Academia.edu no longer supports Internet Explorer.
To browse Academia.edu and the wider internet faster and more securely, please take a few seconds to upgrade your browser .
Enter the email address you signed up with and we'll email you a reset link.
- We're Hiring!
- Help Center
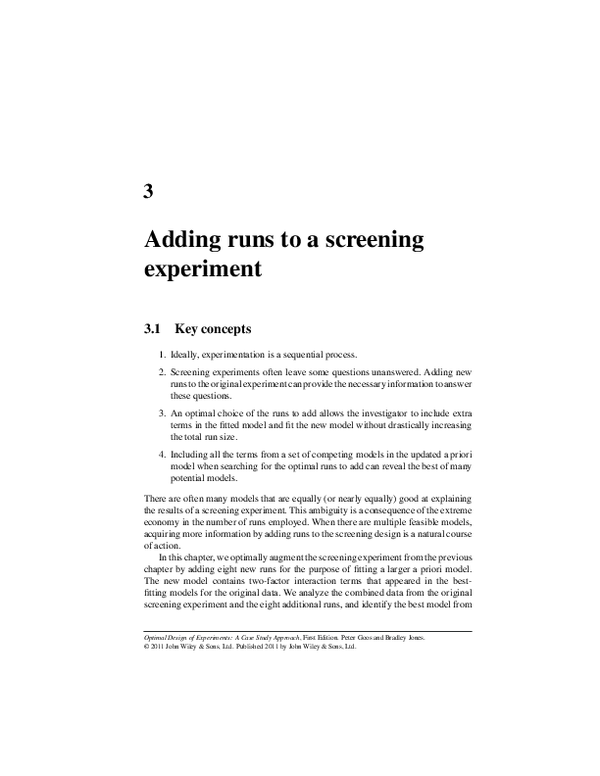
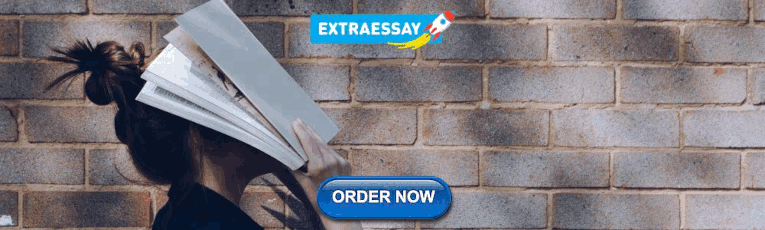
Optimal Design of Experiments: A Case Study Approach by Peter Goos and Bradley Jones

