Academia.edu no longer supports Internet Explorer.
To browse Academia.edu and the wider internet faster and more securely, please take a few seconds to upgrade your browser .
Enter the email address you signed up with and we'll email you a reset link.
- We're Hiring!
- Help Center
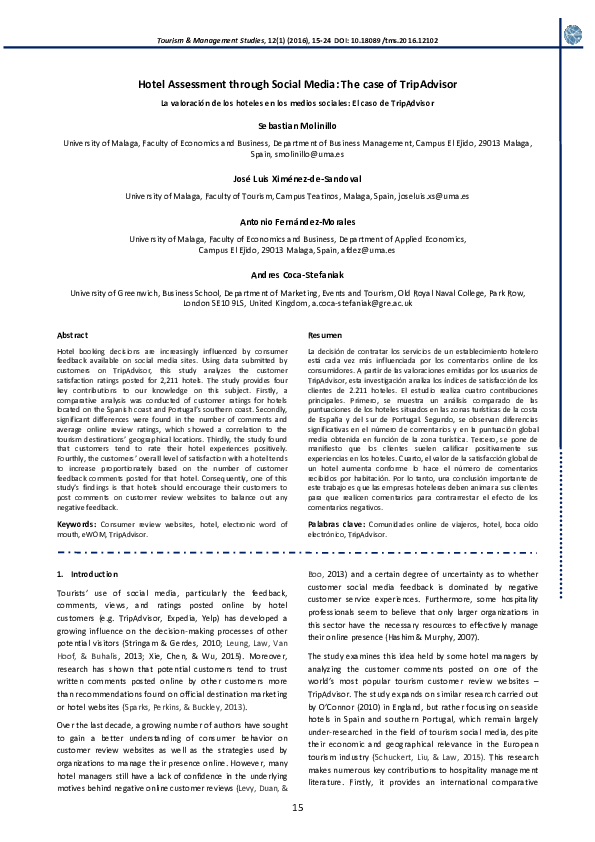
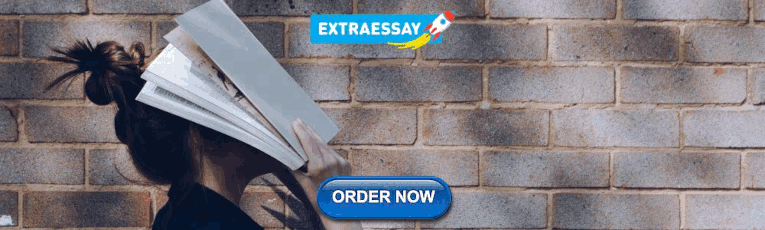
Hotel Assessment through Social Media: The case of TripAdvisor

Hotel booking decisions are increasingly influenced by consumer feedback available on social media sites. Using data submitted by customers on TripAdvisor, this study analyzes the customer satisfaction ratings posted for 2,211 hotels. The study provides four key contributions to our knowledge on this subject. Firstly, a comparative analysis was conducted of customer ratings for hotels located on the Spanish coast and Portugal's southern coast. Secondly, significant differences were found in the number of comments and average online review ratings, which showed a correlation to the tourism destinations' geographical locations. Thirdly, the study found that customers tend to rate their hotel experiences positively. Fourthly, the customers' overall level of satisfaction with a hotel tends to increase proportionately based on the number of customer feedback comments posted for that hotel. Consequently, one of this study's findings is that hotels should encourage their customers to post comments on customer review websites to balance out any negative feedback. Resumen La decisión de contratar los servicios de un establecimiento hotelero está cada vez más influenciada por los comentarios online de los consumidores. A partir de las valoraciones emitidas por los usuarios de TripAdvisor, esta investigación analiza los índices de satisfacción de los clientes de 2.211 hoteles. El estudio realiza cuatro contribuciones principales. Primero, se muestra un análisis comparado de las puntuaciones de los hoteles situados en las zonas turísticas de la costa de España y del sur de Portugal. Segundo, se observan diferencias significativas en el número de comentarios y en la puntuación global media obtenida en función de la zona turística. Tercero, se pone de manifiesto que los clientes suelen calificar positivamente sus experiencias en los hoteles. Cuarto, el valor de la satisfacción global de un hotel aumenta conforme lo hace el número de comentarios recibidos por habitación. Por lo tanto, una conclusión importante de este trabajo es que las empresas hoteleras deben animar a sus clientes para que realicen comentarios para contrarrestar el efecto de los comentarios negativos.
Related Papers
Jose Luis Orozco
International tourism has a significant impact on the Spanish economy, both in GDP and in employment. International visitors mostly use English to communicate with the staff of the hotels where they are staying. The development of ICTs and the emergence of the web 2.0 have meant a revolution in the management of the reputation of tourism companies. The aim of this study was to analyse how Spanish hotels manage messages in English from their international guests published on TripAdvisor. The results suggest that, in general, the performance of Spanish hotels is appropriate. However, some aspects that could be improved to raise the quality of responses are presented.
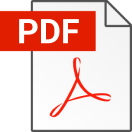
Yale Tiradentes
JMRA Publication
Universal Journal of Computational Mathematics
Alma Braimllari (Spaho)
Online reviews are used by customers as an important source of information during the travel and tourism decision-making process. This research main objective is to analyze the online ratings of hotels in Tirana and Durrës, Albania. Data from Booking.com were collected for 132 hotels with 30 or more online reviews during the period of time 29 November-2 December 2017. Mean of the overall online rating of hotels was 8.65 with a standard deviation of 0.636. About 62.4% of online reviewers of hotels in Tirana have scored from 9 to 10, and 44% of online reviewers of hotels in Durrës have scored from 9 to 10. Results of two econometric models indicated that hotel's size and location negatively impact the overall online rating of the hotels, whereas the hotel's category, the number of online reviews and year the hotel was welcomed by Booking.com positively impact the overall online rating of hotels. These findings are useful for hotel guests and potential customers, and also for hotels' managers in developing appropriate marketing strategies.
Sérgio Moro
This study presents a data mining approach for modeling TripAdvisor score using 504 reviews published in 2015 for the 21 hotels located in the Strip, Las Vegas. Nineteen quantitative features characterizing the reviews, hotels and the users were prepared and used for feeding a support vector machine for modeling the score. The results achieved reveal the model demonstrated adequate predictive performance. Therefore, a sensitivity analysis was applied over the model for extracting useful knowledge translated into features' relevance for the score. The findings unveiled user features related to TripAdvisor membership experience play a key role in influencing the scores granted, clearly surpassing hotel features. Also, both seasonality and the day of the week were found to influence scores. Such knowledge may be helpful in directing efforts to answer online reviews in alignment with hotel strategies, by profiling the reviews according to the member and review date.
Innovative Research Publications
During the travel and tourism decision making process, the online reviews are used as an important source of information by hotel guests. The aim of this study was to analyze the online ratings of hotels in Tirana. The average overall online rating for hotels was 8.82. Results of chi square test indicated that hotel category was related to the overall online rating. The logistic model results indicated that hotel's size negatively impact the overall online rating, whereas the hotel's category positively impact the overall online rating of the hotels in Tirana. These findings are useful for hotel guests, potential customers and hotels' managers.
