7 Important Methods in Psychology With Examples
10 Real Life Examples Of Gestalt Principles
Examples of a Line in Real Life
Add comment cancel reply.
June 2, 2003
What is game theory and what are some of its applications?
Saul I. Gass, professor emeritus at the University of Maryland's Robert H. Smith School of Business, explains.
Game: A competitive activity involving skill, chance, or endurance on the part of two or more persons who play according to a set of rules, usually for their own amusement or for that of spectators ( The Random House Dictionary of the English Language, 1967).
Consider the following real-world competitive situations: missile defense, sales price wars for new cars, energy regulation, auditing tax payers, the TV show "Survivor," terrorism, NASCAR racing, labor- management negotiations, military conflicts, bidding at auction, arbitration, advertising, elections and voting, agricultural crop selection, conflict resolution, stock market, insurance, and telecommunications. What do they have in common?
On supporting science journalism
If you're enjoying this article, consider supporting our award-winning journalism by subscribing . By purchasing a subscription you are helping to ensure the future of impactful stories about the discoveries and ideas shaping our world today.
A basic example helps to illustrate the point. After learning how to play the game tick-tack-toe, you probably discovered a strategy of play that enables you to achieve at least a draw and even win if your opponent makes a mistake and you notice it. Sticking to that strategy ensures that you will not lose.
This simple game illustrates the essential aspects of what is now called game theory. In it, a game is the set of rules that describe it. An instance of the game from beginning to end is known as a play of the game. And a pure strategy--such as the one you found for tick-tack-toe--is an overall plan specifying moves to be taken in all eventualities that can arise in a play of the game. A game is said to have perfect information if, throughout its play, all the rules, possible choices, and past history of play by any player are known to all participants. Games like tick-tack-toe, backgammon and chess are games with perfect information and such games are solved by pure strategies. But whereas you may be able to describe all such pure strategies for tick-tack-toe, it is not possible to do so for chess, hence the latter's age-old intrigue.
Games without perfect information, such as matching pennies, stone-paper-scissors or poker offer the players a challenge because there is no pure strategy that ensures a win. For matching pennies you have two pure strategies: play heads or tails. For stone-paper-scissors you have three pure strategies: play stone or paper or scissors. In both instances you cannot just continually play a pure strategy like heads or stone because your opponent will soon catch on and play the associated winning strategy. What to do? We soon learn to try to confound our opponent by randomizing our choice of strategy for each play (for heads-tails, just toss the coin in the air and see what happens for a 50-50 split). There are also other ways to control how we randomize. For example, for stone-paper-scissors we can toss a six-sided die and decide to select stone half the time (the numbers 1, 2 or 3 are tossed), select paper one third of the time (the numbers 4 or 5 are tossed) or select scissors one sixth of the time (the number 6 is tossed). Doing so would tend to hide your choice from your opponent. But, by mixing strategies in this manner, should you expect to win or lose in the long run? What is the optimal mix of strategies you should play? How much would you expect to win? This is where the modern mathematical theory of games comes into play.
Games such as heads-tails and stone-paper-scissors are called two-person zero-sum games. Zero-sum means that any money Player 1 wins (or loses) is exactly the same amount of money that Player 2 loses (or wins). That is, no money is created or lost by playing the game. Most parlor games are many-person zero-sum games (but if you are playing poker in a gambling hall, with the hall taking a certain percentage of the pot to cover its overhead, the game is not zero-sum). For two-person zero-sum games, the 20th century¿s most famous mathematician, John von Neumann, proved that all such games have optimal strategies for both players, with an associated expected value of the game. Here the optimal strategy, given that the game is being played many times, is a specialized random mix of the individual pure strategies. The value of the game, denoted by v, is the value that a player, say Player 1, is guaranteed to at least win if he sticks to the designated optimal mix of strategies no matter what mix of strategies Player 2 uses. Similarly, Player 2 is guaranteed not to lose more than v if he sticks to the designated optimal mix of strategies no matter what mix of strategies Player 1 uses. If v is a positive amount, then Player 1 can expect to win that amount, averaged out over many plays, and Player 2 can expect to lose that amount. The opposite is the case if v is a negative amount. Such a game is said to be fair if v = 0. That is, both players can expect to win 0 over a long run of plays. The mathematical description of a zero-sum two-person game is not difficult to construct, and determining the optimal strategies and the value of the game is computationally straightforward. We can show that heads-tails is a fair game and that both players have the same optimal mix of strategies that randomizes the selection of heads or tails 50 percent of the time for each. Stone-paper-scissors is also a fair game and both players have optimal strategies that employ each choice one third of the time. Not all zero-sum games are fair, although most two-person zero-sum parlor games are fair games. So why do we then play them? They are fun, we like the competition, and, since we usually play for a short period of time, the average winnings could be different than 0. Try your hand at the following game that has a v = 1/5.
The Skin Game: Two players are each provided with an ace of diamonds and an ace of clubs. Player 1 is also given the two of diamonds and Player 2 the two of clubs. In a play of the game, Player 1 shows one card, and Player 2, ignorant of Player 1¿s choice, shows one card. Player 1 wins if the suits match, and Player 2 wins if they do not. The amount (payoff) that is won is the numerical value of the card of the winner. But, if the two deuces are shown, the payoff is zero. [Here, if the payoffs are in dollars, Player 1 can expect to win $0.20. This game is a carnival hustler¿s (Player 1) favorite; his optimal mixed strategy is to never play the ace of diamonds, play the ace of clubs 60 percent of the time, and the two of diamonds 40 percent of the time.]
The power of game theory goes way beyond the analysis of such relatively simple games, but complications do arise. We can have many-person competitive situations in which the players can form coalitions and cooperate against the other players; many-person games that are nonzero-sum; games with an infinite number of strategies; and two-person nonzero sum games, to name a few. Mathematical analysis of such games has led to a generalization of von Neumann¿s optimal solution result for two-person zero-sum games called an equilibrium solution. An equilibrium solution is a set of mixed strategies, one for each player, such that each player has no reason to deviate from that strategy, assuming all the other players stick to their equilibrium strategy. We then have the important generalization of a solution for game theory: Any many-person non-cooperative finite strategy game has at least one equilibrium solution. This result was proven by John Nash and was pictured in the movie, A Beautiful Mind. The book ( A Beautiful Mind, by Sylvia Nasar; Simon & Schuster, 1998) provides a more realistic and better-told story.
By now you have concluded that the answer to the opening question on competitive situations is "game theory." Aspects of all the cited areas have been subjected to analysis using the techniques of game theory. The web site www.gametheory.net lists about 200 fairly recent references organized into 20 categories. It is important to note, however, that for many competitive situations game theory does not really solve the problem at hand. Instead, it helps to illuminate the problem and offers us a different way of interpreting the competitive interactions and possible results. Game theory is a standard tool of analysis for professionals working in the fields of operations research, economics, finance, regulation, military, insurance, retail marketing, politics, conflict analysis, and energy, to name a few. For further information about game theory see the aforementioned web site and http://william-king.www.drexel.edu/top/eco/game/game.html.
Game Theory in Politics
- First Online: 05 August 2023
Cite this chapter
- Tshilidzi Marwala 2
518 Accesses
1 Altmetric
Game theory has become an indispensable tool in international politics, offering valuable insights into the complex and strategic decision-making processes that govern the interactions between nations, international organizations, and non-state actors. As a mathematical framework for understanding strategic behavior and predicting outcomes, game theory allows scholars and policymakers to model and analyze the myriad of factors that influence the actions and choices of actors on the international stage. This chapter explores the role of game theory in international politics, highlighting its key concepts, applications, and contributions to our understanding of global dynamics. Additionally, we discuss the potential limitations and challenges of applying game theory in the intricate and ever-changing landscape of international politics.
