Fast food in the diet: Implications and solutions for families
Affiliation.
- 1 School of Nursing, University of Minnesota, 308 Harvard Street SE, Minneapolis, MN 55455, USA. Electronic address: [email protected].
- PMID: 29630965
- DOI: 10.1016/j.physbeh.2018.04.005
Fast food is omnipresent in the United States (U.S.) and contributes to poor dietary quality and poor health among youth and adults alike. Children need adults to teach them good eating habits to attain and maintain good health by introducing them to healthful foods and being good role models. The fast food industry, through vast funds and advertising, contribute to challenges parents face to provide healthful foods for their families and thwart our best efforts to meet health goals. Research shows fast food consumption is influenced by lack of cooking confidence, time pressures, and perceptions of ease and convenience. We need practical strategies to help parents and children make healthier food choices. As a product of conference proceedings, this paper provides a non-exhaustive narrative summary of the fast food marketplace and marketing, the contributions of fast food to diet and health, struggles with healthful eating among families, and possible solutions of how we can help children and parents empower themselves to have healthier lives.
Keywords: Cooking; Eating; Families; Fast-food; Health; Intervention.
Copyright © 2018. Published by Elsevier Inc.
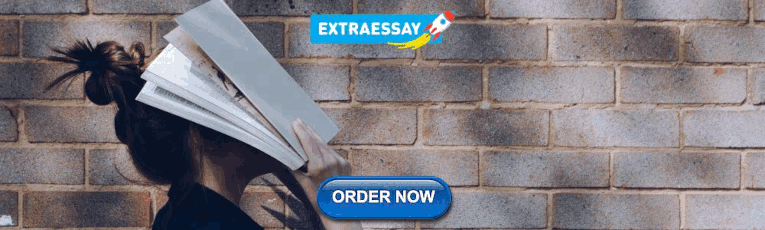
Publication types
- Research Support, N.I.H., Extramural
- Fast Foods* / economics
- Feeding Behavior*
- Health Promotion*
- Healthy Lifestyle
Grants and funding
- R01 DK084000/DK/NIDDK NIH HHS/United States
Fast food in the diet: Implications and solutions for families
- Academics (Nursing)
Research output : Contribution to journal › Article › peer-review
Fast food is omnipresent in the United States (U.S.) and contributes to poor dietary quality and poor health among youth and adults alike. Children need adults to teach them good eating habits to attain and maintain good health by introducing them to healthful foods and being good role models. The fast food industry, through vast funds and advertising, contribute to challenges parents face to provide healthful foods for their families and thwart our best efforts to meet health goals. Research shows fast food consumption is influenced by lack of cooking confidence, time pressures, and perceptions of ease and convenience. We need practical strategies to help parents and children make healthier food choices. As a product of conference proceedings, this paper provides a non-exhaustive narrative summary of the fast food marketplace and marketing, the contributions of fast food to diet and health, struggles with healthful eating among families, and possible solutions of how we can help children and parents empower themselves to have healthier lives.
Bibliographical note
- Intervention
Publisher link
- 10.1016/j.physbeh.2018.04.005
Other files and links
- Link to publication in Scopus
- Link to the citations in Scopus
Fingerprint
- Fast Food Keyphrases 100%
- Healthful Foods Keyphrases 50%
- United States Keyphrases 25%
- Healthy Food Choice Keyphrases 25%
- Dietary Quality Keyphrases 25%
- Healthy Life Keyphrases 25%
- Conference Proceedings Keyphrases 25%
- Health Goals Keyphrases 25%
T1 - Fast food in the diet
T2 - Implications and solutions for families
AU - Fulkerson, Jayne A.
N1 - Publisher Copyright: © 2018
PY - 2018/9/1
Y1 - 2018/9/1
N2 - Fast food is omnipresent in the United States (U.S.) and contributes to poor dietary quality and poor health among youth and adults alike. Children need adults to teach them good eating habits to attain and maintain good health by introducing them to healthful foods and being good role models. The fast food industry, through vast funds and advertising, contribute to challenges parents face to provide healthful foods for their families and thwart our best efforts to meet health goals. Research shows fast food consumption is influenced by lack of cooking confidence, time pressures, and perceptions of ease and convenience. We need practical strategies to help parents and children make healthier food choices. As a product of conference proceedings, this paper provides a non-exhaustive narrative summary of the fast food marketplace and marketing, the contributions of fast food to diet and health, struggles with healthful eating among families, and possible solutions of how we can help children and parents empower themselves to have healthier lives.
AB - Fast food is omnipresent in the United States (U.S.) and contributes to poor dietary quality and poor health among youth and adults alike. Children need adults to teach them good eating habits to attain and maintain good health by introducing them to healthful foods and being good role models. The fast food industry, through vast funds and advertising, contribute to challenges parents face to provide healthful foods for their families and thwart our best efforts to meet health goals. Research shows fast food consumption is influenced by lack of cooking confidence, time pressures, and perceptions of ease and convenience. We need practical strategies to help parents and children make healthier food choices. As a product of conference proceedings, this paper provides a non-exhaustive narrative summary of the fast food marketplace and marketing, the contributions of fast food to diet and health, struggles with healthful eating among families, and possible solutions of how we can help children and parents empower themselves to have healthier lives.
KW - Cooking
KW - Eating
KW - Families
KW - Fast-food
KW - Health
KW - Intervention
UR - http://www.scopus.com/inward/record.url?scp=85045717537&partnerID=8YFLogxK
UR - http://www.scopus.com/inward/citedby.url?scp=85045717537&partnerID=8YFLogxK
U2 - 10.1016/j.physbeh.2018.04.005
DO - 10.1016/j.physbeh.2018.04.005
M3 - Article
C2 - 29630965
AN - SCOPUS:85045717537
SN - 0031-9384
JO - Physiology and Behavior
JF - Physiology and Behavior

An official website of the United States government
The .gov means it’s official. Federal government websites often end in .gov or .mil. Before sharing sensitive information, make sure you’re on a federal government site.
The site is secure. The https:// ensures that you are connecting to the official website and that any information you provide is encrypted and transmitted securely.
- Publications
- Account settings
Preview improvements coming to the PMC website in October 2024. Learn More or Try it out now .
- Advanced Search
- Journal List
- BMC Med Inform Decis Mak
- v.21(Suppl 7); 2021

The ontology of fast food facts: conceptualization of nutritional fast food data for consumers and semantic web applications
Muhammad amith.
1 School of Biomedical Informatics, The University of Texas Health Science Center at Houston, 7000 Fannin St, Suite 600, Houston, TX 77030 USA
Chidinma Onye
2 Surveillance and Epidemiology Unit, Office of Surveillance, Science, and Technology, Harris County Public Health, 2223 West Loop South, Houston, TX 77027 USA
Tracey Ledoux
3 Department of Health and Human Performance, University of Houston, 3875 Holman St. Rm 104 Garrison, Houston, TX 77204 USA
Grace Xiong
4 Department of Neuroscience, University of Texas, 110 Inner Campus Drive, Austin, TX 78705 USA
Associated Data
The ontology of fast food facts generated during the current study are available in the Github repository, https://github.com/UTHealth-Ontology/OFFF .
Fast food with its abundance and availability to consumers may have health consequences due to the high calorie intake which is a major contributor to life threatening diseases. Providing nutritional information has some impact on consumer decisions to self regulate and promote healthier diets, and thus, government regulations have mandated the publishing of nutritional content to assist consumers, including for fast food. However, fast food nutritional information is fragmented, and we realize a benefit to collate nutritional data to synthesize knowledge for individuals.
We developed the ontology of fast food facts as an opportunity to standardize knowledge of fast food and link nutritional data that could be analyzed and aggregated for the information needs of consumers and experts. The ontology is based on metadata from 21 fast food establishment nutritional resources and authored in OWL2 using Protégé.
Three evaluators reviewed the logical structure of the ontology through natural language translation of the axioms. While there is majority agreement (76.1% pairwise agreement) of the veracity of the ontology, we identified 103 out of the 430 statements that were erroneous. We revised the ontology and publicably published the initial release of the ontology. The ontology has 413 classes, 21 object properties, 13 data properties, and 494 logical axioms.
With the initial release of the ontology of fast food facts we discuss some future visions with the continued evolution of this knowledge base, and the challenges we plan to address, like the management and publication of voluminous amount of semantically linked fast food nutritional data.
Fast food or “limited service” restaurants provide foods that are mass-produced and served quickly. These establishments allow for food and drink to be consumed on-premises, taken out, or delivered to the customer, and offer a wide selection of food choices. Healthier menu offerings include salads and lean grilled meats yet pizza, hamburgers, and fries remain the most commonly purchased items. Over the past 3 decades, Americans have increased their intake of foods prepared outside the home and currently, 36% of US adults eat at fast-food restaurants each day [ 1 ]. These rates are similar in children with one-third of US children consuming fast food each day [ 2 ].
Fast foods are popular among people of most age groups due to their low cost, consistency, and convenience [ 3 ]. The majority of research shows that fast food consumption is linked to excess weight gain, poor diet quality, and mortality. Recent research suggests that improvements in nutrition labeling have resulted in the availability of healthier items for consumers to choose from [ 4 ].
The following literature review examines the effect of fast food on individual health and the impact of nutrition labeling on consumer health outcomes.
Impact of fast food on health
Food is essential for every human being and the quality of the food consumed has a significant impact on an individual’s health. Diet quality is based on adherence to national nutritional recommendations and dietary guidelines that promote health, meet nutrient needs, and prevent disease. Certain types of fast foods can lead to excess weight gain, where a change in weight status occurs that causes an individual to be categorized as overweight or obese according to body mass index. Excess weight gain puts people at risk for developing diseases and conditions that increase one’s likelihood of death or mortality due to cardiovascular diseases. Some research suggests that living in areas that are densely populated with fast food restaurants can impact individual health due to the increased accessibility of fast food for consumption, and also position the motivation for the development of a consumer-centric ontology of fast food nutritional information.
Excess weight gain
The overall results of the studies indicate that there is an association between the consumption of fast-food and excess weight gain. A prospective cohort study conducted by [ 2 ] among 541 pre-school-age children found that weight status increased in children who consumed fast food more frequently during the week.
Of the studies conducted among adults Bhutani and colleagues [ 5 ] reported a significant positive relationship between the frequency of eating at fast-food restaurants and increased BMI among 1,418 individuals in a cross-sectional study.
Fast food density
A systematic review examined 31 articles to look at the relationship between retail food establishments around schools and the occurrence of overweight and obesity in school aged children [ 6 ]. Fourteen studies observed a direct association between proximity or density and excess weight.
Similarly, a systematic review conducted by William et al. [ 7 ] examined 20 articles to observe the associations between the retail food environment and body weight. 72 associations were observed with 43 showing a positive relationship. Nineteen of the positive relationships were significant. The authors concluded that there was some evidence of the retail food environment having an effect on children’s bodyweight.
Mazidi et al. [ 8 ] conducted a cross-sectional study that evaluated the association between the neighborhood density of fast-food restaurants and obesity prevalence among neighborhood residents. The authors initially observed a negative association which they attributed to the confounding variables of affluence and education. Once the co-founding variables were removed there was no association found between the density of fast-food restaurants and obesity prevalence.
An ecological analysis conducted aimed to examine the relationship between fast food density and the prevalence of type 2 diabetes among counties in South Carolina [ 9 ]. The author’s found a significant negative association between fast-food restaurant density and prevalence of type 2 diabetes. The authors found these results to be unexpected and cited individual behavioral decisions as affecting the prevalence of type 2 diabetes.
Another cross-sectional study explored whether an association existed between mortality from stroke or cardiovascular disease and fast-food density in the United States [ 10 ]. The authors found that increased fast-food density was associated with an increased risk of death from both stroke and cardiovascular disease along with an increased prevalence of type 2 diabetes. While it was concluded that an association existed it was also determined that the impact of opening a new fast-food restaurant was exceedingly small.
Despite the large number of studies, the findings are mixed and there is limited evidence to suggest that a relationship exists between the food environment and individual health.
Diet quality
The evaluation conducted by Barnes and associates examined the association between fast-food consumption and diet quality among working adults [ 11 ]. The authors’ determined that there was a significant inverse association between the frequency of fast-food consumption and diet quality.
A cross-sectional study conducted by Vercammen and colleagues examined 1479 combination meals offered by 34 US fast-food and fast-casual restaurant chains [ 1 ]. Three options were examined for each combination meal (1) default (2) low-calorie option and (3) high-calorie option. The meals were found to be high in sodium, calories, sugar, and saturated fat. The authors concluded that nearly all the combinations exceeded the daily recommended limits for calories and sodium.
Todd et al. [ 4 ] found that in 2013–2014 working adults had greatly increased the amount of fast food they were consuming and yet were experiencing a decrease in their intake of saturated fat and cholesterol by significant amounts. The authors believe these decreases in saturated fat and cholesterol despite higher intake of fast food may suggest an improvement in the quality of fast foods. The authors attributed the improvements to regulations regarding menu labeling beginning in 2008. This may indicate that menu labeling can lead to improvements in the quality of fast food. The authors offer that menu labeling improves consumers’ ability to recognize low-energy food items and suggest that this may compel restaurants to reformulate their menu items by lowering their energy content [ 4 ].
In a prospective cohort study conducted among 69,582 adults increased fast-food consumption was associated with mortality [ 12 ]. It was also observed that there was an association between increased fast-food consumption and cardiovascular disease-specific mortality.
Impact of nutritional information on health outcomes
Access to nutritional information impacts the way people manage their health through diet. Individuals with chronic diseases can monitor their intake of nutrients such as sodium and sugar to slow the progression of disease, while those without chronic illnesses can use nutritional information for disease prevention. Nutritional information also influences decisions regarding what foods people choose to buy and eat.
Food management
A prospective cohort study conducted by Amuta-Jiminez et al. [ 13 ] observed a relationship between healthy dietary behaviors and the use of food labels among adults diagnosed with cancer. The findings in the study suggest that individuals that used food labels were more likely to engage in health eating behaviors such as consuming more fruits and vegetables and consuming fewer sodas. The findings were significant for all 3 dietary behaviors. A cross-sectional study by Byrd et al. [ 14 ] found that consumers that reported taking action to reduce their sodium intake are more likely to use menu nutrition information compared to consumers that reported taking no action to decrease sodium intake.
A randomized controlled trial performed by Kollannoor-Samuel et al. [ 15 ] found that the use of food labels leads to improved diet quality and improvements in blood glucose control among adults with type 2 diabetes. The findings were statistically significant at the between-individual level.
A cross-sectional study among 1817 adults conducted by Christoph et al. [ 16 ] found that the use of nutritional information was associated with both healthy and unhealthy behaviors involving weight control. The authors also found that these nutrition facts were positively associated with binge eating in women and negatively associated with intuitive eating in men. Christoph et al. [ 16 ] suggest that men who use external cues such as nutritional information when choosing food may be less likely to pay attention to internal cues while consuming food. Contrastingly, women may rely on both or neither of these cues when choosing or consuming food. The authors touch on earlier evidence indicating that individuals with eating disorders and weight concerns may be notably influenced by exposure to menu labeling.
Food choices
Byrd et al. [ 17 ] observed that adding calorie information along with the numeric sodium content of meals to menus resulted in both beneficial and detrimental outcomes. The authors found that consumers who perceived lower calorie and lower sodium foods as tasty were more likely to choose a meal lower in sodium than those who perceive higher calorie and sodium foods as tasty.
Kollannoor-Samuel et al. [ 18 ] conducted a prospective cohort study with 12,686 youths and young adults. The authors hypothesized that the use of nutritional labels would be associated with a decreased risk of diabetes in young adults who were not reported to have diabetes at baseline. The authors found evidence that suggested the use of nutrition labels was associated with a lower long-term risk of diabetes.
A randomized controlled trial conducted by Musicus et al. [ 19 ] found that red stop sign and traffic light warning labels were associated with a significant decrease in the amount of sodium ordered. The authors also found that the use of warning labels increased knowledge about sodium content and increased abilities to distinguish between high and low sodium meals even without labels.
Review summary Due to convenience and availability, more Americans are relying on fast food as a part of their regular diet. Despite the fact that nearly all fast-food restaurants have health promoting items on their menus (e.g., salad), the most commonly purchased items contain excessive amounts of saturated fat, sugar, and sodium. Frequent consumption of fast food has been related to excess weight gain, poor diet quality, and increased mortality risks. However, some research suggests tools such as nutritional labels may empower consumers to make healthier fast-food choices.
While fast food restaurants have incorporated healthier menu items in recent years, studies have shown that there is a link between fast food consumption and poor diet quality. This is attributed to the high levels of sodium, sugar, calories, and saturated fat within menu items. In addition, this poor diet quality associated with fast food consumption is linked to excess weight gain as well as increased mortality risks related to cardiovascular disease.
Ontologies and big data
Noted earlier nutritional labels, or more generally nutritional information and facts, that can be presented to the health consumers is one factor that can be integral to their decisions to make healthy food decisions. Given the amount of fast food establishments, in addition to non-fast food restaurants, health consumers have a variety of options to potentially decide on nutritional and diet choices. This is also compounded with the amount of choices that fast food restaurants have, emerging new fast food restaurant chains and independent venues ( e.g ., “food trucks”).
We focus this work on how to handle the heterogeneous and volume of fast food nutritional data, and methods to collate the vast amount of evolving data to be available and query-able. Fundamentally, this is a Big Data topic that shares some features of Big Data ( velocity , volume , and variety ). We expect due to the market demand that fast food items (along with the nutritional information) will change and also increase with more choices from the individual restaurants and from emerging establishments ( velocity and volume features of Big Data). In addition, We assume that with limited amount of nutritional information presented, if the nutritional data was linked to other external extended data sources, the amount of data would predictably increase further. For many restaurants, the nutritional information is presented in varying formats—static and dynamic websites, PDF downloads, siloed websites, etc., and there also regional menu options to accommodate a segment of the world population, but with no accessible solution to aggregate the information for analysis and decision making ( variety in Big Data).
In this paper we propose the use of an ontology that can facilitate linked data of nutritional information, and provide methods to query the data across the heterogeneous fast food nutritional sources. Ontologies are software artifacts referred to as “formal, explicit specialization of a shared conceptualization” [ 20 ]. In a recent article, Hitzler elaborates that “an ontology is really a knowledge base (in the sense of symbolic artificial intelligence) of concepts (that is, types of classes, such as ’mammals’ and ’live birth’) and their relationships (such as, ’mammals give live birth’), specified in a knowledge representation language based on formal logic” [ 21 ].
Simply, ontologies utilize symbolic terminologies (e.g. “mammals” and “live birth”) to represent concepts (unit of thought) and first order predicate logic (e.g. “give”) to imbue consistent meaning between concepts. Abstractly this generates a network graph of domain information from the relational links between the concepts. Semantic technology like OWL2 and RDF support authoring of the ontologies and a machine-based syntax for machines to share and interpret standardized knowledge of a domain. Within the framework of the Big Data features, ontologies address data variety through standardizing and normalizing heterogeneous data sources and linking to other sources, velocity with flexibility to change schema to accommodate fast growth of data, and also volume through semantic web technologies like nanopublications.
We discuss the development of an ontology for fast food data and information that aims to normalize and standardize the knowledge of fast food nutritional information. We label this ontology, the ontology of fast food facts (OFFF). OFFF is based on the structure of open-sourced consumer-centric nutritional information presented on fast food websites. Aside from formalizing and having a shared conceptualization of fast food nutritional knowledge, the availability of this knowledge base contributes to future use cases that can potentially benefit health consumers and expert-class researchers and clinicians.
Related food ontologies
While our research focus is solely aimed for the fast food domain, there are a few “general” food ontologies. The most prominent is FoodOn [ 22 ], a BFO (basic formal ontology)-based ontology that has an expansive knowledge base covering various aspects of food knowledge, including the agricultural origin of individual food items. Another is a basic application ontology (Food Ontology) from the British Broadcasting Corporation’s Ontologies for food and recipes [ 23 ]. The Recipe Ontology [ 24 ] is an application ontology for a serious gaming project. This ontology models rules and information related to recipes and supports personalized player profiles. The Food Ontology Knowledge Base [ 25 ] is a basic model of food nutritional information for basic food types from the Republic of Turkey’s Ministry of Food database. The Food and Agriculture Organization of the United Nations provides a linked terminology for expert researchers that covers over 35,000 concepts called AGROVOC [ 26 ]. The Food Product Ontology [ 27 ] extends the well-known GoodRelations ontology [ 28 ] to represent concepts for food products, its pricing, and the associated business entity. Its purpose is to help companies publish and share their food product items using a formal, standard model. Open Food Facts [ 29 ] is a crowd-sourced and free terminology source of international food product that rely on volunteers, yet, it is presumed that it may be prone to potential errors due to its crowd-sourced approach and lacks any mechanism to verify the information [ 30 ]. Thailand-based Food-Oriented Ontology-Driven System for food menu items is an application ontology for a nutritional recommender system for individuals with diabetes. Similarly for recommender systems, FoodKG [ 31 ] is an big data knowledge graph that incorporates a large recipe dataset, the United States Department of Agriculture’s National Nutrient Database for Standard Reference, and the FoodOn ontology. While not a food ontology, the Ontology of Nutritional Studies [ 32 ] is another BFO-based ontology that intends to normalize terminologies of nutritional studies to advance data analysis purposes for expert researchers.
Research objective
The ontology of fast food facts is focused on the pertinent information that health consumers are concerned about, reflected in nutritional labels of fast food. In addition, we represented the knowledge gathered from basic questions that health consumers inquire to enrich the ontology further. In the later sections, we discuss how our work with this ontology will be used as a linked accessible data source, and for patient-facing tools that leverage our previous ontology-based technology for dialogue systems (“chat bots”). Lastly, more specifically, this ontology is solely focused on modeling fast food data and their corresponding nutritional information. This paper discusses the enrichment of our initial effort [ 33 ] with expanding and improving the model and further enriching the ontology to address basic information needs of the health consumer that our initial effort did not incorporate.
To initiate the design of our ontology, we analyzed consumer-centric nutritional content from fast food establishments—(McDonalds, Dairy Queen, Chick Fil-A, Wendy’s, Taco Bell, Arby’s, Blimpie, Carl’s Jr, Checkers & Rally’s, Church’s, Jack in the Box, Jollibee, Popeye’s, Raising Cane’s, White Castle, and Panda Express). Their websites provide nutritional data either as a web page or downloadable PDF. We devised the meta-data structure from the sources and identified main concepts and relationships between the concepts using the Food and Drug Administration’s standard national nutritional label as a guide [ 34 ]. This activity yielded the meta-level abstraction of the ontology (See Fig. 1 ).

Meta-level of the ontology of fast food facts showing the representation of the Nutritional Fact concept and its relationship with the Fast Food concept. Dark yellow node indicates additional subclasses
The central concept of the meta-level of this ontology is Nutritional Fact . This concept contains relationships with Nutritional Component , Ingredient , and Allergens . The meta-level of the ontology was authored using Protégé [ 35 ]. Later, we evaluated the veracity of the meta-level structure of the ontology using Hootation [ 36 ], a natural language generation software library for ontologies. Three evaluators (CO, GX, and CT) independently reviewed the translated natural language statements ( S h r e d d e d _ C h i c k e n _ B u r r i t o ⊆ B u r r i t o translated to “ every shredded chicken burrito is a burrito ”) that expresses each logical axiom encoded in the ontology. Each evaluator was given a spreadsheet with the translated axioms, and were instructed to label the statements—“Yes” whether the logical axiom is accurate, “No” if the axiom is not accurate, or “X” if the evaluator was unsure.
Nutritional component
The Nutritional Component has several subclasses alluding to various nutritional data found on food labels—protein, cholesterol, fats, minerals, etc. Essentially, Nutritional Component describes the amount of nutrients that a particular food item has. This is expressed through a data property of Amount (in milligram, gram, ounces, etc.) We also denote amounts of vitamins and minerals contained in a food item—iron, vitamin A, calcium, potassium, etc. This was limited to the vitamins and minerals that are disclosed from the online sources.
Nutritional Fact includes allergen information that is represented as Nutritional Fact → has allergens → Allergens . In many examples from the online sources, allergen content is denoted as a binary representation (i.e., yes or no if a food item has gluten, diary, etc.). The Allergens concept has several Boolean data types to flag the food item for allergen content (soy, msg, egg, diary, etc).
Ingredient information for food items is represented in the ontology with Nutritional Fact → has ingredients → Ingredient . The Ingredient class covers any component listed from the nutritional data such as bread, eggs, corn syrup, caffeine, etc. This concept also has a self-directed relationship has ingredients if certain ingredients contained other ingredients. For example, bread can contain milk and eggs ( e.g. , bread → has ingredients → milk and bread → has ingredients → egg ). Lastly, the Ingredient concept has a link to Allergens using has allergens . If a food item has milk, it can be indicated with having an allergic reaction of diary.
