The role of data science in healthcare advancements: applications, benefits, and future prospects
Affiliations.
- 1 Department of Electrical and Electronics Engineering, Manipal Institute of Technology, Manipal Academy of Higher Education, Manipal, Karnataka, India.
- 2 Department of Humanities and Management, Manipal Institute of Technology, Manipal Academy of Higher Education, Manipal, Karnataka, India.
- 3 Department of Oral Medicine and Radiology, Manipal College of Dental Sciences, Manipal, Manipal Academy of Higher Education, Manipal, Karnataka, India. [email protected].
- 4 Department of Urology, Father Muller Medical College, Mangalore, Karnataka, India.
- 5 Department of Radiation Oncology, Massachusetts General Hospital, Boston, MA, USA.
- 6 Department of Oral Medicine and Radiology, Manipal College of Dental Sciences, Manipal, Manipal Academy of Higher Education, Manipal, Karnataka, India.
- 7 Department of Mechanical and Manufacturing Engineering, Manipal Institute of Technology, Manipal Academy of Higher Education, Manipal, Karnataka, India.
- 8 Department of Urology, University Hospital Southampton NHS Trust, Southampton, UK.
- PMID: 34398394
- PMCID: PMC9308575
- DOI: 10.1007/s11845-021-02730-z
Data science is an interdisciplinary field that extracts knowledge and insights from many structural and unstructured data, using scientific methods, data mining techniques, machine-learning algorithms, and big data. The healthcare industry generates large datasets of useful information on patient demography, treatment plans, results of medical examinations, insurance, etc. The data collected from the Internet of Things (IoT) devices attract the attention of data scientists. Data science provides aid to process, manage, analyze, and assimilate the large quantities of fragmented, structured, and unstructured data created by healthcare systems. This data requires effective management and analysis to acquire factual results. The process of data cleansing, data mining, data preparation, and data analysis used in healthcare applications is reviewed and discussed in the article. The article provides an insight into the status and prospects of big data analytics in healthcare, highlights the advantages, describes the frameworks and techniques used, briefs about the challenges faced currently, and discusses viable solutions. Data science and big data analytics can provide practical insights and aid in the decision-making of strategic decisions concerning the health system. It helps build a comprehensive view of patients, consumers, and clinicians. Data-driven decision-making opens up new possibilities to boost healthcare quality.
Keywords: Big data; Data analytics; Data mining; Healthcare; Healthcare informatics.
© 2021. The Author(s).
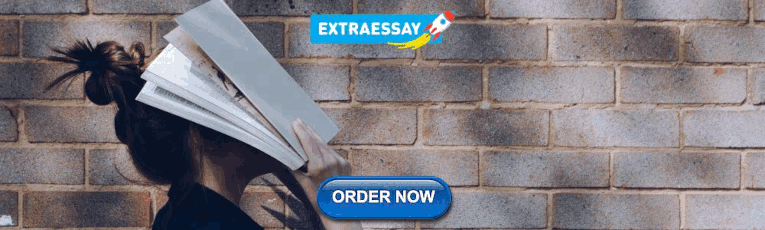
Publication types
- Data Mining / methods
- Data Science*
- Delivery of Health Care
- Machine Learning
- Open access
- Published: 06 January 2022
The use of Big Data Analytics in healthcare
- Kornelia Batko ORCID: orcid.org/0000-0001-6561-3826 1 &
- Andrzej Ślęzak 2
Journal of Big Data volume 9 , Article number: 3 ( 2022 ) Cite this article
69k Accesses
93 Citations
28 Altmetric
Metrics details
The introduction of Big Data Analytics (BDA) in healthcare will allow to use new technologies both in treatment of patients and health management. The paper aims at analyzing the possibilities of using Big Data Analytics in healthcare. The research is based on a critical analysis of the literature, as well as the presentation of selected results of direct research on the use of Big Data Analytics in medical facilities. The direct research was carried out based on research questionnaire and conducted on a sample of 217 medical facilities in Poland. Literature studies have shown that the use of Big Data Analytics can bring many benefits to medical facilities, while direct research has shown that medical facilities in Poland are moving towards data-based healthcare because they use structured and unstructured data, reach for analytics in the administrative, business and clinical area. The research positively confirmed that medical facilities are working on both structural data and unstructured data. The following kinds and sources of data can be distinguished: from databases, transaction data, unstructured content of emails and documents, data from devices and sensors. However, the use of data from social media is lower as in their activity they reach for analytics, not only in the administrative and business but also in the clinical area. It clearly shows that the decisions made in medical facilities are highly data-driven. The results of the study confirm what has been analyzed in the literature that medical facilities are moving towards data-based healthcare, together with its benefits.
Introduction
The main contribution of this paper is to present an analytical overview of using structured and unstructured data (Big Data) analytics in medical facilities in Poland. Medical facilities use both structured and unstructured data in their practice. Structured data has a predetermined schema, it is extensive, freeform, and comes in variety of forms [ 27 ]. In contrast, unstructured data, referred to as Big Data (BD), does not fit into the typical data processing format. Big Data is a massive amount of data sets that cannot be stored, processed, or analyzed using traditional tools. It remains stored but not analyzed. Due to the lack of a well-defined schema, it is difficult to search and analyze such data and, therefore, it requires a specific technology and method to transform it into value [ 20 , 68 ]. Integrating data stored in both structured and unstructured formats can add significant value to an organization [ 27 ]. Organizations must approach unstructured data in a different way. Therefore, the potential is seen in Big Data Analytics (BDA). Big Data Analytics are techniques and tools used to analyze and extract information from Big Data. The results of Big Data analysis can be used to predict the future. They also help in creating trends about the past. When it comes to healthcare, it allows to analyze large datasets from thousands of patients, identifying clusters and correlation between datasets, as well as developing predictive models using data mining techniques [ 60 ].
This paper is the first study to consolidate and characterize the use of Big Data from different perspectives. The first part consists of a brief literature review of studies on Big Data (BD) and Big Data Analytics (BDA), while the second part presents results of direct research aimed at diagnosing the use of big data analyses in medical facilities in Poland.
Healthcare is a complex system with varied stakeholders: patients, doctors, hospitals, pharmaceutical companies and healthcare decision-makers. This sector is also limited by strict rules and regulations. However, worldwide one may observe a departure from the traditional doctor-patient approach. The doctor becomes a partner and the patient is involved in the therapeutic process [ 14 ]. Healthcare is no longer focused solely on the treatment of patients. The priority for decision-makers should be to promote proper health attitudes and prevent diseases that can be avoided [ 81 ]. This became visible and important especially during the Covid-19 pandemic [ 44 ].
The next challenges that healthcare will have to face is the growing number of elderly people and a decline in fertility. Fertility rates in the country are found below the reproductive minimum necessary to keep the population stable [ 10 ]. The reflection of both effects, namely the increase in age and lower fertility rates, are demographic load indicators, which is constantly growing. Forecasts show that providing healthcare in the form it is provided today will become impossible in the next 20 years [ 70 ]. It is especially visible now during the Covid-19 pandemic when healthcare faced quite a challenge related to the analysis of huge data amounts and the need to identify trends and predict the spread of the coronavirus. The pandemic showed it even more that patients should have access to information about their health condition, the possibility of digital analysis of this data and access to reliable medical support online. Health monitoring and cooperation with doctors in order to prevent diseases can actually revolutionize the healthcare system. One of the most important aspects of the change necessary in healthcare is putting the patient in the center of the system.
Technology is not enough to achieve these goals. Therefore, changes should be made not only at the technological level but also in the management and design of complete healthcare processes and what is more, they should affect the business models of service providers. The use of Big Data Analytics is becoming more and more common in enterprises [ 17 , 54 ]. However, medical enterprises still cannot keep up with the information needs of patients, clinicians, administrators and the creator’s policy. The adoption of a Big Data approach would allow the implementation of personalized and precise medicine based on personalized information, delivered in real time and tailored to individual patients.
To achieve this goal, it is necessary to implement systems that will be able to learn quickly about the data generated by people within clinical care and everyday life. This will enable data-driven decision making, receiving better personalized predictions about prognosis and responses to treatments; a deeper understanding of the complex factors and their interactions that influence health at the patient level, the health system and society, enhanced approaches to detecting safety problems with drugs and devices, as well as more effective methods of comparing prevention, diagnostic, and treatment options [ 40 ].
In the literature, there is a lot of research showing what opportunities can be offered to companies by big data analysis and what data can be analyzed. However, there are few studies showing how data analysis in the area of healthcare is performed, what data is used by medical facilities and what analyses and in which areas they carry out. This paper aims to fill this gap by presenting the results of research carried out in medical facilities in Poland. The goal is to analyze the possibilities of using Big Data Analytics in healthcare, especially in Polish conditions. In particular, the paper is aimed at determining what data is processed by medical facilities in Poland, what analyses they perform and in what areas, and how they assess their analytical maturity. In order to achieve this goal, a critical analysis of the literature was performed, and the direct research was based on a research questionnaire conducted on a sample of 217 medical facilities in Poland. It was hypothesized that medical facilities in Poland are working on both structured and unstructured data and moving towards data-based healthcare and its benefits. Examining the maturity of healthcare facilities in the use of Big Data and Big Data Analytics is crucial in determining the potential future benefits that the healthcare sector can gain from Big Data Analytics. There is also a pressing need to predicate whether, in the coming years, healthcare will be able to cope with the threats and challenges it faces.
This paper is divided into eight parts. The first is the introduction which provides background and the general problem statement of this research. In the second part, this paper discusses considerations on use of Big Data and Big Data Analytics in Healthcare, and then, in the third part, it moves on to challenges and potential benefits of using Big Data Analytics in healthcare. The next part involves the explanation of the proposed method. The result of direct research and discussion are presented in the fifth part, while the following part of the paper is the conclusion. The seventh part of the paper presents practical implications. The final section of the paper provides limitations and directions for future research.
Considerations on use Big Data and Big Data Analytics in the healthcare
In recent years one can observe a constantly increasing demand for solutions offering effective analytical tools. This trend is also noticeable in the analysis of large volumes of data (Big Data, BD). Organizations are looking for ways to use the power of Big Data to improve their decision making, competitive advantage or business performance [ 7 , 54 ]. Big Data is considered to offer potential solutions to public and private organizations, however, still not much is known about the outcome of the practical use of Big Data in different types of organizations [ 24 ].
As already mentioned, in recent years, healthcare management worldwide has been changed from a disease-centered model to a patient-centered model, even in value-based healthcare delivery model [ 68 ]. In order to meet the requirements of this model and provide effective patient-centered care, it is necessary to manage and analyze healthcare Big Data.
The issue often raised when it comes to the use of data in healthcare is the appropriate use of Big Data. Healthcare has always generated huge amounts of data and nowadays, the introduction of electronic medical records, as well as the huge amount of data sent by various types of sensors or generated by patients in social media causes data streams to constantly grow. Also, the medical industry generates significant amounts of data, including clinical records, medical images, genomic data and health behaviors. Proper use of the data will allow healthcare organizations to support clinical decision-making, disease surveillance, and public health management. The challenge posed by clinical data processing involves not only the quantity of data but also the difficulty in processing it.
In the literature one can find many different definitions of Big Data. This concept has evolved in recent years, however, it is still not clearly understood. Nevertheless, despite the range and differences in definitions, Big Data can be treated as a: large amount of digital data, large data sets, tool, technology or phenomenon (cultural or technological.
Big Data can be considered as massive and continually generated digital datasets that are produced via interactions with online technologies [ 53 ]. Big Data can be defined as datasets that are of such large sizes that they pose challenges in traditional storage and analysis techniques [ 28 ]. A similar opinion about Big Data was presented by Ohlhorst who sees Big Data as extremely large data sets, possible neither to manage nor to analyze with traditional data processing tools [ 57 ]. In his opinion, the bigger the data set, the more difficult it is to gain any value from it.
In turn, Knapp perceived Big Data as tools, processes and procedures that allow an organization to create, manipulate and manage very large data sets and storage facilities [ 38 ]. From this point of view, Big Data is identified as a tool to gather information from different databases and processes, allowing users to manage large amounts of data.
Similar perception of the term ‘Big Data’ is shown by Carter. According to him, Big Data technologies refer to a new generation of technologies and architectures, designed to economically extract value from very large volumes of a wide variety of data by enabling high velocity capture, discovery and/or analysis [ 13 ].
Jordan combines these two approaches by identifying Big Data as a complex system, as it needs data bases for data to be stored in, programs and tools to be managed, as well as expertise and personnel able to retrieve useful information and visualization to be understood [ 37 ].
Following the definition of Laney for Big Data, it can be state that: it is large amount of data generated in very fast motion and it contains a lot of content [ 43 ]. Such data comes from unstructured sources, such as stream of clicks on the web, social networks (Twitter, blogs, Facebook), video recordings from the shops, recording of calls in a call center, real time information from various kinds of sensors, RFID, GPS devices, mobile phones and other devices that identify and monitor something [ 8 ]. Big Data is a powerful digital data silo, raw, collected with all sorts of sources, unstructured and difficult, or even impossible, to analyze using conventional techniques used so far to relational databases.
While describing Big Data, it cannot be overlooked that the term refers more to a phenomenon than to specific technology. Therefore, instead of defining this phenomenon, trying to describe them, more authors are describing Big Data by giving them characteristics included a collection of V’s related to its nature [ 2 , 3 , 23 , 25 , 58 ]:
Volume (refers to the amount of data and is one of the biggest challenges in Big Data Analytics),
Velocity (speed with which new data is generated, the challenge is to be able to manage data effectively and in real time),
Variety (heterogeneity of data, many different types of healthcare data, the challenge is to derive insights by looking at all available heterogenous data in a holistic manner),
Variability (inconsistency of data, the challenge is to correct the interpretation of data that can vary significantly depending on the context),
Veracity (how trustworthy the data is, quality of the data),
Visualization (ability to interpret data and resulting insights, challenging for Big Data due to its other features as described above).
Value (the goal of Big Data Analytics is to discover the hidden knowledge from huge amounts of data).
Big Data is defined as an information asset with high volume, velocity, and variety, which requires specific technology and method for its transformation into value [ 21 , 77 ]. Big Data is also a collection of information about high-volume, high volatility or high diversity, requiring new forms of processing in order to support decision-making, discovering new phenomena and process optimization [ 5 , 7 ]. Big Data is too large for traditional data-processing systems and software tools to capture, store, manage and analyze, therefore it requires new technologies [ 28 , 50 , 61 ] to manage (capture, aggregate, process) its volume, velocity and variety [ 9 ].
Undoubtedly, Big Data differs from the data sources used so far by organizations. Therefore, organizations must approach this type of unstructured data in a different way. First of all, organizations must start to see data as flows and not stocks—this entails the need to implement the so-called streaming analytics [ 48 ]. The mentioned features make it necessary to use new IT tools that allow the fullest use of new data [ 58 ]. The Big Data idea, inseparable from the huge increase in data available to various organizations or individuals, creates opportunities for access to valuable analyses, conclusions and enables making more accurate decisions [ 6 , 11 , 59 ].
The Big Data concept is constantly evolving and currently it does not focus on huge amounts of data, but rather on the process of creating value from this data [ 52 ]. Big Data is collected from various sources that have different data properties and are processed by different organizational units, resulting in creation of a Big Data chain [ 36 ]. The aim of the organizations is to manage, process and analyze Big Data. In the healthcare sector, Big Data streams consist of various types of data, namely [ 8 , 51 ]:
clinical data, i.e. data obtained from electronic medical records, data from hospital information systems, image centers, laboratories, pharmacies and other organizations providing health services, patient generated health data, physician’s free-text notes, genomic data, physiological monitoring data [ 4 ],
biometric data provided from various types of devices that monitor weight, pressure, glucose level, etc.,
financial data, constituting a full record of economic operations reflecting the conducted activity,
data from scientific research activities, i.e. results of research, including drug research, design of medical devices and new methods of treatment,
data provided by patients, including description of preferences, level of satisfaction, information from systems for self-monitoring of their activity: exercises, sleep, meals consumed, etc.
data from social media.
These data are provided not only by patients but also by organizations and institutions, as well as by various types of monitoring devices, sensors or instruments [ 16 ]. Data that has been generated so far in the healthcare sector is stored in both paper and digital form. Thus, the essence and the specificity of the process of Big Data analyses means that organizations need to face new technological and organizational challenges [ 67 ]. The healthcare sector has always generated huge amounts of data and this is connected, among others, with the need to store medical records of patients. However, the problem with Big Data in healthcare is not limited to an overwhelming volume but also an unprecedented diversity in terms of types, data formats and speed with which it should be analyzed in order to provide the necessary information on an ongoing basis [ 3 ]. It is also difficult to apply traditional tools and methods for management of unstructured data [ 67 ]. Due to the diversity and quantity of data sources that are growing all the time, advanced analytical tools and technologies, as well as Big Data analysis methods which can meet and exceed the possibilities of managing healthcare data, are needed [ 3 , 68 ].
Therefore, the potential is seen in Big Data analyses, especially in the aspect of improving the quality of medical care, saving lives or reducing costs [ 30 ]. Extracting from this tangle of given association rules, patterns and trends will allow health service providers and other stakeholders in the healthcare sector to offer more accurate and more insightful diagnoses of patients, personalized treatment, monitoring of the patients, preventive medicine, support of medical research and health population, as well as better quality of medical services and patient care while, at the same time, the ability to reduce costs (Fig. 1 ).
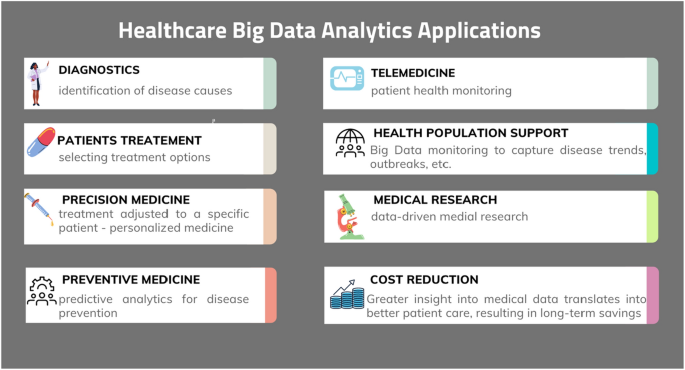
(Source: Own elaboration)
Healthcare Big Data Analytics applications
The main challenge with Big Data is how to handle such a large amount of information and use it to make data-driven decisions in plenty of areas [ 64 ]. In the context of healthcare data, another major challenge is to adjust big data storage, analysis, presentation of analysis results and inference basing on them in a clinical setting. Data analytics systems implemented in healthcare are designed to describe, integrate and present complex data in an appropriate way so that it can be understood better (Fig. 2 ). This would improve the efficiency of acquiring, storing, analyzing and visualizing big data from healthcare [ 71 ].
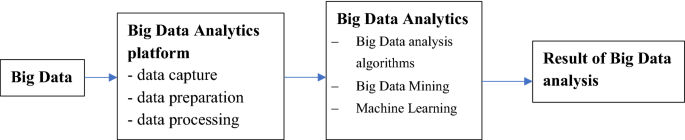
Process of Big Data Analytics
The result of data processing with the use of Big Data Analytics is appropriate data storytelling which may contribute to making decisions with both lower risk and data support. This, in turn, can benefit healthcare stakeholders. To take advantage of the potential massive amounts of data in healthcare and to ensure that the right intervention to the right patient is properly timed, personalized, and potentially beneficial to all components of the healthcare system such as the payer, patient, and management, analytics of large datasets must connect communities involved in data analytics and healthcare informatics [ 49 ]. Big Data Analytics can provide insight into clinical data and thus facilitate informed decision-making about the diagnosis and treatment of patients, prevention of diseases or others. Big Data Analytics can also improve the efficiency of healthcare organizations by realizing the data potential [ 3 , 62 ].
Big Data Analytics in medicine and healthcare refers to the integration and analysis of a large amount of complex heterogeneous data, such as various omics (genomics, epigenomics, transcriptomics, proteomics, metabolomics, interactomics, pharmacogenetics, deasomics), biomedical data, talemedicine data (sensors, medical equipment data) and electronic health records data [ 46 , 65 ].
When analyzing the phenomenon of Big Data in the healthcare sector, it should be noted that it can be considered from the point of view of three areas: epidemiological, clinical and business.
From a clinical point of view, the Big Data analysis aims to improve the health and condition of patients, enable long-term predictions about their health status and implementation of appropriate therapeutic procedures. Ultimately, the use of data analysis in medicine is to allow the adaptation of therapy to a specific patient, that is personalized medicine (precision, personalized medicine).
From an epidemiological point of view, it is desirable to obtain an accurate prognosis of morbidity in order to implement preventive programs in advance.
In the business context, Big Data analysis may enable offering personalized packages of commercial services or determining the probability of individual disease and infection occurrence. It is worth noting that Big Data means not only the collection and processing of data but, most of all, the inference and visualization of data necessary to obtain specific business benefits.
In order to introduce new management methods and new solutions in terms of effectiveness and transparency, it becomes necessary to make data more accessible, digital, searchable, as well as analyzed and visualized.
Erickson and Rothberg state that the information and data do not reveal their full value until insights are drawn from them. Data becomes useful when it enhances decision making and decision making is enhanced only when analytical techniques are used and an element of human interaction is applied [ 22 ].
