- Skip to content
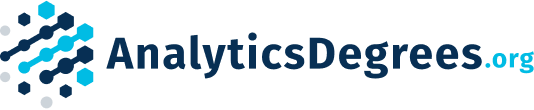
AnalyticsDegrees.org
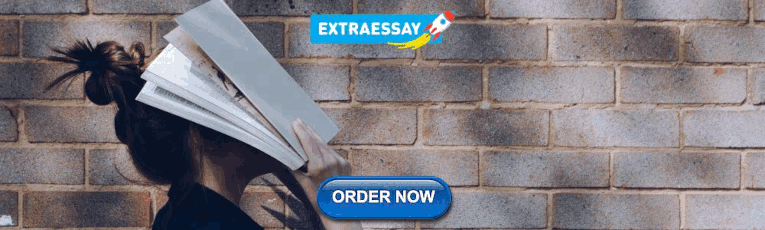
PhD in Data Analytics Programs
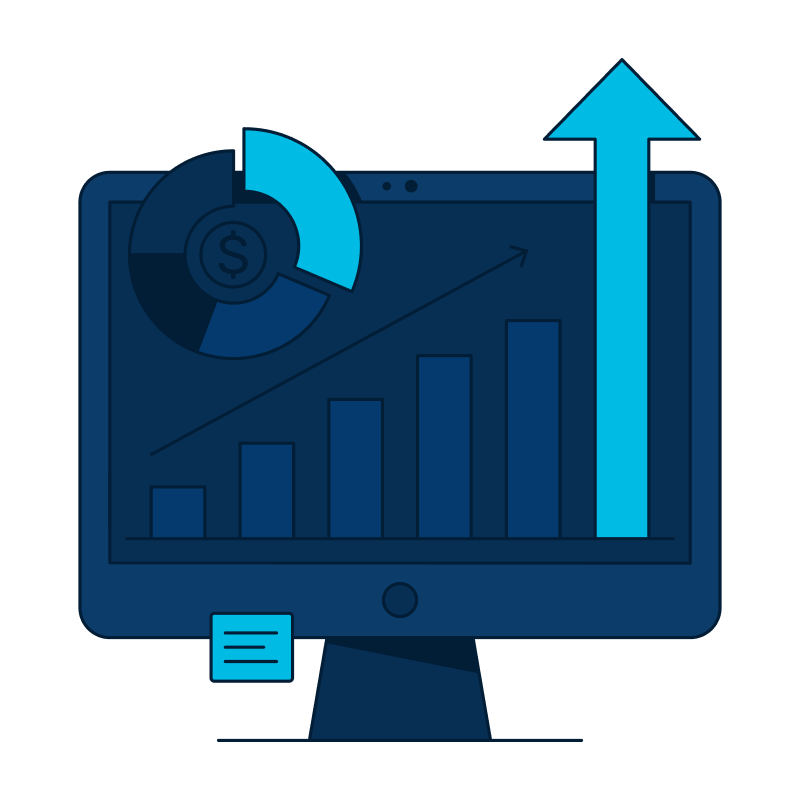
On This Page:
You’re an analytics professional with a talent for research. You’re considering a PhD in Data Analytics as the next logical step in your career, but you’d like to know more about the practicals. Explore different types of analytics doctorates . Dig into details on timelines , coursework , and the dissertation process . Learn about admissions requirements and funding options , including fully-funded doctorates. Find answers to questions about online degrees and employment avenues after graduation. Or skip ahead to our listings of all the PhD in Data Analytics programs in the country.
What Are PhD in Data Analytics Programs?
A PhD in Data Analytics or a closely related field is an interdisciplinary doctorate that focuses on cutting-edge research in the realms of advanced analytics, statistical computing, big data, and data science. Doctoral students in analytics:
- Push the boundaries of analytics in order to solve complex societal & organizational problems and transform decision-making
- Train to be expert practitioners in big data technologies, newly developed statistical methods, and “out of the box” analytical thinking
- Become analytics & data science professors at universities, senior analytics consultants in industry, and government advisors
Can You Earn a PhD in Data Analytics?
Yes. Doctoral programs in data analytics are available, but they are rare. The most popular title for a degree in the realm of data is the PhD in Data Science . Data science is a highly inventive field that builds on analytical foundations, so it makes sense to consider a doctoral program that focuses on innovation & self-guided discoveries.
When you do find a PhD with the word “analytics” in the title , you’re still going to be looking at a doctorate that intersects with the field of data science. Massive data sets, complicated analytics processes, sophisticated predictive models—doctoral students in analytics are schooled in all of these areas (and more).
Note: PhD programs are focused on original research and high-level thinking. If you want a workplace qualification, consider a Master’s in Data Analytics .
Types of Data Analytics Doctorate Programs
We’ve listed some common titles for doctorates in analytics, but we recommend you check the curriculum links in our listings and learn which department/s are offering the program. You should also look up the faculty’s research interests to see if they align with your own ideas for PhD projects. For example:
- If the degree is offered by the Department of Computer Science, a PhD in Data Analytics might be heavy on research into ethics, bias, AI, and building intelligent systems.
- If the degree is offered in partnership with the School of Business, a PhD in Data Analytics could be preoccupied with Machine Learning (ML), risk analysis, and econometrics.
The title of the PhD plays second fiddle to the department.
PhD in Analytics
A PhD in Analytics can often cut across multiple data-driven domains. Think of fields like Business Analytics, Data Science, Operations Research, and more. For instance, at the University of Notre Dame , doctoral students in analytics are able to access a large number of analytics research labs (e.g. gaming, human behavior, data & society, business, etc.) and collaborate with all kinds of partners.
PhD in Big Data Analytics
Doctorates in Big Data Analytics tend to focus on advanced systems & technologies that deal with processing big data (e.g. statistical computing, data mining, etc.), as well as their applications to real-world problems. Some universities, like the University of South Florida , are also interested in examining the human & social implications of analytics (e.g. ethical usage).
PhD in Analytics & Data Science
A PhD in Analytics and Data Science or a PhD in Data Science, Analytics & Engineering is a way for universities to combine data expertise from multiple departments. Yes, advanced analytics & big data processes will be addressed in the curriculum. But you’ll also find a strong emphasis on programming, algorithm creation, and systems development.
PhD in Data Science
Doctoral programs in data science may have more of a “design & develop” feel than analytics doctorates. In addition to exploring advanced analytics & big data applications, PhD in Data Science students are often interested in designing new information systems & tools (e.g. dashboards), creating their own algorithms & models, and exploring the boundaries of AI & Machine Learning (ML).
Note: Interested in industry & corporate analytics applications? Check out the guide to the PhD in Business Analytics .
How Doctorates in Data Analytics Work: Curriculum & Dissertation
Degree structure.
PhD programs in data analytics contain 6 key elements that take 4-5 years to complete on a full-time schedule. You will have to tackle each stage (e.g. core coursework) before you can proceed to the next one (e.g. qualifying exam).
Core Coursework
Qualifying/comprehensive exam, dissertation proposal, dissertation, dissertation defense.
- Year 1: Core coursework and first-year research papers. Assignment of a faculty mentor.
- Year 2: Core coursework, electives, second-year research papers, and the qualifying exam.
- Year 3: Any remaining coursework. Preparing research projects for publication. Dissertation proposal.
- Year 4: Dissertation work under the guidance of a dissertation advisor and advisory committee.
- Year 5: Dissertation work. Research papers & conference submissions. Dissertation defense.
Sample Curriculum
A PhD in Data Analytics or a closely related field will always contain a set of courses in advanced analytics & data science subjects. These courses can come from multiple departments (e.g. Computer Science, Mathematics & Statistics, Industrial Engineering, Psychology, etc.). Examples include:
- Big Data Analytics
- Data Mining
- Theoretical Statistics
- Statistical Computing
- Machine Learning
- Database Systems
- Information Assurance & Security
These are just a few sample course titles! Use the curriculum links in our listings to get a feel for each program’s unique flavor.
Once you’ve tackled the fundamentals of core coursework , you’ll usually be able to choose high-level electives in your particular research interests. For instance, the University of Central Florida offers electives in:
- Advanced computing (e.g. Parallel & Cloud Computation)
- Sophisticated analytics applications (e.g. Interactive Data Visualization)
- Industries (e.g. Industrial Engineering Analytics for Healthcare)
With some programs, you can customize your doctorate to a remarkable extent.
A qualifying exam is designed to test your knowledge of core coursework . It might take the form of a traditional exam, a paper and/or a project. For example, at the University of South Florida , PhD students are required to report on the results of a real-world, big data analytics project and include codes & systems that were developed in the process.
You’ll be required to develop an original idea for a research- or project-based dissertation and present your dissertation proposal to a dissertation advisory committee—experienced faculty members and (occasionally) outside experts who are interested in your area of work.
- A research-based dissertation will explore new realms of analytics research and potential applications.
- A project-based dissertation will involve work on a real-life project—this may be created at a research center or be suggested by an industry partner.
The dissertation proposal often takes the form of a written outline and an oral defense/presentation. If the committee accepts your proposal, you can get to work on your dissertation.
A PhD dissertation is a piece of original research that makes a significant contribution to the theory & practice of a field. In the world of data analytics & data science, dissertations can be research-based or project-based.
Dissertation Titles
Examples of real-life PhD in Data Analytics & Data Science dissertation titles include:
- A Credit Analysis of the Unbanked and Underbanked: An Argument for Alternative Data
- Novel Statistical and Machine Learning Methods for the Forecasting and Analysis of Major League Baseball Player Performance
- Optimal Analytical Methods for High Accuracy Cardiac Disease Classification and Treatment Based on ECG Data
- The Intelligent Management of Crowd-Powered Machine Learning
- Forecasting the Prices of Cryptocurrencies using a Novel Parameter Optimization of VARIMA Models
- Classification with Large Sparse Datasets: Convergence Analysis and Scalable Algorithms
While you are writing up your dissertation, many universities will also expect you to be submitting related research papers to peer-reviewed journals & industry conferences.
The final step in the PhD process is the dissertation defense. You’ll be required to present your dissertation findings to your dissertation advisory committee and defend your research ideas in an oral & visual presentation. This will be followed by questions and a discussion.
It’s not as intimidating as it sounds. By this stage in your education, you will know your research inside-out and will have brainstormed many of the potential questions with your dissertation advisor. You can prepare for a defense by observing other student defenses, practicing with mock presentations, and reading up on the work of committee members.
PhD in Data Analytics: Admissions
Doctorate in data analytics: what it takes to get in.
Every PhD program in data analytics is going to have a unique set of admissions requirements! When you’re putting together a shortlist of doctorates, use the admissions links in our listings to save yourself time & trouble. You can decide if the program suits your level of expertise and education.
Doctoral programs in tech-driven disciplines—especially ones that are fully funded —are extremely competitive. You can stand out from the crowd by:
- Examining your entire application to see if you can make up for weaknesses (e.g. lower grades) with strengths (e.g. real-world projects)
- Matching your research interests to the university, department & research labs offering the program
- Collaborating with experienced analytics practitioners to co-author papers & publications
- Attending industry events and making connections that will help in your research
- Earning professional certificates to fill in any skills gaps
Degree Requirements
Your degree should be in a discipline that’s relevant to your area of research interest in the PhD. For a data analytics doctorate, that might mean a degree in statistics, data analytics, computer science, economics, or similar. The standard GPA requirement is 3.0 GPA or higher.
- Bachelor’s Degree Entry: Some doctoral programs in data analytics & data science are willing to consider applicants with a bachelor’s degree.
- Master’s Degree Entry: Some doctoral programs are only looking for candidates with a master’s degree.
If you’re an undergraduate and you like the look of a PhD that only accepts master’s candidates, ask the program coordinator if you can earn an MS through the same university. Most doctoral programs have a “Master’s Along the Way” option.
Skills & Proficiencies
PhD candidates in analytics must be ready to tackle advanced coursework and high-level research. So universities will usually want to see evidence of proficiency/course credits in:
- Statistics, calculus & linear algebra
- Common analytical programming languages (e.g. R, Python, SAS, etc.)
- Analytics fundamentals (e.g. database management systems)
If you don’t have an undergraduate or master’s degree in analytics or a closely related field, universities will be poring over your transcripts & résumé to make sure you can handle any technical coursework.
General Requirements
In addition to your degree transcripts, almost all PhD programs in data analytics & data science fields will want to see:
- GRE or GMAT scores
- Letters of recommendation
- Statement of purpose
- TOEFL scores for non-English speaking international applicants
PhD in Data Analytics: Tuition & Funding
How to fund the phd.
Doctoral programs in data analytics & data science fall into 2 broad categories:
- Fully funded PhD programs
- Tuition-driven PhD programs
As you might expect, fully funded doctorate programs at strong universities are hard to get into!
Fully Funded PhD Programs
A number of STEM doctorates at research universities are fully funded. The university will waive all tuition costs and provide you with a living stipend as compensation for teaching & research activities. Many PhD students work as Teaching Assistants (TAs) and Research Assistant (RAs) during their doctoral studies.
Talk to the PhD program coordinator and check the fine print when you’re considering these programs.
- You may (or may not) qualify for on-campus housing and university health insurance.
- You may (or may not) qualify for conference stipends, overseas internships, and other perks.
- You may (or may not) be expected to pay for miscellaneous university fees.
- You may receive funding for Years 1-4 of your degree, but Year 5 support could be conditional on strong academic performance.
Tuition-Driven PhD Programs
You’ll also find doctoral programs in analytics & data science that do not offer any funding. They’ll expect you to pay for the degree out of your own pocket. At a private university, a PhD could cost upwards of $60,000-$80,000 in tuition alone.
So tread carefully! If you don’t qualify for fully funded PhD programs and you believe that a doctorate is essential for your career goals, consider applying to a PhD program at a public university in your state—UCF’s in-state tuition for a PhD in Big Data Analytics is very reasonable.
You will also need to look into postgraduate loans, private scholarships & fellowships, employer reimbursement, and teaching & research job opportunities to offset your costs.
Online PhD in Data Analytics Programs
Can you earn an online phd in data analytics.
Yes—but we would caution against them. There are a few universities that offer online doctorates in data analytics, but they tend to be for-profit (e.g. Colorado Tech) or focused on executive-level training instead of research (e.g. DBA in Data Analytics from the University of the Southwest).
You’ll have a little more luck in finding online doctorates in data science, but they still won’t be offered by top-tier universities.
Why Are Online PhD Programs in Analytics Hard to Find?
Prestigious research universities & high-ranking schools are very cautious about maintaining their reputation for quality. They want doctoral students in data analytics & data science to:
- Attend classes in advanced topics, ask questions, and follow-up with faculty
- Have unfettered access to the university’s research centers, labs, and technical facilities
- Be able to teach undergraduates and conduct research in-person
- Meet with their dissertation advisor on a regular basis
- Network with visiting experts and fellow students
We agree with them. At this level, we highly recommend you choose an on-campus doctoral degree.
Career Prospects for PhD in Data Analytics Graduates
A PhD in Data Analytics or a closely related field is a super-specialized degree. You don’t need a doctorate to pursue a career in analytics & data science. Many senior-level practitioners simply have a degree like a Master’s in Data Analytics (or a similar title) and a lot of on-the-job experience.
However, a doctorate in analytics is an excellent choice for aspiring:
- University Professors: If you wish to teach analytics & data science at a college or university, you will probably need a research-focused doctorate. At the University of Notre Dame, 80% of its PhD in Analytics graduates go into academia.
- High-Level Researchers: PhD graduates work in think tanks, industry research labs, and university research centers where exciting discoveries are taking place.
- Data Science & Analytics Consultants: You may wish to act in an advisory capacity for Wall Street, Silicon Valley, and other major centers of industry.
- Senior Research Positions: Some jobs in major tech companies, data-intensive businesses & financial companies (e.g. Senior Statistician) will require top-level research skills.
PhD Data Analytics FAQs
What should i look for in a data analytics doctoral program.
When you’re starting to put together a shortlist of doctoral programs, consider the following aspects:
- Funding Options: The best choice is going to be a fully funded PhD from a highly ranked & highly regarded university that includes teaching & research assistantships.
- Departmental Reputation: Which schools & departments are offering the degree? What kinds of unique benefits do they offer students? How much research funding do they receive?
- Faculty Expertise: Faculty profiles will be posted on the PhD program website. Read their bios, meet them for a virtual coffee, and learn more about their research & industry work. These people will become your advisors & mentors.
- Access to Resources: Will you have access to top-of-the-line analytics tools, commercial resources, and large-scale infrastructures? Can you work on projects within a major analytics research lab or center?
- Career Preparation: A strong PhD program will prepare you for the job market after graduation. Does the curriculum include opportunities for you to submit research papers to peer-reviewed journals? Does it offer stipends for conference travel? Does it bring in visiting experts for seminars?
What is a STEM Doctorate?
STEM stands for Science, Technology, Engineering & Mathematics. A STEM doctorate is any PhD—including the PhD in Data Analytics and the PhD in Data Science—that contains at least 50% of coursework in these fields.
- Are you an international student? Ask if the doctoral program has a “STEM designation” from the U.S. Department of Homeland Security (DHS). Students on an F-1 Visa can apply for Optional Practical Training (OPT) /temporary employment after graduation. Having a STEM-designated degree extends the OPT period from 12 months to 36 months.
- STEM programs often receive a fair amount of funding from the government and private industries. That means universities may be able to offer fully funded PhD programs to multiple students.
Is a PhD in Data Analytics Worth It?
Only if you have a specific career goal in mind. A PhD in Data Analytics or a closely related field is going to be time-consuming, challenging, and heavy on research. At least 4-5 years of your life will be devoted to earning it, so you and your family need to be prepared for the journey.
Unsure about your decision? Talk to analytics professionals who have already gone through the PhD gauntlet. You’ll find doctoral graduates on LinkedIn, at industry conferences , and within faculty directories on university websites. Be prepared to talk to them about your research interests and your goals.
