TEACHING MATERIALS
Blue ocean pedagogical materials, used in nearly 3,000 universities and in almost every country in the world, go beyond the standard case-based method. Our multimedia cases and interactive exercises are designed to help you build a deeper understanding of key blue ocean concepts, from blue ocean strategy to nondisruptive creation, developed by world-renowned professors Chan Kim and Renée Mauborgne . Currently, with over 20 Harvard bestselling cases .
CASES BY TOPIC
TEACHING GUIDE
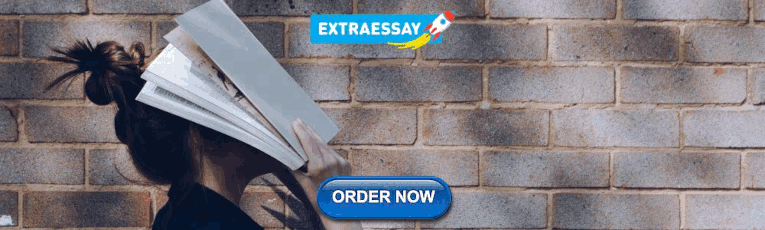
DRIVING THE FUTURE
Driving the future: how autonomous vehicles will change industries and strategy.
Author(s): KIM, W. Chan, MAUBORGNE, Renée, CHEN, Guoli, OLENICK, Michael
Case study trailer
Self-driving cars are moving from science fiction to showroom fact, or at least to a car summoning platform. Waymo, the self-driving car division of Google, has ordered 82,000 self-driving cars for delivery through 2020. Cruise Automation, from General Motors, is perfecting its own fleet. Countless companies are driving full-throttle into the future. This case explores whether self-driving cars (autonomous vehicles or AVs) are a red ocean or blue ocean opportunity, and explains the difference between technological innovation and value innovation . It will prompt students to think about disruptive innovation and nondisruptive market creation , and why inventors of major technological innovations throughout history have often failed to meaningfully monetize their inventions.
HBSP | Case Centre | INSEAD
Teaching Note
Case Centre | INSEAD
Autonomous driving’s future: Convenient and connected
The dream of seeing fleets of driverless cars efficiently delivering people to their destinations has captured consumers’ imaginations and fueled billions of dollars in investment in recent years. But even after some setbacks that have pushed out timelines for autonomous-vehicle (AV) launches and delayed customer adoption, the mobility community still broadly agrees that autonomous driving (AD) has the potential to transform transportation , consumer behavior, and society at large.
About the authors
Because of this, AD could create massive value for the auto industry, generating hundreds of billions of dollars before the end of this decade, McKinsey research shows. 1 McKinsey Center for Future Mobility analysis. To realize the consumer and commercial benefits of autonomous driving, however, auto OEMs and suppliers may need to develop new sales and business strategies, acquire new technological capabilities, and address concerns about safety.
This report, which focuses on the private-passenger-car segment of the AD market, examines the potential for autonomous technologies to disrupt the passenger car market. It also outlines critical success factors that every auto OEM, supplier, and tech provider should know in order to win in the AD passenger car market. (Other McKinsey publications explore the potential of shared AVs such as robo-taxis and robo-shuttles , as well as autonomous trucks and autonomous last-mile delivery.)
Autonomous driving could produce substantial value for drivers, the auto industry, and society
AD could revolutionize the way consumers experience mobility. AD systems may make driving safer, more convenient, and more enjoyable. Hours on the road previously spent driving could be used to video call a friend, watch a funny movie, or even work. For employees with long commutes, driving an AV might increase worker productivity and even shorten the workday. Since workers can perform their jobs from an autonomous car, they could more easily move farther away from the office, which, in turn, could attract more people to rural areas and suburbs. AD might also improve mobility for elderly drivers, providing them with mobility options that go beyond public transportation or car-sharing services. Safety might also increase, with one study showing that the growing adoption of advanced driver-assistance systems (ADAS) in Europe could reduce the number of accidents by about 15 percent by 2030. 2 Tom Seymour, “Crash repair market to reduce by 17% by 2030 due to advanced driver systems, says ICDP,” Automotive Management Online , April 7, 2018.
Along with these consumer benefits, AD may also generate additional value for the auto industry. Today, most cars only include basic ADAS features, but major advancements in AD capabilities are on the horizon. Vehicles will ultimately achieve Society of Automotive Engineers (SAE) Level 4 (L4), or driverless control under certain conditions. Consumers want access to AD features and are willing to pay for them, according to a 2021 McKinsey consumer survey. Growing demand for AD systems could create billions of dollars in revenue. Vehicles with lidar-based Level 2+ (L2+) capabilities contain approximately $1,500 to $2,000 in component costs, and even more for cars with Level 3 (L3) and L4 options. Based on consumer interest in AD features and commercial solutions available on the market today, ADAS and AD could generate between $300 billion and $400 billion in the passenger car market by 2035, according to McKinsey analysis 3 McKinsey Center for Future Mobility analysis. Total revenue potential is derived from McKinsey’s base case on ADAS and AD installation rates, with the share of OEM subscription offerings at a 100 percent installation rate, and actual customer take rate and pricing assumptions by segment and ADAS/AD features. Prices are assumed to decline over time as features become industry standards and because of overall cost digression. (Exhibit 1).
The knock-on effects of autonomous cars on other industries could be significant. For example, by reducing the number of car accidents and collisions, AD technology could limit the number of consumers requiring roadside assistance and repairs. That may put pressure on those types of businesses as consumer adoption of AD rises. In addition, consumers with self-driving cars might not be required to pay steep insurance premiums, since handing over control of vehicles to AD systems might mean that individual drivers could no longer be held liable for car accidents. As a consequence, new business-to-business insurance models may arise for autonomous travel.
Several automakers are already piloting new insurance products. These companies are gleaning insights on driving behavior from autonomous technology and making personalized offers to their consumers. Since OEMs control the AD system, its performance, and the data that it generates (such as the real-time performance of drivers), auto companies can precisely tailor insurance policies to their consumers, giving them a significant advantage over external insurance providers.
How AD could transform the passenger car market
Given today’s high levels of uncertainty in the auto industry, McKinsey has developed three scenarios for autonomous-passenger car sales based upon varying levels of technology availability, customer adoption, and regulatory support (Exhibit 2). In our delayed scenario, automakers further push out AV launch timelines, and consumer adoption remains low. This scenario projects that in 2030, only 4 percent of new passenger cars sold are installed with L3+ AD functions, with that figure increasing to 17 percent in 2035.
Our base scenario assumes that OEMs can meet their announced timelines for AV launches, with a medium level of customer adoption despite the high costs of AD systems. By 2030, 12 percent of new passenger cars are sold with L3+ autonomous technologies, and 37 percent have advanced AD technologies in 2035.
Would you like to learn more about the McKinsey Center for Future Mobility ?
Finally, in our accelerated scenario, OEMs debut new AVs quickly, with sizable revenues coming in through new business models (for example, pay as you go, which offers AD on demand, or new subscription services). In this scenario, most premium automakers preinstall hardware that makes fully autonomous driving possible when the software is ready to upgrade. In this scenario, 20 percent of passenger cars sold in 2030 include advanced AD technologies, and 57 percent have them by 2035.
Delivering higher levels of automation
For automakers focused on delivering vehicles with higher levels of automation, there is enormous potential for growth. Consumers interested in the convenience of hands-free driving might want cars with more advanced autonomous functions (including L2+, L3, and L4), which give the autonomous system greater control over driving tasks. Costs for sensors and high-performance computers are decreasing, while safety standards for AD technologies are continuing to advance. (For instance, standards currently available for traffic jam pilots, which allow autonomous vehicles to navigate through stop-and-go traffic while maintaining a safe distance from other cars, could soon extend to other advanced AD functions.) Taken together, these factors could help the auto industry introduce more advanced autonomous features to a broad range of vehicles over time.
Based on McKinsey’s sales scenarios, L3 and L4 systems for driving on highways will likely be more commonly available in the private-passenger-car segment by around 2025 in Europe and North America, even though the first applications are just now coming into market. (One luxury European brand offers an L3 conditional self-driving system but restricts usage to certain well-marked highways and at reduced speeds.)
About the McKinsey Center for Future Mobility
These insights were developed by the McKinsey Center for Future Mobility (MCFM). Since 2011, the MCFM has worked with stakeholders across the mobility ecosystem by providing independent and integrated evidence about possible future-mobility scenarios. With our unique, bottom-up modeling approach, our insights enable an end-to-end analytics journey through the future of mobility—from consumer needs to modal mix across urban and rural areas, sales, value pools, and life cycle sustainability. Contact us , if you are interested in getting full access to our market insights via the McKinsey Mobility Insights Portal.
Steep up-front costs for developing L3 and L4 driving systems suggest that auto companies’ efforts to commercialize more advanced AD systems may first be limited to premium-vehicle segments. Additional hardware- and software-licensing costs per vehicle for L3 and L4 systems could reach $5,000 or more during the early rollout phase, with development and validation costs likely exceeding more than $1 billion. Because the sticker price on these vehicles is likely to be high, there might be greater commercial potential in offering L2+ systems. These autonomous systems somewhat blur the lines between standard ADAS and automated driving, allowing drivers to take their hands off the wheel for certain periods in areas permitted by law.
L2+ systems are already available from several OEMs, with many other vehicle launches planned over the next few years. If equipped with sufficient sensor and computing power, the technology developed for L2+ systems could also contribute to the development of L3 systems. This is the approach taken by several Chinese disruptor OEMs. These companies are launching vehicles that offer L2+ systems pre-equipped with lidar sensors. The vehicles are likely to reach L3 functionality relatively soon, since the companies are likely using their on-road fleet of enhanced L2+ vehicles to collect data to learn how to handle rare edge cases, or to run the L3 system in shadow mode.
Where true L3 systems are not available, developers might also offer a combination of L2+ and L3 features. This may include an L2+ feature for automated driving on highways and in cities, together with an L3 feature for use in traffic jams.
Car buyers are highly interested in AD features
Consumers benefit from using AD systems in many ways, including greater levels of safety; ease of operation for parking, merging, and other maneuvers; additional fuel savings because of the autonomous system’s ability to maintain optimal speeds; and more quality time. Consumers understand these benefits and continue to be highly willing to consider using AD features, according to our research.
Consumers benefit from using AD systems in many ways, such as greater levels of safety, and some are highly willing to consider using AD features.
In McKinsey’s 2021 autonomous driving, connectivity, electrification, and shared mobility (ACES) 4 Timo Möller, Asutosh Padhi, Dickon Pinner, and Andreas Tschiesner, “ The future of mobility is at our doorstep ,” December 19, 2019. survey, which polled more than 25,000 consumers about their mobility preferences, about a quarter of respondents said they are very likely to choose an advanced AD feature when purchasing their next vehicle. Two-thirds of these highly interested customers would pay a one-time fee of $10,000 or an equivalent subscription rate for an L4 highway pilot, which provides hands-free driving on highways under certain conditions (Exhibit 3). This represents a price point and willingness to pay that is consistent with a few top-of-the-line AD vehicles launched in the past few years, as well as with our value-based pricing model.
Since consumers have such different lifestyles and needs, AD systems may benefit some consumers far more than others, making them much more likely to pay for AD features. For instance, a sales manager who drives 30,000 miles a year and upgrades to an autonomous car could use all of that time previously spent driving to contact new leads or to create in-depth sales strategies with his or her team. On the other hand, a parent who uses a car primarily for shopping or for driving the kids to school might be more reluctant to pay for AD features.
Exploring the values of different consumer personas could enable OEMs and dealerships to tailor their value propositions and pricing schemes. For instance, they might implement a flexible pricing model that includes a fixed one-time fee, subscription offerings, and, potentially, an on-demand option such as paying an hourly rate for each use of a traffic jam pilot. Our research indicates that consumers prefer having different pricing options. Of the highly interested consumers, 20 percent of ACES survey respondents said they would prefer to purchase ADAS features through a subscription, while nearly 30 percent said they would prefer to pay each time they use a feature. In addition, one in four respondents said they would like to be able to unlock additional ADAS features even after purchasing a new car.
Although consumers continue to be very interested in autonomous driving, they are also adopting more cautious and realistic attitudes toward self-driving cars. For the first time in five years, consumers are less willing to consider driving a fully autonomous vehicle, our ACES consumer surveys show. Readiness to switch to a private AV is down by almost ten percentage points, with 26 percent of respondents saying they would prefer to switch to a fully autonomous car in 2021, compared with 35 percent in 2020 (Exhibit 4).
Our ACES consumer research also reveals that trust in the safety of AVs is down by five percentage points, and that the share of consumers who support government regulation of fully self-driving cars has declined by about 15 percentage points. While safety concerns are top of mind, consumers also want opportunities to test-drive AD systems and more information about the technology. To help customers become more comfortable with AVs, OEMs may need to offer hands-on experiences with AVs, address safety concerns, and educate consumers about how autonomous driving works.
Regulatory support is critical
Support from regulators is essential to overcoming AD safety concerns, creating a trusted and safe ecosystem, and implementing global standards. So far, most public officials have strongly advocated for the inclusion of ADAS capabilities in existing regulations, laying the groundwork for autonomous driving. This has resulted in a much higher penetration of ADAS functions, both in passenger cars and commercial vehicles.
The auto industry and public authorities agree on autonomous driving’s potential to save lives . Today, basic SAE L1 and L2 ADAS features are increasingly coming under regulation. This includes Europe’s Vehicle General Safety Regulation, along with Europe and North America’s New Car Assessment Program (NCAP), a voluntary program that establishes standards for car safety. NCAP is a key advocate for the integration of active safety systems in passenger cars.
In 2020 and 2022, OEMs seeking the NCAP’s highest, five-star safety rating were faced with the challenges of implementing features such as automatic emergency braking (AEB) and automatic emergency steering (AES). As a result, US and European OEMs in all segments have developed these features, with more than 90 percent of all European- and American-made cars offering L1 capabilities as a baseline.
There is already sufficient regulation to enable companies to pilot robo-shuttle services in cities, primarily in the United States, China, Israel, and now in Europe. Companies will continue their test-and-learn cycles with the robo-shuttle pilots and move into a phase of stable operations over the next few years. Still missing, however, are global standards regarding AD functions for use in private vehicles, although many regulators are working on them.
The United Nations Economic Commission for Europe has a rule on automated lane-keeping systems that regulates the introduction of L3 AD for speeds up to 60 kilometers per hour. In addition, the UN’s World Forum for Harmonization of Vehicle Regulations (WP.29) is working on additional regulation for using AD functions at higher speeds. This group plans to extend the use of advanced autonomous systems to speeds of up to 130 kilometers per hour, with the rule coming into force in 2023. Germany has also offered comprehensive legislation on AD that has allowed one European OEM to launch the first true L3 feature in a current model. Similar legislation exists in Japan and has recently been authorized in France. The development of global AD standards for private-passenger vehicles is clearly in motion.
Succeeding in the passenger car market
To succeed in the autonomous passenger car market, OEMs and suppliers will likely need to change how they operate. This may require a new approach to R&D that focuses on software-driven development processes, a plan to make use of fleet data, and flexible, feature-rich offerings across vehicle segments that consider consumers’ varying price points. Decoupling the development of hardware components and software for AD platforms could allow automakers and suppliers to keep design costs more feasible, since the AV architecture could then be reused.
To win over consumers, auto companies could also develop a customer-centered, go-to-market strategy. Moreover, leaders might explore different ownership models and sales methods with the end-to-end (E2E) business case in mind, taking into account the entire life cycle of the autonomous vehicle. Finally, leaders may also need to create an organization that will support all of the above changes.
Creating a new R&D strategy
Succeeding in AD may require OEMs to make a mindset change. Simply put, the old ways of doing things are no longer valid. Successful OEMs should focus on building up in-house competencies such as excellence in software development. Although the automotive industry has honed its ability to split development work among multiple partners and suppliers, the sheer complexity of an L3- or L4-capable AD stack limits the potential for partnering with many different specialists.
Indeed, developing AD capabilities requires much stronger ownership of the entire ecosystem, as well as the ability to codevelop hardware and software—in particular, chips and neural networks. This suggests leading OEMs should either develop strong in-house capabilities or form partnerships with leading tech players tasked with delivering the entire driving platform.
OEMs would also benefit from holistically managing their road maps for developing AD features and portfolios of offerings. They should ensure that the AD architecture is flexible and reusable where possible. Moreover, to stay competitive over a vehicle platform’s life cycle, systems must be easy to upgrade. Developing new strategies to collect fleet data, selecting relevant testing scenarios, and using the data to train and validate the AD system are also likely to be essential.
Developing customer-centered, go-to-market strategies
OEMs and their dealer networks should work to dispel the many uncertainties faced by consumers when deciding to buy a car with AD capabilities. Although consumers remain highly interested in AD, most buyers have not yet driven an autonomous car. Consumers receive many different (and sometimes contradictory) messages throughout the car-buying journey, from sources that hype up the technology to those that tout significant safety concerns. To win the trust of consumers, OEMs and dealerships may need to deliver additional sales training so that employees can pitch AD systems to customers and explain the technologies in enough detail to alleviate customer concerns.
Enabling customers to experience AD firsthand is critical, so auto companies may want to offer a test-drive that introduces the AD platform. Changing the business model from offering one-time licensing to an ongoing subscription plan could make it easier for customers to afford an AV and provide additional upside for OEMs. Our research indicates that in the future, all three business models (one-time sales, subscription pricing, and pay per use) may generate significant revenue. This implies that OEMs and other companies might need to adapt their go-to-market approach in order to sell subscriptions. OEMs might consider offering subscriptions that go beyond AD features and potential vehicle ownership, such as in-car connectivity.
Making an end-to-end business case
With new forms of revenue coming in through subscriptions and pay-per-use offerings, OEMs may need to rethink how they calculate the business case for their vehicles and shift toward E2E marketing strategies. That means considering subscription pricing and length, sales of upgrades, software maintenance, and potential upselling to more advanced systems. For subscription pricing, OEMs are likely to face higher up-front costs, since they have to equip all vehicles with the technologies that will make AD features run. In return, they might expect higher customer use and revenues over the vehicle’s life cycle.
Based on McKinsey’s customer research and business case studies, AD subscription models may initially be economically viable only for premium D-segment vehicles (large cars such as sedans or wagons) and above, particularly those that already exhibit higher revenues from ADAS/AD functions. OEMs may need to adjust their internal key performance indicators (KPI), financing structures, and strategies for communicating with investors, since the E2E business model reduces short-term profitability in exchange for long-term revenue.
Reorganizing the company
Software is the key differentiator for AD, so organizations must excel in several areas: attracting coding talent, the development process, and capabilities in simulation and validation. It’s worth noting that leading AD players do not necessarily have the biggest development teams. In fact, the development teams deployed by leading disruptive players are often significantly smaller than those of some other large OEM groups and tier-one suppliers. This highlights the importance of having the right talent, combined with effective development processes and best-in-class tool chains.
Experience suggests that deploying more resources can backfire, creating additional fragmentation and making communication needlessly complex for companies managing development projects. This is why it is often not a winning formula to install managers experienced in hardware development or embedded-software development to lead AD systems’ software development teams.
Implications for suppliers
Suppliers may also need to adapt to new industry success factors. They face fierce competition for full-stack solutions that may likely lead to a consolidation of players. To compete, suppliers must be focused and nimble. They might benefit from offering different delivery models to OEM customers, from stand-alone hardware solutions to fully integrated hardware–software solutions. In return, new opportunities may open up for developing joint business models closer to end customers, potentially including the possibility of revenue sharing.
For state-of-the-art AD solutions, companies will need access to large amounts of fleet data to train algorithms to achieve low-enough failure rates. While OEMs have fleet access and only need to find suitable technology to extract data from their customer fleets, suppliers must depend on partners or key customers to gain access. Consequently, it is mission critical for suppliers seeking to develop state-of-the-art AD systems to recruit a close lead customer early on for codevelopment and fleet access.
A lack of access to substantial amounts of fleet data, funding, and sufficient talent will probably limit the number of companies that can successfully offer full-stack AD systems. The result may be a “winner takes most” market dynamic. Companies with the best access to data and funding will likely enjoy a strong competitive advantage over those that do not have this information, since they will have a better chance to advance their technology and get ahead of their competitors.
As a result, the number of successful suppliers or tech companies delivering a full AD system could likely remain limited to a handful of companies, in both the West and China. For the first generation of AD systems, joint development of software and the required chips may help the full system achieve better performance and efficiency, with a lower risk of late integration issues. This could further limit the number of potential industry winners.
Achieving long-term success may also require suppliers to articulate their competitive advantage and strategies. A targeted approach may yield higher returns.
Achieving long-term success may also require suppliers to articulate their competitive advantage, value proposition, and strategies. They should decide whether or not to become a full-stack player for the most advanced systems or concentrate on dedicated areas of the stack, which could be either hardware components or software elements. Our research shows that a targeted approach may yield higher returns for many suppliers and potentially offer substantial and attractive value pools. The total value of the passenger car AD components market could reach $55 billion to $80 billion by 2030, based on a detailed analysis that assumes a medium AD adoption scenario (Exhibit 5). In this scenario, most of the revenue would be generated by control units.
OEMs often follow different strategies for their lower-end ADAS and higher-end AD solutions, so suppliers that want to play across the entire technology stack may need to work within flexible delivery models. This could include supplying components, delivering stand-alone software functions such as parking, or delivering fully integrated ADAS or AD stacks. While delivering components is a business model that allows partnering with many different OEMs, supplying targeted software solutions or fully integrated software stacks is only possible when OEMs have decided to outsource.
Because most leading OEMs in AD development use in-house development for their most advanced systems, the number of potential customers for full-stack solutions is quite limited. For singular functions or add-ons (for example, parking or less sophisticated ADAS systems), there is a larger range of customers looking for suppliers. ADAS and AD systems are highly dependent on software, so supply chain monetization strategies could change. For instance, instead of charging a one-time fee for each component, suppliers might charge for performing regular system updates. They might even transition to a revenue-sharing model, which would increase the financial incentive to keep features up to date.
New technology companies are also entering a market previously reserved for tier-one automotive suppliers. Tech companies currently active in the passenger car market are mainly starting from a system-on-chip competency and building the software suite on top. There is also the chance that, in the future, L4 robo-taxi and robo-shuttle technology providers may enter the auto-supply market, but these companies will need to evaluate the applicability of AD technologies and cost positions against what customers require from passenger cars.

What’s next for autonomous vehicles?
At first glance, these new tech companies may appear to threaten incumbent tier-one auto suppliers, since they compete for business from the same OEMs. But tech companies and incumbent tier-one suppliers could potentially benefit from new partnership opportunities in which they provide complementary capabilities in software and hardware development that would also help to industrialize AD solutions.
Securing a leading position as an AD supplier will likely be challenging. It may require companies to develop strong capabilities in technology and economies of scale to attain a position as cost leader. But as suppliers begin to talk to OEMs about equipping fleets with new technologies, additional new business opportunities—including profit sharing—could arise. Critically, suppliers could benefit from a new operating model for working with OEMs that ensures sufficient upside beyond just sharing risks, since suppliers do not have the direct access to car buyers or drivers that would allow them to communicate certain value propositions.
High potential, high uncertainty
New AD technologies have tremendous potential to provide new levels of safety and convenience for consumers, generate significant value within the auto industry, and transform how people travel. At the same time, the dynamic and rapidly evolving AD passenger car market is producing high levels of uncertainty. All companies in the AD value chain—from automakers and suppliers to technology providers—must have clear, well-aligned strategies. Companies seeking to win in the autonomous passenger car market could benefit from a targeted value proposition, a clear vision of where the market is heading (including well-developed scenarios that cover the next ten years at minimum), and an understanding of what consumers want most.
To start, companies can evaluate their starting positions against their longer-term business goals and priorities. The result should be an AD portfolio strategy, feature road map, and detailed implementation plan that addresses each critical success factor. Companies will likely benefit from securing key capabilities, revamping the organization, updating internal processes, and developing external relationships with partners and regulators. With OEMs regularly revisiting timelines for rolling out new AD vehicles, companies may also need to frequently review and update their business strategies. Success in AD is not a given. But to realize the full promise of autonomous driving, forward-looking companies and regulatory bodies can pave the way.
Johannes Deichmann is a partner in McKinsey’s Stuttgart office; Eike Ebel is a consultant in the Frankfurt office, where Kersten Heineke is a partner; Ruth Heuss is a senior partner in the Berlin office; and Martin Kellner is an associate partner in the Munich office, where Fabian Steiner is a consultant.
This article was edited by Belinda Yu, an editor in McKinsey’s Atlanta office.
Explore a career with us
Related articles.

The road to affordable autonomous mobility
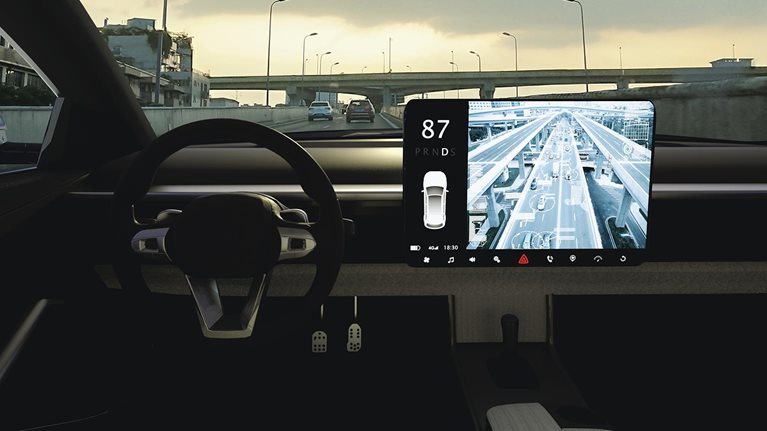
Automotive semiconductors for the autonomous age

