Sampling Methods In Reseach: Types, Techniques, & Examples
Saul Mcleod, PhD
Editor-in-Chief for Simply Psychology
BSc (Hons) Psychology, MRes, PhD, University of Manchester
Saul Mcleod, PhD., is a qualified psychology teacher with over 18 years of experience in further and higher education. He has been published in peer-reviewed journals, including the Journal of Clinical Psychology.
Learn about our Editorial Process
Olivia Guy-Evans, MSc
Associate Editor for Simply Psychology
BSc (Hons) Psychology, MSc Psychology of Education
Olivia Guy-Evans is a writer and associate editor for Simply Psychology. She has previously worked in healthcare and educational sectors.
On This Page:
Sampling methods in psychology refer to strategies used to select a subset of individuals (a sample) from a larger population, to study and draw inferences about the entire population. Common methods include random sampling, stratified sampling, cluster sampling, and convenience sampling. Proper sampling ensures representative, generalizable, and valid research results.
- Sampling : the process of selecting a representative group from the population under study.
- Target population : the total group of individuals from which the sample might be drawn.
- Sample: a subset of individuals selected from a larger population for study or investigation. Those included in the sample are termed “participants.”
- Generalizability : the ability to apply research findings from a sample to the broader target population, contingent on the sample being representative of that population.
For instance, if the advert for volunteers is published in the New York Times, this limits how much the study’s findings can be generalized to the whole population, because NYT readers may not represent the entire population in certain respects (e.g., politically, socio-economically).
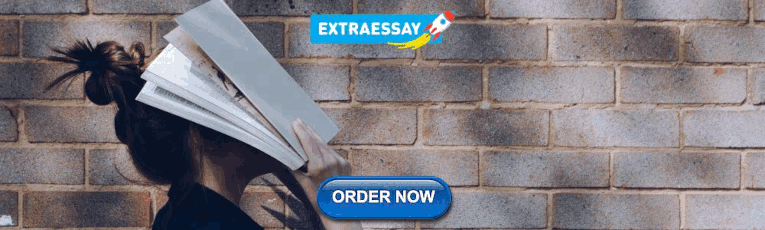
The Purpose of Sampling
We are interested in learning about large groups of people with something in common in psychological research. We call the group interested in studying our “target population.”
In some types of research, the target population might be as broad as all humans. Still, in other types of research, the target population might be a smaller group, such as teenagers, preschool children, or people who misuse drugs.
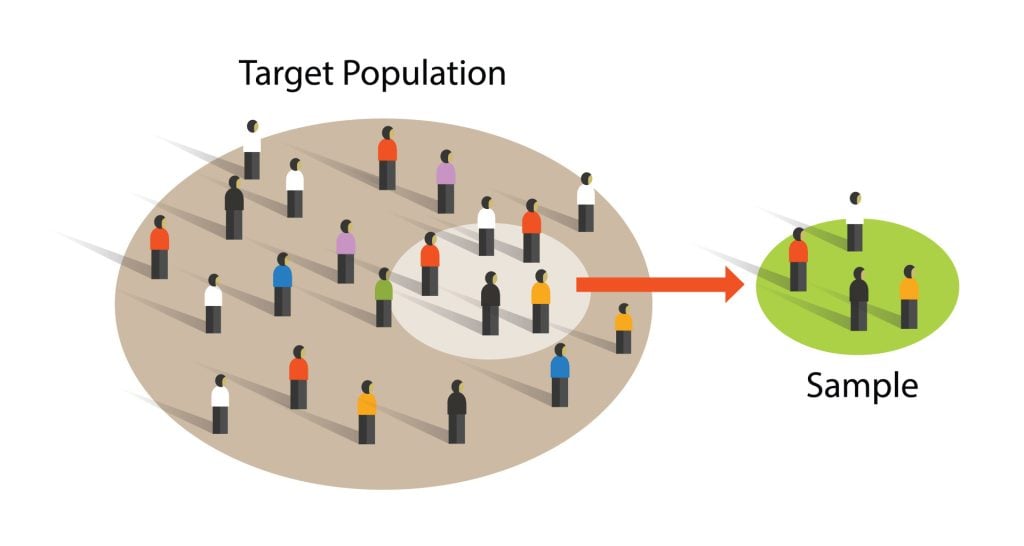
Studying every person in a target population is more or less impossible. Hence, psychologists select a sample or sub-group of the population that is likely to be representative of the target population we are interested in.
This is important because we want to generalize from the sample to the target population. The more representative the sample, the more confident the researcher can be that the results can be generalized to the target population.
One of the problems that can occur when selecting a sample from a target population is sampling bias. Sampling bias refers to situations where the sample does not reflect the characteristics of the target population.
Many psychology studies have a biased sample because they have used an opportunity sample that comprises university students as their participants (e.g., Asch ).
OK, so you’ve thought up this brilliant psychological study and designed it perfectly. But who will you try it out on, and how will you select your participants?
There are various sampling methods. The one chosen will depend on a number of factors (such as time, money, etc.).
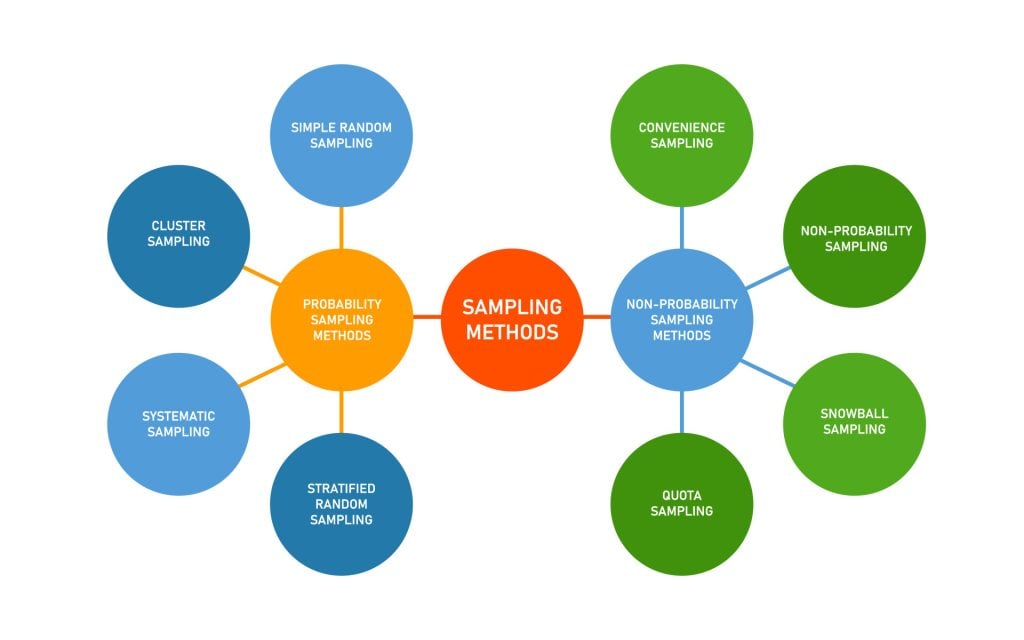
Random Sampling
Random sampling is a type of probability sampling where everyone in the entire target population has an equal chance of being selected.
This is similar to the national lottery. If the “population” is everyone who bought a lottery ticket, then everyone has an equal chance of winning the lottery (assuming they all have one ticket each).
Random samples require naming or numbering the target population and then using some raffle method to choose those to make up the sample. Random samples are the best method of selecting your sample from the population of interest.
- The advantages are that your sample should represent the target population and eliminate sampling bias.
- The disadvantage is that it is very difficult to achieve (i.e., time, effort, and money).
Stratified Sampling
During stratified sampling , the researcher identifies the different types of people that make up the target population and works out the proportions needed for the sample to be representative.
A list is made of each variable (e.g., IQ, gender, etc.) that might have an effect on the research. For example, if we are interested in the money spent on books by undergraduates, then the main subject studied may be an important variable.
For example, students studying English Literature may spend more money on books than engineering students, so if we use a large percentage of English students or engineering students, our results will not be accurate.
We have to determine the relative percentage of each group at a university, e.g., Engineering 10%, Social Sciences 15%, English 20%, Sciences 25%, Languages 10%, Law 5%, and Medicine 15%. The sample must then contain all these groups in the same proportion as the target population (university students).
- The disadvantage of stratified sampling is that gathering such a sample would be extremely time-consuming and difficult to do. This method is rarely used in Psychology.
- However, the advantage is that the sample should be highly representative of the target population, and therefore we can generalize from the results obtained.
Opportunity Sampling
Opportunity sampling is a method in which participants are chosen based on their ease of availability and proximity to the researcher, rather than using random or systematic criteria. It’s a type of convenience sampling .
An opportunity sample is obtained by asking members of the population of interest if they would participate in your research. An example would be selecting a sample of students from those coming out of the library.
- This is a quick and easy way of choosing participants (advantage)
- It may not provide a representative sample and could be biased (disadvantage).
Systematic Sampling
Systematic sampling is a method where every nth individual is selected from a list or sequence to form a sample, ensuring even and regular intervals between chosen subjects.
Participants are systematically selected (i.e., orderly/logical) from the target population, like every nth participant on a list of names.
To take a systematic sample, you list all the population members and then decide upon a sample you would like. By dividing the number of people in the population by the number of people you want in your sample, you get a number we will call n.
If you take every nth name, you will get a systematic sample of the correct size. If, for example, you wanted to sample 150 children from a school of 1,500, you would take every 10th name.
- The advantage of this method is that it should provide a representative sample.
Sample size
The sample size is a critical factor in determining the reliability and validity of a study’s findings. While increasing the sample size can enhance the generalizability of results, it’s also essential to balance practical considerations, such as resource constraints and diminishing returns from ever-larger samples.
Reliability and Validity
Reliability refers to the consistency and reproducibility of research findings across different occasions, researchers, or instruments. A small sample size may lead to inconsistent results due to increased susceptibility to random error or the influence of outliers. In contrast, a larger sample minimizes these errors, promoting more reliable results.
Validity pertains to the accuracy and truthfulness of research findings. For a study to be valid, it should accurately measure what it intends to do. A small, unrepresentative sample can compromise external validity, meaning the results don’t generalize well to the larger population. A larger sample captures more variability, ensuring that specific subgroups or anomalies don’t overly influence results.
Practical Considerations
Resource Constraints : Larger samples demand more time, money, and resources. Data collection becomes more extensive, data analysis more complex, and logistics more challenging.
Diminishing Returns : While increasing the sample size generally leads to improved accuracy and precision, there’s a point where adding more participants yields only marginal benefits. For instance, going from 50 to 500 participants might significantly boost a study’s robustness, but jumping from 10,000 to 10,500 might not offer a comparable advantage, especially considering the added costs.

- En español – ExME
- Em português – EME
What are sampling methods and how do you choose the best one?
Posted on 18th November 2020 by Mohamed Khalifa
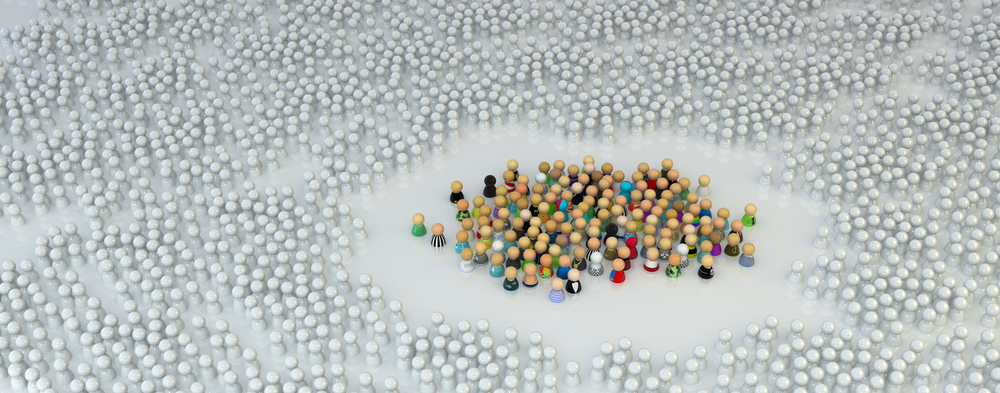
This tutorial will introduce sampling methods and potential sampling errors to avoid when conducting medical research.
Introduction to sampling methods
Examples of different sampling methods, choosing the best sampling method.
It is important to understand why we sample the population; for example, studies are built to investigate the relationships between risk factors and disease. In other words, we want to find out if this is a true association, while still aiming for the minimum risk for errors such as: chance, bias or confounding .
However, it would not be feasible to experiment on the whole population, we would need to take a good sample and aim to reduce the risk of having errors by proper sampling technique.
What is a sampling frame?
A sampling frame is a record of the target population containing all participants of interest. In other words, it is a list from which we can extract a sample.
What makes a good sample?
A good sample should be a representative subset of the population we are interested in studying, therefore, with each participant having equal chance of being randomly selected into the study.
We could choose a sampling method based on whether we want to account for sampling bias; a random sampling method is often preferred over a non-random method for this reason. Random sampling examples include: simple, systematic, stratified, and cluster sampling. Non-random sampling methods are liable to bias, and common examples include: convenience, purposive, snowballing, and quota sampling. For the purposes of this blog we will be focusing on random sampling methods .
Example: We want to conduct an experimental trial in a small population such as: employees in a company, or students in a college. We include everyone in a list and use a random number generator to select the participants
Advantages: Generalisable results possible, random sampling, the sampling frame is the whole population, every participant has an equal probability of being selected
Disadvantages: Less precise than stratified method, less representative than the systematic method
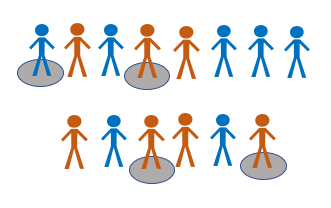
Example: Every nth patient entering the out-patient clinic is selected and included in our sample
Advantages: More feasible than simple or stratified methods, sampling frame is not always required
Disadvantages: Generalisability may decrease if baseline characteristics repeat across every nth participant
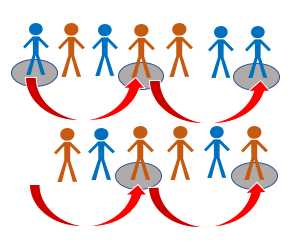
Example: We have a big population (a city) and we want to ensure representativeness of all groups with a pre-determined characteristic such as: age groups, ethnic origin, and gender
Advantages: Inclusive of strata (subgroups), reliable and generalisable results
Disadvantages: Does not work well with multiple variables
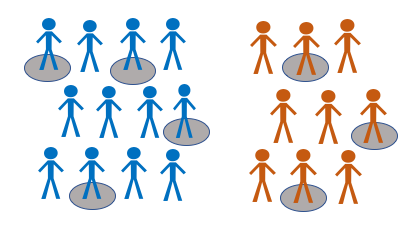
Example: 10 schools have the same number of students across the county. We can randomly select 3 out of 10 schools as our clusters
Advantages: Readily doable with most budgets, does not require a sampling frame
Disadvantages: Results may not be reliable nor generalisable
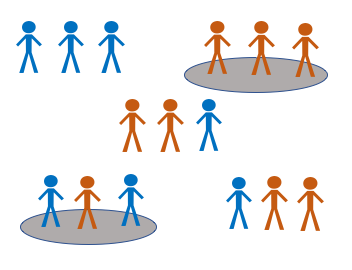
How can you identify sampling errors?
Non-random selection increases the probability of sampling (selection) bias if the sample does not represent the population we want to study. We could avoid this by random sampling and ensuring representativeness of our sample with regards to sample size.
An inadequate sample size decreases the confidence in our results as we may think there is no significant difference when actually there is. This type two error results from having a small sample size, or from participants dropping out of the sample.
In medical research of disease, if we select people with certain diseases while strictly excluding participants with other co-morbidities, we run the risk of diagnostic purity bias where important sub-groups of the population are not represented.
Furthermore, measurement bias may occur during re-collection of risk factors by participants (recall bias) or assessment of outcome where people who live longer are associated with treatment success, when in fact people who died were not included in the sample or data analysis (survivors bias).
By following the steps below we could choose the best sampling method for our study in an orderly fashion.
Research objectiveness
Firstly, a refined research question and goal would help us define our population of interest. If our calculated sample size is small then it would be easier to get a random sample. If, however, the sample size is large, then we should check if our budget and resources can handle a random sampling method.
Sampling frame availability
Secondly, we need to check for availability of a sampling frame (Simple), if not, could we make a list of our own (Stratified). If neither option is possible, we could still use other random sampling methods, for instance, systematic or cluster sampling.
Study design
Moreover, we could consider the prevalence of the topic (exposure or outcome) in the population, and what would be the suitable study design. In addition, checking if our target population is widely varied in its baseline characteristics. For example, a population with large ethnic subgroups could best be studied using a stratified sampling method.
Random sampling
Finally, the best sampling method is always the one that could best answer our research question while also allowing for others to make use of our results (generalisability of results). When we cannot afford a random sampling method, we can always choose from the non-random sampling methods.
To sum up, we now understand that choosing between random or non-random sampling methods is multifactorial. We might often be tempted to choose a convenience sample from the start, but that would not only decrease precision of our results, and would make us miss out on producing research that is more robust and reliable.
References (pdf)
Mohamed Khalifa
Leave a reply cancel reply.
Your email address will not be published. Required fields are marked *
Save my name, email, and website in this browser for the next time I comment.
No Comments on What are sampling methods and how do you choose the best one?
Thank you for this overview. A concise approach for research.
really helps! am an ecology student preparing to write my lab report for sampling.
I learned a lot to the given presentation.. It’s very comprehensive… Thanks for sharing…
Very informative and useful for my study. Thank you
Oversimplified info on sampling methods. Probabilistic of the sampling and sampling of samples by chance does rest solely on the random methods. Factors such as the random visits or presentation of the potential participants at clinics or sites could be sufficiently random in nature and should be used for the sake of efficiency and feasibility. Nevertheless, this approach has to be taken only after careful thoughts. Representativeness of the study samples have to be checked at the end or during reporting by comparing it to the published larger studies or register of some kind in/from the local population.
Thank you so much Mr.mohamed very useful and informative article
Subscribe to our newsletter
You will receive our monthly newsletter and free access to Trip Premium.
Related Articles
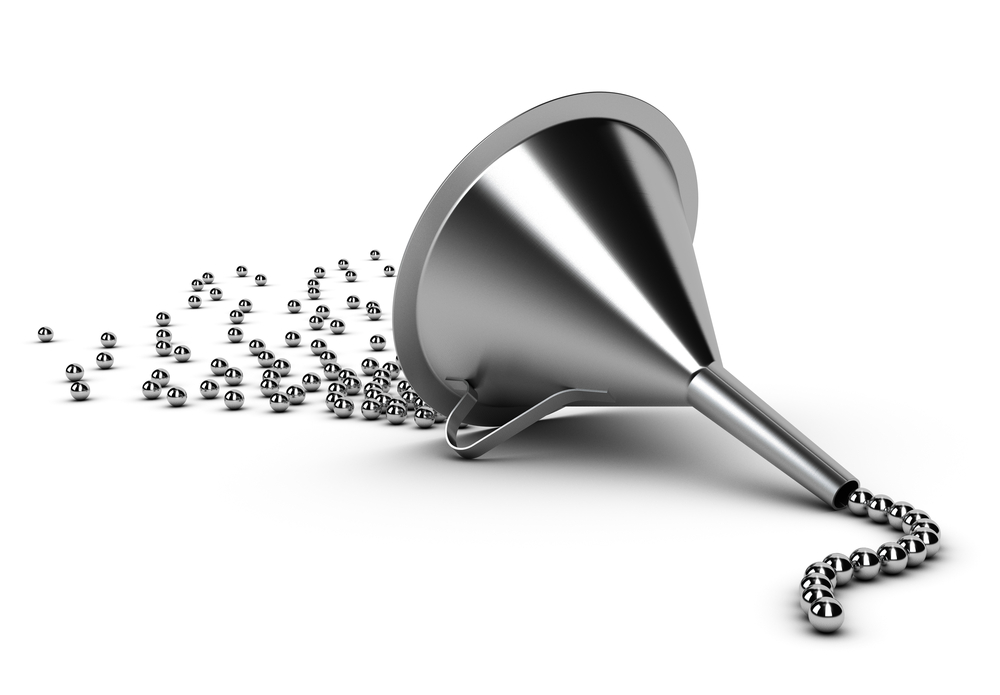
How to read a funnel plot
This blog introduces you to funnel plots, guiding you through how to read them and what may cause them to look asymmetrical.
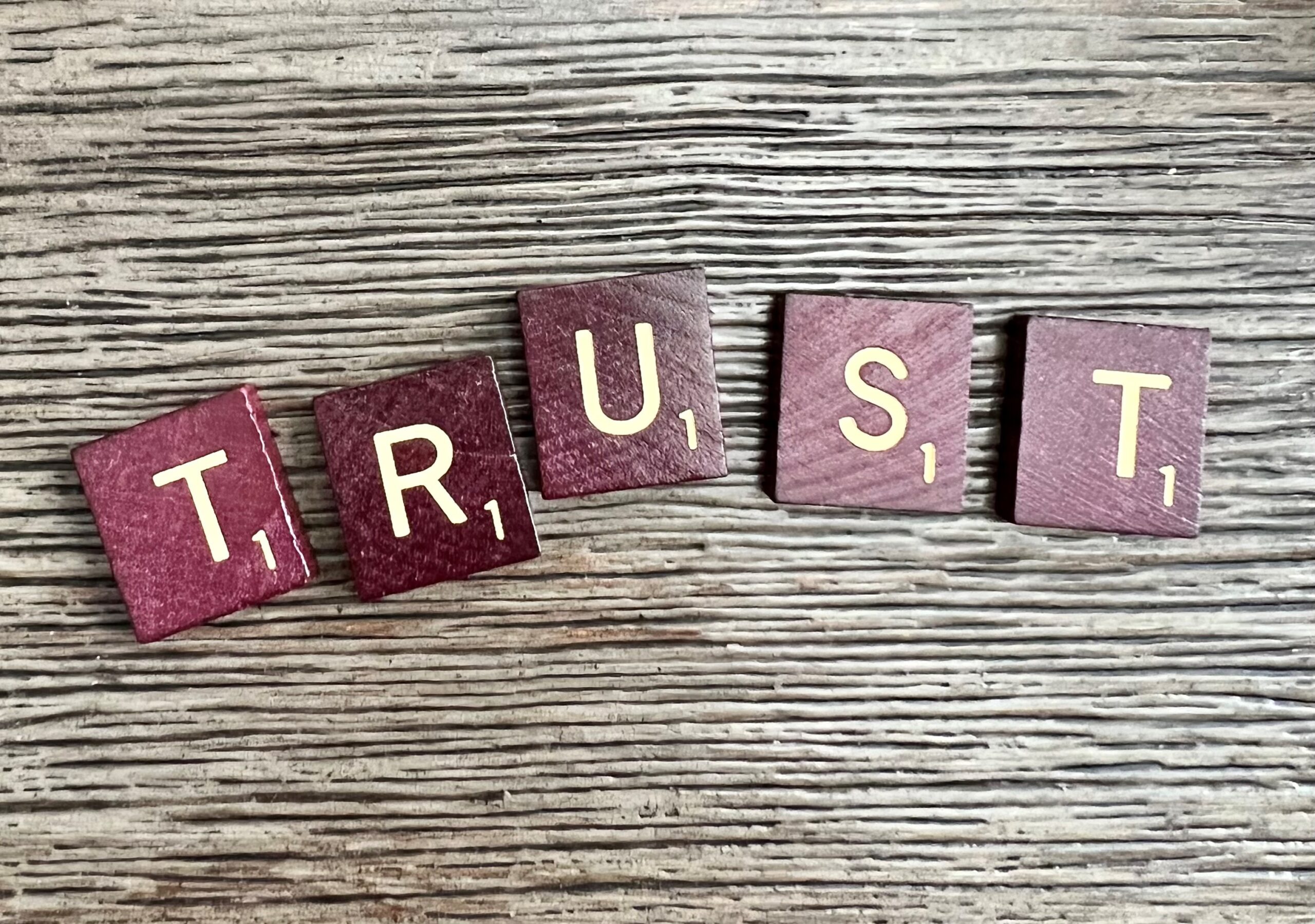
Internal and external validity: what are they and how do they differ?
Is this study valid? Can I trust this study’s methods and design? Can I apply the results of this study to other contexts? Learn more about internal and external validity in research to help you answer these questions when you next look at a paper.
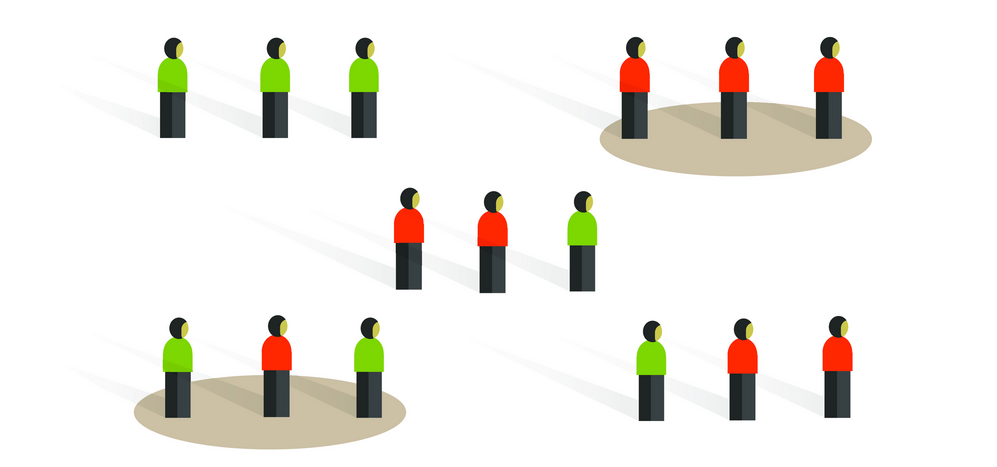
Cluster Randomized Trials: Concepts
This blog summarizes the concepts of cluster randomization, and the logistical and statistical considerations while designing a cluster randomized controlled trial.
Have a language expert improve your writing
Run a free plagiarism check in 10 minutes, automatically generate references for free.
- Knowledge Base
- Methodology
- Sampling Methods | Types, Techniques, & Examples
Sampling Methods | Types, Techniques, & Examples
Published on 3 May 2022 by Shona McCombes . Revised on 10 October 2022.
When you conduct research about a group of people, it’s rarely possible to collect data from every person in that group. Instead, you select a sample. The sample is the group of individuals who will actually participate in the research.
To draw valid conclusions from your results, you have to carefully decide how you will select a sample that is representative of the group as a whole. There are two types of sampling methods:
- Probability sampling involves random selection, allowing you to make strong statistical inferences about the whole group. It minimises the risk of selection bias .
- Non-probability sampling involves non-random selection based on convenience or other criteria, allowing you to easily collect data.
You should clearly explain how you selected your sample in the methodology section of your paper or thesis.
Table of contents
Population vs sample, probability sampling methods, non-probability sampling methods, frequently asked questions about sampling.
First, you need to understand the difference between a population and a sample , and identify the target population of your research.
- The population is the entire group that you want to draw conclusions about.
- The sample is the specific group of individuals that you will collect data from.
The population can be defined in terms of geographical location, age, income, and many other characteristics.
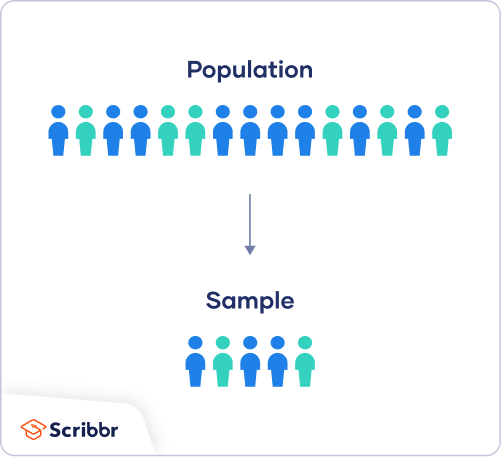
It is important to carefully define your target population according to the purpose and practicalities of your project.
