Thank you for visiting nature.com. You are using a browser version with limited support for CSS. To obtain the best experience, we recommend you use a more up to date browser (or turn off compatibility mode in Internet Explorer). In the meantime, to ensure continued support, we are displaying the site without styles and JavaScript.
- View all journals
- My Account Login
- Explore content
- About the journal
- Publish with us
- Sign up for alerts
- Review Article
- Open access
- Published: 08 June 2021
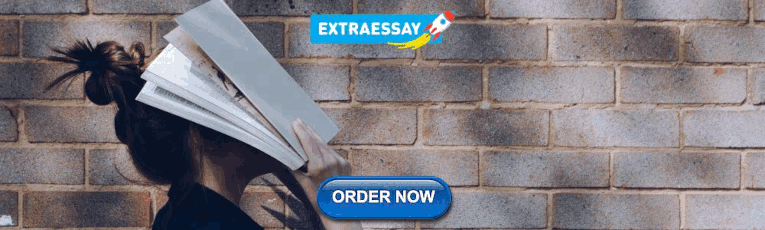
Metacognition: ideas and insights from neuro- and educational sciences
- Damien S. Fleur ORCID: orcid.org/0000-0003-4836-5255 1 , 2 ,
- Bert Bredeweg ORCID: orcid.org/0000-0002-5281-2786 1 , 3 &
- Wouter van den Bos 2 , 4
npj Science of Learning volume 6 , Article number: 13 ( 2021 ) Cite this article
35k Accesses
44 Citations
11 Altmetric
Metrics details
- Human behaviour
- Interdisciplinary studies
Metacognition comprises both the ability to be aware of one’s cognitive processes (metacognitive knowledge) and to regulate them (metacognitive control). Research in educational sciences has amassed a large body of evidence on the importance of metacognition in learning and academic achievement. More recently, metacognition has been studied from experimental and cognitive neuroscience perspectives. This research has started to identify brain regions that encode metacognitive processes. However, the educational and neuroscience disciplines have largely developed separately with little exchange and communication. In this article, we review the literature on metacognition in educational and cognitive neuroscience and identify entry points for synthesis. We argue that to improve our understanding of metacognition, future research needs to (i) investigate the degree to which different protocols relate to the similar or different metacognitive constructs and processes, (ii) implement experiments to identify neural substrates necessary for metacognition based on protocols used in educational sciences, (iii) study the effects of training metacognitive knowledge in the brain, and (iv) perform developmental research in the metacognitive brain and compare it with the existing developmental literature from educational sciences regarding the domain-generality of metacognition.
Similar content being viewed by others
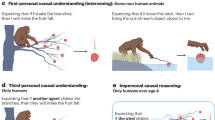
The development of human causal learning and reasoning
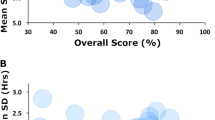
Sleep quality, duration, and consistency are associated with better academic performance in college students
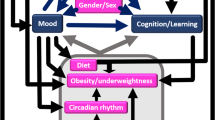
Effect of sleep and mood on academic performance—at interface of physiology, psychology, and education
Introduction.
Metacognition is defined as “thinking about thinking” or the ability to monitor and control one’s cognitive processes 1 and plays an important role in learning and education 2 , 3 , 4 . For instance, high performers tend to present better metacognitive abilities (especially control) than low performers in diverse educational activities 5 , 6 , 7 , 8 , 9 . Recently, there has been a lot of progress in studying the neural mechanisms of metacognition 10 , 11 , yet it is unclear at this point how these results may inform educational sciences or interventions. Given the potential benefits of metacognition, it is important to get a better understanding of how metacognition works and of how training can be useful.
The interest in bridging cognitive neuroscience and educational practices has increased in the past two decades, spanning a large number of studies grouped under the umbrella term of educational neuroscience 12 , 13 , 14 . With it, researchers have brought forward issues that are viewed as critical for the discipline to improve education. Recurring issues that may impede the relevance of neural insights for educational practices concern external validity 15 , 16 , theoretical discrepancies 17 and differences in terms of the domains of (meta)cognition operationalised (specific or general) 15 . This is important because, in recent years, brain research is starting to orient itself towards training metacognitive abilities that would translate into real-life benefits. However, direct links between metacognition in the brain and metacognition in domains such as education have still to be made. As for educational sciences, a large body of literature on metacognitive training is available, yet we still need clear insights about what works and why. While studies suggest that training metacognitive abilities results in higher academic achievement 18 , other interventions show mixed results 19 , 20 . Moreover, little is known about the long-term effects of, or transfer effects, of these interventions. A better understanding of the cognitive processes involved in metacognition and how they are expressed in the brain may provide insights in these regards.
Within cognitive neuroscience, there has been a long tradition of studying executive functions (EF), which are closely related to metacognitive processes 21 . Similar to metacognition, EF shows a positive relationship with learning at school. For instance, performance in laboratory tasks involving error monitoring, inhibition and working memory (i.e. processes that monitor and regulate cognition) are associated with academic achievement in pre-school children 22 . More recently, researchers have studied metacognition in terms of introspective judgements about performance in a task 10 . Although the neural correlates of such behaviour are being revealed 10 , 11 , little is known about how behaviour during such tasks relates to academic achievement.
Educational and cognitive neuroscientists study metacognition in different contexts using different methods. Indeed, while the latter investigate metacognition via behavioural task, the former mainly rely on introspective questionnaires. The extent to which these different operationalisations of metacognition match and reflect the same processes is unclear. As a result, the external validity of methodologies used in cognitive neuroscience is also unclear 16 . We argue that neurocognitive research on metacognition has a lot of potential to provide insights in mechanisms relevant in educational contexts, and that theoretical and methodological exchange between the two disciplines can benefit neuroscientific research in terms of ecological validity.
For these reasons, we investigate the literature through the lenses of external validity, theoretical discrepancies, domain generality and metacognitive training. Research on metacognition in cognitive neuroscience and educational sciences are reviewed separately. First, we investigate how metacognition is operationalised with respect to the common framework introduced by Nelson and Narens 23 (see Fig. 1 ). We then discuss the existing body of evidence regarding metacognitive training. Finally, we compare findings in both fields, highlight gaps and shortcomings, and propose avenues for research relying on crossovers of the two disciplines.
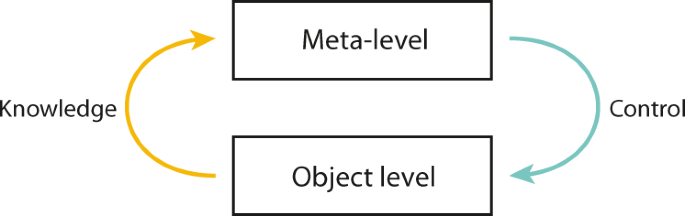
Meta-knowledge is characterised as the upward flow from object-level to meta-level. Meta-control is characterised as the downward flow from meta-level to object-level. Metacognition is therefore conceptualised as the bottom-up monitoring and top-down control of object-level processes. Adapted from Nelson and Narens’ cognitive psychology model of metacognition 23 .
In cognitive neuroscience, metacognition is divided into two main components 5 , 24 , which originate from the seminal works of Flavell on metamemory 25 , 26 . First, metacognitive knowledge (henceforth, meta-knowledge) is defined as the knowledge individuals have of their own cognitive processes and their ability to monitor and reflect on them. Second, metacognitive control (henceforth, meta-control) consists of someone’s self-regulatory mechanisms, such as planning and adapting behaviour based on outcomes 5 , 27 . Following Nelson and Narens’ definition 23 , meta-knowledge is characterised as the flow and processing of information from the object level to the meta-level, and meta-control as the flow from the meta-level to the object level 28 , 29 , 30 (Fig. 1 ). The object-level encompasses cognitive functions such as recognition and discrimination of objects, decision-making, semantic encoding, and spatial representation. On the meta-level, information originating from the object level is processed and top-down regulation on object-level functions is imposed 28 , 29 , 30 .
Educational researchers have mainly investigated metacognition through the lens of Self-Regulated Learning theory (SRL) 3 , 4 , which shares common conceptual roots with the theoretical framework used in cognitive neuroscience but varies from it in several ways 31 . First, SRL is constrained to learning activities, usually within educational settings. Second, metacognition is merely one of three components, with “motivation to learn” and “behavioural processes”, that enable individuals to learn in a self-directed manner 3 . In SRL, metacognition is defined as setting goals, planning, organising, self-monitoring and self-evaluating “at various points during the acquisition” 3 . The distinction between meta-knowledge and meta-control is not formally laid down although reference is often made to a “self-oriented feedback loop” describing the relationship between reflecting and regulating processes that resembles Nelson and Narens’ model (Fig. 1 ) 3 , 23 . In order to facilitate the comparison of operational definitions, we will refer to meta-knowledge in educational sciences when protocols operationalise self-awareness and knowledge of strategies, and to meta-control when they operationalise the selection and use of learning strategies and planning. For an in-depth discussion on metacognition and SRL, we refer to Dinsmore et al. 31 .
Metacognition in cognitive neuroscience
Operational definitions.
In cognitive neuroscience, research in metacognition is split into two tracks 32 . One track mainly studies meta-knowledge by investigating the neural basis of introspective judgements about one’s own cognition (i.e., metacognitive judgements), and meta-control with experiments involving cognitive offloading. In these experiments, subjects can perform actions such as set reminders, making notes and delegating tasks 33 , 34 , or report their desire for them 35 . Some research has investigated how metacognitive judgements can influence subsequent cognitive behaviour (i.e., a downward stream from the meta-level to the object level), but only one study so far has explored how this relationship is mapped in the brain 35 . In the other track, researchers investigate EF, also referred to as cognitive control 30 , 36 , which is closely related to metacognition. Note however that EF are often not framed in metacognitive terms in the literature 37 (but see ref. 30 ). For the sake of concision, we limit our review to operational definitions that have been used in neuroscientific studies.
Metacognitive judgements
Cognitive neuroscientists have been using paradigms in which subjects make judgements on how confident they are with regards to their learning of some given material 10 . These judgements are commonly referred to as metacognitive judgements , which can be viewed as a form of meta-knowledge (for reviews see Schwartz 38 and Nelson 39 ). Historically, researchers mostly resorted to paradigms known as Feelings of Knowing (FOK) 40 and Judgements of Learning (JOL) 41 . FOK reflect the belief of a subject to knowing the answer to a question or a problem and being able to recognise it from a list of alternatives, despite being unable to explicitly recall it 40 . Here, metacognitive judgement is thus made after retrieval attempt. In contrast, JOL are prospective judgements during learning of one’s ability to successfully recall an item on subsequent testing 41 .
More recently, cognitive neuroscientists have used paradigms in which subjects make retrospective metacognitive judgements on their performance in a two-alternative Forced Choice task (2-AFC) 42 . In 2-AFCs, subjects are asked to choose which of two presented options has the highest criterion value. Different domains can be involved, such as perception (e.g., visual or auditory) and memory. For example, subjects may be instructed to visually discriminate which one of two boxes contains more dots 43 , identify higher contrast Gabor patches 44 , or recognise novel words from words that were previously learned 45 (Fig. 2 ). The subjects engage in metacognitive judgements by rating how confident they are relative to their decision in the task. Based on their responses, one can evaluate a subject’s metacognitive sensitivity (the ability to discriminate one’s own correct and incorrect judgements), metacognitive bias (the overall level of confidence during a task), and metacognitive efficiency (the level of metacognitive sensitivity when controlling for task performance 46 ; Fig. 3 ). Note that sensitivity and bias are independent aspects of metacognition, meaning that two subjects may display the same levels of metacognitive sensitivity, but one may be biased towards high confidence while the other is biased towards low confidence. Because metacognitive sensitivity is affected by the difficulty of the task (one subject tends to display greater metacognitive sensitivity in easy tasks than difficult ones and different subjects may find a task more or less easy), metacognitive efficiency is an important measure as it allows researchers to compare metacognitive abilities between subjects and between domains. The most commonly used methods to assess metacognitive sensitivity during retrospective judgements are the receiver operating curve (ROC) and meta- d ′. 46 Both derive from signal detection theory (SDT) 47 which allows Type 1 sensitivity, or d’ ′ (how a subject can discriminate between stimulus alternatives, i.e. object-level processes) to be differentiated from metacognitive sensitivity (a judgement on the correctness of this decision) 48 . Importantly, only comparing meta- d ′ to d ′ seems to give reliable assessments metacognitive efficiency 49 . A ratio of 1 between meta- d’ ′ and d’ ′, indicates that a subject was perfectly able to discriminate between their correct and incorrect judgements. A ratio of 0.8 suggests that 80% of the task-related sensory evidence was available for the metacognitive judgements. Table 1 provides an overview of the different types of tasks and protocols with regards to the type of metacognitive process they operationalise. These operationalisations of meta-knowledge are used in combination with brain imaging methods (functional and structural magnetic resonance imaging; fMRI; MRI) to identify brain regions associated with metacognitive activity and metacognitive abilities 10 , 50 . Alternatively, transcranial magnetic stimulation (TMS) can be used to temporarily deactivate chosen brain regions and test whether this affects metacognitive abilities in given tasks 51 , 52 .
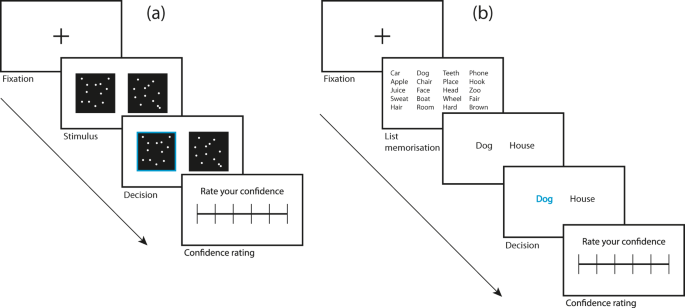
a Visual perception task: subjects choose the box containing the most (randomly generated) dots. Subjects then rate their confidence in their decision. b Memory task: subjects learn a list of words. In the next screen, they have to identify which of two words shown was present on the list. The subjects then rate their confidence in their decision.
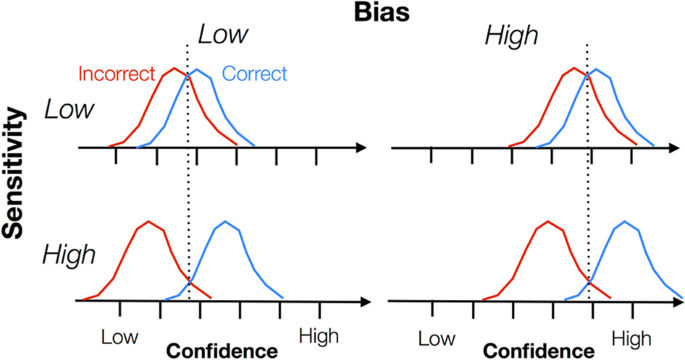
The red and blue curves represent the distribution of confidence ratings for incorrect and correct trials, respectively. A larger distance between the two curves denotes higher sensitivity. Displacement to the left and right denote biases towards low confidence (low metacognitive bias) and high confidence (high metacognitive bias), respectively (retrieved from Fig. 1 in Fleming and Lau 46 ). We repeat the disclaimer of the original authors that this figure is not a statistically accurate description of correct and incorrect responses, which are typically not normally distributed 46 , 47 .
A recent meta-analysis analysed 47 neuroimaging studies on metacognition and identified a domain-general network associated with high vs. low confidence ratings in both decision-making tasks (perception 2-AFC) and memory tasks (JOL, FOK) 11 . This network includes the medial and lateral prefrontal cortex (mPFC and lPFC, respectively), precuneus and insula. In contrast, the right anterior dorsolateral PFC (dlPFC) was specifically involved in decision-making tasks, and the bilateral parahippocampal cortex was specific to memory tasks. In addition, prospective judgements were associated with the posterior mPFC, left dlPFC and right insula, whereas retrospective judgements were associated with bilateral parahippocampal cortex and left inferior frontal gyrus. Finally, emerging evidence suggests a role of the right rostrolateral PFC (rlPFC) 53 , 54 , anterior PFC (aPFC) 44 , 45 , 55 , 56 , dorsal anterior cingulate cortex (dACC) 54 , 55 and precuneus 45 , 55 in metacognitive sensitivity (meta- d ′, ROC). In addition, several studies suggest that the aPFC relates to metacognition specifically in perception-related 2-AFC tasks, whereas the precuneus is engaged specifically in memory-related 2-AFC tasks 45 , 55 , 56 . This may suggest that metacognitive processes engage some regions in a domain-specific manner, while other regions are domain-general. For educational scientists, this could mean that some domains of metacognition may be more relevant for learning and, granted sufficient plasticity of the associated brain regions, that targeting them during interventions may show more substantial benefits. Note that rating one’s confidence and metacognitive sensitivity likely involve additional, peripheral cognitive processes instead of purely metacognitive ones. These regions are therefore associated with metacognition but not uniquely per se. Notably, a recent meta-analysis 50 suggests that domain-specific and domain-general signals may rather share common circuitry, but that their neural signature varies depending on the type of task or activity, showing that domain-generality in metacognition is complex and still needs to be better understood.
