Society Homepage About Public Health Policy Contact
Data-driven hypothesis generation in clinical research: what we learned from a human subject study, article sidebar.
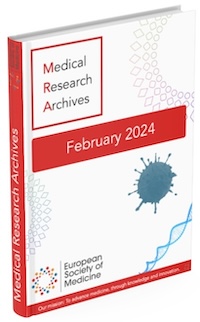
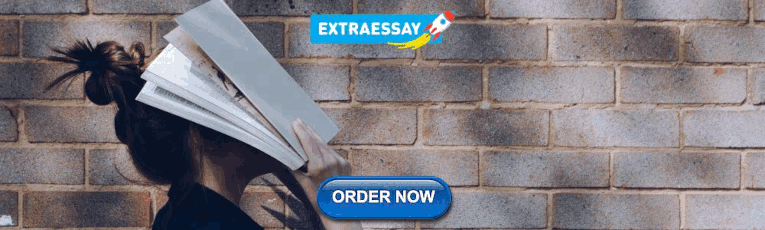
Submit your own article
Register as an author to reserve your spot in the next issue of the Medical Research Archives.
Join the Society
The European Society of Medicine is more than a professional association. We are a community. Our members work in countries across the globe, yet are united by a common goal: to promote health and health equity, around the world.
Join Europe’s leading medical society and discover the many advantages of membership, including free article publication.
Main Article Content
Hypothesis generation is an early and critical step in any hypothesis-driven clinical research project. Because it is not yet a well-understood cognitive process, the need to improve the process goes unrecognized. Without an impactful hypothesis, the significance of any research project can be questionable, regardless of the rigor or diligence applied in other steps of the study, e.g., study design, data collection, and result analysis. In this perspective article, the authors provide a literature review on the following topics first: scientific thinking, reasoning, medical reasoning, literature-based discovery, and a field study to explore scientific thinking and discovery. Over the years, scientific thinking has shown excellent progress in cognitive science and its applied areas: education, medicine, and biomedical research. However, a review of the literature reveals the lack of original studies on hypothesis generation in clinical research. The authors then summarize their first human participant study exploring data-driven hypothesis generation by clinical researchers in a simulated setting. The results indicate that a secondary data analytical tool, VIADS—a visual interactive analytic tool for filtering, summarizing, and visualizing large health data sets coded with hierarchical terminologies, can shorten the time participants need, on average, to generate a hypothesis and also requires fewer cognitive events to generate each hypothesis. As a counterpoint, this exploration also indicates that the quality ratings of the hypotheses thus generated carry significantly lower ratings for feasibility when applying VIADS. Despite its small scale, the study confirmed the feasibility of conducting a human participant study directly to explore the hypothesis generation process in clinical research. This study provides supporting evidence to conduct a larger-scale study with a specifically designed tool to facilitate the hypothesis-generation process among inexperienced clinical researchers. A larger study could provide generalizable evidence, which in turn can potentially improve clinical research productivity and overall clinical research enterprise.
Article Details
The Medical Research Archives grants authors the right to publish and reproduce the unrevised contribution in whole or in part at any time and in any form for any scholarly non-commercial purpose with the condition that all publications of the contribution include a full citation to the journal as published by the Medical Research Archives .
- Urgent Support
Engineering Graduate Studies
Hypothesis and Experimental Design
Jump to: Activity Examples | Resources
Two important elements of The Scientific Method that will help you design your research approach more efficiently are “Generating Hypotheses” and “Designing Controlled Experiments” to test these hypotheses. A well-designed experiment that you deeply understand will save time and resources and facilitate easier data analysis/interpretation. Many people reading this may be working on a project that focuses on designing a product, or discovery research where the hypothesis it is not immediately obvious. We encourage you to read on however as the exercise of generating a hypothesis will likely help you think about the assumptions you are making in your research and the physical principles your work builds upon.
These activities will help you …
- Begin formulating an appropriate hypothesis related to your research.
- Apply a systematic process for designing experiments.
What is a Hypothesis?
A hypothesis is an “educated guess/prediction” or “ proposed explanation ” of how a system will behave based on the available evidence . A hypothesis is a starting point for further investigation and testing because a hypothesis makes a prediction about the behavior of a measurable outcome of an experiment. A hypothesis should be:
- Testable – you can design an experiment to test it
- Falsifiable – it can be proven wrong (note it cannot be “proved”)
- Useful – the outcome must give valuable information
A useful hypothesis may relate to the underlying question of your research. For example:
“We hypothesize that therapy resistant cell populations will be enriched in hypoxic microenvironments. “
“We hypothesize that increasing the number of boreholes simulated in 3D geological models minimizes the variation of the geological model results.”
Some research projects do not have an obvious hypothesis to test, but the design strategy/concept chosen is based on an underlying assumption about how the system being designed works (i.e. the hypothesis). For example:
“We hypothesize that decreasing the baking temperature of the photoresist layer will reduce thermal expansion and device cracking”
In this case the researcher is troubleshooting poor device quality and is proposing to vary different fabrication parameters (one being baking temperature). Understanding the assumptions (working hypotheses) of why different variables might improve device quality is useful as it provides a basis to prioritize what variables to focus on first. The core goal of this research is not to test a specific hypothesis, but using the scientific method to troubleshoot a design challenge will enable the researcher to understand the parameters that control the behavior of different designs and to identify a design that is successful more efficiently.
In all the examples above, the hypothesis helps to guide the design of a useful and interpretable experiment with appropriate controls that rule out alternative explanations of the experimental observation. Hypotheses are therefore likely essential and useful parts of all research projects.
Suggested Activity – Create a Hypothesis for Your Research
Estimated time: 30 mins
- Write down the parameters you are varying or testing in your experimental system or model and how you think the behaviour of the system is going to vary with these parameters.
- (Alternative) If your project goal is to design a device, write down the parameters you believe control whether the device will work.
- (Alternative) If your project goal involves optimizing a process, write down the underlying physics or chemistry controlling the process you are studying.
- With these parameters in mind, write down the key assumption(s) you are making about how your system works.
- Try to formulate each one of these assumptions into a hypothesis that might be useful for your research project. If you have multiple aims each one may have a separate hypothesis. Make sure the hypothesis meets each of the three key elements above.
- Share your hypothesis with a peer or your supervisor to discuss if this is a good hypothesis – is it testable? Does it make a useful prediction? Does it capture the key underlying assumptions your research is based upon?
Remember that writing a good research hypothesis is challenging and will take a lot of careful thought about the underlying science that governs your system.
Designing Experiments
Designing experiments appropriately is very important to avoid wasting resources (time!) and to ensure results can be interpreted correctly. It is often very useful to discuss the design of your planned experiments in your meetings with your supervisor to get feedback before you start doing experiments. This will also ensure you and your supervisor have a consistent understanding of experimental design and that all the appropriate controls required to interpret your data have been considered.
The factors that must be considered when you design experiments is going to depend on your specific area of research. S ome important things to think about when designing experiments include:
Rationale: What is the purpose of this experiment? Is this the best experiment I can do? Does my experiment answer any question ? Does this experiment help answer the question I am trying to ask? What hypothesis am I trying to test?
Will my experiment be interpretable? What controls can I use to distinguish my results from other potential explanations? Can I add a control to distinguish between explanations? Can I add a control to further test my hypothesis?
Is my experiment/model rigorous? What is the sensitivity of the method I am using and can it measure accurately what I want to measure? What outcomes (metrics) will I measure and is this measurement appropriate? How many replicates (technical replicates versus independent replicates) will I do? Am I only changing the variable that I am testing? What am I keeping constant? What statistical tests do I plan to carry out and what considerations are needed? Is my statistical design appropriate (power analysis, sufficient replicates)?
What logistics do I need to consider? Are the equipment/resources I need available? Do I need additional training or equipment access? Are there important safety or ethical issues/permits to consider? Are pilot experiments needed to assess feasibility and what would these be? What is my planned experimental protocol and are there important timing issues to consider? What experimental outputs and parameters need to be documented throughout experiment?
This list is not exhaustive and you should consider what is missing for your particular situation.
Suggested Activity – Design an Experiment Using a Template
Estimated time: 45 min
- Explore the excel template for experimental design ( Resource 1 ) or modelling ( Resource 2 ). A template like this is very useful for keeping track of protocols as well as improving the reproducibility of your experiments. Note this template is simply a starting point to get you thinking systematically and should be adapted to best suit your needs.
- Fill out the template for an experiment or modelling project you are planning to complete soon.
- Consider how you can modify this template to be more applicable to your specific project.
- Using the template document, explain your experimental design/model design to a peer or your supervisor. Let them ask questions to understand your design and provide feedback. Alternatively, if there is a part of your design that you are unclear about this is a great starting point for a targeted and efficient discussion with your supervisor.
- Revise your design based on feedback.
Activity Examples
© 2024 Faculty of Applied Science and Engineering
- U of T Home
- Accessibility
- Student Data Practices
- Website Feedback
- First Online: 01 January 2024
Cite this chapter
- Hiroshi Ishikawa 3
Part of the book series: Studies in Big Data ((SBD,volume 139))
147 Accesses
This chapter will explain the definition and properties of a hypothesis, the related concepts, and basic methods of hypothesis generation as follows.
Describe the definition, properties, and life cycle of a hypothesis.
Describe relationships between a hypothesis and a theory, a model, and data.
Categorize and explain research questions that provide hints for hypothesis generation.
Explain how to visualize data and analysis results.
Explain the philosophy of science and scientific methods in relation to hypothesis generation in science.
Explain deduction, induction, plausible reasoning, and analogy concretely as reasoning methods useful for hypothesis generation.
Explain problem solving as hypothesis generation methods by using familiar examples.
This is a preview of subscription content, log in via an institution to check access.
Access this chapter
- Available as PDF
- Read on any device
- Instant download
- Own it forever
- Available as EPUB and PDF
- Durable hardcover edition
- Dispatched in 3 to 5 business days
- Free shipping worldwide - see info
Tax calculation will be finalised at checkout
Purchases are for personal use only
Institutional subscriptions
Aufmann RN, Lockwood JS et al (2018) Mathematical excursions. CENGAGE
Google Scholar
Bortolotti L (2008) An introduction to the philosophy of science. Polity
Cairo A (2016) The truthful art: data, charts, and maps for communication. New Riders
Cellucci C (2017) Rethinking knowledge: the heuristic view. Springer
Chang M (2014) Principles of scientific methods. CRC Press
Crease RP (2010) The great equations: breakthroughs in science from Pythagoras to Heisenberg. W. W. Norton & Company
Danks D, Ippoliti E (eds) Building theories: Heuristics and hypotheses in sciences. Springer
Diggle PJ, Chetwynd AG (2011) Statistics and scientific method: an introduction for students and researchers. Oxford University Press
DOAJ (2022) Directory of open access journal. https://doaj.org/ Accessed 2022
Gilchrist P, Wheaton B (2011) Lifestyle sport, public policy and youth engagement: examining the emergence of Parkour. Int J Sport Policy Polit 3(1):109–131. https://doi.org/10.1080/19406940.2010.547866
Article Google Scholar
Google Maps. https://www.google.com/maps Accessed 2022.
Ishikawa H (2015) Social big data mining. CRC Press
Järvinen P (2008) Mapping research questions to research methods. In: Avison D, Kasper GM, Pernici B, Ramos I, Roode D (eds) Advances in information systems research, education and practice. Proceedings of IFIP 20th world computer congress, TC 8, information systems, vol 274. Springer. https://doi.org/10.1007/978-0-387-09682-7-9_3
JAXA (2022) Martian moons eXploration. http://www.mmx.jaxa.jp/en/ . Accessed 2022
Lewton T (2020) How the bits of quantum gravity can buzz. Quanta Magazine. 2020. https://www.quantamagazine.org/gravitons-revealed-in-the-noise-of-gravitational-waves-20200723/ . Accessed 2022
Mahajan S (2014) The art of insight in science and engineering: Mastering complexity. The MIT Press
Méndez A, Rivera–Valentín EG (2017) The equilibrium temperature of planets in elliptical orbits. Astrophys J Lett 837(1)
NASA (2022) Mars sample return. https://www.jpl.nasa.gov/missions/mars-sample-return-msr Accessed 2022
OpenStreetMap (2022). https://www.openstreetmap.org . Accessed 2022
Pólya G (2009) Mathematics and plausible reasoning: vol I: induction and analogy in mathematics. Ishi Press
Pólya G, Conway JH (2014) How to solve it. Princeton University Press
Rehm J (2019) The four fundamental forces of nature. Live science https://www.livescience.com/the-fundamental-forces-of-nature.html
Sadler-Smith E (2015) Wallas’ four-stage model of the creative process: more than meets the eye? Creat Res J 27(4):342–352. https://doi.org/10.1080/10400419.2015.1087277
Siegel E, This is why physicists think string theory might be our ‘theory of everything.’ Forbes, 2018. https://www.forbes.com/sites/startswithabang/2018/05/31/this-is-why-physicists-think-string-theory-might-be-our-theory-of-everything/?sh=b01d79758c25
Zeitz P (2006) The art and craft of problem solving. Wiley
Download references
Author information
Authors and affiliations.
Department of Systems Design, Tokyo Metropolitan University, Hino, Tokyo, Japan
Hiroshi Ishikawa
You can also search for this author in PubMed Google Scholar
Corresponding author
Correspondence to Hiroshi Ishikawa .
Rights and permissions
Reprints and permissions
Copyright information
© 2024 The Author(s), under exclusive license to Springer Nature Switzerland AG
About this chapter
Ishikawa, H. (2024). Hypothesis. In: Hypothesis Generation and Interpretation. Studies in Big Data, vol 139. Springer, Cham. https://doi.org/10.1007/978-3-031-43540-9_2
Download citation
DOI : https://doi.org/10.1007/978-3-031-43540-9_2
Published : 01 January 2024
Publisher Name : Springer, Cham
Print ISBN : 978-3-031-43539-3
Online ISBN : 978-3-031-43540-9
eBook Packages : Computer Science Computer Science (R0)
Share this chapter
Anyone you share the following link with will be able to read this content:
Sorry, a shareable link is not currently available for this article.
Provided by the Springer Nature SharedIt content-sharing initiative
- Publish with us
Policies and ethics
- Find a journal
- Track your research