Eva Martin-Fuentes
C. Michael Hall , Stefan Gössling
Gössling, S., Andersson, A-C., & Hall, C.M., The Manager's Dilemma: A conceptualization of online review manipulation strategies. Current Issues in Tourism, DOI: 10.1080/13683500.2015.1127337 [The version provided here is the submitted manuscript. For the authoritative version please consult the journal website] Online evaluations are one of the most important innovations in tourism in recent years, often combining a review/rating (business-specific evaluation) and a ranking (inter-business comparison). As online reputation determines economic success, tourism managers may be tempted to manipulate online content. This paper presents the results from a qualitative study involving 20 hotel managers in southern Sweden, and their perspectives on manipulation. Results confirm that there exists a wide range of review manipulation strategies, many of which are difficult to control. Even though only few managers appear to systematically manipulate, online evaluations represent a significant challenge for businesses, as they introduce direct competition and foster consumer judgment cultures. It is postulated that managers will increasingly find themselves in a Prisoner’s dilemma, representing a situation where engaging in manipulation is the most rational choice in an increasingly competitive market situation.
Tourism & Management Studies
The tourism industry has been strongly impacted by the consumer use of review sites. Since review travel sites such as TripAdvisor allowed accommodations to create own profiles with information, hotels began incorporating these actions into their engaging customers programming. Despite the benefits that review sites can offer to customers, hotels and accommodations, its implementation is not developed and exploited in all its possibilities, and little is known about hotels use of this review sites. This study explores the topic trough quantitative methodology, conducting multiple correlation analysis of data obtained from a sample of 301 hotel managers. Managers consider they are committed to this type of platforms and are capable of use it accurately. Also it was found evidence of correlation between use of TripAdvisor and hotel characteristics (size, ownership structure, and category). Furthermore, multiple regression analysis shows competence and commitment as the most important predictors of use intensity. Resumen La industria del turismo se ha visto fuertemente afectada por el uso las plataformas de valoración por parte de los consumidores. Dado que sitios como TripAdvisor permiten a los alojamientos crear perfiles propios, los hoteles han comenzado a incorporar estas acciones en sus programas de compromiso y fidelización de clientes. A pesar de los beneficios que estas webs pueden ofrecer tanto a clientes como a hoteles, su implementación no se ha desarrollado en todas sus posibilidades, y poco se sabe sobre el uso que de ellas hacen los hoteles. Esta investigación explora este tema mediante una metodología cuantitativa, realizando análisis de correlación múltiple con datos obtenidos de 301 gerentes de hoteles. Los gerentes consideran que están comprometidos con este tipo de plataformas y son capaces de usarlas con precisión. Además, encontramos evidencias de correlación entre el uso de TripAdvisor y las características del hotel (tamaño, estructura de propiedad y categoría). Igualmente, el análisis de regresión múltiple muestra la capacidad y el compromiso como los predictores más importantes de la intensidad de su uso.
Rosanna Leung
This study aims to identify and categorize service failures from luxury hotels in Taiwan. A theoretical framework is proposed by adapting marketing mix elements. A total of 411 negative comments were found and extracted from four popular online review websites from 20 luxury hotels in Taipei. The content analysis method was employed for data analysis. The study results show that majority of luxury hotels in Taiwan encounter critical product issues, especially concerning the condition of facilities and equipment offered in the guest rooms. Furthermore, cleanliness of the hotel rooms requires greater attention, especially the cleanliness of room carpets, which could affect the hotel guests' comfort during their stay. The findings and recommendations can provide luxury hotel practitioners in Taiwan a clearer picture about the service problems faced and enable them to conduct further investigations for further improvements. So far, existing studies are lacking of a systematic framework in demonstrating the service failures from online reviews. This research attempts to fill this gap.
RELATED PAPERS
Journal of Hospitality Marketing & Management
International Journal of Hospitality Management
Carlos Flavian
Dialogmarketing Perspektiven 2010/ …
Matt Muskat , Birgit Muskat
Anil Bilgihan
Aree Binprathan
Stanislav Ivanov
International Journal of Economics, Commerce and Management - United Kingdom
Journal of Vacation Marketing
Fabio Cassia , Francesca Magno
Bernadeth Petriana
Journal of Travel & Tourism Marketing
Alexander Trupp
International Journal of Hospitality & Tourism Systems
Publishing India Group
Sustainability
José Ramón Saura
Encontros Cientificos Tourism Management Studies
Francisco dos Anjos , Jéssica Meira
Anindya Ghose
Professor Dimitrios Buhalis
Nemanja Stanisic
Dafnis N Coudounaris
International Journal of Hospitality & Tourism Administration
Valentin VASSORT
Pablo Flores Limberger
SYED ZAWAAHAR BIN MOHAMED RABIK
alexandria.unisg.ch
Brigitte Stangl
ayzhan merbek
International Journal of Engineering & Technology
Angela S H Lee , Zuraini Zainol
Journal of Spatial and Organizational Dynamics
Journal of Tourism, Sustainability and Well-being (JTSW)
Roberta Minazzi
Hsiangting Chen , Kelly Virginia Phelan
Economic Problems of Tourism
Andrzej Mateusz Wajda , Simone Puorto
Betsy Stringam , Keith Mandabach
African Journal of Hospitality, Tourism and Leisure
Shohel Md. Nafi , Tanvir Ahmed
Roslizawati Che Aziz
Strategies of Adaptation in Tourist Communication. Leiden, Brill
Giuliana Fiorentino
RELATED TOPICS
- We're Hiring!
- Help Center
- Find new research papers in:
- Health Sciences
- Earth Sciences
- Cognitive Science
- Mathematics
- Computer Science
- Academia ©2024

Hotel assessment through social media – TripAdvisor as a case study
- Sebastian Molinillo University of Malaga Andalucia Tech Faculty of Economics and Business Management http://orcid.org/0000-0001-9132-5190
- Antonio Fernández-Morales University of Malaga Andalucia Tech Faculty of Economics and Business Management
- José Luis Ximénez-de-Sandoval University of Malaga Faculty of Tourism
- Andres Coca-Stefaniak University of Greenwich Business School
Hotel booking decisions are increasingly influenced by consumers’ feedback available on social media sites. Using data submitted by TripAdvisor users, this study provides four key contributions to knowledge. Firstly, a comparative analysis was carried out of customer ratings received by hotels located on Spain’s coastal areas as well as Portugal’s southern coast. Secondly, significant differences were found in the number of comments as well as the average online review rating score awarded, which were found to be related to the geographical location of each tourism destination. Thirdly, it is found that customers tend to rate their experiences in hotels positively. Fourthly, the level of overall customer satisfaction with a hotel tends to increase proportionately to the number of customer feedback comments posted for that hotel. As a result of this, one of the findings of this study is that hotels should encourage their customers to post comments on online customer review websites to balance out any negative feedback received.