If the population is very large, demographically mixed, and geographically dispersed, it might be difficult to gain access to a representative sample.
Sampling frame
The sampling frame is the actual list of individuals that the sample will be drawn from. Ideally, it should include the entire target population (and nobody who is not part of that population).
You are doing research on working conditions at Company X. Your population is all 1,000 employees of the company. Your sampling frame is the company’s HR database, which lists the names and contact details of every employee.
Sample size
The number of individuals you should include in your sample depends on various factors, including the size and variability of the population and your research design. There are different sample size calculators and formulas depending on what you want to achieve with statistical analysis .
Prevent plagiarism, run a free check.
Probability sampling means that every member of the population has a chance of being selected. It is mainly used in quantitative research . If you want to produce results that are representative of the whole population, probability sampling techniques are the most valid choice.
There are four main types of probability sample.
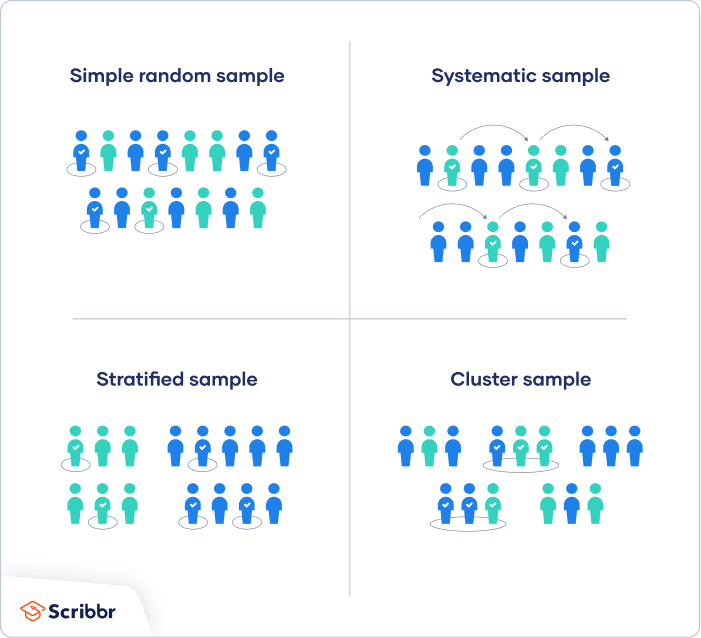
1. Simple random sampling
In a simple random sample , every member of the population has an equal chance of being selected. Your sampling frame should include the whole population.
To conduct this type of sampling, you can use tools like random number generators or other techniques that are based entirely on chance.
You want to select a simple random sample of 100 employees of Company X. You assign a number to every employee in the company database from 1 to 1000, and use a random number generator to select 100 numbers.
2. Systematic sampling
Systematic sampling is similar to simple random sampling, but it is usually slightly easier to conduct. Every member of the population is listed with a number, but instead of randomly generating numbers, individuals are chosen at regular intervals.
All employees of the company are listed in alphabetical order. From the first 10 numbers, you randomly select a starting point: number 6. From number 6 onwards, every 10th person on the list is selected (6, 16, 26, 36, and so on), and you end up with a sample of 100 people.
If you use this technique, it is important to make sure that there is no hidden pattern in the list that might skew the sample. For example, if the HR database groups employees by team, and team members are listed in order of seniority, there is a risk that your interval might skip over people in junior roles, resulting in a sample that is skewed towards senior employees.
3. Stratified sampling
Stratified sampling involves dividing the population into subpopulations that may differ in important ways. It allows you draw more precise conclusions by ensuring that every subgroup is properly represented in the sample.
To use this sampling method, you divide the population into subgroups (called strata) based on the relevant characteristic (e.g., gender, age range, income bracket, job role).
Based on the overall proportions of the population, you calculate how many people should be sampled from each subgroup. Then you use random or systematic sampling to select a sample from each subgroup.
The company has 800 female employees and 200 male employees. You want to ensure that the sample reflects the gender balance of the company, so you sort the population into two strata based on gender. Then you use random sampling on each group, selecting 80 women and 20 men, which gives you a representative sample of 100 people.
4. Cluster sampling
Cluster sampling also involves dividing the population into subgroups, but each subgroup should have similar characteristics to the whole sample. Instead of sampling individuals from each subgroup, you randomly select entire subgroups.
If it is practically possible, you might include every individual from each sampled cluster. If the clusters themselves are large, you can also sample individuals from within each cluster using one of the techniques above. This is called multistage sampling .
This method is good for dealing with large and dispersed populations, but there is more risk of error in the sample, as there could be substantial differences between clusters. It’s difficult to guarantee that the sampled clusters are really representative of the whole population.
The company has offices in 10 cities across the country (all with roughly the same number of employees in similar roles). You don’t have the capacity to travel to every office to collect your data, so you use random sampling to select 3 offices – these are your clusters.
In a non-probability sample , individuals are selected based on non-random criteria, and not every individual has a chance of being included.
This type of sample is easier and cheaper to access, but it has a higher risk of sampling bias . That means the inferences you can make about the population are weaker than with probability samples, and your conclusions may be more limited. If you use a non-probability sample, you should still aim to make it as representative of the population as possible.
Non-probability sampling techniques are often used in exploratory and qualitative research . In these types of research, the aim is not to test a hypothesis about a broad population, but to develop an initial understanding of a small or under-researched population.
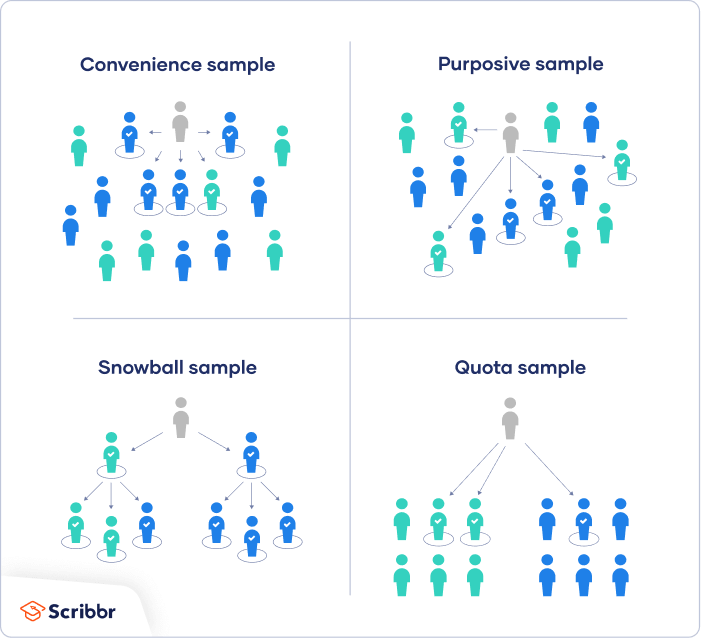
1. Convenience sampling
A convenience sample simply includes the individuals who happen to be most accessible to the researcher.
This is an easy and inexpensive way to gather initial data, but there is no way to tell if the sample is representative of the population, so it can’t produce generalisable results.
You are researching opinions about student support services in your university, so after each of your classes, you ask your fellow students to complete a survey on the topic. This is a convenient way to gather data, but as you only surveyed students taking the same classes as you at the same level, the sample is not representative of all the students at your university.
2. Voluntary response sampling
Similar to a convenience sample, a voluntary response sample is mainly based on ease of access. Instead of the researcher choosing participants and directly contacting them, people volunteer themselves (e.g., by responding to a public online survey).
Voluntary response samples are always at least somewhat biased, as some people will inherently be more likely to volunteer than others.
You send out the survey to all students at your university and many students decide to complete it. This can certainly give you some insight into the topic, but the people who responded are more likely to be those who have strong opinions about the student support services, so you can’t be sure that their opinions are representative of all students.
3. Purposive sampling
Purposive sampling , also known as judgement sampling, involves the researcher using their expertise to select a sample that is most useful to the purposes of the research.
It is often used in qualitative research , where the researcher wants to gain detailed knowledge about a specific phenomenon rather than make statistical inferences, or where the population is very small and specific. An effective purposive sample must have clear criteria and rationale for inclusion.
You want to know more about the opinions and experiences of students with a disability at your university, so you purposely select a number of students with different support needs in order to gather a varied range of data on their experiences with student services.
4. Snowball sampling
If the population is hard to access, snowball sampling can be used to recruit participants via other participants. The number of people you have access to ‘snowballs’ as you get in contact with more people.
You are researching experiences of homelessness in your city. Since there is no list of all homeless people in the city, probability sampling isn’t possible. You meet one person who agrees to participate in the research, and she puts you in contact with other homeless people she knows in the area.
A sample is a subset of individuals from a larger population. Sampling means selecting the group that you will actually collect data from in your research.
For example, if you are researching the opinions of students in your university, you could survey a sample of 100 students.
Statistical sampling allows you to test a hypothesis about the characteristics of a population. There are various sampling methods you can use to ensure that your sample is representative of the population as a whole.
Samples are used to make inferences about populations . Samples are easier to collect data from because they are practical, cost-effective, convenient, and manageable.
Probability sampling means that every member of the target population has a known chance of being included in the sample.
Probability sampling methods include simple random sampling , systematic sampling , stratified sampling , and cluster sampling .
In non-probability sampling , the sample is selected based on non-random criteria, and not every member of the population has a chance of being included.
Common non-probability sampling methods include convenience sampling , voluntary response sampling, purposive sampling , snowball sampling , and quota sampling .
Sampling bias occurs when some members of a population are systematically more likely to be selected in a sample than others.
Cite this Scribbr article
If you want to cite this source, you can copy and paste the citation or click the ‘Cite this Scribbr article’ button to automatically add the citation to our free Reference Generator.
McCombes, S. (2022, October 10). Sampling Methods | Types, Techniques, & Examples. Scribbr. Retrieved 22 April 2024, from https://www.scribbr.co.uk/research-methods/sampling/
Is this article helpful?
Shona McCombes
Other students also liked, what is quantitative research | definition & methods, a quick guide to experimental design | 5 steps & examples, controlled experiments | methods & examples of control.
If you're seeing this message, it means we're having trouble loading external resources on our website.
If you're behind a web filter, please make sure that the domains *.kastatic.org and *.kasandbox.org are unblocked.
To log in and use all the features of Khan Academy, please enable JavaScript in your browser.
Statistics and probability
Course: statistics and probability > unit 6.
- Picking fairly
- Using probability to make fair decisions
- Techniques for generating a simple random sample
- Simple random samples
- Techniques for random sampling and avoiding bias
- Sampling methods
Sampling methods review
- Samples and surveys
What are sampling methods?
Bad ways to sample.
- (Choice A) Convenience sampling A Convenience sampling
- (Choice B) Voluntary response sampling B Voluntary response sampling
Good ways to sample
- (Choice A) Simple random sampling A Simple random sampling
- (Choice B) Stratified random sampling B Stratified random sampling
- (Choice C) Cluster random sampling C Cluster random sampling
- (Choice D) Systematic random sampling D Systematic random sampling
Want to join the conversation?
- Upvote Button navigates to signup page
- Downvote Button navigates to signup page
- Flag Button navigates to signup page

An overview of sampling methods
Last updated
27 February 2023
Reviewed by
Cathy Heath
When researching perceptions or attributes of a product, service, or people, you have two options:
Survey every person in your chosen group (the target market, or population), collate your responses, and reach your conclusions.
Select a smaller group from within your target market and use their answers to represent everyone. This option is sampling .
Sampling saves you time and money. When you use the sampling method, the whole population being studied is called the sampling frame .
The sample you choose should represent your target market, or the sampling frame, well enough to do one of the following:
Generalize your findings across the sampling frame and use them as though you had surveyed everyone
Use the findings to decide on your next step, which might involve more in-depth sampling
Make research less tedious
Dovetail streamlines research to help you uncover and share actionable insights
How was sampling developed?
Valery Glivenko and Francesco Cantelli, two mathematicians studying probability theory in the early 1900s, devised the sampling method. Their research showed that a properly chosen sample of people would reflect the larger group’s status, opinions, decisions, and decision-making steps.
They proved you don't need to survey the entire target market, thereby saving the rest of us a lot of time and money.
- Why is sampling important?
We’ve already touched on the fact that sampling saves you time and money. When you get reliable results quickly, you can act on them sooner. And the money you save can pay for something else.
It’s often easier to survey a sample than a whole population. Sample inferences can be more reliable than those you get from a very large group because you can choose your samples carefully and scientifically.
Sampling is also useful because it is often impossible to survey the entire population. You probably have no choice but to collect only a sample in the first place.
Because you’re working with fewer people, you can collect richer data, which makes your research more accurate. You can:
Ask more questions
Go into more detail
Seek opinions instead of just collecting facts
Observe user behaviors
Double-check your findings if you need to
In short, sampling works! Let's take a look at the most common sampling methods.
- Types of sampling methods
There are two main sampling methods: probability sampling and non-probability sampling. These can be further refined, which we'll cover shortly. You can then decide which approach best suits your research project.
Probability sampling method
Probability sampling is used in quantitative research , so it provides data on the survey topic in terms of numbers. Probability relates to mathematics, hence the name ‘quantitative research’. Subjects are asked questions like:
How many boxes of candy do you buy at one time?
How often do you shop for candy?
How much would you pay for a box of candy?
This method is also called random sampling because everyone in the target market has an equal chance of being chosen for the survey. It is designed to reduce sampling error for the most important variables. You should, therefore, get results that fairly reflect the larger population.
Non-probability sampling method
In this method, not everyone has an equal chance of being part of the sample. It's usually easier (and cheaper) to select people for the sample group. You choose people who are more likely to be involved in or know more about the topic you’re researching.
Non-probability sampling is used for qualitative research. Qualitative data is generated by questions like:
Where do you usually shop for candy (supermarket, gas station, etc.?)
Which candy brand do you usually buy?
Why do you like that brand?
- Probability sampling methods
Here are five ways of doing probability sampling:
Simple random sampling (basic probability sampling)
Systematic sampling
Stratified sampling.
Cluster sampling
Multi-stage sampling
Simple random sampling.
There are three basic steps to simple random sampling:
Choose your sampling frame.
Decide on your sample size. Make sure it is large enough to give you reliable data.
Randomly choose your sample participants.
You could put all their names in a hat, shake the hat to mix the names, and pull out however many names you want in your sample (without looking!)
You could be more scientific by giving each participant a number and then using a random number generator program to choose the numbers.
Instead of choosing names or numbers, you decide beforehand on a selection method. For example, collect all the names in your sampling frame and start at, for example, the fifth person on the list, then choose every fourth name or every tenth name. Alternatively, you could choose everyone whose last name begins with randomly-selected initials, such as A, G, or W.
Choose your system of selecting names, and away you go.
This is a more sophisticated way to choose your sample. You break the sampling frame down into important subgroups or strata . Then, decide how many you want in your sample, and choose an equal number (or a proportionate number) from each subgroup.
For example, you want to survey how many people in a geographic area buy candy, so you compile a list of everyone in that area. You then break that list down into, for example, males and females, then into pre-teens, teenagers, young adults, senior citizens, etc. who are male or female.
So, if there are 1,000 young male adults and 2,000 young female adults in the whole sampling frame, you may want to choose 100 males and 200 females to keep the proportions balanced. You then choose the individual survey participants through the systematic sampling method.
Clustered sampling
This method is used when you want to subdivide a sample into smaller groups or clusters that are geographically or organizationally related.
Let’s say you’re doing quantitative research into candy sales. You could choose your sample participants from urban, suburban, or rural populations. This would give you three geographic clusters from which to select your participants.
This is a more refined way of doing cluster sampling. Let’s say you have your urban cluster, which is your primary sampling unit. You can subdivide this into a secondary sampling unit, say, participants who typically buy their candy in supermarkets. You could then further subdivide this group into your ultimate sampling unit. Finally, you select the actual survey participants from this unit.
- Uses of probability sampling
Probability sampling has three main advantages:
It helps minimizes the likelihood of sampling bias. How you choose your sample determines the quality of your results. Probability sampling gives you an unbiased, randomly selected sample of your target market.
It allows you to create representative samples and subgroups within a sample out of a large or diverse target market.
It lets you use sophisticated statistical methods to select as close to perfect samples as possible.
- Non-probability sampling methods
To recap, with non-probability sampling, you choose people for your sample in a non-random way, so not everyone in your sampling frame has an equal chance of being chosen. Your research findings, therefore, may not be as representative overall as probability sampling, but you may not want them to be.
Sampling bias is not a concern if all potential survey participants share similar traits. For example, you may want to specifically focus on young male adults who spend more than others on candy. In addition, it is usually a cheaper and quicker method because you don't have to work out a complex selection system that represents the entire population in that community.
Researchers do need to be mindful of carefully considering the strengths and limitations of each method before selecting a sampling technique.
Non-probability sampling is best for exploratory research , such as at the beginning of a research project.
There are five main types of non-probability sampling methods:
Convenience sampling
Purposive sampling, voluntary response sampling, snowball sampling, quota sampling.
The strategy of convenience sampling is to choose your sample quickly and efficiently, using the least effort, usually to save money.
Let's say you want to survey the opinions of 100 millennials about a particular topic. You could send out a questionnaire over the social media platforms millennials use. Ask respondents to confirm their birth year at the top of their response sheet and, when you have your 100 responses, begin your analysis. Or you could visit restaurants and bars where millennials spend their evenings and sign people up.
A drawback of convenience sampling is that it may not yield results that apply to a broader population.
This method relies on your judgment to choose the most likely sample to deliver the most useful results. You must know enough about the survey goals and the sampling frame to choose the most appropriate sample respondents.
Your knowledge and experience save you time because you know your ideal sample candidates, so you should get high-quality results.
This method is similar to convenience sampling, but it is based on potential sample members volunteering rather than you looking for people.
You make it known you want to do a survey on a particular topic for a particular reason and wait until enough people volunteer. Then you give them the questionnaire or arrange interviews to ask your questions directly.
Snowball sampling involves asking selected participants to refer others who may qualify for the survey. This method is best used when there is no sampling frame available. It is also useful when the researcher doesn’t know much about the target population.
Let's say you want to research a niche topic that involves people who may be difficult to locate. For our candy example, this could be young males who buy a lot of candy, go rock climbing during the day, and watch adventure movies at night. You ask each participant to name others they know who do the same things, so you can contact them. As you make contact with more people, your sample 'snowballs' until you have all the names you need.
This sampling method involves collecting the specific number of units (quotas) from your predetermined subpopulations. Quota sampling is a way of ensuring that your sample accurately represents the sampling frame.
- Uses of non-probability sampling
You can use non-probability sampling when you:
Want to do a quick test to see if a more detailed and sophisticated survey may be worthwhile
Want to explore an idea to see if it 'has legs'
Launch a pilot study
Do some initial qualitative research
Have little time or money available (half a loaf is better than no bread at all)
Want to see if the initial results will help you justify a longer, more detailed, and more expensive research project
- The main types of sampling bias, and how to avoid them
Sampling bias can fog or limit your research results. This will have an impact when you generalize your results across the whole target market. The two main causes of sampling bias are faulty research design and poor data collection or recording. They can affect probability and non-probability sampling.
Faulty research
If a surveyor chooses participants inappropriately, the results will not reflect the population as a whole.
A famous example is the 1948 presidential race. A telephone survey was conducted to see which candidate had more support. The problem with the research design was that, in 1948, most people with telephones were wealthy, and their opinions were very different from voters as a whole. The research implied Dewey would win, but it was Truman who became president.
Poor data collection or recording
This problem speaks for itself. The survey may be well structured, the sample groups appropriate, the questions clear and easy to understand, and the cluster sizes appropriate. But if surveyors check the wrong boxes when they get an answer or if the entire subgroup results are lost, the survey results will be biased.
How do you minimize bias in sampling?
To get results you can rely on, you must:
Know enough about your target market
Choose one or more sample surveys to cover the whole target market properly
Choose enough people in each sample so your results mirror your target market
Have content validity . This means the content of your questions must be direct and efficiently worded. If it isn’t, the viability of your survey could be questioned. That would also be a waste of time and money, so make the wording of your questions your top focus.
If using probability sampling, make sure your sampling frame includes everyone it should and that your random sampling selection process includes the right proportion of the subgroups
If using non-probability sampling, focus on fairness, equality, and completeness in identifying your samples and subgroups. Then balance those criteria against simple convenience or other relevant factors.
What are the five types of sampling bias?
Self-selection bias. If you mass-mail questionnaires to everyone in the sample, you’re more likely to get results from people with extrovert or activist personalities and not from introverts or pragmatists. So if your convenience sampling focuses on getting your quota responses quickly, it may be skewed.
Non-response bias. Unhappy customers, stressed-out employees, or other sub-groups may not want to cooperate or they may pull out early.
Undercoverage bias. If your survey is done, say, via email or social media platforms, it will miss people without internet access, such as those living in rural areas, the elderly, or lower-income groups.
Survivorship bias. Unsuccessful people are less likely to take part. Another example may be a researcher excluding results that don’t support the overall goal. If the CEO wants to tell the shareholders about a successful product or project at the AGM, some less positive survey results may go “missing” (to take an extreme example.) The result is that your data will reflect an overly optimistic representation of the truth.
Pre-screening bias. If the researcher, whose experience and knowledge are being used to pre-select respondents in a judgmental sampling, focuses more on convenience than judgment, the results may be compromised.
How do you minimize sampling bias?
Focus on the bullet points in the next section and:
Make survey questionnaires as direct, easy, short, and available as possible, so participants are more likely to complete them accurately and send them back
Follow up with the people who have been selected but have not returned their responses
Ignore any pressure that may produce bias
- How do you decide on the type of sampling to use?
Use the ideas you've gleaned from this article to give yourself a platform, then choose the best method to meet your goals while staying within your time and cost limits.
If it isn't obvious which method you should choose, use this strategy:
Clarify your research goals
Clarify how accurate your research results must be to reach your goals
Evaluate your goals against time and budget
List the two or three most obvious sampling methods that will work for you
Confirm the availability of your resources (researchers, computer time, etc.)
Compare each of the possible methods with your goals, accuracy, precision, resource, time, and cost constraints
Make your decision
- The takeaway
Effective market research is the basis of successful marketing, advertising, and future productivity. By selecting the most appropriate sampling methods, you will collect the most useful market data and make the most effective decisions.
Get started today
Go from raw data to valuable insights with a flexible research platform
Editor’s picks
Last updated: 21 December 2023
Last updated: 16 December 2023
Last updated: 6 October 2023
Last updated: 25 November 2023
Last updated: 12 May 2023
Last updated: 15 February 2024
Last updated: 11 March 2024
Last updated: 12 December 2023
Last updated: 18 May 2023
Last updated: 6 March 2024
Last updated: 10 April 2023
Last updated: 20 December 2023
Latest articles
Related topics, log in or sign up.
Get started for free
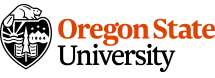
Chapter 5. Sampling
Introduction.
Most Americans will experience unemployment at some point in their lives. Sarah Damaske ( 2021 ) was interested in learning about how men and women experience unemployment differently. To answer this question, she interviewed unemployed people. After conducting a “pilot study” with twenty interviewees, she realized she was also interested in finding out how working-class and middle-class persons experienced unemployment differently. She found one hundred persons through local unemployment offices. She purposefully selected a roughly equal number of men and women and working-class and middle-class persons for the study. This would allow her to make the kinds of comparisons she was interested in. She further refined her selection of persons to interview:
I decided that I needed to be able to focus my attention on gender and class; therefore, I interviewed only people born between 1962 and 1987 (ages 28–52, the prime working and child-rearing years), those who worked full-time before their job loss, those who experienced an involuntary job loss during the past year, and those who did not lose a job for cause (e.g., were not fired because of their behavior at work). ( 244 )
The people she ultimately interviewed compose her sample. They represent (“sample”) the larger population of the involuntarily unemployed. This “theoretically informed stratified sampling design” allowed Damaske “to achieve relatively equal distribution of participation across gender and class,” but it came with some limitations. For one, the unemployment centers were located in primarily White areas of the country, so there were very few persons of color interviewed. Qualitative researchers must make these kinds of decisions all the time—who to include and who not to include. There is never an absolutely correct decision, as the choice is linked to the particular research question posed by the particular researcher, although some sampling choices are more compelling than others. In this case, Damaske made the choice to foreground both gender and class rather than compare all middle-class men and women or women of color from different class positions or just talk to White men. She leaves the door open for other researchers to sample differently. Because science is a collective enterprise, it is most likely someone will be inspired to conduct a similar study as Damaske’s but with an entirely different sample.
This chapter is all about sampling. After you have developed a research question and have a general idea of how you will collect data (observations or interviews), how do you go about actually finding people and sites to study? Although there is no “correct number” of people to interview, the sample should follow the research question and research design. You might remember studying sampling in a quantitative research course. Sampling is important here too, but it works a bit differently. Unlike quantitative research, qualitative research involves nonprobability sampling. This chapter explains why this is so and what qualities instead make a good sample for qualitative research.
Quick Terms Refresher
- The population is the entire group that you want to draw conclusions about.
- The sample is the specific group of individuals that you will collect data from.
- Sampling frame is the actual list of individuals that the sample will be drawn from. Ideally, it should include the entire target population (and nobody who is not part of that population).
- Sample size is how many individuals (or units) are included in your sample.
The “Who” of Your Research Study
After you have turned your general research interest into an actual research question and identified an approach you want to take to answer that question, you will need to specify the people you will be interviewing or observing. In most qualitative research, the objects of your study will indeed be people. In some cases, however, your objects might be content left by people (e.g., diaries, yearbooks, photographs) or documents (official or unofficial) or even institutions (e.g., schools, medical centers) and locations (e.g., nation-states, cities). Chances are, whatever “people, places, or things” are the objects of your study, you will not really be able to talk to, observe, or follow every single individual/object of the entire population of interest. You will need to create a sample of the population . Sampling in qualitative research has different purposes and goals than sampling in quantitative research. Sampling in both allows you to say something of interest about a population without having to include the entire population in your sample.
We begin this chapter with the case of a population of interest composed of actual people. After we have a better understanding of populations and samples that involve real people, we’ll discuss sampling in other types of qualitative research, such as archival research, content analysis, and case studies. We’ll then move to a larger discussion about the difference between sampling in qualitative research generally versus quantitative research, then we’ll move on to the idea of “theoretical” generalizability, and finally, we’ll conclude with some practical tips on the correct “number” to include in one’s sample.
Sampling People
To help think through samples, let’s imagine we want to know more about “vaccine hesitancy.” We’ve all lived through 2020 and 2021, and we know that a sizable number of people in the United States (and elsewhere) were slow to accept vaccines, even when these were freely available. By some accounts, about one-third of Americans initially refused vaccination. Why is this so? Well, as I write this in the summer of 2021, we know that some people actively refused the vaccination, thinking it was harmful or part of a government plot. Others were simply lazy or dismissed the necessity. And still others were worried about harmful side effects. The general population of interest here (all adult Americans who were not vaccinated by August 2021) may be as many as eighty million people. We clearly cannot talk to all of them. So we will have to narrow the number to something manageable. How can we do this?
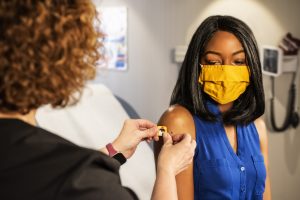
First, we have to think about our actual research question and the form of research we are conducting. I am going to begin with a quantitative research question. Quantitative research questions tend to be simpler to visualize, at least when we are first starting out doing social science research. So let us say we want to know what percentage of each kind of resistance is out there and how race or class or gender affects vaccine hesitancy. Again, we don’t have the ability to talk to everyone. But harnessing what we know about normal probability distributions (see quantitative methods for more on this), we can find this out through a sample that represents the general population. We can’t really address these particular questions if we only talk to White women who go to college with us. And if you are really trying to generalize the specific findings of your sample to the larger population, you will have to employ probability sampling , a sampling technique where a researcher sets a selection of a few criteria and chooses members of a population randomly. Why randomly? If truly random, all the members have an equal opportunity to be a part of the sample, and thus we avoid the problem of having only our friends and neighbors (who may be very different from other people in the population) in the study. Mathematically, there is going to be a certain number that will be large enough to allow us to generalize our particular findings from our sample population to the population at large. It might surprise you how small that number can be. Election polls of no more than one thousand people are routinely used to predict actual election outcomes of millions of people. Below that number, however, you will not be able to make generalizations. Talking to five people at random is simply not enough people to predict a presidential election.