This is a preview of subscription content, log in via an institution to check access.
Access this chapter
Subscribe and save.
- Get 10 units per month
- Download Article/Chapter or eBook
- 1 Unit = 1 Article or 1 Chapter
- Cancel anytime
- Available as PDF
- Read on any device
- Instant download
- Own it forever
- Available as EPUB and PDF
- Durable hardcover edition
- Dispatched in 3 to 5 business days
- Free shipping worldwide - see info
Tax calculation will be finalised at checkout
Purchases are for personal use only
Institutional subscriptions
https://www.jfklibrary.org/learn/about-jfk/jfk-in-history/the-cold-war .
https://www.cfr.org/global-conflict-tracker/conflict/territorial-disputes-south-china-sea .
http://www.nuclearfiles.org/menu/key-issues/nuclear-weapons/history/cold-war/strategy/strategy-mutual-assured-destruction.htm .
Adler, I. (2013). The equivalence of linear programs and zero-sum games. International Journal of Game Theory, 42 (1), 165.
Article Google Scholar
Amadae, S. M. (2016). Prisoners of reason: Game theory and neoliberal political economy . Cambridge University Press.
Google Scholar
Aumann, R. J. (1987). Game theory. In J. Eatwell, M. Milgate, & P. Newman (Eds.), The new Palgrave: A dictionary of economics . Macmillan.
Beckenkamp, M., Hennig‐Schmidt, H., & Maier-Rigaud, F. P. (2007). Cooperation in symmetric and asymmetric prisoner’s dilemma games. MPI Collective Goods Preprint (2006/25).
Bernheim, B. D., Peleg, B., & Whinston, M. D. (1987). Coalition-proof Nash equilibria I. concepts. Journal of Economic Theory , 42 (1), 1–12.
Bierman, H. S., & Fernandez, L. F. (1998). Game theory with economic applications . Addison-Wesley.
Camerer, C. F. (2011). Behavioral game theory: Experiments in strategic interaction . Princeton University Press.
Carmona, G., & Podczeck, K. (2009). On the existence of pure-strategy equilibria in large games. Journal of Economic Theory, 144 (3), 1300–1319.
Chess, D. M. (1988). Simulating the evolution of behavior: The iterated prisoners’ dilemma problem. Complex Systems, 2 (6), 663–670.
Collins, R. W. (2022). The Prisoner’s Dilemma paradox: Rationality, morality, and reciprocity. Think, 21 (61), 45–55.
Dutta, P. K. (1999). Strategies and games: Theory and practice . MIT Press.
Fisher, R. A. (1930). The genetical theory of natural selection . Clarendon Press.
Book Google Scholar
Harsanyi, J. C. (1973). Oddness of the number of equilibrium points: A new proof. International Journal of Game Theory, 2 (1), 235–250.
Hew, S. L., & White, L. B. (2008). Cooperative resource allocation games in shared networks: Symmetric and asymmetric fair bargaining models. IEEE Transactions on Wireless Communications, 7 (11), 4166–4175.
Kreps, D. M. (1987). Nash equilibrium. In The new Palgrave dictionary of economics . Palgrave Macmillan.
Marwala, T. (2013). Multi-agent approaches to economic modeling: Game theory, ensembles, evolution and the stock market. In Economic modeling using artificial intelligence methods (pp. 195–213). Springer.
Marwala, T., & Hurwitz, E. (2017). Game theory. In Artificial intelligence and economic theory: Skynet in the market (pp. 75–88). Springer.
Munoz-Garcia, F., & Toro-Gonzalez, D. (2019). Pure strategy Nash equilibrium and simultaneous-move games with complete information. In Strategy and game theory: Practice exercises with answers (pp. 39–86). Springer.
Nash, J. F., Jr. (1950). Equilibrium points in n-person games. Proceedings of the National Academy of Sciences, 36 (1), 48–49.
Olson, E. S. (2010). Zero-sum game: The rise of the world’s largest derivatives exchange . Wiley.
Poundstone, W. (1993). Prisoner’s dilemma: John von Neumann, game theory, and the puzzle of the bomb . Anchor.
Rapoport, A., & Chammah, A. M. (1966). The game of chicken. American Behavioral Scientist, 10 (3), 10–28.
Rapoport, A., Chammah, A. M., & Orwant, C. J. (1965). Prisoner’s dilemma: A study in conflict and cooperation (Vol. 165). University of Michigan Press.
Schneider, M., & Shields, T. (2022). Motives for cooperation in the one-shot Prisoner’s Dilemma. Journal of Behavioral Finance, 23 (4), 438–456.
Sun, C. H. (2020). Simultaneous and sequential choice in a symmetric two-player game with canyon-shaped payoffs. The Japanese Economic Review, 71 (2), 191–219.
Washburn, A. (2014). Single person background. Two-Person Zero-Sum Games , 1–4.
Wilson, R. (1971). Computing equilibria of n-person games. SIAM Journal on Applied Mathematics, 21 (1), 80–87.
Download references
Author information
Authors and affiliations.
United Nations University, Tokyo, Japan
Tshilidzi Marwala
You can also search for this author in PubMed Google Scholar
Corresponding author
Correspondence to Tshilidzi Marwala .
Rights and permissions
Reprints and permissions
Copyright information
© 2023 The Author(s), under exclusive license to Springer Nature Singapore Pte Ltd.
About this chapter
Marwala, T. (2023). Game Theory in Politics. In: Artificial Intelligence, Game Theory and Mechanism Design in Politics. Palgrave Macmillan, Singapore. https://doi.org/10.1007/978-981-99-5103-1_2
Download citation
DOI : https://doi.org/10.1007/978-981-99-5103-1_2
Published : 05 August 2023
Publisher Name : Palgrave Macmillan, Singapore
Print ISBN : 978-981-99-5102-4
Online ISBN : 978-981-99-5103-1
eBook Packages : Political Science and International Studies Political Science and International Studies (R0)
Share this chapter
Anyone you share the following link with will be able to read this content:
Sorry, a shareable link is not currently available for this article.
Provided by the Springer Nature SharedIt content-sharing initiative
- Publish with us
Policies and ethics
- Find a journal
- Track your research
Grab your spot at the free arXiv Accessibility Forum
Help | Advanced Search
Computer Science > Computer Science and Game Theory
Title: a case study of agent-based models for evolutionary game theory.
Abstract: Evolutionary game theory is a mathematical toolkit to analyse the interactions that an individual agent has in a population and how the composition of strategies in this population evolves over time. While it can provide neat solutions to simple problems, in more complicated situations where assumptions such as infinite population size may be relaxed, deriving analytic solutions can be intractable. In this short paper, we present a game with complex interactions and examine how an agent-based model may be used as a heuristic technique to find evolutionarily stable states.
Comments: | 6 pages, 1 figure, accepted to Conference of Social Simulation 2021 |
Subjects: | Computer Science and Game Theory (cs.GT); Multiagent Systems (cs.MA) |
Cite as: | [cs.GT] |
(or [cs.GT] for this version) | |
Focus to learn more arXiv-issued DOI via DataCite |
Submission history
Access paper:.
- Other Formats

References & Citations
- Google Scholar
- Semantic Scholar
DBLP - CS Bibliography
Bibtex formatted citation.

Bibliographic and Citation Tools
Code, data and media associated with this article, recommenders and search tools.
- Institution
arXivLabs: experimental projects with community collaborators
arXivLabs is a framework that allows collaborators to develop and share new arXiv features directly on our website.