All Phd in Data Analytics Programs
Arizona state university.
School of Computing and Augmented Intelligence
Tempe, Arizona
PhD in Data Science, Analytics, and Engineering
University of arizona.
Department of Biosystems Engineering
Tucson, Arizona
PhD in Biosystems Analytics & Technology
University of central florida.
College of Sciences
Orlando, Florida
University of South Florida-Main Campus
Muma College of Business
Tampa, Florida
Georgia State University
Robinson College of Business
Atlanta, Georgia
PhD in Business Administration & Digital Innovation - Data Science & Analytics
Kennesaw state university.
School of Data Science and Analytics
Kennesaw, Georgia
Doctor of Philosophy in Analytics and Data Science
University of notre dame.
Mendoza College of Business
Notre Dame, Indiana
University of Kansas
School of Business
Lawrence, Kansas
PhD in Analytics and Operations
Central michigan university.
College of Science and Engineering
Mount Pleasant, Michigan
PhD in Statistics and Analytics
North carolina, north carolina state university at raleigh.
Center for Geospatial Analytics
Raleigh, North Carolina
PhD in Geospatial Analytics
Pennsylvania, pennsylvania state university-main campus.
College of the Liberal Arts
University Park, Pennsylvania
PhD in Human Development and Family Studies and Social Data Analytics
Phd in informatics and social data analytics, phd in political science and social data analytics, phd in psychology and social data analytics, phd in social data analytics, phd in sociology and social data analytics, phd in statistics and social data analytics.
DiscoverDataScience.org
Guide to Applying for a Ph.D in Big Data
By Kat Campise, Data Scientist, Ph.D.
Ph.D. programs, in general, are a strenuous undertaking. You’ll spend between 4 to 7 years, on average, in deep and highly structured research on one topic with specific writing requirements. These won’t be blogs or superficial articles waxing poetic about the trials and tribulation of AI. You’ll be expected to publish and present your research to the highest levels of academia who will undoubtedly relish (at least some scholars will) in debating — if not outright challenging — every aspect of the research you conducted.
None of this is meant to scare you away from embarking on the Ph.D. journey. Rather, this is to prepare you for many years of sacrifice and, to be forthright, stress. Ph.D. completion rates hover around 50% . However, this statistic may be more promising depending on the graduate school you choose to attend and the program you intend to complete. For example, Duke University has Ph.D. completion rates as high as 95% .
By the conclusion of your Ph.D., however, you’ll be positioned as one of the leading experts in your chosen area of research. While this doesn’t make you omniscient or omnipotent (too many scholars conflate expertise with being downright arrogant), you will have more knowledge about a given subject than those at the bachelor’s or master’s degree levels. This knowledge is granular, meaning that through your applied research, you will have accrued greater understanding of the nuances involved in the problems you’ve studied at great length.
A Ph.D. is creational. The expectation is that you’ll create or discover something new in your research area. For example, if you’re in the midst of a Ph.D. in Data Science, deriving a brand new AI system, and then discussing how you arrived at this via your dissertation — which you will defend — is what a Ph.D. program will demand of you.
Should You Apply to a Ph.D. Program?
Most Ph.D. programs require full-time study. This will leave very little room for additional employment responsibilities, e.g., having a part-time job or attempting to work full-time. You won’t merely be reading others’ research and then repeating or summarizing it. You’ll critically analyze the strengths and weaknesses of their research, and then use it to inform your research design, development, and implementation. You’re building a brand new solution to a particular problem.
Many Ph.D. programs have a stipulation that you will be part of a teaching cadre, meaning you’ll be teaching either bachelor’s or master’s level students in your discipline. This is in addition to your research and writing. While these may be paid, the teaching assignments don’t tend to be as lucrative as jobs within private industry. For instance, the Bureau of Labor Statistics reports that the median salary for data scientists is $100,910 as of May 2021. It’s extremely unlikely that you’ll earn that type of salary within your Ph.D. in Data Science program.
The flip side of this is that you can reach a six-figure salary once you complete your Ph.D. if you’re willing to take on the opportunity cost during your Ph.D. program. In fact, BLS data says the highest-earning data scientists have salaries of $167,040 or more.
So, should you apply?
If you are certain of the program, which includes having an idea as to what you want to research, you enjoy focusing on a problem (almost endlessly) and creating new solutions, and you’re willing to spend around 6 years of your life constantly reading, analyzing, writing, publishing, and presenting, then start by reviewing the next steps of the application process.
Step 1: Finalize School and Program Choice
Although there are a growing number of online programs, Ph.D. programs are still primarily an onsite experience for the sciences, technology, engineering, and math (STEM) disciplines. So, most Ph.D. applicants will need to take the university location into consideration along with the availability of the specific Ph.D. program.
Regarding program choice, ideally, you should have either a bachelor’s or master’s degree in a related discipline. In many cases, one of the application requirements is for you to have completed specific courses (or a directly relevant degree). Using data science as an example, all Ph.D. programs in data science currently require the completion of Calculus (at the very least, Calculus I), Linear Algebra, and advanced statistics.
Some programs go further and have programming requirements (Python, Java, R, etc.) along with coursework in data structures and algorithms. It’s rare to jump from a B.A. in English to a Ph.D. in Computer Science (or Data Science); not because someone isn’t capable of doing so, but due to the major “catch up” required in terms of extensive practicum in the subject. A Ph.D. is already rigorous without you needing to take a series of prerequisite courses.
Review the current professors’ research interests and publications. One of them is likely to be your advisor and you’ll need to invite others to be a part of your dissertation committee (if the Ph.D. is structured in that way). This will also help you to generate research ideas of your own while also helping your application to “connect” with the department’s goals and objectives.
Additionally, peruse the required courses. If you can find the syllabi for those courses, read through them thoroughly. Note the journals and journal articles they reference. If you can find them (many are locked away in pay for view gateways such as JSTOR, but Google Scholar may have them available for free via PDF), then start reading! Doing so will clue you in on both the professor’s research area — especially if they are an author for one or more of the articles — and the focus of both the particular course and the Ph.D. program.
Remember, the department and its constituents want a high Ph.D. completion rate (which also holds true for master’s and bachelor’s degrees). The prestige factor attracts more students and more students translate into more funding. It’s not all about the money, of course. But, they do strongly prefer candidates who will successfully complete the program and earn their Ph.D.
While you should read the program requirements carefully. Don’t hesitate to gather questions that you can’t find answers to (specifically about the program itself rather than “how do I apply”) and send an email to the Department Chair. Keep in mind that if this is in the middle of a semester, it may take them time to respond to you as they also have teaching, research, and other bureaucratic duties.
Step 2: Review the Application Process
Depending on the department’s website layout, usually, it’s pretty easy to find their “How to Apply” section. Wherever that is located, make sure you find it and review the materials you’ll need to send along with your application. Thus far, just about every U.S. university has an option for applying online (we’ve yet to find one who doesn’t accept online applications). An overwhelming majority of Ph.D. programs require the documents discussed in the steps below. As such, you’ll need to set aside additional time, and money, so that you’ll have all of the requisite materials.
Step 3: Gather Your Transcripts
All U.S. universities are going to ask for official transcripts. During the online application process, you may be asked to upload unofficial transcripts for review by their admission committee. Subsequently, the Graduate Department will request your official transcripts upon admission acceptance. If you have any gaps in education or there was a semester or two where you weren’t performing very well academically, this can be briefly (and professionally) addressed in your Statement of Interest or Letter of Intent; more will be included on this topic below.
Step 4: Test Scores
Some Ph.D. programs are moving away from the GRE testing requirement. Others will accept GMAT test results in lieu of GRE scores. But, STEM programs aren’t likely to abandon the GRE as part of the application process. You’ll need to pay close attention to any cutoff scores listed by the department and whether you should take the General GRE or its Subject Tests .
Depending on where you are located in the world, GRE fees range from $205 to $230 . Subject Tests are $150 per subject. That aside, you’ll also need to spend time in test preparation mode which can be as little as 50 hours and as high as 120 hours. Your test preparation needs are unique and depend on many different factors. Most students perform better on one section over the other, e.g., if you have a Bachelor’s Degree in Math, the Quant section may be a breeze but your performance on the Verbal section may not be as stellar.
Also, keep the application due date in mind when scheduling your GRE test. Give yourself time to retake the test if need be while also ensuring that your test scores are received by the university before the application due date.
Step 5: Writing Samples, Resumes/CVs, and Letters of Intent
It cannot be overstated that scholarly work at the Ph.D. level requires a mind-numbing amount of writing (and research!). The department admission committee wants to determine if you can write at an academic level and if you have begun to form research interests. Essentially, they want to understand why you want to enter the Ph.D. program and how your studies will align with your career goals. All of this is part of determining not only your commitment but also your readiness.
Having industry experience is a bonus which is one of the reasons they ask for a resume or CV. As much as a Ph.D. seems to be “ivory tower” pontificating — admittedly, it can be — students who have some hands-on experience in the particular research area tend to have more successful outcomes — as do students who have a set of clear goals and objectives.
If you don’t have an academic writing sample, then this is the time to reach out to the Department Chair to determine what you should write about for application purposes. If you’ve completed a master’s degree, you should have your thesis to send. Some departments will explicitly state what the writing sample should contain. Summarily, if for some reason you don’t have a sample readily available, be prepared to create one.
What the department committee is likely not seeking is for you to have an already formed dissertation topic. If they’re seasoned academics, as they should be, they’re keenly aware that research interests evolve over time. But, as long as you have some direction, e.g., “I’m interested in researching how AI facial recognition can be accurately and equitably deployed in determining the likelihood of criminal activity”, then you’ll have a higher probability of making it to the acceptance pile.
Step 6: Letters of Recommendation
Sometimes referred to as “Letters of Reference” department requirements vary on the number and type of recommendation letters to include with your application. Usually, you’re required to send 3. Since you’re applying for admission into academia, recommendations from prior professors are the prevailing preference. However, an increasing number of universities also accept references from employers if they can include how your employment experience has prepared you for your intended academic studies.
The “how” of routing the reference or recommendation letters differs between universities. Some will still require that the letters are sent via postal mail directly to the department. But, there’s a shift towards simply uploading the letters as a PDF directly to your online graduate application.
Remember Self Care
Your application is viewed from a holistic perspective. Although GRE scores can be part of the admission consideration equation, most universities don’t view you as merely a test score number (which is one reason some are foregoing that requirement). As mentioned elsewhere, the department does want a high graduation rate along with generating scholars who are well-regarded in their expertise. The department admission committees are aware of the blood, sweat, and tears that committing to a Ph.D. program requires.
There is a high probability that you’ll experience disorienting moments including imposter syndrome. Life doesn’t always flow smoothly and definitely doesn’t stop just because you’re in the middle of your Ph.D. in Statistics (or whichever discipline you’ve chosen). It’s perfectly feasible to speak with your advisor about taking a short break from your studies so you can enact self-care. Only you can know and determine if that’s an action (or inaction) you need to take so you can return to your program revived and ready for the next set of challenges.
2021 US Bureau of Labor Statistics salary and employment figures for data scientists reflect national data, not school-specific information. Conditions in your area may vary. Data accessed January 2023.
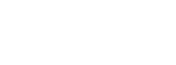
- Related Programs

Explore your training options in 10 minutes Get Started
- Graduate Stories
- Partner Spotlights
- Bootcamp Prep
- Bootcamp Admissions
- University Bootcamps
- Coding Tools
- Software Engineering
- Web Development
- Data Science
- Tech Guides
- Tech Resources
- Career Advice
- Online Learning
- Internships
- Apprenticeships
- Tech Salaries
- Associate Degree
- Bachelor's Degree
- Master's Degree
- University Admissions
- Best Schools
- Certifications
- Bootcamp Financing
- Higher Ed Financing
- Scholarships
- Financial Aid
- Best Coding Bootcamps
- Best Online Bootcamps
- Best Web Design Bootcamps
- Best Data Science Bootcamps
- Best Technology Sales Bootcamps
- Best Data Analytics Bootcamps
- Best Cybersecurity Bootcamps
- Best Digital Marketing Bootcamps
- Los Angeles
- San Francisco
- Browse All Locations
- Digital Marketing
- Machine Learning
- See All Subjects
- Bootcamps 101
- Full-Stack Development
- Career Changes
- View all Career Discussions
- Mobile App Development
- Cybersecurity
- Product Management
- UX/UI Design
- What is a Coding Bootcamp?
- Are Coding Bootcamps Worth It?
- How to Choose a Coding Bootcamp
- Best Online Coding Bootcamps and Courses
- Best Free Bootcamps and Coding Training
- Coding Bootcamp vs. Community College
- Coding Bootcamp vs. Self-Learning
- Bootcamps vs. Certifications: Compared
- What Is a Coding Bootcamp Job Guarantee?
- How to Pay for Coding Bootcamp
- Ultimate Guide to Coding Bootcamp Loans
- Best Coding Bootcamp Scholarships and Grants
- Education Stipends for Coding Bootcamps
- Get Your Coding Bootcamp Sponsored by Your Employer
- GI Bill and Coding Bootcamps
- Tech Intevriews
- Our Enterprise Solution
- Connect With Us
- Publication
- Reskill America
- Partner With Us
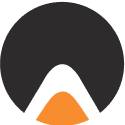
- Resource Center
- Bachelor’s Degree
- Master’s Degree
Best Doctorates in Data Science: Top PhD Programs, Career Paths, and Salaries
If you are tech-savvy and like to stay up-to-date on the latest developments in the computing field, you might be interested in getting a data science job. The industry is interdisciplinary, with those working within it proficient in statistics, computer science, and operations research.
As such, this career isn’t pursued casually, as extensive education is required to become a data scientist and enter the industry. While some jobs can be obtained with a master’s degree, earning one of the best PhDs in Data Science is a much better option if you want the freedom to be able to conduct your own research.
Find your bootcamp match
Within your data science PhD studies, you will spend the latter two years of the four-year program doing your own unique research and writing a dissertation on your findings, preparing you to do the same in the real world. Those with a master’s degree, by contrast, don’t have as many creative liberties and don’t usually develop their own research, but rather analyze existing studies.
With the amount of schooling and skills required to work within the field, you might be wondering what a PhD in Data Science Salary looks like. Below, we’ll discuss the top schools for getting a PhD in Data Science, as well as the career outlook once you get your degree.
What Is a PhD in Data Science?
A PhD in Data Science is a four- or five-year, full-time degree pursued after a bachelor’s or master’s degree. Faculty in university PhD programs often like students to have a prior master’s degree, but if not, they might offer integrated master’s and PhD studies.
Within a PhD in Data Science program, you’ll spend the first two years of your program learning foundational knowledge, taking advanced courses in statistics, computer programming, data mining, research methodology, and so much more. The latter two years of the degree involve conducting your own unique research. You will then record your findings in a dissertation and defend your research before a committee to get your doctorate.
How to Get Into a Data Science PhD Program: Admission Requirements
You can get into a Data Science PhD program by meeting a university’s admission requirements, which will differ between each school. Typically, the minimum educational requirement is a bachelor’s degree in a related STEM degree, but most programs prefer a prior master’s degree.
If you don’t have a master’s degree, it is highly recommended to at least be proficient in a coding language and to have taken classes in calculus, statistics, and engineering. You will also need to have a minimum 3.0 GPA across your postsecondary studies and send the school your academic transcripts. There are also supplemental materials you would need for an application. These include a statement of purpose, two or three letters of recommendation, GRE scores, and a professional resume.
PhD in Data Science Admission Requirements
- A postsecondary degree in a related field
- Academic transcripts
- Graduate record examination (GRE) scores
- Coursework in data structures, algorithms, calculus, and linear algebra
- A background in a programming language
- Letters of recommendation
- Admission essays
- Personal statement
Data Science PhD Acceptance Rates: How Hard Is It to Get Into a PhD Program in Data Science?
It can be hard to get into a PhD program in Data Science. PhD programs within universities are very exclusive. While they receive a sea of applications, most schools only accept about a dozen of them. For example, Yale, one of the best schools in the country, had over 300 applicants but only made around 13 offers. As such, it is wise to apply for multiple PhD programs in order to increase your chances of getting an offer of admission.
How to Get Into the Best Universities
[query_class_embed] how-to-get-into-*school
Best PhDs in Data Science: In Brief
Best universities for data science phds: where to get a phd in data science.
Since data science is a new field of scientific inquiry, it can be difficult to find the best universities for getting a data science PhD. In order to help you on your educational path, we’ve listed the 10 best schools for a data science PhD, below.
Arizona State University (ASU) was ranked the nation's most innovative university by US News & World Report. Originally founded in 1885, ASU has grown to now offer more than 160 programs at the graduate level in everything from art to engineering. The graduate school is well-known for its research work.
PhD in Data Science, Analytics, and Engineering
This program is geared toward those who want to work in either the data science industry, academia, or government to solve real-world problems through data-informed methods. The 12 credits of core courses within this program include data mining, statistics, security and assurance of information, and database management.
If you want to focus on engineering, you’ll take nine credits across cloud computing, database systems, and databases for web and other multimedia. If you want to focus on analytics, you'll take Machine Learning Statistics, Regression Analytics, and Data Visualization.
As a culmination of your studies, you’d produce a thesis. This requires you to propose a topic of study to the dissertation supervisory committee, and upon passing their comprehensive exam, you can begin your research. Ten days before you are to defend your dissertation, which must come less than a year after completing your 60th credit, you’ll submit a version of it to the committee for review.
