Society Homepage About Public Health Policy Contact
Data-driven hypothesis generation in clinical research: what we learned from a human subject study, article sidebar.
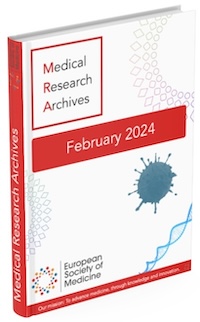
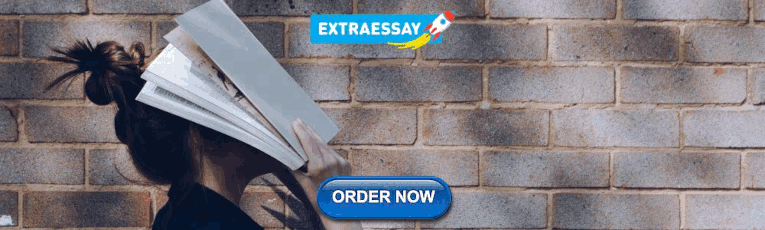
Submit your own article
Register as an author to reserve your spot in the next issue of the Medical Research Archives.
Join the Society
The European Society of Medicine is more than a professional association. We are a community. Our members work in countries across the globe, yet are united by a common goal: to promote health and health equity, around the world.
Join Europe’s leading medical society and discover the many advantages of membership, including free article publication.
Main Article Content
Hypothesis generation is an early and critical step in any hypothesis-driven clinical research project. Because it is not yet a well-understood cognitive process, the need to improve the process goes unrecognized. Without an impactful hypothesis, the significance of any research project can be questionable, regardless of the rigor or diligence applied in other steps of the study, e.g., study design, data collection, and result analysis. In this perspective article, the authors provide a literature review on the following topics first: scientific thinking, reasoning, medical reasoning, literature-based discovery, and a field study to explore scientific thinking and discovery. Over the years, scientific thinking has shown excellent progress in cognitive science and its applied areas: education, medicine, and biomedical research. However, a review of the literature reveals the lack of original studies on hypothesis generation in clinical research. The authors then summarize their first human participant study exploring data-driven hypothesis generation by clinical researchers in a simulated setting. The results indicate that a secondary data analytical tool, VIADS—a visual interactive analytic tool for filtering, summarizing, and visualizing large health data sets coded with hierarchical terminologies, can shorten the time participants need, on average, to generate a hypothesis and also requires fewer cognitive events to generate each hypothesis. As a counterpoint, this exploration also indicates that the quality ratings of the hypotheses thus generated carry significantly lower ratings for feasibility when applying VIADS. Despite its small scale, the study confirmed the feasibility of conducting a human participant study directly to explore the hypothesis generation process in clinical research. This study provides supporting evidence to conduct a larger-scale study with a specifically designed tool to facilitate the hypothesis-generation process among inexperienced clinical researchers. A larger study could provide generalizable evidence, which in turn can potentially improve clinical research productivity and overall clinical research enterprise.
Article Details
The Medical Research Archives grants authors the right to publish and reproduce the unrevised contribution in whole or in part at any time and in any form for any scholarly non-commercial purpose with the condition that all publications of the contribution include a full citation to the journal as published by the Medical Research Archives .
- Urgent Support
Engineering Graduate Studies
Hypothesis and Experimental Design
Jump to: Activity Examples | Resources
Two important elements of The Scientific Method that will help you design your research approach more efficiently are “Generating Hypotheses” and “Designing Controlled Experiments” to test these hypotheses. A well-designed experiment that you deeply understand will save time and resources and facilitate easier data analysis/interpretation. Many people reading this may be working on a project that focuses on designing a product, or discovery research where the hypothesis it is not immediately obvious. We encourage you to read on however as the exercise of generating a hypothesis will likely help you think about the assumptions you are making in your research and the physical principles your work builds upon.
These activities will help you …
- Begin formulating an appropriate hypothesis related to your research.
- Apply a systematic process for designing experiments.
What is a Hypothesis?
A hypothesis is an “educated guess/prediction” or “ proposed explanation ” of how a system will behave based on the available evidence . A hypothesis is a starting point for further investigation and testing because a hypothesis makes a prediction about the behavior of a measurable outcome of an experiment. A hypothesis should be:
- Testable – you can design an experiment to test it
- Falsifiable – it can be proven wrong (note it cannot be “proved”)
- Useful – the outcome must give valuable information
A useful hypothesis may relate to the underlying question of your research. For example:
“We hypothesize that therapy resistant cell populations will be enriched in hypoxic microenvironments. “
“We hypothesize that increasing the number of boreholes simulated in 3D geological models minimizes the variation of the geological model results.”