In order to answer quantitative research questions of causality, one must employ probability sampling. Quantitative researchers try to generalize their findings to a larger population. Samples are designed with that in mind. Qualitative researchers ask very different questions, though. Qualitative research questions are not about “how many” of a certain group do X (in this case, what percentage of the unvaccinated hesitate for concern about safety rather than reject vaccination on political grounds). Qualitative research employs nonprobability sampling . By definition, not everyone has an equal opportunity to be included in the sample. The researcher might select White women they go to college with to provide insight into racial and gender dynamics at play. Whatever is found by doing so will not be generalizable to everyone who has not been vaccinated, or even all White women who have not been vaccinated, or even all White women who have not been vaccinated who are in this particular college. That is not the point of qualitative research at all. This is a really important distinction, so I will repeat in bold: Qualitative researchers are not trying to statistically generalize specific findings to a larger population . They have not failed when their sample cannot be generalized, as that is not the point at all.
In the previous paragraph, I said it would be perfectly acceptable for a qualitative researcher to interview five White women with whom she goes to college about their vaccine hesitancy “to provide insight into racial and gender dynamics at play.” The key word here is “insight.” Rather than use a sample as a stand-in for the general population, as quantitative researchers do, the qualitative researcher uses the sample to gain insight into a process or phenomenon. The qualitative researcher is not going to be content with simply asking each of the women to state her reason for not being vaccinated and then draw conclusions that, because one in five of these women were concerned about their health, one in five of all people were also concerned about their health. That would be, frankly, a very poor study indeed. Rather, the qualitative researcher might sit down with each of the women and conduct a lengthy interview about what the vaccine means to her, why she is hesitant, how she manages her hesitancy (how she explains it to her friends), what she thinks about others who are unvaccinated, what she thinks of those who have been vaccinated, and what she knows or thinks she knows about COVID-19. The researcher might include specific interview questions about the college context, about their status as White women, about the political beliefs they hold about racism in the US, and about how their own political affiliations may or may not provide narrative scripts about “protective whiteness.” There are many interesting things to ask and learn about and many things to discover. Where a quantitative researcher begins with clear parameters to set their population and guide their sample selection process, the qualitative researcher is discovering new parameters, making it impossible to engage in probability sampling.
Looking at it this way, sampling for qualitative researchers needs to be more strategic. More theoretically informed. What persons can be interviewed or observed that would provide maximum insight into what is still unknown? In other words, qualitative researchers think through what cases they could learn the most from, and those are the cases selected to study: “What would be ‘bias’ in statistical sampling, and therefore a weakness, becomes intended focus in qualitative sampling, and therefore a strength. The logic and power of purposeful sampling like in selecting information-rich cases for study in depth. Information-rich cases are those from which one can learn a great deal about issues of central importance to the purpose of the inquiry, thus the term purposeful sampling” ( Patton 2002:230 ; emphases in the original).
Before selecting your sample, though, it is important to clearly identify the general population of interest. You need to know this before you can determine the sample. In our example case, it is “adult Americans who have not yet been vaccinated.” Depending on the specific qualitative research question, however, it might be “adult Americans who have been vaccinated for political reasons” or even “college students who have not been vaccinated.” What insights are you seeking? Do you want to know how politics is affecting vaccination? Or do you want to understand how people manage being an outlier in a particular setting (unvaccinated where vaccinations are heavily encouraged if not required)? More clearly stated, your population should align with your research question . Think back to the opening story about Damaske’s work studying the unemployed. She drew her sample narrowly to address the particular questions she was interested in pursuing. Knowing your questions or, at a minimum, why you are interested in the topic will allow you to draw the best sample possible to achieve insight.
Once you have your population in mind, how do you go about getting people to agree to be in your sample? In qualitative research, it is permissible to find people by convenience. Just ask for people who fit your sample criteria and see who shows up. Or reach out to friends and colleagues and see if they know anyone that fits. Don’t let the name convenience sampling mislead you; this is not exactly “easy,” and it is certainly a valid form of sampling in qualitative research. The more unknowns you have about what you will find, the more convenience sampling makes sense. If you don’t know how race or class or political affiliation might matter, and your population is unvaccinated college students, you can construct a sample of college students by placing an advertisement in the student paper or posting a flyer on a notice board. Whoever answers is your sample. That is what is meant by a convenience sample. A common variation of convenience sampling is snowball sampling . This is particularly useful if your target population is hard to find. Let’s say you posted a flyer about your study and only two college students responded. You could then ask those two students for referrals. They tell their friends, and those friends tell other friends, and, like a snowball, your sample gets bigger and bigger.
Researcher Note
Gaining Access: When Your Friend Is Your Research Subject
My early experience with qualitative research was rather unique. At that time, I needed to do a project that required me to interview first-generation college students, and my friends, with whom I had been sharing a dorm for two years, just perfectly fell into the sample category. Thus, I just asked them and easily “gained my access” to the research subject; I know them, we are friends, and I am part of them. I am an insider. I also thought, “Well, since I am part of the group, I can easily understand their language and norms, I can capture their honesty, read their nonverbal cues well, will get more information, as they will be more opened to me because they trust me.” All in all, easy access with rich information. But, gosh, I did not realize that my status as an insider came with a price! When structuring the interview questions, I began to realize that rather than focusing on the unique experiences of my friends, I mostly based the questions on my own experiences, assuming we have similar if not the same experiences. I began to struggle with my objectivity and even questioned my role; am I doing this as part of the group or as a researcher? I came to know later that my status as an insider or my “positionality” may impact my research. It not only shapes the process of data collection but might heavily influence my interpretation of the data. I came to realize that although my inside status came with a lot of benefits (especially for access), it could also bring some drawbacks.
—Dede Setiono, PhD student focusing on international development and environmental policy, Oregon State University
The more you know about what you might find, the more strategic you can be. If you wanted to compare how politically conservative and politically liberal college students explained their vaccine hesitancy, for example, you might construct a sample purposively, finding an equal number of both types of students so that you can make those comparisons in your analysis. This is what Damaske ( 2021 ) did. You could still use convenience or snowball sampling as a way of recruitment. Post a flyer at the conservative student club and then ask for referrals from the one student that agrees to be interviewed. As with convenience sampling, there are variations of purposive sampling as well as other names used (e.g., judgment, quota, stratified, criterion, theoretical). Try not to get bogged down in the nomenclature; instead, focus on identifying the general population that matches your research question and then using a sampling method that is most likely to provide insight, given the types of questions you have.
There are all kinds of ways of being strategic with sampling in qualitative research. Here are a few of my favorite techniques for maximizing insight:
- Consider using “extreme” or “deviant” cases. Maybe your college houses a prominent anti-vaxxer who has written about and demonstrated against the college’s policy on vaccines. You could learn a lot from that single case (depending on your research question, of course).
- Consider “intensity”: people and cases and circumstances where your questions are more likely to feature prominently (but not extremely or deviantly). For example, you could compare those who volunteer at local Republican and Democratic election headquarters during an election season in a study on why party matters. Those who volunteer are more likely to have something to say than those who are more apathetic.
- Maximize variation, as with the case of “politically liberal” versus “politically conservative,” or include an array of social locations (young vs. old; Northwest vs. Southeast region). This kind of heterogeneity sampling can capture and describe the central themes that cut across the variations: any common patterns that emerge, even in this wildly mismatched sample, are probably important to note!
- Rather than maximize the variation, you could select a small homogenous sample to describe some particular subgroup in depth. Focus groups are often the best form of data collection for homogeneity sampling.
- Think about which cases are “critical” or politically important—ones that “if it happens here, it would happen anywhere” or a case that is politically sensitive, as with the single “blue” (Democratic) county in a “red” (Republican) state. In both, you are choosing a site that would yield the most information and have the greatest impact on the development of knowledge.
- On the other hand, sometimes you want to select the “typical”—the typical college student, for example. You are trying to not generalize from the typical but illustrate aspects that may be typical of this case or group. When selecting for typicality, be clear with yourself about why the typical matches your research questions (and who might be excluded or marginalized in doing so).
- Finally, it is often a good idea to look for disconfirming cases : if you are at the stage where you have a hypothesis (of sorts), you might select those who do not fit your hypothesis—you will surely learn something important there. They may be “exceptions that prove the rule” or exceptions that force you to alter your findings in order to make sense of these additional cases.
In addition to all these sampling variations, there is the theoretical approach taken by grounded theorists in which the researcher samples comparative people (or events) on the basis of their potential to represent important theoretical constructs. The sample, one can say, is by definition representative of the phenomenon of interest. It accompanies the constant comparative method of analysis. In the words of the funders of Grounded Theory , “Theoretical sampling is sampling on the basis of the emerging concepts, with the aim being to explore the dimensional range or varied conditions along which the properties of the concepts vary” ( Strauss and Corbin 1998:73 ).
When Your Population is Not Composed of People
I think it is easiest for most people to think of populations and samples in terms of people, but sometimes our units of analysis are not actually people. They could be places or institutions. Even so, you might still want to talk to people or observe the actions of people to understand those places or institutions. Or not! In the case of content analyses (see chapter 17), you won’t even have people involved at all but rather documents or films or photographs or news clippings. Everything we have covered about sampling applies to other units of analysis too. Let’s work through some examples.
Case Studies
When constructing a case study, it is helpful to think of your cases as sample populations in the same way that we considered people above. If, for example, you are comparing campus climates for diversity, your overall population may be “four-year college campuses in the US,” and from there you might decide to study three college campuses as your sample. Which three? Will you use purposeful sampling (perhaps [1] selecting three colleges in Oregon that are different sizes or [2] selecting three colleges across the US located in different political cultures or [3] varying the three colleges by racial makeup of the student body)? Or will you select three colleges at random, out of convenience? There are justifiable reasons for all approaches.
As with people, there are different ways of maximizing insight in your sample selection. Think about the following rationales: typical, diverse, extreme, deviant, influential, crucial, or even embodying a particular “pathway” ( Gerring 2008 ). When choosing a case or particular research site, Rubin ( 2021 ) suggests you bear in mind, first, what you are leaving out by selecting this particular case/site; second, what you might be overemphasizing by studying this case/site and not another; and, finally, whether you truly need to worry about either of those things—“that is, what are the sources of bias and how bad are they for what you are trying to do?” ( 89 ).
Once you have selected your cases, you may still want to include interviews with specific people or observations at particular sites within those cases. Then you go through possible sampling approaches all over again to determine which people will be contacted.
Content: Documents, Narrative Accounts, And So On
Although not often discussed as sampling, your selection of documents and other units to use in various content/historical analyses is subject to similar considerations. When you are asking quantitative-type questions (percentages and proportionalities of a general population), you will want to follow probabilistic sampling. For example, I created a random sample of accounts posted on the website studentloanjustice.org to delineate the types of problems people were having with student debt ( Hurst 2007 ). Even though my data was qualitative (narratives of student debt), I was actually asking a quantitative-type research question, so it was important that my sample was representative of the larger population (debtors who posted on the website). On the other hand, when you are asking qualitative-type questions, the selection process should be very different. In that case, use nonprobabilistic techniques, either convenience (where you are really new to this data and do not have the ability to set comparative criteria or even know what a deviant case would be) or some variant of purposive sampling. Let’s say you were interested in the visual representation of women in media published in the 1950s. You could select a national magazine like Time for a “typical” representation (and for its convenience, as all issues are freely available on the web and easy to search). Or you could compare one magazine known for its feminist content versus one antifeminist. The point is, sample selection is important even when you are not interviewing or observing people.
Goals of Qualitative Sampling versus Goals of Quantitative Sampling
We have already discussed some of the differences in the goals of quantitative and qualitative sampling above, but it is worth further discussion. The quantitative researcher seeks a sample that is representative of the population of interest so that they may properly generalize the results (e.g., if 80 percent of first-gen students in the sample were concerned with costs of college, then we can say there is a strong likelihood that 80 percent of first-gen students nationally are concerned with costs of college). The qualitative researcher does not seek to generalize in this way . They may want a representative sample because they are interested in typical responses or behaviors of the population of interest, but they may very well not want a representative sample at all. They might want an “extreme” or deviant case to highlight what could go wrong with a particular situation, or maybe they want to examine just one case as a way of understanding what elements might be of interest in further research. When thinking of your sample, you will have to know why you are selecting the units, and this relates back to your research question or sets of questions. It has nothing to do with having a representative sample to generalize results. You may be tempted—or it may be suggested to you by a quantitatively minded member of your committee—to create as large and representative a sample as you possibly can to earn credibility from quantitative researchers. Ignore this temptation or suggestion. The only thing you should be considering is what sample will best bring insight into the questions guiding your research. This has implications for the number of people (or units) in your study as well, which is the topic of the next section.
What is the Correct “Number” to Sample?
Because we are not trying to create a generalizable representative sample, the guidelines for the “number” of people to interview or news stories to code are also a bit more nebulous. There are some brilliant insightful studies out there with an n of 1 (meaning one person or one account used as the entire set of data). This is particularly so in the case of autoethnography, a variation of ethnographic research that uses the researcher’s own subject position and experiences as the basis of data collection and analysis. But it is true for all forms of qualitative research. There are no hard-and-fast rules here. The number to include is what is relevant and insightful to your particular study.
That said, humans do not thrive well under such ambiguity, and there are a few helpful suggestions that can be made. First, many qualitative researchers talk about “saturation” as the end point for data collection. You stop adding participants when you are no longer getting any new information (or so very little that the cost of adding another interview subject or spending another day in the field exceeds any likely benefits to the research). The term saturation was first used here by Glaser and Strauss ( 1967 ), the founders of Grounded Theory. Here is their explanation: “The criterion for judging when to stop sampling the different groups pertinent to a category is the category’s theoretical saturation . Saturation means that no additional data are being found whereby the sociologist can develop properties of the category. As he [or she] sees similar instances over and over again, the researcher becomes empirically confident that a category is saturated. [They go] out of [their] way to look for groups that stretch diversity of data as far as possible, just to make certain that saturation is based on the widest possible range of data on the category” ( 61 ).
It makes sense that the term was developed by grounded theorists, since this approach is rather more open-ended than other approaches used by qualitative researchers. With so much left open, having a guideline of “stop collecting data when you don’t find anything new” is reasonable. However, saturation can’t help much when first setting out your sample. How do you know how many people to contact to interview? What number will you put down in your institutional review board (IRB) protocol (see chapter 8)? You may guess how many people or units it will take to reach saturation, but there really is no way to know in advance. The best you can do is think about your population and your questions and look at what others have done with similar populations and questions.
Here are some suggestions to use as a starting point: For phenomenological studies, try to interview at least ten people for each major category or group of people . If you are comparing male-identified, female-identified, and gender-neutral college students in a study on gender regimes in social clubs, that means you might want to design a sample of thirty students, ten from each group. This is the minimum suggested number. Damaske’s ( 2021 ) sample of one hundred allows room for up to twenty-five participants in each of four “buckets” (e.g., working-class*female, working-class*male, middle-class*female, middle-class*male). If there is more than one comparative group (e.g., you are comparing students attending three different colleges, and you are comparing White and Black students in each), you can sometimes reduce the number for each group in your sample to five for, in this case, thirty total students. But that is really a bare minimum you will want to go. A lot of people will not trust you with only “five” cases in a bucket. Lareau ( 2021:24 ) advises a minimum of seven or nine for each bucket (or “cell,” in her words). The point is to think about what your analyses might look like and how comfortable you will be with a certain number of persons fitting each category.
Because qualitative research takes so much time and effort, it is rare for a beginning researcher to include more than thirty to fifty people or units in the study. You may not be able to conduct all the comparisons you might want simply because you cannot manage a larger sample. In that case, the limits of who you can reach or what you can include may influence you to rethink an original overcomplicated research design. Rather than include students from every racial group on a campus, for example, you might want to sample strategically, thinking about the most contrast (insightful), possibly excluding majority-race (White) students entirely, and simply using previous literature to fill in gaps in our understanding. For example, one of my former students was interested in discovering how race and class worked at a predominantly White institution (PWI). Due to time constraints, she simplified her study from an original sample frame of middle-class and working-class domestic Black and international African students (four buckets) to a sample frame of domestic Black and international African students (two buckets), allowing the complexities of class to come through individual accounts rather than from part of the sample frame. She wisely decided not to include White students in the sample, as her focus was on how minoritized students navigated the PWI. She was able to successfully complete her project and develop insights from the data with fewer than twenty interviewees. [1]
But what if you had unlimited time and resources? Would it always be better to interview more people or include more accounts, documents, and units of analysis? No! Your sample size should reflect your research question and the goals you have set yourself. Larger numbers can sometimes work against your goals. If, for example, you want to help bring out individual stories of success against the odds, adding more people to the analysis can end up drowning out those individual stories. Sometimes, the perfect size really is one (or three, or five). It really depends on what you are trying to discover and achieve in your study. Furthermore, studies of one hundred or more (people, documents, accounts, etc.) can sometimes be mistaken for quantitative research. Inevitably, the large sample size will push the researcher into simplifying the data numerically. And readers will begin to expect generalizability from such a large sample.
To summarize, “There are no rules for sample size in qualitative inquiry. Sample size depends on what you want to know, the purpose of the inquiry, what’s at stake, what will be useful, what will have credibility, and what can be done with available time and resources” ( Patton 2002:244 ).
How did you find/construct a sample?
Since qualitative researchers work with comparatively small sample sizes, getting your sample right is rather important. Yet it is also difficult to accomplish. For instance, a key question you need to ask yourself is whether you want a homogeneous or heterogeneous sample. In other words, do you want to include people in your study who are by and large the same, or do you want to have diversity in your sample?
For many years, I have studied the experiences of students who were the first in their families to attend university. There is a rather large number of sampling decisions I need to consider before starting the study. (1) Should I only talk to first-in-family students, or should I have a comparison group of students who are not first-in-family? (2) Do I need to strive for a gender distribution that matches undergraduate enrollment patterns? (3) Should I include participants that reflect diversity in gender identity and sexuality? (4) How about racial diversity? First-in-family status is strongly related to some ethnic or racial identity. (5) And how about areas of study?
As you can see, if I wanted to accommodate all these differences and get enough study participants in each category, I would quickly end up with a sample size of hundreds, which is not feasible in most qualitative research. In the end, for me, the most important decision was to maximize the voices of first-in-family students, which meant that I only included them in my sample. As for the other categories, I figured it was going to be hard enough to find first-in-family students, so I started recruiting with an open mind and an understanding that I may have to accept a lack of gender, sexuality, or racial diversity and then not be able to say anything about these issues. But I would definitely be able to speak about the experiences of being first-in-family.
—Wolfgang Lehmann, author of “Habitus Transformation and Hidden Injuries”
Examples of “Sample” Sections in Journal Articles
Think about some of the studies you have read in college, especially those with rich stories and accounts about people’s lives. Do you know how the people were selected to be the focus of those stories? If the account was published by an academic press (e.g., University of California Press or Princeton University Press) or in an academic journal, chances are that the author included a description of their sample selection. You can usually find these in a methodological appendix (book) or a section on “research methods” (article).
Here are two examples from recent books and one example from a recent article:
Example 1 . In It’s Not like I’m Poor: How Working Families Make Ends Meet in a Post-welfare World , the research team employed a mixed methods approach to understand how parents use the earned income tax credit, a refundable tax credit designed to provide relief for low- to moderate-income working people ( Halpern-Meekin et al. 2015 ). At the end of their book, their first appendix is “Introduction to Boston and the Research Project.” After describing the context of the study, they include the following description of their sample selection:
In June 2007, we drew 120 names at random from the roughly 332 surveys we gathered between February and April. Within each racial and ethnic group, we aimed for one-third married couples with children and two-thirds unmarried parents. We sent each of these families a letter informing them of the opportunity to participate in the in-depth portion of our study and then began calling the home and cell phone numbers they provided us on the surveys and knocking on the doors of the addresses they provided.…In the end, we interviewed 115 of the 120 families originally selected for the in-depth interview sample (the remaining five families declined to participate). ( 22 )
Was their sample selection based on convenience or purpose? Why do you think it was important for them to tell you that five families declined to be interviewed? There is actually a trick here, as the names were pulled randomly from a survey whose sample design was probabilistic. Why is this important to know? What can we say about the representativeness or the uniqueness of whatever findings are reported here?
Example 2 . In When Diversity Drops , Park ( 2013 ) examines the impact of decreasing campus diversity on the lives of college students. She does this through a case study of one student club, the InterVarsity Christian Fellowship (IVCF), at one university (“California University,” a pseudonym). Here is her description:
I supplemented participant observation with individual in-depth interviews with sixty IVCF associates, including thirty-four current students, eight former and current staff members, eleven alumni, and seven regional or national staff members. The racial/ethnic breakdown was twenty-five Asian Americans (41.6 percent), one Armenian (1.6 percent), twelve people who were black (20.0 percent), eight Latino/as (13.3 percent), three South Asian Americans (5.0 percent), and eleven people who were white (18.3 percent). Twenty-nine were men, and thirty-one were women. Looking back, I note that the higher number of Asian Americans reflected both the group’s racial/ethnic composition and my relative ease about approaching them for interviews. ( 156 )
How can you tell this is a convenience sample? What else do you note about the sample selection from this description?
Example 3. The last example is taken from an article published in the journal Research in Higher Education . Published articles tend to be more formal than books, at least when it comes to the presentation of qualitative research. In this article, Lawson ( 2021 ) is seeking to understand why female-identified college students drop out of majors that are dominated by male-identified students (e.g., engineering, computer science, music theory). Here is the entire relevant section of the article:
Method Participants Data were collected as part of a larger study designed to better understand the daily experiences of women in MDMs [male-dominated majors].…Participants included 120 students from a midsize, Midwestern University. This sample included 40 women and 40 men from MDMs—defined as any major where at least 2/3 of students are men at both the university and nationally—and 40 women from GNMs—defined as any may where 40–60% of students are women at both the university and nationally.… Procedure A multi-faceted approach was used to recruit participants; participants were sent targeted emails (obtained based on participants’ reported gender and major listings), campus-wide emails sent through the University’s Communication Center, flyers, and in-class presentations. Recruitment materials stated that the research focused on the daily experiences of college students, including classroom experiences, stressors, positive experiences, departmental contexts, and career aspirations. Interested participants were directed to email the study coordinator to verify eligibility (at least 18 years old, man/woman in MDM or woman in GNM, access to a smartphone). Sixteen interested individuals were not eligible for the study due to the gender/major combination. ( 482ff .)
What method of sample selection was used by Lawson? Why is it important to define “MDM” at the outset? How does this definition relate to sampling? Why were interested participants directed to the study coordinator to verify eligibility?
Final Words
I have found that students often find it difficult to be specific enough when defining and choosing their sample. It might help to think about your sample design and sample recruitment like a cookbook. You want all the details there so that someone else can pick up your study and conduct it as you intended. That person could be yourself, but this analogy might work better if you have someone else in mind. When I am writing down recipes, I often think of my sister and try to convey the details she would need to duplicate the dish. We share a grandmother whose recipes are full of handwritten notes in the margins, in spidery ink, that tell us what bowl to use when or where things could go wrong. Describe your sample clearly, convey the steps required accurately, and then add any other details that will help keep you on track and remind you why you have chosen to limit possible interviewees to those of a certain age or class or location. Imagine actually going out and getting your sample (making your dish). Do you have all the necessary details to get started?
Table 5.1. Sampling Type and Strategies
Further Readings
Fusch, Patricia I., and Lawrence R. Ness. 2015. “Are We There Yet? Data Saturation in Qualitative Research.” Qualitative Report 20(9):1408–1416.
Saunders, Benjamin, Julius Sim, Tom Kinstone, Shula Baker, Jackie Waterfield, Bernadette Bartlam, Heather Burroughs, and Clare Jinks. 2018. “Saturation in Qualitative Research: Exploring Its Conceptualization and Operationalization.” Quality & Quantity 52(4):1893–1907.
- Rubin ( 2021 ) suggests a minimum of twenty interviews (but safer with thirty) for an interview-based study and a minimum of three to six months in the field for ethnographic studies. For a content-based study, she suggests between five hundred and one thousand documents, although some will be “very small” ( 243–244 ). ↵
The process of selecting people or other units of analysis to represent a larger population. In quantitative research, this representation is taken quite literally, as statistically representative. In qualitative research, in contrast, sample selection is often made based on potential to generate insight about a particular topic or phenomenon.
The actual list of individuals that the sample will be drawn from. Ideally, it should include the entire target population (and nobody who is not part of that population). Sampling frames can differ from the larger population when specific exclusions are inherent, as in the case of pulling names randomly from voter registration rolls where not everyone is a registered voter. This difference in frame and population can undercut the generalizability of quantitative results.
The specific group of individuals that you will collect data from. Contrast population.
The large group of interest to the researcher. Although it will likely be impossible to design a study that incorporates or reaches all members of the population of interest, this should be clearly defined at the outset of a study so that a reasonable sample of the population can be taken. For example, if one is studying working-class college students, the sample may include twenty such students attending a particular college, while the population is “working-class college students.” In quantitative research, clearly defining the general population of interest is a necessary step in generalizing results from a sample. In qualitative research, defining the population is conceptually important for clarity.
A sampling strategy in which the sample is chosen to represent (numerically) the larger population from which it is drawn by random selection. Each person in the population has an equal chance of making it into the sample. This is often done through a lottery or other chance mechanisms (e.g., a random selection of every twelfth name on an alphabetical list of voters). Also known as random sampling .
The selection of research participants or other data sources based on availability or accessibility, in contrast to purposive sampling .
A sample generated non-randomly by asking participants to help recruit more participants the idea being that a person who fits your sampling criteria probably knows other people with similar criteria.
Broad codes that are assigned to the main issues emerging in the data; identifying themes is often part of initial coding .
A form of case selection focusing on examples that do not fit the emerging patterns. This allows the researcher to evaluate rival explanations or to define the limitations of their research findings. While disconfirming cases are found (not sought out), researchers should expand their analysis or rethink their theories to include/explain them.
A methodological tradition of inquiry and approach to analyzing qualitative data in which theories emerge from a rigorous and systematic process of induction. This approach was pioneered by the sociologists Glaser and Strauss (1967). The elements of theory generated from comparative analysis of data are, first, conceptual categories and their properties and, second, hypotheses or generalized relations among the categories and their properties – “The constant comparing of many groups draws the [researcher’s] attention to their many similarities and differences. Considering these leads [the researcher] to generate abstract categories and their properties, which, since they emerge from the data, will clearly be important to a theory explaining the kind of behavior under observation.” (36).
The result of probability sampling, in which a sample is chosen to represent (numerically) the larger population from which it is drawn by random selection. Each person in the population has an equal chance of making it into the random sample. This is often done through a lottery or other chance mechanisms (e.g., the random selection of every twelfth name on an alphabetical list of voters). This is typically not required in qualitative research but rather essential for the generalizability of quantitative research.
A form of case selection or purposeful sampling in which cases that are unusual or special in some way are chosen to highlight processes or to illuminate gaps in our knowledge of a phenomenon. See also extreme case .
The point at which you can conclude data collection because every person you are interviewing, the interaction you are observing, or content you are analyzing merely confirms what you have already noted. Achieving saturation is often used as the justification for the final sample size.
The accuracy with which results or findings can be transferred to situations or people other than those originally studied. Qualitative studies generally are unable to use (and are uninterested in) statistical generalizability where the sample population is said to be able to predict or stand in for a larger population of interest. Instead, qualitative researchers often discuss “theoretical generalizability,” in which the findings of a particular study can shed light on processes and mechanisms that may be at play in other settings. See also statistical generalization and theoretical generalization .