PhD in Data Science, Analytics, and Engineering Overview
- Program Length: 4-6 years
- Acceptance Rate: 88% (school acceptance rate)
- Tuition: $11,720/year (in state); $23,544/year (out of state)
- PhD Funding Opportunities: Awards, fellowships, and scholarships
PhD in Data Science, Analytics, and Engineering Admission Requirements
- Application
- Application fee
- Official transcripts
- Three letters of recommendation
- Letter of intent
- GRE scores
Bowling Green State University (BGSU) offers more than 20 PhD programs in a variety of disciplines including engineering, psychology, business, and music. Since its beginnings in 1910, BGSU has been noted for its engineering and scientific research, being one of eight universities in the nation with the Carnegie Foundation’s Community Engagement Classification.
PhD in Data Science
This research-oriented program is interdisciplinary, incorporating teachings from applied statistics, operations research, and computer science. A unique aspect of BGSU’s program is that you’ll need to take ethics classes in order to understand the moral ramifications of gathering data and in communications to learn to effectively present their findings. Before beginning your 16- to 30-credit dissertation, you will need to pass the qualifying examination that involves oral and written sections.
PhD in Data Science Overview
- Program Length: 4-5 years
- Acceptance Rate: 79% (school acceptance rate)
- Tuition and Fees: $523/credit (in state); $856/credit (out of state)
- PhD Funding Opportunities: Assistantships, scholarships, fellowship
- Payment of $45 application fee
- Minimum GPA of 3.0
- Official or unofficial transcripts from previous institutions
- Graduate Record Examination (GRE) scores
- Graduate Management Admission Test (GMAT) scores
- Three letters of recommendation
- Resume
Chapman offers a variety of graduate programs, with 66 master’s and seven doctoral degrees in disciplines like business, law, education, and health sciences. It was founded in 1861 and is known for its research, with more than 31,000 citations from its 5,283 publications. Chapman University is also known for its strong alumni network, which can help graduates find jobs and networking opportunities.
PhD in Computational and Data Sciences
The PhD in computational and data sciences is designed for students who want to work in fields like population genetics, earth systems, biotechnology, bioinformatics, and economic science. The curriculum includes coursework in mathematical modeling, mining data, data analysis, and computational science, as well as research and thesis guidance from faculty.
The program is structured so that students can specialize in an area of computational science that interests them, such as scientific computing, data science, or machine learning, allowing students to also choose their dissertation topic. Before becoming doctoral candidates, students take qualifying exams for their core curriculum and do presentations on their elective courses.
PhD in Computational Data Sciences Overview
- Acceptance Rate: 60% (school acceptance rate)
- Tuition and Fees: $32,400 tuition
- PhD Funding Opportunities: Assistantships, work-study, loans
PhD in Computational and Data Sciences Admission Requirements
- Proof of satisfactory coursework in computer programming, differential equations, and statistics
- $60 application fee
- Two letters of recommendation
- 750-word statement of interest
This circa 1889 school offers more than 130 programs at the graduate level, with 1,687 students currently taking on one of its 50-plus doctoral programs. The school has various innovation clusters of research types, including those related to environmental sustainability, innovations in health, data science and cyberinfrastructure, transportation, and advanced manufacturing.
PhD in Biomedical Data Science and Informatics
This joint program through the college and the Medical University of South Carolina (MUSC) aims to teach students how to remedy issues in medicine through the combined study of information and computer sciences. Courses within the program will cover statistical theory, data management, machine learning, and bioinformatics.
Students spend the first two years doing coursework, the third year completing professional development training and research electives, and the fourth year solely researching. Research, seminars, and lab rotations will consist of 24 credit hours. Before completion of the program you’ll need to take a qualifying exam alongside proposing, writing, and defending your dissertation.
PhD in Biomedical Data Science and Informatics Overview
- Acceptance Rate: 49% (school acceptance rate)
- Tuition and Fees: $691/credit (in state); $1491/credit (out of state)
- PhD Funding Opportunities: Assistantships, scholarships and fellowships
PhD in Biomedical Data Science and Informatics Admission Requirements
- Bachelor’s degree in a STEM field, with one year of calculus and biology classes
- Graduate record examination (GRE) scores
- Prior computer programming work experience or coursework
- Work or research experience (recommended)
- Personal essay
- Two or three letters of recommendation
This university provides more than 30 doctoral programs just within the College of Science. Something unique to the circa 1949 school—known for its research in physics, immunology, molecular medicine, and biodiversity—is that its staff encourages research teams to incorporate members across various disciplines, bringing the insight and strengths of those respective fields together.
PhD in Computational Sciences and Informatics
Throughout this 72-credit program in the Department of Computational and Data Sciences, you’ll choose two out of four core courses in statistical and scientific visualization, advanced computing, databases, or numerical methods, and then choose from a rotating list of emphasis courses. Emphasis classes might cover topics like knowledge mining, statistical inference, or Bayesian inference decision theory.
By the end of your first year, you’ll need to obtain a research advisor, then get your proposal approved by the department committee to be considered a candidate for a PhD. A month before defending their dissertations, students conduct a pre-defense to get final revision recommendations.
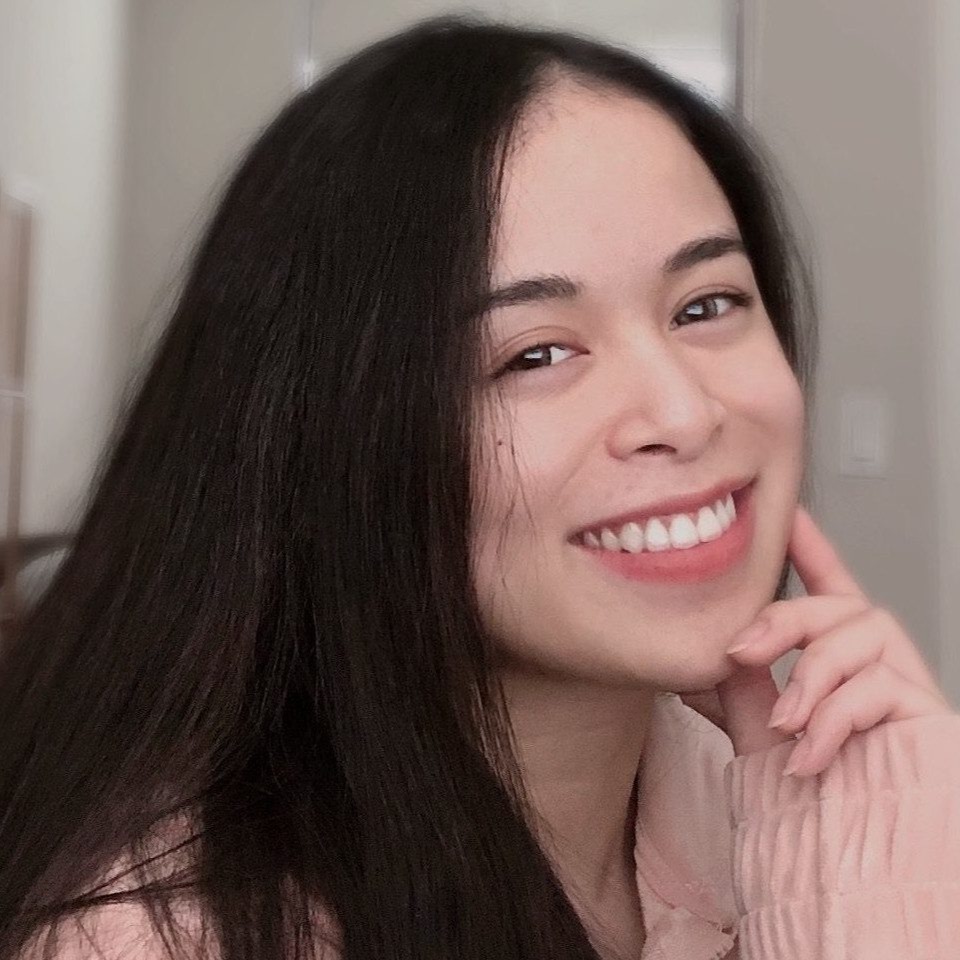
"Career Karma entered my life when I needed it most and quickly helped me match with a bootcamp. Two months after graduating, I found my dream job that aligned with my values and goals in life!"
Venus, Software Engineer at Rockbot
PhD in Computational Sciences and Informatics Overview
- Acceptance Rate: 91% (school acceptance rate)
- Tuition and Fees: $12,594/year (in state); $33,906/year (out of state)
- PhD Funding Opportunities: Fellowships, assistantships, lecturer positions, faculty grants, scholarships, work-study
PhD in Computational Sciences and Informatics Admission Requirements
- Mathematics background
- Knowledge of programming languages such as C, C++, and Python
- Personal statement
Though Harrisburg only joined the Commonwealth of Pennsylvania in 2001, the private, not-for-profit university has grown to have an enrollment of 4,000 students from over 100 countries. While it only offers three PhD degree programs in data science, computational science, and information systems engineering, it is known for its impressive research in supercomputer datamining, aquaponics, and virtual reality.
PhD in Data Sciences
This program strives to teach PhD candidates diverse methods of data science and train them to be able to apply their analytical knowledge across disciplines beyond data science. The first two years of the program are Harrisbug's Analytics Master's Degree, and students apply for the actual PhD in the final semester of that program.
If, however, students have a prior master’s degree from Harrisburg in computer science, they can transfer those credits and complete this four-to-five year doctoral degree in a shorter period. After completing the classwork portion of the degree, taking labs, seminars, classes, and doing fieldwork, they’ll begin their dissertation research. The defense of their dissertation will function as their final exam.
PhD in Data Sciences Overview
- Acceptance Rate: N/A
- Tuition and Fees : $800/credit hour (in state); $4,800/credit hour (out of state)
- PhD Funding Opportunities: Scholarships, grants, loans, work-study
PhD in Data Sciences Admission Requirements
- GRE/GMAT (strongly recommended)
- Essay on career goals
- Proof of research potential (courses or projects)
- Minimum master’s degree GPA of 3.3
- A letter of intent
The 1969-founded Indiana University-Purdue University Indianapolis (IUPUI) is an eponymous merger between the two schools and offers 550 programs across all levels. Of those, 57 are PhDs, covering everything from American studies and economics to addiction neuroscience and epidemiology. Some of their latest research breakthroughs were in the fields of informatics and computing, cardiology, nanosystems, and artificial intelligence.
With data science being a field in its infancy, IUPU’s School of Informatics and Computing strives to have its graduates be leaders within this ever-evolving industry. Students will take classes in system analysis and design, monitoring social media, and data mining and visualization.
PhD candidates can collaborate with professors on groundbreaking research in information infrastructures, Android science, computer security, machine learning, dataset integration, and computational social science. After earning this interdisciplinary degree, doctoral graduates will be ready to work in academia, health care, or even business intelligence.
- Acceptance Rate: 84% (school acceptance rate)
- Tuition and Fees: $425/credit (in state) ; $1,350.00/credit (out of state)
- PhD Funding Opportunities: Faculty grants, work-study, loans, internal funding, foundation or corporate funding, funding agencies
- GPA of 3.5 or higher
- GRE scores in the 70th percentile or higher for all sections
- Bachelor’s degree (master’s degree preferred)
- Completed classes in computer programming, statistics theory, linear algebra, and multivariable calculus
- Online application
- 500- to 750-word statement of purpose
Founded in 1966, Kennesaw provides more than 170 programs to its 40,000-plus students. Its 11 doctoral programs include studies in computer science, education, engineering, international diplomacy, business administration, and more. The core of the university's studies relates to technology and computing, medicine, human well-being and development, and sustainability.
Doctoral Degree in Analytics and Data Science
This interdisciplinary program combines business, math, stats, and computer science to make for well-rounded PhD candidates. Furthering that mission, the school also teaches written and oral communication skills to help graduates thrive in business or research fields.
In the 78-credit program, students will take classes on machine learning, mining data, analyzing big data, and graph theory in their first year. This is followed by 21 credits of electives in their second year. Though students often participate in research projects during their first two years, the latter two of their programs will involve independently-led studies for their dissertation.
Doctoral Degree in Analytics and Data Science Overview
- Acceptance Rate: 82% (school acceptance rate)
- Tuition and Fees: Qualified students are given a research stipend and waived tuition
- PhD Funding Opportunities: Foundations and institutes, corporate programs, scholarships, grants, loans, clearinghouses, coalitions, research stipends
Doctoral Degree in Analytics and Data Science Admission Requirements
- Master’s degree in computational-related discipline
- If no master’s degree, apply to the combined Master’s Degree in Applied Statistics or Computer Science program
- Strong proficiency in a programming language like Python
- Online application and $60 application fee
- Official transcripts from previous colleges or universities
- The graduate record examination (GRE) scores
- Statement of purpose
- Completion of math courses through Calculus II
- SAS Certification (preferred)
This 1874-founded school has 150 graduate programs, with 50-plus PhD programs in disciplines like medicine, physics, economics, and chemical physics. They have research programs in more than just pure and applied mathematics, as they also perform studies on wildfires, disinfectants, and autoregulation.
PhD in Statistics and Data Science
Prospective employees in academia, business, or government should consider this interdisciplinary research-based program in the Department of Mathematics and Statistics in the College of Science. The 72 credit hours of this degree are broken up into 48 hours of classwork covering topics like linear models, statistical theory and computing, and quantitative methods, 30 of which should be at the 700-level. There are 24 dissertation credits and 24 master’s classes from a previously finished graduate degree.
In order to continue within the PhD program after the third year, candidate hopefuls will need to pass a written qualifying test. After the qualifying test, students need to score highly on an oral exam in their chosen concentration before submitting and defending their dissertations.
PhD in Statistics and Data Science Overview
- Program Length: 4-6 years (8 years max)
- Acceptance Rate: 88% for overall school
- Tuition and Fees: $305.50/credit
- PhD Funding Opportunities: Work-study, assistantships, scholarships, stipends, tuition waiver, subsidized medical plan
PhD in Statistics and Data Science Admission Requirements
- Online application
- Bachelor’s and master’s degree transcripts
- Mathematics test scores (recommended)
- Financial aid application
Founded in 1701, Yale University is one of the oldest universities in the United States and is ranked fifth-best school in the nation by US News & World Report. Yale has 12 different professional schools and 73 different graduate degree programs. The university is especially well-known for its research in the humanities, environmental science, social sciences, and biotechnology.
PhD program in Statistics and Data Science
Students entering this degree program will focus on probability, statistics, information theory, data mining, machine learning, neural networks, and more as their foundational studies. After that, students take elective classes on one-off special topics classes that change between semesters.
Those in the program need to take an oral and practical exam in their first year and begin their dissertation work in either their second or third year. This is usually a five-year program, with students getting a dissertation fellowship in their fifth year. Yale is a very exclusive school, and last year only made between 12 and 14 offers to the 300 applicants it received. As such, applying to other schools, in addition to Yale, would be a wise choice.
PhD program in Statistics and Data Science Overview
- Program Length: 5 years
- Acceptance Rate: 5% (school acceptance rate)
- Tuition and Fees: $45,700/year (waived through provided fellowship)
- PhD Funding Opportunities : PhD students get a fellowship that covers all tuition through first five years in addition to an annual stipend of $36,000, Teaching fellowships, stipends, and health care benefits
PhD program in Statistics and Data Science Admission Requirements
- Graduate record examination (GRE) scores (optional)
- Strong mathematical background
- Unofficial transcripts from previous colleges
Can You Get a PhD in Data Science Online?
Yes, you can get a PhD in Data Science online. There are a few fully-online PhD programs in data science provided by schools like Northcentral University. If you wish to pursue your PhD online but haven’t been accepted into a program for data science, you can consider a computer science program that has a concentration in data science. Since data science is a subset of computer science, you would learn the same foundational skills in either program.
Best Online PhD Programs in Data Science
How long does it take to get a phd in data science.
It typically takes four to five years to complete a PhD in Data Science. While four years is the standard for most schools, some programs take a fifth year to complete due to the exhaustive research conducted. Most of the programs we’ve covered above require students to complete between 70 to 80 credits.
While that only requires between eight and 10 credits per semester, students’ schedules are filled with doing research, being a teacher’s assistant, and completing a fellowship. The amount of coursework required, the research component, and the dissertation are all factors that can affect the time it takes to earn a PhD in Data Science.
Is a PhD in Data Science Hard?
Yes, a PhD in Data Science is hard as it involves taking incredibly technical classes and conducting your own novel research within data science. The academic discipline is the merging of computer science, statistics, operations research, and more, meaning that successful students must be proficient in a wide range of technical skills.
How Much Does It Cost to Get a PhD in Data Science?
It costs, on average, $19,314 per year to get a PhD in Data Science , according to the National Center of Education Statistics. The cost will change depending on the type of school a student attends. If a doctoral student studies at a public university, the tuition is only $12,171, on average. By contrast, if a doctoral student studies at a private institution, tuition costs about $14,208 at for-profit universities, and $27,776 for nonprofit universities.
How to Pay for a PhD in Data Science: PhD Funding Options
There are many different avenues students can look into to pay for a PhD in Data Science. Some schools, such as Yale and Kennesaw State University, waive tuition for eligible students, and might even give students a yearly stipend. Another common option is to do an assistantship, in which you’d work within your data science department by teaching or doing research.
Students can also apply for various scholarships or grants to help cut down the cost of tuition. While scholarships for undergraduate students are typically merit-based, PhD funding is achieved through a student’s specific field, supporting their research and cutting tuition costs.
Best Online Master’s Degrees
[query_class_embed] online-*subject-masters-degrees
What Is the Difference Between a Data Science Master’s Degree and PhD?
The difference between a data science master’s degree and PhD is that the former program only takes about two years to complete, while the latter is the educational step past a master’s degree that takes at least four years to complete. In fact, the first two years of a PhD program are usually a master’s degree program.
As such, some schools prefer applicants to have master’s degrees to cut down on the length of time. A master’s degree, and the first two years of a PhD program, are more so classroom-based. For PhD students, this is when students learn the foundations they’ll need to conduct their own research in the final two years of their program.