Want to create or adapt books like this? Learn more about how Pressbooks supports open publishing practices.
Developing a Hypothesis
Rajiv S. Jhangiani; I-Chant A. Chiang; Carrie Cuttler; and Dana C. Leighton
Learning Objectives
- Distinguish between a theory and a hypothesis.
- Discover how theories are used to generate hypotheses and how the results of studies can be used to further inform theories.
- Understand the characteristics of a good hypothesis.
Theories and Hypotheses
Before describing how to develop a hypothesis, it is important to distinguish between a theory and a hypothesis. A theory is a coherent explanation or interpretation of one or more phenomena. Although theories can take a variety of forms, one thing they have in common is that they go beyond the phenomena they explain by including variables, structures, processes, functions, or organizing principles that have not been observed directly. Consider, for example, Zajonc’s theory of social facilitation and social inhibition (1965) [1] . He proposed that being watched by others while performing a task creates a general state of physiological arousal, which increases the likelihood of the dominant (most likely) response. So for highly practiced tasks, being watched increases the tendency to make correct responses, but for relatively unpracticed tasks, being watched increases the tendency to make incorrect responses. Notice that this theory—which has come to be called drive theory—provides an explanation of both social facilitation and social inhibition that goes beyond the phenomena themselves by including concepts such as “arousal” and “dominant response,” along with processes such as the effect of arousal on the dominant response.
Outside of science, referring to an idea as a theory often implies that it is untested—perhaps no more than a wild guess. In science, however, the term theory has no such implication. A theory is simply an explanation or interpretation of a set of phenomena. It can be untested, but it can also be extensively tested, well supported, and accepted as an accurate description of the world by the scientific community. The theory of evolution by natural selection, for example, is a theory because it is an explanation of the diversity of life on earth—not because it is untested or unsupported by scientific research. On the contrary, the evidence for this theory is overwhelmingly positive and nearly all scientists accept its basic assumptions as accurate. Similarly, the “germ theory” of disease is a theory because it is an explanation of the origin of various diseases, not because there is any doubt that many diseases are caused by microorganisms that infect the body.
A hypothesis , on the other hand, is a specific prediction about a new phenomenon that should be observed if a particular theory is accurate. It is an explanation that relies on just a few key concepts. Hypotheses are often specific predictions about what will happen in a particular study. They are developed by considering existing evidence and using reasoning to infer what will happen in the specific context of interest. Hypotheses are often but not always derived from theories. So a hypothesis is often a prediction based on a theory but some hypotheses are a-theoretical and only after a set of observations have been made, is a theory developed. This is because theories are broad in nature and they explain larger bodies of data. So if our research question is really original then we may need to collect some data and make some observations before we can develop a broader theory.
Theories and hypotheses always have this if-then relationship. “ If drive theory is correct, then cockroaches should run through a straight runway faster, and a branching runway more slowly, when other cockroaches are present.” Although hypotheses are usually expressed as statements, they can always be rephrased as questions. “Do cockroaches run through a straight runway faster when other cockroaches are present?” Thus deriving hypotheses from theories is an excellent way of generating interesting research questions.
But how do researchers derive hypotheses from theories? One way is to generate a research question using the techniques discussed in this chapter and then ask whether any theory implies an answer to that question. For example, you might wonder whether expressive writing about positive experiences improves health as much as expressive writing about traumatic experiences. Although this question is an interesting one on its own, you might then ask whether the habituation theory—the idea that expressive writing causes people to habituate to negative thoughts and feelings—implies an answer. In this case, it seems clear that if the habituation theory is correct, then expressive writing about positive experiences should not be effective because it would not cause people to habituate to negative thoughts and feelings. A second way to derive hypotheses from theories is to focus on some component of the theory that has not yet been directly observed. For example, a researcher could focus on the process of habituation—perhaps hypothesizing that people should show fewer signs of emotional distress with each new writing session.
Among the very best hypotheses are those that distinguish between competing theories. For example, Norbert Schwarz and his colleagues considered two theories of how people make judgments about themselves, such as how assertive they are (Schwarz et al., 1991) [2] . Both theories held that such judgments are based on relevant examples that people bring to mind. However, one theory was that people base their judgments on the number of examples they bring to mind and the other was that people base their judgments on how easily they bring those examples to mind. To test these theories, the researchers asked people to recall either six times when they were assertive (which is easy for most people) or 12 times (which is difficult for most people). Then they asked them to judge their own assertiveness. Note that the number-of-examples theory implies that people who recalled 12 examples should judge themselves to be more assertive because they recalled more examples, but the ease-of-examples theory implies that participants who recalled six examples should judge themselves as more assertive because recalling the examples was easier. Thus the two theories made opposite predictions so that only one of the predictions could be confirmed. The surprising result was that participants who recalled fewer examples judged themselves to be more assertive—providing particularly convincing evidence in favor of the ease-of-retrieval theory over the number-of-examples theory.
Theory Testing
The primary way that scientific researchers use theories is sometimes called the hypothetico-deductive method (although this term is much more likely to be used by philosophers of science than by scientists themselves). Researchers begin with a set of phenomena and either construct a theory to explain or interpret them or choose an existing theory to work with. They then make a prediction about some new phenomenon that should be observed if the theory is correct. Again, this prediction is called a hypothesis. The researchers then conduct an empirical study to test the hypothesis. Finally, they reevaluate the theory in light of the new results and revise it if necessary. This process is usually conceptualized as a cycle because the researchers can then derive a new hypothesis from the revised theory, conduct a new empirical study to test the hypothesis, and so on. As Figure 2.3 shows, this approach meshes nicely with the model of scientific research in psychology presented earlier in the textbook—creating a more detailed model of “theoretically motivated” or “theory-driven” research.
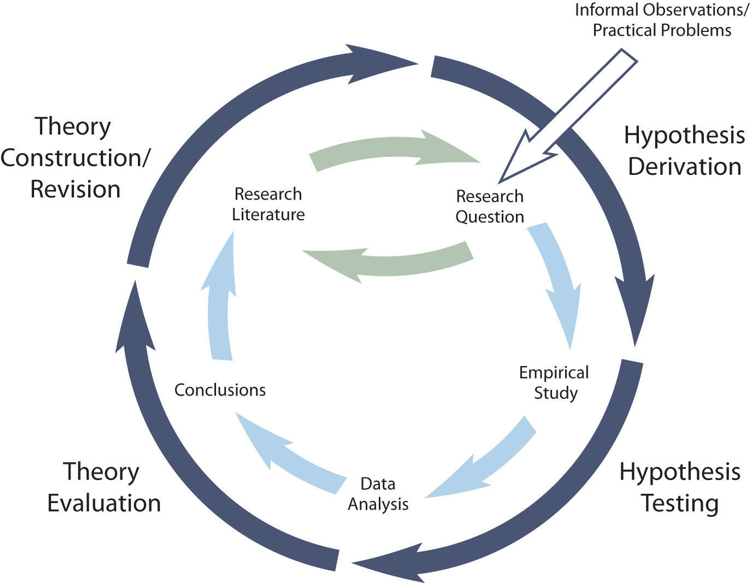
As an example, let us consider Zajonc’s research on social facilitation and inhibition. He started with a somewhat contradictory pattern of results from the research literature. He then constructed his drive theory, according to which being watched by others while performing a task causes physiological arousal, which increases an organism’s tendency to make the dominant response. This theory predicts social facilitation for well-learned tasks and social inhibition for poorly learned tasks. He now had a theory that organized previous results in a meaningful way—but he still needed to test it. He hypothesized that if his theory was correct, he should observe that the presence of others improves performance in a simple laboratory task but inhibits performance in a difficult version of the very same laboratory task. To test this hypothesis, one of the studies he conducted used cockroaches as subjects (Zajonc, Heingartner, & Herman, 1969) [3] . The cockroaches ran either down a straight runway (an easy task for a cockroach) or through a cross-shaped maze (a difficult task for a cockroach) to escape into a dark chamber when a light was shined on them. They did this either while alone or in the presence of other cockroaches in clear plastic “audience boxes.” Zajonc found that cockroaches in the straight runway reached their goal more quickly in the presence of other cockroaches, but cockroaches in the cross-shaped maze reached their goal more slowly when they were in the presence of other cockroaches. Thus he confirmed his hypothesis and provided support for his drive theory. (Zajonc also showed that drive theory existed in humans [Zajonc & Sales, 1966] [4] in many other studies afterward).
Incorporating Theory into Your Research
When you write your research report or plan your presentation, be aware that there are two basic ways that researchers usually include theory. The first is to raise a research question, answer that question by conducting a new study, and then offer one or more theories (usually more) to explain or interpret the results. This format works well for applied research questions and for research questions that existing theories do not address. The second way is to describe one or more existing theories, derive a hypothesis from one of those theories, test the hypothesis in a new study, and finally reevaluate the theory. This format works well when there is an existing theory that addresses the research question—especially if the resulting hypothesis is surprising or conflicts with a hypothesis derived from a different theory.
To use theories in your research will not only give you guidance in coming up with experiment ideas and possible projects, but it lends legitimacy to your work. Psychologists have been interested in a variety of human behaviors and have developed many theories along the way. Using established theories will help you break new ground as a researcher, not limit you from developing your own ideas.
Characteristics of a Good Hypothesis
There are three general characteristics of a good hypothesis. First, a good hypothesis must be testable and falsifiable . We must be able to test the hypothesis using the methods of science and if you’ll recall Popper’s falsifiability criterion, it must be possible to gather evidence that will disconfirm the hypothesis if it is indeed false. Second, a good hypothesis must be logical. As described above, hypotheses are more than just a random guess. Hypotheses should be informed by previous theories or observations and logical reasoning. Typically, we begin with a broad and general theory and use deductive reasoning to generate a more specific hypothesis to test based on that theory. Occasionally, however, when there is no theory to inform our hypothesis, we use inductive reasoning which involves using specific observations or research findings to form a more general hypothesis. Finally, the hypothesis should be positive. That is, the hypothesis should make a positive statement about the existence of a relationship or effect, rather than a statement that a relationship or effect does not exist. As scientists, we don’t set out to show that relationships do not exist or that effects do not occur so our hypotheses should not be worded in a way to suggest that an effect or relationship does not exist. The nature of science is to assume that something does not exist and then seek to find evidence to prove this wrong, to show that it really does exist. That may seem backward to you but that is the nature of the scientific method. The underlying reason for this is beyond the scope of this chapter but it has to do with statistical theory.
- Zajonc, R. B. (1965). Social facilitation. Science, 149 , 269–274 ↵
- Schwarz, N., Bless, H., Strack, F., Klumpp, G., Rittenauer-Schatka, H., & Simons, A. (1991). Ease of retrieval as information: Another look at the availability heuristic. Journal of Personality and Social Psychology, 61 , 195–202. ↵
- Zajonc, R. B., Heingartner, A., & Herman, E. M. (1969). Social enhancement and impairment of performance in the cockroach. Journal of Personality and Social Psychology, 13 , 83–92. ↵
- Zajonc, R.B. & Sales, S.M. (1966). Social facilitation of dominant and subordinate responses. Journal of Experimental Social Psychology, 2 , 160-168. ↵
A coherent explanation or interpretation of one or more phenomena.
A specific prediction about a new phenomenon that should be observed if a particular theory is accurate.
A cyclical process of theory development, starting with an observed phenomenon, then developing or using a theory to make a specific prediction of what should happen if that theory is correct, testing that prediction, refining the theory in light of the findings, and using that refined theory to develop new hypotheses, and so on.
The ability to test the hypothesis using the methods of science and the possibility to gather evidence that will disconfirm the hypothesis if it is indeed false.
Developing a Hypothesis Copyright © 2022 by Rajiv S. Jhangiani; I-Chant A. Chiang; Carrie Cuttler; and Dana C. Leighton is licensed under a Creative Commons Attribution-NonCommercial-ShareAlike 4.0 International License , except where otherwise noted.
Share This Book
Thank you for visiting nature.com. You are using a browser version with limited support for CSS. To obtain the best experience, we recommend you use a more up to date browser (or turn off compatibility mode in Internet Explorer). In the meantime, to ensure continued support, we are displaying the site without styles and JavaScript.
- View all journals
- Explore content
- About the journal
- Publish with us
- Sign up for alerts
- NATURE INDEX
- 17 November 2023
Hypotheses devised by AI could find ‘blind spots’ in research
- Matthew Hutson 0
Matthew Hutson is a science writer based in New York City.
You can also search for this author in PubMed Google Scholar
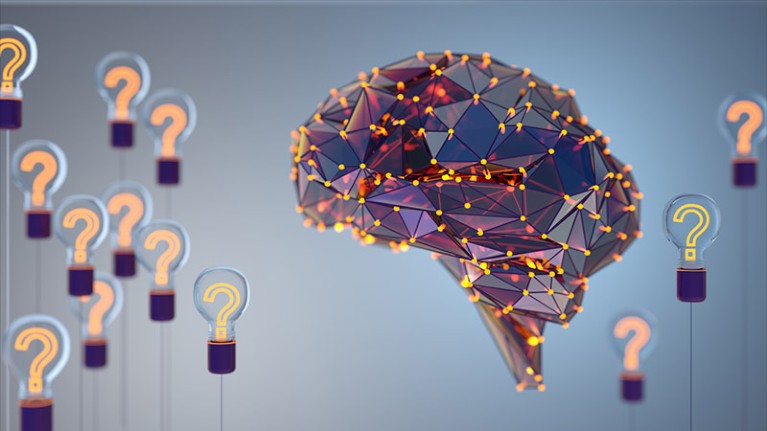
Credit: Olemedia/Getty
In early October, as the Nobel Foundation announced the recipients of this year’s Nobel prizes, a group of researchers, including a previous laureate, met in Stockholm to discuss how artificial intelligence (AI) might have an increasingly creative role in the scientific process. The workshop, led in part by Hiroaki Kitano, a biologist and chief executive of Sony AI in Tokyo, considered creating prizes for AIs and AI–human collaborations that produce world-class science. Two years earlier, Kitano proposed the Nobel Turing Challenge 1 : the creation of highly autonomous systems (‘AI scientists’) with the potential to make Nobel-worthy discoveries by 2050.
It’s easy to imagine that AI could perform some of the necessary steps in scientific discovery. Researchers already use it to search the literature, automate data collection, run statistical analyses and even draft parts of papers. Generating hypotheses — a task that typically requires a creative spark to ask interesting and important questions — poses a more complex challenge. For Sendhil Mullainathan, an economist at the University of Chicago Booth School of Business in Illinois, “it’s probably been the single most exhilarating kind of research I’ve ever done in my life”.
Network effects
AI systems capable of generating hypotheses go back more than four decades. In the 1980s, Don Swanson, an information scientist at the University of Chicago, pioneered literature-based discovery — a text-mining exercise that aimed to sift ‘undiscovered public knowledge’ from the scientific literature. If some research papers say that A causes B, and others that B causes C, for example, one might hypothesize that A causes C. Swanson created software called Arrowsmith that searched collections of published papers for such indirect connections and proposed, for instance, that fish oil, which reduces blood viscosity, might treat Raynaud’s syndrome, in which blood vessels narrow in response to cold 2 . Subsequent experiments proved the hypothesis correct.
Literature-based discovery and other computational techniques can organize existing findings into ‘knowledge graphs’, networks of nodes representing, say, molecules and properties. AI can analyse these networks and propose undiscovered links between molecule nodes and property nodes. This process powers much of modern drug discovery, as well as the task of assigning functions to genes. A review article published in Nature 3 earlier this year explores other ways in which AI has generated hypotheses, such as proposing simple formulae that can organize noisy data points and predicting how proteins will fold up. Researchers have automated hypothesis generation in particle physics, materials science, biology, chemistry and other fields.
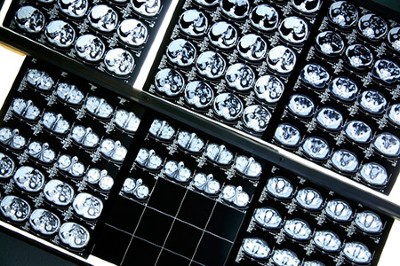
An AI revolution is brewing in medicine. What will it look like?
One approach is to use AI to help scientists brainstorm. This is a task that large language models — AI systems trained on large amounts of text to produce new text — are well suited for, says Yolanda Gil, a computer scientist at the University of Southern California in Los Angeles who has worked on AI scientists. Language models can produce inaccurate information and present it as real, but this ‘hallucination’ isn’t necessarily bad, Mullainathan says. It signifies, he says, “‘here’s a kind of thing that looks true’. That’s exactly what a hypothesis is.”
Blind spots are where AI might prove most useful. James Evans, a sociologist at the University of Chicago, has pushed AI to make ‘alien’ hypotheses — those that a human would be unlikely to make. In a paper published earlier this year in Nature Human Behaviour 4 , he and his colleague Jamshid Sourati built knowledge graphs containing not just materials and properties, but also researchers. Evans and Sourati’s algorithm traversed these networks, looking for hidden shortcuts between materials and properties. The aim was to maximize the plausibility of AI-devised hypotheses being true while minimizing the chances that researchers would hit on them naturally. For instance, if scientists who are studying a particular drug are only distantly connected to those studying a disease that it might cure, then the drug’s potential would ordinarily take much longer to discover.
When Evans and Sourati fed data published up to 2001 to their AI, they found that about 30% of its predictions about drug repurposing and the electrical properties of materials had been uncovered by researchers, roughly six to ten years later. The system can be tuned to make predictions that are more likely to be correct but also less of a leap, on the basis of concurrent findings and collaborations, Evans says. But “if we’re predicting what people are going to do next year, that just feels like a scoop machine”, he adds. He’s more interested in how the technology can take science in entirely new directions.
Keep it simple
Scientific hypotheses lie on a spectrum, from the concrete and specific (‘this protein will fold up in this way’) to the abstract and general (‘gravity accelerates all objects that have mass’). Until now, AI has produced more of the former. There’s another spectrum of hypotheses, partially aligned with the first, which ranges from the uninterpretable (these thousand factors lead to this result) to the clear (a simple formula or sentence). Evans argues that if a machine makes useful predictions about individual cases — “if you get all of these particular chemicals together, boom, you get this very strange effect” — but can’t explain why those cases work, that’s a technological feat rather than science. Mullainathan makes a similar point. In some fields, the underlying principles, such as the mechanics of protein folding, are understood and scientists just want AI to solve the practical problem of running complex computations that determine how bits of proteins will move around. But in fields in which the fundamentals remain hidden, such as medicine and social science, scientists want AI to identify rules that can be applied to fresh situations, Mullainathan says.
In a paper presented in September 5 at the Economics of Artificial Intelligence Conference in Toronto, Canada, Mullainathan and Jens Ludwig, an economist at the University of Chicago, described a method for AI and humans to collaboratively generate broad, clear hypotheses. In a proof of concept, they sought hypotheses related to characteristics of defendants’ faces that might influence a judge’s decision to free or detain them before trial. Given mugshots of past defendants, as well the judges’ decisions, an algorithm found that numerous subtle facial features correlated with judges’ decisions. The AI generated new mugshots with those features cranked either up or down, and human participants were asked to describe the general differences between them. Defendants likely to be freed were found to be more “well-groomed” and “heavy-faced”. Mullainathan says the method could be applied to other complex data sets, such as electrocardiograms, to find markers of an impending heart attack that doctors might not otherwise know to look for. “I love that paper,” Evans says. “That’s an interesting class of hypothesis generation.”
In science, experimentation and hypothesis generation often form an iterative cycle: a researcher asks a question, collects data and adjusts the question or asks a fresh one. Ross King, a computer scientist at Chalmers University of Technology in Gothenburg, Sweden, aims to complete this loop by building robotic systems that can perform experiments using mechanized arms 6 . One system, called Adam, automated experiments on microbe growth. Another, called Eve, tackled drug discovery. In one experiment, Eve helped to reveal the mechanism by which a toothpaste ingredient called triclosan can be used to fight malaria.
Robot scientists
King is now developing Genesis, a robotic system that experiments with yeast. Genesis will formulate and test hypotheses related to the biology of yeast by growing actual yeast cells in 10,000 bioreactors at a time, adjusting factors such as environmental conditions or making genome edits, and measuring characteristics such as gene expression. Conceivably, the hypotheses could involve many subtle factors, but King says they tend to involve a single gene or protein whose effects mirror those in human cells, which would make the discoveries potentially applicable in drug development. King, who is on the organizing committee of the Nobel Turing Challenge, says that these “robot scientists” have the potential to be more consistent, unbiased, cheap, efficient and transparent than humans.
Researchers see several hurdles to and opportunities for progress. AI systems that generate hypotheses often rely on machine learning, which usually requires a lot of data. Making more papers and data sets openly available would help, but scientists also need to build AI that doesn’t just operate by matching patterns but can also reason about the physical world, says Rose Yu, a computer scientist at the University of California, San Diego. Gil agrees that AI systems should not be driven only by data — they should also be guided by known laws. “That’s a very powerful way to include scientific knowledge into AI systems,” she says.
As data gathering becomes more automated, Evans predicts that automating hypothesis generation will become increasingly important. Giant telescopes and robotic labs collect more measurements than humans can handle. “We naturally have to scale up intelligent, adaptive questions”, he says, “if we don’t want to waste that capacity.”
doi: https://doi.org/10.1038/d41586-023-03596-0
Kitano, H. npj Syst. Biol. Appl. 7 , 29 (2021).
Article PubMed Google Scholar
Swanson, D. R. Perspect. Biol. Med. 30 , 7–18 (1986).
Wang, H. et al. Nature 620 , 47–60 (2023).
Sourati, J. & Evans, J. A. Nature Hum. Behav. 7 , 1682–1696 (2023).
Ludwig, J. & Mullainathan, S. Working Paper 31017 (National Bureau of Economic Research, 2023).
King, R., Peter, O. & Courtney, P. in Artificial Intelligence in Science 129–139 (OECD Publishing, 2023).
Download references
Related Articles
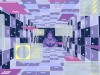
- Machine learning
- Computer science
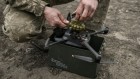
Lethal AI weapons are here: how can we control them?
News Feature 23 APR 24
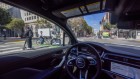
Will AI accelerate or delay the race to net-zero emissions?
Comment 22 APR 24
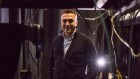
AI’s keen diagnostic eye
Outlook 18 APR 24
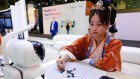
AI now beats humans at basic tasks — new benchmarks are needed, says major report
News 15 APR 24
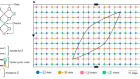
High-threshold and low-overhead fault-tolerant quantum memory
Article 27 MAR 24
Three reasons why AI doesn’t model human language
Correspondence 19 MAR 24
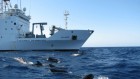
NATO is boosting AI and climate research as scientific diplomacy remains on ice
News Explainer 25 APR 24
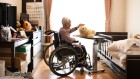
Are robots the solution to the crisis in older-person care?
Outlook 25 APR 24
Junior Group Leader
The Imagine Institute is a leading European research centre dedicated to genetic diseases, with the primary objective to better understand and trea...
Paris, Ile-de-France (FR)
Imagine Institute
Director of the Czech Advanced Technology and Research Institute of Palacký University Olomouc
The Rector of Palacký University Olomouc announces a Call for the Position of Director of the Czech Advanced Technology and Research Institute of P...
Czech Republic (CZ)
Palacký University Olomouc
Course lecturer for INFH 5000
The HKUST(GZ) Information Hub is recruiting course lecturer for INFH 5000: Information Science and Technology: Essentials and Trends.
Guangzhou, Guangdong, China
The Hong Kong University of Science and Technology (Guangzhou)
Suzhou Institute of Systems Medicine Seeking High-level Talents
Full Professor, Associate Professor, Assistant Professor
Suzhou, Jiangsu, China
Suzhou Institute of Systems Medicine (ISM)
Postdoctoral Fellowships: Early Diagnosis and Precision Oncology of Gastrointestinal Cancers
We currently have multiple postdoctoral fellowship positions within the multidisciplinary research team headed by Dr. Ajay Goel, professor and foun...
Monrovia, California
Beckman Research Institute, City of Hope, Goel Lab
Sign up for the Nature Briefing newsletter — what matters in science, free to your inbox daily.
Quick links
- Explore articles by subject
- Guide to authors
- Editorial policies
- Bipolar Disorder
- Therapy Center
- When To See a Therapist
- Types of Therapy
- Best Online Therapy
- Best Couples Therapy
- Best Family Therapy
- Managing Stress
- Sleep and Dreaming
- Understanding Emotions
- Self-Improvement
- Healthy Relationships
- Student Resources
- Personality Types
- Guided Meditations
- Verywell Mind Insights
- 2023 Verywell Mind 25
- Mental Health in the Classroom
- Editorial Process
- Meet Our Review Board
- Crisis Support
How to Write a Great Hypothesis
Hypothesis Definition, Format, Examples, and Tips
Kendra Cherry, MS, is a psychosocial rehabilitation specialist, psychology educator, and author of the "Everything Psychology Book."
:max_bytes(150000):strip_icc():format(webp)/IMG_9791-89504ab694d54b66bbd72cb84ffb860e.jpg)
Amy Morin, LCSW, is a psychotherapist and international bestselling author. Her books, including "13 Things Mentally Strong People Don't Do," have been translated into more than 40 languages. Her TEDx talk, "The Secret of Becoming Mentally Strong," is one of the most viewed talks of all time.
:max_bytes(150000):strip_icc():format(webp)/VW-MIND-Amy-2b338105f1ee493f94d7e333e410fa76.jpg)
Verywell / Alex Dos Diaz
- The Scientific Method
Hypothesis Format
Falsifiability of a hypothesis.
- Operationalization
Hypothesis Types
Hypotheses examples.
- Collecting Data
A hypothesis is a tentative statement about the relationship between two or more variables. It is a specific, testable prediction about what you expect to happen in a study. It is a preliminary answer to your question that helps guide the research process.
Consider a study designed to examine the relationship between sleep deprivation and test performance. The hypothesis might be: "This study is designed to assess the hypothesis that sleep-deprived people will perform worse on a test than individuals who are not sleep-deprived."
At a Glance