Author Biographies
Associate Professor of Marketing
Department of Business Management
Department of Applied Economics (Statistics and Econometrics 15)
PhD Candidate
Program in Tourism Management and Planning
Senior Lecturer
Department of Marketing, Events and Tourism
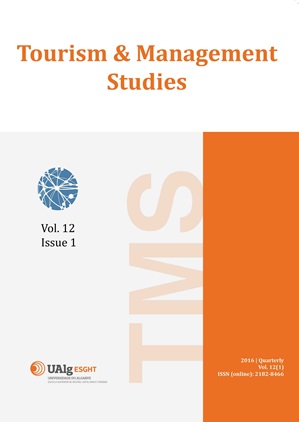
Copyright (c) 2016 Tourism & Management Studies
This work is licensed under a Creative Commons Attribution-NoDerivatives 4.0 International License .
The journal retains published articles’ copyrights, but they are simultaneously licensed under the Creative Commons Attribution License (CC BY-NC-ND), which allows individuals’ to share the relevant papers as long as authorship and publication in this journal are duly acknowledged.
How to Cite
- Endnote/Zotero/Mendeley (RIS)
Most read articles by the same author(s)
- Sebastian Molinillo, Francisco Liébana-Cabanillas, Rafael Anaya-Sánchez, Destination Image on the DMO's Platforms: Official Website and Social Media , Tourism & Management Studies: Vol. 13 No. 3 (2017)
- Javier Perez-Aranda, María Vallespín Arán, Sebastian Molinillo, A proposal for measuring hotels’ managerial responses to User-Generated-Content Reviews , Tourism & Management Studies: Vol. 14 (2018): Special Issue on Hospitality
Institutions
Conferences
TMS ALGARVE 2022
TMS ALGARVE 2018
TMS ALGARVE 2016
TMS ALGARVE 2014
TMS ALGARVE 2013
TMS ALGARVE 2012
TMS ALGARVE 2011
Do DMOs Promote the Right Aspects of the Destination? A Study of Instagram Photography with a Visual Classifier
- Conference paper
- Open Access
- First Online: 07 January 2022
- Cite this conference paper
You have full access to this open access conference paper
- Lyndon Nixon ORCID: orcid.org/0000-0001-7091-4543 4
Included in the following conference series:
- ENTER22 e-Tourism Conference
As global travel emerges from the pandemic, pent up interest in travel will lead to consumers making their choice between global destinations. Instagram is a key source of destination inspiration. DMO marketing success on this channel relies on projecting a destination image that resonates with this target group. However, usual text-based marketing intelligence on this channel does not work as content is consumed first and foremost as a visual projection. The author has built a deep learning based visual classifier for destination image measurement from photos. In this paper, we compare projected and perceived destination images in Instagram photography for four of the most Instagrammed destinations worldwide. We find that whereas the projected destination image aligns well to the perceived image, there are specific aspects of the destinations that are of more interest to Instagrammers than reflected in the current destination marketing.
You have full access to this open access chapter, Download conference paper PDF
Similar content being viewed by others
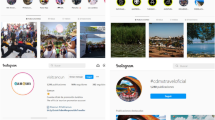
Quantifying differences between UGC and DMO’s image content on Instagram using deep learning
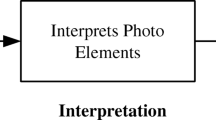
Is a picture worth a thousand views? Measuring the effects of travel photos on user engagement using deep learning algorithms
The Impact of Visual Social Media on the Projected Image of a Destination: The Case of Mexico City on Instagram
- Destination image
- Visual classification
- Instagram photography
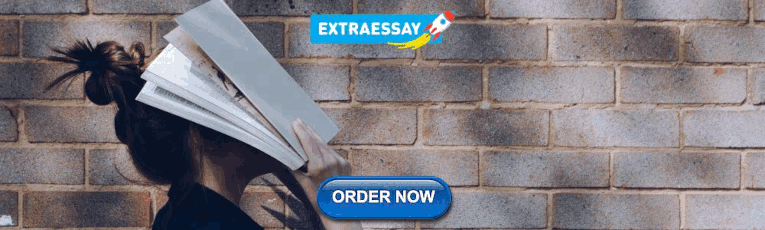
1 Introduction
Instagram may be the most significant source of destination inspiration for a significant number of travel-interested Internet users, especially in the 18–34 year old age group Footnote 1 . After all, 48% of Instagram users rely on the images and videos that they see on the social media platform to inform their travel decision making and 35% of users use the platform to discover new places Footnote 2 . It is therefore not surprising that destination marketing organisations (DMOs) have expended significant effort in marketing on this channel. Instagram is a highly visual social network and therefore content marketing is focused on the use of photography and videos, more so than the text of postings. When an Instagram user consumes destination marketing content, the intention of the marketer is that the visually projected image of the destination resonates with them and increases their interest and positive attitude towards the destination – leading to an intent to visit the destination. Multiple case studies have backed up the assumption that an optimally marketed destination on Instagram can lead to an increase in visitor numbers Footnote 3 . Indeed, Instagram is now being blamed for causing overtourism in certain “Instagrammable” locations Footnote 4 . A challenge for DMOs or other stakeholders is to know if they are indeed marketing their destination optimally on Instagram. A rise in visitor numbers can only suggest marketing success a posteriori. Marketing intelligence – the analysis of marketing data to identify the factors of marketing success – has classically made use of analysis of text (of reviews, or of social media postings) and quantifiable metrics (e.g., in social networks number of likes, comments and shares) [ 15 ]. However, the primary component of Instagram marketing is visual: the photo or video used in the posting. Ignoring this component in marketing intelligence in Instagram would mean the primary factor for the marketing success is being excluded from the analysis.
In this paper, our assumption is that the target audience for the destination marketing – the Instagram users – reveal the aspects they are most interested in and find most appealing in a visit to the destination through their own choice of the photography they post to the social network as part of their touristic experience. Photography has been long understood as an expression of the photographer’s mental image of the destination [ 19 ]. They take photos of the aspects they consider most indicative, and this is now even more the case in social networks where the user chooses which of their photos they want to post and share with others. Therefore, the content of Instagram photography from a destination (user generated content, or UGC) can be analysed for the “perceived destination image”. Equally, the content posted by the DMO on its Instagram channel can be subject to the same analysis to measure the “projected destination image” [ 11 ]. Common marketing theory says that marketers want to influence consumer attitudes towards their product and can measure the success of this influence by surveying consumers after a marketing campaign and determining if attitudes have become closer to the marketing message. In e-tourism, this has translated as marketers defining their intended “projected destination image” and measuring how close the “perceived destination image” comes to it after a marketing campaign. However, such thinking persists the assumption that marketers are the primary conveyors of the destinations image when in fact today’s traveller has a destination image which is strongly influenced by the UGC they consume about that destination [ 14 ], especially from social networks [ 25 ]. Therefore, we turn this theory on its head and argue that, in fact, destination marketers should inform themselves of the “perceived destination image” of their target audience and, given that this reflects the that resonate most with those consumers, aim to align their “projected destination image” to it.