2012, International Statistical Review
Optimal experimental design
- Byran Smucker 1 ,
- Martin Krzywinski 2 &
- Naomi Altman 3
Nature Methods volume 15 , pages 559–560 ( 2018 ) Cite this article
25k Accesses
53 Citations
21 Altmetric
Metrics details
Customize the experiment for the setting instead of adjusting the setting to fit a classical design.
You have full access to this article via your institution.
To maximize the chance for success in an experiment, good experimental design is needed. However, the presence of unique constraints may prevent mapping the experimental scenario onto a classical design. In these cases, we can use optimal design: a powerful, general-purpose tool that offers an attractive alternative to classical design and provides a framework within which to obtain high-quality, statistically grounded designs under nonstandard conditions. It can flexibly accommodate constraints, is connected to statistical quantities of interest and often mimics intuitive classical designs.
For example, suppose we wish to test the effects of a drug’s concentration in the range 0–100 ng/ml on the growth of cells. The cells will be grown with the drug in test tubes, arranged on a rack with four shelves. Our goal may be to determine whether the drug has an effect and precisely estimate the effect size or to identify the concentration at which the response is optimal. We will address both by finding designs that are optimal for regression parameter estimation as well as designs optimal for prediction precision.
To illustrate how constraints may influence our design, suppose that the shelves receive different amounts of light, which might lead to systematic variation between shelves. The shelf would therefore be a natural block 1 . Since we don’t expect such systematic variation within a shelf, the order of tubes on a shelf can be randomized. Furthermore, each shelf can only hold nine test tubes. The experimental design question, then, is: What should be the drug concentration in each of the 36 tubes?
If concentration were a categorical factor, we could compare the mean response at nine concentrations—a traditional randomized complete block design (RCBD) 1 . However, because concentration is actually continuous, discrete levels unduly limit which concentrations are studied and reduce our ability to detect an effect and estimate the concentration that produces an optimal response. Classical designs, like full factorials or RCBDs, assume an ideal and simple experimental setup, which may be inappropriate for all experimental goals or untenable in the presence of constraints.
Optimal design provides a principled approach to accommodating the entire range of concentrations and making full use of each shelf’s capacity. It can incorporate a variety of constraints such as sample size restrictions (e.g., the lab has a limited supply of test tubes), awkward blocking structures (e.g., shelves have different capacities) or disallowed treatment combinations (e.g., certain combinations of factor levels may be infeasible or otherwise undesirable).
To assist in describing optimal design, let’s review some terminology. The drug is a ‘factor’, and particular concentrations are ‘levels’. A particular combination of factor levels is a ‘treatment’ (with just a single factor, a treatment is simply a factor level) applied to an ‘experimental unit’, which is a test tube. The shelves are ‘blocks’, which are collections of experimental units that are similar in traits (e.g., light level) that might affect the experimental outcome 1 . The possible set of treatments that could be chosen is the ‘design space’. A ‘run’ is the execution of a single experimental unit, and the ‘sample size’ is the number of runs in the experiment.
Optimal design optimizes a numerical criterion, which typically relates to the variance or other statistically relevant properties of the design, and uses as input the number of runs, the factors and their possible levels, block structure (if any), and a hypothesized form of the relationship between the response and the factors. Two of the most common criteria are the D-criterion and the I-criterion. They are fundamentally different: the D-criterion relates to the variance of factor effects, and the I-criterion addresses the precision of predictions.
To understand the D-criterion (determinant), suppose we have a quadratic regression model 2 with parameters β 1 and β 2 that relate the factor to the response (for simplicity, ignore β 0 , the intercept). Our estimates of these parameters, \(\hat \beta _1\) and \(\hat \beta _2\) , will have error and, assuming the model error variance is known, the D-optimal design minimizes the area of the ellipse that defines the joint confidence interval for the parameters (Fig. 1 ). This area will include the true values of both β 1 and β 2 in 95% (or some other desired proportion) of repeated executions of the design, and its size and shape are a function of the data’s overall variance and the design.