In terms of the role of metacognitive judgements on future behaviour, one study found that brain patterns associated with the desire for cognitive offloading (i.e., meta-control) partially overlap with those associated with meta-knowledge (metacognitive judgements of confidence), suggesting that meta-control is driven by either non-metacognitive, in addition to metacognitive, processes or by a combination of different domain-specific meta-knowledge processes 35 .
Executive function
In EF, processes such as error detection/monitoring and effort monitoring can be related to meta-knowledge while error correction, inhibitory control, and resource allocation can be related to meta-control 36 . To activate these processes, participants are asked to perform tasks in laboratory settings such as Flanker tasks, Stroop tasks, Demand Selection tasks and Motion Discrimination tasks (Fig. 4 ). Neural correlates of EF are investigated by having subjects perform such tasks while their brain activity is recorded with fMRI or electroencephalography (EEG). Additionally, patients with brain lesions can be tested against healthy participants to evaluate the functional role of the impaired regions 57 .
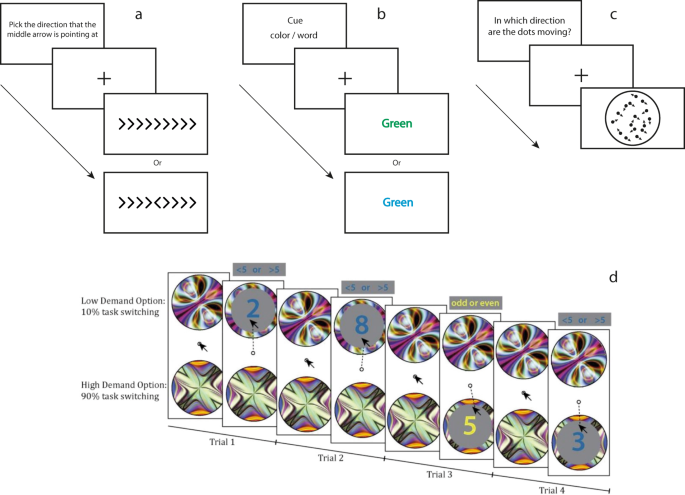
a Flanker task: subjects indicate the direction to which the arrow in the middle points. b Stroop task: subjects are presented with the name of colour printed in a colour that either matches or mismatches the name. Subjects are asked to give the name of the written colour or the printed colour. c Motion Discrimination task: subjects have to determine in which direction the dots are going with variating levels of noise. d Example of a Demand Selection task: in both options subjects have to switch between two tasks. Task one, subjects determine whether the number shown is higher or lower than 5. Task two, subjects determine whether the number is odd or even. The two options (low and high demand) differ in their degree of task switching, meaning the effort required. Subjects are allowed to switch between the two options. Note, the type of task is solely indicated by the colour of the number and that the subjects are not explicitly told about the difference in effort between the two options (retrieved from Fig. 1c in Froböse et al. 58 ).
In a review article on the neural basis of EF (in which they are defined as meta-control), Shimamura argues that a network of regions composed of the aPFC, ACC, ventrolateral PFC (vlPFC) and dlPFC is involved in the regulations of cognition 30 . These regions are not only interconnected but are also intricately connected to cortical and subcortical regions outside of the PFC. The vlPFC was shown to play an important role in “selecting and maintaining information in working memory”, whereas the dlPFC is involved in “manipulating and updating information in working memory” 30 . The ACC has been proposed to monitor cognitive conflict (e.g. in a Stroop task or a Flanker task), and the dlPFC to regulate it 58 , 59 . In particular, activity in the ACC in conflict monitoring (meta-knowledge) seems to contribute to control of cognition (meta-control) in the dlPFC 60 , 61 and to “bias behavioural decision-making toward cognitively efficient tasks and strategies” (p. 356) 62 . In a recent fMRI study, subjects performed a motion discrimination task (Fig. 4c ) 63 . After deciding on the direction of the motion, they were presented additional motion (i.e. post-decisional evidence) and then were asked to rate their confidence in their initial choice. The post-decisional evidence was encoded in the activity of the posterior medial frontal cortex (pMFC; meta-knowledge), while lateral aPFC (meta-control) modulated the impact of this evidence on subsequent confidence rating 63 . Finally, results from a meta-analysis study on cognitive control identified functional connectivity between the pMFC, associated with monitoring and informing other regions about the need for regulation, and the lPFC that would effectively regulate cognition 64 .
Online vs. offline metacognition
While the processes engaged during tasks such as those used in EF research can be considered as metacognitive in the sense that they are higher-order functions that monitor and control lower cognitive processes, scientists have argued that they are not functionally equivalent to metacognitive judgements 10 , 11 , 65 , 66 . Indeed, engaging in metacognitive judgements requires subjects to reflect on past or future activities. As such, metacognitive judgements can be considered as offline metacognitive processes. In contrast, high-order processes involved in decision-making tasks such as used in EF research are arguably largely made on the fly, or online , at a rapid pace and subjects do not need to reflect on their actions to perform them. Hence, we propose to explicitly distinguish online and offline processes. Other researchers have shared a similar view and some have proposed models for metacognition that make similar distinctions 65 , 66 , 67 , 68 . The functional difference between online and offline metacognition is supported by some evidence. For instance, event-related brain potential (ERP) studies suggest that error negativities are associated with error detection in general, whereas an increased error positivity specifically encodes error that subjects could report upon 69 , 70 . Furthermore, brain-imaging studies suggest that the MFC and ACC are involved in online meta-knowledge, while the aPFC and lPFC seem to be activated when subjects engage in more offline meta-knowledge and meta-control, respectively 63 , 71 , 72 . An overview of the different tasks can be found in Table 1 and a list of different studies on metacognition can be found in Supplementary Table 1 (organised in terms of the type of processes investigated, the protocols and brain measures used, along with the brain regions identified). Figure 5 illustrates the different brain regions associated with meta-knowledge and meta-control, distinguishing between what we consider to be online and offline processes. This distinction is often not made explicitly but it will be specifically helpful when building bridges between cognitive neuroscience and educational sciences.
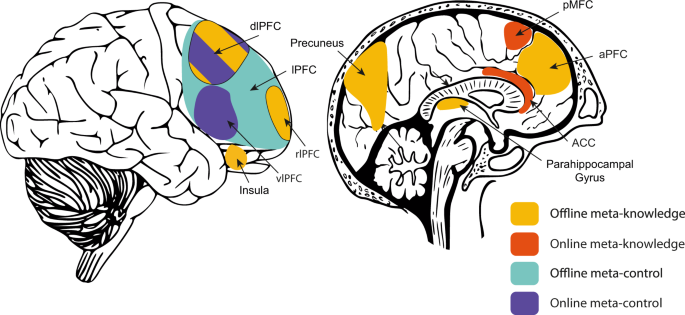
The regions are divided into online meta-knowledge and meta-control, and offline meta-knowledge and meta-control following the distinctions introduced earlier. Some regions have been reported to be related to both offline and online processes and are therefore given a striped pattern.
Training metacognition
There are extensive accounts in the literature of efforts to improve EF components such as inhibitory control, attention shifting and working memory 22 . While working memory does not directly reflect metacognitive abilities, its training is often hypothesised to improve general cognitive abilities and academic achievement. However, most meta-analyses found that training methods lead only to weak, non-lasting effects on cognitive control 73 , 74 , 75 . One meta-analysis did find evidence of near-transfer following EF training in children (in particular working memory, inhibitory control and cognitive flexibility), but found no evidence of far-transfer 20 . According to this study, training on one component leads to improved abilities in that same component but not in other EF components. Regarding adults, however, one meta-analysis suggests that EF training in general and working memory training specifically may both lead to significant near- and far-transfer effects 76 . On a neural level, a meta-analysis showed that cognitive training resulted in decreased brain activity in brain regions associated with EF 77 . According to the authors, this indicates that “training interventions reduce demands on externally focused attention” (p. 193) 77 .
With regards to meta-knowledge, several studies have reported increased task-related metacognitive abilities after training. For example, researchers found that subjects who received feedback on their metacognitive judgements regarding a perceptual decision-making task displayed better metacognitive accuracy, not only in the trained task but also in an untrained memory task 78 . Related, Baird and colleagues 79 found that a two-week mindfulness meditation training lead to enhanced meta-knowledge in the memory domain, but not the perceptual domain. The authors link these results to evidence of increased grey matter density in the aPFC in meditation practitioners.
Research on metacognition in cognitive science has mainly been studied through the lens of metacognitive judgements and EF (specifically performance monitoring and cognitive control). Meta-knowledge is commonly activated in subjects by asking them to rate their confidence in having successfully performed a task. A distinction is made between metacognitive sensitivity, metacognitive bias and metacognitive efficacy. Monitoring and regulating processes in EF are mainly operationalised with behavioural tasks such as Flanker tasks, Stroop tasks, Motion Discrimination tasks and Demand Selection tasks. In addition, metacognitive judgements can be viewed as offline processes in that they require the subject to reflect on her cognition and develop meta-representations. In contrast, EF can be considered as mostly online metacognitive processes because monitoring and regulation mostly happen rapidly without the need for reflective thinking.
Although there is some evidence for domain specificity, other studies have suggested that there is a single network of regions involved in all meta-cognitive tasks, but differentially activated in different task contexts. Comparing research on meta-knowledge and meta-control also suggest that some regions play a crucial role in both knowledge and regulation (Fig. 5 ). We have also identified a specific set of regions that are involved in either offline or online meta-knowledge. The evidence in favour of metacognitive training, while mixed, is interesting. In particular, research on offline meta-knowledge training involving self-reflection and metacognitive accuracy has shown some promising results. The regions that show structural changes after training, were those that we earlier identified as being part of the metacognition network. EF training does seem to show far-transfer effects at least in adults, but the relevance for everyday life activity is still unclear.
One major limitation of current research in metacognition is ecological validity. It is unclear to what extent the operationalisations reviewed above reflect real-life metacognition. For instance, are people who can accurately judge their performance on a behavioural task also able to accurately assess how they performed during an exam? Are people with high levels of error regulation and inhibitory control able to learn more efficiently? Note that criticism on the ecological validity of neurocognitive operationalisations extends beyond metacognition research 16 . A solution for improving validity may be to compare operationalisations of metacognition in cognitive neuroscience with the ones in educational sciences, which have shown clear links with learning in formal education. This also applies to metacognitive training.
Metacognition in educational sciences
The most popular protocols used to measure metacognition in educational sciences are self-report questionnaires or interviews, learning journals and thinking-aloud protocols 31 , 80 . During interviews, subjects are asked to answer questions regarding hypothetical situations 81 . In learning journals, students write about their learning experience and their thoughts on learning 82 , 83 . In thinking-aloud protocols, subjects are asked to verbalise their thoughts while performing a problem-solving task 80 . Each of these instruments can be used to study meta-knowledge and meta-control. For instance, one of the most widely used questionnaires, the Metacognitive Awareness Inventory (MAI) 42 , operationalises “Flavellian” metacognition and has dedicated scales for meta-knowledge and meta-control (also popular are the MSLQ 84 and LASSI 85 which operate under SRL). The meta-knowledge scale of the MAI operationalises knowledge of strategies (e.g., “ I am aware of what strategies I use when I study ”) and self-awareness (e.g., “ I am a good judge of how well I understand something ”); the meta-control scale operationalises planning (e.g., “ I set a goal before I begin a task ”) and use of learning strategies (e.g., “ I summarize what I’ve learned after I finish ”). Learning journals, self-report questionnaires and interviews involve offline metacognition. Thinking aloud, though not engaging the same degree self-reflection, also involves offline metacognition in the sense that online processes are verbalised, which necessitate offline processing (see Table 1 for an overview and Supplementary Table 2 for more details).
More recently, methodologies borrowed from cognitive neuroscience have been introduced to study EF in educational settings 22 , 86 . In particular, researchers used classic cognitive control tasks such as the Stroop task (for a meta-analysis 86 ). Most of the studied components are related to meta-control and not meta-knowledge. For instance, the BRIEF 87 is a questionnaire completed by parents and teachers which assesses different subdomains of EF: (1) inhibition, shifting, and emotional control which can be viewed as online metacognitive control, and (2) planning, organisation of materials, and monitoring, which can be viewed as offline meta-control 87 .
Assessment of metacognition is usually compared against metrics of academic performance such as grades or scores on designated tasks. A recent meta-analysis reported a weak correlation of self-report questionnaires and interviews with academic performance whereas think-aloud protocols correlated highly 88 . Offline meta-knowledge processes operationalised by learning journals were found to be positively associated with academic achievement when related to reflection on learning activities but negatively associated when related to reflection on learning materials, indicating that the type of reflection is important 89 . EF have been associated with abilities in mathematics (mainly) and reading comprehension 86 . However, the literature points towards contrary directions as to what specific EF component is involved in academic achievement. This may be due to the different groups that were studied, to different operationalisations or to different theoretical underpinnings for EF 86 . For instance, online and offline metacognitive processes, which are not systematically distinguished in the literature, may play different roles in academic achievement. Moreover, the bulk of research focussed on young children with few studies on adolescents 86 and EF may play a role at varying extents at different stages of life.
A critical question in educational sciences is that of the nature of the relationship between metacognition and academic achievement to understand whether learning at school can be enhanced by training metacognitive abilities. Does higher metacognition lead to higher academic achievement? Do these features evolve in parallel? Developmental research provides valuable insights into the formation of metacognitive abilities that can inform training designs in terms of what aspect of metacognition should be supported and the age at which interventions may yield the best results. First, meta-knowledge seems to emerge around the age of 5, meta-control around 8, and both develop over the years 90 , with evidence for the development of meta-knowledge into adolescence 91 . Furthermore, current theories propose that meta-knowledge abilities are initially highly domain-dependent and gradually become more domain-independent as knowledge and experience are acquired and linked between domains 32 . Meta-control is believed to evolve in a similar fashion 90 , 92 .
Common methods used to train offline metacognition are direct instruction of metacognition, metacognitive prompts and learning journals. In addition, research has been done on the use of (self-directed) feedback as a means to induce self-reflection in students, mainly in computer-supported settings 93 . Interestingly, learning journals appear to be used for both assessing and fostering metacognition. Metacognitive instruction consists of teaching learners’ strategies to “activate” their metacognition. Metacognitive prompts most often consist of text pieces that are sent at specific times and that trigger reflection (offline meta-knowledge) on learning behaviour in the form of a question, hint or reminder.
Meta-analyses have investigated the effects of direct metacognitive instruction on students’ use of learning strategies and academic outcomes 18 , 94 , 95 . Their findings show that metacognitive instruction can have a positive effect on learning abilities and achievement within a population ranging from primary schoolers to university students. In particular, interventions lead to the highest effect sizes when they both (i) instructed a combination of metacognitive strategies with an emphasis on planning strategies (offline meta-control) and (ii) “provided students with knowledge about strategies” (offline meta-knowledge) and “illustrated the benefits of applying the trained strategies, or even stimulated metacognitive reasoning” (p.114) 18 . The longer the duration of the intervention, the more effective they were. The strongest effects on academic performance were observed in the context of mathematics, followed by reading and writing.
While metacognitive prompts and learning journals make up the larger part of the literature on metacognitive training 96 , meta-analyses that specifically investigate their effectiveness have yet to be performed. Nonetheless, evidence suggests that such interventions can be successful. Researchers found that metacognitive prompts fostered the use of metacognitive strategies (offline meta-control) and that the combination of cognitive and metacognitive prompts improved learning outcomes 97 . Another experiment showed that students who received metacognitive prompts performed more metacognitive activities inside the learning environment and displayed better transfer performance immediately after the intervention 98 . A similar study using self-directed prompts showed enhanced transfer performance that was still observable 3 weeks after the intervention 99 .