Self-driving Vehicles
Main navigation, related documents.
2015 Study Panel Charge
June 2016 Interim Summary
Download Full Report
[ go to the annotated version ]
Since the 1930s, science fiction writers dreamed of a future with self-driving cars, and building them has been a challenge for the AI community since the 1960s. By the 2000s, the dream of autonomous vehicles became a reality in the sea and sky, and even on Mars, but self-driving cars existed only as research prototypes in labs. Driving in a city was considered to be a problem too complex for automation due to factors like pedestrians, heavy traffic, and the many unexpected events that can happen outside of the car’s control. Although the technological components required to make such autonomous driving possible were available in 2000—and indeed some autonomous car prototypes existed [30] [31] [32] —few predicted that mainstream companies would be developing and deploying autonomous cars by 2015. During the first Defense Advanced Research Projects Agency (DARPA) “grand challenge” on autonomous driving in 2004, research teams failed to complete the challenge in a limited desert setting.
But in eight short years, from 2004-2012, speedy and surprising progress occurred in both academia and industry. Advances in sensing technology and machine learning for perception tasks has sped progress and, as a result, Google’s autonomous vehicles and Tesla’s semi-autonomous cars are driving on city streets today. Google’s self-driving cars, which have logged more than 1,500,000 miles (300,000 miles without an accident), [33] are completely autonomous—no human input needed. Tesla has widely released self-driving capability to existing cars with a software update. [34] Their cars are semi-autonomous, with human drivers expected to stay engaged and take over if they detect a potential problem. It is not yet clear whether this semi-autonomous approach is sustainable, since as people become more confident in the cars' capabilities, they are likely to pay less attention to the road, and become less reliable when they are most needed. The first traffic fatality involving an autonomous car, which occurred in June of 2016, brought this question into sharper focus. [35]
In the near future, sensing algorithms will achieve super-human performance for capabilities required for driving. Automated perception, including vision, is already near or at human-level performance for well-defined tasks such as recognition and tracking. Advances in perception will be followed by algorithmic improvements in higher level reasoning capabilities such as planning. A recent report predicts self-driving cars to be widely adopted by 2020. [36] And the adoption of self-driving capabilities won’t be limited to personal transportation. We will see self-driving and remotely controlled delivery vehicles, flying vehicles, and trucks. Peer-to-peer transportation services (e.g. ridesharing) are also likely to utilize self-driving vehicles. Beyond self-driving cars, advances in robotics will facilitate the creation and adoption of other types of autonomous vehicles, including robots and drones.
It is not yet clear how much better self-driving cars need to become to encourage broad acceptance. The collaboration required in semi-self-driving cars and its implications for the cognitive load of human drivers is not well understood. But if future self-driving cars are adopted with the predicted speed, and they exceed human-level performance in driving, other significant societal changes will follow. Self-driving cars will eliminate one of the biggest causes of accidental death and injury in United States, and lengthen people’s life expectancy. On average, a commuter in US spends twenty-five minutes driving each way. [37] With self-driving car technology, people will have more time to work or entertain themselves during their commutes. And the increased comfort and decreased cognitive load with self-driving cars and shared transportation may affect where people choose to live. The reduced need for parking may affect the way cities and public spaces are designed. Self-driving cars may also serve to increase the freedom and mobility of different subgroups of the population, including youth, elderly and disabled.
Self-driving cars and peer-to-peer transportation services may eliminate the need to own a vehicle. The effect on total car use is hard to predict. Trips of empty vehicles and people’s increased willingness to travel may lead to more total miles driven. Alternatively, shared autonomous vehicles—people using cars as a service rather than owning their own—may reduce total miles, especially if combined with well-constructed incentives, such as tolls or discounts, to spread out travel demand, share trips, and reduce congestion. The availability of shared transportation may displace the need for public transportation—or public transportation may change form towards personal rapid transit, already available in four cities, [38] which uses small capacity vehicles to transport people on demand and point-to-point between many stations. [39]
As autonomous vehicles become more widespread, questions will arise over their security, including how to ensure that technologies are safe and properly tested under different road conditions prior to their release. Autonomous vehicles and the connected transportation infrastructure will create a new venue for hackers to exploit vulnerabilities to attack. There are also ethical questions involved in programming cars to act in situations in which human injury or death is inevitable, especially when there are split-second choices to be made regarding whom to put at risk. The legal systems in most states in the US do not have rules covering self-driving cars. As of 2016, four states in the US (Nevada, Florida, California, and Michigan), Ontario in Canada, the United Kingdom, France, and Switzerland have passed rules for the testing of self-driving cars on public roads. Even these laws do not address issues about responsibility and assignment of blame for an accident for self-driving and semi-self-driving cars. [40]
[30] "Navlab," Wikipedia , last updated June 4, 2016, accessed August 1, 2016, https://en.wikipedia.org/wiki/Navlab .
[31] "Navlab: The Carnegie Mellon University Navigation Laboratory," Carnegie Mellon University, accessed August 1, 2016, http://www.cs.cmu.edu/afs/cs/project/alv/www/ .
[32] "Eureka Prometheus Project," Wikipedia , last modified February 12, 2016, accessed August 1, 2016, https://en.wikipedia.org/wiki/Eureka_Prometheus_Project .
[33] “Google Self-Driving Car Project,” Google, accessed August 1, 2016, https://www.google.com/selfdrivingcar/ .
[34] Molly McHugh, "Tesla’s Cars Now Drive Themselves, Kinda," Wired , October 14, 2015, accessed August 1, 2016, http://www.wired.com/2015/10/tesla-self-driving-over-air-update-live/ .
[35] Anjali Singhvi and Karl Russell, "Inside the Self-Driving Tesla Fatal Accident," The New York Times , Last updated July 12, 2016, accessed August 1, 2016, http://www.nytimes.com/interactive/2016/07/01/business/inside-tesla-accident.html .
[36] John Greenough, "10 million self-driving cars will be on the road by 2020," Business Insider , June 15, 2016, accessed August 1, 2016, http://www.businessinsider.com/report-10-million-self-driving-cars-will-be-on-the-road-by-2020-2015-5-6 .
[37] Brian McKenzie and Melanie Rapino, "Commuting in the United States: 2009," American Community Survey Reports , United States Census Bureau, September 2011, accessed August 1, 2016, https://www.census.gov/prod/2011pubs/acs-15.pdf .
[38] Morgantown, West Virginia; Masdar City, UAE; London, England; and Suncheon, South Korea.
[39] "Personal rapid transit," Wikipedia , Last modified July 18, 2016, accessed August 1, 2016, https://en.wikipedia.org/wiki/Personal_rapid_transit .
[40] Patrick Lin, "The Ethics of Autonomous Cars," The Atlantic , October 8, 2013, accessed August 1, 2016, http://www.theatlantic.com/technology/archive/2013/10/the-ethics-of-autonomous-cars/280360/ .
Cite This Report
Peter Stone, Rodney Brooks, Erik Brynjolfsson, Ryan Calo, Oren Etzioni, Greg Hager, Julia Hirschberg, Shivaram Kalyanakrishnan, Ece Kamar, Sarit Kraus, Kevin Leyton-Brown, David Parkes, William Press, AnnaLee Saxenian, Julie Shah, Milind Tambe, and Astro Teller. "Artificial Intelligence and Life in 2030." One Hundred Year Study on Artificial Intelligence: Report of the 2015-2016 Study Panel, Stanford University, Stanford, CA, September 2016. Doc: http://ai100.stanford.edu/2016-report . Accessed: September 6, 2016.
Report Authors
AI100 Standing Committee and Study Panel
© 2016 by Stanford University. Artificial Intelligence and Life in 2030 is made available under a Creative Commons Attribution-NoDerivatives 4.0 License (International): https://creativecommons.org/licenses/by-nd/4.0/ .
Thank you for visiting nature.com. You are using a browser version with limited support for CSS. To obtain the best experience, we recommend you use a more up to date browser (or turn off compatibility mode in Internet Explorer). In the meantime, to ensure continued support, we are displaying the site without styles and JavaScript.
- View all journals
- Explore content
- About the journal
- Publish with us
- Sign up for alerts
- 24 October 2018
Self-driving car dilemmas reveal that moral choices are not universal
You can also search for this author in PubMed Google Scholar
When a driver slams on the brakes to avoid hitting a pedestrian crossing the road illegally, she is making a moral decision that shifts risk from the pedestrian to the people in the car. Self-driving cars might soon have to make such ethical judgments on their own — but settling on a universal moral code for the vehicles could be a thorny task, suggests a survey of 2.3 million people from around the world.
Access options
Access Nature and 54 other Nature Portfolio journals
Get Nature+, our best-value online-access subscription
24,99 € / 30 days
cancel any time
Subscribe to this journal
Receive 51 print issues and online access
185,98 € per year
only 3,65 € per issue
Rent or buy this article
Prices vary by article type
Prices may be subject to local taxes which are calculated during checkout
Nature 562 , 469-470 (2018)
doi: https://doi.org/10.1038/d41586-018-07135-0
Awad, E. et al . Nature https://doi.org/10.1038/s41586-018-0637-6 (2018).
Article Google Scholar
Bonnefon, J. et al. Science 352 , 1573-1576 (2016).
Article PubMed Google Scholar
Download references
Reprints and permissions
Related Articles
Autonomous vehicles: No drivers required
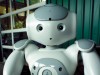
Editorial: Road test
- Computer science
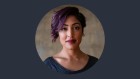
AI-fuelled election campaigns are here — where are the rules?
World View 09 APR 24
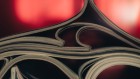
How papers with doctored images can affect scientific reviews
News 28 MAR 24
Superconductivity case shows the need for zero tolerance of toxic lab culture
Correspondence 26 MAR 24
How to break big tech’s stranglehold on AI in academia
Correspondence 09 APR 24
Use fines from EU social-media act to fund research on adolescent mental health
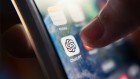
‘Without these tools, I’d be lost’: how generative AI aids in accessibility
Technology Feature 08 APR 24
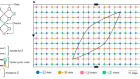
High-threshold and low-overhead fault-tolerant quantum memory
Article 27 MAR 24
Three reasons why AI doesn’t model human language
Correspondence 19 MAR 24
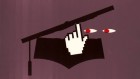
So … you’ve been hacked
Technology Feature 19 MAR 24
Junior Group Leader Position at IMBA - Institute of Molecular Biotechnology
The Institute of Molecular Biotechnology (IMBA) is one of Europe’s leading institutes for basic research in the life sciences. IMBA is located on t...
Austria (AT)
IMBA - Institute of Molecular Biotechnology
Husbandry Technician I
Memphis, Tennessee
St. Jude Children's Research Hospital (St. Jude)
Lead Researcher – Department of Bone Marrow Transplantation & Cellular Therapy
Researcher in the center for in vivo imaging and therapy, scientist or lead researcher (protein engineering, hematology, shengdar q. tsai lab).
Sign up for the Nature Briefing newsletter — what matters in science, free to your inbox daily.
Quick links
- Explore articles by subject
- Guide to authors
- Editorial policies
Suggestions or feedback?
MIT News | Massachusetts Institute of Technology
- Machine learning
- Social justice
- Black holes
- Classes and programs
Departments
- Aeronautics and Astronautics
- Brain and Cognitive Sciences
- Architecture
- Political Science
- Mechanical Engineering
Centers, Labs, & Programs
- Abdul Latif Jameel Poverty Action Lab (J-PAL)
- Picower Institute for Learning and Memory
- Lincoln Laboratory
- School of Architecture + Planning
- School of Engineering
- School of Humanities, Arts, and Social Sciences
- Sloan School of Management
- School of Science
- MIT Schwarzman College of Computing
Exploring new methods for increasing safety and reliability of autonomous vehicles
Press contact :.
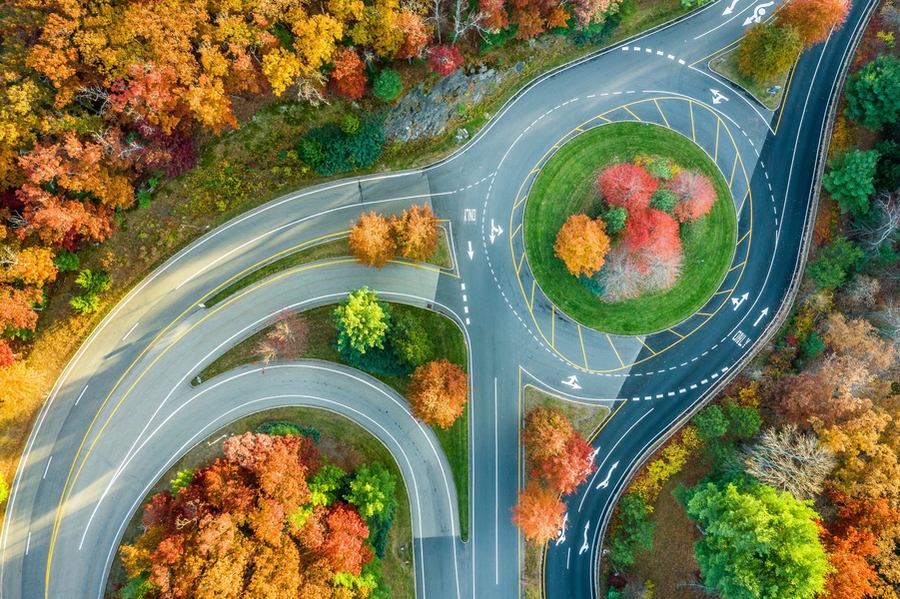
Previous image Next image
When we think of getting on the road in our cars, our first thoughts may not be that fellow drivers are particularly safe or careful — but human drivers are more reliable than one may expect. For each fatal car crash in the United States, motor vehicles log a whopping hundred million miles on the road.
Human reliability also plays a role in how autonomous vehicles are integrated in the traffic system, especially around safety considerations. Human drivers continue to surpass autonomous vehicles in their ability to make quick decisions and perceive complex environments: Autonomous vehicles are known to struggle with seemingly common tasks, such as taking on- or off-ramps, or turning left in the face of oncoming traffic. Despite these enormous challenges, embracing autonomous vehicles in the future could yield great benefits, like clearing congested highways; enhancing freedom and mobility for non-drivers; and boosting driving efficiency, an important piece in fighting climate change.
MIT engineer Cathy Wu envisions ways that autonomous vehicles could be deployed with their current shortcomings, without experiencing a dip in safety. “I started thinking more about the bottlenecks. It’s very clear that the main barrier to deployment of autonomous vehicles is safety and reliability,” Wu says.
One path forward may be to introduce a hybrid system, in which autonomous vehicles handle easier scenarios on their own, like cruising on the highway, while transferring more complicated maneuvers to remote human operators. Wu, who is a member of the Laboratory for Information and Decision Systems (LIDS), a Gilbert W. Winslow Assistant Professor of Civil and Environmental Engineering (CEE) and a member of the MIT Institute for Data, Systems, and Society (IDSS), likens this approach to air traffic controllers on the ground directing commercial aircraft.
In a paper published April 12 in IEEE Transactions on Robotics , Wu and co-authors Cameron Hickert and Sirui Li (both graduate students at LIDS) introduced a framework for how remote human supervision could be scaled to make a hybrid system efficient without compromising passenger safety. They noted that if autonomous vehicles were able to coordinate with each other on the road, they could reduce the number of moments in which humans needed to intervene.
Humans and cars: finding a balance that’s just right
For the project, Wu, Hickert, and Li sought to tackle a maneuver that autonomous vehicles often struggle to complete. They decided to focus on merging, specifically when vehicles use an on-ramp to enter a highway. In real life, merging cars must accelerate or slow down in order to avoid crashing into cars already on the road. In this scenario, if an autonomous vehicle was about to merge into traffic, remote human supervisors could momentarily take control of the vehicle to ensure a safe merge. In order to evaluate the efficiency of such a system, particularly while guaranteeing safety, the team specified the maximum amount of time each human supervisor would be expected to spend on a single merge. They were interested in understanding whether a small number of remote human supervisors could successfully manage a larger group of autonomous vehicles, and the extent to which this human-to-car ratio could be improved while still safely covering every merge.
With more autonomous vehicles in use, one might assume a need for more remote supervisors. But in scenarios where autonomous vehicles coordinated with each other, the team found that cars could significantly reduce the number of times humans needed to step in. For example, a coordinating autonomous vehicle already on a highway could adjust its speed to make room for a merging car, eliminating a risky merging situation altogether.
The team substantiated the potential to safely scale remote supervision in two theorems. First, using a mathematical framework known as queuing theory, the researchers formulated an expression to capture the probability of a given number of supervisors failing to handle all merges pooled together from multiple cars. This way, the researchers were able to assess how many remote supervisors would be needed in order to cover every potential merge conflict, depending on the number of autonomous vehicles in use. The researchers derived a second theorem to quantify the influence of cooperative autonomous vehicles on surrounding traffic for boosting reliability, to assist cars attempting to merge.
When the team modeled a scenario in which 30 percent of cars on the road were cooperative autonomous vehicles, they estimated that a ratio of one human supervisor to every 47 autonomous vehicles could cover 99.9999 percent of merging cases. But this level of coverage drops below 99 percent, an unacceptable range, in scenarios where autonomous vehicles did not cooperate with each other.
“If vehicles were to coordinate and basically prevent the need for supervision, that’s actually the best way to improve reliability,” Wu says.
Cruising toward the future
The team decided to focus on merging not only because it’s a challenge for autonomous vehicles, but also because it’s a well-defined task associated with a less-daunting scenario: driving on the highway. About half of the total miles traveled in the United States occur on interstates and other freeways. Since highways allow higher speeds than city roads, Wu says, “If you can fully automate highway driving … you give people back about a third of their driving time.”
If it became feasible for autonomous vehicles to cruise unsupervised for most highway driving, the challenge of safely navigating complex or unexpected moments would remain. For instance, “you [would] need to be able to handle the start and end of the highway driving,” Wu says. You would also need to be able to manage times when passengers zone out or fall asleep, making them unable to quickly take over controls should it be needed. But if remote human supervisors could guide autonomous vehicles at key moments, passengers may never have to touch the wheel. Besides merging, other challenging situations on the highway include changing lanes and overtaking slower cars on the road.
Although remote supervision and coordinated autonomous vehicles are hypotheticals for high-speed operations, and not currently in use, Wu hopes that thinking about these topics can encourage growth in the field.
“This gives us some more confidence that the autonomous driving experience can happen,” Wu says. “I think we need to be more creative about what we mean by ‘autonomous vehicles.’ We want to give people back their time — safely. We want the benefits, we don’t strictly want something that drives autonomously.”
Share this news article on:
Related links.
- Laboratory for Information and Decision Systems
- Institute for Data, Systems, and Society
- Department of Civil and Environmental Engineering
Related Topics
- Autonomous vehicles
- Technology and society
- Human-computer interaction
- Civil and environmental engineering
- Laboratory for Information and Decision Systems (LIDS)
Related Articles
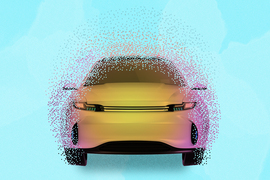
Computers that power self-driving cars could be a huge driver of global carbon emissions
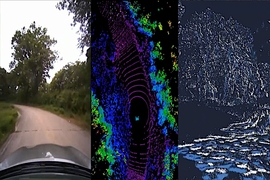
Researchers release open-source photorealistic simulator for autonomous driving
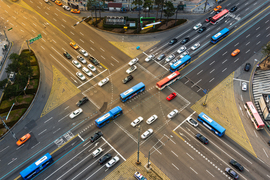
On the road to cleaner, greener, and faster driving
Previous item Next item
More MIT News
A biomedical engineer pivots from human movement to women’s health
Read full story →
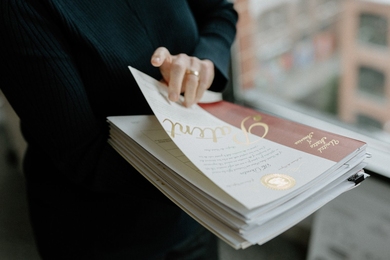
MIT tops among single-campus universities in US patents granted
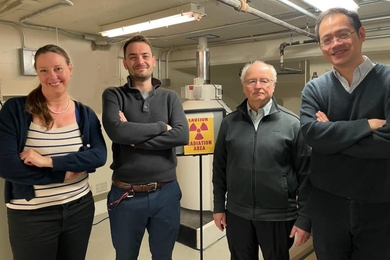
A new way to detect radiation involving cheap ceramics
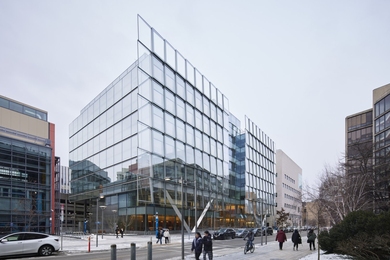
A crossroads for computing at MIT
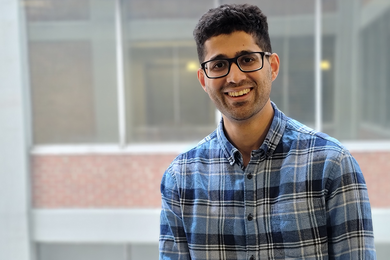
Growing our donated organ supply
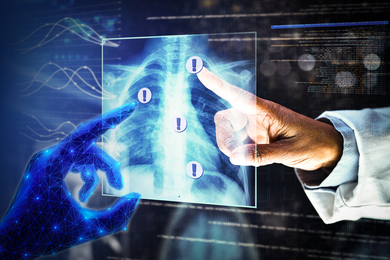
New AI method captures uncertainty in medical images
- More news on MIT News homepage →
Massachusetts Institute of Technology 77 Massachusetts Avenue, Cambridge, MA, USA
- Map (opens in new window)
- Events (opens in new window)
- People (opens in new window)
- Careers (opens in new window)
- Accessibility
- Social Media Hub
- MIT on Facebook
- MIT on YouTube
- MIT on Instagram
Play with a live Neptune project -> Take a tour 📈
Self-Driving Cars With Convolutional Neural Networks (CNN)
Humanity has been waiting for self-driving cars for several decades. Thanks to the extremely fast evolution of technology, this idea recently went from “possible” to “commercially available in a Tesla”.
Deep learning is one of the main technologies that enabled self-driving. It’s a versatile tool that can solve almost any problem – it can be used in physics, for example, the proton-proton collision in the Large Hadron Collider, just as well as in Google Lens to classify pictures. Deep learning is a technology that can help solve almost any type of science or engineering problem.
In this article, we’ll focus on deep learning algorithms in self-driving cars – convolutional neural networks (CNN). CNN is the primary algorithm that these systems use to recognize and classify different parts of the road, and to make appropriate decisions.
Along the way, we’ll see how Tesla, Waymo, and Nvidia use CNN algorithms to make their cars driverless or autonomous.
You may also like
Experiment Tracking for Systems Powering Self-Driving Vehicles [Case Study with Waabi]
How do self-driving cars work?
The first self-driving car was invented in 1989, it was the Automatic Land Vehicle in Neural Network (ALVINN). It used neural networks to detect lines, segment the environment, navigate itself, and drive. It worked well, but it was limited by slow processing powers and insufficient data.
With today’s high-performance graphics cards, processors, and huge amounts of data, self-driving is more powerful than ever. If it becomes mainstream, it will reduce traffic congestion and increase road safety.
Self-driving cars are autonomous decision-making systems. They can process streams of data from different sensors such as cameras, LiDAR, RADAR, GPS, or inertia sensors. This data is then modeled using deep learning algorithms, which then make decisions relevant to the environment the car is in.

The image above shows a modular perception-planning-action pipeline used to make driving decisions. The key components of this method are the different sensors that fetch data from the environment.
To understand the workings of self-driving cars, we need to examine the four main parts:
Perception
Localization.
- High-level path planning
- Behaviour Arbitration
- Motion Controllers
One of the most important properties that self-driving cars must have is perception , which helps the car see the world around itself, as well as recognize and classify the things that it sees. In order to make good decisions, the car needs to recognize objects instantly.
So, the car needs to see and classify traffic lights, pedestrians, road signs, walkways, parking spots, lanes, and much more. Not only that, it also needs to know the exact distance between itself and the objects around it. Perception is more than just seeing and classifying, it enables the system to evaluate the distance and decide to either slow down or brake.
To achieve such a high level of perception, a self-driving car must have three sensors:
The camera provides vision to the car, enabling multiple tasks like classification, segmentation, and localization . The cameras need to be high-resolution and represent the environment accurately.
In order to make sure that the car receives visual information from every side: front, back, left, and right, the cameras are stitched together to get a 360-degree view of the entire environment. These cameras provide a wide-range view as far as 200 meters as well as a short-range view for more focused perception.

In some tasks like parking, the camera also provides a panoramic view for better decision-making.
Even though the cameras do all the perception related tasks, it’s hardly of any use during extreme conditions like fog, heavy rain, and especially at night time. During extreme conditions, all that cameras capture is noise and discrepancies, which can be life-threatening.
To overcome these limitations, we need sensors that can work without light and also measure distance.
LiDAR stands for Light Detection And Ranging, it’s a method to measure the distance of objects by firing a laser beam and then measuring how long it takes for it to be reflected by something.
A camera can only provide the car with images of what’s going around itself. When it’s combined with the LiDAR sensor, it gains depth in the images – it suddenly has a 3D perception of what’s going on around the car.
So, LiDAR perceives spatial information . And when this data is fed into deep neural networks, the car can predict the actions of the objects or vehicles close to it. This sort of technology is very useful in a complex driving scenario, like a multi-exit intersection, where the car can analyze all other cars and make the appropriate, safest decision.
In 2019, Elon Musk openly stated that “ anyone relying on LiDARs are doomed… ”. Why? Well, LiDARs have limitations that can be catastrophic. For example, the LiDAR sensor uses lasers or light to measure the distance of the nearby object. It will work at night and in dark environments, but it can still fail when there’s noise from rain or fog. That’s why we also need a RADAR sensor.
Radio detection and ranging (RADAR) is a key component in many military and consumer applications. It was first used by the military to detect objects. It calculates distance using radio wave signals . Today, it’s used in many vehicles and has become a primary component of the self-driving car.
RADARs are highly effective because they use radio waves instead of lasers, so they work in any conditions.

It’s important to understand that radars are noisy sensors. This means that even if the camera sees no obstacle, the radar will detect some obstacles.

The image above shows the self-driving car (in green) using LiDAR to detect objects around and to calculate the distance and shape of the object. Compare the same scene, but captured with the RADAR sensor below, and you can see a lot of unnecessary noise.

The RADAR data should be cleaned in order to make good decisions and predictions. We need to separate weak signals from strong ones; this is called thresholding . We also use Fast Fourier Transforms (FFT) to filter and interpret the signal.
If you look at the below above, you’ll notice that the RADAR and LiDAR signals are point-based data. This data should be clustered so that it can be interpreted nicely. Clustering algorithms such as Euclidean Clustering or K means Clustering are used to achieve this task.

Localization algorithms in self-driving cars calculate the position and orientation of the vehicle as it navigates – a science known as Visual Odometry (VO).
VO works by matching key points in consecutive video frames. With each frame, the key points are used as the input to a mapping algorithm. The mapping algorithm, such as Simultaneous localization and mapping (SLAM), computes the position and orientation of each object nearby with respect to the previous frame and helps to classify roads, pedestrians, and other objects around.

Deep learning is generally used to improve the performance of VO, and to classify different objects. Neural networks, such as PoseNet and VLocNet++, are some of the frameworks that use point data to estimate the 3D position and orientation. These estimated 3D positions and orientations can be used to derive scene semantics, as seen in the image below.

Understanding human drivers is a very complex task. It involves emotions rather than logic, and these are all fueled with reactions . It becomes very uncertain what the next action will be of the drivers or pedestrians nearby, so a system that can predict the actions of other road users can be very important for road safety.
The car has a 360-degree view of its environment that enables it to perceive and capture all the information and process it. Once fed into the deep learning algorithm, it can come up with all the possible moves that other road users might make. It’s like a game where the player has a finite number of moves and tries to find the best move to defeat the opponent.
The sensors in self-driving cars enable them to perform tasks like image classification, object detection, segmentation, and localization. With various forms of data representation, the car can make predictions of the object around it.
A deep learning algorithm can model such information (images and cloud data points from LiDARs and RADARs) during training. The same model, but during inference, can help the car to prepare for all the possible moves which involve braking, halting, slowing down, changing lanes, and so on.
The role of deep learning is to interpret complex vision tasks, localize itself in the environment, enhance perception, and actuate kinematic maneuvers in self-driving cars. This ensures road safety and easy commute as well.
But the tricky part is to choose the correct action out of a finite number of actions.
Decision-making
Decision-making is vital in self-driving cars. They need a system that’s dynamic and precise in an uncertain environment. It needs to take into account that not all sensor readings will be true, and that humans can make unpredictable choices while driving. These things can’t be measured directly. Even if we could measure them, we can’t predict them with good accuracy.

The image above shows a self-driving car moving towards an intersection. Another car, in blue, is also moving towards the intersection. In this scenario, the self-driving car has to predict whether the other car will go straight, left, or right. In each case, the car has to decide what maneuver it should perform to prevent a collision.
In order to make a decision, the car should have enough information so that it can select the necessary set of actions. We learned that the sensors help the car to collect information and deep learning algorithms can be used for localization and prediction.
To recap, localization enables the car to know its initial position, and prediction creates an n number of possible actions or moves based on the environment. The question remains: which option is best out of the many predicted actions?
When it comes to making decisions, we use deep reinforcement learning (DRL). More specifically, a decision-making algorithm called the Markov decision process (MDP) lies at the heart of DRL (we’ll learn more about MDP in a later section where we talk about reinforcement learning).
Usually, an MDP is used to predict the future behavior of the road-users. We should keep in mind that the scenario can get very complex if the number of objects, especially moving ones, increases. This eventually increases the number of possible moves for the self-driving car itself.
In order to tackle the problem of finding the best move for itself, the deep learning model is optimized with Bayesian optimization . There are also situations where the framework, consisting of both a hidden Markov model and Bayesian Optimization, is used for decision-making.
In general, decision-making in self-driving cars is a hierarchical process. This process has four components:
- Path or Route planning : Essentially, route planning is the first of four decisions that the car must make. Entering the environment, the car should plan the best possible route from its current position to the requested destination. The idea is to find an optimal solution among all the other solutions.
- Behaviour Arbitration : Once the route is planned, the car needs to navigate itself through the route. The car knows about the static elements, like roads, intersections, average road congestion and more, but it can’t know exactly what the other road users are going to be doing throughout the journey. This uncertainty in the behavior of other road users is solved by using probabilistic planning algorithms like MDPs.
- Motion Planning : Once the behavior layer decides how to navigate through a certain route, the motion planning system orchestrates the motion of the car. The motion of the car must be feasible and comfortable for the passenger. Motion planning includes speed of the vehicle, lane-changing, and more, all of which should be relevant to the environment the car is in.
- Vehicle Control : Vehicle control is used to execute the reference path from the motion planning system.

CNNs used for self-driving cars
Convolutional neural networks (CNN) are used to model spatial information, such as images. CNNs are very good at extracting features from images, and they’re often seen as universal non-linear function approximators.
CNNs can capture different patterns as the depth of the network increases. For example, the layers at the beginning of the network will capture edges, while the deep layers will capture more complex features like the shape of the objects (leaves in trees, or tires on a vehicle). This is the reason why CNNs are the main algorithm in self-driving cars.
The key component of the CNN is the convolutional layer itself. It has a convolutional kernel which is often called the filter matrix . The filter matrix is convolved with a local region of the input image which can be defined as:

Where:
- the operator * represents the convolution operation,
- w is the filter matrix and b is the bias,
- x is the input,
- y is the output.
The dimension of the filter matrix in practice is usually 3X3 or 5X5. During the training process, the filter matrix will constantly update itself to get a reasonable weight. One of the properties of CNN is that the weights are shareable. The same weight parameters can be used to represent two different transformations in the network. The shared parameter saves a lot of processing space; they can produce more diverse feature representations learned by the network.
The output of the CNN is usually fed to a nonlinear activation function . The activation function enables the network to solve the linear inseparable problems, and these functions can represent high-dimensional manifolds in lower-dimensional manifolds. Commonly used activation functions are Sigmoid, Tanh, and ReLU, which are listed as follows:

It’s worth mentioning that the ReLU is the preferred activation function, because it converges faster compared to the other activation functions. In addition to that, the output of the convolution layer is modified by the max-pooling layer which keeps more information about the input image, like the background and texture.
The three important CNN properties that make them versatile and a primary component of self-driving cars are:
- local receptive fields,
- shared weights,
- spatial sampling .
These properties reduce overfitting and store representations and features that are vital for image classification, segmentation, localization, and more.

Next, we’ll discuss three CNN networks that are used by three companies pioneering self-driving cars:
- HydraNet by Tesla
- ChauffeurNet by Google Waymo
- Nvidia Self driving car
HydraNet – semantic segmentation for self-driving cars
HydraNet was introduced by Ravi et al. in 2018 . It was developed for semantic segmentation, for improving computational efficiency during inference time.

HydraNets is dynamic architecture so it can have different CNN networks, each assigned to different tasks. These blocks or networks are called branches. The idea of HydraNet is to get various inputs and feed them into a task-specific CNN network.
Take the context of self-driving cars. One input dataset can be of static environments like trees and road-railing, another can be of the road and the lanes, another of traffic lights and road, and so on. These inputs are trained in different branches. During the inference time, the gate chooses which branches to run, and the combiner aggregates branch outputs and makes a final decision.
In the case of Tesla, they have modified this network slightly because it’s difficult to segregate data for the individual tasks during inference. To overcome that problem, engineers at Tesla developed a shared backbone. The shared backbones are usually modified ResNet-50 blocks.
This HydraNet is trained on all the object’s data. There are task-specific heads that allow the model to predict task-specific outputs. The heads are based on semantic segmentation architecture like the U-Net.

The Tesla HydraNet can also project a birds-eye, meaning it can create a 3D view of the environment from any angle, giving the car much more dimensionality to navigate properly. It’s important to know that Tesla doesn’t use LiDAR sensors. It has only two sensors, a camera and a radar. Although LiDAR explicitly creates depth perception for the car, Tesla’s hydranet is so efficient that it can stitch all the visual information from the 8 cameras in it and create depth perception.

ChauffeurNet: training self-driving car using imitation learning
ChauffeurNet is an RNN-based neural network used by Google Waymo, however, CNN is actually one of the core components here and it’s used to extract features from the perception system.
The CNN in ChauffeurNet is described as a convolutional feature network, or FeatureNet, that extracts contextual feature representation shared by the other networks. These representations are then fed to a recurrent agent network (AgentRNN) that iteratively yields the prediction of successive points in the driving trajectory.
The idea behind this network is to train a self-driving car using imitation learning. In the paper released by Bansal et al “ ChauffeurNet: Learning to Drive by Imitating the Best and Synthesizing the Worst ”, they argue that training a self-driving car even with 30 million examples is not enough. In order to tackle that limitation, the authors trained the car in synthetic data. This synthetic data introduced deviations such as introducing perturbation to the trajectory path, adding obstacles, introducing unnatural scenes, etc. They found that such synthetic data was able to train the car much more efficiently than the normal data.
Usually, self-driving has an end-to-end process as we saw earlier, where the perception system is part of a deep learning algorithm along with planning and controlling. In the case of ChauffeurNet, the perception system is not a part of the end-to-end process; instead, it’s a mid-level system where the network can have different variations of input from the perception system.

ChauffeurNet yields a driving trajectory by observing a mid-level representation of the scene from the sensors, using the input along with synthetic data to imitate an expert driver.

In the image above, the cyan path depicts the input route, green box is the self-driving car, blue dots are the agent’s past route or position, and green dots are the predicted future routes or positions.
Essentially, a mid-level representation doesn’t directly use raw sensor data as input, factoring out the perception task, so we can combine real and simulated data for easier transfer learning. This way, the network can create a high-level bird’s eye view of the environment which ultimately yields better decisions.
Nvidia self-driving car: a minimalist approach towards self-driving cars
Nvidia also uses a Convolution Neural Network as a primary algorithm for its self-driving car. But unlike Tesla, it uses 3 cameras, one on each side and one at the front. See the image below.

The network is capable of operating inroads that don’t have lane markings, including parking lots. It can also learn features and representations that are necessary for detecting useful road features.
Compared to the explicit decomposition of the problem such as lane marking detection, path planning, and control, this end-to-end system optimizes all processing steps at the same time.
Better performance is the result of internal components self-optimizing to maximize overall system performance, instead of optimizing human-selected intermediate criteria like lane detection. Such criteria understandably are selected for ease of human interpretation, which doesn’t automatically guarantee maximum system performance. Smaller networks are possible because the system learns to solve the problem with a minimal number of processing steps.
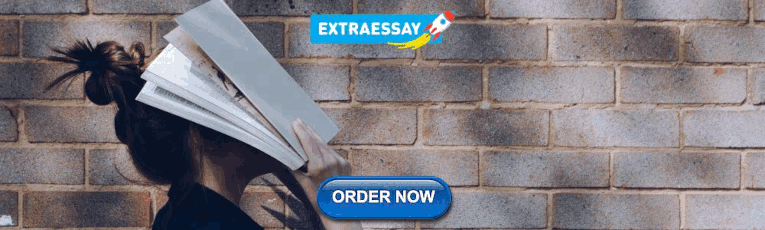
Reinforcement learning used for self-driving cars
Reinforcement learning (RL) is a type of machine learning where an agent learns by exploring and interacting with the environment. In this case, the self-driving car is an agent .
Explore more applications of RL
10 Real-Life Applications of Reinforcement Learning
7 Applications of Reinforcement Learning in Finance and Trading
We discussed earlier how the neural network predicts a number of actions from the perception data. But, choosing an appropriate action requires deep reinforcement learning (DRL). At the core of DRL, we have three important variables:
- State describes the current situation in a given time. In this case, it would be a position on the road.
- Action describes all the possible moves that the car can make.
- Reward is feedback that the car receives whenever it takes a certain action.
Generally, the agent is not told what to do or what actions to take. So far as we have seen, in supervised learning, the algorithm maps input to the output. In DRL, the algorithm learns by exploring the environment and each interaction yields a certain reward. The reward can be both positive and negative. The goal of the DRL is to maximize the cumulative rewards.
In self-driving cars, the same procedure is followed: the network is trained on perception data, where it learns what decision it should make. Because the CNNs are very good at extracting features of representations from the input, DRL algorithms can be trained on those representations. Training a DRL algorithm on these representations can yield good results because these extracted representations are the transformation of higher-dimensional manifolds into simpler lower-dimensional manifolds. Training on lower representation yields efficiency which is required at the inference.
One key point to remember is that self-driving cars can’t be trained in real-world scenarios or roads because they will be extremely dangerous. Instead, self-driving cars are trained on a simulator where there’s no risk at all.
Some open-source simulators are:

These cars (agents) are trained for thousands of epochs with highly difficult simulations before they’re deployed in the real world.
During training, the agent (the car) learns by taking a certain action in a certain state. Based on this state-action pair, it receives a reward . This process happens over and over again. Each time the agent updates its memory of rewards. This is called the policy .
The policy is described as how the agent makes decisions. It’s a decision-making rule. The policy defines the behaviour of the agent at a given time.
For every negative decision the agent makes, the policy is changed. So in order to avoid the negative rewards, the agent checks the quality of a certain action. This is measured by the state-value function. State-value can be measured using the Bellman Expectation Equation.
The Bellman expectation equation, along with Markov Decision Process (MDP), makes up the two core concepts of DRL. But when it comes to self-driving cars, we have to keep in mind that the observations from the perception data should be mapped with the appropriate action and not just map the underlying state to the action. This is where a partially observed decision process or a Partially Observable Markov Decision Process (POMDP) is required, which can make decisions based on the observation.
Partially Observable Markov Decision Process used for self-driving cars
The Markov Decision Process gives us a way to sequentialize decision-making. When the agent interacts with the environment, it does so sequentially over time. Each time the agent interacts with the environment, it gives some representation of the environment state. Given the representation of the state, the agent selects the action to take, as in the image below.

The action taken is transitioned into some new state and the agent is given a reward. This process of evaluating a state, taking action, changing states, and rewarding is repeated. Throughout the process, it’s the agent’s goal to maximize the total amount of rewards.
Let’s get a more constructive idea of the whole process:
- At a give time t, the state of the environment is at St
- The agent observes the current state St and selects an action At
- The environment is then transitioned into a new state St+1, simultaneously the agent is rewarded Rt
In a partially observable Markov decision process (POMDP), the agent senses the environment state with observations received from the perception data and takes a certain action followed by receiving a reward.
The POMDP has six components and it can be denoted as POMDP M:= (I, S, A, R, P, γ), where,
- I: Observations
- S: Finite set of states
- A: Finite set of actions
- R: Reward function
- P: transition probability function
- γ – discounting factor for future rewards.
The objective of DRL is to find the desired policy that maximizes the reward at each given time step or, in other words, to find an optimal value-action function (Q-function).
Q-learning used for self-driving cars
Q-learning is one of the most commonly used DRL algorithms for self-driving cars. It comes under the category of model-free learning . In model-free learning, the agent will try to approximate the optimal state-action pair. The policy still determines which action-value pairs or Q-value are visited and updated (see the equation below). The goal is to find optimal policy by interacting with the environment while modifying the same when the agent makes an error.
With enough samples or observation data, Q-learning will learn optimal state-action value pairs. In practice, Q-learning has been shown to converge to the optimum state-action values for a MDP with probability 1, provided that all actions in all states are infinitely available.
Q-learning can be described in the following equation:

α ∈ [0,1] is the learning rate. It controls the degree to which Q values are updated at a given t.