Thus, healthcare has experienced much progress in usage and analysis of data. A large-scale digitalization and transparency in this sector is a key statement of almost all countries governments policies. For centuries, the treatment of patients was based on the judgment of doctors who made treatment decisions. In recent years, however, Evidence-Based Medicine has become more and more important as a result of it being related to the systematic analysis of clinical data and decision-making treatment based on the best available information [ 42 ]. In the healthcare sector, Big Data Analytics is expected to improve the quality of life and reduce operational costs [ 72 , 82 ]. Big Data Analytics enables organizations to improve and increase their understanding of the information contained in data. It also helps identify data that provides insightful insights for current as well as future decisions [ 28 ].
Big Data Analytics refers to technologies that are grounded mostly in data mining: text mining, web mining, process mining, audio and video analytics, statistical analysis, network analytics, social media analytics and web analytics [ 16 , 25 , 31 ]. Different data mining techniques can be applied on heterogeneous healthcare data sets, such as: anomaly detection, clustering, classification, association rules as well as summarization and visualization of those Big Data sets [ 65 ]. Modern data analytics techniques explore and leverage unique data characteristics even from high-speed data streams and sensor data [ 15 , 16 , 31 , 55 ]. Big Data can be used, for example, for better diagnosis in the context of comprehensive patient data, disease prevention and telemedicine (in particular when using real-time alerts for immediate care), monitoring patients at home, preventing unnecessary hospital visits, integrating medical imaging for a wider diagnosis, creating predictive analytics, reducing fraud and improving data security, better strategic planning and increasing patients’ involvement in their own health.
Big Data Analytics in healthcare can be divided into [ 33 , 73 , 74 ]:
descriptive analytics in healthcare is used to understand past and current healthcare decisions, converting data into useful information for understanding and analyzing healthcare decisions, outcomes and quality, as well as making informed decisions [ 33 ]. It can be used to create reports (i.e. about patients’ hospitalizations, physicians’ performance, utilization management), visualization, customized reports, drill down tables, or running queries on the basis of historical data.
predictive analytics operates on past performance in an effort to predict the future by examining historical or summarized health data, detecting patterns of relationships in these data, and then extrapolating these relationships to forecast. It can be used to i.e. predict the response of different patient groups to different drugs (dosages) or reactions (clinical trials), anticipate risk and find relationships in health data and detect hidden patterns [ 62 ]. In this way, it is possible to predict the epidemic spread, anticipate service contracts and plan healthcare resources. Predictive analytics is used in proper diagnosis and for appropriate treatments to be given to patients suffering from certain diseases [ 39 ].
prescriptive analytics—occurs when health problems involve too many choices or alternatives. It uses health and medical knowledge in addition to data or information. Prescriptive analytics is used in many areas of healthcare, including drug prescriptions and treatment alternatives. Personalized medicine and evidence-based medicine are both supported by prescriptive analytics.
discovery analytics—utilizes knowledge about knowledge to discover new “inventions” like drugs (drug discovery), previously unknown diseases and medical conditions, alternative treatments, etc.
Although the models and tools used in descriptive, predictive, prescriptive, and discovery analytics are different, many applications involve all four of them [ 62 ]. Big Data Analytics in healthcare can help enable personalized medicine by identifying optimal patient-specific treatments. This can influence the improvement of life standards, reduce waste of healthcare resources and save costs of healthcare [ 56 , 63 , 71 ]. The introduction of large data analysis gives new analytical possibilities in terms of scope, flexibility and visualization. Techniques such as data mining (computational pattern discovery process in large data sets) facilitate inductive reasoning and analysis of exploratory data, enabling scientists to identify data patterns that are independent of specific hypotheses. As a result, predictive analysis and real-time analysis becomes possible, making it easier for medical staff to start early treatments and reduce potential morbidity and mortality. In addition, document analysis, statistical modeling, discovering patterns and topics in document collections and data in the EHR, as well as an inductive approach can help identify and discover relationships between health phenomena.
Advanced analytical techniques can be used for a large amount of existing (but not yet analytical) data on patient health and related medical data to achieve a better understanding of the information and results obtained, as well as to design optimal clinical pathways [ 62 ]. Big Data Analytics in healthcare integrates analysis of several scientific areas such as bioinformatics, medical imaging, sensor informatics, medical informatics and health informatics [ 65 ]. Big Data Analytics in healthcare allows to analyze large datasets from thousands of patients, identifying clusters and correlation between datasets, as well as developing predictive models using data mining techniques [ 65 ]. Discussing all the techniques used for Big Data Analytics goes beyond the scope of a single article [ 25 ].
The success of Big Data analysis and its accuracy depend heavily on the tools and techniques used to analyze the ability to provide reliable, up-to-date and meaningful information to various stakeholders [ 12 ]. It is believed that the implementation of big data analytics by healthcare organizations could bring many benefits in the upcoming years, including lowering health care costs, better diagnosis and prediction of diseases and their spread, improving patient care and developing protocols to prevent re-hospitalization, optimizing staff, optimizing equipment, forecasting the need for hospital beds, operating rooms, treatments, and improving the drug supply chain [ 71 ].
Challenges and potential benefits of using Big Data Analytics in healthcare
Modern analytics gives possibilities not only to have insight in historical data, but also to have information necessary to generate insight into what may happen in the future. Even when it comes to prediction of evidence-based actions. The emphasis on reform has prompted payers and suppliers to pursue data analysis to reduce risk, detect fraud, improve efficiency and save lives. Everyone—payers, providers, even patients—are focusing on doing more with fewer resources. Thus, some areas in which enhanced data and analytics can yield the greatest results include various healthcare stakeholders (Table 1 ).
Healthcare organizations see the opportunity to grow through investments in Big Data Analytics. In recent years, by collecting medical data of patients, converting them into Big Data and applying appropriate algorithms, reliable information has been generated that helps patients, physicians and stakeholders in the health sector to identify values and opportunities [ 31 ]. It is worth noting that there are many changes and challenges in the structure of the healthcare sector. Digitization and effective use of Big Data in healthcare can bring benefits to every stakeholder in this sector. A single doctor would benefit the same as the entire healthcare system. Potential opportunities to achieve benefits and effects from Big Data in healthcare can be divided into four groups [ 8 ]:
Improving the quality of healthcare services:
assessment of diagnoses made by doctors and the manner of treatment of diseases indicated by them based on the decision support system working on Big Data collections,
detection of more effective, from a medical point of view, and more cost-effective ways to diagnose and treat patients,
analysis of large volumes of data to reach practical information useful for identifying needs, introducing new health services, preventing and overcoming crises,
prediction of the incidence of diseases,
detecting trends that lead to an improvement in health and lifestyle of the society,
analysis of the human genome for the introduction of personalized treatment.
Supporting the work of medical personnel
doctors’ comparison of current medical cases to cases from the past for better diagnosis and treatment adjustment,
detection of diseases at earlier stages when they can be more easily and quickly cured,
detecting epidemiological risks and improving control of pathogenic spots and reaction rates,
identification of patients who are predicted to have the highest risk of specific, life-threatening diseases by collating data on the history of the most common diseases, in healing people with reports entering insurance companies,
health management of each patient individually (personalized medicine) and health management of the whole society,
capturing and analyzing large amounts of data from hospitals and homes in real time, life monitoring devices to monitor safety and predict adverse events,
analysis of patient profiles to identify people for whom prevention should be applied, lifestyle change or preventive care approach,
the ability to predict the occurrence of specific diseases or worsening of patients’ results,
predicting disease progression and its determinants, estimating the risk of complications,
detecting drug interactions and their side effects.
Supporting scientific and research activity
supporting work on new drugs and clinical trials thanks to the possibility of analyzing “all data” instead of selecting a test sample,
the ability to identify patients with specific, biological features that will take part in specialized clinical trials,
selecting a group of patients for which the tested drug is likely to have the desired effect and no side effects,
using modeling and predictive analysis to design better drugs and devices.
Business and management
reduction of costs and counteracting abuse and counseling practices,
faster and more effective identification of incorrect or unauthorized financial operations in order to prevent abuse and eliminate errors,
increase in profitability by detecting patients generating high costs or identifying doctors whose work, procedures and treatment methods cost the most and offering them solutions that reduce the amount of money spent,
identification of unnecessary medical activities and procedures, e.g. duplicate tests.
According to research conducted by Wang, Kung and Byrd, Big Data Analytics benefits can be classified into five categories: IT infrastructure benefits (reducing system redundancy, avoiding unnecessary IT costs, transferring data quickly among healthcare IT systems, better use of healthcare systems, processing standardization among various healthcare IT systems, reducing IT maintenance costs regarding data storage), operational benefits (improving the quality and accuracy of clinical decisions, processing a large number of health records in seconds, reducing the time of patient travel, immediate access to clinical data to analyze, shortening the time of diagnostic test, reductions in surgery-related hospitalizations, exploring inconceivable new research avenues), organizational benefits (detecting interoperability problems much more quickly than traditional manual methods, improving cross-functional communication and collaboration among administrative staffs, researchers, clinicians and IT staffs, enabling data sharing with other institutions and adding new services, content sources and research partners), managerial benefits (gaining quick insights about changing healthcare trends in the market, providing members of the board and heads of department with sound decision-support information on the daily clinical setting, optimizing business growth-related decisions) and strategic benefits (providing a big picture view of treatment delivery for meeting future need, creating high competitive healthcare services) [ 73 ].
The above specification does not constitute a full list of potential areas of use of Big Data Analysis in healthcare because the possibilities of using analysis are practically unlimited. In addition, advanced analytical tools allow to analyze data from all possible sources and conduct cross-analyses to provide better data insights [ 26 ]. For example, a cross-analysis can refer to a combination of patient characteristics, as well as costs and care results that can help identify the best, in medical terms, and the most cost-effective treatment or treatments and this may allow a better adjustment of the service provider’s offer [ 62 ].
In turn, the analysis of patient profiles (e.g. segmentation and predictive modeling) allows identification of people who should be subject to prophylaxis, prevention or should change their lifestyle [ 8 ]. Shortened list of benefits for Big Data Analytics in healthcare is presented in paper [ 3 ] and consists of: better performance, day-to-day guides, detection of diseases in early stages, making predictive analytics, cost effectiveness, Evidence Based Medicine and effectiveness in patient treatment.
Summarizing, healthcare big data represents a huge potential for the transformation of healthcare: improvement of patients’ results, prediction of outbreaks of epidemics, valuable insights, avoidance of preventable diseases, reduction of the cost of healthcare delivery and improvement of the quality of life in general [ 1 ]. Big Data also generates many challenges such as difficulties in data capture, data storage, data analysis and data visualization [ 15 ]. The main challenges are connected with the issues of: data structure (Big Data should be user-friendly, transparent, and menu-driven but it is fragmented, dispersed, rarely standardized and difficult to aggregate and analyze), security (data security, privacy and sensitivity of healthcare data, there are significant concerns related to confidentiality), data standardization (data is stored in formats that are not compatible with all applications and technologies), storage and transfers (especially costs associated with securing, storing, and transferring unstructured data), managerial skills, such as data governance, lack of appropriate analytical skills and problems with Real-Time Analytics (health care is to be able to utilize Big Data in real time) [ 4 , 34 , 41 ].
The research is based on a critical analysis of the literature, as well as the presentation of selected results of direct research on the use of Big Data Analytics in medical facilities in Poland.
Presented research results are part of a larger questionnaire form on Big Data Analytics. The direct research was based on an interview questionnaire which contained 100 questions with 5-point Likert scale (1—strongly disagree, 2—I rather disagree, 3—I do not agree, nor disagree, 4—I rather agree, 5—I definitely agree) and 4 metrics questions. The study was conducted in December 2018 on a sample of 217 medical facilities (110 private, 107 public). The research was conducted by a specialized market research agency: Center for Research and Expertise of the University of Economics in Katowice.
When it comes to direct research, the selected entities included entities financed from public sources—the National Health Fund (23.5%), and entities operating commercially (11.5%). In the surveyed group of entities, more than a half (64.9%) are hybrid financed, both from public and commercial sources. The diversity of the research sample also applies to the size of the entities, defined by the number of employees. Taking into account proportions of the surveyed entities, it should be noted that in the sector structure, medium-sized (10–50 employees—34% of the sample) and large (51–250 employees—27%) entities dominate. The research was of all-Poland nature, and the entities included in the research sample come from all of the voivodships. The largest group were entities from Łódzkie (32%), Śląskie (18%) and Mazowieckie (18%) voivodships, as these voivodships have the largest number of medical institutions. Other regions of the country were represented by single units. The selection of the research sample was random—layered. As part of medical facilities database, groups of private and public medical facilities have been identified and the ones to which the questionnaire was targeted were drawn from each of these groups. The analyses were performed using the GNU PSPP 0.10.2 software.
The aim of the study was to determine whether medical facilities in Poland use Big Data Analytics and if so, in which areas. Characteristics of the research sample is presented in Table 2 .
The research is non-exhaustive due to the incomplete and uneven regional distribution of the samples, overrepresented in three voivodeships (Łódzkie, Mazowieckie and Śląskie). The size of the research sample (217 entities) allows the authors of the paper to formulate specific conclusions on the use of Big Data in the process of its management.
For the purpose of this paper, the following research hypotheses were formulated: (1) medical facilities in Poland are working on both structured and unstructured data (2) medical facilities in Poland are moving towards data-based healthcare and its benefits.
The paper poses the following research questions and statements that coincide with the selected questions from the research questionnaire:
From what sources do medical facilities obtain data? What types of data are used by the particular organization, whether structured or unstructured, and to what extent?
From what sources do medical facilities obtain data?
In which area organizations are using data and analytical systems (clinical or business)?
Is data analytics performed based on historical data or are predictive analyses also performed?
Determining whether administrative and medical staff receive complete, accurate and reliable data in a timely manner?
Determining whether real-time analyses are performed to support the particular organization’s activities.
Results and discussion
On the basis of the literature analysis and research study, a set of questions and statements related to the researched area was formulated. The results from the surveys show that medical facilities use a variety of data sources in their operations. These sources are both structured and unstructured data (Table 3 ).
According to the data provided by the respondents, considering the first statement made in the questionnaire, almost half of the medical institutions (47.58%) agreed that they rather collect and use structured data (e.g. databases and data warehouses, reports to external entities) and 10.57% entirely agree with this statement. As much as 23.35% of representatives of medical institutions stated “I agree or disagree”. Other medical facilities do not collect and use structured data (7.93%) and 6.17% strongly disagree with the first statement. Also, the median calculated based on the obtained results (median: 4), proves that medical facilities in Poland collect and use structured data (Table 4 ).
In turn, 28.19% of the medical institutions agreed that they rather collect and use unstructured data and as much as 9.25% entirely agree with this statement. The number of representatives of medical institutions that stated “I agree or disagree” was 27.31%. Other medical facilities do not collect and use structured data (17.18%) and 13.66% strongly disagree with the first statement. In the case of unstructured data the median is 3, which means that the collection and use of this type of data by medical facilities in Poland is lower.
In the further part of the analysis, it was checked whether the size of the medical facility and form of ownership have an impact on whether it analyzes unstructured data (Tables 4 and 5 ). In order to find this out, correlation coefficients were calculated.
Based on the calculations, it can be concluded that there is a small statistically monotonic correlation between the size of the medical facility and its collection and use of structured data (p < 0.001; τ = 0.16). This means that the use of structured data is slightly increasing in larger medical facilities. The size of the medical facility is more important according to use of unstructured data (p < 0.001; τ = 0.23) (Table 4 .).
To determine whether the form of medical facility ownership affects data collection, the Mann–Whitney U test was used. The calculations show that the form of ownership does not affect what data the organization collects and uses (Table 5 ).
Detailed information on the sources of from which medical facilities collect and use data is presented in the Table 6 .
The questionnaire results show that medical facilities are especially using information published in databases, reports to external units and transaction data, but they also use unstructured data from e-mails, medical devices, sensors, phone calls, audio and video data (Table 6 ). Data from social media, RFID and geolocation data are used to a small extent. Similar findings are concluded in the literature studies.
From the analysis of the answers given by the respondents, more than half of the medical facilities have integrated hospital system (HIS) implemented. As much as 43.61% use integrated hospital system and 16.30% use it extensively (Table 7 ). 19.38% of exanimated medical facilities do not use it at all. Moreover, most of the examined medical facilities (34.80% use it, 32.16% use extensively) conduct medical documentation in an electronic form, which gives an opportunity to use data analytics. Only 4.85% of medical facilities don’t use it at all.
Other problems that needed to be investigated were: whether medical facilities in Poland use data analytics? If so, in what form and in what areas? (Table 8 ). The analysis of answers given by the respondents about the potential of data analytics in medical facilities shows that a similar number of medical facilities use data analytics in administration and business (31.72% agreed with the statement no. 5 and 12.33% strongly agreed) as in the clinical area (33.04% agreed with the statement no. 6 and 12.33% strongly agreed). When considering decision-making issues, 35.24% agree with the statement "the organization uses data and analytical systems to support business decisions” and 8.37% of respondents strongly agree. Almost 40.09% agree with the statement that “the organization uses data and analytical systems to support clinical decisions (in the field of diagnostics and therapy)” and 15.42% of respondents strongly agree. Exanimated medical facilities use in their activity analytics based both on historical data (33.48% agree with statement 7 and 12.78% strongly agree) and predictive analytics (33.04% agrees with the statement number 8 and 15.86% strongly agree). Detailed results are presented in Table 8 .
Medical facilities focus on development in the field of data processing, as they confirm that they conduct analytical planning processes systematically and analyze new opportunities for strategic use of analytics in business and clinical activities (38.33% rather agree and 10.57% strongly agree with this statement). The situation is different with real-time data analysis, here, the situation is not so optimistic. Only 28.19% rather agree and 14.10% strongly agree with the statement that real-time analyses are performed to support an organization’s activities.
When considering whether a facility’s performance in the clinical area depends on the form of ownership, it can be concluded that taking the average and the Mann–Whitney U test depends. A higher degree of use of analyses in the clinical area can be observed in public institutions.
Whether a medical facility performs a descriptive or predictive analysis do not depend on the form of ownership (p > 0.05). It can be concluded that when analyzing the mean and median, they are higher in public facilities, than in private ones. What is more, the Mann–Whitney U test shows that these variables are dependent from each other (p < 0.05) (Table 9 ).
When considering whether a facility’s performance in the clinical area depends on its size, it can be concluded that taking the Kendall’s Tau (τ) it depends (p < 0.001; τ = 0.22), and the correlation is weak but statistically important. This means that the use of data and analytical systems to support clinical decisions (in the field of diagnostics and therapy) increases with the increase of size of the medical facility. A similar relationship, but even less powerful, can be found in the use of descriptive and predictive analyses (Table 10 ).
Considering the results of research in the area of analytical maturity of medical facilities, 8.81% of medical facilities stated that they are at the first level of maturity, i.e. an organization has developed analytical skills and does not perform analyses. As much as 13.66% of medical facilities confirmed that they have poor analytical skills, while 38.33% of the medical facility has located itself at level 3, meaning that “there is a lot to do in analytics”. On the other hand, 28.19% believe that analytical capabilities are well developed and 6.61% stated that analytics are at the highest level and the analytical capabilities are very well developed. Detailed data is presented in Table 11 . Average amounts to 3.11 and Median to 3.
The results of the research have enabled the formulation of following conclusions. Medical facilities in Poland are working on both structured and unstructured data. This data comes from databases, transactions, unstructured content of emails and documents, devices and sensors. However, the use of data from social media is smaller. In their activity, they reach for analytics in the administrative and business, as well as in the clinical area. Also, the decisions made are largely data-driven.
In summary, analysis of the literature that the benefits that medical facilities can get using Big Data Analytics in their activities relate primarily to patients, physicians and medical facilities. It can be confirmed that: patients will be better informed, will receive treatments that will work for them, will have prescribed medications that work for them and not be given unnecessary medications [ 78 ]. Physician roles will likely change to more of a consultant than decision maker. They will advise, warn, and help individual patients and have more time to form positive and lasting relationships with their patients in order to help people. Medical facilities will see changes as well, for example in fewer unnecessary hospitalizations, resulting initially in less revenue, but after the market adjusts, also the accomplishment [ 78 ]. The use of Big Data Analytics can literally revolutionize the way healthcare is practiced for better health and disease reduction.
The analysis of the latest data reveals that data analytics increase the accuracy of diagnoses. Physicians can use predictive algorithms to help them make more accurate diagnoses [ 45 ]. Moreover, it could be helpful in preventive medicine and public health because with early intervention, many diseases can be prevented or ameliorated [ 29 ]. Predictive analytics also allows to identify risk factors for a given patient, and with this knowledge patients will be able to change their lives what, in turn, may contribute to the fact that population disease patterns may dramatically change, resulting in savings in medical costs. Moreover, personalized medicine is the best solution for an individual patient seeking treatment. It can help doctors decide the exact treatments for those individuals. Better diagnoses and more targeted treatments will naturally lead to increases in good outcomes and fewer resources used, including doctors’ time.
The quantitative analysis of the research carried out and presented in this article made it possible to determine whether medical facilities in Poland use Big Data Analytics and if so, in which areas. Thanks to the results obtained it was possible to formulate the following conclusions. Medical facilities are working on both structured and unstructured data, which comes from databases, transactions, unstructured content of emails and documents, devices and sensors. According to analytics, they reach for analytics in the administrative and business, as well as in the clinical area. It clearly showed that the decisions made are largely data-driven. The results of the study confirm what has been analyzed in the literature. Medical facilities are moving towards data-based healthcare and its benefits.