Measuring destination image from Instagram photography is quite different from analysis of text or statistics. In the following chapter, we look at destination image in e-tourism research and how visual media classification has been researched in the field of computing. Section 3 introduces the author’s implementation of a deep learning-based visual classifier for measuring destination image and its evaluation results for accurate classification of tourism photography. Section 4 explains our experiment: we take four top Instagram destinations and download sets of DMO and UGC photos. We describe the measured destination image as multidimensional vectors and compare projected and perceived images including for selected features. We identify differences between the destination marketing and UGC. Section 5 concludes this paper with a look at our findings, what DMOs can learn from this for their future destination marketing and which limitations and future work remain.
2 State of the Art
Destination image has been a subject of tourism research since several decades [ 5 ]. It has been defined as the mental construct a person has of a destination and is sourced initially from indirect sources, i.e., before they visit the destination and form an opinion [ 8 ]. From the perspective of a group, the destination image may be considered the ideas and feelings most expressed about the destination (e.g., Costa Rica may be most typically associated with jungle and adventure). Destination image has been important as a concept to tourism stakeholders as a correlation is assumed between a positive image and an increased intention to visit [ 16 ]. Originally, destination marketers considered their marketing as significant in determining the image of their destination among a target audience, as their marketing materials were a primary source of impressions about the destination for someone who was exploring the option to visit. Nowadays this no longer holds as the Web and digital photography has led to an explosion of destination imagery from tourists which is globally distributed and easily consumed on popular Websites. This imagery is now dominant in forming an initial destination image among connected consumers [ 13 ]. Destination marketers now need to accept that Internet users participate in the co-creation of the destination brand [ 1 ], referring to the influence on the destination image of the user content being shared online [ 2 ].
Initial destination image research used either surveys and other solicitation techniques from the public or expert knowledge to define the attributes people commonly associate with destinations [ 8 ]. Measurement of destination image consists of determining a value for each attribute. Given a classification of those attributes, surveys were also used to directly extract the destination image for a given destination from a group of people, e.g. [ 3 ]. As more destination related content became available online through Websites, researchers turned from survey-based approaches to data-based approaches where they collected the data from the Web and used software tools to analyse it. Text analysis could be used with collections of reviews of tourism POIs (points of interest), e.g. [ 15 ]. However, this proves less effective with multimedia content (images and videos) where the only text that might be available would be an associated description, tags or even the content of the comments on that multimedia item.
Tourism researchers have long been aware of the importance of photography in understanding how visitors perceive a destination [ 6 ]. The choice of elements in the photos are seen as reflecting the photographer’s own idea of what is most important about the destination [ 19 ]. Initial research on destination image and photography solicited directly from participants their impressions from observing a certain photo, a technique known as PEI (Photo Elicitation Interview), which has also been applied with Instagram [ 9 ]. Later work manually classified the photos found on Websites according to their content or theme, e.g. [ 21 , 22 ]. Such efforts were largely small scale and did not concur in how to classify the photos, nor aligned their classifications to other research on destination image attributes [ 20 ].
With the advent of social networks and photo sharing, the scale of available photography about destinations has expanded greatly. The Web also drove “big data” research where analysis could be performed on larger scale data collections that could be sourced online and hence provide more accurate results. However, in the research domain of image understanding, accessible and accurate software systems have only emerged with the advent of deep learning-based approaches [ 24 ]. These use neural networks (AI systems that seek to replicate the neural activity of the brain) with multiple processing layers (hence “deep”) to ‘learn’ to identify the content of images based on training with large datasets of already classified images. State of the art deep learning classifiers have been trained with the ImageNet dataset, a set of 1.3 million photos annotated according to a list of 1 000 visual categories, reporting high levels of accuracy when evaluated against previously unseen image sets Footnote 5 . The classification abilities of deep neural networks are also available to users via Web based APIs hosted by companies such as Google (Cloud Vision), IBM (Watson Visual Recognition) or Microsoft (Azure Computer Vision). They tend to offer object recognition, i.e., classification of images based on the detection of visible objects, drawing from concept sets in the tens of thousands.
Tourism research using deep learning classifiers to analyse larger photo sets has only appeared in the past few years, cf. [ 7 , 12 , 26 , 27 , 28 ]. Off-the-shelf pre-trained classifiers have been used which return generic concepts for each image, largely based on the ImageNet classification. A few papers have acknowledged that this is not directly useable in the touristic context where destination image is defined as an aggregation of more general cognitive attributes (such as “entertainment”) rather than the specific objects explicitly visible in the image (such as “guitar”, “turntables” or “stage”). The classifiers are not trained specifically on tourist photography and the few experiments to measure the accuracy of a classifier when used in the tourism domain report lower figures than for the ImageNet classification for which evaluation results are reported. [ 18 ] evaluated two pre-trained classifiers with Instagram photography of Vienna and found f1 measures (the harmonic mean of precision and recall) of 0.54. [ 12 ] found an accuracy score of 28% for their classifier when tested specifically in the use case of touristic destination image. Past work has also not mapped the labels returned by the off-the-shelf classifiers to destination image attributes, a task made more complex by the lack of any official listing of all labels.
There is the option to train a deep learning neural network specifically for visual classification in some new domain, given that the ImageNet pre-training is accepted to be too generic when classification is to be done for a specific use case. While networks could be trained from scratch, transfer learning is a common approach to reach high accuracy with less training cycles. Here, we start with the pre-trained model from ImageNet and then train the new model with the domain-specific annotated images. However, to the best of the author’s knowledge, no-one has trained a visual classifier specifically for destination image measurement. In the next chapter, we introduce the author’s implementation and evaluation of such a system.
3 Implementation of a Visual Classifier for Destination Image
Given the lack of an accurate classifier for destination image from photography, we decided to implement our own. While the leading benchmark systems in (general) visual classification tend to be highly complex (hundreds of layers and ten of millions of parameters) they also need expensive, powerful hardware to run. In our case, we are considering a more specific task (narrow domain) and aim for a model which can be trained and used with more common computing resources (e.g., a laptop with one GPU, or in cloud services such as Google Colab). We develop in Python, using the Keras open-source library for developing deep learning networks.
The standard workflow for a deep learning model is to prepare training data, build a pipeline to input the data, build a model, train the model with the data, test the model and then iterate on improving the model. To prepare training data, we need to decide on the set of visual categories for which the classifier will be trained then prepare a set of data for training which consists of tourism photography where each photo is annotated as belonging to one of those visual categories (every photo is considered as belonging to a single category. Multi-label classification is a subject for future work). Finally, train a model with the data and measure the accuracy on the test data set (we follow a standard convention of taking 20% of the data for testing).