A term used by IRBs to denote all materials aimed at recruiting participants into a research study (including printed advertisements, scripts, audio or video tapes, or websites). Copies of this material are required in research protocols submitted to IRB.
Introduction to Qualitative Research Methods Copyright © 2023 by Allison Hurst is licensed under a Creative Commons Attribution-ShareAlike 4.0 International License , except where otherwise noted.
- Privacy Policy

Home » Research Methods – Types, Examples and Guide
Research Methods – Types, Examples and Guide
Table of Contents

Research Methods
Definition:
Research Methods refer to the techniques, procedures, and processes used by researchers to collect , analyze, and interpret data in order to answer research questions or test hypotheses. The methods used in research can vary depending on the research questions, the type of data that is being collected, and the research design.
Types of Research Methods
Types of Research Methods are as follows:
Qualitative research Method
Qualitative research methods are used to collect and analyze non-numerical data. This type of research is useful when the objective is to explore the meaning of phenomena, understand the experiences of individuals, or gain insights into complex social processes. Qualitative research methods include interviews, focus groups, ethnography, and content analysis.
Quantitative Research Method
Quantitative research methods are used to collect and analyze numerical data. This type of research is useful when the objective is to test a hypothesis, determine cause-and-effect relationships, and measure the prevalence of certain phenomena. Quantitative research methods include surveys, experiments, and secondary data analysis.
Mixed Method Research
Mixed Method Research refers to the combination of both qualitative and quantitative research methods in a single study. This approach aims to overcome the limitations of each individual method and to provide a more comprehensive understanding of the research topic. This approach allows researchers to gather both quantitative data, which is often used to test hypotheses and make generalizations about a population, and qualitative data, which provides a more in-depth understanding of the experiences and perspectives of individuals.
Key Differences Between Research Methods
The following Table shows the key differences between Quantitative, Qualitative and Mixed Research Methods
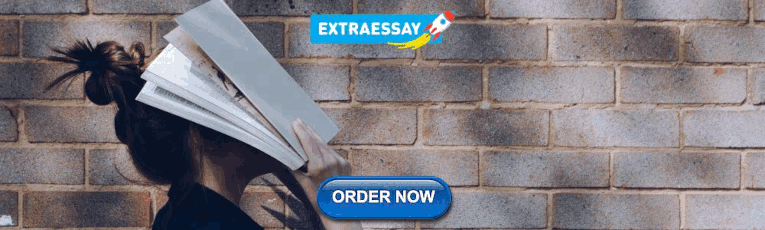
Examples of Research Methods
Examples of Research Methods are as follows:
Qualitative Research Example:
A researcher wants to study the experience of cancer patients during their treatment. They conduct in-depth interviews with patients to gather data on their emotional state, coping mechanisms, and support systems.
Quantitative Research Example:
A company wants to determine the effectiveness of a new advertisement campaign. They survey a large group of people, asking them to rate their awareness of the product and their likelihood of purchasing it.
Mixed Research Example:
A university wants to evaluate the effectiveness of a new teaching method in improving student performance. They collect both quantitative data (such as test scores) and qualitative data (such as feedback from students and teachers) to get a complete picture of the impact of the new method.
Applications of Research Methods
Research methods are used in various fields to investigate, analyze, and answer research questions. Here are some examples of how research methods are applied in different fields:
- Psychology : Research methods are widely used in psychology to study human behavior, emotions, and mental processes. For example, researchers may use experiments, surveys, and observational studies to understand how people behave in different situations, how they respond to different stimuli, and how their brains process information.
- Sociology : Sociologists use research methods to study social phenomena, such as social inequality, social change, and social relationships. Researchers may use surveys, interviews, and observational studies to collect data on social attitudes, beliefs, and behaviors.
- Medicine : Research methods are essential in medical research to study diseases, test new treatments, and evaluate their effectiveness. Researchers may use clinical trials, case studies, and laboratory experiments to collect data on the efficacy and safety of different medical treatments.
- Education : Research methods are used in education to understand how students learn, how teachers teach, and how educational policies affect student outcomes. Researchers may use surveys, experiments, and observational studies to collect data on student performance, teacher effectiveness, and educational programs.
- Business : Research methods are used in business to understand consumer behavior, market trends, and business strategies. Researchers may use surveys, focus groups, and observational studies to collect data on consumer preferences, market trends, and industry competition.
- Environmental science : Research methods are used in environmental science to study the natural world and its ecosystems. Researchers may use field studies, laboratory experiments, and observational studies to collect data on environmental factors, such as air and water quality, and the impact of human activities on the environment.
- Political science : Research methods are used in political science to study political systems, institutions, and behavior. Researchers may use surveys, experiments, and observational studies to collect data on political attitudes, voting behavior, and the impact of policies on society.
Purpose of Research Methods
Research methods serve several purposes, including:
- Identify research problems: Research methods are used to identify research problems or questions that need to be addressed through empirical investigation.
- Develop hypotheses: Research methods help researchers develop hypotheses, which are tentative explanations for the observed phenomenon or relationship.
- Collect data: Research methods enable researchers to collect data in a systematic and objective way, which is necessary to test hypotheses and draw meaningful conclusions.
- Analyze data: Research methods provide tools and techniques for analyzing data, such as statistical analysis, content analysis, and discourse analysis.
- Test hypotheses: Research methods allow researchers to test hypotheses by examining the relationships between variables in a systematic and controlled manner.
- Draw conclusions : Research methods facilitate the drawing of conclusions based on empirical evidence and help researchers make generalizations about a population based on their sample data.
- Enhance understanding: Research methods contribute to the development of knowledge and enhance our understanding of various phenomena and relationships, which can inform policy, practice, and theory.
When to Use Research Methods
Research methods are used when you need to gather information or data to answer a question or to gain insights into a particular phenomenon.
Here are some situations when research methods may be appropriate:
- To investigate a problem : Research methods can be used to investigate a problem or a research question in a particular field. This can help in identifying the root cause of the problem and developing solutions.
- To gather data: Research methods can be used to collect data on a particular subject. This can be done through surveys, interviews, observations, experiments, and more.
- To evaluate programs : Research methods can be used to evaluate the effectiveness of a program, intervention, or policy. This can help in determining whether the program is meeting its goals and objectives.
- To explore new areas : Research methods can be used to explore new areas of inquiry or to test new hypotheses. This can help in advancing knowledge in a particular field.
- To make informed decisions : Research methods can be used to gather information and data to support informed decision-making. This can be useful in various fields such as healthcare, business, and education.
Advantages of Research Methods
Research methods provide several advantages, including:
- Objectivity : Research methods enable researchers to gather data in a systematic and objective manner, minimizing personal biases and subjectivity. This leads to more reliable and valid results.
- Replicability : A key advantage of research methods is that they allow for replication of studies by other researchers. This helps to confirm the validity of the findings and ensures that the results are not specific to the particular research team.
- Generalizability : Research methods enable researchers to gather data from a representative sample of the population, allowing for generalizability of the findings to a larger population. This increases the external validity of the research.
- Precision : Research methods enable researchers to gather data using standardized procedures, ensuring that the data is accurate and precise. This allows researchers to make accurate predictions and draw meaningful conclusions.
- Efficiency : Research methods enable researchers to gather data efficiently, saving time and resources. This is especially important when studying large populations or complex phenomena.
- Innovation : Research methods enable researchers to develop new techniques and tools for data collection and analysis, leading to innovation and advancement in the field.
About the author
Muhammad Hassan
Researcher, Academic Writer, Web developer
You may also like

Questionnaire – Definition, Types, and Examples

Data Collection – Methods Types and Examples

Delimitations in Research – Types, Examples and...

Research Process – Steps, Examples and Tips

Research Design – Types, Methods and Examples

Institutional Review Board – Application Sample...

Research Methodology Example
Detailed Walkthrough + Free Methodology Chapter Template
If you’re working on a dissertation or thesis and are looking for an example of a research methodology chapter , you’ve come to the right place.
In this video, we walk you through a research methodology from a dissertation that earned full distinction , step by step. We start off by discussing the core components of a research methodology by unpacking our free methodology chapter template . We then progress to the sample research methodology to show how these concepts are applied in an actual dissertation, thesis or research project.
If you’re currently working on your research methodology chapter, you may also find the following resources useful:
- Research methodology 101 : an introductory video discussing what a methodology is and the role it plays within a dissertation
- Research design 101 : an overview of the most common research designs for both qualitative and quantitative studies
- Variables 101 : an introductory video covering the different types of variables that exist within research.
- Sampling 101 : an overview of the main sampling methods
- Methodology tips : a video discussion covering various tips to help you write a high-quality methodology chapter
- Private coaching : Get hands-on help with your research methodology
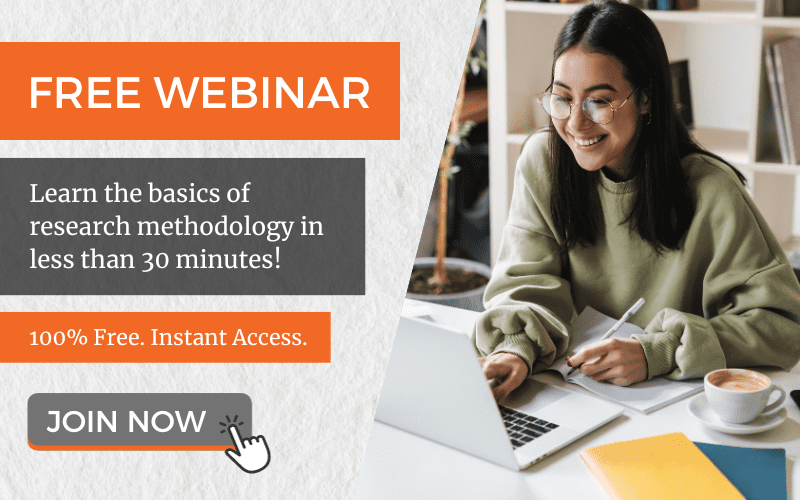
FAQ: Research Methodology Example
Research methodology example: frequently asked questions, is the sample research methodology real.
Yes. The chapter example is an extract from a Master’s-level dissertation for an MBA program. A few minor edits have been made to protect the privacy of the sponsoring organisation, but these have no material impact on the research methodology.
Can I replicate this methodology for my dissertation?
As we discuss in the video, every research methodology will be different, depending on the research aims, objectives and research questions. Therefore, you’ll need to tailor your literature review to suit your specific context.
You can learn more about the basics of writing a research methodology chapter here .
Where can I find more examples of research methodologies?
The best place to find more examples of methodology chapters would be within dissertation/thesis databases. These databases include dissertations, theses and research projects that have successfully passed the assessment criteria for the respective university, meaning that you have at least some sort of quality assurance.
The Open Access Thesis Database (OATD) is a good starting point.
How do I get the research methodology chapter template?
You can access our free methodology chapter template here .
Is the methodology template really free?
Yes. There is no cost for the template and you are free to use it as you wish.
You Might Also Like:
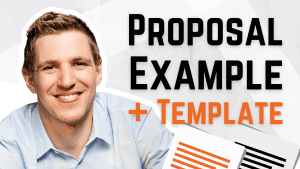
Submit a Comment Cancel reply
Your email address will not be published. Required fields are marked *
Save my name, email, and website in this browser for the next time I comment.
- Print Friendly
- Skip to main content
- Skip to primary sidebar
- Skip to footer
- QuestionPro

- Solutions Industries Gaming Automotive Sports and events Education Government Travel & Hospitality Financial Services Healthcare Cannabis Technology Use Case NPS+ Communities Audience Contactless surveys Mobile LivePolls Member Experience GDPR Positive People Science 360 Feedback Surveys
- Resources Blog eBooks Survey Templates Case Studies Training Help center

Home Market Research
Sample: Definition, Types, Formula & Examples
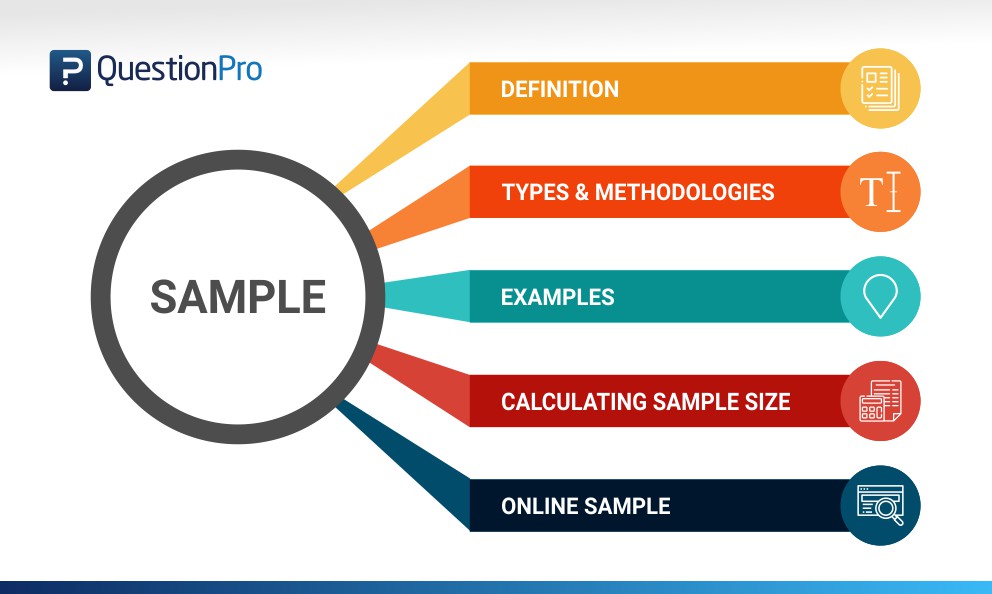
How often do researchers look for the right survey respondents, either for a market research study or an existing survey in the field? The sample or the respondents of this research may be selected from a set of customers or users that are known or unknown.
You may often know your typical respondent profile but don’t have access to the respondents to complete your research study. At such times, researchers and research teams reach out to specialized organizations to access their panel of respondents or buy respondents from them to complete research studies and surveys.
These could be general population respondents that match demographic criteria or respondents based on specific criteria. Such respondents are imperative to the success of research studies.
This article discusses in detail the different types of samples, sampling methods, and examples of each. It also mentions the steps to calculate the size, the details of an online sample, and the advantages of using them.
Content Index
- What is a sample?
Probability sampling methodologies with examples
Non-probability sampling methodologies with examples.
- How to determine a sample size
- Calculating sample size
- Sampling advantages
What is a Sample?
A sample is a smaller set of data that a researcher chooses or selects from a larger population using a pre-defined selection bias method. These elements are known as sample points, sampling units, or observations.
Creating a sample is an efficient method of conducting research . Researching the whole population is often impossible, costly, and time-consuming. Hence, examining the sample provides insights the researcher can apply to the entire population.
For example, if a cell phone manufacturer wants to conduct a feature research study among students in US Universities. An in-depth research study must be conducted if the researcher is looking for features that the students use, features they would like to see, and the price they are willing to pay.
This step is imperative to understand the features that need development, the features that require an upgrade, the device’s pricing, and the go-to-market strategy.
In 2016/17 alone, there were 24.7 million students enrolled in universities across the US. It is impossible to research all these students; the time spent would make the new device redundant, and the money spent on development would render the study useless.
Creating a sample of universities by geographical location and further creating a sample of these students from these universities provides a large enough number of students for research.
Typically, the population for market research is enormous. Making an enumeration of the whole population is practically impossible. The sample usually represents a manageable size of this population. Researchers then collect data from these samples through surveys, polls, and questionnaires and extrapolate this data analysis to the broader community.
LEARN ABOUT: Survey Sampling
Types of Samples: Selection methodologies with examples
The process of deriving a sample is called a sampling method. Sampling forms an integral part of the research design as this method derives the quantitative and qualitative data that can be collected as part of a research study. Sampling methods are characterized into two distinct approaches: probability sampling and non-probability sampling.
Probability sampling is a method of deriving a sample where the objects are selected from a population-based on probability theory. This method includes everyone in the population, and everyone has an equal chance of being selected. Hence, there is no bias whatsoever in this type of sample.
Each person in the population can subsequently be a part of the research. The selection criteria are decided at the outset of the market research study and form an important component of research.
LEARN ABOUT: Action Research
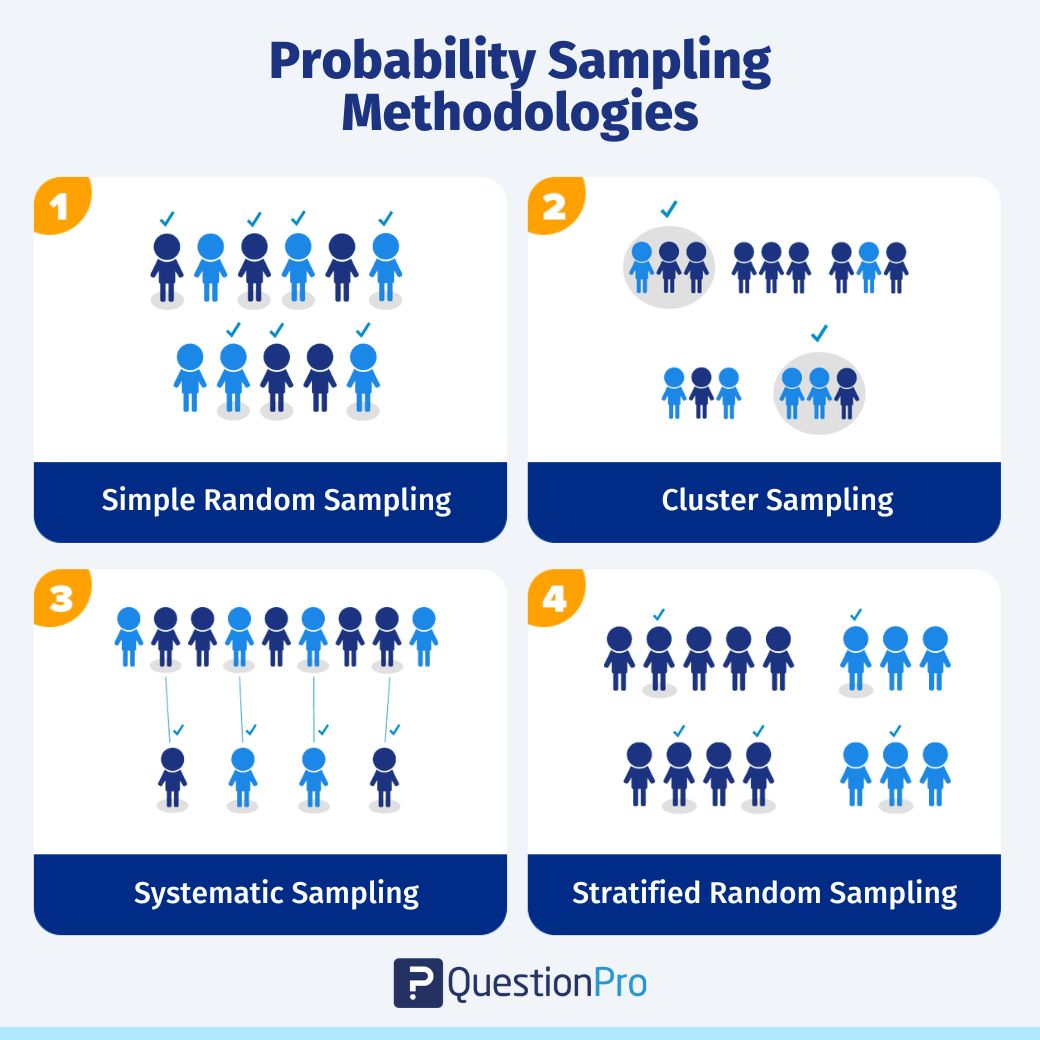
Probability sampling can be further classified into four distinct types of samples. They are:
- Simple random sampling: The most straightforward way of selecting a sample is simple random sampling . In this method, each member has an equal chance of participating in the study. The objects in this sample population are chosen randomly, and each member has the same probability of being selected. For example, if a university dean would like to collect feedback from students about their perception of the teachers and level of education, all 1000 students in the University could be a part of this sample. Any 100 students can be selected randomly to be a part of this sample.
- Cluster sampling: Cluster sampling is a type of sampling method where the respondent population is divided into equal clusters. Clusters are identified and included in a sample based on defining demographic parameters such as age, location, sex, etc. This makes it extremely easy for a survey creator to derive practical inferences from the feedback. For example, if the FDA wants to collect data about adverse side effects from drugs, they can divide the mainland US into distinctive cluster analysis , like states. Research studies are then administered to respondents in these clusters. This type of generating a sample makes the data collection in-depth and provides easy-to-consume and act-upon, insights.
- Systematic sampling: Systematic sampling is a sampling method where the researcher chooses respondents at equal intervals from a population. The approach to selecting the sample is to pick a starting point and then pick respondents at a pre-defined sample interval. For example, while selecting 1,000 volunteers for the Olympics from an application list of 10,000 people, each applicant is given a count of 1 to 10,000. Then starting from 1 and selecting each respondent with an interval of 10, a sample of 1,000 volunteers can be obtained.
- Stratified random sampling: Stratified random sampling is a method of dividing the respondent population into distinctive but pre-defined parameters in the research design phase. In this method, the respondents don’t overlap but collectively represent the whole population. For example, a researcher looking to analyze people from different socioeconomic backgrounds can distinguish respondents by their annual salaries. This forms smaller groups of people or samples, and then some objects from these samples can be used for the research study.
LEARN ABOUT: Purposive Sampling
The non-probability sampling method uses the researcher’s discretion to select a sample. This type of sample is derived mostly from the researcher’s or statistician’s ability to get to this sample.
This type of sampling is used for preliminary research where the primary objective is to derive a hypothesis about the topic in research. Here each member does not have an equal chance of being a part of the sample population, and those parameters are known only post-selection to the sample.
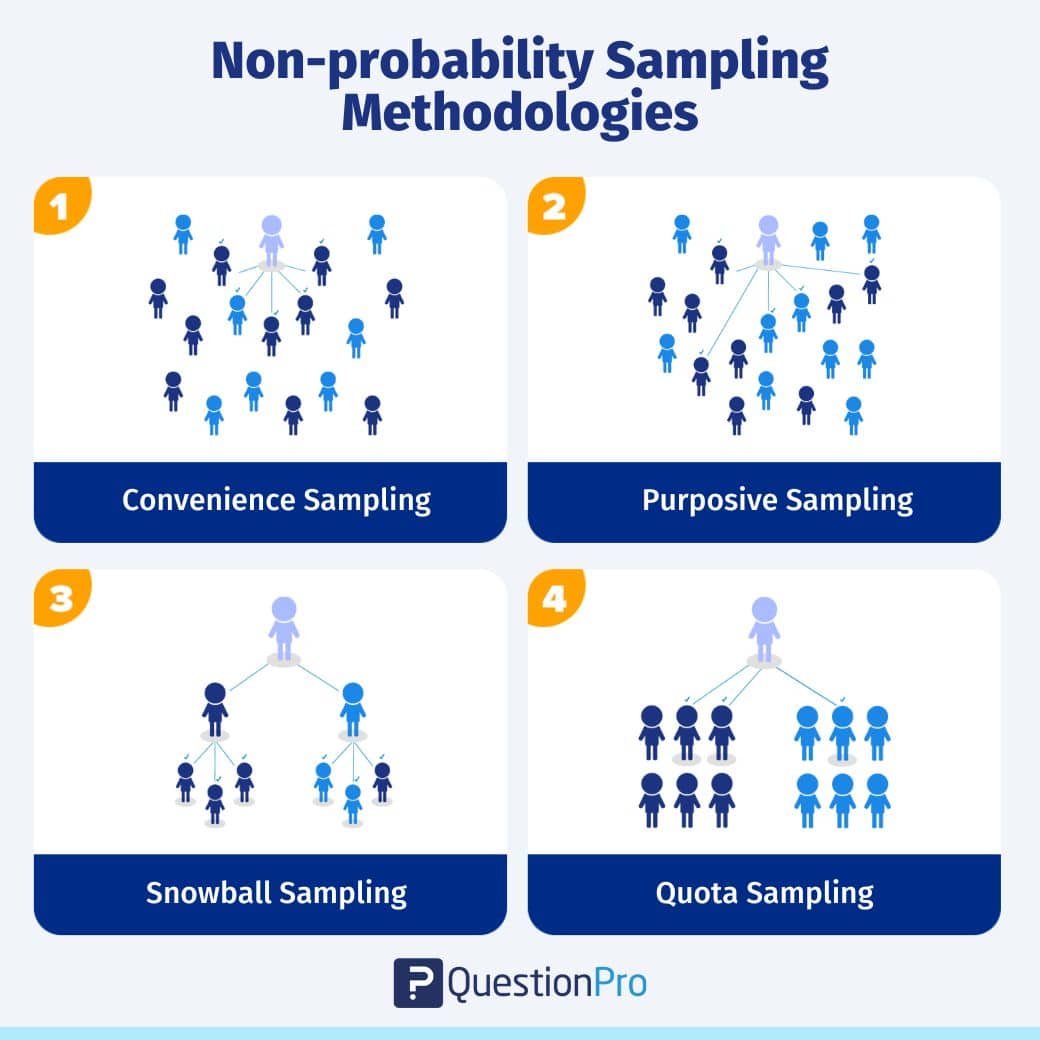
We can classify non-probability sampling into four distinct types of samples. They are:
- Convenience sampling: Convenience sampling , in easy terms, stands for the convenience of a researcher accessing a respondent. There is no scientific method for deriving this sample. Researchers have nearly no authority over selecting the sample elements, and it’s purely done based on proximity and not representativeness.
This non-probability sampling method is used when there is time and costs limitations in collecting feedback. For example, researchers that are conducting a mall-intercept survey to understand the probability of using a fragrance from a perfume manufacturer. In this sampling method, the sample respondents are chosen based on their proximity to the survey desk and willingness to participate in the research.
- Judgemental/purposive sampling: The judgemental or purposive sampling method is a method of developing a sample purely on the basis and discretion of the researcher purely, based on the nature of the study along with his/her understanding of the target audience. This sampling method selects people who only fit the research criteria and end objectives, and the remaining are kept out.
For example, if the research topic is understanding what University a student prefers for Masters, if the question asked is “Would you like to do your Masters?” anything other than a response, “Yes” to this question, everyone else is excluded from this study.
- Snowball sampling: Snowball sampling or chain-referral sampling is defined as a non-probability sampling technique in which the samples have rare traits. This is a sampling technique in which existing subjects provide referrals to recruit samples required for a research study.
For example, while collecting feedback about a sensitive topic like AIDS, respondents aren’t forthcoming with information. In this case, the researcher can recruit people with an understanding or knowledge of such people and collect information from them or ask them to collect information.
- Quota sampling: Quota sampling is a method of collecting a sample where the researcher has the liberty to select a sample based on their strata. The primary characteristic of this method is that two people cannot exist under two different conditions. For example, when a shoe manufacturer would like to understand millennials’ perception of the brand with other parameters like comfort, pricing, etc. It selects only females who are millennials for this study as the research objective is to collect feedback about women’s shoes.
How to determine a Sample Size
As we have learned above, the right sample size determination is essential for the success of data collection in a market research study. But is there a correct number for the sample size? What parameters decide the sample size? What are the distribution methods of the survey?
To understand all of this and make an informed calculation of the right sample size, it is first essential to understand four important variables that form the basic characteristics of a sample. They are:
- Population size: The population size is all the people that can be considered for the research study. This number, in most cases, runs into huge amounts. For example, the population of the United States is 327 million. But in market research, it is impossible to consider all of them for the research study.
- The margin of error (confidence interval): The margin of error is depicted by a percentage that is a statistical inference about the confidence of what number of the population depicts the actual views of the whole population. This percentage helps towards the statistical analysis in selecting a sample and how much sampling error in this would be acceptable.
LEARN ABOUT: Research Process Steps
- Confidence level: This metric measures where the actual mean falls within a confidence interval. The most common confidence intervals are 90%, 95%, and 99%.