In conclusion, Big Data Analytics has the potential for positive impact and global implications in healthcare. Future research on the use of Big Data in medical facilities will concern the definition of strategies adopted by medical facilities to promote and implement such solutions, as well as the benefits they gain from the use of Big Data analysis and how the perspectives in this area are seen.
Practical implications
This work sought to narrow the gap that exists in analyzing the possibility of using Big Data Analytics in healthcare. Showing how medical facilities in Poland are doing in this respect is an element that is part of global research carried out in this area, including [ 29 , 32 , 60 ].
Limitations and future directions
The research described in this article does not fully exhaust the questions related to the use of Big Data Analytics in Polish healthcare facilities. Only some of the dimensions characterizing the use of data by medical facilities in Poland have been examined. In order to get the full picture, it would be necessary to examine the results of using structured and unstructured data analytics in healthcare. Future research may examine the benefits that medical institutions achieve as a result of the analysis of structured and unstructured data in the clinical and management areas and what limitations they encounter in these areas. For this purpose, it is planned to conduct in-depth interviews with chosen medical facilities in Poland. These facilities could give additional data for empirical analyses based more on their suggestions. Further research should also include medical institutions from beyond the borders of Poland, enabling international comparative analyses.
Future research in the healthcare field has virtually endless possibilities. These regard the use of Big Data Analytics to diagnose specific conditions [ 47 , 66 , 69 , 76 ], propose an approach that can be used in other healthcare applications and create mechanisms to identify “patients like me” [ 75 , 80 ]. Big Data Analytics could also be used for studies related to the spread of pandemics, the efficacy of covid treatment [ 18 , 79 ], or psychology and psychiatry studies, e.g. emotion recognition [ 35 ].
Availability of data and materials
The datasets for this study are available on request to the corresponding author.
Abouelmehdi K, Beni-Hessane A, Khaloufi H. Big healthcare data: preserving security and privacy. J Big Data. 2018. https://doi.org/10.1186/s40537-017-0110-7 .
Article Google Scholar
Agrawal A, Choudhary A. Health services data: big data analytics for deriving predictive healthcare insights. Health Serv Eval. 2019. https://doi.org/10.1007/978-1-4899-7673-4_2-1 .
Al Mayahi S, Al-Badi A, Tarhini A. Exploring the potential benefits of big data analytics in providing smart healthcare. In: Miraz MH, Excell P, Ware A, Ali M, Soomro S, editors. Emerging technologies in computing—first international conference, iCETiC 2018, proceedings (Lecture Notes of the Institute for Computer Sciences, Social-Informatics and Telecommunications Engineering, LNICST). Cham: Springer; 2018. p. 247–58. https://doi.org/10.1007/978-3-319-95450-9_21 .
Bainbridge M. Big data challenges for clinical and precision medicine. In: Househ M, Kushniruk A, Borycki E, editors. Big data, big challenges: a healthcare perspective: background, issues, solutions and research directions. Cham: Springer; 2019. p. 17–31.
Google Scholar
Bartuś K, Batko K, Lorek P. Business intelligence systems: barriers during implementation. In: Jabłoński M, editor. Strategic performance management new concept and contemporary trends. New York: Nova Science Publishers; 2017. p. 299–327. ISBN: 978-1-53612-681-5.
Bartuś K, Batko K, Lorek P. Diagnoza wykorzystania big data w organizacjach-wybrane wyniki badań. Informatyka Ekonomiczna. 2017;3(45):9–20.
Bartuś K, Batko K, Lorek P. Wykorzystanie rozwiązań business intelligence, competitive intelligence i big data w przedsiębiorstwach województwa śląskiego. Przegląd Organizacji. 2018;2:33–9.
Batko K. Możliwości wykorzystania Big Data w ochronie zdrowia. Roczniki Kolegium Analiz Ekonomicznych. 2016;42:267–82.
Bi Z, Cochran D. Big data analytics with applications. J Manag Anal. 2014;1(4):249–65. https://doi.org/10.1080/23270012.2014.992985 .
Boerma T, Requejo J, Victora CG, Amouzou A, Asha G, Agyepong I, Borghi J. Countdown to 2030: tracking progress towards universal coverage for reproductive, maternal, newborn, and child health. Lancet. 2018;391(10129):1538–48.
Bollier D, Firestone CM. The promise and peril of big data. Washington, D.C: Aspen Institute, Communications and Society Program; 2010. p. 1–66.
Bose R. Competitive intelligence process and tools for intelligence analysis. Ind Manag Data Syst. 2008;108(4):510–28.
Carter P. Big data analytics: future architectures, skills and roadmaps for the CIO: in white paper, IDC sponsored by SAS. 2011. p. 1–16.
Castro EM, Van Regenmortel T, Vanhaecht K, Sermeus W, Van Hecke A. Patient empowerment, patient participation and patient-centeredness in hospital care: a concept analysis based on a literature review. Patient Educ Couns. 2016;99(12):1923–39.
Chen H, Chiang RH, Storey VC. Business intelligence and analytics: from big data to big impact. MIS Q. 2012;36(4):1165–88.
Chen CP, Zhang CY. Data-intensive applications, challenges, techniques and technologies: a survey on big data. Inf Sci. 2014;275:314–47.
Chomiak-Orsa I, Mrozek B. Główne perspektywy wykorzystania big data w mediach społecznościowych. Informatyka Ekonomiczna. 2017;3(45):44–54.
Corsi A, de Souza FF, Pagani RN, et al. Big data analytics as a tool for fighting pandemics: a systematic review of literature. J Ambient Intell Hum Comput. 2021;12:9163–80. https://doi.org/10.1007/s12652-020-02617-4 .
Davenport TH, Harris JG. Competing on analytics, the new science of winning. Boston: Harvard Business School Publishing Corporation; 2007.
Davenport TH. Big data at work: dispelling the myths, uncovering the opportunities. Boston: Harvard Business School Publishing; 2014.
De Cnudde S, Martens D. Loyal to your city? A data mining analysis of a public service loyalty program. Decis Support Syst. 2015;73:74–84.
Erickson S, Rothberg H. Data, information, and intelligence. In: Rodriguez E, editor. The analytics process. Boca Raton: Auerbach Publications; 2017. p. 111–26.
Fang H, Zhang Z, Wang CJ, Daneshmand M, Wang C, Wang H. A survey of big data research. IEEE Netw. 2015;29(5):6–9.
Fredriksson C. Organizational knowledge creation with big data. A case study of the concept and practical use of big data in a local government context. 2016. https://www.abo.fi/fakultet/media/22103/fredriksson.pdf .
Gandomi A, Haider M. Beyond the hype: big data concepts, methods, and analytics. Int J Inf Manag. 2015;35(2):137–44.
Groves P, Kayyali B, Knott D, Van Kuiken S. The ‘big data’ revolution in healthcare. Accelerating value and innovation. 2015. http://www.pharmatalents.es/assets/files/Big_Data_Revolution.pdf (Reading: 10.04.2019).
Gupta V, Rathmore N. Deriving business intelligence from unstructured data. Int J Inf Comput Technol. 2013;3(9):971–6.
Gupta V, Singh VK, Ghose U, Mukhija P. A quantitative and text-based characterization of big data research. J Intell Fuzzy Syst. 2019;36:4659–75.
Hampel HOBS, O’Bryant SE, Castrillo JI, Ritchie C, Rojkova K, Broich K, Escott-Price V. PRECISION MEDICINE-the golden gate for detection, treatment and prevention of Alzheimer’s disease. J Prev Alzheimer’s Dis. 2016;3(4):243.
Harerimana GB, Jang J, Kim W, Park HK. Health big data analytics: a technology survey. IEEE Access. 2018;6:65661–78. https://doi.org/10.1109/ACCESS.2018.2878254 .
Hu H, Wen Y, Chua TS, Li X. Toward scalable systems for big data analytics: a technology tutorial. IEEE Access. 2014;2:652–87.
Hussain S, Hussain M, Afzal M, Hussain J, Bang J, Seung H, Lee S. Semantic preservation of standardized healthcare documents in big data. Int J Med Inform. 2019;129:133–45. https://doi.org/10.1016/j.ijmedinf.2019.05.024 .
Islam MS, Hasan MM, Wang X, Germack H. A systematic review on healthcare analytics: application and theoretical perspective of data mining. In: Healthcare. Basel: Multidisciplinary Digital Publishing Institute; 2018. p. 54.
Ismail A, Shehab A, El-Henawy IM. Healthcare analysis in smart big data analytics: reviews, challenges and recommendations. In: Security in smart cities: models, applications, and challenges. Cham: Springer; 2019. p. 27–45.
Jain N, Gupta V, Shubham S, et al. Understanding cartoon emotion using integrated deep neural network on large dataset. Neural Comput Appl. 2021. https://doi.org/10.1007/s00521-021-06003-9 .
Janssen M, van der Voort H, Wahyudi A. Factors influencing big data decision-making quality. J Bus Res. 2017;70:338–45.
Jordan SR. Beneficence and the expert bureaucracy. Public Integr. 2014;16(4):375–94. https://doi.org/10.2753/PIN1099-9922160404 .
Knapp MM. Big data. J Electron Resourc Med Libr. 2013;10(4):215–22.
Koti MS, Alamma BH. Predictive analytics techniques using big data for healthcare databases. In: Smart intelligent computing and applications. New York: Springer; 2019. p. 679–86.
Krumholz HM. Big data and new knowledge in medicine: the thinking, training, and tools needed for a learning health system. Health Aff. 2014;33(7):1163–70.
Kruse CS, Goswamy R, Raval YJ, Marawi S. Challenges and opportunities of big data in healthcare: a systematic review. JMIR Med Inform. 2016;4(4):e38.
Kyoungyoung J, Gang HK. Potentiality of big data in the medical sector: focus on how to reshape the healthcare system. Healthc Inform Res. 2013;19(2):79–85.
Laney D. Application delivery strategies 2011. http://blogs.gartner.com/doug-laney/files/2012/01/ad949-3D-Data-Management-Controlling-Data-Volume-Velocity-and-Variety.pdf .
Lee IK, Wang CC, Lin MC, Kung CT, Lan KC, Lee CT. Effective strategies to prevent coronavirus disease-2019 (COVID-19) outbreak in hospital. J Hosp Infect. 2020;105(1):102.
Lerner I, Veil R, Nguyen DP, Luu VP, Jantzen R. Revolution in health care: how will data science impact doctor-patient relationships? Front Public Health. 2018;6:99.
Lytras MD, Papadopoulou P, editors. Applying big data analytics in bioinformatics and medicine. IGI Global: Hershey; 2017.
Ma K, et al. Big data in multiple sclerosis: development of a web-based longitudinal study viewer in an imaging informatics-based eFolder system for complex data analysis and management. In: Proceedings volume 9418, medical imaging 2015: PACS and imaging informatics: next generation and innovations. 2015. p. 941809. https://doi.org/10.1117/12.2082650 .
Mach-Król M. Analiza i strategia big data w organizacjach. In: Studia i Materiały Polskiego Stowarzyszenia Zarządzania Wiedzą. 2015;74:43–55.
Madsen LB. Data-driven healthcare: how analytics and BI are transforming the industry. Hoboken: Wiley; 2014.
Manyika J, Chui M, Brown B, Bughin J, Dobbs R, Roxburgh C, Hung BA. Big data: the next frontier for innovation, competition, and productivity. Washington: McKinsey Global Institute; 2011.
Marconi K, Dobra M, Thompson C. The use of big data in healthcare. In: Liebowitz J, editor. Big data and business analytics. Boca Raton: CRC Press; 2012. p. 229–48.
Mehta N, Pandit A. Concurrence of big data analytics and healthcare: a systematic review. Int J Med Inform. 2018;114:57–65.
Michel M, Lupton D. Toward a manifesto for the ‘public understanding of big data.’ Public Underst Sci. 2016;25(1):104–16. https://doi.org/10.1177/0963662515609005 .
Mikalef P, Krogstie J. Big data analytics as an enabler of process innovation capabilities: a configurational approach. In: International conference on business process management. Cham: Springer; 2018. p. 426–41.
Mohammadi M, Al-Fuqaha A, Sorour S, Guizani M. Deep learning for IoT big data and streaming analytics: a survey. IEEE Commun Surv Tutor. 2018;20(4):2923–60.
Nambiar R, Bhardwaj R, Sethi A, Vargheese R. A look at challenges and opportunities of big data analytics in healthcare. In: 2013 IEEE international conference on big data; 2013. p. 17–22.
Ohlhorst F. Big data analytics: turning big data into big money, vol. 65. Hoboken: Wiley; 2012.
Olszak C, Mach-Król M. A conceptual framework for assessing an organization’s readiness to adopt big data. Sustainability. 2018;10(10):3734.
Olszak CM. Toward better understanding and use of business intelligence in organizations. Inf Syst Manag. 2016;33(2):105–23.
Palanisamy V, Thirunavukarasu R. Implications of big data analytics in developing healthcare frameworks—a review. J King Saud Univ Comput Inf Sci. 2017;31(4):415–25.
Provost F, Fawcett T. Data science and its relationship to big data and data-driven decisionmaking. Big Data. 2013;1(1):51–9.
Raghupathi W, Raghupathi V. An overview of health analytics. J Health Med Inform. 2013;4:132. https://doi.org/10.4172/2157-7420.1000132 .
Raghupathi W, Raghupathi V. Big data analytics in healthcare: promise and potential. Health Inf Sci Syst. 2014;2(1):3.
Ratia M, Myllärniemi J. Beyond IC 4.0: the future potential of BI-tool utilization in the private healthcare, conference: proceedings IFKAD, 2018 at: Delft, The Netherlands.
Ristevski B, Chen M. Big data analytics in medicine and healthcare. J Integr Bioinform. 2018. https://doi.org/10.1515/jib-2017-0030 .
Rumsfeld JS, Joynt KE, Maddox TM. Big data analytics to improve cardiovascular care: promise and challenges. Nat Rev Cardiol. 2016;13(6):350–9. https://doi.org/10.1038/nrcardio.2016.42 .
Schmarzo B. Big data: understanding how data powers big business. Indianapolis: Wiley; 2013.
Senthilkumar SA, Rai BK, Meshram AA, Gunasekaran A, Chandrakumarmangalam S. Big data in healthcare management: a review of literature. Am J Theor Appl Bus. 2018;4:57–69.
Shubham S, Jain N, Gupta V, et al. Identify glomeruli in human kidney tissue images using a deep learning approach. Soft Comput. 2021. https://doi.org/10.1007/s00500-021-06143-z .
Thuemmler C. The case for health 4.0. In: Thuemmler C, Bai C, editors. Health 4.0: how virtualization and big data are revolutionizing healthcare. New York: Springer; 2017.
Tsai CW, Lai CF, Chao HC, et al. Big data analytics: a survey. J Big Data. 2015;2:21. https://doi.org/10.1186/s40537-015-0030-3 .
Wamba SF, Gunasekaran A, Akter S, Ji-fan RS, Dubey R, Childe SJ. Big data analytics and firm performance: effects of dynamic capabilities. J Bus Res. 2017;70:356–65.
Wang Y, Byrd TA. Business analytics-enabled decision-making effectiveness through knowledge absorptive capacity in health care. J Knowl Manag. 2017;21(3):517–39.
Wang Y, Kung L, Wang W, Yu C, Cegielski CG. An integrated big data analytics-enabled transformation model: application to healthcare. Inf Manag. 2018;55(1):64–79.
Wicks P, et al. Scaling PatientsLikeMe via a “generalized platform” for members with chronic illness: web-based survey study of benefits arising. J Med Internet Res. 2018;20(5):e175.
Willems SM, et al. The potential use of big data in oncology. Oral Oncol. 2019;98:8–12. https://doi.org/10.1016/j.oraloncology.2019.09.003 .
Williams N, Ferdinand NP, Croft R. Project management maturity in the age of big data. Int J Manag Proj Bus. 2014;7(2):311–7.
Winters-Miner LA. Seven ways predictive analytics can improve healthcare. Medical predictive analytics have the potential to revolutionize healthcare around the world. 2014. https://www.elsevier.com/connect/seven-ways-predictive-analytics-can-improve-healthcare (Reading: 15.04.2019).
Wu J, et al. Application of big data technology for COVID-19 prevention and control in China: lessons and recommendations. J Med Internet Res. 2020;22(10): e21980.
Yan L, Peng J, Tan Y. Network dynamics: how can we find patients like us? Inf Syst Res. 2015;26(3):496–512.
Yang JJ, Li J, Mulder J, Wang Y, Chen S, Wu H, Pan H. Emerging information technologies for enhanced healthcare. Comput Ind. 2015;69:3–11.
Zhang Q, Yang LT, Chen Z, Li P. A survey on deep learning for big data. Inf Fusion. 2018;42:146–57.
Download references
Acknowledgements
We would like to thank those who have touched our science paths.
This research was fully funded as statutory activity—subsidy of Ministry of Science and Higher Education granted for Technical University of Czestochowa on maintaining research potential in 2018. Research Number: BS/PB–622/3020/2014/P. Publication fee for the paper was financed by the University of Economics in Katowice.
Author information
Authors and affiliations.
Department of Business Informatics, University of Economics in Katowice, Katowice, Poland
Kornelia Batko
Department of Biomedical Processes and Systems, Institute of Health and Nutrition Sciences, Częstochowa University of Technology, Częstochowa, Poland
Andrzej Ślęzak
You can also search for this author in PubMed Google Scholar
Contributions
KB proposed the concept of research and its design. The manuscript was prepared by KB with the consultation of AŚ. AŚ reviewed the manuscript for getting its fine shape. KB prepared the manuscript in the contexts such as definition of intellectual content, literature search, data acquisition, data analysis, and so on. AŚ obtained research funding. Both authors read and approved the final manuscript.
Corresponding author
Correspondence to Kornelia Batko .
Ethics declarations
Ethics approval and consent to participate.
Not applicable.
Consent for publication
Competing interests.
The author declares no conflict of interest.
Additional information
Publisher's note.
Springer Nature remains neutral with regard to jurisdictional claims in published maps and institutional affiliations.
Rights and permissions
Open Access This article is licensed under a Creative Commons Attribution 4.0 International License, which permits use, sharing, adaptation, distribution and reproduction in any medium or format, as long as you give appropriate credit to the original author(s) and the source, provide a link to the Creative Commons licence, and indicate if changes were made. The images or other third party material in this article are included in the article's Creative Commons licence, unless indicated otherwise in a credit line to the material. If material is not included in the article's Creative Commons licence and your intended use is not permitted by statutory regulation or exceeds the permitted use, you will need to obtain permission directly from the copyright holder. To view a copy of this licence, visit http://creativecommons.org/licenses/by/4.0/ .
Reprints and permissions
About this article
Cite this article.
Batko, K., Ślęzak, A. The use of Big Data Analytics in healthcare. J Big Data 9 , 3 (2022). https://doi.org/10.1186/s40537-021-00553-4
Download citation
Received : 28 August 2021
Accepted : 19 December 2021
Published : 06 January 2022
DOI : https://doi.org/10.1186/s40537-021-00553-4
Share this article
Anyone you share the following link with will be able to read this content:
Sorry, a shareable link is not currently available for this article.
Provided by the Springer Nature SharedIt content-sharing initiative
- Big Data Analytics
- Data-driven healthcare
Data science methods in healthcare: A research perspective [ICEST 2021 Invited speaker ]
Ieee account.
- Change Username/Password
- Update Address
Purchase Details
- Payment Options
- Order History
- View Purchased Documents
Profile Information
- Communications Preferences
- Profession and Education
- Technical Interests
- US & Canada: +1 800 678 4333
- Worldwide: +1 732 981 0060
- Contact & Support
- About IEEE Xplore
- Accessibility
- Terms of Use
- Nondiscrimination Policy
- Privacy & Opting Out of Cookies
A not-for-profit organization, IEEE is the world's largest technical professional organization dedicated to advancing technology for the benefit of humanity. © Copyright 2024 IEEE - All rights reserved. Use of this web site signifies your agreement to the terms and conditions.
22 Big Data in Healthcare Examples and Applications
Companies are turning to big data to reimagine every aspect of healthcare.

Big data is being utilized more and more in every industry , but the role it’s playing in healthcare may end up having the greatest impact on our lives.
Researchers, hospitals and physicians are turning to a vast network of healthcare data to understand clinical context, prevent future health issues and even find new treatment options. While there are many ways data is being used to impact healthcare, we’ve rounded up five areas — along with a few examples of companies and organizations working within each area — where big data is taking on some of the major challenges in healthcare.
Big Data in Healthcare Applications and Examples
Big data in healthcare applications.
- Cancer Research
- Disease Detection
- Population Health
- Pharmaceutical Research and Development
- Health Insurance Risk Assessment
Big Data and Cancer Research
Nearly all of us have been impacted by cancer in some way, and the search for new ways to combat the disease is in high gear. Researchers in both the private and public sector are dedicated to everything from researching a cure to finding more effective treatment options. Big data has changed the way these researchers understand the disease, providing access to patient information, trends and patterns never accessible before. The following are just a few of the companies using big data to make headway in the fight against cancer.
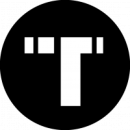
Location: Chicago, Illinois
Tempus is building the largest library of molecular and clinical data in the world with the goal of providing medical professionals with more clinical context for each patient’s cancer case. The Tempus platform collects and organizes data from lab reports, clinical notes, radiology scans and pathology images, accelerating oncology research and helping physicians make more personalized and informed treatment plans.