Fast food concept
The meta-level, described above, serves as a framework for consumer nutritional information. For fast food items, we created a class called Fast Food that subclass an entity of Food . This concept is used to describe the types of fast food in most of the fast food establishments. We also included a concept called Fast Food Restaurant (linked to Fast Food through offered by ) which is a descendant class of Agent , following how other ontologies like PROV-O (Provenance ontology) [ 37 ] and FOAF (Friend of a friend ontology) [ 38 ] represent organizations ( Agent > Organization > Business ). Based on our limited selection of fast food venues, we identified 10 basic fast food categories (See Fig. 2 )— Fast Food Sandwich , Fast Food Taco , Fast Food Chicken , Fast Food Condiments , Fast Food Salad , Fast Food Beverage , Fast Food Burrito , Fast Food Sides , Fast Food Breakfast , Fast Food Desserts , and Fast Food Asian Dish . Fast Food is linked to Nutritional Fact with the object property of has Nutritional Fact to associate fast food items with nutritional data.

Detailed Meta-level of the Ontology of Fast Food Facts showing the sub-categories for Fast Food . Dark yellow node indicates additional subclasses
Instance data model
The nutritional data items from the menus of the fast food establishments were represented as unique instances for the ontology. The aforementioned fast food establishments has nutritional facts either on the website or as a PDF download. We transferred the data from the sources to a spreadsheet for import. We used the Protégé plugin, Cellfie [ 39 ], to preform the mass import of the food data. We created a set of custom import rules for Cellfie to normalize and add the data to the ontology.
Figure 3 shows an example of a final imported data from the collected data, and Fig. 4 visualizes a sample instance data from the Ontology of Fast Food Facts. The food item instantiates the type of fast food. In the example above, Iced Coffee McDonald’s (Small) is an instance of Coffee which is a type of Beverage . This data item links to other nutritional and allergen information using hasNutritionalInfo and hasAllergens , as shown in Fig. 4 . In the example, trans fat information ( TransFat_IcedCoffeeMcDonaldsSmall ) is associated with the aforementioned iced coffee, and it also indicates the amount of trans fat. The same is with allergen information ( Allergen_IcedCoffeeMcDonaldsSmall ) denoting there is diary allergen. This instance for iced coffee has _datum appended to it, and for the nutritional information there is a corresponding prefix ( Allergen_ , TransFat_ , etc.).

Instance level of the Ontology of Fast Food Facts. Screenshot shows an example of an imported instance for an apple pie item from a major fast food company

Visualization of a sample instance from the ontology of fast food facts. Suffix and prefix are bold-faced to highlight the type of instance information
Enrichment From common nutrition questions
Consumer nutrition questions were gathered by performing Google web searches using the phrases “Frequently asked nutrition questions” and “Most common nutrition questions”. Six sources were selected, each of which listed frequently asked nutrition related questions that were answered by registered nutritionists or registered dieticians. The sources included Consumer Reports [ 40 ], The Washington Post [ 41 ], North Dakota State University [ 42 – 44 ], The U.S. Department of Agriculture [ 45 ], The European Food Information Council [ 46 ], and Harvard School of Public Health [ 47 ]. The eight sources resulted in 41 questions that were narrowed down to include 19 questions that were used to expand the ontology. Table 1 lists the final 19 questions which were related to outcomes associated with sugar, sodium, and fats.
19 Consumer questions used to expand the ontology of fast food facts
Figure 5 models the additional concepts and relationships to OFFF. This included the addition of two new concepts: Health Outcomes , and Diet Quality which were extended through the existing concept of Nutritional Component . This also included additional subclasses of the aforementioned concepts, all of which are shown in Fig. 5 . These derivations were encoded into the ontology of fast food facts using Protégé.

A concept map outlining the additional concepts derived from the consumer nutrition questions. For readability purposes, the class restriction definitions are listed
Health outcomes
The Health Outcomes concept consists of subclasses that represent various adverse health outcomes that are associated with diet quality. These outcomes include obesity, tooth decay, hypertension, type 2 diabetes, osteoporosis, overweight, heart disease, and raised cholesterol levels. Health Outcomes is linked to Nutritional Component using Influence .
The Diet Quality concept consists of subclasses that represent attributes identified as contributing to a poor quality diet—excess trans-fat, excess fat, excess sugar, and excess sodium. Each subclass is linked to a subclass of the Health Outcomes concept using the object property causedBy , raises and lowers . Figure 5 lists the class restrictions for these subclasses.
Daily value and daily value percentage
The Daily Value data property expresses the daily recommended value of a nutritional component. Nutritional Component is extended using the Daily Value data property (positive integer value). Similarly, we also included the percentage of the Daily Value ( Daily Value Percentage ) as a decimal value type.
The ontology of fast food facts (OFFF) contained 413 classes, 21 object properties, 13 data properties, and 494 logical axioms. The three evaluators independently annotated 430 natural language statements. We annotated each statement in terms of (0) whether the statement was not accurately expressed by the ontology and (1) whether the statement was accurately expressed by the ontology. Statements that elicited a response of “don’t know” were annotated as (0). The evaluators achieved substantial intercoder reliability with an average pairwise percent agreement of 76.1%. Pairwise agreement was 73% for raters 1 and 2, 84.7% for raters 2 and 3, and 70.7% for raters 1 and 3. Intercoder reliability was calculated using ReCal3 0.1 Alpha for 3+ Coders.
In total average among the raters, our evaluators assessed that the ontology accounted to 73.0% accurate number of statements (mean of 56.5%, 81.2%, and 81.2%). To our knowledge, we assume that most of the conceptual level structure is accurate. However, we reviewed the statements that had complete disagreement (all evaluators believed or were not sure the statement was accurate) or majority disagreement (two of the three evaluators believed or were not sure the statement was accurate). We accounted 103 statements in all that fit this criteria, and we reviewed the issues with these statements to assess the source of the inaccuracy of OFFF.
We noted three types of issues with OFFF’s accuracy—(1) poor labeling of the entities that could have benefited with more elaborate labels for better expression of the axiom, (2) mislabeling that expressed information that did not reflect the world (e.g., M o c h a ⊆ C o f f e e _ F a c t , “ every mocha is a coffee fact ”), and 3) logical errors and possible confusion and contention where the issue was not the label but an issue with association of the statement that may have led to confusion or misunderstanding (e.g., D Q _ T r e a t z z a _ P i z z a ⊆ C a k e , “ every dqtreatzza pizza is a cake ”). For the third case, there were 24 statements that fit that category. For the first and second case, there were 48 and 31 statements respectively.
We revised the ontology based on these majority disagreements of the veracity. From our preliminary work, the model of our ontology represented polyhierarchical of fast food facts instead of representing them as entities of food. We oriented the ontology model to reflect a sound organization of fast food, and this is reflected in the design we discussed earlier. This addressed most of the second issue of mislabeling. With issues revolving around more elaboration of the labels, we added more expressive labels by adding terms, like modifying T a c o ⊆ S a l a d (“ every taco is a salad ”) to T a c o _ S a l a d ⊆ S a l a d ( ≈ “ every taco salad is a salad ”). For the third issue there were a combination of authoring errors, like duplicate yet erroneous statements, and misunderstanding of the accuracy of the statement. For authoring errors, we had a statement like M u s h r o o m _ S w i s s _ B u r g e r ⊆ M u s h r o o m (“ every mushroom swiss burger is a mushroom ”), which were later deleted due to being a duplicate and an error. There were statements that relied on particular knowledge of the food item from a certain restaurants (like Dairy Queen and Whataburger) that used their own nomenclature (e.g., A p p l e _ B i t e s ⊆ A p p l e _ S l i c e s or H a s h _ B r o w n _ S t i c k s _ W h a t a b u r g e r s ). Also for the third issue, there were statements that might have been accurate depending on prior understanding the definition of the concepts. For example, OFFF has H o t _ D o g as a type of F a s t _ F o o d _ S a n d w i c h —if one were to understand that a sandwich is a breaded food item with some type of non-bread filling. Another example is Pretzel or O a t m e a l _ B a r as type of F a s t _ F o o d _ S i d e may be true if there was prior knowledge that these items were available as side item with the entree. All in all, we removed any specific concepts that were vendor-specific nomenclature to ensure normalization and accuracy of the OFFF. The latest version of this ontology is available at the git repository link, https://github.com/UTHealth-Ontology/OFFF.
We developed an ontology called the ontology of fast food facts (OFFF) that models nutritional data of fast food items. This initial work was seeded from nutritional sources published from McDonald’s, Dairy Queen, Chick Fil-A, Wendy’s, Taco Bell, McDonalds, Dairy Queen, Chick Fil-A, Wendy’s, Taco Bell, Arby’s, Blimpie, Carl’s Jr, Checkers & Rally’s, Church’s, Jack in the Box, Jollibee, Popeye’s, Raising Cane’s, White Castle, and Panda Express. With these sources we were able to model the meta-level abstraction that expresses a model of nutritional data and fast food, and then later import the source data onto the conceptual meta-level structure of the ontology with reusable Cellfie scripts. We evaluated the accuracy of the nutritional knowledge in the ontology, and determined if the ontology fulfills the requirements of the competency questions. Most of the knowledge of the nutritional data were accurate, but only about three-fourths of the competency questions were answered with “Yes”.
While the ontology is derived from numerous open-sourced resources from fast food establishments, the ontology is still relatively broad and may need further elaboration of concepts. For example, S o f t _ D r i n k s and Hamburger concepts will need additional specific sub-concepts and perhaps class-level restriction definitions to describe “creative” fast food items. Another example is B r e a k f a s t _ P l a t t e r which is likely to include portions of other fast food items, which signals that this class and others like it will need to be expanded to be more descriptive. There is also unconventional fast food venues, like “food trucks” and emerging multi-ethnic fusion venues, that may have unique or ethnic offerings that could challenge the model of the Ontology of Fast Food Facts. We also do not account for regional offerings (e.g., McDonald’s in Japan) that have items that could expand the model further. We foresee this ontology to further evolve with the next few iterations towards a standard ontology model of fast food that can link nutritional data for analysis and decision making.
A noted feature of ontologies is interoperability, to link and extend data sources. There exist certain concepts that enable OFFF to be link to other ontologies. For example, in Fig. 6 we have one of the Ingredients concept, “high fructose corn syrup ingredient” is linked as an equivalent class to the RxNORM’s [ 48 , 49 ] version of “high fructose corn syrup” to show how an ingredient of a fast food data item (like a soft drink) can be extended with existing knowledge bases of other ontologies. Furthermore assuming RxNORM is extended and linked to other ontologies it can further elaborate more meaning to a fast food ingredient leading to possible analytical and research possibilities for clinical informatics studies. In addition there is an opportunity in linking to biomedical ontologies with the chemical nutritional entities of OFFF that could enhance future analytical endeavors. A major food ontology, the FoodOn ontology [ 22 ], aims to be a comprehensive, realist model of food information ranging from processing of food, distribution, packaging, cooking processes, physical attributes, etc. FoodOn is an ontology for expert level research on food and agriculture. We foresee the possibility of utilizing OFFF to link to FoodOn for traceability analysis for fast food information and also possibly extend the scope of OFFF.

An example displaying concepts from the Ontology of Fast Food Facts linking to other ontologies like RxNORM
Our challenge we have encountered is the management of imported data. Currently, we have collected nutritional data from 21 sources. The import process toward a merged ontology with the data was predicted to be large and unwieldy based on imports of six of the 21 dataset sources. Our upcoming goal with this work is to push most of the data for public accessibility and consumption. One potential possibility we plan to investigate is to publish nutritional data as nanopublication named graphs and have the data as a persistent store on peer-to-peer network.
The vision of the Semantic Web entails data linked across servers of the World Wide Web to provide meaningful description of data [ 50 ]. Portions of the web, like the Open Linked Data Cloud, are early realizations of the Semantic Web vision. Nanopublications builds upon on the vision of the Semantic Web but with a focus on linked scientific assertions over a peer-to-peer decentralized network. A nanopublication is “defined as a small data container consisting of three parts: an assertion part containing the main content in the form of an atomic piece of formally represented data (e.g., an observed effect of a drug on a disease); a provenance part that describes how this piece of data came about (e.g., how it was measured); and a publication info part that give meta-information about the nanopublication as a whole” [ 51 ].
With our modeled nutritional data on the nanopublication servers there is a an opportunity to have a reliable, persistent, and queryable source to utilize the fast food nutritional information [ 51 , 52 ]. Our immediate goal is to develop a custom publication pipeline that will convert our ontology of fast food facts into atomic nanopublication graph formats (.trig) that are linked together. Figure 7 summarizes this next step where a populated OFFF with instance data is decomposed to nanopublication graph formats. Each nanopublication is composed of four basic parts—a header content (gray part of Fig. 7 ), the assertion content that have one fast food fact (blue-ish part of Fig. 7 ), the provenance content (reddish-part of Fig. 7 ), and the nanopublication metadata (yellow part of Fig. 7 ). Each nanopublication will be assigned a Trusty URI [ 53 ], a cryptographic hash value that are immutable and permanent identifiers of digital content to identify the nanopublication of a fast food fact from OFFF. This identifier will also be utilized to link associated data. In the example of Fig. 7 , the nanopublication is linked to the allergen information, and potentially to other nutritional data and metadata. We intend with the proposed pipeline to have formalized publication procedure where when there is new data, it will automatically be converted to verified before public release. Subsequently with published fast food nanopublications, we will investigate tools for querying and management which is lacking but of interest with nanopublication research and development [ 54 ].

A summary of future proposed publishing pipeline to facilitate the conversion of facts from the Ontology of Fast Food Facts to nanopublication files for distribution
In a separate domain, we (MA, CT) have worked on interactive patient-facing technology for vaccine education and counseling. One of the tools from that endeavor is a dialogue system engine [ 55 ] that we envision to automate lightweight evidence-based nutritional counseling to assist in curbing eating behaviors in individuals who have diseases caused by diet and nutritional factors. We intend to use the Ontology of Fast Food Facts to supplement nutritional information that could furnish fast food nutritional information in the automated interactive dialogue. Supplementing the dialogue system engine was a question-answering (QA) subsystem that responded to health consumer questions and generated simple natural language responses from the vaccine knowledge base’s triples [ 55 ]. While we recognize that merely answering questions will not impact behavior the way formalized evidenced-based counseling would, we also plan on repurposing the technology to assess the portability and performance of our QA system.
Poor eating habits either from overeating or food choices has a possible impact on the onset of serious life threatening disease and impacting the quality of life of patients. There is research that alludes that the presentation of nutritional information could have an impact on healthy eating behavior. Yet the copious amount of nutritional information along with potential extended data, complicates any effort to aggregate and centralize nutritional information for consumers and experts to utilize.
We embarked on the development of an ontology of fast food data that aims to normalize and standardize heterogeneous data sources of fast food information, and facilitate high volume and rapid changing amount of nutritional fast food data. The ontology was reviewed to assess the logical construction of the polyhierarchical conceptual level of fast food and fast food nutritional data. While majority of our reviewers perceived most of the ontology’s knowledge to be accurate, a small minority of statements suffered from improper labeling that skewed the logic of the embedded axioms. We revised the ontology and it is available at https://github.com/UTHealth-Ontology/OFFF. This ontology serves as a first step toward potential future direction to completely realize a persistent queryable, public source of linked consumer-centric nutrition data of fast food.
Acknowledgements
Some of the initial data from a previous conference proceeding [ 33 ] was incorporated, redesigned and extended in the work presented in this paper toward a mature evolution of this ontology model.
About this supplement
This article has been published as part of BMC Medical Informatics and Decision Making Volume 21 Supplement 7 2021:Selected articles from the Fifth International Workshop on SemanticsPowered Data Mining and Analytics (SEPDA 2020). The full contents of the supplement are available at https://bmcmedinformdecismak.biomedcentral.com/articles/supplements/volume-21-supplement-7 .
Abbreviations
Authors' contributions.
MA developed the initial draft. CO and TL provided additions and feedback of the initial draft. CO, GX, and CT reviewed the data. MA, CO, CT, GX, and TL provided revisions of the subsequent draft. All authors read and approved the final manuscript.
This research was funded by the National Library of Medicine of the National Institutes of Health under Award Numbers R01LM011829, and the National Institute of Allergy and Infectious Diseases of the National Institutes of Health under Award Number R01AI130460 and R01AI130460-03S1. Publication costs are funded by National Institute on Aging under Award Numbers R1RF1AG072799. The National Library of Medicine of the National Institutes of Health and National Institute of Allergy and Infectious Diseases of the National Institutes of Health, and National Institute on Aging did not play any role in the design of the study, the collection, analysis, and interpretation of data, or in writing of the manuscript.
Availability of data and materials
Declarations.
Not applicable.
The authors declare that they have no competing interests.
Publisher's Note
Springer Nature remains neutral with regard to jurisdictional claims in published maps and institutional affiliations.
Contributor Information
Muhammad Amith, Email: [email protected] .
Chidinma Onye, Email: [email protected] .
Tracey Ledoux, Email: ude.hu.lartnec@xuodelat .
Grace Xiong, Email: moc.liamg@54321gnoixg .
Cui Tao, Email: [email protected] .
Food and Diet
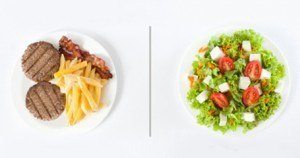
Beyond Willpower: Diet Quality and Quantity Matter
It’s no secret that the amount of calories people eat and drink has a direct impact on their weight: Consume the same number of calories that the body burns over time, and weight stays stable. Consume more than the body burns, weight goes up. Less, weight goes down. But what about the type of calories: Does it matter whether they come from specific nutrients-fat, protein, or carbohydrate? Specific foods-whole grains or potato chips? Specific diets-the Mediterranean diet or the “Twinkie” diet? And what about when or where people consume their calories: Does eating breakfast make it easier to control weight? Does eating at fast-food restaurants make it harder?
There’s ample research on foods and diet patterns that protect against heart disease, stroke, diabetes, and other chronic conditions. The good news is that many of the foods that help prevent disease also seem to help with weight control-foods like whole grains, vegetables, fruits, and nuts. And many of the foods that increase disease risk-chief among them, refined grains and sugary drinks-are also factors in weight gain.Conventional wisdom says that since a calorie is a calorie, regardless of its source, the best advice for weight control is simply to eat less and exercise more. Yet emerging research suggests that some foods and eating patterns may make it easier to keep calories in check, while others may make people more likely to overeat.
This article briefly reviews the research on dietary intake and weight control, highlighting diet strategies that also help prevent chronic disease.
Macronutrients and Weight: Do Carbs, Protein, or Fat Matter?
When people eat controlled diets in laboratory studies, the percentage of calories from fat, protein, and carbohydrate do not seem to matter for weight loss. In studies where people can freely choose what they eat, there may be some benefits to a higher protein, lower carbohydrate approach. For chronic disease prevention, though, the quality and food sources of these nutrients matters more than their relative quantity in the diet. And the latest research suggests that the same diet quality message applies for weight control.
Dietary Fat and Weight
Low-fat diets have long been touted as the key to a healthy weight and to good health. But the evidence just isn’t there: Over the past 30 years in the U.S., the percentage of calories from fat in people’s diets has gone down, but obesity rates have skyrocketed. ( 1 , 2 ) Carefully conducted clinical trials have found that following a low-fat diet does not make it any easier to lose weight than following a moderate- or high-fat diet. In fact, study volunteers who follow moderate- or high-fat diets lose just as much weight, and in some studies a bit more, as those who follow low-fat diets. ( 3 , 4 ) And when it comes to disease prevention, low-fat diets don’t appear to offer any special benefits. ( 5 )
Part of the problem with low-fat diets is that they are often high in carbohydrate, especially from rapidly digested sources, such as white bread and white rice. And diets high in such foods increase the risk of weight gain, diabetes, and heart disease. (See Carbohydrates and Weight , below.)
For good health, the type of fat people eat is far more important that the amount (see box), and there’s some evidence that the same may be true for weight control. ( 6 – 9 ) In the Nurses’ Health Study, for example, which followed 42,000 middle-age and older women for eight years, increased consumption of unhealthy fats-trans fats, especially, but also saturated fats-was linked to weight gain, but increased consumption of healthy fats-monounsaturated and polyunsaturated fat-was not. ( 6 )
Protein and Weight
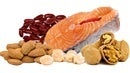
Higher protein diets seem to have some advantages for weight loss, though more so in short-term trials; in longer term studies, high-protein diets seem to perform equally well as other types of diets. ( 3 , 4 ) High-protein diets tend to be low in carbohydrate and high in fat, so it is difficult to tease apart the benefits of eating lots of protein from those of eating more fat or less carbohydrate. But there are a few reasons why eating a higher percentage of calories from protein may help with weight control:
- More satiety: People tend to feel fuller, on fewer calories, after eating protein than they do after eating carbohydrate or fat. ( 10 )
- Greater thermic effect: It takes more energy to metabolize and store protein than other macronutrients, and this may help people increase the energy they burn each day. ( 10 , 11 )
- Improved body composition: Protein seems to help people hang on to lean muscle during weight loss, and this, too, can help boost the energy-burned side of the energy balance equation. ( 11 )
Higher protein, lower carbohydrate diets improve blood lipid profiles and other metabolic markers, so they may help prevent heart disease and diabetes. ( 4 , 12 , 13 ) But some high-protein foods are healthier than others: High intakes of red meat and processed meat are associated with an increased risk of heart disease, diabetes, and colon cancer. ( 14 – 16 )
Replacing red and processed meat with nuts, beans, fish, or poultry seems to lower the risk of heart disease and diabetes. ( 14 , 16 ) And this diet strategy may help with weight control, too, according to a recent study from the Harvard School of Public Health. Researchers tracked the diet and lifestyle habits of 120,000 men and women for up to 20 years, looking at how small changes contributed to weight gain over time. ( 9 ) People who ate more red and processed meat over the course of the study gained more weight-about a pound extra every four years. People who ate more nuts over the course of the study gained less weight-about a half pound less every four years.
Carbohydrates and Weight
Lower carbohydrate, higher protein diets may have some weight loss advantages in the short term. ( 3 , 4 ) Yet when it comes to preventing weight gain and chronic disease, carbohydrate quality is much more important than carbohydrate quantity.
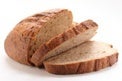
Read more about carbohydrates on The Nutrition Source
Milled, refined grains and the foods made with them-white rice, white bread, white pasta, processed breakfast cereals, and the like-are rich in rapidly digested carbohydrate. So are potatoes and sugary drinks. The scientific term for this is that they have a high glycemic index and glycemic load. Such foods cause fast and furious increases in blood sugar and insulin that, in the short term, can cause hunger to spike and can lead to overeating-and over the long term, increase the risk of weight gain, diabetes, and heart disease. ( 17 – 19 )
For example, in the diet and lifestyle change study, people who increased their consumption of French fries, potatoes and potato chips, sugary drinks, and refined grains gained more weight over time-an extra 3.4, 1.3, 1.0, and 0.6 pounds every four years, respectively. ( 9 ) People who decreased their intake of these foods gained less weight.
Specific Foods that Make It Easier or Harder to Control Weight
There’s growing evidence that specific food choices may help with weight control. The good news is that many of the foods that are beneficial for weight control also help prevent heart disease, diabetes, and other chronic diseases. Conversely, foods and drinks that contribute to weight gain—chief among them, refined grains and sugary drinks—also contribute to chronic disease.
Whole Grains, Fruits and Vegetables, and Weight
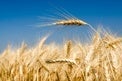
Read more about whole grains on The Nutrition Source
Whole grains-whole wheat, brown rice, barley, and the like, especially in their less-processed forms-are digested more slowly than refined grains. So they have a gentler effect on blood sugar and insulin, which may help keep hunger at bay. The same is true for most vegetables and fruits. These “slow carb” foods have bountiful benefits for disease prevention, and there’s also evidence that they can help prevent weight gain.
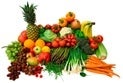
Read more about vegetables and fruits on The Nutrition Source
The weight control evidence is stronger for whole grains than it is for fruits and vegetables. ( 20 – 22 ) The most recent support comes from the Harvard School of Public Health diet and lifestyle change study: People who increased their intake of whole grains, whole fruits (not fruit juice), and vegetables over the course of the 20-year study gained less weight-0.4, 0.5, and 0.2 pounds less every four years, respectively. ( 9 )
Of course, the calories from whole grains, whole fruits, and vegetables don’t disappear. What’s likely happening is that when people increase their intake of these foods, they cut back on calories from other foods. Fiber may be responsible for these foods’ weight control benefits, since fiber slows digestion, helping to curb hunger. Fruits and vegetables are also high in water, which may help people feel fuller on fewer calories.
Nuts and Weight
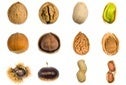
Read more about nuts on The Nutrition Source
Nuts pack a lot of calories into a small package and are high in fat, so they were once considered taboo for dieters. As it turns out, studies find that eating nuts does not lead to weight gain and may instead help with weight control, perhaps because nuts are rich in protein and fiber, both of which may help people feel fuller and less hungry. ( 9 , 23 – 25 ) People who regularly eat nuts are less likely to have heart attacks or die from heart disease than those who rarely eat them, which is another reason to include nuts in a healthy diet. ( 19 )
Dairy and Weight
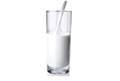
Read more about calcium and milk on The Nutrition Source
The U.S. dairy industry has aggressively promoted the weight-loss benefits of milk and other dairy products, based largely on findings from short-term studies it has funded. ( 26 , 27 ) But a recent review of nearly 50 randomized trials finds little evidence that high dairy or calcium intakes help with weight loss. ( 28 ) Similarly, most long-term follow-up studies have not found that dairy or calcium protect against weight gain, ( 29 – 32 ) and one study in adolescents found high milk intakes to be associated with increased body mass index. ( 33 )
One exception is the recent dietary and lifestyle change study from the Harvard School of Public Health, which found that people who increased their yogurt intake gained less weight; increases in milk and cheese intake, however, did not appear to promote weight loss or gain. ( 9 ) It’s possible that the beneficial bacteria in yogurt may influence weight control, but more research is needed.