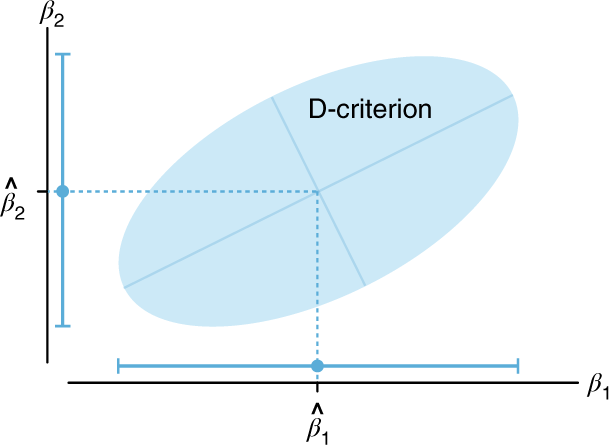
The ellipse can be projected onto each axis to obtain the familiar one-dimensional confidence intervals for each parameter (shown as blue points with error bars). The D-criterion reduces the variance of the parameter estimates and/or the correlation between the estimates by minimizing the area of the ellipse.
On the other hand, the I-criterion (integrated variance) is used when the experimental goal is to make precise predictions of the response, rather than to obtain precise estimates of the model parameters. An I-optimal design chooses the set of runs to minimize the average variance in prediction across the joint range of the factors. The prediction variance is a function of several elements: the data’s overall error variance, the factor levels at which we are predicting, and also the design itself. This criterion is more complicated mathematically because it involves integration.
For both criteria, numerical heuristics are used in the optimization but they do not guarantee a global optimum. For most scenarios, however, near-optimal designs are adequate and not hard to obtain.
Returning to our example, suppose we wish to obtain a precise estimate of our drug’s effect on the mean response. If we expect that the effect is linear (our model has one parameter of interest, β 1 , which is the slope), the D-optimal design places either four or five experimental units in each block at the low level (0 ng/ml) and the remaining units at the high level (100 ng/ml). Thus, to obtain a precise estimate of β 1 , we want to place the concentration values as far apart as possible in order to stabilize the estimate. Assigning four or five units of each concentration to each shelf helps to reduce the confounding of drug and shelf effects.
One downside to this simple low–high design is its inability to detect departures from linearity. If we expect that, after accounting for block differences, the relationship between the response and the factor may be curvilinear (with both a linear and quadratic term: y = β 0 + β 1 x + β 2 x 2 + ε , where ε is the error and β 0 is the intercept, which we'll ignore here; we also omit the block terms for the sake of simplicity), the D-optimal design is 3–3–3 (at 0, 50 and 100 ng/ml, respectively) within each block.
In many settings, the goal is to learn about whether and how factors affect the response (i.e., whether β 1 and/or β 2 are non-zero and, if so, how far from zero they are), in which case the D-criterion is a good choice. In other cases, the goal is to find the level of the factors that optimizes the response, in which case a design that produces more precise predictions is better. The I-criterion, which minimizes the average prediction variance across the design region, is a natural choice.
In our example, the I-optimal design for the linear model is equivalent to that generated by the D-criterion: within each block, it allocates either four or five units to the low level and the rest to the high level. However, the I-optimal design for the model that includes both linear and quadratic effects is 2–5–2 within each block; that is, it places two experimental units at the low and high levels of the factor and places five in the center.
The quality of these designs in terms of their prediction variance can be compared using fraction of design space (FDS) plots 3 . We show this plot for the D- and I-optimal designs for the quadratic case (Fig. 2a ). A point on an FDS plot gives the proportion of the design space (the fraction of the 0–100 ng/ml interval, across the blocks) that has a prediction variance less than or equal to the value on the y axis. For instance, the I-optimal design yields a lower median prediction variance than the D-optimal design: at most 0.13 for 50% of the design space as compared to 0.15. Because of the extra runs at 50 ng/ml, the I-optimal design has a lower prediction variance in the middle of the region than the D-optimal design, but variance is higher near the edges (Fig. 2b ).
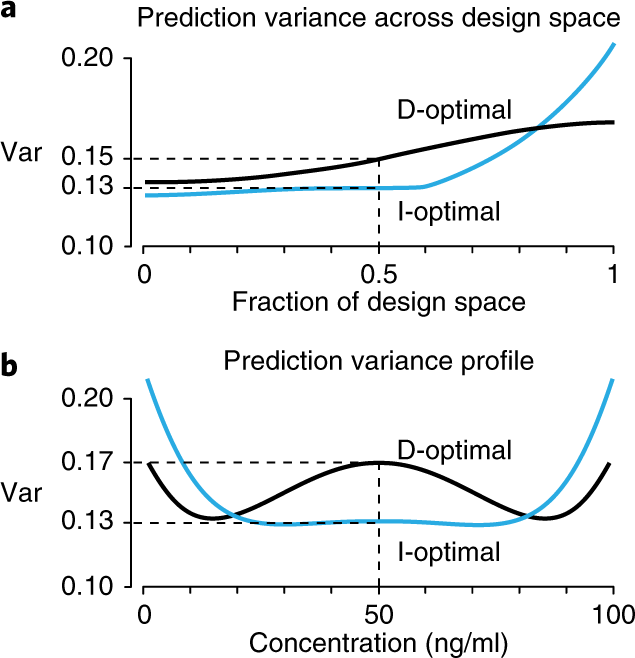
a , Prediction variance as a function of the fraction of design space (FDS). b , The variance profile across the range of concentrations for both designs.
Our one-factor blocking example demonstrates the basics of optimal design. A more realistic experiment might involve the same blocking structure but three factors—each with a specified range—and a goal to determine how the response is impacted by the factors and their interactions. We want to study the factors in combination; otherwise, any interactions between them will go undetected and the statistical efficiency to estimate factor effects is reduced.