Several studies suggest that learning journals can positively enhance metacognition. Subjects who kept a learning journal displayed stronger high meta-control and meta-knowledge on learning tasks and tended to reach higher academic outcomes 100 , 101 , 102 . However, how the learning journal is used seems to be critical; good instructions are crucial 97 , 103 , and subjects who simply summarise their learning activity benefit less from the intervention than subjects who reflect about their knowledge, learning and learning goals 104 . An overview of studies using learning journals and metacognitive prompts to train metacognition can be found in Supplementary Table 3 .
In recent years, educational neuroscience researchers have tried to determine whether training and improvements in EF can lead to learning facilitation and higher academic achievement. Training may consist of having students continually perform behavioural tasks either in the lab, at home, or at school. Current evidence in favour of training EF is mixed, with only anecdotal evidence for positive effects 105 . A meta-analysis did not show evidence for a causal relationship between EF and academic achievement 19 , but suggested that the relationship is bidirectional, meaning that the two are “mutually supportive” 106 .
A recent review article has identified several gaps and shortcoming in the literature on metacognitive training 96 . Overall, research in metacognitive training has been mainly invested in developing learners’ meta-control rather than meta-knowledge. Furthermore, most of the interventions were done in the context of science learning. Critically, there appears to be a lack of studies that employed randomised control designs, such that the effects of metacognitive training intervention are often difficult to evaluate. In addition, research overwhelmingly investigated metacognitive prompts and learning journals in adults 96 , while interventions on EF mainly focused on young children 22 . Lastly, meta-analyses evaluating the effectiveness of metacognitive training have so far focused on metacognitive instruction on children. There is thus a clear disbalance between the meta-analyses performed and the scope of the literature available.
An important caveat of educational sciences research is that metacognition is not typically framed in terms of online and offline metacognition. Therefore, it can be unclear whether protocols operationalise online or offline processes and whether interventions tend to benefit more online or offline metacognition. There is also confusion in terms of what processes qualify as EF and definitions of it vary substantially 86 . For instance, Clements and colleagues mention work on SRL to illustrate research in EF in relation to academic achievement but the two spawn from different lines of research, one rooted in metacognition and socio-cognitive theory 31 and the other in the cognitive (neuro)science of decision-making. In addition, the MSLQ, as discussed above, assesses offline metacognition along with other components relevant to SRL, whereas EF can be mainly understood as online metacognition (see Table 1 ), which on the neural level may rely on different circuitry.
Investigating offline metacognition tends to be carried out in school settings whereas evaluating EF (e.g., Stroop task, and BRIEF) is performed in the lab. Common to all protocols for offline metacognition is that they consist of a form of self-report from the learner, either during the learning activity (thinking-aloud protocols) or after the learning activity (questionnaires, interviews and learning journals). Questionnaires are popular protocols due to how easy they are to administer but have been criticised to provide biased evaluations of metacognitive abilities. In contrast, learning journals evaluate the degree to which learners engage in reflective thinking and may therefore be less prone to bias. Lastly, it is unclear to what extent thinking-aloud protocols are sensitive to online metacognitive processes, such as on-the-fly error correction and effort regulation. The strength of the relationship between metacognitive abilities and academic achievement varies depending on how metacognition is operationalised. Self-report questionnaires and interviews are weakly related to achievement whereas thinking-aloud protocols and EF are strongly related to it.
Based on the well-documented relationship between metacognition and academic achievement, educational scientists hypothesised that fostering metacognition may improve learning and academic achievement, and thus performed metacognitive training interventions. The most prevalent training protocols are direct metacognitive instruction, learning journals, and metacognitive prompts, which aim to induce and foster offline metacognitive processes such as self-reflection, planning and selecting learning strategies. In addition, researchers have investigated whether training EF, either through tasks or embedded in the curriculum, results in higher academic proficiency and achievement. While a large body of evidence suggests that metacognitive instruction, learning journals and metacognitive prompts can successfully improve academic achievement, interventions designed around EF training show mixed results. Future research investigating EF training in different age categories may clarify this situation. These various degrees of success of interventions may indicate that offline metacognition is more easily trainable than online metacognition and plays a more important role in educational settings. Investigating the effects of different methods, offline and online, on the neural level, may provide researchers with insights into the trainability of different metacognitive processes.
In this article, we reviewed the literature on metacognition in educational sciences and cognitive neuroscience with the aim to investigate gaps in current research and propose ways to address them through the exchange of insights between the two disciplines and interdisciplinary approaches. The main aspects analysed were operational definitions of metacognition and metacognitive training, through the lens of metacognitive knowledge and metacognitive control. Our review also highlighted an additional construct in the form of the distinction between online metacognition (on the fly and largely automatic) and offline metacognition (slower, reflective and requiring meta-representations). In cognitive neuroscience, research has focused on metacognitive judgements (mainly offline) and EF (mainly online). Metacognition is operationalised with tasks carried out in the lab and are mapped onto brain functions. In contrast, research in educational sciences typically measures metacognition in the context of learning activities, mostly in schools and universities. More recently, EF has been studied in educational settings to investigate its role in academic achievement and whether training it may benefit learning. Evidence on the latter is however mixed. Regarding metacognitive training in general, evidence from both disciplines suggests that interventions fostering learners’ self-reflection and knowledge of their learning behaviour (i.e., offline meta-knowledge) may best benefit them and increase academic achievement.
We focused on four aspects of research that could benefit from an interdisciplinary approach between the two areas: (i) validity and reliability of research protocols, (ii) under-researched dimensions of metacognition, (iii) metacognitive training, and (iv) domain-specificity vs. domain generality of metacognitive abilities. To tackle these issue, we propose four avenues for integrated research: (i) investigate the degree to which different protocols relate to similar or different metacognitive constructs, (ii) implement designs and perform experiments to identify neural substrates necessary for offline meta-control by for example borrowing protocols used in educational sciences, (iii) study the effects of (offline) meta-knowledge training on the brain, and (iv) perform developmental research in the metacognitive brain and compare it with the existing developmental literature in educational sciences regarding the domain-generality of metacognitive processes and metacognitive abilities.
First, neurocognitive research on metacognitive judgements has developed robust operationalisations of offline meta-knowledge. However, these operationalisations often consist of specific tasks (e.g., 2-AFC) carried out in the lab. These tasks are often very narrow and do not resemble the challenges and complexities of behaviours associated with learning in schools and universities. Thus, one may question to what extent they reflect real-life metacognition, and to what extent protocols developed in educational sciences and cognitive neuroscience actually operationalise the same components of metacognition. We propose that comparing different protocols from both disciplines that are, a priori, operationalising the same types of metacognitive processes can help evaluate the ecological validity of protocols used in cognitive neuroscience, and allow for more holistic assessments of metacognition, provided that it is clear which protocol assesses which construct. Degrees of correlation between different protocols, within and between disciplines, may allow researchers to assess to what extent they reflect the same metacognitive constructs and also identify what protocols are most appropriate to study a specific construct. For example, a relation between meta- d ′ metacognitive sensitivity in a 2-AFC task and the meta-knowledge subscale of the MAI, would provide external validity to the former. Moreover, educational scientists would be provided with bias-free tools to assess metacognition. These tools may enable researchers to further investigate to what extent metacognitive bias, sensitivity and efficiency each play a role in education settings. In contrast, a low correlation may highlight a difference in domain between the two measures of metacognition. For instance, metacognitive judgements in brain research are made in isolated behaviour, and meta-d’ can thus be viewed to reflect “local” metacognitive sensitivity. It is also unclear to what extent processes involved in these decision-making tasks cover those taking place in a learning environment. When answering self-reported questionnaires, however, subjects make metacognitive judgements on a large set of (learning) activities, and the measures may thus resemble more “global” or domain-general metacognitive sensitivity. In addition, learners in educational settings tend to receive feedback — immediate or delayed — on their learning activities and performance, which is generally not the case for cognitive neuroscience protocols. Therefore, investigating metacognitive judgements in the presence of performance or social feedback may allow researchers to better understand the metacognitive processes at play in educational settings. Devising a global measure of metacognition in the lab by aggregating subjects’ metacognitive abilities in different domains or investigating to what extent local metacognition may affect global metacognition could improve ecological validity significantly. By investigating the neural correlates of educational measures of metacognition, researchers may be able to better understand to what extent the constructs studied in the two disciplines are related. It is indeed possible that, though weakly correlated, the meta-knowledge scale of the MAI and meta-d’ share a common neural basis.
Second, our review highlights gaps in the literature of both disciplines regarding the research of certain types of metacognitive processes. There is a lack of research in offline meta-control (or strategic regulation of cognition) in neuroscience, whereas this construct is widely studied in educational sciences. More specifically, while there exists research on EF related to planning (e.g. 107 ), common experimental designs make it hard to disentangle online from offline metacognitive processes. A few studies have implemented subject reports (e.g., awareness of error or desire for reminders) to pin-point the neural substrates specifically involved in offline meta-control and the current evidence points at a role of the lPFC. More research implementing similar designs may clarify this construct. Alternatively, researchers may exploit educational sciences protocols, such as self-report questionnaires, learning journals, metacognitive prompts and feedback to investigate offline meta-control processes in the brain and their relation to academic proficiency and achievement.
Third, there is only one study known to us on the training of meta-knowledge in the lab 78 . In contrast, meta-knowledge training in educational sciences have been widely studied, in particular with metacognitive prompts and learning journals, although a systematic review would be needed to identify the benefits for learning. Relative to cognitive neuroscience, studies suggest that offline meta-knowledge trained in and outside the lab (i.e., metacognitive judgements and meditation, respectively) transfer to meta-knowledge in other lab tasks. The case of meditation is particularly interesting since meditation has been demonstrated to beneficiate varied aspects of everyday life 108 . Given its importance for efficient regulation of cognition, training (offline) meta-knowledge may present the largest benefits to academic achievement. Hence, it is important to investigate development in the brain relative to meta-knowledge training. Evidence on metacognitive training in educational sciences tends to suggest that offline metacognition is more “plastic” and may therefore benefit learning more than online metacognition. Furthermore, it is important to have a good understanding of the developmental trajectory of metacognitive abilities — not only on a behavioural level but also on a neural level — to identify critical periods for successful training. Doing so would also allow researchers to investigate the potential differences in terms of plasticity that we mention above. Currently, the developmental trajectory of metacognition is under-studied in cognitive neuroscience with only one study that found an overlap between the neural correlates of metacognition in adults and children 109 . On a side note, future research could explore the potential role of genetic factors in metacognitive abilities to better understand to what extent and under what constraints they can be trained.
Fourth, domain-specific and domain-general aspects of metacognitive processes should be further investigated. Educational scientists have studied the development of metacognition in learners and have concluded that metacognitive abilities are domain-specific at the beginning (meaning that their quality depends on the type of learning activity, like mathematics vs. writing) and progressively evolve towards domain-general abilities as knowledge and expertise increase. Similarly, neurocognitive evidence points towards a common network for (offline) metacognitive knowledge which engages the different regions at varying degrees depending on the domain of the activity (i.e., perception, memory, etc.). Investigating this network from a developmental perspective and comparing findings with the existing behavioural literature may improve our understanding of the metacognitive brain and link the two bodies of evidence. It may also enable researchers to identify stages of life more suitable for certain types of metacognitive intervention.
Dunlosky, J. & Metcalfe, J. Metacognition (SAGE Publications, 2008).
Pintrich, P. R. The role of metacognitive knowledge in learning, teaching, and assessing. Theory Into Pract. 41 , 219–225 (2002).
Article Google Scholar
Zimmerman, B. J. Self-regulated learning and academic achievement: an overview. Educ. Psychol. 25 , 3–17 (1990).
Zimmerman, B. J. & Schunk, D. H. Self-Regulated Learning and Academic Achievement: Theoretical Perspectives (Routledge, 2001).
Baker, L. & Brown, A. L. Metacognitive Skills and Reading. In Handbook of Reading Research Vol. 1 (ed. Pearson, P. D.) 353–395 (Longman, 1984).
Mckeown, M. G. & Beck, I. L. The role of metacognition in understanding and supporting reading comprehension. In Handbook of Metacognition in Education (eds Hacker, D. J., Dunlosky, J. & Graesser, A. C.) 19–37 (Routledge, 2009).
Desoete, A., Roeyers, H. & Buysse, A. Metacognition and mathematical problem solving in grade 3. J. Learn. Disabil. 34 , 435–447 (2001).
Article CAS PubMed Google Scholar
Veenman, M., Kok, R. & Blöte, A. W. The relation between intellectual and metacognitive skills in early adolescence. Instructional Sci. 33 , 193–211 (2005).
Harris, K. R., Graham, S., Brindle, M. & Sandmel, K. Metacognition and children’s writing. In Handbook of metacognition in education 131–153 (Routledge, 2009).
Fleming, S. M. & Dolan, R. J. The neural basis of metacognitive ability. Philos. Trans. R. Soc. B 367 , 1338–1349 (2012).
Vaccaro, A. G. & Fleming, S. M. Thinking about thinking: a coordinate-based meta-analysis of neuroimaging studies of metacognitive judgements. Brain Neurosci. Adv. 2 , 10.1177%2F2398212818810591 (2018).
Ferrari, M. What can neuroscience bring to education? Educ. Philos. Theory 43 , 31–36 (2011).
Zadina, J. N. The emerging role of educational neuroscience in education reform. Psicol. Educ. 21 , 71–77 (2015).
Meulen, A., van der, Krabbendam, L. & Ruyter, Dde Educational neuroscience: its position, aims and expectations. Br. J. Educ. Stud. 63 , 229–243 (2015).
Varma, S., McCandliss, B. D. & Schwartz, D. L. Scientific and pragmatic challenges for bridging education and neuroscience. Educ. Res. 37 , 140–152 (2008).
van Atteveldt, N., van Kesteren, M. T. R., Braams, B. & Krabbendam, L. Neuroimaging of learning and development: improving ecological validity. Frontline Learn. Res. 6 , 186–203 (2018).
Article PubMed PubMed Central Google Scholar
Hruby, G. G. Three requirements for justifying an educational neuroscience. Br. J. Educ. Psychol. 82 , 1–23 (2012).
Article PubMed Google Scholar
Dignath, C., Buettner, G. & Langfeldt, H.-P. How can primary school students learn self-regulated learning strategies most effectively?: A meta-analysis on self-regulation training programmes. Educ. Res. Rev. 3 , 101–129 (2008).
Jacob, R. & Parkinson, J. The potential for school-based interventions that target executive function to improve academic achievement: a review. Rev. Educ. Res. 85 , 512–552 (2015).
Kassai, R., Futo, J., Demetrovics, Z. & Takacs, Z. K. A meta-analysis of the experimental evidence on the near- and far-transfer effects among children’s executive function skills. Psychol. Bull. 145 , 165–188 (2019).
Roebers, C. M. Executive function and metacognition: towards a unifying framework of cognitive self-regulation. Dev. Rev. 45 , 31–51 (2017).
Clements, D. H., Sarama, J. & Germeroth, C. Learning executive function and early mathematics: directions of causal relations. Early Child. Res. Q. 36 , 79–90 (2016).
Nelson, T. O. & Narens, L. Metamemory. In Perspectives on the development of memory and cognition (ed. R. V. Kail & J. W. Hag) 3–33 (Hillsdale, N.J.: Erlbaum, 1977).
Baird, J. R. Improving learning through enhanced metacognition: a classroom study. Eur. J. Sci. Educ. 8 , 263–282 (1986).
Flavell, J. H. & Wellman, H. M. Metamemory (1975).
Flavell, J. H. Metacognition and cognitive monitoring: a new area of cognitive–developmental inquiry. Am. Psychol. 34 , 906 (1979).
Livingston, J. A. Metacognition: An Overview. (2003).
Nelson, T. O. Metamemory: a theoretical framework and new findings. In Psychology of Learning and Motivation Vol. 26 (ed. Bower, G. H.) 125–173 (Academic Press, 1990).
Nelson, T. O. & Narens, L. Why investigate metacognition. In Metacognition: Knowing About Knowing (eds Metcalfe, J. & Shimamura, A. P.) 1–25 (MIT Press, 1994).