Both individuals and organizations that work with arXivLabs have embraced and accepted our values of openness, community, excellence, and user data privacy. arXiv is committed to these values and only works with partners that adhere to them.
Have an idea for a project that will add value for arXiv's community? Learn more about arXivLabs .
Academia.edu no longer supports Internet Explorer.
To browse Academia.edu and the wider internet faster and more securely, please take a few seconds to upgrade your browser .
Enter the email address you signed up with and we'll email you a reset link.
- We're Hiring!
- Help Center
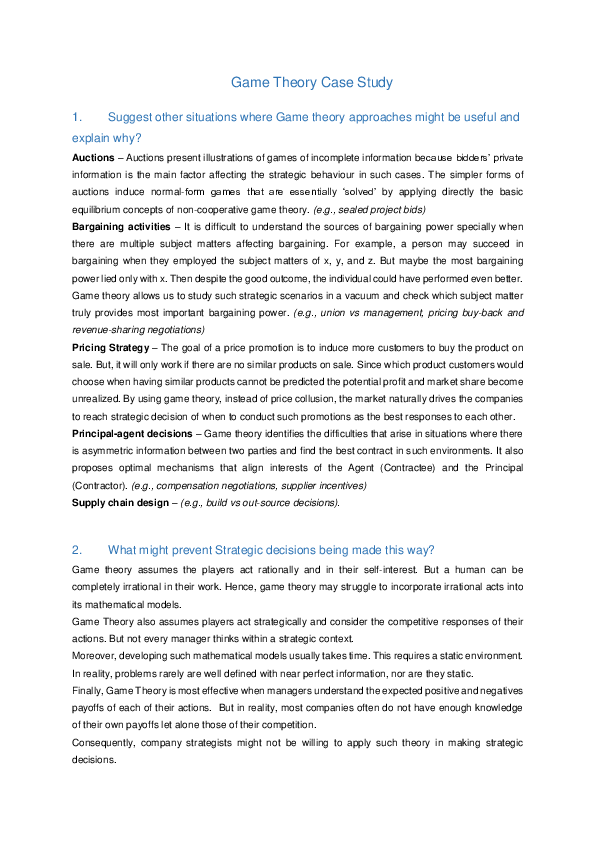
Game Theory Case Study

Related Papers
Christof Weinhardt
From 12.11.06 to 17.11.06, the Dagstuhl Seminar 06461 ``Negotiation and Market Engineering'' was held in the International Conference and Research Center (IBFI), Schloss Dagstuhl. During the seminar, several participants presented their current research, and ongoing work and open problems were discussed. Abstracts of the presentations given during the seminar as well as abstracts of seminar results and ideas are put together in this paper. The first section describes the seminar topics and goals in general. Links to extended abstracts or full papers are provided, if available.
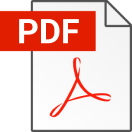
IOP Conference Series Materials Science and Engineering
Hubert Anysz
The process of negotiation can be analyzed considering some scientific issues (e.g. psychological, sociological). When a construction subcontract is negotiated, as it is business activity, the rational choices should be considered more than other factors influencing the decisions of the negotiating parties. A game theory supports rational decision making based on calculations, expected values and strategic choices. An experiment was carried with the participation of the students of Civil Engineering Faculty. Five different games were prepared. The students started playing with the "nature". Then the probabilities of the "nature" strategies were introduced. Finally, they played three one-on-one sets of repetitive games. The results prove that knowing the needs of the opposite player (knowing his/her possible strategies) leads to a win-win result where the score of both players is maximized. The experiment and its result are analyzed in the paper. The multiple negotiations, and then multiple cooperation of a general contractor with the same subcontractor on construction projects is an often case. These parties discuss the rules of their cooperation formalized in subcontracts several times. The typical image of a general contractor's need is a low price given by a subcontractor. In fact, it the is only one of the needs. Moreover, it is not a rare case where flexibility of engagement on a building site or a high number of equipment units or favorable terms of payment are desired by a general contractor more than the low price given by a subcontractor. A subcontractor's standing can be different too. They can search for profitable cooperation or they have to provide work for some brigades not to have them unengaged. They can afford long term payment or they have to be paid in advance. The experiment proves that disclosure of the needs (strategies) by negotiating parties leads to achievement of higher gain for both of them (when the game is repetitive). The paper sets the basis for modelling negotiations of a construction subcontract based on a game theory. 1. Introduction Scientific approaches to the negotiation processes (psychological, sociological) and a theory of rational choices-a game theory-were opposed to proposed way of solving conflicts presented in the book [1] gave inspiration to this article. The experiment based on [2], [3], [4] (set of the games and descriptions to them) was prepared to illustrate the students-the experiment participants-that the information about possible strategies of opposite player (negotiating party) can improve a lot the score achieved by both parties. In order to achieve top result in a final game, players were required to choose also the strategies where in a simple attempt they lost. Review of modern application of a game theory has shown the wide spectrum of its possible use [5] supporting decision making. Models based on the game theory were
Lecture Notes in Business Information Processing
In this dissertation, we pay a particular attention to the application of game theory in three multi-agent negotiation problems: minimum cost spanning tree, data envelopment analysis (DEA), and partner selection in airline alliances, where the first two problems assume for an abstract agent. In Chapter 2, we characterize the decentralized rule in the minimum cost spanning tree problem, which is introduced by Feltkamp et al. [1994b]. In Chapter 3, we first improve the DEA game in Nakabayashi and Tone [2006] by proposing an alternative scheme and then focus on analyzing the solutions by our new game theoretic approaches to weight assignments in DEA problems. Lastly, the main concern in Chapter 4 is how the service quality might affect an airline’s decision making in the selection of its partner during the formation of airline alliances.
IOP Conference Series: Materials Science and Engineering
Dan Savescu
Paper presents some aspects regarding negotiation process used in technological transfer, negotiations strategies, partners orientation in negotiation time, WIN-WIN, or WIN-LOOSE, or LOOSE-LOOSE position, tactics and technics used in negotiation.
Alejandro Jofre
Supply Chain
Pierpaolo Pontrandolfo
Wojciech Zaluski
Proceedings of IACCM International Academic Symposiums on Contract & Commercial, Edited by D Lowe, ISBN 978-1-934697-01-6, pp 25-44
David J Lowe
It is widely acknowledged that the ability to clearly articulate a profession’s knowledge base is crucial when defining a discrete professional group. As with project management and supply chain management, the knowledge based of the commercial and contract management (C&CM) function is an amalgam of theories, concepts and models drawn from the research and emerging practice of a variety of academic disciplines. To investigate the interrelationship between and relevance of these theories and concepts to the C&CM function/profession, and the general level of awareness of them among practitioners, a global survey of the IACCM’s membership was conducted in October 2007. The results of the survey presented in this paper reveal that the most familiar theories, concepts and models were: leadership, best value/value for money, competitive advantage, governance, strategy, globalisation, core competence, commitment, creativity, and negotiation theory. The academic disciplines associated with the most familiar 25 items were: organisational behaviour, strategic management, economics, marketing, law, operations management and accountancy. Overall, the results indicate a high degree of homogeneity in the respondent’s familiarity with the items irrespective of their location or prior level of education. Significant differences were, however, established in 33 of the items between subgroups based on the function of the C&CM practitioner. Generally, these differences could have been predicted, for example, C&CM practitioners supporting procurement were found to be more familiar with procurement theory, strategic purchasing, supply chains, supplier segmentation and the theory of incentives etc. compare to those practitioners who support sales or both sales and procurement. In terms of the relevance of the theories, concepts and models to the C&CM function/profession, negotiation analysis, problem-solving interest-based negotiation, negotiation theory, best value/value for money, trust, competitive advantage, and contract law model were considered to be highly relevant. The academic disciplines associated with the most relevant 25 items were: organisational behaviour, strategic management, operations management, economics, marketing, law, and operational research.