Sugar-Sweetened Beverages and Weight
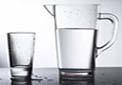
Read more about healthy drinks on The Nutrition Source
There’s convincing evidence that sugary drinks increase the risk of weight gain, obesity, and diabetes: ( 34 – 36 ) A systematic review and meta-analysis of 88 studies found “clear associations of soft drink intake with increased caloric intake and body weight.” ( 34 ) In children and adolescents, a more recent meta analysis estimates that for every additional 12-ounce serving of sugary beverage consumed each day, body mass index increases by 0.08 units. ( 35 ) Another meta analysis finds that adults who regularly drink sugared beverages have a 26 percent higher risk of developing type 2 diabetes than people who rarely drink sugared beverages. ( 36 ) Emerging evidence also suggests that high sugary beverage intake increases the risk of heart disease. ( 37 )
Like refined grains and potatoes, sugary beverages are high in rapidly-digested carbohydrate. (See Carbohydrates and Weight , above.) Research suggests that when that carbohydrate is delivered in liquid form, rather than solid form, it is not as satiating, and people don’t eat less to compensate for the extra calories. ( 38 )
These findings on sugary drinks are alarming, given that children and adults are drinking ever-larger quantities of them: In the U.S., sugared beverages made up about 4 percent of daily calorie intake in the 1970s, but by 2001, represented about 9 percent of calories. ( 36 ) The most recent data find that on any given day, half of Americans consume some type of sugared beverage, 25 percent consume at least 200 calories from sugared drinks, and 5 percent of consume at least 567 calories-the equivalent of four cans of sugary soda. ( 39 )
The good news is that studies in children and adults have also shown that cutting back on sugary drinks can lead to weight loss. ( 40 , 41 ) Sugary drinks have become an important target for obesity prevention efforts, prompting discussions of policy initiatives such as taxing soda. ( 42 )
Fruit Juice and Weight
It’s important to note that fruit juices are not a better option for weight control than sugar-sweetened beverages. Ounce for ounce, fruit juices-even those that are 100 percent fruit juice, with no added sugar- are as high in sugar and calories as sugary sodas. So it’s no surprise that a recent Harvard School of Public Health study, which tracked the diet and lifestyle habits of 120,000 men and women for up to 20 years, found that people who increased their intake of fruit juice gained more weight over time than people who did not. ( 9 ) Pediatricians and public health advocates recommend that children and adults limit fruit juice to just a small glass a day, if they consume it at all.
Alcohol and Weight
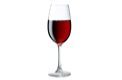
Read more about alcohol on The Nutrition Source
Even though most alcoholic beverages have more calories per ounce than sugar-sweetened beverages, there’s no clear-cut evidence that moderate drinking contributes to weight gain. While the recent diet and lifestyle change study found that people who increased their alcohol intake gained more weight over time, the findings varied by type of alcohol. ( 9 ) In most previous prospective studies, there was no difference in weight gain over time between light-to-moderate drinkers and nondrinkers, or the light-to-moderate drinkers gained less weight than nondrinkers. ( 43 – 47 )
Diet Patterns, Portion Size, and Weight
People don’t eat nutrients or foods in isolation. They eat meals that fall into an overall eating pattern, and researchers have begun exploring whether particular diet or meal patterns help with weight control or contribute to weight gain. Portion sizes have also increased dramatically over the past three decades, as has consumption of fast food-U.S. children, for example, consume a greater percentage of calories from fast food than they do from school food ( 48 )-and these trends are also thought to be contributors to the obesity epidemic.
Dietary Patterns and Weight
So-called “prudent” dietary patterns-diets that feature whole grains, vegetables, and fruits-seem to protect against weight gain, whereas “Western-style” dietary patterns-with more red meat or processed meat, sugared drinks, sweets, refined carbohydrates, or potatoes-have been linked to obesity. ( 49 – 52 ) The Western-style dietary pattern is also linked to increased risk of heart disease, diabetes, and other chronic conditions.
Following a Mediterranean-style diet, well-documented to protect against chronic disease, ( 53 ) appears to be promising for weight control, too. The traditional Mediterranean-style diet is higher in fat (about 40 percent of calories) than the typical American diet (34 percent of calories ( 54 )), but most of the fat comes from olive oil and other plant sources. The diet is also rich in fruits, vegetables, nuts, beans, and fish. A 2008 systematic review found that in most (but not all) studies, people who followed a Mediterranean-style diet had lower rates of obesity or more weight loss. ( 55 ) There is no single “Mediterranean” diet, however, and studies often use different definitions, so more research is needed.
Breakfast, Meal Frequency, Snacking, and Weight
There is some evidence that skipping breakfast increases the risk of weight gain and obesity, though the evidence is stronger in children, especially teens, than it is in adults. ( 56 ) Meal frequency and snacking have increased over the past 30 years in the U.S. ( 57 )-on average, children get 27 percent of their daily calories from snacks, primarily from desserts and sugary drinks, and increasingly from salty snacks and candy. But there have been conflicting findings on the relationship between meal frequency, snacking, and weight control, and more research is needed. ( 56 )
Portion Sizes and Weight
Since the 1970s, portion sizes have increased both for food eaten at home and for food eaten away from home, in adults and children. ( 58 , 59 ) Short-term studies clearly demonstrate that when people are served larger portions, they eat more. One study, for example, gave moviegoers containers of stale popcorn in either large or medium-sized buckets; people reported that they did not like the taste of the popcorn-and even so, those who received large containers ate about 30 percent more popcorn than those who received medium-sized containers. ( 60 ) Another study showed that people given larger beverages tended to drink significantly more, but did not decrease their subsequent food consumption . ( 67 ) An additional study provided evidence that when provided with larger portion sizes, people tended to eat more, with no decrease in later food intake. ( 68 ) There is an intuitive appeal to the idea that portion sizes increase obesity, but long-term prospective studies would help to strengthen this hypothesis.
Fast Food and Weight
Fast food is known for its large portions, low prices, high palatability, and high sugar content, and there’s evidence from studies in teens and adults that frequent fast-food consumption contributes to overeating and weight gain. ( 61 – 66 ) The CARDIA study, for example, followed 3,000 young adults for 13 years. People who had higher fast-food-intake levels at the start of the study weighed an average of about 13 pounds more than people who had the lowest fast-food-intake levels. They also had larger waist circumferences and greater increases in triglycercides, and double the odds of developing metabolic syndrome. ( 62 ) More research is needed to tease apart the effect of eating fast food itself from the effect of the neighborhood people live in, or other individual traits that may make people more likely to eat fast food.
The Bottom Line: Healthy Diet Can Prevent Weight Gain and Chronic Disease
Weight gain in adulthood is often gradual, about a pound a year ( 9 )-too slow of a gain for most people to notice, but one that can add up, over time, to a weighty personal and public health problem. There’s increasing evidence that the same healthful food choices and diet patterns that help prevent heart disease, diabetes, and other chronic conditions may also help to prevent weight gain:
- Choose minimally processed, whole foods-whole grains, vegetables, fruits, nuts, healthful sources of protein (fish, poultry, beans), and plant oils.
- Limit sugared beverages, refined grains, potatoes, red and processed meats, and other highly processed foods, such as fast food.
Though the contribution of any one diet change to weight control may be small, together, the changes could add up to a considerable effect, over time and across the whole society. ( 9 ) Since people’s food choices are shaped by their surroundings, it’s imperative for governments to promote policy and environmental changes that make healthy foods more accessible and decrease the availability and marketing of unhealthful foods.
1. Willett WC, Leibel RL. Dietary fat is not a major determinant of body fat. Am J Med . 2002;113 Suppl 9B:47S-59S.
2. Melanson EL, Astrup A, Donahoo WT. The relationship between dietary fat and fatty acid intake and body weight, diabetes, and the metabolic syndrome. Ann Nutr Metab . 2009;55:229-43.
3. Sacks FM, Bray GA, Carey VJ, et al. Comparison of weight-loss diets with different compositions of fat, protein, and carbohydrates. N Engl J Med . 2009;360:859-73.
4. Shai I, Schwarzfuchs D, Henkin Y, et al. Weight loss with a low-carbohydrate, Mediterranean, or low-fat diet. N Engl J Med . 2008;359:229-41.
5. Howard BV, Manson JE, Stefanick ML, et al. Low-fat dietary pattern and weight change over 7 years: the Women’s Health Initiative Dietary Modification Trial. JAMA . 2006;295:39-49.
6. Field AE, Willett WC, Lissner L, Colditz GA. Dietary fat and weight gain among women in the Nurses’ Health Study. Obesity (Silver Spring) . 2007;15:967-76.
7. Koh-Banerjee P, Chu NF, Spiegelman D, et al. Prospective study of the association of changes in dietary intake, physical activity, alcohol consumption, and smoking with 9-y gain in waist circumference among 16 587 US men. Am J Clin Nutr . 2003;78:719-27.
8. Thompson AK, Minihane AM, Williams CM. Trans fatty acids and weight gain. Int J Obes (Lond) . 2011;35:315-24.
9. Mozaffarian D, Hao T, Rimm EB, Willett WC, Hu FB. Changes in diet and lifestyle and long-term weight gain in women and men. N Engl J Med . 2011;364:2392-404.
10. Halton TL, Hu FB. The effects of high protein diets on thermogenesis, satiety and weight loss: a critical review. J Am Coll Nutr . 2004;23:373-85.
11. Westerterp-Plantenga MS, Nieuwenhuizen A, Tome D, Soenen S, Westerterp KR. Dietary protein, weight loss, and weight maintenance. Annu Rev Nutr . 2009;29:21-41.
12. Furtado JD, Campos H, Appel LJ, et al. Effect of protein, unsaturated fat, and carbohydrate intakes on plasma apolipoprotein B and VLDL and LDL containing apolipoprotein C-III: results from the OmniHeart Trial. Am J Clin Nutr . 2008;87:1623-30.
13. Appel LJ, Sacks FM, Carey VJ, et al. Effects of protein, monounsaturated fat, and carbohydrate intake on blood pressure and serum lipids: results of the OmniHeart randomized trial. JAMA . 2005;294:2455-64.
14. Bernstein AM, Sun Q, Hu FB, Stampfer MJ, Manson JE, Willett WC. Major dietary protein sources and risk of coronary heart disease in women. Circulation . 2010;122:876-83.
15. Aune D, Ursin G, Veierod MB. Meat consumption and the risk of type 2 diabetes: a systematic review and meta-analysis of cohort studies. Diabetologia . 2009;52:2277-87.
16. Pan A, Sun Q, Bernstein AM, et al. Red meat consumption and risk of type 2 diabetes: 3 cohorts of US adults and an updated meta-analysis. Am J Clin Nutr . 2011;94(4):1088-96.
17. Abete I, Astrup A, Martinez JA, Thorsdottir I, Zulet MA. Obesity and the metabolic syndrome: role of different dietary macronutrient distribution patterns and specific nutritional components on weight loss and maintenance. Nutr Rev . 2010;68:214-31.
18. Barclay AW, Petocz P, McMillan-Price J, et al. Glycemic index, glycemic load, and chronic disease risk—a meta-analysis of observational studies. Am J Clin Nutr . 2008;87:627-37.
19. Mente A, de Koning L, Shannon HS, Anand SS. A systematic review of the evidence supporting a causal link between dietary factors and coronary heart disease. Arch Intern Med . 2009;169:659-69.
20. Koh-Banerjee P, Franz M, Sampson L, et al. Changes in whole-grain, bran, and cereal fiber consumption in relation to 8-y weight gain among men. Am J Clin Nutr . 2004;80:1237-45.
21. Liu S, Willett WC, Manson JE, Hu FB, Rosner B, Colditz G. Relation between changes in intakes of dietary fiber and grain products and changes in weight and development of obesity among middle-aged women. Am J Clin Nutr . 2003;78:920-7.
22. Ledoux TA, Hingle MD, Baranowski T. Relationship of fruit and vegetable intake with adiposity: a systematic review. Obes Rev . 2011;12:e143-50.
23. Mattes RD, Kris-Etherton PM, Foster GD. Impact of peanuts and tree nuts on body weight and healthy weight loss in adults. J Nutr . 2008;138:1741S-5S.
24. Bes-Rastrollo M, Sabate J, Gomez-Gracia E, Alonso A, Martinez JA, Martinez-Gonzalez MA. Nut consumption and weight gain in a Mediterranean cohort: The SUN study. Obesity (Silver Spring) . 2007;15:107-16.
25. Bes-Rastrollo M, Wedick NM, Martinez-Gonzalez MA, Li TY, Sampson L, Hu FB. Prospective study of nut consumption, long-term weight change, and obesity risk in women. Am J Clin Nutr . 2009;89:1913-19.
26. Zemel MB, Shi H, Greer B, Dirienzo D, Zemel PC. Regulation of adiposity by dietary calcium. FASEB J . 2000;14:1132-8.
27. Zemel MB, Thompson W, Milstead A, Morris K, Campbell P. Calcium and dairy acceleration of weight and fat loss during energy restriction in obese adults. Obes Res . 2004;12:582-90.
28. Lanou AJ, Barnard ND. Dairy and weight loss hypothesis: an evaluation of the clinical trials. Nutr Rev . 2008;66:272-9.
29. Phillips SM, Bandini LG, Cyr H, Colclough-Douglas S, Naumova E, Must A. Dairy food consumption and body weight and fatness studied longitudinally over the adolescent period. Int J Obes Relat Metab Disord . 2003;27:1106-13.
30. Rajpathak SN, Rimm EB, Rosner B, Willett WC, Hu FB. Calcium and dairy intakes in relation to long-term weight gain in US men. Am J Clin Nutr . 2006;83:559-66.
31. Snijder MB, van Dam RM, Stehouwer CD, Hiddink GJ, Heine RJ, Dekker JM. A prospective study of dairy consumption in relation to changes in metabolic risk factors: the Hoorn Study. Obesity (Silver Spring) . 2008;16:706-9.
32. Boon N, Koppes LL, Saris WH, Van Mechelen W. The relation between calcium intake and body composition in a Dutch population: The Amsterdam Growth and Health Longitudinal Study. Am J Epidemiol . 2005;162:27-32.
33. Berkey CS, Rockett HR, Willett WC, Colditz GA. Milk, dairy fat, dietary calcium, and weight gain: a longitudinal study of adolescents. Arch Pediatr Adolesc Med . 2005;159:543-50.
34. Vartanian LR, Schwartz MB, Brownell KD. Effects of soft drink consumption on nutrition and health: a systematic review and meta-analysis. Am J Public Health . 2007;97:667-75.
35. Malik VS, Willett WC, Hu FB. Sugar-sweetened beverages and BMI in children and adolescents: reanalyses of a meta-analysis. Am J Clin Nutr . 2009;89:438-9; author reply 9-40.
36. Hu FB, Malik VS. Sugar-sweetened beverages and risk of obesity and type 2 diabetes: epidemiologic evidence. Physiol Behav . 2010;100:47-54.
37. Malik VS, Popkin BM, Bray GA, Despres JP, Willett WC, Hu FB. Sugar-sweetened beverages and risk of metabolic syndrome and type 2 diabetes: a meta-analysis. Diabetes Care . 2010;33:2477-83.
38. Pan A, Hu FB. Effects of carbohydrates on satiety: differences between liquid and solid food. Curr Opin Clin Nutr Metab Care . 2011;14:385-90.
39. Ogden CL KB, Carroll MD, Park S. Consumption of sugar drinks in the United States , 2005-2008. Hyattsville, MD: National Center for Health Statistics; 2011.
40. Chen L, Appel LJ, Loria C, et al. Reduction in consumption of sugar-sweetened beverages is associated with weight loss: the PREMIER trial. Am J Clin Nutr . 2009;89:1299-306.
41. Ebbeling CB, Feldman HA, Osganian SK, Chomitz VR, Ellenbogen SJ, Ludwig DS. Effects of decreasing sugar-sweetened beverage consumption on body weight in adolescents: a randomized, controlled pilot study. Pediatrics . 2006;117:673-80.
42. Brownell KD, Farley T, Willett WC, et al. The public health and economic benefits of taxing sugar-sweetened beverages. N Engl J Med . 2009;361:1599-605.
43. Wang L, Lee IM, Manson JE, Buring JE, Sesso HD. Alcohol consumption, weight gain, and risk of becoming overweight in middle-aged and older women. Arch Intern Med . 2010;170:453-61.
44. Liu S, Serdula MK, Williamson DF, Mokdad AH, Byers T. A prospective study of alcohol intake and change in body weight among US adults. Am J Epidemiol . 1994;140:912-20.
45. Wannamethee SG, Field AE, Colditz GA, Rimm EB. Alcohol intake and 8-year weight gain in women: a prospective study. Obes Res . 2004;12:1386-96.
46. Lewis CE, Smith DE, Wallace DD, Williams OD, Bild DE, Jacobs DR, Jr. Seven-year trends in body weight and associations with lifestyle and behavioral characteristics in black and white young adults: the CARDIA study. Am J Public Health . 1997;87:635-42.
47. Bes-Rastrollo M, Sanchez-Villegas A, Gomez-Gracia E, Martinez JA, Pajares RM, Martinez-Gonzalez MA. Predictors of weight gain in a Mediterranean cohort: the Seguimiento Universidad de Navarra Study 1. Am J Clin Nutr . 2006;83:362-70; quiz 94-5.
48. Poti JM, Popkin BM. Trends in Energy Intake among US Children by Eating Location and Food Source, 1977-2006. J Am Diet Assoc . 2011;111:1156-64.
49. Schulze MB, Fung TT, Manson JE, Willett WC, Hu FB. Dietary patterns and changes in body weight in women. Obesity (Silver Spring) . 2006;14:1444-53.
50. Newby PK, Muller D, Hallfrisch J, Andres R, Tucker KL. Food patterns measured by factor analysis and anthropometric changes in adults. Am J Clin Nutr . 2004;80:504-13.
51. Schulz M, Nothlings U, Hoffmann K, Bergmann MM, Boeing H. Identification of a food pattern characterized by high-fiber and low-fat food choices associated with low prospective weight change in the EPIC-Potsdam cohort. J Nutr . 2005;135:1183-9.
52. Newby PK, Muller D, Hallfrisch J, Qiao N, Andres R, Tucker KL. Dietary patterns and changes in body mass index and waist circumference in adults. Am J Clin Nutr . 2003;77:1417-25.
53. Sofi F, Abbate R, Gensini GF, Casini A. Accruing evidence on benefits of adherence to the Mediterranean diet on health: an updated systematic review and meta-analysis. Am J Clin Nutr . 2010;92:1189-96.
54. Wright JD WC-Y. Trends in intake of energy and macronutrients in adults from 1999-2000 through 2007-2008 . Hyattsville, MD: National Center for Health Statistics; 2010.
55. Buckland G, Bach A, Serra-Majem L. Obesity and the Mediterranean diet: a systematic review of observational and intervention studies. Obes Rev . 2008;9:582-93.
56. Dietary Guidelines for Americans Advisory Committee. Report of the DGAC on the Dietary Guidelines for Americans , 2010 ; 2010.
57. Popkin BM, Duffey KJ. Does hunger and satiety drive eating anymore? Increasing eating occasions and decreasing time between eating occasions in the United States. Am J Clin Nutr . 2010;91:1342-7.
58. Nielsen SJ, Popkin BM. Patterns and trends in food portion sizes, 1977-1998. JAMA . 2003;289:450-3.
59. Piernas C, Popkin BM. Food portion patterns and trends among U.S. children and the relationship to total eating occasion size, 1977-2006. J Nutr . 2011;141:1159-64.
60. Wansink B, Kim J. Bad popcorn in big buckets: portion size can influence intake as much as taste. J Nutr Educ Behav . 2005;37:242-5.
61. Duffey KJ, Gordon-Larsen P, Jacobs DR, Jr., Williams OD, Popkin BM. Differential associations of fast food and restaurant food consumption with 3-y change in body mass index: the Coronary Artery Risk Development in Young Adults Study. Am J Clin Nutr . 2007;85:201-8.
62. Duffey KJ, Gordon-Larsen P, Steffen LM, Jacobs DR, Jr., Popkin BM. Regular consumption from fast food establishments relative to other restaurants is differentially associated with metabolic outcomes in young adults. J Nutr . 2009;139:2113-8.
63. Taveras EM, Berkey CS, Rifas-Shiman SL, et al. Association of consumption of fried food away from home with body mass index and diet quality in older children and adolescents. Pediatrics . 2005;116:e518-24.
64. French SA, Harnack L, Jeffery RW. Fast food restaurant use among women in the Pound of Prevention study: dietary, behavioral and demographic correlates. Int J Obes Relat Metab Disord . 2000;24:1353-9.
65. Pereira MA, Kartashov AI, Ebbeling CB, et al. Fast-food habits, weight gain, and insulin resistance (the CARDIA study): 15-year prospective analysis. Lancet . 2005;365:36-42.
66. Rosenheck R. Fast food consumption and increased caloric intake: a systematic review of a trajectory towards weight gain and obesity risk. Obes Rev . 2008;9:535-47.
67. Rolls, B. J., L. S. Roe, et al. (2007). “The effect of large portion sizes on energy intake is sustained for 11 days.” Obesity (Silver Spring) 15(6): 1535-1543.
68. Ello-Martin, J. A., J. H. Ledikwe, et al. (2005). “The influence of food portion size and energy density on energy intake: implications for weight management.” Am J Clin Nutr 82(1 Suppl): 236S-241S.
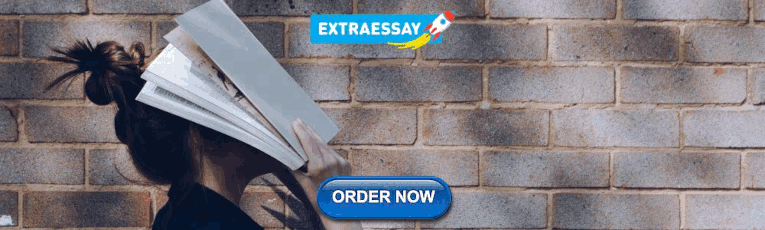
Fasting-like diet lowers risk factors for disease, reduces biological age in humans: Study
C ycles of a diet that mimics fasting can reduce signs of immune system aging, as well as insulin resistance and liver fat in humans, resulting in a lower biological age, according to a new USC Leonard Davis School of Gerontology-led study.
The study, published in Nature Communications on Feb. 20, adds to the body of evidence supporting the beneficial effects of the fasting-mimicking diet (FMD).
The FMD is a five-day diet high in unsaturated fats and low in overall calories, protein, and carbohydrates and is designed to mimic the effects of a water-only fast while still providing necessary nutrients and making it much easier for people to complete the fast. The diet was developed by the laboratory of USC Leonard Davis School Professor Valter Longo, the senior author of the new study.
"This is the first study to show that a food-based intervention that does not require chronic dietary or other lifestyle changes can make people biologically younger, based on both changes in risk factors for aging and disease and on a validated method developed by the Levine group to assess biological age," Longo said.
Previous research led by Longo has indicated that brief, periodic FMD cycles are associated with a range of beneficial effects. They can:
- Promote stem cell regeneration
- Lessen chemotherapy side effects
- Reduce the signs of dementia in mice
In addition, the FMD cycles can lower the risk factors for cancer, diabetes, heart disease and other age-related diseases in humans.
The Longo lab also had previously shown that one or two cycles of the FMD for five days a month increased the healthspan and lifespan of mice on either a normal or Western diet, but the effects of the FMD on aging and biological age, liver fat, and immune system aging in humans were unknown until now.
Lower disease risks and more youthful cells
The study analyzed the diet's effects in two clinical trial populations, each with men and women between the ages of 18 and 70. Patients who were randomized to the fasting-mimicking diet underwent 3–4 monthly cycles, adhering to the FMD for 5 days, then ate a normal diet for 25 days.
The FMD is comprised of plant-based soups, energy bars, energy drinks, chip snacks, and tea portioned out for 5 days as well as a supplement providing high levels of minerals, vitamins, and essential fatty acids. Patients in the control groups were instructed to eat either a normal or Mediterranean-style diet.
An analysis of blood samples from trial participants showed that patients in the FMD group had lower diabetes risk factors, including less insulin resistance and lower HbA1c results. Magnetic resonance imaging also revealed a decrease in abdominal fat as well as fat within the liver, improvements associated with a reduced risk of metabolic syndrome. In addition, the FMD cycles appeared to increase the lymphoid-to-myeloid ratio—an indicator of a more youthful immune system.