- Standard deviation: This metric covers the variance in a survey. A safe number to consider is .5, which would mean that the sample size has to be that large.
Calculating Sample Size
To calculate the sample size, you need the following parameters.
- Z-score: The Z-score value can be found here .
- Standard deviation
- Margin of error
- Confidence level
To calculate use the sample size, use this formula:
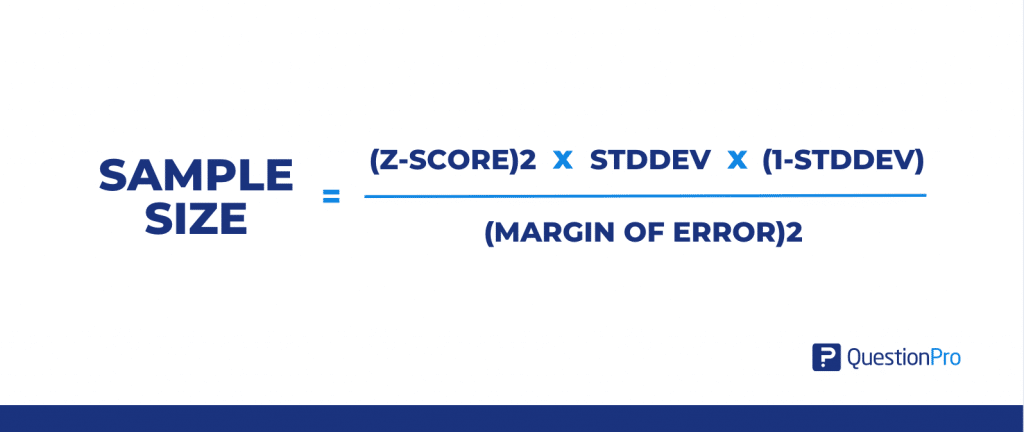
Sample Size = (Z-score)2 * StdDev*(1-StdDev) / (margin of error)2
Consider the confidence level of 90%, standard deviation of .6 and margin of error, +/-4%
((1.64)2 x .6(.6)) / (.04)2
( 2.68x .0.36) / .0016
.9648 / .0016
603 respondents are needed and that becomes your sample size.
Try our sample size calculator to give population, margin of error calculator , and confidence level.
LEARN MORE: Population vs Sample
Sampling Advantages
As shown above, there are many advantages to sampling. Some of the most significant advantages are:
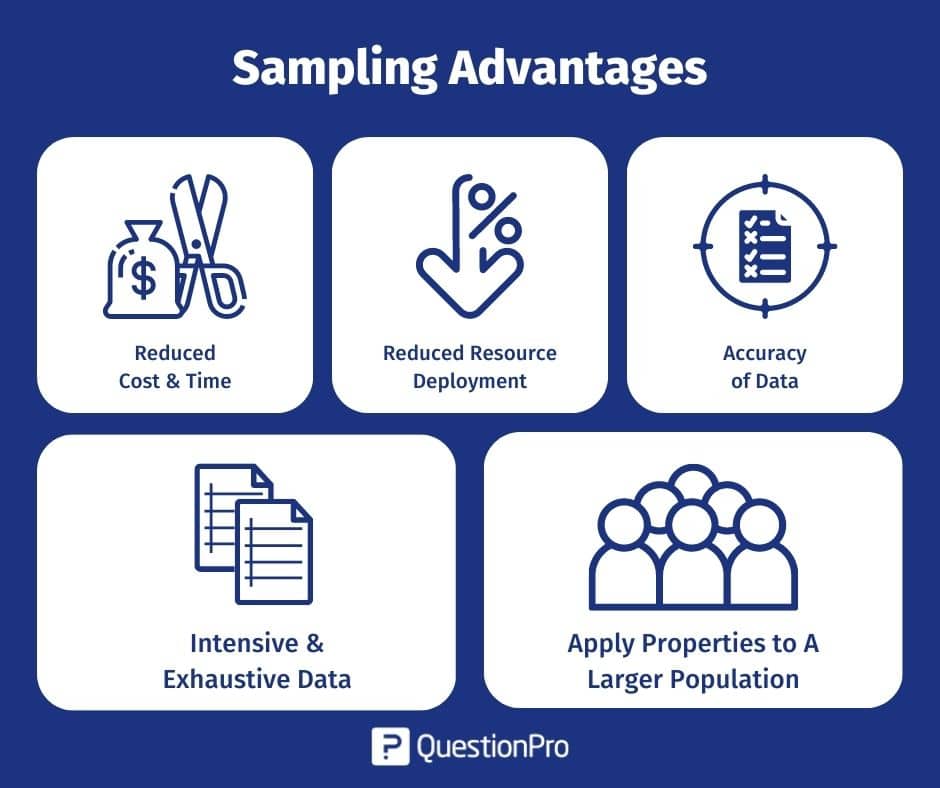
- Reduced cost & time: Since using a sample reduces the number of people that have to be reached out to, it reduces cost and time. Imagine the time saved between researching with a population of millions vs. conducting a research study using a sample.
- Reduced resource deployment: It is obvious that if the number of people involved in a research study is much lower due to the sample, the resources required are also much less. The workforce needed to research the sample is much less than the workforce needed to study the whole population .
- Accuracy of data: Since the sample indicates the population, the data collected is accurate. Also, since the respondent is willing to participate, the survey dropout rate is much lower, which increases the validity and accuracy of the data.
- Intensive & exhaustive data: Since there are lesser respondents, the data collected from a sample is intense and thorough. More time and effort are given to each respondent rather than collecting data from many people.
- Apply properties to a larger population: Since the sample is indicative of the broader population, it is safe to say that the data collected and analyzed from the sample can be applied to the larger population, which would hold true.
To collect accurate data for research, filter bad panelists, and eliminate sampling bias by applying different control measures. If you need any help arranging a sample audience for your next market research project, contact us at [email protected] . We have more than 22 million panelists across the world!
In conclusion, a sample is a subset of a population that is used to represent the characteristics of the entire population. Sampling is essential in research and data analysis to make inferences about a population based on a smaller group of individuals. There are different types of sampling, such as probability sampling, non-probability sampling, and others, each with its own advantages and disadvantages.
Choosing the right sampling method depends on the research question, budget, and resources is important. Furthermore, the sample size plays a crucial role in the accuracy and generalizability of the findings.
This article has provided a comprehensive overview of the definition, types, formula, and examples of sampling. By understanding the different types of sampling and the formulas used to calculate sample size, researchers and analysts can make more informed decisions when conducting research and data unit of analysis .
Sampling is an important tool that enables researchers to make inferences about a population based on a smaller group of individuals. With the right sampling method and sample size, researchers can ensure that their findings are accurate and generalizable to the population.
Utilize one of QuestionPro’s many survey questionnaire samples to help you complete your survey.
When creating online surveys for your customers, employees, or students, one of the biggest mistakes you can make is asking the wrong questions. Different businesses and organizations have different needs required for their surveys.
If you ask irrelevant questions to participants, they’re more likely to drop out before completing the survey. A questionnaire sample template will help set you up for a successful survey.
LEARN MORE SIGN UP FREE
MORE LIKE THIS
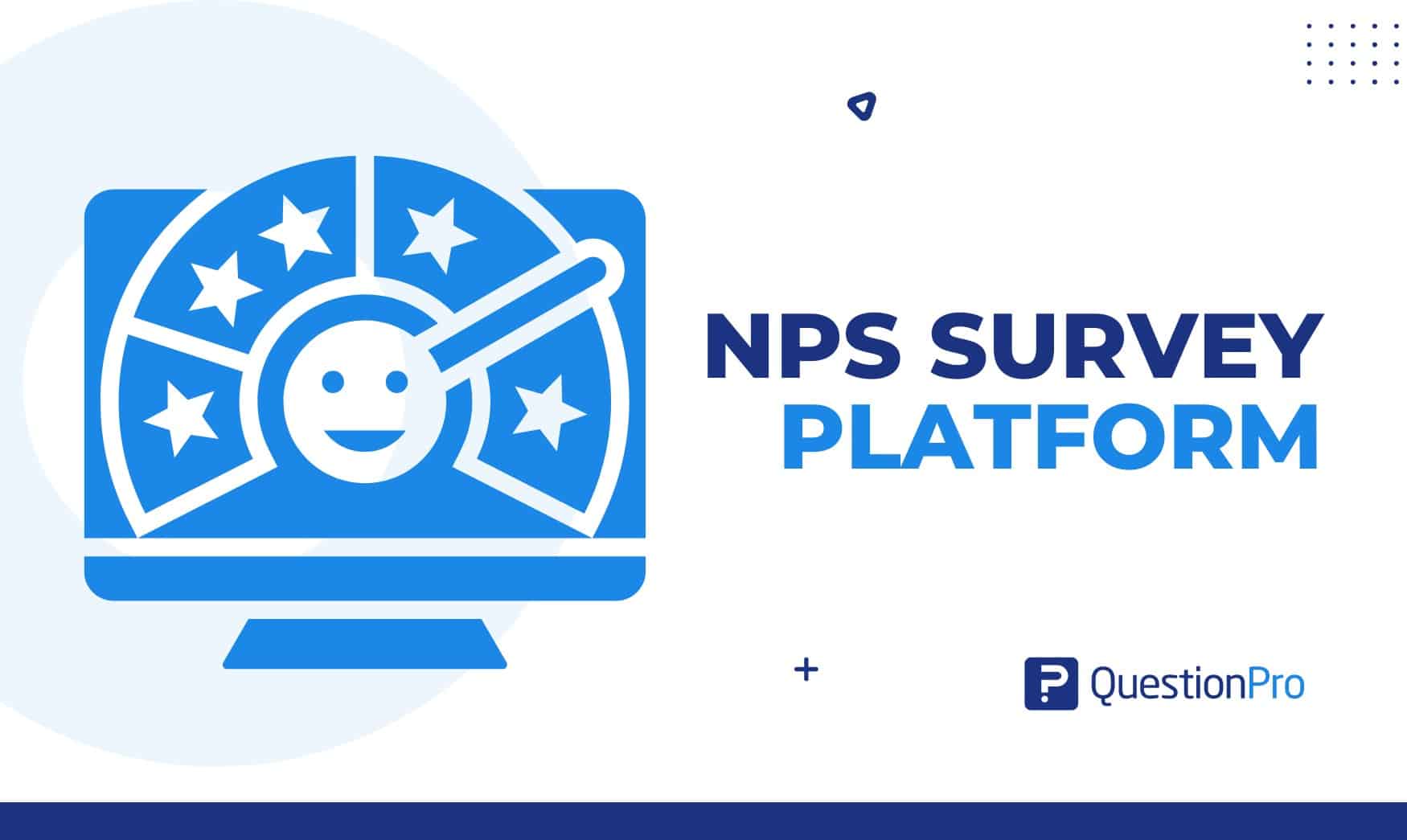
NPS Survey Platform: Types, Tips, 11 Best Platforms & Tools
Apr 26, 2024
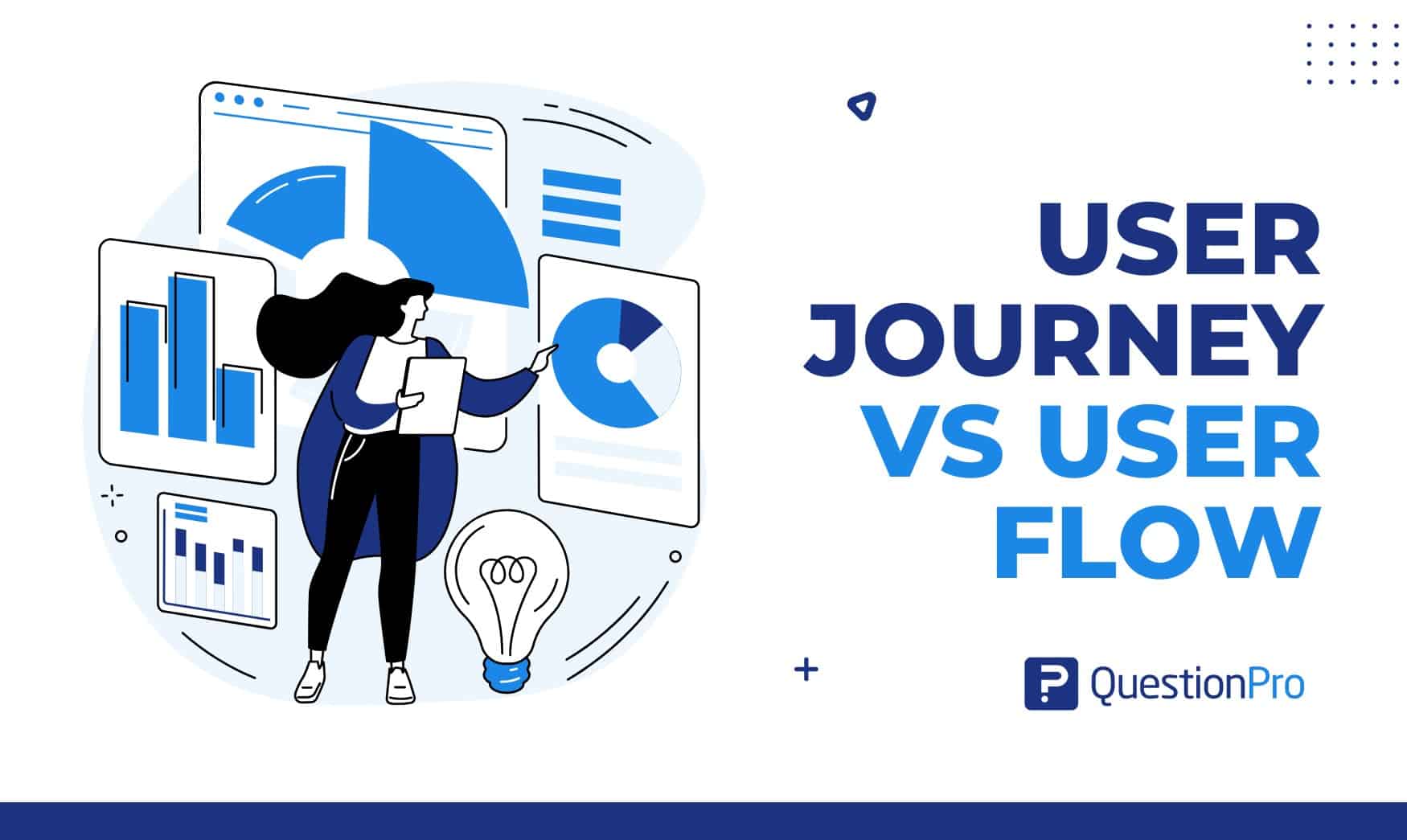
User Journey vs User Flow: Differences and Similarities
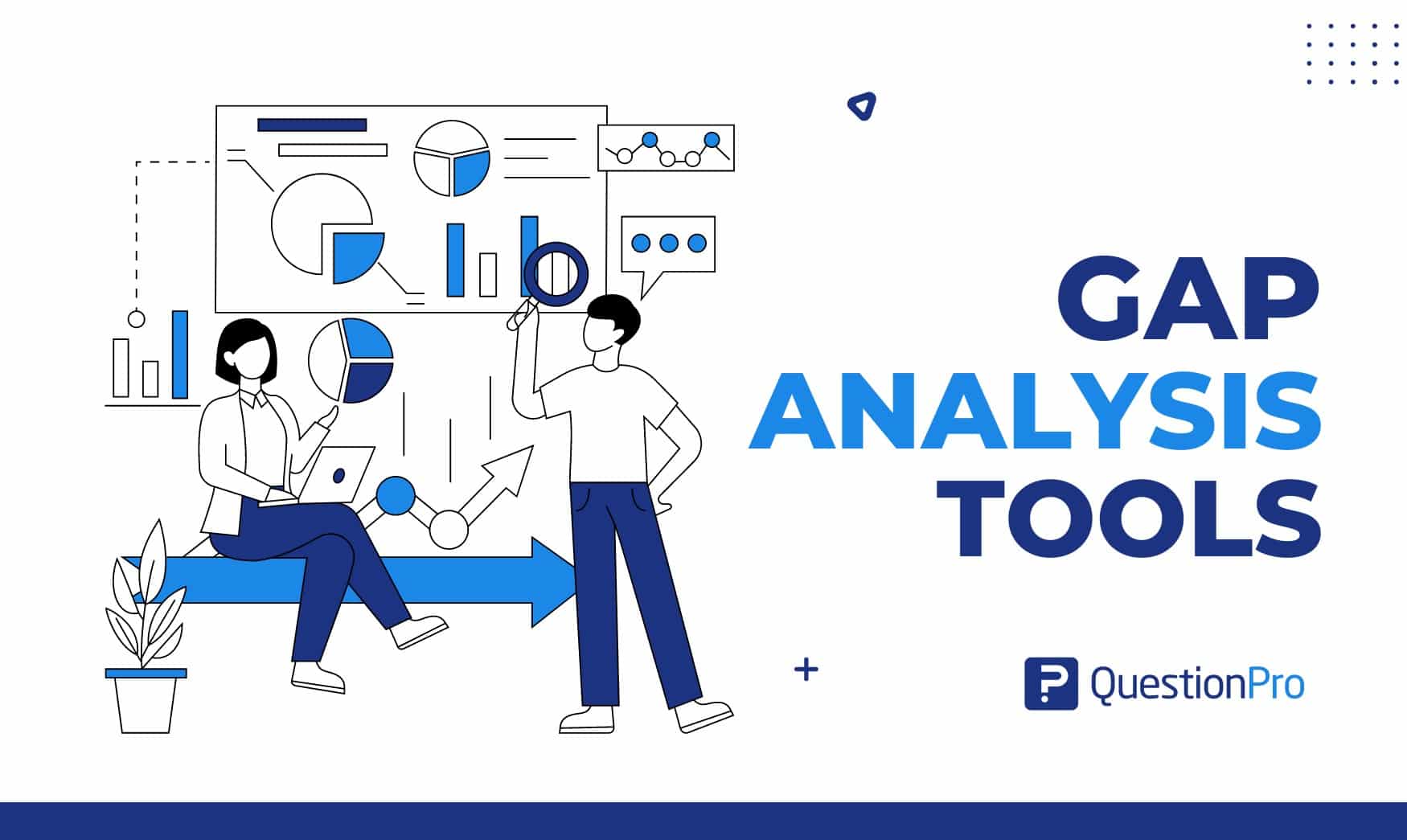
Best 7 Gap Analysis Tools to Empower Your Business
Apr 25, 2024
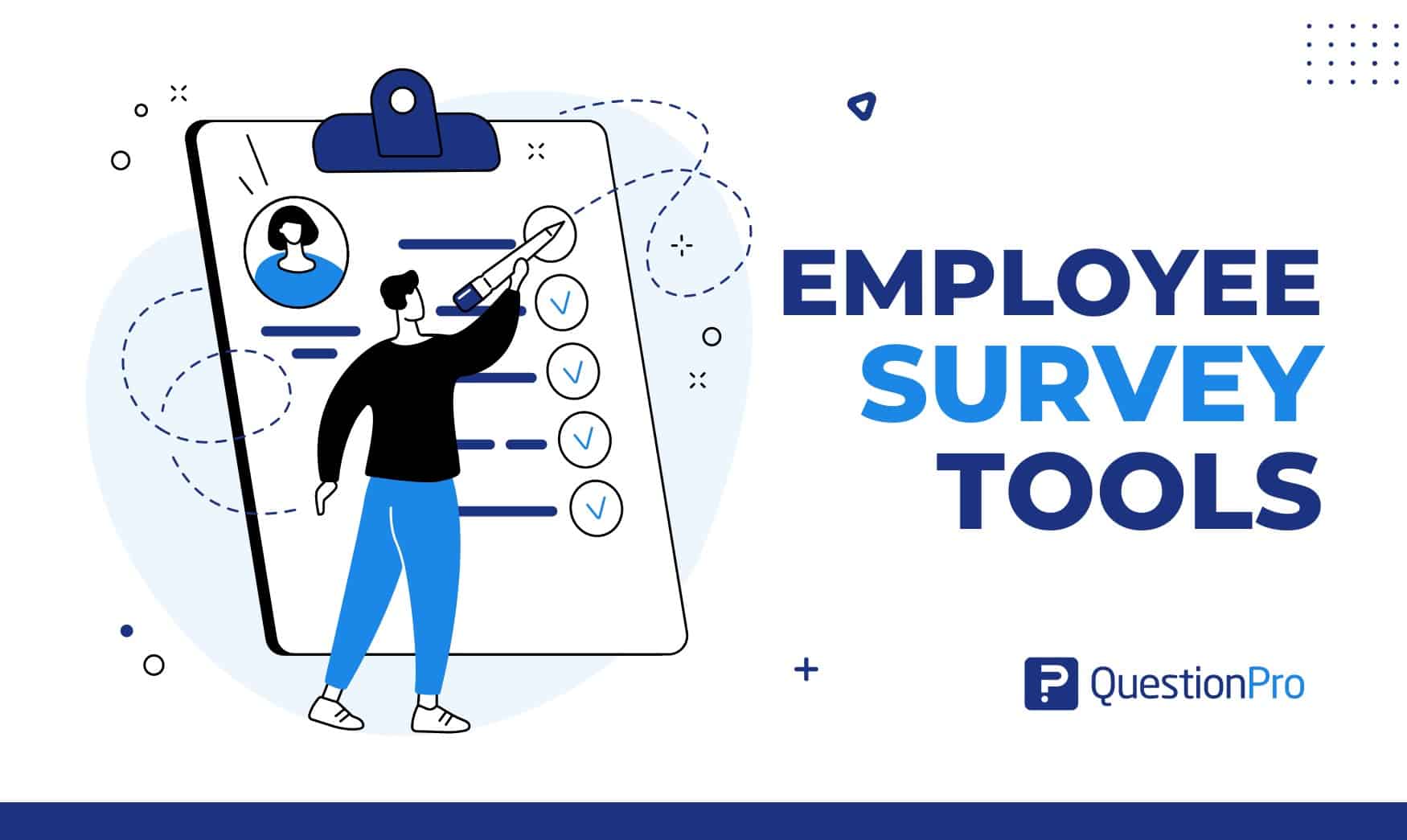
12 Best Employee Survey Tools for Organizational Excellence
Other categories.
- Academic Research
- Artificial Intelligence
- Assessments
- Brand Awareness
- Case Studies
- Communities
- Consumer Insights
- Customer effort score
- Customer Engagement
- Customer Experience
- Customer Loyalty
- Customer Research
- Customer Satisfaction
- Employee Benefits
- Employee Engagement
- Employee Retention
- Friday Five
- General Data Protection Regulation
- Insights Hub
- Life@QuestionPro
- Market Research
- Mobile diaries
- Mobile Surveys
- New Features
- Online Communities
- Question Types
- Questionnaire
- QuestionPro Products
- Release Notes
- Research Tools and Apps
- Revenue at Risk
- Survey Templates
- Training Tips
- Uncategorized
- Video Learning Series
- What’s Coming Up
- Workforce Intelligence
Numbers, Facts and Trends Shaping Your World
Read our research on:
Full Topic List
Regions & Countries
- Publications
- Our Methods
- Short Reads
- Tools & Resources
Read Our Research On:
Methodology: 2023 focus groups of Asian Americans
Methodology: 2022-23 survey of asian americans, table of contents.
- About the focus groups
- Participant recruitment procedures
- Moderator and interpreter qualification
- Data analysis
- Sample design
- Data collection
- Weighting and variance estimation
- Analysis of Asians living in poverty
- Acknowledgments
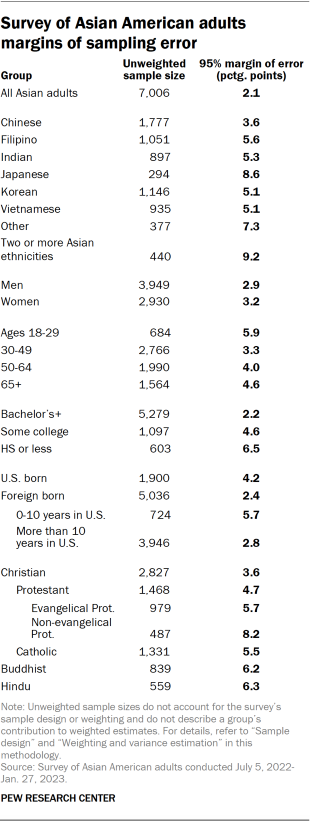
The survey analysis is drawn from a national cross-sectional survey conducted for Pew Research Center by Westat. The sampling design of the survey was an address-based sampling (ABS) approach, supplemented by list samples, to reach a nationally representative group of respondents. The survey was fielded July 5, 2022, through Jan. 27, 2023. Self-administered screening interviews were conducted with a total of 36,469 U.S. adults either online or by mail, resulting in 7,006 interviews with Asian American adults. It is these 7,006 Asian Americans who are the focus of this report. After accounting for the complex sample design and loss of precision due to weighting, the margin of sampling error for these respondents is plus or minus 2.1 percentage points at the 95% level of confidence.
The survey was administered in two stages. In the first stage, a short screening survey was administered to a national sample of U.S. adults to collect basic demographics and determine a respondent’s eligibility for the extended survey of Asian Americans. Screener respondents were considered eligible for the extended survey if they self-identified as Asian (alone or in combination with any other race or ethnicity). Note that all individuals who self-identified as Asian were asked to complete the extended survey.
To maintain consistency with the Census Bureau’s definition of “Asian,” individuals responding as Asian but who self-identified with origins that did not meet the bureau’s official standards prior to the 2020 decennial census were considered ineligible and were not asked to complete the extended survey or were removed from the final sample. Those excluded were people solely of Southwest Asian descent (e.g., Lebanese, Saudi), those with Central Asian origins (e.g., Afghan, Uzbek) as well as various other non-Asian origins. The impact of excluding these groups is small, as together they represent about 1%-2% of the national U.S. Asian population, according to a Pew Research Center analysis of the 2021 American Community Survey.
Eligible survey respondents were asked in the extended survey how they identified ethnically (for example: Chinese, Filipino, Indian, Korean, Vietnamese, or some other ethnicity with a write-in option). Note that survey respondents were asked about their ethnicity rather than nationality. For example, those classified as Chinese in the survey are those self-identifying as of Chinese ethnicity, rather than necessarily being a citizen or former citizen of the People’s Republic of China. Since this is an ethnicity, classification of survey respondents as Chinese also includes those who are Taiwanese.
The research plan for this project was submitted to Westat’s institutional review board (IRB), which is an independent committee of experts that specializes in helping to protect the rights of research participants. Due to the minimal risks associated with this questionnaire content and the population of interest, this research underwent an expedited review and received approval (approval #FWA 00005551).
Throughout this methodology statement, the terms “extended survey” and “extended questionnaire” refer to the extended survey of Asian Americans that is the focus of this report, and “eligible adults” and “eligible respondents” refer to those individuals who met its eligibility criteria, unless otherwise noted.
The survey had a complex sample design constructed to maximize efficiency in reaching Asian American adults while also supporting reliable, national estimates for the population as a whole and for the five largest ethnic groups (Chinese, Filipino, Indian, Korean and Vietnamese). Asian American adults include those who self-identify as Asian, either alone or in combination with other races or Hispanic identity.
The main sample frame of the 2022-2023 Asian American Survey is an address-based sample (ABS). The ABS frame of addresses was derived from the USPS Computerized Delivery Sequence file. It is maintained by Marketing Systems Group (MSG) and is updated monthly. MSG geocodes their entire ABS frame, so block, block group, and census tract characteristics from the decennial census and the American Community Survey (ACS) could be appended to addresses and used for sampling and data collection.
All addresses on the ABS frame were geocoded to a census tract. Census tracts were then grouped into three strata based on the density of Asian American adults, defined as the proportion of Asian American adults among all adults in the tract. The three strata were defined as:
- High density: Tracts with an Asian American adult density of 10% or higher
- Medium density: Tracts with a density 3% to less than 10%
- Low density: Tracts with a density less than 3%
Mailing addresses in census tracts from the lowest density stratum, strata 3, were excluded from the sampling frame. As a result, the frame excluded 54.1% of the 2020 census tracts, 49.1% of the U.S. adult population, including 9.1% of adults who self-identified as Asian alone or in combination with other races or Hispanic ethnicity. For the largest five Asian ethnic subgroups, Filipinos had the largest percentage of excluded adults, with 6.8%, while Indians had the lowest with 4.2% of the adults. Addresses were then sampled from the two remaining strata. This stratification and the assignment of differential sampling rates to the strata were critical design components because of the rareness of the Asian American adult population.