Eirik Romstad
Loading Preview
Sorry, preview is currently unavailable. You can download the paper by clicking the button above.
RELATED PAPERS
Journal of Education, Humanities and Social Sciences
Project Management Journal
jaakko kujala
Journal of Economic Behavior & Organization
Antonio Cabrales
LEONIDAS ANTHOPOULOS
Impact of Sustainability on Project Evaluation
Herbert Robinson
teresa sharpe
Negotiation Journal
James Sebenius
Asian Journal of Management Cases
Cyril Joyce Fernandez
Amin Shalchi
MOHAMMED AUDU
Dmitry Ignatov
Construction Management and Economics
Mieke Hoezen , Hans Voordijk
Ren-Jye Dzeng
lewis kornhauser
AARF Publications Journals
IFIP Advances in Information and Communication Technology
Jose Fonseca
William Samuelson
Tomasz Małkus
David M Boje
Technological and Economic Development of Economy
Jolanta Tamosaitiene
Innovative Issues and Approaches in Social Sciences
Bozidar lenarčič
International Journal of Project Management
Abdul Rauf Abdul Karim
RELATED TOPICS
- We're Hiring!
- Help Center
- Find new research papers in:
- Health Sciences
- Earth Sciences
- Cognitive Science
- Mathematics
- Computer Science
- Academia ©2024
Information
- Author Services
Initiatives
You are accessing a machine-readable page. In order to be human-readable, please install an RSS reader.
All articles published by MDPI are made immediately available worldwide under an open access license. No special permission is required to reuse all or part of the article published by MDPI, including figures and tables. For articles published under an open access Creative Common CC BY license, any part of the article may be reused without permission provided that the original article is clearly cited. For more information, please refer to https://www.mdpi.com/openaccess .
Feature papers represent the most advanced research with significant potential for high impact in the field. A Feature Paper should be a substantial original Article that involves several techniques or approaches, provides an outlook for future research directions and describes possible research applications.
Feature papers are submitted upon individual invitation or recommendation by the scientific editors and must receive positive feedback from the reviewers.
Editor’s Choice articles are based on recommendations by the scientific editors of MDPI journals from around the world. Editors select a small number of articles recently published in the journal that they believe will be particularly interesting to readers, or important in the respective research area. The aim is to provide a snapshot of some of the most exciting work published in the various research areas of the journal.
Original Submission Date Received: .
- Active Journals
- Find a Journal
- Proceedings Series
- For Authors
- For Reviewers
- For Editors
- For Librarians
- For Publishers
- For Societies
- For Conference Organizers
- Open Access Policy
- Institutional Open Access Program
- Special Issues Guidelines
- Editorial Process
- Research and Publication Ethics
- Article Processing Charges
- Testimonials
- Preprints.org
- SciProfiles
- Encyclopedia
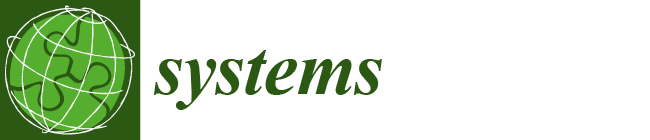
Article Menu
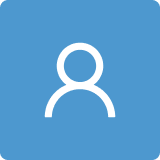
- Subscribe SciFeed
- Recommended Articles
- Google Scholar
- on Google Scholar
- Table of Contents
Find support for a specific problem in the support section of our website.
Please let us know what you think of our products and services.
Visit our dedicated information section to learn more about MDPI.
JSmol Viewer
A pricing model study of shared parking area charge based on game theory.
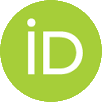
1. Introduction
2. materials and methods, 2.1. parking pricing tripartite participant gaming relationships, 2.2. decision-making behavior of parking lot participants, 2.2.1. a model of travelers’ parking behavioral choices, 2.2.2. shared parking operating platform revenue model, 2.2.3. shared parking operating platform revenue model, 2.3. shared parking charge pricing model, 2.3.1. a multi-tier pricing model in shared parking domains based on game theory, 2.3.2. determination of weights, 2.3.3. determination of parameters, 2.3.4. model solution, 3. case study, 3.1. parameter input, 3.2. comparison and analysis, 3.2.1. programmatic comparison, 3.2.2. weighting calculations, 3.2.3. model solution, 3.2.4. analysis of results, 4. conclusions, author contributions, data availability statement, conflicts of interest.
- He, K.; Ren, G. Travel behavior choice under the condition of dynamic reservation parking pricing. J. Transp. Eng. Inf. 2020 , 18 , 53–60. [ Google Scholar ]
- Li, H.; Ning, X.; Yu, L.; Chen, H. Combined flexible working arrangements and time-dependent parking charges. J. Transp. Syst. Eng. Inf. Technol. 2020 , 20 , 1–7. [ Google Scholar ]
- Hensher, D.A.; King, J. Parking demand and responsiveness to supply, pricing and location in the Sydney central business district. Transp. Res. Part A Policy Pract. 2001 , 35 , 177–196. [ Google Scholar ] [ CrossRef ]
- Xu, H.; Yu, J.; Xu, W.; Zhang, K. Charging strategy of park-and-ride facilities with consideration to ride-sharing incentive and heterogeneous cost-sharing proportion. Syst. Eng.-Theory Pract. 2023 , 43 , 1220–1231. [ Google Scholar ]
- Hu, X.; Li, C.; An, S. Pricing model of urban parking project in public-private partnership mode considering interests of multiple participants. J. Transp. Eng. Inf. 2023 , 21 , 113–124. [ Google Scholar ]
- Proulx, F.R.; Cavagnolo, B.; Torres-Montoya, M. Impact of parking prices and transit fares on mode choice at the university of California, Berkeley. Transp. Res. Rec. J. Transp. Res. Board 2014 , 2469 , 41–48. [ Google Scholar ] [ CrossRef ]
- Wang, J.; Jin, S.; Li, W.; Chen, F. Parking pricing method of public parking lots based on multi-aspect comprehensive benefit maximization. J. Traffic Transp. Eng. 2017 , 17 , 126–135. [ Google Scholar ]
- Tsai, J.F.; Chu, C.-P. Economic analysis of collecting parking fees by a private firm. Transp. Res. Part A Policy Pract. 2006 , 40 , 690–697. [ Google Scholar ] [ CrossRef ]
- Qian, Z.; Xiao, F.; Zhang, H.M. The economics of parking provision for the morning commute. Transp. Res. Part A Policy Pract. 2011 , 45 , 861–879. [ Google Scholar ] [ CrossRef ]
- Qian, Z.; Xiao, F.; Zhang, H.M. Managing morning commute traffic with parking. Transp. Res. Part B Methodol. 2012 , 46 , 894–916. [ Google Scholar ] [ CrossRef ]
- Zhu, C.; Jia, B.; Han, L. Parking space allocation and pricing based on Stackelberg game. J. Transp. Syst. Eng. Inf. Technol. 2015 , 15 , 19–24. [ Google Scholar ]
- Daniel, M.; Bai, Y.; Ouyang, Y. Parking space management via dynamic performance-based pricing. Transp. Res. Procedia 2015 , 7 , 170–191. [ Google Scholar ]
- Zong, F.; He, Y.; Yuan, Y. Dependence of parking pricing on land use and time of day. Sustainability 2015 , 7 , 9587–9607. [ Google Scholar ] [ CrossRef ]
- Wang, Y.; Jia, S.; Li, J.; Wei, R. A model of network assignment for multi-mode travel considering roadside parking charge game. J. Transp. Inf. Saf. 2019 , 37 , 88–94. [ Google Scholar ]
- Sowmya, K.; Dhabu, M.M. Multistage game model based dynamic pricing for car parking slot to control congestion. Sustainability 2022 , 14 , 11808. [ Google Scholar ] [ CrossRef ]
- Liu, Z.; Xu, C.; Chen, Y. A study on data pricing model using utility method. Price Theory Pract. 2022 , 11 , 164–167. [ Google Scholar ]