A hypothesis is crucial to scientific research because it offers a clear direction for what the researchers are looking to find. This allows them to design experiments to test their predictions and add to our scientific knowledge about the world. This article explores how a hypothesis is used in psychology research, how to write a good hypothesis, and the different types of hypotheses you might use.
The Hypothesis in the Scientific Method
In the scientific method , whether it involves research in psychology, biology, or some other area, a hypothesis represents what the researchers think will happen in an experiment. The scientific method involves the following steps:
- Forming a question
- Performing background research
- Creating a hypothesis
- Designing an experiment
- Collecting data
- Analyzing the results
- Drawing conclusions
- Communicating the results
The hypothesis is a prediction, but it involves more than a guess. Most of the time, the hypothesis begins with a question which is then explored through background research. At this point, researchers then begin to develop a testable hypothesis.
Unless you are creating an exploratory study, your hypothesis should always explain what you expect to happen.
In a study exploring the effects of a particular drug, the hypothesis might be that researchers expect the drug to have some type of effect on the symptoms of a specific illness. In psychology, the hypothesis might focus on how a certain aspect of the environment might influence a particular behavior.
Remember, a hypothesis does not have to be correct. While the hypothesis predicts what the researchers expect to see, the goal of the research is to determine whether this guess is right or wrong. When conducting an experiment, researchers might explore numerous factors to determine which ones might contribute to the ultimate outcome.
In many cases, researchers may find that the results of an experiment do not support the original hypothesis. When writing up these results, the researchers might suggest other options that should be explored in future studies.
In many cases, researchers might draw a hypothesis from a specific theory or build on previous research. For example, prior research has shown that stress can impact the immune system. So a researcher might hypothesize: "People with high-stress levels will be more likely to contract a common cold after being exposed to the virus than people who have low-stress levels."
In other instances, researchers might look at commonly held beliefs or folk wisdom. "Birds of a feather flock together" is one example of folk adage that a psychologist might try to investigate. The researcher might pose a specific hypothesis that "People tend to select romantic partners who are similar to them in interests and educational level."
Elements of a Good Hypothesis
So how do you write a good hypothesis? When trying to come up with a hypothesis for your research or experiments, ask yourself the following questions:
- Is your hypothesis based on your research on a topic?
- Can your hypothesis be tested?
- Does your hypothesis include independent and dependent variables?
Before you come up with a specific hypothesis, spend some time doing background research. Once you have completed a literature review, start thinking about potential questions you still have. Pay attention to the discussion section in the journal articles you read . Many authors will suggest questions that still need to be explored.
How to Formulate a Good Hypothesis
To form a hypothesis, you should take these steps:
- Collect as many observations about a topic or problem as you can.
- Evaluate these observations and look for possible causes of the problem.
- Create a list of possible explanations that you might want to explore.
- After you have developed some possible hypotheses, think of ways that you could confirm or disprove each hypothesis through experimentation. This is known as falsifiability.
In the scientific method , falsifiability is an important part of any valid hypothesis. In order to test a claim scientifically, it must be possible that the claim could be proven false.
Students sometimes confuse the idea of falsifiability with the idea that it means that something is false, which is not the case. What falsifiability means is that if something was false, then it is possible to demonstrate that it is false.
One of the hallmarks of pseudoscience is that it makes claims that cannot be refuted or proven false.
The Importance of Operational Definitions
A variable is a factor or element that can be changed and manipulated in ways that are observable and measurable. However, the researcher must also define how the variable will be manipulated and measured in the study.
Operational definitions are specific definitions for all relevant factors in a study. This process helps make vague or ambiguous concepts detailed and measurable.
For example, a researcher might operationally define the variable " test anxiety " as the results of a self-report measure of anxiety experienced during an exam. A "study habits" variable might be defined by the amount of studying that actually occurs as measured by time.
These precise descriptions are important because many things can be measured in various ways. Clearly defining these variables and how they are measured helps ensure that other researchers can replicate your results.
Replicability
One of the basic principles of any type of scientific research is that the results must be replicable.
Replication means repeating an experiment in the same way to produce the same results. By clearly detailing the specifics of how the variables were measured and manipulated, other researchers can better understand the results and repeat the study if needed.
Some variables are more difficult than others to define. For example, how would you operationally define a variable such as aggression ? For obvious ethical reasons, researchers cannot create a situation in which a person behaves aggressively toward others.
To measure this variable, the researcher must devise a measurement that assesses aggressive behavior without harming others. The researcher might utilize a simulated task to measure aggressiveness in this situation.
Hypothesis Checklist
- Does your hypothesis focus on something that you can actually test?
- Does your hypothesis include both an independent and dependent variable?
- Can you manipulate the variables?
- Can your hypothesis be tested without violating ethical standards?
The hypothesis you use will depend on what you are investigating and hoping to find. Some of the main types of hypotheses that you might use include:
- Simple hypothesis : This type of hypothesis suggests there is a relationship between one independent variable and one dependent variable.
- Complex hypothesis : This type suggests a relationship between three or more variables, such as two independent and dependent variables.
- Null hypothesis : This hypothesis suggests no relationship exists between two or more variables.
- Alternative hypothesis : This hypothesis states the opposite of the null hypothesis.
- Statistical hypothesis : This hypothesis uses statistical analysis to evaluate a representative population sample and then generalizes the findings to the larger group.
- Logical hypothesis : This hypothesis assumes a relationship between variables without collecting data or evidence.
A hypothesis often follows a basic format of "If {this happens} then {this will happen}." One way to structure your hypothesis is to describe what will happen to the dependent variable if you change the independent variable .
The basic format might be: "If {these changes are made to a certain independent variable}, then we will observe {a change in a specific dependent variable}."
A few examples of simple hypotheses:
- "Students who eat breakfast will perform better on a math exam than students who do not eat breakfast."
- "Students who experience test anxiety before an English exam will get lower scores than students who do not experience test anxiety."
- "Motorists who talk on the phone while driving will be more likely to make errors on a driving course than those who do not talk on the phone."
- "Children who receive a new reading intervention will have higher reading scores than students who do not receive the intervention."
Examples of a complex hypothesis include:
- "People with high-sugar diets and sedentary activity levels are more likely to develop depression."
- "Younger people who are regularly exposed to green, outdoor areas have better subjective well-being than older adults who have limited exposure to green spaces."
Examples of a null hypothesis include:
- "There is no difference in anxiety levels between people who take St. John's wort supplements and those who do not."
- "There is no difference in scores on a memory recall task between children and adults."
- "There is no difference in aggression levels between children who play first-person shooter games and those who do not."
Examples of an alternative hypothesis:
- "People who take St. John's wort supplements will have less anxiety than those who do not."
- "Adults will perform better on a memory task than children."
- "Children who play first-person shooter games will show higher levels of aggression than children who do not."
Collecting Data on Your Hypothesis
Once a researcher has formed a testable hypothesis, the next step is to select a research design and start collecting data. The research method depends largely on exactly what they are studying. There are two basic types of research methods: descriptive research and experimental research.
Descriptive Research Methods
Descriptive research such as case studies , naturalistic observations , and surveys are often used when conducting an experiment is difficult or impossible. These methods are best used to describe different aspects of a behavior or psychological phenomenon.
Once a researcher has collected data using descriptive methods, a correlational study can examine how the variables are related. This research method might be used to investigate a hypothesis that is difficult to test experimentally.
Experimental Research Methods
Experimental methods are used to demonstrate causal relationships between variables. In an experiment, the researcher systematically manipulates a variable of interest (known as the independent variable) and measures the effect on another variable (known as the dependent variable).
Unlike correlational studies, which can only be used to determine if there is a relationship between two variables, experimental methods can be used to determine the actual nature of the relationship—whether changes in one variable actually cause another to change.
The hypothesis is a critical part of any scientific exploration. It represents what researchers expect to find in a study or experiment. In situations where the hypothesis is unsupported by the research, the research still has value. Such research helps us better understand how different aspects of the natural world relate to one another. It also helps us develop new hypotheses that can then be tested in the future.
Thompson WH, Skau S. On the scope of scientific hypotheses . R Soc Open Sci . 2023;10(8):230607. doi:10.1098/rsos.230607
Taran S, Adhikari NKJ, Fan E. Falsifiability in medicine: what clinicians can learn from Karl Popper [published correction appears in Intensive Care Med. 2021 Jun 17;:]. Intensive Care Med . 2021;47(9):1054-1056. doi:10.1007/s00134-021-06432-z
Eyler AA. Research Methods for Public Health . 1st ed. Springer Publishing Company; 2020. doi:10.1891/9780826182067.0004
Nosek BA, Errington TM. What is replication ? PLoS Biol . 2020;18(3):e3000691. doi:10.1371/journal.pbio.3000691
Aggarwal R, Ranganathan P. Study designs: Part 2 - Descriptive studies . Perspect Clin Res . 2019;10(1):34-36. doi:10.4103/picr.PICR_154_18
Nevid J. Psychology: Concepts and Applications. Wadworth, 2013.
By Kendra Cherry, MSEd Kendra Cherry, MS, is a psychosocial rehabilitation specialist, psychology educator, and author of the "Everything Psychology Book."
- Data, AI, & Machine Learning
- Managing Technology
- Social Responsibility
- Workplace, Teams, & Culture
- AI & Machine Learning
- Diversity & Inclusion
- Big ideas Research Projects
- Artificial Intelligence and Business Strategy
- Responsible AI
- Future of the Workforce
- Future of Leadership
- All Research Projects
- AI in Action
- Most Popular
- The Truth Behind the Nursing Crisis
- Work/23: The Big Shift
- Coaching for the Future-Forward Leader
- Measuring Culture
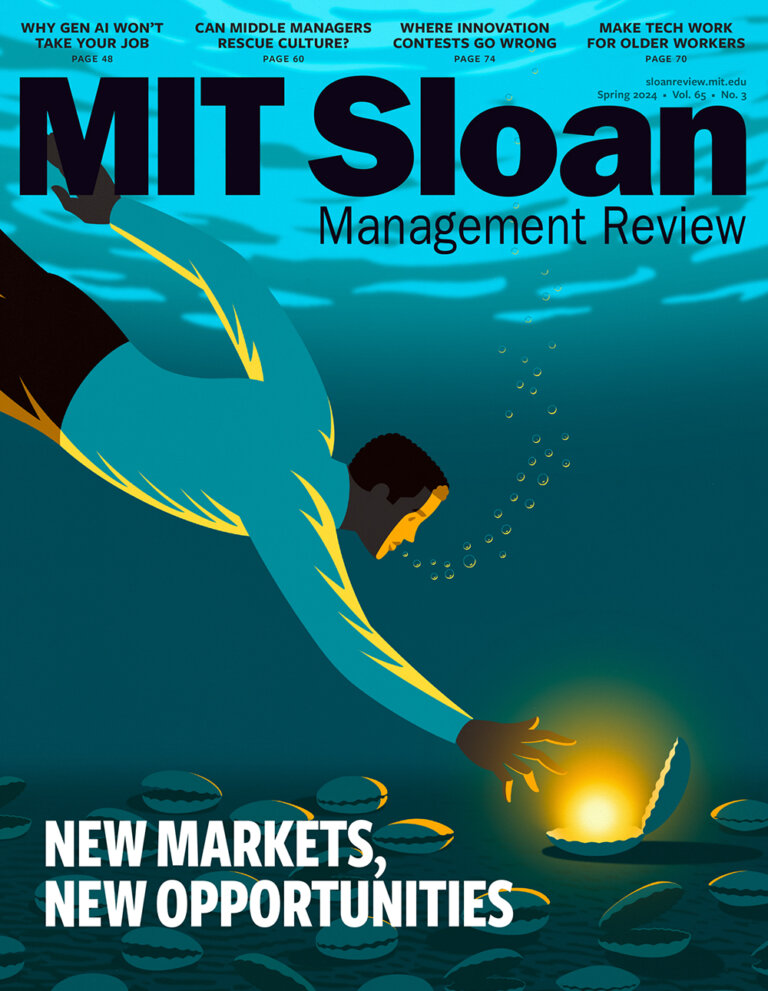
The spring 2024 issue’s special report looks at how to take advantage of market opportunities in the digital space, and provides advice on building culture and friendships at work; maximizing the benefits of LLMs, corporate venture capital initiatives, and innovation contests; and scaling automation and digital health platform.
- Past Issues
- Upcoming Events
- Video Archive
- Me, Myself, and AI
- Three Big Points
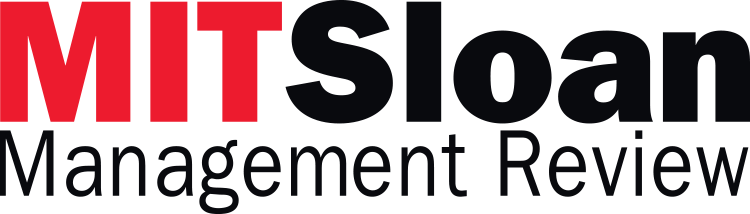
Why Hypotheses Beat Goals
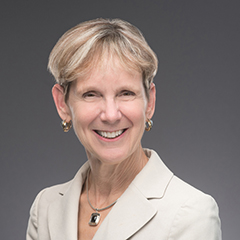
- Developing Strategy
- Skills & Learning
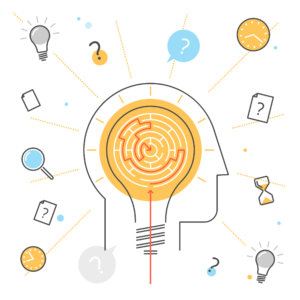
Not long ago, it became fashionable to embrace failure as a sign of a company’s willingness to take risks. This trend lost favor as executives recognized that what they wanted was learning, not necessarily failure. Every failure can be attributed to a raft of missteps, and many failures do not automatically contribute to future success.
Certainly, if companies want to aggressively pursue learning, they must accept that failures will happen. But the practice of simply setting goals and then being nonchalant if they fail is inadequate.
Instead, companies should focus organizational energy on hypothesis generation and testing. Hypotheses force individuals to articulate in advance why they believe a given course of action will succeed. A failure then exposes an incorrect hypothesis — which can more reliably convert into organizational learning.
What Exactly Is a Hypothesis?
When my son was in second grade, his teacher regularly introduced topics by asking students to state some initial assumptions. For example, she introduced a unit on whales by asking: How big is a blue whale? The students all knew blue whales were big, but how big? Guesses ranged from the size of the classroom to the size of two elephants to the length of all the students in class lined up in a row. Students then set out to measure the classroom and the length of the row they formed, and they looked up the size of an elephant. They compared their results with the measurements of the whale and learned how close their estimates were.
Note that in this example, there is much more going on than just learning the size of a whale. Students were learning to recognize assumptions, make intelligent guesses based on those assumptions, determine how to test the accuracy of their guesses, and then assess the results.
This is the essence of hypothesis generation. A hypothesis emerges from a set of underlying assumptions. It is an articulation of how those assumptions are expected to play out in a given context. In short, a hypothesis is an intelligent, articulated guess that is the basis for taking action and assessing outcomes.
Get Updates on Transformative Leadership
Evidence-based resources that can help you lead your team more effectively, delivered to your inbox monthly.
Please enter a valid email address
Thank you for signing up
Privacy Policy
Hypothesis generation in companies becomes powerful if people are forced to articulate and justify their assumptions. It makes the path from hypothesis to expected outcomes clear enough that, should the anticipated outcomes fail to materialize, people will agree that the hypothesis was faulty.
Building a culture of effective hypothesizing can lead to more thoughtful actions and a better understanding of outcomes. Not only will failures be more likely to lead to future successes, but successes will foster future successes.
Why Is Hypothesis Generation Important?
Digital technologies are creating new business opportunities, but as I’ve noted in earlier columns , companies must experiment to learn both what is possible and what customers want. Most companies are relying on empowered, agile teams to conduct these experiments. That’s because teams can rapidly hypothesize, test, and learn.
Hypothesis generation contrasts starkly with more traditional management approaches designed for process optimization. Process optimization involves telling employees both what to do and how to do it. Process optimization is fine for stable business processes that have been standardized for consistency. (Standardized processes can usually be automated, specifically because they are stable.) Increasingly, however, companies need their people to steer efforts that involve uncertainty and change. That’s when organizational learning and hypothesis generation are particularly important.
Shifting to a culture that encourages empowered teams to hypothesize isn’t easy. Established hierarchies have developed managers accustomed to directing employees on how to accomplish their objectives. Those managers invariably rose to power by being the smartest person in the room. Such managers can struggle with the requirements for leading empowered teams. They may recognize the need to hold teams accountable for outcomes rather than specific tasks, but they may not be clear about how to guide team efforts.
Some newer companies have baked this concept into their organizational structure. Leaders at the Swedish digital music service Spotify note that it is essential to provide clear missions to teams . A clear mission sets up a team to articulate measurable goals. Teams can then hypothesize how they can best accomplish those goals. The role of leaders is to quiz teams about their hypotheses and challenge their logic if those hypotheses appear to lack support.
A leader at another company told me that accountability for outcomes starts with hypotheses. If a team cannot articulate what it intends to do and what outcomes it anticipates, it is unlikely that team will deliver on its mission. In short, the success of empowered teams depends upon management shifting from directing employees to guiding their development of hypotheses. This is how leaders hold their teams accountable for outcomes.
Members of empowered teams are not the only people who need to hone their ability to hypothesize. Leaders in companies that want to seize digital opportunities are learning through their experiments which strategies hold real promise for future success. They must, in effect, hypothesize about what will make the company successful in a digital economy. If they take the next step and articulate those hypotheses and establish metrics for assessing the outcomes of their actions, they will facilitate learning about the company’s long-term success. Hypothesis generation can become a critical competency throughout a company.
How Does a Company Become Proficient at Hypothesizing?
Most business leaders have embraced the importance of evidence-based decision-making. But developing a culture of evidence-based decision-making by promoting hypothesis generation is a new challenge.
For one thing, many hypotheses are sloppy. While many people naturally hypothesize and take actions based on their hypotheses, their underlying assumptions may go unexamined. Often, they don’t clearly articulate the premise itself. The better hypotheses are straightforward and succinctly written. They’re pointed about the suppositions they’re based on. And they’re shared, allowing an audience to examine the assumptions (are they accurate?) and the postulate itself (is it an intelligent, articulated guess that is the basis for taking action and assessing outcomes?).
Related Articles
Seven-Eleven Japan offers a case in how do to hypotheses right.
For over 30 years, Seven-Eleven Japan was the most profitable retailer in Japan. It achieved that stature by relying on each store’s salesclerks to decide what items to stock on that store’s shelves. Many of the salesclerks were part-time, but they were each responsible for maximizing turnover for one part of the store’s inventory, and they received detailed reports so they could monitor their own performance.
The language of hypothesis formulation was part of their process. Each week, Seven-Eleven Japan counselors visited the stores and asked salesclerks three questions:
- What did you hypothesize this week? (That is, what did you order?)
- How did you do? (That is, did you sell what you ordered?)
- How will you do better next week? (That is, how will you incorporate the learning?)
By repeatedly asking these questions and checking the data for results, counselors helped people throughout the company hypothesize, test, and learn. The result was consistently strong inventory turnover and profitability.
How can other companies get started on this path? Evidence-based decision-making requires data — good data, as the Seven-Eleven Japan example shows. But rather than get bogged down with the limits of a company’s data, I would argue that companies can start to change their culture by constantly exposing individual hypotheses. Those hypotheses will highlight what data matters most — and the need of teams to test hypotheses will help generate enthusiasm for cleaning up bad data. A sense of accountability for generating and testing hypotheses then fosters a culture of evidence-based decision-making.
The uncertainties and speed of change in the current business environment render traditional management approaches ineffective. To create the agile, evidence-based, learning culture your business needs to succeed in a digital economy, I suggest that instead of asking What is your goal? you make it a habit to ask What is your hypothesis?
About the Author
Jeanne Ross is principal research scientist for MIT’s Center for Information Systems Research . Follow CISR on Twitter @mit_cisr .
More Like This
Add a comment cancel reply.
You must sign in to post a comment. First time here? Sign up for a free account : Comment on articles and get access to many more articles.
Comment (1)
Richard jones.
Hypothesis Maker Online
Looking for a hypothesis maker? This online tool for students will help you formulate a beautiful hypothesis quickly, efficiently, and for free.
Are you looking for an effective hypothesis maker online? Worry no more; try our online tool for students and formulate your hypothesis within no time.
- 🔎 How to Use the Tool?
- ⚗️ What Is a Hypothesis in Science?
👍 What Does a Good Hypothesis Mean?
- 🧭 Steps to Making a Good Hypothesis
🔗 References
📄 hypothesis maker: how to use it.
Our hypothesis maker is a simple and efficient tool you can access online for free.
If you want to create a research hypothesis quickly, you should fill out the research details in the given fields on the hypothesis generator.
Below are the fields you should complete to generate your hypothesis:
- Who or what is your research based on? For instance, the subject can be research group 1.
- What does the subject (research group 1) do?
- What does the subject affect? - This shows the predicted outcome, which is the object.
- Who or what will be compared with research group 1? (research group 2).
Once you fill the in the fields, you can click the ‘Make a hypothesis’ tab and get your results.
⚗️ What Is a Hypothesis in the Scientific Method?
A hypothesis is a statement describing an expectation or prediction of your research through observation.
It is similar to academic speculation and reasoning that discloses the outcome of your scientific test . An effective hypothesis, therefore, should be crafted carefully and with precision.
A good hypothesis should have dependent and independent variables . These variables are the elements you will test in your research method – it can be a concept, an event, or an object as long as it is observable.
You can observe the dependent variables while the independent variables keep changing during the experiment.
In a nutshell, a hypothesis directs and organizes the research methods you will use, forming a large section of research paper writing.
Hypothesis vs. Theory
A hypothesis is a realistic expectation that researchers make before any investigation. It is formulated and tested to prove whether the statement is true. A theory, on the other hand, is a factual principle supported by evidence. Thus, a theory is more fact-backed compared to a hypothesis.
Another difference is that a hypothesis is presented as a single statement , while a theory can be an assortment of things . Hypotheses are based on future possibilities toward a specific projection, but the results are uncertain. Theories are verified with undisputable results because of proper substantiation.
When it comes to data, a hypothesis relies on limited information , while a theory is established on an extensive data set tested on various conditions.
You should observe the stated assumption to prove its accuracy.
Since hypotheses have observable variables, their outcome is usually based on a specific occurrence. Conversely, theories are grounded on a general principle involving multiple experiments and research tests.
This general principle can apply to many specific cases.
The primary purpose of formulating a hypothesis is to present a tentative prediction for researchers to explore further through tests and observations. Theories, in their turn, aim to explain plausible occurrences in the form of a scientific study.
It would help to rely on several criteria to establish a good hypothesis. Below are the parameters you should use to analyze the quality of your hypothesis.
🧭 6 Steps to Making a Good Hypothesis
Writing a hypothesis becomes way simpler if you follow a tried-and-tested algorithm. Let’s explore how you can formulate a good hypothesis in a few steps:
Step #1: Ask Questions
The first step in hypothesis creation is asking real questions about the surrounding reality.
Why do things happen as they do? What are the causes of some occurrences?
Your curiosity will trigger great questions that you can use to formulate a stellar hypothesis. So, ensure you pick a research topic of interest to scrutinize the world’s phenomena, processes, and events.
Step #2: Do Initial Research
Carry out preliminary research and gather essential background information about your topic of choice.
The extent of the information you collect will depend on what you want to prove.
Your initial research can be complete with a few academic books or a simple Internet search for quick answers with relevant statistics.
Still, keep in mind that in this phase, it is too early to prove or disapprove of your hypothesis.
Step #3: Identify Your Variables
Now that you have a basic understanding of the topic, choose the dependent and independent variables.
Take note that independent variables are the ones you can’t control, so understand the limitations of your test before settling on a final hypothesis.
Step #4: Formulate Your Hypothesis
You can write your hypothesis as an ‘if – then’ expression . Presenting any hypothesis in this format is reliable since it describes the cause-and-effect you want to test.
For instance: If I study every day, then I will get good grades.
Step #5: Gather Relevant Data
Once you have identified your variables and formulated the hypothesis, you can start the experiment. Remember, the conclusion you make will be a proof or rebuttal of your initial assumption.
So, gather relevant information, whether for a simple or statistical hypothesis, because you need to back your statement.
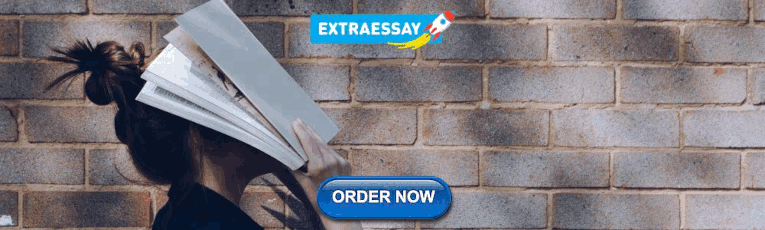
Step #6: Record Your Findings
Finally, write down your conclusions in a research paper .
Outline in detail whether the test has proved or disproved your hypothesis.
Edit and proofread your work, using a plagiarism checker to ensure the authenticity of your text.
We hope that the above tips will be useful for you. Note that if you need to conduct business analysis, you can use the free templates we’ve prepared: SWOT , PESTLE , VRIO , SOAR , and Porter’s 5 Forces .
❓ Hypothesis Formulator FAQ
Updated: Oct 25th, 2023
- How to Write a Hypothesis in 6 Steps - Grammarly
- Forming a Good Hypothesis for Scientific Research
- The Hypothesis in Science Writing
- Scientific Method: Step 3: HYPOTHESIS - Subject Guides
- Hypothesis Template & Examples - Video & Lesson Transcript
- Free Essays
- Writing Tools
- Lit. Guides
- Donate a Paper
- Referencing Guides
- Free Textbooks
- Tongue Twisters
- Job Openings
- Expert Application
- Video Contest
- Writing Scholarship
- Discount Codes
- IvyPanda Shop
- Terms and Conditions
- Privacy Policy
- Cookies Policy
- Copyright Principles
- DMCA Request
- Service Notice
Use our hypothesis maker whenever you need to formulate a hypothesis for your study. We offer a very simple tool where you just need to provide basic info about your variables, subjects, and predicted outcomes. The rest is on us. Get a perfect hypothesis in no time!
Type of Research projects Part 2: Hypothesis-driven versus hypothesis-generating research (1 August 2018)
How to Generate and Validate Product Hypotheses