Without the blocking constraint, a typical strategy would be to specify and use a high and low level for each factor and to perform an experiment using several replicates of the 2 3 = 8 treatment combinations. This is a classical two-level factorial design 4 that under reasonable assumptions provides ample power to detect factor effects and two-factor interactions. Unfortunately, this design doesn’t map to our scenario and can’t use the full nine-unit capacity of each shelf—unlike an optimal design, which can (Fig. 3 ).
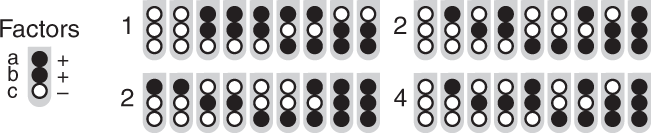
The D-optimal design that assigns three factors (a–c) at two levels each—low (unfilled circles) and high (filled circles)—to nine tubes on each of four shelves. The shelves are blocks and the design accounts for the main effects of the three factors and the three two-factor interactions. Each treatment is replicated at least four times, with treatments in tubes 3–7 on each shelf replicated five times.
In unconstrained settings where a classical design would be appropriate, optimal designs often turn out to be the same as their traditional counterparts. For instance, any RCBD 1 is both D- and I-optimal. Or, for a design with a sample size of 24, three factors, no blocks, and an assumed model that includes the three factor effects and all of the two-factor interactions, both the D- and I-criteria yield as optimal the two-level full-factorial design with three replicates.
So far, we have described optimal designs conceptually but have not discussed the details of how to construct them or how to analyze them 5 . Specialized software to construct optimal designs is widely available and accessible. To analyze the designs we’ve discussed—with continuous factors—it is necessary to use regression 2 (rather than ANOVA) to meaningfully relate the response to the factors. This approach allows the researcher to identify large main effects or quadratic terms and even two-factor interactions.
Optimal designs are not a panacea. There is no guarantee that (i) the experiment can achieve good power, (ii) the model form is valid and (iii) the criterion reflects the objectives of the experiment. Optimal design requires careful thought about the experiment. However, in an experiment with constraints, these assumptions can usually be specified reasonably.
Krzywinski, M. & Altman, N. Nat. Methods 11 , 699–700 (2014).
Article PubMed CAS Google Scholar
Krzywinski, M. & Altman, N. Nat. Methods 12 , 1103–1104 (2015).
Zahran, A., Anderson-Cook, C. M. & Myers, R. H. J. Qual. Tech. 35 , 377–386 (2003).
Article Google Scholar
Krzywinski, M. & Altman, N. Nat. Methods 11 , 1187–1188 (2014).
Goos, P. & Jones, B. Optimal Design of Experiments: A Case Study Approach (John Wiley & Sons, Chichester, UK, 2011).
Download references
Author information
Authors and affiliations.
Associate Professor of Statistics at Miami University, Oxford, OH, USA
Byran Smucker
Staff scientist at Canada’s Michael Smith Genome Sciences Centre, Vancouver, British Columbia, Canada
Martin Krzywinski
Professor of Statistics at The Pennsylvania State University, University Park, PA, USA
Naomi Altman
You can also search for this author in PubMed Google Scholar
Corresponding author
Correspondence to Martin Krzywinski .
Ethics declarations
Competing interests.
The authors declare no competing interests.
Rights and permissions
Reprints and permissions
About this article
Cite this article.
Smucker, B., Krzywinski, M. & Altman, N. Optimal experimental design. Nat Methods 15 , 559–560 (2018). https://doi.org/10.1038/s41592-018-0083-2
Download citation
Published : 31 July 2018
Issue Date : August 2018
DOI : https://doi.org/10.1038/s41592-018-0083-2
Share this article
Anyone you share the following link with will be able to read this content:
Sorry, a shareable link is not currently available for this article.
Provided by the Springer Nature SharedIt content-sharing initiative
This article is cited by
Optimal experimental design for precise parameter estimation in competitive cross-reaction equilibria.
- Somaye Vali Zade
- Hamid Abdollahi
Journal of the Iranian Chemical Society (2024)
Augmented region of interest for untargeted metabolomics mass spectrometry (AriumMS) of multi-platform-based CE-MS and LC-MS data
- Lukas Naumann
- Adrian Haun
- Christian Neusüß
Analytical and Bioanalytical Chemistry (2023)
A microfluidic optimal experimental design platform for forward design of cell-free genetic networks
- Bob van Sluijs
- Roel J. M. Maas
- Wilhelm T. S. Huck
Nature Communications (2022)
Advances, challenges and opportunities in creating data for trustworthy AI
- Weixin Liang
- Girmaw Abebe Tadesse
Nature Machine Intelligence (2022)
Real-time Adaptive Design Optimization Within Functional MRI Experiments
- Per B. Sederberg
- Brandon M. Turner
Computational Brain & Behavior (2020)
Quick links
- Explore articles by subject
- Guide to authors
- Editorial policies
Sign up for the Nature Briefing newsletter — what matters in science, free to your inbox daily.