Despite oversampling of the high- and medium-density Asian American strata in the ABS sample, the ABS sample was not expected to efficiently yield the required number of completed interviews for some ethnic subgroups. Therefore, the ABS sample was supplemented with samples from the specialized surname list frames maintained by the MSG. These list frames identify households using commercial databases linked to addresses and telephone numbers. The individuals’ surnames in these lists could be classified by likely ethnic origin. Westat requested MSG to produce five list frames: Chinese, Filipino, Indian, Korean and Vietnamese. The lists were subset to include only cases with a mailing address. Addresses sampled from the lists, unlike those sampled from the ABS frame, were not limited to high- and medium-density census tracts.
Once an address was sampled from either the ABS frame or the surname lists, an invitation was mailed to the address. The invitation requested that the adult in the household with the next birthday complete the survey.
To maximize response, the survey used a sequential mixed-mode protocol in which sampled households were first directed to respond online and later mailed a paper version of the questionnaire if they did not respond online.

The first mailing was a letter introducing the survey and providing the information necessary (URL and unique PIN) for online response. A pre-incentive of $2 was included in the mailing. This and remaining screener recruitment letters focused on the screener survey, without mentioning the possibility of eligibility for a longer survey and associated promised incentive, since most people would only be asked to complete the short screening survey. It was important for all households to complete the screening survey, not just those who identify as Asian American. As such, the invitation did not mention that the extended survey would focus on topics surrounding the Asian American experience. The invitation was generic to minimize the risk of nonresponse bias due to topic salience bias.
After one week, Westat sent a postcard reminder to all sampled individuals, followed three weeks later by a reminder letter to nonrespondents. Approximately 8.5 weeks after the initial mailing, Westat sent nonrespondents a paper version screening survey, which was a four-page booklet (one folded 11×17 paper) and a postage-paid return envelope in addition to the cover letter. If no response was obtained from those four mailings, no further contact was made.
Eligible adults who completed the screening interview on the web were immediately asked to continue with the extended questionnaire. If an eligible adult completed the screener online but did not complete the extended interview, Westat sent them a reminder letter. This was performed on a rolling basis when it had been at least one week since the web breakoff. Names were not collected until the end of the web survey, so these letters were addressed to “Recent Participant.”
If an eligible respondent completed a paper screener, Westat mailed them the extended survey and a postage-paid return envelope. This was sent weekly as completed paper screeners arrived. Westat followed these paper mailings with a reminder postcard. Later, Westat sent a final paper version via FedEx to eligible adults who had not completed the extended interview online or by paper.
A pre-incentive of $2 (in the form of two $1 bills) was sent to all sampled addresses with the first letter, which provided information about how to complete the survey online. This and subsequent screener invitations only referred to the pre-incentive without reference to the possibility of later promised incentives.
Respondents who completed the screening survey and were found eligible were offered a promised incentive of $10 to go on and complete the extended survey. All participants who completed the extended web survey were offered their choice of a $10 Amazon.com gift code instantly or $10 cash mailed. All participants who completed the survey via paper were mailed a $10 cash incentive.
In December 2022 a mailing was added for eligible respondents who had completed a screener questionnaire, either by web or paper but who had not yet completed the extended survey. It was sent to those who had received their last mailing in the standard sequence at least four weeks earlier. It included a cover letter, a paper copy of the extended survey, and a business reply envelope, and was assembled in a 9×12 envelope with a $1 bill made visible through the envelope window.
In the last month of data collection, an additional mailing was added to boost the number of Vietnamese respondents. A random sample of 4,000 addresses from the Vietnamese surname list and 2,000 addresses from the ABS frame who were flagged as likely Vietnamese were sent another copy of the first invitation letter, which contained web login credentials but no paper copy of the screener. This was sent in a No. 10 envelope with a wide window and was assembled with a $1 bill visible through the envelope window.
The mail and web screening and extended surveys were developed in English and translated into Chinese (Simplified and Traditional), Hindi, Korean, Tagalog and Vietnamese. For web, the landing page was displayed in English initially but included banners at the top and bottom of the page that allowed respondents to change the displayed language. Once in the survey, a dropdown button at the top of each page was available to respondents to toggle between languages.
The paper surveys were also formatted into all six languages. Recipients thought to be more likely to use a specific language option, based on supplemental information in the sampling frame or their address location, were sent a paper screener in that language in addition to an English screener questionnaire. Those receiving a paper extended instrument were sent the extended survey in the language in which the screener was completed. For web, respondents continued in their selected language from the screener.
Household-level weighting
The first step in weighting was creating a base weight for each sampled mailing address to account for its probability of selection into the sample. The base weight for mailing address k is called BW k and is defined as the inverse of its probability of selection. The ABS sample addresses had a probability of selection based on the stratum from which they were sampled. The supplemental samples (i.e., Chinese, Filipino, Indian, Korean and Vietnamese surname lists) also had a probability of selection from the list frames. Because all of the addresses in the list frames are also included in the ABS frame, these addresses had multiple opportunities for these addresses to be selected, and the base weights include an adjustment to account for their higher probability of selection.
Each sampled mailing address was assigned to one of four categories according to its final screener disposition. The categories were 1) household with a completed screener interview, 2) household with an incomplete screener interview, 3) ineligible (i.e., not a household, which were primarily postmaster returns), and 4) addresses for which status was unknown (i.e., addresses that were not identified as undeliverable by the USPS but from which no survey response was received).
The second step in the weighting process was adjusting the base weight to account for occupied households among those with unknown eligibility (category 4). Previous ABS studies have found that about 13% of all addresses in the ABS frame were either vacant or not home to anyone in the civilian, non-institutionalized adult population. For this survey, it was assumed that 87% of all sampled addresses from the ABS frame were eligible households. However, this value was not appropriate for the addresses sampled from the list frames, which were expected to have a higher proportion of households as these were maintained lists. For the list samples, the occupied household rate was computed as the proportion of list cases in category 3 compared to all resolved list cases (i.e., the sum of categories 1 through 3). The base weights for the share of category 4 addresses (unknown eligibility) assumed to be eligible were then allocated to cases in categories 1 and 2 (known households) so that the sum of the combined category 1 and 2 base weights equaled the number of addresses assumed to be eligible in each frame. The category 3 ineligible addresses were given a weight of zero.
The next step was adjusting for nonresponse for households without a completed screener interview to create a final household weight. This adjustment allocated the weights of nonrespondents (category 2) to those of respondents (category 1) within classes defined by the cross-classification of sampling strata, census region, and sample type (e.g., ABS and list supplemental samples). Those classes with fewer than 50 sampled addresses or large adjustment factors were collapsed with nearby cells within the sample type. Given the large variance in the household weights among the medium density ABS stratum, final household weights for addresses within this stratum were capped at 300.
Weighting of extended survey respondents
The extended interview nonresponse adjustment began by assigning each case that completed the screener interview to one of three dispositions: 1) eligible adult completed the extended interview; 2) eligible adult did not complete the extended interview; and 3) not eligible for the extended interview.
An initial adult base weight was calculated for the cases with a completed extended interview as the product of the truncated number of adults in the household (max value of 3) and the household weight. This adjustment accounted for selecting one adult in each household.
The final step in the adult weighting was calibrating the adult weights for those who completed the extended interview so that the calibrated weights (i.e., the estimated number of adults) aligned with benchmarks for non-institutionalized Asian adults from the 2016-2020 American Community Surveys Public Use Microdata Sample (PUMS). Specifically, raking was used to calibrate the weights on the following dimensions:
- Ethnic group (Chinese, Filipino, Indian, Japanese, Korean, Vietnamese, other single Asian ethnicities, and multiple Asian ethnicities)
- Collapsed ethnic group (Chinese, Filipino, Indian, Korean, Vietnamese, all other single and multiple Asian ethnicities) by age group
- Collapsed ethnic group by sex
- Collapsed ethnic group by census region
- Collapsed ethnic group by education
- Collapsed ethnic group by housing tenure
- Collapsed ethnic group by nativity
- Income group by number of persons in the household
The control totals used in raking were based on the entire population of Asian American adults (including those who live in the excluded stratum) to correct for both extended interview nonresponse and undercoverage from excluding the low-density stratum in the ABS frame.
Variance estimation
Because the modeled estimates used in the weighting are themselves subject to sampling error, variance estimation and tests of statistical significance were performed using the grouped jackknife estimator ( JK 2). One hundred sets of replicates were created by deleting a group of cases within each stratum from each replicate and doubling the weights for a corresponding set of cases in the same stratum. The entire weighting and modeling process was performed on the full sample and then separately repeated for each replicate. The result is a total of 101 separate weights for each respondent that have incorporated the variability from the complex sample design. 1
Response rates
Westat assigned all sampled cases a result code for their participation in the screener, and then they assigned a result for the extended questionnaire for those who were eligible for the survey of Asian Americans. Two of the dispositions warrant some discussion. One is the category “4.313 No such address.” This category is for addresses that were returned by the U.S. Postal Service as not being deliverable. This status indicates the address, which was on the USPS Delivery Sequence File at the time of sampling, currently is not occupied or no longer exists. The second category is “4.90 Other.” This category contains 588 addresses that were never mailed because they had a drop count of greater than four. Drop points are addresses with multiple households that share the same address. The information available in the ABS frame on drop points is limited to the number of drop points at the address, without information on the type of households at the drop point, or how they should be labeled for mailing purposes. In this survey, all drop points were eligible for sampling, but only those with drop point counts of four or fewer were mailed. Westat treated drop point counts of five or more as out of scope, and no mailing was done for those addresses.
Westat used the disposition results to compute response rates consistent with AAPOR definitions. The response rates are weighted by the base weight to account for the differential sampling in this survey. The AAPOR RR3 response rate to the screening interview was 17.0%. 2 The RR1 response rate to the extended Asian American interview (77.9%) is the number of eligible adults completing the questionnaire over the total sampled for that extended questionnaire. The overall response rate is the product of the screener response rate and the conditional response rate for the extended questionnaire. The overall response rate for the Asian American sample in the Pew Research Center survey was 13.3% (17.0% x 77.9%).
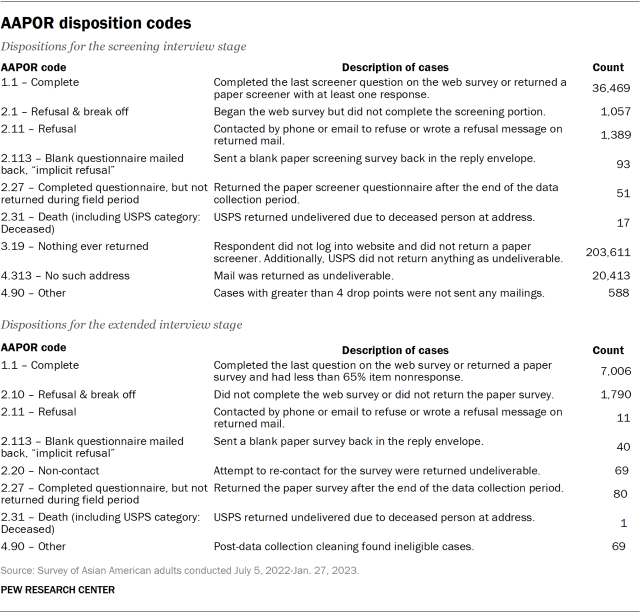
Survey analysis of Asian adults living in poverty is based on 561 respondents of the 2022-23 survey of Asian Americans whose approximate family income falls at or below the 2022 federal poverty line published by the U.S. Department of Health and Human Services (HHS).
The survey asked respondents to choose their family income brackets in the 12 months prior to the survey. These income brackets were converted into dollars in the following ways:
- For those reporting a family income of less than $12,500, $12,499 was used as a proxy for their family income.
- For respondents reporting income brackets that are between $12,500 and $149,999, the midpoint of the selected income bracket was used as a proxy. For example, if they chose “$12,500 to $14,999,” $13,750 was used.
- For respondents reporting a family income of $150,000 or more, $150,000 was used.
The survey also asked respondents how many adults ages 18 or older live in their household including themselves, from one to 10 adults. Additionally, the survey asked how many children under 18 live in their household, from zero to 10 children. These responses were used to calculate their total family (household) size. Asian adults were categorized as “living near or below the poverty line” if their approximate family income, after being adjusted for family size, falls at or below 100% of the 2022 federal poverty line. Respondents with a household size of four were categorized as “living near or below the poverty line” if their approximate family income is $27,750 or less. 3 All Asian adults whose approximate family income is $12,499 were categorized as “living near or below” the poverty line regardless of family size, since those respondents have an income under the 2022 federal poverty line for a family of one. All Asian adults who meet the criteria above are used for the analysis of Asians in poverty, irrespective of their status as students or not.
A number of sensitivity checks were performed to test the robustness of the findings, and the main conclusions were consistently upheld. These sensitivity checks included using the poverty thresholds published by the Census Bureau instead of the poverty line published by HHS to define Asians in poverty, and excluding full-time students from the analysis even if their family income falls at or below the poverty line.
- For additional details on jackknife replication, refer to Rust, K.F., and J.N.K. Rao. 1996. “ Variance estimation for complex surveys using replication techniques .” Statistical Methods in Medical Research. ↩
- The weighted share of unscreened households assumed to be eligible for the screener interview (occupied “e”) was 87%. ↩
- The U.S. Department of Health and Human Services has separate poverty guidelines for the 48 contiguous states and the District of Columbia, Alaska and Hawaii. For all respondents in the 2022-23 survey of Asian Americans, poverty status was determined by applying the federal poverty line for the 48 contiguous states and D.C., regardless of respondents’ state of residence. ↩
Sign up for our weekly newsletter
Fresh data delivery Saturday mornings
Sign up for The Briefing
Weekly updates on the world of news & information
- Asian Americans
- Homeownership & Renting
- Income & Wages
- Income, Wealth & Poverty
- Personal Finances
Key facts about Asian Americans living in poverty
1 in 10: redefining the asian american dream (short film), the hardships and dreams of asian americans living in poverty, key facts about asian american eligible voters in 2024, striking findings from 2023, most popular, report materials.
- Moderator Guide
1615 L St. NW, Suite 800 Washington, DC 20036 USA (+1) 202-419-4300 | Main (+1) 202-857-8562 | Fax (+1) 202-419-4372 | Media Inquiries
Research Topics
- Age & Generations
- Coronavirus (COVID-19)
- Economy & Work
- Family & Relationships
- Gender & LGBTQ
- Immigration & Migration
- International Affairs
- Internet & Technology
- Methodological Research
- News Habits & Media
- Non-U.S. Governments
- Other Topics
- Politics & Policy
- Race & Ethnicity
- Email Newsletters
ABOUT PEW RESEARCH CENTER Pew Research Center is a nonpartisan fact tank that informs the public about the issues, attitudes and trends shaping the world. It conducts public opinion polling, demographic research, media content analysis and other empirical social science research. Pew Research Center does not take policy positions. It is a subsidiary of The Pew Charitable Trusts .
Copyright 2024 Pew Research Center
Terms & Conditions
Privacy Policy
Cookie Settings
Reprints, Permissions & Use Policy
Certification of the total element mass fractions in UME EnvCRM 03 soil sample via a joint research project
- Practitioner's Report
- Published: 23 April 2024
Cite this article
- Alper Isleyen 1 ,
- Suleyman Z. Can 1 ,
- Oktay Cankur 1 ,
- Murat Tunc 1 ,
- Jochen Vogl 2 ,
- Maren Koenig 2 ,
- Milena Horvat 3 ,
- Radojko Jacimovic 3 ,
- Tea Zuliani 3 ,
- Vesna Fajon 3 ,
- Aida Jotanovic 4 ,
- Luka Gaževic 5 ,
- Milena Milosevic 5 ,
- Maria Ochsenkuehn–Petropoulou 6 ,
- Fotis Tsopelas 6 ,
- Theopisti Lymberopoulou 6 ,
- Lamprini-Areti Tsakanika 6 ,
- Olga Serifi 6 ,
- Klaus M. Ochsenkuehn 6 ,
- Ewa Bulska 7 ,
- Anna Tomiak 7 ,
- Eliza Kurek 7 ,
- Zehra Cakılbahçe 1 ,
- Gokhan Aktas 1 ,
- Hatice Altuntas 1 ,
- Elif Basaran 1 ,
- Barıs Kısacık 1 &
- Zeynep Gumus 1
19 Accesses
Explore all metrics
Soil certified reference material (CRM), UME EnvCRM 03 was produced by a collaborative approach among national metrology institutes, designated institutes and university research laboratories within the scope of the EMPIR project: Matrix Reference Materials for Environmental Analysis. This paper presents the sampling and processing methodology, homogeneity, stability, characterization campaign, the assignment of property values and their associated uncertainties in compliance with ISO 17034:2016. The material processing methodology involves blending a natural soil sample with a contaminated soil sample obtained by spiking elemental solutions for 8 elements (Cd, Co, Cu, Hg, Ni, Pb, Sb and Zn) to reach the level of warning risk monitoring values specified for metals and metalloids of soils in Europe. Comparative homogeneity and stability test data were obtained by two different institutes, ensuring the reliability and back up of the data. The certified values and associated expanded uncertainties for the total mass fractions of thirteen elements (As, Cd, Co, Cr, Cu, Fe, Hg, Mn, Ni, Pb, Sb, V and Zn) are established. The developed CRM can be used for the development and validation of measurement procedures for the determination of the total mass fractions of elements in soil and also for quality control/assurance purposes. The developed CRM is the first example of a soil material originating from Türkiye.
This is a preview of subscription content, log in via an institution to check access.
Access this article
Price includes VAT (Russian Federation)
Instant access to the full article PDF.
Rent this article via DeepDyve
Institutional subscriptions
Matrix reference materials for environmental analysis EURAMET project page. https://www.euramet.org/research-innovation/search-research-projects/details/project/matrix-reference-materials-for-environmental-analysis
ISO 17034 (2016) General requirements for the competence of reference materials producers
Vassileva E, Azemard S, Mandjukov P (2017) Certification for trace elements and methyl mercury mass fractions in IAEA-456 marine sediment sample Accred. Qual Assur 23:29–37
Google Scholar
Mackey EA, Christopher SJ, Lindstrom RM, Long SE, Marlow AF, Murphy KE, Paul RL, Popelka-Filcoff RS, Rabb SA, Sieber JR, Spatz RO, Tomlin BE, Wood LJ, Yu LL, Zeisler R, Yen JH, Wilson SA, Adams MG, Brown ZA, Lamothe PL, Taggart JE, Jones C, Nebelsick J (2010) NIST special publication 260–172, certification of three NIST renewal soil standard reference materials for element content: SRM 2709a San Joaquin Soil, SRM 2710a Montana Soil I, and SRM 2711a Montana Soil II
Birgersson-Liebich A, Venelinov T, Santoro A, Held A (2010) Certification report, the certification of the mass fraction of the total content and the aqua regia extractable content of As, Cd, Co, Cr, Cu, Mn, Ni, Pb and Zn in Loam soil certified reference material ERM ® -CC141
Scharf H, Lück D, Bremser W (2006) Bericht zur Zertifizierung der gesamtgehalte und der mit königswasser extrahierbaren gehalte der elemente As, Cd, Co, Cr, Cu, Hg, Mn, Ni, Pb und Zn in einer bodenprobe Zertifiziertes Referenzmaterial BAM-U110
Griepink B, Muntau H, Vercoutere K (1994) Final report, certification of the total contents (mass fractions) of Cd, Co, Cu, Pb, Mn, Hg and Ni and the aqua regia soluble contents (mass fractions) of Cd, Pb, Ni and Zn in a light sandy soil CRM 142R
Semenkov IN, Koroleva TV (2019) International environmental legislation on the content of chemical elements in soils: guidelines and schemes. Eurasian Soil Sci 52(10):1289–1297
Article CAS Google Scholar
Carlon C (Ed.) (2007) Derivation methods of soil screening values in Europe. A review and evaluation of national procedures towards harmonization. European Commission Joint Research Centre, Ispra
Karaca A, Türkmen C, Arcak S, Haktanır K, Topçuoğlu B, Yıldız H (2009) The determination of the effect of Cayirhan coal-fired power plant emission on heavy metals and sulphur contents of regional soils. Ankara Üniversitesi Çevrebilimleri Dergisi 1(1):25–41
Article Google Scholar
Lamberty A, Schimmel H, Pauwels J (1998) The study of the stability of reference materials by isochronous measurements. Fres J Anal Chem 360:359–361
ISO Guide 35 (2017) Reference materials — guidance for characterization and assessment of homogeneity and stability
Linsinger TPJ, Pauwels J, Van der Veen AMH, Schimmel H, Lamberty A (2001) Homogeneity and stability of reference materials. Accred Qual Assur 6:20–25
Certificate of the Reference Material UME EnvCRM 03-Soil. https://rm.ume.tubitak.gov.tr/sertifika/ume_crm_envcrm03_certificate.pdf
International vocabulary of metrology - basic and general concepts and associated terms, 3rd ed (VIM 3). Available from https://www.bipm.org or as ISO/IEC guide 99-12:2007
ISO/TC 334 Position Paper (2023) The need for assessment of commutability of reference materials. https://committee.iso.org/files/live/sites/tc334/files/ISO-TC334_Commutability_document_2023-03.pdf
Download references
Acknowledgements
The work of this study is part of the project 14RPT03-EnvCRM, which was funded within the framework of the EMPIR. The EMPIR initiative is co-funded by the European Union’s Horizon 2020 research and innovation programme and the EMPIR Participating States. Authors thank to TUBITAK UME intern trainees Esma Eroğlu, Büşra Bıyıklı, Onur Uygun, H. Merve Kırbaş for their dedicated work during the processing of the soil material and Doğan Meriç for the logistics and supply of the soil material. We dedicate this article to the memory of Prof. Osman Yavuz Ataman, a doyen of analytical chemistry, who encouraged and directed us in producing reference materials.
European Metrology Programme for Innovation and Research, 14RPT03-EnvCRM.
Author information
Authors and affiliations.
TÜBİTAK UME-Ulusal Metroloji Enstitüsü, Kocaeli, Türkiye
Alper Isleyen, Suleyman Z. Can, Oktay Cankur, Murat Tunc, Zehra Cakılbahçe, Gokhan Aktas, Hatice Altuntas, Elif Basaran, Barıs Kısacık & Zeynep Gumus
BAM-Bundesanstalt für Materialforschung und –prüfung, Berlin, Germany
Jochen Vogl & Maren Koenig
IJS-Institute Jozef Stefan, Ljubljana, Slovenia
Milena Horvat, Radojko Jacimovic, Tea Zuliani & Vesna Fajon
IMBIH- Institute of Metrology, Sarajevo, Bosnia and Herzegovina
Aida Jotanovic
Directorate of Measures and Precious Metals, MoE-DMDM- Ministry of Economy, Beograde, Serbia
Luka Gaževic & Milena Milosevic
NTUA-National Technical University of Athens, Athens, Greece
Maria Ochsenkuehn–Petropoulou, Fotis Tsopelas, Theopisti Lymberopoulou, Lamprini-Areti Tsakanika, Olga Serifi & Klaus M. Ochsenkuehn
UWAR-University of Warsaw, Warsaw, Poland
Ewa Bulska, Anna Tomiak & Eliza Kurek
You can also search for this author in PubMed Google Scholar
Contributions
A.I. wrote the main manuscript text. AI., Z.C., G.A., H.A., E.B., B.K., Z.G. contributed to the material processing of the soil CRM. S.Z.C., O.C., M.T., J.V., M.K., M.H., R.J., T.Z., V.F., A.J., L.G., M.M., M.O-P., F.T., T.L., L-A.T., O.S.,K.M.O., E.B., A.T., E.K. contributed to the analysis and data evaluation. All authors reviewed the manuscript.
Corresponding author
Correspondence to Alper Isleyen .
Ethics declarations
Conflict of interest.
The authors declare no competing interests.
Additional information
Publisher's note.
Springer Nature remains neutral with regard to jurisdictional claims in published maps and institutional affiliations.
Supplementary Information
Below is the link to the electronic supplementary material.
Supplementary file1 (DOCX 2407 KB)
Rights and permissions.
Reprints and permissions
About this article
Isleyen, A., Can, S.Z., Cankur, O. et al. Certification of the total element mass fractions in UME EnvCRM 03 soil sample via a joint research project. Accred Qual Assur (2024). https://doi.org/10.1007/s00769-024-01597-8
Download citation
Received : 26 December 2023
Accepted : 02 April 2024
Published : 23 April 2024
DOI : https://doi.org/10.1007/s00769-024-01597-8
Share this article
Anyone you share the following link with will be able to read this content:
Sorry, a shareable link is not currently available for this article.
Provided by the Springer Nature SharedIt content-sharing initiative
- Total element content
- Certification
- Environmental pollution monitoring
- Find a journal
- Publish with us
- Track your research
- Open access
- Published: 26 April 2024
Associations of the circulating levels of cytokines with the risk of myeloproliferative neoplasms: a bidirectional mendelian-randomization study
- Hao Xiong 1 , 2 ,
- Huitao Zhang 2 ,
- Jun Bai 1 ,
- Yanhong Li 1 ,
- Lijuan Li 1 &
- Liansheng Zhang 1
BMC Cancer volume 24 , Article number: 531 ( 2024 ) Cite this article
47 Accesses
Metrics details
In the pathogenesis of myeloproliferative neoplasms (MPN), inflammation plays an important role. However, it is unclear whether there is a causal link between inflammation and MPNs. We used a bidirectional, two-sample Mendelian randomization (MR) approach to investigate the causal relationship between systemic inflammatory cytokines and myeloproliferative neoplasms.
A genome-wide association study (GWAS) of 8293 European participants identified genetic instrumental variables for circulating cytokines and growth factors. Summary statistics of MPN were obtained from a GWAS including 1086 cases and 407,155 controls of European ancestry. The inverse-variance-weighted method was mainly used to compute odds ratios (OR) and 95% confidence intervals (Cl).
Our results showed that higher Interleukin-2 receptor, alpha subunit ( IL-2rα ) levels, and higher Interferon gamma-induced protein 10 ( IP-10 ) levels were associated with an increased risk of MPN (OR = 1.36,95%CI = 1.03–1.81, P = 0.032; OR = 1.55,95%CI = 1.09–2.22, P = 0.015; respectively).In addition, Genetically predicted MPN promotes expression of the inflammatory cytokines interleukin-10 ( IL-10 ) (BETA = 0.033, 95% CI = 0.003 ~ 0.064, P = 0.032) and monokine induced by interferon-gamma ( MIG ) (BETA = 0.052, 95% CI = 0.002–0.102, P = 0.043) and, on activation, normal T cells express and secrete RANTES (BETA = 0.055, 95% CI = 0.0090.1, P = 0.018).
Our findings suggest that cytokines are essential to the pathophysiology of MPN. More research is required if these biomarkers can be used to prevent and treat MPN.
Peer Review reports
Introduction
The chronic hematological malignancies known as myeloproliferative neoplasms (MPN), which include polycythemia vera (PV), essential thrombocythemia (ET), and myelofibrosis (MF), advance at varying rates [ 1 ]. The incidence rates of PV, ET, and PMF are estimated to be 0.5 to 4.0, 1.1 to 2.0, and 0.3 to 2.0 per 100,000 people, respectively. It is reported that nearly 10–15% of patients with MPN progress to AML [ 2 ], more than 20% will develop thrombosis during the disease, and approximately 6.2% of newly diagnosed patients will suffer hemorrhage [ 3 , 4 ]. The presence of these symptoms mentioned above raises the rate of disability and mortality in MPN patients [ 5 ] and imposes a huge economic burden on the family and society. The most common feature of MPN is hyperactivation of Janus kinase 2 ( JAK2 ) signaling, which is caused by acquired mutations in JAK2 , MPL , and CALR [ 6 ]. However, clinically used JAK2 inhibitors such as Ruxolitinib and Fedratinib have limited efficacy, high toxicity, and are prone to drug resistance [ 6 , 7 , 8 ]. Therefore, increased awareness of the pathogenic components may offer clues for halting the disease's course and creating novel treatments.