It’s important to remember that the agent will discover the good and bad actions through trial and error.
Self-driving cars aim to revolutionize car travel by making it safe and efficient. In this article, we outlined some of the key components such as LiDAR, RADAR, cameras, and most importantly – the algorithms that make self-driving cars possible.
While it’s promising, there’s still a lot of room for improvement. For example, current self-driving cars are at level-2 out of level-5 of advancement, which means that there still has to be a human ready to intervene if necessary.
Few things need to be taken care of:
- The algorithms used are not yet optimal enough to perceive roads and lanes because some roads lack markings and other signs.
- The optimal sensing modality for localization, mapping, and perception still lack accuracy and efficiency.
- Vehicle-to-vehicle communication is still a dream, but work is being done in this area as well.
- The field of human-machine interaction is not explored enough, with many open, unsolved problems.
Still, the technology we’ve developed so far is amazing. And with orchestrated efforts, we can ensure that self-driving systems will be safe, robust, and revolutionary.
Further reading:
- A Survey of Deep Learning Techniques for Autonomous Driving
- A Survey of Autonomous Driving: Common Practices and Emerging Technologies
- Decision Making for Autonomous Driving considering Interaction and Uncertain Prediction of Surrounding Vehicles
- Autonomous car using CNN deep learning algorithm
- Deep Reinforcement Learning for Autonomous Driving: A Survey
- The WIRED Guide to Self-Driving Cars
- Deep Learning for Self-Driving Cars
- Training Self Driving Cars using Reinforcement Learning
- A (Long) Peek into Reinforcement Learning
- Tesla Statistics: What You Should Know About Safety, Pricing and More
Was the article useful?
More about self-driving cars with convolutional neural networks (cnn), check out our product resources and related articles below:, how to optimize gpu usage during model training with neptune.ai, zero-shot and few-shot learning with llms, llmops: what it is, why it matters, and how to implement it, the real cost of self-hosting mlflow, explore more content topics:, manage your model metadata in a single place.
Join 50,000+ ML Engineers & Data Scientists using Neptune to easily log, compare, register, and share ML metadata.
The folly of trolleys: Ethical challenges and autonomous vehicles
Subscribe to techstream, heather m. roff heather m. roff former brookings expert @hmroff.
December 17, 2018
Scholars and pundits tend to focus on one ethical challenge for autonomous cars: the Trolley Problem. Unfortunately, the focus on this ethical problem alone seems to blind experts and practitioners alike from grappling with other unaddressed ethical challenges. This paper argues that one must take the technology on its own terms. Once we understand how these vehicles work—that is, the mathematics behind their learning—we will see how there are many other design choices that are far more morally important.
Table of Contents Introduction I. It’s all about the POMDP II. The follies of trolleys III. The value functions Conclusion
- 43 min read
Introduction
Often when anyone hears about the ethics of autonomous cars the first thing to enter the conversation is “the Trolley Problem.” The Trolley Problem is a thought experiment where someone is presented with two situations that present nominally similar choices and potential consequences (Foot 1967; Kamm 1989; Kamm 2007; Otsuka 2008; Parfit 2011; Thompson 1976; Thompson 1985; Unger 1996). Situation A (known as Switch ), is where a runaway trolley is driving down a track and will run into and kill five workmen unless an observer flips a switch and diverts the train down a sidetrack that will only kill one workman. Situation B (known as Bridge ) has the observer crossing over a bridge, where she sees that the five people will be killed unless she pushes a rather large and plump individual off off the bridge onto the tracks below, thereby stopping the train and saving the five. Most philosophers agree that it is morally permissible to kill the one in Switch , but others (including most laypeople) think that it is impermissible to push the plump person in Bridge (Kagan 1989). The case has the same effects: kill one to save the five. This discrepancy in intuition has led to much spilled ink over “the problem” and led to an entire enquiry in “Trolleyology.”
Applied to autonomous cars, at first glance, the Trolley Problem seems like a natural fit. Indeed, it could be the sine qua non ethical issue for philosophers, lawyers, and engineers alike. However, if I am correct, the introduction of the Trolley is more like a death knell of any serious conversation about ethics and autonomous cars. The Trolley Problem detracts from understanding about how autonomous cars actually work, how they “think,” how much influence humans have over their decision-making processes, and the real ethical issues that face those advocating the advancement and deployment of autonomous cars in cities and towns the globe over. Instead of thinking about runaway trolleys and killing bystanders, we should have a better grounding in the technology itself. Once we have that, then we see how new—more complex and nuanced—ethical questions arise. Ones that look very little like trolleys.
I argue that we need to understand that autonomous vehicles (AVs) will be making sequential decisions in a dynamic environment under conditions of uncertainty. Once we understand that the car is not a human, and that the decision is not a single-shot, black and white one, but one that will be made at the intersection of multiple overlapping probability distributions, we will see that “judgments” about what courses of action to take are going to be not only computationally difficult, but highly context dependent and, perhaps, unknowable by a human engineer a priori . Once we can disabuse ourselves of thinking the problem is an aberrant one-off ethical dilemma, we can begin to interrogate the foreseeability of other types of ethical and social dilemmas.
The Trolley Problem detracts from understanding about how autonomous cars actually work, how they “think,” and the real ethical issues that face those advocating the advancement and deployment of autonomous cars.
This paper is organized into three parts. Part one argues that we should look at one promising area of robotic decision theory and control: Partially Observed Markov Decision Processes (POMDPs) as the most likely mathematical model an autonomous vehicle will use. 1 After explaining what this model does and looks like, section two argues that the functioning of such systems does not comport with the assumptions of the Trolley Problem. This entails, then, that viewing ethical concerns about AVs from this perspective is incorrect and will blind us to more pressing concerns. Finally, part three argues that we need to interrogate what we decide are the objective and value functions of these systems. Moreover, we need to make transparent how we use the mathematical models to get the systems to learn and to make value trade-offs. For it seems absurd to place an AV as the bearer of a moral obligation not to kill anyone, but it is not absurd to interrogate the engineers who chose various objectives to guide their systems. I conclude with some observations of the types of ethical problems that will arise with AVs, and none have the form of the Trolley.
I. It’s all about the POMDP
A Partially Observed Markov Decision Process (POMDP) is a variant of a Markov Decision Process (MDP). The MDP model is a useful mathematical model for various control and planning problems in engineering and computing. For instance, the MDP is useful in an environment that is fully observable , has discrete time intervals, and few choices of action in various conditions (Puterman 2005). We could think of an MDP model being useful in something like a game of chess or tic-tac-toe. The algorithm knows the environment fully (the board, pieces, rules) and waits for its opponent to make a move. Once that move is made, the algorithm can calculate all the potential moves it has in front of it, discounting for future moves and then taking the “best” or “optimal” decision to counter.
Unfortunately, many real-world environments are not like tic-tac-toe or chess. Moreover, when we have robotic systems, like AVs, even with many sensors attached to them, the system itself cannot have complete knowledge of its environment. There is incomplete knowledge due to limitations in the range and fidelity of the sensors, occlusions, and latency (the time it takes to process the sensor readings, the continuous, dynamic environment will have changed.) Moreover, a robot in this situation makes a decision using the current observations as well as a history of previous actions and observations. In more precise terms, a system is measuring everything it can at a particular state ( s ), and the finite set of states S = { s1 , …, sn } is the environment. When a system observes itself in s , and takes an action a , it then transitions to a new state, s’, and can take action a2 ( s’, a2 ). The set of possible actions is A = { a1 , …, ak }. Thus at any given point, a system is deciding which action to take based on its present state, its prior state (if there is one), and its expected future transitioned state. The crucial difference here is that a POMDP is working in an environment where the system (or agent) has incomplete knowledge and uncertainty, and is working from probabilities; in essence, an AV is not working from an MDP, it would more than likely be working from a POMDP.
How does a system know which action to take? There are a variety of potential ways, but I will focus on one here: reinforcement learning. For a POMDP using reinforcement learning, the autonomous vehicle learns through a receipt of some reward signal. Systems like this that use POMDPs have reward (or sometimes ‘cost’) signals that tell them which actions to pursue (or avoid). But these signals are based on probability distributions of which acts in the current state, s, will lead to more rewards in a future state, sn, discounted for future acts. Let’s take an example here to explain in easier terms. I may reason that I am tired but need to finish this paper (state), so I could decide to take a nap right now (an act in my possible set of actions), and then get up later to finish the paper. However, I also do not know if I will have restful sleep, oversleep, or feel worse when I get up, thereby frustrating my plans for my paper even more, though the thought of an immediate nap could give me an immediate reward (sleep and rest). The optimal decision (or, in POMDP speak, “policy”), could be to sleep right now. However, that is not actually correct. Rather, the POMDP requires that one pick the most optimal policy under conditions of uncertainty, for sequential decisions tasks, and discounted for future reward states. In short, the optimal policy would be for me to instead grab a cup of coffee, finish my paper, and then go to bed early because I will actually maximize my total amount of sleep by not napping during the day and getting my work done quickly.
Yet the learning really only takes place once a decision is made and there is feedback to the system. In essence, there is a requirement of some sort of system memory about past behavior and reward feedback. How robust this memory needs to be is specific to tasks and systems, but what we can say at a general level is that the POMDP needs to have a belief about its actions based on posterior observations and their corresponding distributions. 2 In short, the belief “is a sufficient statistic of the history,” without the agent actually having to remember every single possible action and observation (Spaan 2012).
Yet to build an adequate learning system, there is a need for many experiences to build robust beliefs. In my case of fatigue and academic writing, if I did not have a long history of experiences with writing academic papers and fatigue, one may think that whatever decision I take is at best considered a random one (that is, its 50/50 as to whether I act on my best policy). Yet, since I have a very long history of academic paper writing and fatigue, as well as the concomitant rewards and costs of prior decisions, I can accurately predict which action will actually maximize my reward. I know my best policy. This is because policies, generally, map beliefs to actions (Sutton and Barto 1998; Spaan 2012). 3 Yet mathematically, what we really mean is that a policy, ∏ , is a continuous set of probability distributions over the entire set of states ( S ). And an optimal policy is that policy that maximizes my rewards.
Related Content
January 24, 2017
Annibel Rice, Adie Tomer
October 16, 2017
Darrell M. West
September 29, 2016
That function then becomes a value function, that is, a function of how an agent’s action at its initial belief state ( b0 ), populates its expected reward return once it receives feedback and updates its beliefs about various states and observations, and thus it improves on its policy. Then it continues this pattern, again and again and again, until it can begin to better predict what acts will maximize its reward. Ostensibly, this structure permits a system to learn how to act in a world that is uncertain, noisy, messy, and not fully observable. The role of the engineer is to define the task or objective in such a way that the artificial agent following a POMDP model can take a series of actions and learn which ones correspond with the correct observations about its state of the world and act accordingly, despite uncertainty.
The AV world will undoubtedly make greater use of POMDPs in their software architectures. While there is a myriad of computational techniques to choose from, probabilistic ones like POMDPs have proven themselves amongst the leading candidates to build and field autonomous robots. As Thrun (2000) explains, “the probabilistic approach […] is the idea of representing information through probability densities” around the areas of perception and control, and “probabilistic robotics has led to fielded systems with unprecedented levels of autonomy and robustness.” For instance, recently Cunningham et al. used POMDPs to create a multi-policy decision-making process for autonomous driving that estimated when to pass a slower vehicle as well as how to merge into traffic taking into account driving preferences such as reaching goals quickly and rider comfort (Cunningham et al. 2015).
It is well known, however, that POMDPs are computationally inefficient and that as the complexity of a problem increases, some problems may be intractable. To account for this, most researchers make certain assumptions about the world and the mathematics to make the problems computable, or they use heuristic approximations to ease policy searches (Hausrecht 2000). Yet when one approximates, in any sense, there is no guarantee that a system will act in a precisely optimal manner. However we manipulate the mathematics, we pay a cost in one domain or another: either computationally or for the pursuit of the best outcomes.
There is no guarantee that something completely novel and unforeseen will not confuse an AV or cause it to act in unforeseeable ways.
The important thing to note in all of this discussion is that any AV that is running on a probabilistic method like a set or architecture of POMDPs is going to be doing two things. First, it is not making “decisions” with complete knowledge, such as in the Trolley case. Rather, it is choosing a probability of some act changing a state of affairs. In essence, decisions are made in dynamic conditions of uncertainty. Second, this means that for AVs controlled by a learning system to operate effectively, substantial amounts of “episodes” or training in various environments under various similar and different situations are required for them to make “good” decisions when they are fielded on a road. That is, they need to be able to draw from a very rich history of interactions to extrapolate out from them in a forward-looking and predictive way. However, since they are learning systems, there is no guarantee that something completely novel and unforeseen will not confuse an AV or cause it to act in unforeseeable ways. In the case of the Trolley, we can immediately begin to see the differences between how a bystander may reason and how an AV does.
II. The follies of trolleys
Patrick Lin (2017) defends the use of the Trolley Problem as an “intuition pump” to get us to think about what sorts of principles we ought to be programming into AVs. He argues that using thought experiments like this “isolates and stress-tests a couple of assumptions about how driverless cars should handle unavoidable crashes, as rare as they might be. It teases out the questions of (1) whether numbers matter and (2) whether killing is worse than letting die.” Additionally, he notes that because driverless cars are the creations of humans over time, “programmers and designers of automated cars […] do have the time to get it right and therefore bear more responsibility for bad outcomes,” thereby bootstrapping some resolution to whether there was sufficient intentionality for the act to be judged as morally right or wrong.
While I agree with Lin’s assessment that many cases in philosophy are not designed for real-world scenarios, but to isolate and press upon our intuitions, this does not mean that they are well suited for all purposes. As Peter Singer notes, reducing “philosophy…to the level of solving the chess puzzle” is rather unhelpful, for “there are things that are more important” (Singer 2010). We need to take special care to see the asymmetries between cases like the Trolley Problem and algorithms that are not moral agents but make morally important decisions. The first and easiest way to see this is to acknowledge that an AV utilizing something like a POMDP in a dynamic environment is not making a decision at one point in time but is making sequential decisions. It is making a choice based on a set of probability distributions about what act will give it the highest reward function (or minimize the most cost) based upon prior knowledge, present observations and likely future states. Unlike the Trolley cases, where there is one decision to make at one point in time, this is not how autonomous cars actually operate.
We need to take special care to see the asymmetries between cases like the Trolley Problem and algorithms that are not moral agents but make morally important decisions.
Second, and more bluntly, we’d have to model trolley-like problems in a variety of situations, and train them in those situations (or episodes) hundreds, maybe thousands, of times to get the system to learn what to do in that instance. It would not just magically make the “right” decision in that instance because the math and the prior set of observations would not, in fact could not, permit it to do so. We actually have to pre-specify what “right” is for it to learn what to do. This is because the types of algorithms we use are optimizing by their nature. They want to find the most optimal strategy to maximize its reward function, and this learning, by the way, means that it needs to make many mistakes. 4 For instance, one set of researchers at Carnegie Mellon University refused to use simulations to teach an autonomous aerial vehicle to fly and navigate. Rather, they allowed it to crash, over 11,500 times, to learn simple self-supervised policies to navigate (Gandhi, Pinto and Gupta 2017). Indeed this learning-by-doing is exactly what much of the testing of self-driving cars in real road conditions is also directed towards: real life learning and not mere simulations. Yet we are not asking the cars to go crashing into people or to choose whether it is better to kill five men or five pregnant women. 5
Moreover, even if one decided to simulate these Trolley cases again and again, and diversify them to some sort of sufficient degree, we must acknowledge the simple but strict point that unless one already knows the right answer, the math cannot help. Also, I am hard pressed to find any philosophers who all agree on the one way of living and the correct moral code for over 2,000 years, or even to find agreement on what to do in the Trolley Problem. 6 What is even worse is that if we take the opinion that our intuitions ought to guide us in finding data for these moral dilemmas, we will not in fact, find reliable data. This can easily be seen with two simple examples to show how people do not in fact act consistently: the Allais Paradox and the Ellsberg Paradox. Both of these paradoxes challenge the basic axioms that Von Neumann and Morgenstern (1944) posited for their theory of expected utility. Expected utility theory basically states that people will choose an outcome based on whether that outcome’s expected utility is higher than all other potential outcomes. In short, it means people are utility maximizers. 7 In the Allais Paradox, we find that in a given experiment people fail to actually act consistently to maximize their utility (or achieve preference satisfaction) and thus they violate the substitution axiom of the theory. (Allais 1953). In the Ellsberg Paradox, people end up choosing when they cannot actually infer the probabilities that will maximize their preferences; thus violating the axioms of completeness and monotonicity (Ellsberg 1961).
One may object here and claim that utilitarianism is not the only moral theory, and that we do not in fact want utility maximizing self-driving cars. We’d rather have cars that respect rights and lives, more akin to a virtue ethics or deontological approach to ethics. But if that is so, then we have done away with the need for Trolley Problems at the outset. It is impermissible to kill anyone if that is true, despite the numbers. Or we merely state a priori that lesser evil justifications win the day, and thus in extremis we have averted the problem (Kamm 2007; Frowe 2015). Or, if we grant that self-driving cars, relying on a mathematical descendent from classic act utilitarianism, end up calculating as an act utilitarian would, then there appears to be no problem–the numbers win the day. Wait, wait, one responds, this is all too quick. Clearly we feel that self-driving cars ought not to kill anyone, the Trolley Problem stands, and they still might find themselves in situations where they have no choice but to kill someone, so who ought it to be?
Here again, I cite that we are stuck in an uncomfortable position vis a vis the need for data and training versus the need to know what morality dictates for us as true: do we want to model moral dilemmas or do we want to solve them? If it is the former, we can do this indefinitely. We can model moral dilemmas and ask people to partake in experiments, but that only tells us the empirical reality of what those people think. And that may be a significantly different answer than what morality dictates one ought do. If it is the latter, I am still extremely skeptical that this is the right framework to be discussing the ethical quandaries that arise with self-driving cars. Perhaps the Trolley Problem is nothing more than an unsolvable distraction from the question of safety thresholds and other types of ethical questions regarding the second or third order effects of automotive automation in society. 8
Indeed, if I am correct, then the entire set up of a moral dilemma for a non-moral agent to “choose” the best action is a false choice because there is no one choice that any engineer could foreseeably plan for. What is more, even if the engineer were to exhibit enough foresight and build a learning system that could pick up on subtle clues from interactions with the environment and mass amounts of data, this assumes that we have actually figured out which action is the right one to take! We’ve classified data as “good” or “bad” and fed that to a system. Yet we haven’t as human moral agents decided this at all, for there is debate in each situation about what one ought to do, as well as uncertainty . Trolley Problems are constructed in such a way where the agent has one choice, and knows with certainty what will happen should she make that choice. Moreover, that choice is constructed as a dilemma: it appears that no matter what choice she makes, she will end up doing some form of wrong. Under real-world driving conditions, this will rarely in fact be the case. And if we attempt to find a solution through the available technological means, all we have done is to show a huge amount of data to the system and have it optimize its behavior for the task it has been assigned to complete. If viewed in this way, modeling moral dilemmas as tasks and optimization appears morally repugnant.
More importantly for our purposes here, we must be explicitly clear that AI is not human. Even if an AI were a moral agent (and we agreed on what that looked like), the anthropomorphism presumed in the AV Trolley case is actually blinding us to some of the real dangers. For in classic moral philosophy Trolley cases, we assume from the outset that there is: (i) a moral agent confronted with the choice; (ii) that this moral agent is self-aware with a history of acting in the world, understands concepts, and possesses enough intelligence to contextually identify when trifle constraints are trumped by significant moral ones and (iii) the intelligence can, in some sense, balance or measure seemingly (or truly) incommensurable goods and conflicting obligations. Moreover, as Barbara Fried (2012) summarizes about the structure of Trolley Problems:
The hypotheticals typically share a number of features beyond the basic dilemma of third party harm/harm tradeoffs. These include the consequences of the available choices are stipulated to be known with certainty ex ante; that the actors are all individuals (as opposed to institutions); that the would-be victims (of the harm we impose by our actions or allow to occur by our inaction) are generally identifiable individuals in close proximity to the would-be actor(s); and that the causal chain between act and harm is fairly direct and apparent. In addition, actors usually face a one-off decision about how to act. That is to say, readers are typically not invited to consider the consequences of scaling up the moral principle by which the immediate dilemma is resolved to a large number of (or large-number) cases.
Yet not only are all the attributes noted above well beyond the present day capabilities of any AI systems, the situation in which an AV operates fails to comport with any and all of the assumptions in trolley-like cases (Roff 2017). There is a disjuncture between saying that humans will “program” the AV to make the “correct” moral choice, thereby bootstrapping the Trolley case to AVs, and between claiming that an AV is a learning automata that is sufficiently capable to make morally important decisions on the order of the Trolley problem. Moreover, we cannot just throw up our hands and ask what the best consequences will render, for in that case there is no real “problem” at issue: if one is a consequentialist, save the five over the one, no questions asked.
It is unhelpful to continue to focus and to insist that the Trolley Problem exhausts the moral landscape for ethical questions with regard to AVs and their deployment.
It is unhelpful to continue to focus and to insist that the Trolley Problem exhausts the moral landscape for ethical questions with regard to AVs and their deployment. All AIs can do is to bring into relief existing tensions in our everyday lives that we tend to assume away. This may be due to our human limitations in seeing underlying structures and social systems because we cannot take in such large amounts of data. AI, however, is able to find novel patterns in large data and plan based on that data. This data may reflect our biases, or it may simply be an aggregation of whatever situations the AV has encountered. The only thing AVs require of humans is to make explicit what tasks we require of it and what the rewards and objectives are; we do not require the AV to tell us that these are our goals, rewards and objectives. Unfortunately, this distinction is not something that is often made explicit.
Rather, the debate often oscillates between whether the human agents ought to “program” the right answer, or whether the learning system can in fact make the morally correct answer. If it is the former, we must admit it ignores the fact that a learning system does not operate in such straightforward terms. It is a learning system that will be constrained by its sensors, its experience, and the various architectures and sub-architectures of its system. But it will be acting in real time, away from its developers, and in a wide and dynamic environment, and so the human(s) responsible for its behavior will have, at best, mediated and distanced (if any) responsibility for that system’s behaviors (Matthais 2004).
If it is the latter, I have attempted to show here that the learning AV does not possess the types of qualities relevant for the aberrant Trolley case. Even if we were to train it on large amounts and variants of the Trolley case, there will always be situations that can arise that may not produce the estimated or intended decision by the human. This is simple mathematics. For one can only make generalizations about behaviors—particularly messy human behaviors that may give rise to AV Trolley like cases—when there is a significantly large dataset (we call this the law of large numbers). Unfortunately, this means that there is no way to know what one individual data point (or driver) will do in any given circumstance. And the Trolley case is always the unusual individual outlier. So, while there is value to be found in thinking through the moral questions related to Trolley-like cases, there are also limits to it as well, particularly with regard to decision weights, policies and moral uncertainty.
III. The value functions
If we agree that the Trolley Problem offers little guidance on the wider social issues at hand, particularly the value of a massive change and scientific research, then we can begin to acknowledge the wide-ranging issues that society will face with autonomous cars. As Kate Crawford and Ryan Calo (2016) explain, “autonomous systems are [already] changing workplaces, streets and schools. We need to ensure that those changes are beneficial, before they are built further into the infrastructure of everyday life.” In short, we need to identify the values that we want to actualize through the engineering, design and deployment of technologies, like self-driving cars. There is thus a double entendre at work here: we know that the software running these systems will be trying to maximize their value functions, but we also need to ensure that they are maximizing society’s too.
We know that the software running these systems will be trying to maximize their value functions, but we also need to ensure that they are maximizing society’s too.
So what are the values that we want to maximize with autonomous cars? Most obviously, we want cars to be better drivers than people. With over 5.5 million crashes per year and over 30,000 deaths in the U.S. alone, safety appears to be the primary motivation for automating driving. Over 40 percent of fatal crashes involve “some combination of alcohol, distraction, drug involvement and/or fatigue” (Fagnant and Kockelman 2015). That means that if everyone were using self-driving vehicles, at least in the U.S., there could be at least a 12,000 reduction in fatalities per year. Ostensibly, saving life is a paramount value. 9
But exactly how this occurs, as well as the attendant effects of policies, infrastructure choices, and technological development are all value loaded endeavors. There is not simply an innovative technological “fix” here. We cannot “encode” ethics and wash our hands of it. The innovation, rather, needs to come from the intersection of humanities, social sciences, and policy, working alongside engineering. This is because the values that we want to uphold must first be identified, contested, and understood. Richard Feynman famously said, “I cannot create that which I do not understand.” Meaning, we cannot create, or perhaps better, recreate those things of which we are ignorant.
Indeed, I would go so far as to push Crawford and Calo in their use of the word “infrastructure” and suggest that it is in fact the normative infrastructure that is of greatest importance. Normative here has two meanings that we ought to keep in mind: (i) the philosophical or moral “ought;” and (ii) the Foucauldian “normalization” approach that identifies norms as those concepts or values that seek to control and judge our behavior (Foucault 1975). These are two very different notions of “normative,” but both are crucially important for the identification of value and the creation of value functions for autonomous technologies.
From the moral perspective, one must be able to identify all those moral values that ought to be operationalized in not merely the autonomous vehicle system , but the adjudication methods that one will use when these values come into conflict. This is not, some might think, a return to the Trolley Problem. Rather, it is a technological value choice on how one decides to design a system to select a course of action. In multi-objective learning systems, there often occur situations where objectives (that is tasks or behaviors to accomplish) conflict with one another, are correlated, or even endogenous. The engineer must design a way of finding a way of prioritizing particular objectives or creating a system for tradeoffs, such as whether to conserve energy or to maintain comfort (Moffaert and Nowé 2014). 10 How they do so is a matter of mathematics, but it is also a choice about whether they are privileging particular kinds of mathematics that in turn privilege particular kinds of behaviors (such as satisficing).
Additionally, shifting focus away from tragic and rare events like Trolley cases, allows us to open up more systemic and “garden variety” problems that we need to consider for reducing harm and ensuring safety. As Allen Wood (2011) argues, most people would never have to face a Trolley case if there were safer trollies, the inability for passersby to have access to switches and good signage to “prevent anyone from being in places where they might be killed or injured by a runaway train or trolley.” In short, we need to think about use, design, and interaction for the daily experience for consumers, users, or bystanders of the technology. We must understand how AVs could change the design, layout and make-up of cities and towns, and what effects those may have on everything from access to basic resources to increasing forms of inequality.
From the Foucauldian perspective, things become slightly more interesting, and this is where I think many of the ethical concerns begin to come into view. The norms that govern how we act, the assumptions we make about the appropriateness of the actions or behaviors of others, and the value that we assign to those judgments is here a matter of empirical assessment (Foucault 1971; Foucault 1975). For instance, public opinion surveys are conduits telling us what people “think” about something. Less obvious, however, are the ways in which we subtly adjust our behavior without speaking or, in some instances, even thinking from cultural and societal cues. These are the kinds of norms Foucault is concerned about. These kinds of norms are the ones that show up in large datasets, in biases, in “patterns of life.” And it is these kinds of norms, which are the hardest ones to identify, that are the stickiest ones to change.
How this matters for autonomous vehicles lays in the assumptions that engineers make about human behavior, human values, or even what “appropriate” looks like. From a value-sensitive design (VSD) standpoint, one may consider not only the question of lethal harm to passengers or bystanders, but a myriad of values like privacy, security, trust, civil and political rights, emotional well-being, environmental sustainability, beauty, social capital, fairness, and democratic value. For VSD seeks to encapsulate not only the conceptual aspects of the values a particular technology will bring (or affect), but also how “technological properties and underlying mechanisms support or hinder human values” (Friedman, Kahn, Borning 2001).
But one will note that in all of these choices, Trolley Problems have no place. For instance, many of the social and ethical implications of AVs can be extremely subtle or simply obvious. Note the idea recently circulated by the firm Deloitte: AVs will impact retail and goods delivery services (Deloitte 2017). As it argues, retailers will attempt to use AVs to increase catchment areas, provide higher levels of customer service by sending cars to customers, cut down on delivery time, or act as “neighborhood centers” akin to a mobile corner store that delivers goods to one’s home. In essence, retailers can better cater to their customers and “nondrivers are not ‘forced’ to take the bus, subway, train or bike anymore […] and this will impact footfall and therefore (convenience) stores” (Deloitte 2017).
Yet this foreseen benefit from AVs may only apply to already affluent individuals living in reasonable proximity to affluent retail outlets. It certainly will struggle to find economic incentives in “food deserts” where low-income individuals without access to transport live at increasingly difficult distances from supermarkets or grocery stores (Economic Research Service U.S. Department of Agriculture 2017). Given that these individuals currently do not possess transport and suffer from lack of access to fresh foods and vegetables does not bode well for their ability to afford to pay prices for automation and delivery, or perhaps increased prices for the luxury of being ferried to and fro. This may in effect have more deleterious effects on poverty and the widening gap between the rich and poor, increasing rather than decreasing the areas now considered as “food deserts.”
To be sure, there is much speculation about how AVs will actually provide net benefits for society.
To be sure, there is much speculation about how AVs will actually provide net benefits for society. Many reports, from a variety of perspectives, estimate that AVs will ensure that all the parking garages are turned into beautiful parks and garden spaces (Marshall 2017), and the elderly, disabled and vulnerable have access to safe and reliable transport (Madia 2017, Anderson 2014, West 2016, Bertoncello and Wee 2015, UK House of Lords 2017). But less attention appears to be paid to how the present limitations of the technology will require substantial reformulation to urban planning, infrastructure, and the lives and communities of those around (and absent from) AVs. Hyper-loops for AVs, for example, may require pedestrian overpasses, or, as one researcher suggests, “electric fences,” to keep pedestrians from crossing at the street level (Scheider 2017). Others suggest that increased adoption of AVs will need to be cost and environmentally beneficial, so they will need to be communal and operated in larger ride shares (Small 2017). If this is so, then questions about the presence of surveillance and intervention for potential crimes, harassment, or other offensive behavior would seem to arise.
All of these seemingly small side or indirect effects of AVs will normalize usage, engender rules of behavior, systems of power, and place particular values over others. In the Foucauldian sense, the adoption and deployment of the AV will begin to change the organization and construction of “collective infrastructure” and this will require some form of governmental rationality—an imposition of structures of power—on society (Foucault 1982). For this sort of urban planning, merely to permit the greater adoption of AVs is a political choice; it will enable a “certain allocation of people in space, a canalization of their circulation, as well as the coding of their reciprocal relations” (Foucault 1982). Thus making these types of decisions transparent and apparent to the designers and engineers of AVs will help them to see the assumptions that they make about the world and what they and others value in it.