Every product owner knows that it takes effort to build something that'll cater to user needs. You'll have to make many tough calls if you wish to grow the company and evolve the product so it delivers more value. But how do you decide what to change in the product, your marketing strategy, or the overall direction to succeed? And how do you make a product that truly resonates with your target audience?
There are many unknowns in business, so many fundamental decisions start from a simple "what if?". But they can't be based on guesses, as you need some proof to fill in the blanks reasonably.
Because there's no universal recipe for successfully building a product, teams collect data, do research, study the dynamics, and generate hypotheses according to the given facts. They then take corresponding actions to find out whether they were right or wrong, make conclusions, and most likely restart the process again.
On this page, we thoroughly inspect product hypotheses. We'll go over what they are, how to create hypothesis statements and validate them, and what goes after this step.
What Is a Hypothesis in Product Management?
A hypothesis in product development and product management is a statement or assumption about the product, planned feature, market, or customer (e.g., their needs, behavior, or expectations) that you can put to the test, evaluate, and base your further decisions on . This may, for instance, regard the upcoming product changes as well as the impact they can result in.
A hypothesis implies that there is limited knowledge. Hence, the teams need to undergo testing activities to validate their ideas and confirm whether they are true or false.
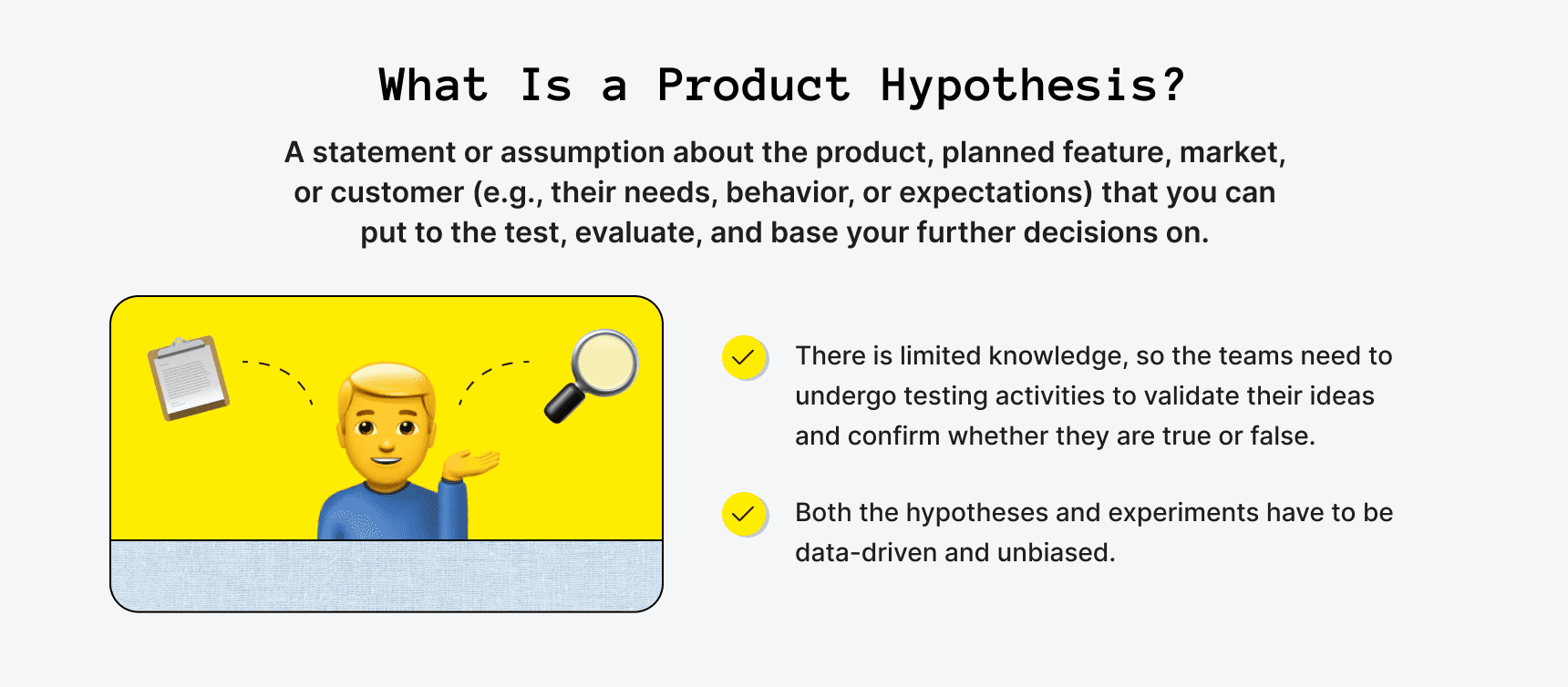
Hypotheses guide the product development process and may point at important findings to help build a better product that'll serve user needs. In essence, teams create hypothesis statements in an attempt to improve the offering, boost engagement, increase revenue, find product-market fit quicker, or for other business-related reasons.
It's sort of like an experiment with trial and error, yet, it is data-driven and should be unbiased . This means that teams don't make assumptions out of the blue. Instead, they turn to the collected data, conducted market research , and factual information, which helps avoid completely missing the mark. The obtained results are then carefully analyzed and may influence decision-making.
Such experiments backed by data and analysis are an integral aspect of successful product development and allow startups or businesses to dodge costly startup mistakes .
When do teams create hypothesis statements and validate them? To some extent, hypothesis testing is an ongoing process to work on constantly. It may occur during various product development life cycle stages, from early phases like initiation to late ones like scaling.
In any event, the key here is learning how to generate hypothesis statements and validate them effectively. We'll go over this in more detail later on.
Idea vs. Hypothesis Compared
You might be wondering whether ideas and hypotheses are the same thing. Well, there are a few distinctions.
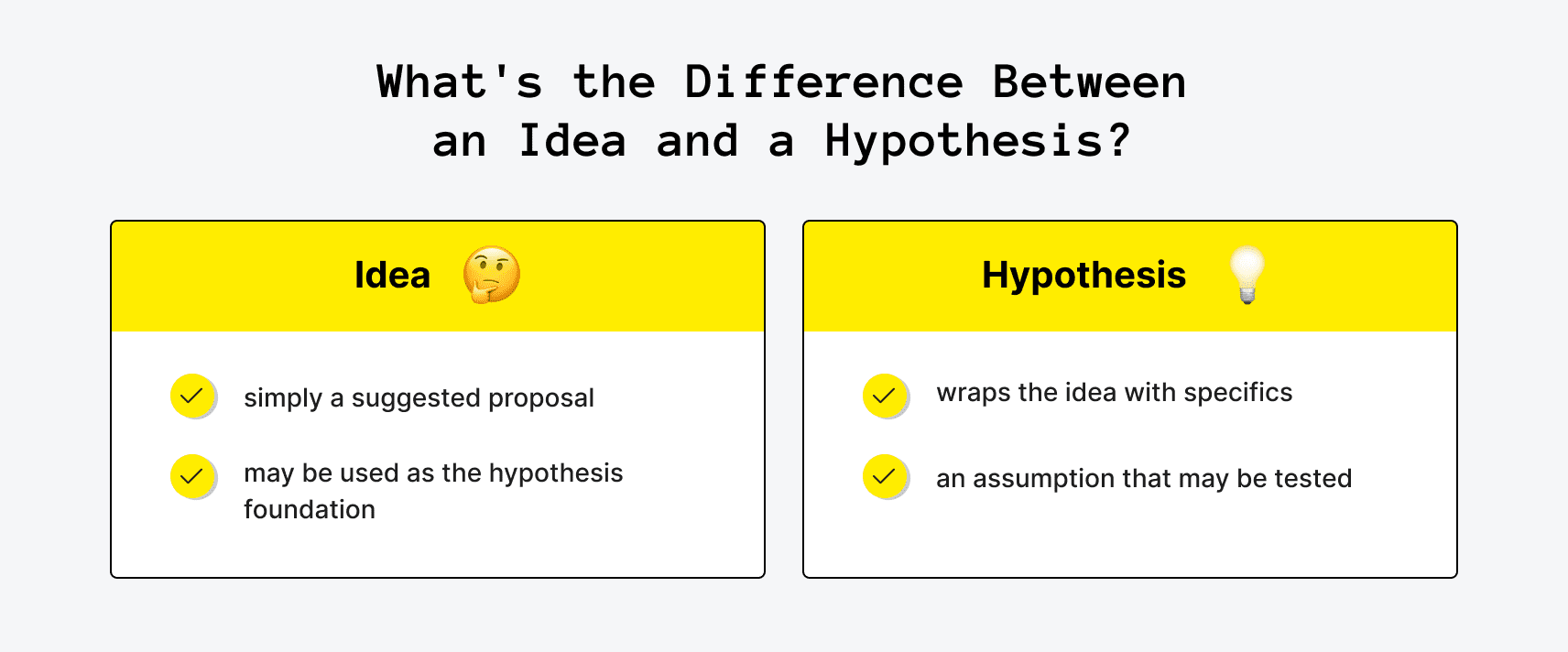
An idea is simply a suggested proposal. Say, a teammate comes up with something you can bring to life during a brainstorming session or pitches in a suggestion like "How about we shorten the checkout process?". You can jot down such ideas and then consider working on them if they'll truly make a difference and improve the product, strategy, or result in other business benefits. Ideas may thus be used as the hypothesis foundation when you decide to prove a concept.
A hypothesis is the next step, when an idea gets wrapped with specifics to become an assumption that may be tested. As such, you can refine the idea by adding details to it. The previously mentioned idea can be worded into a product hypothesis statement like: "The cart abandonment rate is high, and many users flee at checkout. But if we shorten the checkout process by cutting down the number of steps to only two and get rid of four excessive fields, we'll simplify the user journey, boost satisfaction, and may get up to 15% more completed orders".
A hypothesis is something you can test in an attempt to reach a certain goal. Testing isn't obligatory in this scenario, of course, but the idea may be tested if you weigh the pros and cons and decide that the required effort is worth a try. We'll explain how to create hypothesis statements next.
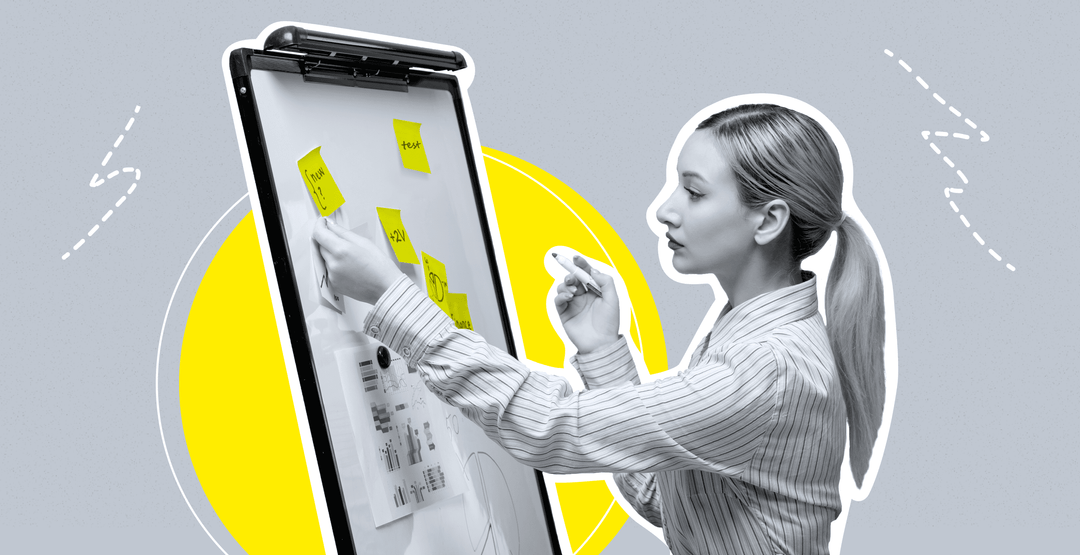
How to Generate a Hypothesis for a Product
The last thing those developing a product want is to invest time and effort into something that won't bring any visible results, fall short of customer expectations, or won't live up to their needs. Therefore, to increase the chances of achieving a successful outcome and product-led growth , teams may need to revisit their product development approach by optimizing one of the starting points of the process: learning to make reasonable product hypotheses.
If the entire procedure is structured, this may assist you during such stages as the discovery phase and raise the odds of reaching your product goals and setting your business up for success. Yet, what's the entire process like?
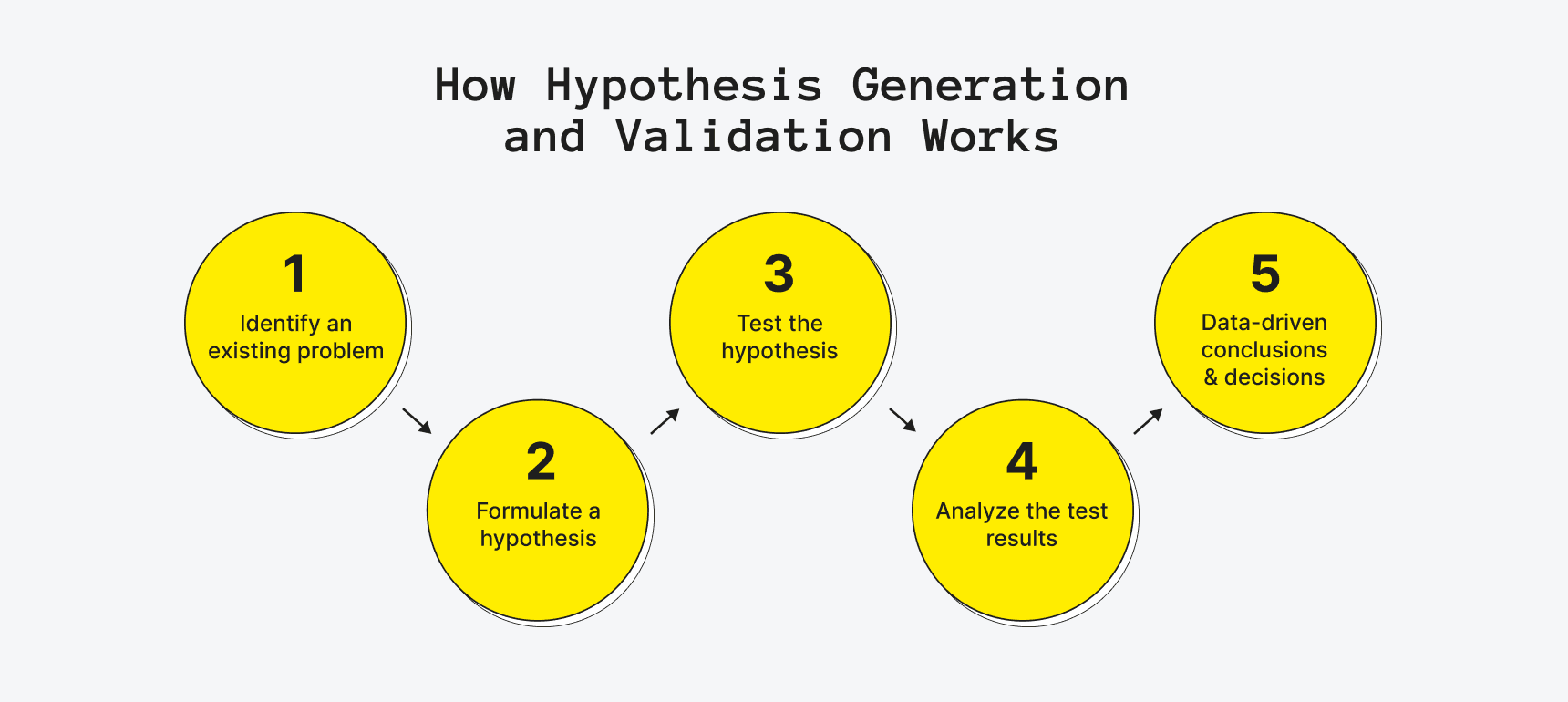
- It all starts with identifying an existing problem . Is there a product area that's experiencing a downfall, a visible trend, or a market gap? Are users often complaining about something in their feedback? Or is there something you're willing to change (say, if you aim to get more profit, increase engagement, optimize a process, expand to a new market, or reach your OKRs and KPIs faster)?
- Teams then need to work on formulating a hypothesis . They put the statement into concise and short wording that describes what is expected to achieve. Importantly, it has to be relevant, actionable, backed by data, and without generalizations.
- Next, they have to test the hypothesis by running experiments to validate it (for instance, via A/B or multivariate testing, prototyping, feedback collection, or other ways).
- Then, the obtained results of the test must be analyzed . Did one element or page version outperform the other? Depending on what you're testing, you can look into various merits or product performance metrics (such as the click rate, bounce rate, or the number of sign-ups) to assess whether your prediction was correct.
- Finally, the teams can make conclusions that could lead to data-driven decisions. For example, they can make corresponding changes or roll back a step.
How Else Can You Generate Product Hypotheses?
Such processes imply sharing ideas when a problem is spotted by digging deep into facts and studying the possible risks, goals, benefits, and outcomes. You may apply various MVP tools like (FigJam, Notion, or Miro) that were designed to simplify brainstorming sessions, systemize pitched suggestions, and keep everyone organized without losing any ideas.
Besides, you can settle on one of the many frameworks that facilitate decision-making processes , ideation phases, or feature prioritization . Such frameworks are best applicable if you need to test your assumptions and structure the validation process. These are a few common ones if you're looking toward a systematic approach:
- Business Model Canvas (used to establish the foundation of the business model and helps find answers to vitals like your value proposition, finding the right customer segment, or the ways to make revenue);
- Lean Startup framework (the lean startup framework uses a diagram-like format for capturing major processes and can be handy for testing various hypotheses like how much value a product brings or assumptions on personas, the problem, growth, etc.);
- Design Thinking Process (is all about interactive learning and involves getting an in-depth understanding of the customer needs and pain points, which can be formulated into hypotheses followed by simple prototypes and tests).
Need a hand with product development?
Upsilon's team of pros is ready to share our expertise in building tech products.
How to Make a Hypothesis Statement for a Product
Once you've indicated the addressable problem or opportunity and broken down the issue in focus, you need to work on formulating the hypotheses and associated tasks. By the way, it works the same way if you want to prove that something will be false (a.k.a null hypothesis).
If you're unsure how to write a hypothesis statement, let's explore the essential steps that'll set you on the right track.
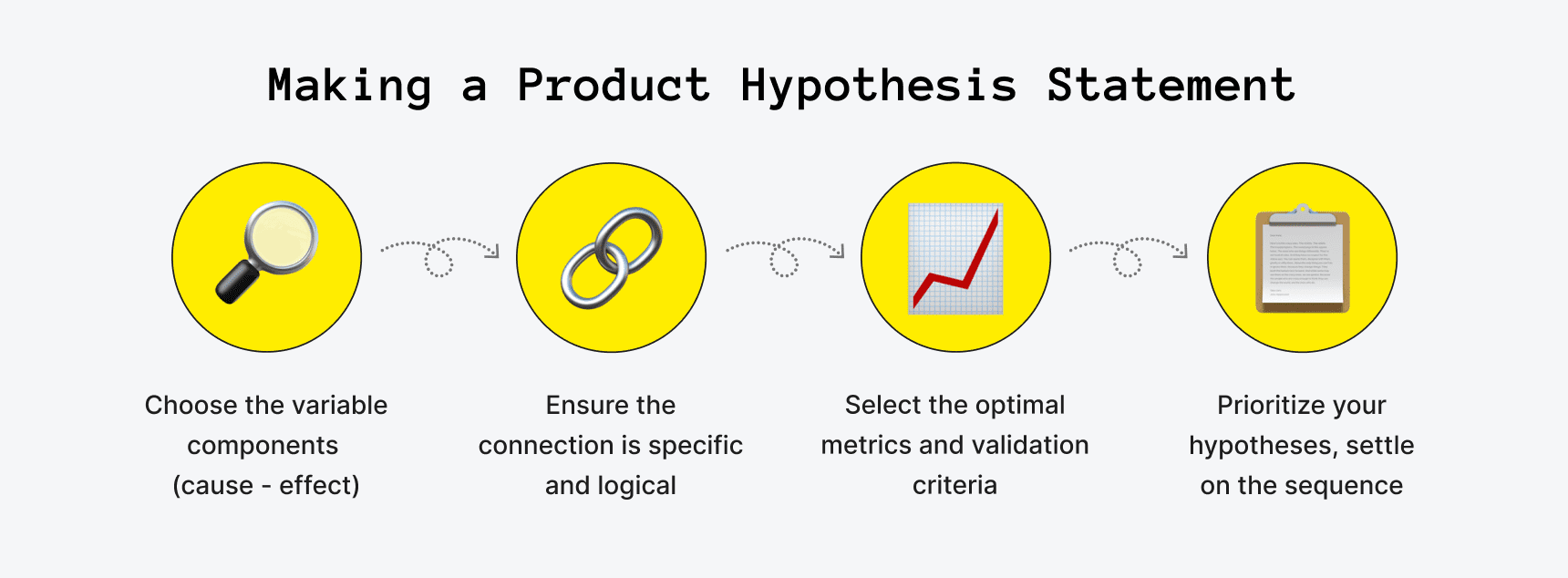
Step 1: Allocate the Variable Components
Product hypotheses are generally different for each case, so begin by pinpointing the major variables, i.e., the cause and effect . You'll need to outline what you think is supposed to happen if a change or action gets implemented.
Put simply, the "cause" is what you're planning to change, and the "effect" is what will indicate whether the change is bringing in the expected results. Falling back on the example we brought up earlier, the ineffective checkout process can be the cause, while the increased percentage of completed orders is the metric that'll show the effect.
Make sure to also note such vital points as:
- what the problem and solution are;
- what are the benefits or the expected impact/successful outcome;
- which user group is affected;
- what are the risks;
- what kind of experiments can help test the hypothesis;
- what can measure whether you were right or wrong.
Step 2: Ensure the Connection Is Specific and Logical
Mind that generic connections that lack specifics will get you nowhere. So if you're thinking about how to word a hypothesis statement, make sure that the cause and effect include clear reasons and a logical dependency .
Think about what can be the precise and link showing why A affects B. In our checkout example, it could be: fewer steps in the checkout and the removed excessive fields will speed up the process, help avoid confusion, irritate users less, and lead to more completed orders. That's much more explicit than just stating the fact that the checkout needs to be changed to get more completed orders.
Step 3: Decide on the Data You'll Collect
Certainly, multiple things can be used to measure the effect. Therefore, you need to choose the optimal metrics and validation criteria that'll best envision if you're moving in the right direction.
If you need a tip on how to create hypothesis statements that won't result in a waste of time, try to avoid vagueness and be as specific as you can when selecting what can best measure and assess the results of your hypothesis test. The criteria must be measurable and tied to the hypotheses . This can be a realistic percentage or number (say, you expect a 15% increase in completed orders or 2x fewer cart abandonment cases during the checkout phase).
Once again, if you're not realistic, then you might end up misinterpreting the results. Remember that sometimes an increase that's even as little as 2% can make a huge difference, so why make 50% the merit if it's not achievable in the first place?
Step 4: Settle on the Sequence
It's quite common that you'll end up with multiple product hypotheses. Some are more important than others, of course, and some will require more effort and input.
Therefore, just as with the features on your product development roadmap , prioritize your hypotheses according to their impact and importance. Then, group and order them, especially if the results of some hypotheses influence others on your list.
Product Hypothesis Examples
To demonstrate how to formulate your assumptions clearly, here are several more apart from the example of a hypothesis statement given above:
- Adding a wishlist feature to the cart with the possibility to send a gift hint to friends via email will increase the likelihood of making a sale and bring in additional sign-ups.
- Placing a limited-time promo code banner stripe on the home page will increase the number of sales in March.
- Moving up the call to action element on the landing page and changing the button text will increase the click-through rate twice.
- By highlighting a new way to use the product, we'll target a niche customer segment (i.e., single parents under 30) and acquire 5% more leads.
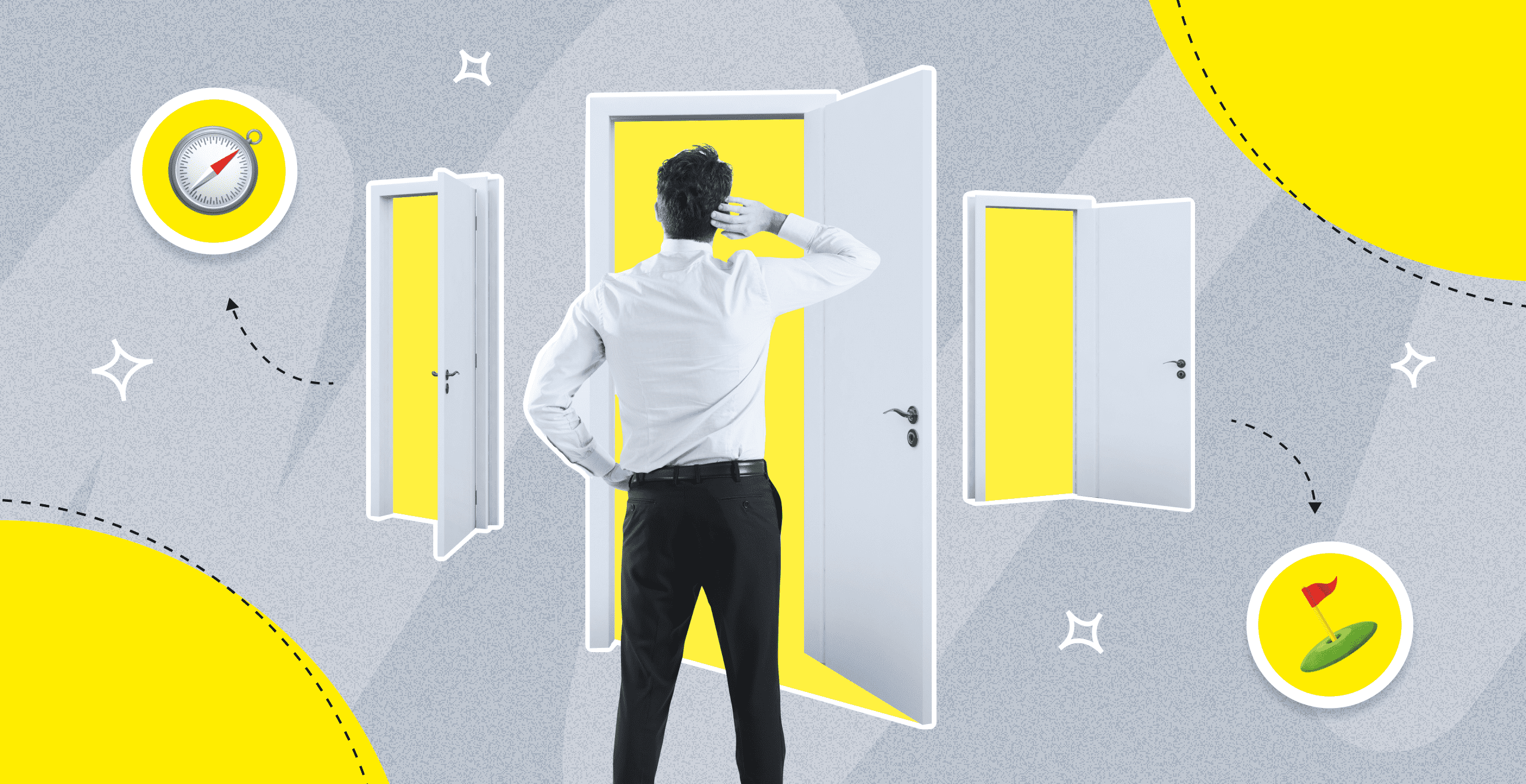
How to Validate Hypothesis Statements: The Process Explained
There are multiple options when it comes to validating hypothesis statements. To get appropriate results, you have to come up with the right experiment that'll help you test the hypothesis. You'll need a control group or people who represent your target audience segments or groups to participate (otherwise, your results might not be accurate).
What can serve as the experiment you may run? Experiments may take tons of different forms, and you'll need to choose the one that clicks best with your hypothesis goals (and your available resources, of course). The same goes for how long you'll have to carry out the test (say, a time period of two months or as little as two weeks). Here are several to get you started.
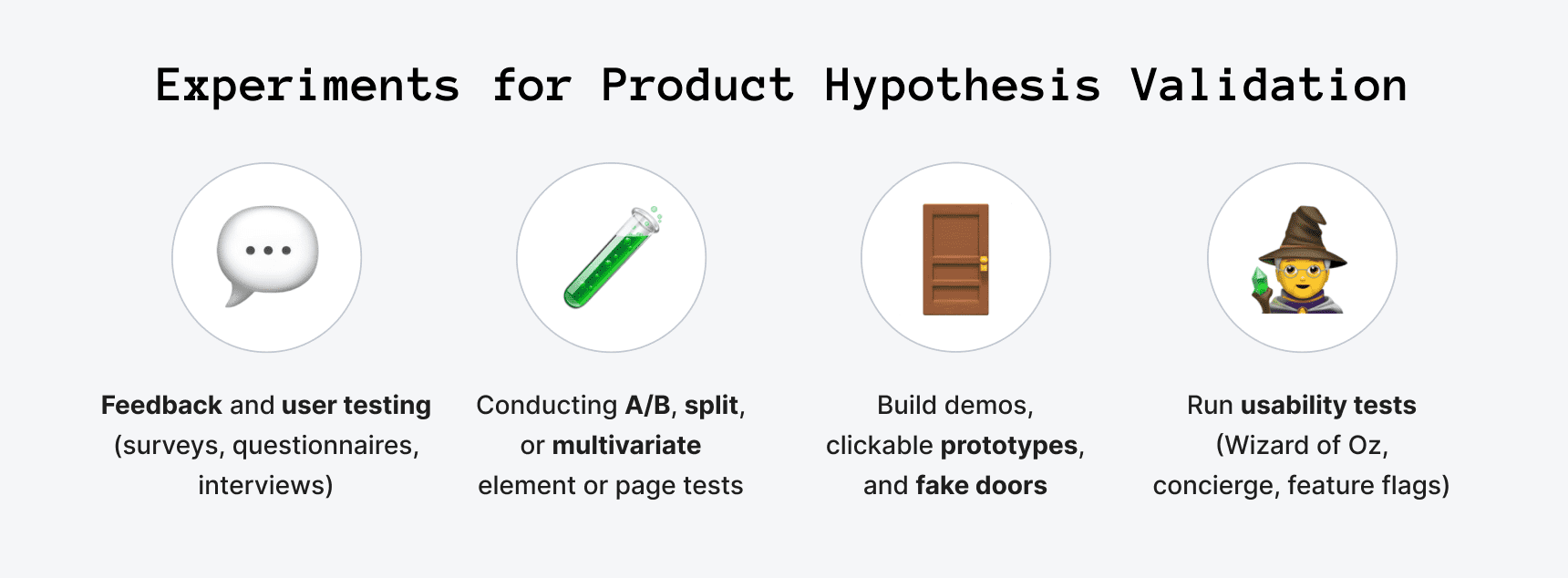
Feedback and User Testing
Talking to users, potential customers, or members of your own online startup community can be another way to test your hypotheses. You may use surveys, questionnaires, or opt for more extensive interviews to validate hypothesis statements and find out what people think. This assumption validation approach involves your existing or potential users and might require some additional time, but can bring you many insights.
Conduct A/B or Multivariate Tests
One of the experiments you may develop involves making more than one version of an element or page to see which option resonates with the users more. As such, you can have a call to action block with different wording or play around with the colors, imagery, visuals, and other things.
To run such split experiments, you can apply tools like VWO that allows to easily construct alternative designs and split what your users see (e.g., one half of the users will see version one, while the other half will see version two). You can track various metrics and apply heatmaps, click maps, and screen recordings to learn more about user response and behavior. Mind, though, that the key to such tests is to get as many users as you can give the tests time. Don't jump to conclusions too soon or if very few people participated in your experiment.
Build Prototypes and Fake Doors
Demos and clickable prototypes can be a great way to save time and money on costly feature or product development. A prototype also allows you to refine the design. However, they can also serve as experiments for validating hypotheses, collecting data, and getting feedback.
For instance, if you have a new feature in mind and want to ensure there is interest, you can utilize such MVP types as fake doors . Make a short demo recording of the feature and place it on your landing page to track interest or test how many people sign up.
Usability Testing
Similarly, you can run experiments to observe how users interact with the feature, page, product, etc. Usually, such experiments are held on prototype testing platforms with a focus group representing your target visitors. By showing a prototype or early version of the design to users, you can view how people use the solution, where they face problems, or what they don't understand. This may be very helpful if you have hypotheses regarding redesigns and user experience improvements before you move on from prototype to MVP development.
You can even take it a few steps further and build a barebone feature version that people can really interact with, yet you'll be the one behind the curtain to make it happen. There were many MVP examples when companies applied Wizard of Oz or concierge MVPs to validate their hypotheses.
Or you can actually develop some functionality but release it for only a limited number of people to see. This is referred to as a feature flag , which can show really specific results but is effort-intensive.
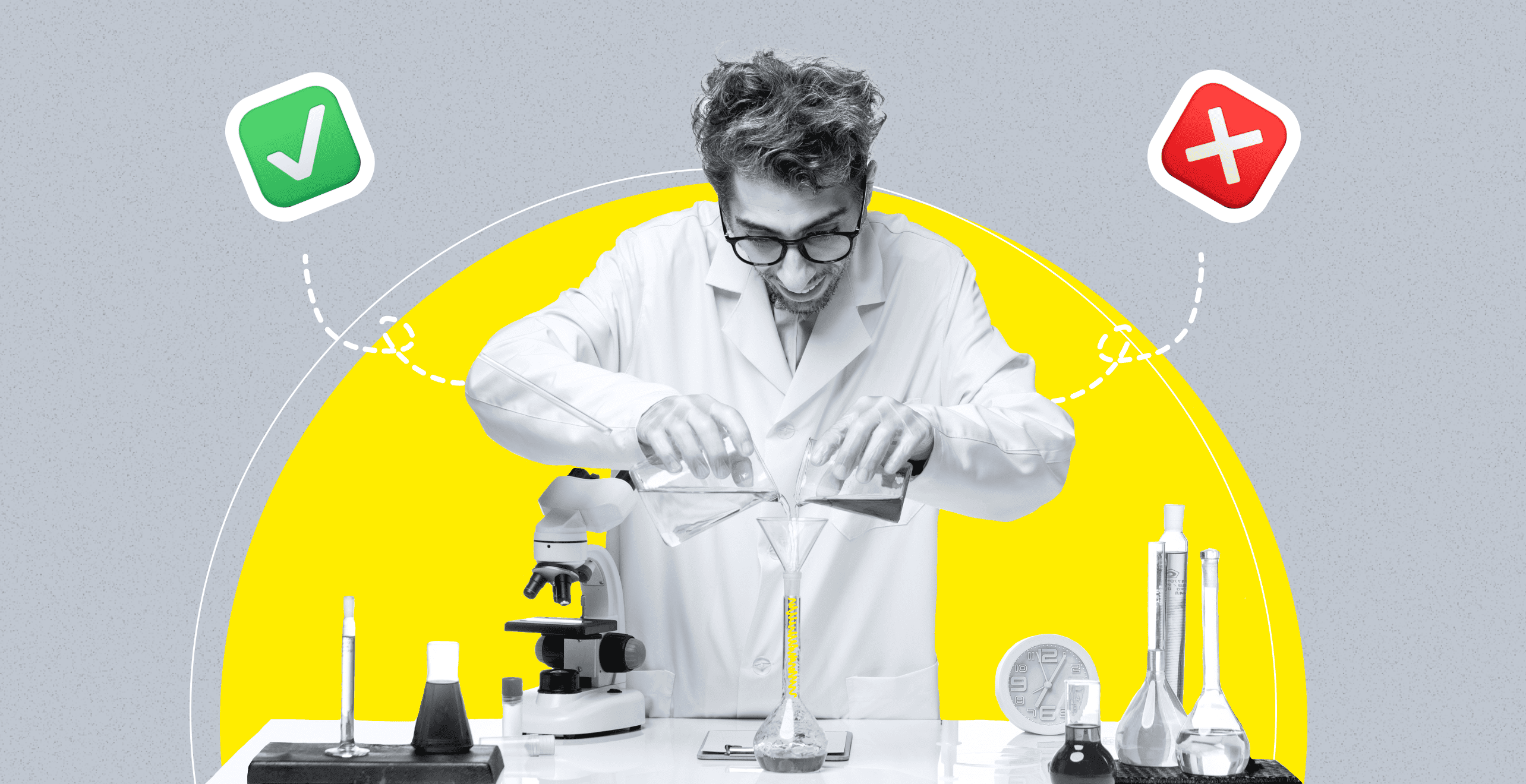
What Comes After Hypothesis Validation?
Analysis is what you move on to once you've run the experiment. This is the time to review the collected data, metrics, and feedback to validate (or invalidate) the hypothesis.
You have to evaluate the experiment's results to determine whether your product hypotheses were valid or not. For example, if you were testing two versions of an element design, color scheme, or copy, look into which one performed best.
It is crucial to be certain that you have enough data to draw conclusions, though, and that it's accurate and unbiased . Because if you don't, this may be a sign that your experiment needs to be run for some additional time, be altered, or held once again. You won't want to make a solid decision based on uncertain or misleading results, right?
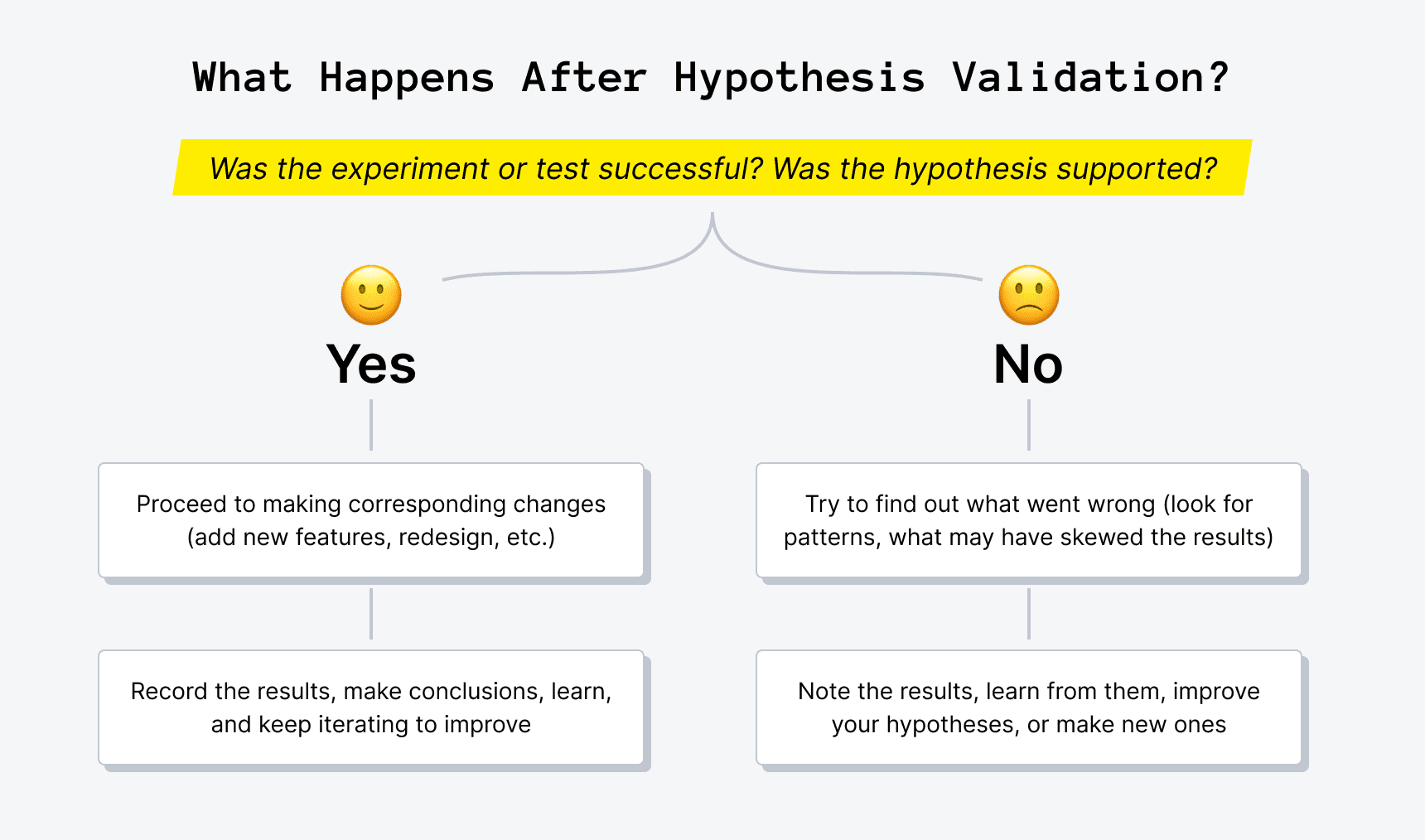
- If the hypothesis was supported , proceed to making corresponding changes (such as implementing a new feature, changing the design, rephrasing your copy, etc.). Remember that your aim was to learn and iterate to improve.
- If your hypothesis was proven false , think of it as a valuable learning experience. The main goal is to learn from the results and be able to adjust your processes accordingly. Dig deep to find out what went wrong, look for patterns and things that may have skewed the results. But if all signs show that you were wrong with your hypothesis, accept this outcome as a fact, and move on. This can help you make conclusions on how to better formulate your product hypotheses next time. Don't be too judgemental, though, as a failed experiment might only mean that you need to improve the current hypothesis, revise it, or create a new one based on the results of this experiment, and run the process once more.
On another note, make sure to record your hypotheses and experiment results . Some companies use CRMs to jot down the key findings, while others use something as simple as Google Docs. Either way, this can be your single source of truth that can help you avoid running the same experiments or allow you to compare results over time.
Have doubts about how to bring your product to life?
Upsilon's team of pros can help you build a product most optimally.
Final Thoughts on Product Hypotheses
The hypothesis-driven approach in product development is a great way to avoid uncalled-for risks and pricey mistakes. You can back up your assumptions with facts, observe your target audience's reactions, and be more certain that this move will deliver value.
However, this only makes sense if the validation of hypothesis statements is backed by relevant data that'll allow you to determine whether the hypothesis is valid or not. By doing so, you can be certain that you're developing and testing hypotheses to accelerate your product management and avoiding decisions based on guesswork.
Certainly, a failed experiment may bring you just as much knowledge and findings as one that succeeds. Teams have to learn from their mistakes, boost their hypothesis generation and testing knowledge, and make improvements according to the results of their experiments. This is an ongoing process, of course, as no product can grow if it isn't iterated and improved.
If you're only planning to or are currently building a product, Upsilon can lend you a helping hand. Our team has years of experience providing product development services for growth-stage startups and building MVPs for early-stage businesses , so you can use our expertise and knowledge to dodge many mistakes. Don't be shy to contact us to discuss your needs!
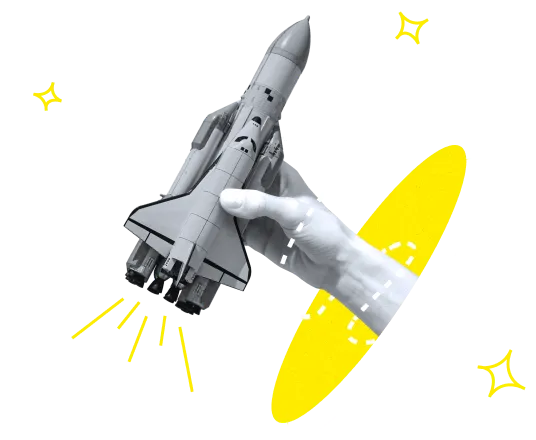
How to Prototype a Product: Steps, Tips, and Best Practices
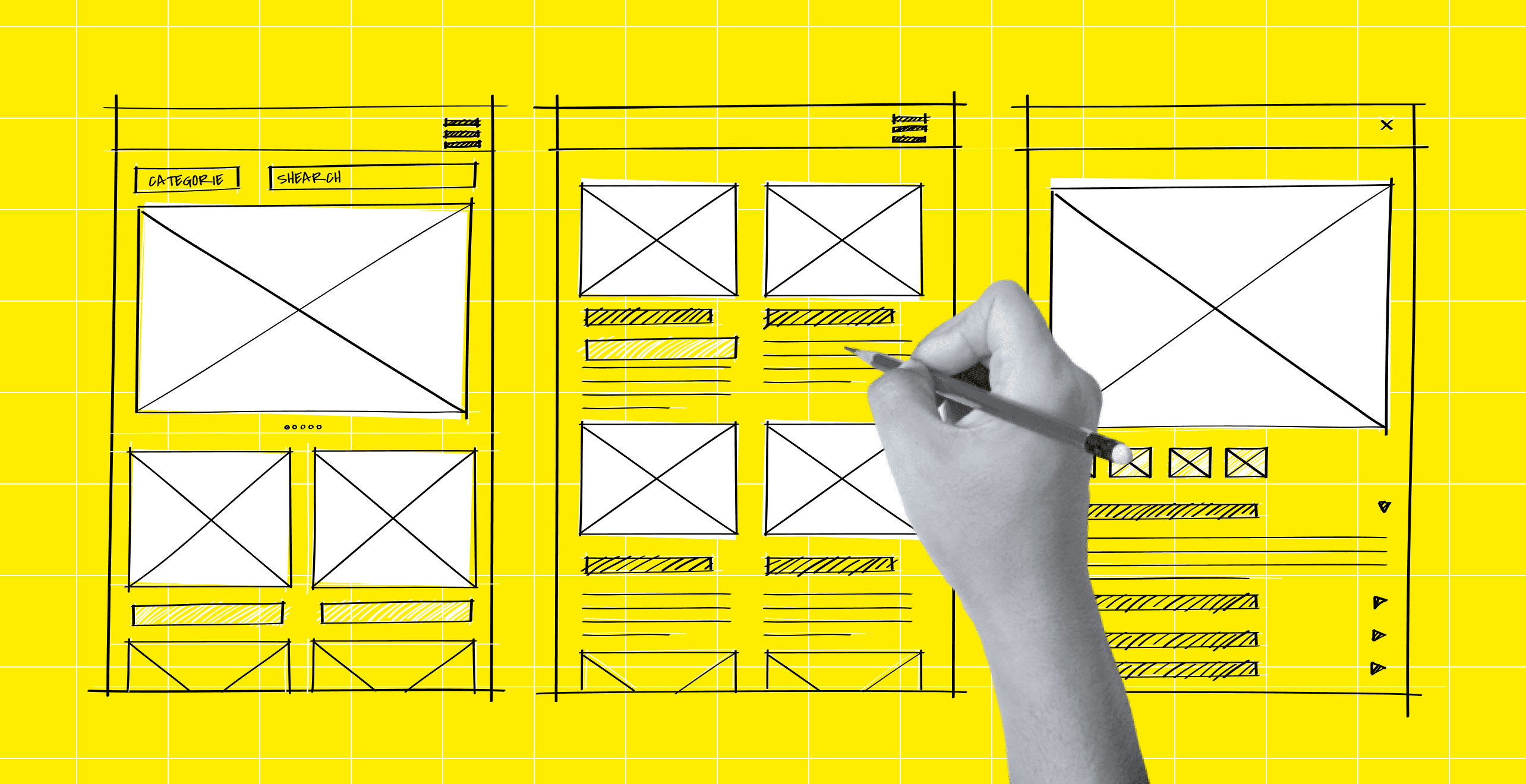
What Is Wireframing and Its Role in Product Development
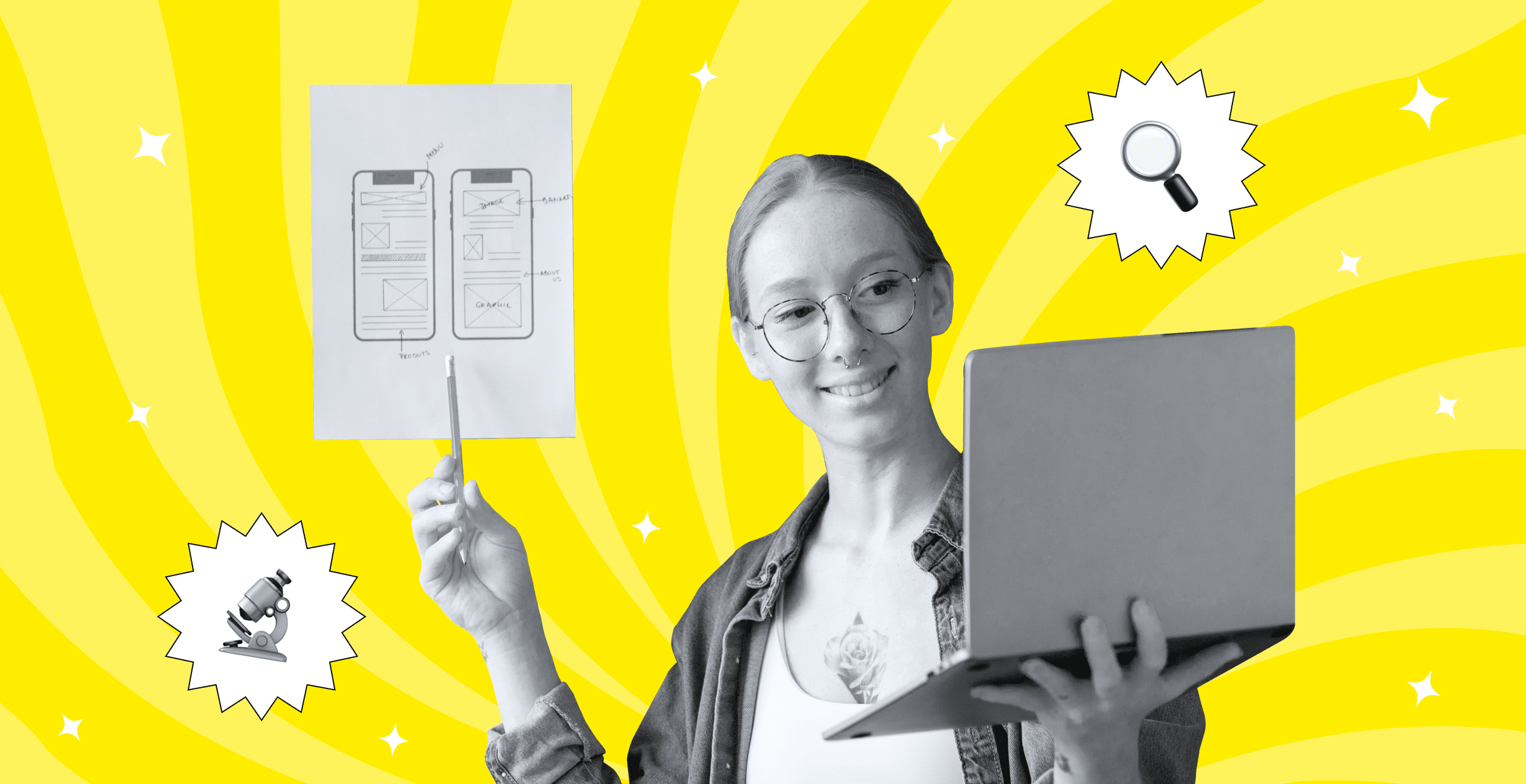
UX Discovery: Deliverables, Process, and Importance
Never miss an update.