Further statistical analysis of the results from both clinical studies showed that FMD participants had reduced their biological age—a measure of how well one's cells and tissues are functioning, as opposed to chronological age—by 2.5 years on average.
"This study shows for the first time evidence for biological age reduction from two different clinical trials, accompanied by evidence of rejuvenation of metabolic and immune function," Longo said.
The study, conducted by first authors Sebastian Brandhorst, USC Leonard Davis research associate professor, and Morgan E. Levine, founding principal investigator of Altos Labs and USC Leonard Davis Ph.D. alumna, lends more support to the FMD's potential as a short-term periodic, achievable dietary intervention that can help people lessen their disease risk and improve their health without extensive lifestyle changes, Longo said.
"Although many doctors are already recommending the FMD in the United States and Europe, these findings should encourage many more health care professionals to recommend FMD cycles to patients with higher than desired levels of disease risk factors as well as to the general population that may be interested in increased function and younger age," Longo said.
More information: Fasting-mimicking diet causes hepatic and blood markers changes indicating reduced biological age and disease risk, Nature Communications (2024). DOI: 10.1038/s41467-024-45260-9
Provided by University of Southern California

A .gov website belongs to an official government organization in the United States.
A lock ( ) or https:// means you've safely connected to the .gov website. Share sensitive information only on official, secure websites.
- About Healthy Growth and Weight
- Water and Healthier Drinks
- Healthy Eating for a Healthy Weight
- Steps for Losing Weight
- Tips for Physical Activity and Your Weight
- Tips for Parents and Caregivers
- External Resources
- Be Sugar Smart
- Rethink Your Drink
About Healthy Weight and Growth
Parents and caregivers can help children have healthy growth and a healthy weight.
- Achieving healthy growth and weight includes healthy eating, physical activity, optimal sleep, and stress reduction.
- There are several ways to eat well, be physically active, and maintain a healthy weight.
Why it's important
Healthy eating, physical activity, optimal sleep , and stress reduction are important to achieving optimal health and managing weight as you age. Several other factors may also affect weight gain.
People who have obesity, compared to those with a healthy weight, are at increased risk for many serious diseases and health conditions .
In addition to the tips below, see other information that can help.
Eat healthy foods
Healthy eating means incorporating a variety of healthy foods into your eating pattern. Fad diets promise fast results. However, such diets limit your nutritional intake, can be unhealthy, and tend to fail in the long run. See these tips instead:
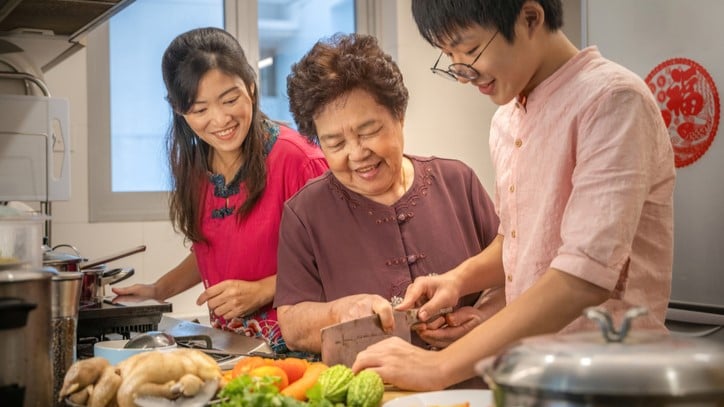
Tips for Healthy Eating for a Healthy Weight
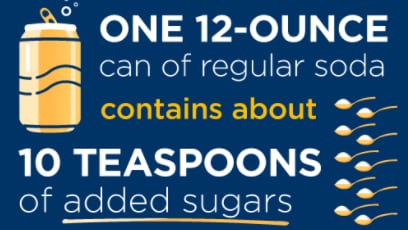
Be Smart About Sugar
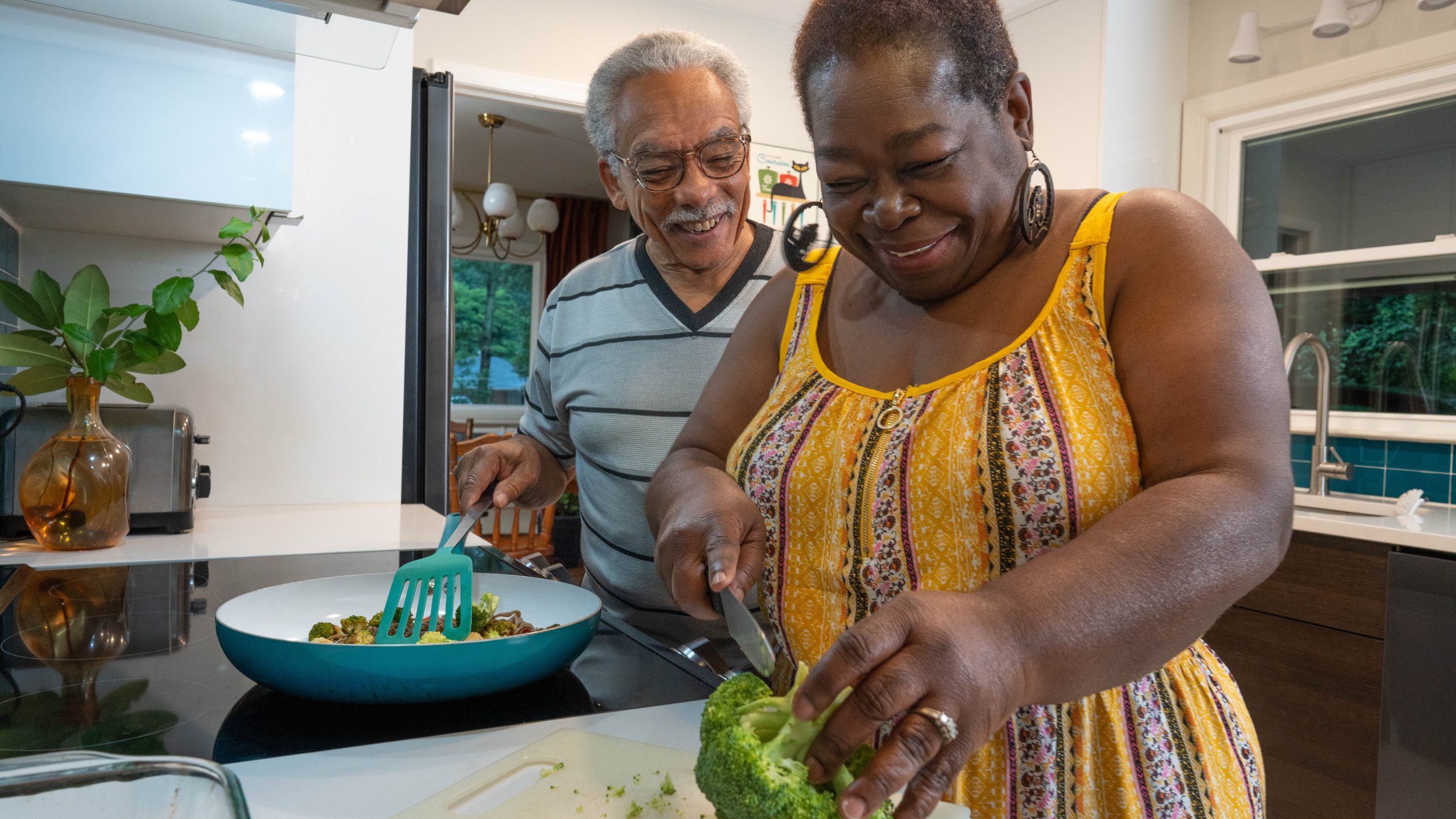
How to Have Healthier Meals and Snacks
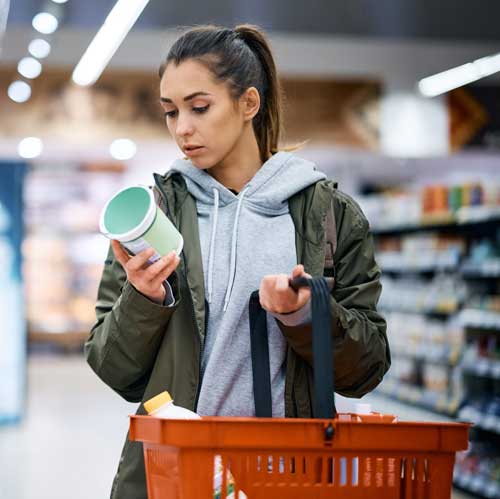
Nutrition Facts Label and Your Health
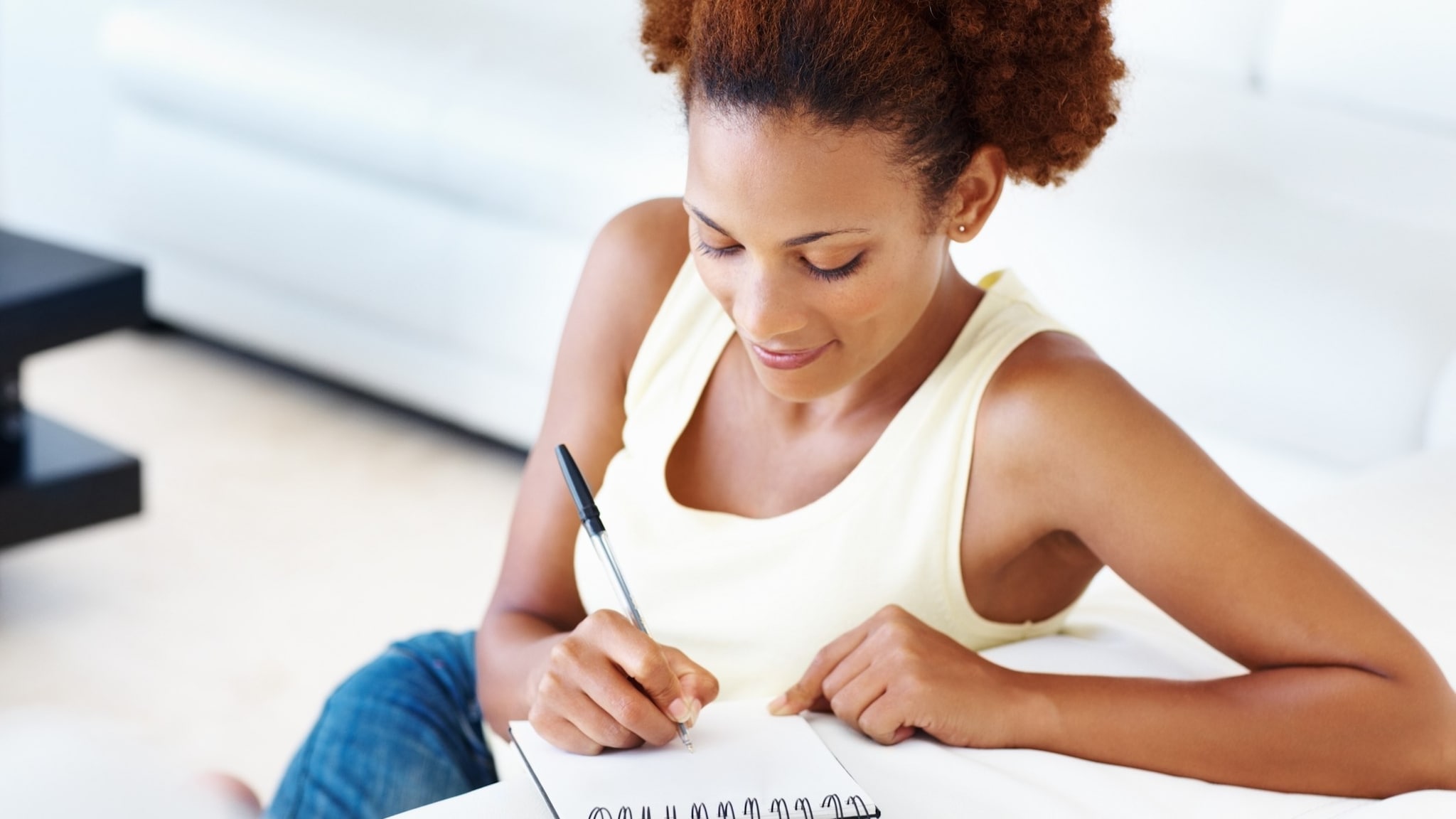
Steps for Improving Your Eating Habits
Limit sugary drinks
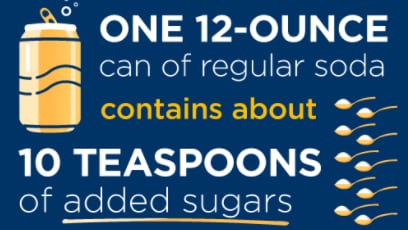
About Water and Healthier Drinks
Tracking food and drinks
Be physically active.
How much physical activity you need depends on your age and whether you are trying to maintain your weight or lose weight. Walking is often a good way to add more physical activity to your lifestyle.
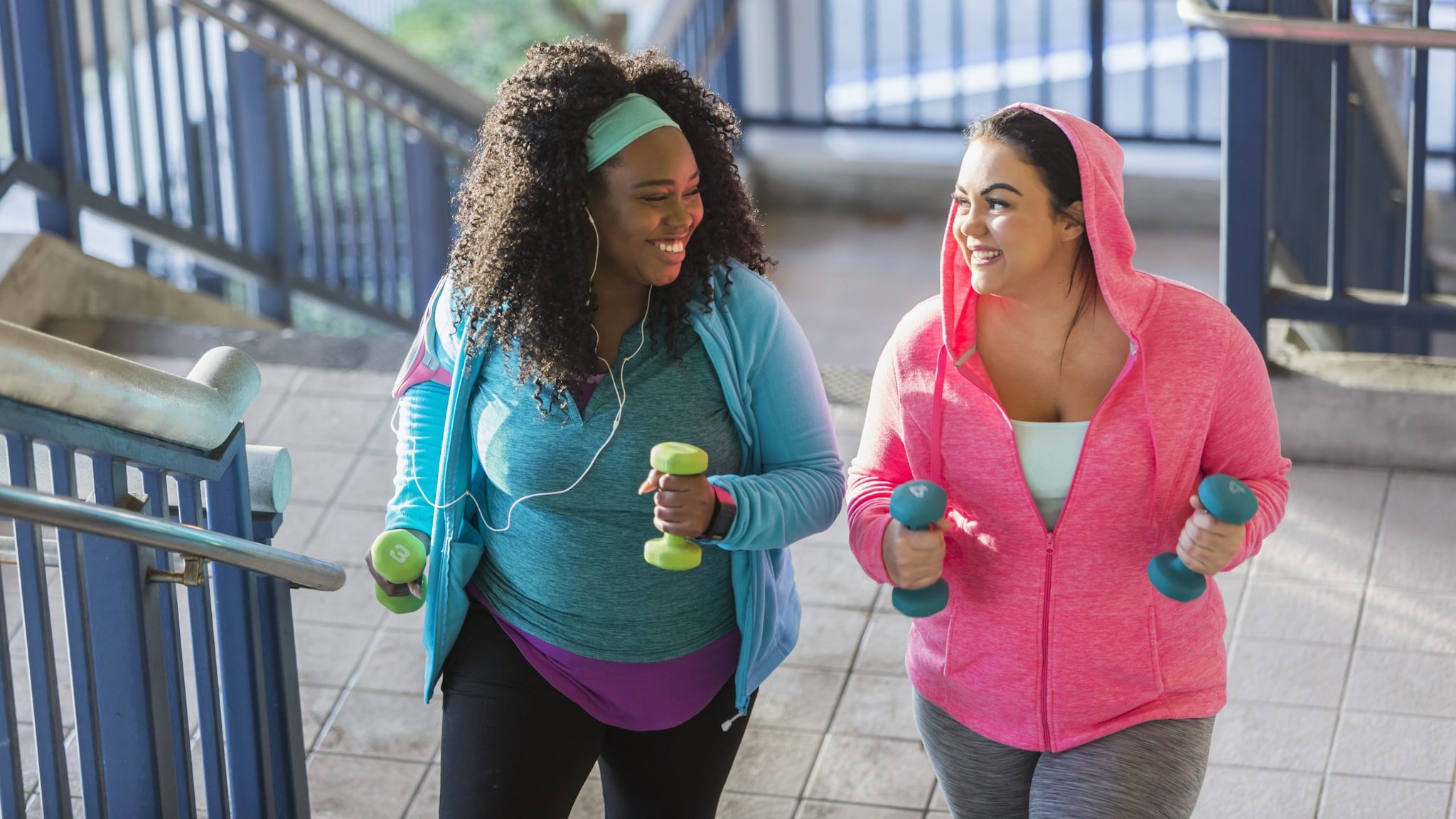
Physical Activity and Your Weight and Health
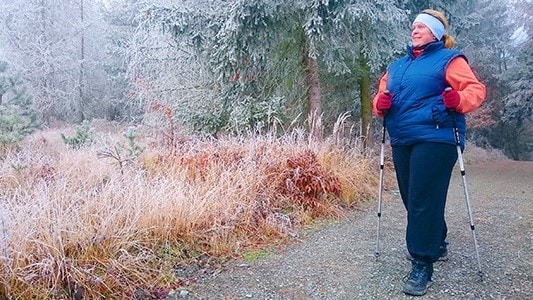
Steps for Getting Started With Physical Activity
Tracking physical activity
Manage your weight.
You can use the body mass index (BMI) calculator as a screening tool for your weight. See more information to help manage your weight.
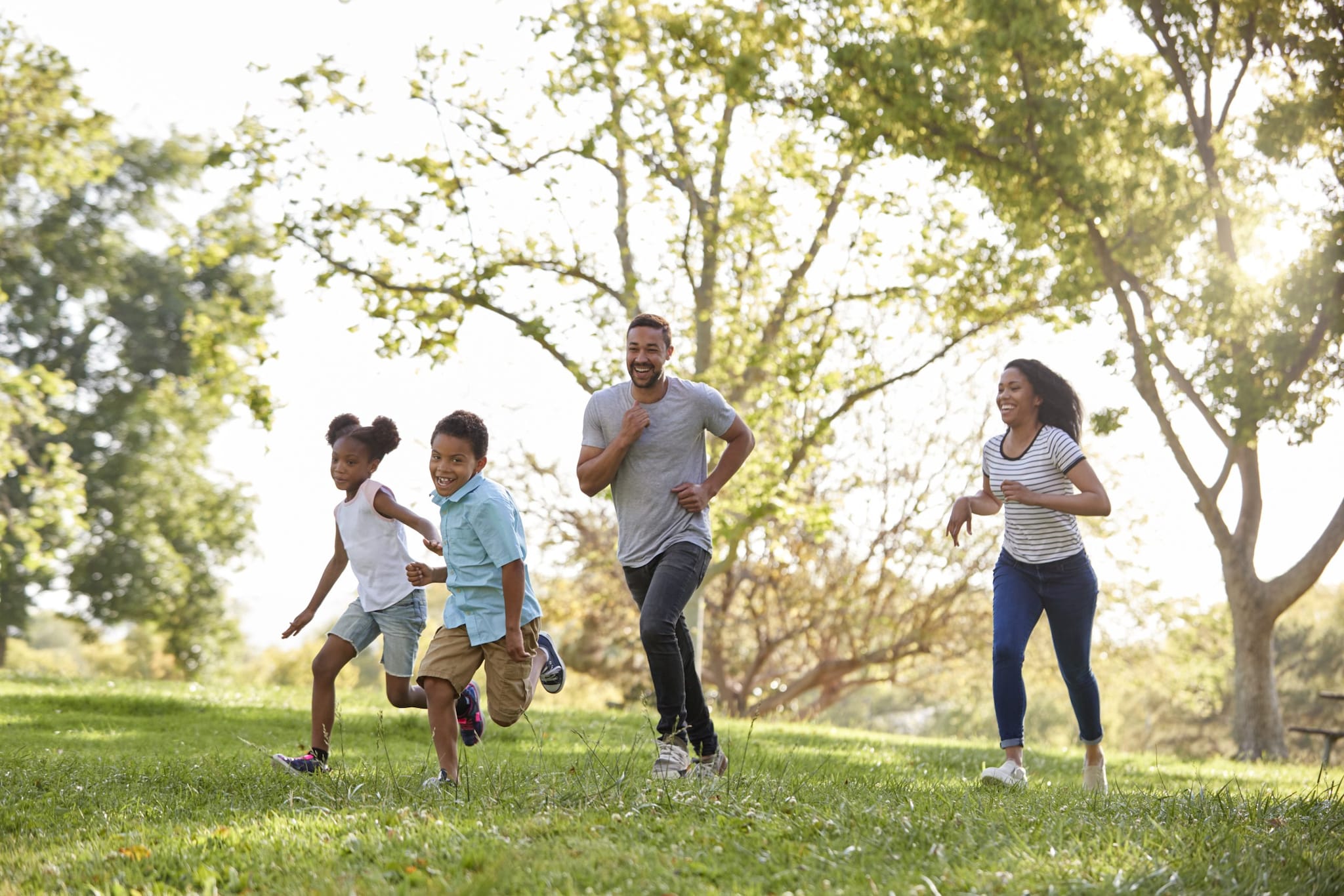
Tips for Maintaining Healthy Weight
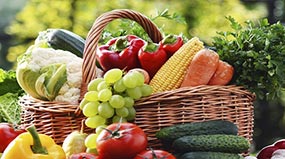
Healthy Habits: Fruits and Vegetables to Manage Weight
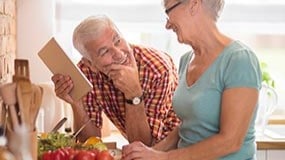
Tips for Keeping Weight Off
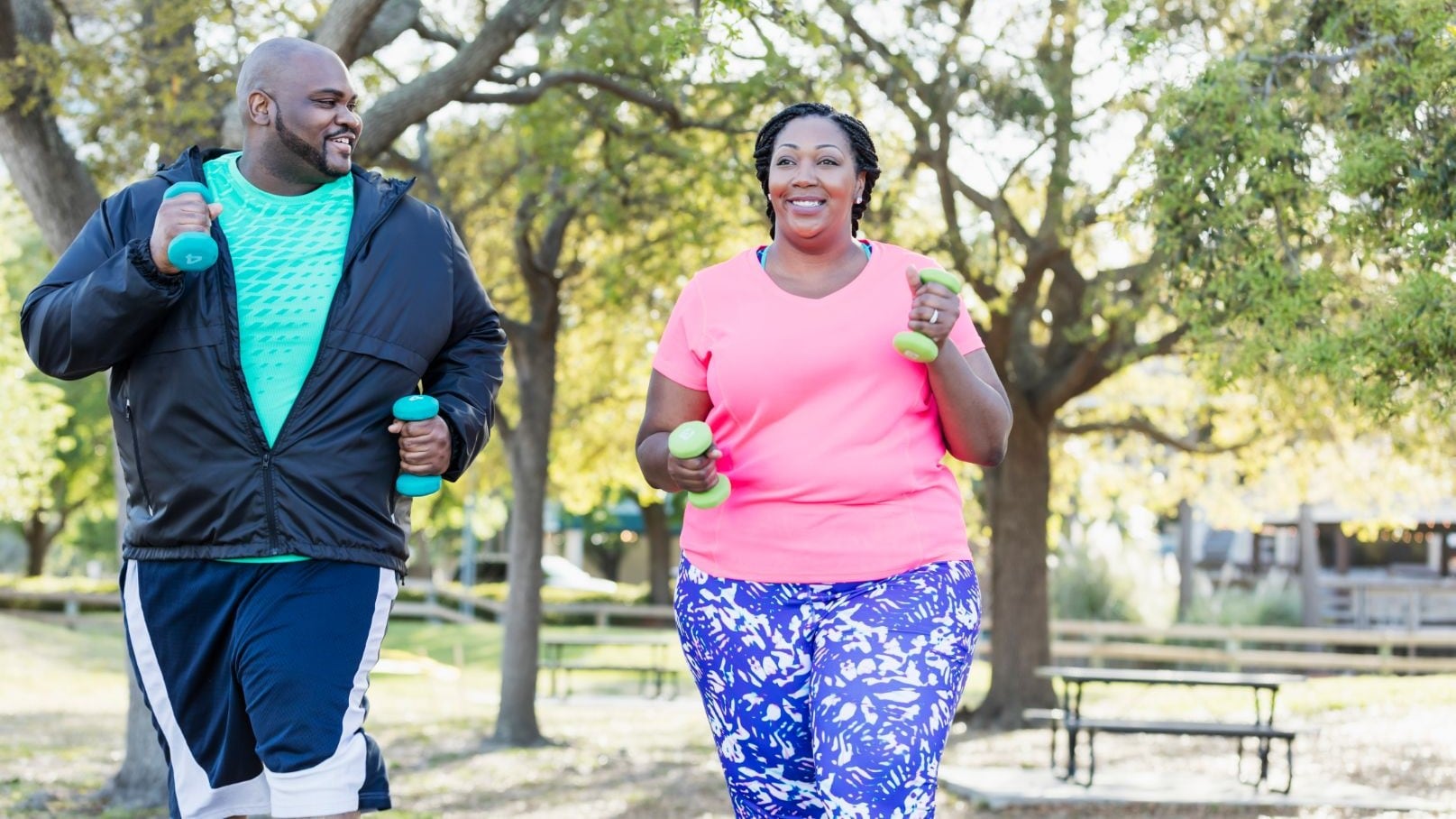
Tips for Cutting Calories
Help children
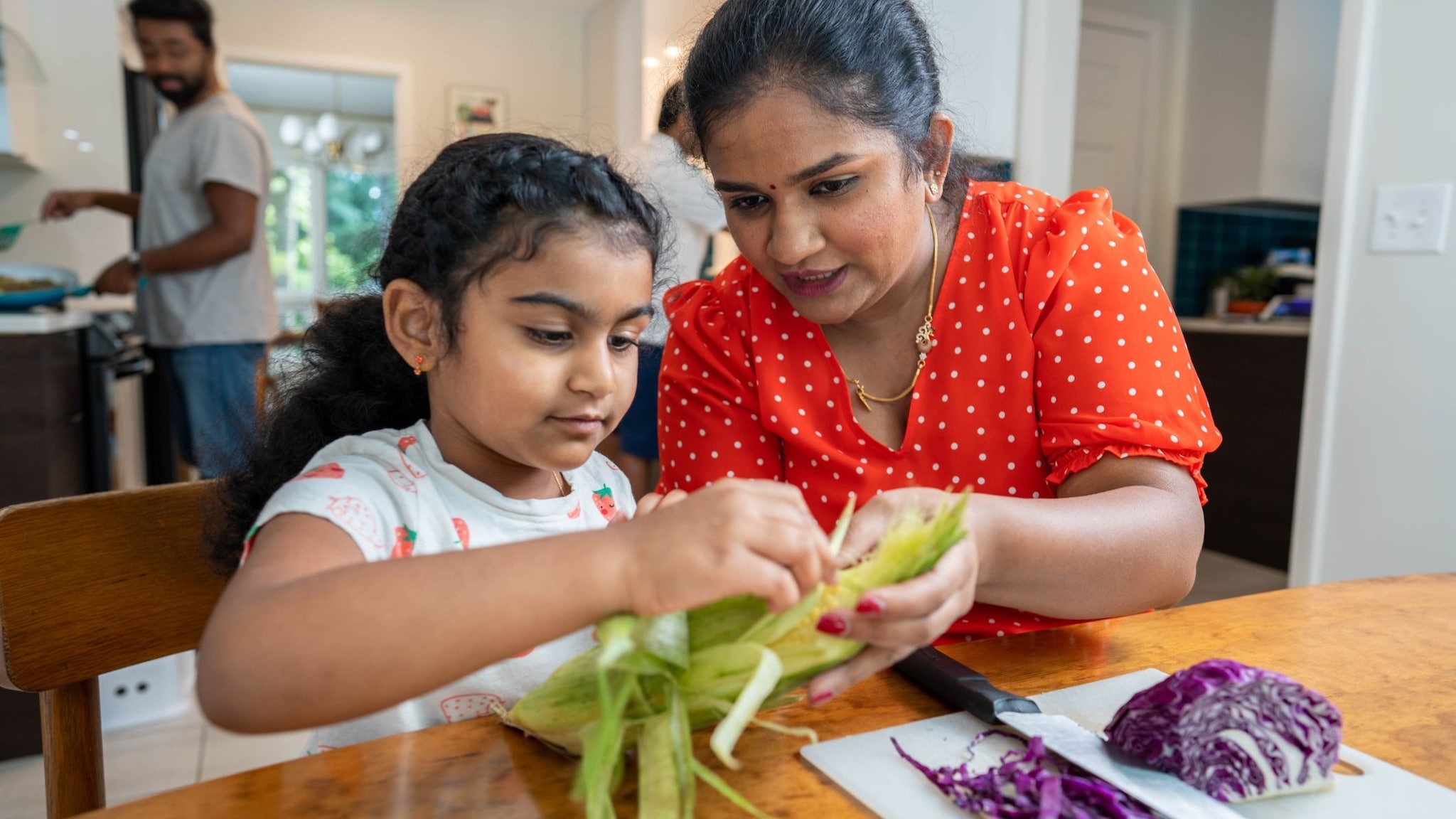
Tips to Support Healthy Routines for Children and Teens

Preventing Childhood Obesity: 6 Things Families Can Do
Adopt healthy habits
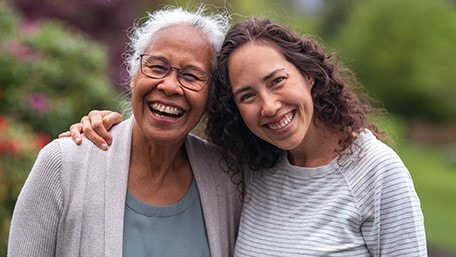
Healthy Habits: Enhancing Immunity
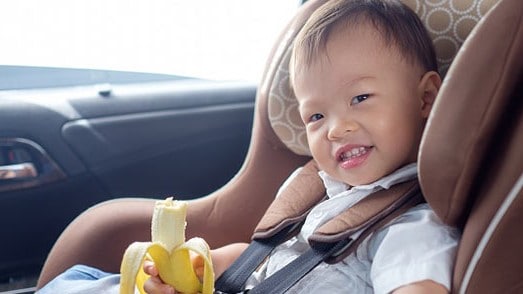
Tips for Healthy Holiday Travel
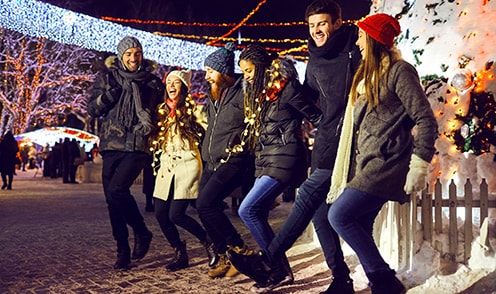
How to Have Healthier Holidays in 1-2-3!