Master’s vs PhD in Data Science Job Outlook
The job outlook for people with a Master’s or PhD in Data Science is very positive. Data science is a new scientific field, so workers within its industries are in high demand. For example, computer and information research scientists , which have a minimum requirement of a master’s degree, should see their careers grow by 22 percent between 2020 and 2030, according to the US Bureau of Labor Statistics (BLS).
Medical scientists , which have a minimum educational requirement of a PhD, should see job growth of 17 percent between 2020 and 2030. While The PhD job outlook is lower in this instance, a PhD is highly desirable, which is evident by the salary discrepancy below.
Difference in Salary for Data Science Master’s vs PhD
A PhD typically leads to a higher salary than a master’s degree. For example, the US Bureau of Labor Statistics reports that computer and information research scientists average $131,490 per year, while medical scientists in the scientific research and development services industry make $129,800. By contrast, those with a master’s degree tend to earn an average salary of $106,000 , according to PayScale.
While the BLS states that the minimum educational requirement for that job is a master’s degree, typically those with master’s degrees work in analyzing existing data. With a PhD, you can conduct research within this innovative field.
Related Data Science Degrees
[query_class_embed] https://careerkarma.com/blog/data-science-bachelors-degrees/ https://careerkarma.com/blog/best-online-data-science-bachelors-degrees/ https://careerkarma.com/blog/data-science-masters-degrees/
Why Should You Get a PhD in Data Science?
You should get a PhD in Data Science because you will be one of the pioneering leaders in this budding field. While a master’s program teaches students how to analyze data, a PhD program empowers students to do their own research. Read below for why you should get a PhD in Data Science.
Reasons for Getting a PhD in Data Science
- Lead the Field. Data science is a new field, so those that get a doctoral degree will be at the forefront of new developments. As you’ll be analyzing data, it’s incredibly exciting to think that your PhD research will be groundbreaking.
- Ever-evolving job: Technology is constantly advancing at incredible speeds, so being the one to learn about these advancements will never get old. With artificial intelligence technologies on the rise, one can only imagine in just 10 years’ time how different our understanding of data science will be.
- Specialize in interest. As students go along their educational paths, they go from learning foundational knowledge to increasingly specific information. Thus, if you’re passionate about a subset of data science but didn’t get to focus on it in your bachelor’s or master’s degree program, a PhD is the perfect opportunity to study, research, and work within your interests.
- High salaries. As the field of data science grows, the need for data science experts will also increase. PhD graduates will be uniquely equipped for the industry’s changing landscape and will be highly sought-after.
- Research opportunities. While this is an enriching hands-on experience, it lays the groundwork for you to be able to conduct your own studies in the latter two years of your program. You will be able to follow your passions rather than just helping a faculty member succeed in their work.
- Job Market. The BLS projects that job openings in computer and information research sciences will grow by 22 percent from 2020 to 2030. In getting a PhD, you will be a more competitive applicant than those with a lesser degree. It’s likely that you can even negotiate higher salaries because of your specialties.
Getting a PhD in Data Science: Data Science PhD Coursework
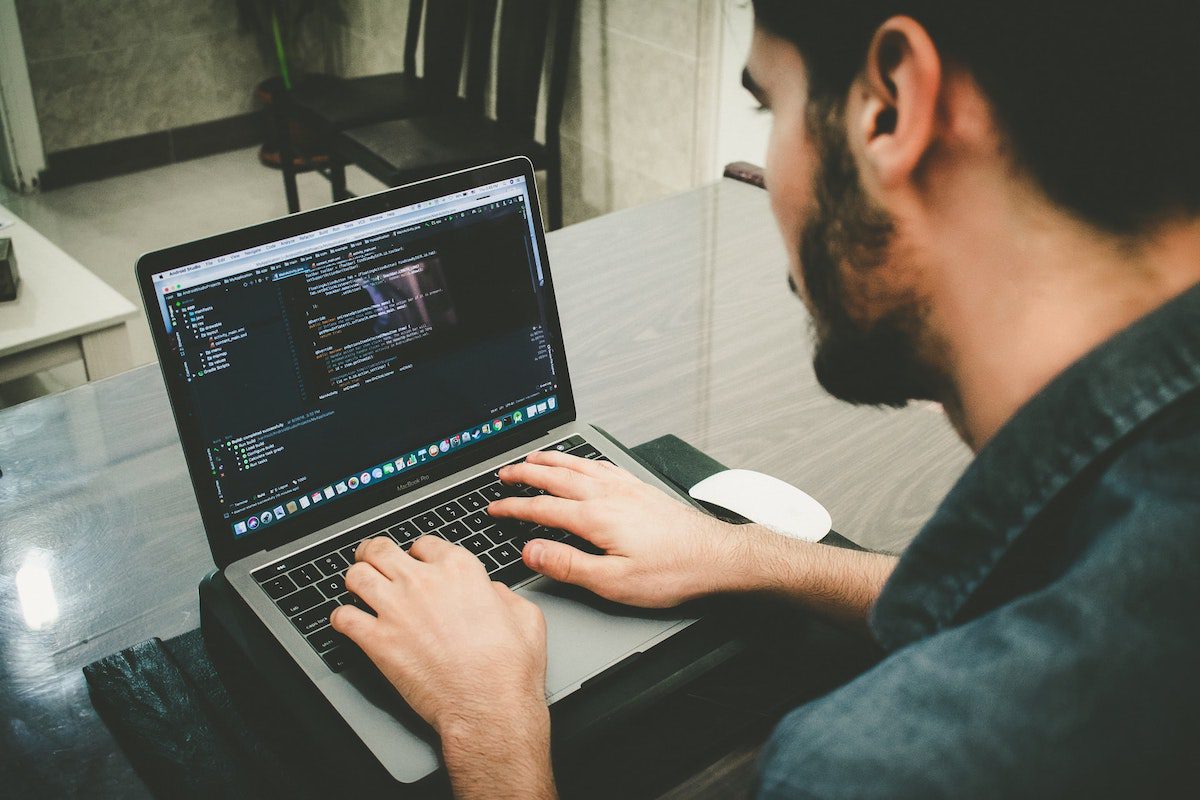
Data science is an interdisciplinary field, involving bioinformatics, computer science, statistics, and operations research. As such, the coursework PhD students undertake is diverse, including data mining, bioinformatics, ethics, and data visualization. Below, we’ll discuss some of the common classes found throughout most PhD programs in data science.
Introduction to Data Science
This class will teach you the baseline information you’ll need to know to advance in your data science career. Since you’re in an advanced degree program, you’ll likely be working with real data from case studies. You’ll take that information and learn how to build and manage databases, visualize data, and run statistical analyses.
Data Mining
Raw data, though important, isn’t useful until it can be contextualized and analyzed. Data mining is also called “knowledge discovery,” meaning that mining is the process of digging through mounds of data to learn information. Students will code, select and visualize data, use machine learning, and clean information to make novel findings.
Bioinformatics
As the combination of terms implies, bioinformatics is where biology and informatics meet and involves the study of biological data. This field of study is essential for those that want to go into medicine as data scientists. If you haven’t yet completed your bachelor’s degree, pursuing one of the best undergraduate degrees in bioinformatics is a wise choice.
Ethics of Data Science
Since data science involves collecting and storing information, mostly on people, there are possible moral ramifications to this. Within an ethics class, you’ll learn the proper methodology for conducting research to assure that your work meets codes of conduct.
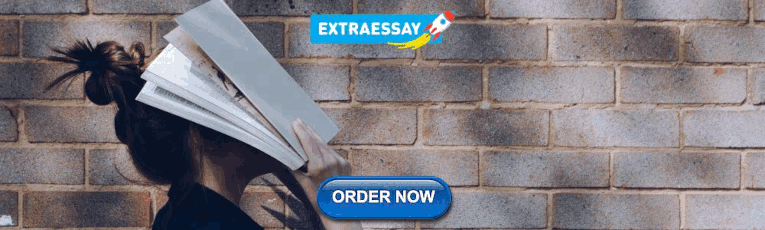
Data Visualization
An important aspect of conducting research is being able to articulate your discoveries. Through visualization in programming languages like R, you’ll learn how to plot data and make reports. This process helps you organize your findings as well as snuff out any errors made during computation.
Best Master’s Degrees
[query_class_embed] *subject-masters-degrees
How to Get a PhD in Data Science: Doctoral Program Requirements
You can get a PhD in Data Science by meeting your chosen university’s degree requirements. Though these can vary, there are commonalities across different schools, such as completing a set number of credits, taking exams, and crafting a dissertation. We’ll now go into more detail about these common components of the doctoral degree.
Data Science PhD programs typically require the completion of 70 and 80 credit hours. This is often split down the middle, with the first half of credits being done in a classroom, and the latter half being done through your research and dissertation.
While full-time undergraduate students take 15 or so credits per semester, the number of PhD students is lower as they conduct work outside of classroom hours through assistantships. Candidates typically complete this degree studying full-time for four to five years, taking between eight and 10 credits per semester. Most schools have a cap on the maximum number of years a student has to complete their PhD. For example, University of Nevada’s maximum allowance is eight years.
Before entering a PhD program, students already have a bachelor’s or master’s degree in a relevant STEM discipline. With the basics in coding languages, a statistical method, calculus, and engineering out of the way, doctorate students can take a deep dive into more difficult and focused courses. Some examples of classes PhD students will take include machine learning, data visualization, and bioinformatics.
Often students will be able to choose a specialization to narrow the focus of their research. This allows them to take more niche classes on topics like asymptotics, stochastic processes, and Bayes theorem. After completing two years of classwork, students then begin their dissertation studies.
In order to be considered candidates for a PhD, students will need to pass exams between the first and third year of their degree program. The tests, which often consist of a verbal exam and written assessment, determine what the candidate-hopefuls have learned so far and whether they will be effective researchers with the school’s department.
After completing two years of coursework and passing their qualifying exams, PhD candidates begin research for their eventual dissertations. Candidates collaborate with a chosen faculty member to help guide them in their approved topic of study. Students then write about their findings in a dissertation or thesis, which they will need to defend in front of a committee before being considered doctors.
Potential Careers With an Data Science Degree
[query_class_embed] how-to-become-a-*profession
PhD in Data Science Salary and Job Outlook
The salary and job outlook for those with a PhD in Data Science is very positive. The field of study is fairly new, and while there might not be many jobs that specifically require a PhD, having a terminal degree will make you a competitive applicant for prospective employers.
Data science PhD holders can work as medical scientists in the scientific research and development services industry or as information and computer research scientists. For the former industry, which requires a PhD, there will be a 17-percent increase in job openings between 2020 and 2030, per the US Bureau of Labor Statistics (BLS). The US BLS reports that computational and informational research sciences will see a 22-percent increase in job openings during that decade. Average salaries for those professionals are $116,430 and $131,490, respectively.
Though the salary for those in computational and informational research sciences is higher and typically only requires a master’s degree, those with a PhD are more likely to work in those positions. This is because PhD holders often conduct research, having done so in their doctoral programs, while former graduate students often analyze existing findings instead.
What Can You Do With a PhD in Data Science?
With a PhD in Data Science, there are a plethora of jobs within reach . The field of study is interdisciplinary, meaning that you’d be equipped with the skills to thrive in careers relating to computer science, bioinformatics, engineering, data management, and so much more. Let’s discuss further some of the highest-paying jobs that you can get with a PhD in Data Science.
Best Jobs with a PhD in Data Science
- Computer and Information Research Scientist
- Mathematicians/Statistician
- Medical Scientist
- Machine Learning Engineer
- Data Scientist
What Is the Average Salary for a PhD in Data Science?
The average salary for a PhD in Data Science is around $131,000. Payscale reports this is the average salary for those with a PhD in Computer Science, and since data science is a specialization of computer science, one can infer the salaries would be similar.
While $131,000 can be the expected salary for those with a Doctorate in Computer Science—and data science, by extension—the average salary you might earn will depend on a few variables. These include the amount of work experience you have, the industry you are working in, the organization you are working for, and the region of the country you are working in.
Highest-Paying Data Science Jobs for PhD Grads
Best data science jobs with a doctorate.
The best data science jobs with a doctorate are as a computer and information research scientist, mathematician or statistician, medical scientist, machine learning engineer, or data scientist. All of the above careers earn over $100,000 per year, but the actual salary a job might offer can differ.
These professionals are found across health care, corporate, and scientific fields and work to optimize the computer systems for their organization. This is done through distilling overly-complicated algorithms, troubleshooting issues with other engineers, and conducting research into developing new electronic programs.
Though these jobs usually have a minimum education requirement of a master’s degree, those with a PhD are likely to also populate this sector and will likely be given preference by employers. This is because those with a PhD conduct research more frequently than those with a master’s degree, who usually analyze existing data.
- Salary with a Data Science PhD: $131,490
- Job Outlook: 22% job growth from 2020 to 2030
- Number of Jobs: 33,000
- Highest-Paying States: Oregon, Texas, Arizona, Washington, Massachusetts
While both statisticians and data scientists analyze sets of information, a difference between the two is that while the field of computational statistics can be broad, data science is more focused on computer science and machine learning. They do, however, use the same methodology for analysis, so with your PhD in Data Science, you’d be equipped to be a statistician in a variety of industries.
According to the US Bureau of Labor Statistics, statisticians and mathematicians that work in technical, professional, and scientific services make on average $129,800, and those that work in life, engineering, and physical science development and research earn $114,770 per year.
- Salary with a Data Science PhD: $129,800
- Job Outlook: 33% job growth from 2020 to 2030
- Number of Jobs: 44,800
- Highest-Paying States: Connecticut, New York, Massachusetts, Wyoming, and California
Working in what the BLS calls the “ scientific research and development services industry ,” you could use your data science know-how in the medical field, especially if you concentrated or did your dissertation in health care. You’d likely data mine through patient information, analyzing it to then make recommendations to those in your health system organization.
- Salary with a Data Science PhD: $116,430
- Job Outlook: 17% job growth from 2020 to 2030
- Number of Jobs: 133,900
- Highest-Paying States: Maine, New Jersey, Tennessee, Connecticut, Delaware
Artificial intelligence is growing alongside the data science field. Pursuing this career would allow you to be able to help foster artificial intelligence (AI) programs. You’d code your own AI system, teaching it how to analyze large amounts of data and how the system should respond to it.
- Salary with a Data Science PhD: $112,709
- Job Outlook: 22% job growth from 2020 to 2030
- Number of Jobs: 33,000 (for computer and information research scientists)
- Highest-Paying States: Oregon, Texas, Arizona, Washington, Massachusetts (for computer and information research scientists)
Those that work within this field combine their knowledge of informatics, computer programming, data mining and management, and more to conduct research by studying data sets. Data Scientists can work in business, devising avenues to optimize profits by looking at reports.health care, presenting their studies to guide decisions bettering the medical system; and academia, working to innovate this budding field of study.
- Salary with a Data Science PhD: $108,660
- Number of Jobs: 105,980
- Highest-Paying States: New Jersey, New York, Delaware, Washington, California
Is a PhD in Data Science Worth It?
Yes, a PhD in Data Science is much worth it. Though not all data science jobs require a PhD, with some upper-level careers only requiring a master’s degree, you would have an advantage over others with lower levels of education. You’d have experience conducting your own research method, which would prepare you for running your own studies in the real world.
Most with master’s degrees don’t actually develop their own studies, rather, they analyze existing information. A PhD would give you a competitive edge, making you a more impressive candidate to prospective employers. You’d be more likely to get hired, and more plausibly able to negotiate a higher salary.
Data science is a new field of inquiry, so by having a doctorate in it, you would be at the forefront of the technological advancements within the industry. You would likely make at least $100,000 yearly in data science and have the interdisciplinary skills to work in other industries if you desire.
Additional Reading About Data Science
[query_class_embed] https://careerkarma.com/blog/introduction-to-data-science/ https://careerkarma.com/blog/how-to-get-a-job-in-data-science/ https://careerkarma.com/blog/data-science-degree/
PhD in Data Science FAQ
Yes, many data scientists have PhDs, but it is not a requirement for many jobs in the industry. Some require only master’s degrees instead, but there are advantages to having a PhD. With a PhD, you’ll have conducted your own research to get your doctorate, allowing you to more easily create your own studies in the real world. Those with a master’s degree, by contrast, usually only analyze existing studies, giving you less creative liberties with what you work on.
There are many career options for those with a PhD in Data Science, as the course of study is interdisciplinary. This means that those with the degree are taught technical skills that can apply to multiple different industries. Often, those with a data science degree go on to work in either business, medicine, or academia.
The US Bureau of Labor Statistics (BLS) reports that the median annual salary for data scientists is $131,490 . This report states that those within this job often have a master’s degree, meaning that with your PhD, you’d be a more impressive candidate and be able to negotiate a higher salary. Additionally, the BLS estimates that demand for data scientists will grow by 22 percent from 2020 to 2030, a much higher rate than the national average of eight percent.
A PhD is the best degree to become a data scientist if you want to conduct your own studies. Many within data science have lesser degrees, usually a master’s and sometimes a bachelor’s, which in turn gives them less responsibility within the field. If you have a scientific inquiry that you are passionate about and want the freedom to study what you want, a PhD is the best degree to obtain.
About us: Career Karma is a platform designed to help job seekers find, research, and connect with job training programs to advance their careers. Learn about the CK publication .
What's Next?

Get matched with top bootcamps
Ask a question to our community, take our careers quiz.