Navigation Menu
Search code, repositories, users, issues, pull requests..., provide feedback.
We read every piece of feedback, and take your input very seriously.
Saved searches
Use saved searches to filter your results more quickly.
To see all available qualifiers, see our documentation .
- Notifications
This repository contains the code and data for the paper "ChatGPT4PCG 2 Competition: Prompt Engineering for Science Birds Level Generation".
chatgpt4pcg/experiments-2024
Folders and files, repository files navigation, chatgpt4pcg 2 competition: prompt engineering for science birds level generation.
This repository contains the code and datasets for the paper "ChatGPT4PCG 2 Competition: Prompt Engineering for Science Birds Level Generation" accepted at IEEE CoG 2024 .
Pittawat Taveekitworachai, Febri Abdullah, Mury F. Dewantoro, Yi Xia, Pratch Suntichaikul, Ruck Thawonmas, Julian Togelius, and Jochen Renz
This paper presents the second ChatGPT4PCG competition at the 2024 IEEE Conference on Games. In this edition of the competition, we follow the first edition, but make several improvements and changes. We introduce a new evaluation metric along with allowing a more flexible format for participants' submissions and making several improvements to the evaluation pipeline. Continuing from the first edition, we aim to foster and explore the realm of prompt engineering (PE) for procedural content generation (PCG). While the first competition saw success, it was hindered by various limitations; we aim to mitigate these limitations in this edition. We introduce diversity as a new metric to discourage submissions aimed at producing repetitive structures. Furthermore, we allow submission of a Python program instead of a prompt text file for greater flexibility in implementing advanced PE approaches, which may require control flow, including conditions and iterations. We also make several improvements to the evaluation pipeline with a better classifier for similarity evaluation and better-performing function signatures. We thoroughly evaluate the effectiveness of the new metric and the improved classifier. Additionally, we perform an ablation study to select a function signature to instruct ChatGPT for level generation. Finally, we provide implementation examples of various PE techniques in Python and evaluate their preliminary performance. We hope this competition serves as a resource and platform for learning about PE and PCG in general.
File structure
Installation and usage.
- Create a virtual environment (if needed):
and activate it:
- Copy .env.example and rename it to .env. . Follow instructions on this page to obtain your own OpenAI API key.
- Install the requirements:
- Run the code in the directories as needed.
- Jupyter Notebook 96.3%
- Python 3.7%