Some research projects do not have an obvious hypothesis to test, but the design strategy/concept chosen is based on an underlying assumption about how the system being designed works (i.e. the hypothesis). For example:
“We hypothesize that decreasing the baking temperature of the photoresist layer will reduce thermal expansion and device cracking”
In this case the researcher is troubleshooting poor device quality and is proposing to vary different fabrication parameters (one being baking temperature). Understanding the assumptions (working hypotheses) of why different variables might improve device quality is useful as it provides a basis to prioritize what variables to focus on first. The core goal of this research is not to test a specific hypothesis, but using the scientific method to troubleshoot a design challenge will enable the researcher to understand the parameters that control the behavior of different designs and to identify a design that is successful more efficiently.
In all the examples above, the hypothesis helps to guide the design of a useful and interpretable experiment with appropriate controls that rule out alternative explanations of the experimental observation. Hypotheses are therefore likely essential and useful parts of all research projects.
Suggested Activity – Create a Hypothesis for Your Research
Estimated time: 30 mins
- Write down the parameters you are varying or testing in your experimental system or model and how you think the behaviour of the system is going to vary with these parameters.
- (Alternative) If your project goal is to design a device, write down the parameters you believe control whether the device will work.
- (Alternative) If your project goal involves optimizing a process, write down the underlying physics or chemistry controlling the process you are studying.
- With these parameters in mind, write down the key assumption(s) you are making about how your system works.
- Try to formulate each one of these assumptions into a hypothesis that might be useful for your research project. If you have multiple aims each one may have a separate hypothesis. Make sure the hypothesis meets each of the three key elements above.
- Share your hypothesis with a peer or your supervisor to discuss if this is a good hypothesis – is it testable? Does it make a useful prediction? Does it capture the key underlying assumptions your research is based upon?
Remember that writing a good research hypothesis is challenging and will take a lot of careful thought about the underlying science that governs your system.
Designing Experiments
Designing experiments appropriately is very important to avoid wasting resources (time!) and to ensure results can be interpreted correctly. It is often very useful to discuss the design of your planned experiments in your meetings with your supervisor to get feedback before you start doing experiments. This will also ensure you and your supervisor have a consistent understanding of experimental design and that all the appropriate controls required to interpret your data have been considered.
The factors that must be considered when you design experiments is going to depend on your specific area of research. S ome important things to think about when designing experiments include:
Rationale: What is the purpose of this experiment? Is this the best experiment I can do? Does my experiment answer any question ? Does this experiment help answer the question I am trying to ask? What hypothesis am I trying to test?
Will my experiment be interpretable? What controls can I use to distinguish my results from other potential explanations? Can I add a control to distinguish between explanations? Can I add a control to further test my hypothesis?
Is my experiment/model rigorous? What is the sensitivity of the method I am using and can it measure accurately what I want to measure? What outcomes (metrics) will I measure and is this measurement appropriate? How many replicates (technical replicates versus independent replicates) will I do? Am I only changing the variable that I am testing? What am I keeping constant? What statistical tests do I plan to carry out and what considerations are needed? Is my statistical design appropriate (power analysis, sufficient replicates)?
What logistics do I need to consider? Are the equipment/resources I need available? Do I need additional training or equipment access? Are there important safety or ethical issues/permits to consider? Are pilot experiments needed to assess feasibility and what would these be? What is my planned experimental protocol and are there important timing issues to consider? What experimental outputs and parameters need to be documented throughout experiment?
This list is not exhaustive and you should consider what is missing for your particular situation.
Suggested Activity – Design an Experiment Using a Template
Estimated time: 45 min
- Explore the excel template for experimental design ( Resource 1 ) or modelling ( Resource 2 ). A template like this is very useful for keeping track of protocols as well as improving the reproducibility of your experiments. Note this template is simply a starting point to get you thinking systematically and should be adapted to best suit your needs.
- Fill out the template for an experiment or modelling project you are planning to complete soon.
- Consider how you can modify this template to be more applicable to your specific project.