- Shao, Z. Game theory application in traffic management. Road Traffic Saf. 2006 , 6 , 8–10. [ Google Scholar ]
- Zahra, J.; Amirhossein, A.; Philippe, C.; Khoo, M.B.C. Monitoring the coefficient of variation: A literature review. Comput. Ind. Eng. 2021 , 161 , 107600. [ Google Scholar ]
- Kui, H.; Wang, J.; Wang, Y.; E, W.; Gao, L. Vehicle fuel consumption model based on urban road operations. J. Jilin Univ. Eng. Technol. Ed. 2009 , 39 , 1146–1150. [ Google Scholar ]
- Zong, F.; Juan, Z.; Zhang, H.; Jia, H. Calculation and application of value of travel time. J. Transp. Syst. Eng. Inf. Technol. 2009 , 9 , 114–119. [ Google Scholar ]
- Zhou, Z. Study on Functional Design and Key Models of Urban Parking Guidance Information System. Ph.D. Thesis, Southeast University, Nanjing, China, 2004. [ Google Scholar ]
- Feng, H.; Zhu, C. Model of curb parking pricing in urban center district of China. J. Transp. Syst. Eng. Inf. Technol. 2008 , 8 , 129–135. [ Google Scholar ]
- Sun, C.; Zhang, P.; Shi, Y.; Chang, Y. Sensor location strategy and scaling rate inference for origin-destination demand estimation. IEEE Trans. Intell. Transp. Syst. 2021 , 22 , 3455–3467. [ Google Scholar ] [ CrossRef ]
- Sun, C.; Chu, Z.; Zhang, P.; Chang, Y. Day-to-day traffic user equilibrium model considering influence of intelligent highways and advanced traveler information systems. J. Cent. South Univ. 2022 , 29 , 1376–1388. [ Google Scholar ] [ CrossRef ]
- An, S.; Ma, T.; Yin, J. Parking fee pricing model for urban cities in China. J. Harbin Inst. Technol. 2000 , 32 , 65–69. [ Google Scholar ]
- Shin, J.H.; Jun, H.B.; Kim, J.G. Dynamic control of intelligent parking guidance using neural network predictive control. Comput. Ind. Eng. 2018 , 120 , 15–30. [ Google Scholar ] [ CrossRef ]
Click here to enlarge figure
Parameters | Define |
---|---|
Operating costs per unit of time for shared berths in shared parking lots ($/h) | |
Parking fees per time interval | |
Shared parking fees for parking lot z in the parking domain | |
Unit cost of driving | |
Unit fuel consumption cost | |
Unit cost of walking | |
Average walking speed | |
Average travel speed | |
Fuel price ($/L) | |
Number of shared parking spaces in shared parking lot z | |
Distance from shared parking lot z to destination des | |
Average parking time for parking users | |
Actual traffic on section d (pcu/h) | |
Actual capacity of road section d (pcu/h) | |
Parking costs for parking users | |
Shared parking operating platform profit | |
Management utility within a shared parking system | |
Deviation of the objective values of parking costs, parking operating platform profit, and management utility | |
Upper and lower limits for optimal parking saturation in shared parking lots | |
Upper and lower limits of optimal road saturation | |
Parking user travel time from starting point O to shared parking lot z(h) | |
Time through intersection (h) | |
Travel time on road segment (h) | |
Parking user seek time in shared parking lot z (h) | |
Walking time of parking users from shared parking lot z to destination des (h) | |
Number of parking requests | |
Number of parking users in shared parking lot z |
Section Number | Road Traffic Volume (pcu/h) | Roadway Free Travel Time (s) | Mobility (pcu/h) | Section Number | Road Traffic Volume (pcu/h) | Roadway Free Travel Time (s) | Mobility (pcu/h) |
---|---|---|---|---|---|---|---|
0–1 | 432 | 32.1 | 1800 | 3–6 | 340 | 52.2 | 700 |
1–2 | 854 | 24.2 | 900 | 4–7 | 1221 | 51.3 | 1700 |
1–3 | 142 | 61.4 | 700 | 5–8 | 690 | 62.1 | 1600 |
2–4 | 1221 | 32.8 | 1700 | 6–7 | 520 | 61.2 | 1300 |
4–3 | 352 | 42.9 | 1300 | 8–B | 120 | 20.3 | 700 |
4–5 | 540 | 36.7 | 1300 |
Intersection Number | Signal Cycle Length | Direction of Traffic Flow | Green Signal Ratio | Intersection Number | Signal Cycle Length | Direction of Traffic Flow | Green Signal Ratio |
---|---|---|---|---|---|---|---|
1 | —— | —— | —— | 5 | —— | —— | —— |
2 | 140 | north and south | Straight 0.2 | 6 | 70 | north and south | 0.6 |
Left 0.2 | east and west | 0.4 | |||||
east and west | Straight 0.25 | 7 | 110 | north and south | 0.3 | ||
Left 0.2 | east and west | Straight 0.4 | |||||
3 | —— | —— | —— | Left 0.3 | |||
4 | 110 | north and south | 0.2 | 8 | 80 | north and south | 0.3 |
east and west | Straight 0.4 | east and west | 0.6 | ||||
Left 0.35 |
Trails | Nodal | Trails | Nodal | Trails | Nodal | Trails | Nodal |
---|---|---|---|---|---|---|---|
1 | O1367D | 3 | O1247D | 5 | O13458D | 7 | O2458D |
2 | O1347D | 4 | O247D | 6 | O12458D |
Serial Number | Number of Shared Berths | Free-Flow Down-Stream Parking Search Time | Walking Time | Shared Berth Operating Costs | Average Parking Time |
---|---|---|---|---|---|
Shared parking lot A | 300 | 0.2 | 0.15 | 0.35 | 6.0 |
Shared parking lot B | 250 | 0.1 | 0.05 | 0.5 | 5.8 |
Parameter Name | Parameter Value | Parameter Name | Parameter Value |
---|---|---|---|
Fuel Costs for Parking User Driving (CNY/h) | 28 | Upper limit of optimal road saturation | 0.6 |
Value cost of time for parking users (CNY/h) | 22 | Lower limit of optimal road saturation | 0.4 |
Maximum fee rate for shared parking (CNY/h) | 9 | Upper limit of optimal parking saturation | 0.8 |
Lower limit of fee rate for shared parking (CNY/h) | 1 | Lower limit of optimal parking saturation | 0.7 |
Standard Deviation | Average Value | Coefficient of Variation | Weights | |
---|---|---|---|---|
Travel Costs | 1927.1578 | 13,552.5867 | 0.1422 | 0.1719 |
Total Operating Profit | 301.5099 | 912.6079 | 0.3304 | 0.3994 |
Parking Saturation | 0.1569 | 0.4946 | 0.3173 | 0.3836 |
Road Saturation | 0.0586 | 1.5673 | 0.0374 | 0.0452 |
Programmatic | Shared Parking | Charge Rate (CNY) | Parking Requirements (Vehicles) | Deviation | Travel Costs (CNY) | Operating Profit (CNY) | Parking Saturation Difference | Difference in Road Saturation |
---|---|---|---|---|---|---|---|---|
No. 1 | 1 | 145 | 1.7504 | 7038.3 | −126.9 | 0.2886 | 1.5059 | |
No. 2 | 1 | 155 | ||||||
No. 1 | 9 | 145 | 0.8982 | 21,031.5 | 2265.1 | 0.2886 | 1.5059 | |
No. 2 | 9 | 155 | ||||||
No. 1 | 2.7 | 160 | 1.4998 | 10,443.9 | 453.7 | 0.2457 | 1.4847 | |
No. 2 | 3.2 | 140 | ||||||
No. 1 | 1 | 163 | 1.6789 | 7551.4 | −41.9 | 0.2521 | 1.4827 | |
No. 2 | 1.6 | 137 | ||||||
Reference [ ] | No. 1 | 8.6 | 153 | 0.8831 | 20,317.3 | 2235.6 | 0.2697 | 1.4931 |
No. 2 | 8.9 | 147 | ||||||
Minimum deviation | No. 1 | 8.5 | 160 | 0.8155 | 20,589.0 | 2187.9 | 0.2457 | 1.4847 |
No. 2 | 9 | 140 |
The statements, opinions and data contained in all publications are solely those of the individual author(s) and contributor(s) and not of MDPI and/or the editor(s). MDPI and/or the editor(s) disclaim responsibility for any injury to people or property resulting from any ideas, methods, instructions or products referred to in the content. |
Share and Cite
Sun, C.; Jing, H.; Yin, H. A Pricing Model Study of Shared Parking Area Charge Based on Game Theory. Systems 2024 , 12 , 269. https://doi.org/10.3390/systems12080269
Sun C, Jing H, Yin H. A Pricing Model Study of Shared Parking Area Charge Based on Game Theory. Systems . 2024; 12(8):269. https://doi.org/10.3390/systems12080269
Sun, Chao, Haodong Jing, and Haowei Yin. 2024. "A Pricing Model Study of Shared Parking Area Charge Based on Game Theory" Systems 12, no. 8: 269. https://doi.org/10.3390/systems12080269
Article Metrics
Article access statistics, further information, mdpi initiatives, follow mdpi.