An official website of the United States government
The .gov means it’s official. Federal government websites often end in .gov or .mil. Before sharing sensitive information, make sure you’re on a federal government site.
The site is secure. The https:// ensures that you are connecting to the official website and that any information you provide is encrypted and transmitted securely.
- Publications
- Account settings
Preview improvements coming to the PMC website in October 2024. Learn More or Try it out now .
- Advanced Search
- Journal List
- PMC10635246

This is a preprint.
How do clinical researchers generate data-driven scientific hypotheses cognitive events using think-aloud protocol.
1 Department of Public Health Sciences, Clemson University, Clemson, SC
Brooke N. Draghi
Mytchell a. ernst, vimla l. patel.
2 Cognitive Studies in Medicine and Public Health, The New York Academy of Medicine, New York City, NY
James J. Cimino
3 Informatics Institute, School of Medicine, University of Alabama, Birmingham, Birmingham, AL
Jay H. Shubrook
4 College of Osteopathic Medicine, Touro University, Vallejo, CA
Yuchun Zhou
5 Patton College of Education, Ohio University, Athens, OH
6 Russ College of Engineering and Technology, Ohio University, Athens, OH
Sonsoles De Lacalle
7 Department of Health Science, California State University Channel Islands, Camarillo, CA
Associated Data
Objectives:.
This study aims to identify the cognitive events related to information use (e.g., “Analyze data”, “Seek connection”) during hypothesis generation among clinical researchers. Specifically, we describe hypothesis generation using cognitive event counts and compare them between groups.
The participants used the same datasets, followed the same scripts, used VIADS (a v isual i nteractive a nalysis tool for filtering and summarizing large d ata s ets coded with hierarchical terminologies) or other analytical tools (as control) to analyze the datasets, and came up with hypotheses while following the think-aloud protocol. Their screen activities and audio were recorded and then transcribed and coded for cognitive events.
The VIADS group exhibited the lowest mean number of cognitive events per hypothesis and the smallest standard deviation. The experienced clinical researchers had approximately 10% more valid hypotheses than the inexperienced group. The VIADS users among the inexperienced clinical researchers exhibit a similar trend as the experienced clinical researchers in terms of the number of cognitive events and their respective percentages out of all the cognitive events. The highest percentages of cognitive events in hypothesis generation were “Using analysis results” (30%) and “Seeking connections” (23%).
Conclusion:
VIADS helped inexperienced clinical researchers use fewer cognitive events to generate hypotheses than the control group. This suggests that VIADS may guide participants to be more structured during hypothesis generation compared with the control group. The results provide evidence to explain the shorter average time needed by the VIADS group in generating each hypothesis.
Introduction
A research hypothesis is an educated guess regarding relationships among different variables [ 1 , 2 ]. A research question typically comprises one to several scientific hypotheses that drive the direction of most research projects [ 1 , 3 – 5 ]. If we consider the life cycle of a research project, hypothesis generation constitutes its starting point. Without a significant, insightful, and novel hypothesis to begin with, it is difficult to have an impactful research project regardless of the study design, experiment implementation, and results analysis. Therefore, hypothesis generation plays a critical role in a research project. There are several studies investigating the mechanism of the generation of scientific hypotheses by researchers, both in science (e.g., Dunbar and Khar [ 6 , 7 ]) and in clinical medicine (e.g., Joseph and Patel [ 8 , 9 ]). However, none of these studies address how an analytic tool can be used to facilitate the hypothesis-generation process.
At least two categories of hypothesis are used frequently in scientific research. One is a hypothesis originating from experimental observations, e.g., any unusual phenomena observed during experiments in the context of “wet lab”. The other category is a hypothesis originating from the context of data analysis, for example, studies in epidemiology, genomics, and informatics [ 10 – 12 ]. Observations of unique or unusual phenomena in the first category and observations of trends in the second category are both critical in developing hypotheses [ 7 , 13 ]. Herein, we focus on the hypothesis generation within the second category.
In the past decades, there has been much work toward understanding scientific thinking and reasoning, medical reasoning, analogy, and working memory [ 7 , 14 ]. Educational settings and math problems were used to explore the reasoning process [ 15 – 17 ]. However, scientific hypothesis generation was not addressed, and the mechanism of explicit cognitive processes during scientific hypothesis generation remains unclear. The main differences between scientific reasoning and hypothesis generation include: a) the starting points of the two processes are different; many studies involving scientific reasoning start from an existing problem or puzzle [ 17 – 20 ], whereas data-driven hypothesis generation searches for a problem or a focus area to begin, named as open discovery by; Henry et al. [ 21 ]; b) the mechanisms between the start and end points of the two processes may differ, with convergent thinking used more in scientific reasoning when a question or a puzzle needs to be solved [ 7 ] and divergent thinking used more in data-driven scientific hypothesis generation. Meanwhile, hypothesis generation in medical diagnosis starts with a presented medical case or symptoms [ 19 , 22 ], which is similar to scientific reasoning.
We previously developed a conceptual framework for scientific hypothesis generation and its contributing factors [ 23 ]. Researchers have explored the possibilities of automatically generating scientific hypotheses in the past [ 10 , 24 – 28 ]; however, these authors recognized the challenges faced by an automated tool for such an advanced cognitive process [ 24 , 29 , 30 ].
Our study aims to obtain a better understanding regarding the scientific hypothesis generation process in clinical research. Considering hypotheses can directly impact and guide the direction of any research project, the findings of this work can potentially impact the clinical research enterprise. The research protocol [ 31 ], VIADS [ 32 – 34 ] (a v isual i nteractive a nalytic tool for filtering and summarizing large health d ata s ets coded with hierarchical terminologies—VIADS, a secondary data analytical tool developed by our team) usability [ 35 ], and quality evaluation of the hypotheses generated by participants [ 23 ] have all been published. This manuscript focuses on the cognitive events used by experienced and inexperienced clinical researchers during hypothesis generation.
Study flow and data sets used
The 2 × 2 study compared the hypothesis generation process of the clinical researchers with and without VIADS on the same datasets ( Appendix A ), with the same study scripts ( Appendix B ), and within the same timeframe (2 hours/study session), and they all followed the think-aloud method. The participants were separated into experienced and inexperienced clinical researchers based on predetermined criteria[ 31 ], e.g., years of experience and number of publications as significant contributors. The data were extracted from the National Ambulatory Medical Care Survey (NAMCS) conducted by the Centers for Disease Control and Prevention in 2005 and 2015 [ 36 ]. We preprocessed the NAMCS data sets by calculating and aggregating the ICD-9-CM diagnostic and procedural codes and their frequencies. The participants were asked to analyze the data and generate hypotheses and articulate their mind and actions during the process, i.e., study sessions. The screen activities and conversations between participants and the study facilitator were recorded via BBFlashback. The recordings were transcribed by a professional service.
Cognitive events coding for the hypothesis generation recordings
Based on the experience of conducting all study sessions, initial data analysis, the feedback from the investigation team, and literature review [ 1 , 13 , 37 – 41 ], a preliminary conceptual framework of the cognitive hypothesis generation process was developed before coding ( Figure 1 ). The conceptual framework served as a foundational framework to formulate the initial codes and code groups ( Appendix C ) that were used to code the transcriptions of the recordings, mainly for cognitive events (e.g., seek connections, analogy) in the hypothesis generation process. For example, “Analogy” was used when a participant compared one’s last study with the analysis results in front of him/her. “Use PICOT” was used when a participant used PICOT (i.e., patient, intervention, comparison, outcome, type of study) to formulate an idea into a formal hypothesis.