- Using the template document, explain your experimental design/model design to a peer or your supervisor. Let them ask questions to understand your design and provide feedback. Alternatively, if there is a part of your design that you are unclear about this is a great starting point for a targeted and efficient discussion with your supervisor.
- Revise your design based on feedback.
Activity Examples
© 2024 Faculty of Applied Science and Engineering
- U of T Home
- Accessibility
- Student Data Practices
- Website Feedback
Have a language expert improve your writing
Run a free plagiarism check in 10 minutes, automatically generate references for free.
- Knowledge Base
- Methodology
Research Design | Step-by-Step Guide with Examples
Published on 5 May 2022 by Shona McCombes . Revised on 20 March 2023.
A research design is a strategy for answering your research question using empirical data. Creating a research design means making decisions about:
- Your overall aims and approach
- The type of research design you’ll use
- Your sampling methods or criteria for selecting subjects
- Your data collection methods
- The procedures you’ll follow to collect data
- Your data analysis methods
A well-planned research design helps ensure that your methods match your research aims and that you use the right kind of analysis for your data.
Table of contents
Step 1: consider your aims and approach, step 2: choose a type of research design, step 3: identify your population and sampling method, step 4: choose your data collection methods, step 5: plan your data collection procedures, step 6: decide on your data analysis strategies, frequently asked questions.
- Introduction
Before you can start designing your research, you should already have a clear idea of the research question you want to investigate.
There are many different ways you could go about answering this question. Your research design choices should be driven by your aims and priorities – start by thinking carefully about what you want to achieve.
The first choice you need to make is whether you’ll take a qualitative or quantitative approach.
Qualitative research designs tend to be more flexible and inductive , allowing you to adjust your approach based on what you find throughout the research process.
Quantitative research designs tend to be more fixed and deductive , with variables and hypotheses clearly defined in advance of data collection.
It’s also possible to use a mixed methods design that integrates aspects of both approaches. By combining qualitative and quantitative insights, you can gain a more complete picture of the problem you’re studying and strengthen the credibility of your conclusions.
Practical and ethical considerations when designing research
As well as scientific considerations, you need to think practically when designing your research. If your research involves people or animals, you also need to consider research ethics .
- How much time do you have to collect data and write up the research?
- Will you be able to gain access to the data you need (e.g., by travelling to a specific location or contacting specific people)?
- Do you have the necessary research skills (e.g., statistical analysis or interview techniques)?
- Will you need ethical approval ?
At each stage of the research design process, make sure that your choices are practically feasible.
Prevent plagiarism, run a free check.
Within both qualitative and quantitative approaches, there are several types of research design to choose from. Each type provides a framework for the overall shape of your research.
Types of quantitative research designs
Quantitative designs can be split into four main types. Experimental and quasi-experimental designs allow you to test cause-and-effect relationships, while descriptive and correlational designs allow you to measure variables and describe relationships between them.
With descriptive and correlational designs, you can get a clear picture of characteristics, trends, and relationships as they exist in the real world. However, you can’t draw conclusions about cause and effect (because correlation doesn’t imply causation ).
Experiments are the strongest way to test cause-and-effect relationships without the risk of other variables influencing the results. However, their controlled conditions may not always reflect how things work in the real world. They’re often also more difficult and expensive to implement.
Types of qualitative research designs
Qualitative designs are less strictly defined. This approach is about gaining a rich, detailed understanding of a specific context or phenomenon, and you can often be more creative and flexible in designing your research.
The table below shows some common types of qualitative design. They often have similar approaches in terms of data collection, but focus on different aspects when analysing the data.
Your research design should clearly define who or what your research will focus on, and how you’ll go about choosing your participants or subjects.
In research, a population is the entire group that you want to draw conclusions about, while a sample is the smaller group of individuals you’ll actually collect data from.
Defining the population
A population can be made up of anything you want to study – plants, animals, organisations, texts, countries, etc. In the social sciences, it most often refers to a group of people.
For example, will you focus on people from a specific demographic, region, or background? Are you interested in people with a certain job or medical condition, or users of a particular product?
The more precisely you define your population, the easier it will be to gather a representative sample.
Sampling methods
Even with a narrowly defined population, it’s rarely possible to collect data from every individual. Instead, you’ll collect data from a sample.
To select a sample, there are two main approaches: probability sampling and non-probability sampling . The sampling method you use affects how confidently you can generalise your results to the population as a whole.