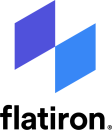
Flatiron Health
Location: New York, New York
Flatiron Health utilizes billions of data points from cancer patients to enhance research and gain new insights for patient care. Their solutions connect all players in the treatment of cancer, from oncologists and hospitals to academics and life science researchers, enabling them to learn from each patient.
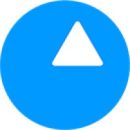
Oncora Medical
Location: Philadelphia, Pennsylvania
Oncora Medical is simplifying workflows for oncologists by blending machine learning, automation and big data into a single platform. With the company’s data analysis tools, oncologists can compile data and quickly add information to a patient’s health records. As a result, oncologists can review a patient’s radiology and pathology history faster, delivering more timely and personalized care to cancer patients.
Related Reading Big Data in Education: 9 Companies Delivering Insights to the Classroom
Big Data and Early Disease Detection
Early detection for diseases and complications is crucial for successful treatment. Whether it’s cancer, multiple sclerosis or a number of other conditions, screenings and other exams are often vital in staying ahead of disease. Here are a few examples of companies leveraging big data to improve early detection of disease and complications in patients.
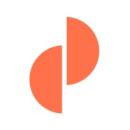
Location: Irving, Texas
Pieces is a cloud-based software company that collects data throughout the entire patient journey to improve both the quality and cost of care. The company’s flagship product, Pieces Decision Sciences, is a clinical engine that makes decisions and recommendations based on a variety of data such as lab results, vitals, and structured and unstructured data. The platform consistently works to identify possible interventions while also learning from clinical outcomes.
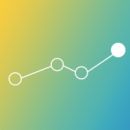
PeraHealth: The Rothman Index
Location: Charlotte, North Carolina
PeraHealth is the creator of the Rothman Index, a peer-reviewed, universal scoring system for the overall health of a patient. The score takes the data within electronic health records, vitals, lab results and nursing assessments to assign a score. The scores are provided in a visual graph and updated in real time to identify changes and keep track of the details, helping patients avoid complications.
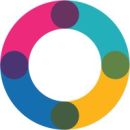
Prognos applies artificial intelligence to clinical data and manages Prognos Factor — a hub for multi-sourced diagnostic data. Their AI platform helps physicians apply treatments earlier, displays clinical trial opportunities, suggests therapy options and exposes care gaps for more than 30 conditions.
Big Data and Population Health
Different from public health, which focuses on how society can ensure healthier people, population health studies the patterns and conditions that affect the overall health of groups . Big data is an essential part of understanding population health because without data, patterns are difficult to pinpoint. The following are just a few examples of companies that are aggregating and organizing data to help healthcare organizations and researchers identify the patterns that can improve health conditions.
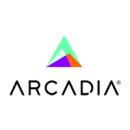
Location: Fully Remote
Arcadia ’s big data platform provides organizations throughout the healthcare landscape with actionable insights that enable them to “make more strategic decisions in support of their financial, clinical, and operational objectives.” In the area of population health management, for example, Arcadia’s analytics capabilities make it possible to identify and overcome care gaps.
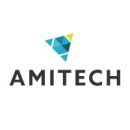
Amitech Solutions
Location: Creve Coeur, Missouri
Amitech Solutions applies data to the health field in multiple ways, from modern data management to healthcare analytics. Specifically, Amitech utilizes data for population health management solutions, combining physical and behavioral health data to identify risks and engage patients in their own healthcare.
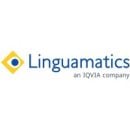
Linguamatics
Location: Marlborough, Massachusetts
Linguamatics mines the untapped, unstructured data in electronic health records for research and solutions in population health. By using natural language processing , Linguamatics can use unstructured patient data to identify lifestyle factors, build predictive models and detect high-risk patients.
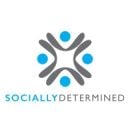
Socially Determined
Location: Washington, D.C.
Socially Determined takes a more holistic approach to population health by supplying healthcare organizations with social risk intelligence. The company’s platform SocialScape measures factors such as patients’ access to housing, transportation and food. Healthcare groups can then craft their strategies around these variables to deliver tailored care to specific populations.
Big Data and Pharmaceutical Research
Whether it be vaccines, synthetic insulin or simple antihistamines, medicines produced by the pharmaceutical industry play an important role in the treatment of disease. New drug discovery and creation depends on data to assess the viability and effectiveness of treatments. The following companies are using big data to help enhance pharmaceutical companies with research and development .
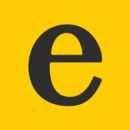
Location: San Mateo, California
Evidation has a mobile app that rewards users for healthy behaviors, provides access to health insights and offers opportunities to contribute to health research. Through Evidation, researchers can access everyday health data that informs their work and enables them to discover new ways of diagnosing, treating and managing various medical conditions. The company prioritizes giving app users control over their data, asking them for consent before their data can be accessed.
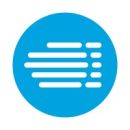
Location: Durham, North Carolina
IQVIA builds links between analytics, data and technology, so pharmacy leaders can complete faster and more effective clinical research. Besides boasting a dense healthcare database , the company leverages AI and machine learning to pinpoint the ideal patients for specific trials. Pharmacists can then run decentralized trials, compile data with IQVIA’s devices and jumpstart the research and development process.
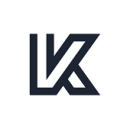
Kalderos is challenging the cost of pharmaceuticals through its drug discount management platform, which collects data from multiple sources and stakeholders to improve transparency among patients. Drug manufacturers, covered entities and payers can use the platform to collaborate too. The company hopes to promote trust and equity within the healthcare industry.
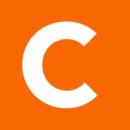
Location: Santa Clara, California
Although now part of the Cloudera family due to a merger , the Hortonworks data platform continues to help pharmaceutical companies and researchers gain a better view of pharmaceutical data. Because billions of records are integrated and made accessible, companies can answer questions that weren’t possible before. This sparks more effective research for clinical trials, improved safety, faster time to market and better health outcomes .
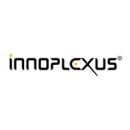
Location: Iselin, New Jersey (U.S. office)
Innoplexus is the creator of the iPlexus discovery tool that organizes millions of publications, articles, dissertations, thousands of clinical trials, drug profiles and congress articles into a concept-based research platform. The tool helps pharmaceutical companies find the relevant information needed for research and new drug discovery.
Related Reading 9 Examples of Big Data in Media and Entertainment
Big Data and Health Records
When it comes to healthcare and specifically health insurance , risk is often a large contributing factor in how patients access care. The following are a few examples of companies using big data to gain more insight into risk and ensure accuracy in adjustments.
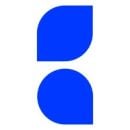
Blubyrd is designed to help surgical facilities and clinical practices compile and exchange data efficiently and securely. This data includes appointment schedules, procedure codes and equipment inventory.
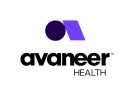
Avaneer Health
Avaneer Health works to improve the efficiency of data flow in the healthcare industry by giving network participants access to administrative help and secured transactions. Founded in 2020 by a collective of top healthcare industry leaders — including CVS, Anthem, Cleveland Clinic and more — the company’s platform relies on blockchain.
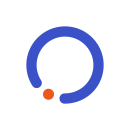
Particle Health
Particle Health makes an API platform that brings together patient records into a single secure place. With a simple query, developers can access clean and actionable data sets to use. The goal is for healthcare providers to use the data to make more meaningful recommendations to their patients.
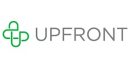
Upfront Healthcare
Upfront Healthcare ’s software platform uses data-driven personalization to improve communications between healthcare professionals and patients. For example, Upfront collects data — such as patient-reported outcomes, behavioral patterns, psychographic segments — and uses it to deliver relevant and timely messages to patients, whether it’s a reminder or a call to action.
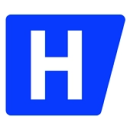
Human API streamlines the underwriting process by allowing teams to sift through detailed electronic health records. A health intelligence platform reviews patients’ health backgrounds with automated features and pinpoints any underlying conditions. This workflow reduces the time it takes to complete each application, leading to higher placement rates, larger volumes of applicants and improved customer experiences.
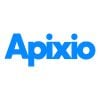
Apixio ’s data acquisition technology wrangles medical data from millions of files, claims, PDFs and other health records. With this information, Apixio’s coding application provides more accurate risk adjustment for healthcare providers.
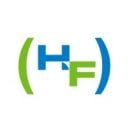
Health Fidelity
Health Fidelity helps healthcare providers and institutions find risks normally concealed in clinical charts. Their technology uses natural language processing to extract 100 percent of data within clinical charts and identify problems in care, assessment and documentation, which provides improved visibility for risk adjustment.
Rose Velazquez contributed reporting to this story.
Recent Healthcare Technology Articles
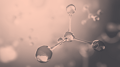
Data science methodologies in smart healthcare: a review
- Review Paper
- Published: 24 February 2022
- Volume 12 , pages 329–344, ( 2022 )
Cite this article
- Prasanta Kumar Parida ORCID: orcid.org/0000-0002-8676-0144 1 ,
- Lingraj Dora 1 ,
- Monorama Swain 2 ,
- Sanjay Agrawal 3 &
- Rutuparna Panda 3
629 Accesses
3 Citations
Explore all metrics
Data Science methodologies empower the representation and quantitative investigation in the field of smart healthcare. It is of extraordinary noteworthiness for early detection/classification of diseases for treatment planning. Nonetheless, there is a strong need to provide an in-depth survey of the existing methodologies highlighting their pros and cons to make them attractive for the researchers. The present study is an effort in this direction. The contribution made in this paper provides a new research path to the data science methodologies in smart healthcare, as opposed to the existing review articles. Recently, medical imaging investigation used deep learning (DL), as it defeats the limitations of the visual evaluation and the conventional machine learning methods for smart healthcare services. Research and methodologies are yet to be explored for the development of e-health. In this review paper, the uses of data science techniques in the clinical practice are highlighted. Also, it focuses on the state-of-the-art methods in the DL-based detection/classification of diseases. We emphasize the trends and challenges in smart healthcare as well as compare some baseline methods. This paper may be beneficial for the researchers to explore many more ideas covering all aspects of data science methodologies and applications for smart healthcare.
This is a preview of subscription content, log in via an institution to check access.
Access this article
Price includes VAT (Russian Federation)
Instant access to the full article PDF.
Rent this article via DeepDyve
Institutional subscriptions
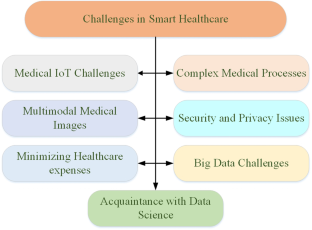
Similar content being viewed by others
Deep Learning for Health Care in Disease Identification: A Review
Deep Learning in Healthcare Informatics
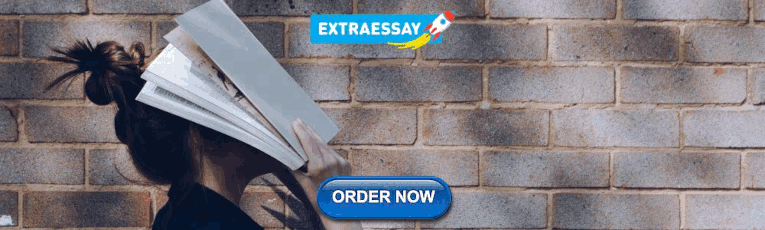
Deep Learning in Healthcare
Al-Dulaimi J, Cosmas J. Smart safety & health care in cities. Procedia Comput Sci. 2016;98:259–66.
Article Google Scholar
Mallow JA, Theeke LA, Theeke E, Mallow BK. The effectiveness of mI SMART: A nurse practitioner led technology intervention for multiple chronic conditions in primary care. Int J Nurs Sci. 2018;5(2):131–7.
Google Scholar
Sicari S, Rizzardi A, Grieco L, Piro G, Coen-Porisini A. A policy enforcement framework for internet of things applications in the smart health. Smart Health. 2017;3:39–74.
Lytras MD, Chui KT, Visvizi A. Data analytics in smart healthcare: The recent developments and beyond. 2019.
Syed L, Jabeen S, Manimala S, Elsayed HA. Data science algorithms and techniques for smart healthcare using IoT and big data analytics. In: Smart Techniques for a Smarter Planet. Springer; 2019. p. 211–241.
Tian S, Yang W, Le Grange JM, Wang P, Huang W, Ye Z. Smart healthcare: making medical care more intelligent. Global Health Journal. 2019.
Chui KT, Alhalabi W, Pang SSH, Pablos POD, Liu RW, Zhao M. Disease diagnosis in smart healthcare: Innovation, technologies and applications. Sustainability. 2017;9(12):2309.
Rayan Z, Alfonse M, Salem ABM. Machine learning approaches in smart health. Procedia Comput Sci. 2019;154:361–8.
Sundaravadivel P, Kougianos E, Mohanty SP, Ganapathiraju MK. Everything you wanted to know about smart health care: Evaluating the different technologies and components of the internet of things for better health. IEEE Consum Electron Mag. 2017;7(1):18–28.
Afrati FN, Ullman JD. Optimizing joins in a map-reduce environment. In: Proceedings of the 13th International Conference on Extending Database Technology. 2010. p. 99–110.
Chen LM. Overview of basic methods for data science. In: Mathematical problems in data science. Springer; 2015. p. 17–37.
Aborokbah MM, Al-Mutairi S, Sangaiah AK, Samuel OW. Adaptive context aware decision computing paradigm for intensive health care delivery in smart cities-a case analysis. Sustain Cities Soc. 2018;41:919–24.
Medhane DV, Sangaiah AK. Search space-based multi-objective optimization evolutionary algorithm. Comput Electr Eng. 2017;58:126–43.
Sangaiah AK, Thangavelu AK. An adaptive neuro-fuzzy approach to evaluation of team-level service climate in GSD projects. Neural Comput Applic. 2014;25(3–4):573–83.
Sangaiah AK, Thangavelu AK, Gao XZ, Anbazhagan N, Durai MS. An ANFIS approach for evaluation of team-level service climate in GSD projects using Taguchi-genetic learning algorithm. Appl Soft Comput. 2015;30:628–35.
Sampri A, Mavragani A, Tsagarakis KP. Evaluating google trends as a tool for integrating the “smart health” concept in the smart cities’ governance in USA. Procedia Eng. 2016;162:585–92.
Manikandan R, Patan R, Gandomi AH, Sivanesan P, Kalyanaraman H. Hash polynomial two factor decision tree using IoT for smart health care scheduling. Expert Syst Appl. 2020;141:112924.
Tokognon CA, Gao B, Tian GY, Yan Y. Structural health monitoring framework based on internet of things: a survey. IEEE Internet Things J. 2017;4(3):619–35.
Pal D, Funilkul S, Charoenkitkarn N, Kanthamanon P. Internet-of-things and smart homes for elderly healthcare: an end user perspective. IEEE Access. 2018;6:10483–96.
Enshaeifar S, Zoha A, Markides A, Skillman S, Acton ST, Elsaleh T, Hassanpour M, Ahrabian A, Kenny M, Klein S, et al. Health management and pattern analysis of daily living activities of people with dementia using in-home sensors and machine learning techniques. PloS One. 2018;13(5).
Rodrigues JJ, Segundo DBDR, Junqueira HA, Sabino MH, Prince RM, Al-Muhtadi J, De Albuquerque VHC. Enabling technologies for the internet of health things. IEEE Access. 2018;6:13129–41.
Übeyli ED. Implementing automated diagnostic systems for breast cancer detection. Expert Syst Appl. 2007;33(4):1054–62.
Polat K, Güneş S. Breast cancer diagnosis using least square support vector machine. Digit Signal Process. 2007;17(4):694–701.
Setiono R. Generating concise and accurate classification rules for breast cancer diagnosis. Artif Intell Med. 2000;18(3):205–19.
Sheikhpour R, Sarram MA, Sheikhpour R. Particle swarm optimization for bandwidth determination and feature selection of kernel density estimation based classifiers in diagnosis of breast cancer. Appl Soft Comput. 2016;40:113–31.
Article MATH Google Scholar
Dheeba J, Singh NA, Selvi ST. Computer-aided detection of breast cancer on mammograms: a swarm intelligence optimized wavelet neural network approach. J Biomed Inform. 2014;49:45–52.
Mert A, Kılıç N, Bilgili E, Akan A. Breast cancer detection with reduced feature set. Comput Math Methods Med. 2015;2015.
Nahato KB, Harichandran KN, Arputharaj K. Knowledge mining from clinical datasets using rough sets and backpropagation neural network. Comput Math Methods Med. 2015;2015.
Badnjevic A, Gurbeta L, Custovic E. An expert diagnostic system to automatically identify asthma and chronic obstructive pulmonary disease in clinical settings. Sci Rep. 2018;8(1):1–9.
Badnjević A, Pokvić LG, Hasičić M, Bandić L, Mašetić Z, Kovačević Ž, Kevrić J, Pecchia L. Evidence-based clinical engineering: machine learning algorithms for prediction of defibrillator performance. Biomed Signal Process Control. 2019;54:101629.
Catic A, Gurbeta L, Kurtovic-Kozaric A, Mehmedbasic S, Badnjevic A. Application of neural networks for classification of Patau, Edwards, Down, Turner and Klinefelter syndrome based on first trimester maternal serum screening data, ultrasonographic findings and patient demographics. BMC Med Genet. 2018;11(1):1–12.
Kovačević Ž, Pokvić LG, Spahić L, Badnjević A. Prediction of medical device performance using machine learning techniques: infant incubator case study. Heal Technol. 2020;10(1):151–5.
Stokes K, Castaldo R, Franzese M, Salvatore M, Fico G, Pokvic LG, Badnjevic A, Pecchia L. A machine learning model for supporting symptom-based referral and diagnosis of bronchitis and pneumonia in limited resource settings. Biocybern Biomed Eng. 2021;41(4):1288–302.
Litjens G, Kooi T, Bejnordi BE, Setio AAA, Ciompi F, Ghafoorian M, Van Der Laak JA, Van Ginneken B, Sánchez CI. A survey on deep learning in medical image analysis. Med Image Anal. 2017;42:60–88.
Winkels M, Cohen TS. Pulmonary nodule detection in CT scans with equivariant CNNS. Med Image Anal. 2019;55:15–26.
Simard PY, Steinkraus D, Platt JC, et al. Best practices for convolutional neural networks applied to visual document analysis. In: ICDAR, vol 3. 2003.
Kumar A, Singh SK, Saxena S, Lakshmanan K, Sangaiah AK, Chauhan H, Shrivastava S, Singh RK. Deep feature learning for histopathological image classification of canine mammary tumors and human breast cancer. Inf Sci. 2020;508:405–21.
Khan S, Islam N, Jan Z, Din IU, Rodrigues JJC. A novel deep learning based framework for the detection and classification of breast cancer using transfer learning. Pattern Recogn Lett. 2019;125:1–6.
Wang P, Song Q, Li Y, Lv S, Wang J, Li L, Zhang H. Cross-task extreme learning machine for breast cancer image classification with deep convolutional features. Biomed Signal Process Control. 2020a;57:101789.
Beevi KS, Nair MS, Bindu G. Automatic mitosis detection in breast histopathology images using convolutional neural network based deep transfer learning. Bioprocess Biosyst Eng. 2019;39(1):214–23.
Li H, Zhuang S, Da Li, Zhao J, Ma Y. Benign and malignant classification of mammogram images based on deep learning. Biomed Signal Process Control. 2019;51:347–54.
Abdel-Zaher AM, Eldeib AM. Breast cancer classification using deep belief networks. Expert Systems with Applications. 2016;46:139–44.
Havaei M, Davy A, Warde-Farley D, Biard A, Courville A, Bengio Y, Pal C, Jodoin PM, Larochelle H. Brain tumor segmentation with deep neural networks. Med Image Anal. 2017;35:18–31.
Valverde S, Cabezas M, Roura E, González-Villà S, Pareto D, Vilanova JC, Ramió-Torrentà L, Rovira À, Oliver A, Lladó X. Improving automated multiple sclerosis lesion segmentation with a cascaded 3D convolutional neural network approach. NeuroImage. 2017;155:159–68.
Wachinger C, Reuter M, Klein T. Deepnat: Deep convolutional neural network for segmenting neuroanatomy. NeuroImage. 2018;170:434–45.
Trebeschi S, van Griethuysen JJ, Lambregts DM, Lahaye MJ, Parmar C, Bakers FC, Peters NH, Beets-Tan RG, Aerts HJ. Author correction: deep learning for fully-automated localization and segmentation of rectal cancer on multiparametric MR. Sci Rep. 2018;8(1):1.
Christ PF, Ettlinger F, Grün F, Elshaera MEA, Lipkova J, Schlecht S, Ahmaddy F, Tatavarty S, Bickel M, Bilic P, et al. Automatic liver and tumor segmentation of CT and MRI volumes using cascaded fully convolutional neural networks. arXiv preprint arXiv:170205970 . 2017.
Ronneberger O, Fischer P, Brox T. U-net: Convolutional networks for biomedical image segmentation. In: International Conference on Medical Image Computing and Computer-Assisted Intervention, Springer; 2015. p. 234–241.