To choose the visual categories for the classifier, we want to use the commonly accepted cognitive attributes of destination image as defined in the research literature. However, there is no single, widely accepted definition of destination image attributes. We start with the factors influencing destination image defined by [ 4 ] as the authors aimed for a comprehensive aggregation of all attributes that are considered in a destination. [ 17 ] wanted to measure destination image from Instagram photography (through a survey) and took a subset of the list in [ 4 ] which was best suited to cognitive identification (tangibly visible in an image). This led to a new list of 53 attributes where some attributes were more specific than [ 4 ] (e.g., “Flora and Fauna” became “Plants & Flowers”, “Animals” and “Trees”). In a further refinement step, the author took this list of 53 attributes and cleaned it to 18 visually distinct categories based on aggregations (e.g., “Concerts” and “Cinema/Theatre” could be grouped into “Entertainment”) and filtering out categories that are difficult to generalise for classification (e.g., “Arts and Crafts” and “Traditions” would look very different from place to place) (Table 1 ):
To create the training data, we have noted that available classifiers have used public large scale annotated photo datasets such as ImageNet. However, these datasets are not annotated with tourism photography and destination image in mind and tend towards generic object detection tasks such as identifying a “car”, “dog” or “football”. Therefore, we found it important to source a new image training dataset specifically for our task. There is no golden rule for how many images are needed for training each category but following the TensorFlow tutorial for visual classification Footnote 6 where 3670 photos were used to train for 5 classes i.e., 734 photos per class, we also aimed to average at several hundred photos for each category. To find photos suitably representative of tourism photography in each category, we used Google Images search with the conjunctive query “tourism AND (label)” where label is the name of the visual category (categories like “plants & flowers” were split into two queries). The Fatkun Batch Bild plugin was used to download 500–800 images per category and the result was manually filtered to colour photographs without any overlays, leading to 100–500 photos per category for the training dataset. The final training dataset has 4949 photos, or an average of 275 photos per category. After training, the photos were discarded.
The initial architecture is a Convolutional Neural Network (CNN) with three convolution blocks, a max pooling layer in each, a fully connected layer on top with 128 units activated by a relu activation function and, in our case another fully connected layer with 18 units which outputs the result (a confidence value for each of the 18 visual categories). While the initial CNN had an accuracy score of 63–68% on the test dataset (after 20 epochs), we decided to explore the use of transfer learning to train our new model on top of the visual classification already learnt by an existing, re-usable model. Several models are available in Keras for transfer learning and after experimenting with results from a few options, we chose InceptionResNetV2 which reports the best results on the ImageNet validation dataset yet is not the most complex to train with (56 million parameters) Footnote 7 . Further experimentation with the settings for the model training led us to choose max pooling and making all layers trainable. We loaded InceptionResNetV2 without the last two convolutional layers and added three convolution blocks with a max pooling layer in each, as in our original CNN. We run this new model for 10 epochs, and this model scored 90% accuracy on the test dataset.
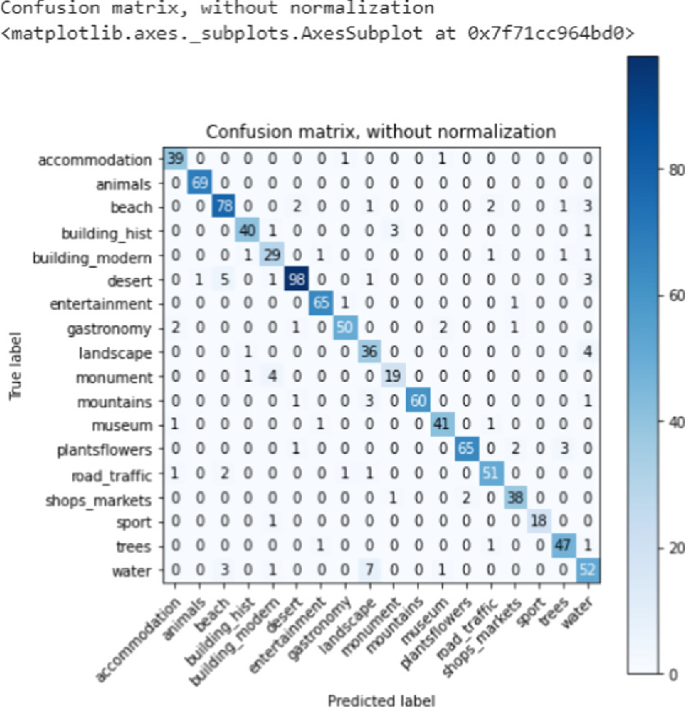
Confusion matrix for classification of the test dataset
We produced a confusion matrix to check if the accuracy is consistent across the categories (Fig. 1 . NB the 18 visual categories can be seen on the vertical axis in the order they are classified). In the test dataset (983 images), 29 out of 34 images (85%) labelled building_modern and 19 out of 24 images (79%) labelled monument were predicted correctly with the main confusion being 4 monument images labelled as building_modern (out of 24). Other confusions visible in the matrix are desert images labelled as beach (5), landscape as water (4) and water as landscape (7), all of which are understandable given the common-sense similarities (deserts and beaches both have sand, landscapes also contain water). Overall, the classifier has correctly labelled the vast majority of images and has been consistent across categories.
4 Experiment
Having made available a visual classification model that can annotate photography according to a shortlist of destination image cognitive attributes with high accuracy, we will use the classifier to compare the perceived and projected destination image of a number of top destinations according to the Instagram photos posted by users and by the DMO respectively. Based on lists of most Instagrammed locations, we chose the cities of Paris, Barcelona and New York as well as the country of the Maldives, which also complement one another in the sense of each being quite distinct as a destination (Paris: romantic, historical; Barcelona: beach, modernism; New York: urban, entertainment; Maldives: water, relaxation). We use a Python library to download Instagram photos for each destination (i) from its official DMO account and (ii) according to the DMO recommended travel hashtag (this helps focus our data collection of tourism-related photography). We discard the photos once we have classified them. Table 2 shows the selected account and hashtag and the number of photos acquired for each (the downloading took place in the second half of August 2021):
The classifier labels each individual photo with a set of 18 confidence scores (on a scale of 0 to 1), one for each visual category (in the order seen in Fig. 1 ). We accept one label per photo in that we select the visual category with the highest confidence score. Given an input of multiple photos, we can produce an array of 18 integers where each integer is the sum of photos labelled with a respective visual category. Vectors are representations of objects in multi-dimensional space. Following the use of vectors to represent data items in embedding layers of neural networks, where the items can be subsequently compared and learnt about (e.g., similar items are closer together and can be clustered), we will interpret the destination image array produced by the visual classifier as an 18-dimensional vector. Such a vector can be understood as a set of feature weights where the features are the visual categories and the weights are the presence of the feature in the dataset (to allow for comparison, all features need to be at the same scale so we take the no. of photos labelled with the feature divided by no. photos in the dataset to produce for every feature a value between 0 and 1). For example, the vector produced for the projected destination image (the DMO account) of Maldives is: [0.01663366 0.00871287 0.3829703 0.00435644 0.02336634 0.02336634 0.02930693 0.02534653 0.02336634 0.01029703 0.0150495 0.00475248 0.03960396 0.01584158 0.01346535 0.01029703 0.00990099 0.34336634] . The two dominant features are clearly beach (in the 3 rd position with a value of 0.383) and water (in the last position with a value of 0.343) as all other features (all other destination image attributes) are not larger than 0.04. We can say that the DMO marketing of the Maldives (projected image) is heavily based on the beach and water attributes. We can use the cosine similarity of two vectors to determine how close the projected image (DMO) is to the perceived image (UGC) of the destination. Cosine similarity measures similarity in terms of the orientation of the vectors rather than the magnitude, which makes sense for the representation of destination image in n-dimensional space since each weight represents a feature of the destination and the overall image is determined by the comparative relationship of the feature values (e.g., beach and water is stronger than everything else) rather than the absolute values themselves. The resulting cosine similarity measure between the Maldives’ projected and perceived destination images is 0.983 (cf. Table 3 ), indicating no significant differences. It seems Maldives visitors equally post in their majority beach and water photos. We can consider the Maldives case a validation of our approach with the visual classifier, as the Maldives as a destination is arguably limited to the two visual categories of beach and water (it seems neither accommodation nor gastronomy form a focus of destination photography there) and therefore it could be expected that these two features dominate both the projected and perceived images of the Maldives. How about cities like New York, Paris or Barcelona, which contain many different features that could be subjects of photography? Do the DMOs present a different image of the city than the visitors themselves? Table 3 shows the cosine similarity for each destination image, i.e., the closeness of the vectors of the destinations projected destination image (DMO account) and perceived destination image (UGC).