Probability sampling is the most statistically valid option, but it’s often difficult to achieve unless you’re dealing with a very small and accessible population.
For practical reasons, many studies use non-probability sampling, but it’s important to be aware of the limitations and carefully consider potential biases. You should always make an effort to gather a sample that’s as representative as possible of the population.
Case selection in qualitative research
In some types of qualitative designs, sampling may not be relevant.
For example, in an ethnography or a case study, your aim is to deeply understand a specific context, not to generalise to a population. Instead of sampling, you may simply aim to collect as much data as possible about the context you are studying.
In these types of design, you still have to carefully consider your choice of case or community. You should have a clear rationale for why this particular case is suitable for answering your research question.
For example, you might choose a case study that reveals an unusual or neglected aspect of your research problem, or you might choose several very similar or very different cases in order to compare them.
Data collection methods are ways of directly measuring variables and gathering information. They allow you to gain first-hand knowledge and original insights into your research problem.
You can choose just one data collection method, or use several methods in the same study.
Survey methods
Surveys allow you to collect data about opinions, behaviours, experiences, and characteristics by asking people directly. There are two main survey methods to choose from: questionnaires and interviews.
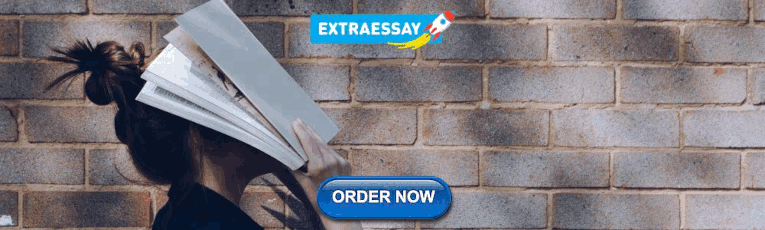
Observation methods
Observations allow you to collect data unobtrusively, observing characteristics, behaviours, or social interactions without relying on self-reporting.
Observations may be conducted in real time, taking notes as you observe, or you might make audiovisual recordings for later analysis. They can be qualitative or quantitative.
Other methods of data collection
There are many other ways you might collect data depending on your field and topic.
If you’re not sure which methods will work best for your research design, try reading some papers in your field to see what data collection methods they used.
Secondary data
If you don’t have the time or resources to collect data from the population you’re interested in, you can also choose to use secondary data that other researchers already collected – for example, datasets from government surveys or previous studies on your topic.
With this raw data, you can do your own analysis to answer new research questions that weren’t addressed by the original study.
Using secondary data can expand the scope of your research, as you may be able to access much larger and more varied samples than you could collect yourself.
However, it also means you don’t have any control over which variables to measure or how to measure them, so the conclusions you can draw may be limited.
As well as deciding on your methods, you need to plan exactly how you’ll use these methods to collect data that’s consistent, accurate, and unbiased.
Planning systematic procedures is especially important in quantitative research, where you need to precisely define your variables and ensure your measurements are reliable and valid.
Operationalisation
Some variables, like height or age, are easily measured. But often you’ll be dealing with more abstract concepts, like satisfaction, anxiety, or competence. Operationalisation means turning these fuzzy ideas into measurable indicators.
If you’re using observations , which events or actions will you count?
If you’re using surveys , which questions will you ask and what range of responses will be offered?
You may also choose to use or adapt existing materials designed to measure the concept you’re interested in – for example, questionnaires or inventories whose reliability and validity has already been established.
Reliability and validity
Reliability means your results can be consistently reproduced , while validity means that you’re actually measuring the concept you’re interested in.
For valid and reliable results, your measurement materials should be thoroughly researched and carefully designed. Plan your procedures to make sure you carry out the same steps in the same way for each participant.
If you’re developing a new questionnaire or other instrument to measure a specific concept, running a pilot study allows you to check its validity and reliability in advance.
Sampling procedures
As well as choosing an appropriate sampling method, you need a concrete plan for how you’ll actually contact and recruit your selected sample.
That means making decisions about things like:
- How many participants do you need for an adequate sample size?
- What inclusion and exclusion criteria will you use to identify eligible participants?
- How will you contact your sample – by mail, online, by phone, or in person?
If you’re using a probability sampling method, it’s important that everyone who is randomly selected actually participates in the study. How will you ensure a high response rate?
If you’re using a non-probability method, how will you avoid bias and ensure a representative sample?