Chen L, Wu Y, DSouza AM, Abidin AZ, Wismüller A, Xu C. MRI tumor segmentation with densely connected 3D CNN. In: Medical imaging 2018: image processing, international society for optics and photonics, vol. 10574. 2018. p. 105741F.
Jégou S, Drozdzal M, Vazquez D, Romero A, Bengio Y. The one hundred layers tiramisu: Fully convolutional densenets for semantic segmentation. In: Proceedings of the IEEE Conference on Computer Vision and Pattern Recognition Workshops. 2017. p. 11–19.
Li Z, Wang Y, Yu J, Guo Y, Cao W. Deep learning based radiomics (DLR) and its usage in noninvasive IDH1 prediction for low grade glioma. Sci Rep. 2017;7(1):1–11.
Clark T, Wong A, Haider MA, Khalvati F. Fully deep convolutional neural networks for segmentation of the prostate gland in diffusion-weighted MR images. In: International Conference Image Analysis and Recognition. Springer; 2017. p. 97–104.
McKinley R, Wepfer R, Gundersen T, Wagner F, Chan A, Wiest R, Reyes M. Nabla-net: A deep dag-like convolutional architecture for biomedical image segmentation. In: International Workshop on Brainlesion: Glioma, Multiple Sclerosis, Stroke and Traumatic Brain Injuries. Springer; 2016. p. 119–128.
Zhang Q, Cui Z, Niu X, Geng S, Qiao Y. Image segmentation with pyramid dilated convolution based on ResNet and U-Net. In: International Conference on Neural Information Processing. Springer; 2017. p. 364–372.
Deniz CM, Xiang S, Hallyburton RS, Welbeck A, Babb JS, Honig S, Cho K, Chang G. Segmentation of the proximal femur from MR images using deep convolutional neural networks. Sci Rep. 2018;8(1):1–14.
Milletari F, Ahmadi SA, Kroll C, Plate A, Rozanski V, Maiostre J, Levin J, Dietrich O, Ertl-Wagner B, Bötzel K, et al. Hough-CNN: deep learning for segmentation of deep brain regions in MRI and ultrasound. Comput Vis Image Underst. 2017;164:92–102.
Yang X, Yu L, Wu L, Wang Y, Ni D, Qin J, Heng PA. Fine-grained recurrent neural networks for automatic prostate segmentation in ultrasound images. In: Thirty-First AAAI Conference on Artificial Intelligence. 2017b.
Poudel RP, Lamata P, Montana G. Recurrent fully convolutional neural networks for multi-slice MRI cardiac segmentation. In: Reconstruction, segmentation, and analysis of medical images. Springer; 2016. p. 83–94.
Mazurowski MA, Buda M, Saha A, Bashir MR. Deep learning in radiology: an overview of the concepts and a survey of the state of the art with focus on MRI. J Magn Reson Imaging. 2019;49(4):939–54.
Antropova N, Huynh BQ, Giger ML. A deep feature fusion methodology for breast cancer diagnosis demonstrated on three imaging modality datasets. Med Phys. 2017;44(10):5162–71.
Paul R, Hawkins SH, Balagurunathan Y, Schabath MB, Gillies RJ, Hall LO, Goldgof DB. Deep feature transfer learning in combination with traditional features predicts survival among patients with lung adenocarcinoma. Tomography. 2016;2(4):388.
Chi J, Walia E, Babyn P, Wang J, Groot G, Eramian M. Thyroid nodule classification in ultrasound images by fine-tuning deep convolutional neural network. J Digit Imaging. 2017;30(4):477–86.
Kumar A, Kim J, Lyndon D, Fulham M, Feng D. An ensemble of fine-tuned convolutional neural networks for medical image classification. IEEE J Biomed Health Inform. 2016;21(1):31–40.
Zhu Z, Harowicz M, Zhang J, Saha A, Grimm LJ, Hwang ES, Mazurowski MA. Deep learning analysis of breast MRIS for prediction of occult invasive disease in ductal carcinoma in situ. Comput Biol Med. 2019;115:103498.
Krizhevsky A, Sutskever I, Hinton GE. Imagenet classification with deep convolutional neural networks. In: Advances in neural information processing systems. 2012. p. 1097–1105.
Simonyan K, Zisserman A. Very deep convolutional networks for large-scale image recognition. arXiv preprint arXiv:14091556 . 2014.
Suk HI, Lee SW, Shen D, Initiative ADN, et al. Deep ensemble learning of sparse regression models for brain disease diagnosis. Med Image Anal. 2017;37:101–13.
Khawaldeh S, Pervaiz U, Rafiq A, Alkhawaldeh RS. Noninvasive grading of glioma tumor using magnetic resonance imaging with convolutional neural networks. Appl Sci. 2018;8(1):27.
González G, Ash SY, Vegas-Sánchez-Ferrero G, Onieva Onieva J, Rahaghi FN, Ross JC, Díaz A, San José Estépar R, Washko GR. Disease staging and prognosis in smokers using deep learning in chest computed tomography. Am J Respir Crit Care Med. 2018;197(2):193–203.
He K, Zhang X, Ren S, Sun J. Deep residual learning for image recognition. In: Proceedings of the IEEE conference on computer vision and pattern recognition. 2016. p. 770–778.
Szegedy C, Vanhoucke V, Ioffe S, Shlens J, Wojna Z. Rethinking the inception architecture for computer vision. In: Proceedings of the IEEE Conference on Computer Vision and Pattern Recognition. 2016. p. 2818–2826.
Szegedy C, Ioffe S, Vanhoucke V, Alemi AA. Inception-v4, inception-resnet and the impact of residual connections on learning. In: Thirty-first AAAI conference on artificial intelligence. 2017.
Kim Y. Convolutional neural networks for sentence classification. arXiv preprint arXiv:14085882 . 2014.
Rajpurkar P, Irvin J, Zhu K, Yang B, Mehta H, Duan T, Ding D, Bagul A, Langlotz C, Shpanskaya K, et al. Chexnet: Radiologist-level pneumonia detection on chest x-rays with deep learning. arXiv preprint arXiv:171105225 . 2017.
Bengio Y, Lamblin P, Popovici D, Larochelle H. Greedy layer-wise training of deep networks. In: Advances in neural information processing systems. 2007. p. 153–160.
Hinton GE, Salakhutdinov RR. Reducing the dimensionality of data with neural networks. Science. 2006;313(5786):504–7.
Article MathSciNet MATH Google Scholar
Ortiz A, Munilla J, Martínez-Murcia FJ, Górriz JM, Ramírez J, Initiative ADN, et al. Learning longitudinal MRI patterns by SICE and deep learning: Assessing the Alzheimer’s disease progression. In: Annual Conference on Medical Image Understanding and Analysis. Springer; 2017. p. 413–424.
Kumar D, Wong A, Clausi DA. Lung nodule classification using deep features in CT images. In: 2015 12th Conference on Computer and Robot Vision. IEEE; 2015. p. 133–138.
Yoo Y, Tang LY, Brosch T, Li DK, Kolind S, Vavasour I, Rauscher A, MacKay AL, Traboulsee A, Tam RC. Deep learning of joint myelin and t1w MRI features in normal-appearing brain tissue to distinguish between multiple sclerosis patients and healthy controls. NeuroImage: Clinical. 2018;17:169–178.
Bi X, Li S, Xiao B, Li Y, Wang G, Ma X. Computer aided Alzheimer’s disease diagnosis by an unsupervised deep learning technology. Neurocomputing. 2019.
Ismael SAA, Mohammed A, Hefny H (2020) An enhanced deep learning approach for brain cancer MRI images classification using residual networks. Artif Intell Med. 102:101779.
Wang Z, Li M, Wang H, Jiang H, Yao Y, Zhang H, Xin J. Breast cancer detection using extreme learning machine based on feature fusion with CNN deep features. IEEE Access. 2019;7:105146–58.
Zhang D, Zou L, Zhou X, He F. Integrating feature selection and feature extraction methods with deep learning to predict clinical outcome of breast cancer. IEEE Access. 2018;6:28936–44.
Kwak JT, Hewitt SM. Nuclear architecture analysis of prostate cancer via convolutional neural networks. IEEE Access. 2017;5:18526–33.
Rakhlin A, Shvets A, Iglovikov V, Kalinin AA. Deep convolutional neural networks for breast cancer histology image analysis. In: International Conference Image Analysis and Recognition. Springer; 2018. p. 737–744.
Kaur P, Singh G, Kaur P. Intellectual detection and validation of automated mammogram breast cancer images by multi-class SVM using deep learning classification. Inform Med Unlocked. 2019;16:100151.
Wang Y, Choi EJ, Choi Y, Zhang H, Jin GY, Ko SB. Breast cancer classification in automated breast ultrasound using multiview convolutional neural network with transfer learning. Ultrasound Med Biol. 2020b;46(5):1119–1132
Shen L, He M, Shen N, Yousefi N, Wang C, Liu G. Optimal breast tumor diagnosis using discrete wavelet transform and deep belief network based on improved sunflower optimization method. Biomed Signal Process Control. 2020;60:101953.
Özyurt F, Sert E, Avcı D. An expert system for brain tumor detection: Fuzzy c-means with super resolution and convolutional neural network with extreme learning machine. Med Hypotheses. 2020;134:109433.
Sharif MI, Li JP, Khan MA, Saleem MA. Active deep neural network features selection for segmentation and recognition of brain tumors using MRI images. Pattern Recogn Lett. 2020;129:181–9.
Ghassemi N, Shoeibi A, Rouhani M. Deep neural network with generative adversarial networks pre-training for brain tumor classification based on MR images. Biomed Signal Process Control. 2020;57:101678.
Raja PS, et al. Brain tumor classification using a hybrid deep autoencoder with Bayesian fuzzy clustering-based segmentation approach. Biocybernetics and Biomedical Engineering. 2020;40(1):440–53.
Kumar S, Mankame DP. Optimization driven deep convolution neural network for brain tumor classification. Biocybern Biomed Eng. 2020;40(3):1190–204.
Hashemzehi R, Mahdavi SJS, Kheirabadi M, Kamel SR. Detection of brain tumors from MRI images base on deep learning using hybrid model CNN and NADE. Biocybern Biomed Eng. 2020;40(3):1225–32.
Maharjan S, Alsadoon A, Prasad P, Al-Dalain T, Alsadoon OH. A novel enhanced softmax loss function for brain tumour detection using deep learning. J Neurosci Methods. 2020;330:108520.
Çinar A, Yildirim M. Detection of tumors on brain MRI images using the hybrid convolutional neural network architecture. Med Hypotheses. 2020;139:109684.
Hussain S, Anwar SM, Majid M. Segmentation of glioma tumors in brain using deep convolutional neural network. Neurocomputing. 2018;282:248–61.
Yang X, Liu C, Wang Z, Yang J, Le Min H, Wang L, Cheng KTT. Co-trained convolutional neural networks for automated detection of prostate cancer in multi-parametric MRI. Med Image Anal. 2017a;42:212–227
Salvi M, Molinari F, Acharya UR, Molinaro L, Meiburger KM. Impact of stain normalization and patch selection on the performance of convolutional neural networks in histological breast and prostate cancer classification. Computer Methods and Programs in Biomedicine Update. 2021;1:100004.
Duran-Lopez L, Dominguez-Morales JP, Conde-Martin AF, Vicente-Diaz S, Linares-Barranco A. Prometeo: A CNN-based computer-aided diagnosis system for WSI prostate cancer detection. IEEE Access. 2020;8:128613–28.
Karimi D, Nir G, Fazli L, Black PC, Goldenberg L, Salcudean SE. Deep learning-based gleason grading of prostate cancer from histopathology images-role of multiscale decision aggregation and data augmentation. IEEE J Biomed Health Inform. 2019;24(5):1413–26.
Chen J, Wan Z, Zhang J, Li W, Chen Y, Li Y, Duan Y. Medical image segmentation and reconstruction of prostate tumor based on 3D AlexNet. Comput Methods Prog Biomed. 2021;200:105878.
Dhengre N, Sinha S, Chinni B, Dogra V, Rao N. Computer aided detection of prostate cancer using multiwavelength photoacoustic data with convolutional neural network. Biomed Signal Process Control. 2020;60:101952.
Deepak S, Ameer P. Brain tumor classification using deep CNN features via transfer learning. Comput Biol Med. 2019;111:103345.
Download references
The authors did not receive support from any organization for the submitted work. No funding was received to assist with the preparation of this manuscript. No funding was received for conducting this study. No funds, grants, or other support was received.
Author information
Authors and affiliations.
Department of Electrical and Electronics Engineering, Veer Surendra Sai University of Technology, Burla, India
Prasanta Kumar Parida & Lingraj Dora
Department of Electronics and Communication Engineering, Silicon Institute of Technology, Bhubaneswar, India
Monorama Swain
Department of Electronics and Telecommunication Engineering, Veer Surendra Sai University of Technology, Burla, India
Sanjay Agrawal & Rutuparna Panda
You can also search for this author in PubMed Google Scholar
Corresponding author
Correspondence to Rutuparna Panda .
Ethics declarations
Informed consent, research involving human and animal participants, conflicts of interest.
The authors declare that they have no conflict of interest.
Additional information
Publisher’s note.
Springer Nature remains neutral with regard to jurisdictional claims in published maps and institutional affiliations.
Rights and permissions
Reprints and permissions
About this article
Parida, P.K., Dora, L., Swain, M. et al. Data science methodologies in smart healthcare: a review. Health Technol. 12 , 329–344 (2022). https://doi.org/10.1007/s12553-022-00648-9
Download citation
Received : 10 September 2021
Accepted : 08 February 2022
Published : 24 February 2022
Issue Date : March 2022
DOI : https://doi.org/10.1007/s12553-022-00648-9
Share this article
Anyone you share the following link with will be able to read this content:
Sorry, a shareable link is not currently available for this article.
Provided by the Springer Nature SharedIt content-sharing initiative
- Data science technique
- Machine learning
- Deep learning
- Smart healthcare
- Find a journal
- Publish with us
- Track your research
- MyU : For Students, Faculty, and Staff
Fall 2024 CSCI Special Topics Courses
Cloud computing.
Meeting Time: 09:45 AM‑11:00 AM TTh Instructor: Ali Anwar Course Description: Cloud computing serves many large-scale applications ranging from search engines like Google to social networking websites like Facebook to online stores like Amazon. More recently, cloud computing has emerged as an essential technology to enable emerging fields such as Artificial Intelligence (AI), the Internet of Things (IoT), and Machine Learning. The exponential growth of data availability and demands for security and speed has made the cloud computing paradigm necessary for reliable, financially economical, and scalable computation. The dynamicity and flexibility of Cloud computing have opened up many new forms of deploying applications on infrastructure that cloud service providers offer, such as renting of computation resources and serverless computing. This course will cover the fundamentals of cloud services management and cloud software development, including but not limited to design patterns, application programming interfaces, and underlying middleware technologies. More specifically, we will cover the topics of cloud computing service models, data centers resource management, task scheduling, resource virtualization, SLAs, cloud security, software defined networks and storage, cloud storage, and programming models. We will also discuss data center design and management strategies, which enable the economic and technological benefits of cloud computing. Lastly, we will study cloud storage concepts like data distribution, durability, consistency, and redundancy. Registration Prerequisites: CS upper div, CompE upper div., EE upper div., EE grad, ITI upper div., Univ. honors student, or dept. permission; no cr for grads in CSci. Complete the following Google form to request a permission number from the instructor ( https://forms.gle/6BvbUwEkBK41tPJ17 ).
CSCI 5980/8980
Machine learning for healthcare: concepts and applications.
Meeting Time: 11:15 AM‑12:30 PM TTh Instructor: Yogatheesan Varatharajah Course Description: Machine Learning is transforming healthcare. This course will introduce students to a range of healthcare problems that can be tackled using machine learning, different health data modalities, relevant machine learning paradigms, and the unique challenges presented by healthcare applications. Applications we will cover include risk stratification, disease progression modeling, precision medicine, diagnosis, prognosis, subtype discovery, and improving clinical workflows. We will also cover research topics such as explainability, causality, trust, robustness, and fairness.
Registration Prerequisites: CSCI 5521 or equivalent. Complete the following Google form to request a permission number from the instructor ( https://forms.gle/z8X9pVZfCWMpQQ6o6 ).
Visualization with AI
Meeting Time: 04:00 PM‑05:15 PM TTh Instructor: Qianwen Wang Course Description: This course aims to investigate how visualization techniques and AI technologies work together to enhance understanding, insights, or outcomes.
This is a seminar style course consisting of lectures, paper presentation, and interactive discussion of the selected papers. Students will also work on a group project where they propose a research idea, survey related studies, and present initial results.
This course will cover the application of visualization to better understand AI models and data, and the use of AI to improve visualization processes. Readings for the course cover papers from the top venues of AI, Visualization, and HCI, topics including AI explainability, reliability, and Human-AI collaboration. This course is designed for PhD students, Masters students, and advanced undergraduates who want to dig into research.
Registration Prerequisites: Complete the following Google form to request a permission number from the instructor ( https://forms.gle/YTF5EZFUbQRJhHBYA ). Although the class is primarily intended for PhD students, motivated juniors/seniors and MS students who are interested in this topic are welcome to apply, ensuring they detail their qualifications for the course.
Visualizations for Intelligent AR Systems
Meeting Time: 04:00 PM‑05:15 PM MW Instructor: Zhu-Tian Chen Course Description: This course aims to explore the role of Data Visualization as a pivotal interface for enhancing human-data and human-AI interactions within Augmented Reality (AR) systems, thereby transforming a broad spectrum of activities in both professional and daily contexts. Structured as a seminar, the course consists of two main components: the theoretical and conceptual foundations delivered through lectures, paper readings, and discussions; and the hands-on experience gained through small assignments and group projects. This class is designed to be highly interactive, and AR devices will be provided to facilitate hands-on learning. Participants will have the opportunity to experience AR systems, develop cutting-edge AR interfaces, explore AI integration, and apply human-centric design principles. The course is designed to advance students' technical skills in AR and AI, as well as their understanding of how these technologies can be leveraged to enrich human experiences across various domains. Students will be encouraged to create innovative projects with the potential for submission to research conferences.
Registration Prerequisites: Complete the following Google form to request a permission number from the instructor ( https://forms.gle/Y81FGaJivoqMQYtq5 ). Students are expected to have a solid foundation in either data visualization, computer graphics, computer vision, or HCI. Having expertise in all would be perfect! However, a robust interest and eagerness to delve into these subjects can be equally valuable, even though it means you need to learn some basic concepts independently.
Sustainable Computing: A Systems View
Meeting Time: 09:45 AM‑11:00 AM Instructor: Abhishek Chandra Course Description: In recent years, there has been a dramatic increase in the pervasiveness, scale, and distribution of computing infrastructure: ranging from cloud, HPC systems, and data centers to edge computing and pervasive computing in the form of micro-data centers, mobile phones, sensors, and IoT devices embedded in the environment around us. The growing amount of computing, storage, and networking demand leads to increased energy usage, carbon emissions, and natural resource consumption. To reduce their environmental impact, there is a growing need to make computing systems sustainable. In this course, we will examine sustainable computing from a systems perspective. We will examine a number of questions: • How can we design and build sustainable computing systems? • How can we manage resources efficiently? • What system software and algorithms can reduce computational needs? Topics of interest would include: • Sustainable system design and architectures • Sustainability-aware systems software and management • Sustainability in large-scale distributed computing (clouds, data centers, HPC) • Sustainability in dispersed computing (edge, mobile computing, sensors/IoT)
Registration Prerequisites: This course is targeted towards students with a strong interest in computer systems (Operating Systems, Distributed Systems, Networking, Databases, etc.). Background in Operating Systems (Equivalent of CSCI 5103) and basic understanding of Computer Networking (Equivalent of CSCI 4211) is required.
- Future undergraduate students
- Future transfer students
- Future graduate students
- Future international students
- Diversity and Inclusion Opportunities
- Learn abroad
- Living Learning Communities
- Mentor programs
- Programs for women
- Student groups
- Visit, Apply & Next Steps
- Information for current students
- Departments and majors overview
- Departments
- Undergraduate majors
- Graduate programs
- Integrated Degree Programs
- Additional degree-granting programs
- Online learning
- Academic Advising overview
- Academic Advising FAQ
- Academic Advising Blog
- Appointments and drop-ins
- Academic support
- Commencement
- Four-year plans
- Honors advising
- Policies, procedures, and forms
- Career Services overview
- Resumes and cover letters
- Jobs and internships
- Interviews and job offers
- CSE Career Fair
- Major and career exploration
- Graduate school
- Collegiate Life overview
- Scholarships
- Diversity & Inclusivity Alliance
- Anderson Student Innovation Labs
- Information for alumni
- Get engaged with CSE
- Upcoming events
- CSE Alumni Society Board
- Alumni volunteer interest form
- Golden Medallion Society Reunion
- 50-Year Reunion
- Alumni honors and awards
- Outstanding Achievement
- Alumni Service
- Distinguished Leadership
- Honorary Doctorate Degrees
- Nobel Laureates
- Alumni resources
- Alumni career resources
- Alumni news outlets
- CSE branded clothing
- International alumni resources
- Inventing Tomorrow magazine
- Update your info
- CSE giving overview
- Why give to CSE?