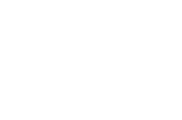
Subscribe to receive issue release notifications and newsletters from MDPI journals
- Election 2024
- Entertainment
- Newsletters
- Photography
- AP Buyline Personal Finance
- AP Buyline Shopping
- Press Releases
- Israel-Hamas War
- Russia-Ukraine War
- Global elections
- Asia Pacific
- Latin America
- Middle East
- Delegate Tracker
- AP & Elections
- 2024 Paris Olympic Games
- Auto Racing
- Movie reviews
- Book reviews
- Financial Markets
- Business Highlights
- Financial wellness
- Artificial Intelligence
- Social Media
FACT FOCUS: A look at false claims around Kamala Harris and her campaign for the White House
Democrats are quickly rallying around Vice President Kamala Harris as their likely presidential nominee after President Joe Biden’s ground-shaking decision to bow out of the 2024 race.
Vice President Kamala Harris arrives to speak from the South Lawn of the White House in Washington, Monday, July 22, 2024, during an event with NCAA college athletes. This is her first public appearance since President Joe Biden endorsed her to be the next presidential nominee of the Democratic Party. (AP Photo/Alex Brandon)
- Copy Link copied
The announcement that Vice President Kamala Harris will seek the Democratic nomination for president is inspiring a wave of false claims about her eligibility and her background. Some first emerged years ago, while others only surfaced after President Joe Biden’s decision to end his bid for a second term.
Here’s a look at the facts.
CLAIM: Harris is not an American citizen and therefore cannot serve as commander in chief.
THE FACTS: Completely false . Harris is a natural born U.S. citizen. She was born on Oct. 20, 1964, in Oakland, California, according to a copy of her birth certificate, obtained by The Associated Press.
Her mother, a cancer researcher from India, and her father, an economist from Jamaica, met as graduate students at the University of California, Berkeley.
Under the 14th Amendment to the Constitution, anyone born on U.S. soil is considered a natural born U.S. citizen and eligible to serve as either the vice president or president.
“All persons born or naturalized in the United States, and subject to the jurisdiction thereof, are citizens of the United States and of the State wherein they reside,” reads the amendment.
There is no question or legitimate debate about whether a citizen like Harris is eligible to serve as president or vice president, said Jessica Levinson, a professor at Loyola Law School.
“So many legal questions are really nuanced — this isn’t one of those situations,” Levinson told the AP on Monday.
Still, social media posts making the debunked assertion that Harris cannot serve as president went viral soon after Biden announced Sunday that he was dropping out of the race and would back Harris for president.
“Kamala Harris is not eligible to run for President,” read one post on X that was liked more than 34,000 times. “Neither of her parents were natural born American citizens when she was born.”
False assertions about Harris’ eligibility began circulating in 2019 when she launched her bid for the presidency. They got a boost, thanks in part to then-President Donald Trump, when Biden selected her as his running mate.
“I heard today that she doesn’t meet the requirements,” the Republican said of Harris in 2019.
CLAIM: Harris is not Black.
THE FACTS: This is false. Harris is Black and Indian . Her father, Donald Harris, is a Black man who was born in Jamaica. Shyamala Gopalan, her mother, was born in southern India. Harris has spoken publicly for many years, including in her 2019 autobiography , about how she identifies with the heritage of both her parents.
What to know about the 2024 Election
- Democracy: American democracy has overcome big stress tests since 2020. More challenges lie ahead in 2024.
- AP’s Role: The Associated Press is the most trusted source of information on election night, with a history of accuracy dating to 1848. Learn more.
- Stay informed. Keep your pulse on the news with breaking news email alerts. Sign up here .
Despite ample evidence to the contrary, social media users are making erroneous claims about Harris’ race.
“Just a reminder that Kamala Harris @KamalaHarris isn’t black,” reads one X post that had received approximately 42,000 likes and 20,400 shares as of Monday. “She Indian American. She pretends to be black as part of the delusional, Democrat DEI quota.”
But Harris is both Black and Indian. Indeed, she is the first woman, Black person and person of South Asian descent to serve as vice president. This fact is highlighted in her biography on WhiteHouse.gov and she has spoken about her ethnicity on many occasions.
Harris wrote in her autobiography, “The Truths We Hold: An American Journey,” that she identifies with the heritage of both her mother and father.
“My mother, grandparents, aunts, and uncle instilled us with pride in our South Asian roots,” she wrote. “Our classical Indian names harked back to our heritage, and we were raised with a strong awareness and appreciation for Indian culture.”
In the next paragraph, she adds, “My mother understood very well that she was raising two black daughters.” Harris again refers to herself as a “black woman” in the book’s next chapter.
CLAIM: Harris got her start by having an affair with a married man, California politician Willie Brown.
THE FACTS: This is missing some important context. Brown was separated from his wife during the relationship, which was not a secret.
Brown, 90, is a former mayor of San Francisco who was serving as speaker of the California State Assembly in the 1990s when he and Harris were in a relationship. Brown had separated from his wife in 1982.
“Yes, we dated. It was more than 20 years ago,” Brown wrote in 2020 in the San Francisco Chronicle under the article title, “Sure, I dated Kamala Harris. So what?”
He wrote that he supported Harris’ first race to be San Francisco district attorney — just as he has supported a long list of other California politicians, including former House Speaker Nancy Pelosi, former Sen. Dianne Feinstein and Gov. Gavin Newsom.
Harris, 59, was state attorney general from 2011-2017 and served in the Senate from 2017 until 2021, when she became vice president. She has been married to Doug Emhoff since 2014.