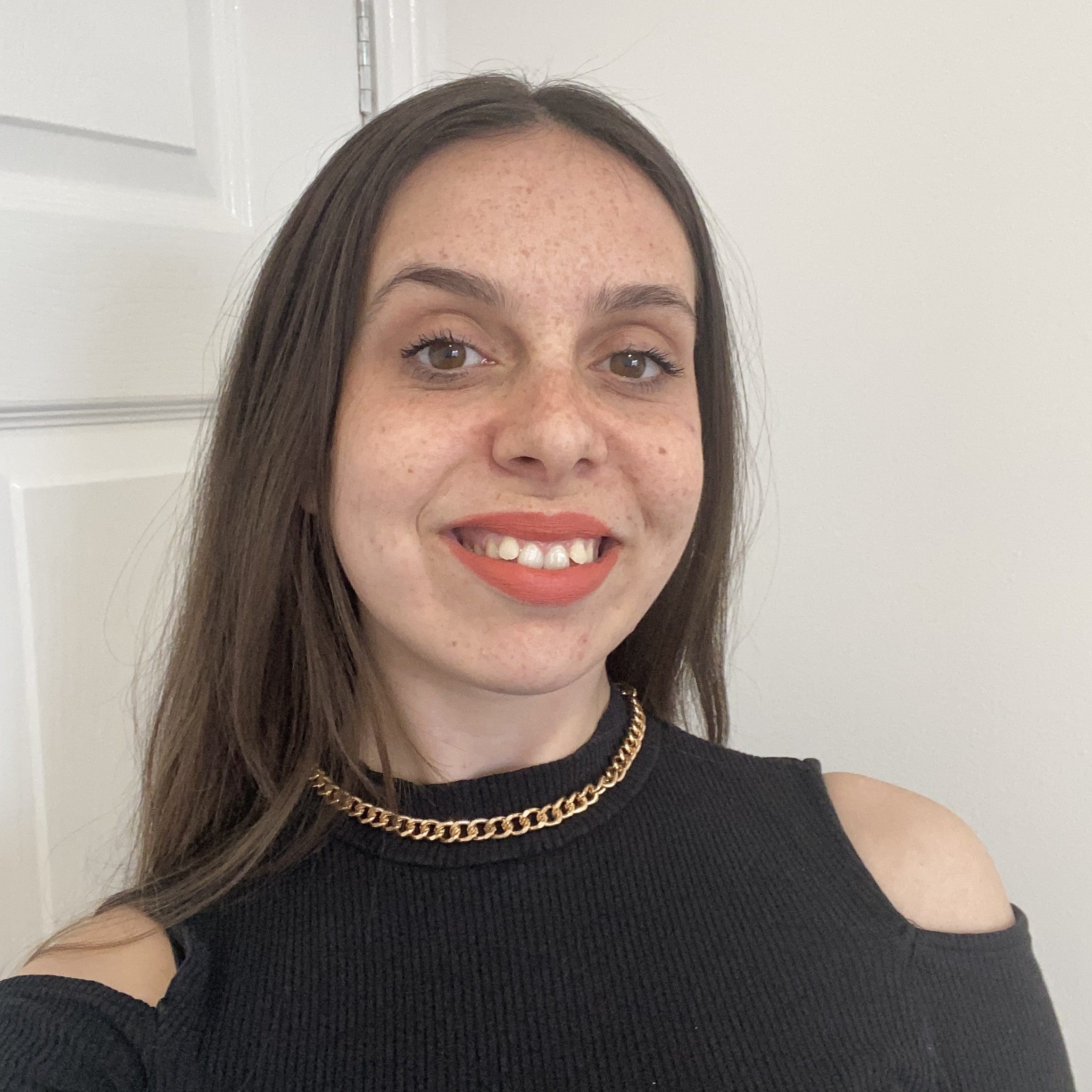
Leave a Reply Cancel reply
Your email address will not be published. Required fields are marked *
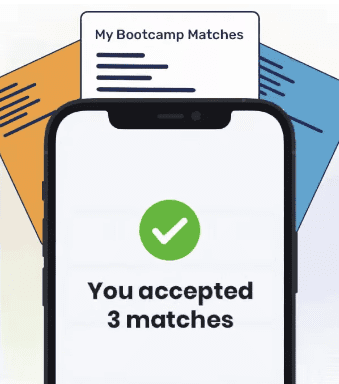
- UC Berkeley
- Sign Up to Volunteer
- I School Slack
- Alumni News
- Alumni Events
- Alumni Accounts
- Career Support
- Academic Mission
- Diversity & Inclusion Resources
- DEIBJ Leadership
- Featured Faculty
- Featured Alumni
- Work at the I School
- Subscribe to Email Announcements
- Logos & Style Guide
- Directions & Parking
The School of Information is UC Berkeley’s newest professional school. Located in the center of campus, the I School is a graduate research and education community committed to expanding access to information and to improving its usability, reliability, and credibility while preserving security and privacy.
- Career Outcomes
- Degree Requirements
- Paths Through the MIMS Degree
- Final Project
- Funding Your Education
- Admissions Events
- Request Information
- Capstone Project
- Jack Larson Data for Good Fellowship
- Tuition & Fees
- Women in MIDS
- MIDS Curriculum News
- MICS Student News
- Dissertations
- Applied Data Science Certificate
- ICTD Certificate
- Citizen Clinic
The School of Information offers four degrees:
The Master of Information Management and Systems (MIMS) program educates information professionals to provide leadership for an information-driven world.
The Master of Information and Data Science (MIDS) is an online degree preparing data science professionals to solve real-world problems. The 5th Year MIDS program is a streamlined path to a MIDS degree for Cal undergraduates.
The Master of Information and Cybersecurity (MICS) is an online degree preparing cybersecurity leaders for complex cybersecurity challenges.
Our Ph.D. in Information Science is a research program for next-generation scholars of the information age.
- Spring 2024 Course Schedule
- Summer 2024 Course Schedule
- Fall 2024 Course Schedule
The School of Information's courses bridge the disciplines of information and computer science, design, social sciences, management, law, and policy. We welcome interest in our graduate-level Information classes from current UC Berkeley graduate and undergraduate students and community members. More information about signing up for classes.
- Ladder & Adjunct Faculty
- MIMS Students
- MIDS Students
- 5th Year MIDS Students
- MICS Students
- Ph.D. Students

- Publications
- Centers & Labs
- Computer-mediated Communication
- Data Science
- Entrepreneurship
- Human-computer Interaction (HCI)
- Information Economics
- Information Organization
- Information Policy
- Information Retrieval & Search
- Information Visualization
- Social & Cultural Studies
- Technology for Developing Regions
- User Experience Research
Research by faculty members and doctoral students keeps the I School on the vanguard of contemporary information needs and solutions.
The I School is also home to several active centers and labs, including the Center for Long-Term Cybersecurity (CLTC) , the Center for Technology, Society & Policy , and the BioSENSE Lab .
- Why Hire I School?
- Request a Resume Book
- Leadership Development Program
- Mailing List
- For Nonprofit and Government Employers
- Jobscan & Applicant Tracking Systems
- Resume & LinkedIn Review
- Resume Book
I School graduate students and alumni have expertise in data science, user experience design & research, product management, engineering, information policy, cybersecurity, and more — learn more about hiring I School students and alumni .
- Press Coverage
- I School Voices
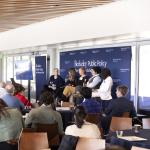
The Goldman School of Public Policy, the CITRIS Policy Lab, and the School of Information hosted the inaugural UC...
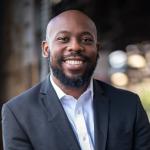
Dr. Diag Davenport has been appointed as an assistant professor at UC Berkeley as part of a joint search in...
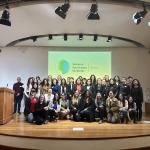
At the Women in Data Science conference held at UC Berkeley this past week, four educators affiliated with the...
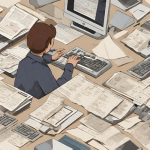
When the Bancroft Library received over 100,000 Japanese-American internment “individual record” forms (WRA-26) from...
- Distinguished Lecture Series
- I School Lectures
- Information Access Seminars
- CLTC Events
- Women in MIDS Events
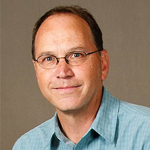
Ph.D. in Information Science
Ph.d. community.
Ph.D. students are knowledge architects and respected contributors to our information society, with a vision of expanding access to quality information, an appreciation for diverse perspectives, and the spirit of collaboration.
You Belong at Berkeley
The I School is a welcoming community of students, faculty, and staff from a wide variety of backgrounds, nations, cultures, and experiences.
The doctoral program is a research-oriented program in which the student chooses specific fields of specialization, prepares sufficiently in the literature and the research of those fields to pass a qualifying examination, and completes original research culminating in the written dissertation. The degree of Doctor of Philosophy is conferred in recognition of a candidate's grasp of a broad field of learning and distinguished accomplishment in that field through contribution of an original piece of research revealing high critical ability and powers of imagination and synthesis.
The Ideal Place for Breakthrough Thinking
School of Information offers an ideal environment for information scholars , on the campus of a preeminent, forward-thinking research institution .
Dedicated to cross-disciplinary research, breakthrough thinking, and creative collaboration, the I School actively shapes the information frontier and has a track record of scholarly ideas, solutions, and policy counsel that make information more accessible, manageable, and useful.
Rigorous academics instill the theoretical and research capabilities required to advance diverse interests — from information design, architecture, and assurance, to human-computer interaction and the social, economic, and public policy implications of information. Ph.D. students work closely with faculty recognized as information pioneers.
Interdisciplinary thinking and partnership are central to the I School approach, so doctoral research often engages exceptional UC Berkeley schools and departments beyond the I School, from journalism, business, and law to computing, engineering, humanities, and social sciences.
On average, I School students complete the Ph.D. degree in 6 years.
- Semester 1–4 : Breadth, major, & minor coursework
- Semester 4–5 : Prelim research paper & exam
- Semester 6–8 : Qualifying exam
- Semester 10–12 : Complete & present dissertation
Detailed degree requirements & timeline
Areas of Study
Major and minor areas include:
- Human-Computer Interaction
- Information Economics and Policy
- Information Law and Policy
- Information Organization and Retrieval
- Information Systems Design
- Social Aspects of Information
- Information and Communication Technologies and Development
Your Career
I School Ph.D. graduates go on to careers in academia, industry, or the public sector.
Recent Ph.D. graduates hold tenure-track faculty positions at the world’s leading universities, as well as leading research positions in industry, academia, and public-interest organizations.
More about Ph.D. career outcomes
- MIMS Program
- 5th Year MIDS Program
- MIDS Program
- MICS Program
- Graduate Certificates

“I think we can do a better job of using the Internet to tap into how people are doing, how they’re feeling, and what matters to them — online democracy, in a way, but minus the hype.”
—Galen Panger Ph.D. 2017
Download printable flier
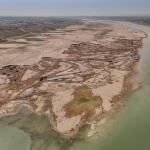
New Research Aims to Curb Illegal Sand Mining with Data-Driven Mapping Tools
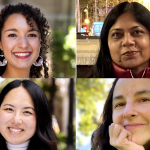
I School Representatives Lead DEIBJ Initiatives
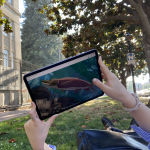
Human-Computer Interaction Research De-Centers Humans to Give Nature a Voice
Last updated:.
- Application

- Weill Cornell Medicine

MS in Health Informatics
Transforming healthcare through data and information technology.
Our MS in Health Informatics program is focused on the application of information technology and data science in healthcare delivery. We study, develop, and improve healthcare information technologies. To apply these information technologies effectively, we also study human and organizational behavior.
Core Training
Our core curriculum covers three domains:
- Information technology and data science: Students study statistical methods, machine learning, data management, and informatics standards and technology infrastructure.
- Health and healthcare: Students learn about domestic and global healthcare, shadow physicians and visit hospitals.
- Human and organizational behavior: Students cover human factors, human-computer interaction and diffusion of innovation to learn how to position information systems for success.
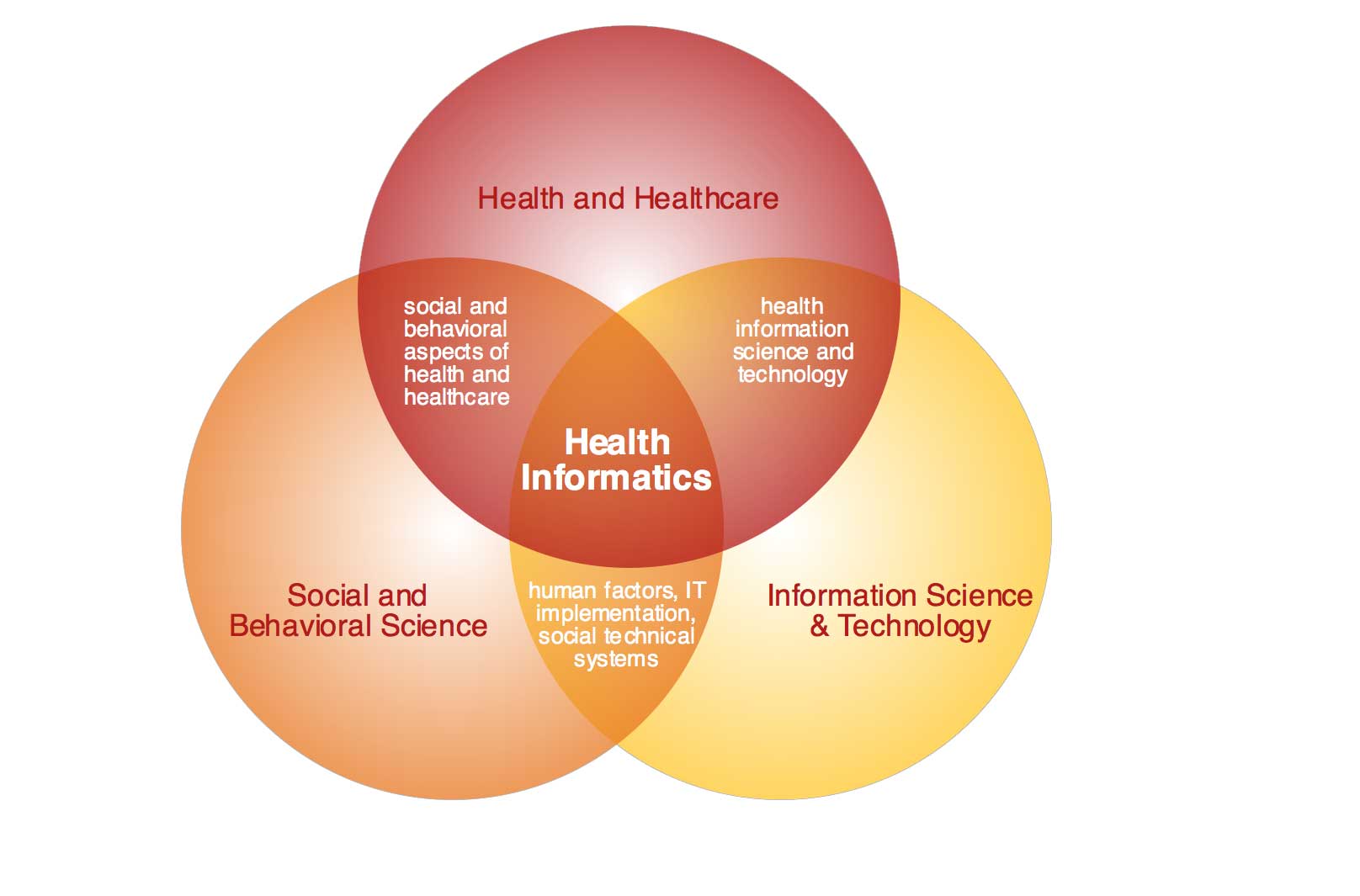
Our program offers innovative key informatics skills blended with healthcare system knowledge. Students study cutting-edge topics in health informatics such as statistical and machine learning, data management, and consumer informatics, culminating in a hands-on capstone project with clients from our industry partners.
Collaboration is at the core of our program, with students and faculty from a range of fields, working with a wide array of collaborating NYC institutes. This diversity creates a unique learning environment. To get a sense of our culture, please take a look at our Admissions Information .
Our alumni hold positions in data and policy analysis, health information technology, process improvement, consulting, and more at healthcare institutions and startups. Many alumni pursue doctoral studies.
Students can complete the health informatics curriculum in 12 months.
Research Projects

Prerequisites for Admission
Information sessions, alumni outcomes, program director.
Jose Florez-Arango, MD, PhD, MS
HI 1 Year Student - Recommended Curriculum Progression
Students are recommended to follow the schedule below in order to ensure eligibility for graduation. The Education Team will monitor progression, but it is ultimately the student’s responsibility to track their progression to ensure they meet graduation requirements. Course offerings and course availability are subject to change.
Typical course load is 15 credits
Introduction to Biostatistics with STATA Lab (HBDS 5001) OR Biostatistics I with R Lab (HBDS 5005) - Required
Introduction to Biostatistics with STATA Lab Course Director: Arindam RoyChoudhury, PhD 4 credits
An introduction to the fundamentals of biostatistics with primary emphasis on understanding of statistical concepts behind data analytic principles. This course will be accompanied with a Stata lab to explore, visualize and perform statistical analysis with data. Lectures and discussions will focus on the following: exploratory data analysis; basic concepts of statistics; construction of hypothesis tests and confidence intervals; the development of statistical methods for analyzing data; and development of mathematical models used to relate a response variable to explanatory or descriptive variables.
Biostatistics I with R Lab Course Director: Xi Kathy Zhou, PhD 4 credits
This course provides an introduction to important topics in biostatistical concepts and reasoning. Specific topics include tools for describing central tendency and variability in data, probability distributions, sampling distributions, estimation, and hypothesis testing. Assignments will involve computation using the R programming language.
Introduction to Health Informatics (HINF 5001) - Required
Course Director: Marianne Sharko MD, MS 3 credits
Health informatics is the body of knowledge that concerns the acquisition, storage, management and use of information in, about and for human health, and the design and management of related information systems to advance the understanding and practice of healthcare, public health, consumer health and biomedical research. The discipline of health informatics sits at the intersection of several fields of research – including health and biomedical science, information and computer science, and sociotechnical and cognitive sciences. In recent years we have witnessed how the collection, storage and usage of digital health data has exponentially grown. Increases in the complexity of health information systems have driven growth in demand for a specialized workforce. This course introduces the field of health informatics and provides students with the basic knowledge and skills to pursue a professional career in this field and apply informatics methods and tools in their health professional practice.