- College priorities
- Give online now
- External relations
- Giving priorities
- Donor stories
- Impact of giving
- Ways to give to CSE
- Matching gifts
- CSE directories
- Invest in your company and the future
- Recruit our students
- Connect with researchers
- K-12 initiatives
- Diversity initiatives
- Research news
- Give to CSE
- CSE priorities
- Corporate relations
- Information for faculty and staff
- Administrative offices overview
- Office of the Dean
- Academic affairs
- Finance and Operations
- Communications
- Human resources
- Undergraduate programs and student services
- CSE Committees
- CSE policies overview
- Academic policies
- Faculty hiring and tenure policies
- Finance policies and information
- Graduate education policies
- Human resources policies
- Research policies
- Research overview
- Research centers and facilities
- Research proposal submission process
- Research safety
- Award-winning CSE faculty
- National academies
- University awards
- Honorary professorships
- Collegiate awards
- Other CSE honors and awards
- Staff awards
- Performance Management Process
- Work. With Flexibility in CSE
- K-12 outreach overview
- Summer camps
- Outreach events
- Enrichment programs
- Field trips and tours
- CSE K-12 Virtual Classroom Resources
- Educator development
- Sponsor an event
Main navigation
- For journalists
- For faculty and staff
- Experts guide
Millions of gamers advance biomedical research

- Tweet Widget
Leveraging gamers and video game technology can dramatically boost scientific research according to a new study published today in Nature Biotechnology .
4.5 million gamers around the world have advanced medical science by helping to reconstruct microbial evolutionary histories using a minigame included inside the critically and commercially successful video game, Borderlands 3 . Their playing has led to a significantly refined estimate of the relationships of microbes in the human gut. The results of this collaboration will both substantially advance our knowledge of the microbiome and improve on the AI programs that will be used to carry out this work in future.
Tracing the evolutionary relationships of bacteria
By playing Borderlands Science , a mini-game within the looter-shooter video game Borderlands 3 , these players have helped trace the evolutionary relationships of more than a million different kinds of bacteria that live in the human gut, some of which play a crucial role in our health. This information represents an exponential increase in what we have discovered about the microbiome up till now. By aligning rows of tiles which represent the genetic building blocks of different microbes, humans have been able to take on tasks that even the best existing computer algorithms have been unable to solve yet.
The project was led by McGill University researchers, developed in collaboration with Gearbox Entertainment Company , an award-winning interactive entertainment company, and Massively Multiplayer Online Science ( MMOS ) , a Swiss IT company connecting scientists to video games), and supported by the expertise and genomic material from the Microsetta Initiative led by Rob Knight from the Departments of Pediatrics, Bioengineering, and Computer Science & Engineering at the University of California San Diego.
Humans improve on existing algorithms and lay groundwork for the future
Not only have the gamers improved on the results produced by the existing programs used to analyze DNA sequences, but they are also helping lay the groundwork for improved AI programs that can be used in future.
“We didn’t know whether the players of a popular game like Borderlands 3 would be interested or whether the results would be good enough to improve on what was already known about microbial evolution. But we’ve been amazed by the results.” says Jérôme Waldispühl , an associate professor in McGill’s School of Computer Science and senior author on the paper published today. “In half a day, the Borderlands Science players collected five times more data about microbial DNA sequences than our earlier game, Phylo , had collected over a 10-year period.”
The idea for integrating DNA analysis into a commercial video game with mass market appeal came from Attila Szantner, an adjunct professor in McGill’s School of Computer Science and CEO and co-founder of MMOS . “As almost half of the world population is playing with videogames, it is of utmost importance that we find new creative ways to extract value from all this time and brainpower that we spend gaming,” says Szantner. “ Borderlands Science shows how far we can get by teaming up with the game industry and its communities to tackle the big challenges of our times.”
“Gearbox’s developers were eager to engage millions of Borderlands players globally with our creation of an appealing in-game experience to demonstrate how clever minds playing Borderlands are capable of producing tangible, useful, and valuable scientific data at a level not approachable with non-interactive technology and mediums,” said Randy Pitchford , founder and CEO of Gearbox Entertainment Company. “I'm proud that Borderlands Science has become one of the largest and most accomplished citizen science projects of all time, forecasting the opportunity for similar projects in future video games and pushing the boundaries of the positive effect that video games can make on the world.”
Relating microbes to disease and lifestyle
The tens of trillions of microbes that colonize our bodies play a crucial role in maintaining human health. But microbial communities can change over time in response to factors such as diet, medications, and lifestyle habits.
Because of the sheer number of microbes involved, scientists are still only in the early days of being able to identify which microorganisms are affected by, or can affect, which conditions.
Which is why the researchers’ project and the results from the gamers are so important.
“We expect to be able to use this information to relate specific kinds of microbes to what we eat, to how we age, and to the many diseases ranging from inflammatory bowel disease to Alzheimer’s that we now know microbes to be involved in,” adds Knight, who also directs the Center for Microbiome Innovation at the UC San Diego. “Because evolution is a great guide to function, having a better tree relating our microbes to one another gives us a more precise view of what they are doing within and around us.”
Building communities to advance knowledge
“Here we have 4.5 million people who contributed to science. In a sense, this result is theirs too and they should feel proud about it,” says Waldispühl . “It shows that we can fight the fear or misconceptions that members of the public may have about science and start building communities who work collectively to advance knowledge.”
“ Borderlands Science created an incredible opportunity to engage with citizen scientists on a novel and important problem, using data generated by a separate massive citizen science project,” adds Daniel McDonald, the Scientific Director of the Microsetta Initiative. “These results demonstrate the remarkable value of open access data, and the scale of what is possible with inclusive practices in scientific endeavors.”
“ Improving microbial phylogeny with citizen science within a mass-market video game ” by Roman Sarrazin-Gendron et al was published in Nature Biotechnology DOI: 10.1038/s41587-024-02175-6
The research was funded in part by Genome Canada and Génome Québec.
About McGill University
Founded in Montreal, Quebec, in 1821, McGill University is Canada’s top ranked medical doctoral university. McGill is consistently ranked as one of the top universities, both nationally and internationally. It is a world-renowned institution of higher learning with research activities spanning three campuses, 12 faculties, 14 professional schools, 300 programs of study and over 39,000 students, including more than 10,400 graduate students. McGill attracts students from over 150 countries around the world, its 12,000 international students making up 30% of the student body. Over half of McGill students claim a first language other than English, including approximately 20% of our students who say French is their mother tongue.
Contact Information
- News releases
- Faculty of Science
Related Content

Ehab Abouheif named Fellow of the American Association for the Advancement of Science

Divisive diagnosis raised in George Floyd case under scrutiny

Clearing the air: wind farms more land efficient than previously thought

Experts: Planet vs. Plastics | Earth Day 2024

Experts: Canadian federal budget 2024

Expert: Instagram cracks down on teen sextortion
Department and university information, institutional communications.

- McGill Reporter
- Office of the President
- Office of the Provost
- McGill experts guide
- About McGill
- Quick facts
- Administration
A third of China's urban population at risk of city sinking, new satellite data shows
L and subsidence is overlooked as a hazard in cities, according to scientists from the University of East Anglia (UEA) and Virginia Tech. Writing in the journal Science, Prof Robert Nicholls of the Tyndall Center for Climate Change Research at UEA and Prof Manoochehr Shirzaei of Virginia Tech and United Nations University for Water, Environment and Health, Ontario, highlight the importance of a new research paper analyzing satellite data that accurately and consistently maps land movement across China.
While they say in their comment article that consistently measuring subsidence is a great achievement, they argue it is only the start of finding solutions. Predicting future subsidence requires models that consider all drivers, including human activities and climate change, and how they might change with time.
The research paper, published in the same issue, considers 82 cities with a collective population of nearly 700 million people. The results show that 45% of the urban areas that were analyzed are sinking, with 16% falling at a rate of 10mm a year or more.
Nationally, roughly 270 million urban residents are estimated to be affected, with nearly 70 million experiencing rapid subsidence of 10mm a year or more. Hotspots include Beijing and Tianjin.
Coastal cities such as Tianjin are especially affected as sinking land reinforces climate change and sea-level rise. The sinking of sea defenses is one reason why Hurricane Katrina's flooding brought such devastation and death-toll to New Orleans in 2005.
Shanghai—China's biggest city—has subsided up to 3m over the past century and continues to subside today. When subsidence is combined with sea-level rise, the urban area in China below sea level could triple in size by 2120, affecting 55 to 128 million residents. This could be catastrophic without a strong societal response.
"Subsidence jeopardizes the structural integrity of buildings and critical infrastructure and exacerbates the impacts of climate change in terms of flooding, particularly in coastal cities where it reinforces sea-level rise," said Prof Nicholls, who was not involved in the study, but whose research focuses on sea-level rise, coastal erosion and flooding, and how communities can adapt to these changes.
The subsidence is mainly caused by human action in the cities. Groundwater withdrawal, which lowers the water table is considered the most important driver of subsidence, combined with geology and weight of buildings.
In Osaka and Tokyo, groundwater withdrawal was stopped in the 1970s and city subsidence has ceased or greatly reduced, showing this is an effective mitigation strategy. Traffic vibration and tunneling are potentially also a local contributing factor—Beijing has sinking of 45mm a year near subways and highways. Natural upward or downward land movement also occurs but is generally much smaller than human-induced changes.
While human-induced subsidence was known in China before this study, Profs Nicholls and Shirzaei say these new results reinforce the need for a national response. This problem happens in susceptible cities outside China and is a widespread problem across the world.
They call for the research community to move from measurement to understanding implications and supporting responses. The new satellite measurements are delivering new detailed subsidence data but the methods to use this information to work with city planners to address these problems need much more development. Affected coastal cities in China and more widely need particular attention.
"Many cities and areas worldwide are developing strategies for managing the risks of climate change and sea-level rise," said Prof Nicholls. "We need to learn from this experience to also address the threat of subsidence which is more common than currently recognized."
More information: Robert J. Nicholls, Assessing and responding to human-induced subsidence, Science (2024). DOI: 10.1126/science.ado9986 . www.science.org/doi/10.1126/science.ado9986
Provided by University of East Anglia

An official website of the United States government
The .gov means it’s official. Federal government websites often end in .gov or .mil. Before sharing sensitive information, make sure you’re on a federal government site.
The site is secure. The https:// ensures that you are connecting to the official website and that any information you provide is encrypted and transmitted securely.
- Publications
- Account settings
Preview improvements coming to the PMC website in October 2024. Learn More or Try it out now .
- Advanced Search
- Journal List
- Springer Nature - PMC COVID-19 Collection

The use of Big Data Analytics in healthcare
Kornelia batko.
1 Department of Business Informatics, University of Economics in Katowice, Katowice, Poland
Andrzej Ślęzak
2 Department of Biomedical Processes and Systems, Institute of Health and Nutrition Sciences, Częstochowa University of Technology, Częstochowa, Poland
Associated Data
The datasets for this study are available on request to the corresponding author.
The introduction of Big Data Analytics (BDA) in healthcare will allow to use new technologies both in treatment of patients and health management. The paper aims at analyzing the possibilities of using Big Data Analytics in healthcare. The research is based on a critical analysis of the literature, as well as the presentation of selected results of direct research on the use of Big Data Analytics in medical facilities. The direct research was carried out based on research questionnaire and conducted on a sample of 217 medical facilities in Poland. Literature studies have shown that the use of Big Data Analytics can bring many benefits to medical facilities, while direct research has shown that medical facilities in Poland are moving towards data-based healthcare because they use structured and unstructured data, reach for analytics in the administrative, business and clinical area. The research positively confirmed that medical facilities are working on both structural data and unstructured data. The following kinds and sources of data can be distinguished: from databases, transaction data, unstructured content of emails and documents, data from devices and sensors. However, the use of data from social media is lower as in their activity they reach for analytics, not only in the administrative and business but also in the clinical area. It clearly shows that the decisions made in medical facilities are highly data-driven. The results of the study confirm what has been analyzed in the literature that medical facilities are moving towards data-based healthcare, together with its benefits.
Introduction
The main contribution of this paper is to present an analytical overview of using structured and unstructured data (Big Data) analytics in medical facilities in Poland. Medical facilities use both structured and unstructured data in their practice. Structured data has a predetermined schema, it is extensive, freeform, and comes in variety of forms [ 27 ]. In contrast, unstructured data, referred to as Big Data (BD), does not fit into the typical data processing format. Big Data is a massive amount of data sets that cannot be stored, processed, or analyzed using traditional tools. It remains stored but not analyzed. Due to the lack of a well-defined schema, it is difficult to search and analyze such data and, therefore, it requires a specific technology and method to transform it into value [ 20 , 68 ]. Integrating data stored in both structured and unstructured formats can add significant value to an organization [ 27 ]. Organizations must approach unstructured data in a different way. Therefore, the potential is seen in Big Data Analytics (BDA). Big Data Analytics are techniques and tools used to analyze and extract information from Big Data. The results of Big Data analysis can be used to predict the future. They also help in creating trends about the past. When it comes to healthcare, it allows to analyze large datasets from thousands of patients, identifying clusters and correlation between datasets, as well as developing predictive models using data mining techniques [ 60 ].
This paper is the first study to consolidate and characterize the use of Big Data from different perspectives. The first part consists of a brief literature review of studies on Big Data (BD) and Big Data Analytics (BDA), while the second part presents results of direct research aimed at diagnosing the use of big data analyses in medical facilities in Poland.
Healthcare is a complex system with varied stakeholders: patients, doctors, hospitals, pharmaceutical companies and healthcare decision-makers. This sector is also limited by strict rules and regulations. However, worldwide one may observe a departure from the traditional doctor-patient approach. The doctor becomes a partner and the patient is involved in the therapeutic process [ 14 ]. Healthcare is no longer focused solely on the treatment of patients. The priority for decision-makers should be to promote proper health attitudes and prevent diseases that can be avoided [ 81 ]. This became visible and important especially during the Covid-19 pandemic [ 44 ].
The next challenges that healthcare will have to face is the growing number of elderly people and a decline in fertility. Fertility rates in the country are found below the reproductive minimum necessary to keep the population stable [ 10 ]. The reflection of both effects, namely the increase in age and lower fertility rates, are demographic load indicators, which is constantly growing. Forecasts show that providing healthcare in the form it is provided today will become impossible in the next 20 years [ 70 ]. It is especially visible now during the Covid-19 pandemic when healthcare faced quite a challenge related to the analysis of huge data amounts and the need to identify trends and predict the spread of the coronavirus. The pandemic showed it even more that patients should have access to information about their health condition, the possibility of digital analysis of this data and access to reliable medical support online. Health monitoring and cooperation with doctors in order to prevent diseases can actually revolutionize the healthcare system. One of the most important aspects of the change necessary in healthcare is putting the patient in the center of the system.
Technology is not enough to achieve these goals. Therefore, changes should be made not only at the technological level but also in the management and design of complete healthcare processes and what is more, they should affect the business models of service providers. The use of Big Data Analytics is becoming more and more common in enterprises [ 17 , 54 ]. However, medical enterprises still cannot keep up with the information needs of patients, clinicians, administrators and the creator’s policy. The adoption of a Big Data approach would allow the implementation of personalized and precise medicine based on personalized information, delivered in real time and tailored to individual patients.
To achieve this goal, it is necessary to implement systems that will be able to learn quickly about the data generated by people within clinical care and everyday life. This will enable data-driven decision making, receiving better personalized predictions about prognosis and responses to treatments; a deeper understanding of the complex factors and their interactions that influence health at the patient level, the health system and society, enhanced approaches to detecting safety problems with drugs and devices, as well as more effective methods of comparing prevention, diagnostic, and treatment options [ 40 ].
In the literature, there is a lot of research showing what opportunities can be offered to companies by big data analysis and what data can be analyzed. However, there are few studies showing how data analysis in the area of healthcare is performed, what data is used by medical facilities and what analyses and in which areas they carry out. This paper aims to fill this gap by presenting the results of research carried out in medical facilities in Poland. The goal is to analyze the possibilities of using Big Data Analytics in healthcare, especially in Polish conditions. In particular, the paper is aimed at determining what data is processed by medical facilities in Poland, what analyses they perform and in what areas, and how they assess their analytical maturity. In order to achieve this goal, a critical analysis of the literature was performed, and the direct research was based on a research questionnaire conducted on a sample of 217 medical facilities in Poland. It was hypothesized that medical facilities in Poland are working on both structured and unstructured data and moving towards data-based healthcare and its benefits. Examining the maturity of healthcare facilities in the use of Big Data and Big Data Analytics is crucial in determining the potential future benefits that the healthcare sector can gain from Big Data Analytics. There is also a pressing need to predicate whether, in the coming years, healthcare will be able to cope with the threats and challenges it faces.
This paper is divided into eight parts. The first is the introduction which provides background and the general problem statement of this research. In the second part, this paper discusses considerations on use of Big Data and Big Data Analytics in Healthcare, and then, in the third part, it moves on to challenges and potential benefits of using Big Data Analytics in healthcare. The next part involves the explanation of the proposed method. The result of direct research and discussion are presented in the fifth part, while the following part of the paper is the conclusion. The seventh part of the paper presents practical implications. The final section of the paper provides limitations and directions for future research.
Considerations on use Big Data and Big Data Analytics in the healthcare
In recent years one can observe a constantly increasing demand for solutions offering effective analytical tools. This trend is also noticeable in the analysis of large volumes of data (Big Data, BD). Organizations are looking for ways to use the power of Big Data to improve their decision making, competitive advantage or business performance [ 7 , 54 ]. Big Data is considered to offer potential solutions to public and private organizations, however, still not much is known about the outcome of the practical use of Big Data in different types of organizations [ 24 ].
As already mentioned, in recent years, healthcare management worldwide has been changed from a disease-centered model to a patient-centered model, even in value-based healthcare delivery model [ 68 ]. In order to meet the requirements of this model and provide effective patient-centered care, it is necessary to manage and analyze healthcare Big Data.
The issue often raised when it comes to the use of data in healthcare is the appropriate use of Big Data. Healthcare has always generated huge amounts of data and nowadays, the introduction of electronic medical records, as well as the huge amount of data sent by various types of sensors or generated by patients in social media causes data streams to constantly grow. Also, the medical industry generates significant amounts of data, including clinical records, medical images, genomic data and health behaviors. Proper use of the data will allow healthcare organizations to support clinical decision-making, disease surveillance, and public health management. The challenge posed by clinical data processing involves not only the quantity of data but also the difficulty in processing it.
In the literature one can find many different definitions of Big Data. This concept has evolved in recent years, however, it is still not clearly understood. Nevertheless, despite the range and differences in definitions, Big Data can be treated as a: large amount of digital data, large data sets, tool, technology or phenomenon (cultural or technological.
Big Data can be considered as massive and continually generated digital datasets that are produced via interactions with online technologies [ 53 ]. Big Data can be defined as datasets that are of such large sizes that they pose challenges in traditional storage and analysis techniques [ 28 ]. A similar opinion about Big Data was presented by Ohlhorst who sees Big Data as extremely large data sets, possible neither to manage nor to analyze with traditional data processing tools [ 57 ]. In his opinion, the bigger the data set, the more difficult it is to gain any value from it.
In turn, Knapp perceived Big Data as tools, processes and procedures that allow an organization to create, manipulate and manage very large data sets and storage facilities [ 38 ]. From this point of view, Big Data is identified as a tool to gather information from different databases and processes, allowing users to manage large amounts of data.
Similar perception of the term ‘Big Data’ is shown by Carter. According to him, Big Data technologies refer to a new generation of technologies and architectures, designed to economically extract value from very large volumes of a wide variety of data by enabling high velocity capture, discovery and/or analysis [ 13 ].
Jordan combines these two approaches by identifying Big Data as a complex system, as it needs data bases for data to be stored in, programs and tools to be managed, as well as expertise and personnel able to retrieve useful information and visualization to be understood [ 37 ].
Following the definition of Laney for Big Data, it can be state that: it is large amount of data generated in very fast motion and it contains a lot of content [ 43 ]. Such data comes from unstructured sources, such as stream of clicks on the web, social networks (Twitter, blogs, Facebook), video recordings from the shops, recording of calls in a call center, real time information from various kinds of sensors, RFID, GPS devices, mobile phones and other devices that identify and monitor something [ 8 ]. Big Data is a powerful digital data silo, raw, collected with all sorts of sources, unstructured and difficult, or even impossible, to analyze using conventional techniques used so far to relational databases.
While describing Big Data, it cannot be overlooked that the term refers more to a phenomenon than to specific technology. Therefore, instead of defining this phenomenon, trying to describe them, more authors are describing Big Data by giving them characteristics included a collection of V’s related to its nature [ 2 , 3 , 23 , 25 , 58 ]:
- Volume (refers to the amount of data and is one of the biggest challenges in Big Data Analytics),
- Velocity (speed with which new data is generated, the challenge is to be able to manage data effectively and in real time),
- Variety (heterogeneity of data, many different types of healthcare data, the challenge is to derive insights by looking at all available heterogenous data in a holistic manner),
- Variability (inconsistency of data, the challenge is to correct the interpretation of data that can vary significantly depending on the context),
- Veracity (how trustworthy the data is, quality of the data),
- Visualization (ability to interpret data and resulting insights, challenging for Big Data due to its other features as described above).
- Value (the goal of Big Data Analytics is to discover the hidden knowledge from huge amounts of data).