Harris’ critics have used the past relationship to question her qualifications, as Fox News personality Tomi Lahren did when she wrote on social media in 2019: “Kamala did you fight for ideals or did you sleep your way to the top with Willie Brown.” Lahren later apologized for the comment.
Trump and some of his supporters have also highlighted the nearly three-decade old relationship in recent attacks on Harris .
CLAIM: An Inside Edition clip of television host Montel Williams holding hands with Harris and another woman is proof that Harris was his “side piece.”
THE FACTS: The clip shows Montel with Harris and his daughter, Ashley Williams. Harris and Williams, a former marine who hosted “The Montel Williams Show” for more than a decade, dated briefly in the early 2000s.
In the clip, taken from a 2019 Inside Edition segment , Williams can be seen posing for photographs and holding hands with both women as they arrive at the 2001 Eighth Annual Race to Erase MS in Los Angeles.
But social media users are misrepresenting the clip, using it as alleged evidence that Harris was Montel’s “side piece” — a term used to describe a person, typically a woman, who has a sexual relationship with a man in a monogamous relationship.
Williams addressed the false claims in an X post on Monday, writing in reference to the Inside Edition clip, “as most of you know, that is my daughter to my right.” Getty Images photos from the Los Angeles gala identify the women as Harris and Ashley Williams.
In 2019, Williams described his relationship with Harris in a post on X, then known as Twitter.
“@KamalaHarris and I briefly dated about 20 years ago when we were both single,” he wrote in an X post at the time. “So what? I have great respect for Sen. Harris. I have to wonder if the same stories about her dating history would have been written if she were a male candidate?”
CLAIM: Harris promised to inflict the “vengeance of a nation” on Trump supporters.
THE FACTS: A fabricated quote attributed to Harris is spreading online five years after it first surfaced.
In the quote, Harris supposedly promises that if Trump is defeated in 2020, Trump supporters will be targeted by the federal government: “Once Trump’s gone and we have regained our rightful place in the White House, look out if you supported him and endorsed his actions, because we’ll be coming for you next. You will feel the vengeance of a nation.”
The quote was shared again on social media this week. One post on X containing an image of the quote was shared more than 22,000 times as of Monday afternoon.
The remarks didn’t come from Harris , but from a satirical article published online in August 2019. Shortly after, Trump supporters like musician Ted Nugent reposted the comments without noting they were fake.
CLAIM: A video shows Harris saying in a speech: “Today is today. And yesterday was today yesterday. Tomorrow will be today tomorrow. So live today, so the future today will be as the past today as it is tomorrow.”
THE FACTS: Harris never said this. Footage from a 2023 rally on reproductive rights at Howard University, her alma mater, was altered to make it seem as though she did.
In the days after Harris headlined the Washington rally, Republicans mocked a real clip of her speech, with one critic dubbing her remarks a “word salad,” the AP reported at the time .
Harris says in the clip: “So I think it’s very important — as you have heard from so many incredible leaders — for us, at every moment in time, and certainly this one, to see the moment in time in which we exist and are present, and to be able to contextualize it, to understand where we exist in the history and in the moment as it relates not only to the past, but the future.”
NARAL Pro-Choice America, an abortion rights nonprofit whose president also spoke at the rally, livestreamed the original footage. It shows Harris making the “moment in time” remark, but not the “today is today” comment.
The White House’s transcript of Harris’ remarks also does not include the statement from the altered video. Harris’ appearance at the event came the same day that Biden announced their reelection bid .
Find AP Fact Checks here: https://apnews.com/APFactCheck .
Improving Generalization in Game Agents with Data Augmentation in Imitation Learning
This presentation was delivered at the IEEE World Congress on Computational Intelligence , which was held on 5 July 2024 in Yokohama, Japan.
Authors: Derek Yadgaroff, Alessandro Sestini, Konrad Tollmar, Ayça Özçelikkale, and Linus Gisslén. Presented by Alessandro Sestini.
How do we efficiently train in-game AI agents to handle new situations that they haven’t been trained on?
Imitation learning is an effective approach for training game-playing agents and, consequently, for efficient game production. However, generalization – the ability to perform well in related but unseen scenarios – is an essential requirement that remains an unsolved challenge for game AI. Generalization is difficult for imitation learning agents because it requires the algorithm to take meaningful actions outside of the training distribution.
In this paper, we propose a solution to this challenge. Inspired by the success of data augmentation in supervised learning, we augment the training data so the distribution of states and actions in the dataset better represents the real state-action distribution.
This study evaluates methods for combining and applying data augmentations to improve the generalization of imitation learning agents. It also provides a performance benchmark of these augmentations across several 3D environments. These results demonstrate that data augmentation is a promising framework for improving generalization in imitation learning agents.
Download the research paper (PDF 1.6 MB).
Download the slide deck (PDF 10 MB).
Related News
Ea presents at siggraph 2024 with seed, frostbite, and ea sports., towards optimal training distribution for photo-to-face models, incorporating ml research into audio production: exflowsions case study.
- Share full article
For more audio journalism and storytelling, download New York Times Audio , a new iOS app available for news subscribers.

- Apple Podcasts
- Google Podcasts
The Sunday Read: ‘The Kidnapping I Can’t Escape’
Fifty years ago, her father’s friend was taken at gunpoint on long island. then he went on with his life — and that’s the part that haunts her..

By Taffy Brodesser-Akner
Read by Gabra Zackman
Produced by Adrienne Hurst and Aaron Esposito
Narration produced by Krish Seenivasan
Edited by John Woo
Original music by Aaron Esposito
Engineered by Marion Lozano and Ted Blaisdell
Listen and follow The Daily Apple Podcasts | Spotify | Amazon Music | YouTube
On Nov. 12, 1974, Taffy Brodesser-Akner’s father’s childhood friend Jack Teich was kidnapped out of his driveway in the nicest part of the nicest part of Long Island. He was arriving home from work when two men forced him into their car at gunpoint and took him to a house where they chained and interrogated him.
On the second day of his kidnapping, Jack’s wife, Janet, received a call from someone demanding a ransom of $750,000, and a few days later, Janet and Jack’s brother Buddy dropped the money off at Penn Station under F.B.I. surveillance. The F.B.I. did not catch the kidnapper, but afterward, he decided to let Jack go.
Jack was home safe. He had survived his kidnapping. But the actual kidnapping is not what this story is about, if you can believe it. It’s about surviving what you survived, which is also known as the rest of your life.
There are a lot of ways to listen to ‘The Daily.’ Here’s how.
We want to hear from you. Tune in, and tell us what you think. Email us at [email protected] . Follow Michael Barbaro on X: @mikiebarb . And if you’re interested in advertising with The Daily, write to us at [email protected] .
Additional production for The Sunday Read was contributed by Isabella Anderson, Anna Diamond, Sarah Diamond, Elena Hecht, Emma Kehlbeck, Tanya Pérez, Frannie Carr Toth and Krish Seenivasan.