Spanish language material.
Food Assistance and Food System Resources .
Learn how to eat healthy with MyPlate.
My Plate Plan customized to you.
Healthy Weight and Growth
Eating well and being physically active contribute to healthy growth in childhood and good health throughout life. See how to get started.
Thank you for visiting nature.com. You are using a browser version with limited support for CSS. To obtain the best experience, we recommend you use a more up to date browser (or turn off compatibility mode in Internet Explorer). In the meantime, to ensure continued support, we are displaying the site without styles and JavaScript.
- View all journals
- My Account Login
- Explore content
- About the journal
- Publish with us
- Sign up for alerts
- Open access
- Published: 18 January 2022
Large-scale diet tracking data reveal disparate associations between food environment and diet
- Tim Althoff ORCID: orcid.org/0000-0003-4793-2289 1 ,
- Hamed Nilforoshan 2 ,
- Jenna Hua 3 , 4 &
- Jure Leskovec ORCID: orcid.org/0000-0002-5411-923X 2 , 5
Nature Communications volume 13 , Article number: 267 ( 2022 ) Cite this article
22k Accesses
31 Citations
115 Altmetric
Metrics details
- Epidemiology
- Risk factors
An unhealthy diet is a major risk factor for chronic diseases including cardiovascular disease, type 2 diabetes, and cancer 1 , 2 , 3 , 4 . Limited access to healthy food options may contribute to unhealthy diets 5 , 6 . Studying diets is challenging, typically restricted to small sample sizes, single locations, and non-uniform design across studies, and has led to mixed results on the impact of the food environment 7 , 8 , 9 , 10 , 11 , 12 , 13 , 14 , 15 , 16 , 17 , 18 , 19 , 20 , 21 , 22 , 23 . Here we leverage smartphones to track diet health, operationalized through the self-reported consumption of fresh fruits and vegetables, fast food and soda, as well as body-mass index status in a country-wide observational study of 1,164,926 U.S. participants (MyFitnessPal app users) and 2.3 billion food entries to study the independent contributions of fast food and grocery store access, income and education to diet health outcomes. This study constitutes the largest nationwide study examining the relationship between the food environment and diet to date. We find that higher access to grocery stores, lower access to fast food, higher income and college education are independently associated with higher consumption of fresh fruits and vegetables, lower consumption of fast food and soda, and lower likelihood of being affected by overweight and obesity. However, these associations vary significantly across zip codes with predominantly Black, Hispanic or white populations. For instance, high grocery store access has a significantly larger association with higher fruit and vegetable consumption in zip codes with predominantly Hispanic populations (7.4% difference) and Black populations (10.2% difference) in contrast to zip codes with predominantly white populations (1.7% difference). Policy targeted at improving food access, income and education may increase healthy eating, but intervention allocation may need to be optimized for specific subpopulations and locations.
Similar content being viewed by others
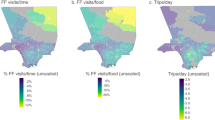
Population mobility data provides meaningful indicators of fast food intake and diet-related diseases in diverse populations
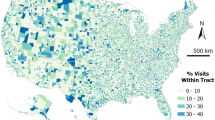
Integrating human activity into food environments can better predict cardiometabolic diseases in the United States
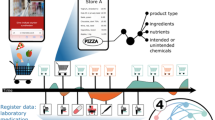
Assessing household lifestyle exposures from consumer purchases, the My Purchases cohort
Introduction.
Dietary factors significantly contribute to risk of mortality and chronic diseases such as cardiovascular diseases, type 2 diabetes and cancer globally 1 , 2 , 3 . Emerging evidence suggests that the built and food environment, behavioral, and socioeconomic factors significantly affect diet 7 . Prior studies of the food environment and diet have led to mixed results 7 , 8 , 9 , 10 , 11 , 12 , 13 , 14 , 15 , 16 , 17 , 18 , 19 , 20 , 21 , 22 , 23 , and very few used nationally representative samples. These mixed results are potentially attributed to methodological limitations of small sample size, differences in geographic contexts, study population, and non-uniform measurements of both the food environment and diet across studies. Therefore, research with larger sample size and using improved and consistent methods and measurements is needed 9 , 24 , 25 .
Commercially available and widely used mobile applications allow the tracking of health behaviors and population health 26 , as recently demonstrated in physical activity 27 , 28 , sleep 29 , 30 , COVID-19 pandemic response 31 , 32 , 33 , women’s health 34 , as well as diet research 35 , 36 , 37 , 38 , 39 , 40 . With ever increasing smartphone ownership in the U.S. 41 and the availability of immense geospatial data, there are now unprecedented opportunities to combine various data on individual diets, population characteristics (gender and ethnicity), socioeconomic status (income and educational attainment), as well as food environment at large scale. Interrogation of these rich data resources to examine geographical and other forms of heterogeneity in the effect of food environments on health could lead to the development and implementation of cost-effective interventions 42 . Here, we leverage large-scale smartphone-based food journals of 1,164,926 participants across 9822 U.S. zip codes (Fig. 1 ) and combine several Internet data sources to quantify the independent associations of food (grocery and fast food) access, income and educational attainment with food consumption and body-mass index (BMI) status (Fig. 2 ). This study constitutes the largest nationwide study examining the relationship between the food environment and diet to date.
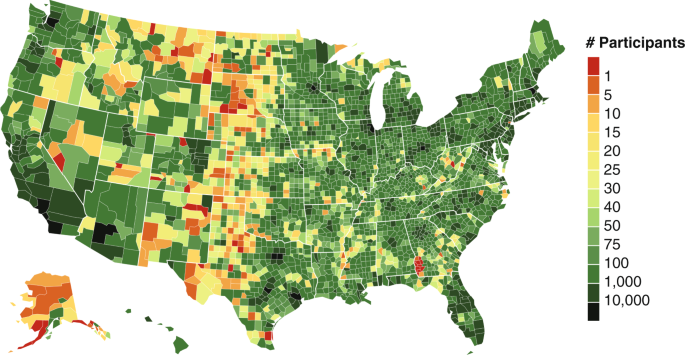
A choropleth showing the number of participants in each U.S. county. This country-wide observational study included 1,164,926 participants across 9822 U.S. zip codes that collectively logged 2.3 billion food entries for an average of 197 days each. This study constitutes the largest nationwide study examining the relationship between food environment and diet to date (e.g., with 511% more counties represented compared to BRFSS data 93 ).
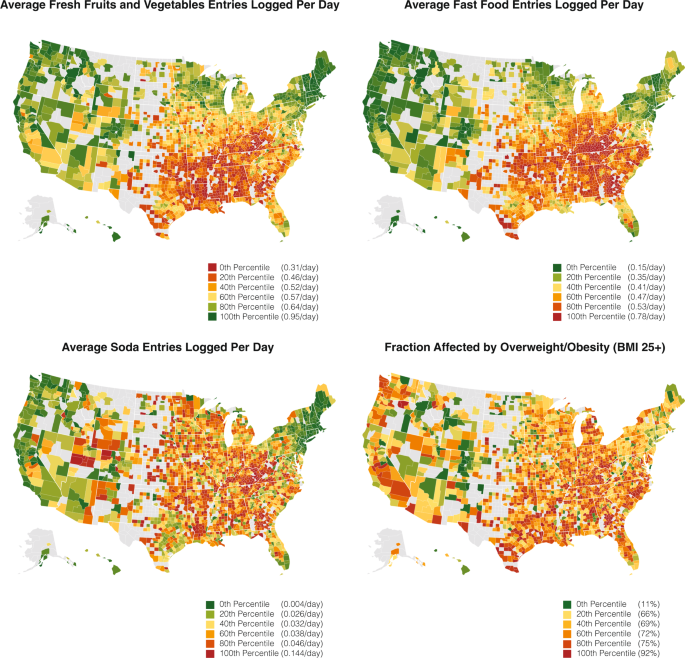
A set of choropleths showing the main study outcomes of the number of entries that are classified as fresh fruit and vegetables, fast food, and soda consumption as well as the fraction affected by overweight/obesity (BMI > 25) participants across the USA by counties with more than 30 participants. We observe that food consumption healthfulness varies significantly across counties in the United States.
Data validation: diet tracking data correlates with existing large-scale measures
To determine the ability of our dataset to identify relationships between fast food, grocery store access, income, educational attainment and diet health outcomes, we confirmed that this studies’ smartphone-based food logs correlate with existing large-scale survey measures and purchase data. Specifically, the fraction of fresh fruits and vegetables (F&V) that participants logged is correlated with Behavioral Risk Factor Surveillance System (BRFSS) survey data 43 (Fig. 3 a; Pearson Correlation R = 0.63, p < 10 −5 ; Two-sided Student’s t -test; Methods). Further, the reported BMI of MyFitnessPal (MFP) participants is correlated with BRFSS survey data 44 (Fig. 3 b; Pearson Correlation R = 0.78, p < 10 −5 ; Two-sided Student’s t -test; Methods). Lastly, the digital food logs data replicate previous findings of relative consumption differences in low-income, low-access food deserts based on Nielsen purchase data 45 (Fig. 3 c; Pearson Correlation R = 0.88, p < 0.01; Two-sided Student’s t -test; Methods). These results demonstrate that smartphone-based food logs are highly correlated with existing, gold-standard survey measures and purchase data.
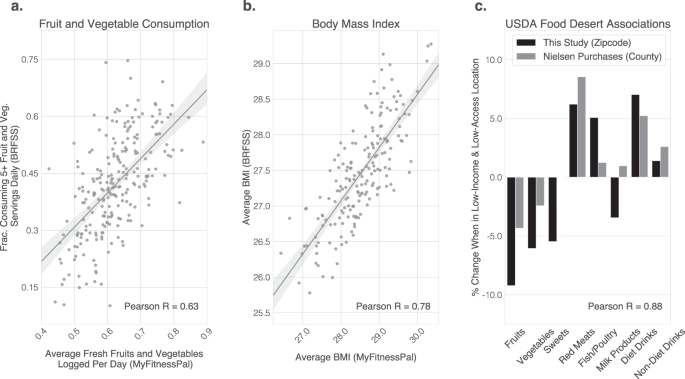
a Fraction of fresh fruits and vegetables logged is correlated with BRFSS survey data 43 (Pearson Correlation R = 0.63, p < 10 −5 ; Two-sided Student’s t -test; Methods). b Body-mass index of smartphone cohort is correlated with BRFSS survey data 44 (Pearson Correlation R = 0.78, p < 10 −5 ; Two-sided Student’s t -test; Methods). Lines in a , b show best linear fit along with shaded 95% bootstrap confidence intervals. c Digital food logs replicate previous findings of relative consumption differences in low-income, low-access food deserts based on Nielsen purchase data 45 (Pearson Correlation R = 0.88, p < 0.01; Two-sided Student’s t -test; Methods). These results demonstrate that smartphone-based food logs are highly correlated with existing, gold-standard survey measures and purchase data.
Associations between food environment, demographics and diet
Using these data across all 9822 U.S. codes, we found that high income, high educational attainment, high grocery store access, and low fast food access were independently associated with higher consumption of fresh F&V, lower consumption of fast food and soda, and lower prevalence of BMI levels categorized as overweight or obesity (Fig. 4 ; BMI > 25). The only exception to this pattern was a very slight (0.6%) positive difference in BMI levels categorized as overweight or obesity associated with income. Specifically, in zip codes of above median grocery store access participants logged 3.4% more F&V, 7.6% less fast food, 6.4% less soda and were 2.4% less likely to be affected by overweight or obesity (all P < 0.001). In zip codes of below median fast food access participants logged 5.3% more F&V, 6.2% less fast food, 13.3% less soda and were 1.5% less likely to be affected by overweight or obesity (all P < 0.001). In zip codes of above median education, participants logged 9.2% more F&V, 8.5% less fast food, 13.8% less soda and were 13.1% less likely to be affected by overweight or obesity (all P < 0.001). Finally, in zip codes of above median household income (referred to as higher income below), participants logged 3.3% more F&V, 6.8% less fast food, 8.6% less soda (all P < 0.001), but had a 0.6% higher likelihood of being affected by overweight or obesity ( P = 0.006). Note that the reported effect size are based on comparing above and below median zip codes for any given factor. We found a general pattern of consistent, and in many cases higher effect sizes when comparing top versus bottom quartiles (Supplementary Fig. 2 ), suggesting the possibility of a dose-response relationships across most considered variables. We found that zip codes with high educational attainment levels compared to low educational attainment levels had the largest relative positive differences across F&V, fast food, soda, and BMI levels categorized as overweight or obesity.
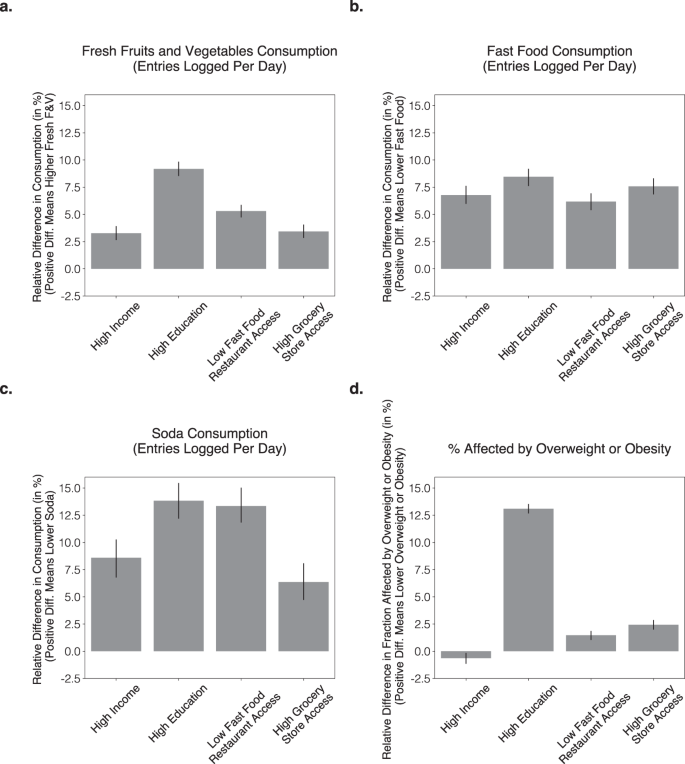
Independent contributions of high income (median family income higher than or equal to $70,241), high educational attainment (fraction of population with college education 29.8% or higher), high grocery store access (fraction of population that is closer than 0.5 miles from nearest grocery store is greater than or equal to than 20.3%), and low fast food access (less than or equal to 5.0% of all businesses are fast-food chains) on relative difference in consumption of a fresh fruits and vegetables, b fast food, c soda, and d relative difference in fraction affected by overweight or obesity (BMI > 25). Cut points correspond to median values. Y -axes are oriented such that consistently higher is better. Estimates are based on matching experiments controlling for all but one treatment variable, across N = 4911 matched pairs of zip codes (Methods). Bar height corresponds to mean values; error bars correspond to 95% bootstrap confidence intervals (Methods). While the most highly predictive factors vary across outcomes, only high educational attainment was associated with a sizeable difference of 13.1% in the fraction affected by overweight or obesity.
Significant differences across zip codes with predominantly Black, Hispanic, and Non-Hispanic white populations
We separately repeated our data analyses within zip codes with predominantly Black (3.7%), Hispanic (5.6%), and non-Hispanic white populations (78.4%) (Fig. 5 ). Results within zipcodes with predominantly non-Hispanic white populations closely resembled results within the overall population, since most zip codes in this study had predominantly white populations (78.4%; not unlike the overall U.S. population at 61.3%) 46 . However, restricting our analyses to zip codes with predominantly Black and Hispanic populations led to significantly different findings. Specifically, within zip codes with predominantly Black populations we found associations of higher income in the inverse direction of the population average and towards low healthful food consumption, across four out of four outcome variables, resulting in lower F&V consumption (−6.5%), higher fast food consumption (5.5%), and higher likelihood of BMI levels categorized as overweight or obesity (8.1%). Higher income was also associated with higher soda consumption (14.1%) but was not statistically significant ( P = 0.061). On the other hand, low fast food access and high educational attainment access were generally associated with higher diet health, with low fast food access correlating with the highest significant negative difference in fast food consumption (−12.0%) and high educational attainment with the highest positive difference in fresh fruit and vegetable consumption (11.2%), although lower fast food access was associated with worse outcomes for one of the outcome variables. Specifically, lower fast food access was associated with a slightly higher likelihood of being affected by overweight or obesity (3.1%). Higher grocery store access had a positive association with diet health across all outcome variables in zip codes with predominantly Black populations, and was associated with higher F&V consumption (10.2%), lower fast food consumption (12.6%), lower likelihood of BMI levels categorized as overweight or obesity (9.0%), and lower soda consumption (5.3%), although the association with soda consumption was not statistically significant ( P = 0.060).
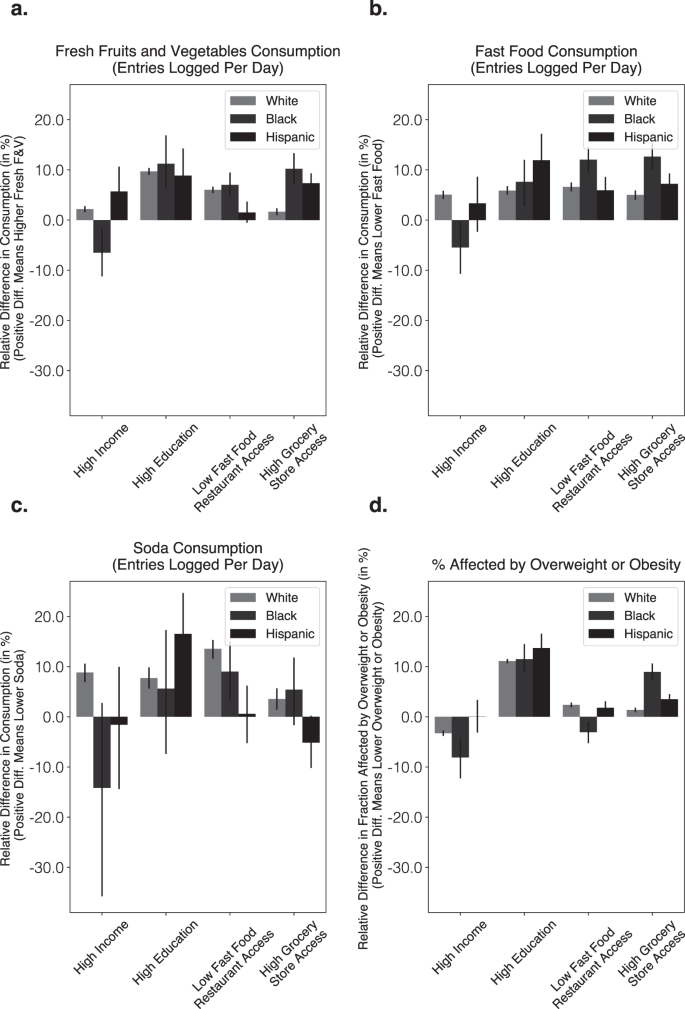
Independent contributions of high income (median family income higher than or equal to $70,241), high educational attainment (fraction of population with college education 29.8% or higher), high grocery store access (fraction of population that is closer than 0.5 miles from nearest grocery store is greater than or equal to than 20.3%), and low fast food access (less than or equal to 5.0% of all businesses are fast-food chains) on relative difference in consumption of a fresh fruits and vegetables, b fast food, c soda, and d relative difference in fraction affected by overweight or obesity (BMI > 25). Cut points correspond to median values. Y -axes are oriented such that consistently higher is better. Estimates are based on matching experiments controlling for all but one treatment variable, across N = 4277, 4102, 3510, 3205 matched pairs of non-Hispanic white-majority zip codes, treated on income, educational attainment, fast food access, grocery store access respectively; N = 42, 74, 259, 259 matched pairs of Black-majority zip codes, treated on income, educational attainment, fast food access, grocery store access respectively; N = 67, 61, 297, 471 matched pairs of Hispanic-majority zip codes, treated on income, educational attainment, fast food access, grocery store access respectively (Methods). Bar height corresponds to mean values; error bars correspond to 95% bootstrap confidence intervals (Methods). We observe significant differences in outcomes between zip codes with predominantly Black, Hispanic, and non-Hispanic white populations.