The chronic inflammatory environment is one of the typical features of myeloproliferative neoplasms, where inflammation is tightly intertwined with tumor clones, providing a permissive micro-environment for disease progression [ 9 , 10 , 11 ]. Inflammatory cytokines are essential immune mediators in the physiology and disease process of MPN and not only play a significant role in inflammatory pathology but are also inextricably linked to the development of the disease [ 9 , 12 ]. GM-CSF , IL-1 , IL-4 , IL-5 , IL-6 , IL-10 , IFN-2 , MIP-1 , IL-12 , and TNF-α were shown to have higher cytokine levels in treatment-naive patients in all three MPN groups when compared to age-matched control participants, according to an observational study [ 9 ]. In addition, serum IL-2 and soluble IL-2 receptor alpha ( sIL-2rα ) increased as patients with MPNs progressed to advanced clinical stages [ 13 ], and serum IL-2 , sIL-2rα , and IL-6 levels were positively correlated with bone marrow neovascularization, indicating that increased inflammatory responses may be connected to the course of MPN disease [ 14 ], suggesting that MPN patients may benefit from using cytokines as a tool for illness monitoring [ 15 ]. However, little is known about the mechanisms and duration of inflammation in MPNs [ 16 ]. The origins of the increased cytokine production in MPNs (alterations, others?) and whether inflammation may occur before the development of JAK2/CALR/MPL gene mutations are still up for dispute. Observational studies are prone to common biases such as reverse causality and residual confounding [ 17 ] and have limitations such as small sample sizes and short follow-up periods. These studies, however, only addressed a small subset of inflammatory cytokines and did not take into account how other physical factors can affect changes in inflammatory cytokine levels. Determining whether variations in inflammatory cytokines cause the development of MPN or whether MPN development influences the microenvironment and causes variations in inflammatory cytokines is crucial. Investigating the precise nature of the connection between inflammatory cytokines and MPN is crucial from a therapeutic standpoint given the lack of knowledge regarding the etiology of MPN.
To establish a link between inflammatory cytokines and MPN, we applied Mendelian randomization (MR).MR has the advantage of reducing confounding variables and measurement error, as well as addressing the limitations of traditional observational studies mentioned above. This approach can effectively avoid bias caused by reverse causality [ 18 ]. The greatest level of evidence hierarchy outside of randomized controlled trials is provided by MR, which uses genetic variation as an instrumental variable (IV), which has been a dependable tool for getting reliable estimates of the causal influence of numerous risk variables on health [ 19 ]. In the current investigation, we used a two-sample MR design to methodically evaluate the potential causal link between inflammatory cytokines and MPN risk. Additionally, reverse MR analysis was done to determine how MPN affected cytokines.
Study design
The Mendelian randomization design method uses publicly available datasets from extensive genome-wide association studies (GWAS) for risk factors and disease to examine whether exposure has a causal effect on disease emergence. As a genetic instrumental variable analysis, MR Uses single nucleotide polymorphisms (SNPs) as instrumental variables for the risk factor of interest. SNP S are randomly assigned at meiosis and are not subject to reverse causality bias, so the Mendelian randomization approach can overcome unmeasured confounders and lead to more reliable causal inferences [ 20 ].
To determine the relationship between inflammatory cytokine levels and the risk of MPN, we conducted a bidirectional Mendelian randomized trial. As the summary statistics from published research are made available to the public, the institutional review board did not need to approve our study's ethics further. In Supplementary Table 1 , the features of the data used in this investigation are displayed.
Data sources and instruments
The data on inflammatory cytokines was obtained from a meta-analysis published in 2017 that summarized data from genome-wide association studies (GWAS) carried out with three Finnish cohorts (YFS and FINRISK, 1997 and 2002), totaling 8,293 Finns. The original publication has information about the cytokine assays, inclusion standards, etc. [ 21 ]. In MR analysis, P -values are used to measure whether there is an association between genetic variants and exposure factors. We set the P value to find the genetic variant loci associated with the trait. The independence of the selected instrumental variables was further ensured by removing all SNPs with linkage disequilibrium (LD) to avoid biased results (parameters kb = 250, r 2 = 0.001). Calculate the F-statistic [using the formula: F = (N-2)*R2/(1-R2), N is the sample size]to assess the extent of weak instrument bias, F > 10 suggests that full instrumental SNPs are sufficiently strong to lessen any potential bias, while an F-statistic ≤ 10 implies weak instruments [ 22 ].Initially, we used P < 5 × 10 –8 as a criterion to look for instrumental factors, and we discovered that the majority of cytokines had either no SNPs or only a few SNPs [ 3 ]. To get as many cytokines as possible into the study. Furthermore, we chose IVs using a permissive significance criterion ( P < 5 × 10 –6 ). Further, we use the parameter kb = 250, r 2 = 0.001 to eliminate the linkage disequilibrium among variables. Supplementary Tables 2 and 3 provide extensive details on the features of the IVs.
- Myeloproliferative neoplasms
The MPN Patients' data were obtained from publicly available GWAS data, which we downloaded from open GWAS ( https://gwas.mrcieu.ac.uk/ ). The data come from UK Biobank, which is a cohort study conducted between 2006 and 2010. The study collected in-depth genetic and phenotypic data on approximately 500,000 people across the United Kingdom. The data we downloaded for analysis included 1086 MPN patients and 407,155 controls [ 23 ]. The original article describes the criteria and procedures for the data's quality control [ 23 ]. Erythrocytosis, primary thrombocythemia, myelofibrosis, chronic myeloid leukemia, and chronic myeloproliferative illness are all included in the UKBB's description of the MPN phenotype. Those who had polycythemia vera, essential thrombocythemia, myelofibrosis, chronic myeloid leukemia, or malignant mastocytosis were additionally labeled as cases if they had self-reported cancer, self-reported sickness code, or histology of cancer tumor code. In the MPN dataset, we screened SNPs as instrumental variables according to the following criteria: ( P < 5 × 10 –8 , r 2 = 0.001, kb = 250 kb). Five SNPs were kept as separate MPN IVs. Inverse MR analyses were performed using these SNPs to examine the genetic influence of MPN on the amount of cytokines.
Bioinformatics analysis
We use the data set from the GEO database for gene expression spectrum analysis ( https://www.ncbi.nlm.nih.gov/geo/query/acc.cgi ). The GSE103237 dataset is based on the GPL13667 platform and consists of 26 polycythemia vera, 24 essential thrombocythemia, and 15 normal bone marrow samples. The GSE136335 dataset is based on the GPL17586 platform consisting of 8 myelofibrosis patients and 6 normal bone marrow samples. We used the "Ggpubr" package to create violin plots to visualize the expression of IP-10 , and IL-2ra in the three subtypes of MPN. The diagnostic value of IP-10 and IL-2ra was analyzed by the receiver operating characteristic (ROC) curve. The expression of genes was analyzed using the "pROC" package (v1.18.2), and genes with the area under the curve (AUC) > 0.7 were considered potential diagnostic markers.
Statistical analysis
We harmonized the effect of SNPs on inflammatory cytokines and MPNs before the Mendelian randomization analysis. Furthermore, we used the two-sample MR software package for MR analysis. The inverse variance weighted(IVW) methods were used to assess potential causality, while weighted median and MR-Egger regression methods were used as complementary methods for causality between inflammatory cytokines and MPN [ 24 ]. The MR-Egger method was used for multiple validity testing. We used Cochran's Q test to assess heterogeneity in IVW [ 25 ]. MR-PRESSO was used to detect the presence of outliers [ 26 ], and leave-one-out analyses were used to verify whether the causal effect depended on a single variant. We also performed instrumental strength tests using the F statistic, with F > 10 indicating sufficient strength [ 27 ]. Eventually, if the IVW method result is significant ( P < 0.05), even if the findings of other techniques are not significant and there is no pleiotropy or heterogeneity discovered, it may be regarded as a good result, given that the beta values of the other methods are in the same direction [ 28 ].
Based on the number of cytokines, we used the Bonferroni approach to compensate for multiple comparisons and set statistical significance at a P -value < 1.22 × 10 −3 (0.05/41) level. If a P -value was between 1.22 × 10 −3 and 0.05, we considered suggestive evidence for a potential causal association. The majority of the work mentioned above was completed using the R analysis program (version 4.3.0), which was applied to the relevant R package, including Two-sample MR, data array, etc.
Instrumental variables
Figure 1 offers a flowchart of the full-text logic. In the study of the effect of inflammatory factors on MPN, we screened instrumental variables according to the criteria of P < 5 × 10 –6 and r 2 < 0.001. A total of 354 SNPs linked to 41 cytokines were used for MR analysis after being harmonized with the outcome variable MPN (Supplementary Table 1 ).To analyze the effect of MPN on inflammatory factors, we used MPN as an exposure factor, By using such criteria ( P < 5 × 10 –8 , r 2 < 0.001), a total of five SNPs were obtained for subsequent analyses, and specific SNP information can be found in Supplementary Table 2 . These SNPs' F statistics, which ranged from 20.77 to 781.85(Table 1 ), showed that the instrument was sufficiently reliable to rule out the possibility of a null relationship brought on by instrument bias.
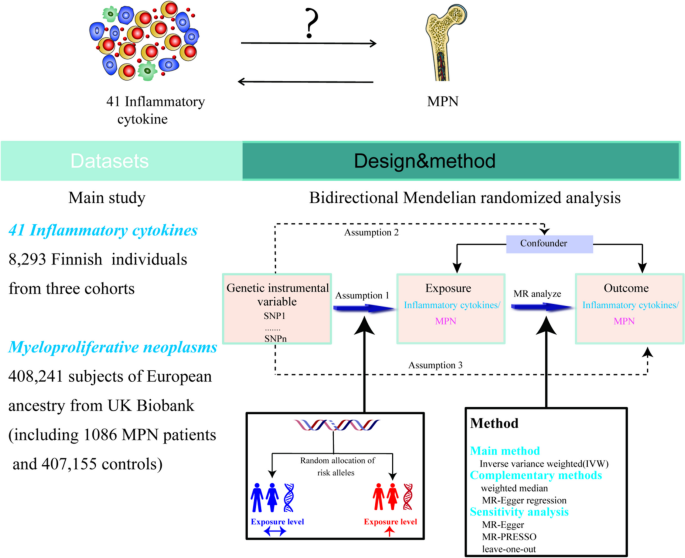
Study design overview and assumptions for MR Design. Assumption 1: IVs were not related to Confounders; Assumntion2: a strong correlation between iVs and exposure; Assumption 3: IVs can affect outcomes only through exposure and not through other pathways
Effect of inflammatory cytokines on MPN
We explored the effects of 41 cytokines on MPN sequentially using a two-sample Mendelian randomization analysis (Supplementary Table 3 ). Only two cytokines (Interleukin-2 receptor, alpha subunit ( IL − 2rα ), and interferon gamma-induced protein 10 ( IP-10 ) revealed suggestive associations with MPN risk after the Bonferroni correction. Genetically determined higher levels of circulating IL-2rα are suggestively positively associated with MPN risk [odds ratio (OR): 1.365,95% confidence interval (CI): 1.029–1.814, P = 0.032]. The other two complementary analytical methods Weighted median and MR Egger also obtained similar but not statistically significant results (Fig. 2 A). Furthermore, Cochran's Q test did not reveal any heterogeneity ( P = 0.268). A directional pleiotropy was also not discovered (MR egger-intercept = 0.021, P for MR egger-intercept = 0.710; P for MR PRESSO global test = 0.528) (Supplementary Table 5 ). Removing one SNP did not significantly change the results in the leave-one-out sensitivity analysis (Fig. 3 A). Sensitivity analyses were conducted using the leave-one-out analyses to assess the reliability and stability of the results. The overall effect of the remaining instrumental variables was calculated by removing each SNP stepwise and observing whether the results changed after removing a single SNP. The results showed that removing individual SNPs did not significantly change the results of the exclusion sensitivity analyses (Fig. 3 A). We did not find secondary phenotypes associated with SNPs that were used as instrumental variables on Pheno-Scanner's website.
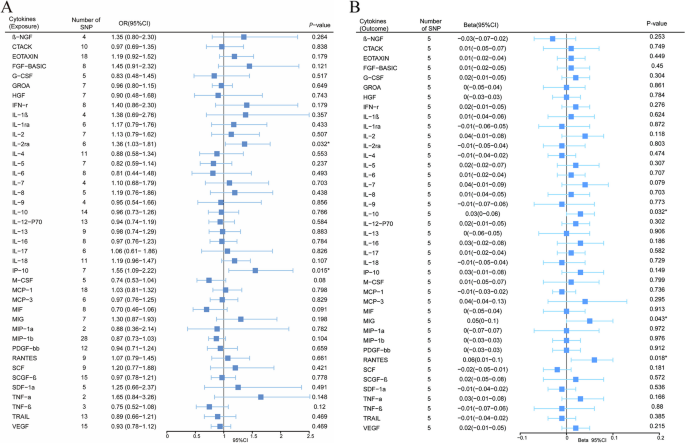
Forest plot of Mendelian randomization analysis of the correlation between inflammatory cytokines and risk of myeloproliferative neoplasms (MR analysis method using IVW). A : Effects of 41 inflammatory cytokines on myeloproliferative tumors. B :Effects of myeloproliferative neoplasms on 41 inflammatory cytokines(* P < 0.05)
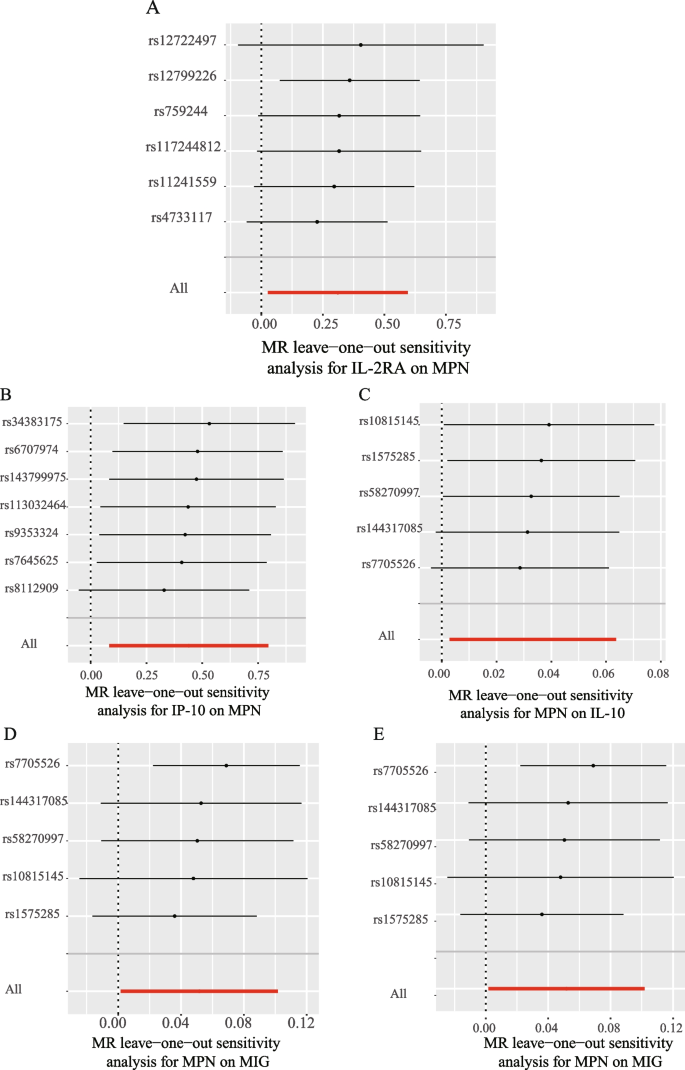
Leave-one-out analysis of bidirectional mendelian randomization in cytokines and MPN.The left side of the forest plot represents the SNPs for which the leave-one-out analysis was performed, and the short line parallel to the x-axis represents the 95% confidence interval for the OR/beta value of the MR analysis after excluding the corresponding SNPs. As shown in the figure, the overall error line does not change much after excluding each SNP, and all OR/beta values are on the 0 side, indicating that the results are reliable ( A ):Forest plots for the exposure of IL-2ra . B Forest plots for the exposure of IP-10 . C Forest plots for the outcome of IL-10 . D Forest plots for the outcome of MIG . E Forest plots for the outcome of RANTES
We also observed a suggestive association between genetically determined higher circulating IP-10 and a 55.3% increased risk of MPN (OR:1.553, 95% CI:1.088–2.216, P = 0.015), and it did not show heterogeneity (Cochrane Q test, P = 0.706)(Fig. 2 A); nor did it show directional pleiotropy (MR egger-intercept = 0.085, P for MR egger-intercept = 0.305, P for MR PRESSO global test = 0.668) (Supplementary Table 5 ). Sensitivity analysis was conducted using leave-one-out studies, and the results showed that no individual study had any impact (Fig. 3 B). We did not identify the SNP associated with other phenotypes on the Pheno-Scanner website, indicating that it does not increase the risk of MPN through the non-exposure pathway.
Effect of MPN on inflammatory cytokines
In analyzing the effect of MPN on inflammatory factors, We found a suggestive association between genetically predicted MPN and levels of the cytokines IL-10 , MIG , and RANTES . Genetically predicted MPN were suggestively associated with levels of interleukin-10 ( IL-10 ) (BETA = 0.033,95% CI = 0.003 ~ 0.064, P = 0.032) and Monokine induced by interferon-gamma ( MIG ) (BETA = 0.052,95% CI = 0.002–0.102, P = 0.043) and Regulated on activation, normal T Cell expressed and secreted ( RANTES ) (BETA = 0.055,95% CI = 0.009 − 0.1, P = 0.018) using IVW methods. It is worth paying attention to the fact that in the RANTES analysis, although the MR-egger results were not statistically significant, the direction of the MR-egger results was inconsistent with the IVW results, suggesting that the RANTES results may be unreliable (Fig. 2 B). In these findings, there was no indication of pleiotropy or heterogeneity. Supplementary Tables 4 and 6 provides a summary of the abovementioned findings. The leave-one-out analysis, meanwhile, revealed that all SNPs contributed to consistent causal estimates. (Fig. 3 C-E). The analysis mentioned above demonstrated the validity of the results.
The expression of IP-10 and IL-2ra in various subtypes of MPN and their diagnostic value
By analysing the effect of inflammatory cytokines on MPN, we found that higher levels of IP-10 , IL-2ra were associated with increased risk of MPN. However, due to data limitations, we did not have the opportunity to further analyse the effect of IP-10 , IL-2ra on the risk of developing each subtype of MPN using the MR approach. Therefore, in order to investigate the expression of IP-10 and IL-2ra in each subtype of MPN and their diagnostic value, we analyzed their expression in healthy individuals and the three subtypes of MPN and their diagnostic value based on data from the GEO database, as shown in Fig. 4 . Our results showed that IP-10 and IL-2ra were elevated in ET, PV, and MF compared with normal subjects. The highest diagnostic efficacy for MF was based on the expression of IP-10 and IL-2ra (IP10: AUC = 0.958, IL-2ra : AUC = 0.938); the areas under the ROC curves of IP-10 and IL-2ra in ET, PV, and MF were greater than 0.7, suggesting that IP-10 and IL-2ra may be used as potential diagnostic markers of MPN.
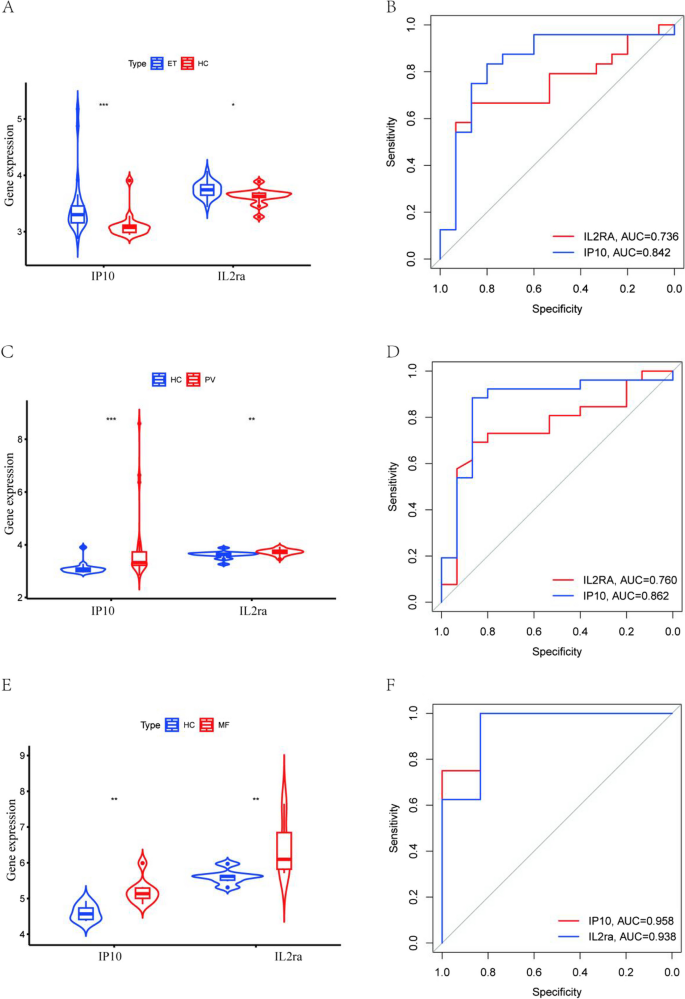
The GEO dataset analyses the expression of IP10 and IL2ra in each subtype of MPN and their diagnostic value.A: Expression of IP10 and IL2ra in ET and healthy donors; B: ROC curve of the prediction model based on IP10 and IL2ra to distinguish ET from healthy donors; C:IP10 and IL2ra expression in PV and healthy donors; D: ROC curve of the prediction model based on IP10 and IL2ra to distinguish PV from healthy donors; E:IP10 and IL2ra expression in MF and healthy donors; F: ROC curve of the prediction model based on IP10 and IL2ra to distinguish MF from healthy donors; *** P < 0.001, ** P < 0.01,* P < 0.05,HC: Healthy donors
Using publicly available pooled data from GWAS, we conducted a bidirectional two-sample MR analysis of the potential causative relationship between inflammatory cytokines and MPNs, and our study supported a causal association between inflammatory cytokines and MPNs. We found suggestive evidence that levels of the genetically predicted circulating cytokines IL-2rα , and IP-10 have a risk effect on MPNs. Reverse MR analysis found suggestive evidence of a positive causal effect of MPN on levels of the circulating cytokines IL-10 , MIG , and RANTES . These findings passed sensitivity analyses and were not affected by heterogeneity or horizontal pleiotropy. To our knowledge, this investigation is anticipated to be the broadest and most thorough MR evaluation of links between genetically inflammatory cytokines and MPN risk to date.
The CXC chemokine family member interferon gamma-induced protein 10 ( IP-10 ) is crucial for cell growth and proliferation [ 29 ]. IP-10 combines with the CXCR3 receptor, being a key driver in cancer and autoimmune regulation [ 30 ]. Several observational studies have demonstrated the presence of aberrant IP-10 expression in MPN patients, especially in PMF and PV, where IP-10 expression is significantly elevated [ 31 , 32 ]. Meanwhile, the serum level of IP-10 was also correlated with the disease progression of MPN [ 32 ]. Our MR analysis suggests that elevated IP-10 levels may contribute to MPN disease progression, which is consistent with results derived from observational studies. Previous basic research can explain our findings and the phenomena of observational studies in terms of pathogenesis. IP-10 expression is reported to be required for the activation of the JAK signaling pathway [ 33 ]and its level correlates with JAK2V617F status [ 9 , 34 ]. Therefore, JAK inhibition can reduce downstream chemokine IP-10 production by disrupting T cell-induced macrophage activation [ 35 ]. However, stromal cells in the microenvironment can protect MPN clonal cells from JAK2 inhibitors by secreting IP-10 , which can promote disease progression. These discoveries underscore the importance of researching IP-10 as a potential therapeutic target in the MPN tumor microenvironment and highlight the necessity of further studies on the exact mechanism of its role in MPN oncogenesis.
Our study also reveals a potential association between IL2rα and increased risk of MPN disease. IL2rα is an important component of IL-2R , a high-affinity receptor molecule highly expressed by activated T lymphocytes [ 36 ], and plays an important role in the regulation of T cell differentiation. Increasing IL-2rα expression on antigen-presenting cells (APCs) enhances the formation of memory T cells [ 37 ], and mutations in IL-2rα lead to decreased T cell function [ 38 ]. Accordingly, IL-2rα levels are associated with T-cell, B-cell, and immune system activation [ 36 ]. It has been demonstrated that conditions linked to cellular immune activation correlate with increased IL-2rα [ 39 , 40 ]. Additionally, a few observational studies have shown a connection between IL2r and MPN. Katerina et al. found that serum levels of IL-2rα were significantly elevated in patients with MPN compared to normal individuals [ 14 ], which was confirmed by further studies, where IL2rα levels were correlated with overall survival in patients with MF in MPN [ 41 ], and levels of IL-2ra in patients with MPN were positively correlated with disease progression and bone marrow angiogenesis [ 42 ]. According to the findings of our MR investigation, elevated levels of IL-2rα in the circulatory system may accelerate the development of MPN disease. This finding is not only consistent with the results of observational studies but also compensates for the shortcomings of small sample sizes and potential confounders in the observational studies mentioned above and provides more reliable evidence for the association between IL2rα and MPN at the level of genetics, emphasizing the importance and necessity of further investigating the role of IL2rα in the development of MPN disease.
Both our analyses and previous studies suggest that IP-10 and IL2rα may play an important role in MPN disease development. Considering the heterogeneity of the three subtypes of MPN, we sought to explore the effects of IP-10 and IL2rα on the disease risk of each subtype of MPN. Unfortunately, due to the limitation of the dataset, we had no way to further explore the relationship between IP-10 and IL2rα and different subtypes of MPN. Therefore, we initially analyzed the expression and diagnostic value of IP-10 and IL2rα in each subtype of MPN using the GEO database. We were surprised to find that IP-10 and IL2rα not only had elevated expression in the three subtypes of MPN compared to healthy individuals, but also had the potential to serve as independent biomarkers. This is consistent with our MR analysis that high expression of IP-10 , IL2rα increases the risk of MPN disease. This greatly encourages our confidence in further exploring the role of IL2rα , IP-10 in MPN at a later stage.
Positive MR analysis has revealed the role of inflammatory cytokines, particularly IP-10 and IL-2rα , in MPN disease progression. Indeed, MPN cells can also release large amounts of pro-inflammatory products, which in turn cause genomic instability and drive clonal myeloproliferation [ 43 , 44 ].To explore the effect of MPN disease on inflammatory cytokines, we performed a reverse MR analysis. The inverse MR analysis showed a potential positive correlation between genetically predicted MPN and the levels of cytokines IL-10 , MIG , and RANTES , and that MPN could promote slightly increased levels of the above cytokines. Inverse MR analysis revealed a potential positive correlation between genetically predicted MPN and levels of the cytokines IL-10 , MIG , and RANTES , with MPN promoting slightly elevated levels of the aforementioned cytokines, which is consistent with observational findings [ 9 , 45 , 46 , 47 ]. Our review of the literature revealed that aberrantly expressed IL-10 , MIG , and RANTES are all associated with premature atherosclerosis, a devastating consequence of chronic inflammation in the MPN [ 48 , 49 , 50 ].MIG binds to the receptor CXCR3 and not only participates in the recruitment of T cells to peripheral sites of inflammation [ 51 ]but also chemotactically recruits monocytes/macrophages to sites of inflammation. Activated inflammatory cells release pro-inflammatory factors to induce an inflammatory response [ 52 ], which promotes atherosclerosis. RANTES is one of the chemokines highly expressed upon platelet activation, and RANTES released by activated platelets facilitates the formation of atherosclerotic lesions by platelet-monocyte aggregation [ 53 , 54 ], and RANTES also regulates local inflammatory processes and atherosclerosis progression by mediating CD4 + T-cell homing [ 55 ]. It is interesting to note that IL-10 appears to be a protective factor against atherosclerosis, a common clinical symptom of MPN, and that, as an anti-inflammatory cytokine, IL-10 can attenuate atherosclerotic lesions by preventing dilation of inflamed areas, decreasing the size of plaques, and other mechanisms [ 56 ]. Specifically, IL-10 attenuates atherosclerotic lesions by inhibiting macrophage activation, as well as inhibiting the expression of matrix metalloproteinases, proinflammatory cytokines, and cyclooxygenase-2 in lipid-loaded and activated macrophage foam cells [ 57 , 58 ]. Therefore, it is necessary to investigate the correlation and mechanism between the elevated circulating levels of MPN-promoting inflammatory cytokines IL-10 , MIG , and RANTES and the common clinical complications of MPN, and to provide the possibility of targeting the above cytokines to alleviate the clinical complications.