- Engineering & Transportation
- Engineering

Enjoy fast, free delivery, exclusive deals, and award-winning movies & TV shows with Prime Try Prime and start saving today with fast, free delivery
Amazon Prime includes:
Fast, FREE Delivery is available to Prime members. To join, select "Try Amazon Prime and start saving today with Fast, FREE Delivery" below the Add to Cart button.
- Cardmembers earn 5% Back at Amazon.com with a Prime Credit Card.
- Unlimited Free Two-Day Delivery
- Streaming of thousands of movies and TV shows with limited ads on Prime Video.
- A Kindle book to borrow for free each month - with no due dates
- Listen to over 2 million songs and hundreds of playlists
- Unlimited photo storage with anywhere access
Important: Your credit card will NOT be charged when you start your free trial or if you cancel during the trial period. If you're happy with Amazon Prime, do nothing. At the end of the free trial, your membership will automatically upgrade to a monthly membership.
Buy new: $76.81 $76.81 FREE delivery May 8 - 10 Ships from: Amazon.com Sold by: Amazon.com
Return this item for free.
Free returns are available for the shipping address you chose. You can return the item for any reason in new and unused condition: no shipping charges
- Go to your orders and start the return
- Select the return method
Buy used: $71.21
Fulfillment by Amazon (FBA) is a service we offer sellers that lets them store their products in Amazon's fulfillment centers, and we directly pack, ship, and provide customer service for these products. Something we hope you'll especially enjoy: FBA items qualify for FREE Shipping and Amazon Prime.
If you're a seller, Fulfillment by Amazon can help you grow your business. Learn more about the program.

Download the free Kindle app and start reading Kindle books instantly on your smartphone, tablet, or computer - no Kindle device required .
Read instantly on your browser with Kindle for Web.
Using your mobile phone camera - scan the code below and download the Kindle app.

Image Unavailable

- To view this video download Flash Player

Follow the author

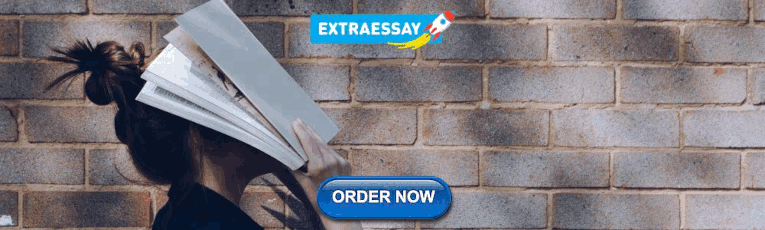
Optimal Design of Experiments: A Case Study Approach 1st Edition
Purchase options and add-ons.
Prague's magnificent synagogues and Old Jewish Cemetery attract millions of visitors each year, and travelers who venture beyond the capital find physical evidence of once vibrant Jewish communities in towns and villages throughout today's Czech Republic. For those seeking to learn more about the people who once lived and died at those sites, however, there has until now been no comprehensive account in English of the region's Jews.
Prague and Beyond presents a new and accessible history of the Jews of the Bohemian Lands written by an international team of scholars. It offers a multifaceted account of the Jewish people in a region that has been, over the centuries, a part of the Holy Roman Empire and the Habsburg Monarchy, was constituted as the democratic Czechoslovakia in the years following the First World War, became the Nazi Protectorate of Bohemia and Moravia and later a postwar Communist state, and is today's Czech Republic. This ever-changing landscape provides the backdrop for a historical reinterpretation that emphasizes the rootedness of Jews in the Bohemian Lands, the intricate variety of their social, economic, and cultural relationships, their negotiations with state power, the connections that existed among Jewish communities, and the close, if often conflictual, ties between Jews and their non-Jewish neighbors.
Prague and Beyond is written in a narrative style with a focus on several unifying themes across the periods. These include migration and mobility; the shape of social networks; religious life and education; civic rights, citizenship, and Jewish autonomy; gender and the family; popular culture; and memory and commemorative practices. Collectively these perspectives work to revise conventional understandings of Central Europe's Jewish past and present, and more fully capture the diversity and multivalence of life in the Bohemian Lands.
- ISBN-10 0470744618
- ISBN-13 978-0470744611
- Edition 1st
- Publisher Wiley
- Publication date August 8, 2011
- Language English
- Dimensions 6 x 0.88 x 9 inches
- Print length 304 pages
- See all details