In contrast, within zip codes with predominantly Hispanic populations we found a significant association between higher, above-median, income and higher F&V consumption (5.7%), but not with the remaining three outcome variables. Zip codes with higher proportion of people with high educational attainment had the most positive association with diet health across all variables. Specifically, higher educational attainment was associated with higher F&V consumption (8.9%), lower fast food consumption (11.9%), lower soda consumption (16.5%), and lower likelihood of BMI levels categorized as overweight or obesity (13.7%). Higher grocery store access and lower fast food access had similar effect sizes as on the overall population for some outcome variables (i.e. similar associations with likelihood of BMI levels categorized as overweight or obesity and fast food consumption). However, in some cases the magnitude of association was higher (i.e. grocery store access was associated with 7.4% higher F&V consumption in areas with predominantly Hispanic population, which is more than twice than the difference within the overall population) and in others, unlike the overall population, there was no significant association (i.e. no significant relationship between fast food access on soda consumption, or between fast food access and F&V consumption).
Few factors were consistently associated with better outcomes across all three subpopulations. Across all three groups, F&V consumption was significantly higher in zip codes with high grocery store access and high educational attainment. Fast food consumption was lower across all potential intervention targets besides higher income. Soda consumption was lowest most with lower fast food access for Black and white-majority zip codes, whereas it was lowest with higher educational attainment in Hispanic zip codes. Lastly, BMI levels categorized as overweight or obesity were far lower with higher educational attainment levels compared to all other intervention targets, across all three groups.
Commercially available and widely used mobile applications and devices enable the individuals to track their own health, and in aggregate may inform our understanding of population health. These emerging data sources capture health behaviors from millions of participants 26 and have uniquely enabled large-scale research studies, including in physical activity 27 , 28 , sleep 29 , 30 , COVID-19 pandemic response 31 , 32 , 33 , women’s health 34 , as well as diet research 35 , 36 , 37 , 38 , 39 , 40 .
While many of our results were consistent with previous studies 47 , 48 , 49 , importantly, we found that zip codes with higher proportion of people with high educational attainment had the largest relative difference in the likelihood of BMI levels categorized as overweight or obesity (13.1% lower). It is well established that social determinants of health are linked to obesity 50 , 51 , 52 . As an important component of social determinants of health, our study suggests that having higher educational attainment is the most predictive of reduced overweight and obesity for all ethnicities.
When we restricted our analyses to zip codes with predominantly Black, Hispanic, and non-Hispanic white populations, we found the independent associations of food access, income and educational attainment with food consumption and BMI status varied significantly across these three groups. These findings suggest that tailored intervention strategies are needed based on neighborhood population distributions, assets and contexts.
Within zip codes with predominantly Black populations, the association between having higher income and diet health was negative. Having higher income was associated with lower F&V consumption, higher fast food and soda consumption, and higher likelihood of overweight and obesity. This could be explained by the “diminishing return hypothesis”, which suggests that Black people receive fewer protective health benefits from increases in SES than white people 53 , 54 . A combination of factors, including neighborhood economic disadvantage 55 , 56 , racial/ethnic discrimination 57 , 58 , and stress associated with educational attainment and mobility 59 , may prevent Black people from higher SES backgrounds from achieving their fullest health potential relative to white people 60 .
Within zip codes with predominantly Hispanic populations, higher income was not associated with lower likelihood of BMI levels categorized as overweight or obesity. The absence of a relationship between higher income and BMI, compared to in zip codes with predominantly Black and non-Hispanic white populations, could be partially explained by the “Hispanic health paradox” and “Hispanic health advantage” 61 , 62 , 63 , 64 , 65 , 66 . The Hispanic health paradox suggests that even though the first-generation Hispanic people have lower SES, they experience better health outcomes including lower prevalence of cardiovascular diseases, asthma, diabetes and cancer compared to those who were U.S.-born 61 , 62 , 63 . Hispanic health advantage suggests that Hispanic people have lower rates of harmful health behaviors, such as smoking, which in turn positively influence other health outcomes compared to non-Hispanic white people 61 , 64 , 65 , 66 . Additionally, through acculturation or adopting American culture, Hispanic immigrants may engage in less healthy behaviors, which in turn put themselves at higher risk for chronic diseases 61 , 62 , 63 , 67 , 68 , 69 , 70 , 71 .
While it is challenging to close the education and income gaps, establishing more grocery stores and limiting fast food restaurant access may help improve diet health across the population. Previous reviews suggested that government policies that addressing food affordability and purchase, such as the Healthy Food Financing Initiative (HFFI), increasing food stamp (SNAP) benefit and provide incentives to create healthy retail food environment have been effective in reducing food insecurity and dietary behaviors 72 , 73 , 74 , 75 , 76 , 77 . While several studies showed that the establishments of new supermarkets had little improvement in BMI 78 , 79 , 80 ; however, the investments in the new supermarkets have improved economic opportunity and social cohesion 81 , 82 , 83 . Our results showed that higher grocery store access was associated with 2–3 times higher fresh fruit and vegetable consumption and lower fast food consumption more for Black people than for white people. Although previous literature has shown null effects of grocery store access 84 , 85 , these studies have focused on the general population, which is white-skewed. Therefore, policies and strategies in increasing grocery store access and decreasing fast food access could potentially be the most effective approaches in changing dietary habits among locations with predominantly Black populations.
Furthermore, having more grocery store access and lower fast food access, in the food environment may work in synergistic ways that may lead to even lower obesity prevalence and obesity-related lifestyle and behavior changes. This is demonstrated in a recent study by Cantor et al. that HFFI boosted the effects of SNAP participation on improving food security and healthy food choices in food desserts 86 . This synergy could be multiplied when combining with effective education programs that could potentially lower obesity prevalence further by increasing individuals’ SES (e.g., income and educational attainment) 87 , 88 , health literacy and behaviors 87 , 88 , 89 , 90 , 91 , as well as sense of control and empowerment 92 .
Due to the cross-sectional nature of the study, we were not able to make any causal inferences between SES, food environment variables, dietary behavior, and BMI, as unobserved neighborhood and individual demographic and social characteristics could lead to confounding. However, we used a matching-based approach to mimic a quasi-experimental design to disentangle the individual associations of income, educational attainment and food access with participants’ food consumption. Our analysis did not include other demographic variables such as gender and age, as both variables were naturally balanced across treatment and control groups and we observed minimal zipcode-level correlations between age/gender and any of our four outcome measures (Supplementary Table 13 ). In addition, we confirmed that results were virtually identical (Pearson Correlation R = 0.95), when explicitly controlling for age and gender in our matching-based approach. However, we jointly considered the potential impacts of neighborhood income, neighborhood educational attainment and food environment access on participants’ food consumption with consistent measures across the U.S., whereas previously published studies examined one or a few at a time. Our study population, based on a sample of MFP users, is an imperfect representation of the United States national population. Comparing our study population to nationally representative survey data, we found that our study population had significant overlap with the U.S. national population in terms of population demographics, educational attainment and BMI status, but that it was skewed towards women and higher income (Supplementary Table 3 ). We used individuals’ food loggings to estimate their consumption (specifically, the number of food entries as the logged amount consumed varied highly across foods without standardization; e.g., specifying weight, volume, or number). Food loggings may not capture what individuals actually ate and participants may be particularly motivated or care about their diet and weight. Importantly, we conducted multiple validation experiments through comparisons with high quality and highly representative datasets which demonstrated high correlations to gold-standard approaches (Fig. 3 ). The majority of food environment studies used screeners, food frequency questionnaires or 24-h recalls for dietary assessment, and very few used diaries 9 . In contrast, our participants logged their food intakes for an average of 197 days each. We also harnessed other large datasets such as Yelp to examine participants’ food environments. Considering both the strengths and limitations of this study, more research is needed especially based on longitudinal study design and detailed individual level data to enable causal inference and precise interpretation of the results.
In conclusion, we analyzed 2.3 billion food intake logs and BMIs from 1.2 million MFP smartphone app participants over 7 years across 9822 zip codes in relation to educational attainment, ethnicity, income, and food environment access. Our analyses indicated that higher access to grocery stores, lower access to fast food, higher income and educational attainment were independently associated with higher consumption of fresh F&V, lower consumption of fast food and soda, and lower likelihood of being affected by overweight or obesity, but that these associations varied significantly across zip codes with predominantly Black, Hispanic and white subpopulations. Policy targeted at improving food access, income and education may increase healthy eating. However, intervention allocation may need to be optimized for specific subpopulations and locations.
Study design and population
We conducted a United States countrywide cross-sectional study of participants’ self-reported food intake and BMI in relation to zip code level demographic (educational attainment, ethnicity), socioeconomic (income), and food environment factors (grocery store and fast food access) by combining datasets from MFP, US Census, USDA and Yelp.
Overall, this cross-sectional matching-based study analyzed 2.3 billion food intake logs from U.S. smartphone participants over 7 years across 9822 zip codes, which is 24% of overall USA zip codes (U.S. has a total of 41,692 zip codes). Participants were users of the MFP app, a free application for tracking caloric intake. We analyzed anonymized, retrospective data collected during a 7-year observation period between 2010 and 2016 that were aggregated to the zip code level. Comparing our study population to nationally representative survey data, we found that our study population had significant overlap with the U.S. national population in terms of population demographics, educational attainment and BMI status, but that it was skewed towards women and higher income (Supplementary Table 3 ). Our matching-based statistical methodology controls for observed biases between comparison groups in terms of income, educational attainment, grocery store access, and fast food access (Methods: Statistical Analysis). Data handling and analysis was conducted in accordance with MFP policies and with the guidelines of the Stanford University Institutional Review Board.
Study data: MyFitnessPal
We compute outcome measures of food consumption and BMI status from 2.3 billion food intake logs by a sample of 1,164,926 U.S. participants of the MFP smartphone application to quantify food consumption across 9822 zip codes. The scale and geographic distribution of our study participants, as well as our outcome measures, are illustrated in Figs. 1 and 2 respectively. To ensure participant privacy as well as reliability of our measures, we decided to only include zip codes in which we had access to 30 or more participant food logs, which reduced the dataset size from 27,027 zip codes (spanning 3117 counties) to the final 9822 zip codes (spanning 1730, or 55% of all counties in the United States). Nevertheless, the geographical breadth of this dataset far exceeds existing food surveys. For example, our final dataset contained 511% more counties than the BRFSS survey of 283 counties, with 370% more participants per county on average 93 . While size and coverage compare favorably to BRFSS, it is important to understand what is not covered by our study. Figure 1 illustrates that our study lacks representation in the Midwest of the USA as well as in Alaska. In our study data, we further observed under-representation of zip codes with majority non-white population (Supplementary Table 3 ) and rural zip codes (RUCA codes 7 through 10 94 , 95 ), as well as over-representation of high-income zip codes (median family income higher than $70,241).
During the observation period from January 1, 2010 to November 15, 2016, the average participant logged 9.30 entries into their digital food journal per day. The average participant used the app for 197 days. All participants in this sample used the app for at least 10 days. We classified the 2.3 billion food intake entries into three categories of public health interest, fresh F&V, fast food, and sugary non-diet soda, and excluded them from analysis if they did not match these categories. Our classification method is consistent with USDA MyPlate with one divergence of the exclusion of juices. The healthiness of juice as a fruit and vegetable serving is contested due to its sugar content and limited nutritional profile 96 , 97 , 98 . For more details on the definition of a food entry, our classification method, and the choice of outcome measure, see Details on outcome measures subsection in Methods.
We intentionally use a cross-sectional rather than longitudinal study design, since fine-grained and large-scale temporal data on changes in the food environment were not available.
Study data: demographic and socioeconomic factors
We obtained data on demographic and socioeconomic factors from CensusReporter 99 . Specifically, for each zip code in our data set we obtained median family income, fraction of population with college education (Bachelor’s degree or higher), and fraction of population that is white (not including Hispanic), Black, or Hispanic from the 2010 to 2014 American Community Survey’s census tract estimates 99 . While data were available only on zip code level, previous studies have shown that area-level income measures are meaningful for health outcomes and describe unique socioeconomic inequities 100 .
Study data: grocery store and fast food access
Grocery store access was defined as the fraction of population that is more than 0.5 miles away from a grocery store following the food desert status definitions from the USDA Food Access Research Atlas 101 . Contrary to the USDA definition, we found evidence that even in rural zipcodes, the fraction of population greater than 0.5 miles away from grocery stores has the strongest association with food consumption (compared to 10 and 20 miles away), and thus we used 0.5 miles as the threshold across rural and urban zipcodes (Methods: Details on food environment measures). We measured fast food access through the fraction of restaurants that are fast food restaurants within a sample from Yelp, querying the nearest 1000 businesses from the zip code’s center, up to a maximum radius of 40 km (25 miles). See subsections Data Validation and reproducing State-of-the-art Measures using Population-scale Digital Food Logs for details and validation of these objective food environment measures.
We release all data aggregated at zipcode level in order to enable validation, follow-up research, and use by policy makers.
Details on food environment measures
We obtained data on grocery store access (fraction of population that is more than 0.5 miles away from grocery store) and food desert status from the USDA Food Access Research Atlas 101 . A census tract is considered a food desert by the USDA if it is both low-income (defined by Department of Treasury’s New Markets Tax Credit program) and low-access, meaning at least 500 people or 30 percent of residents live more than 0.5 miles from a supermarket in urban areas (10 miles in rural areas) 45 .
Although the USDA uses different thresholds for urban and rural areas (0.5 and 10 miles respectively), we found that even in rural zipcodes (defined as USDA rural-urban continuum RUCA scores of 7 through 10 94 , 95 ), the fraction of population that is farther than 0.5 miles from grocery stores had the highest correlation to fruit and vegetable consumption (Pearson Correlation R = −0.20), compared to 1 miles (Pearson Correlation R = −0.17), 10 miles ( R = −0.05), and 20 miles (Pearson Correlation R = 0.03). This suggests that the fraction of the population farther than 0.5 miles from a grocery store has the strongest relationship with healthy food consumption, even in rural zipcodes . Hence, we decided used 0.5 miles distance as a standard measure of grocery store access for rural and urban zip codes, contrary to the USDA definition. We subsequently sanity checked for any downstream confounding of urbanicity in our primary matching experiment of above/below median grocery store access, and found a negligible difference (Standardized Mean Difference (SMD) of 0.18) in urbanicity between control and treatment, suggesting that the effect size was not due to grocery store distance functioning as a proxy for urbanicity, but rather directly due to differential grocery store access.
We aggregated these data from a census tract level to a zip code level using USPS Crosswalk data, which provides a list of all census tracts which overlap with a single zip code 102 . We related these data on census tract level to the zip code level by taking the weighted average of each census tract food environment measure (both grocery store access and food desert status), weighted by the number of people in the tract 102 . For instance, if zip code A overlapped with Census Tract A (2500 people, food desert) and Census Tract B (7500 people, not a food desert), the food desert measure of zip code A would be estimated as 25%. We defined the binary threshold for food desert, used in Fig. 3 , as 50% or higher.
We measured fast food access through the fraction of restaurants in a zip-code that are fast food restaurants. Data on local restaurants and businesses were obtained through the Yelp API 103 . For each zip code, we consider up to 1000 restaurant businesses that are nearest to the zip code center up to a distance of 40 km (67.8% of zip code queries resulted in 1000 restaurant businesses within 40 km; Yelp API results are restricted to 1000 results). This resulted in a varying sample radius depending on urbanicity. For example, Urban zipcodes (RUCA code of 1) had an average effective centroid size of 15 miles, which we calculated by taking the distance from the zipcode center to the furthest restaurant returned by Yelp. We further used Yelp-based environment variables that we expected not to influence food consumption, such as the availability of waterproofing services, countertop installers, or electronic stores, as null experiments to demonstrate discriminant validity of our statistical analysis pipeline (see Supplementary Fig. 3 ).
Details on outcome measures (food consumption and BMI status)
We used 2.3 billion food intake logs by a sample of 1,164,926 U.S. participants of the MFP smartphone application to quantify food consumption across 9822 zip codes. During the observation period from January 1, 2010 to November 15, 2016, the average participant logged 9.30 entries into their digital food journal per day. The average participant used the app for 197 days. All participants in this sample used the app for at least 10 days.
Clustering of food consumption observations within individuals and zip codes was handled through multiple levels of aggregation. First we aggregated within participant and day (i.e., someone eating a banana at breakfast and another for dinner), then we aggregated across all days with tracking within each participant, and then across all participants within one zip code. We computed non-parametric confidence intervals and p -values through bootstrapping with 1000 replications on zip code level (last level of aggregation) 104 .
The unit of analysis for each zipcode was the average number of daily entries per person. An entry is a single food consumption event logged in the app MFP. Each entry contains a separate food component (e.g., banana, yogurt, hamburger, ...), brand name (e.g., “Campbells”), description (e.g., “Chicken Soup”), serving size unit (e.g., “cup”), and number of servings (e.g., “1”). Supplementary Fig. 1 shows the application interface for logging a food entry (e.g., 1 Banana from Whole Foods). We decided to use entries based on the observation that there was little variance in the number of servings per food category logged by participants in a single entry, and since the amount consumed varied highly across foods without standardization (e.g., specifying weight, volume, or number). Participants typically log “standard portion sizes” of each food individually (e.g., one bowl of cereal, one banana) on the MFP app. For example, for participants that logged a banana, and listed the serving size as “Banana”, the median entry was for 1 banana, the mean was for 0.88 bananas, and 95% of food entries were for between 0.5 to 1.5 bananas. The MFP app strongly encourages this behavior through a large library of foods to log that follows these standard portion sizes.
We classified all entries into three categories using brand name and description, and three separate binary classifiers: fresh F&V (through a proprietary classifier by MFP which used key words in the brand name and description), fast food (if the brand name contained the name of a fast food chain listed in Supplementary Table 8 , and sugary (non-diet) soda (if the brand name contained the name of a soda drink listed in Supplementary Table 9 and the description did not contain “diet”, “lite”, “light”, or “zero”). In all cases, descriptions, as well fast food and soda drink keywords, were normalized by lower-casing and removing punctuation. Each binary classifier thus took a food entry as input (i.e., “Coca Cola, Diet Cherry Coke, 8oz”) and outputted a binary label (i.e., soda or NOT soda). Entries which were predicted to be in none of the three categories based on all three models were excluded from the study.
Our classification method for fresh F&V is consistent with USDA MyPlate. The only divergence from USDA MyPlate is that we intentionally excluded juices, for which MFP has a separate classifier, which does not separate sweetened juice drinks or sports drinks and 100% juice. For our definition of Fresh F&V, we chose to exclude juices because the healthiness of juice as a fruit and vegetable serving is contested 96 , 98 , as even 100% fruit juices are typically high in sugar and calories, and low in fiber, and vegetable juices are often mixed with other high-sugar ingredients. We thus took a conservative approach to estimating diet healthiness by excluding these food entries.
We evaluated the accuracy of each of the three binary classification model by estimating the precision (# True Positive / # Predicted Positive) from a random sample of 50 entries belonging to each category. Precision estimates are summarized in Supplementary Table 1 , and Supplementary Tables 10 , 11 , and 12 show random samples of 50 food items from all elements predicted to be in each category (where asterisk “*” indicates an incorrect prediction). Note that across 2.3 billion food logs it was not possible to measure recall, but were able to measure precision by manually inspecting the food entry brand and description and assigning it a category.
We then calculated the average number of food entries logged per participant, per day, for each of the F&V, fast food, and soda categories (e.g. average number of F&V logged per participant per day), excluding days in which the participant was inactive (i.e., consistently did not log anything). Finally, we aggregated these participant-level measures to the zip code level by taking the mean of each category’s measure for all participants in each zip code. We further used BMI health in each zip code as a BMI status outcome, specifically the fraction of participants in a zip code which are affected by overweight or obesity (BMI > 25). BMI was self-reported by participants of the smartphone application (99.92% of participants did report BMI). Supplementary Table 2 shows basic summary statistics for the outcome measures used in this study. In our statistical analyses, we compared two sets of zip codes that differ in a dimension of interest (e.g., grocery store access access) as treatment and control group and use the relative difference in F&V consumption, fast food consumption, soda consumption, and BMI health of the treated group relative to the control group. To generate confidence intervals, as well as to compute p -values to test for statistical significance of differences in outcome, we use non-parametric bootstrap resampling with 1000 replications 104 . Specifically, we follow the method proposed by Austin and Small 105 , which is to draw bootstrap samples post-matching from the matched pairs in the propensity-score-matched sample after the Genetic Matching stage 106 . We confirmed the validity of this method empirically by also calculating t -tests for each experiment, which gave qualitatively similar results. We note that we perform bootstrapping on zip code level (highest level of aggregation). While, multilevel bootstrapping methods exist, they do not scale well with our dataset size of 2.3 billion food items. However, due to the large number of 9822 zip codes our analyses are well-powered statistically even with bootstrapping at zip code level.
Data validation
We find that our study population has significant overlap with the U.S. national population (Supplementary Table 3 ) but is skewed towards women and higher income. We demonstrate that food consumption measured based on this population are highly correlated with state-of-the-art measures (Fig. 3 ). Smartphone apps such as MFP feature large databases with nutritional information and can be used to track one’s diet over time. Previous studies have compared app-reported diet measures to traditional measures including 24-h dietary recalls and food composition tables. These studies found that both measures tend to be highly correlated 107 , 108 , but that app-reported measures tend to underestimate certain macro- and micronutrients 107 , 108 , especially in populations that were previously unfamiliar with the smartphone applications 109 . In contrast, this study leverages a sample of existing participants of the smartphone app MFP. Yelp data has been used in measures of food environment 110 and a study in Detroit found Yelp data to be more accurate than commercially available databases such as Reference USA 111 . This study uses a combination of MFP data to capture food consumption, Yelp, and USDA data to capture food environment, and Census data to capture basic demographics. As a preliminary, basic test, we investigated correlations between the Mexican food consumption, the fraction of Mexican restaurants, and the fraction of Hispanic people in the population, on a zip code level. We found that Mexican food consumption (entries labeled as Mexican food by a proprietary MFP classifier, logged per participant, per day) was correlated with the fraction of Mexican restaurants (Pearson Correlation R = 0.72; < 10 −4 ) and the fraction of Hispanic people in the population (Pearson Correlation R = 0.54; P < 10 −4 ). Further, the fraction of Mexican restaurants was correlated with the fraction of Hispanic people in the population as well (Pearson Correlation R = 0.51; P < 10 −4 ).
Reproducing state-of-the-art measures using population-scale digital food logs
A primary concern in studying diet health via food logs is the bias inherent to the MFP population, which is not a representative sample of the US population. To investigate the applicability of population-scale digital food logs to study the relationship between food environment, income and educational attainment with food consumption, we measured the correlation between our smartphone app-based measures and state-of-the-art measures of food consumption including the BRFSS, based on representative surveys of over 350,000 adults in the United States 43 , 44 , and the Nielsen Homescan data 112 , which is a nationally representative panel survey of the grocery purchases of 169,000 unique households across the United States, based on UPC records of all consumer packaged goods participants purchased from any outlet (Fig. 3 ). We used the latest survey data from BRFSS 43 , 44 available at the county-level. Specifically, we used variables FV5SRV from BRFSS 2011 representing the faction of people eating five or more servings of fresh fruit and vegetables 43 , and BMI5 from BRFSS 2012 representing BMI 44 . We compare against BRFSS rather than National Health and Nutrition Examination Survey (NHANES), since BRFSS is significantly larger than NHANES, it is remotely administered matching our study, and it has much better geographical coverage than NHANES and geographical comparisons are central to our study. Despite these advantages, no reference dataset is without limitations 113 , 114 , 115 , motivating this study’s use of large-scale digital food journals.
Comparing our data to BRFSS on county level, we found moderate to high correlations between the amount of fresh F&V consumed (Fig. 3 a, Pearson Correlation R = 0.63, p < 10 −5 ) and BMI (Fig. 3 b, Pearson Correlation R = 0.78, p < 10 −5 ). We further compared to published results by the USDA 45 , which used data from the 2010 Nielsen Homescan Panel Survey that captured household food purchases for in-home consumption (but did not capture restaurants and fast food purchases). We attempted to reproduce published findings on the differences in low-income, low-access communities (food deserts) compared to non-low-income, non-low-access communities 45 across categories of fruit, vegetable, sweets, red meat, fish/poultry, milk products, diet drinks, and non-diet drinks (Table 4 in Rahkovsky and Snyder 45 ). We used proprietary MFP classifiers to categorize foods logged into these categories. We found that our app-based food logs were very highly correlated with previously published results (Fig. 3 c, R = 0.88, p < 0.01) and that the absolute differences between food deserts and non-food deserts were stronger in the MFP data compared to Nielsen purchase data. Overall, these results demonstrate convergent validity and suggest that the employed non-representative sample of population-scale digital food logs can reproduce the basic dynamics of traditional, state-of-the-art measures, and they can do so at massive scale and comparatively low cost.