Ethics is all around us because it is a practical activity for human behavior. From all of the decisions that humans make, from the mundane to the morally important, there are values that affect and shape our common world. If viewed from this perspective, humans are constantly engaging in a sequential decision-making problem, trading off values all the time—not making one-off decisions intermittently. As I have tried to argue here, thinking about ethics in this one-shot problem, extremely tragic case scenario, is unhelpful at best. It distracts us from identifying the real safety problems and value tradeoffs we need to be considering with the adoption of new technologies throughout society.
In the case of autonomous vehicles, we ought to consider how the technology actually works, how the machine actually makes decisions. Once we do this, we see that the application of Trolleyology to this problem is not only a distraction, it is a fallacy.
In the case of AVs, we ought to consider how the technology actually works, how the machine actually makes decisions. Once we do this, we see that the application of Trolleyology to this problem is not only a distraction, it is a fallacy. We aren’t looking at the tradeoffs correctly, for we have multiple competing values that may be incommensurable. It is not whether a car ought to kill one to save five, but how the introduction of the technology will shape and change the rights, lives, and benefits of all those around it. Thus, the set-up of a Trolley Problem for AVs ought to be considered a red herring for anyone considering the ethical implications of autonomous vehicles, or even AI generally, because the aggregation of goods/harms in Trolley cases doesn’t travel to the real world in that way. They fail to scale, they are incommensurate, they are ridden with uncertainty and causality is fairly tricky when we want to consider second- and third-order effects. Thus, if we want to get serious about ethics and AVs, we need to flip the switch on this case.
Acknowledgements
I owe special thanks to Patrick Lin, Adam Henschke, Ryan Jenkins, Kate Crawford, Ryan Calo, Sean Legassick, Iason Gabriel and Raia Hadsel. Thank you all for your keen minds, insights, and feedback.
Reference List
Allais, Par M. (1953). “Le Comportement de L’Homme Rationnel Devant Le Risque: Critique Des Postulats et Axiomes De L’Ecole Americaine” Econometrica , 21(4): 503-546.
Anderson, James M. (2014) “Self-Driving Vehicles Offer Potential Benefits, Policy Challenges for Lawmakers” Rand Corporation , Santa Montica, CA. Available online at: https://www.rand.org/news/press/2014/01/06.html. Accessed 17 January 2018.
Bertoncello, Michele. and Dominik Wee. (2015) “Ten Ways Autonomous Driving Could Redefine the Automotive World” McKinsey & Company, June. Available online at: https://www.mckinsey.com/industries/automotive-and-assembly/our-insights/ten-ways-autonomous-driving-could-redefine-the-automotive-world. Accessed 15 January 2018.
Crawford, Kate and Ryan Calo. (2016). “There is a Blind Spot in AI Research” Nature , Vol. 538, October. Available online at: https://www.nature.com/polopoly_fs/1.20805!/menu/main/topColumns/topLeftColumn/pdf/538311a.pdf. Accessed 15 January 2018.
Cunningham, Alexander G. Enric Galceran, Ryan Eustice, Edwin Olson. (2015). “MPDM: Mulitpolicy Decision-Making in Dynamic, Uncertain Environments for Autonomous Driving” Robotics and Automation (ICRA) IEEE International Conference on Robotics and Automation, Seattle, USA.
Economic Research Service (ERS), U.S. Department of Agriculture (USDA). (2017). Food Access Research Atlas , Available online at: https://www.ers.usda.gov/data-products/food-access-research-atlas/. Accessed 17 January 2018.
Ellsberg, Daniel. (1961). “Risk, Ambiguity, and the Savage Axioms” The Quarterly Journal of Economics , 75(4): 643-669.
Fagnant, Daniel J. and Kara Kockelman. (2015). “Preparing a Nation for Autonomous Vehicles: Opportunities, Barriers and Policy Recommendations.” Transportation Research Part A , Vol. 77: 167-181.
Foot, Philippa. (1967). “The Problem of Abortion and the Doctrine of Double Effect” Oxford Review, Vol, 5: 5-15.
Foucault, Michel. (1971). The Archeology of Knowledge and the Discourse of Language , (Trns.) A. M. Sheridan Smith, (1972 edition), New York, NY: Pantheon Books.
——. (1975). Discipline & Punish: The Birth of the Prison (1977 edition), New York, NY: Vintage Books.
——. (1982). “Interview with Michel Foucault on ‘Space, Knowledge, and Power’ from Skyline in (Ed.) Paul Rabinow, The Foucault Reader (1984), New York, NY: Pantheon Books.
Fried, Barbara. (2012). “What Does Matter? The Case for Killing the Trolley Problem (Or Letting it Die), The Philosophical Quarterly , 62(248): 506.
Frowe, Helen. (2015). “Claim Rights, Duties, and Lesser Evil Justifications” The Aristotelian Society , 89(1): 267-285.
Gandhi, Dhiraj. Lerrel Pinto and Abhinav Gupta. (2017). “Learning to Fly by Crashing” Available online at: https://arxiv.org/pdf/1704.05588.pdf. Accessed 18 January 2018.
Goldman, A.I. (1979). What is Justified Belief?. In: (Eds). Pappas G.S. Justification and Knowledge . Philosophical Studies Series in Philosophy, vol 17. Springer, Dordrecht.
Hausrecht, Milos. (2000) “Value-Function Approximations for Partially Observable Markov Decision Processes” Journal of Artificial Intelligence Research , Vol. 13: 33-94.
Kagan, Shelly. (1989). The Limits of Morality, Oxford: Oxford University Press.
Kamm, Francis. (1989). “Harming Some to Save Others” Philosophical Studies , Vol. 57: 227-260.
——. (2007). Intricate Ethics: Rights, Responsibilities, and Permissible Harm, Oxford: Oxford University Press.
Lin, Patrick. (2017). “Robot Cars and Fake Ethical Dilemmas” Forbes Magazine , 3, April. Available online at: https://www.forbes.com/sites/patricklin/2017/04/03/robot-cars-and-fake-ethical-dilemmas/#3bdf4f2413a2. Accessed 12 January 2018.
Madia, Eric. (2017). “How Autonomous Cars and Buses Will Change Urban Planning (Industry Perspective) Future Structure , 24 May. Available online at: http://www.govtech.com/fs/perspectives/how-autonomous-cars-buses-will-change-urban-planning-industry-perspective.html. Accessed 16 January 2018.
Marshall, Aarian. (2017). “How to Design Streets for Humans—and Self-Driving Cars” Wired Magazine , 30 October. Available online at: https://www.wired.com/story/nacto-streets-self-driving-cars/. Accessed 22 November 2017.
Otsuka, Michael. (2008). “Double Effect, Triple Effect and the Trolley Problem: Squaring the Circle in Looping Cases” Utilitias , 20(1): 92-110.
Parfit, Darek. (2011). On What Matters , Vol. 1 and 2. Oxford: Oxford University Press.
Puterman, Martin L. (2005). Markov Decision Processes: Discrete Stochastic Dynamic Programming , London: John Wiley & Sons.
Roff, Heather M. (2017). “How Understanding Animals Can Help Us to Make the Most out of Artificial Intelligence” The Conversation , 30 March. Available online at: https://theconversation.com/how-understanding-animals-can-help-us-make-the-most-of-artificial-intelligence-74742. Accessed 12 December 2017.
Science and Technology Select Committee, United Kingdom House of Lords (2016-2017). “Connected and Autonomous Vehicles: The Future?” Government of the United Kingdom. Available online at: https://publications.parliament.uk/pa/ld201617/ldselect/ldsctech/115/115.pdf. Accessed 15 January 2018.
Singer, Peter. (2010). Interview in Philosophy Bites , (Eds.) Edmonds, D. and N. Warburton, Oxford: Oxford University Press.
Small, Andrew. (2017). “The Self-Driving Dilemma” CityLab Available online at: https://www.citylab.com/transportation/2017/05/the-self-driving-dilemma/525171/. Accessed 15 January 2018.
Spaan, Matthijs. (2012). “Partially Observable Markov Decision Processes” in (Eds). M.A. Wiering and M. van Otterlo, Reinforcement Learning: State of the Art , London: Springer Verlag.
Sutton, Richard and Andrew Barto. (1998). Reinforcement Learning: An Introduction , Cambridge: MIT Press. Thompson, Judith Jarvis (1976). “Killing, Letting Die and the Trolley Problem” The Monist , 59(2): 204-217.
——. (1985). “The Trolley Problem” The Yale Law Journal , 95(6): 1395-1415. Thrun, Sebastian. (2000). “Probabilistic Algorithms in Robotics,” AI Magazine , 21(4).
Tuinder, Marike. (2017). “The Impact on Retail” Deloitte.
Unger, Peter. (1996). Living High and Letting Die , Oxford: Oxford University Press.
Van Moffaert, Kristof., Ann Nowé. (2014). “Multi-Objective Reinforcement Learning Using Sets of Pareto Dominating Policies” Journal of Machine Learning Research , Vol. 15: 3663-3692.
Von Neumann, John and Oskar Morgenstern. (1944). Theory of Games and Economic Behavior , Princeton, NJ: Princeton University Press.
West, Darrell M. (2016). “Securing the Future of Driverless Cars” Brookings Institution, 29 September Available online at: https://www.brookings.edu/research/securing-the-future-of-driverless-cars/. Accessed 15 January 2018.
Wood, Allen. (2011). “Humanity as an End in Itself” in (Ed.) Darek Parfit, On What Matters , Vol. 2, Oxford: Oxford University Press.
- There are of course many machine learning techniques one could use, and for the sake of brevity I am simplifying various artificial intelligence techniques, like deep learning, and other technical details to focus on a classic case of the POMDP, in no way is this meant to be a panacea.
- There are techniques for learning memoryless policies. However, it is uncertain how well these would scale, particularly in the AV case. Of course, the word belief is anthropomorphic in this sense, but the system will have a memory of past actions and some estimation of likely future outcomes. We can liken this to Goldman’s explanation of a “belief-forming-process” (Goldman 1979).
- We might also just want to call belief the relevant information in the state the agent finds itself in, so the belief is the state/action pair with the memory of prior state/action pairs.
- One could object and say that the model could be specified in an expert However, this approach would be very brittle to novel circumstances and does not get the benefit of adaptive learning and experimentation. I thank Mykel Kochenderfer for pressing me on this point.
- Moral Machine is a project out of Massachusetts Institute of Technology that aims to take discussion of ethics and self-driving cars further “by providing a platform for 1) building a crowd-sourced picture of human opinion on how machines should make decisions when faced with moral dilemmas, and 2) crowd-sourcing assembly and discussion of potential scenarios of moral consequence.” Unfortunately, the entire premise for this, as I am arguing here, is faulty. Cf:. http://moralmachine.mit.edu.
- While we have some experimental philosophy issuing surveys to reveal what people’s preferences would be in a Trolley case, while there is a majority of agreement for one to throw the switch, this does not mean that the opinion on this matter is the morally correct action to take. The very limited case sampled (the classic kill one to save the five), is contrived. Thus even if we were to model a variety of such cases, it is highly unlikely that an AV would encounter any one of them, let alone provide moral cover for the action taken. Cf: https://philpapers.org/surveys/results.pl.
- Of course, one could also object that decision theory is normative and not descriptive. But this objection seems misplaced, as if people thought it prescriptive there would not be so much work on the descriptive side of the equation, particularly in the fields of economics and political science.
- I thank Ryan Calo and Kate Crawford for pushing me on these points and the helpful.
- There are some who may argue that if someone has wronged another or poses a wrongful lethal threat to another, they have forfeited their right not to be lethally harmed in response. If that is so, then there appears to be something weightier than “life” at work in this balance, for one will certainly perish. Rather, what seems of greater value in this scenario is that of rights and justice.
- It also seems amusing that the acronym for their approach to solving this problem is a “MORL” (multi objective reinforcement learning) algorithm.
Artificial Intelligence
Governance Studies
Center for Technology Innovation
Artificial Intelligence and Emerging Technology Initiative
Tom Wheeler
April 9, 2024
April 4, 2024
Nicol Turner Lee
March 28, 2024
Advertisement
Self-Driving Vehicles—an Ethical Overview
- Research Article
- Open access
- Published: 12 August 2021
- Volume 34 , pages 1383–1408, ( 2021 )
Cite this article
You have full access to this open access article
- Sven Ove Hansson ORCID: orcid.org/0000-0003-0071-3919 1 ,
- Matts-Åke Belin 1 , 2 &
- Björn Lundgren ORCID: orcid.org/0000-0001-5830-3432 3 , 4 , 5
59k Accesses
30 Citations
32 Altmetric
Explore all metrics
The introduction of self-driving vehicles gives rise to a large number of ethical issues that go beyond the common, extremely narrow, focus on improbable dilemma-like scenarios. This article provides a broad overview of realistic ethical issues related to self-driving vehicles. Some of the major topics covered are as follows: Strong opinions for and against driverless cars may give rise to severe social and political conflicts. A low tolerance for accidents caused by driverless vehicles may delay the introduction of driverless systems that would substantially reduce the risks. Trade-offs will arise between safety and other requirement on the road traffic system. Over-reliance on the swift collision-avoiding reactions of self-driving vehicles can induce people to take dangerous actions, such as stepping out in front of a car, relying on its fast braking. Children travelling alone can violate safety instructions such as the use of seatbelts. Digital information about routes and destinations can be used to convey commercial and political messages to car users. If fast passage can be bought, then socio-economic segregation of road traffic may result. Terrorists and other criminals can hack into a vehicle and make it crash. They can also use self-driving vehicles for instance to carry bombs to their designed places of detonation or to wreak havoc on a country’s road system.
Similar content being viewed by others
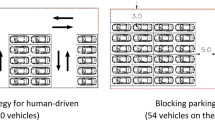
Exploring the implications of autonomous vehicles: a comprehensive review
Kareem Othman
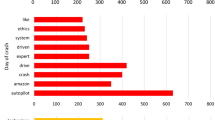
Public acceptance and perception of autonomous vehicles: a comprehensive review
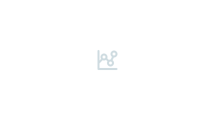
The Future of Transportation: Ethical, Legal, Social and Economic Impacts of Self-driving Vehicles in the Year 2025
Avoid common mistakes on your manuscript.
1 Introduction
Self-driving vehicles have been predicted to radically change our patterns of travelling and transportation (Gruel & Stanford, 2016 ; Pernestål & Kristoffersson, 2019 ). Their introduction will be a protracted process involving massive investments in vehicles and infrastructure, as well as changes in ingrained behaviours and attitudes. There will probably be a decades-long period of gradual introduction, in which fully automated operation of road vehicles will only be allowed in limited segments of the road system, such as specially designated highways or highway lanes, and small areas such as parking facilities where velocities will be kept low (Kyriakidis et al., 2019 ).
This will be a momentous technological transformation. It calls for major efforts to anticipate and evaluate social changes that may potentially accompany the introduction of the new technology. As part of these endeavours, ethical and public policy aspects of the technology itself and of various scenarios for its introduction need to be explored (Palm & Hansson, 2006 ). This article presents an overview of plausible challenges and opportunities that can potentially result from the introduction of self-driving (driverless, autonomous) road vehicles. Our purpose is to broaden the discussion from a focus on the crash behaviour of vehicles to the many types of social change that the new technology can be involved in. We have studied the ethical literature on the topic, and reflected on the social and ethical implications of topics brought up in the technical and policy-oriented literature. This search resulted in a fairly extensive (but of necessity not exhaustive) list of issues, many of which do not seem to have been discussed previously in the ethical literature. Footnote 1 In what follows we begin by discussing the changes in responsibility ascriptions that can be expected (“Sect. 2 ”), since such changes will determine much of the ethical framework for the new technology. After that we discuss potential positive and negative reactions to automated vehicles (“Sect. 3 ”) and the trade-offs between safety and other requirements on a new road traffic system (“Sect. 4 ”). We then turn to the important ethical issues that arise from the possibility of external control of autonomous vehicles (“Sect. 5 ”) and from the large amounts of person-related data that will be collected in vehicles and road management systems (“Sect. 6 ”). This is followed by chapters on human health and the environment (“Sect. 7 ”), social and labour market relations (“Sect. 8 ”), and criminality (“Sect. 9 ”). Our conclusions are summarized in “Sect. 10 ”.
2 Responsibility for Safety
Much of the discussion on self-driving vehicles has been concerned with issues of responsibility. In the currently on-going tests on public roads, there is always a person on the driver’s seat, called a “safety driver” or “steward”, who is required to follow the traffic and be prepared to take over control immediately if the need arises. The safety driver has essentially the same legal responsibilities as the driver of a conventional vehicle. However, this is seen as a temporary solution, and the automobile industry aims at releasing the safety driver, so that all occupants of the vehicle can be passengers. Such a step would seem implausible unless and until automatic driving has achieved a markedly higher level of safety than human driving. Possibly, this will first be attained only in certain parts of the road system (e.g. motorways), and fully automatic driving may then initially be allowed only there.
If and when this happens, a radically new situation will arise with respect to responsibility. If there is no driver who controls the vehicle, who is then responsible for the safety of its passengers and of those who travel or walk on the same roads? If a car is “driven” by a computer possessing artificial intelligence, does that intelligence constitute an entity that can be held responsible? What are the responsibilities of the vehicle’s current owner? Its manufacturer? The owner and manager of the road system? The organization running the traffic control centre that the vehicle communicates with?
Even without automatic vehicles, traditional assumptions about responsibilities in road traffic have been subject to change in the last few decades. Traditionally, drivers and others moving on the roads have been taken to carry almost the whole burden of responsibility (Melcher et al., 2015 , p. 2868). Footnote 2 Vision Zero, which was introduced in Sweden 1997 and is now adopted in numerous countries, states, and cities around the world, aims at eliminating all fatalities and serious injuries in road traffic. It puts much more emphasis than previous approaches on the responsibilities of road builders and managers, vehicle manufacturers, and others who contribute to creating and maintaining the traffic system, or use it professionally (Belin et al., 2012 ; Rosencrantz et al., 2007 ). Future changes in responsibility ascriptions will have to be seen in that perspective.
In order to analyse the responsibility issues connected with automated road traffic, we need to distinguish between two fundamentally different types of responsibility, namely, task responsibility and blame responsibility (Dworkin, 1981 ; Goodin, 1987 ; Hansson, 2022 ). Having a task responsibility means to be obliged to do something. Having a blame responsibility means that one is to be blamed if something goes wrong. Blame responsibility is often associated with punishments or with duties to compensate. Blame responsibility is also often called “backwards-looking responsibility”, and task responsibility can be called “forwards-looking responsibility”.
These two major forms of responsibility coincide in many practical situations, but in particular in complex social situations, they can be born by different agents. For instance, suppose that a motorist who drives too fast kills a child crossing a road on its way to school. In the subsequent trial, the driver will be held (blame) responsible for the act. And of course the driver is (task) responsible for not driving dangerously again. But that is not enough. We also need to prevent the same type of accident from happening again, with other drivers. This is not something that the culpable driver can do. Instead, measures are needed in the traffic system. We may have reasons to introduce traffic lights, speed bumps, or perhaps a pedestrian underpass. The task responsibility for these measures falls to decision-makers, such as public authorities. In cases like this, blame and task responsibility part company.
What will happen with our responsibility ascriptions when driverless cars are introduced? One thing should be clear: since the users of fully automated vehicles have no control over the vehicle, other than their choice of a destination, it would be difficult to hold them responsible either for safety (task responsibility) or for accidents (blame responsibility) (Gurney, 2017 ). We do not usually hold people responsible for what they cannot control (King, 2014 ). Footnote 3 There are three major alternatives for what we can do instead. First, we can hold other persons responsible instead. The most obvious candidates are the vehicle manufacturers and the people responsible for the road system (including the communication and coordination systems used to guide the vehicles). The second option is to hold the artificial intelligence built into the vehicles responsible. The third is to treat traffic accidents in the same way as natural accidents such as tsunamis and strokes of lightning, for which no one is held responsible. In Matthias’ ( 2004 ) terminology, this would mean that there is a “responsibility gap” for these accidents. Several authors have warned that self-driving vehicles may come with a responsibility gap (Coeckelbergh, 2016 ; de Jong, 2020 ).
Although the future is always difficult to predict, the first option is by far the most probable one. Previous experience shows that this is how we usually react when a person to whom we assigned responsibility is replaced by an automatic system. For instance, if an aviation accident unfolds after the pilot turned on the autopilot, we do not blame the artificial intelligence that took over the flight, and neither do we treat the failure as a natural event. Instead, we will probably put blame on those who directed the construction, testing, installation, service, and updating of the artificial intelligence. Such an approach is not unknown in road traffic. In the past few decades, proponents of the Vision Zero approach to traffic safety have had some success in achieving an analogous transfer of responsibility to vehicle and road system providers, although human drivers are still in place.
It cannot be excluded that future, perhaps more human-like, artificial agents will be assigned blame or task responsibility in the same way as human agents (Nyholm, 2018a , pp. 1209–1210). However, in the foreseeable future, the systems running our vehicles do not seem to be plausible candidates for being so treated. These will be systems taking and executing orders given to them by humans. There does not seem to be any need for them to express emotions, make self-reflective observations, or exhibit other behaviours that could make us see them as our peers. Footnote 4 It should also be noted that current approaches to automatic driving are predominantly based on pre-programmed response patterns, with little or no scope for autonomous learning. This is typical for safety-critical software. The cases in which it appears to be difficult to assign responsibility for an artificial agent to its creator(s) are those that involve extensive machine learning, which means that the programmers who constructed the software have no chance of predicting its behaviour.
We should therefore assume that for driverless vehicles, the responsibilities now assigned to drivers will for the most part be transferred to the constructors and maintainers of the vehicles and the roads and communication systems on which they depend (Bonnefon et al., 2020 , pp. 53–63; Crane et al., 2017 ; Luetge, 2017 , p. 503; Marchant & Lindor, 2012 ). Footnote 5 This also seems to be what the automobile industry expects to happen (Atiyeh, 2015 ; Nyholm, 2018c ). It will have the interesting consequence that blame responsibility and task responsibility will be more closely aligned with each other since they are carried by the same organization (Nyholm & Smids, 2016 , p. 1284n). The responsibility of manufacturers can either be based on products liability or on some new legal principle, such as Gurney’s ( 2017 ) proposal that in liability cases, the manufacturers of autonomous vehicles should be treated as drivers of those vehicles. Abraham and Rabin ( 2019 ) suggested a new legal concept, “manufacturer enterprise responsibility” that would involve a strict liability compensation system for injuries attributable to autonomous vehicles. Some authors, notably Danaher ( 2016 ) and de Jong ( 2020 ), have put focus on the “retribution gap”, i.e. the lack of mechanisms to identify individual persons that are punishable for a crash caused by an autonomous vehicle. This part of the responsibility gap cannot be so easily filled by a corporate entity as the parts concerning compensation (another part of blame responsibility) of future improvements (task responsibility). However, finding someone to punish is not necessarily as important as compensating victims and reducing the risks of future crashes.
It is much less clear how responsibilities will be assigned in near-automated driving, in which a human in the driver’s seat is constantly prepared to take over control of the vehicle in the case of an emergency (Nyholm, 2018a , p. 1214). However, although this may be adequate for test driving, it is unclear whether the same system can be introduced on a mass scale. Human interventions will tend to be slow, probably often slower than if the human is driving, and such interventions may also worsen rather than improve the outcome of a dangerous situation (Hevelke & Nida-Rümelin, 2015 ; Sparrow & Howard, 2017 , pp. 207–208). It is highly doubtful whether such arrangements satisfy the requirement of “meaningful human control” that is frequently referred to in the AI literature (Mecacci & Santoni de Sio 2020 ). Since meaningful control is a standard criterion for both blame and task responsibility, it is therefore also doubtful whether either type of responsibility can be assigned to a person sitting in the driver’s seat under such conditions (Hevelke & Nida-Rümelin, 2015 ).
3 What Can and Should Be Accepted?
Although the automotive industry and public traffic administrations are planning for automatized road traffic, its introduction will, at least in democracies, ultimately depend on how public attitudes will develop. Some studies indicate that large parts of the population in most countries have a fairly positive attitude to autonomous vehicles (Kyriakidis et al., 2015 ). However, such studies should be interpreted with caution. Not many have any experience of self-driving vehicles, and no one has experience of their large-scale introduction into a traffic system. Furthermore, other studies indicate a less positive attitude (Edmonds, 2019 ).
Public attitudes to accidents involving autonomous vehicles will be important, perhaps decisive, for the introduction of such vehicles in regular traffic. Will we accept the same frequency of serious accidents with self-driving cars as that which is now tolerated for vehicles driven by humans? There are several reasons to believe that we will not. Already today, tolerance for safety-critical vehicle malfunctions is low. Manufacturers recall car models to repair faults with a comparatively low probability of causing an accident. They would probably encounter severe public relations problems if they did not. Previous attempts to limit such recalls to cases when they have a favourable cost–benefit profile have proved disastrous to the manufacturer’s public relations (Smith, 2017 ). The public tends to expect much lower failure rates in vehicle technology than in the behaviour of drivers (Liu et al., 2019 ). This difference is by no means irrational, since technological systems can be constructed to be much more predictable, and in that sense more reliable, than humans. Footnote 6
Another reason to put high demands on the safety features of driverless vehicles is that improvements in technology are much more generalizable than improvements in human behaviour. Suppose that a motorist drives over a child at dusk because of problems with his eyesight. This may be reason enough for him to change his way of driving, or to buy new eyeglasses. If his eyesight cannot be sufficiently improved, it is a reason for authorities to withdraw his driver’s licence. However, all these measures will only affect this particular driver. In contrast, if a similar accident occurs due to some problem with the information processing in an automatized vehicle, then improvements to avoid similar accidents in the future will apply (at least) to all new vehicles of the same type. The fact that a crash with a self-driving vehicles cannot be written off as an exception due to reckless behaviour may also contribute to higher demands on the safety of these vehicles.
In addition to these rational reasons for high safety requirements on driverless vehicles, public attitudes may be influenced by factors such as fear of novelties or a particular revulsion to being killed by a machine. There have already been cases of enraged opponents slashing tyres, throwing rocks, standing in front of a car to stop it, and pointing guns at travellers sitting in a self-driving car, largely due to safety concerns (Cuthbertson, 2018 ). At least one company has left its self-driving test vehicles unmarked in order to avoid sabotage (Connor, 2016 ).
All this can combine to heighten the safety requirements on self-driving vehicles. This was confirmed in a study indicating that self-driving vehicles would have to reduce current traffic fatalities by 75–80% in order to be tolerated by the public in China (Liu et al., 2019 ). Potentially, requirements of safety improvement may turn out to be so high that they delay the introduction of driverless systems even if these systems would in fact substantially reduce the risks. Such delays can be ethically quite problematic (Brooks, 2017a ; Hicks, 2018 , p. 67).
To the extent that future driverless vehicles satisfy such augmented safety requirements, the public’s tolerance of accidents with humanly driven vehicles may be affected. If a much lower accident rate is shown to be possible in automatized road traffic, then demands for safer driving can be expected to gain momentum. This can lead to measures that reduce the risks of conventional driving, such as alcohol interlocks, speed limiters, and advanced driver assistance technologies. Insurance will become more expensive for human-driven than self-driving cars if the former are involved in more accidents. There may also be proposals to exclude human-driven vehicles from parts of the road net, or even to prohibit them altogether. According to Sparrow and Howard ( 2017 , p. 206), when self-driving cars pose a smaller risk to other road-users than what conventional cars do, “then it should be illegal to drive them: at that point human drivers will be the moral equivalent of drunk robots” (Cf. Müller & Gogoll, 2020 ; Nyholm & Smids, 2020 ).
On the other hand, strong negative reactions to driverless cars can be expected to develop in segments of the population. In road traffic as we know it, drivers communicate with each other and with unprotected persons in various informal ways. Drivers show other drivers that they are leaving them space to change lanes, and pedestrians tend to wait for drivers to signal that they have seen them before stepping into the street. Similarly, drivers react to pedestrians showing that they wait for the vehicle to pass (Brooks, 2017a ; b ; Färber, 2015 , p. 143; Färber, 2016 , p. 140). Inability of automatic vehicles to take part, as senders or receivers, in such communications, may give rise to reactions against their presence in the streets. There may also be disapprovals of patterns of movement that differ from the driving styles of most human drivers, such as strictly following speed limits and other traffic laws, and accelerating and decelerating slowly in order to save energy (Nyholm & Smids, 2020 ; Prakken, 2017 ).
Furthermore, negative reactions can have their grounds in worries about the social and psychological effects of dependence on artificial intelligence, or about the uncertainties pertaining to risks of sabotage or large accidents due to a breakdown of the system. There are signs that significant reactions of this nature may arise. According to a study conducted by the American Automobile Association, three out of four Americans are afraid of riding a fully autonomous car (Edmonds, 2019 ). Such attitudes may be connected with other misgivings about a future, more technocentric society. Such reactions should not be underestimated. The experience of genetically modified crops in Europe shows that resistance to new technologies can delay their introduction several decades, despite extensive experience of safe use (Hansson, 2016 ).
Attitudes to automatized road traffic can also be influenced by devotion to the activity of driving. For some people, driving a motor vehicle is an important source of pride and self-fulfilment. The “right to drive a car” is important in their lives (Borenstein et al., 2019 , p. 392; Edensor, 2004 ; Moor, 2016 ). Notably, this does not necessarily involve negativity to mixed traffic, as long as one is allowed to drive oneself, and the “pleasure of driving” is not too much thwarted by the self-driving vehicles and the arrangements made for them. The “Human Driving Manifesto” that was published in 2018 argued explicitly for mixed traffic, claiming that “[t]he same technology that enables self-driving cars will allow humans to retain control within the safe confines of automation” (Roy, 2018 ). However, from an ethical (but perhaps not a political) point of view, the pleasures of driving would tend to be lightweight considerations in comparison with the avoidance of fatalities on the road.
All this adds up to prospects for severe social and political conflicts on the automatization of road traffic. Judging by previous introductions of contested technology, there is a clear risk that this can develop into a trench war between parties with impassioned and uncompromising positions. If driverless cars achieve a much better safety record than conventional vehicles—otherwise their introduction seems unlikely—then proponents will be invigorated by the safety statistics and will see little reason to make concessions that would be costly in terms of human lives. On the other hand, opponents motivated by abhorrence of a more technology dependent society cannot be expected to look for compromises. Dealing with the terms of such an entrenched clash of social ideals may well be the dominant issue of ethical involvement in road traffic automatization. Needless to say, rash and badly prepared introductions of self-driving vehicles could potentially trigger an escalation of such conflicts.
4 Safety and the Trade-Offs of Constructing a Traffic System
In the construction of a new traffic system, safety will be a major concern, and possibly the most discussed aspect in public deliberations. However, there will also be other specifications of what the traffic system should achieve. Just as in the existing traffic system, this will in practice often lead to trade-offs between safety and other objectives. Since safety is an ethical requirement, all such trade-offs have a considerable ethical component. In a new traffic system, they will have to be made with a considerably higher priority for safety than in the current system with its dreadful death toll.
Many of the more specific features of self-driving vehicles, such as short reaction time and abilities to communicate with other vehicles, can be used both to enhance safety and to increase speed. For instance, driving on city roads and other roads with unprotected travellers, such as pedestrians and cyclists, will always be subject to a speed–safety trade-off (Flipse & Puylaert, 2018 , p. 55). With sufficiently low speeds, fatal car–pedestrian collisions can virtually be eradicated. Probably, passengers of driverless vehicles would not tolerate such low speeds. They can also cause annoyance and possibly risky behaviour by the drivers of conventional vehicles. On the other hand, if the tolerance for fatal accidents becomes much lower for self-driving than for humanly driven vehicles (as discussed above), then demands for such low speeds can be expected. As noted by Goodall ( 2016 , pp. 815–816), since fast transportation in city areas is beneficial to many types of businesses, the speed–safety trade-off will be accompanied by an economy–safety trade-off connected with the efficiency of logistics.
Increased separation between pedestrians and motor vehicles can efficiently reduce accident risks. The introduction of inner city zones, similar to pedestrian zones but allowing for automatized vehicles driving at very low speeds and giving way to pedestrians, could possibly solve the safety problem and the need for transportation of goods. However, such zones may not be easily accepted by people who wish to reach city destinations with conventionally driven vehicles. This can lead to an accessibility–safety trade-off.
Self-driving vehicles can drive close to each other in a caravan, where the first vehicle sends out instructions to brake or accelerate, so that these operations are performed simultaneously by the whole row of vehicles. This technology (“platooning”) can significantly reduce congestion and thereby travel time. However, an efficient use of this mechanism will inevitably tend to reduce safety margins (Hasan et al., 2019 ; Hu et al., 2021 ). This will give rise to a speed–safety trade-off, but also to an economy–safety trade-off concerning infrastructure investments.
Even if accidents due to incoordination in fast-moving vehicle caravans will be very unusual, the effects can be enormous. This may place road traffic in a situation more similar to that of civil aviation, whose safety considerations are dominated by rare but potentially very large accidents (Lin, 2015 , p. 80; Lin, 2016 , p. 80). There may then be incentives to limit the number of vehicles in a caravan, and thereby the size of a maximal accident, although such a limitation may not decrease the expected total number of fatalities in these rare accidents. Discussions on such measures will involve a small–vs.–large–accidents trade-off.
Already in today’s traffic system there are large differences in safety between different cars. Important safety features are present in some car models but not in others. Some of these safety features, such as crumple zones, safety cells, and airbags, reduce the severity of the injuries affecting drivers and passengers (crashworthiness). Others, such as driver monitoring systems and anti-lock braking systems, reduce the probability of accidents (crash avoidance). Many of the crash avoidance features that are now installed on human-driven cars can be seen as forerunners of components that will be integrated into fully autonomous driving systems. The efficiency of the total crash avoidance system of self-driving cars will be crucial for the extent to which these vehicles can be introduced into road traffic. Like all other features, those affecting crash avoidance can be expected to differ between car models. New models will expectedly have better crash avoidance systems. Expensive car models may be equipped with better systems than less expensive ones; for instance, they may have better and more costly sensors (Holstein et al., 2018 ).