There is a close similarity in all cases, suggesting the projected and perceived destination images are well aligned. On one hand, DMOs can achieve this by reusing UGC in their own destination marketing, a common approach today on platforms like Instagram. On the other hand, it suggests that Instagram users do share the same perception of the destination as the one being promoted by the destination marketers – a possible proof of the notion of the hermeneutic circle of representation [ 23 ]. This is the idea that we replicate and reinforce the media depictions we already know (in Paris, we have to photograph the Eiffel Tower, etc.). [ 10 ] considered that tourist photography may either reflect or inform a destination image since, consciously or unconsciously, tourists look for scenes that replicate their existing perceptions. While this result is positive for the DMO, there may some differences in the images which we can explore by looking at specific features (visual categories) in the destination image vector. Figure 2 shows comparisons of the city destination images along three features that all of the cities are known for: gastronomy, historical buildings and modern buildings.
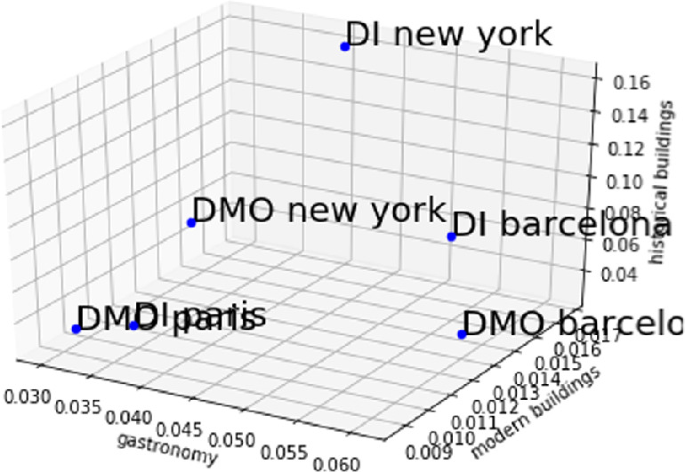
3D plot of the destination images of the cities along three features (DI = perceived image, DMO = projected image)
While the projected DMO imagery of Paris is closest to the perceived image in UGC (both points almost overlap), Instagrammers focus more on all three features than the DMO in New York (it may be the DMO is actively working on promoting other features of NYC) whereas in Barcelona the DMO tends to feature more gastronomy than the perceived destination image (which more strongly features both historical and modern buildings in the city). In particular, the New York DMO might be advised to focus more on gastronomy and historical buildings in NYC as this is certainly of more interest to Instagrammers, and the Barcelona DMO should consider that Instagrammers are attracted to both the historical and modern architecture of the city more than is reflected in their destination marketing.
5 Conclusions
In this paper, we described our own deep learning visual classifier for tourism photography which can be used to measure destination image and demonstrated its accuracy. We used this classifier with both DMO and UGC photography from Instagram for four popular destinations and considered how the resulting destination image, measured as an 18-dimensional vector, may be analysed for destination marketing insights. We found that projected and perceived images align well in Instagram, so DMOs are actively projecting an image which resonates well with Instagram users’ perceptions of the destination. However, along individual features differences can be found. Assuming DMOs are well advised to align their projected images to the perceived image on Instagram, New York could promote more gastronomy and historical buildings and Barcelona could promote more its architecture, both historical and modern. We believe the measurement of destination image using our visual classifier can provide new insights into how destinations are presented through visual media such as photographs, which is an area of Internet marketing still not satisfactorily covered in business intelligence systems. Other experiments could be to compare the images of different destinations, to identify changes in destination images over time, or differences in destination image across different groups. As a limitation, we restricted our destination image attributes to 18 categories which were not only visually distinct but also globally consistent. Categories like arts & crafts or traditions were not considered as their visual appearance would vary greatly from place to place. Future work would be to both further improve the accuracy of the classifier, especially considering very visually different destinations, and to add further categories (related to the previous point, possibly ad hoc based on the classification task). The work could also be applied to video, which is ‘moving images’, although the temporal dimension should also be considered (i.e., how long a feature is visible for). The Python code for training a CNN with transfer learning for destination image classification as well as the weights of our best performing model (90% accuracy) are made available publicly on Github https://github.com/lyndonnixon/VisualDestinationClassifier as well as a labelled set of Flickr photographs for evaluating visual classifiers on Google Drive https://bit.ly/visualdestination . We hope thereby to encourage further research in visual classification for destination image and further experiments with tourism photography and videos.
62.8% of the users are aged 18–34 according to the Digital Internet Snapshot 2021 https://datareportal.com/reports/digital-2021-global-overview-report (last accessed on 24 March 2021).
Rezab, J. “Instagram: The Place To Be For Travel Brands”. Available online. https://www.mediapost.com/publications/article/263167/instagram-the-place-to-be-for-travel-brands.html (last accessed on 28 July 2021. Requires free sign-in.).
e.g. Iceland and New Zealand case studies from “Insta tourism explained: What, why and where”. Available online. https://tourismteacher.com/insta-tourism/ (last accessed on 28 July 2021).
Tenbarge, K. “15 destinations Instagram has helped ruin” Available online. https://www.insider.com/travel-destinations-instagram-influencers-ruined-2019-7 (last accessed on 28 July 2021).
https://keras.io/api/applications/ lists pre-trained models available in the Python library Keras with top-5 accuracy scores of 89 to 96%.
https://www.tensorflow.org/tutorials/images/classification .
InceptionResNetV2 Simple Introduction, by Zahra Elhamraoui, Medium, May 16, 2020. https://medium.com/@zahraelhamraoui1997/inceptionresnetv2-simple-introduction-9a2000edcdb6 (last accessed August 31, 2021).