Statistical analysis
In this large-scale observational study, we used a matching-based approach 116 , 117 to disentangle contributions of income, educational attainment, grocery store access, and fast food access on food consumption. We considered multiple statistical strategies, including regression modeling and propensity score matching. We decided to employ a full matching on all variables, which avoids parametric assumptions and is a more conservative method for matching than for example propensity score-based techniques 117 . To estimate the treatment effects of each of these factors, we divided all available zip codes into treatment and control groups based on a median split; that is, we estimated the difference in outcomes between matched above-median and below-median zip codes. We created matched pairs of zip codes by selecting a zip code in the control group that is closely matched (i.e., less than 0.25 SMD between the treated and control groups) 117 to the zip code in the treatment group across all factors, except the treatment factor of interest. Since we repeated this matching process for each zip code in the treatment group, this approach estimated the Average Treatment Effect on the Treated (ATT). Through this process, we attempted to eliminate variation of plausible influences and to isolate the effect of interest. We repeated this process for each treatment of interest; for example for the results presented in Fig. 4 , we performed four matchings, one for each of income, educational attainment, grocery store access and fast food access. For the sub-population experiments (Fig. 5 ), we repeated the same method on the subset of the zip codes in which the majority of inhabitants were of a particular ethnic group. Lastly, although we considered controlling for age and gender in the matching procedure, as these are related to diet health at the individual-level, we did not include them in our final analysis after observing (1) minimal zipcode-level correlations between age/gender and any of our four outcome measures (Supplementary Table 13 ; largest Pearson Correlation was 0.12) and (2) virtually identical results (Pearson Correlation R = 0.95) when comparing before and after controlling for age and gender by adding them the genetic matching algorithm. See subsection on Details on Matching Approach for further details and statistics that demonstrate that treatment and control groups were well-balanced on observed covariates after matching.
We tested discriminant validity of our statistical approach by measuring the effect of null-treatments that should not have any impact on food consumption. We chose examples of null-treatments by selecting variables that had little correlation with study independent variables (income, educational attainment, grocery store access, fast food access) and were plausibly unrelated to food consumption. This selection process lead to use of the fraction of countertop installers, electronics stores, and waterproofing services nearby as measured through Yelp. Applying our analysis pipeline to these null-treatments, we found that all of these null-treatments had zero effect on food consumption. This demonstrated that our statistical analysis approach did not produce measurements that it was not supposed to measure; that is, discriminant validity (Supplementary Fig. 3 and Supplementary Table 7 ).
Details on matching approach
Specifically, we use a one-to-one Genetic Matching approach, 106 with replacement, and use the mean of the SMD between treatment and control groups, across all matched variables, as the Genetic Matching balance metric in order to maximize balance (overlap) between the treated and the control units. Some definitions of SMD use the standard deviation in the overall population before matching 116 . However, we choose the standard deviation in the control group post-matching, which typically is much smaller and therefore gives more conservative estimates of balance between treated and control units 118 .
After matching, we evaluated the quality of balance between the treated and the control units by the SMD across each of the variables that were controlled for and included in the matching process. A good balance between treated and control groups was defined as a SMD of less than 0.25 standard deviations 117 across each variable. By default, we do not enforce a caliper in order to minimize bias in matching process, although in rare cases in which a good balance was not achieved, a caliper was enforced, starting at 2.5 standard deviations between matched and controlled units, and decreased by 0.1 until the matched and control groups had a SMD smaller than 0.25 across all matched variables.
For the vast majority of matching experiments the SMD across all matched variables was well below 0.25, with a mean of 0.040 and median of 0.016 for the four overall population matching experiments. The SMD for the ethnicity-majority zipcode experiments was slightly higher, but still very significantly below 0.25 across all 12 experiments, with a mean of 0.055 and median of 0.036. Thus, no caliper was necessary to ensure a good balance, with the exception of one out of the 12 of sub-population experiments (white, high educational attainment). Detailed balancing statistics for each of the matches are available in the Supplementary Information (Supplementary Tables 14 – 36b ), as well as a supplementary matching experiment in which a top/bottom quartile split was used instead of a median split (Supplementary Fig. 2 ).
Details on the use of zip codes
A zip code is a postal code used by the US Postal Services. Zip codes consist of 5 digits and were introduced in their current form in 1983 in order to provide granular demarcations of US geography for mail purposes 119 . Most previous surveys such as BRFSS aggregate individuals at the less fine-grained levels of granularity: city, county, or MSA (Metropolitan statistical area) level. By contrast, we chose to use zip codes in order to study diet health and obesity at a more fine-grained level of analysis. As a point of reference, there are currently 41,692 zip codes in the USA compared to 3143 counties and county equivalents (i.e., 13.2 zip codes per county on average). Zip codes are on average 91 square miles and contain 7872 people 120 , compared to counties and county-equivalents which are on average 1208 square miles and contain 104,422 people 121 . Neighboring zip codes which may be in the same county have sharply contrasting demographics 122 . A zip code-level analysis better enables us to measure the disparate impacts of educational attainment, income, and food environment on diet health and obesity, and to stratify our analyses by ethnicity.
The content is solely the responsibility of the authors and does not necessarily represent the official views of the NIH or sponsors.
Reporting summary
Further information on research design is available in the Nature Research Reporting Summary linked to this article.
Data availability
Data are available at http://snap.stanford.edu/dietdisparities .
Code availability
Code is available at http://snap.stanford.edu/dietdisparities .
Afshin, A. et al. Health effects of dietary risks in 195 countries, 1990–2017: a systematic analysis for the global burden of disease study 2017. Lancet 393 , 1958–1972 (2019).
Article Google Scholar
Micha, R. et al. Association between dietary factors and mortality from heart disease, stroke, and type 2 diabetes in the united states. JAMA 317 , 912–924 (2017).
Article PubMed PubMed Central Google Scholar
Jayedi, A., Soltani, S., Abdolshahi, A. & Shab-Bidar, S. Healthy and unhealthy dietary patterns and the risk of chronic disease: an umbrella review of meta-analyses of prospective cohort studies. Br. J. Nutr. 124 , 1133–1144 (2020).
Article CAS PubMed Google Scholar
Beaglehole, R. et al. Priority actions for the non-communicable disease crisis. Lancet 377 , 1438–1447 (2011).
Article PubMed Google Scholar
Fleischer, N. L., Roux, A. V. D., Alazraqui, M. & Spinelli, H. Social patterning of chronic disease risk factors in a latin american city. J. Urban Health 85 , 923 (2008).
Sallis, J. F. & Glanz, K. The role of built environments in physical activity, eating, and obesity in childhood. Future Child. 16 , 89–108 (2006).
Fleischhacker, S. E., Evenson, K. R., Rodriguez, D. A. & Ammerman, A. S. A systematic review of fast food access studies. Obes. Rev. 12 , e460–e471 (2011).
Odoms-Young, A., Singleton, C. R., Springfield, S., McNabb, L. & Thompson, T. Retail environments as a venue for obesity prevention. Curr. Obes. Rep. 5 , 184–191 (2016).
Kirkpatrick, S. I. et al. Dietary assessment in food environment research: a systematic review. Am. J. Preventive Med. 46 , 94–102 (2014).
Caspi, C. E., Sorensen, G., Subramanian, S. & Kawachi, I. The local food environment and diet: a systematic review. Health Place 18 , 1172–1187 (2012).
Feng, J., Glass, T. A., Curriero, F. C., Stewart, W. F. & Schwartz, B. S. The built environment and obesity: a systematic review of the epidemiologic evidence. Health Place 16 , 175–190 (2010).
Cummins, S. & Macintyre, S. Food environments and obesity-"neighbourhood or nation? Int. J. Epidemiol. 35 , 100–104 (2006).
Charreire, H. et al. Measuring the food environment using geographical information systems: a methodological review. Public Health Nutr. 13 , 1773–1785 (2010).
Kelly, B., Flood, V. M. & Yeatman, H. Measuring local food environments: an overview of available methods and measures. Health Place 17 , 1284–1293 (2011).
McKinnon, R. A., Reedy, J., Morrissette, M. A., Lytle, L. A. & Yaroch, A. L. Measures of the food environment: a compilation of the literature, 1990–2007. Am. J. Preventive Med. 36 , S124–S133 (2009).
Elinder, L. S. & Jansson, M. Obesogenic environments–aspects on measurement and indicators. Public Health Nutr. 12 , 307–315 (2009).
PubMed Google Scholar
Gittelsohn, J. & Sharma, S. Physical, consumer, and social aspects of measuring the food environment among diverse low-income populations. Am. J. Preventive Med. 36 , S161–S165 (2009).
Gustafson, A., Hankins, S. & Jilcott, S. Measures of the consumer food store environment: a systematic review of the evidence 2000–2011. J. Community health 37 , 897–911 (2012).
Lytle, L. A. Measuring the food environment: state of the science. Am. J. Preventive Med. 36 , S134–S144 (2009).
Odoms-Young, A. M., Zenk, S. & Mason, M. Measuring food availability and access in african-american communities: implications for intervention and policy. Am. J. Preventive Med. 36 , S145–S150 (2009).
Ohri-Vachaspati, P. & Leviton, L. C. Measuring food environments: a guide to available instruments. Am. J. Health Promotion 24 , 410–426 (2010).
Sharkey, J. R. Measuring potential access to food stores and food-service places in rural areas in the us. Am. J. Preventive Med. 36 , S151–S155 (2009).
Kamphuis, C. B. et al. Environmental determinants of fruit and vegetable consumption among adults: a systematic review. Br. J. Nutr. 96 , 620–635 (2006).
CAS PubMed Google Scholar
Lytle, L. A. & Sokol, R. L. Measures of the food environment: a systematic review of the field, 2007–2015. Health Place 44 , 18–34 (2017).
Kumanyika, S. K. Environmental influences on childhood obesity: ethnic and cultural influences in context. Physiol. Behav. 94 , 61–70 (2008).
Hicks, J. L. et al. Best practices for analyzing large-scale health data from wearables and smartphone apps. NPJ Digital Med. 2 , 1–12 (2019).
Althoff, T., Hicks, J. L., King, A. C., Delp, S. L. & Leskovec, J. Large-scale physical activity data reveal worldwide activity inequality. Nature 547 , 336–339 (2017).
Article ADS CAS PubMed PubMed Central Google Scholar
Althoff, T., White, R. W. & Horvitz, E. Influence of pokémon go on physical activity: study and implications. J. Med. Internet Res. 18 , e315 (2016).
Althoff, T., Horvitz, E., White, R. W. & Zeitzer, J. Harnessing the web for population-scale physiological sensing: a case study of sleep and performance. In Proceedings of the 26th international conference on World Wide Web . 113–122 (International World Wide Web Conferences Steering Committee, 2017).
Althoff, T., Horvitz, E. & White, R. W. Psychomotor function measured via online activity predicts motor vehicle fatality risk. NPJ Digital Med. 1 , 1–2 (2018).
Bento, A. I. et al. Evidence from internet search data shows information-seeking responses to news of local covid-19 cases. Proc. Natl Acad. Sci. 117 , 11220–11222 (2020).
Article CAS PubMed PubMed Central Google Scholar
Chang, S. et al. Mobility network models of covid-19 explain inequities and inform reopening. Nature 589 , 82–87 (2021).
Article ADS CAS PubMed Google Scholar
Suh, J., Horvitz, E., White, R. W. & Althoff, T. Population-scale study of human needs during the covid-19 pandemic: Analysis and implications. In Proceedings of the 14th ACM International Conference on Web Search and Dat a Mining . 4–12 (Association for Computing Machinery, 2021).
Pierson, E., Althoff, T., Thomas, D., Hillard, P. & Leskovec, J. Daily, weekly, seasonal and menstrual cycles in women’s mood, behaviour and vital signs. Nat. Hum. Behav. 5 , 716–725 (2021).
Aiello, L. M., Schifanella, R., Quercia, D. & Del Prete, L. Large-scale and high-resolution analysis of food purchases and health outcomes. EPJ Data Sci. 8 , 14 (2019).
West, R., White, R. W. & Horvitz, E. From cookies to cooks: Insights on dietary patterns via analysis of web usage logs. In Proceedings of the 22nd international conference on World Wide Web . 1399–1410 (International World Wide Web Conferences Steering Committee, 2013).
Abbar, S., Mejova, Y. & Weber, I. You tweet what you eat: Studying food consumption through twitter. In Proceedings of the 33rd Annual ACM Conference on Human Factors in Computing Systems . 3197–3206 (Association for Computing Machinery, 2015).
De Choudhury, M., Sharma, S. & Kiciman, E. Characterizing dietary choices, nutrition, and language in food deserts via social media. In Proceedings of the 19th ACM conference on computer-supported cooperative work & social computing . 1157–1170 (Association for Computing Machinery, 2016).
Gordon, M., Althoff, T. & Leskovec, J. Goal-setting and achievement in activity tracking apps: a case study of MyFitnessPal. In The World Wide Web Conference . 571–582 (International World Wide Web Conferences Steering Committee, 2019).
Allcott, H. et al. Food deserts and the causes of nutritional inequality. Q. J. Econ. 134 , 1793–1844 (2019).
Article MATH Google Scholar
Sasson, C. et al. American heart association diabetes and cardiometabolic health summit: Summary and recommendations. J. Am. Heart Assoc. 7 , e009271 (2018).
Mason, K. E., Pearce, N. & Cummins, S. Associations between fast food and physical activity environments and adiposity in mid-life: cross-sectional, observational evidence from uk biobank. Lancet Public Health 3 , e24–e33 (2018).
CDC. Behavioral risk factor surveillance system survey questionnaire (2011). https://www.cdc.gov/brfss/smart/smart_2011.htm . Accessed 18 Aug 2017.
CDC. Behavioral risk factor surveillance system survey questionnaire (2012). https://www.cdc.gov/brfss/smart/smart_2012.htm . Accessed 18 Aug 2017.
Rahkovsky, I. & Snyder, S. Food choices and store proximity (2015). https://www.ers.usda.gov/webdocs/publications/45432/53943_err195.pdf?v=42276/ . Accessed 31 Dec 2019.
USCB. Quickfacts (2017). https://www.census.gov/quickfacts/fact/table/US/PST045219 .
Larson, N. I., Story, M. T. & Nelson, M. C. Neighborhood environments: disparities in access to healthy foods in the us. Am. J. Preventive Med. 36 , 74–81 (2009).
Mackenbach, J. D. et al. A systematic review on socioeconomic differences in the association between the food environment and dietary behaviors. Nutrients 11 , 2215 (2019).
Article PubMed Central Google Scholar
Meyer, K. A. et al. Sociodemographic differences in fast food price sensitivity. JAMA Intern. Med. 174 , 434–442 (2014).
Lakerveld, J. & Mackenbach, J. The upstream determinants of adult obesity. Obes. Facts 10 , 216–222 (2017).
Cockerham, W. C., Hamby, B. W. & Oates, G. R. The social determinants of chronic disease. Am. J. Preventive Med. 52 , S5–S12 (2017).
Yusuf, Z. I. et al. Social determinants of overweight and obesity among children in the united states. Int. J. Matern. Child Health AIDS 9 , 22 (2020).
Farmer, M. M. & Ferraro, K. F. Are racial disparities in health conditional on socioeconomic status? Soc. Sci. Med. 60 , 191–204 (2005).
Shuey, K. M. & Willson, A. E. Cumulative disadvantage and black-white disparities in life-course health trajectories. Res. Aging 30 , 200–225 (2008).
Do, D. P. et al. Does place explain racial health disparities? quantifying the contribution of residential context to the black/white health gap in the united states. Soc. Sci. Med. 67 , 1258–1268 (2008).
Williams, D. R. & Collins, C. Us socioeconomic and racial differences in health: patterns and explanations. Annu. Rev. Sociol. 21 , 349–386 (1995).
Bratter, J. L. & Gorman, B. K. Is discrimination an equal opportunity risk? racial experiences, socioeconomic status, and health status among black and white adults. J. Health Soc. Behav. 52 , 365–382 (2011).
Williams, D. R., Neighbors, H. W. & Jackson, J. S. Racial/ethnic discrimination and health: findings from community studies. Am. J. Public Health 93 , 200–208 (2003).
Pearson, J. A. Can’t buy me whiteness: New lessons from the titanic on race, ethnicity, and health. Du Bois Rev.: Soc. Sci. Res. Race 5 , 27–47 (2008).
Boen, C. The role of socioeconomic factors in black-white health inequities across the life course: Point-in-time measures, long-term exposures, and differential health returns. Soc. Sci. Med. 170 , 63–76 (2016).
Lommel, L. L., Thompson, L., Chen, J.-L., Waters, C. & Carrico, A. Acculturation, inflammation, and self-rated health in mexican american immigrants. J. Immigr. Minority Health 21 , 1052–1060 (2019).
Bostean, G. Does selective migration explain the hispanic paradox? a comparative analysis of mexicans in the us and mexico. J. Immigr. Minority Health 15 , 624–635 (2013).
Ruiz, J. M., Steffen, P. & Smith, T. B. Hispanic mortality paradox: a systematic review and meta-analysis of the longitudinal literature. Am. J. Public Health 103 , e52–e60 (2013).
Min, J. W., Rhee, S., Lee, S. E., Rhee, J. & Tran, T. Comparative analysis on determinants of self-rated health among non-hispanic white, hispanic, and asian american older adults. J. Immigr. Minority Health 16 , 365–372 (2014).
Kimbro, R. T., Gorman, B. K. & Schachter, A. Acculturation and self-rated health among latino and asian immigrants to the united states. Soc. Probl. 59 , 341–363 (2012).
Brewer, J. V. et al. Contributors to self-reported health in a racially and ethnically diverse population: focus on hispanics. Ann. Epidemiol. 23 , 19–24 (2013).
Article MathSciNet PubMed Google Scholar
Ridker, P. M. C-reactive protein: a simple test to help predict risk of heart attack and stroke. Circulation 108 , e81–e85 (2003).
Viruell-Fuentes, E. A. Beyond acculturation: immigration, discrimination, and health research among mexicans in the united states. Soc. Sci. Med. 65 , 1524–1535 (2007).
Rodriguez, F. et al. Association of educational attainment and cardiovascular risk in hispanic individuals: Findings from the cooper center longitudinal study. JAMA Cardiol. 4 , 43–50 (2019).
Lutsey, P. L. et al. Associations of acculturation and socioeconomic status with subclinical cardiovascular disease in the multi-ethnic study of atherosclerosis. Am. J. Public Health 98 , 1963–1970 (2008).
Koya, D. L. & Egede, L. E. Association between length of residence and cardiovascular disease risk factors among an ethnically diverse group of united states immigrants. J. Gen. Intern. Med. 22 , 841–846 (2007).
Moran, A. et al. Financial incentives increase purchases of fruit and vegetables among lower-income households with children. Health Aff. 38 , 1557–1566 (2019).
Moran, A. J. et al. Associations between governmental policies to improve the nutritional quality of supermarket purchases and individual, retailer, and community health outcomes: An integrative review. Int. J. Environ. Res. Public Health 17 , 7493 (2020).
Phipps, E. J. et al. Impact of a rewards-based incentive program on promoting fruit and vegetable purchases. Am. J. Public Health 105 , 166–172 (2015).
Steele-Adjognon, M. & Weatherspoon, D. Double up food bucks program effects on snap recipients’ fruit and vegetable purchases. BMC Public Health 17 , 1–7 (2017).
Wilde, P., Klerman, J. A., Olsho, L. E. & Bartlett, S. Explaining the impact of usda’s healthy incentives pilot on different spending outcomes. Appl. Economic Perspect. Policy 38 , 655–672 (2015).
Polacsek, M. et al. A supermarket double-dollar incentive program increases purchases of fresh fruits and vegetables among low-income families with children: the healthy double study. J. Nutr. Educ. Behav. 50 , 217–228 (2018).
Cummins, S., Flint, E. & Matthews, S. A. New neighborhood grocery store increased awareness of food access but did not alter dietary habits or obesity. Health Aff. 33 , 283–291 (2014).
Dubowitz, T. et al. Changes in diet after introduction of a full service supermarket in a food desert. Health Aff. 34 , 1858 (2015).
Zhang, Y. T. et al. Is a reduction in distance to nearest supermarket associated with bmi change among type 2 diabetes patients? Health Place 40 , 15–20 (2016).
Rogus, S., Athens, J., Cantor, J. & Elbel, B. Measuring micro-level effects of a new supermarket: do residents within 0.5 mile have improved dietary behaviors? J. Acad. Nutr. Dietetics 118 , 1037–1046 (2018).
Chrisinger, B. A mixed-method assessment of a new supermarket in a food desert: contributions to everyday life and health. J. Urban Health 93 , 425–437 (2016).
Giang, T., Karpyn, A., Laurison, H. B., Hillier, A. & Perry, R. D. Closing the grocery gap in underserved communities: the creation of the pennsylvania fresh food financing initiative. J. Public Health Manag. Pract. 14 , 272–279 (2008).
Jack, D. et al. Socio-economic status, neighbourhood food environments and consumption of fruits and vegetables in new york city. Public health Nutr. 16 , 1197–1205 (2013).
Strome, S., Johns, T., Scicchitano, M. J. & Shelnutt, K. Elements of access: the effects of food outlet proximity, transportation, and realized access on fresh fruit and vegetable consumption in food deserts. Int. Q. Community Health Educ. 37 , 61–70 (2016).
Cantor, J. et al. Snap participants improved food security and diet after a full-service supermarket opened in an urban food desert: Study examines impact grocery store opening had on food security and diet of supplemental nutrition assistance program participants living in an urban food desert. Health Aff. 39 , 1386–1394 (2020).
Chandola, T., Clarke, P., Morris, J. & Blane, D. Pathways between education and health: a causal modelling approach. J. R. Stat. Soc.: Ser. A 169 , 337–359 (2006).
Article MathSciNet Google Scholar
Cutler, D. M. & Lleras-Muney, A. Education and health: evaluating theories and evidence. Working Paper Series, Working Paper 12352 National Bureau of Economic Research. http://www.nber.org/papers/w12352 (2006).
Kenkel, D. S. Health behavior, health knowledge, and schooling. J. Political Econ. 99 , 287–305 (1991).
Fletcher, J. M. & Frisvold, D. E. Higher education and health investments: does more schooling affect preventive health care use? J. Hum. Cap. 3 , 144–176 (2009).
Lleras-Muney, A. The relationship between education and adult mortality in the united states. Rev. Economic Stud. 72 , 189–221 (2005).
Mirowsky, J. Education, Social Status, and Health (Routledge, 2017).
CDC. Behavioral risk factor surveillance system survey questionnaire. https://www.cdc.gov/brfss . Accessed 18 Aug 2017.
Weeks, W. B. et al. Differences in health-related quality of life in rural and urban veterans. Am. J. Public Health 94 , 1762–1767 (2004).
Cromartie, J. Rural-urban commuting area codes (2020). https://www.ers.usda.gov/data-products/rural-urban-commuting-area-codes.aspx .
Ferris, H. A., Isganaitis, E. & Brown, F. Time for an end to juice in the special supplemental nutrition program for women, infants, and children. JAMA pediatrics 171 , 509–510 (2017).
Caswell, H. The role of fruit juice in the diet: an overview. Nutr. Bull. 34 , 273–288 (2009).
Guasch-Ferré, M. & Hu, F. B. Are fruit juices just as unhealthy as sugar-sweetened beverages? JAMA Netw. Open 2 , e193109–e193109 (2019).
Bureau, U. C. American community survey 5-year estimates (2014). http://censusreporter.org . Accessed 30 Aug 2017.
Buajitti, E., Chiodo, S. & Rosella, L. C. Agreement between area-and individual-level income measures in a population-based cohort: implications for population health research. SSM-Popul. Health 10 , 100553 (2020).
Rhone, A. Food access research atlas documentation (2015). https://www.ers.usda.gov/data-products/food-access-research-atlas/documentation/ . Accessed 20 Aug 2017.
Din, A. HUD USPS Zip Code Crosswalk Files (2017). https://www.huduser.gov/portal/datasets/usps_crosswalk.html . Accessed 10 Aug 2017.
Yelp. Yelp Fusion V3 API (2017). https://www.yelp.com/dataset/documentation/main/ . Accessed 30 Aug 2017.
Efron, B. & Tibshirani, R. J. An introduction to the bootstrap (CRC press, 1994).
Austin, P. C. & Small, D. S. The use of bootstrapping when using propensity-score matching without replacement: a simulation study. Stat. Med. 33 , 4306–4319 (2014).
Article MathSciNet PubMed PubMed Central Google Scholar
Diamond, A. & Sekhon, J. S. Genetic matching for estimating causal effects: a general multivariate matching method for achieving balance in observational studies. Rev. Econ. Stat . 95 , 932–945 (2013).