Big Data is defined as an information asset with high volume, velocity, and variety, which requires specific technology and method for its transformation into value [ 21 , 77 ]. Big Data is also a collection of information about high-volume, high volatility or high diversity, requiring new forms of processing in order to support decision-making, discovering new phenomena and process optimization [ 5 , 7 ]. Big Data is too large for traditional data-processing systems and software tools to capture, store, manage and analyze, therefore it requires new technologies [ 28 , 50 , 61 ] to manage (capture, aggregate, process) its volume, velocity and variety [ 9 ].
Undoubtedly, Big Data differs from the data sources used so far by organizations. Therefore, organizations must approach this type of unstructured data in a different way. First of all, organizations must start to see data as flows and not stocks—this entails the need to implement the so-called streaming analytics [ 48 ]. The mentioned features make it necessary to use new IT tools that allow the fullest use of new data [ 58 ]. The Big Data idea, inseparable from the huge increase in data available to various organizations or individuals, creates opportunities for access to valuable analyses, conclusions and enables making more accurate decisions [ 6 , 11 , 59 ].
The Big Data concept is constantly evolving and currently it does not focus on huge amounts of data, but rather on the process of creating value from this data [ 52 ]. Big Data is collected from various sources that have different data properties and are processed by different organizational units, resulting in creation of a Big Data chain [ 36 ]. The aim of the organizations is to manage, process and analyze Big Data. In the healthcare sector, Big Data streams consist of various types of data, namely [ 8 , 51 ]:
- clinical data, i.e. data obtained from electronic medical records, data from hospital information systems, image centers, laboratories, pharmacies and other organizations providing health services, patient generated health data, physician’s free-text notes, genomic data, physiological monitoring data [ 4 ],
- biometric data provided from various types of devices that monitor weight, pressure, glucose level, etc.,
- financial data, constituting a full record of economic operations reflecting the conducted activity,
- data from scientific research activities, i.e. results of research, including drug research, design of medical devices and new methods of treatment,
- data provided by patients, including description of preferences, level of satisfaction, information from systems for self-monitoring of their activity: exercises, sleep, meals consumed, etc.
- data from social media.
These data are provided not only by patients but also by organizations and institutions, as well as by various types of monitoring devices, sensors or instruments [ 16 ]. Data that has been generated so far in the healthcare sector is stored in both paper and digital form. Thus, the essence and the specificity of the process of Big Data analyses means that organizations need to face new technological and organizational challenges [ 67 ]. The healthcare sector has always generated huge amounts of data and this is connected, among others, with the need to store medical records of patients. However, the problem with Big Data in healthcare is not limited to an overwhelming volume but also an unprecedented diversity in terms of types, data formats and speed with which it should be analyzed in order to provide the necessary information on an ongoing basis [ 3 ]. It is also difficult to apply traditional tools and methods for management of unstructured data [ 67 ]. Due to the diversity and quantity of data sources that are growing all the time, advanced analytical tools and technologies, as well as Big Data analysis methods which can meet and exceed the possibilities of managing healthcare data, are needed [ 3 , 68 ].
Therefore, the potential is seen in Big Data analyses, especially in the aspect of improving the quality of medical care, saving lives or reducing costs [ 30 ]. Extracting from this tangle of given association rules, patterns and trends will allow health service providers and other stakeholders in the healthcare sector to offer more accurate and more insightful diagnoses of patients, personalized treatment, monitoring of the patients, preventive medicine, support of medical research and health population, as well as better quality of medical services and patient care while, at the same time, the ability to reduce costs (Fig. 1 ).

Healthcare Big Data Analytics applications
(Source: Own elaboration)
The main challenge with Big Data is how to handle such a large amount of information and use it to make data-driven decisions in plenty of areas [ 64 ]. In the context of healthcare data, another major challenge is to adjust big data storage, analysis, presentation of analysis results and inference basing on them in a clinical setting. Data analytics systems implemented in healthcare are designed to describe, integrate and present complex data in an appropriate way so that it can be understood better (Fig. 2 ). This would improve the efficiency of acquiring, storing, analyzing and visualizing big data from healthcare [ 71 ].

Process of Big Data Analytics
The result of data processing with the use of Big Data Analytics is appropriate data storytelling which may contribute to making decisions with both lower risk and data support. This, in turn, can benefit healthcare stakeholders. To take advantage of the potential massive amounts of data in healthcare and to ensure that the right intervention to the right patient is properly timed, personalized, and potentially beneficial to all components of the healthcare system such as the payer, patient, and management, analytics of large datasets must connect communities involved in data analytics and healthcare informatics [ 49 ]. Big Data Analytics can provide insight into clinical data and thus facilitate informed decision-making about the diagnosis and treatment of patients, prevention of diseases or others. Big Data Analytics can also improve the efficiency of healthcare organizations by realizing the data potential [ 3 , 62 ].
Big Data Analytics in medicine and healthcare refers to the integration and analysis of a large amount of complex heterogeneous data, such as various omics (genomics, epigenomics, transcriptomics, proteomics, metabolomics, interactomics, pharmacogenetics, deasomics), biomedical data, talemedicine data (sensors, medical equipment data) and electronic health records data [ 46 , 65 ].
When analyzing the phenomenon of Big Data in the healthcare sector, it should be noted that it can be considered from the point of view of three areas: epidemiological, clinical and business.
From a clinical point of view, the Big Data analysis aims to improve the health and condition of patients, enable long-term predictions about their health status and implementation of appropriate therapeutic procedures. Ultimately, the use of data analysis in medicine is to allow the adaptation of therapy to a specific patient, that is personalized medicine (precision, personalized medicine).
From an epidemiological point of view, it is desirable to obtain an accurate prognosis of morbidity in order to implement preventive programs in advance.
In the business context, Big Data analysis may enable offering personalized packages of commercial services or determining the probability of individual disease and infection occurrence. It is worth noting that Big Data means not only the collection and processing of data but, most of all, the inference and visualization of data necessary to obtain specific business benefits.
In order to introduce new management methods and new solutions in terms of effectiveness and transparency, it becomes necessary to make data more accessible, digital, searchable, as well as analyzed and visualized.
Erickson and Rothberg state that the information and data do not reveal their full value until insights are drawn from them. Data becomes useful when it enhances decision making and decision making is enhanced only when analytical techniques are used and an element of human interaction is applied [ 22 ].
Thus, healthcare has experienced much progress in usage and analysis of data. A large-scale digitalization and transparency in this sector is a key statement of almost all countries governments policies. For centuries, the treatment of patients was based on the judgment of doctors who made treatment decisions. In recent years, however, Evidence-Based Medicine has become more and more important as a result of it being related to the systematic analysis of clinical data and decision-making treatment based on the best available information [ 42 ]. In the healthcare sector, Big Data Analytics is expected to improve the quality of life and reduce operational costs [ 72 , 82 ]. Big Data Analytics enables organizations to improve and increase their understanding of the information contained in data. It also helps identify data that provides insightful insights for current as well as future decisions [ 28 ].
Big Data Analytics refers to technologies that are grounded mostly in data mining: text mining, web mining, process mining, audio and video analytics, statistical analysis, network analytics, social media analytics and web analytics [ 16 , 25 , 31 ]. Different data mining techniques can be applied on heterogeneous healthcare data sets, such as: anomaly detection, clustering, classification, association rules as well as summarization and visualization of those Big Data sets [ 65 ]. Modern data analytics techniques explore and leverage unique data characteristics even from high-speed data streams and sensor data [ 15 , 16 , 31 , 55 ]. Big Data can be used, for example, for better diagnosis in the context of comprehensive patient data, disease prevention and telemedicine (in particular when using real-time alerts for immediate care), monitoring patients at home, preventing unnecessary hospital visits, integrating medical imaging for a wider diagnosis, creating predictive analytics, reducing fraud and improving data security, better strategic planning and increasing patients’ involvement in their own health.
Big Data Analytics in healthcare can be divided into [ 33 , 73 , 74 ]:
- descriptive analytics in healthcare is used to understand past and current healthcare decisions, converting data into useful information for understanding and analyzing healthcare decisions, outcomes and quality, as well as making informed decisions [ 33 ]. It can be used to create reports (i.e. about patients’ hospitalizations, physicians’ performance, utilization management), visualization, customized reports, drill down tables, or running queries on the basis of historical data.
- predictive analytics operates on past performance in an effort to predict the future by examining historical or summarized health data, detecting patterns of relationships in these data, and then extrapolating these relationships to forecast. It can be used to i.e. predict the response of different patient groups to different drugs (dosages) or reactions (clinical trials), anticipate risk and find relationships in health data and detect hidden patterns [ 62 ]. In this way, it is possible to predict the epidemic spread, anticipate service contracts and plan healthcare resources. Predictive analytics is used in proper diagnosis and for appropriate treatments to be given to patients suffering from certain diseases [ 39 ].
- prescriptive analytics—occurs when health problems involve too many choices or alternatives. It uses health and medical knowledge in addition to data or information. Prescriptive analytics is used in many areas of healthcare, including drug prescriptions and treatment alternatives. Personalized medicine and evidence-based medicine are both supported by prescriptive analytics.
- discovery analytics—utilizes knowledge about knowledge to discover new “inventions” like drugs (drug discovery), previously unknown diseases and medical conditions, alternative treatments, etc.
Although the models and tools used in descriptive, predictive, prescriptive, and discovery analytics are different, many applications involve all four of them [ 62 ]. Big Data Analytics in healthcare can help enable personalized medicine by identifying optimal patient-specific treatments. This can influence the improvement of life standards, reduce waste of healthcare resources and save costs of healthcare [ 56 , 63 , 71 ]. The introduction of large data analysis gives new analytical possibilities in terms of scope, flexibility and visualization. Techniques such as data mining (computational pattern discovery process in large data sets) facilitate inductive reasoning and analysis of exploratory data, enabling scientists to identify data patterns that are independent of specific hypotheses. As a result, predictive analysis and real-time analysis becomes possible, making it easier for medical staff to start early treatments and reduce potential morbidity and mortality. In addition, document analysis, statistical modeling, discovering patterns and topics in document collections and data in the EHR, as well as an inductive approach can help identify and discover relationships between health phenomena.
Advanced analytical techniques can be used for a large amount of existing (but not yet analytical) data on patient health and related medical data to achieve a better understanding of the information and results obtained, as well as to design optimal clinical pathways [ 62 ]. Big Data Analytics in healthcare integrates analysis of several scientific areas such as bioinformatics, medical imaging, sensor informatics, medical informatics and health informatics [ 65 ]. Big Data Analytics in healthcare allows to analyze large datasets from thousands of patients, identifying clusters and correlation between datasets, as well as developing predictive models using data mining techniques [ 65 ]. Discussing all the techniques used for Big Data Analytics goes beyond the scope of a single article [ 25 ].
The success of Big Data analysis and its accuracy depend heavily on the tools and techniques used to analyze the ability to provide reliable, up-to-date and meaningful information to various stakeholders [ 12 ]. It is believed that the implementation of big data analytics by healthcare organizations could bring many benefits in the upcoming years, including lowering health care costs, better diagnosis and prediction of diseases and their spread, improving patient care and developing protocols to prevent re-hospitalization, optimizing staff, optimizing equipment, forecasting the need for hospital beds, operating rooms, treatments, and improving the drug supply chain [ 71 ].
Challenges and potential benefits of using Big Data Analytics in healthcare
Modern analytics gives possibilities not only to have insight in historical data, but also to have information necessary to generate insight into what may happen in the future. Even when it comes to prediction of evidence-based actions. The emphasis on reform has prompted payers and suppliers to pursue data analysis to reduce risk, detect fraud, improve efficiency and save lives. Everyone—payers, providers, even patients—are focusing on doing more with fewer resources. Thus, some areas in which enhanced data and analytics can yield the greatest results include various healthcare stakeholders (Table (Table1 1 ).
The use of analytics by various healthcare stakeholders
Source: own elaboration on the basis of [ 19 , 20 ]
Healthcare organizations see the opportunity to grow through investments in Big Data Analytics. In recent years, by collecting medical data of patients, converting them into Big Data and applying appropriate algorithms, reliable information has been generated that helps patients, physicians and stakeholders in the health sector to identify values and opportunities [ 31 ]. It is worth noting that there are many changes and challenges in the structure of the healthcare sector. Digitization and effective use of Big Data in healthcare can bring benefits to every stakeholder in this sector. A single doctor would benefit the same as the entire healthcare system. Potential opportunities to achieve benefits and effects from Big Data in healthcare can be divided into four groups [ 8 ]:
- assessment of diagnoses made by doctors and the manner of treatment of diseases indicated by them based on the decision support system working on Big Data collections,
- detection of more effective, from a medical point of view, and more cost-effective ways to diagnose and treat patients,
- analysis of large volumes of data to reach practical information useful for identifying needs, introducing new health services, preventing and overcoming crises,
- prediction of the incidence of diseases,
- detecting trends that lead to an improvement in health and lifestyle of the society,
- analysis of the human genome for the introduction of personalized treatment.
- doctors’ comparison of current medical cases to cases from the past for better diagnosis and treatment adjustment,
- detection of diseases at earlier stages when they can be more easily and quickly cured,
- detecting epidemiological risks and improving control of pathogenic spots and reaction rates,
- identification of patients who are predicted to have the highest risk of specific, life-threatening diseases by collating data on the history of the most common diseases, in healing people with reports entering insurance companies,
- health management of each patient individually (personalized medicine) and health management of the whole society,
- capturing and analyzing large amounts of data from hospitals and homes in real time, life monitoring devices to monitor safety and predict adverse events,
- analysis of patient profiles to identify people for whom prevention should be applied, lifestyle change or preventive care approach,
- the ability to predict the occurrence of specific diseases or worsening of patients’ results,
- predicting disease progression and its determinants, estimating the risk of complications,
- detecting drug interactions and their side effects.
- supporting work on new drugs and clinical trials thanks to the possibility of analyzing “all data” instead of selecting a test sample,
- the ability to identify patients with specific, biological features that will take part in specialized clinical trials,
- selecting a group of patients for which the tested drug is likely to have the desired effect and no side effects,
- using modeling and predictive analysis to design better drugs and devices.
- reduction of costs and counteracting abuse and counseling practices,
- faster and more effective identification of incorrect or unauthorized financial operations in order to prevent abuse and eliminate errors,
- increase in profitability by detecting patients generating high costs or identifying doctors whose work, procedures and treatment methods cost the most and offering them solutions that reduce the amount of money spent,
- identification of unnecessary medical activities and procedures, e.g. duplicate tests.
According to research conducted by Wang, Kung and Byrd, Big Data Analytics benefits can be classified into five categories: IT infrastructure benefits (reducing system redundancy, avoiding unnecessary IT costs, transferring data quickly among healthcare IT systems, better use of healthcare systems, processing standardization among various healthcare IT systems, reducing IT maintenance costs regarding data storage), operational benefits (improving the quality and accuracy of clinical decisions, processing a large number of health records in seconds, reducing the time of patient travel, immediate access to clinical data to analyze, shortening the time of diagnostic test, reductions in surgery-related hospitalizations, exploring inconceivable new research avenues), organizational benefits (detecting interoperability problems much more quickly than traditional manual methods, improving cross-functional communication and collaboration among administrative staffs, researchers, clinicians and IT staffs, enabling data sharing with other institutions and adding new services, content sources and research partners), managerial benefits (gaining quick insights about changing healthcare trends in the market, providing members of the board and heads of department with sound decision-support information on the daily clinical setting, optimizing business growth-related decisions) and strategic benefits (providing a big picture view of treatment delivery for meeting future need, creating high competitive healthcare services) [ 73 ].
The above specification does not constitute a full list of potential areas of use of Big Data Analysis in healthcare because the possibilities of using analysis are practically unlimited. In addition, advanced analytical tools allow to analyze data from all possible sources and conduct cross-analyses to provide better data insights [ 26 ]. For example, a cross-analysis can refer to a combination of patient characteristics, as well as costs and care results that can help identify the best, in medical terms, and the most cost-effective treatment or treatments and this may allow a better adjustment of the service provider’s offer [ 62 ].
In turn, the analysis of patient profiles (e.g. segmentation and predictive modeling) allows identification of people who should be subject to prophylaxis, prevention or should change their lifestyle [ 8 ]. Shortened list of benefits for Big Data Analytics in healthcare is presented in paper [ 3 ] and consists of: better performance, day-to-day guides, detection of diseases in early stages, making predictive analytics, cost effectiveness, Evidence Based Medicine and effectiveness in patient treatment.
Summarizing, healthcare big data represents a huge potential for the transformation of healthcare: improvement of patients’ results, prediction of outbreaks of epidemics, valuable insights, avoidance of preventable diseases, reduction of the cost of healthcare delivery and improvement of the quality of life in general [ 1 ]. Big Data also generates many challenges such as difficulties in data capture, data storage, data analysis and data visualization [ 15 ]. The main challenges are connected with the issues of: data structure (Big Data should be user-friendly, transparent, and menu-driven but it is fragmented, dispersed, rarely standardized and difficult to aggregate and analyze), security (data security, privacy and sensitivity of healthcare data, there are significant concerns related to confidentiality), data standardization (data is stored in formats that are not compatible with all applications and technologies), storage and transfers (especially costs associated with securing, storing, and transferring unstructured data), managerial skills, such as data governance, lack of appropriate analytical skills and problems with Real-Time Analytics (health care is to be able to utilize Big Data in real time) [ 4 , 34 , 41 ].
The research is based on a critical analysis of the literature, as well as the presentation of selected results of direct research on the use of Big Data Analytics in medical facilities in Poland.
Presented research results are part of a larger questionnaire form on Big Data Analytics. The direct research was based on an interview questionnaire which contained 100 questions with 5-point Likert scale (1—strongly disagree, 2—I rather disagree, 3—I do not agree, nor disagree, 4—I rather agree, 5—I definitely agree) and 4 metrics questions. The study was conducted in December 2018 on a sample of 217 medical facilities (110 private, 107 public). The research was conducted by a specialized market research agency: Center for Research and Expertise of the University of Economics in Katowice.
When it comes to direct research, the selected entities included entities financed from public sources—the National Health Fund (23.5%), and entities operating commercially (11.5%). In the surveyed group of entities, more than a half (64.9%) are hybrid financed, both from public and commercial sources. The diversity of the research sample also applies to the size of the entities, defined by the number of employees. Taking into account proportions of the surveyed entities, it should be noted that in the sector structure, medium-sized (10–50 employees—34% of the sample) and large (51–250 employees—27%) entities dominate. The research was of all-Poland nature, and the entities included in the research sample come from all of the voivodships. The largest group were entities from Łódzkie (32%), Śląskie (18%) and Mazowieckie (18%) voivodships, as these voivodships have the largest number of medical institutions. Other regions of the country were represented by single units. The selection of the research sample was random—layered. As part of medical facilities database, groups of private and public medical facilities have been identified and the ones to which the questionnaire was targeted were drawn from each of these groups. The analyses were performed using the GNU PSPP 0.10.2 software.
The aim of the study was to determine whether medical facilities in Poland use Big Data Analytics and if so, in which areas. Characteristics of the research sample is presented in Table Table2 2 .
Characteristics of the research sample
The research is non-exhaustive due to the incomplete and uneven regional distribution of the samples, overrepresented in three voivodeships (Łódzkie, Mazowieckie and Śląskie). The size of the research sample (217 entities) allows the authors of the paper to formulate specific conclusions on the use of Big Data in the process of its management.
For the purpose of this paper, the following research hypotheses were formulated: (1) medical facilities in Poland are working on both structured and unstructured data (2) medical facilities in Poland are moving towards data-based healthcare and its benefits.
The paper poses the following research questions and statements that coincide with the selected questions from the research questionnaire:
- From what sources do medical facilities obtain data? What types of data are used by the particular organization, whether structured or unstructured, and to what extent?
- From what sources do medical facilities obtain data?
- In which area organizations are using data and analytical systems (clinical or business)?
- Is data analytics performed based on historical data or are predictive analyses also performed?
- Determining whether administrative and medical staff receive complete, accurate and reliable data in a timely manner?
- Determining whether real-time analyses are performed to support the particular organization’s activities.
Results and discussion
On the basis of the literature analysis and research study, a set of questions and statements related to the researched area was formulated. The results from the surveys show that medical facilities use a variety of data sources in their operations. These sources are both structured and unstructured data (Table (Table3 3 ).
Type of data sources used in medical facility (%)
1—strongly disagree, 2—I disagree, 3—I agree or disagree, 4—I rather agree, 5—I strongly agree
According to the data provided by the respondents, considering the first statement made in the questionnaire, almost half of the medical institutions (47.58%) agreed that they rather collect and use structured data (e.g. databases and data warehouses, reports to external entities) and 10.57% entirely agree with this statement. As much as 23.35% of representatives of medical institutions stated “I agree or disagree”. Other medical facilities do not collect and use structured data (7.93%) and 6.17% strongly disagree with the first statement. Also, the median calculated based on the obtained results (median: 4), proves that medical facilities in Poland collect and use structured data (Table (Table4 4 ).
Collection and use of data determined by the size of medical facility (number of employees)
In turn, 28.19% of the medical institutions agreed that they rather collect and use unstructured data and as much as 9.25% entirely agree with this statement. The number of representatives of medical institutions that stated “I agree or disagree” was 27.31%. Other medical facilities do not collect and use structured data (17.18%) and 13.66% strongly disagree with the first statement. In the case of unstructured data the median is 3, which means that the collection and use of this type of data by medical facilities in Poland is lower.