Frequently bought together

Customers who viewed this item also viewed

Editorial Reviews
"A long-needed, comprehensive, and beautifully written history of the Jews in the Czech lands by an international group of scholars. Combining intricate detail with multi-century narrative sweep, Prague and Beyond is an extraordinary read."—Helen Epstein, author of Where She Came From: A Daughter's Search for her Mother's History
" Prague and Beyond is an impressive work, offering a well-conceived and well-executed overview of the long history of Jews in the Czech lands. The book should be greeted with enthusiasm not only by Czech historians and historians of modern European Jewry, but by European historians more generally and by other readers with an interest in the lost world of pre-Holocaust Europe. All will find something to learn here."—David Rechter, University of Oxford
"There is simply no other integral narrative history of the Jews in the 'Bohemian lands.' Prague and Beyond will fill lacunae on reading lists in European and Jewish history, history of the Holocaust, and Central European political science."—Moshe Rosman, Bar-Ilan University
From the Inside Flap
This is an engaging and informative book on the modern practice of experimental design. The authors' writing style is entertaining, the consulting dialogs are extremely enjoyable, and the technical material is presented brilliantly but not overwhelmingly. The book is a joy to read. Everyone who practices or teaches DOE should read this book. -- Douglas C. Montgomery , Regents Professor, Department of Industrial Engineering, Arizona State University
This book is the compelling story of two consultants in dialog as they show their clients how to leave the roads of textbook experimental design and fly the direct route of optimal design as enabled by computer-based methods. -- John Sall , Executive Vice President and Cofounder, SAS Institute
This book puts cutting-edge optimal design of experiments techniques into the hands of the practitioner. Ten real-world design scenarios, which Goos and Jones present as consulting session conversations with clients, easily engage and absorb the reader. A behind-the-scenes look at various technical treasures accompanies each scenario. -- Marie Gaudard , Professor Emeritus, University of New Hampshire
Each chapter begins with a realistic experimental situation being informally discussed on site by local engineers and statistical consultants. Next an optimal experimental design is constructed and the data with full detailed analysis provided. Statisticians and para-statisticians alike should enjoy this book. Clearly a new day is dawning in the art and practice of experimental design. -- J. Stuart Hunter , Professor Emeritus, Princeton University
From the Back Cover
"This is an engaging and informative book on the modern practice of experimental design. The authors' writing style is entertaining, the consulting dialogs are extremely enjoyable, and the technical material is presented brilliantly but not overwhelmingly. The book is a joy to read. Everyone who practices or teaches DOE should read this book." -- Douglas C. Montgomery , Regents Professor, Department of Industrial Engineering, Arizona State University
"This book is the compelling story of two consultants in dialog as they show their clients how to leave the roads of textbook experimental design and fly the direct route of optimal design as enabled by computer-based methods." -- John Sall , Executive Vice President and Cofounder, SAS Institute
"This book puts cutting-edge optimal design of experiments techniques into the hands of the practitioner. Ten real-world design scenarios, which Goos and Jones present as consulting session conversations with clients, easily engage and absorb the reader. A behind-the-scenes look at various technical treasures accompanies each scenario." -- Marie Gaudard , Professor Emeritus, University of New Hampshire
"Each chapter begins with a realistic experimental situation being informally discussed on site by local engineers and statistical consultants. Next an optimal experimental design is constructed and the data with full detailed analysis provided. Statisticians and para-statisticians alike should enjoy this book. Clearly a new day is dawning in the art and practice of experimental design." -- J. Stuart Hunter , Professor Emeritus, Princeton University
About the Author
Product details.
- Publisher : Wiley; 1st edition (August 8, 2011)
- Language : English
- Hardcover : 304 pages
- ISBN-10 : 0470744618
- ISBN-13 : 978-0470744611
- Item Weight : 1.22 pounds
- Dimensions : 6 x 0.88 x 9 inches
- #137 in Scientific Experiments & Projects
- #705 in History of Judaism
- #1,184 in Industrial Manufacturing Systems
Videos for this product

Click to play video

Optimal Design of Experiments: A Case Study Approach
Merchant Video
About the author
Discover more of the author’s books, see similar authors, read author blogs and more
Customer reviews
Customer Reviews, including Product Star Ratings help customers to learn more about the product and decide whether it is the right product for them.
To calculate the overall star rating and percentage breakdown by star, we don’t use a simple average. Instead, our system considers things like how recent a review is and if the reviewer bought the item on Amazon. It also analyzed reviews to verify trustworthiness.
Reviews with images

- Sort reviews by Top reviews Most recent Top reviews
Top reviews from the United States
There was a problem filtering reviews right now. please try again later..