Abbate T, Codini A, Aquilani B (2019) Knowledge co-creation in Open Innovation digital platforms: processes, tools and services. J Bus Ind Mark 34:1434–1447. https://doi.org/10.1108/jbim-09-2018-0276
Article Google Scholar
Agrawal A, Kaushik A, Rahman Z (2015) Co-creation of social value through integration of stakeholders. Procedia Soc Behav Sci 189:442–448. https://doi.org/10.1016/j.sbspro.2015.03.198
Baloglu S, McCleary K (1999) A model of destination image formation. Ann Tour Res 26(4):868–897. https://doi.org/10.1016/s0160-7383(99)00030-4
Beerli A, Martín JD (2004) Factors influencing destination image. Ann Tour Res 31(3):657–681. https://doi.org/10.1016/j.annals.2004.01.010
Crompton JL (1979) Motivations for pleasure vacation. Ann Tour Res 6(4):408–424. https://doi.org/10.1016/0160-7383(79)90004-5
Day J, Skidmore S, Koller T (2002) Image selection in destination positioning: a new approach. J Vacat Mark 8(2):177–186. https://doi.org/10.1177/135676670200800207
Deng N, Li X (2018) Feeling a destination through the “right” photos: a machine learning model for DMOs’ photo selection. Tour Manag 65:267–278. https://doi.org/10.1016/j.tourman.2017.09.010
Echtner CM, Ritchie JB (1991) The meaning and measurement of destination image. J Tour Stud 2(2):2–12
Google Scholar
Fatanti MN, Suyadnya, IW (2015) Beyond user gaze: how instagram creates tourism destination brand? Procedia Soc Behav Sci 211:1089–1095. https://doi.org/10.1016/j.sbspro.2015.11.145
Garrod B (2008) Understanding the relationship between tourism destination imagery and tourist photography. J Travel Res 47(3):346–358. https://doi.org/10.1177/0047287508322785
Kim H, Richardson SL (2003) Motion picture impacts on destination image. Ann Tour Res 30(1):216–237. https://doi.org/10.1016/s0160-7383(02)00062-2
Kim D, Kang Y, Park Y, Kim N, Lee J (2019) Understanding tourists’ urban images with geotagged photos using convolutional neural networks. Spat Inf Res 28(2):241–255. https://doi.org/10.1007/s41324-019-00285-x
Marchiori E, Cantoni L (2015) The role of prior experience in the perception of a tourism destination in user-generated content. J Destin Mark Manag 4(3):194–201. https://doi.org/10.1016/j.jdmm.2015.06.001
Marine-Roig E, Anton Clavé S (2015) Tourism analytics with massive user-generated content: a case study of Barcelona. J Destin Mark Manag 4(3):162–172. https://doi.org/10.1016/j.jdmm.2015.06.004
Miguéns J, Baggio R, Costa C (2008) Social media and tourism destinations: TripAdvisor case study. Adv Tour Res 26(28):1–6
Molinillo S, Liébana-Cabanillas F, Anaya-Sánchez R, Buhalis D (2018) DMO online platforms: image and intention to visit. Tour Manag 65:116–130. https://doi.org/10.1016/j.tourman.2017.09.021
Nixon L, Popova A, Önder I (2017) How instagram influences visual destination image: a case study of Jordan and Costa Rica. In: ENTER2017 conference, Rome, Italy
Nixon L (2018) Assessing the usefulness of online image annotation services for destination image measurement. In: ENTER2018 conference, Jönköping, Sweden
Pan S, Lee J, Tsai H (2014) Travel photos: Motivations, image dimensions, and affective qualities of places. Tour Manag 40:59–69. https://doi.org/10.1016/j.tourman.2013.05.007
Picazo P, Moreno-Gil S (2017) Analysis of the projected image of tourism destinations on photographs: a literature review to prepare for the future. J Vacat Mark 25(1):3–24. https://doi.org/10.1177/1356766717736350
Stepchenkova S, Zhan F (2013) Visual destination images of Peru: comparative content analysis of DMO and user-generated photography. Tour Manag 36:590–601. https://doi.org/10.1016/j.tourman.2012.08.006
Tussyadiah P (2010) Destination-promoted and visitor-generated images - do they represent similar stories? In: Tourism and visual culture, vol 2. Method and cases, Chap. 13
Urry J (2002) The tourist gaze. Sage. https://doi.org/10.1079/9781845936112.0156
Voulodimos A, Doulamis N, Doulamis A, Protopapadakis E (2018) Deep learning for computer vision: a brief review. Comput Intell Neurosci 2018. https://doi.org/10.1155/2018/7068349
Xiang Z, Magnini VP, Fesenmaier DR (2015) Information technology and consumer behavior in travel and tourism: insights from travel planning using the internet. J Retail Consum Serv 22:244–249. https://doi.org/10.1016/j.jretconser.2014.08.005
Xiao X, Fang C, Lin H (2020) Characterizing tourism destination image using photos’ visual content. ISPRS Int J Geo Inf 9(12):730. https://doi.org/10.3390/ijgi9120730
Zhang K, Chen Y, Li C (2019) Discovering the tourists’ behaviors and perceptions in a tourism destination by analyzing photos’ visual content with a computer deep learning model: the case of Beijing. Tour Manag 75:595–608. https://doi.org/10.1016/j.tourman.2019.07.002
Zhang K, Chen Y, Lin Z (2020) Mapping destination images and behavioral patterns from user-generated photos: a computer vision approach. Asia Pac J Tour Res 25(11):1199–1214. https://doi.org/10.1080/10941665.2020.1838586
Download references
Author information
Authors and affiliations.
School of Applied Data Science, MODUL University Vienna, Am Kahlenberg 1, 1190, Vienna, Austria
Lyndon Nixon
You can also search for this author in PubMed Google Scholar
Corresponding author
Correspondence to Lyndon Nixon .
Editor information
Editors and affiliations.
Modul Private University Vienna, Wien, Wien, Austria
Jason L. Stienmetz
University of Lleida, Lleida, Spain
Berta Ferrer-Rosell
Free University of Bozen-Bolzano, Bolzano, Italy
David Massimo
Rights and permissions
Open Access This chapter is licensed under the terms of the Creative Commons Attribution 4.0 International License ( http://creativecommons.org/licenses/by/4.0/ ), which permits use, sharing, adaptation, distribution and reproduction in any medium or format, as long as you give appropriate credit to the original author(s) and the source, provide a link to the Creative Commons license and indicate if changes were made.
The images or other third party material in this chapter are included in the chapter's Creative Commons license, unless indicated otherwise in a credit line to the material. If material is not included in the chapter's Creative Commons license and your intended use is not permitted by statutory regulation or exceeds the permitted use, you will need to obtain permission directly from the copyright holder.
Reprints and permissions
Copyright information
© 2022 The Author(s)
About this paper
Cite this paper.
Nixon, L. (2022). Do DMOs Promote the Right Aspects of the Destination? A Study of Instagram Photography with a Visual Classifier. In: Stienmetz, J.L., Ferrer-Rosell, B., Massimo, D. (eds) Information and Communication Technologies in Tourism 2022. ENTER 2022. Springer, Cham. https://doi.org/10.1007/978-3-030-94751-4_16
Download citation
DOI : https://doi.org/10.1007/978-3-030-94751-4_16
Published : 07 January 2022
Publisher Name : Springer, Cham
Print ISBN : 978-3-030-94750-7
Online ISBN : 978-3-030-94751-4
eBook Packages : Business and Management Business and Management (R0)
Share this paper
Anyone you share the following link with will be able to read this content:
Sorry, a shareable link is not currently available for this article.