Our study has several advantages. (1) The link between inflammatory cytokines and MPN risk is explained for the first time in magnetic resonance research. (2) Unlike observational studies, our study minimized confounders and reverse causality, providing a reliable causal relationship between MPN and inflammatory cytokines. (3) Our research data were sourced from the openly available GWAS database, which houses a significant volume of original research data, and thus gives this study a solid guarantee.
There are also some limitations to our study. In the first place, all participants in the dataset we used were of European ethnicity, which limits our ability to generalise our findings to other ethnicities. It is well known that Mendelian randomisation investigates the effect of genotypic variation (exposure) on phenotype (outcome) from a genetic perspective. Bias caused by confounding variables or reverse causality is avoided [ 18 ].In reality, however, it is well known that the level of gene expression determines the unique characteristics of a cell, that differences in disease prevalence between populations are associated with the frequency of alleles that regulate polymorphisms, and that differences in allele frequencies between racial groups have highly significant phenotypic consequences [ 59 ].Significant differences in gene expression phenotypes have been reported for at least 25 percent of genes between Europeans and Asians, and specific genetic variants (allele frequencies) between populations are the main cause of these differences [ 59 ].Therefore, in Mendelian randomisation analyses, genetic differences in quantitative phenotypes between different ethnic groups may be functionally equally important. Environmental, genetic, dietary, and lifestyle factors in different racial groups may influence phenotypic results [ 60 ], so we think it is unavoidable that the results of MR may differ between races due to residual confounding and selection bias [ 61 , 62 ].This is our limitation in this study. Therefore, whether elevated IP-10 and IL-2ra increase the risk of MPN prevalence in other populations requires specific analyses of gene expression variation for particular populations. Future studies will also need to enhance the analysis of gene expression variation between populations to improve understanding of the underlying genetics and population differences observed in complex genetic diseases. In the second, there are three subtypes of MPN, and due to the limitations of the GWAS dataset, we have not specifically stratified to explore the relationship between cytokines and the different subtypes of MPN. Finally, after Bonferroni correction, no cytokines showed statistically significant associations with MPN risk, and only two of them (IP-10, IL-2rα) showed suggestive associations.
In conclusion, our study suggests that elevated circulating levels of IP-10 and IL-2rα are associated with a high risk of MPN. Potential positive correlation between genetically predicted MPN and levels of the cytokines IL-10 , MIG , and RANTES . Our results show that cytokines play a significant role in the pathophysiology of MPN. Further research is required on the potential use of these biomarkers for the prevention and treatment of MPN.
Availability of data and materials
The datasets analyzed during the current study are available in the FinnGen repository, More details in FinnGen are described at https://r9.finngen.fi .
Grinfeld J, et al. Classification and Personalized Prognosis in Myeloproliferative Neoplasms. N Engl J Med. 2018;379(15):1416–30.
Article CAS PubMed PubMed Central Google Scholar
Tefferi A. Myeloproliferative neoplasms: A decade of discoveries and treatment advances. Am J Hematol. 2016;91(1):50–8.
Article CAS PubMed Google Scholar
Rungjirajittranon T, et al. A systematic review and meta-analysis of the prevalence of thrombosis and bleeding at diagnosis of Philadelphia-negative myeloproliferative neoplasms. BMC Cancer. 2019;19(1):184.
Article PubMed PubMed Central Google Scholar
Abdul-Rahim AH, et al. National institutes of health stroke scale item profiles as predictor of patient outcome: external validation on independent trial data. Stroke. 2015;46(2):395–400.
Article PubMed Google Scholar
Papageorgiou L, et al. Thrombotic and Hemorrhagic Issues Associated with Myeloproliferative Neoplasms. Clin Appl Thromb Hemost. 2022;28:10760296221097968.
Brkic S, Meyer SC. Challenges and Perspectives for Therapeutic Targeting of Myeloproliferative Neoplasms. Hemasphere. 2021;5(1):e516.
Vannucchi AM, et al. Ruxolitinib versus standard therapy for the treatment of polycythemia vera. N Engl J Med. 2015;372(5):426–35.
Verstovsek S, et al. Long-term treatment with ruxolitinib for patients with myelofibrosis: 5-year update from the randomized, double-blind, placebo-controlled, phase 3 COMFORT-I trial. J Hematol Oncol. 2017;10(1):55.
Cacemiro MDC, et al. Philadelphia-negative myeloproliferative neoplasms as disorders marked by cytokine modulation. Hematol Transfus Cell Ther. 2018;40(2):120–31.
Braun LM, Zeiser R. Immunotherapy in Myeloproliferative Diseases. Cells. 2020;9(6):1559.
Mantovani A, et al. Cancer-related inflammation. Nature. 2008;454(7203):436–44.
Mantovani A, et al. Interleukin-1 and Related Cytokines in the Regulation of Inflammation and Immunity. Immunity. 2019;50(4):778–95.
Nachbaur DM, et al. Serum levels of interleukin-6 in multiple myeloma and other hematological disorders: correlation with disease activity and other prognostic parameters. Ann Hematol. 1991;62(2–3):54–8.
Panteli KE, et al. Serum interleukin (IL)-1, IL-2, sIL-2Ra, IL-6 and thrombopoietin levels in patients with chronic myeloproliferative diseases. Br J Haematol. 2005;130(5):709–15.
Ramanathan G, Fleischman AG. The Microenvironment in Myeloproliferative Neoplasms. Hematol Oncol Clin North Am. 2021;35(2):205–16.
Hasselbalch HC. Chronic inflammation as a promotor of mutagenesis in essential thrombocythemia, polycythemia vera and myelofibrosis. A human inflammation model for cancer development? Leuk Res. 2013;37(2):214–20.
Smith GD, Ebrahim S. “Mendelian randomization”: can genetic epidemiology contribute to understanding environmental determinants of disease? Int J Epidemiol. 2003;32(1):1–22.
Davey Smith G, Hemani G. Mendelian randomization: genetic anchors for causal inference in epidemiological studies. Hum Mol Genet. 2014;23(R1):R89-98.
Davies N.M, Holmes M.V, Davey Smith G. Reading Mendelian randomisation studies: a guide, glossary, and checklist for clinicians. BMJ. 2018;362:k601.
Sun Y, et al. Assessing the causal role of hypertension on left atrial and left ventricular structure and function: A two-sample Mendelian randomization study. Front Cardiovasc Med. 2022;9:1006380.
Ahola-Olli A, et al. Genome-wide Association Study Identifies 27 Loci Influencing Concentrations of Circulating Cytokines and Growth Factors. Am J Hum Genet. 2017;100(1):40–50.
Zhang Y, et al. Causal Association Between Tea Consumption and Kidney Function: A Mendelian Randomization Study. Front Nutr. 2022;9:801591.
Bycroft C, et al. The UK Biobank resource with deep phenotyping and genomic data. Nature. 2018;562(7726):203–9.
Larsson SC, et al. Type 2 diabetes, glucose, insulin, BMI, and ischemic stroke subtypes: Mendelian randomization study. Neurology. 2017;89(5):454–60.
Bowden J, Hemani G, Davey Smith G. Invited Commentary: Detecting Individual and Global Horizontal Pleiotropy in Mendelian Randomization-A Job for the Humble Heterogeneity Statistic? Am J Epidemiol. 2018;187(12):2681–5.
PubMed PubMed Central Google Scholar
Verbanck M, et al. Detection of widespread horizontal pleiotropy in causal relationships inferred from Mendelian randomization between complex traits and diseases. Nat Genet. 2018;50(5):693–8.
Pierce BL, Ahsan H, Vanderweele TJ. Power and instrument strength requirements for Mendelian randomization studies using multiple genetic variants. Int J Epidemiol. 2011;40(3):740–52.
Chen X, et al. Depression and prostate cancer risk: A Mendelian randomization study. Cancer Med. 2020;9(23):9160–7.
Liu M, et al. CXCL10/IP-10 in infectious diseases pathogenesis and potential therapeutic implications. Cytokine Growth Factor Rev. 2011;22(3):121–30.
CAS PubMed PubMed Central Google Scholar
Karin N, Razon H. Chemokines beyond chemo-attraction: CXCL10 and its significant role in cancer and autoimmunity. Cytokine. 2018;109:24–8.
Obro NF, et al. Longitudinal Cytokine Profiling Identifies GRO-alpha and EGF as Potential Biomarkers of Disease Progression in Essential Thrombocythemia. Hemasphere. 2020;4(3):e371.
Tefferi A, et al. Circulating interleukin (IL)-8, IL-2R, IL-12, and IL-15 levels are independently prognostic in primary myelofibrosis: a comprehensive cytokine profiling study. J Clin Oncol. 2011;29(10):1356–63.
Schnoder TM, et al. Cell autonomous expression of CXCL-10 in JAK2V617F-mutated MPN. J Cancer Res Clin Oncol. 2017;143(5):807–20.
Allain-Maillet S, et al. Anti-Glucosylsphingosine Autoimmunity, JAK2V617F-Dependent Interleukin-1beta and JAK2V617F-Independent Cytokines in Myeloproliferative Neoplasms. Cancers (Basel). 2020;12(9):2446.
Nyirenda MH, et al. JAK inhibitors disrupt T cell-induced proinflammatory macrophage activation. RMD Open. 2023;9(1):e002671.
Waldmann TA. The structure, function, and expression of interleukin-2 receptors on normal and malignant lymphocytes. Science. 1986;232(4751):727–32.
Jones MC, et al. CD4 Effector TCR Avidity for Peptide on APC Determines the Level of Memory Generated. J Immunol. 2023;210(12):1950–61.
Picard C, Casanova JL. Inherited disorders of cytokines. Curr Opin Pediatr. 2004;16(6):648–58.
Semenzato G, et al. High serum levels of soluble interleukin 2 receptor in patients with B chronic lymphocytic leukemia. Blood. 1987;70(2):396–400.
Steis RG, et al. Serum soluble IL-2 receptor as a tumor marker in patients with hairy cell leukemia. Blood. 1988;71(5):1304–9.
Wang JC, Wang A. Plasma soluble interleukin-2 receptor in patients with primary myelofibrosis. Br J Haematol. 1994;86(2):380–2.
Bourantas KL, et al. Serum beta-2-microglobulin, TNF-alpha and interleukins in myeloproliferative disorders. Eur J Haematol. 1999;63(1):19–25.
Masselli E, et al. Protein kinase Cvarepsilon inhibition restores megakaryocytic differentiation of hematopoietic progenitors from primary myelofibrosis patients. Leukemia. 2015;29(11):2192–201.
Lussana F, Rambaldi A. Inflammation and myeloproliferative neoplasms. J Autoimmun. 2017;85:58–63.
Pourcelot E, et al. Cytokine profiles in polycythemia vera and essential thrombocythemia patients: clinical implications. Exp Hematol. 2014;42(5):360–8.
Gangemi S, et al. Evaluation of interleukin-23 plasma levels in patients with polycythemia vera and essential thrombocythemia. Cell Immunol. 2012;278(1–2):91–4.
Kleppe M, et al. JAK-STAT pathway activation in malignant and nonmalignant cells contributes to MPN pathogenesis and therapeutic response. Cancer Discov. 2015;5(3):316–31.
Li Q, et al. Activation of macrophage TBK1-HIF-1alpha-mediated IL-17/IL-10 signaling by hyperglycemia aggravates the complexity of coronary atherosclerosis: An in vivo and in vitro study. FASEB J. 2021;35(5):e21609.
Koenen RR, et al. Disrupting functional interactions between platelet chemokines inhibits atherosclerosis in hyperlipidemic mice. Nat Med. 2009;15(1):97–103.
Shi H, et al. CRISPR/Cas9 based blockade of IL-10 signaling impairs lipid and tissue homeostasis to accelerate atherosclerosis. Front Immunol. 2022;13:999470.
Park MK, et al. The CXC chemokine murine monokine induced by IFN-gamma (CXC chemokine ligand 9) is made by APCs, targets lymphocytes including activated B cells, and supports antibody responses to a bacterial pathogen in vivo. J Immunol. 2002;169(3):1433–43.
Koper OM, et al. CXCL9, CXCL10, CXCL11, and their receptor (CXCR3) in neuroinflammation and neurodegeneration. Adv Clin Exp Med. 2018;27(6):849–56.
von Hundelshausen P, et al. RANTES deposition by platelets triggers monocyte arrest on inflamed and atherosclerotic endothelium. Circulation. 2001;103(13):1772–7.
Article Google Scholar
Huo Y, et al. Circulating activated platelets exacerbate atherosclerosis in mice deficient in apolipoprotein E. Nat Med. 2003;9(1):61–7.
Ley K. Role of the adaptive immune system in atherosclerosis. Biochem Soc Trans. 2020;48(5):2273–81.
Xu S, et al. The role of interleukin-10 family members in cardiovascular diseases. Int Immunopharmacol. 2021;94:107475.
Hansson GK. Immune mechanisms in atherosclerosis. Arterioscler Thromb Vasc Biol. 2001;21(12):1876–90.
Han X, Boisvert WA. Interleukin-10 protects against atherosclerosis by modulating multiple atherogenic macrophage function. Thromb Haemost. 2015;113(3):505–12.
Spielman RS, et al. Common genetic variants account for differences in gene expression among ethnic groups. Nat Genet. 2007;39(2):226–31.
Zhao Y, et al. Associations between type 2 diabetes mellitus and chronic liver diseases: evidence from a Mendelian ranldomization study in Europeans and East Asians. Front Endocrinol (Lausanne). 2024;15:1338465.
Au Yeung SL, et al. Evaluating the role of non-alcoholic fatty liver disease in cardiovascular diseases and type 2 diabetes: a Mendelian randomization study in Europeans and East Asians. Int J Epidemiol. 2023;52(3):921–31.
Ford I, et al. The inverse relationship between alanine aminotransferase in the normal range and adverse cardiovascular and non-cardiovascular outcomes. Int J Epidemiol. 2011;40(6):1530–8.
Download references
Acknowledgements
We appreciate all investigators for sharing these data in our study.
This study was supported by Commissioned by the National Clinical Medical Research Center for Hematological Diseases (grant no. 2021WWA01) and the Construction of the clinical medical research center of the Gansu Science and Technology project (grant no. 21JR7RA435) and the Gansu Province Science and technology project natural science foundation (grant no. 21JR11RA104) and Lanzhou science and technology development plan project(grant no. 2020-ZD-99) and The Second Hospital of Lanzhou University "Cui-ying Science and Technology Innovation" Program (grant no. 2020QN-13).
Author information
Authors and affiliations.
Department of Hematology, The Second Hospital of Lanzhou University, Lanzhou, China
Hao Xiong, Jun Bai, Yanhong Li, Lijuan Li & Liansheng Zhang
Department of Hematology, The Affiliated Hospital of Southwest Medical University, Luzhou, China
Hao Xiong & Huitao Zhang
You can also search for this author in PubMed Google Scholar
Contributions
LSZ and LJL conceived, designed, and supervised the whole study. HX,HTZ performed the analyses. JB and YHL interpreted the results, and contributed in this work in study design, data interpretation, and manuscript writing.HX and HTZ wrote the manuscript. All authors provided critical comments and approved the final manuscript.
Corresponding authors
Correspondence to Lijuan Li or Liansheng Zhang .
Ethics declarations
Ethics approval and consent for participate.
Not applicable.
Consent for publication
The authors have given consent for publication.
Competing interests
The authors declare no competing interests.
Additional information
Publisher’s note.
Springer Nature remains neutral with regard to jurisdictional claims in published maps and institutional affiliations.
Supplementary Information
Supplementary material 1, supplementary material 2, supplementary material 3, supplementary material 4, supplementary material 5, supplementary material 6, rights and permissions.
Open Access This article is licensed under a Creative Commons Attribution 4.0 International License, which permits use, sharing, adaptation, distribution and reproduction in any medium or format, as long as you give appropriate credit to the original author(s) and the source, provide a link to the Creative Commons licence, and indicate if changes were made. The images or other third party material in this article are included in the article's Creative Commons licence, unless indicated otherwise in a credit line to the material. If material is not included in the article's Creative Commons licence and your intended use is not permitted by statutory regulation or exceeds the permitted use, you will need to obtain permission directly from the copyright holder. To view a copy of this licence, visit http://creativecommons.org/licenses/by/4.0/ . The Creative Commons Public Domain Dedication waiver ( http://creativecommons.org/publicdomain/zero/1.0/ ) applies to the data made available in this article, unless otherwise stated in a credit line to the data.
Reprints and permissions
About this article
Cite this article.
Xiong, H., Zhang, H., Bai, J. et al. Associations of the circulating levels of cytokines with the risk of myeloproliferative neoplasms: a bidirectional mendelian-randomization study. BMC Cancer 24 , 531 (2024). https://doi.org/10.1186/s12885-024-12301-x
Download citation
Received : 17 August 2023
Accepted : 22 April 2024
Published : 26 April 2024
DOI : https://doi.org/10.1186/s12885-024-12301-x
Share this article
Anyone you share the following link with will be able to read this content:
Sorry, a shareable link is not currently available for this article.
Provided by the Springer Nature SharedIt content-sharing initiative
- Mendelian randomization
- Inflammatory
ISSN: 1471-2407
- Submission enquiries: [email protected]
- General enquiries: [email protected]
ORIGINAL RESEARCH article
This article is part of the research topic.
Advances in Infrared Lasers and Their Applications
Fine Grained Analysis Method for Unmanned Aerial Vehicle Measurement Based on Laser-based Light Scattering Particle Sensing Provisionally Accepted
- 1 Qinhuangdao Tianda Environmental Research Protection Institute Co., LTD, China
The final, formatted version of the article will be published soon.
As an effective particle measurement method, laser-based particle sensors combined with unmanned aerial vehicles (UAVs) can be used for measuring air quality in near ground space. In order to assess the air quality between flight trajectories, a new fine-grained analysis method, Co-KNN-DNN is proposed to present the continuous distribution of air quality in more detail. First of all, the overall scheme was designed, M30T UAV was selected to carry the portable air quality monitoring equipment, with laser-based laser particulate matter sensor and Mini2, to collect AQI and related attributes of the near-ground layer in the selected research area, to do the necessary processing of the collected data, to build a data set suitable for model input, etc., to train and optimize the model, and to carry out practical application of the model. Based on the spatial dimension-based air quality finegrained analysis model Co-KNN-DNN, three experiments were conducted at different altitudes within the study area. 290 samples from each altitude data set were randomly selected to form the initial marker sample set, and 200 samples from each altitude were randomly selected as the test sample set. The remaining samples were unlabeled sample sets. The experimental results show that the average R-squared value can reach 0.99. The effectiveness and practicability of the Co-KNN-DNN model were verified by application research.
Keywords: air quality fine-grained, Sniffer4D Mini2, M30T UAV, Laser Particulate Matter Sensor, Co-KNN-DNN
Received: 06 Apr 2024; Accepted: 26 Apr 2024.
Copyright: © 2024 Jia, Song and Liu. This is an open-access article distributed under the terms of the Creative Commons Attribution License (CC BY) . The use, distribution or reproduction in other forums is permitted, provided the original author(s) or licensor are credited and that the original publication in this journal is cited, in accordance with accepted academic practice. No use, distribution or reproduction is permitted which does not comply with these terms.
* Correspondence: Mr. Xutao Jia, Qinhuangdao Tianda Environmental Research Protection Institute Co., LTD, Qinhuangdao, China
People also looked at
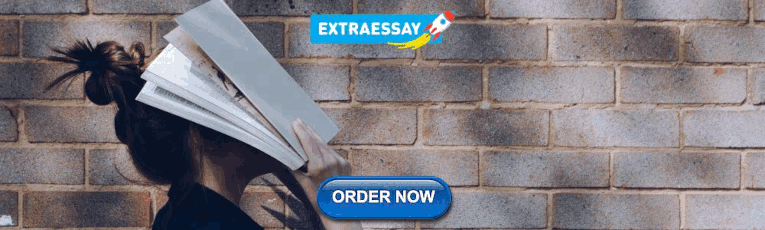
IMAGES
VIDEO
COMMENTS
The sample is the group of individuals who will actually participate in the research. To draw valid conclusions from your results, you have to carefully decide how you will select a sample that is representative of the group as a whole. This is called a sampling method. There are two primary types of sampling methods that you can use in your ...
The sample should be selected randomly, or if using a non-random method, every effort should be made to minimize bias and ensure that the sample is representative of the population. Collect data: Once the sample has been selected, collect data from each member of the sample using appropriate research methods (e.g., surveys, interviews ...
Sampling methods in psychology refer to strategies used to select a subset of individuals (a sample) from a larger population, to study and draw inferences about the entire population. Common methods include random sampling, stratified sampling, cluster sampling, and convenience sampling. Proper sampling ensures representative, generalizable, and valid research results.
Firstly, a refined research question and goal would help us define our population of interest. If our calculated sample size is small then it would be easier to get a random sample. If, however, the sample size is large, then we should check if our budget and resources can handle a random sampling method. Sampling frame availability
Simple random sampling. Simple random sampling involves selecting participants in a completely random fashion, where each participant has an equal chance of being selected.Basically, this sampling method is the equivalent of pulling names out of a hat, except that you can do it digitally.For example, if you had a list of 500 people, you could use a random number generator to draw a list of 50 ...
1. Simple random sampling. In a simple random sample, every member of the population has an equal chance of being selected. Your sampling frame should include the whole population. To conduct this type of sampling, you can use tools like random number generators or other techniques that are based entirely on chance.
Understand sampling methods in research, from simple random sampling to stratified, systematic, and cluster sampling. Learn how these sampling techniques boost data accuracy and representation, ensuring robust, reliable results. Check this article to learn about the different sampling method techniques, types and examples.
Research methods are specific procedures for collecting and analyzing data. Developing your research methods is an integral part of your research design. When planning your methods, there are two key decisions you will make. First, decide how you will collect data. Your methods depend on what type of data you need to answer your research question:
A sample is the subset of the population that you actually measure, test, or evaluate and base your results. Sampling methods are how you obtain your sample. Before beginning your study, carefully define the population because your results apply to the target population. You can define your population as narrowly as necessary to meet the needs ...
Revised on December 18, 2023. A simple random sample is a randomly selected subset of a population. In this sampling method, each member of the population has an exactly equal chance of being selected. This method is the most straightforward of all the probability sampling methods, since it only involves a single random selection and requires ...
Bad ways to sample. Convenience sample: The researcher chooses a sample that is readily available in some non-random way. Example—A researcher polls people as they walk by on the street. Why it's probably biased: The location and time of day and other factors may produce a biased sample of people. Voluntary response sample: The researcher ...
Evaluate your goals against time and budget. List the two or three most obvious sampling methods that will work for you. Confirm the availability of your resources (researchers, computer time, etc.) Compare each of the possible methods with your goals, accuracy, precision, resource, time, and cost constraints.
Research methodology formats can vary depending on the specific requirements of the research project, but the following is a basic example of a structure for a research methodology section: ... This is a research methodology that involves the collection of data from a sample of individuals using questionnaires or interviews. Survey research is ...
As we mentioned, research methodology refers to the collection of practical decisions regarding what data you'll collect, from who, how you'll collect it and how you'll analyse it. Research design, on the other hand, is more about the overall strategy you'll adopt in your study. For example, whether you'll use an experimental design ...
Sampling in qualitative research has different purposes and goals than sampling in quantitative research. Sampling in both allows you to say something of interest about a population without having to include the entire population in your sample. We begin this chapter with the case of a population of interest composed of actual people.
Quantitative research methods are used to collect and analyze numerical data. This type of research is useful when the objective is to test a hypothesis, determine cause-and-effect relationships, and measure the prevalence of certain phenomena. Quantitative research methods include surveys, experiments, and secondary data analysis.
In this video, we walk you through a research methodology from a dissertation that earned full distinction, step by step. We start off by discussing the core components of a research methodology by unpacking our free methodology chapter template. We then progress to the sample research methodology to show how these concepts are applied in an ...
What is a Sample? A sample is a smaller set of data that a researcher chooses or selects from a larger population using a pre-defined selection bias method. These elements are known as sample points, sampling units, or observations. Creating a sample is an efficient method of conducting research. Researching the whole population is often ...
The methodology section should clearly show why your methods suit your objectives and convince the reader that you chose the best possible approach to answering your problem statement and research questions. 2. Cite relevant sources. Your methodology can be strengthened by referencing existing research in your field. This can help you to:
The main purpose of sampling in research is to make the research process doable. The research sample helps to reduce bias, accurately present the population and is cost-effective.
The overall response rate for the Asian American sample in the Pew Research Center survey was 13.3% (17.0% x 77.9%). Analysis of Asians living in poverty Survey analysis of Asian adults living in poverty is based on 561 respondents of the 2022-23 survey of Asian Americans whose approximate family income falls at or below the 2022 federal ...
To build on this research, we test the impact of freedom of domestic movement and democracy on forest loss from 2001 to 2014 for a sample of 107 low- and middle-income nations. We find support for the idea that having more freedom of movement decreases forest loss in more democratic nations compared to less democratic nations.
The method retains feasibility by projecting the resulting points onto a feasible set. The a.s. convergence of AN-SPS method is proved without the assumption of a bounded feasible set or bounded iterates. Preliminary numerical results on Hinge loss problems reveal the advantages of the proposed adaptive scheme.
Step 1: Consider your aims and approach. Step 2: Choose a type of research design. Step 3: Identify your population and sampling method. Step 4: Choose your data collection methods. Step 5: Plan your data collection procedures. Step 6: Decide on your data analysis strategies. Other interesting articles.
The participating laboratories used validated measurement procedures (including potentially primary methods such as ID ICP-MS, ID-TIMS and k 0-INAA) and were allowed to apply the sample preparation method of their choice.Each laboratory received two bottles of samples which were selected such from the whole set of samples that they represent the whole produced batch.
Objective In the pathogenesis of myeloproliferative neoplasms (MPN), inflammation plays an important role. However, it is unclear whether there is a causal link between inflammation and MPNs. We used a bidirectional, two-sample Mendelian randomization (MR) approach to investigate the causal relationship between systemic inflammatory cytokines and myeloproliferative neoplasms. Methods A genome ...
Survey research means collecting information about a group of people by asking them questions and analyzing the results. To conduct an effective survey, follow these six steps: Determine who will participate in the survey. Decide the type of survey (mail, online, or in-person) Design the survey questions and layout.
As an effective particle measurement method, laser-based particle sensors combined with unmanned aerial vehicles (UAVs) can be used for measuring air quality in near ground space. In order to assess the air quality between flight trajectories, a new fine-grained analysis method, Co-KNN-DNN is proposed to present the continuous distribution of air quality in more detail. First of all, the ...
Qualitative research methods. Each of the research approaches involve using one or more data collection methods.These are some of the most common qualitative methods: Observations: recording what you have seen, heard, or encountered in detailed field notes. Interviews: personally asking people questions in one-on-one conversations. Focus groups: asking questions and generating discussion among ...