Top reviews from other countries

- Amazon Newsletter
- About Amazon
- Accessibility
- Sustainability
- Press Center
- Investor Relations
- Amazon Devices
- Amazon Science
- Sell on Amazon
- Sell apps on Amazon
- Supply to Amazon
- Protect & Build Your Brand
- Become an Affiliate
- Become a Delivery Driver
- Start a Package Delivery Business
- Advertise Your Products
- Self-Publish with Us
- Become an Amazon Hub Partner
- › See More Ways to Make Money
- Amazon Visa
- Amazon Store Card
- Amazon Secured Card
- Amazon Business Card
- Shop with Points
- Credit Card Marketplace
- Reload Your Balance
- Amazon Currency Converter
- Your Account
- Your Orders
- Shipping Rates & Policies
- Amazon Prime
- Returns & Replacements
- Manage Your Content and Devices
- Recalls and Product Safety Alerts
- Conditions of Use
- Privacy Notice
- Consumer Health Data Privacy Disclosure
- Your Ads Privacy Choices
4 DOE Case Studies
Peter Goos presents four case studies from the Optimal Design of Experiments book he co-authored with Bradley Jones. He introduces each case by describing the type of experiment and when and why it is useful. Next, he demonstrates how to set up the experiment in the JMP software and how to analyze the resulting data.
Presentation material taken from Optimal Design of Experiments: A Case Study Approach by P. Goos and B. Jones .
CASE STUDIES
Yield maximization experiment: a response surface design in an irregularly shaped factor region.
The presenter designs an experiment for investigating a chemical reaction using a full cubic model in two factors. Because many factor-level combinations are known to be infeasible in advance, the two factors cannot be varied completely independently of each other.
Stability Improvement Experiment: A Screening Design in Blocks
The presenter creates a blocking design to determine what factors impact the stability of a vitamin. The process mean shifts randomly from day to day and the investigator wants to explicitly account for these shifts in the statistical model.
Wind Tunnel Experiment: A Split-plot Design
The presenter designs an experiment run in a wind tunnel where it is possible to vary certain factors without shutting down the tunnel and other factors can only be changed by shutting down the tunnel.
Laser Etching Experiment: A Definitive Screening Design in Blocks
The presenter creates a definitive screening design to determine optimal settings for a laser etching process.
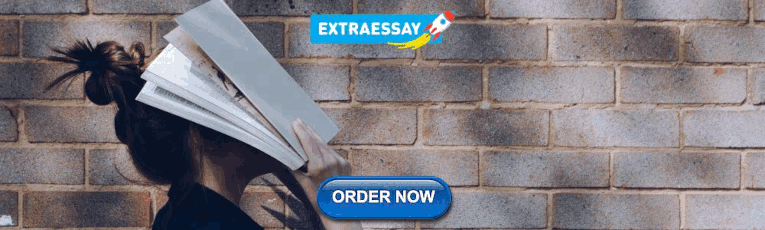
IMAGES
VIDEO
COMMENTS
Using optimal experimental design, as we did in the extraction experiment case study, is such an approach: it allows the researcher to create a follow-up experiment that will give the most precise estimates for any set of main effects, interaction effects, and/or polynomial effects (such as quadratic effects; see Chapter 4).
Optimal design of experiments: a case study approach / Peter Goos and Bradley Jones. p. cm. Includes bibliographical references and index. ISBN 978--470-74461-1 (hardback) 1. Industrial engineering-Experiments-Computer-aided design. 2. Experimental design-Data processing. 3. Industrial engineering-Case studies. I. Jones, Bradley. II ...
Optimal Design of Experiments A Case Study Approach. P1: OTA/XYZ P2: ABC JWST075-FM JWST075-Goos June 6, 2011 8:54 Printer Name: Yet to Come ... Optimal design of experiments: a case study approach / Peter Goos and Bradley Jones. p. cm. Includes bibliographical references and index. ISBN 978--470-74461-1 (hardback) 1. Industrial engineering ...
Peter Goos, Department of Mathematics, Statistics and Actuarial Sciences of the Faculty of Applied Economics of the University of Antwerp. His main research topic is the optimal design of experiments. He has published a book as well as several methodological articles on the design and analysis of blocked and split-plot experiments.
Optimal Design of Experiments: A Case Study Approach Peter Goos, Bradley Jones E-Book 978-1-119-97616-5 June 2011 $83.00 Hardcover 978--470-74461-1 August 2011 Print-on-demand $103.95 O-Book 978-1-119-97401-7 June 2011 Available on Wiley Online Library DESCRIPTION "This is an engaging and informative book on the modern practice of experimental ...
Request PDF | Optimal Design of Experiments: A Case-Study Approach | "This is an engaging and informative book on the modern practice of experimental design. The authors' writing style is ...
A comparative experiment on the design of a response surface design in an irregularly shaped design region and the effect of the mixture constraint on the model results in a robust and optimal process experiment. Preface. Acknowledgments. 1 A simple comparative experiment. 1.1 Key concepts. 1.2 The setup of a comparative experiment. 1.3 Summary. 2 An optimal screening experiment. 2.1 Key ...