In the further part of the analysis, it was checked whether the size of the medical facility and form of ownership have an impact on whether it analyzes unstructured data (Tables (Tables4 4 and and5). 5 ). In order to find this out, correlation coefficients were calculated.
Collection and use of data determined by the form of ownership of medical facility
Based on the calculations, it can be concluded that there is a small statistically monotonic correlation between the size of the medical facility and its collection and use of structured data (p < 0.001; τ = 0.16). This means that the use of structured data is slightly increasing in larger medical facilities. The size of the medical facility is more important according to use of unstructured data (p < 0.001; τ = 0.23) (Table (Table4 4 .).
To determine whether the form of medical facility ownership affects data collection, the Mann–Whitney U test was used. The calculations show that the form of ownership does not affect what data the organization collects and uses (Table (Table5 5 ).
Detailed information on the sources of from which medical facilities collect and use data is presented in the Table Table6 6 .
Data sources used in medical facility
1—we do not use at all, 5—we use extensively
The questionnaire results show that medical facilities are especially using information published in databases, reports to external units and transaction data, but they also use unstructured data from e-mails, medical devices, sensors, phone calls, audio and video data (Table (Table6). 6 ). Data from social media, RFID and geolocation data are used to a small extent. Similar findings are concluded in the literature studies.
From the analysis of the answers given by the respondents, more than half of the medical facilities have integrated hospital system (HIS) implemented. As much as 43.61% use integrated hospital system and 16.30% use it extensively (Table (Table7). 7 ). 19.38% of exanimated medical facilities do not use it at all. Moreover, most of the examined medical facilities (34.80% use it, 32.16% use extensively) conduct medical documentation in an electronic form, which gives an opportunity to use data analytics. Only 4.85% of medical facilities don’t use it at all.
The use of HIS and electronic documentation in medical facilities (%)
Other problems that needed to be investigated were: whether medical facilities in Poland use data analytics? If so, in what form and in what areas? (Table (Table8). 8 ). The analysis of answers given by the respondents about the potential of data analytics in medical facilities shows that a similar number of medical facilities use data analytics in administration and business (31.72% agreed with the statement no. 5 and 12.33% strongly agreed) as in the clinical area (33.04% agreed with the statement no. 6 and 12.33% strongly agreed). When considering decision-making issues, 35.24% agree with the statement "the organization uses data and analytical systems to support business decisions” and 8.37% of respondents strongly agree. Almost 40.09% agree with the statement that “the organization uses data and analytical systems to support clinical decisions (in the field of diagnostics and therapy)” and 15.42% of respondents strongly agree. Exanimated medical facilities use in their activity analytics based both on historical data (33.48% agree with statement 7 and 12.78% strongly agree) and predictive analytics (33.04% agrees with the statement number 8 and 15.86% strongly agree). Detailed results are presented in Table Table8 8 .
Conditions of using Big Data Analytics in medical facilities (%)
Medical facilities focus on development in the field of data processing, as they confirm that they conduct analytical planning processes systematically and analyze new opportunities for strategic use of analytics in business and clinical activities (38.33% rather agree and 10.57% strongly agree with this statement). The situation is different with real-time data analysis, here, the situation is not so optimistic. Only 28.19% rather agree and 14.10% strongly agree with the statement that real-time analyses are performed to support an organization’s activities.
When considering whether a facility’s performance in the clinical area depends on the form of ownership, it can be concluded that taking the average and the Mann–Whitney U test depends. A higher degree of use of analyses in the clinical area can be observed in public institutions.
Whether a medical facility performs a descriptive or predictive analysis do not depend on the form of ownership (p > 0.05). It can be concluded that when analyzing the mean and median, they are higher in public facilities, than in private ones. What is more, the Mann–Whitney U test shows that these variables are dependent from each other (p < 0.05) (Table (Table9 9 ).
Conditions of using Big Data Analytics in medical facilities determined by the form of ownership of medical facility
When considering whether a facility’s performance in the clinical area depends on its size, it can be concluded that taking the Kendall’s Tau (τ) it depends (p < 0.001; τ = 0.22), and the correlation is weak but statistically important. This means that the use of data and analytical systems to support clinical decisions (in the field of diagnostics and therapy) increases with the increase of size of the medical facility. A similar relationship, but even less powerful, can be found in the use of descriptive and predictive analyses (Table (Table10 10 ).
Conditions of using Big Data Analytics in medical facilities determined by the size of medical facility (number of employees)
Considering the results of research in the area of analytical maturity of medical facilities, 8.81% of medical facilities stated that they are at the first level of maturity, i.e. an organization has developed analytical skills and does not perform analyses. As much as 13.66% of medical facilities confirmed that they have poor analytical skills, while 38.33% of the medical facility has located itself at level 3, meaning that “there is a lot to do in analytics”. On the other hand, 28.19% believe that analytical capabilities are well developed and 6.61% stated that analytics are at the highest level and the analytical capabilities are very well developed. Detailed data is presented in Table Table11. 11 . Average amounts to 3.11 and Median to 3.
Analytical maturity of examined medical facilities (%)
The results of the research have enabled the formulation of following conclusions. Medical facilities in Poland are working on both structured and unstructured data. This data comes from databases, transactions, unstructured content of emails and documents, devices and sensors. However, the use of data from social media is smaller. In their activity, they reach for analytics in the administrative and business, as well as in the clinical area. Also, the decisions made are largely data-driven.
In summary, analysis of the literature that the benefits that medical facilities can get using Big Data Analytics in their activities relate primarily to patients, physicians and medical facilities. It can be confirmed that: patients will be better informed, will receive treatments that will work for them, will have prescribed medications that work for them and not be given unnecessary medications [ 78 ]. Physician roles will likely change to more of a consultant than decision maker. They will advise, warn, and help individual patients and have more time to form positive and lasting relationships with their patients in order to help people. Medical facilities will see changes as well, for example in fewer unnecessary hospitalizations, resulting initially in less revenue, but after the market adjusts, also the accomplishment [ 78 ]. The use of Big Data Analytics can literally revolutionize the way healthcare is practiced for better health and disease reduction.
The analysis of the latest data reveals that data analytics increase the accuracy of diagnoses. Physicians can use predictive algorithms to help them make more accurate diagnoses [ 45 ]. Moreover, it could be helpful in preventive medicine and public health because with early intervention, many diseases can be prevented or ameliorated [ 29 ]. Predictive analytics also allows to identify risk factors for a given patient, and with this knowledge patients will be able to change their lives what, in turn, may contribute to the fact that population disease patterns may dramatically change, resulting in savings in medical costs. Moreover, personalized medicine is the best solution for an individual patient seeking treatment. It can help doctors decide the exact treatments for those individuals. Better diagnoses and more targeted treatments will naturally lead to increases in good outcomes and fewer resources used, including doctors’ time.
The quantitative analysis of the research carried out and presented in this article made it possible to determine whether medical facilities in Poland use Big Data Analytics and if so, in which areas. Thanks to the results obtained it was possible to formulate the following conclusions. Medical facilities are working on both structured and unstructured data, which comes from databases, transactions, unstructured content of emails and documents, devices and sensors. According to analytics, they reach for analytics in the administrative and business, as well as in the clinical area. It clearly showed that the decisions made are largely data-driven. The results of the study confirm what has been analyzed in the literature. Medical facilities are moving towards data-based healthcare and its benefits.
In conclusion, Big Data Analytics has the potential for positive impact and global implications in healthcare. Future research on the use of Big Data in medical facilities will concern the definition of strategies adopted by medical facilities to promote and implement such solutions, as well as the benefits they gain from the use of Big Data analysis and how the perspectives in this area are seen.
Practical implications
This work sought to narrow the gap that exists in analyzing the possibility of using Big Data Analytics in healthcare. Showing how medical facilities in Poland are doing in this respect is an element that is part of global research carried out in this area, including [ 29 , 32 , 60 ].
Limitations and future directions
The research described in this article does not fully exhaust the questions related to the use of Big Data Analytics in Polish healthcare facilities. Only some of the dimensions characterizing the use of data by medical facilities in Poland have been examined. In order to get the full picture, it would be necessary to examine the results of using structured and unstructured data analytics in healthcare. Future research may examine the benefits that medical institutions achieve as a result of the analysis of structured and unstructured data in the clinical and management areas and what limitations they encounter in these areas. For this purpose, it is planned to conduct in-depth interviews with chosen medical facilities in Poland. These facilities could give additional data for empirical analyses based more on their suggestions. Further research should also include medical institutions from beyond the borders of Poland, enabling international comparative analyses.
Future research in the healthcare field has virtually endless possibilities. These regard the use of Big Data Analytics to diagnose specific conditions [ 47 , 66 , 69 , 76 ], propose an approach that can be used in other healthcare applications and create mechanisms to identify “patients like me” [ 75 , 80 ]. Big Data Analytics could also be used for studies related to the spread of pandemics, the efficacy of covid treatment [ 18 , 79 ], or psychology and psychiatry studies, e.g. emotion recognition [ 35 ].
Acknowledgements
We would like to thank those who have touched our science paths.
Authors’ contributions
KB proposed the concept of research and its design. The manuscript was prepared by KB with the consultation of AŚ. AŚ reviewed the manuscript for getting its fine shape. KB prepared the manuscript in the contexts such as definition of intellectual content, literature search, data acquisition, data analysis, and so on. AŚ obtained research funding. Both authors read and approved the final manuscript.
This research was fully funded as statutory activity—subsidy of Ministry of Science and Higher Education granted for Technical University of Czestochowa on maintaining research potential in 2018. Research Number: BS/PB–622/3020/2014/P. Publication fee for the paper was financed by the University of Economics in Katowice.
Availability of data and materials
Declarations.
Not applicable.
The author declares no conflict of interest.
Publisher's Note
Springer Nature remains neutral with regard to jurisdictional claims in published maps and institutional affiliations.
Contributor Information
Kornelia Batko, Email: [email protected] .
Andrzej Ślęzak, Email: moc.liamg@25kazelsa .

- News Releases
A third of China’s urban population at risk of city sinking, new satellite data shows
University of East Anglia
Land subsidence is overlooked as a hazard in cities, according to scientists from the University of East Anglia (UEA) and Virginia Tech.
Writing in the journal Science, Prof Robert Nicholls of the Tyndall Centre for Climate Change Research at UEA and Prof Manoochehr Shirzaei of Virginia Tech and United Nations University for Water, Environment and Health, Ontario, highlight the importance of a new research paper analysing satellite data that accurately and consistently maps land movement across China.
While they say in their comment article that consistently measuring subsidence is a great achievement, they argue it is only the start of finding solutions. Predicting future subsidence requires models that consider all drivers, including human activities and climate change, and how they might change with time.
The research paper, published in the same issue, considers 82 cities with a collective population of nearly 700 million people. The results show that 45% of the urban areas that were analysed are sinking, with 16% falling at a rate of 10mm a year or more.
Nationally, roughly 270 million urban residents are estimated to be affected, with nearly 70 million experiencing rapid subsidence of 10mm a year or more. Hotspots include Beijing and Tianjin.
Coastal cities such as Tianjin are especially affected as sinking land reinforces climate change and sea-level rise. The sinking of sea defences is one reason why Hurricane Katrina’s flooding brought such devastation and death-toll to New Orleans in 2005.
Shanghai – China’s biggest city – has subsided up to 3m over the past century and continues to subside today. When subsidence is combined with sea-level rise, the urban area in China below sea level could triple in size by 2120, affecting 55 to 128 million residents. This could be catastrophic without a strong societal response.
"Subsidence jeopardises the structural integrity of buildings and critical infrastructure and exacerbates the impacts of climate change in terms of flooding, particularly in coastal cities where it reinforces sea-level rise," said Prof Nicholls, who was not involved in the study, but whose research focusses on sea-level rise, coastal erosion and flooding, and how communities can adapt to these changes.
The subsidence is mainly caused by human action in the cities. Groundwater withdrawal, that lowers the water table is considered the most important driver of subsidence, combined with geology and weight of buildings.
In Osaka and Tokyo, groundwater withdrawal was stopped in the 1970s and city subsidence has ceased or greatly reduced showing this is an effective mitigation strategy. Traffic vibration and tunnelling is potentially also a local contributing factor - Beijing has sinking of 45mm a year near subways and highways. Natural upward or downward land movement also occurs but is generally much smaller than human induced changes.
While human-induced subsidence was known in China before this study, Profs Nicholls and Shirzaei say these new results reinforce the need for a national response. This problem happens in susceptible cities outside China and is a widespread problem across the world.
They call for the research community to move from measurement to understanding implications and supporting responses. The new satellite measurements are delivering new detailed subsidence data but the methods to use this information to work with city planners to address these problems needs much more development. Affected coastal cities in China and more widely need particular attention.
“Many cities and areas worldwide are developing strategies for managing the risks of climate change and sea-level rise,” said Prof Nicholls. “We need to learn from this experience to also address the threat of subsidence which is more common than currently recognised.”
10.1126/science.ado9986
Method of Research
Commentary/editorial
Article Title
Earth’s sinking surface: China’s major cities show considerable subsidence from human activities
Article Publication Date
18-Apr-2024
Disclaimer: AAAS and EurekAlert! are not responsible for the accuracy of news releases posted to EurekAlert! by contributing institutions or for the use of any information through the EurekAlert system.
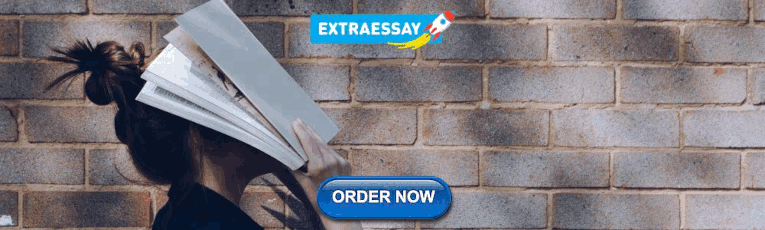
IMAGES
VIDEO
COMMENTS
Introduction. The evolution in the digital era has led to the confluence of healthcare and technology resulting in the emergence of newer data-related applications [].Due to the voluminous amounts of clinical data generated from the health care sector like the Electronic Health Records (EHR) of patients, prescriptions, clinical reports, information about the purchase of medicines, medical ...
Data science is an interdisciplinary field that extracts knowledge and insights from many structural and unstructured data, using scientific methods, data mining techniques, machine-learning algorithms, and big data. The healthcare industry generates large datasets of useful information on patient demography, treatment plans, results of medical examinations, insurance, etc. The data collected ...
Abstract. Data science is an interdisciplinary field that applies numerous techniques, such as machine learning (ML), neural networks (NN) and artificial intelligence (AI), to create value, based ...
Data science is an interdisciplinary field that applies numerous techniques, such as machine learning (ML), neural networks (NN) and artificial intelligence (AI), to create value, based on extracting knowledge and insights from available 'big' data [].The recent advances in data science and AI have had a major impact on healthcare already, as can be seen in the recent biomedical literature [].
Data science is an interdisciplinary subject that uses scientific methodologies, data mining techniques, machine-learning algorithms, and large amounts of data to extract information and insights.
1. Introduction. The use of data science methodologies in medicine and public health has been enabled by the wide availability of big data of human mobility, contact tracing, medical imaging, virology, drug screening, bioinformatics, electronic health records and scientific literature along with the ever-growing computing power [1-4].With these advances, the huge passion of researchers and ...
In this context, the recent use of Data Science technologies for healthcare is providing mutual benefits to both patients and medical professionals, improving prevention and treatment for several kinds of diseases. ... Web: ESWC 2018 Satellite Events - ESWC 2018 Satellite Events, Heraklion, Crete, June 3-7, 2018. Revised Selected Papers, pp ...
Environmental Research and Public Health Editorial Data Science in Healthcare: COVID-19 and Beyond Tim Hulsen Department of Hospital Services & Informatics, Philips Research, 5656AE Eindhoven, The Netherlands; [email protected] Data science is an interdisciplinary field that applies numerous techniques, such as
data science, and practice modules created on standardized clinical, genomic, and basic science data sets. Understanding data heterogeneity (accuracy and formatting), data fragmen-tation (multiple databases and multiple stakeholders), data (Circ Cardiovasc Qual Outcomes. 2016;9:683-687. DOI: 10.1161/CIRCOUTCOMES.116.003081.)
using (Big) Data Science technologies in the healthcare sector, including several recommendations: † Breaking down data silos in healthcare. Access to high-quality, large health-care datasets will optimize care processes, disease diagnosis, personalized care and in general the healthcare system. Furthermore, true transformation of the
Data science is an interdisciplinary field that extracts knowledge and insights from many structural and unstructured data, using scientific methods, data mining techniques, machine-learning algorithms, and big data. ... The role of data science in healthcare advancements: applications, benefits, and future prospects Ir J Med Sci. 2022 Aug;191 ...
Data Science in Healthcare: a Systematic Review. Sihem Ben Sassi a, , Nacim Y anes a, RIADI Laboratory, National School of Computer Sciences, La Manouba University, La Manoub a, 2010, Tunisia ...
2. Literature Review of Healthcare Data. Multiple forms of healthcare data include biomedical signals, genomic data, sensing data, biomedical images, and social media [].Genomic data analysis lets someone realize more about genetic markers, disease condition, consanguinity, and mutations; clinical text mining converts data from practical medical notes from disorganized format to applicable ...
The introduction of Big Data Analytics (BDA) in healthcare will allow to use new technologies both in treatment of patients and health management. The paper aims at analyzing the possibilities of using Big Data Analytics in healthcare. The research is based on a critical analysis of the literature, as well as the presentation of selected results of direct research on the use of Big Data ...
Problems in health data accumulation. Prior research observed several issues related to big data accumulated in healthcare, such as data quality (Sabharwal, Gupta, and Thirunavukkarasu Citation 2016) and data quantity (Gopal et al. Citation 2019). However, there is a lack of research into the types of problems that may occur during data ...
The use of Big Data in healthcare poses new ethical and legal challenges because of the personal nature of the information enclosed. Ethical and legal challenges include the risk to compromise privacy, personal autonomy, as well as effects on public demand for transparency, trust and fairness while using Big Data. 16.
Summary form only given, as follows. The complete presentation was not made available for publication as part of the conference proceedings. In recent times, artificial intelligence and data analysis approaches are gaining special attention in the healthcare context. Innovative methods in this direction are being introduced as a problem solver for different healthcare targets, including ...
Key Words: Data Science, Algorithms, Applic ations in healthcare. SAMRIDDHI : A Journal of Phys ical Sci ences, E ngineering and T echnology, (2021); DOI : 10.1809 /samriddhi.v13iS1.14
Location: Irving, Texas Pieces is a cloud-based software company that collects data throughout the entire patient journey to improve both the quality and cost of care. The company's flagship product, Pieces Decision Sciences, is a clinical engine that makes decisions and recommendations based on a variety of data such as lab results, vitals, and structured and unstructured data.
The contribution made in this paper provides a new research path to the data science methodologies in smart healthcare, as opposed to the existing review articles. Recently, medical imaging investigation used deep learning (DL), as it defeats the limitations of the visual evaluation and the conventional machine learning methods for smart ...
Search 217,797,568 papers from all fields of science. Search. Sign In Create ... This paper summarizes the recent promising applications of AI and big data in medical health and electronic health, which have potentially added value to diagnosis and patient care. ... Semantic Scholar is a free, AI-powered research tool for scientific literature ...
This paper conducted a bibliometric analysis of personality and safety behavior research in the Web of Science database during the period of 2000-2023. A total of 1972 publications were screened from the Web of Science database, which covered 9992 authors, 867 journals, 114 countries/regions, and 3141 organizations. The annual growth trend and distribution of subject categories were analyzed ...
CSCI 5980 Cloud ComputingMeeting Time: 09:45 AM‑11:00 AM TTh Instructor: Ali AnwarCourse Description: Cloud computing serves many large-scale applications ranging from search engines like Google to social networking websites like Facebook to online stores like Amazon. More recently, cloud computing has emerged as an essential technology to enable emerging fields such as Artificial ...
Introduction. We are living in the age of "data science and advanced analytics", where almost everything in our daily lives is digitally recorded as data [].Thus the current electronic world is a wealth of various kinds of data, such as business data, financial data, healthcare data, multimedia data, internet of things (IoT) data, cybersecurity data, social media data, etc [].
Largest global citizen science project accelerates knowledge of human microbiome Leveraging gamers and video game technology can dramatically boost scientific research according to a new study published today in Nature Biotechnology. 4.5 million gamers around the world have advanced medical science by helping to reconstruct microbial evolutionary histories using a minigame included inside the ...
Abstract. This book seeks to promote the exploitation of data science in healthcare systems. The focus is on advancing the automated analytical methods used to extract new knowledge from data for ...
The research paper, published in the same issue, considers 82 cities with a collective population of nearly 700 million people. The results show that 45% of the urban areas that were analyzed are ...
Big Data analytics can improve patient outcomes, advance and personalize care, improve provider relation ships with. patients, and reduce medical sp ending. This paper introduces he althcare data ...
The paper aims at analyzing the possibilities of using Big Data Analytics in healthcare. The research is based on a critical analysis of the literature, as well as the presentation of selected results of direct research on the use of Big Data Analytics in medical facilities. ... For the purpose of this paper, the following research hypotheses ...
Land subsidence is overlooked as a hazard in cities, according to scientists from the University of East Anglia (UEA) and Virginia Tech. Writing in the journal Science, Prof Robert Nicholls of the ...