Initial version of the framework on cognitive events during hypothesis generation
The transcription of one study session was utilized as a pilot coding case to set the initial coding principles ( Appendix D ). The pilot coding sessions were used as training sessions for the two coders as well. The rest of the transcriptions were coded by the two coders independently and separately first. The two coders compared their coding results, discussed any discrepancies, and reached a consensus on coding later by including the study facilitator and modifying the coding principles. More codes and code groups were added while the coding progressed. After coding all the study session transcripts, the two coders also organized each hypothesis generation as an independent process and labeled the cognitive events during each hypothesis generation. We investigated the possible hypothesis generation processes based on coded cognitive events.
Data analytics strategy
This study used the cognitive events and the aggregated frequencies of these events to demonstrate the possible hypothesis generation process. While analyzing the cognitive events, we considered the results from four levels: (1) each hypothesis generation as a unit and we examined all hypotheses (n = 199), (2) each participant as a unit and all participants (n = 16) as a unit, (3) the group of participants who used VIADS as a unit (n = 9), and (4) the group of participants who did not use VIADS as a unit (n = 7). Correspondingly, the results were also organized at these four levels. We performed independent t-tests to compare the cognitive events between participants (a) in the VIADS and control groups and (b) between the experienced (3 participants, 36 hypotheses) and inexperienced clinical researchers (13 participants, 163 hypotheses). The study sessions of two participants’ (in the control group, both were inexperienced clinical researchers) were missing from the coding data because of technical failures resulting in partial recording of their study sessions, and their data were excluded from the analysis.
All hypotheses were rated by an expert panel of seven members using the same metrics for quality evaluation [ 23 , 42 ]. We deemed a hypothesis as invalid if three or more experts rated it as 1 (the lowest rating) on validity (significance and feasibility are two additional dimensions used for evaluation) of the hypothesis. However, we included the analysis of the result for all the hypotheses and valid hypotheses.
Ethics statement
The study was approved by the Institutional Review Board of Clemson University, South Carolina (IRB2020–056) and Ohio University Institutional Review Boards (18-X-192).
Hypothesis generation framework
Figure 2 is a refined and evolving version of the initial framework shown in Figure 1 , our preliminary understanding of hypothesis generation. Figure 2 was instrumental in directly guiding the coding of the cognitive events. The predominant cognitive events within the processing evidence category include “Using analysis results” (30%), “Seeking connections” (23%), and “Analyze data” (20.81%, Figure 2 ). Appendix E illustrates the processes and events used percentages while generating hypotheses. Appendix F presents individual cognitive events used for all hypotheses and valid hypotheses, respectively.

Cognitive process frameworks for scientific hypothesis generation in clinical research; the highest percentages of cognitive events used by clinical researchers were highlighted.
Overall cognitive events usage during hypothesis generation
Sixteen participants generated 199 hypotheses during the 2-hour study sessions, with 163 originating from the inexperienced groups ( Table 1 ). We used 20 distinct codes, i.e., cognitive events and 6 code groups ( Figure 2 ). Appendix C showcases the comprehensive codebook. Appendix D delineates the rationale and principles established during the coding phase. In total, 1216 times of cognitive events were applied across the 199 hypotheses. On average, inexperienced clinical researchers in the control group applied 7.38 cognitive events per hypothesis. Conversely, inexperienced clinical researchers in the VIADS group used 4.48 (p< 0.001 versus control) cognitive events per hypothesis with the lowest standard deviation (SD, 2.43). Experienced clinical researchers employed 6.15 (p < 0.01 versus junior VIADS) cognitive events per hypothesis. Notably, the inexperienced clinical researchers in the control group demonstrated the highest average number of cognitive event usage with the largest SD (5.02), whether we considered all hypotheses or just valid ones ( Table 1 ). The experienced participants have approximately 10% higher valid hypotheses (72.22% vs. 63.19%) than junior participants.
Group-wise comparison of cognitive events used while generating hypotheses
Note: SD, standard deviation;
Cognitive events comparison between VIADS and non-VIADS participants
Furthermore, we compared the percentages of cognitive event count between the VIADS and non-VIADS groups among inexperienced clinical researchers ( Figure 3 ). “Use analysis results” (31.3% vs.27.1%, p < 0.001), “Seek connections” (25.4% vs. 17.8%, p < 0.001), and “Analyze data” (22.1% vs. 21.1%) were the events with the highest percentages. The “Seek connections”, “Use analysis results”, and “Pause/think” (3.8% vs. 9.3%, p < 0.05) all show statistical differences between the VIADS and control groups by t tests. Our results indicate that the participants in the VIADS group registered higher event counts during “Preparation”, when “Analyzing results”, and when “Seeking connections”. Conversely, the control group exhibited greater event counts in categories such as “Needing further study”, “Inferring”, “Pausing”, “Using checklists”, and “Using PICOT”.

Comparing cognitive events generated by VIADS and control groups among inexperienced clinical researchers while generating hypotheses
Cognitive events comparison between experienced and inexperienced clinical researchers
We also examined the differences between experienced and inexperienced clinical researchers regarding the percentages of cognitive events they used ( Figure 4 ). “Use analysis results” (31.7% vs. 29.4%, p < 0.01), “Seek connections” (27.6% vs. 21.9%, p < 0.01), and “Analyze data” (17.5% vs. 21.6%, p< 0.01)) were events with the highest percentages of use. The data suggest that experienced clinical researchers exhibit higher percentages regarding these cognitive events: “Using analysis results”, “Seeking connections”, “Inferring”, and “Pausing”. Conversely, inexperienced clinical researchers demonstrated elevated percentages in cognitive events such as “Preparation”, “Data analysis”, “Utilizing suggestions”, “Utilizing checklists”, and “Utilizing PICOT”.

Comparison of cognitive events between experienced and inexperienced clinical researchers while generating hypotheses
Summary of results
The inexperienced clinical researchers in the VIADS group used the fewest cognitive events to generate each hypothesis on average versus the control group (p < 0.001) and the experienced clinical researchers (p < 0.01, Table 2). The most frequently used cognitive events were “Use analysis results” (29.85%), “Seek connections” (23.03%), and “Analyze data” (20.81%) during hypothesis generation ( Figure 2 ). It seems the inexperienced clinical researchers in the VIADS group demonstrated a similar trend to experienced clinical researchers ( Figures 3 and and4 4 ).
Results interpretation
Several findings of this study were notable. The experienced clinical researchers had a 10% higher percentage of valid hypotheses than the inexperienced clinical researchers (72.22% vs. 63.19%; Table 1 ), consistent with proposition and experience. Another interesting phenomenon is regarding the average cognitive events used by the different groups: the junior VIADS group used far fewer events per hypothesis than the control or experienced groups (4.38 vs. 7.38 vs. 6.15, Table 1 ) and exhibited the lowest SD. This is highly significant as it indicates that the VIADS group, despite comprising inexperienced clinical researchers, used fewer cognitive events to generate each hypothesis on average. This result supports our hypothesis that VIADS facilitates hypothesis generation. In addition, this result supports our findings that the VIADS group used a shorter time to generate each hypothesis on average [ 23 ].
Our results show clinical researchers spent ≥ 70% of cognitive events to process evidence during hypothesis generation. The top three cognitive events used by clinical researchers during hypothesis generation included “Using analysis results” (29.85%), “Seeking connections” (23.03%), and “Analyzing data” (20.81%, Figure 2 ).
Figure 3 presents the cognitive events and their distributions between the VIADS and control groups comprising the inexperienced clinical researchers. The participants in the VIADS group showed a higher number of cognitive events for interpreting the results, and the participants in the control group showed a higher number of cognitive events for external help, such as checklists and PICOT, during hypothesis generation. Figures 3 and and4 4 show that the VIADS group exhibits similar cognitive event trends with those of the experienced group in terms of “Using analysis results” and “Seeking connections”:
- VIADS versus control: 31.35% versus 27.11% (p< 0.001);
- experienced versus inexperienced: 31.71% versus 29.38% (p < 0.01)
- VIADS versus control: 25.38% versus 17.78% (p< 0.001);
- experienced versus inexperienced: 27.64% versus 21.86% (p< 0.01).
The results indicate that VIADS may help inexperienced clinical researchers move in a direction that aligns more with that of experienced clinical researchers. A more carefully designed study is needed to support or deny such a statement. However, it appears that the current quantitative evidence of cognitive events and their distributions among all cognitive events support such a trend.
Significance of the work
We consider this study to have the following significance: 1) developed the cognitive framework for hypothesis generation in the clinical research context and provided quantitative evidence through cognitive events for the framework; 2) identified and elaborated evidence-based cognitive mechanisms that might be underneath hypothesis generation; 3) identified that experienced clinical researchers possess a considerably higher valid rate of hypothesis generated in a 2-hour window than the inexperienced clinical researchers; 4) demonstrated that VIADS may help inexperienced clinical researchers to use fewer cognitive events than participants without using in hypothesis generation, which indicates VIADS provides a structured way of thinking during hypothesis generation; and 5) established the baseline measures of cognitive events in hypothesis generation and the following events were used in descending order: processing evidence, seeking evidence, and preparation.
Comparing to other studies
Patel et al. have explored medical reasoning through diagnoses, which have significantly influenced the design of the current study [ 7 , 8 , 20 , 22 ]. From their studies, we know that there were differences in the reasoning processes and thinking steps between experienced and inexperienced clinicians in medical diagnosis [ 9 , 19 , 22 , 43 , 44 ]. Therefore, we separated the participants into experienced and inexperienced groups before assigning them randomly into VIADS or control groups. The findings of this study mostly align with those of Patel et al. despite our different settings, medical diagnosis versus scientific hypothesis generation in clinical research. The experienced participants used fewer cognitive events than inexperienced participants on average, although the VIADS group used the lowest number of cognitive events despite comprising inexperienced clinical researchers.
Klahr and Dunbar’s landmark study published in 1988 [ 6 ] also enlightened our study [ 6 ]. Their study taught participants to use an electronic device. The participants had to figure out an unencountered function of the device. The process was employed to study hypothesis generation, reasoning, and testing iteratively. They concluded that searching memory and using results from prior experiments are critical for hypothesis generation. The primary differences between our studies lay in two folds: (1) the tasks for the participants (2) and the types of hypotheses generated. In the Klahr and Dunbar’s study, hypotheses had correct answers, i.e., problem-solving with one or multiple correct answers. Most likely, the participants used convergent thinking [ 7 ]. Their study used a simulated lab environment to assess scientific thinking. Conversely, the hypothesis generation in our study is open discovery without correct answers. The participants in our study used more divergent thinking during the process [ 7 ]. The hypothesis generation process in our study was substantially messier, unpredictable, and challenging to consistently evaluate comparing to their well-defined problems.
Limitations and challenges
One of the main limitations is only three experienced clinical researchers participated in our study who generated 36 hypotheses. We compared the inexperienced and experienced groups regarding all the hypotheses and cognitive events used. However, we could not compare the cognitive events between the VIADS and control groups among the experienced clinical researchers. We made similar efforts to recruit inexperienced and experienced clinical researchers via comparable platforms; however, the recruitment results were considerably worse in the experienced group.
Another limitation of the study was that the information could be captured via the think-aloud protocol. We acknowledge that we only captured the verbalized events during the study sessions, which is a subset of the conscious process and a small portion of the real process. Our coding, aggregation, and analysis are based on the captured events.
In addition, we also faced challenges in terms of unexpected technical failure and unpredictability because this was a human-participation study. The audio recordings of two participants were partial because of a technical failure. One mitigation strategy that could be used was to conduct a test recording each time for every participant, which can be particularly critical if a new device is used in the middle of the study.
Future work
Several avenues for future research emerge from our study. First, we aim to explore the sequence pattern of cognitive events to furnish additional insights into hypothesis generation. Furthermore, juxtaposing the frequencies of cognitive events with the quality evaluation results of the generated hypotheses might illuminate the potential patterns, further enriching our understanding of the process. Finally, a larger scale study encompassing a larger participant sample size and situated in a more natural environment can enhance the robustness of our findings.
Experienced clinical researchers exhibit a higher valid hypothesis rate than inexperienced clinical researchers. The VIADS group of inexperienced clinical researchers used the fewest cognitive events with the lowest standard deviation to generate each hypothesis compared with experienced and inexperienced clinical researchers not using VIADS. This efficiency is further underscored by the VIADS group taking the least average time to generate a hypothesis. Notably, the VIADS inexperienced cohort mirrored the trend observed in experienced clinical researchers in terms of cognitive event distribution. Such findings indicate that VIADS may provide structured guidance during hypothesis generation. Further studies, ideally on a grander scale and in a more natural environment, could offer a deeper understanding of the process. Our research provides foundational metrics on cognitive event measures during hypothesis generation in clinical research, demonstrating the viability of executing such experiments in a simulated setting and unraveling the intricacies of the hypothesis generation process through these experiments.
What is already known on this topic:
how hypotheses were generated when solving a puzzle or a medical case and the reasoning differences between experienced and inexperienced physicians.
What this study adds:
Our study facilitates our understanding of how clinical researchers generate hypotheses with secondary data analytical tools and datasets, the cognitive events used during hypothesis generation in an open discovery context.
How this study might affect research, practice, or policy:
Our work suggests secondary data analytical tools and visualization may facilitate hypothesis generation among inexperienced clinical researchers regarding the number of hypotheses, average time, and the cognitive events needed per hypothesis.
Supplementary Material
Supplement 1, supplement 2, supplement 3, supplement 4, supplement 5, supplement 6, acknowledgments.
We want to thank all participants sincerely for their precious time, courage, and expertise in helping us understand this critical but less-known hypothesis generation process better. This project received support from the National Library of Medicine (R15LM012941) and was funded partially by the National Institute of General Medical Sciences of the National Institutes of Health (P20 GM121342). The intellectual environment and research training resources provided by the NIH/NLM T15 SC BIDS4Health (T15LM013977) enriched this work.
Appendices:
Appendix A : Datasets used by participants during study sessions
Appendix B : Study session scripts followed by all participants
Appendix C : Codes and code group used during study session transcription analysis
Appendix D : Rationale and guidelines for coding data-driven hypothesis generation recordings
Appendix E : Cognitive events and their percentages during hypothesis generation in clinical research
Appendix F : Cognitive events used while generating data-driven hypotheses

Generative A.I. Arrives in the Gene Editing World of CRISPR
Much as ChatGPT generates poetry, a new A.I. system devises blueprints for microscopic mechanisms that can edit your DNA.
The physical structure of OpenCRISPR-1, a gene editor created by A.I. technology from Profluent. Credit... Video by Profluent Bio
Supported by
- Share full article

By Cade Metz
Has reported on the intersection of A.I. and health care for a decade.
- April 22, 2024
Generative A.I. technologies can write poetry and computer programs or create images of teddy bears and videos of cartoon characters that look like something from a Hollywood movie.
Now, new A.I. technology is generating blueprints for microscopic biological mechanisms that can edit your DNA, pointing to a future when scientists can battle illness and diseases with even greater precision and speed than they can today.
Described in a research paper published on Monday by a Berkeley, Calif., startup called Profluent, the technology is based on the same methods that drive ChatGPT, the online chatbot that launched the A.I. boom after its release in 2022 . The company is expected to present the paper next month at the annual meeting of the American Society of Gene and Cell Therapy.
Much as ChatGPT learns to generate language by analyzing Wikipedia articles, books and chat logs, Profluent’s technology creates new gene editors after analyzing enormous amounts of biological data, including microscopic mechanisms that scientists already use to edit human DNA.
These gene editors are based on Nobel Prize-winning methods involving biological mechanisms called CRISPR. Technology based on CRISPR is already changing how scientists study and fight illness and disease , providing a way of altering genes that cause hereditary conditions, such as sickle cell anemia and blindness.

Previously, CRISPR methods used mechanisms found in nature — biological material gleaned from bacteria that allows these microscopic organisms to fight off germs.
“They have never existed on Earth,” said James Fraser, a professor and chair of the department of bioengineering and therapeutic sciences at the University of California, San Francisco, who has read Profluent’s research paper. “The system has learned from nature to create them, but they are new.”
The hope is that the technology will eventually produce gene editors that are more nimble and more powerful than those that have been honed over billions of years of evolution.
On Monday, Profluent also said that it had used one of these A.I.-generated gene editors to edit human DNA and that it was “open sourcing” this editor, called OpenCRISPR-1. That means it is allowing individuals, academic labs and companies to experiment with the technology for free.
A.I. researchers often open source the underlying software that drives their A.I. systems , because it allows others to build on their work and accelerate the development of new technologies. But it is less common for biological labs and pharmaceutical companies to open source inventions like OpenCRISPR-1.
Though Profluent is open sourcing the gene editors generated by its A.I. technology, it is not open sourcing the A.I. technology itself.