Shimamura, A. P. A Neurocognitive approach to metacognitive monitoring and control. In Handbook of Metamemory and Memory (eds Dunlosky, J. & Bjork, R. A.) (Routledge, 2014).
Dinsmore, D. L., Alexander, P. A. & Loughlin, S. M. Focusing the conceptual lens on metacognition, self-regulation, and self-regulated learning. Educ. Psychol. Rev. 20 , 391–409 (2008).
Borkowski, J. G., Chan, L. K. & Muthukrishna, N. A process-oriented model of metacognition: links between motivation and executive functioning. In (Gregory Schraw & James C. Impara) Issues in the Measurement of Metacognition 1–42 (Buros Institute of Mental Measurements, 2000).
Risko, E. F. & Gilbert, S. J. Cognitive offloading. Trends Cogn. Sci. 20 , 676–688 (2016).
Gilbert, S. J. et al. Optimal use of reminders: metacognition, effort, and cognitive offloading. J. Exp. Psychol. 149 , 501 (2020).
Boldt, A. & Gilbert, S. Distinct and overlapping neural correlates of metacognitive monitoring and metacognitive control. Preprint at bioRxiv https://psyarxiv.com/3dz9b/ (2020).
Fernandez-Duque, D., Baird, J. A. & Posner, M. I. Executive attention and metacognitive regulation. Conscious Cogn. 9 , 288–307 (2000).
Baker, L., Zeliger-Kandasamy, A. & DeWyngaert, L. U. Neuroimaging evidence of comprehension monitoring. Psihol. teme 23 , 167–187 (2014).
Google Scholar
Schwartz, B. L. Sources of information in metamemory: Judgments of learning and feelings of knowing. Psychon. Bull. Rev. 1 , 357–375 (1994).
Nelson, T. O. Metamemory, psychology of. In International Encyclopedia of the Social & Behavioral Sciences (eds Smelser, N. J. & Baltes, P. B.) 9733–9738 (Pergamon, 2001).
Hart, J. T. Memory and the feeling-of-knowing experience. J. Educ. Psychol. 56 , 208 (1965).
Arbuckle, T. Y. & Cuddy, L. L. Discrimination of item strength at time of presentation. J. Exp. Psychol. 81 , 126 (1969).
Fechner, G. T. Elemente der Psychophysik (Breitkopf & Härtel, 1860).
Rouault, M., Seow, T., Gillan, C. M. & Fleming, S. M. Psychiatric symptom dimensions are associated with dissociable shifts in metacognition but not task performance. Biol. Psychiatry 84 , 443–451 (2018).
Fleming, S. M., Weil, R. S., Nagy, Z., Dolan, R. J. & Rees, G. Relating introspective accuracy to individual differences in brain structure. Science 329 , 1541–1543 (2010).
Article CAS PubMed PubMed Central Google Scholar
McCurdy, L. Y. et al. Anatomical coupling between distinct metacognitive systems for memory and visual perception. J. Neurosci. 33 , 1897–1906 (2013).
Fleming, S. M. & Lau, H. C. How to measure metacognition. Front. Hum. Neurosci. 8 https://doi.org/10.3389/fnhum.2014.00443 (2014).
Galvin, S. J., Podd, J. V., Drga, V. & Whitmore, J. Type 2 tasks in the theory of signal detectability: discrimination between correct and incorrect decisions. Psychon. Bull. Rev. 10 , 843–876 (2003).
Metcalfe, J. & Schwartz, B. L. The ghost in the machine: self-reflective consciousness and the neuroscience of metacognition. In (eds Dunlosky, J. & Tauber, S. K.) Oxford Handbook of Metamemory 407–424 (Oxford University Press, 2016).
Maniscalco, B. & Lau, H. A signal detection theoretic approach for estimating metacognitive sensitivity from confidence ratings. Conscious Cognition 21 , 422–430 (2012).
Rouault, M., McWilliams, A., Allen, M. G. & Fleming, S. M. Human metacognition across domains: insights from individual differences and neuroimaging. Personal. Neurosci. 1 https://doi.org/10.1017/pen.2018.16 (2018).
Rounis, E., Maniscalco, B., Rothwell, J. C., Passingham, R. E. & Lau, H. Theta-burst transcranial magnetic stimulation to the prefrontal cortex impairs metacognitive visual awareness. Cogn. Neurosci. 1 , 165–175 (2010).
Ye, Q., Zou, F., Lau, H., Hu, Y. & Kwok, S. C. Causal evidence for mnemonic metacognition in human precuneus. J. Neurosci. 38 , 6379–6387 (2018).
Fleming, S. M., Huijgen, J. & Dolan, R. J. Prefrontal contributions to metacognition in perceptual decision making. J. Neurosci. 32 , 6117–6125 (2012).
Morales, J., Lau, H. & Fleming, S. M. Domain-general and domain-specific patterns of activity supporting metacognition in human prefrontal cortex. J. Neurosci. 38 , 3534–3546 (2018).
Baird, B., Smallwood, J., Gorgolewski, K. J. & Margulies, D. S. Medial and lateral networks in anterior prefrontal cortex support metacognitive ability for memory and perception. J. Neurosci. 33 , 16657–16665 (2013).
Fleming, S. M., Ryu, J., Golfinos, J. G. & Blackmon, K. E. Domain-specific impairment in metacognitive accuracy following anterior prefrontal lesions. Brain 137 , 2811–2822 (2014).
Baldo, J. V., Shimamura, A. P., Delis, D. C., Kramer, J. & Kaplan, E. Verbal and design fluency in patients with frontal lobe lesions. J. Int. Neuropsychol. Soc. 7 , 586–596 (2001).
Froböse, M. I. et al. Catecholaminergic modulation of the avoidance of cognitive control. J. Exp. Psychol. Gen. 147 , 1763 (2018).
Botvinick, M. M., Braver, T. S., Barch, D. M., Carter, C. S. & Cohen, J. D. Conflict monitoring and cognitive control. Psychol. Rev. 108 , 624 (2001).
Kerns, J. G. et al. Anterior cingulate conflict monitoring and adjustments in control. Science 303 , 1023–1026 (2004).
Yeung, N. Conflict monitoring and cognitive control. In The Oxford Handbook of Cognitive Neuroscience: The Cutting Edges Vol. 2 (eds Ochsner, K. N. & Kosslyn, S.) 275–299 (Oxford University Press, 2014).
Botvinick, M. M. Conflict monitoring and decision making: reconciling two perspectives on anterior cingulate function. Cogn. Affect. Behav. Neurosci. 7 , 356–366 (2007).
Fleming, S. M., van der Putten, E. J. & Daw, N. D. Neural mediators of changes of mind about perceptual decisions. Nat. Neurosci. 21 , 617–624 (2018).
Ridderinkhof, K. R., Ullsperger, M., Crone, E. A. & Nieuwenhuis, S. The role of the medial frontal cortex in cognitive control. Science 306 , 443–447 (2004).
Koriat, A. The feeling of knowing: some metatheoretical implications for consciousness and control. Conscious Cogn. 9 , 149–171 (2000).
Thompson, V. A., Evans, J. & Frankish, K. Dual process theories: a metacognitive perspective. Ariel 137 , 51–43 (2009).
Arango-Muñoz, S. Two levels of metacognition. Philosophia 39 , 71–82 (2011).
Shea, N. et al. Supra-personal cognitive control and metacognition. Trends Cogn. Sci. 18 , 186–193 (2014).
Nieuwenhuis, S., Ridderinkhof, K. R., Blom, J., Band, G. P. & Kok, A. Error-related brain potentials are differentially related to awareness of response errors: evidence from an antisaccade task. Psychophysiology 38 , 752–760 (2001).
Overbeek, T. J., Nieuwenhuis, S. & Ridderinkhof, K. R. Dissociable components of error processing: on the functional significance of the Pe vis-à-vis the ERN/Ne. J. Psychophysiol. 19 , 319–329 (2005).
McGuire, J. T. & Botvinick, M. M. Prefrontal cortex, cognitive control, and the registration of decision costs. Proc. Natl Acad. Sci. USA 107 , 7922–7926 (2010).
Hester, R., Foxe, J. J., Molholm, S., Shpaner, M. & Garavan, H. Neural mechanisms involved in error processing: a comparison of errors made with and without awareness. Neuroimage 27 , 602–608 (2005).
Melby-Lervåg, M. & Hulme, C. Is working memory training effective? A meta-analytic review. Dev. Psychol. 49 , 270 (2013).
Soveri, A., Antfolk, J., Karlsson, L., Salo, B. & Laine, M. Working memory training revisited: a multi-level meta-analysis of n-back training studies. Psychon. Bull. Rev. 24 , 1077–1096 (2017).
Schwaighofer, M., Fischer, F. & Bühner, M. Does working memory training transfer? A meta-analysis including training conditions as moderators. Educ. Psychol. 50 , 138–166 (2015).
Karbach, J. & Verhaeghen, P. Making working memory work: a meta-analysis of executive-control and working memory training in older adults. Psychol. Sci. 25 , 2027–2037 (2014).
Patel, R., Spreng, R. N. & Turner, G. R. Functional brain changes following cognitive and motor skills training: a quantitative meta-analysis. Neurorehabil Neural Repair 27 , 187–199 (2013).
Carpenter, J. et al. Domain-general enhancements of metacognitive ability through adaptive training. J. Exp. Psychol. 148 , 51–64 (2019).
Baird, B., Mrazek, M. D., Phillips, D. T. & Schooler, J. W. Domain-specific enhancement of metacognitive ability following meditation training. J. Exp. Psychol. 143 , 1972 (2014).
Winne, P. H. & Perry, N. E. Measuring self-regulated learning. In Handbook of Self-Regulation (eds Boekaerts, M., Pintrich, P. R. & Zeidner, M.) Ch. 16, 531–566 (Academic Press, 2000).
Zimmerman, B. J. & Martinez-Pons, M. Development of a structured interview for assessing student use of self-regulated learning strategies. Am. Educ. Res. J. 23 , 614–628 (1986).
Park, C. Engaging students in the learning process: the learning journal. J. Geogr. High. Educ. 27 , 183–199 (2003).
Article CAS Google Scholar
Harrison, G. M. & Vallin, L. M. Evaluating the metacognitive awareness inventory using empirical factor-structure evidence. Metacogn. Learn. 13 , 15–38 (2018).
Pintrich, P. R., Smith, D. A. F., Garcia, T. & Mckeachie, W. J. Reliability and predictive validity of the motivated strategies for learning questionnaire (MSLQ). Educ. Psychol. Meas. 53 , 801–813 (1993).
Prevatt, F., Petscher, Y., Proctor, B. E., Hurst, A. & Adams, K. The revised Learning and Study Strategies Inventory: an evaluation of competing models. Educ. Psychol. Meas. 66 , 448–458 (2006).
Baggetta, P. & Alexander, P. A. Conceptualization and operationalization of executive function. Mind Brain Educ. 10 , 10–33 (2016).
Gioia, G. A., Isquith, P. K., Guy, S. C. & Kenworthy, L. Test review behavior rating inventory of executive function. Child Neuropsychol. 6 , 235–238 (2000).
Ohtani, K. & Hisasaka, T. Beyond intelligence: a meta-analytic review of the relationship among metacognition, intelligence, and academic performance. Metacogn. Learn. 13 , 179–212 (2018).
Dianovsky, M. T. & Wink, D. J. Student learning through journal writing in a general education chemistry course for pre-elementary education majors. Sci. Educ. 96 , 543–565 (2012).
Veenman, M. V. J., Van Hout-Wolters, B. H. A. M. & Afflerbach, P. Metacognition and learning: conceptual and methodological considerations. Metacogn Learn. 1 , 3–14 (2006).
Weil, L. G. et al. The development of metacognitive ability in adolescence. Conscious Cogn. 22 , 264–271 (2013).
Veenman, M. & Spaans, M. A. Relation between intellectual and metacognitive skills: Age and task differences. Learn. Individ. Differ. 15 , 159–176 (2005).
Verbert, K. et al. Learning dashboards: an overview and future research opportunities. Personal. Ubiquitous Comput. 18 , 1499–1514 (2014).
Dignath, C. & Büttner, G. Components of fostering self-regulated learning among students. A meta-analysis on intervention studies at primary and secondary school level. Metacogn. Learn. 3 , 231–264 (2008).
Hattie, J., Biggs, J. & Purdie, N. Effects of learning skills interventions on student learning: a meta-analysis. Rev. Educ. Res. 66 , 99–136 (1996).
Zohar, A. & Barzilai, S. A review of research on metacognition in science education: current and future directions. Stud. Sci. Educ. 49 , 121–169 (2013).
Berthold, K., Nückles, M. & Renkl, A. Do learning protocols support learning strategies and outcomes? The role of cognitive and metacognitive prompts. Learn. Instr. 17 , 564–577 (2007).
Bannert, M. & Mengelkamp, C. Scaffolding hypermedia learning through metacognitive prompts. In International Handbook of Metacognition and Learning Technologies Vol. 28 (eds Azevedo, R. & Aleven, V.) 171–186 (Springer New York, 2013).
Bannert, M., Sonnenberg, C., Mengelkamp, C. & Pieger, E. Short- and long-term effects of students’ self-directed metacognitive prompts on navigation behavior and learning performance. Comput. Hum. Behav. 52 , 293–306 (2015).
McCrindle, A. R. & Christensen, C. A. The impact of learning journals on metacognitive and cognitive processes and learning performance. Learn. Instr. 5 , 167–185 (1995).
Connor-Greene, P. A. Making connections: evaluating the effectiveness of journal writing in enhancing student learning. Teach. Psychol. 27 , 44–46 (2000).
Wong, B. Y. L., Kuperis, S., Jamieson, D., Keller, L. & Cull-Hewitt, R. Effects of guided journal writing on students’ story understanding. J. Educ. Res. 95 , 179–191 (2002).
Nückles, M., Schwonke, R., Berthold, K. & Renkl, A. The use of public learning diaries in blended learning. J. Educ. Media 29 , 49–66 (2004).
Cantrell, R. J., Fusaro, J. A. & Dougherty, E. A. Exploring the effectiveness of journal writing on learning social studies: a comparative study. Read. Psychol. 21 , 1–11 (2000).
Blair, C. Executive function and early childhood education. Curr. Opin. Behav. Sci. 10 , 102–107 (2016).
Clements, D. H., Sarama, J., Unlu, F. & Layzer, C. The Efficacy of an Intervention Synthesizing Scaffolding Designed to Promote Self-Regulation with an Early Mathematics Curriculum: Effects on Executive Function (Society for Research on Educational Effectiveness, 2012).
Newman, S. D., Carpenter, P. A., Varma, S. & Just, M. A. Frontal and parietal participation in problem solving in the Tower of London: fMRI and computational modeling of planning and high-level perception. Neuropsychologia 41 , 1668–1682 (2003).
Sedlmeier, P. et al. The psychological effects of meditation: a meta-analysis. Psychol. Bull. 138 , 1139 (2012).
Bellon, E., Fias, W., Ansari, D. & Smedt, B. D. The neural basis of metacognitive monitoring during arithmetic in the developing brain. Hum. Brain Mapp. 41 , 4562–4573 (2020).
Download references
Acknowledgements
We would like to thank the University of Amsterdam for supporting this research through the Interdisciplinary Doctorate Agreement grant. W.v.d.B. is further supported by the Jacobs Foundation, European Research Council (grant no. ERC-2018-StG-803338), the European Union Horizon 2020 research and innovation programme (grant no. DiGYMATEX-870578), and the Netherlands Organization for Scientific Research (grant no. NWO-VIDI 016.Vidi.185.068).
Author information
Authors and affiliations.
Informatics Institute, University of Amsterdam, Amsterdam, the Netherlands
Damien S. Fleur & Bert Bredeweg
Departement of Psychology, University of Amsterdam, Amsterdam, the Netherlands
Damien S. Fleur & Wouter van den Bos
Faculty of Education, Amsterdam University of Applied Sciences, Amsterdam, the Netherlands
Bert Bredeweg
Center for Adaptive Rationality, Max Planck Institute for Human Development, Berlin, Germany
Wouter van den Bos
You can also search for this author in PubMed Google Scholar
Contributions
D.S.F., B.B. and W.v.d.B. conceived the main conceptual idea of this review article. D.S.F. wrote the manuscript with inputs from and under the supervision of B.B. and W.v.d.B.