Taffy Brodesser-Akner is a features writer for The New York Times Magazine, based in New York. More about Taffy Brodesser-Akner
Advertisement
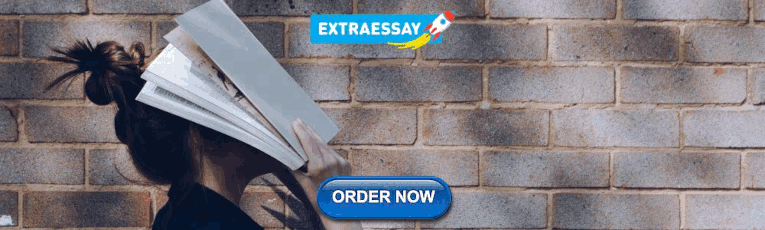
IMAGES
VIDEO
COMMENTS
Chen TC, Lin YC, Wang LC (2012) The analysis of BOT strategies based on game theory - case study on Taiwan's high speed railway project. J Civil Eng Manag 18(5):662-674. Cohen N (2015) Bargaining and informal interactions in the national budget: A game theory analysis of the Israeli case. Int Rev Administr Sci 81(1):58-78
Games of Threats. by Elon Kohlberg and Abraham Neyman. The Shapley value is the most widely studied solution concept of cooperative game theory, with applications to cost allocation, fair division, voting, etc. It is defined on coalitional games, which are the standards objects of the theory. The authors extend the Shapley value solution beyond ...
Game theory for HR case studies. Note: The complete calculation of the formulas cannot be given due to space limitations. However, sufficient guidelines are provided to build your own cases and calculate the formulas. Please consult with your data science team for production implementation. Case study 1: Talent acquisition
Game theory is a branch of mathematics used to study strategic decision making. In a game-theoretic setting, two or more players take turns making decisions to maximize their payoff. The game-theoretic solution is usually presented as a combination of strategies that each player should use in the game. The game-theoretic solutions are based on ...
Game theory as a management tool has been around for more than 50 years. Today, most university business students are introduced to the idea through the classic "prisoner's dilemma.". This and similar exercises have instilled the idea that game theory generates a single solution representing the best outcome for reasonable players.
The game theory elements and learning theories provide the basis for a general design framework in Section 4. In Section 5, we introduce two case studies where game theory-based interventions were conducted with stakeholders from diverse contexts to examine different multi-actor problems in very different and unique ways. For each case, we ...
Journal of Game Theory 2017, 6 (1): 7-14. DOI: 10.5923/j.jgt.20170601. 2. A Case for Behavioural Game Theory. Sarah Bonau. Faculty of Business and Economics, University of Pecs, Pecs, Hungary ...
Abstract and Figures. When one starts to study Game Theory, a game called Prisoner's Dilemma is one of the first examples to be introduced. This report was written just after I completed Stanford ...
Elizabeth Bondi, Hoon Oh, Haifeng Xu, Fei Fang, Bistra Dilkina, and Milind Tambe. 2019. "Using Game Theory in Real Time in the Real World: A Conservation Case Study (Demonstration) ." In International Conference on Autonomous Agents and Multiagent Systems (Demonstration) (AAMAS-19).
2. An exemplar: a course in game theory Game Theory as a topic is well suited to approaches that use activities involving participants as players to introduce the concepts, rules and strategies for particular games and/or theorems presented. In Brokaw and Merz (2004), one such activity is presented: a game that allows players to grasp the
Social networks emerge as a result of actors' linking decisions. We propose a game-theoretical model of socio-strategic network formation on directed weighted graphs, in which every actors ...
Several case studies on the application of GT to game design have also been conducted. One of the most popular is the Beer Game (Sterman, 1989), which has formed the basis for further studies on optimization (Meng, Ye, & Xie, 2010; Thompson & Badizadegan, 2015) and the modelling artificial intelligence (Kimbrough, Wu, & Zhong, 2002).
1.2 QUESTION AND HYPOTHESIS. This research paper regarding the Strategic Decision Making "Game Theory" is written to study how firms can take decision and collaborate to increase their profit, but at the same time how firms can determinate the market to find a better position in the market.
Strategy. Game theory is the study of mathematical models of strategic interactions. [1] It has applications in many fields of social science, and is used extensively in economics, logic, systems science and computer science. [2] Initially, game theory addressed two-person zero-sum games, in which a participant's gains or losses are exactly ...
The two main players in this game of pricing decisions are the retailers and the consumers. The customers want the goods at the best prices, and the retailer makes use of the best pricing tactic. 2. Collective Bargaining. One of the best examples of game theory is negotiation or collective bargaining.
The game theory could be an efficient tool for solving this issue. In this case study, linear programming and game theory havebeen used as the modeling approach to mitigate the hurdles and to look for the better solution to this burning issue. From the game model and Nash equilibrium we can see the 6. th.
This is where the modern mathematical theory of games comes into play. Games such as heads-tails and stone-paper-scissors are called two-person zero-sum games. Zero-sum means that any money Player ...
The concepts of game theory (rationality etc) now pervade much of social science, so that Professor Zagare's exposition of game theory and its applications (intended to "convert the unconverted and initiate the uninitiated") is very welcome. He provides methods for analysing the structure of the game; considers zero and nonzero-sum games ...
Therefore, accurately identifying whether a case is best represented as a simultaneous or sequential game is a crucial step in the strategic analysis of international politics. ... Game theory has been used to study the formation and effectiveness of international institutions and regimes. For example, the collective action problem, which ...
A Case Study of Agent-Based Models for Evolutionary Game Theory. Jacobus Smit, Ed Plumb. Evolutionary game theory is a mathematical toolkit to analyse the interactions that an individual agent has in a population and how the composition of strategies in this population evolves over time. While it can provide neat solutions to simple problems ...
foundations of game theory and analyze the game theory strategies for the decision making in business firms. Keywords: Strategy, Payoff Matrix, Behavior Model , Decision Making , Zero -Sum Games . Game Theory Strategies for Decision Making - A Case Study N. Santosh Ranganath Faculty Member, Dept. of Commerce and Management Studies Dr.B.R ...
Game Theory Case Study 1. Suggest other situations where Game theory approaches might be useful and explain why? Auctions - Auctions present illustrations of games of incomplete information because bidders' private information is the main factor affecting the strategic behaviour in such cases. The simpler forms of auctions induce normal ...
In this study, a tripartite decision-making parking pricing model was developed based on Game Theory to comprehensively reflect the impact of parking pricing on private car travelers, parking lot operators, and traffic managers. Utility theory is introduced to analyze the behavioral characteristics of the tripartite participants in parking pricing. A parking behavior model for private car ...
Cooperative game theory approach towards capital equipment procurement in construction projects: a concrete batch plant case study. Mahdi Rouhparvar a Ministry of Roads and Urban Development, ... This study explores how contractors can benefit from joint resource procurement in construction projects, particularly in case with capital-intensive ...
After the 1968 Mexico City Games and 1972 Munich games were marked by deadly violence and the 1976 Montreal Games experienced dramatic cost overruns, there were hardly any takers to serve as a ...
The announcement that Vice President Kamala Harris will seek the Democratic nomination for president is inspiring a wave of false claims about her eligibility and her background. Some first emerged years ago, while others only surfaced after President Joe Biden's decision to end his bid for a second term.. Here's a look at the facts. ___ CLAIM: Harris is not an American citizen and ...
401 Games Case Study; 401 Games Case Study. 753 Words 4 Pages. When positioning 401 Games, we are using a combination of bases for our position method. We chose user categories, and usage occasions. We first chose user categories because this specific positioning approach highlights the ideal target consumer and suggests that our products are ...
Imitation learning is an effective approach for training game-playing agents and, consequently, for efficient game production. However, generalization - the ability to perform well in related but unseen scenarios - is an essential requirement that remains an unsolved challenge for game AI.
A CASE STUDY OF TENCENT'S MOBILE PAYMENT INTERNATIONAL EXPANSION BASED ON DIFFUSION OF INNOVATIONS THEORY ZHANG SHIMAN Graduate School, Siam University [email protected] Abstract: The rapid development of mobile payment systems has transformed the global financial landscape, and Tencent's WeChat Pay is at the forefront of this revolution.
The Sunday Read: 'A Republican Election Clerk vs. Trump Die-Hards in a World of Lies'