Currently, our tolerance is in practice fairly high for large differences in the risks that different vehicles expose other road users to, due to variations in equipment as well as in driver skills and behaviour. In many countries, a minimal technical safety level is ensured by compulsory periodic motor vehicle inspections, which include checks of brakes and other basic requirements. However, there are still large differences between vehicle types and models for instance in driver monitoring systems and anti-lock braking systems. In general, new cars have a higher standard than old cars in these respects. Recalls to update old cars to the technical safety standards of new cars are, to our knowledge, not practised anywhere. Footnote 7 Software updates in old vehicles may become a difficult issue, in particular for vehicles that outlive their manufacturing company (Smith, 2014 ). Today, most accidents are ascribed to human failures (Rolison et al., 2018 ). When the majority of crashes are ascribed to vehicle failures, prohibition of inferior vehicle types will be a much more obvious way to improve safety. Doing so will be good for safety, but achieving the higher safety level will be costly. To the extent that the higher costs for safety will prevent people with low incomes from owning motor vehicles, it can also involve an equity–safety trade-off.
The protection of passengers against accident risks will have to be implemented in a new situation in driverless cars. There may no longer be a person present in the vehicle who is responsible for the safety of all passengers. Presumably, this also means that there will no longer be a need for one sober person in the car. We can foresee trade-offs between, on the one hand, passengers’ free choice of activities and behaviour in the vehicle, and on the other hand, the measures required for their safety, in short freedom–safety trade-offs. A car or a bus can be occupied by a company of befuddled daredevils trying to bypass whatever features the vehicle has been equipped with to prevent dangerous behaviour such as leaning out of windows or throwing out objects. The introduction of mechanisms to detect and prevent dangerous behaviour, such as non-belted travel, can be conceived as privacy intrusive, and we then have a privacy–safety trade-off. It should be noted, however, that such mechanisms have an important function for minors travelling alone. Children may easily indulge in unsafe behaviour, such as travelling without a seat belt, and standard anti-paternalist arguments are not applicable to under-age persons. Vehicle-to-vehicle and vehicle-to-infrastructure communication can give rise to another privacy–safety trade-off; see “Sect. 6 ”.
Just like human drivers, self-driving vehicles can become involved in traffic situations where an accident cannot be avoided, and a fast reaction is needed in order to reduce its consequences as far as possible. A considerable number of ethics papers have been devoted to cases in which this reaction has to deal with an ethical dilemma, for instance between driving either into two elderly persons or one child. Footnote 8 Such dilemmas are virtually unheard of in the history of human driving. The reason for this is that the dilemmatic situations are extremely rare in practice. In order for such a situation to arise, two unexpected human obstacles will have to be perceived simultaneously and with about the same degree of certainty, so that the (human or artificial) agent’s first reaction will take both into account. Furthermore, there have to be two reasonably controlled options to choose between. As excellently explained by Davnall ( 2020 ), such situations are extremely rare. In almost all situations when a crash is imminent, the most important reaction is to decrease the car’s speed as much as possible in order to reduce its momentum. The choice is therefore between braking maximally without swerving and braking maximally and at the same time swerving. The latter option has severe disadvantages: swerving reduces the efficiency of braking, so that the collision will take place with a larger momentum. Swerving leads to loss of control, so that (in sharp contrast to the unrealistic examples in this literature) the car’s trajectory becomes unpredictable. This can lead to skidding, spinning, and a sideways collision that is not alleviated by the crumple zones at the car’s front. The chances for pedestrians and others to move out of harm’s way are also smaller if the car is spinning and skidding. In summary, the self-driving car “does not face a decision between hitting an object in front of it and hitting an object off to one side. Instead, the decision is better described as being between a controlled manoeuvre—one which can be proven with generality to result in the lowest impact speed of any available option—and a wildly uncontrolled one.” (Davnall, 2020 , pp. 442–443). Due to the physics of braking and crashing, the situation is very much the same for self-driving systems as it is for human drivers. Consequently, the need for including deliberations on this type of dilemmas does not seem to be larger in the programming of automatized vehicles than in driver’s education Footnote 9 (Brooks, 2017a ). Discussions of such dilemmatic situations seem to have been driven by theoretical considerations, rather than by attempts to identify the ethical problems arising in automated road traffic. Footnote 10 The ethical problems of crash avoidance, in particular the speed–safety trade-offs and the other trade-offs described above, will in all probability be much more important and should therefore be at the centre of the ethical discussion.
5 External Control of Driverless Vehicles
We typically think of an automated car as a vehicle following the directions of the human being who instructs it, both concerning the destination and the route. However, it will not be difficult to construct systems in which the decisions by individual drivers can be overridden by the traffic guidance system. In the case of a traffic jam on a particular road section, driverless vehicles can be redirected to uncongested roads. Such automatic redirection will be much more efficient than sending messages to the passengers who will then have to choose whether or not to follow the recommended new route. However, enforced redirection of a vehicle due to congestion may be conceived as an infringement on the freedom of its occupants. It is both possible and desirable to retain a personal choice for the road users in that case.
The ability of emergency service vehicles to reach their destination as quickly as possible is often a matter of life or death. In a fully automatized road traffic system, both the velocity of the blue light vehicles and the safety of other travellers can be substantially increased if all other vehicles on the pertinent roads are kept out of way through external control by the traffic guidance system. In addition, such external control of vehicles can be used for various law enforcement purposes, such as stopping a car at the roadside in order to arrest a traveller or to search for drugs, contraband or stolen goods. It has been predicted that such remote seizure can decrease the risk of deadly violence when a car is stopped by the police (Joh, 2019 , p. 309).
Arguably, this does not differ from what the police already have the authority to do. They can redirect traffic for purposes such as avoiding congestion, and they can stop a vehicle to arrest a driver or passenger or search for objects to be confiscated. If there is continuous electronic communication between the targeted vehicle(s) and a traffic guidance system, then it will be possible to inform the travellers of the reasons for the external interference and the expected consequences for their continued journey. This is a distinct advantage as compared to traditional police action on roads. Furthermore, taking control of a suspect’s vehicle and bringing it to the roadside is a much safer method than traditional high-speed pursuits. Car chases have a yearly death toll of about 100 per year in the USA alone. Between a fourth and half of those killed are innocent bystanders or road users (Hutson et al., 2007 ; Lyneham & Hewitt-Rau, 2013 ; Rice et al., 2015 ). From an ethical point of view, a reduction in these numbers is of course most desirable.
However, as the risks involved in stopping a vehicle become smaller, there may be moves to use the method for many more purposes than what traditional car chases are used for (namely, to capture persons trying to escape law enforcement). For instance, vehicles can be stopped in order to seize foreign nationals without a valid visa, persons suspected of having committed a minor misdemeanour, or a person whose travel destination indicates an intention to violate a restraining order (Holstein et al., 2018 ). The purposes for which law enforcement agencies can take over control of a vehicle, and the procedures for decisions to do so, will therefore have to be determined, based on a balance between the interests of law enforcement and other legitimate interests.
6 Information Handling
The potential advantages of self-driving vehicles can only be realized with well-developed communication systems. Vehicle-to-vehicle (inter-vehicle) communication can be used to avoid crashes and organize platooning. Vehicle-to-road-management communication systems can provide updated local information on traffic and accessibility. Both types of communication can complement the information gathered by the vehicle itself. Information about obstacles ahead can be obtained before they are registered by the car’s own sensors. Furthermore, sensor or sensor interpretation errors can be detected by comparison with information from other cars or from the roadside. If vehicle-to-road-management systems are interconnected on a large scale, then they can also be used for optimizing the traffic flow (van Wyk et al., 2020 ).
However, like all large-scale handling of person-related information, the collection and processing of traffic information can give rise to considerable privacy intrusions (Zimmer, 2005 ). Today, it is still largely possible to travel anonymously. A person who drives a private car does not necessarily leave any electronic traces, and the same applies to someone travelling by collective transportation (unless she pays with a payment card or a personal travel card) or by taxi (unless she pays with a payment card or the taxi has video surveillance).
All this will be different in an automatized traffic system. Self-driving vehicles will depend on geopositioning transponders operating in a highly standardized fashion (Borenstein et al., 2019 , p. 384), and possibly on centralized communication systems that keep track of each vehicle’s planned route and destination (Luetge, 2017 , p. 554). For privately owned cars, this information will be linkable to the owner. It can potentially be accessed by the road manager and by authorities. The situation will be similar for cars that are rented on a short-term or long-term basis. Just as today, companies renting out vehicles for personal use will register the identity of their customers. Furthermore, there will presumably be an incentive to install video surveillance systems in driverless vehicles—in particular buses—in order to deal with potential disturbances.
Geopositioning of persons can be highly sensitive. It can reveal memberships in religious or political organizations, as well as sensitive private relationships. For a member of a cult, a criminal or extreme political organization, disclosure of visits to an organization offering exit counselling can be life-threatening. The disclosure of travel destinations can be equally dangerous for a person who has obtained a new identity, for instance in a witness protection programme or a programme protecting women from harassment by ex-husbands. More generally, freedom to travel without being surveilled—by government, companies, or private persons—is arguably one of the values universally cherished in liberal societies (Sobel, 2014 ).
Geopositioning data can also potentially be used for commercial purposes. Currently, web browsing data on a person’s movements in the virtual space of the internet is used to tailor a massive flow of advertisements (Véliz, 2019 ; Vold & Whittlestone, 2019 ). With geopositioning data, our movements in real space can be used in the same way (Gillespie, 2016 ). Sellers and rental providers of vehicles will have economic incentives to include an advertisement function over which they retain control, so that they can sell space on it. For instance, after a car has been parked outside a timber yard, the owner or renter of the car would receive commercial messages from other construction stores. A (devotional or touristic) visit to a church or a mosque could be followed by messages from proselytizing organizations etc. Political ads could be individualized, based for instance on the combination of past travel and web surfing habits. These commercial messages could be conveyed via loudspeakers or screens in the car, or through other media connected with the person who owns or rents the vehicle. It is not inconceivable that such personalized commercials may become as ineluctable for travellers as the (personalized) commercials are today for the web surfer and the (impersonal) ads for the newspaper reader (King, 2011 ; Svarcas, 2012 ). Car manufacturers are already developing recommender systems that deliver commercial information based on the recipient’s previous behaviour. Such systems can be installed in both human-driven and self-driving cars (Vrščaj et al., 2020 ). In addition, ride-sharing can be tailored, based on personal information for instance from web browsing, which is used to find a suitable travel companion (Moor, 2016 ; Soteropoulos et al., 2019 , p. 46). However, we still have a (political) choice whether we want our real-world movements to be registered and used for such purposes.
A person going by a driverless car may have a destination that is less precise than a specific address, such as “a grocery” or “a place on the way to the final destination where I can buy some flowers”. Such destinations leave open for considerable commercial opportunities of the same types that are currently used on web browsers and social media. The car-traveller can then find herself driven, not to the closest grocery or flower shop, but to a store further away that has paid for being directed to. Travellers can also be offered to stop at places, for instance restaurants, for which they have not expressed any desire. There will be strong incentives for the sellers and renters of vehicles to display such services. But in this case as well, we still have an option to decide (politically) what types of messages our future travels should impose on us.
If the coordination between automatized vehicles is efficient, then the vast majority of accidents will probably result from collisions with cars driven by humans and with unprotected travellers such as pedestrians, cyclists, motorcyclists, and horseback riders. An obvious solution to this would be for non-autonomous vehicles, pedestrians etc. to carry a transponder that communicates with motor vehicles in order to avoid collisions (Morhart & Biebl, 2011 ). Parents may wish to provide their children with transponders in order to ensure their safety. It is not inconceivable that demands may arise to make transponders mandatory for certain types of vehicles (such as motorcycles), or for persons walking, cycling or horse-riding on particularly dangerous roads. Obviously, personal transponders would give rise to much the same privacy issues as vehicle-bound geopositioning.
7 Effects on Health and the Environment
To the extent that public transportation such as fixed route buses is replaced by self-driving vehicles that are called to the user’s location, there will no longer be a need to walk to and from a bus stop or a train or subway station. Such walks are an important part of the physical exercise performed by large parts of the population. Reducing the amount of exercise from an already suboptimal level can have negative health effects (Sallis et al., 2012 ). This may call for counter-measures, such as making residential areas car-free (Nieuwenhuijsen & Khreis, 2016 ).
The distribution between road traffic and other modes of traffic, in particular aviation and rail-bound traffic, may change due to the introduction of self-driving vehicles, but it is not possible to foresee what direction such changes will take. If road traffic replaces air-trips, then this will have positive environmental and climate effects. If it replaces rail traffic, then the effect may go in the opposite direction.
It seems plausible that self-driving vehicles will have better energy efficiency than vehicles driven by humans (Urmson & Whittaker, 2008 ). It has also been proposed that electric vehicles will be more attractive if they are self-driven so that they can “recharge themselves” when they are not needed (Brown et al., 2014 ). However, it is also plausible that the total mileage will increase (ibid.). The effects of automatized road traffic on the climate and the environment will also depend on several other factors, such as the distribution between privately owned and rentable vehicles (Zhang et al., 2018 ), and the extent of car- and ride-sharing (Fagnant & Kockelman, 2018 ). The introduction of a traffic management system that coordinates travel will make it easier than in the current system to arrange ride-sharing. However, if most of the vehicles continue to be privately owned (or long-time rented), then incentives to ride-sharing may be insufficient, and car travelling may continue to be as inefficient as today in terms of the number of passengers per vehicle. If traffic is mostly organized with cars hired for each occasion, similar to the current taxi system, then large-scale ride-sharing can more easily be organized and made economically attractive. Needless to say, the choice between these alternatives is a policy decision that need not be left to the market. The climate crisis provides strong reasons to support ride-sharing for instance with incentives in the transport fare system (Greenwald & Kornhauser, 2019 ). However, it is doubtful whether improved energy efficiency and increased car- and ride-sharing can outweigh the increased mileage that is expected to follow with the introduction of self-driving vehicles. At any rate, increased use of climate-friendlier modes of transportation, such as trains and bicycles, is necessary to achieve climate objectives.
A routing system for automatized traffic can be constructed to ensure that each vehicle reaches its destination as soon as possible. Alternatively, it can be tailored to achieve energy efficiency. This will mean lower velocities and fewer accelerations and decelerations, and therefore also increased travel time. Policy-makers will have to decide whether to leave this choice to the individual vehicle user (just as the same decision is left to individual drivers in the present system), or to regulate it in some way. Such a regulation can for instance impose a minimal priority to be assigned to energy conservation in all motor vehicles, or it can involve some form of taxation incurring additional costs on energy-inefficient transportation. Probably, platooning will be so energy-efficient that there will be strong reasons for policy-makers to consider the introduction of a unified speed on major highways (Brown et al., 2014 ).
Both road lighting and exterior automotive lighting can be substantially reduced in an automatized road traffic system (Sparrow & Howard, 2017 , p. 212). This will reduce energy consumption, and it will also lead to a reduction in light pollution (Stone et al., 2020 ). No large effects on the noise pollution emitted from each vehicle can be expected, since the noise level depends primarily on the energy source and the type of motor, rather than on whether the vehicle is automatized or conventionally driven. An increase in road traffic, which is a plausible consequence of automation, will lead to increased noise pollution.
8 Social and Labour Market Consequences
The introduction of self-driving vehicles will have important social consequences. Perhaps most obviously, people who cannot travel alone on roads today will be able to do so. Parents may wish to allow children to go alone by a driverless car. This can make it possible for children to visit relatives or friends, or take part in various activities, even when there is no grown-up available who has the time to accompany them (Harb et al., 2018 ). However, traffic situations can arise in which it is not safe for children to travel alone in a self-driving vehicle. Therefore, a regulation setting a minimal age for the oldest person travelling in a driverless vehicle may be required (Gasser, 2015 , pp. 571–572; Gasser, 2016 , pp. 548–549).
The effects for people with disabilities would seem to be more unequivocally positive. Costly adaptations of vehicles can to a large part be dispensed with. A considerable number of people who cannot drive a car will be able to go on their own in a self-driving car (Mladenovic & McPherson, 2016 , p. 1137). This will increase their mobility, and it can potentially have positive effects on their well-being and social connectedness.
On the negative side, an automatized road traffic system makes it possible to introduce new social divisions among travellers. We already have divisions between more and less affordable manners of travelling on-board the same vehicle. However, although those who travel first or business class on trains and airplanes have more legroom, and (on airplanes) receive more drinks and presumably better food, they leave and arrive at the same time. If there is a traffic delay, first class passengers are not sent off in a separate vehicle, leaving the second (or “tourist”) class passengers behind. A road management system will of course ensure the swift passage of emergency vehicles when other vehicles have to travel slowly, but will it also offer swift passage to those who can afford a “first” or “business” option for their travel? There will certainly be economic incentives to provide such services for those who can pay for them (Dietrich & Weisswange, 2019 ; Mladenovic & McPherson, 2016 ). The negative effects on social cohesion and solidarity of such a system should not be underestimated. Fortunately, the choice whether to allow such shortcuts for the prosperous is a political decision yet to be made.
Sensors currently in use tend to be less reliable in detecting dark-skinned than light-skinned pedestrians (Cuthbertson, 2019 ). This will expose dark-skinned pedestrians to higher risks than others. The probable cause of this defect is that too few dark-skinned faces have been included in the training sets used when the sensor software was developed. This is a problem that will urgently have to be eliminated.
New and more comfortable travel opportunities can give rise to changes in the relative attractiveness of different residential districts, possibly with areas further from city centres gaining in attractiveness (Heinrichs, 2015 , pp. 230–231; Heinrichs, 2016 , pp. 223–224; Soteropoulos et al., 2019 , p. 42). There may also be effects on the localization choices of firms, including shops and entertainment facilities. Changes in the use of urban space may have effects on social segregation, which are difficult to foresee but should be at the focus in urban planning.
As in other branches of industry, automatization of the traffic system will lead to a decreased need of personnel. Driving professions such as those of a bus driver, lorry driver or taxi driver will gradually diminish. For instance, it has been estimated that 5 million Americans work at least part time as drivers (Eisenstein, 2017 ). That is about 3% of the workforce. Even a partial and gradual replacement of these jobs by automatized vehicles will require solutions such as training schemes and other forms of labour market policies (Hicks, 2018 , p. 67; Ryan, 2020 ). If such measures are not taken, or are not efficient enough, the result will be unemployment, with its accompanying social problems. Footnote 11 It should be noted that other branches of industry are expected to undergo a similar process at the same time. The labour market effects of automatized road traffic can therefore be seen as part of the much larger question whether and how the labour market can be readjusted at sufficient pace to deal with the effects of artificial intelligence and its attendant automatization (Pavlidou et al., 2011 ).
However, self-driving vehicles may also have a positive effect on the supply side of the labour market. To the extent that travel becomes faster and/or more convenient, workers will be willing to take jobs at larger distance from home, thus facilitating matching on the labour market. Affordable travel opportunities to workplaces can make it possible for underprivileged people to escape poverty (Epting, 2019 , p. 393).
It is highly uncertain what effects the introduction of self-driving cars will have on employment in the automotive industry. A decrease in the number of cars produced would have a negative impact on employment. However, as noted in “Sect. 2 ”, the industry is expected to have a much higher post-production involvement in self-driving than in human-driven cars. This should have positive effects on employment in the automobile industry. However, parts of this effect may be due to a transfer of employments from other branches of industry. Furthermore, the automotive industry is at the same time subject to other developments that affect the size of its labour force, in particular the automatization of its production processes and economic developments in third-world countries that increase the number of potential users and owners of motor vehicles. The total effect of all these developments is uncertain.
9 Criminality
Almost invariably, major social changes give rise to new forms of criminality that threaten human welfare. We have no reason to believe that vehicle automatization will be an exception from this. Four important potential variants of criminality are illegal transportation, unauthorized access to data, sabotage, and new forms of auto theft.
Automated vehicles can be used for illegal transportation tasks, for instance smuggling and the delivery of drugs, stolen goods, and contraband. For law enforcement, this can give rise to new challenges. Police inspection of vehicles with no traveller will be less intrusive than inspection of vehicles containing humans, but privacy concerns will nevertheless have to be taken into account.
The most obvious way to steal data from a vehicle is to hack into its computer system, either by surreptitious physical connection or using its links to other vehicles and to the traffic guidance system (Jafarnejad et al., 2015 ). If the system contains sensitive information, such as geopositioned travel logs, then this information can be used for instance for blackmailing or for arranging an “accident” at a place to which the owner returns regularly. Information about individual travel patterns obtained from hacking of the traffic guidance system can be used in the same ways.
All self-driving vehicles depend on sensor and software technology, both of which are sensitive to manipulation. Physical sensor manipulation can be performed in order to make the vehicle dysfunctional or (worse) to hurt or kill its passengers (Petit & Shladover, 2015 ). The effects of such manipulation (as well as other forms of sensor malfunction) can to a large extent be eliminated with sensor redundancy. By comparing the inputs from several sensors with overlapping functionalities, sensor malfunctioning can be detected.
Software manipulation can be performed for various criminal purposes, for instance to make the vehicle inoperable, to make it crash, or to direct the vehicle to a destination undesired by the passengers, for instance with the intent of frightening or kidnapping travellers (Crane et al., 2017 , pp. 239–251; Jafarnejad et al., 2015 ; Joh, 2019 , p. 313). Such manipulations can be connected with terrorism or organized crime. The prospect of being helplessly driven at high speed to an unknown place would seem to be scary enough to intimidate a witness. The risk of such software manipulation should be taken seriously. In addition to the usual measures to prevent, detect, contain and respond to an attack, vehicles can be provided with an overriding option for passengers to order it to stop at the nearest place where it can be safely parked (Kiss, 2019 ).
Vehicles without passengers can be used for criminal and terrorist attacks, such as driving at high speed into a crowd, or carrying a bomb to a place where it will be detonated (instead of having it carried by a suicide bomber) (Joh, 2019 , pp. 306–307; Ryan, 2020 ). Some such crimes will require software manipulation, which criminals can be expected to perform on vehicles in their own possession. Therefore, systems that detect and report attempts to alter the software will have to be an essential component of the security system (Straub et al., 2017 ).
Software manipulation performed by insiders in the automotive industry is much more difficult to prevent. In the recent diesel emission scandals, prominent motor vehicle industries were capable of illegal manipulation of software, sanctioned on top level in the business hierarchies (Bovens, 2016 ). Since car manufacturers have much to lose from a bad safety record, they do not have an incentive to manipulate software in a way that leads to serious accidents. However, they may have an incentive to manipulate vehicle-to-road-management information in ways that avoid unfavourable reporting to statistical systems based on these communications. Manufacturers working under an authoritarian regime may be ordered to provide exported vehicles with software backdoors that can be used in a potential future conflict to create havoc in another country’s traffic system.
Terrorists or enemy states can hack the traffic guidance system (rather than individual vehicles) in order to sabotage a country’s road traffic. They can for instance stop or redirect transportation of goods, or they can direct targeted vehicles to deadly collisions. This is a serious security problem that requires at least two types of responses. First, traffic guidance systems have to be made as inaccessible as possible to attacks. Secondly, vehicle-to-vehicle communication systems should include warning signals sent out from crashing vehicles, giving rise to crash-avoiding reactions in vehicles in the vicinity.
Automatized cars need to be protected against unauthorized access. Privately owned cars can be equipped with face recognition or other bioidentification systems that only allow certain persons to start a ride (similar systems can exclude unauthorized persons from driving a conventional car, Park et al., 2017 ). Companies renting out self-driving cars will have strong incentives to install identification mechanisms that ensure proper payment and make it possible to trace customers who have done damage to the vehicle. Auto theft may therefore become much more difficult to get away with. This may lead to an increased prevalence of kidnappings with the sole purpose of using the kidnapped person to direct a self-driving car to a desired destination.
In mixed traffic, some roads or lanes may be reserved for driverless vehicles. The traffic on such roads may potentially run at higher speed than the highest speed allowed on roads that are open to conventionally driven cars. Illegal human driving on such roads can give rise to considerable risks, and will therefore have to be strictly forbidden. One potential new form of criminality is driving on such roads, as a form of street racing. There may also be other ways for human drivers to exploit the fast reactions of self-driving vehicles. Safety margins can be transgressed for the thrill of it or in order to pass queues and reach a destination faster (Lin, 2015 , p. 81; Lin, 2016 , p. 81; Sparrow & Howard, 2017 , p. 211). Pedestrians may develop over-reliance on the reactions of self-driving vehicles, and step out in front of a vehicle with an insufficient safety margin, relying on its fast braking (Färber, 2015 , p. 143; Färber, 2016 , p. 138; Loh & Misselhorn, 2019 ). Such over-trust in autonomous systems may offset the safety gains that are obtainable with automated road traffic. Measures against it may run into ethical problems concerning paternalism and intrusiveness.
10 Conclusion
In this final section, we will summarize some of the major ethical issues that require further deliberations.
10.1 Responsibility
The introduction of automated road traffic will give rise to large changes in responsibility ascriptions concerning accidents and traffic safety. Probably, the responsibilities now assigned to drivers will for the most part be transferred to the constructors and maintainers of vehicles, roads, and communication systems.
10.2 Public Attitudes
We can expect a much lower tolerance for crashes caused by driverless vehicles than for crashes attributable to errors by human drivers. Such high safety requirements may postpone the introduction of driverless systems even if these systems in fact substantially reduce the risks.
Public opinion will also be influenced by other issues than safety. Apprehensions about a future society dominated by increasingly autonomous technology can lead to resistance against self-driving vehicles. Such resistance can also be fuelled by aberrant “behaviour” of self-driving cars, and by wishes to retain human driving as a source of pride and self-fulfilment. On the other hand, if human driving coexists with much safer automated traffic, it may be put under pressure to become safer. There may also be proposals to limit human driving or to prohibit it altogether. All this can add up to severe social and political conflicts on automatized road traffic. Rash and badly prepared introductions of self-driving vehicles can potentially lead to an escalation of such conflicts.
10.3 Safety
The short reaction times of self-driving vehicles can be used to enhance safety or to increase speed. A trade-off between safety and speed will have to be struck. This applies to platooning on highways, and also to vehicle movements in the vicinity of pedestrians.
A fully automatic vehicle can carry passengers that could not travel alone in a conventional car, for instance a group of inebriated daredevils, or children unaccompanied by adults. It may then be difficult to ensure safety, for instance that seatbelts are used and that no one leans out of a window.
Over-reliance on the swift collision-avoiding reactions of self-driving cars can induce people to take dangerous actions. Pedestrians may step out in front of a vehicle, relying on its fast braking. Motorists may choose to drive (illegally) on roads or lanes reserved for automatic vehicles.
10.4 Control
The police will probably be able stop a self-driving vehicle by taking control of it electronically. This is much safer than traditional high-speed pursuits. However, the purposes and procedures for decisions to halt a vehicle will have to be based on a balance between the interests of law enforcement and other legitimate interests.
More ominously, criminals can take control over a vehicle in order to make it crash or become inoperable. Terrorists or enemy states can use self-driving vehicles to redirect the transportation of important goods, drive into crowds, carry bombs to their designed places of detonation, or create a general havoc in a country’s road system.
10.5 Information
Extensive information about routes and destinations will have to be collected in order to optimize the movements of self-driving vehicles. Such information can be misused or hacked. It can for instance be used to convey commercial and political messages to car users. An authoritarian state can use it to keep track of the opposition.
The safety of pedestrians, cyclists, and people travelling in conventional motor vehicles can be improved if they carry transponders that keep self-driving vehicles in their vicinity informed of their positions and movements. Such transponders will give rise to the same issues concerning privacy as the transponders in self-driving vehicles.
10.6 Social Justice
Vehicle types and models will differ in their crash avoidance systems, expectedly with newer and more expensive models having the best systems. It will be technically possible to allow cars with better safety features to operate on different places or at higher speeds than other cars. Socio-economic segregation of road traffic can potentially have considerable negative effects on social cohesion.
The need for professional drivers will gradually decrease, and many will lose their employments. This will require solutions such as training schemes and other forms of labour market policies.
In general, the ethical implications of introducing autonomous vehicles are not inherent in the technology itself, but will depend to a large extent on social choices, not least the decisions of law-makers. Choices have to be made for instance on the required level of safety, the distribution of responsibilities between infrastructure providers and vehicle manufacturers and providers, the organization of traffic control, trade-offs between privacy and other interests, and the adjustment of the traffic sector as a whole to climate and environmental policies. It is essential that these decisions be made in the public interest and based on thorough investigations of the issues at hand. There is also an urgent need for further ethical and social research that penetrates the full range of potential issues that the introduction of autonomous vehicles can give rise to, including key ethical issues such as equity, privacy, acceptability of risk, responsibility, and the social mechanisms for dealing with trade-offs and value conflicts.
Availability of Data and Material
This research is based on publicly available texts that are listed in the bibliography.
Code Availability
Not applicable.
For a previous review focusing on crashes with self-driving cars, see Nyholm ( 2018b , c ). For a comprehensive scenario-based treatment, see Ryan ( 2020 ).
Husak ( 2004 ) highlighted the unacceptably high level of risk-taking in the current road traffic system, but laid the responsibility on individual road-users, arguing for instance that trips taken for “frivolous purposes” (p. 352), such as recreational travels by car, are morally objectionable. In contrast, Vision Zero emphasizes the responsibility of those who can transform the traffic system and make it safer.
Hevelke and Nida-Rümelin ( 2015 ) proposed a form of collective (blame) responsibility, shared by all users of fully automated vehicles. However, such shared responsibility can only be implemented through an insurance-based compensation system. It cannot include the possibility of criminal charges. This does not seem to be a plausible way to deal with offences that may potentially include the causation of deaths and serious injuries.
Tigard ( 2020 ) proposed that in cases when a technological system has failed, we can “demand answers from the system itself” and even “hold AI to account by imposing sanctions, correcting undesirable behavioral patterns acquired, and generally seeing that the target of our response works to improve for the future.” Although this may be possible as a purely intellectual venture, it is difficult to see how the emotional components of responsibility ascriptions could be established in relation to software.
Possibly, large companies that rent out cars will take on more extensive responsibilities than private car owners, whether or not these companies are owned by the car industry.
However, it does not follow that machines necessarily perform better in a complex environment where unpredictable disturbances may require reactions that cannot be pre-programmed. Arguably, road traffic is such a complex environment, in particular mixed traffic with both driverless and conventional vehicles.
In Sweden between 1975 and 2007, recycling of older vehicles was rewarded with a bonus. This was primarily for environmental reasons, but the bonus also contributed to the disposal of vehicles lacking modern safety equipment.
See Nyholm ( 2018b , c ) and Davnall ( 2020 , pp. 431–434) for references and systematic reviews of this literature.
The most plausible scenario in which an ethical dilemma could arise seems to be sudden loss of braking power. This is a rare event in human driving and it is not expected to become more common in self-driving vehicles (Davnall 2020 ). The dilemmas that it can give rise to do not seem to be a common topic in drivers’ education.
For further clarifications of the lack of realism of these deliberations, see Gasser ( 2015 , p. 556), Goodall ( 2016 ), Hansson ( 2012 , p. 44), Hern ( 2016 ), Himmelreich ( 2018 ), and Nyholm and Smids ( 2016 ). For a well-articulated contrary view, see Keeling ( 2020 ). Keeling does not take into account the problems with swerving discussed above, and seems to grossly overestimate the frequency of cases with a controlled choice between different ways to crash.