Data management
It’s also important to create a data management plan for organising and storing your data.
Will you need to transcribe interviews or perform data entry for observations? You should anonymise and safeguard any sensitive data, and make sure it’s backed up regularly.
Keeping your data well organised will save time when it comes to analysing them. It can also help other researchers validate and add to your findings.
On their own, raw data can’t answer your research question. The last step of designing your research is planning how you’ll analyse the data.
Quantitative data analysis
In quantitative research, you’ll most likely use some form of statistical analysis . With statistics, you can summarise your sample data, make estimates, and test hypotheses.
Using descriptive statistics , you can summarise your sample data in terms of:
- The distribution of the data (e.g., the frequency of each score on a test)
- The central tendency of the data (e.g., the mean to describe the average score)
- The variability of the data (e.g., the standard deviation to describe how spread out the scores are)
The specific calculations you can do depend on the level of measurement of your variables.
Using inferential statistics , you can:
- Make estimates about the population based on your sample data.
- Test hypotheses about a relationship between variables.
Regression and correlation tests look for associations between two or more variables, while comparison tests (such as t tests and ANOVAs ) look for differences in the outcomes of different groups.
Your choice of statistical test depends on various aspects of your research design, including the types of variables you’re dealing with and the distribution of your data.
Qualitative data analysis
In qualitative research, your data will usually be very dense with information and ideas. Instead of summing it up in numbers, you’ll need to comb through the data in detail, interpret its meanings, identify patterns, and extract the parts that are most relevant to your research question.
Two of the most common approaches to doing this are thematic analysis and discourse analysis .
There are many other ways of analysing qualitative data depending on the aims of your research. To get a sense of potential approaches, try reading some qualitative research papers in your field.
A sample is a subset of individuals from a larger population. Sampling means selecting the group that you will actually collect data from in your research.
For example, if you are researching the opinions of students in your university, you could survey a sample of 100 students.
Statistical sampling allows you to test a hypothesis about the characteristics of a population. There are various sampling methods you can use to ensure that your sample is representative of the population as a whole.
Operationalisation means turning abstract conceptual ideas into measurable observations.
For example, the concept of social anxiety isn’t directly observable, but it can be operationally defined in terms of self-rating scores, behavioural avoidance of crowded places, or physical anxiety symptoms in social situations.
Before collecting data , it’s important to consider how you will operationalise the variables that you want to measure.
The research methods you use depend on the type of data you need to answer your research question .
- If you want to measure something or test a hypothesis , use quantitative methods . If you want to explore ideas, thoughts, and meanings, use qualitative methods .
- If you want to analyse a large amount of readily available data, use secondary data. If you want data specific to your purposes with control over how they are generated, collect primary data.
- If you want to establish cause-and-effect relationships between variables , use experimental methods. If you want to understand the characteristics of a research subject, use descriptive methods.
Cite this Scribbr article
If you want to cite this source, you can copy and paste the citation or click the ‘Cite this Scribbr article’ button to automatically add the citation to our free Reference Generator.
McCombes, S. (2023, March 20). Research Design | Step-by-Step Guide with Examples. Scribbr. Retrieved 22 April 2024, from https://www.scribbr.co.uk/research-methods/research-design/
Is this article helpful?
Shona McCombes
Hypothesis Generation and Interpretation
Design Principles and Patterns for Big Data Applications
- © 2024
- Hiroshi Ishikawa 0
Department of Systems Design, Tokyo Metropolitan University, Hino, Japan
You can also search for this author in PubMed Google Scholar
- Provides an integrated perspective on why decisions are made and how the process is modeled
- Presentation of design patterns enables use in a wide variety of big-data applications
- Multiple practical use cases indicate the broad real-world significance of the methods presented
Part of the book series: Studies in Big Data (SBD, volume 139)
1163 Accesses
This is a preview of subscription content, log in via an institution to check access.
Access this book
- Available as EPUB and PDF
- Read on any device
- Instant download
- Own it forever
- Durable hardcover edition
- Dispatched in 3 to 5 business days
- Free shipping worldwide - see info
Tax calculation will be finalised at checkout
Other ways to access
Licence this eBook for your library
Institutional subscriptions
Table of contents (8 chapters)
Front matter, basic concept.
Hiroshi Ishikawa
Science and Hypothesis
Machine learning and integrated approach, hypothesis generation by difference, methods for integrated hypothesis generation, interpretation, back matter.