Provided by the Springer Nature SharedIt content-sharing initiative
- Publish with us
Policies and ethics
- Find a journal
- Track your research
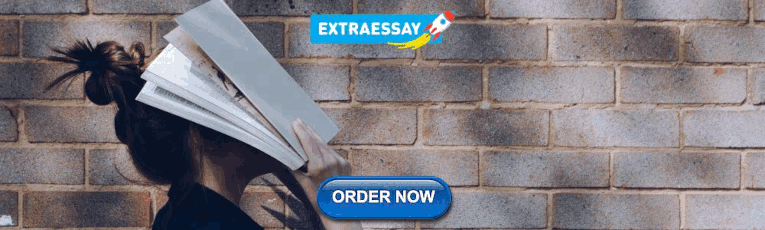
COMMENTS
Social media and T ourism Destinations: T ripAdvisor Case S tudy. J. Miguéns, R. Baggio, and C. Costa. Abst ract — Online social networking site are the most popular sites on the internet. The ...
This paper examines CGC on TripAdvisor, with a case study on the city of Lisbon, where it is explored how the users collaborate to image a destination. Online social networking site are the most popular sites on the internet. The second generation of web based services is characterized by having a consumer generated content (CGC), which allow people to share information.
The Tripadvisor ranking order is also based on the popularity of the hotels by tourist preferences, and other -3- J. MIGUÉNS ET AL: SOCIAL MEDIA AND TOURISM DESTINATION: TRIPADVISOR CASE STUDY not available measurement. The results are displayed in Table 1. Hotel rating is actually ordered in accordance with the traditional start rating.
Download Free PDF. View PDF. IASK ATR2008 (Advances in Tourism Research 2008), Aveiro, Portugal, May. 26-28 Social media and Tourism Destinations: TripAdvisor Case Study J. Miguéns, R. Baggio, and C. Costa Abstract — Online social networking site are the most popular sites on the internet.
Social media and Tourism Destinations: TripAdvisor Case Study ARTICLE CITATIONS 7 DOWNLOADS 149 VIEWS 21 3 AUTHORS, INCLUDING: R. Baggio Università commerciale Luigi Bocconi 66 PUBLICATIONS 339 ...
In other words, this study is expected to make some contributions in the following aspects: (1) pointing out the growth of scientific research on destination branding and social media since 2011 until 2021, as well as indicating the part of the world most contributing in terms of research on this topic, and also distinguishing journals that are ...
Abstract. This case study explores TripAdvisor in order to understand its role in social media within the tourism landscape and specifically, in relation to the "Open Innovation in Tourism" phenomenon. The key features, business model and innovation approaches of TripAdvisor are analysed and its value added services for travellers as well ...
Social Media and Tourism Destinations: TripAdvisor Case Study. In: The Proceedings of IASK ART2008 (Advances in Tourism Research 2008). Aveiro , Portugal, May
Researchers seeking robust insights into the spatial distribution of visitors within given destinations are making increasing use of social media user-generated content (UGC) harvested from sources such as TripAdvisor.com, which sheds light on matters such as visitor concentrations and tourist interests.
The influence of social media on travel decision-making has attracted much attention from tourism scholars. A recent literature analysis has suggested that most of such studies have focused on the impact of social media on behavioural intention with very limited studies on actual behaviour (Leung et al., 2019).Furthermore, relatively little insight has been put on the roles of social media in ...
This document discusses social media and tourism, using TripAdvisor as a case study. It analyzes TripAdvisor reviews and profiles of hotels and forum authors in Lisbon, Portugal. TripAdvisor allows users to generate consumer content by posting reviews, photos, videos and participating in discussion forums about tourism locations and services. This user-generated content provides valuable ...
This study chose TripAdvisor to analyze guest reviews of hotels in Oporto, elected European Best Destination in 2012 and 2014 and the Best European Destination by the Lonely Planet in 2013. ... Social Media and Tourism: The Case of E-Complaints on TripAdvisor (An Extended Abstract). In: Rossi, P. (eds) Marketing at the Confluence between ...
In the form of case studies of TripAdvisor and Booking.com, the current rise of trust in reviews on these review sites was analysed and documented. ... Hubert van Hoof, and Dimitrios Buhalis. 2013. Social Media in Tourism and Hospitality: A Literature Review. Journal of Travel & Tourism Marketing 30: 3-22 ... Social media in destination ...
TripAdvisor. The study expands on similar research carried out H┞ O Cラミミラ (2010) in England, but rather focusing on seaside hotels in Spain and southern Portugal, which remain largely under-researched in the field of tourism social media, despite their economic and geographical relevance in the European
Abstract and Figures. Hotel booking decisions are increasingly influenced by consumer feedback available on social media sites. Using data submitted by customers on TripAdvisor, this study ...
Tourism & Management Studies, 12(1) (2016), 15-24 DOI: 10.18089/tms.2016.12102 Hotel Assessment through Social Media: The case of TripAdvisor La valoración de los hoteles en los medios sociales: El caso de TripAdvisor Sebastian Molinillo University of Malaga, Faculty of Economics and Business, Department of Business Management, Campus El Ejido ...
TABLE 2 - "Social media and Tourism Destination: TripAdvisor Case Study" ... "Social media and Tourism Destination: TripAdvisor Case Study" Skip to search form Skip to main content Skip to account menu. Semantic Scholar's Logo. Search 217,307,042 papers from all fields of science.
The main part of the study focuses on how the National Tourism Organization, the Ministry of Tourism, the prefecture of Thessaly and tourism-related enterprises of the region use social media. The extent of usage and promotion is measured through data collected from Tripadvisor, Facebook and official national tourism websites.
Hotel booking decisions are increasingly influenced by consumers' feedback available on social media sites. Using data submitted by TripAdvisor users, this study provides four key contributions to knowledge. Firstly, a comparative analysis was carried out of customer ratings received by hotels located on Spain's coastal areas as well as Portugal's southern coast.
Semi nyak, as the most popular. destination, has the b iggest total of vacation rentals at 50%. Ranking second among the po pular destinations, Ubud has 15% which is less than Canggu, which is in ...
Instagram may be the most significant source of destination inspiration for a significant number of travel-interested Internet users, especially in the 18-34 year old age group Footnote 1.After all, 48% of Instagram users rely on the images and videos that they see on the social media platform to inform their travel decision making and 35% of users use the platform to discover new places ...
Consumer generated content is rapidly gaining traction as part of the purchase decision making process. After examining the implications for travel businesses, this paper focuses on TripAdvisor.com, the largest online network of travel consumers to establish its current practices and challenges. Using a sample of London hotels, it was shown ...
TripAdvisor.com; (2) Information about the top five popular destinations in Bali with reference to number of reviews, number of restaurants, number of hotels, and number of things to do were gathered.