The project is part of a wider effort to build A.I. technologies that can improve medical care. Scientists at the University of Washington, for instance, are using the methods behind chatbots like OpenAI’s ChatGPT and image generators like Midjourney to create entirely new proteins — the microscopic molecules that drive all human life — as they work to accelerate the development of new vaccines and medicines.
(The New York Times has sued OpenAI and its partner, Microsoft, on claims of copyright infringement involving artificial intelligence systems that generate text.)
Generative A.I. technologies are driven by what scientists call a neural network , a mathematical system that learns skills by analyzing vast amounts of data. The image creator Midjourney, for example, is underpinned by a neural network that has analyzed millions of digital images and the captions that describe each of those images. The system learned to recognize the links between the images and the words. So when you ask it for an image of a rhinoceros leaping off the Golden Gate Bridge, it knows what to do.
Profluent’s technology is driven by a similar A.I. model that learns from sequences of amino acids and nucleic acids — the chemical compounds that define the microscopic biological mechanisms that scientists use to edit genes. Essentially, it analyzes the behavior of CRISPR gene editors pulled from nature and learns how to generate entirely new gene editors.
“These A.I. models learn from sequences — whether those are sequences of characters or words or computer code or amino acids,” said Profluent’s chief executive, Ali Madani, a researcher who previously worked in the A.I. lab at the software giant Salesforce.
Profluent has not yet put these synthetic gene editors through clinical trials, so it is not clear if they can match or exceed the performance of CRISPR. But this proof of concept shows that A.I. models can produce something capable of editing the human genome.
Still, it is unlikely to affect health care in the short term. Fyodor Urnov, a gene editing pioneer and scientific director at the Innovative Genomics Institute at the University of California, Berkeley, said scientists had no shortage of naturally occurring gene editors that they could use to fight illness and disease. The bottleneck, he said, is the cost of pushing these editors through preclinical studies, such as safety, manufacturing and regulatory reviews, before they can be used on patients.
But generative A.I. systems often hold enormous potential because they tend to improve quickly as they learn from increasingly large amounts of data. If technology like Profluent’s continues to improve, it could eventually allow scientists to edit genes in far more precise ways. The hope, Dr. Urnov said, is that this could, in the long term, lead to a world where medicines and treatments are quickly tailored to individual people even faster than we can do today.
“I dream of a world where we have CRISPR on demand within weeks,” he said.
Scientists have long cautioned against using CRISPR for human enhancement because it is a relatively new technology that could potentially have undesired side effects, such as triggering cancer, and have warned against unethical uses, such as genetically modifying human embryos.
This is also a concern with synthetic gene editors. But scientists already have access to everything they need to edit embryos.
“A bad actor, someone who is unethical, is not worried about whether they use an A.I.-created editor or not,” Dr. Fraser said. “They are just going to go ahead and use what’s available.”
Cade Metz writes about artificial intelligence, driverless cars, robotics, virtual reality and other emerging areas of technology. More about Cade Metz
Explore Our Coverage of Artificial Intelligence
News and Analysis
Meta projected that revenue for the current quarter would be lower than what Wall Street anticipated and said it would spend billions of dollars more on its artificial intelligence efforts, even as it reported robust revenue and profits for the first three months of the year.
Microsoft introduced three smaller A.I. models that are part of a technology family the company has named Phi-3. The company said even the smallest of the three performed almost as well as GPT-3.5, the system that underpinned OpenAI’s ChatGPT chatbot.
A new flood of child sexual abuse material created by A.I. is threatening to overwhelm the authorities already held back by antiquated technology and laws. As a result, legislators are working on bills to combat A.I.-generated sexually explicit images of minors.
The Age of A.I.
A new category of apps promises to relieve parents of drudgery, with an assist from A.I . But a family’s grunt work is more human, and valuable, than it seems.
Despite Mark Zuckerberg’s hope for Meta’s A.I. assistant to be the smartest , it struggles with facts, numbers and web search.
Much as ChatGPT generates poetry, a new A.I. system devises blueprints for microscopic mechanisms that can edit your DNA.
Could A.I. change India’s elections? Avatars are addressing voters by name, in whichever of India’s many languages they speak. Experts see potential for misuse in a country already rife with disinformation.
Which A.I. system writes the best computer code or generates the most realistic image? Right now, there’s no easy way to answer those questions, our technology columnist writes .
Advertisement
We've detected unusual activity from your computer network
To continue, please click the box below to let us know you're not a robot.
Why did this happen?
Please make sure your browser supports JavaScript and cookies and that you are not blocking them from loading. For more information you can review our Terms of Service and Cookie Policy .
For inquiries related to this message please contact our support team and provide the reference ID below.
Help | Advanced Search
Computer Science > Computer Vision and Pattern Recognition
Title: id-animator: zero-shot identity-preserving human video generation.
Abstract: Generating high fidelity human video with specified identities has attracted significant attention in the content generation community. However, existing techniques struggle to strike a balance between training efficiency and identity preservation, either requiring tedious case-by-case finetuning or usually missing the identity details in video generation process. In this study, we present ID-Animator, a zero-shot human-video generation approach that can perform personalized video generation given single reference facial image without further training. ID-Animator inherits existing diffusion-based video generation backbones with a face adapter to encode the ID-relevant embeddings from learnable facial latent queries. To facilitate the extraction of identity information in video generation, we introduce an ID-oriented dataset construction pipeline, which incorporates decoupled human attribute and action captioning technique from a constructed facial image pool. Based on this pipeline, a random face reference training method is further devised to precisely capture the ID-relevant embeddings from reference images, thus improving the fidelity and generalization capacity of our model for ID-specific video generation. Extensive experiments demonstrate the superiority of ID-Animator to generate personalized human videos over previous models. Moreover, our method is highly compatible with popular pre-trained T2V models like animatediff and various community backbone models, showing high extendability in real-world applications for video generation where identity preservation is highly desired. Our codes and checkpoints will be released at this https URL .
Submission history
Access paper:.
- HTML (experimental)
- Other Formats

References & Citations
- Google Scholar
- Semantic Scholar
BibTeX formatted citation

Bibliographic and Citation Tools
Code, data and media associated with this article, recommenders and search tools.
- Institution
arXivLabs: experimental projects with community collaborators
arXivLabs is a framework that allows collaborators to develop and share new arXiv features directly on our website.
Both individuals and organizations that work with arXivLabs have embraced and accepted our values of openness, community, excellence, and user data privacy. arXiv is committed to these values and only works with partners that adhere to them.
Have an idea for a project that will add value for arXiv's community? Learn more about arXivLabs .

Morehead State Unveils New Transformational Buildings & Campus Revitalization Projects, Paving the Way for the Next Generation
- 25 April 2024
Morehead State University has come a long way since its founding as a single building in 1887. Over more than 135 years, the institution has remained a “light to the mountains” by growing and evolving to meet its students' academic, social, and residential needs.
That spirit of change, progress, and improvement for future generations of Eagles fuels MSU's current efforts to revitalize its campus.
Renovation projects will improve the campus community's experience over the next several years through strategic budgeting, state funding, and generous private gifts.
“We are actively trying to make a generation leap with our facilities that students can enjoy for years to come ,” said Dr. Jay Morgan, president of Morehead State University."
Construction of a New Science & Engineering Building
Located behind the Howell-McDowell Administration Building, the new $98 million science and engineering building will prepare students for the 21st-century job market and contribute to the region's economic transformation.
This 123,000-square-foot facility will house academic programs, including biology, chemistry, physics, geoscience, computer science, engineering, and manufacturing. Design is underway and expected to be completed this fall. Construction is anticipated to start in Fall 2024 and be completed by Spring 2027. It will replace Lappin Hall and the Lloyd-Cassity Building.
Built in 1937, expanded in 1967 and 1993, and renovated in 1996, Lappin Hall is a four-story classroom, laboratory, and office structure named in honor of Dr. Warren C. Lappin, former academic vice president and faculty member and two-time acting president. MSU built the Lloyd-Cassity Building in 1962. MSU named the three-story classroom and office structure in honor of Lloyd Cassity of Ashland, former chair of the Board of Regents. It is currently the home to the Lane-Volgenau Center for STEM Education, MSUTeach and the 21st Century Center for Manufacturing Systems.
Construction of a New Multidisciplinary Classroom Building
Additionally, Morehead State will begin construction on a new multidisciplinary classroom building. This 120,000-square-foot facility will house the business administration and music programs. The $90 million in state funding becomes available in July 2024, and the programming and design process will soon follow. Construction is anticipated to start in Fall 2025 and conclude in Summer 2028. This facility will replace the Bert T. Combs Building and Baird Music Hall.
MSU constructed the Bert T. Combs Building in 1962 and partially renovated it in 2003. The University named the four-story classroom and office building in honor of former Gov. Bert T. Combs. Built in 1954 and expanded in 1967, Baird Music Hall is a three-story classroom and office building named in honor of Dr. William Jesse Baird, the University's fifth president. It includes Duncan Recital Hall and Fulbright Auditorium.
Residence Halls
MSU plans to construct a new $38 million, 80,000-square-foot residence hall to house 310 students across four floors. The design is currently underway and expected to be completed this summer. Construction should begin in Fall 2024 and be completed by Spring 2026.
MSU is also renovating Cooper Hall and Nunn Hall.
Cooper Hall is undergoing a complete renovation that has a project cost of $10.8 million. Upon completion, the 35,000-square-foot facility will have a capacity of 175 beds. Construction is approximately 50% complete and is expected to be finished in Spring 2025 and will be open to students in the Fall of 2025.
Cooper Hall is a four-story men’s residence hall built in 1965 and named in honor of former U.S. Senator John Sherman Cooper.
Currently under renovation, Nunn Hall, a 105,000-square-foot facility, will remain at its current capacity of 400 beds. The $3.2 million renovation includes:
- New paint
- New countertops and lighting
- Patch, paint, and re-finish the exterior walkways
- New elevator equipment
- New chiller & other mechanical equipment
MSU will complete the project in two phases. The first phase concludes this summer, and the second will be completed by Summer 2025.
The nine-story residence hall was built in 1969 and named in honor of Beula Nunn, wife of Governor Louie B. Nunn.
Razing Cartmell Hall will occur after the new residence hall is completed. The 16-story coed residence hall was built in 1969 and named in honor of Dr. William H. Cartmell of Maysville, the first private citizen to serve as chair of MSU’s Board of Regents.
Other Academic Building Renovations
Both Ginger Hall and the Camden-Carroll Library are undergoing renovations.
Ginger Hall is currently undergoing a $1.75 million renovation of the entire building, including new lighting, ceilings, flooring, and paint on all floors. A new chiller has also been installed, and a full exterior building cleaning has been completed.
Ginger Hall, built in 1968, was named in honor of Dr. Lyman V. Ginger, former state school superintendent and former chair of the MSU Board of Regents.
The Camden-Carroll Library is currently undergoing a $1.15 million renovation. Completed projects include replacing both roof levels and the building’s chiller and installing a fire suppression system for the collections area.
The first floor will have new finishes, flooring, ceilings, lighting, and paint. Plans also include 16 new offices for academic advisors on the first floor. These improvements are scheduled for completion by Fall 2024 and are actively under construction. Second floor renovations will follow once the first floor is completed.
The Camden-Carroll Library was built in 1931 and expanded twice in 1965 and 1978. The five-story structure is named after former U.S. Senator Johnson Camden and former Governor Julian M. Carroll. It is listed on the National Register of Historic Places.
Improved Parking
MSU is razing Wetherby Gymnasium and the Laughlin Health Building to create an entrance to campus and increase parking access for students, faculty, staff, families, and guests. An additional 400 new parking spaces on campus will prioritize safety, allowing fewer students to have to park in the overflow lot and walk across U.S. 60 to get to MSU.
Wetherby and Laughlin have served the community for generations by providing health services and hosting athletic events. However, these aging facilities were difficult to maintain.
MSU built Wetherby Gym in 1956. From its construction until the construction of the Academic Athletic Center in 1981, it served as the primary facility for the University’s intercollegiate athletic programs and the athletic programs for the Breckinridge School on MSU’s campus. It was also the venue for several significant campus events, from commencement to concerts.
Built in 1967, the two-story Laughlin Health Building was a classroom, laboratory and office building named in honor of Robert (Bobby) G. Laughlin, former basketball coach, athletic director, and faculty member.
Plans are in the works to allow Eagles to keep a piece of these facilities through several memorabilia opportunities. For more information about memorabilia , contact the Office of Alumni Relations and Development at 606-783-2033 or [email protected] .
Craft Academy Expansion & Renovations to Alumni Tower
The Craft Academy, led by donations from the Craft family, will be expanding in the Fall 2024. Alumni Tower went through extensive ground floor and partial upper floor renovation to accommodate the academy moving into the tower for future growth. The Craft Family has provided MSU with millions of dollars in generous contributions and the academy continues to lead the way as a top school in the U.S. In the Fall 2023, Alumni Tower became the home of the Craft Academy and its 200 students.
Other Campus Improvements
Last year, MSU began renovation activities for its football facility with the addition of a new artificial surface. With the expansion of the men’s and women’s track program, a new track surface was also added. Jayne Stadium is also undergoing exterior painting and branding along with updating the stadium press boxes. Further enhancements to the football stadium are also planned.
Private donations are funding facility upgrades for the softball and baseball programs. These upgrades include a new scoreboard, fence screen, Big Blue Wall, new turf project, batting cages, field equipment, re-play systems, and new field tarps.
Meanwhile, the Academic-Athletic Center is undergoing a large branding project on the front of the facility.
Elsewhere across campus, renovation is underway on the first floor of the Educational Services Building on E. Main Street to create a new space for the MSU Police Department. The MSUPD will be relocated from Laughlin Health Building in May.
The Kentucky General Assembly also approved significant asset preservation funds for MSU. The University has already used these funds to replace roofs and HVAC units for various buildings, upgrade facilities, renovate space and internal repairs.
MSU President Jay Morgan said the combination of new facilities and new and improved residence halls will enhance the experience for faculty, staff, and Eagles past and present.
“We are actively working to transform our facilities, either by building new ones or renovating existing ones. So that MSU students can benefit for generations to come.”
For more information on MSU’s campus revitalization, visit www.moreheadstate.edu/forward .
Communications & Marketing 606-783-9328 Email Us
Social Media

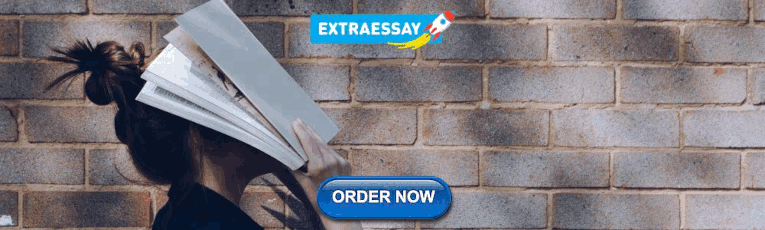
IMAGES
VIDEO
COMMENTS
The basic science hypothesis-testing paradigm. The classical paradigm for basic biological research has been to develop a specific hypothesis that can be tested by the application of a prospectively defined experiment (see Box 1).I suggest that one of the major (although not the only) factors that led to the development of this paradigm is that experimental design was limited by the throughput ...
1. An Overview. Broadly, preclinical research can be classified into two distinct categories depending on the aim and purpose of the experiment, namely, "hypothesis generating" (exploratory) and "hypothesis testing" (confirmatory) research (Fig. 1).Hypothesis generating studies are often scientifically-informed, curiosity and intuition-driven explorations which may generate testable ...
5. Phrase your hypothesis in three ways. To identify the variables, you can write a simple prediction in if…then form. The first part of the sentence states the independent variable and the second part states the dependent variable. If a first-year student starts attending more lectures, then their exam scores will improve.
Hypothesis generation is an early and critical step in any hypothesis-driven clinical research project. Because it is not yet a well-understood cognitive process, the need to improve the process goes unrecognized. Without an impactful hypothesis, the significance of any research project can be questionable, regardless of the rigor or diligence applied in other steps of the study, e.g., study ...
A hypothesis is an "educated guess/prediction" or "proposed explanation" of how a system will behave based on the available evidence. A hypothesis is a starting point for further investigation and testing because a hypothesis makes a prediction about the behavior of a measurable outcome of an experiment. A hypothesis should be:
Generate a hypothesis in advance through pre-analyzing a problem (i.e., generation of a prestage hypothesis ). 3. Collect data related to the prestage hypothesis by appropriate means such as experiment, observation, database search, and Web search (i.e., data collection). 4. Process and transform the collected data as needed. 5.
Scientific hypothesis generation and scientific hypothesis testing are distinct processes 2,5. In clinical research, research questions are often delineated without the support of systematic data analysis (i.e., not data-driven) 2,6,7. Using and analyzing existing data to facilitate scientific
Hypothesis Generation is a literature-based discovery approach that utilizes existing literature to automatically generate implicit biomedical associations and provide reasonable predictions for future research. Despite its potential, current hypothesis generation methods face challenges when applied to research on biological mechanisms. ...
Table of contents. Step 1: Define your variables. Step 2: Write your hypothesis. Step 3: Design your experimental treatments. Step 4: Assign your subjects to treatment groups. Step 5: Measure your dependent variable. Other interesting articles. Frequently asked questions about experiments.
The first is to raise a research question, answer that question by conducting a new study, and then offer one or more theories (usually more) to explain or interpret the results. This format works well for applied research questions and for research questions that existing theories do not address. The second way is to describe one or more ...
There are 5 main steps in hypothesis testing: State your research hypothesis as a null hypothesis and alternate hypothesis (H o) and (H a or H 1 ). Collect data in a way designed to test the hypothesis. Perform an appropriate statistical test. Decide whether to reject or fail to reject your null hypothesis. Present the findings in your results ...
In science, experimentation and hypothesis generation often form an iterative cycle: a researcher asks a question, collects data and adjusts the question or asks a fresh one. ... In one experiment ...
A hypothesis is a tentative statement about the relationship between two or more variables. It is a specific, testable prediction about what you expect to happen in a study. It is a preliminary answer to your question that helps guide the research process. Consider a study designed to examine the relationship between sleep deprivation and test ...
Why Is Hypothesis Generation Important? Digital technologies are creating new business opportunities, but as I've noted in earlier columns, companies must experiment to learn both what is possible and what customers want. Most companies are relying on empowered, agile teams to conduct these experiments.
Creating a research hypothesis to answer a research problem is an iterative process. (Image by rawpixel.com on Freepik) Any research begins with a research question and a research hypothesis.A research question alone may not suffice to design the experiment(s) needed to answer it. A hypothesis is central to the scientific method. But what is a hypothesis?
Our hypothesis maker is a simple and efficient tool you can access online for free. If you want to create a research hypothesis quickly, you should fill out the research details in the given fields on the hypothesis generator. Below are the fields you should complete to generate your hypothesis:
Hypothesis generation: qualitative research to identify potentials of behavioral change regarding energy consumption. The qualitative expressions are based on data that includes semi-structured interviews, observations and social experiments. The energy-saving benefits are considered without a reduction in performance.
What about hypothesis-generating projects. Because these projects take a non-biased approach, these projects are more likely to come up with ground-breaking new mechanisms or concepts. On the other hand, hypothesis-generating research usually involves a longer process. You have to first generate the hypothesis, which requires setting up of the ...
Step 1: Allocate the Variable Components. Product hypotheses are generally different for each case, so begin by pinpointing the major variables, i.e., the cause and effect. You'll need to outline what you think is supposed to happen if a change or action gets implemented.
Meta Horizon OS is the result of a decade of work at Meta to build a next-generation computing platform. To pioneer standalone headsets, we developed technologies like inside-out-tracking and self-tracked controllers. To allow for more natural interaction systems and social presence, we pioneered hand, eye, face and body tracking.
├── experiment_results.zip.002 # This file contains the results of the experiment. ├── few_shot # This directory contains the code for the few-shot prompting experiment. ├── gpt-3.5-turbo-1106-results # This directory contains the output of the experiment using the GPT-3.5-turbo-1106 model.
Generative Commonsense Reasoning (GCR) requires a model to reason about a situation using commonsense knowledge, while generating coherent sentences. Although the quality of the generated sentences is crucial, the diversity of the generation is equally important because it reflects the model's ability to use a range of commonsense knowledge facts. Large Language Models (LLMs) have shown ...
Introduction. A research hypothesis is an educated guess regarding relationships among different variables [1, 2].A research question typically comprises one to several scientific hypotheses that drive the direction of most research projects [1, 3 - 5].If we consider the life cycle of a research project, hypothesis generation constitutes its starting point.
Much as ChatGPT generates poetry, a new A.I. system devises blueprints for microscopic mechanisms that can edit your DNA. The physical structure of OpenCRISPR-1, a gene editor created by A.I ...
The European Union is discussing with member states proposals to sanction key Russian liquefied natural gas projects and a ban on using EU ports to re-export supplies destined for third countries ...
This paper addresses the task of 3D clothed human generation from textural descriptions. Previous works usually encode the human body and clothes as a holistic model and generate the whole model in a single-stage optimization, which makes them struggle for clothing editing and meanwhile lose fine-grained control over the whole generation process. To solve this, we propose a layer-wise clothed ...
Generating high fidelity human video with specified identities has attracted significant attention in the content generation community. However, existing techniques struggle to strike a balance between training efficiency and identity preservation, either requiring tedious case-by-case finetuning or usually missing the identity details in video generation process. In this study, we present ID ...
Renovation projects will improve the campus community's experience over the next several years through strategic budgeting, state funding, and generous private gifts. "We are actively trying to make a generation leap with our facilities that students can enjoy for years to come," said Dr. Jay Morgan, president of Morehead State University."