- Hypothesis Generation
- Hypothesis Interpretation
- Data Engineering
- Data Science
- Data Management
- Machine Learning
- Data Mining
- Design Patterns
- Design Principles
About this book
The novel methods and technologies proposed in Hypothesis Generation and Interpretation are supported by the incorporation of historical perspectives on science and an emphasis on the origin and development of the ideas behind their design principles and patterns.
Authors and Affiliations
About the author.
He has published actively in international, refereed journals and conferences, such as ACM Transactions on Database Systems , IEEE Transactions on Knowledge and Data Engineering , The VLDB Journal , IEEE International Conference on Data Engineering, and ACM SIGSPATIAL and Management of Emergent Digital EcoSystems (MEDES). He has authored and co-authored a dozen books, including Social Big Data Mining (CRC, 2015) and Object-Oriented Database System (Springer-Verlag, 1993).
Bibliographic Information
Book Title : Hypothesis Generation and Interpretation
Book Subtitle : Design Principles and Patterns for Big Data Applications
Authors : Hiroshi Ishikawa
Series Title : Studies in Big Data
DOI : https://doi.org/10.1007/978-3-031-43540-9
Publisher : Springer Cham
eBook Packages : Computer Science , Computer Science (R0)
Copyright Information : The Editor(s) (if applicable) and The Author(s), under exclusive license to Springer Nature Switzerland AG 2024
Hardcover ISBN : 978-3-031-43539-3 Published: 02 February 2024
Softcover ISBN : 978-3-031-43542-3 Due: 31 January 2024
eBook ISBN : 978-3-031-43540-9 Published: 01 January 2024
Series ISSN : 2197-6503
Series E-ISSN : 2197-6511
Edition Number : 1
Number of Pages : XII, 372
Number of Illustrations : 52 b/w illustrations, 125 illustrations in colour
Topics : Theory of Computation , Database Management , Data Mining and Knowledge Discovery , Machine Learning , Big Data , Complex Systems
- Publish with us
Policies and ethics
- Find a journal
- Track your research

An official website of the United States government
The .gov means it’s official. Federal government websites often end in .gov or .mil. Before sharing sensitive information, make sure you’re on a federal government site.
The site is secure. The https:// ensures that you are connecting to the official website and that any information you provide is encrypted and transmitted securely.
- Publications
- Account settings
Preview improvements coming to the PMC website in October 2024. Learn More or Try it out now .
- Advanced Search
- Journal List
- Acta Orthop
- v.90(4); 2019 Aug
Hypothesis-generating and confirmatory studies, Bonferroni correction, and pre-specification of trial endpoints
A p-value presents the outcome of a statistically tested null hypothesis. It indicates how incompatible observed data are with a statistical model defined by a null hypothesis. This hypothesis can, for example, be that 2 parameters have identical values, or that they differ by a specified amount. A low p-value shows that it is unlikely (a high p-value that it is not unlikely) that the observed data are consistent with the null hypothesis. Many null hypotheses are tested in order to generate study hypotheses for further research, others to confirm an already established study hypothesis. The difference between generating and confirming a hypothesis is crucial for the interpretation of the results. Presenting an outcome from a hypothesis-generating study as if it had been produced in a confirmatory study is misleading and represents methodological ignorance or scientific misconduct.
Hypothesis-generating studies differ methodologically from confirmatory studies. A generated hypothesis must be confirmed in a new study. An experiment is usually required for confirmation as an observational study cannot provide unequivocal results. For example, selection and confounding bias can be prevented by randomization and blinding in a clinical trial, but not in an observational study. Confirmatory studies, but not hypothesis-generating studies, also require control of the inflation in the false-positive error risk that is caused by testing multiple null hypotheses. The phenomenon is known as a multiplicity or mass-significance effect. A method for correcting the significance level for the multiplicity effect has been devised by the Italian mathematician Carlo Emilio Bonferroni. The correction (Bender and Lange 2001 ) is often misused in hypothesis-generating studies, often ignored when designing confirmatory studies (which results in underpowered studies), and often inadequately used in laboratory studies, for example when an investigator corrects the significance level for comparing 3 experimental groups by lowering it to 0.05/3 = 0. 017 and believes that this solves the problem of testing 50 null hypotheses, which would have required a corrected significance level of 0.05/50 = 0.001.