Research Methods in Health Informatics (HINF 5004) - Required
Course Director: Yunyu Xiao, PhD 3 credits
Informatics innovations have their desired impact only when they have high quality, are highly usable, are integrated into their organizational setting, and are widely adopted and used. That makes it critical for informatics students to understand not only how informatics innovations work, but also the users and settings in which they are used. Students will learn methods and models for: measuring data and system quality; assessing and predicting technology adoption (what makes technology sticky?); improving humancomputer interaction via human factors engineering; understanding organizational and systemic challenges in the real world; influencing patients’ health behavior and decisions; and assessing quality, safety, and cost outcomes using health services research study designs. In this mixed methods course, students will gain experience using both quantitative and qualitative methods.
Healthcare Organization and Delivery (HPEC 5002) - Required
Course Director: Lisa Kern MD, MPH 3 credits
The goal of this course is to educate students about the complexity and nuances of healthcare delivery. The course will be especially useful for non-clinicians who intend to go into fields that will require a detailed understanding of healthcare. Class sessions will not summarize healthcare; rather, they will analyze healthcare – through themes such as people, time, money, communication, uncertainty, and others. Students will come away from the course with a deeper appreciation of why it is difficult to change healthcare. They will then be able to anticipate the intended and unintended consequences of interventions and policies that they and others might implement.
Master’s Project 1 and Professional Development (HCPR 9010) - Required
Course Director: Faculty 2 credit
This is the culminating capstone course of all masters-level graduate education programs. It has two aims: (1) helping students to discover and develop new and effective ways of managing and working together with all the stakeholders within the healthcare field and (2) helping accelerate a student's development of 12 the context awareness, integrative management, and industry skills that are needed to lead in a rapidly changing healthcare sector. This capstone course puts students in a new organization, one they don’t already know well, and gives them the chance to practice hitting the ground running. This culminating course provides a deeper preparation for the next stages of a student's career. The capstone project will last the entire year: the first term involves matching students with the right project, the second term has students working with their client, and the third term consists of a detailed report and final presentation in front of the client as well as faculty and fellow classmates.
Spring Term
Typical course load is 12 credits
Health Information Standards & Interoperability (HINF 5020) - Required
Course Director: Jyoti Pathak, PhD 3 credits
In modern healthcare. exchange of clinical data across multiple stakeholders — between healthcare organizations, between providers and patients, and among agencies and governmental entities — is pivotal. Health information standards provide the “backbone” to achieve uniform data interoperability and exchange across multiple heterogeneous systems. This course will introduce existing and emerging clinical data modeling, terminology and knowledge representation standards.
Master’s Project 2 (HCPR 9020) - Required
Course Director: Faculty 3 credits
This is the culminating capstone course of all masters-level graduate education programs. It has two aims: (1) helping students to discover and develop new and effective ways of managing and working together with all the stakeholders within the healthcare field and (2) helping accelerate a student's development of the context awareness, integrative management, and industry skills that are needed to lead in a rapidly changing healthcare sector. This capstone course puts students in a new organization, one they don’t already know well, and gives them the chance to practice hitting the ground running. This culminating course provides a deeper preparation for the next stages of a student's career. The capstone project will last the entire year: the first term involves matching students with the right project, the second term has students working with their client, and the third term consists of a detailed report and final presentation.
Natural Language Processing (HINF 5016) - Elective
Course Director: Yiye Zhang, PhD 3 credits
This course introduces students to the field of natural language processing (NLP), applied to the health domain. NLP focuses on text data, which lacks the structure of conventional tabular data. In the health domain text is abundant in electronic health records, the medical literature and on the Web. Important applications of NLP include information extraction (pulling facts out of text) and information retrieval (searching through a collection of texts). The course presents methods for working with text: identifying the elements (words and symbols), recognizing sentence boundaries, parsing syntactic structures, assigning meaning, and establishing the structure of the discourse as a whole. The students build skills with these methods through laboratory work.
Artificial Intelligence in Medicine (HINF 5012) - Elective
Course Director: Fei Wang, PhD 3 credits
Introduces students to a variety of analytic methods for health data using computational tools. The course covers topics in data mining, machine learning, classification, clustering and prediction. Students engage in hands-on exercises using a popular collection of data mining algorithms.
Health Behavior and Consumer Informatics (HINF 5017) - Elective
Consumer health informatics (CHI) is the study of consumer information needs and technologies that provide consumers with the information they need to be more engaged in self-care and healthcare. This introductory CHI course will present an overview of theories of health and information behavior; key concepts and terminology; and main application domains. We will explore how health behavior theories 8 provide a framework for explaining consumers’ health behaviors and how CHI tools that are built with a theoretical foundation can promote health behavior change. The course will cover CHI applications in major application domains including electronic patient portals, mobile health (mHealth), and telehealth. Students will learn how to assess end-user needs and technological practices of potential users who experience health information and technological disparities. Students will also learn how to design for endusers, evaluate CHI applications and research.
Summer Term
Typical course load is 9 credits
Clinical Informatics (HINF 5011) - Required
Course Director: Sameer Malhotra, M.B.B.S., M.A. 3 credits
Prerequisites: Introduction to Health Informatics Clinical information systems such as electronic health records are central to modern healthcare. This course introduces students to the complex infrastructure of clinical information systems, technologies used to improve healthcare quality and safety (including clinical decision support and electronic ordering), and policies surrounding health information technology.
Data Management (SQL) (HINF 5018) - Required
Database systems are central to most organizations’ information systems strategies. At any organizational level, users can expect to have frequent contact with database systems. Therefore, skill in using such systems – understanding their capabilities and limitations, knowing how to access data directly or through technical specialists, knowing how to effectively use the information such systems can provide, and skills in designing new systems and related applications – is a distinct advantage and necessity today. The Relational Database Management System (RDBMS) is one type of database systems that are most often used in healthcare organizations and is the primary focus of this course. An overview of the non-relational database structure will also be given using Python programming language to provide a fuller picture of the current data management landscape. Further, to provide students with opportunities to apply the knowledge they learn from the lectures, various homework assignments, lab assignments, an exam, and a database implementation project will be given.
Master’s Project 3 (HCPR 9030) - Required
This is the culminating capstone course of all masters-level graduate education programs. It has two aims: (1) helping students to discover and develop new and effective ways of managing and working together with all the stakeholders within the healthcare field and (2) helping accelerate a student's development of the context awareness, integrative management, and industry skills that are needed to lead in a rapidly changing healthcare sector. This capstone course puts students in a new organization, one they don’t already know well, and gives them the chance to practice hitting the ground running. This culminating course provides a deeper preparation for the next stages of a student's career. The capstone project will last the entire year: the first term involves matching students with the right project, the second term has students working with their client, and the third term consists of a detailed report and final presentation in front of the client as well as faculty and fellow classmates.
Admissions Information
Academic Calendar
About Our Faculty
Request More Information
Population Health Sciences 402 E. 67th St. New York, NY 10065 Phone: (646) 962-8001
Student Spotlights from this Program
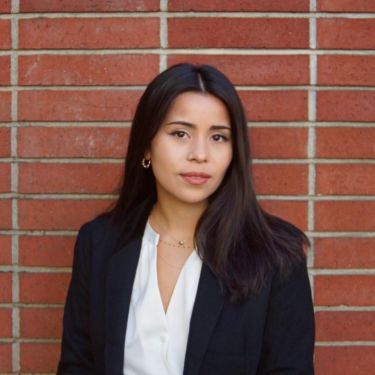
In 2020, Taylor Dang began medical school with plans to become a naturopathic physician. She was passionate about healthcare and was actively involved in political advocacy centered around health justice. However, as one of few students of color in her cohort, Taylor felt isolated at medical school. She made the call to withdraw and identify another path in healthcare. Taylor is now a graduate of the MS in Health Informatics program at Weill Co rnell Medicine (WC M ) , where she has found a way to pursue advocacy through the lens of healthcare systems and information.
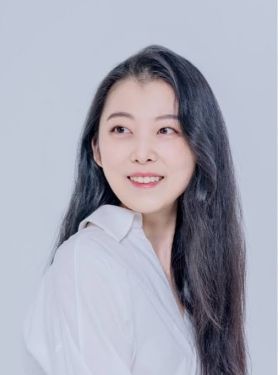
Dayoung Kim became interested in artificial intelligence (AI) while completing her undergraduate degree in computer science at the EWHA Womans University in South Korea. She worked in a bioinformatics and natural language processing (NLP) lab there, which shaped her decision to pursue higher education and further her knowledge of AI. She is now an alumna of the MS in Health Informatics program at Weill Cornell Medicine (WCM ) and works as a machine learning engineer at Boeing.
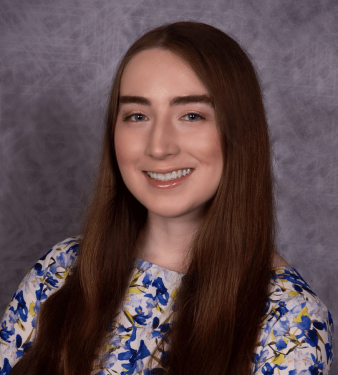
Maya discovered the MS in Health Informatics program while in Austria, where she taught English at a secondary school for two years. While there, she volunteered to tutor Syrian refugees in English, and at welcome centers to help Ukrainian refugees who fled the country when the war began. The experiences were jarring but propelled her to want to do more for others on a global scale.
View all Student Spotlights
Large Multidimensional Datasets inside a Database System (PhD Thesis)
- Kaushik Chakrabarti
January 2001
Many modern database applications deal with large amounts of multidimensional data. Examples include multimedia content-based retrieval (high dimensional multimedia feature data), time-series similarity retrieval, data mining/OLAP and spatial/spatio-temporal applications. To be able to handle multidimensional data efficiently, we need access methods (AMs) to selectively access some data items in a large collection associatively.
Traditional database AMs like B+-tree and hashing are not suitable for multidimensional data as they can handle only one dimensional data. Using multiple B+-trees (one per dimension) or space linearization followed by B+-tree indexing are not efficient solutions. We need multidimensional index structures: those that can index data based on multiple dimensions simultaneously. Most multidimensional index structures proposed so far do not scale beyond 10-15 dimensional spaces and are hence not suitable for high dimensional spaces that arise in modern database applications like multimedia retrieval (e.g., 64-d color histograms), data mining/OLAP (e.g., 52-d bank data in clustering) and time series/scientific/medical applications (e.g., 20-d Space Shuttle data, 64-d Electrocardiogram data). A simple sequential scan through the entire dataset to answer the query is often faster than using a multidimensional index structure.
To address the above need, we design and implement the hybrid tree, a multidimensional index structure that scales to high dimensional spaces. The hybrid tree combines the positive aspects of the two types of multidimensional index structures, namely data partitioning (e.g., R-tree and derivatives) and space partitioning (e.g., kdB-tree and derivatives), to achieve search performance more scalable to high dimensionalities than either of the above techniques. Our experiments show that the hybrid tree scales well to high dimensionalities for real-life datasets.
To achieve further scalability, we develop the local dimensionality reduction (LDR) technique to reduce the dimensionality of high dimensional data. The reduced space can be indexed more effectively using a multidimensional index structure. LDR exploits local, as opposed to global, correlations in the data and hence can reduce dimensionality with significantly lower loss of distance information compared to global dimensionality reduction techniques. This implies fewer false positives and hence significantly better search performance.
Another challenge in multidimensional indexing is handling time-series data which constitutes a major portion of all financial, medical and scientific information. We develop a new dimensionality reduction technique, called Adaptive Piecewise Constant Approximation (APCA), for time series data. APCA takes the idea of LDR one step further; it adapts locally to each time series object in the database and chooses the best reduced-representation for that object. We show how the APCA representation can be indexed using a multidimensional index structure. Our experiments show that APCA outperforms the other techniques by one to two orders of magnitude in terms of search performance.
Before multidimensional index structures can be supported as AMs in ”commercial-strength” database systems, efficient techniques to provide transactional access to data via the index structure must be developed. We develop concurrency control techniques for multidimensional index structures. Our solution, based on granular locking, offers a high degree of concurrency and has a low lock overhead.
An alternate technique to handle huge data volumes and fast search time requirements in multidimensional datasets is approximate query answering. This is especially true for decision support/OLAP applications where queries are usually exploratory in nature; fast approximate answers are often preferred to exact answers that take hours to compute. We develop a wavelet-based approximate query answering tool for DSS data. Our technique constructs compact synopses (comprising of wavelet coefficients) of the relevant database tables and subsequently answers any SQL query by working exclusively on the compact synopses. Our approach provides more accurate answers and faster response times compared to other approximate query answering techniques, namely random sampling and histograms, especially for high dimensional data.
Despite the increasing application need, commercial database management systems (DBMSs) lag far behind in their support for multidimensional data. One of the main reasons is the lack of scalable and effective techniques to manage large amounts of multidimensional data residing inside the DBMS. We believe that the techniques developed in this thesis address that problem. We hope that our solutions will encourage commercial database vendors to provide better support for multidimensional data in the future.
- Follow on Twitter
- Like on Facebook
- Follow on LinkedIn
- Subscribe on Youtube
- Follow on Instagram
- Subscribe to our RSS feed
Share this page:
- Share on Twitter
- Share on Facebook
- Share on LinkedIn
- Share on Reddit

Research Degrees
- The PhD Research Degree Programmes in Information Systems
Research Support
Entrance requirements, requirements for the award of the degree, the phd research degree programme in information systems.
The Doctor of Philosophy (PhD) Research Degree Programme in Information Systems admitted the first group of research students in 1990. It is now among the largest and the most extensive of its kind in the region, with more than 50 students currently enrolled on the programme.
This programme develops scholars who can draw on the concepts and methods of information technology, organizational behavior and strategy, and economics in conducting research on complex issues arising from the design, application and management of information systems in organizations. Particular emphasis is placed on the links between theory and practice and upon the practical application of innovative information systems methods and techniques to business, organizational, and societal problems. The research methodologies employed range from behavioral methods (both qualitative and quantitative), technical modelling to econometrics. Main areas of research include (1) Knowledge and Innovation Management; (2) Electronic/Mobile Commerce; (3) Online Social Networks; (4) IS Related Policy and Economic Analysis; (5) Healthcare; (6) Cyber Security; (7) Big Data; (8) Financial Information Services; (9) Artificial Intelligence; and (10) Technology Innovation and Platform Economy.
Research support is very good by international standards and there is a supportive and congenial environment in which research students pursue their scholarly work. The establishment of “Digital Innovation Lab” in early 2017 aims to collaborate, strengthen and elevate the applied research activities in the department. Departmental staff and research students are active in the international IS research community through their publications in leading IS journals and conferences, along with their participation on editorial boards and conference committees. Research students are supervised by an international team of experienced active researchers. There is also a visiting scholars’ programme through which prominent IS professors worldwide are invited to visit the Department to give seminars and advise on research.
Doctor of Philosophy (PhD)
Applicants seeking admission to PhD studies should:
- be a current MPhil student in the University who is seeking transfer to a PhD programme; or
- hold a higher research degree (or equivalent qualification) from a recognised university; or
- hold a Master’s degree (or equivalent qualification) from a recognised university; or
- hold a Bachelor’s degree with first class honours (or equivalent qualification) from a recognised university.
English Language Proficiency Requirements
Applicants whose first degree was obtained from an institution where the language of teaching is not English are expected to possess a minimum score of 85 in the Test of English as a Foreign Language (TOEFL) OR an overall band score of 6.5 in the International English Language Testing System (IELTS) to meet the English proficiency requirement.
The English requirement from CB website ( https://www.cb.cityu.edu.hk/phd/admission/ )
Applications are accepted throughout the year and will be considered in two rounds (January and April) annually. For detailed application procedures, please visit the home page of the Chow Yei Ching School of Graduate Studies. https://www.cityu.edu.hk/pg/research-degree-programmes
- Successfully completing prescribed coursework requirements;
- Presenting the results of research in a thesis which gives evidence of a sound understanding of the area of study, its context and applicability and makes a contribution to knowledge; and
- Passing an oral examination.
We use cookies to help our site work, to understand how it is used, and to tailor ads that are more relevant to you and your interests.
By accepting, you agree to cookies being stored on your device. You can view details and manage settings at any time on our cookies policy page.

Information and Communication Systems PhD
Key information, full-time - 4 years, part-time - 8 years.
Research brochure
Register for updates
Webinars and events
Why choose this programme
The University of Surrey’s Institute for Communication Systems (ICS), home of the 5G/6G Innovation Centre (5G/6GIC), is the one of the UK’s major academic research centres specialising in information and communications technology. You’ll be taught by leading experts in the field, become part of our thriving community of academics, researchers and PhD students, and gain excellent employment prospects.
We’re specialists in mobile and wireless technologies, including satellite communications and networks, internet of things, cyber security, and future network architectures and protocols. As home to the 5G/6GIC, we’re recognised as world leaders in end-to-end research and practical implementation for 5G/6G mobile communications and beyond.
Our strong track record in research includes high-quality publications, patents and world-class implementations. We’re heavily involved in collaborative research across the UK, Europe, Asia and the Americas, funded by governments and industry. Our PhD graduates secure good positions across industry and academia in the UK and worldwide.
Shanghai Ranking’s Academic Ranking of World Universities (ARWU) 2023 ranked our telecommunication engineering subjects as 51-75 best in the world.