This will not be the case in areas with a large shortage of drivers. Self-driving vehicles have been referred to as a potential solution to driver shortage (Mittal et al., 2018 ).
Abraham, K. S., & Rabin, R. L. (2019). Automated vehicles and manufacturer responsibility for accidents. Virginia Law Review, 105 (1), 127–171.
Google Scholar
Atiyeh, C. (2015). Volvo will take responsibility if its self-driving cars crash. Car and Driver , October 8. https://www.caranddriver.com/news/a15352720/volvo-will-take-responsibility-if-its-self-driving-cars-crash/ . Accessed 30 July 2021
Belin, M. -Å., Tillgren, P., & Vedung, E. (2012). Vision zero – A road safety policy innovation. International Journal of Injury Control and Safety Promotion, 19 (2), 171–179.
Bonnefon, J.-F., Černý, D., Danaher, J., Devillier, N., Johansson, V., Kovacikova, T., Martens, M., Mladenovic, M. N., Palade, P., Reed, N., de Sio, F. S., Tsinorema, S., Wachter, S., & Zawieska, K. (2020). Ethics of connected and automated vehicles: Recommendations on road safety, privacy, fairness, explainability and responsibility . European Commission. https://doi.org/10.2777/035239
Book Google Scholar
Borenstein, J., Herkert, J. R., & Miller, K. W. (2019). Self-driving cars and engineering ethics: The need for a system level analysis. Science and Engineering Ethics, 25 (2), 383–398.
Bovens, L. (2016). The ethics of Dieselgate. Midwest Studies in Philosophy, 40 , 262–283.
Brooks, R. (2017a). Unexpected consequences of self driving cars. https://rodneybrooks.com/unexpected-consequences-of-self-driving-cars/ . Accessed 30 July 2021
Brooks, R. (2017b). Edge cases for self driving cars. https://rodneybrooks.com/edge-cases-for-self-driving-cars/ . Accessed 30 July 2021.
Brown, Austin, Gonder, Jeffrey, & Repac, Brittany. (2014). An analysis of possible energy impacts of automated vehicles. In G. Meyer & S. Beiker (Eds.), Road vehicle automation (pp. 137–153). Springer.
Coeckelbergh, M. (2016). Responsibility and the moral phenomenology of using self-driving cars. Applied Artificial Intelligence, 30 (8), 748–757.
Connor, S. (2016). First self-driving cars will be unmarked so that other drivers don’t bully them. The Guardian , October 30.
Crane, D. A., Logue, K. D., & Pilz, B. C. (2017). A survey of legal issues arising from the deployment of autonomous and connected vehicles. Michigan Telecommunications and Technology Law Review, 23 , 191–320.
Cuthbertson, A. (2018). People are slashing tyres and throwing rocks at self-driving cars in Arizona. The Independent , December 13. https://www.independent.co.uk/life-style/gadgets-and-tech/news/self-driving-cars-waymo-arizona-chandler-vandalism-tyre-slashing-rocks-a8681806.html . Accessed 30 July 2021
Cuthbertson, A. (2019). Self-driving cars more likely to drive into black people, study claims. The Independent , March 6. https://www.independent.co.uk/life-style/gadgets-and-tech/news/self-driving-car-crash-racial-bias-black-people-study-a8810031.html . Accessed 30 July 2021
Danaher, J. (2016). Robots, law and the retribution gap. Ethics and Information Technology, 18 , 299–309.
Davnall, R. (2020). Solving the single-vehicle self-driving car trolley problem using risk theory and vehicle dynamics. Science and Engineering Ethics, 26 , 431–449.
de Jong, R. (2020). The retribution-gap and responsibility-loci related to robots and automated technologies: A reply to Nyholm. Science and Engineering Ethics, 26 (2), 727–735.
Dietrich, M., & Weisswange, T. H. (2019). Distributive justice as an ethical principle for autonomous vehicle behavior beyond hazard scenarios. Ethics and Information Technology, 21 , 227–239.
Dworkin, G. (1981). Voluntary health risks and public policy: Taking risks, assessing responsibility. Hastings Centrer Report, 11 (5), 26–31.
Edensor, T. (2004). Automobility and national identity: Representation, geography and driving practice. Theory, Culture & Society, 21 (4–5), 101120.
Edmonds, E. (2019). Three in four Americans remain afraid of fully self-driving vehicles. AAA Newsroom . https://newsroom.aaa.com/2019/03/americans-fear-self-driving-cars-survey . Accessed 30 July 2021.
Eisenstein, P. A. (2017). Millions of professional drivers will be replaced by self-driving vehicles. NBC News , November 5. https://www.nbcnews.com/business/autos/millions-professional-drivers-will-be-replaced-self-driving-vehicles-n817356 . Accessed 30 July 2021
Epting, S. (2019). Automated vehicles and transportation justice. Philosophy and Technology, 32 (3), 389–403.
Fagnant, D. J., & Kockelman, K. M. (2018). Dynamic ride-sharing and fleet sizing for a system of shared autonomous vehicles in Austin, Texas. Transportation, 45 (1), 143–158.
Färber, Berthold. (2015). Kommunikationsprobleme zwischen autonomen Fahrzeugen und menschlichen Fahrern. In M. Maurer, J. C. Gerdes, B. Lenz, & H. Winner (Eds.), Autonomes Fahren. Technische, rechtliche und gesellschaftliche Aspekte (pp. 127–146). Springer.
Färber, Berthold. (2016). Communication and communication problems between autonomous vehicles and human drivers. In M. Maurer, J. C. Gerdes, B. Lenz, & H. Winner (Eds.), Autonomous driving. Technical, legal and social aspects (pp. 125–144). Springer.
Flipse, S. M., & Puylaert, S. (2018). Organizing a collaborative development of technological design requirements using a constructive dialogue on value profiles: A case in automated vehicle development. Science and Engineering Ethics, 24 (1), 49–72.
Gasser, T. M. (2015). Grundlegende und spezielle Rechtsfragen für autonome Fahrzeuge. In M. Maurer, J. C. Gerdes, B. Lenz, & H. Winner (Eds.), Autonomes Fahren. Technische, rechtliche und gesellschaftliche Aspekte (pp. 543–574). Springer.
Gasser, T. M. (2016). Fundamental and special legal questions for autonomous vehicles. In M. Maurer, J. C. Gerdes, B. Lenz, & H. Winner (Eds.), Autonomous driving. Technical, legal and social aspects (pp. 523–551). Springer.
Gillespie, M. (2016). Shifting automotive landscapes: Privacy and the right to travel in the era of autonomous motor vehicles. Washington University Journal of Law and Policy, 50 , 147–169.
Goodall, N. J. (2016). Away from trolley problems and toward risk management. Applied Artificial Intelligence, 30 (8), 810–821.
Goodin, R. E. (1987). Apportioning responsibilities. Law and Philosophy, 6 , 167–185.
Greenwald, J. M., & Kornhauser, A. (2019). It’s up to us: Policies to improve climate outcomes from automated vehicles. Energy Policy, 127 , 445–451.
Gruel, W., & Stanford, J. M. (2016). Assessing the long-term effects of autonomous vehicles: A speculative approach. Transportation ResearchPprocedia, 13 , 18–29.
Gurney, J. K. (2017). Imputing driverhood. Applying a reasonable driver standard to accidents caused by autonomous vehicles. In P. Lin, K. Abney, & R. Jenkins (Eds.), Robot ethics 2.0: From autonomous cars to artificial intelligence (pp. 51–65). Oxford University Press.
Hansson, S. O. (2012). “A panorama of the philosophy of risk. In S. Roeser, R. Hillerbrand, P. Sandin, & M. Peterson (Eds.), Handbook of risk theory (pp. 27–54). Springer.
Hansson, S. O. (2016). How to be cautious but open to learning: Time to update biotechnology and GMO legislation. Risk Analysis, 36 (8), 1513–1517.
Hansson, S. O. (2022). Responsibility in road traffic. To be published in K. E. Björnberg, S. O. Hansson, M.-Å. Belin, & C. Tingvall (Eds.), Handbook of Vision Zero. Theory, technology and management for a zero casualty policy . Springer.
Harb, M., Xiao, Yu., Circella, G., Mokhtarian, P. L., & Walker, J. L. (2018). Projecting travelers into a world of self-driving vehicles: Estimating travel behavior implications via a naturalistic experiment. Transportation, 45 (6), 1671–1685.
Hasan, S., Balador, A., Girs, S., & Uhlemann, E. (2019). Towards emergency braking as a fail-safe state in platooning: A simulative approach. IEEE 90th Vehicular Technology Conference (VTC2019-Fall).
Heinrichs, D. (2015). Autonomes Fahren und Stadtstruktur. In M. Maurer, J. C. Gerdes, B. Lenz, & H. Winner (Eds.), Autonomes Fahren. Technische, rechtliche und gesellschaftliche Aspekte (pp. 219–239). Springer.
Heinrichs, Dirk. (2016). Autonomous driving and urban land use. In M. Maurer, J. C. Gerdes, B. Lenz, & H. Winner (Eds.), Autonomous driving Technical, legal and social aspects (pp. 213–231). Springer.
Hern, A. (2016). Self-driving cars don’t care about your moral dilemmas. The Guardian , 22 Aug.
Hevelke, A., & Nida-Rümelin, J. (2015). Responsibility for crashes of autonomous vehicles: An ethical analysis. Science and Engineering Ethics, 21 (3), 619–630.
Hicks, D. J. (2018). The safety of autonomous vehicles: Lessons from philosophy of science. IEEE Technology and Society Magazine, 37 (1), 62–69.
Himmelreich, J. (2018). Never mind the trolley: The ethics of autonomous vehicles in mundane situations. Ethical Theory and Moral Practice, 21 (3), 669–684.
Holstein, T., Dodig-Crnkovic, G., & Pelliccione, P. (2018). Ethical and social aspects of self-driving cars. arXiv preprint . https://arxiv.org/pdf/1802.04103.pdf . Accessed 30 July 2021
Hu, M., Zhao, X., Hui, F., Tian, B., Xu, Z., & Zhang, X. (2021). Modeling and analysis on minimum safe distance for platooning vehicles based on field test of communication delay. Journal of Advanced Transportation , article 5543114.
Husak, D. (2004). Vehicles and crashes: Why is this moral issue overlooked? Social Theory and Practice, 30 (3), 351–370.
Hutson, H. R., Rice Jr, P. L., Chana, J. K., Kyriacou, D. N., Chang, Y., & Miller, R. M. (2007). A review of police pursuit fatalities in the United States from 1982–2004. Prehospital Emergency Care, 11 (3), 278–283.
Jafarnejad, S., Codeca, L., Bronzi, W., Frank, R., & Engel, T. (2015). A car hacking experiment: When connectivity meets vulnerability. 2015 IEEE Globecom Workshops .
Joh, E. E. (2019). Automated seizures: Police stops of self-driving cars. New York University Law Review, 94 , 292–314.
Keeling, G. (2020). Why trolley problems matter for the ethics of automated vehicles. Science and Engineering Ethics, 26 (1), 293–307.
King, K. F. (2011). Personal jurisdiction, internet commerce, and privacy: The pervasive legal consequences of modern geolocation technologies. Alabama Law Journal of Science and Technology, 21 , 61–124.
King, M. (2014). Traction without tracing: A (partial) solution for control-based accounts of moral responsibility. European Journal of Philosophy, 22 (3), 463–482.
Kiss, G. (2019). External manipulation recognition modul in self-driving vehicles. In 2019 IEEE 17th international symposium on intelligent systems and informatics (SISY) (pp. 231–234). IEEE.
Kyriakidis, M., Happee, R., & de Winter, J. C. F. (2015). Public opinion on automated driving: Results of an international questionnaire among 5000 respondents. Transportation Research Part F: Traffic Psychology and Behaviour, 32 , 127–140.
Kyriakidis, M., de Winter, J. C. F., Stanton, N., et al. (2019). A human factors perspective on automated driving. Theoretical Issues in Ergonomics Science, 20 (3), 223–249.
Lin, Patrick. (2015). Why ethics matters for autonomous cars. In M. Maurer, J. C. Gerdes, B. Lenz, & H. Winner (Eds.), Autonomes Fahren. Technische, rechtliche und gesellschaftliche Aspekte (pp. 69–85). Springer.
Lin, Patrick. (2016). Why ethics matters for autonomous cars. In M. Maurer, J. C. Gerdes, B. Lenz, & H. Winner (Eds.), Autonomous driving. Technical, legal and social aspects (pp. 69–85). Springer.
Liu, P., Yang, R., & Zhigang, Xu. (2019). How safe is safe enough for self-driving vehicles? Risk Analysis, 39 (2), 315–325.
Loh, W., & Misselhorn, C. (2019). Autonomous driving and perverse incentives. Philosophy and Technology, 32 , 575–590.
Luetge, C. (2017). The German ethics code for automated and connected driving. Philosophy and Technology, 30 (4), 547–558.
Lyneham, M., & Hewitt-Rau, A. (2013). Motor vehicle pursuit-related fatalities in Australia, 2000–11. Trends and Issues in Crime and Criminal Justice, 452 , 1.
Marchant, G. E., & Lindor, R. A. (2012). The coming collision between autonomous vehicles and the liability system. Santa Clara Law Review, 52 , 1321–1340.
Matthias, A. (2004). The responsibility gap: Ascribing responsibility for the actions of learning automata. Ethics and Information Technology, 6 (3), 175–183.
Mecacci, G., & de SantoniSio, F. S. (2020). Meaningful human control as reason-responsiveness: The case of dual-mode vehicles. Ethics and Information Technology, 22 , 103–115.
Melcher, V., Rauh, S., Diederichs, F., Widlroither, H., & Bauer, W. (2015). Take-over requests for automated driving. Procedia Manufacturing, 3 , 2867–2873.
Mittal, N., Udayakumar, P. D., Raghuram, G., & Bajaj, N. (2018). The endemic issue of truck driver shortage – A comparative study between India and the United States. Research in Transportation Economics, 71 , 76–84.
Mladenovic, M. N., & McPherson, T. (2016). Engineering social justice into traffic control for self-driving vehicles? Science and Engineering Ethics, 22 (4), 1131–1149.
Moor, R. (2016). What happens to American myth when you take the driver out of it? The self-driving car and the future of the self. Intelligencer , New York Magazine , October 16. http://nymag.com/selectall/2016/10/is-the-self-driving-car-un-american.html . Accessed 30 July 2021.
Morhart, C., & Biebl, E. (2011). High precision distance measurement for pedestrian protection using cooperative sensors. In S. Lindenmeier & R. Weigel (Eds.), Electromagnetics and network theory 89 and their microwave technology applications (pp. 89–104). Springer.
Müller, J. F., & Gogoll, J. (2020). Should manual driving be (eventually) outlawed? Science and Engineering Ethics, 26 , 1549–1567
Nieuwenhuijsen, M. J., & Khreis, H. (2016). Car free cities: Pathway to healthy urban living. Environment International, 94 , 251–262.
Nyholm, S. (2018a). Attributing agency to automated systems: Reflections on human–robot collaborations and responsibility-loci. Science and Engineering Ethics, 24 (4), 1201–1219.
Nyholm, Sven. (2018b). The ethics of crashes with self-driving cars: A roadmap, I. Philosophy Compass, 13 (7), e12507.
Nyholm, Sven. (2018c). The ethics of crashes with self-driving cars: A roadmap, II. Philosophy Compass, 13 (7), e12506.
Nyholm, S., & Smids, J. (2016). The ethics of accident-algorithms for self-driving cars: An applied trolley problem? Ethical Theory and Moral Practice, 19 (5), 1275–1289.
Nyholm, S., & Smids, J. (2020). Automated cars meet human drivers: responsible human-robot coordination and the ethics of mixed traffic. Ethics and Information Technology J, 22 , 335–344.
Palm, E., & Hansson, S. O. (2006). The case for ethical technology assessment (eTA). Technological Forecasting and Social Change, 73 , 543–558.
Park, S.-H., Kim, J.-H., & Jun, M.-S., et al. (2017). A design of secure authentication method with bio-information in the car sharing environment. In J. J. Park (Ed.), Advances in computer science and ubiquitous computing. Lecture notes in electrical engineering 421 (pp. 205–210). Springer.
Pavlidou, N.-E., Tsaliki, P. V., & Vardalachakis, I. N. (2011). Technical change, unemployment and labor skills. International Journal of Social Economics, 38 (7), 595–606.
Pernestål, A., & Kristoffersson, I. (2019). Effects of driverless vehicles–Comparing simulations to get a broader picture. European Journal of Transport & Infrastructure Research, 19 (1), 1–23.
Petit, J., & Shladover, S. E. (2015). Potential cyberattacks on automated vehicles. IEEE Transactions on Intelligent Transportation Systems, 16 (2), 546–556.
Prakken, H. (2017). On the problem of making autonomous vehicles conform to traffic law. Artificial Intelligence and Law, 25 (3), 341–363.
Rice, T. M., Troszak, L., & Gustafson, B. G. (2015). Epidemiology of law enforcement vehicle collisions in the US and California. Policing: An International Journal of Police Strategies & Management, 38 (3), 425–435.
Rolison, J. J., Regev, S., Moutari, S., & Feeney, A. (2018). What are the factors that contribute to road accidents? An assessment of law enforcement views, ordinary drivers’ opinions, and road accident records. Accident Analysis & Prevention, 115 , 11–24.
Rosencrantz, H., Edvardsson, K., & Hansson, S. O. (2007). Vision zero – Is it irrational? Transportation Research Part A: Policy and Practice, 41 , 559–567.
Roy, A. (2018). This is the human driving manifesto. Driving is a privilege, not a right. Let’s fight to protect it. https://www.thedrive.com/article/18952/this-is-the-human-driving-manifesto . Accessed 30 July 2021
Ryan, M. (2020). The future of transportation: ethical, legal, social and economic impacts of self-driving vehicles in the year 2025. Science and Engineering Ethics, 26 , 1185–1208
Sallis, J. F., Floyd, M. F., Rodríguez, D. A., & Saelens, B. E. (2012). Role of built environments in physical activity, obesity, and cardiovascular disease. Circulation, 125 (5), 729–737.
Smith, B. W. (2014). A legal perspective on three misconceptions in vehicle automation. In G. Meyer & S. Beiker (Eds.), Road vehicle automation (pp. 85–91). Springer.
Smith, B. W. (2017). The trolley and the pinto: Cost-benefit analysis in automated driving and other cyber-physical systems. Texas A&M Law Review, 4, 197–208.
Sobel, R. (2014). The right to travel and privacy: Intersecting fundamental freedoms. The John Marshall Journal of Information Technology and Privacy Law, 30 , 639–666.
Soteropoulos, A., Berger, M., & Ciari, F. (2019). Impacts of automated vehicles on travel behaviour and land use: An international review of modelling studies. Transport Reviews, 39 (1), 29–49.
Sparrow, R., & Howard, M. (2017). When human beings are like drunk robots: Driverless vehicles, ethics, and the future of transport. Transportation Research Part C: Emerging Technologies, 80 , 206–215.
Stone, T., de Sio, F. S., & Vermaas, P. E. (2020). Driving in the dark: Designing autonomous vehicles for reducing light pollution. Science and Engineering Ethics, 26 , 387–403
Straub, J., McMillan, J., Yaniero, B., Schumacher, M., Almosalami, A., Boatey, K., & Hartman, J. (2017). CyberSecurity considerations for an interconnected self-driving car system of systems. In 12th system of systems engineering conference (SoSE). IEEE.
Svarcas, F. (2012). Turning a new leaf: A privacy analysis of Carwings electric vehicle data collection and transmission. Santa Clara Computer and High Technology Law Journal, 29 , 165–197.
Tigard, D. W. (2020). There is no techno-responsibility gap. Philosophy and Technology , published online.
Urmson, C., & Whittaker, W. (2008). Self-driving cars and the urban challenge. IEEE Intelligent Systems, 23 (2), 66–68.
van Wyk, F., Wang, Y., Khojandi, A., & Masoud, N. (2020). Real-time sensor anomaly detection and identification in automated vehicles. IEEE Transactions on Intelligent Transportation Systems, 21 (3), 1264–1276.
Véliz, C. (2019). The internet and privacy. In D. Edmonds (Ed.), Ethics and the contemporary world (pp. 149–159). Routledge.
Vold, K., & Whittlestone, J. (2019). Privacy, autonomy, and personalised targeting: Rethinking how personal data is used. Véliz, C. (Ed.), Report on data, privacy, and the individual in the digital age . Downloaded May 17, 2020 from https://philpapers.org/archive/VOLPAA-2.pdf . Accessed 30 July 2021
Vrščaj, D., Nyholm, S., & Verbong, G. P. J. (2020). Is tomorrow’s car appealing today? Ethical issues and user attitudes beyond automation. AI and Society, 35 , 1033–1046.
Zhang, W., Guhathakurta, S., & Khalil, E. B. (2018). The impact of private autonomous vehicles on vehicle ownership and unoccupied VMT generation. Transportation Research Part C: Emerging Technologies, 90 , 156–165.
Zimmer, M. (2005). Surveillance, privacy and the ethics of vehicle safety communication technologies. Ethics and Information Technology, 7 (4), 201–210.
Download references
Open access funding provided by The Royal Institute of Technology. This research was supported by funding from the Swedish Transport Administration.
Author information
Authors and affiliations.
Division of Philosophy, Royal Institute of Technology (KTH), Stockholm, Sweden
Sven Ove Hansson & Matts-Åke Belin
The Swedish Transport Administration, Borlänge, Sweden
Matts-Åke Belin
The Institute for Futures Studies, Stockholm, Sweden
Björn Lundgren
Department of Historical, Philosophical and Religious Studies, Umeå University, Umeå, Sweden
Department of Philosophy, Stockholm University, Stockholm, Sweden
You can also search for this author in PubMed Google Scholar
Corresponding author
Correspondence to Sven Ove Hansson .
Ethics declarations
Conflict of interest.
The authors declare no competing interests.
Additional information
Publisher’s note.
Springer Nature remains neutral with regard to jurisdictional claims in published maps and institutional affiliations.
Rights and permissions
Open Access This article is licensed under a Creative Commons Attribution 4.0 International License, which permits use, sharing, adaptation, distribution and reproduction in any medium or format, as long as you give appropriate credit to the original author(s) and the source, provide a link to the Creative Commons licence, and indicate if changes were made. The images or other third party material in this article are included in the article's Creative Commons licence, unless indicated otherwise in a credit line to the material. If material is not included in the article's Creative Commons licence and your intended use is not permitted by statutory regulation or exceeds the permitted use, you will need to obtain permission directly from the copyright holder. To view a copy of this licence, visit http://creativecommons.org/licenses/by/4.0/ .
Reprints and permissions
About this article
Hansson, S.O., Belin, MÅ. & Lundgren, B. Self-Driving Vehicles—an Ethical Overview. Philos. Technol. 34 , 1383–1408 (2021). https://doi.org/10.1007/s13347-021-00464-5
Download citation
Received : 25 September 2020
Accepted : 29 June 2021
Published : 12 August 2021
Issue Date : December 2021
DOI : https://doi.org/10.1007/s13347-021-00464-5
Share this article
Anyone you share the following link with will be able to read this content:
Sorry, a shareable link is not currently available for this article.
Provided by the Springer Nature SharedIt content-sharing initiative
- Self-driving vehicles
- Traffic safety
- Automatization
- Future traffic system
- Find a journal
- Publish with us
- Track your research
Self-Driving Cars in Developing Countries: Case Study India
Ieee account.
- Change Username/Password
- Update Address
Purchase Details
- Payment Options
- Order History
- View Purchased Documents
Profile Information
- Communications Preferences
- Profession and Education
- Technical Interests
- US & Canada: +1 800 678 4333
- Worldwide: +1 732 981 0060
- Contact & Support
- About IEEE Xplore
- Accessibility
- Terms of Use
- Nondiscrimination Policy
- Privacy & Opting Out of Cookies
A not-for-profit organization, IEEE is the world's largest technical professional organization dedicated to advancing technology for the benefit of humanity. © Copyright 2024 IEEE - All rights reserved. Use of this web site signifies your agreement to the terms and conditions.
Self-driving car technology: a case study
- Link to current page
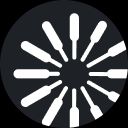
- engineering
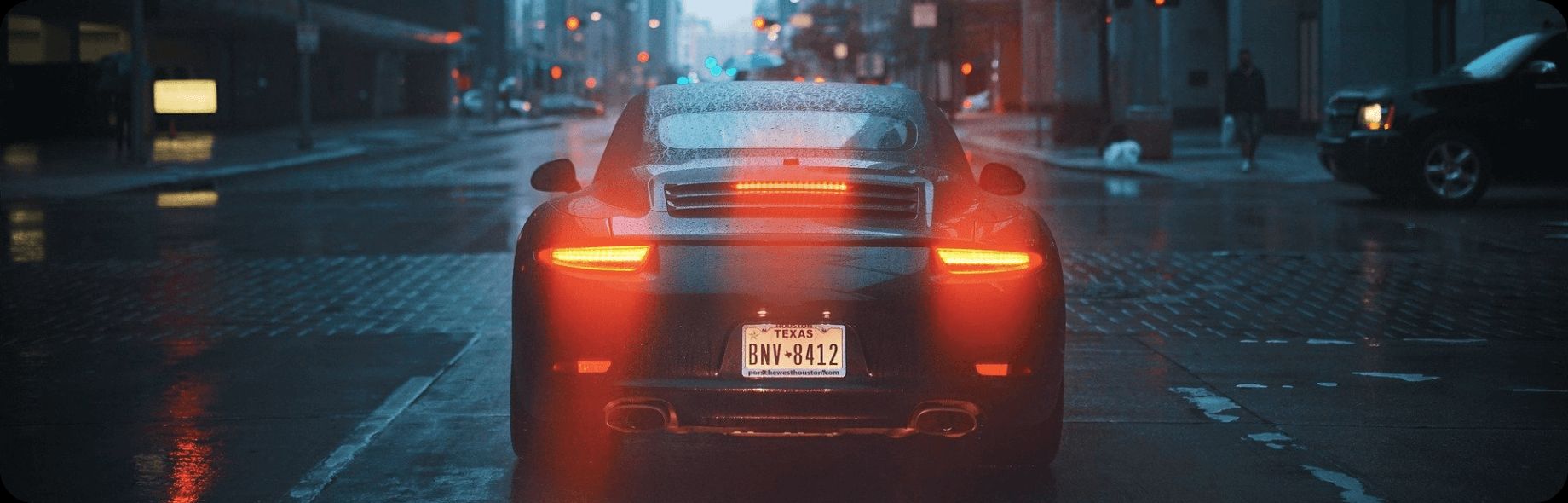
Subscribe to Toloka News
The Yandex Self-Driving Group has been working on cutting-edge technologies for self-driving cars since 2017. But how does Toloka help keep them in the race to develop autonomous vehicles? In this post we'll talk about how the vehicles learn to see the world around them — what kind of data is collected, how it is processed, what algorithms are used, and the role Toloka plays.
Here's a diagram showing what's "under the hood" of self-driving cars:
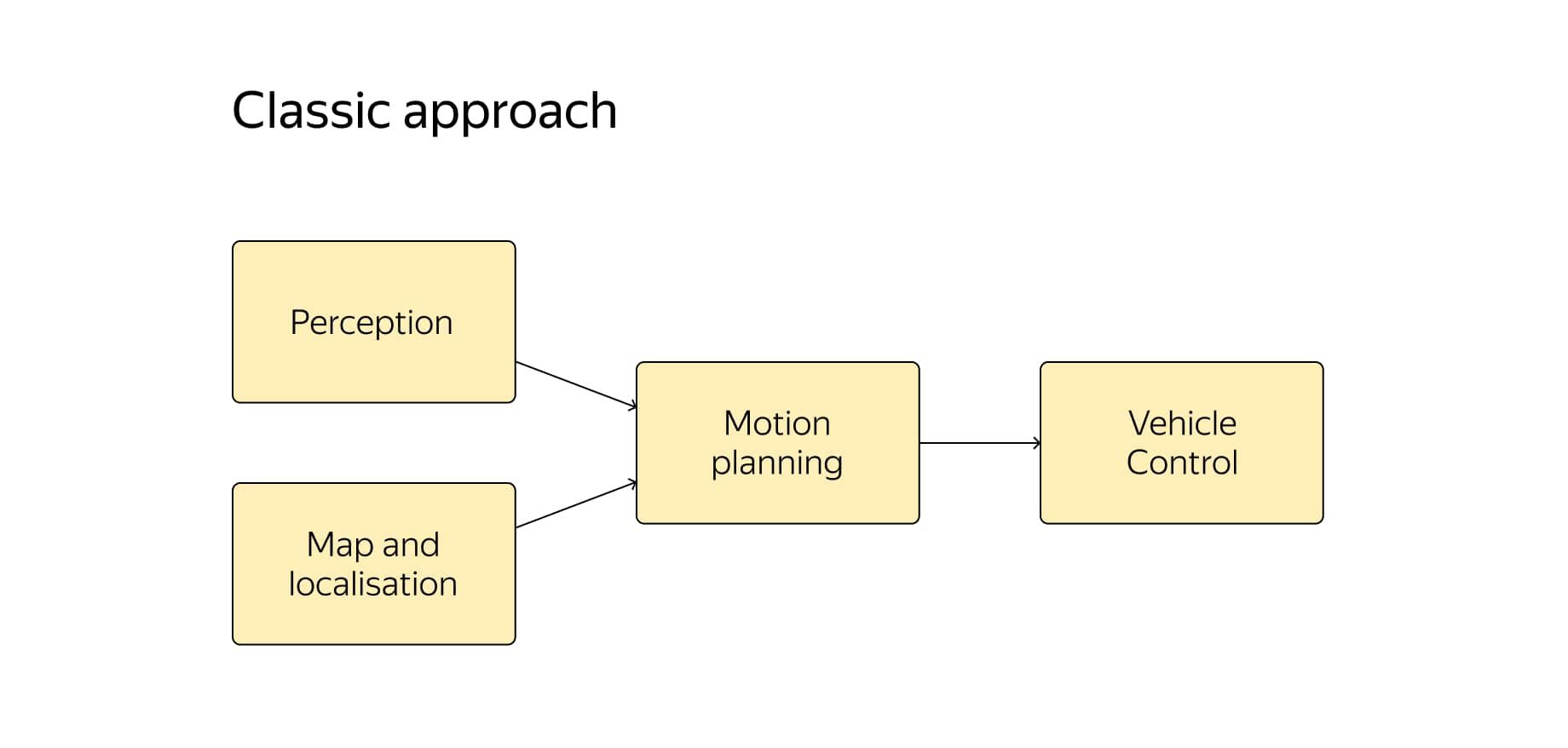
First there's the perception component, which is responsible for detecting what's around the car. Then there are maps and the localization component, which determines exactly where the vehicle is located in the world. Information from these two components is fed into the motion planning component of the car, which decides where to go and what path to take based on real conditions. Then the motion planning component passes the path to the vehicle control component, which sets the steering angle for the path and compensates for the physics of the vehicle. Vehicle control is mostly about physics.
In this post, we'll focus on the perception component and related data analysis. The other components are also extremely important, but the better a car is able to recognize the world around it, the easier it is to do the rest.
So how does the perception component work? First, we need to understand what data is fed into the vehicle. There are many sensors built into the car, but the most widely used ones are cameras, radars, and lidars.
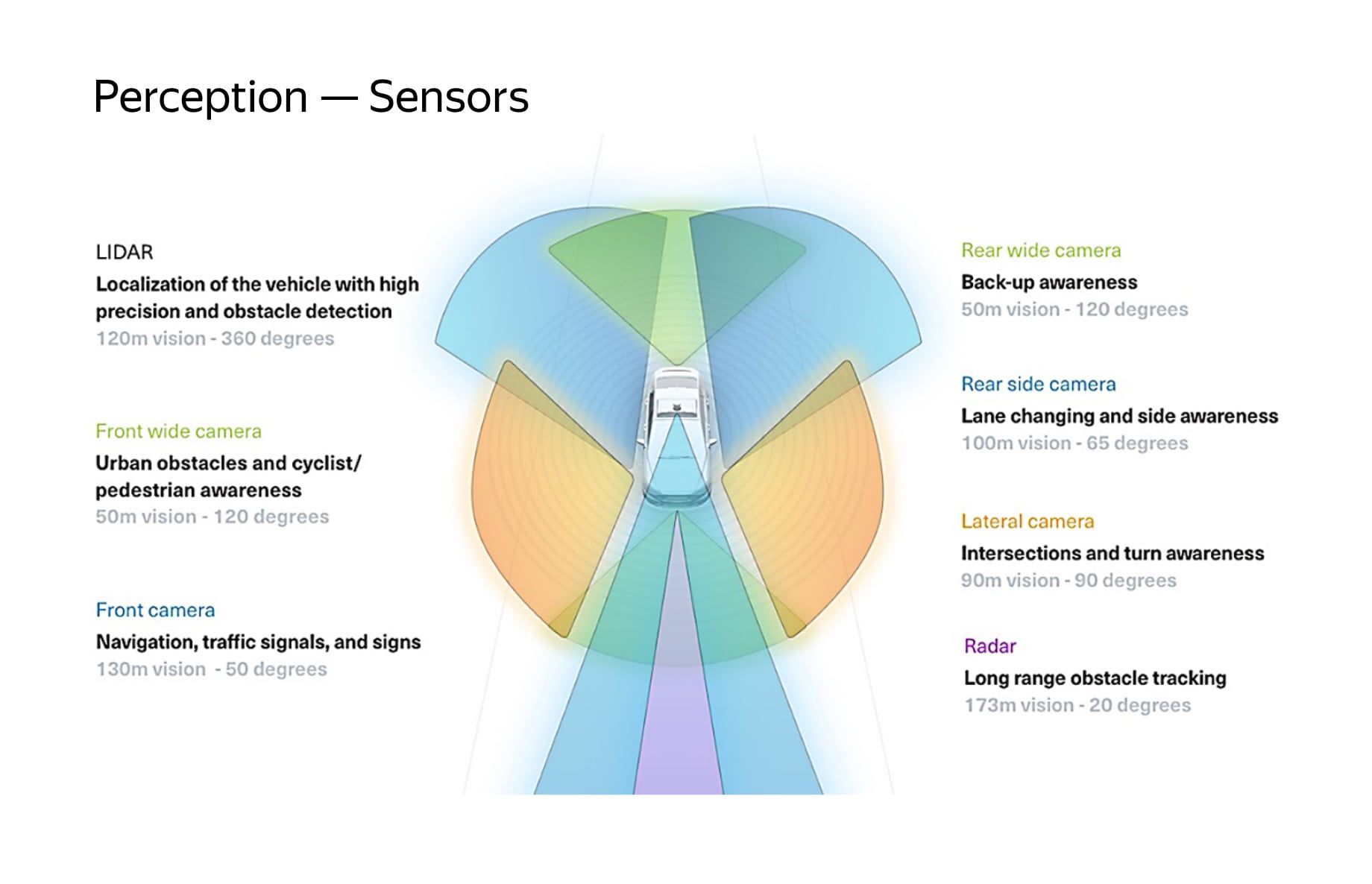
A radar is a production sensor that is widely used in adaptive cruise controls. It's the sensor that detects a car's position based on its solid angle. This sensor works very well on metal items, like cars, but it doesn't work so well on pedestrians. A distinctive feature of radar is that it detects both position and speed; the Doppler effect is used to determine radial velocity. Cameras provide standard video input.
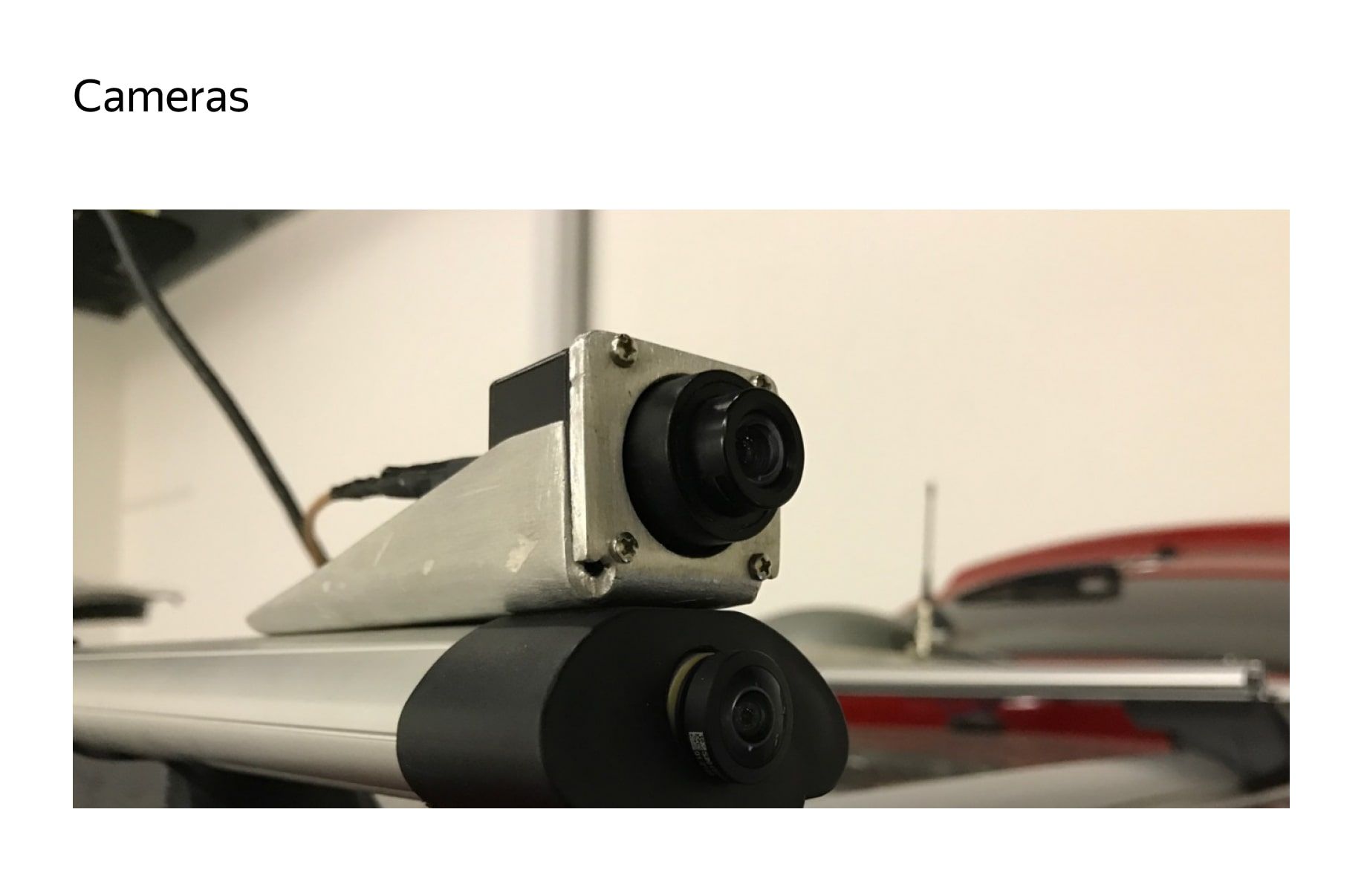
Lidar is more interesting. If you've ever renovated a home, you might be familiar with laser rangefinders that can be mounted on a wall. Inside those rangefinders is a timer that measures how much time it takes for light to travel back and forth to and from a wall, which is used to calculate the distance. There are actually more complex physical principles at work, but the point is that the lidar has many laser rangefinders that are mounted vertically. As the lidar spins, the rangefinders scan the space around them.
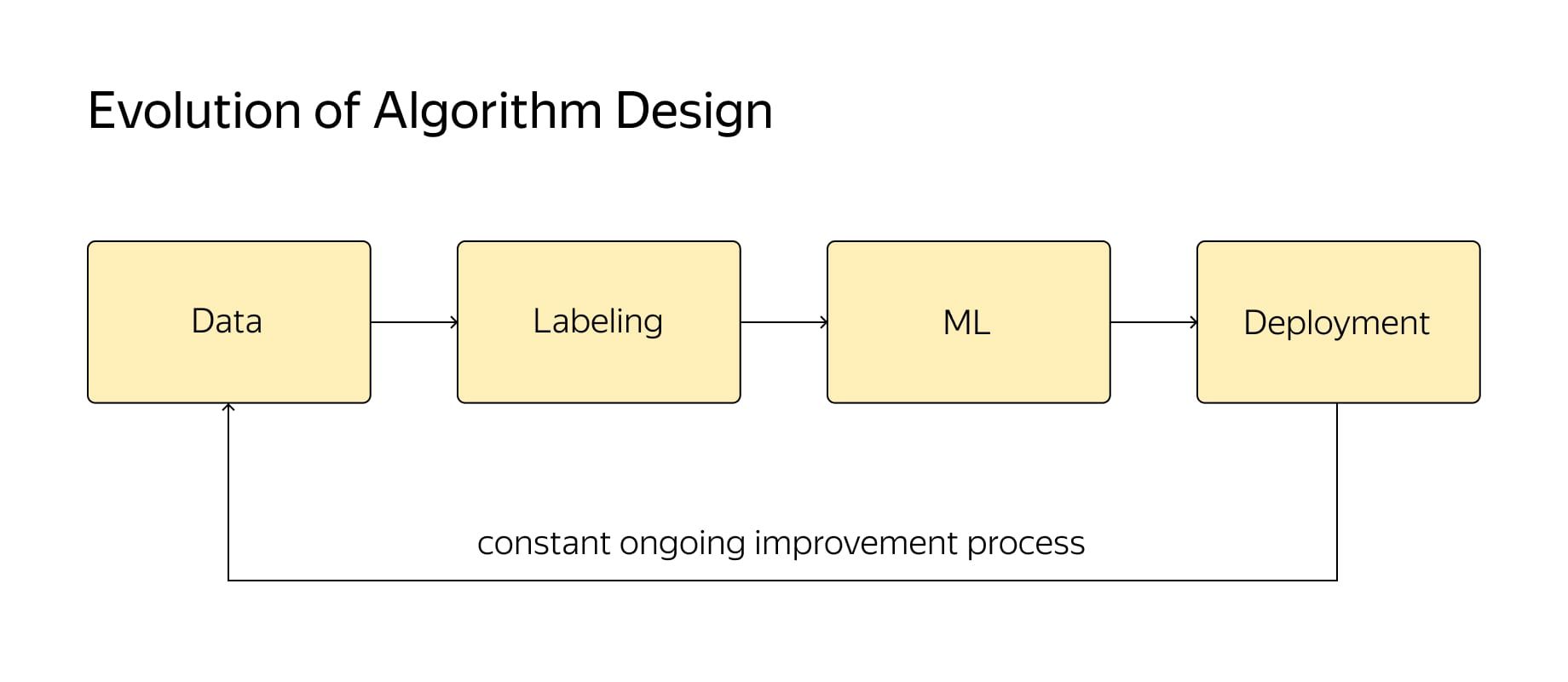
The vehicle has multiple sensors, and each of them generates data. This paves the way to a classic pipeline for training machine learning algorithms.
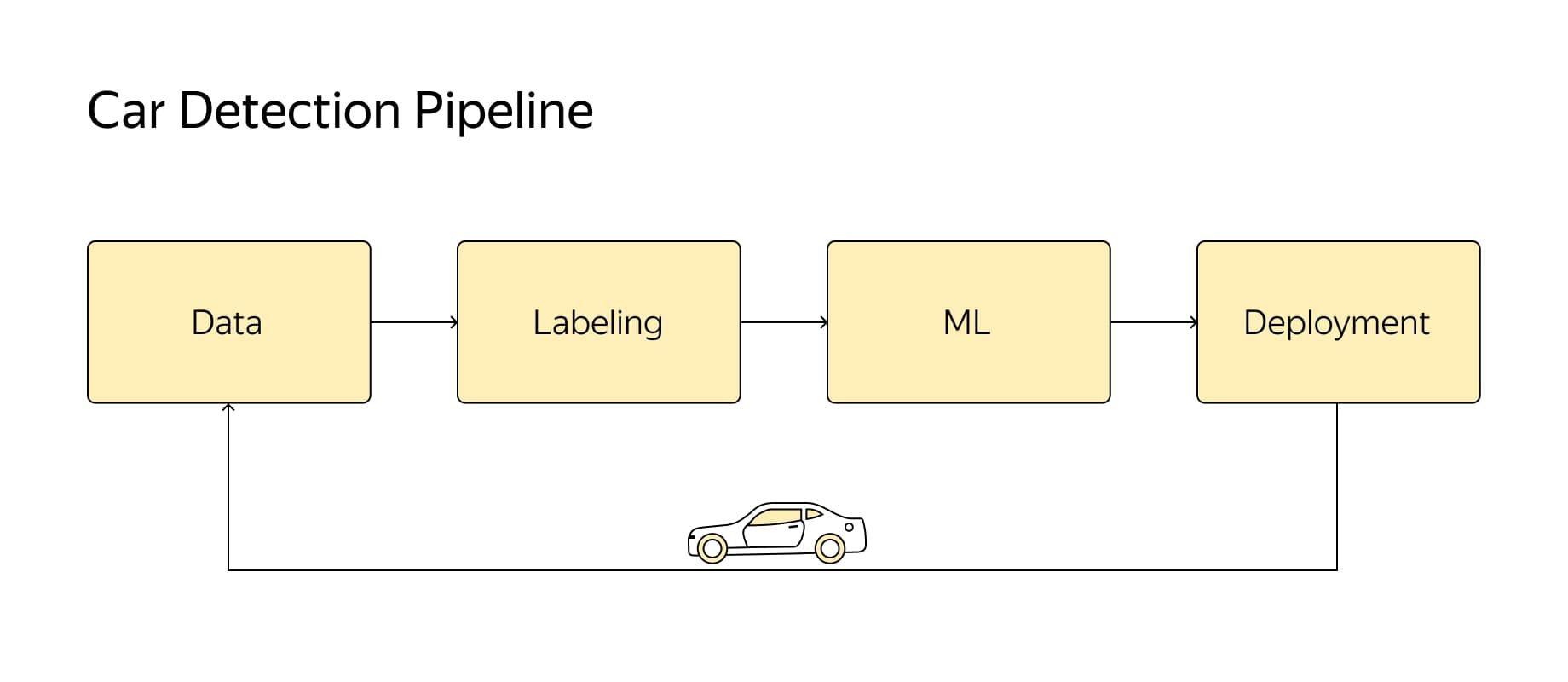
Data must be collected from vehicles, uploaded to the cloud, and sent out for labeling. The labeled data is used to train the model. After it is deployed on the fleet, more data is collected, and this process is repeated over and over. The result should be fed back to the car as soon as possible to keep things running smoothly.
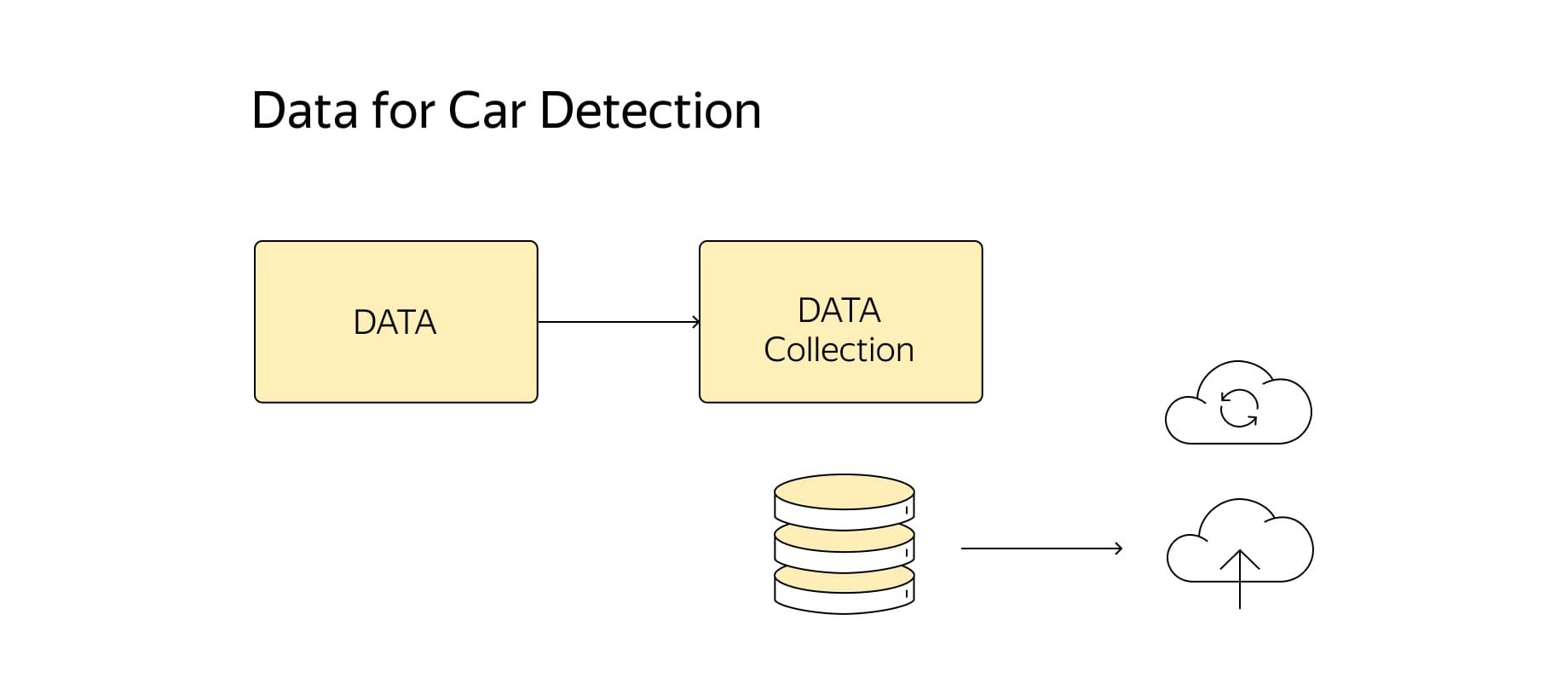
Once the data is collected and uploaded to the cloud, speed is of the essence in data labeling .
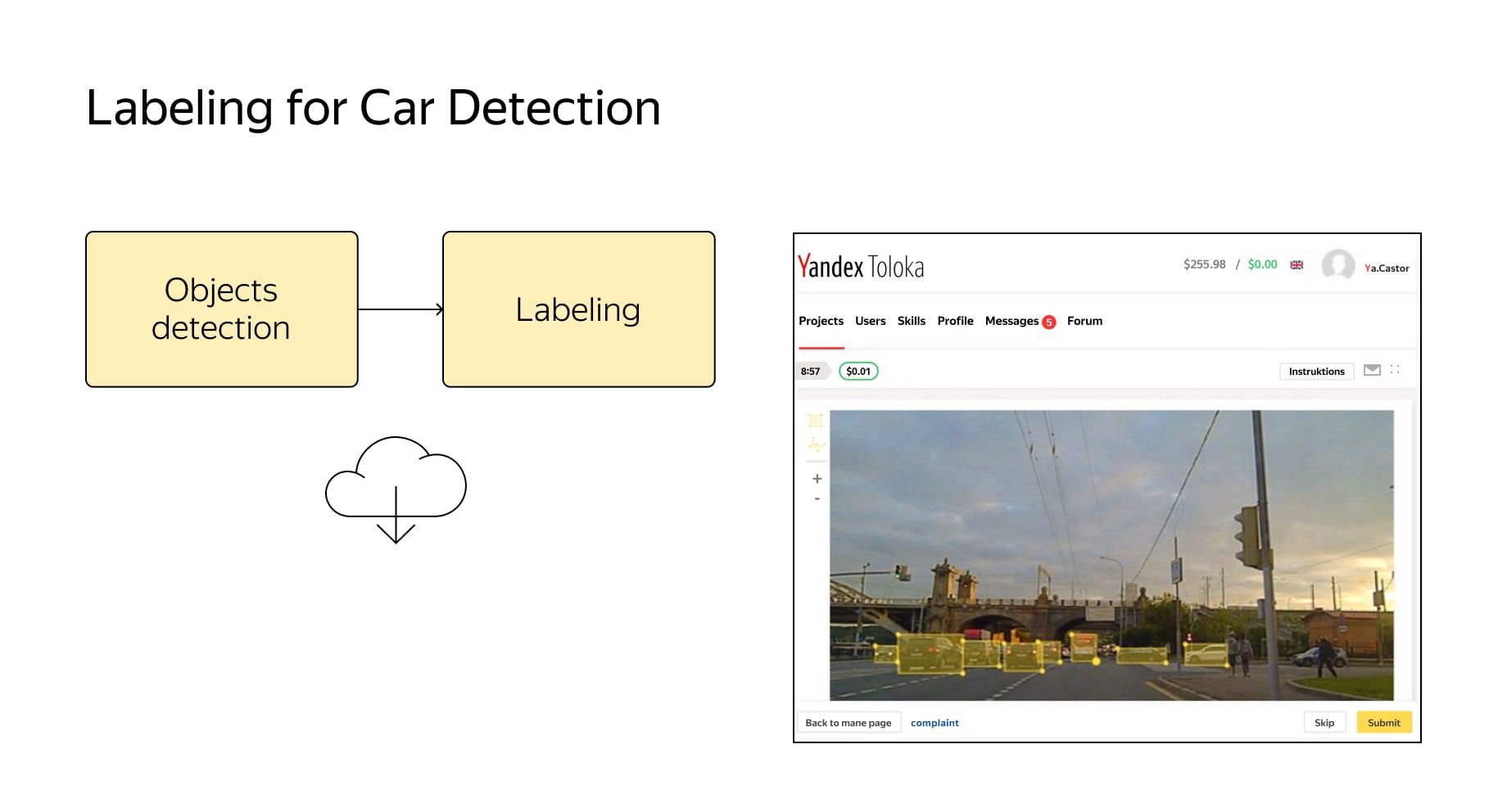
The Yandex development team goes to Toloka to prepare the training data. Tolokers can label large datasets quickly and cheaply. In the case of vehicle detectors, a simple web interface is distributed to tolokers where they select objects in images with bounding boxes, which is fast and easy.
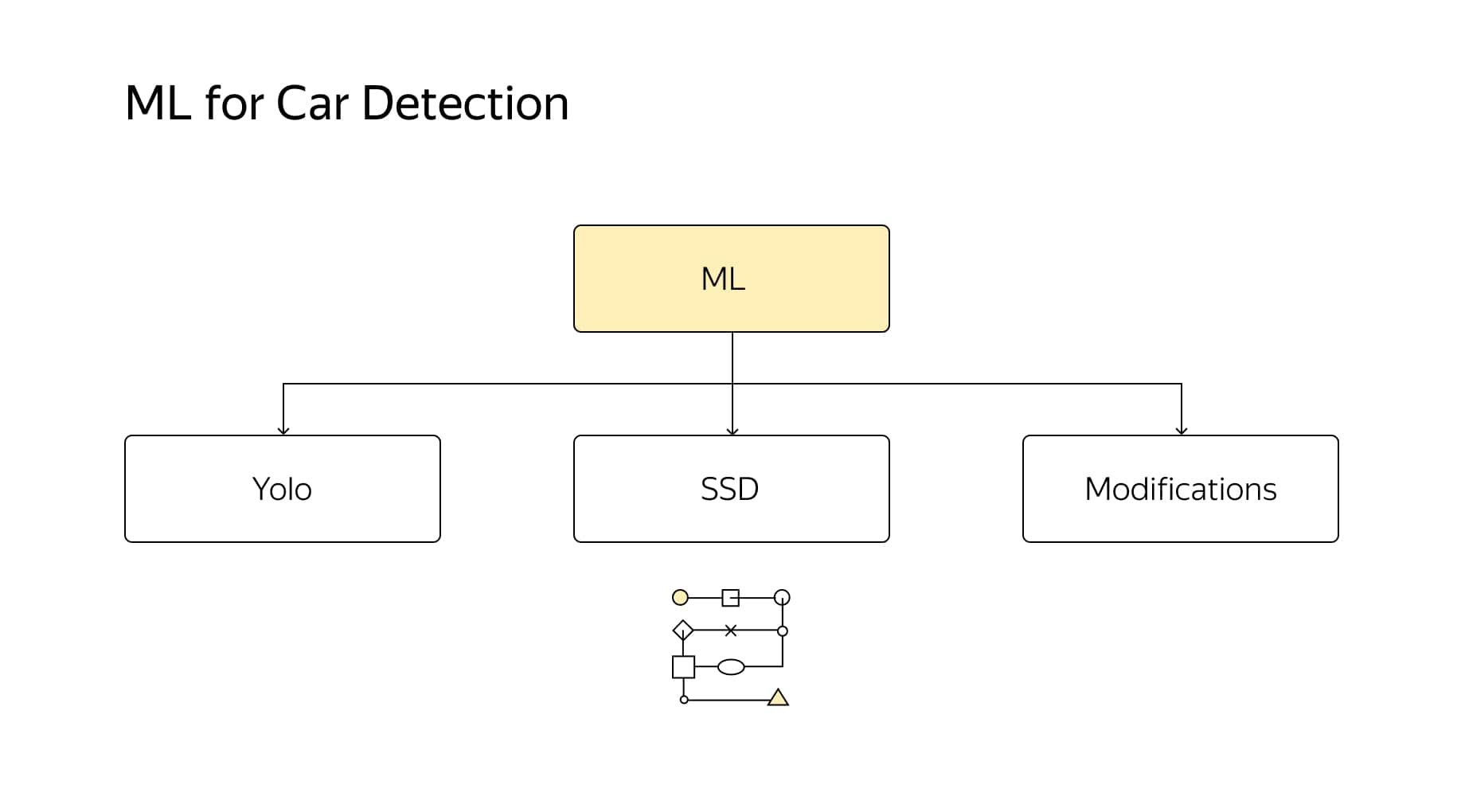
The next step is to choose the machine learning method. There are many fast machine learning methods available, like SSD, Yolo, and their modifications.
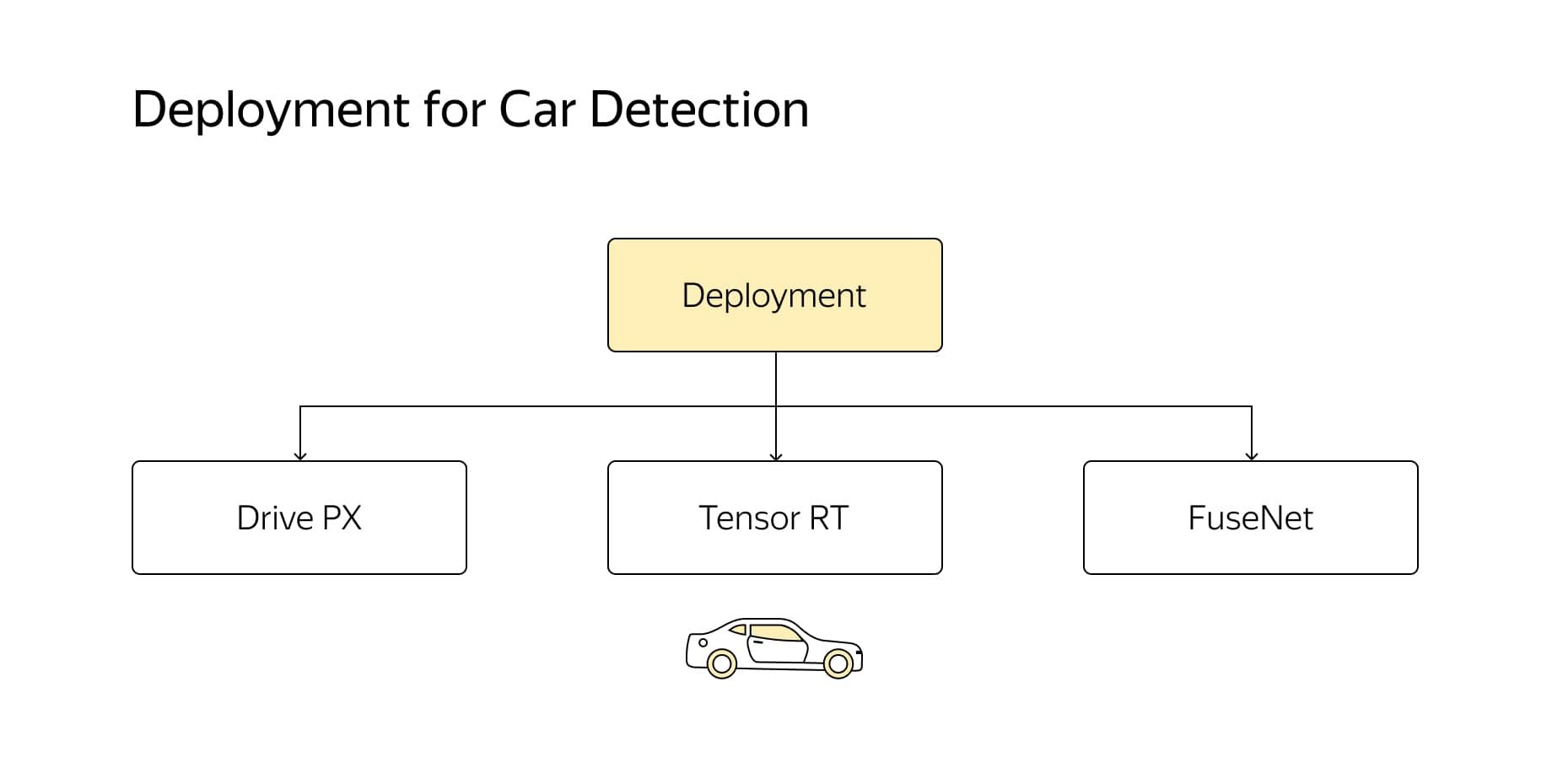
The results are then deployed in the vehicles. There are a lot of cameras involved in order to cover all 360 degrees, and the system must work very quickly to respond to traffic conditions. Various technologies are used: inference engines like Tensor RT, specialized hardware, Drive PX, and FuseNet. Multiple algorithms operate with a unified backend, and compressions are run once. This is a fairly common approach.
In addition to vehicles, the sensors also detect pedestrians and the direction of movement. This algorithm works for a large number of car cameras in real time.
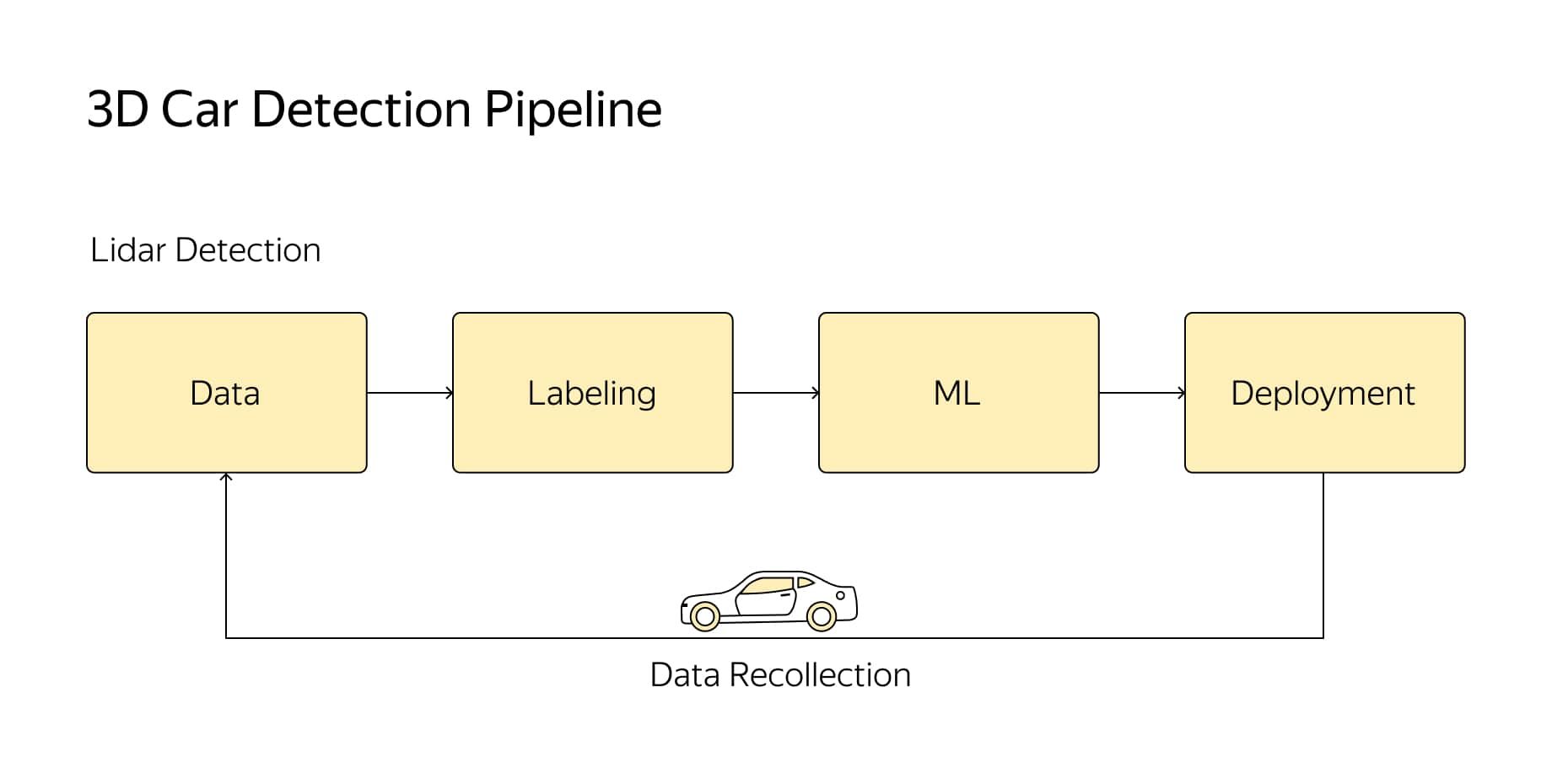
Object detection is essentially a solved problem. There are various algorithms available, as well as lots of competitions on this topic and lots of datasets.
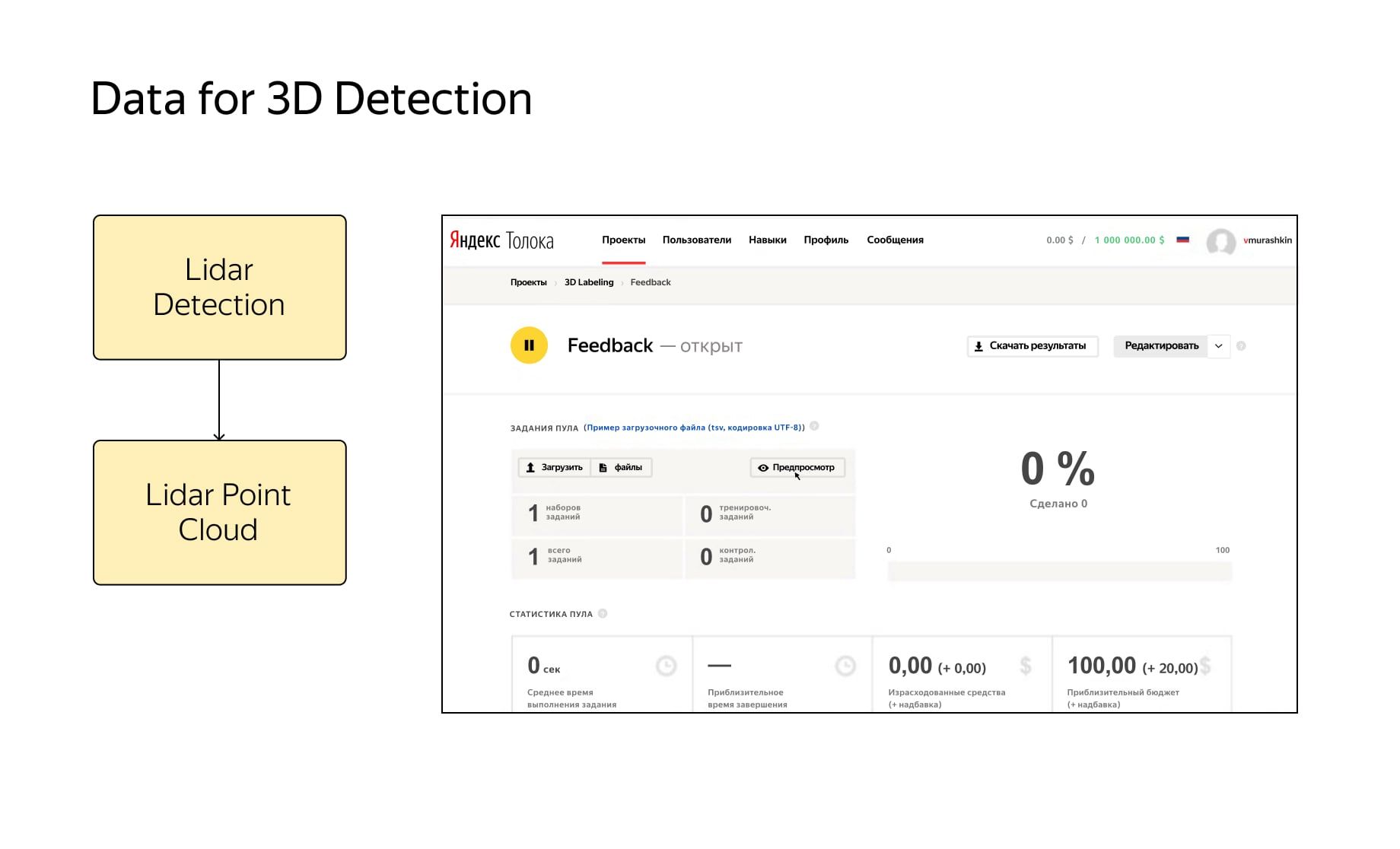
The situation with lidars is more difficult because there was previously only one relevant dataset available: the KITTI dataset. To get started, Yandex developers had to label new data from scratch, and at this point Toloka came to the rescue again.
Labeling a point cloud is not a trivial procedure. Toloka users are not experts in 3D labeling, so it is a challenge to explain how 3D projections work and how they can identify cars in a point cloud, but a good lidar data flow was established.
The next challenge is to take the point clouds with 3D coordinates from around a car and neural networks that are optimized for detection, and feed the point clouds to the neural network as input. Yandex experimented with an approach in which projections are constructed with a birds-eye view of the points and then divided into cells. If there is at least one point in a given cell, it is considered to be occupied.
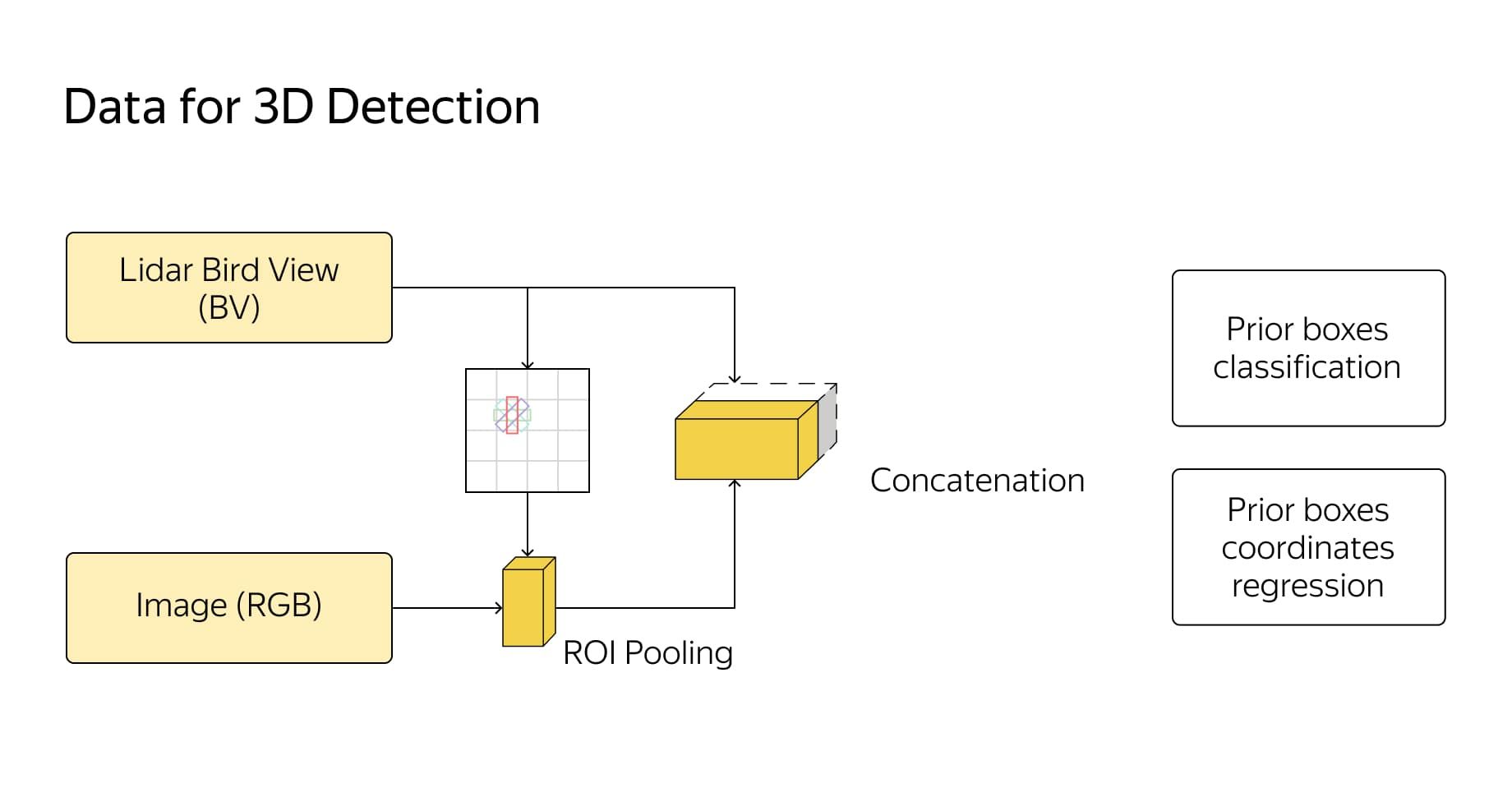
Vertical slices can be added and if there is at least one point in the resulting cube, it can be assigned some kind of characteristic. For example, designating cubes by their topmost point works well. Slices are then fed into a neural network, much like images. There are 14 channels for input and they are handled in the same way as with an SSD, plus an input signal from a network trained for detection. An image is fed into the network, which is trained end-to-end. The output predicts the 3D boxes, their classes, and positions.
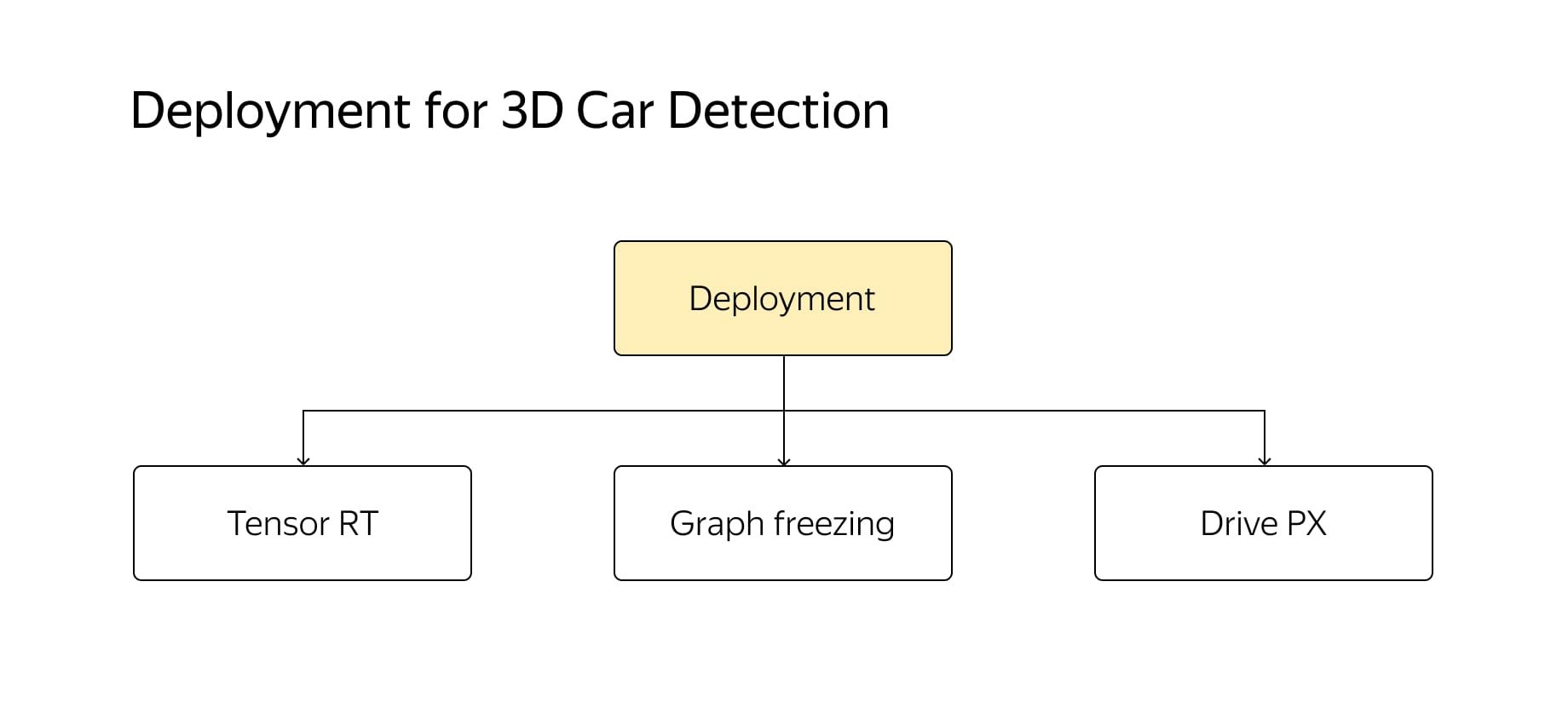
The next step is redeployment in the car system. Here's an example of how it works (this is just training, but it also works in real tests).
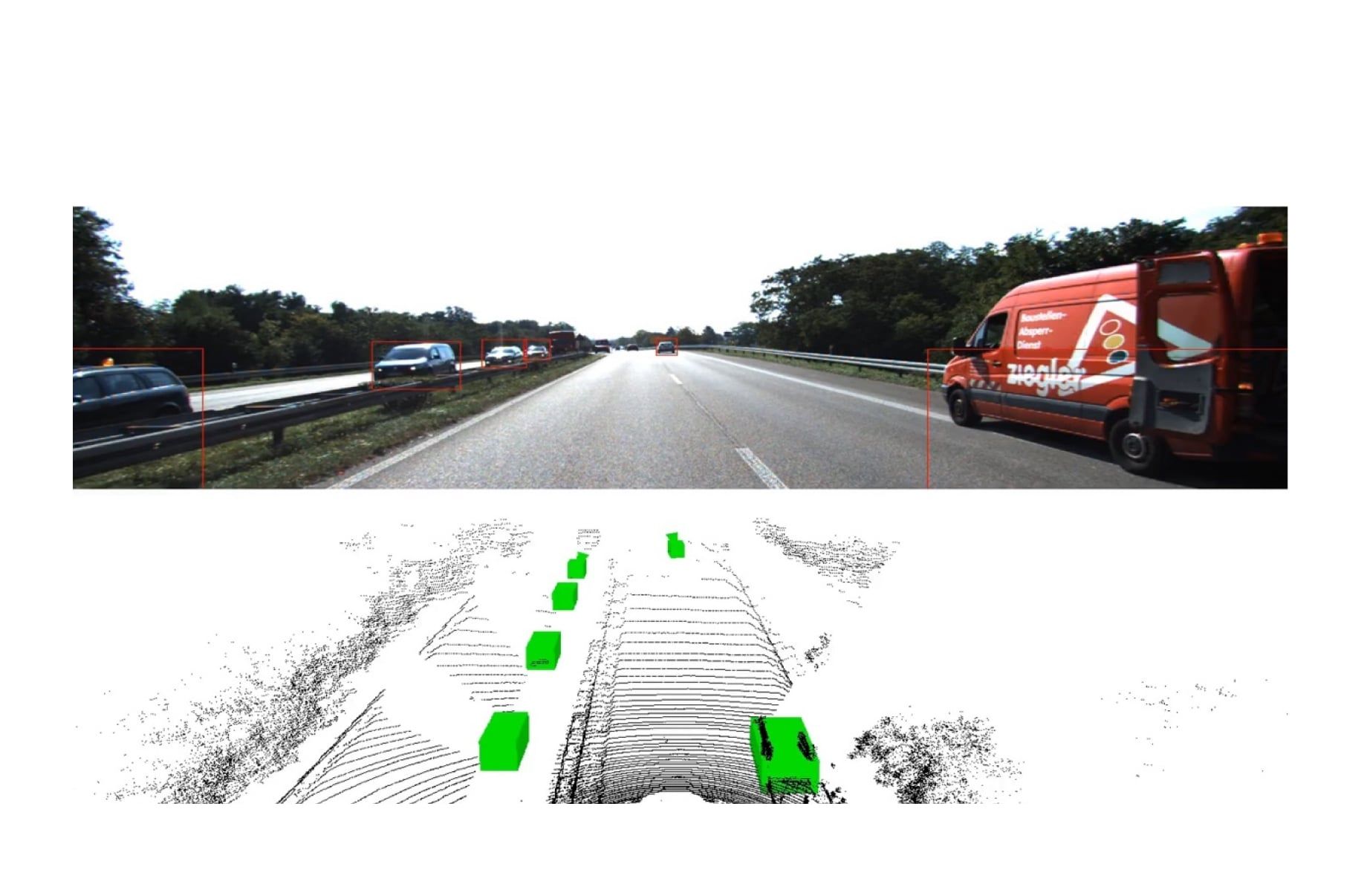
Cars are marked with green boxes.
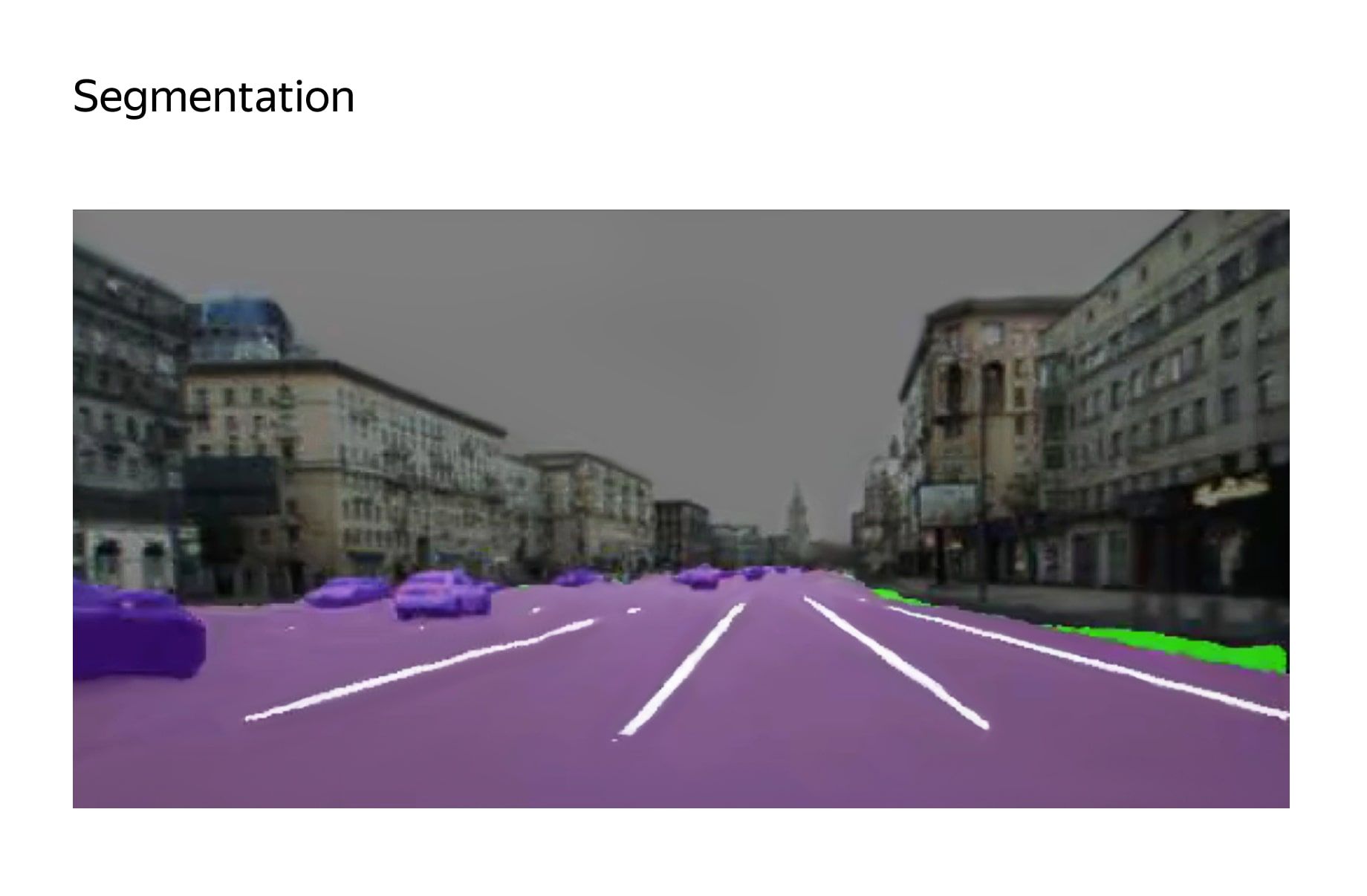
Segmentation is another algorithm that can be used to identify what is in the image and where it is located. Segmentation assigns a class to every pixel. This specific image shows a road and labeled segments. The edges of the road are marked in green, and the cars are marked in purple.
Segmentation has a disadvantage in terms of how it can be fed into motion planning: everything blends together. For instance, if there are cars parked close to one another, there will be one big purple block of cars and it's hard to guess how many there actually are. This leads to another problem — instance segmentation — which involves dividing different entities into parts. Yandex has been working on this, too.
Recent articles
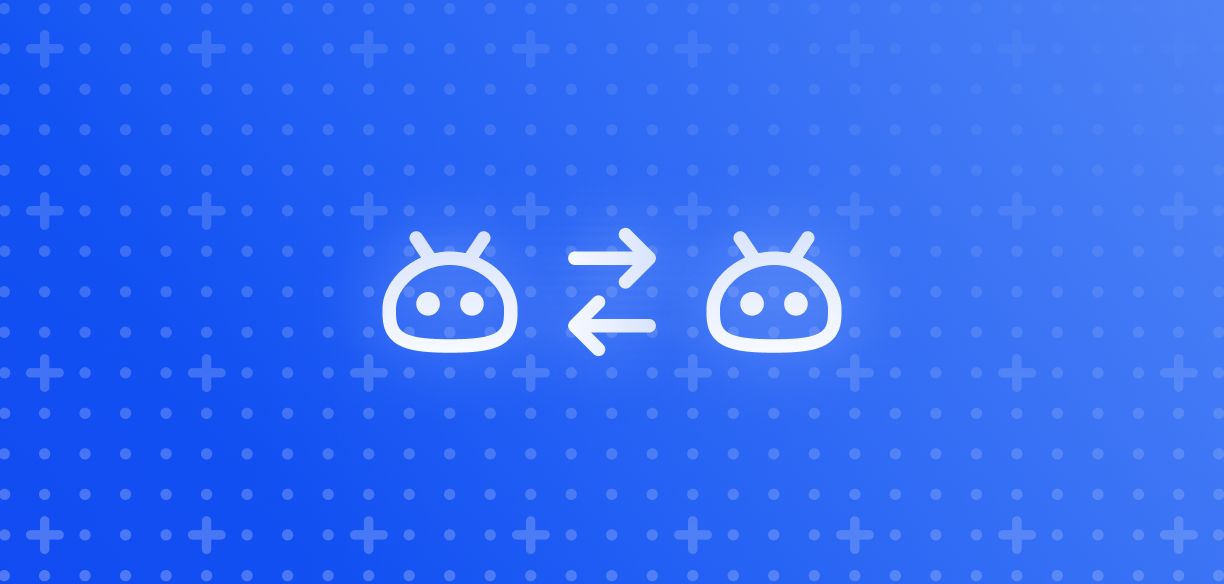
Have a data labeling project?
In 2018 crash, Tesla’s Autopilot just followed the lane lines
Depositions in a civil case over the fatal california crash -- set for trial this week -- explain how the software steers: it’s not fancy.
In Tesla’s marketing materials, the company’s Autopilot driver-assistance system is cast as a technological marvel that uses “advanced cameras, sensors and computing power” to steer, accelerate and brake automatically — even change lanes so “you don’t get stuck behind slow cars or trucks.”
Under oath, however, Tesla engineer Akshay Phatak last year described the software as fairly basic in at least one respect: the way it steers on its own.
“If there are clearly marked lane lines, the system will follow the lane lines,” Phatak said under questioning in July 2023. Tesla’s groundbreaking system, he said, was simply “designed” to follow painted lane lines.
Phatak’s testimony, which was obtained by The Washington Post, came in a deposition for a wrongful-death lawsuit set for trial Tuesday. The case involves a fatal crash in March 2018, when a Tesla in Autopilot careened into a highway barrier near Mountain View, Calif., after getting confused by what the company’s lawyers described in court documents as a “faded and nearly obliterated” lane line.
The driver, Walter Huang, 38, was killed. An investigation by the National Transportation Safety Board later cited Tesla’s failure to limit the use of Autopilot in such conditions as a contributing factor: The company has acknowledged to National Transportation Safety Board that Autopilot is designed for areas with “clear lane markings.”
Phatak’s testimony marks the first time Tesla has publicly explained these design decisions, peeling back the curtain on a system shrouded in secrecy by the company and its controversial CEO, Elon Musk. Musk, Phatak and Tesla did not respond to requests for comment.
Following lane lines is not unique to Tesla: Many modern cars use technology to alert drivers when they’re drifting. But by marketing the technology as “Autopilot,” Tesla may be misleading drivers about the cars’ capabilities — a central allegation in numerous lawsuits headed for trial this year and a key concern of federal safety officials.
For years, Tesla and federal regulators have been aware of problems with Autopilot following lane lines, including cars being guided in the wrong direction of travel and placed in the path of cross-traffic — with sometimes fatal results. Unlike vehicles that are designed to be completely autonomous, like cars from Waymo or Cruise, Teslas do not currently use sensors such as radar or lidar to detect obstacles. Instead, Teslas rely on cameras.
After the crash that killed Huang, Tesla told officials that it updated its software to better recognize “poor and faded” lane markings and to audibly alert drivers when vehicles might lose track of a fading lane. The updates stopped short of forcing the feature to disengage on its own in those situations, however. About two years after Huang died, federal investigators said they could not determine whether those updates would have been sufficient to “accurately and consistently detect unusual or worn lane markings” and therefore prevent Huang’s crash.
Huang, an engineer at Apple, bought his Tesla Model X in fall 2017 and drove it regularly to work along U.S. Highway 101, a crowded multilane freeway that connects San Francisco to the tech hubs of Silicon Valley. On the day of the crash, his car began to drift as a lane line faded. It then picked up a clearer line to the left — putting the car between lanes and on a direct trajectory for a safety barrier separating the highway from an exit onto State Route 85.
Huang’s car hit the barrier at 71 mph, pulverizing its front end , twisting it into unrecognizable heap. Huang was pronounced dead hours later, according to court documents.
In the months preceding the crash, Huang’s vehicle swerved in a similar location eleven times, according to internal Tesla data discussed by Huang’s lawyers during a court hearing last month. According to the data, the car corrected itself seven times. Four other times, it required Huang’s intervention. Huang was allegedly playing a game on his phone when the crash occurred.
The NTSB concluded that driver distraction and Autopilot’s “system limitations” likely led to Huang’s death. In its report, released about two years after the crash, investigators said Tesla’s “ineffective monitoring” of driver engagement also “facilitated the driver’s complacency and inattentiveness.”
Investigators also said that the California Highway Patrol’s failure to report the damaged crash barrier — which was ruined in a previous collision — contributed to the severity of Huang’s injuries.
Huang’s family sued Tesla, alleging wrongful death, and sued the state of California over the damaged crash barrier. The Post obtained copies of several depositions in the case, including testimony which has not been previously reported. Reuters also recently reported on some depositions from the case.
The documents shed light on one of federal regulators and safety officials’ biggest frustrations with Tesla: why Autopilot at times engages on streets where Tesla’s manual says it is not designed to be used. Such areas include streets with cross traffic, urban streets with frequent stoplights and stop signs, and roads without clear lane markings.
In his deposition, Phatak said Autopilot will work wherever the car’s cameras detect lines on the road: “As long as there are painted lane lines, the system will follow them,” he said.
Asked about another crash involving the software, Phatak disputed NTSB’s contention that Autopilot should not have functioned on the road in Florida where driver Jeremy Banner was killed in 2019 when his Tesla barreled into a semi-truck and slid under its trailer. “If I’m not mistaken, that road had painted lane lines,” Phatak said. Banner’s family has filed a wrongful-death lawsuit, which has not yet gone to trial.
Musk has said cars operating in Autopilot are safer than those controlled by humans , a message that several plaintiffs — and some experts — have said creates a false sense of complacency among Tesla drivers. The company has argued that it is not responsible for crashes because it makes clear to Tesla drivers in user manuals and on dashboard screens that they are solely responsible for maintaining control of their car at all times. So far, that argument has prevailed in court, most recently when a California jury found Tesla not liable for a fatal crash that occurred when Autopilot was allegedly engaged.
Autopilot is included in nearly every Tesla. It will steer on streets, follow a set course on freeways and maintain a set speed and distance without human input. It will even change lanes to pass cars and maneuver aggressively in traffic depending on the driving mode selected. It does not stop at stop signs or traffic signals. For an additional $12,000, drivers can purchase a package called Full Self-Driving that can react to traffic signals and gives the vehicles the capability to follow turn-by-turn directions on surface streets.
Since 2017, officials with NTSB have urged Tesla to limit Autopilot use to highways without cross traffic, the areas for which the company’s user manuals specify Autopilot is intended. Asked by an attorney for Huang’s family if Tesla “has decided it’s not going to do anything” on that recommendation, Phatak argued that Tesla was already following the NTSB’s guidance by limiting Autopilot use to roads that have lane lines.
“In my opinion we already are doing that,” Phatak said. “We are already restricting usage of Autopilot.”
A Washington Post investigation last year detailed at least eight fatal or serious Tesla crashes that occurred with Autopilot activated on roads with cross traffic.
Last month, the Government Accountability Office called on the National Highway Traffic Safety Administration, the top auto safety regulator, to provide additional information on driver-assistance systems “to clarify the scope of intended use and the driver’s responsibility to monitor the system and the driving environment while such a system is engaged.”
Phatak’s testimony also shed light on other driver-assist design choices, such as Tesla’s decision to monitor driver attention through sensors that gauge pressure on the steering wheel. Asked repeatedly by the Huang family’s lawyer what tests or studies Tesla performed to ensure the effectiveness of this method, Phatak said it simply tested it with employees.
Other Tesla design decisions have differed from competitors pursuing autonomous vehicles. For one thing, Tesla sells its systems to consumers, while other companies tend to deploy their own fleets as taxis. It also employs a unique, camera-based system and places fewer limits on where the software can be engaged. For example, a spokesperson for Waymo, the Alphabet-owned self-driving car company, said its vehicles operate only in areas that have been rigorously mapped and where the cars have been tested in conditions including fog and rain, a process known as “geo-fencing.”
“We’ve designed our system knowing that lanes and their markings can change, be temporarily occluded, move, and sometimes, disappear completely,” Waymo spokeswoman Katherine Barna said.
California regulators also restrict where these driverless cars can operate, and how fast they can go.
When asked whether Autopilot would use GPS or other mapping systems to ensure a road was suitable for the technology, Phatak said it would not. “It’s not map based,” he said — an answer that diverged from Musk’s statement in a 2016 conference call with reporters that Tesla could turn to GPS as a backup “when the road markings may disappear.” In an audio recording of the call cited by Huang family attorneys, Musk said the cars could rely on satellite navigation “for a few seconds” while searching for lane lines.
Tesla’s heavy reliance on lane lines reflects the broader lack of redundancy within its systems when compared to rivals. The Post has previously reported that Tesla’s decision to omit radar from newer models, at Musk’s behest, culminated in an uptick in crashes.
Rachel Lerman contributed to this report.