In a confirmatory study, it is mandatory to show that the tested hypothesis has been pre-specified. A study protocol or statistical analysis plan should therefore be enclosed with the study report when submitted to a scientific journal for publication. Since 2005 the ICMJE (International Committee of Medical Journal Editors) and the WHO also require registration of clinical trials and their endpoints in a publicly accessible register before enrollment of the first participant. Changing endpoints in a randomized trial after its initiation can in some cases be acceptable, but this is never a trivial problem (Evans 2007 ) and must always be described to the reader. Many authors do not understand the importance of pre-specification and desist from registering their trial, use vague or ambiguous endpoint definitions, redefine the primary endpoint during the analysis, switch primary and secondary outcomes, or present completely new endpoints without mentioning this to the reader. Such publications are simply not credible, but are nevertheless surprisingly common (Ramagopalan et al. 2014 ) even in high impact factor journals (Goldacre et al. 2019 ). A serious editorial evaluation of manuscripts presenting confirmatory results should always include a verification of the endpoint’s pre-specification.
Hypothesis-generating studies are much more common than confirmatory, because the latter are logistically more complex, more laborious, more time-consuming, more expensive, and require more methodological expertise. However, the result of a hypothesis-generating study is just a hypothesis. A hypothesis cannot be generated and confirmed in the same study, and it cannot be confirmed with a new hypothesis-generating study. Confirmatory studies are essential for scientific progress.
- Bender R, Lange S. Adjusting for multiple testing: when and how? J Clin Epidemiol 2001; 54 : 343–9. [ PubMed ] [ Google Scholar ]
- Evans S. When and how can endpoints be changed after initiation of a randomized clinical trial? PLoS Clin Trials 2007; 2 : e18. [ PMC free article ] [ PubMed ] [ Google Scholar ]
- Goldacre B, Drysdale H, Milosevic I, Slade E, Hartley P, Marston C, Powell-Smith A, Heneghan C, Mahtani K R. COMPare: a prospective cohort study correcting and monitoring 58 misreported trials in real time . Trials 2019; 20 : 118. [ PMC free article ] [ PubMed ] [ Google Scholar ]
- Ramagopalan S, Skingsley A P, Handunnetthi L, Klingel M, Magnus D, Pakpoor J, Goldacre B. Prevalence of primary outcome changes in clinical trials registered on ClinicalTrials.gov: a cross-sectional study . F1000Research 2014, 3 : 77. [ PMC free article ] [ PubMed ] [ Google Scholar ]
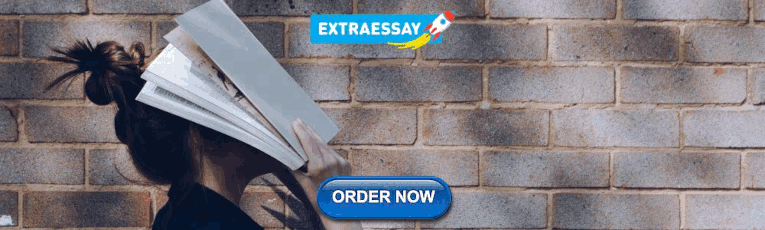
IMAGES
VIDEO
COMMENTS
1. An Overview. Broadly, preclinical research can be classified into two distinct categories depending on the aim and purpose of the experiment, namely, "hypothesis generating" (exploratory) and "hypothesis testing" (confirmatory) research (Fig. 1).Hypothesis generating studies are often scientifically-informed, curiosity and intuition-driven explorations which may generate testable ...
Formulating Hypotheses for Different Study Designs. Generating a testable working hypothesis is the first step towards conducting original research. Such research may prove or disprove the proposed hypothesis. Case reports, case series, online surveys and other observational studies, clinical trials, and narrative reviews help to generate ...
Hypothesis generation is an early and critical step in any hypothesis-driven clinical research project. Because it is not yet a well-understood cognitive process, the need to improve the process goes unrecognized. Without an impactful hypothesis, the significance of any research project can be questionable, regardless of the rigor or diligence applied in other steps of the study, e.g., study ...