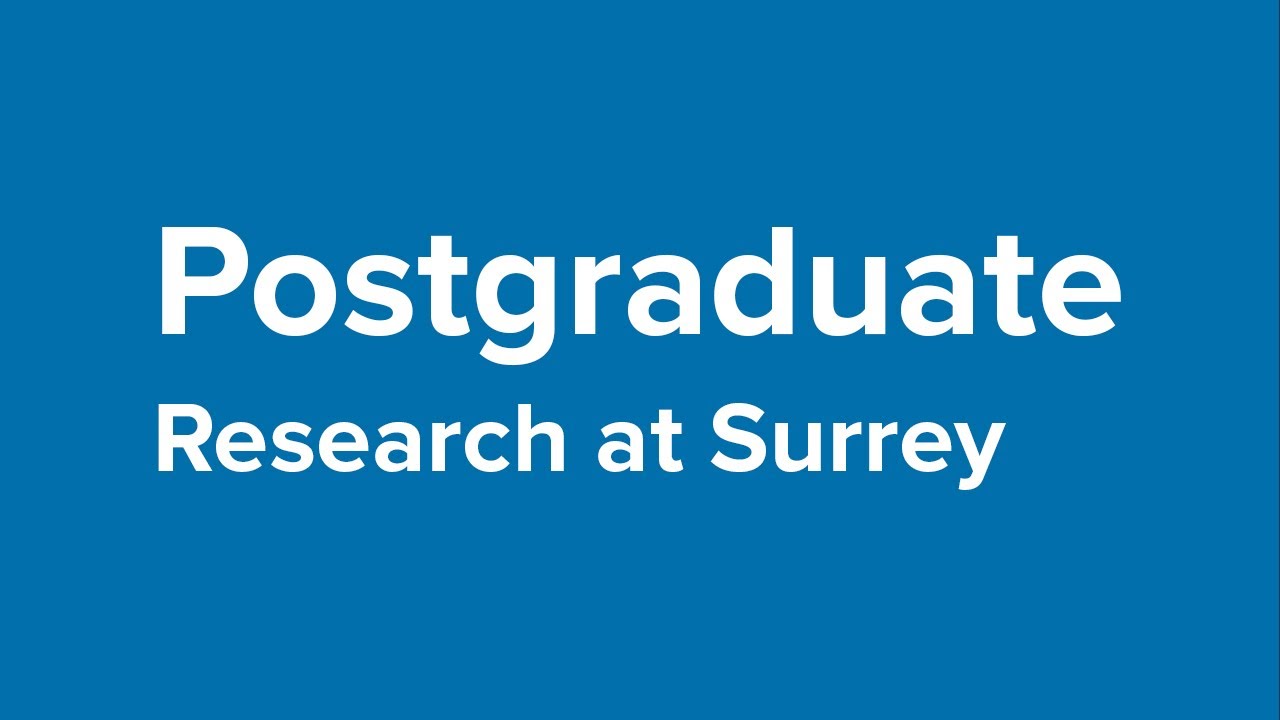
Frequently asked questions about doing a PhD
What you will study
It normally takes three to four years of full-time study to complete this PhD. Your research can be based on a topic of your choice, or may be dictated by industry sponsorship or government funding.
You’ll be assigned two supervisors, who’ll guide you through your studies. Your supervisors will give you direction and experience of the cutting-edge technologies in their areas of expertise. They’ll also give you the support you need to complete your PhD, from giving you guidance and feedback on your work to helping you frame your research proposal. Though you’ll primarily be in contact with your two supervisors, you’ll also work with other staff within ICS and you may have the opportunity to become a team member on research projects.
You’ll be required to write a confirmation report on your research in your first year, and you will be examined by two examiners.
Your final assessment will be based on the presentation of your research in a written thesis, which will be discussed in a viva examination with at least two examiners. You have the option of preparing your thesis as a monograph (one large volume in chapter form) or in publication format (including chapters written for publication), subject to the approval of your supervisors.
Research support
The professional development of postgraduate researchers is supported by the Doctoral College , which provides training in essential skills through its Researcher Development Programme of workshops, mentoring and coaching. A dedicated postgraduate careers and employability team will help you prepare for a successful career after the completion of your PhD.
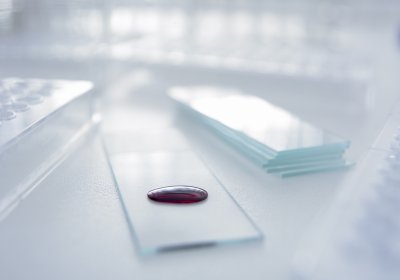
Research themes
We research areas related to information and communication technology, including end-to-end terrestrial, mobile and satellite communications and networks, and their application to verticals such as connected vehicles and health, including:
- 5G-Advanced and 6G
- Integrated satellite and terrestrial 5G and 6G
- Artificial intelligence networking and wireless communications
- Reflective and transmissive intelligent surfaces
- THz communications and components
- Positioning and image sensing
- High-quality time and frequency transmission
- Teleportation
- Connected transportation
- Ultra-Massive MIMO
- Terabits-per-second communications
- Internet of senses
- Network and physical layer security
- Intelligent and high-performance networking and service delivery
- MAC, RRM and RAN management
- Theory and practice of advanced concepts in wireless communications
- Antennas and signal processing.
Our academic staff
See a full list of all our academic staff within the Institute for Communication Systems.
Research centres and groups
Research facilities.
You’ll be allocated space in ICS, which is home to 143 researchers. ICS is one of the world’s major academic research centres dedicated to mobile and wireless communications. You’ll have access to state-of-the-art facilities such as:
- Campus-wide 5G/6G testbed
- Antennas and signal processing
- Networks lab testbed
- Link and system level simulators
- Satellite networking testbed
- Security testbed
- Wireless network testbed
- Internet of things testbed.
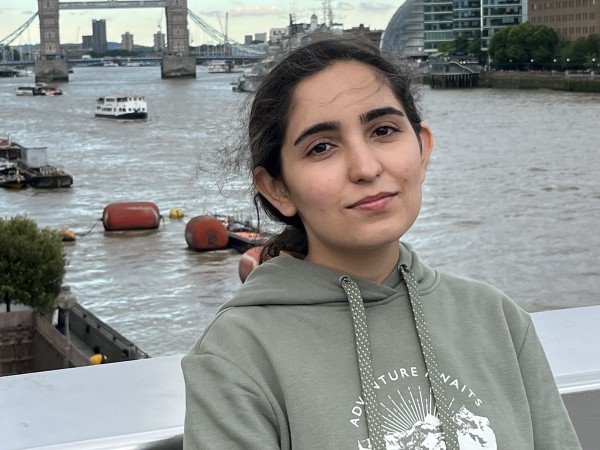
Melika Emami
Having an experienced supervisor in my research area has been invaluable to me throughout my PhD journey... their unwavering support and encouragement have significantly boosted my self-confidence, especially in times when I needed it the most.
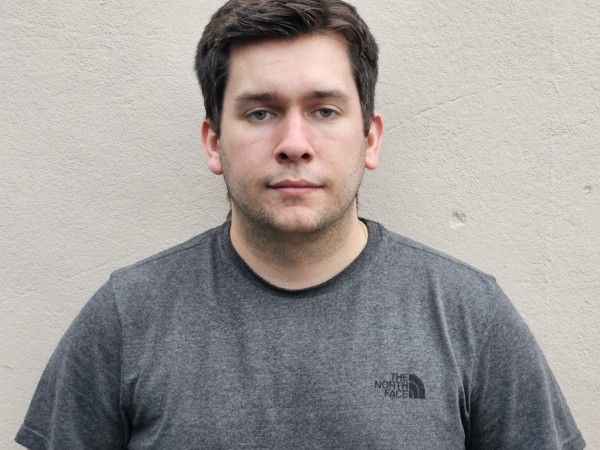
Ryan Fernandez
I knew Surrey would be a good place to do a PhD as the Institute for Communication Systems is a world-leading research centre with an outstanding reputation.
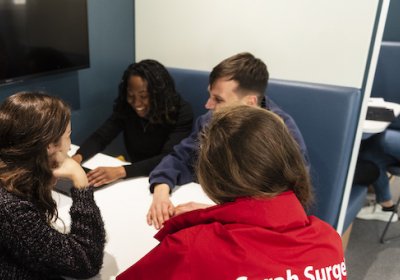
Entry requirements
Applicants are expected to hold a first or upper second-class (2:1) UK degree in a relevant discipline (or equivalent overseas qualification), or a lower-second (2:2) UK degree plus a good UK masters degree - distinction normally required (or equivalent overseas qualification).
International entry requirements by country
English language requirements.
IELTS Academic: 6.5 or above (or equivalent) with 6.0 in each individual category.
These are the English language qualifications and levels that we can accept.
If you do not currently meet the level required for your programme, we offer intensive pre-sessional English language courses , designed to take you to the level of English ability and skill required for your studies here.
Application requirements
Applicants are advised to contact potential supervisors before they submit an application via the website. Please refer to section two of our application guidance .
After registration
Students are initially registered for a PhD with probationary status and, subject to satisfactory progress, subsequently confirmed as having PhD status.
Selection process
Selection is based on applicants:
- Meeting the expected entry requirements
- Being shortlisted through the application screening process
- Completing a successful interview
- Providing suitable references.
Student life
At Surrey we offer the best of both worlds – a friendly campus university, set in beautiful countryside with the convenience and social life of Guildford on your doorstep.
Start date: July 2024
Start date: October 2024
Start date: January 2025
Start date: April 2025
- Annual fees will increase by 4% for each year of study, rounded up to the nearest £100 (subject to legal requirements).
- Any start date other than September will attract a pro-rata fee for that year of entry (75 per cent for January, 50 per cent for April and 25 per cent for July).
View the list of fees for all postgraduate research courses.
Additional costs
There are additional costs that you can expect to incur when studying at Surrey.
A Postgraduate Doctoral Loan can help with course fees and living costs while you study a postgraduate doctoral course.
Apply online
If you are applying for a studentship to work on a particular project, please provide details of the project instead of a research proposal.
Read our application guidance for further information on applying.
To apply online first select the course you'd like to apply for then log in.
1. Select your course
Select the course you wish to apply for.
To apply online sign in or create an account.
Code of practice for research degrees
Surrey’s postgraduate research code of practice sets out the University's policy and procedural framework relating to research degrees. The code defines a set of standard procedures and specific responsibilities covering the academic supervision, administration and assessment of research degrees for all faculties within the University.
Download the code of practice for research degrees (PDF) .
Terms and conditions
When you accept an offer to study at the University of Surrey, you are agreeing to follow our policies and procedures , student regulations , and terms and conditions .
We provide these terms and conditions in two stages:
- First when we make an offer.
- Second when students accept their offer and register to study with us (registration terms and conditions will vary depending on your course and academic year).
View our generic registration terms and conditions (PDF) for the 2023/24 academic year, as a guide on what to expect.
This online prospectus has been published in advance of the academic year to which it applies.
Whilst we have done everything possible to ensure this information is accurate, some changes may happen between publishing and the start of the course.
It is important to check this website for any updates before you apply for a course with us. Read our full disclaimer .
Course location and contact details
Campus location
Stag Hill is the University's main campus and where the majority of our courses are taught.
University of Surrey Admissions
University of Surrey Guildford Surrey GU2 7XH
- Criminal Justice Degrees
- Business Degrees
- Nursing Degrees
- Technology Degrees
- Education Teaching Degrees
- Crime Scene Degrees
- Accounting Degrees
- RN to BSN Degrees
- Degrees in Nutrition
- Computer Science Degrees
- Culinary Arts Degrees
- Bachelors Programs
- Masters Programs
- Online MBA Programs
- Online Certificate Programs
- Associate Programs
- Doctoral Programs
- Bachelor in Accounting
- Criminal Justice Masters
- Finance MBA
- Finance PhD
- Nursing Certificate
- Capella University
- Walden University
- University of Southern California
- Nursing Schools in Texas
- Nursing Schools in California
- Photography Schools in California
- Law Schools in New York
- Medical Schools in North Carolina
- CRNA Schools in Texas
- How to Become a CIA Agent
- How to Become a Corrections Officer
- How to Become a Crime Scene Investigator
- How to Become a Forensic Scientist
- How to Become a Lawyer
- How to Become a Legal Secretary
- How to Become a Nurse Anesthetist
- How to Become a Nurse Practitioner
- How to Become a Paralegal
- How to Become a Police Officer
- How to Become a Private Investigator
- Financial Aid
- Accounting Schools
- Business Administration Courses
- Pharmacist Schools
- Nursing School
- Popular Law Related Searches
- Ten of The Most Enrolled Masters Majors
- Single Moms Guide to Online Education
- Bachelor in Information Systems
- Master in Information Systems
- Associate in Information Systems
- Certificate in Information Systems
- Degree Completion in Information Systems
- Post Bacc Programs in Information Systems
- Careers in Computer Science
- Careers in Information Technology
- Careers in Information Systems
- Careers in Networking
- Careers in Telecommunications
Doctoral in Information Systems
Featured schools.
What is a doctoral of information systems? A doctoral degree of information systems is the highest degree awarded in this field, and includes a specially designed four-year program comprising of two years of academic studies followed by two years of research work. Most PhD candidates hold full time jobs, so the degree program is designed in an executive fashion with classes scheduled for the evenings and weekends. Subjects include information system development, database design and management, information systems integration, telecommunication methods and research techniques. Once the doctoral candidates have completed their research, they participate in a public defense where they justify the methods and techniques employed during their research. What are the future prospects? The field of information systems is one of the fastest growing in the world. Within the United States, this field is expected to grow exponentially due to the increasing digitalization in various businesses and government agencies. The Bureau of Labor Statistics estimates an increase of 17% in employment opportunities with manufacturing companies, financial firms and healthcare services being the leading employers. Average wages in this field currently stand at $110,000 for new PhDs while experienced professionals make almost $200,000 per year.
Q: What are the admission requirements for doctoral in information systems?
A: Individuals who wish to enroll in a doctoral degree in information technology must fulfill the admission requirements of the university. These requirements may include the following: students must have completed a graduate level program with minimum 2.5- 3.0 CGPA, student must have at least 3 letters of recommendations, a portfolio of all research based work must be submitted (if any), students must have a satisfactory SAT score and entry test score, foreign students must have competed a TOEFL course and should be residing within the US.
Q: I wish to enroll in the best phd computing and information system in USA. Can you tell me what I need?
A: PhD programs in computing and information systems are the highest level of education you can go for. The program typically has a duration between 5 to 7 years. You must have a bachelor and masters degree to qualify for admission. Other requirements for admission will vary from institute to institute. Browse through our page for more information.
Ask your Question
- { expandedNavigation=true; activeIndex=0; }"> Research landscape
- { expandedNavigation=true; activeIndex=1; }"> Your goal
- { expandedNavigation=true; activeIndex=2; }"> Plan your stay
- { expandedNavigation=true; activeIndex=3; }"> Success stories
- { expandedNavigation=true; activeIndex=4; }"> Our service
- R&D policy framework
- Research infrastructure
- Research funding system
- Universities
- Universities of applied sciences
- Technical universities
- Top universities
- Fraunhofer-Gesellschaft
- Helmholtz Association
- Leibniz Association
- Max-Planck-Gesellschaft
- Academies of sciences and humanities
- Federal institutions
- State research institutions
- What is R&D in German business?
- Why is collaboration important?
- Which sectors carry out R&D?
- Which are the leading companies?
- How do German businesses compare internationally?
- How is the start-up scene set up?
- How do I start a career?
- Good reasons
- Two ways to get your PhD
- Find your PhD position
How to apply for a PhD
- Funding programmes
- Funding organisations
- Funding databases
- Job portals
- Career options & dual careers
- Funding & awards
- Potential employers
- Research fields
- Entry and residence
- German money-saving tips
- Cost of living
- Social insurance and health
- Bringing your family
- Information for your partner
- Support for families
- Finding a place to live
- Funding opportunities
- Recognition of professional qualifications
- Counselling
- Latest Thinking
- First-hand experiences from international researchers
- On-site consultation
- Our publications
- Research news
- Online talks
- Topics in focus
Find your PhD position in Germany
Before you start your search ....
Before you start your search you should know that there are different PhD models:
- Individual doctorate or
- Structured PhD programmes
What's the difference? Check out our overview of the various ways to do your PhD in Germany
Find your individual doctorate

The "traditional" or "individual" path to a PhD remains the most common in Germany. An individual doctorate involves a thesis or dissertation that is produced under the supervision of one professor . This form of PhD study offers a great deal of flexibility , but demands a high degree of personal initiative and responsibility.
How to find your PhD supervisor
In Germany there is no central admissions or selection office for doctoral students. Therefore, your first step is to find a suitable professor who is willing to be your supervisor.
One way to find a supervisor is to look for a university institute that matches your area of research. The following online search engines might help you find a suitable supervisor:
- GERiT – German research institutions GERiT is a website containing information on approximately 29,000 research institutions in Germany. GERiT allows the user to search easily by location or subject. It provides all the information needed to choose an institution at which to research, study or do a doctorate. www.gerit.org
- Finding a PhD position PhDGermany publishes PhD openings in Germany that specifically target international applicants. Accordingly, in most cases the working language is English. Fluent knowledge of German is only required for certain special positions. PhDGermany helps you find the right PhD opening or supervisor for your doctoral thesis and assists you with the online application process. www.phdgermany.de
- Higher Education Compass This database provides up-to-date information from universities about doctoral opportunities in Germany. The search engine enables you to carry out targeted searches on the basis of departments, admission requirements and form of doctoral thesis. www.higher-education-compass.de
Furthermore, your contacts with your professors or previous university could help direct you to a suitable department or potential supervisor in Germany.
It is also helpful to attend academic conferences in your own subject area. There you will be able to exchange information and make contacts – and perhaps even find a future PhD supervisor.