- Share full article
Advertisement
Supported by
Tesla Settles Lawsuit Over a Fatal Crash Involving Autopilot
A Tesla driver’s family had sought damages for the 2018 crash, which happened while the carmaker’s driver-assistance software was in use.

By Jack Ewing
Tesla on Monday settled a lawsuit that blamed the automaker’s driver-assistance software for the death of a California man in 2018 , averting a trial that would have focused attention on the company’s technology several months before it plans to unveil a self-driving taxi.
The trial stemming from the death of Wei Lun Huang, an Apple software engineer who went by Walter, was scheduled to start Monday with jury selection. The case was one of the most prominent involving Tesla’s Autopilot software, attracting significant public attention and prompting an investigation by the National Transportation Safety Board.
Terms of the settlement with Mr. Huang’s children and other members of his family were not disclosed, and Tesla filed court documents seeking to prevent them from being made public.
Testimony in the trial would have put Tesla’s autonomous driving software under close scrutiny, further fueling a debate about whether the technology makes cars safer or exposes drivers and others to serious injury or death.
Elon Musk, the chief executive of Tesla, has said the company’s self-driving software will generate hundreds of billions of dollars in revenue. Investors have used his claims to justify the company’s lofty stock market valuation. Tesla is worth more than any other carmaker even though its shares have plunged in recent months.
Mr. Musk said on X last week that Tesla would introduce a self-driving taxi, Robotaxi, in August. If Tesla has in fact perfected a vehicle that can ferry passengers without a driver — which many analysts doubt — the development will help answer criticism that the company has been slow to follow up its Model 3 sedan and Model Y sport utility vehicle with new products.
Mr. Huang died after his Tesla Model X, a luxury S.U.V., veered from a highway in Mountain View, Calif., and smashed into a concrete median barrier. In the lawsuit, Mr. Huang’s family blamed defects in Autopilot, which it said lacked technology to avoid a crash. The lawsuit also sought damages from California, arguing that the barrier had been damaged and failed to absorb the impact of the car as it was supposed to.
Lawyers for Mr. Huang and Tesla did not respond to requests for comment late Monday. In legal filings, Tesla said it had settled “to end years of litigation.” The company had indicated in court documents that it planned to offer testimony that Mr. Huang had been playing a video game on his phone when the crash occurred. Lawyers for the family denied that was the case.
While Tesla calls this software Autopilot and a more advanced version Full Self-Driving, neither system makes a car fully autonomous. The systems can accelerate, brake, keep cars in their lanes and perform other functions to varying degrees, but drivers are required to stay engaged and be ready to intervene at any moment.
In December, Tesla recalled more than two million vehicles for a software update under pressure from U.S. regulators who said the automaker had not done enough to ensure that drivers remained attentive when using the systems.
The National Transportation Safety Board’s investigation into the 2018 crash blamed both Tesla and Mr. Huang. The agency said that Autopilot had failed to keep the vehicle in its lane and that its collision-avoidance software had failed to detect a highway barrier. The board also said Mr. Huang had probably been distracted.
Jack Ewing writes about the auto industry with an emphasis on electric vehicles. More about Jack Ewing
Tesla settles with Apple engineer’s family who said Autopilot caused his fatal crash
T esla has settled a high-profile case that was set to put the electric car company and its controversial automated-driving system on trial starting Monday.
Terms of the settlement were not disclosed. Jury selection was set to begin Monday in a wrongful death suit filed by the family of a former Apple engineer who died after his Tesla Model X crashed while the Autopilot feature was engaged. The trial could have lasted several weeks, but the parties settled Monday.
Walter Huang was killed when his Tesla struck a concrete highway median in Silicon Valley on March 23, 2018. The National Transportation Safety Board, in its investigation, found that Autopilot was engaged for nearly 19 minutes before the fatal crash, when the car, traveling at 71 mph, veered off the highway.
The settlement marks another crucial moment for an embattled company that has lost popularity and a third of its market value this year. CEO Elon Musk and the company say that its Autopilot and Full Self-Driving technologies are ahead of the competition and a big reason why Tesla has become the world’s largest electric vehicle maker — just ahead of Chinese rival BYD . But Huang’s family said Tesla oversold its Autopilot technology’s capabilities, and that it is not as safe to use as advertised.
Representatives for Huang’s family and Tesla did not immediately respond to requests for comment.
Tesla has come under intense scrutiny for its Autopilot technology over the six years since Huang’s fatal crash. After a two-year investigation that analyzed 1,000 Tesla crashes while vehicles had Autopilot engaged, the National Highway Traffic Safety Administration said the Autopilot system can give drivers a false sense of security. It can be easily misused in certain dangerous situations when Autopilot may be unable to safely navigate the road, NHTSA found in December 2023.
NHTSA and the National Transportation Safety Board have also been investigating crashes involving Tesla vehicles using the various driver assist features, including a series of crashes into emergency vehicles on the scene of other accidents.
Immediately following the December NHTSA report, Tesla recalled all 2 million of its cars in the United States, giving drivers more warnings when Autopilot is engaged and they are not paying attention to the road or placing their hands on the wheel.
Yet the company maintains that the technology is safe to use when used correctly and reduces fatalities. Autopilot requires drivers to keep their hands on the wheel and Tesla says people who use the automated-driving technology should keep their eyes on the road.
That didn’t happen in the case of Huang’s crash, Tesla has said. In a March 30, 2018, blog post , Tesla said Huang’s hands were not detected on his car’s steering wheel for six seconds prior to the crash. The company said it believes Huang was responsible for the crash because investigators found he was playing a video game on his phone while Autopilot was engaged. Huang did not brake or attempt to steer his car away from the concrete barrier before it crashed.
Although Huang’s family acknowledges he was distracted while the car was driving, they argued Tesla is at fault because it falsely marketed Autopilot as self-driving software. They alleged Tesla knew that Autopilot was not ready for prime time and had flaws that could make its use unsafe.
Tesla did not respond to a request for comment on the allegations.
“Mrs. Huang lost her husband, and two children lost their father because Tesla is beta testing its Autopilot software on live drivers,” said B. Mark Fong, the lawyer who brought the suit filed in California state court in a May 2019 complaint.
If a jury had found in favor of Huang’s family, Tesla could have been forced to pay damages, and they could have added up quickly. Wrongful death suits involving big companies have at times resulted in awards north of $1 billion.
Autopilot’s promise has also helped to boost Tesla’s stock in recent years to make it the most valuable automaker in the world — even as its stock is among the worst-performers in 2024. Musk in October 2023 on a call with analysts said autonomous driving “has the potential to make Tesla the most valuable company in the world by far.”
Tesla’s stock ( TSLA ) rose 5% Monday.
For more CNN news and newsletters create an account at CNN.com
Exclusive: Tesla scraps low-cost car plans amid fierce Chinese EV competition
- Medium Text
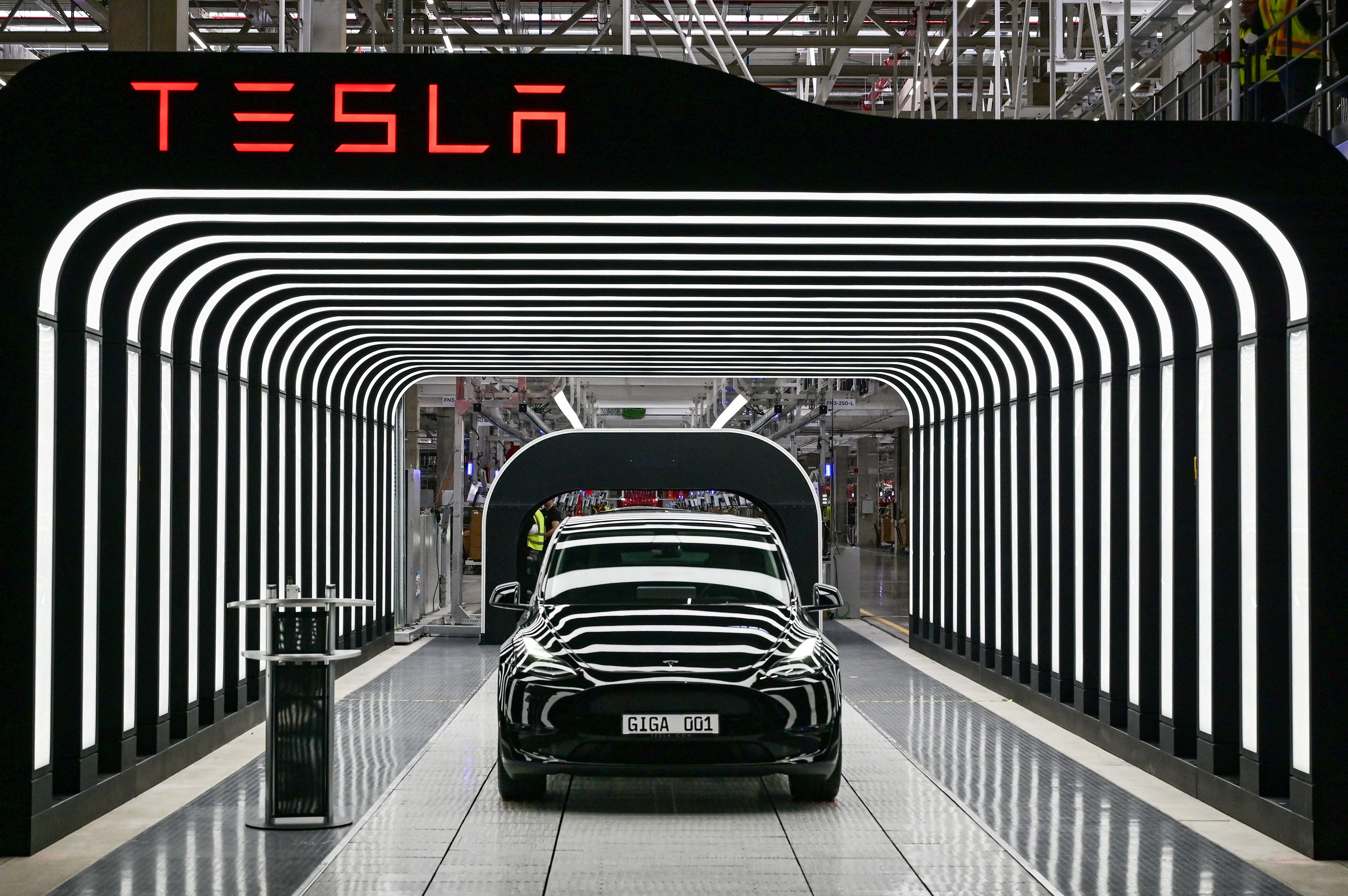
- Entry-level Tesla car won’t be built, three sources tell Reuters
- Tesla to focus on self-driving taxis instead, sources said
- Strategy shift comes as Tesla faces competition from China EV makers including BYD
‘HALT ALL FURTHER ACTIVITIES’
Running late.
Stay up to date with the latest news, trends and innovations that are driving the global automotive industry with the Reuters Auto File newsletter. Sign up here.
Reporting by Hyunjoo Jin in San Francisco, Norihiko Shirouzu in Austin and Ben Klayman in Detroit. Editing by Marla Dickerson and Brian Thevenot.
Our Standards: The Thomson Reuters Trust Principles. New Tab , opens new tab