Broadly, preclinical research can be classified into two distinct categories depending on the aim and purpose of the experiment, namely, "hypothesis generating" (exploratory) and "hypothesis testing" (confirmatory) research (Fig. 1).Hypothesis generating studies are often scientifically-informed, curiosity and intuition-driven explorations which may generate testable theories regarding ...
analysis tool, and the experience levels of study participants during their scientific hypothesis generation processes. Methods: Inexperienced and experienced clinical researchers are recruited. In this 2×2 study design, all participants use the same data sets during scientific hypothesis-generation sessions, following pre-determined scripts.
Hypothesis Testing and Hypothesis Generating Research fruitful avenue for future research endeavors. Such studies will help us narrow the ... Of course, failure to replicate research results with such a design opens the door to numerous possible explanations (the theory is wrong, the measures are faulty or conceptually different, contingencies ...
Abstract. Rigorous research investigation requires a thorough review of the literature on the topic of interest. This promotes development of an original, relevant and feasible hypothesis. Design of an optimal study to test the hypothesis then requires adequate power, freedom from bias, and conduct within a reasonable timeframe with resources ...
naturally occurring event or a proposed outcome of an intervention. 1,2. Hypothesis testing requires choosing the most ap propriate methodology and adequately. powering statistically the study to ...
A hypothesis is a starting point for further investigation and testing because a hypothesis makes a prediction about the behavior of a measurable outcome of an experiment. A hypothesis should be: Testable - you can design an experiment to test it. Falsifiable - it can be proven wrong (note it cannot be "proved")
Introduction. In clinical research, our aim is to design a study, which would be able to derive a valid and meaningful scientific conclusion using appropriate statistical methods that can be translated to the "real world" setting. 1 Before choosing a study design, one must establish aims and objectives of the study, and choose an appropriate target population that is most representative of ...
This chapter argues that the rigor of a study is determined by its ability to persuade skeptics and that researchers should distinguish more clearly between exploratory, data-driven, hypothesis-generating research and confirmatory, theory-driven, hypothesis testing research. Rigorously designed and executed confirmatory studies propel scientific progress by resolving theoretical disagreements.
Study Design Designing a research study is not a simple task. Just as the key elements and ... viewed as hypothesis-generating analyses. Studies carried out in such manner are correctly clas-sified as hypothesis-generating studies. Therefore, once hypotheses are generated, these can then be tested in new, specifically designed studies. ...
5. Phrase your hypothesis in three ways. To identify the variables, you can write a simple prediction in if…then form. The first part of the sentence states the independent variable and the second part states the dependent variable. If a first-year student starts attending more lectures, then their exam scores will improve.
Table of contents. Step 1: Consider your aims and approach. Step 2: Choose a type of research design. Step 3: Identify your population and sampling method. Step 4: Choose your data collection methods. Step 5: Plan your data collection procedures. Step 6: Decide on your data analysis strategies.
A research design is a strategy for answering your research question using empirical data. Creating a research design means making decisions about: Your overall research objectives and approach. Whether you'll rely on primary research or secondary research. Your sampling methods or criteria for selecting subjects. Your data collection methods.
The basic science hypothesis-testing paradigm. The classical paradigm for basic biological research has been to develop a specific hypothesis that can be tested by the application of a prospectively defined experiment (see Box 1).I suggest that one of the major (although not the only) factors that led to the development of this paradigm is that experimental design was limited by the throughput ...
The author uses patterns discovered in a collection of big data applications to provide design principles for hypothesis generation, integrating big data processing and management, machine learning and data mining techniques. ... (those based on applied processes and model generation). Practical case studies are used to demonstrate how ...
After the generation of a hypothesis, it is equally important to appropriately design and adequately power a study (by ensuring a sufficient sample size) in order to test the hypothesis.
Consequently, these objectives determine the study design and research outcome. The development of research questions is a process based on knowledge of current trends, cutting-edge studies, and technological advances in the research field. ... Hypothesis-generating research to establish a framework for hypothesis testing (qualitative research)
Descriptive, observational study designs are useful for only generating hypothesis whereas, analytical, observational study designs are helpful for both generating and testing hypothesis.
This model flows in the following steps: -. Hypothesis generation: qualitative research to identify potentials of behavioral change regarding energy consumption. The qualitative expressions are based on data that includes semi-structured interviews, observations and social experiments. The energy-saving benefits are considered without a ...
Hypothesis-generating studies differ methodologically from confirmatory studies. A generated hypothesis must be confirmed in a new study. An experiment is usually required for confirmation as an observational study cannot provide unequivocal results. For example, selection and confounding bias can be prevented by randomization and blinding in a ...