Find your structured PhD programme

DAAD/Ausserhofer/Himsel
Structured PhD programmes in Germany are frequently very similar to the PhD programmes in English-speaking countries, in which a team of supervisors look after a group of doctoral students . Around 12,000 doctoral students from abroad – roughly one in four – do their PhDs in structured programmes. As a rule, it is possible to complete a doctorate in four to five years.
Where to find your PhD programme
There is no central database of all structured PhD programmes in Germany. You can usually find these programmes directly through the respective universities, graduate schools or non-university research institutions. The German Academic Exchange Service (DAAD) database is also a good place to look. Here you will find a large number of PhD programmes that are specially aimed at international doctoral students.
International doctoral programme database
Are you interested in an international doctoral programme in Germany? This DAAD database presents a selection of roughly 230 international doctoral programmes in Germany. The database can be searched according to different criteria. www.daad.de/international-programmes
Doctoral programmes at universities
Many universities offer structured doctoral programmes, which they publicise on their websites. The Student Advisory Service or Graduate Centre at the respective university will also provide help here. You can find the relevant addresses using the Higher Education Compass provided by the German Rectors’ Conference. www.higher-education-compass.de
DFG-funded research training groups
Research training groups are also funded by the Deutsche Forschungsgemeinschaft (German Research Foundation, DFG) for a period of up to nine years. Their key emphasis is on the qualification of doctoral researchers within the framework of a focused research programme and a structured training strategy. www.dfg.de > Current Research Training Groups
Helmholtz Research Schools, Colleges and Graduate Schools
The Helmholtz Association is Germany’s largest scientific organisation. In collaboration with various institutions of higher education, Helmholtz Association research centres have established structured PhD programmes under the auspices of Helmholtz Graduate Schools, Helmholtz Research Schools and Colleges. www.helmholtz.de > PhD Candidates
Leibniz Graduate Schools
The Leibniz Association connects 97 research institutes that conduct problem-oriented research and provide scientific infrastructure of national and international importance. Together with universities they run structured PhD programmes in Leibniz Graduate Schools. www.leibniz-association.eu > Leibniz Graduate Schools
International Max Planck Research Schools
The Max Planck Society specialises in innovative basic research and its institutes are able to offer up-and-coming researchers excellent infrastructure and support. The website lists the programmes available at International Max Planck Research Schools (IMPRS): www.mpg.de > International Max Planck Research Schools
Max Planck Schools
In Germany, the best researchers in a specific field are often work at different universities and non-university research institutions spread throughout the country. The Max Planck Schools serve as hubs which gather this distributed knowledge. Here, the brightest minds in their fields have come together from within the scientific community to interconnect in faculties made up of active researchers. Students gain access to these unique networks, learn in close personal exchange from leaders in their fields and their peers, and enjoy access to outstanding infrastructure. Currently, three Schools are operating in the fields of Cognition, Matter to Life, and Photonics. www.maxplanckschools.de
Where can I find out about requirements?
Application procedures differ from programme to programme . The precise requirements and deadlines can be found on the website of the respective university, research training group or graduate school. You should therefore first choose a PhD programme and/or graduate school.
You've found the position you want to apply for, but how does applying to a potential supervisor or structured PhD programme work in Germany? Find out more here.
DAAD/Jan Zappner
We help you navigate through the large number of job portals that specialise in openings for academics and scientists. These are some of the sites that may get you started.
DAAD/Uta Konopka
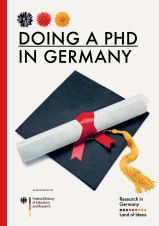
Check out our brochure
Doing a phd in germany (2019, 40 pages).
This booklet for (prospective) international doctoral students presents the different options for doing a doctorate in Germany. It explains the formal requirements and gives some practical advice on finding the right supervisor or doctoral programme. It also outlines different sponsorship and funding options.
- Press Enter to activate screen reader mode.
Finding a Doctoral Position
How do i find a place as a doctoral student.
Unlike at many other universities, the doctorate at ETH Zurich is not organised via an overarching doctoral school. ETH Zurich mainly offers individual doctorates. Each potential doctoral thesis supervisor recruits their doctoral students themselves. In order to be able to complete a doctorate at ETH, a professor at ETH must be willing to supervise your dissertation. With few exceptions, doctorates are linked to a position at ETH or within the ETH-domain. The best way to apply for a doctoral position is to contact the professorship you are interested in directly. To do so, visit the website of the department where you would like to do your doctorate and contact the professors you are considering directly.
- chevron_right ETH departments
- chevron_right Doctoral study programmes
However, doctoral posts are also advertised:
- chevron_right Current vacancies at ETH Zurich
- external page call_made ETH get hired
Helpful information on the topic of applying for a doctorate at ETH is also available in the AVETH survival guide . In any case, please note that the Doctoral Administration does not arrange doctoral positions or mediates between candidates and doctoral thesis supervisors.
Are you already registered as a doctoral student at ETH? See here for further information .
> Contact the Doctoral Administration
Browse Course Material
Course info, instructors.
- Prof. Joseph Ferreira
- Prof. Lorlene Hoyt
Departments
- Urban Studies and Planning
As Taught In
- Information Technology
- Data Mining
- Graphics and Visualization
- Community Development
- Urban Planning
Learning Resource Types
Spatial database management and advanced geographic information systems, course meeting times.
Lectures: 2 sessions / week, 1.5 hours / session
Description
This semester long subject (11.521) is divided into two halves. The first half focuses on learning spatial database management techniques and methods and the second half focuses on using these skills to address a ‘real world,’ client-oriented planning problem. The first half of the semester may be taken separately using the class number 11.523 and the second half may be taken separately as 11.524.
In order to help shape and utilize the information infrastructure that will support the management and development of our metropolitan areas, planners need a basic understanding of the tools and technology for querying, analyzing, and sharing complex databases and maps. Managing online access to large and constantly-changing spatial datasets can be a powerful aid to planning and can facilitate inter-agency cooperation and collaboration in an increasingly decentralized world. But it requires the use of knowledge representation methods, client-server technologies and access control issues that are quite different from what are needed to model and visualize standalone datasets on a personal computer. Hence, planners should acquire basic skills in database management, digital spatial data analysis, and networking.
The 11.523 portion of the semester addresses these issues while retaining a focus on planning (rather than on computer science). This is an intensive, hands-on class that stresses learning by doing. Exercises and examples involving real-world data, maps, and images are used to develop skills with database query languages and the design development and use of structured databases. Class work utilizes web tools, GIS, and database software with lab exercises. Specifically, we will access an Oracle 8i database using SQL (structured query language) and use ArcView for GIS. Each week there are two sixty to ninety-minute classes plus another 90+ minute hands-on lab in an electronic classroom. Class lectures will focus on concepts and case discussion, the scheduled lab time focuses on computer mechanics and skill building. Specific topics during 11.523 include:
- finding, understanding and structuring digital spatial data that are available on the Internet using various browsing, visualization, and data management tools;
- considerable work with relational database technologies and the Structured Query Language (SQL) to design, construct, query, and update urban planning databases;
- some experience with so-called ‘client/server’ and ’enterprise GIS’ technologies for facilitating distributed access to complex spatial data and urban planning applications;
- advanced GIS topics such as 3D visualizations and geospatial web services.
The 11.524 portion of the semester will treat the classroom like a professional planning office, working as a team to produce a two deliverables for their client, Lawrence Community Works, Inc. (LCW), a community development corporation located in the City of Lawrence, Massachusetts. LCW and DUSP recently agreed to work together for the next five years to design and implement a multi-tier web-based planning system that promotes democratic involvement and informs community development projects. Your involvement this semester is critical, because the implementation plan that you craft this semester will serve as the road map for both organizations for years to come and the simple web-based planning tool that you design will engage stakeholders by giving them a better sense of how technologies can aid decision-making processes. Through their project work, students will enhance important professional skills by:
- formulating an implementation plan for a real client;
- designing a simple web-based tool for understanding problems;
- engaging constituents and stakeholders in a real setting;
- integrating theory and practice by evaluating the role of technology in community development;
- learning to communicate effectively within a group and with a professional consultant;
- working with such tools as the WWW, Access, ArcView, ArcIMS, SDE, etc.
11.521 versus 11.523 or 11.524
- The subject 11.521 = 11.523 + 11.524 and you can register for 11.521 or (11.523 plus 11.524) or either one of the half semester modules.
- The subject 11.521 is a full-semester class that earns 12 units ( 3-3-6) of H-level graduate credit
- The subject 11.523 includes classes 1-13 of 11.521 (plus labs) and earns 6 units (2-2-2) of H-level graduate credit. This subject covers the spatial database portion of 11.521
- The subject 11.524 includes classes 14-26 of 11.521 (plus labs) and earns units to be arranged (generally 1-1-4 unless you are taking 11.524 a second time). This subject covers the advanced GIS project portion of 11.521
- The subject 11.521 cannot be taken for credit with 11.523 or 11.524 in the same semester; the subject 11.524 can be taken again in another term. Students who have not decided in which class to enroll should sign up for both 11.521 and 11.523, then drop one of the subjects by the drop date.
Prerequisites
The prerequisite for this class is
- 11.204 : Planning, Communications and Digital Media,
- 11.208 : Introduction to Computers in Public Management II or
- 11.520 : A Workshop on Geographic Information Systems, and
(b) an understanding of analytic methods that most undergraduates acquire via general Institute requirements and MCP students obtain from course from
- 11.220 : Quantitative Reasoning and Statistical Analysis I (which may be taken concurrently).
Requirements
Exercises, class discussions, and projects use real databases and problems taken from current Planning Support Systems (PSS), involving local and regional planning agencies. These data include parcel-level maps, tabular data, and digital orthophotos for all of Boston; land use, wetland, and other environmental planning datasets for the state; and detailed information about the neighborhoods being studied for the project.
The full course includes nine lab exercises; 11.523 students complete the first six labs, and 11.524 students do the last three. Each lab includes an assignment to be turned in. To facilitate a quick turnaround on grading, these assignments will be evaluated on a three-point scale: check-minus, check, check-plus. Students in 11.521 and 11.523 will complete three homework sets and have an examination before Spring Break. Students in 11.521 and 11.524 will complete a half-semester group project during the second half of the term that provides an opportunity to apply GIS and database concepts in a more realistic context. The project concludes with an oral presentation to the client and a written report. In addition to preparing paper maps of various sizes, each team will build a web site to showcase its work.
**Grade is average of 11.523 and 11.524 grades
- 6 Lab Exercises (collectively)………….15%
- 3 Problem Sets (collectively)…………..45%
- Examination…………………………………35%
- Class Participation………………………….5%
- Lab 7………………………………………………………………………….10%
- Lab 8………………………………………………………………………….15%
- Lab 9………………………………………………………………………….15%
- Internal and On-Site Project Presentations………………………10%
- Final Project Part one: Implementation…………………………….25%
- Final Project Part Two: Web-based Planning Application…….25%
Lateness Policy
Turning in assignments promptly is important both for keeping current with the subject matter, which is cumulative, and to keep all students on a level playing field. Hence, we have adopted a strict policy towards credit for assignment that is turned in late.
Lab exercises are typically due one week after the lab is run. A late lab exercise will be accepted up until one week after the original due date for a loss of one grade (e.g., a “check” becomes a “check-minus”). After that, late assignments will receive no credit and will not be accepted.
Late problem sets will have two points deducted for each day (including weekends and holidays) after the due date. Hence, a problem set turned in three days late would lose 6 points. If it would have earned 90 points if turned in on time, it would receive only 84 points under these conditions. Regardless, no problem sets will be accepted after the answers have been posted, typically two weeks after the initial due date.

You are leaving MIT OpenCourseWare
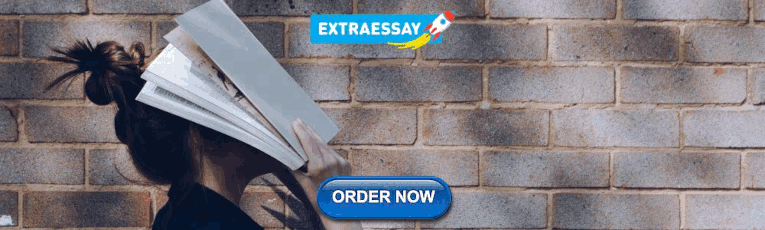
IMAGES
VIDEO
COMMENTS
A PhD in Analytics and Data Science or a PhD in Data Science, Analytics & Engineering is a way for universities to combine data expertise from multiple departments. Yes, advanced analytics & big data processes will be addressed in the curriculum. But you'll also find a strong emphasis on programming, algorithm creation, and systems development.
Step 1: Finalize School and Program Choice. Although there are a growing number of online programs, Ph.D. programs are still primarily an onsite experience for the sciences, technology, engineering, and math (STEM) disciplines. So, most Ph.D. applicants will need to take the university location into consideration along with the availability of ...
It costs, on average, $19,314 per year to get a PhD in Data Science, according to the National Center of Education Statistics. The cost will change depending on the type of school a student attends. If a doctoral student studies at a public university, the tuition is only $12,171, on average.
The School of Information offers four degrees: The Master of Information Management and Systems (MIMS) program educates information professionals to provide leadership for an information-driven world.. The Master of Information and Data Science (MIDS) is an online degree preparing data science professionals to solve real-world problems. The 5th Year MIDS program is a streamlined path to a MIDS ...
The PhD in Information Systems seeks to train future researchers to analyze and understand the multifaceted impact of information systems and technologies on individuals, organizations and society. Students consider the positive and negative consequences of information systems as well as the moral and ethical dilemmas introduced in societies coordinated by and highly dependent upon information ...
An online PhD program is an accessible way to reach the highest levels of educational attainment. ... A 72-credit Ph.D. in systems and engineering management uses a hybrid format to cultivate experts ready to lead in industry, academia, and consulting. ... Prospective students can consult program websites or the Database of Accredited ...
Ist 506 Database systems and Data Analysis (3) Inf 507 Modern Issues in Databases (3) Csi 508 Database Systems I (3) ... The INF PhD program requires each student to meet qualifying requirements, demonstrating foundational knowledge in the core areas of Information Science. The qualifying requirement includes completing each of the four core ...
Course Director: Yiye Zhang, PhD 3 credits. Database systems are central to most organizations' information systems strategies. At any organizational level, users can expect to have frequent contact with database systems. Therefore, skill in using such systems - understanding their capabilities and limitations, knowing how to access data ...
Large Multidimensional Datasets inside a Database System (PhD Thesis) Many modern database applications deal with large amounts of multidimensional data. Examples include multimedia content-based retrieval (high dimensional multimedia feature data), time-series similarity retrieval, data mining/OLAP and spatial/spatio-temporal applications.
Support. Students need three types of support to be successful in a Ph.D. program. The first type of support is structural. Students are provided with a work area, a personal computer, limited teaching responsibilities, and sufficient financial support. Financial Information. The second type of support is research skill development.
The Doctor of Philosophy (PhD) Research Degree Programme in Information Systems admitted the first group of research students in 1990. It is now among the largest and the most extensive of its kind in the region, with more than 50 students currently enrolled on the programme. This programme develops scholars who can draw on the concepts and ...
The University of Surrey's Institute for Communication Systems (ICS), home of the 5G/6G Innovation Centre (5G/6GIC), is the one of the UK's major academic research centres specialising in information and communications technology. You'll be taught by leading experts in the field, become part of our thriving community of academics, researchers and PhD students, and gain excellent ...
Most PhD candidates hold full time jobs, so the degree program is designed in an executive fashion with classes scheduled for the evenings and weekends. Subjects include information system development, database design and management, information systems integration, telecommunication methods and research techniques.
Over the last 80 years, ProQuest has built the world's most comprehensive and renowned dissertations program. ProQuest Dissertations & Theses Global (PQDT Global), continues to grow its repository of 5 million graduate works each year, thanks to the continued contribution from the world's universities, creating an ever-growing resource of emerging research to fuel innovation and new insights.
Required Education: Employers increasingly prefer candidates with a master's degree in data science or a directly related field, such as database management, computer science, or mathematics. 2022 Median Annual Salary: $103,500. Job Outlook (2022-32): +35%. Explore more computer science careers.
Forecasting refers to the estimation of val- ues of a time series at future points in time, so called forecast values or short forecasts. Hereby, the forecast horizon describes the interval from the next point in time up to a given future point in time. A forecasting method is a procedure for computing forecasts from present and past values.
This opportunity is open to PhD students who attend The State University of Campinas (UNICAMP). The internship will take place from February - May and is a remote position. ... Familiarity with the internals and use of caching systems, key-value stores, transactional database systems, and/or storage engines ; Familiarity with the systems and ...
Where to find your PhD programme. There is no central database of all structured PhD programmes in Germany. You can usually find these programmes directly through the respective universities, graduate schools or non-university research institutions. The German Academic Exchange Service (DAAD) database is also a good place to look.
PhD IT is a research-based doctoral course of normally 3 years duration, but may be extended upto 5 years. ... It includes Data Analysis, Database Management, Operating Systems, etc. PhD Computer Science includes all the aspects of Computer Science including Information Technology and Data Science. Along with that, it also includes Computer ...
With few exceptions, doctorates are linked to a position at ETH or within the ETH- domain. The best way to apply for a doctoral position is to contact the professorship you are interested in directly. To do so, visit the website of the department where you would like to do your doctorate and con ...
database systems at the junior, senior, or graduate level, and as a reference book. Our goal is to provide an in-depth and up-to-date presentation of the most important aspects of database systems and applications, and related technologies. We assume that readers are familiar with elementary programming and data-structuring con-
FUTOJNLS 2020 VOLUME- 6, ISSUE- 1. PP- 78 - 89. This paper reports the development of a Database Management System to catalogue. students ' projects thereby eliminating the manual system of ...
The first half focuses on learning spatial database management techniques and methods and the second half focuses on using these skills to address a 'real world,' client-oriented planning problem. ... DUSP recently agreed to work together for the next five years to design and implement a multi-tier web-based planning system that promotes ...