This is an engaging and informative book on the modern practice of experimental design. The authors writing style is entertaining, the consulting dialogs are extremely enjoyable, and the technical material is presented brilliantly but not overwhelmingly. The book is a joy to read. Everyone who practices or teaches DOE should read this book. - Douglas C. Montgomery, Regents Professor ...
"This is an engaging and informative book on the modern practice of experimental design. The authors' writing style is entertaining, the consulting dialogs are extremely enjoyable, and the technical material is presented brilliantly but not overwhelmingly. The book is a joy to read. Everyone who practices or teaches DOE should read this book." - Douglas C. Montgomery, Regents Professor ...
This book ably demonstrates this notion by showing how tailor-made, optimal designs can be effectively employed to meet a client's actual needs. It should be required reading for anyone interested in using the design of experiments in industrial settings." This book demonstrates the utility of the computer-aided optimal design approach using ...
Optimal Design of Experiments: A Case Study Approach by Peter Goos and Bradley Jones. Norman R. Draper, Norman R. Draper. Department of Statistics, University of Wisconsin-Madison, 1300 University Avenue, Madison, WI 53706-1532, USA [email protected] ... PDF. Tools. Request permission; Export citation; Add to favorites; Track citation ...
This paper builds on an alternative principle of utilizing optimal approximate designs for the computation of optimal, or nearly-optimal, exact designs and proves a low-rank property of the associated quadratic forms, which enables us to use AQuA efficiently and apply it to large design spaces.
Optimal Design Of Experiments A Case Study Approach Donald T. Campbell,Julian C. Stanley Optimal Design of Experiments Peter Goos,Bradley Jones,2011-06-28 This is an engaging and informative book on the modern practice of experimental design. The authors' writing style is entertaining, the consulting dialogs are extremely
Optimal Design of Experiments. : Peter Goos, Bradley Jones. Wiley, Jun 13, 2011 - Science - 304 pages. "This is an engaging and informative book on the modern practice of experimental design. The authors' writing style is entertaining, the consulting dialogs are extremely enjoyable, and the technical material is presented brilliantly but not ...
Optimal Design of Experiments: A Case Study approach by Peter Goos and Bradley Jones and Christina M. Mastrangelo. Optimal Design of Experiments: A Case Study ...
Optimal Design of Experiments: A Case Study Approach Willis A. Jensen W. L. Gore & Associates, Inc., 3750 West Kiltie Lane, Flagstaff, Arizona86003-2400 Pages 279-280 | Published online: 21 Nov 2017
Optimal Design of Experiments: A Case Study Approach by Peter Goos and Bradley Jones. Norman R. Draper, Norman R. Draper. Department of Statistics, University of Wisconsin-Madison, 1300 University Avenue, Madison, WI 53706-1532, USA [email protected] ... View the article/chapter PDF and any associated supplements and figures for a period ...
An I-optimal design chooses the set of runs to minimize the average variance in prediction across the joint range of the factors. The prediction variance is a function of several elements: the ...
Optimal Design of Experiments: A Case Study Approach. 1st Edition. by Goos (Author) 4.5 41 ratings. See all formats and editions. "This is an engaging and informative book on the modern practice of experimental design. The authors' writing style is entertaining, the consulting dialogs are extremely enjoyable, and the technical material is ...
He introduces each case by describing the type of experiment and when and why it is useful. Next, he demonstrates how to set up the experiment in the JMP software and how to analyze the resulting data. Presentation material taken from Optimal Design of Experiments: A Case Study Approach by P. Goos and B. Jones.
pdf Excerpt The General Equivalence Theorem provides necessary and sufficient conditions for a moment matrix to be ϕ-optimal for the parameter system of interest in a compact and convex set of competing moment matrices, where ϕ is an information function.
Optimal Design of Experiments: A Case Study Approach by Peter Goos and Bradley Jones. Norman R. Draper, Norman R. Draper. Department of Statistics, University of Wisconsin-Madison, ... PDF. Tools. Request permission; Export citation; Add to favorites; Track citation; Share Share. Give access.
Statistical design of experiments (DoE) aims to develop a near effi-cient design while minimising the number of experiments required. This is an optimal approach especially when there is a need to investigate multiple vari-ables. DoE is a powerful methodology for a wide range of applications, from