Thomson Reuters
Is the Detroit Bureau Chief and North American Transportation Editor, responsible for a team of about 10 reporters covering everything from autos to aerospace to airlines to outer space.
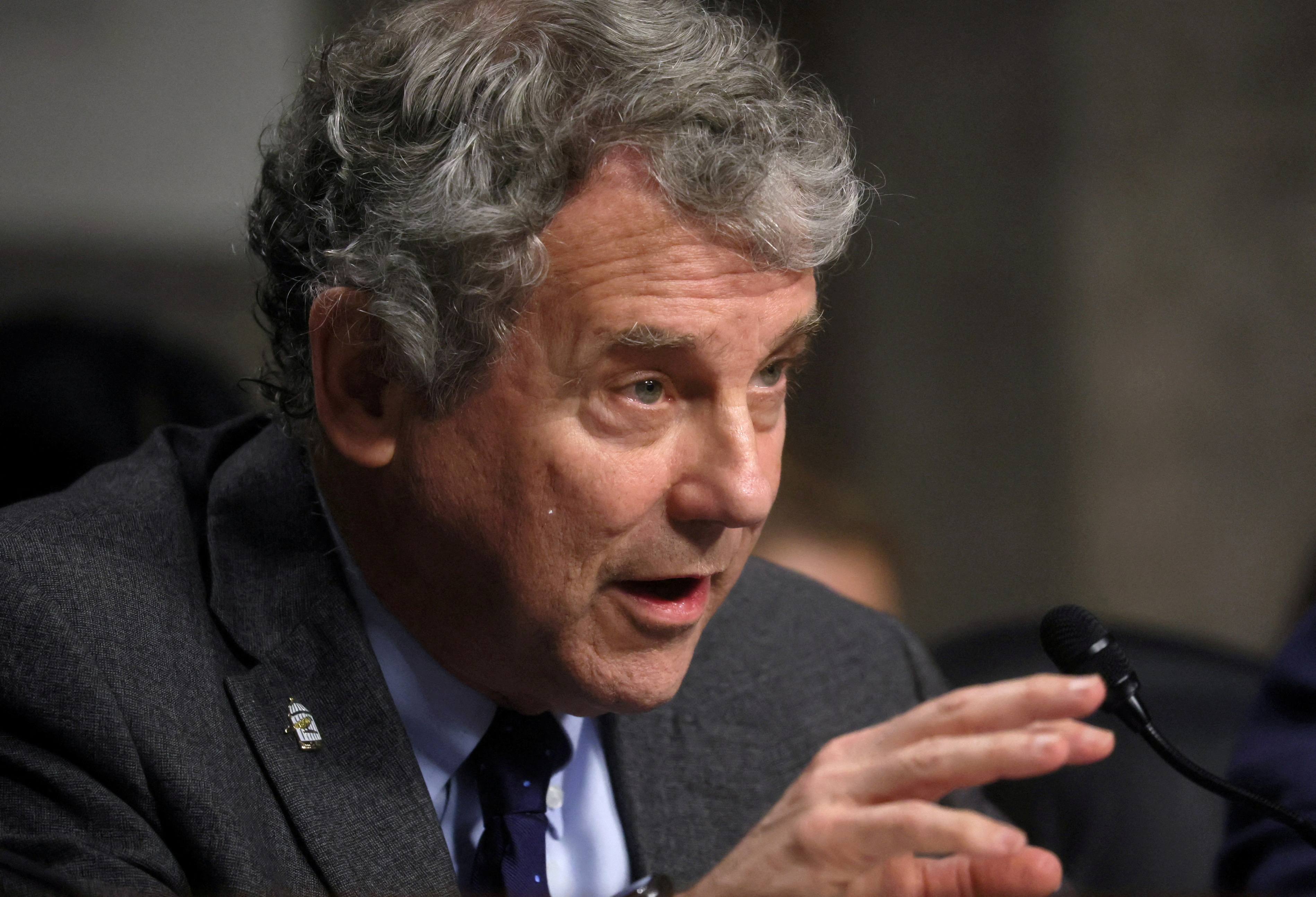
Business Chevron
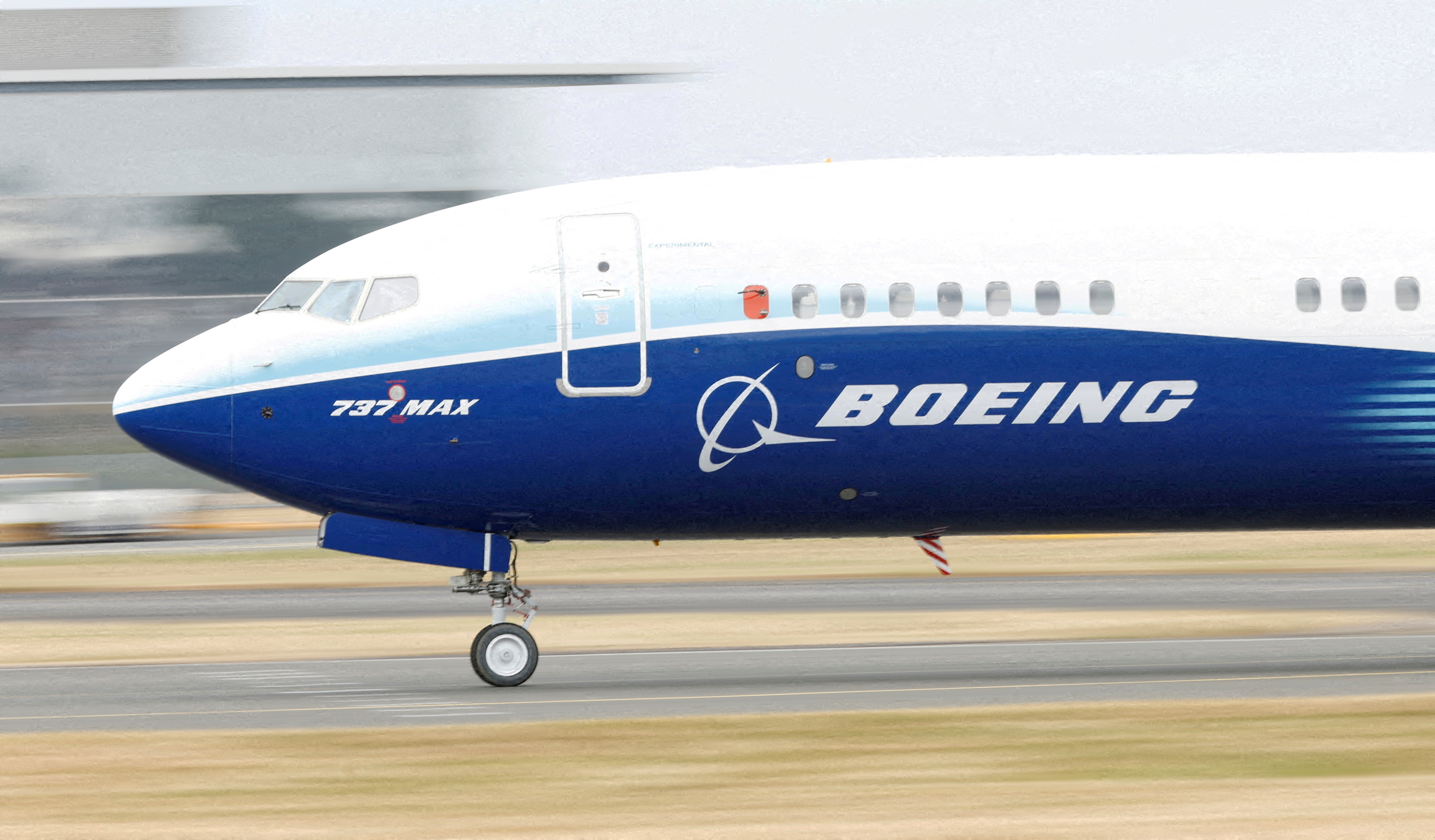
US Senate committee to hold hearing on Boeing safety culture report
The U.S. Senate Commerce Committee said on Thursday it would hold a hearing next week with members of an expert panel that released a report in February criticizing Boeing's safety culture and calling for significant improvements.
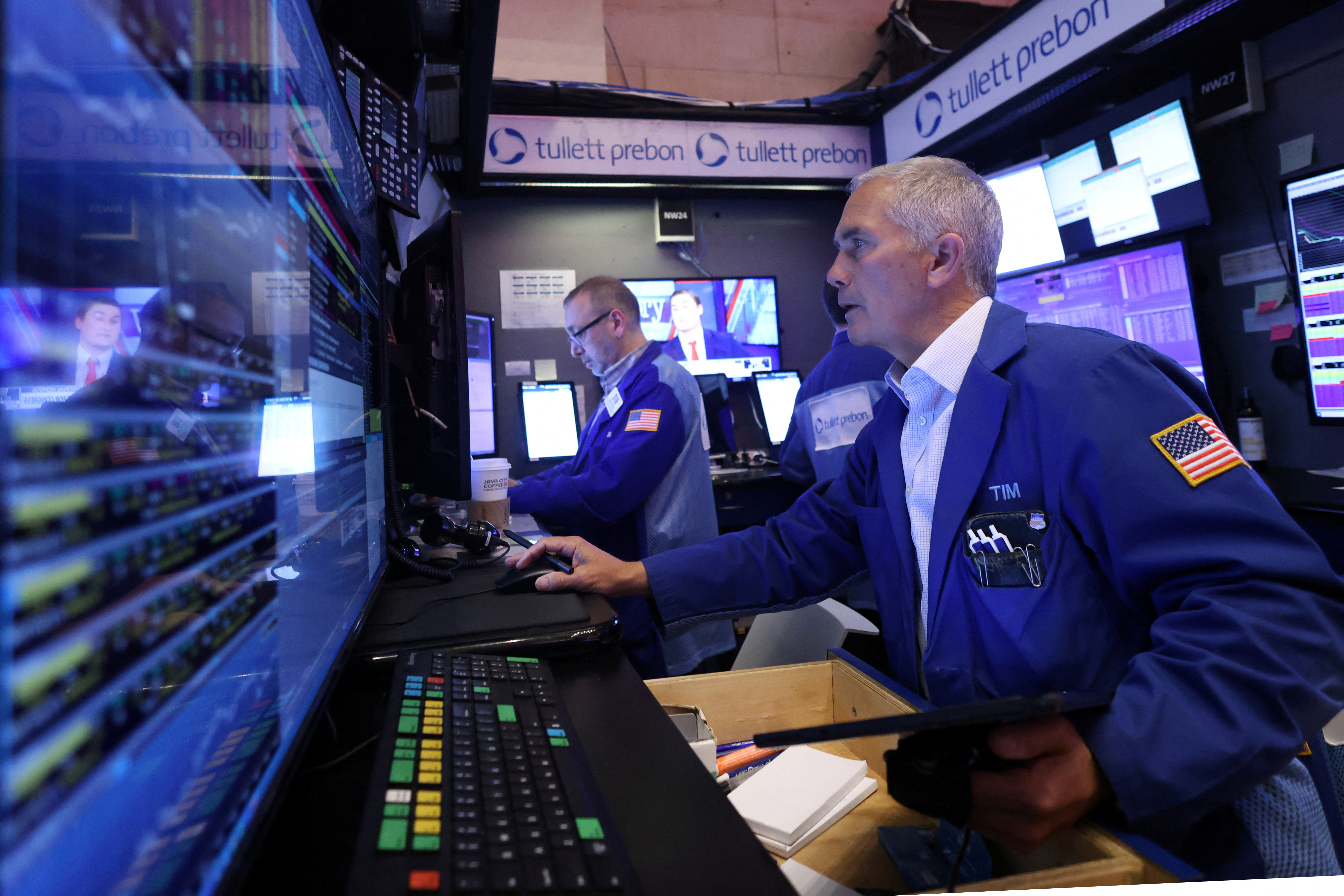
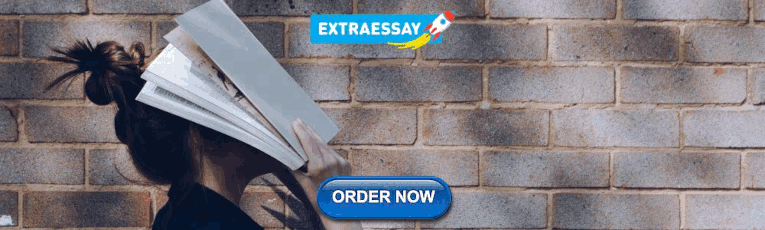
IMAGES
COMMENTS
Stanford professor and head of Google's self-driving car program, wrote: "I envision a future in which our technology is available to everyone, in every car. I envision a future without traffic accidents or congestion."7 Self-driving cars or autonomous vehicles (AVs) use sensors, computers and robotic actuators to
Essentially, a self-driving car needs to perform three actions to be able to replace a human driver: to perceive, to think and to act (Figure 1). These tasks are made possible by a network of high-tech devices such as cameras, computers and controllers. Figure 1: Like a human driver, a self-driving car executes a cycle of perceiving the ...
Waymo, the self-driving car division of Google, has ordered 82,000 self-driving cars for delivery through 2020. Cruise Automation, from General Motors, is perfecting its own fleet. Countless companies are driving full-throttle into the future. This case explores whether self-driving cars (autonomous vehicles or AVs) are a red ocean or blue ...
AV trucking increases driver safety while boosting efficiency beyond what is achieved by even the most proficient human driver. Take a look at some of the possible benefits, according to Deloitte ...
The dream of seeing fleets of driverless cars efficiently delivering people to their destinations has captured consumers' imaginations and fueled billions of dollars in investment in recent years.But even after some setbacks that have pushed out timelines for autonomous-vehicle (AV) launches and delayed customer adoption, the mobility community still broadly agrees that autonomous driving ...
10 are only realized once self-driving cars are adopted en masse. We investigate public attitudes 11 toward self-driving cars using the responses of 107 likely adopters in Berkeley, California as a 12 case study. What do these people find most and least attractive about self-driving cars, and how
Self-driving cars may also serve to increase the freedom and mobility of different subgroups of the population, including youth, elderly and disabled. Self-driving cars and peer-to-peer transportation services may eliminate the need to own a vehicle. The effect on total car use is hard to predict. Trips of empty vehicles and people's ...
Figure 1 exemplifies four traffic situations out of a total of 29 used in our study. In all situations, a self-driving (yellow) car was depicted between two other road users. ... In any case, even ...
Self-driving cars might soon have to make such ethical judgments on their own — but settling on a universal moral code for the vehicles could be a thorny task, suggests a survey of 2.3 million ...
A new study finds human supervisors have the potential to reduce barriers to deploying autonomous vehicles. ... Computers that power self-driving cars could be a huge driver of global carbon emissions. Researchers release open-source photorealistic simulator for autonomous driving . On the road to cleaner, greener, and faster driving ...
4. Continuous Learning: Self-driving cars using deep learning can continuously improve their performance through exposure to diverse scenarios and data. 5. Redundancy and Safety: Deep learning ...
Experiment Tracking for Systems Powering Self-Driving Vehicles [Case Study with Waabi] How do self-driving cars work? The first self-driving car was invented in 1989, it was the Automatic Land Vehicle in Neural Network (ALVINN). It used neural networks to detect lines, segment the environment, navigate itself, and drive.
Moral Machine is a project out of Massachusetts Institute of Technology that aims to take discussion of ethics and self-driving cars further "by providing a platform for 1) building a crowd ...
The introduction of self-driving vehicles gives rise to a large number of ethical issues that go beyond the common, extremely narrow, focus on improbable dilemma-like scenarios. This article provides a broad overview of realistic ethical issues related to self-driving vehicles. Some of the major topics covered are as follows: Strong opinions for and against driverless cars may give rise to ...
This research aims to explain the levels, technologies used, and the impacts and concerns related to the introduction of autonomous vehicles in India. Classification using deep learning and neural networks was done to categorize the collected data into 46 classes of which 43 consist of different road signs and the other 3 namely; unmetalled roads, potholes, and illegal parking. Our research is ...
AI used to be a fanciful concept from science fiction, but now it's becoming a daily reality. One of the most remarkable applications of Machine Learning is the self-driving or autonomous car .Machine learning allows self-driving cars to instantaneously adapt to changing road conditions, while at the same time learning from new road situations.
And image classification is a common way to get data for that. Safety and efficiency of self-driving cars rely on millions of correctly labeled data points. There are many people out on the roads, and all of them play a different role. To create a solution that predicts their behavior, you need a labeled dataset of images depicting them.
Here's a diagram showing what's "under the hood" of self-driving cars: Classic approach. First there's the perception component, which is responsible for detecting what's around the car. Then there are maps and the localization component, which determines exactly where the vehicle is located in the world. Information from these two components ...
Volvo Cars created a concept study for a self-driving car with four different modular interiors that could be built to order, depending on how the customer planned to use it: office, living room ...
Google's self-driving vehicles also identify heavy vehicles, Figure 14 a), and recogn ition of police cars by recognizin g the lights locate d above the car, Figure 14 b).
The case involves a fatal crash in March 2018, ... For example, a spokesperson for Waymo, the Alphabet-owned self-driving car company, said its vehicles operate only in areas that have been ...
Self Driving Car Case Study. 1241 Words5 Pages. What changes could self-driving cars bring in different sectors of the economy? The fundamental usage of transportation is going to change at the future. And now the world is in the inflection point of transportation. This will have implications in all the sections of the economy and this will ...
Lawyers for the family denied that was the case. While Tesla calls this software Autopilot and a more advanced version Full Self-Driving, neither system makes a car fully autonomous. The systems ...
Tesla has settled a high-profile case that was set to put the electric car company and its controversial automated-driving system on trial starting Monday.
Musk said on Friday that Tesla plans to unveil a self-driving robotaxi on August 8, after Reuters reported that Tesla scrapped an inexpensive car plan in favour of robotaxis.
The automaker faces lawsuits and investigations into crashes involving its Autopilot and Full Self-Driving driver-assistance systems, which are not fully autonomous. Tesla has blamed the accidents ...