Teixeira, V., Voci, S. M., Mendes-Netto, R. S. & da Silva, D. G. The relative validity of a food record using the smartphone application MyFitnessPal. Nutr. Dietetics 75 , 219–225 (2018).
Griffiths, C., Harnack, L. & Pereira, M. A. Assessment of the accuracy of nutrient calculations of five popular nutrition tracking applications. Public Health Nutr. 21 , 1495–1502 (2018).
Chen, J., Berkman, W., Bardouh, M., Ng, C. Y. K. & Allman-Farinelli, M. The use of a food logging app in the naturalistic setting fails to provide accurate measurements of nutrients and poses usability challenges. Nutrition 57 , 208–216 (2019).
Nguyen, Q. et al. Social media indicators of the food environment and state health outcomes. Public Health 148 , 120–128 (2017).
Gomez-Lopez, I. N. et al. Using social media to identify sources of healthy food in urban neighborhoods. J. Urban Health 94 , 429–436 (2017).
Einav, L., Leibtag, E. S. & Nevo, A. On the accuracy of Nielsen homescan data. Economic Research Report No. ERR-69. https://www.ers.usda.gov/publications/pub-details/?pubid=46114 (2008).
for Disease Control, C., Prevention et al. Behavioral risk factor surveillance system 2019 summary data quality report. july 16, 2020 (2020).
Mokdad, A. H. The behavioral risk factors surveillance system: past, present, and future. Annu. Rev. Public Health 30 , 43–54 (2009).
Pierannunzi, C., Hu, S. S. & Balluz, L. A systematic review of publications assessing reliability and validity of the behavioral risk factor surveillance system (brfss), 2004–2011. BMC Med. Res. Methodol. 13 , 1–14 (2013).
Austin, P. C. An introduction to propensity score methods for reducing the effects of confounding in observational studies. Multivar. Behav. Res. 46 , 399–424 (2011).
Stuart, E. A. Matching methods for causal inference: a review and a look forward. Stat. Sci.: A Rev. J. Inst. Math. Stat. 25 , 1 (2010).
Article MathSciNet MATH Google Scholar
Glass, G. V. Primary, secondary, and meta-analysis of research. Educ. Researcher 5 , 3–8 (1976).
US Zip Codes History (2012). https://www.zip-codes.com/zip-codes-history.asp . Accessed 11 May 2021.
USPS Postal Facts (2021). https://facts.usps.com/42000-zip-codes/ . Accessed 11 May 2021.
US Census 2012 (2012). https://web.archive.org/web/20130707052113/http://www.census.gov/popest/data/counties/totals/2012/CO-EST2012-alldata.html/ . Accessed 11 May 2021.
Rundle, A. et al. Personal and neighborhood socioeconomic status and indices of neighborhood walk-ability predict body mass index in new york city. Soc. Sci. Med. 67 , 1951–1958 (2008).
Download references
Acknowledgements
T.A. and J.L. were supported by a National Institutes of Health (NIH) grant (U54 EB020405, Mobilize Center, NIH Big Data to Knowledge Center of Excellence). T.A. was supported by the SAP Stanford Graduate Fellowship, NSF grant IIS-1901386, NSF grant CNS-2025022, NIH grant R01MH125179, Bill & Melinda Gates Foundation (INV-004841), and the Office of Naval Research (#N00014-21-1-2154). H.N. was supported by NSF REU #1659585 at the Stanford Center for the Study of Language and Information (CSLI). J.H. is supported by Postdoctoral Fellowship in Cardiovascular Disease Prevention (T32) funded by the National Heart, Lung, and Blood Institute (NHLBI) at the National Institutes of Health (NIH). J.L. was supported by DARPA under Nos. FA865018C7880 (ASED), N660011924033 (MCS); ARO under Nos. W911NF-16-1-0342 (MURI), W911NF-16-1-0171 (DURIP); NSF under Nos. OAC-1835598 (CINES), OAC-1934578 (HDR), CCF-1918940 (Expeditions); Stanford Data Science Initiative, Wu Tsai Neurosciences Institute, Chan Zuckerberg Biohub, Amazon, Boeing, Chase, Docomo, Hitachi, Huawei, JD.com, NVIDIA, Dell. We thank MyFitnessPal for donating the data for independent research and Mitchell Gordon for helping with data preparation.
Author information
Authors and affiliations.
Allen School of Computer Science & Engineering, University of Washington, Seattle, WA, USA
Tim Althoff
Department of Computer Science, Stanford University, Stanford, CA, USA
Hamed Nilforoshan & Jure Leskovec
Stanford Prevention Research Center, Department of Medicine, Stanford University School of Medicine, Stanford, CA, USA
Million Marker Wellness Inc., San Francisco, CA, USA
Chan Zuckerberg Biohub, San Francisco, CA, USA
Jure Leskovec
You can also search for this author in PubMed Google Scholar
Contributions
T.A. and J.L. conceived the study concept and design. T.A. and H.N. conducted the statistical analysis. T.A., H.N., J.H., and J.L. interpreted the data, drafted the manuscript, and critically revised the manuscript for important intellectual content.
Corresponding author
Correspondence to Tim Althoff .
Ethics declarations
Competing interests.
The authors declare no competing interests.
Peer review information
Nature Communications thanks Bridget Kelly, Joreintje Mackenbach, Quynh Nguyen and the other, anonymous, reviewer(s) for their contribution to the peer review of this work.
Additional information
Publisher’s note Springer Nature remains neutral with regard to jurisdictional claims in published maps and institutional affiliations.
Supplementary information
Supplementary information, reporting summary, rights and permissions.
Open Access This article is licensed under a Creative Commons Attribution 4.0 International License, which permits use, sharing, adaptation, distribution and reproduction in any medium or format, as long as you give appropriate credit to the original author(s) and the source, provide a link to the Creative Commons license, and indicate if changes were made. The images or other third party material in this article are included in the article’s Creative Commons license, unless indicated otherwise in a credit line to the material. If material is not included in the article’s Creative Commons license and your intended use is not permitted by statutory regulation or exceeds the permitted use, you will need to obtain permission directly from the copyright holder. To view a copy of this license, visit http://creativecommons.org/licenses/by/4.0/ .
Reprints and permissions
About this article
Cite this article.
Althoff, T., Nilforoshan, H., Hua, J. et al. Large-scale diet tracking data reveal disparate associations between food environment and diet. Nat Commun 13 , 267 (2022). https://doi.org/10.1038/s41467-021-27522-y
Download citation
Received : 20 January 2021
Accepted : 18 November 2021
Published : 18 January 2022
DOI : https://doi.org/10.1038/s41467-021-27522-y
Share this article
Anyone you share the following link with will be able to read this content:
Sorry, a shareable link is not currently available for this article.
Provided by the Springer Nature SharedIt content-sharing initiative
This article is cited by
Multimodal digital phenotyping of diet, physical activity, and glycemia in hispanic/latino adults with or at risk of type 2 diabetes.
- Rony Santiago
npj Digital Medicine (2024)
Diabetic Cardiomyopathy and Cell Death: Focus on Metal-Mediated Cell Death
- Kupper Wintergerst
Cardiovascular Toxicology (2024)
Designing and Evaluating a Hierarchical Framework for Matching Food Outlets across Multi-sourced Geospatial Datasets: a Case Study of San Diego County
- Jiue-An Yang
- Marta M. Jankowska
Journal of Urban Health (2024)
Wearable and digital devices to monitor and treat metabolic diseases
- Ayya Keshet
- Lee Reicher
Nature Metabolism (2023)
Nature Communications (2023)
By submitting a comment you agree to abide by our Terms and Community Guidelines . If you find something abusive or that does not comply with our terms or guidelines please flag it as inappropriate.
Quick links
- Explore articles by subject
- Guide to authors
- Editorial policies
Sign up for the Nature Briefing newsletter — what matters in science, free to your inbox daily.

- Share full article
Advertisement
Supported by
Student Opinion
What Is Your Relationship With Fast Food?
Do you love it, hate it or feel something in between?

By Michael Gonchar
Fast food is seemingly everywhere. One can find a McDonald’s, a Chick-fil-A, a Taco Bell, a Wendy’s, a Dunkin’ or a Domino’s, just to name a few of the most popular chains, in towns and cities across the United States and around the world.
What do you think about fast food? Is it something that improves our lives and makes the world a better place? Or is it a bad thing for our health and a negative influence in our communities?
Brian Gallagher begins the article “ Fast Food Forever: How McHaters Lost the Culture War ” by discussing how the fast food industry faced a strong backlash two decades ago, when the film “Super Size Me,” directed by and starring Morgan Spurlock, became a smash hit. He writes:
Following Mr. Spurlock as he ate nothing but McDonald’s for 30 days — and the ill effects that diet had on his health — the film became the high-water mark in a tide of sentiment against fast food. McDonald’s, specifically, became a symbol for the glossy hegemony of American capitalism both at home and abroad. “McJobs” became a term for low-paying, dead-end positions, “McMansions” for garish, oversize houses. In 1992, the political theorist Benjamin Barber used the term “McWorld” as shorthand for emergent neoliberal dominance; seven years later, protesters against the World Trade Organization seemed to agree, launching a newspaper box through a McDonald’s window during the “Battle of Seattle” marches. Two years after that, Eric Schlosser’s “ Fast Food Nation ” was published. A broad indictment of the entire fast-food industry, the best seller accused the industry of being bad for the environment, rife with labor issues, culturally flattening and culinarily fattening.
The article explains that fast food is still thriving 20 years later:
But two decades later, not only is McDonald’s bigger than ever, with nearly 42,000 global locations, but fast food in general has boomed. There are now some 40 chains with more than 500 locations in the United States. Fast food is the second-largest private employment sector in the country, after hospitals, and 36 percent of Americans — about 84 million people — eat fast food on any given day. The three major appeals of fast food remain intact: It’s cheap, it’s convenient and people like the way it tastes.
Mr. Gallagher also discusses the pivotal role that children play for the fast food industry:
Historically, fast-food companies have been very astute about marketing to children, realizing decades ago that creating customers early means creating customers for life. At the peak of his fame in the 1980s, Ronald McDonald was in some countries more recognizable to children than Mickey Mouse. In 2000, 90 percent of children ages 6 to 9 visited a McDonald’s in a given month. But as Frances Fleming-Milici, the director of marketing initiatives at the UConn Rudd Center for Food Policy and Health, put it, “If it’s marketed to children, it’s probably bad for you.” That became increasingly clear in the mid-2000s. Childhood obesity rates had nearly tripled in 25 years, and the public outcry was growing more urgent. A consortium of large food brands, including McDonald’s, Burger King, PepsiCo and Coca-Cola, tried to get out in front of the problem. They formed the Children’s Food and Beverage Advertising Initiative , and the participating corporations self-imposed limits on advertising to children under 13 (later 12). In place of that marketing to children, though, the big fast-food chains have found something arguably more potent, with McDonald’s, as ever, leading the way. “They’re hyperfocusing on what they call fan-favorite moments, trying to essentially identify how we emotionally connect to McDonald’s,” said Kaitlin Ceckowski, who researches fast-food marketing strategies at Mintel, a market research agency. “What ‘human truths’ exist around their brand?”
Students, read the entire article and then tell us:
Do you like to eat fast food? Why, or why not?
Which fast food chains are your favorites — or least favorites? Why?
When was the last time you ate fast food? In a typical month, how often do you eat it?
Do you think fast food chains make your town or city a better place to live? Why, or why not?
The article discusses paid partnerships between Travis Scott and McDonald’s, Megan Thee Stallion and Popeyes, Ice Spice and Dunkin’, and Lil Nas X and Taco Bell. Do these celebrity ad campaigns make you want to eat fast food more? In general, do you think that fast food advertising is effective — especially with children and teenagers? Why, or why not?
Are you concerned about health issues associated with eating too much fast food, such as diabetes and obesity? Do you think more should be done — by parents, companies or the government — to help limit how much fast food children eat? Why, or why not?
Students 13 and older in the United States and Britain, and 16 and older elsewhere, are invited to comment. All comments are moderated by the Learning Network staff, but please keep in mind that once your comment is accepted, it will be made public and may appear in print.
Find more Student Opinion questions here. Teachers, check out this guide to learn how you can incorporate these prompts into your classroom.
Vegetarian and vegan diets linked to lower risk of heart disease, cancer and death, large review finds
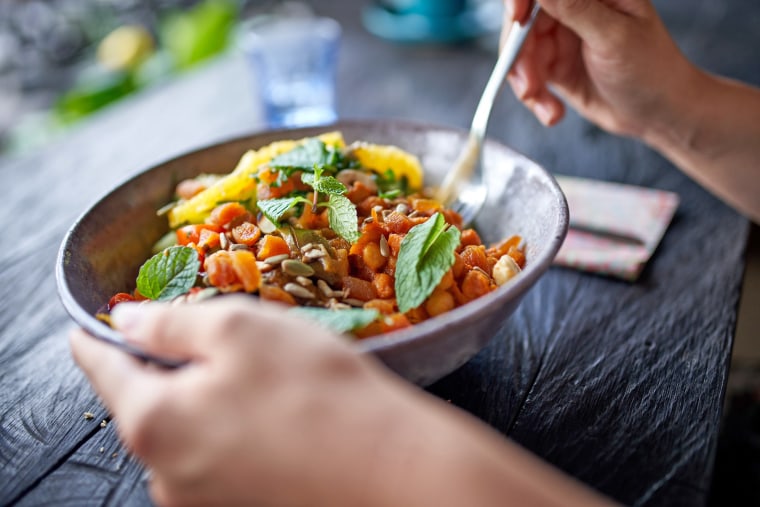
A plant-based diet is associated with a reduced risk of heart disease, cancer and death, according to a large-scale review published Wednesday.
The research , which appears in the journal PLOS ONE, analyzed the results of nearly 50 studies published from 2000 to 2023.
The studies examined the health effects of either vegetarian diets or vegan regimens, which restrict any food derived from animals, including dairy.
A clear consensus emerged: Both eating patterns were associated with a lower risk of cancer and ischemic heart disease (heart problems caused by narrowed arteries). In particular, the diets seemed to reduce the risk of prostate cancer and gastrointestinal cancers like colon cancer. Vegetarian diets were also linked to a lower risk of dying from cardiovascular disease.
In addition, plant-based diets were associated with a reduction in risk factors for heart disease and cancer, including high body weight, inflammation and LDL or “bad” cholesterol.
“This research shows, in general, that a plant-based diet can be beneficial, and taking small steps in that direction can make a difference,” said Matthew Landry, one of the review’s authors and an assistant professor of population health and disease prevention at the University of California, Irvine.
“You don’t have to go completely vegan to see some of these benefits,” he added. “Even reducing a day or two per week of animal-based consumption can have benefits over time.”
However, Dr. Walter Willett, a professor of epidemiology and nutrition at the Harvard T.H. Chan School of Public Health, pointed out that not everyone who follows a plant-based diet eats the same foods, so levels of healthiness still vary.
“A vegetarian diet could be based primarily on refined starches and sugar, which we see to be the worst dietary pattern,” Willett, who was not involved in the new research, said in an email.
A healthy plant-based diet, he said, should consist mostly of whole grains, fruits, vegetables, nuts, soy, beans and non-hydrogenated plant oils.
Why are plant-based diets so healthy?
Researchers are still investigating the mechanisms through which plant-based diets lower the risk of disease.
Some of it may have to do with preventing obesity, which is linked to heart disease and certain cancers . But the benefits likely extend beyond that, Landry said.
“Some of it is independent of weight. Even when weight is maintained or doesn’t change, we still see reductions in some of these other clinical health outcomes, especially when it relates to cardiovascular disease,” he said.
One possible reason is that many fruits and vegetables are high in anti-inflammatory nutrients and antioxidants, which can reduce plaque buildup in the arteries.
Plant-based diets also tend to be high in fiber, which helps lower bad cholesterol, said Brie Turner-McGrievy, a professor of health promotion, education and behavior at the University of South Carolina. She published a study in 2014 which found that plant-based diets can reduce risk factors for heart disease, stroke and Type 2 diabetes. The research was included in the new review.
“Soluble fiber that’s found in things like beans and oats is really a powerful tool to help lower LDL cholesterol levels,” she said.
Turner-McGrievy noted, though, that much of that benefit can only be achieved through eating whole foods: “It’s not like you can take a fiber supplement and hope to have these same outcomes.”
Another benefit of a plant-based diet may come simply from the absence of meat, she said. People who are vegan tend to consume less saturated fat than meat eaters.
“It’s just really hard to lower your saturated fat intake if you’re consuming animal-based foods,” Turner-McGrievy said. “Cheese, for example, is the No. 1 source of saturated fat in the diet.”
Processed meat products such as bacon or salami are also known to raise the risk of cancer , according to the World Health Organization. The agency considers red meat in general to be a “probable human carcinogen.”
Is a vegan or vegetarian diet right for everyone?
According to the Academy of Nutrition and Dietetics, vegetarian and vegan diets are adequate and healthy at all stages of life , including pregnancy, childhood and older adulthood.
But the new review stopped short of recommending plant-based diets for everyone.
“During pregnancy, it’s not recommended based on the data that we have to use a strict vegetarian diet,” said Dr. Federica Guaraldi, one of the review’s authors and an endocrinologist at the IRCCS Institute of Neurological Sciences of Bologna in Italy.
Guaraldi and her co-authors found that the plant-based regimens studied didn’t lower the risk of gestational diabetes or hypertension in pregnant women. One study included in the review suggested that pregnant women who followed a vegetarian diet had lower levels of zinc — which is important for children's growth, development and immune function — than those who ate meat. Another study in the review found that vegetarian mothers had an increased risk of delivering babies with low birthweights.
The review's authors also cautioned that plant-based diets might lead to vitamin B12 deficiencies in the general population. Landry said that can be addressed by taking a B12 supplement.
“From my perspective as a dietitian, a healthy plant-based diet — either vegetarian or vegan — can really meet just about all your vitamin and mineral needs,” he said.

Aria Bendix is the breaking health reporter for NBC News Digital.
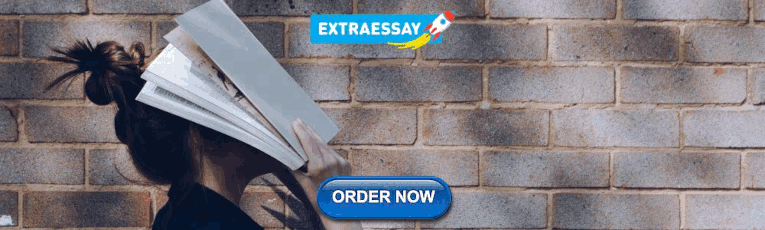
IMAGES
VIDEO
COMMENTS
Fast food has become a significant portion of the world's diet. For example, Table 1 shows the rapid increase in consumption in the United States across all age groups. In the 1970s, an average US adult (aged 18-65 y) consumed fast food on <10% of days, but this had risen to 40.7% of days in 2017-2018. Among US survey participants aged 12 ...
Because of the limited access to supermarkets, they eat more unhealthy fast and processed foods and end up having 7 times the risk of early-life stroke (before age 45), putting people in nursing homes in their 30s, 40s, and 50s. 4 - 7. The vulnerable poor in these areas also have double the risk of heart attack, double the risk of diabetes, and ...
Given the association between the frequency of fast-food consumption and diet quality, it is possible that fast-food consumption might displace healthful food choices for adults. Foods available at fast-food restaurants are energy dense, high in fat and low in fruits and vegetables and fibre (27, 28). Strategies in public health research to ...
The original research articles were reviewed published in English from 1990 to 2014. To search the articles, a number of databases and search engines, including PubMed, Medline, Scopus and Google Scholar were used. ... In animal models, fast food diet induced a phenotype of non-alcoholic fatty liver and steatohepatitis; 43 in this study, fast ...
Fast food tends to be high in salt, sugar, saturated fats, trans fats, calories, and processed preservatives and ingredients. A wealth of well-conducted research has proven the negative health ...
This study aimed to examine trends in the healthiness of U.S. fast food restaurant meals from 2008 to 2017, using the American Heart Association's Heart-Check meal certification criteria. Data ...
Poor diets are a leading cause of morbidity and mortality. Exposure to low-quality food environments saturated with fast food outlets is hypothesized to negatively impact diet. However, food ...
Several studies have been conducted to assess fast food contributions to diet and health. Research of national surveys of our youth, have shown that in 2011-2012, about 12% of their calories came from fast food, with older children ingesting significantly more calories from fast food than younger children [ 27 ].
Eating too much saturated fat can drive up your LDL, or "bad," cholesterol, which puts you at risk for heart disease. The American Heart Association recommends that no more than 6% of your ...
Introduction. Western-style fast food is a factor in dietary patterns portending poor cardiometabolic outcomes in the United States 1 - 3 and more recently in Southeast Asia. 4 The food is calorically dense and served in large portions, typically features meat and processed meat, has highly refined carbohydrates, is generally high in sodium and cholesterol, and has a poor dietary fatty acid ...
Food environments, the spaces where people acquire and consume food, impact diet and related diseases (i.e., nutritional health) 1.To date, research has focused on predefined local and static food ...
The fast food industry, through vast funds and advertising, contribute to challenges parents face to provide healthful foods for their families and thwart our best efforts to meet health goals. Research shows fast food consumption is influenced by lack of cooking confidence, time pressures, and perceptions of ease and convenience.
Background. Globally, young adolescents, especially those in low- and middle-income countries (LMICs), are experiencing a nutritional transition in the form of a dramatic shift in food-consumption patterns from their respective countries' traditional diet to a Westernized diet [Citation 1, Citation 2].Fast food is a common component of Western-style diets, and is energy-dense, nutrient-poor ...
Global industries and technological advancements have contributed to the proliferation of fast food (FF) establishments and ultraprocessed food, associated with poorer diet quality and health outcomes. To investigate FF as an indicator, we compared alternative methods to capture self-reported FF consumption and examined associated socio-demographic factors. We conducted a secondary analysis of ...
The fast food industry, through vast funds and advertising, contribute to challenges parents face to provide healthful foods for their families and thwart our best efforts to meet health goals. Research shows fast food consumption is influenced by lack of cooking confidence, time pressures, and perceptions of ease and convenience.
Consumption of fast foods t wo times or more per. week has been associa ted with 31% highe r. prevalen ce of moderate abdominal obesity in men. and 25% higher preval ence in women 70. Obesity is ...
REVIEW ARTICLE. Fast Foods and their Impact on Health. Ashakiran 1* & Deepthi R. 1 Department of Biochemistry, 2Department of Community Medicine, Sri Devaraj Urs Medical College, Kolar- 563101 ...
The majority of research shows that fast food consumption is linked to excess weight gain, poor diet quality, and mortality. ... The evaluation conducted by Barnes and associates examined the association between fast-food consumption and diet quality among working adults . The authors' determined that there was a significant inverse ...
The weight control evidence is stronger for whole grains than it is for fruits and vegetables. (20-22) The most recent support comes from the Harvard School of Public Health diet and lifestyle change study: People who increased their intake of whole grains, whole fruits (not fruit juice), and vegetables over the course of the 20-year study gained less weight-0.4, 0.5, and 0.2 pounds less ...
But 30 years of research show they don't all have the same impact. ... Overhead view of a large group of healthy raw food for flexitarian mediterranean diet. The composition includes salmon ...
The study, published in Nature Communications on Feb. 20, adds to the body of evidence supporting the beneficial effects of the fasting-mimicking diet (FMD).. The FMD is a five-day diet high in ...
Eat healthy foods. Healthy eating means incorporating a variety of healthy foods into your eating pattern. Fad diets promise fast results. However, such diets limit your nutritional intake, can be unhealthy, and tend to fail in the long run.
Explore diabetes-friendly fast food options at Taco Bell. Get dietitian-approved picks and tips for healthier choices while maintaining your carb budget. diaTribe is a non-profit organization providing free cutting-edge diabetes management tips from diet and exercise, to ways to avoid diabetes complications.
Here we leverage smartphones to track diet health, operationalized through the self-reported consumption of fresh fruits and vegetables, fast food and soda, as well as body-mass index status in a ...
When it comes to fast food, KFC is a go-to spot for fried chicken. Many have come to know that "It's finger lickin' good," as the slogan goes, thanks to Colonel Sanders' signature bone-in fried chicken with 11 herbs and spices. Deliciousness aside, making food choices at KFC may be challenging for those with certain health considerations.
Fast food is seemingly everywhere. One can find a McDonald's, a Chick-fil-A, a Taco Bell, a Wendy's, a Dunkin' or a Domino's, just to name a few of the most popular chains, in towns and ...
This low-calorie diet lasts for five days and consists of eating mostly plant-based unsaturated fats and low quantities of macronutrients like protein and carbs. In addition, you can consume a limited amount of calories from certain foods at specific times, so you're not "fasting" in a traditional sense. (For instance, you can consume 1,100 ...
Vegetarian and vegan diets are associated with a reduced risk of heart disease, cancer and death, according to a large review of research. An analysis of nearly 50 studies suggests that plant ...
Eating a vegan, vegetarian or lacto-ovo vegetarian diet significantly reduces the overall risk of developing cancer, heart disease and dying early from cardiovascular disease, according to a new ...