Corresponding author
Correspondence to Damien S. Fleur .
Ethics declarations
Competing interests.
The authors declare no competing interests.
Additional information
Publisher’s note Springer Nature remains neutral with regard to jurisdictional claims in published maps and institutional affiliations.
Supplementary information
Supplementary materials, rights and permissions.
Open Access This article is licensed under a Creative Commons Attribution 4.0 International License, which permits use, sharing, adaptation, distribution and reproduction in any medium or format, as long as you give appropriate credit to the original author(s) and the source, provide a link to the Creative Commons license, and indicate if changes were made. The images or other third party material in this article are included in the article’s Creative Commons license, unless indicated otherwise in a credit line to the material. If material is not included in the article’s Creative Commons license and your intended use is not permitted by statutory regulation or exceeds the permitted use, you will need to obtain permission directly from the copyright holder. To view a copy of this license, visit http://creativecommons.org/licenses/by/4.0/ .
Reprints and permissions
About this article
Cite this article.
Fleur, D.S., Bredeweg, B. & van den Bos, W. Metacognition: ideas and insights from neuro- and educational sciences. npj Sci. Learn. 6 , 13 (2021). https://doi.org/10.1038/s41539-021-00089-5
Download citation
Received : 06 October 2020
Accepted : 09 April 2021
Published : 08 June 2021
DOI : https://doi.org/10.1038/s41539-021-00089-5
Share this article
Anyone you share the following link with will be able to read this content:
Sorry, a shareable link is not currently available for this article.
Provided by the Springer Nature SharedIt content-sharing initiative
This article is cited by
Relation of life sciences students’ metacognitive monitoring to neural activity during biology error detection.
- Mei Grace Behrendt
- Carrie Clark
- Joseph Dauer
npj Science of Learning (2024)
The many facets of metacognition: comparing multiple measures of metacognition in healthy individuals
- Anneke Terneusen
- Conny Quaedflieg
- Ieke Winkens
Metacognition and Learning (2024)
Towards a common conceptual space for metacognition in perception and memory
- Audrey Mazancieux
- Michael Pereira
- Céline Souchay
Nature Reviews Psychology (2023)
Predictive Validity of Performance-Based Metacognitive Testing is Superior to Self-report: Evidence from Undergraduate Freshman Students
- Marcio Alexander Castillo-Diaz
- Cristiano Mauro Assis Gomes
Trends in Psychology (2023)
Normative data and standardization of an international protocol for the evaluation of metacognition in Spanish-speaking university students: A cross-cultural analysis
- Antonio P. Gutierrez de Blume
- Diana Marcela Montoya Londoño
- Jesus Rivera-Sanchez
Metacognition and Learning (2023)
Quick links
- Explore articles by subject
- Guide to authors
- Editorial policies
Sign up for the Nature Briefing newsletter — what matters in science, free to your inbox daily.

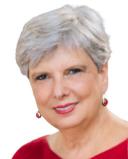
What Is Metacognition? How Does It Help Us Think?
Metacognitive strategies like self-reflection empower students for a lifetime..
Posted October 9, 2020 | Reviewed by Abigail Fagan
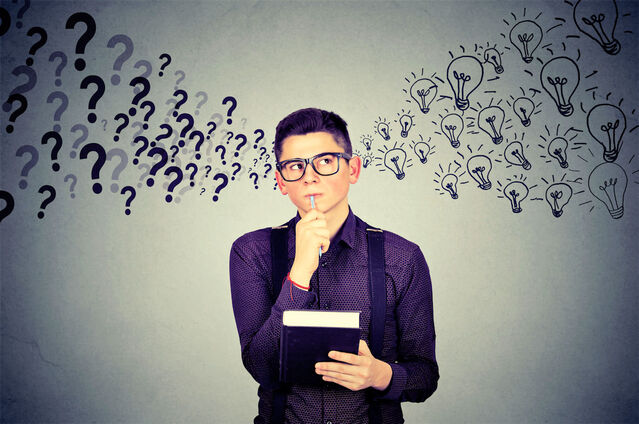
Metacognition is a high order thinking skill that is emerging from the shadows of academia to take its rightful place in classrooms around the world. As online classrooms extend into homes, this is an important time for parents and teachers to understand metacognition and how metacognitive strategies affect learning. These skills enable children to become better thinkers and decision-makers.
Metacognition: The Neglected Skill Set for Empowering Students is a new research-based book by educational consultants Dr. Robin Fogarty and Brian Pete that not only gets to the heart of why metacognition is important but gives teachers and parents insightful strategies for teaching metacognition to children from kindergarten through high school. This article summarizes several concepts from their book and shares three of their thirty strategies to strengthen metacognition.
What Is Metacognition?
Metacognition is the practice of being aware of one’s own thinking. Some scholars refer to it as “thinking about thinking.” Fogarty and Pete give a great everyday example of metacognition:
Think about the last time you reached the bottom of a page and thought to yourself, “I’m not sure what I just read.” Your brain just became aware of something you did not know, so instinctively you might reread the last sentence or rescan the paragraphs of the page. Maybe you will read the page again. In whatever ways you decide to capture the missing information, this momentary awareness of knowing what you know or do not know is called metacognition.
When we notice ourselves having an inner dialogue about our thinking and it prompts us to evaluate our learning or problem-solving processes, we are experiencing metacognition at work. This skill helps us think better, make sound decisions, and solve problems more effectively. In fact, research suggests that as a young person’s metacognitive abilities increase, they achieve at higher levels.
Fogarty and Pete outline three aspects of metacognition that are vital for children to learn: planning, monitoring, and evaluation. They convincingly argue that metacognition is best when it is infused in teaching strategies rather than taught directly. The key is to encourage students to explore and question their own metacognitive strategies in ways that become spontaneous and seemingly unconscious .
Metacognitive skills provide a basis for broader, psychological self-awareness , including how children gain a deeper understanding of themselves and the world around them.
Metacognitive Strategies to Use at Home or School
Fogarty and Pete successfully demystify metacognition and provide simple ways teachers and parents can strengthen children’s abilities to use these higher-order thinking skills. Below is a summary of metacognitive strategies from the three areas of planning, monitoring, and evaluation.
1. Planning Strategies
As students learn to plan, they learn to anticipate the strengths and weaknesses of their ideas. Planning strategies used to strengthen metacognition help students scrutinize plans at a time when they can most easily be changed.
One of ten metacognitive strategies outlined in the book is called “Inking Your Thinking.” It is a simple writing log that requires students to reflect on a lesson they are about to begin. Sample starters may include: “I predict…” “A question I have is…” or “A picture I have of this is…”
Writing logs are also helpful in the middle or end of assignments. For example, “The homework problem that puzzles me is…” “The way I will solve this problem is to…” or “I’m choosing this strategy because…”
2. Monitoring Strategies
Monitoring strategies used to strengthen metacognition help students check their progress and review their thinking at various stages. Different from scrutinizing, this strategy is reflective in nature. It also allows for adjustments while the plan, activity, or assignment is in motion. Monitoring strategies encourage recovery of learning, as in the example cited above when we are reading a book and notice that we forgot what we just read. We can recover our memory by scanning or re-reading.
One of many metacognitive strategies shared by Fogarty and Pete, called the “Alarm Clock,” is used to recover or rethink an idea once the student realizes something is amiss. The idea is to develop internal signals that sound an alarm. This signal prompts the student to recover a thought, rework a math problem, or capture an idea in a chart or picture. Metacognitive reflection involves thinking about “What I did,” then reviewing the pluses and minuses of one’s action. Finally, it means asking, “What other thoughts do I have” moving forward?

Teachers can easily build monitoring strategies into student assignments. Parents can reinforce these strategies too. Remember, the idea is not to tell children what they did correctly or incorrectly. Rather, help children monitor and think about their own learning. These are formative skills that last a lifetime.
3. Evaluation Strategies
According to Fogarty and Pete, the evaluation strategies of metacognition “are much like the mirror in a powder compact. Both serve to magnify the image, allow for careful scrutiny, and provide an up-close and personal view. When one opens the compact and looks in the mirror, only a small portion of the face is reflected back, but that particular part is magnified so that every nuance, every flaw, and every bump is blatantly in view.” Having this enlarged view makes inspection much easier.
When students inspect parts of their work, they learn about the nuances of their thinking processes. They learn to refine their work. They grow in their ability to apply their learning to new situations. “Connecting Elephants” is one of many metacognitive strategies to help students self-evaluate and apply their learning.
In this exercise, the metaphor of three imaginary elephants is used. The elephants are walking together in a circle, connected by the trunk and tail of another elephant. The three elephants represent three vital questions: 1) What is the big idea? 2) How does this connect to other big ideas? 3) How can I use this big idea? Using the image of a “big idea” helps students magnify and synthesize their learning. It encourages them to think about big ways their learning can be applied to new situations.
Metacognition and Self-Reflection
Reflective thinking is at the heart of metacognition. In today’s world of constant chatter, technology and reflective thinking can be at odds. In fact, mobile devices can prevent young people from seeing what is right before their eyes.
John Dewey, a renowned psychologist and education reformer, claimed that experiences alone were not enough. What is critical is an ability to perceive and then weave meaning from the threads of our experiences.
The function of metacognition and self-reflection is to make meaning. The creation of meaning is at the heart of what it means to be human.
Everyone can help foster self-reflection in young people.
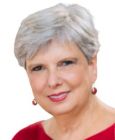
Marilyn Price-Mitchell, Ph.D., is an Institute for Social Innovation Fellow at Fielding Graduate University and author of Tomorrow’s Change Makers.
- Find a Therapist
- Find a Treatment Center
- Find a Psychiatrist
- Find a Support Group
- Find Online Therapy
- United States
- Brooklyn, NY
- Chicago, IL
- Houston, TX
- Los Angeles, CA
- New York, NY
- Portland, OR
- San Diego, CA
- San Francisco, CA
- Seattle, WA
- Washington, DC
- Asperger's
- Bipolar Disorder
- Chronic Pain
- Eating Disorders
- Passive Aggression
- Personality
- Goal Setting
- Positive Psychology
- Stopping Smoking
- Low Sexual Desire
- Relationships
- Child Development
- Therapy Center NEW
- Diagnosis Dictionary
- Types of Therapy
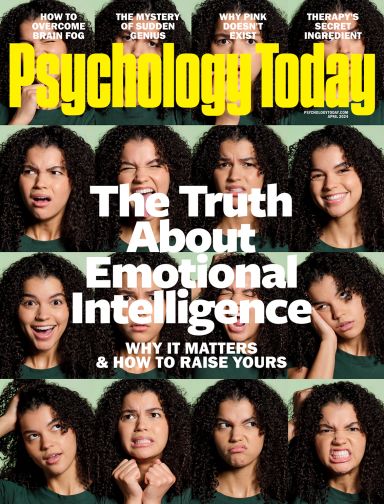
Understanding what emotional intelligence looks like and the steps needed to improve it could light a path to a more emotionally adept world.
- Emotional Intelligence
- Gaslighting
- Affective Forecasting
- Neuroscience
Change Password
Your password must have 8 characters or more and contain 3 of the following:.
- a lower case character,
- an upper case character,
- a special character
Password Changed Successfully
Your password has been changed
- Sign in / Register
Request Username
Can't sign in? Forgot your username?
Enter your email address below and we will send you your username
If the address matches an existing account you will receive an email with instructions to retrieve your username
Metacognition and Self-Efficacy in Action: How First-Year Students Monitor and Use Self-Coaching to Move Past Metacognitive Discomfort During Problem Solving
- Stephanie M. Halmo
- Kira A. Yamini
- Julie Dangremond Stanton
Department of Cellular Biology, University of Georgia, Athens, GA 30602
Search for more papers by this author
*Address correspondence to: Julie Dangremond Stanton ( E-mail Address: [email protected] ).
Stronger metacognitive regulation skills and higher self-efficacy are linked to increased academic achievement. Metacognition and self-efficacy have primarily been studied using retrospective methods, but these methods limit access to students’ in-the-moment metacognition and self-efficacy. We investigated first-year life science students’ metacognition and self-efficacy while they solved challenging problems, and asked: 1) What metacognitive regulation skills are evident when first-year life science students solve problems on their own? and 2) What aspects of learning self-efficacy do first-year life science students reveal when they solve problems on their own? Think-aloud interviews were conducted with 52 first-year life science students across three institutions and analyzed using content analysis. Our results reveal that while first-year life science students plan, monitor, and evaluate when solving challenging problems, they monitor in a myriad of ways. One aspect of self-efficacy, which we call self-coaching, helped students move past the discomfort of monitoring a lack of understanding so they could take action. These verbalizations suggest ways we can encourage students to couple their metacognitive skills and self-efficacy to persist when faced with challenging problems. Based on our findings, we offer recommendations for helping first-year life science students develop and strengthen their metacognition to achieve improved problem-solving performance.
INTRODUCTION
Have you ever asked a student to solve a problem, seen their solution, and then wondered what they were thinking while they were problem solving? As college instructors, we often ask students in our classes to solve problems. Sometimes we gain access to our students’ thought process or cognition through strategic question design and direct prompting. Far less often we gain access to how our students regulate and control their own thinking (metacognition) or their beliefs about their capability to solve the problem (self-efficacy). Retrospective methods can and have been used to access this information from students, but students often cannot remember what they were thinking a week or two later. We lack deep insight into students’ in-the-moment metacognition and self-efficacy because it is challenging to obtain their in-the-moment thoughts.
Educators and students alike are interested in metacognition because of its malleable nature and demonstrated potential to improve academic performance. Not having access to students’ metacognition in-the-moment presents a barrier towards developing effective metacognitive interventions to improve learning. Thus, there is a need to characterize how life science undergraduates use their metacognition during individual problem-solving and to offer evidence-based suggestions to instructors for supporting students’ metacognition. In particular, understanding the metacognitive skills first-year life science students bring to their introductory courses will position us to better support their learning earlier on in their college careers and set them up for future academic success.
Metacognition and Problem Solving
Metacognition, or one’s awareness and control of their own thinking for the purpose of learning ( Cross and Paris, 1988 ), is linked to improved problem-solving performance and academic achievement. In one meta-analysis of studies that spanned developmental stages from elementary school to adulthood, metacognition predicted academic performance when controlling for intelligence ( Ohtani and Hisasaka, 2018 ). In another meta-analysis specific to mathematics, researchers found a significant positive correlation between metacognition and math performance in adolescences, indicating individuals who demonstrated stronger metacognition also performed better on math tasks ( Muncer et al. , 2022 ). The strong connection between metacognition and problem-solving performance and academic achievement represents a potential leverage point for enhancing student learning and success in the life sciences. If we explicitly teach life science undergraduates how to develop and use their metacognition, we can expect to increase the effectiveness of their learning and subsequent academic success. However, in order to provide appropriate guidance, we must first know how students in the target population are employing their metacognition.
Based on one theoretical framework of metacognition, metacognition is comprised of two components: metacognitive knowledge and metacognitive regulation ( Schraw and Moshman, 1995 ). Metacognitive knowledge includes one’s awareness of learning strategies and of themselves as a learner. Metacognitive regulation encompasses how students act on their metacognitive knowledge or the actions they take to learn ( Sandi-Urena et al. , 2011 ). Metacognitive regulation is broken up into three skills: 1) planning how to approach a learning task or goal, 2) monitoring progress towards achieving that learning task or goal, and 3) evaluating achievement of said learning task or goal ( Stanton et al. , 2021 ). These regulation skills can be thought of temporally: planning occurs before learning starts, monitoring occurs during learning, and evaluating takes place after learning has occurred. As biology education researchers, we are particularly interested in life science undergraduates’ metacognitive regulation skills or the actions they take to learn because regulation skills have been shown to have a more dramatic impact on learning than awareness alone ( Dye and Stanton, 2017 ).
Importantly, metacognition is context-dependent, meaning metacognition use may vary depending on factors such as the subject matter or learning task ( Kelemen et al. , 2000 ; Kuhn, 2000 ; Veenman and Spaans, 2005 ). For example, the metacognitive regulation skills a student may use to evaluate their learning after reading a text in their literature course may differ from those skills the same student uses to evaluate their learning on a genetics exam. This is why it is imperative to study metacognition in a particular context, like problem solving in the life sciences.
Metacognition helps a problem solver identify and work with the givens or initial problem state, reach the goal or final problem state, and overcome any obstacles presented in the problem ( Davidson and Sternberg, 1998 ). Specifically, metacognitive regulation skills help a solver select strategies, identify obstacles, and revise their strategies to accomplish a goal. Metacognition and problem solving are often thought of as domain-general skills because of their broad applicability across different disciplines. However, metacognitive skills are first developed in a domain-specific way and then those metacognitive skills can become more generalized over time as they are further developed and honed ( Kuhn, 2000 ; Veenman and Spaans, 2005 ). This is in alignment with research from the problem-solving literature that suggests stronger problem-solving skills are a result of deep knowledge within a domain ( Pressley et al. , 1987 ; Frey et al. , 2022 ). For example, experts are known to classify problems based on deep conceptual features because of their well-developed knowledge base whereas novices tend to classify problems based on superficial features ( Chi et al. , 1981 ). Research on problem solving in chemistry indicates that metacognition and self-efficacy are two key components of successful problem solving ( Rickey and Stacy, 2000 ; Taasoobshirazi and Glynn, 2009 ). College students who achieve greater problem-solving success are those who: 1) use their metacognition to conceptualize problems well, select appropriate strategies, and continually monitor and check their work, and 2) tend to have higher self-efficacy ( Taasoobshirazi and Glynn, 2009 ; Cartrette and Bodner, 2010 ).
Metacognition and Self-efficacy
Self-efficacy, or one’s belief in their capability to carry out a task ( Bandura, 1977 , 1997 ), is another construct that impacts problem solving performance and academic achievement. Research on self-efficacy has revealed its predictive power in regards to performance, academic achievement, and selection of a college major ( Pajares, 1996 ). The large body of research on self-efficacy suggests that students who believe they are capable academically, engage more metacognitive strategies and persist to obtain academic achievement compared with those who do not (e.g., Pintrich and De Groot, 1990 ; Pajares, 2002 ; Huang et al. , 2022 ). In STEM in particular, studies tend to reveal gender differences in self-efficacy with undergraduate men indicating higher self-efficacy in STEM disciplines compared with women ( Stewart et al. , 2020 ). In one study of first-year biology students, women were significantly less confident than men and students’ biology self-efficacy increased over the course of a single semester when measured at the beginning and end of the course ( Ainscough et al. , 2016 ). However, self-efficacy is known to be a dynamic construct, meaning one’s perception of their capability to carry out a task can vary widely across different task types and over time as struggles are encountered and expertise builds for certain tasks ( Yeo and Neal, 2006 ).
Both metacognition and self-efficacy are strong predictors of academic achievement and performance. For example, one study found that students with stronger metacognitive regulation skills and greater self-efficacy beliefs (as measured by self-reported survey responses) perform better and attain greater academic success (as measured by GPA; Coutinho and Neuman, 2008 ). Additionally, self-efficacy beliefs were strong predictors of metacognition, suggesting students with higher self-efficacy used more metacognition. Together, the results from this quantitative study using structural equation modeling of self-reported survey responses suggests that metacognition may act as a mediator in the relationship between self-efficacy and academic achievement ( Coutinho and Neuman, 2008 ).
Most of the research on self-efficacy has been quantitative in nature. In one qualitative study of self-efficacy, interviews were conducted with middle school students to explore the sources of their mathematics self-efficacy beliefs ( Usher, 2009 ). In this study, evidence of self-modeling was found. Self-modeling or visualizing one’s own self-coping during difficult tasks can strengthen one’s belief in their capabilities and can be an even stronger source of self-efficacy than observing a less similar peer succeed ( Bandura, 1997 ). Usher (2009) described self-modeling as students’ internal dialogues or what they say to themselves while doing mathematics. For example, students would tell themselves they can do it and that they would do okay as a way of keeping their confidence up or coaching themselves while doing mathematics. Other researchers have called this efficacy self-talk, or “thoughts or subvocal statements aimed at influencing their efficacy for an ongoing academic task” ( Wolters, 2003 , p. 199). For example, one study found that college students reported saying things to themselves like “You can do it, just keep working” in response to an open-ended questionnaire about how they would maintain effort on a given task ( Wolters, 1998 ; Wolters, 2003 ). As qualitative researchers, we were curious to uncover how both metacognition (planning, monitoring, and evaluating) and self-efficacy (such as self-coaching) might emerge out of more qualitative, in-the-moment data streams.
Methods for Studying Metacognition
Researchers use two main methods to study metacognition: retrospective and in-the-moment methods. Retrospective methods ask learners to reflect on learning they’ve done in the past. In contrast, in-the-moment methods ask learners to reflect on learning they’re currently undertaking ( Veenman et al. , 2006 ). Retrospective methods include self-report data from surveys like the Metacognitive Awareness Inventory ( Schraw and Dennison, 1994 ) or exam “wrappers” or self-evaluations ( Hodges et al. , 2020 ). Whereas in-the-moment methods include think-aloud interviews, which ask students to verbalize all of their thoughts while they solve problems ( Bannert and Mengelkamp, 2008 ; Ku and Ho, 2010 ; Blackford et al. , 2023 ), or online computer chat log-files as groups of students work together to solve problems ( Hurme et al. , 2006 ; Zheng et al. , 2019 ).
Most metacognition research on life science undergraduates, including our own work, has utilized retrospective methods ( Stanton et al. , 2015 , 2019; Dye and Stanton, 2017 ). Important information about first-year life science students’ metacognition has been gleaned using retrospective methods, particularly in regard to planning and evaluating. For example, first-year life science students tend to use strategies that worked for them in high school, even if they do not work for them in college, suggesting first-year life science students may have trouble evaluating their study plans ( Stanton et al. , 2015 ). Additionally, first-year life science students abandon strategies they deem ineffective rather than modifying them for improvement ( Stanton et al. , 2019 ). Lastly, first-year life science students are willing to change their approach to learning, but they may lack knowledge about which approaches are effective or evidence-based ( Tomanek and Montplaisir, 2004 ; Stanton et al. , 2015 ).
In both of the meta-analyses described at the start of this Introduction , the effect sizes were larger for studies that used in-the-moment methods ( Ohtani and Hisasaka, 2018 ; Muncer et al. , 2022 ). This means the predictive power of metacognition for academic performance was more profound for studies that used in-the-moment methods to measure metacognition compared with studies that used retrospective methods. One implication of this finding is that studies using retrospective methods might be failing to capture metacognition’s profound effects on learning and performance. Less research has been done using in-the-moment methods to study metacognition in life science undergraduates likely because of the time-intensive nature of collecting and analyzing data using these methods. One study that used think-aloud methods to investigate biochemistry students’ metacognition when solving open-ended buffer problems found that monitoring was the most commonly used metacognitive regulation skill ( Heidbrink and Weinrich, 2021 ). Another study that used think-aloud methods to explore Dutch third-year medical school students’ metacognition when solving physiology problems about blood flow also revealed a focus on monitoring, with students also planning and evaluating but to a lesser extent ( Versteeg et al. , 2021 ). We hypothesize that in-the-moment methods like think-aloud interviews are likely to reveal greater insight into students monitoring skills because this metacognitive regulation skill occurs during learning tasks. Further investigation into the nature of the metacognition first-year life science students use when solving problems is needed in order to provide guidance to this population and their instructors on how to effectively use and develop their metacognitive regulation skills.
Research Questions
What metacognitive regulation skills are evident when first-year life science students solve problems on their own, what aspects of learning self-efficacy do first-year life science students reveal when they solve problems on their own, research participants & context.
This study is a part of a larger longitudinal research project investigating the development of metacognition in life science undergraduates which was classified by the Institutional Review Board at the University of Georgia (STUDY00006457) and University of North Georgia (2021-003) as exempt. For that project, 52 first-year students at three different institutions in the southeastern United States were recruited from their introductory biology or environmental science courses in the 2021–2022 academic year. Data was collected at three institutions to represent different academic environments because it is known that context can affect metacognition ( Table 1 ). Georgia Gwinnett College is classified as a baccalaureate college predominantly serving undergraduate students, University of Georgia is classified as doctoral R1 institution, and University of North Georgia is classified as a master’s university. Additionally, in our past work we found that first-year students from different institutions differed in their metacognitive skills ( Stanton et al. , 2015 , 2019). Our goal in collecting data from three different institution types was to ensure our qualitative study could be more generalizable than if we had only collected data from one institution.
Students at each institution were invited to complete a survey to provide their contact information, answer the revised 19-item Metacognitive Awareness Inventory ( Harrison and Vallin, 2018 ), 32-item Epistemic Beliefs Inventory ( Schraw et al. , 1995 ), and 8-item Self-efficacy for Learning and Performance subscale from the Motivated Strategies for Learning Questionnaire (MSLQ; Pintrich et al. , 1993 ). They were also asked to self-report their demographic information including their age, gender, race/ethnicity, college experience, intended major, and first-generation status. First-year students who were 18 years or older and majoring in the life sciences were invited to participate in the larger study. We used purposeful sampling to select a sample that matched the demographics of the student body at each institution and also represented a range in metacognitive ability based on students’ responses to the revised Metacognitive Awareness Inventory ( Harrison and Vallin, 2018 ). In total, eight students from Georgia Gwinnett College, 23 students from the University of Georgia, and 21 students from the University of North Georgia participated in the present study ( Table 2 ). Participants received $40 (either in the form of a mailed check, or an electronic Starbucks or Amazon gift card) for their participation in Year 1 of the larger longitudinal study. Their participation in Year 1 included completing the survey, three inventories, and a 2-hour interview, of which the think aloud interview was one quarter of the total interview.
Note: We are using Ebony McGee’s rephrasing of URM as underrepresented racially minoritized groups ( McGee, 2020 ). In our work this means students who self-reported as Black or African American or Hispanic or Latinx. For average high school GPA, institutional data is missing from two GGC students.
Data Collection
All interviews were conducted over Zoom during the 2021–2022 academic year when participants had returned to the classroom. Participants ( n = 52) were asked to think aloud as they solved two challenging biochemistry problems ( Figure 1 ) that have been previously published ( Halmo et al. , 2018 , 2020; Bhatia et al. , 2022 ). We selected two challenging biochemistry problems for first-year students to solve because we know that students do not use metacognition unless they find a learning task challenging ( Carr and Taasoobshirazi, 2008 ). If the problems were easy, they may have solved them quickly without needing to use their metacognition or by employing metacognition that is so automatic they may have a hard time verbalizing it ( Samuels et al. , 2005 ). By having students solve problems we knew would be challenging, we hoped this would trigger them to use and verbalize their metacognition during their problem-solving process. This would enable us to study how they used their metacognition and what they did in response to their metacognition. The problems we selected met this criterion because participants had not yet taken biochemistry.
FIGURE 1. Think-Aloud Problems. Students were asked to think aloud as they solved two challenging biochemistry problems. Panel A depicts the Protein X Problem previously published in Halmo et al. , 2018 and 2020. Panel B depicts the Pathway Flux problem previously published in Bhatia et al. , 2022 . Both problems are open-ended and ask students to make predictions and provide scientific explanations for their predictions.
The problems were open-ended and asked students to make predictions and provide scientific explanations for their predictions about: 1) noncovalent interactions in a folded protein for the Protein X Problem ( Halmo et al. , 2018 , 2020) and 2) negative feedback regulation in a metabolic pathway for the Pathway Flux Problem ( Bhatia et al. , 2022 ). Even though the problems were challenging, we made it clear to students before they began that we were not interested in the correctness of their solutions but rather we were genuinely interested in their thought process. To elicit student thinking after participants fell silent for more than 5 seconds, interviewers used the following two prompts: “What are you thinking (now)?” and “Can you tell me more about that?” ( Ericsson and Simon, 1980 ; Charters, 2003 ). After participants solved the problems, they shared their written solutions with the interviewer using the chat feature in Zoom. Participants were then asked to describe their problem-solving process out loud and respond to up to four reflection questions (see Supplemental Material for full interview protocol). The think-aloud interviews were audio and video recorded and transcribed using a professional, machine-generated transcription service (Temi.com). All transcripts were checked for accuracy by members of the research team before analysis began.
Data Analysis
The resulting transcripts were analyzed by a team of three researchers in three cycles. In the first cycle of data analysis, half of the transcripts were open coded by members of the research team (S.M.H., J.D.S., and K.A.Y.). S.M.H. entered this analysis as a postdoctoral researcher in biology education research with experience in qualitative methods and deep knowledge about student difficulties with the two problems students were asked to solve in this study. J.D.S., an associate professor of cell biology and a biology education researcher, entered this analysis as an educator and metacognition researcher with extensive experience in qualitative methods. K.A.Y. entered this analysis as an undergraduate student double majoring in biology and psychology and as an undergraduate researcher relatively new to qualitative research. During this open coding process, we individually reflected on the contents of the data, remained open to possible directions suggested by our interpretation of the data, and recorded our initial observations using analytic memos ( Saldaña, 2021 ). The research team (S.M.H., J.D.S., and K.A.Y.) then met to discuss our observations from the open coding process and suggest possible codes that were aligned with our observations, knowledge of metacognition and self-efficacy, and our guiding research questions. This discussion led to the development of an initial codebook consisting of inductive codes discerned from the data and deductive codes derived from theory on metacognition and self-efficacy. In the second cycle of data analysis, the codebook was applied to the dataset iteratively by two researchers (S.M.H. and K.A.Y.) using MaxQDA2020 software (VERBI Software; Berlin, Germany) until the codebook stabilized or no new codes or modifications to existing codes were needed. Coding disagreements between the two coders were discussed by all three researchers until consensus was reached. All transcripts were coded to consensus to identify aspects of metacognition and learning self-efficacy that were verbalized by participants. Coding to consensus allowed the team to consider and discuss their diverse interpretations of the data and ensure trustworthiness of the analytic process ( Tracy, 2010 ; Pfeifer and Dolan, 2023 ). In the third and final cycle of analysis, thematic analysis was used to uncover central themes in our dataset. As a part of thematic analysis, two researchers (S.M.H. and K.A.Y.) synthesized one-sentence summaries of each participant’s think aloud interview. Student quotes presented in the Results & Discussion have been lightly edited for clarity, and all names are pseudonyms.
Problem-Solving Performance as One Context for Studying Metacognition
To compare the potential effect of institution and gender on problem solving performance, we scored the final problem solutions, and then interrogated them using R Statistical Software (R Core Team, 2021 ). A one-way ANOVA was performed to compare the effect of institution on problem-solving performance. This analysis revealed that there was not a statistically significant difference in problem-solving performance between the three institutions (F[2, 49] = 0.085, p = 0.92). This indicates students performed similarly on the problems regardless of which institution they attended (Supplemental Data, Table 1 ). Another one-way ANOVA was performed to compare the effect of gender on problem-solving performance which revealed no statistically significant differences in problem-solving performance based on gender (F[1, 50] = 0.956, p = 0.33). Students performed similarly on the problems regardless of their gender (Supplemental Data, Table 2 ). Taken together, this analysis suggests a homogeneous sample in regard to problem-solving performance.
Participants’ final problem solutions were individually scored by two researchers (S.M.H. and K.A.Y.) using an established rubric and scores were discussed until complete consensus was reached. The rubric used to score the problems is available from the corresponding author upon request. The median problem-solving performance of students in our sample was two points on a 10-point rubric. Students in our sample scored low on the rubric because they either failed to answer part of the problem or struggled to provide accurate explanations or evidence to support their predictions. Despite the phrase “provide a scientific explanation to support your prediction” included in the prompt, most students’ solutions contained a prediction, but lacked an explanation. For example, the majority of the solutions for the Protein X problem predicted the noncovalent interaction would be affected by the substitution, but lacked categorization of the relevant amino acids or identification of the noncovalent interactions involved, which are critical problem-solving steps for this problem ( Halmo et al. , 2018 , 2020). The majority of the Pathway Flux solutions also predicted that flux would be affected, but lacked an accurate description of negative feedback inhibition or regulation release of the pathway, which are critical features of this problem ( Bhatia et al. , 2022 ). This lack of accurate explanations is not unexpected. Previous work shows that both introductory biology and biochemistry students struggle to provide accurate explanations to these problems without pedagogical support, and introductory biology students generally struggle more than biochemistry students ( Bhatia et al. , 2022 ; Lemons, personal communication).
RESULTS AND DISCUSSION
To address our first research question, we looked for statements and questions related to the three skills of planning, monitoring, and evaluating in our participants’ think aloud data. Because metacognitive regulation skills encompass how students act on their metacognitive awareness, participants’ explicit awareness was a required aspect when analyzing our data for these skills. For example, the statement “this is a hydrogen bond” does not display awareness of one’s knowledge but rather the knowledge itself (cognition). In contrast, the statement “I know this is a hydrogen bond” does display awareness of one’s knowledge and is therefore considered evidence of metacognition. We found evidence of all three metacognitive regulation skills in our data. First-year life science students plan, monitor, and evaluate when solving challenging problems. However, our data collection method revealed more varied ways in which students monitor. We present our findings for each metacognitive regulation skill ( Table 3 ). For further demonstration of how students use these skills in concert when problem solving, we offer problem-solving vignettes of a student from each institution in Supplemental Data .
Planning: Students did not plan before solving but did assess the task in the moment
Planning how to approach the task of solving problems individually involves selecting strategies to use and when to use them before starting the task ( Stanton et al. , 2021 ). Planning did not appear in our data in a classical sense. This finding is unsurprising because the task was: 1) well-defined, meaning there were a few potentially accurate solutions rather than an abundant number of accurate solutions, 2) straightforward meaning the goal of solving the problem was clearly stated, and 3) relatively short meaning students were not entering and exiting from the task multiple times like they might when studying for an exam. Additionally, the stakes were comparatively low meaning task completion and performance carried little to no weight in participants’ college careers. In other data from this same sample, we know that these participants make plans for high-stakes assessments like exams but often admit to not planning for lower stakes assessments like homework (Stanton, personal communication). Related to the skill of planning, we observed students assessing the task after reading the problem ( Table 3 ). We describe how students assessed the task and provide descriptions of what happened after students planned in this way.
Assessing the task
While we did not observe students explicitly planning their approach to problem solving before beginning the task, we did observe students assessing the task or what other researchers have called “orientation” after reading the problems ( Meijer et al. , 2006 ; Schellings et al. , 2013 ). Students in our study either assessed the task successfully or unsuccessfully. For example, when Gerald states, “So I know that not only do I have to give my answer, but I also have to provide information on how I got my answer …” he successfully identified what the problem was asking him to do by providing a scientific explanation. In contrast, Simone admits her struggle with figuring out what the problem is asking when she states, “I’m still trying to figure out what the question’s asking. I don’t want to give up on this question just yet, but yeah, it’s just kinda hard because I can ’ t figure out what the question is asking me if I don’t know the terminology behind it.” In Simone’s case, the terminology she struggled to understand is what was meant by a scientific explanation. Assessing the task unsuccessfully also involved misinterpreting what the problem asked. This was a frequent issue for students in our sample during the Pathway Flux problem because students inaccurately interpreted the negative feedback loop, which is a known problematic visual representation in biochemistry ( Bhatia et al. , 2022 ). For example, students like Paulina and Kathleen misinterpreted the negative feedback loop as enzyme B no longer functioning when they stated, respectively, “So if enzyme B is taken out of the graph…” , or “…if B cannot catalyze…” Additionally some students misinterpreted the negative feedback loop as a visual cue of the change described in the problem prompt (IV-CoA can no longer bind to enzyme B). This can be seen in the following example quote from Mila: “So I was looking at it and I see what they’re talking about with the IV-CoA no longer binding to enzyme B and I think that’s what that arrow with the circle and the line through it is representing. It’s just telling me that it’s not binding to enzyme B.”
What happened after assessing the task?
Misinterpretations of what the problem was asking like those shared above from Simone, Paulina, Kathleen, and Mila led to inaccurate answers for the Pathway Flux problem. In contrast, when students like Gerald could correctly interpret what the problem asked them to do, this led to more full and accurate answers for both problems. Accurately interpreting what a problem is asking you to do is critical for problem-solving success. A related procedural error identified in other research on written think-aloud protocols from students solving multiple-choice biology problems was categorized as misreading ( Prevost and Lemons, 2016 ).
Implications for Instruction & Research about Planning
In our study, we did not detect evidence of explicit planning beyond assessing the task. This suggests that first-year students’ approaches were either unplanned or automatic ( Samuels et al. , 2005 ). As metacognition researchers and instructors, we find first-year life science students’ absence of planning before solving and presence of assessing the task during solving illuminating. This means planning is likely one area in which we can help first-year life science students grow their metacognitive skills through practice. While we do not anticipate that undergraduate students will be able to plan how to solve a problem that is unfamiliar to them before reading a problem, we do think we can help students develop their planning skills through modeling when solving life science problems.
When modeling problem solving for students, we could make our planning explicit for students by verbalizing how we assess the task and what strategies we plan to use and why. From the problem-solving literature, it is known that experts assess a task by recognizing the deep structure or problem type and what is being asked of them ( Chi et al. , 1981 ; Smith et al. , 2013 ). This likely happens rapidly and automatically for experts through the identification of visual and key word cues. Forcing ourselves to think about what these cues might be and alerting students to them through modeling may help students more rapidly develop expert-level schema, approaches, and planning skills. Providing students with feedback on their assessment of a task and whether or not they misunderstood the problem also seems to be critical for problem-solving success ( Prevost and Lemons, 2016 ). Helping students realize they can plan for smaller tasks like solving a problem by listing the pros and cons of relevant strategies and what order they plan to use selected strategies before they begin could help students narrow the problem solving space, approach the task with focus, and achieve efficiency to become “good strategy users” ( Pressley et al. , 1987 ).
Monitoring: Students monitored in the moment in a myriad of ways
Monitoring progress towards problem-solving involves assessing conceptual understanding during the task ( Stanton et al. , 2021 ). First-year life science students in our study monitored their conceptual understanding during individual problem solving in a myriad of ways. In our analysis, we captured the specific aspects of conceptual understanding students monitored. Students in our sample monitored: 1) relevance, 2) confusion, 3) familiarity, 4) understanding, 5) questions, and 6) correctness ( Table 3 ). We describe each aspect of conceptual understanding that students monitored and we provide descriptions of what happened after students monitored in this way ( Figure 2 ).
FIGURE 2. How monitoring can impact the problem-solving process. The various ways first-year students in this study monitored are depicted as ovals. See Table 3 for detailed descriptions of the ways students monitored. How students in this study acted on their monitoring are shown as rectangles. In most cases, what happened after students monitored determined whether or not problem solving moved forward. Encouraging oneself using positive self-talk, or self-coaching, helped students move past the discomfort associated with monitoring a lack of conceptual understanding (confusion, lack of familiarity, or lack of understanding) and enabled them to use problem-solving strategies, which moved problem solving forward.
Monitoring Relevance
When students monitored relevance, they described what pieces of their own knowledge or aspects of the problem prompts were relevant or irrelevant to their thought process ( Table 3 ). For the Protein X problem, many students monitored the relevance of the provided information about pH. First-year life science students may have focused on this aspect of the problem prompt because pH is a topic often covered in introductory biology classes, which participants were enrolled in at the time of the study. However, students differentially decided whether this information was relevant or irrelevant. Quinn decided this piece of information was relevant: “The pH of the water surrounding it. I think it ’ s important because otherwise it wouldn’t really be mentioned.” In contrast, Ignacio decided the same piece of information was irrelevant: “So the pH has nothing to do with it . The water molecules had nothing to do with it as well. So basically, everything in that first half, everything in that first thing, right there is basically useless. So , I ’ m just going to exclude that information out of my thought process cause the pH has nothing to do with what’s going on right now…” From an instructional perspective, knowing the pH in the Protein X problem is relevant information for determining the ionization state of acidic and basic amino acids, like amino acids D and E shown in the figure, could be helpful. However, this specific problem asked students to consider amino acids A and B, so Ignacio’s decision that the pH was irrelevant may have helped him focus on more central parts of the problem. In addition to monitoring the relevance of the provided information, sometimes students would monitor the relevance of their own knowledge that they brought to bear on the problem. For example, consider the following quote from Regan: “I just think that it might be a hydrogen bond, which has nothing to do with the question .” Regan made this statement during her think aloud for the Protein X problem, which is intriguing because the Protein X problem deals solely with noncovalent interactions like hydrogen bonding.
What happened after monitoring relevance?
Overall, monitoring relevance helped students narrow their focus during problem solving, but could be misleading if done inaccurately like in Regan’s case ( Figure 2 ).
Monitoring Confusion
When students monitored confusion when solving, they expressed a general lack of understanding or knowledge about the problem ( Table 3 ). As Sara put it, “ I have no clue what I’m looking at.” Sometimes monitoring confusion came as an acknowledgement of lack of prior knowledge students felt they needed to solve the problem. Take for instance when Ismail states, “I’ve never really had any prior knowledge on pathway fluxes and like how they work and it obviously doesn ’ t make much sense to me .” Students also expressed confusion about how to approach the problem, which is related to monitoring one’s procedural knowledge. For example, when Harper stated, “ I ’ m not sure how to approach the question ,” she was monitoring a lack of knowledge about how to begin. Similarly, after reading the problem Tiffani shared, “ I am not sure how to solve this one because I’ve actually never done it before…”
What happened after monitoring confusion?
When students monitored their confusion, one of two things happened ( Figure 2 ). Rarely, students would give up on solving altogether. In fact, only one individual (Roland) submitted a final solution that read, “ I have no idea .” More often students persisted despite their confusion. Rereading the problem was a common strategy students in our sample used after identifying general confusion. As Jeffery stated after reading the problem, “I didn’t really understand that, so I’m gonna read that again.” After rereading the problem a few times, Jeffery stated, “Oh, and we have valine here. I didn’t see that before.” Some students like Valentina revealed their rereading strategy rationale after solving, “First I just read it a couple of times because I wasn’t really understanding what it was saying.” After rereading the problem a few times Valentina was able to accurately assess the task by stating “amino acid (A) turns into valine.” When solving, some students linked their general confusion with an inability solve. As Harper shared, “I don’t think that I have enough like basis or learning to where I’m able to answer that question.” Despite making this claim of self-doubt in their ability to solve, Harper monitored in other ways and ultimately came up with a solution beyond a simple, “I don’t know.” In sum, when students acknowledged their confusion in this study, they usually did not stop there. They used their confusion as an indicator to use a strategy, like rereading, to resolve their confusion or as a jumping off point to further monitor by identifying more specifically what they did not understand. Persisting despite confusion is likely dependent on other factors, like self-efficacy.
Monitoring Familiarity
When students monitored familiarity, they described knowledge or aspects of the problem prompt that were familiar or not familiar to them ( Table 3 ). This category also captured when students would describe remembering or forgetting something from class. For example, when Simone states, “ I remember learning covalent bonds in chemistry, but I don ’ t remember right now what that meant” she is acknowledging her familiarity with the term covalent from her chemistry course. Similarly, Oliver acknowledges his familiarity with tertiary structure from his class when solving the Protein X problem. He first shared , “ This reminds me of something that we’ve looked at in class of a tertiary structure. It was shown differently but I do remember something similar to this .” Then later, he acknowledges his lack of familiarity with the term flux when solving the Pathway Fux problem, “That word flux. I ’ ve never heard that word before .” Quinn aptly pointed out that being familiar with a term or recognizing a word in the problem did not equate to her understanding, “I mean, I know amino acids, but that doesn’t… like I recognize the word , but it doesn’t really mean anything to me. And then non-covalent, I recognize the conjunction of words, but again, it's like somewhere deep in there…”
What happened after monitoring familiarity?
When students recognized what was familiar to them in the problem, it sometimes helped them connect to related prior knowledge ( Figure 2 ). In some cases, though, students connected words in the problem that were familiar to them to unrelated prior knowledge. Erika, for example, revealed in her problem reflection that she was familiar with the term mutation in the Protein X problem and formulated her solution based on her knowledge of the different types of DNA mutations, not noncovalent interactions. In this case, Erika’s familiarity with the term mutation and failure to monitor the relevance of this knowledge when problem solving impeded her development of an accurate solution to the problem. This is why Quinn’s recognition that her familiarity with terms does not equate to understanding is critical. This recognition can help students like Erika avoid false feelings of knowing that might come from the rapid and fluent recall of unrelated knowledge ( Reber and Greifeneder, 2017 ). When students recognized parts of the problem they were unfamiliar with, they often searched for familiar terms to use as footholds ( Figure 2 ). For example, Lucy revealed the following in her problem reflection: “So first I tried to look at the beginning introduction to see if I knew anything about the topic. Unfortunately, I did not know anything about it. So, I just tried to look for any trigger words that I did recognize.” After stating this, Lucy said she recognized the words protein and tertiary structure and was able to access some prior knowledge about hydrogen bonds for her solution.
Monitoring Understanding
When students monitored understanding, they described specific pieces of knowledge they either knew or did not know, beyond what was provided in the problem prompt ( Table 3 ). Monitoring understanding is distinct from monitoring confusion. When students displayed awareness of a specific piece of knowledge they did not know (e.g., “I don’t know what these arrows really mean.” ) this was considered monitoring (a lack of) understanding. In contrast, monitoring confusion was a more general awareness of their overall lack of understanding (e.g., “Well, I first look at the image and I ’ m already kind of confused with it [laughs].” ). For example, Kathleen demonstrated an awareness of her understanding about amino acid properties when she said, “ I know that like the different amino acids all have different properties like some are, what’s it called? Like hydrophobic, hydrophilic, and then some are much more reactive.” Willibald monitored his understanding using the mnemonic “when in doubt, van der Waals it out” by sharing, “So, cause I know basically everything has, well not basically everything, but a lot of things have van der Waal forces in them. So that’s why I say that a lot of times. But it’s a temporary dipole, I think.” In contrast, Jeffery monitored his lack of understanding of a specific part of the Pathway Flux figure when he stated, “I guess I don ’ t understand what this dotted arrow is meaning.” Ignoring or misinterpreting the negative feedback loop was a common issue as students solved this problem, so it’s notable that Jeffery acknowledged his lack of understanding about this symbol. When students identified what they knew, the incomplete knowledge they revealed sometimes had the potential to lead to a misunderstanding. Take for example Lucy’s quote: “ I know a hydrogen bond has to have a hydrogen. I know that much. And it looks like they both have hydrogen.” This statement suggests Lucy might be displaying a known misconception about hydrogen bonding – that all hydrogens participate in hydrogen bonding ( Villafañe et al. , 2011 ).
What happened after monitoring understanding?
When students could identify what they knew, they used this information to formulate a solution ( Figure 2 ). When students could identify what they did not know, they either did not know what to do next or they used strategies to move beyond their lack of understanding ( Figure 2 ). Two strategies students used after identifying a lack of understanding included disregarding information and writing what they knew. Kyle disregarded information when he didn’t understand the negative feedback loop in the Pathway Flux problem: “…there is another arrow on the side I see with a little minus sign. I’m not sure what that means… it’s not the same as [the arrows by] A and C. So, I’m just going to disregard it sort of for now. It’s not the same. Just like note that in my mind that it’s not the same.” In this example, Kyle disregards a critical part of the problem, the negative feedback loop, and does not revisit the disregarded information which ultimately led him to an incorrect prediction for this problem. We also saw one example of a student, Elaine, use the strategy of writing what she knew when she was struggling to provide an explanation for her answer: “I should know this more, but I don’t know, like a specific scientific explanation answer, but I’m just going to write what I do know so I can try to organize my thoughts.” Elaine’s focus on writing what she knew allowed her to organize the knowledge she did have into a plausible solution that specified which amino acids would participate in new noncovalent interactions (“I predict there will be a bond between A and B and possibly A and C.” ) despite not knowing “what would be required in order for it to create a new noncovalent interaction with another amino acid.” The strategies that Kyle and Elaine used in response to monitoring a lack of understanding shared the common goal of helping them get unstuck in their problem-solving process.
Monitoring Questions
When students monitored through questions, they asked themselves a question out loud ( Table 3 ). These questions were either about the problem itself or their own knowledge. An example of monitoring through a question about the problem itself comes from Elaine who asked herself after reading the problem and sharing her initial thoughts, “ What is this asking me? ” Elaine’s question helped reorient her to the problem and put herself back on track with answering the question asked. After Edith came to a tentative solution, she asked herself, “But what about the other information? How does that pertain to this? ” which helped her initiate monitoring the relevance of the information provided in the prompt. Students also posed questions to themselves about their own content knowledge. Take for instance Phillip when he asked himself, “So, would noncovalent be ionic bonds or would it be something else? Covalent bonds are sharing a bond, but what does noncovalent mean? ” After Phillip asked himself this question, he reread the problem but ultimately acknowledged he was “not too sure what noncovalent would mean.”
What happened after monitoring questions?
After students posed questions to themselves while solving, they either answered their question or they didn’t ( Figure 2 ). Students who answered their self-posed questions relied on other forms of monitoring and rereading the prompt to do so. For example, after questioning themselves about their conceptual knowledge, some students acknowledged they did not know the answer to their question by monitoring their understanding. Students who did not answer their self-posed questions moved on without answering their question directly out loud.
Monitoring Correctness
When students monitored correctness, they corrected their thinking out loud ( Table 3 ). A prime example of this comes from Kyle’s think aloud, where he corrects his interpretation of the problem not once but twice: “It said the blue one highlighted is actually a valine, which substituted the serine, so that’s valine right there. And then I’m reading the question. No, no, no. It ’ s the other way around. So, serine would substitute the valine and the valine is below… Oh wait wait , I had it right the first time. So, the blue highlighted is this serine and that’s supposed to be there, but a mutation occurs where the valine gets substituted.” Kyle first corrects his interpretation of the problem in the wrong direction but corrects himself again to put him on the right track. Icarus also caught himself reading the problem incorrectly by replacing the word noncovalent with the word covalent, which was a common error students made: “ Oh, wait, I think I read that wrong. I think I read it wrong. Well, yeah. Then that will affect it. I didn’t read the noncovalent part. I just read covalent.” Students also corrected their language use during the think aloud interviews, like Edith: “ because enzyme B is no longer functioning… No, not enzyme B… because IV-CoA is no longer functional and able to bind to enzyme B, the metabolic pathway is halted.” Edith’s correction of her own wording, while minor, is worth noting because students in this study often misinterpreted the Pathway Flux problem to read as “enzyme B no longer works”. There were also instances when students corrected their own knowledge that they brought to bear on the problem. This can be seen in the following quote from Tiffani when she says, “And tertiary structure. It has multiple… No, no, no. That ’ s primary structure. Tertiary structure’s when like the proteins are folded in on each other.”
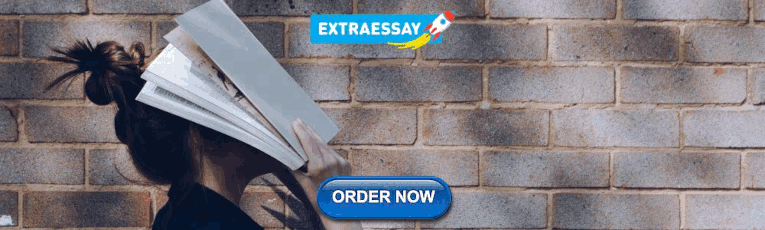
What happened after monitoring correctness?
When students corrected themselves, this resulted in more accurate interpretations of the problem and thus more accurate solutions ( Figure 2 ). Specifically, monitoring correctness helped students avoid common mistakes when assessing the task which was the case for Kyle, Icarus, and Edith described above. When students do not monitor correctness, incorrect ideas can go unchecked throughout their problem-solving process, leading to more inaccurate solutions. In other research, contradicting and misunderstanding content were two procedural errors students experienced when solving multiple-choice biology problems ( Prevost and Lemons, 2016 ), which could be alleviated through monitoring correctness.
Implications for Instruction & Research about Monitoring
Monitoring is the last metacognitive regulation skill to develop, and it develops slowly and well into adulthood ( Schraw, 1998 ). Based on our data, first-year life science students are monitoring in the moment in a myriad of ways. This may suggest that college-aged students have already developed monitoring skills by the time they enter college. This finding has implications for both instruction and research. For instruction, we may need to help our students keep track of and learn what do with the information and insight they glean from their in-situ monitoring when solving life science problems. For example, students in our study could readily identify what they did and did not know, but they sometimes struggled to identify ways in which they could potentially resolve their lack of understanding, confusion, or uncertainty or use this insight in expert-like ways when formulating a solution.
As instructors who teach students about metacognition, we can normalize the temporary discomfort monitoring may bring as an integral part of the learning process and model for students what to do after they monitor. For example, when students glean insight from monitoring familiarity , we could help them learn how to properly use this information so that they do not equate familiarity with understanding when practicing problem solving on their own. This could help students avoid the fluency fallacy or the false sense that they understand something simply because they recognize it or remember learning about it ( Reber and Greifeneder, 2017 ).
The majority of the research on metacognition, including our own, has been conducted using retrospective methods. However, retrospective methods may provide little insight into true monitoring skills since these skills are used during learning rather than after learning has occurred ( Schraw and Moshman, 1995 ; Stanton et al. , 2021 ). More research using in-the-moment methods, which are used widely in the problem-solving literature, are needed to fully understand the rich monitoring skills of life science students and how they may develop over time. The monitoring skills of life science students in both individual and small group settings, and the relationship of monitoring skills across these two settings, warrants further exploration. This seems particularly salient given that questioning and responding to questions seems to be an important aspect of both individual metacognition in the present study and social metacognition in our prior study, which also used in-the-moment methods ( Halmo et al. , 2022 ).
Evaluating: Students evaluated their solution and experience problem solving
Evaluating achievement of individual problem solving involves appraising an implemented plan and how it could be improved for future learning after completing the task ( Stanton et al. , 2021 ). Students in our sample revealed some of the ways they evaluate when solving problems on their own ( Table 3 ). They evaluated both their solution and their experience of problem solving.
Evaluating A Solution
Evaluating a solution occurred when students assessed the accuracy of their solution, double-checked their answer, or rethought their solution ( Table 3 ). While some students evaluated their accuracy in the affirmative (that their solution is right), most students evaluated the accuracy of their solution in the negative (that their solution is wrong). For example, when Kyle states, “ I don ’ t think hydrogen bonding is correct .” Kyle clarified in his problem reflection, “I noticed [valine] did have hydrogens and the only noncovalent interaction I know of is probably hydrogen bonding. So, I just sort of stuck with that and just said more hydrogen bonding would happen with the same oxygen over there [in glutamine].” Through this quote, we see that Kyle went with hydrogen bonding as his prediction because it’s the only noncovalent interaction he could recall. However, Kyle accurately evaluated the accuracy of his solution by noting that hydrogen bonding was not the correct answer. Evaluating accuracy in the negative often seemed like hedging or self-doubt. Take for instance Regan’s quote that she shared right after submitting her final solution: “ The chances of being wrong are 100% , just like, you know [laughs].”
Students also evaluated their solution by double-checking their work. Kyle used a very clearly-defined approach for double checking his work by solving the problem twice: “So that’s just my initial answer I would put, and then what I do next was I ’ d just like reread the question and sort of see if I come up with the same answer after rereading and redoing the problem. So, I’m just going to do that real quick.” Checking one’s work is a well-established problem-solving step that most successful problem solvers undertake ( Cartrette and Bodner, 2010 ; Prevost and Lemons, 2016 ).
Students also evaluated by rethinking their initial solution. In the following case, Mila’s evaluation of her solution did not improve her final answer. Mila initially predicted that the change described in the Pathway Flux problem would affect flux, which is correct. However, she evaluates her solution when she states, “Oh, wait a minute, now that I’m saying this out loud, I don’t think it’ll affect it because I think IV-CoA will be binding to enzyme B or C. Sorry, hold on. Now I ’ m like rethinking my whole answer .” After this evaluation, Mila changes her prediction to “it won’t affect flux” , which is incorrect. In contrast, some students’ evaluations of their solutions resulted in improved final answers. For example, after submitting his solution and during his problem reflection, Willibald states, “Oh, I just noticed. I said there’ll be no effect on the interaction, but then I said van der Waals forces which is an interaction. So, I just contradicted myself in there .” After this recognition, Willibald decides to amend his first solution, ultimately improving his prediction. We also observed one student, Jeffery, evaluating whether or not his solution answered the problem asked, which is notable because we also observed students evaluating in this way when solving problems in small groups ( Halmo et al. , 2022 ): “I guess I can’t say for sure, but I’ll say this new amino acid form[s] a bond with the neighboring amino acids and results in a new protein shape. The only issue with that answer is I feel like I ’ m not really answering the question : Predict any new noncovalent interactions that might occur with such a mutation.” While the above examples of evaluating solution occurred spontaneously without prompting, having students describe their thinking process after solving the problems may have been sufficient to prompt them to evaluate their solution.
What happened after evaluating a solution?
When students evaluated the accuracy of their solution, double-checked their answer, or rethought their solution it helped them recognize potential flaws or mistakes in their answers. After evaluating their solution, they either decided to stick with their original answer or amend their solution. Evaluating a solution often resulted in students adding to or refining their final answer. However, these solution amendments were not always beneficial or in the correct direction because of limited content knowledge. In other work on the metacognition involved in changing answers, answer-changing neither reduced or significantly boosted performance ( Stylianou-Georgiou and Papanastasiou, 2017 ). The fact that Mila’s evaluation of her solution led to a less correct answer, whereas Willibald’s evaluation of his solutions led to a more correct answer further contributes to the variable success of answer-changing on performance.
Evaluating Experience
Evaluating experience occurred when students assessed the difficulty level of the problem or the feelings associated with their thought process ( Table 3 ). This type of evaluation occurred after solving in their problem reflection or in response to the closing questions of the think aloud interview. Students evaluated the problems as difficult based on the confusion, lack of understanding, or low self-efficacy they experienced when solving. For example, Ivy stated, “I just didn’t really have any background knowledge on them, which kind of made it difficult .” In one instance, Willibald’s evaluation of difficulty while amending his solution was followed up with a statement about self-efficacy: “ This one was a difficult one. I told you I’m bad with proteins [laughs].” Students also compared the difficulty of the two problems we asked them to solve. For example, Elena determined that the Pathway Flux problem was easier for her compared with the Protein X problem in her problem reflection: “ I didn ’ t find this question as hard as the last question just cause it was a little bit more simple.” In contrast, Elaine revealed that she found the Protein X problem challenging because of the open-ended nature of the question: “ I just thought that was a little more difficult because it’s just asking me to predict what possibly could happen instead of like something that’s like, definite, like I know the answer to. So, I just tried to think about what I know…” Importantly, Elaine indicated her strategy of thinking about what she knew in the problem in response to her evaluation of difficulty.
Evaluating experience also occurred when students assessed how their feelings were associated with their thought process. The feelings they described were directly tied to aspects of their monitoring. We found that students associated negative emotions (nervousness, worry, and panic) with a lack of understanding or a lack of familiarity. For example, in Renee’s problem reflection, she connected feelings of panic to when she monitored a lack of understanding: “I kind of panicked for a second, not really panicked cause I know this isn’t like graded or anything, but I do not know what a metabolic pathway is.” In contrast, students associated more positive feelings when they reflected on moments of monitoring understanding or familiarity. For example, Renee also stated, “At first I was kind of happy because I knew what was going on.” Additionally, some students revealed their use of a strategy explicitly to engender positive emotions or to avoid negative emotions, like Tabitha: “I looked at the first box, I tried to break it up into certain sections, so I did not get overwhelmed by looking at it.”
What happened after evaluating experience?
When students evaluated their experience problem solving in this study, they usually evaluated the problems as difficult and not easy. Their evaluations of experience were directly connected to aspects of their monitoring while solving. They associated positive emotions and ease with understanding and negative emotions and difficulty with confusion, a lack of familiarity, or a lack of understanding. Additionally, they identified the purpose of some strategy use was to avoid negative experiences. Because their evaluations of experience occurred after solving the problems, most students did not act on this evaluation in the context of this study. We speculate that students may act on evaluations of experience by making plans for future problem solving, but our study design did not necessarily provide students with this opportunity. Exploring how students respond to this kind of evaluation in other study designs would be illuminating.
Implications for Instruction & Research about Evaluating
Our data indicate that some first-year life science students are evaluating their solution and experience after individual problem solving. As instructors, we can encourage students to further evaluate their solutions by prompting them to: 1) rethink or redo a problem to see whether they come up with the same answer or wish to amend their initial solution, and 2) predict whether they think their solution is right or wrong. Encouraging students to evaluate by predicting whether their solution is right or wrong is limited by content knowledge. Therefore, it is imperative to help students develop their self-evaluation accuracy by following up their predictions with immediate feedback to help them become well-calibrated ( Osterhage, 2021 ). Additionally, encouraging students to reflect on their experience solving problems might help students identify and verbalize perceived problem-solving barriers to themselves and their instructors. There is likely a highly individualized level of desirable difficulty for each student where a problem is difficult enough to engage their curiosity and motivation to solve something unknown but also does not generate negative emotions associated with failure that could prevent problem solving from moving forward ( Zepeda et al. , 2020 ; de Bruin et al. , 2023 ). The link between feelings and metacognition in the present study is paralleled in other studies that used retrospective methods and found links between feelings of (dis)comfort and metacognition ( Dye and Stanton, 2017 ). This suggests that the feelings students associate with their metacognition is an important consideration when designing future research studies and interventions. For example, helping students coach themselves through the negative emotions associated with not knowing and pivoting to what they do know might increase the self-efficacy needed for problem-solving persistence.
To address our second research question, we looked for statements related to self-efficacy in our participants’ think aloud data. Self-efficacy is defined as one’s belief in their capability to carry out a specific task ( Bandura, 1997 ). Alternatively, self-efficacy is sometimes operationalized as one’s confidence in performing specific tasks ( Ainscough et al. , 2016 ). While we saw instances of students making high-self efficacy statements (“I’m confident that I was going in somewhat of the right direction”) and low self-efficacy statements (“I’m not gonna understand it anyways”) during their think aloud interviews, we were particularly intrigued by a distinct form of self-efficacy that appeared in our data that we call “self-coaching” ( Table 4 ). We posit that self-coaching is similar to the ideas of self-modeling or efficacy self-talk that other researchers have described in the past ( Wolters, 2003 ; Usher, 2009 ). In our data, these self-encouraging statements either: 1) reassured themselves about a lack of understanding, 2) reassured themselves that it’s okay to be wrong, 3) encouraged themselves to keep going despite not knowing, or 4) reminded themselves of their prior experience. To highlight the role that self-coaching played in problem solving in our dataset, we first present examples where self-coaching was absent and could have been beneficial for the students in our study. Then we present examples where self-coaching was used.
When students monitored without self-coaching, they had hard time moving forward in their problem-solving
When solving the challenging biochemistry problems in this study, first-year life science students often came across pieces of information or parts of the figures that they were unfamiliar with or did not understand. In the Monitoring section, we described how students monitored their understanding and familiarity, but perhaps what is more interesting is how students responded to not knowing and their lack of familiarity ( Figure 2 ). In a handful of cases, we witnessed students get stuck or hung up on what they did not know. We posit that the feeling of not knowing could increase anxiety, cause concern, and increase self-doubt, all of which can negatively impact a student’s self-efficacy and cause them to stop problem solving. One example of this in our data comes from Tiffani. Tiffani stated her lack of knowledge about how to proceed and followed this up with a statement on her lack of ability to solve the problem, “I am actually not sure how to solve this. I do not think I can solve this one.” A few lines later, Tiffani clarified where her lack of understanding rested, but again stated she cannot solve the problem, “I’m not really sure how these type of amino acids pair up, so I can’t really solve it.” In this instance, Tiffani’s lack of understanding is linked to a perceived inability to solve the problem.
Some students also linked not knowing with perceived deficits. For example, in the following quote Chandra linked not knowing how to answer the second part of the Protein X problem with the idea that she is “not very good” with noncovalent interactions: “ I’m not really sure about the second part. I do not know what to say at all for that, to predict any new noncovalent, I’m not very good with noncovalent at all.” When asked where she got stuck during problem solving, Chandra stated, “The “predict any new noncovalent” cause [I’m] not good with bonds. So, I cannot predict anything really.” In Chandra’s case, her lack of understanding was linked to a perceived deficit and inability to solve the problem. As instructors, it is moments like these where we would hope to intervene and help our students persist in problem solving. However, targeted coaching for all students each time they solve a problem can seem like an impossible feat to accomplish in large, lecture-style college classrooms. Therefore, from our data we suggest that encouraging students to self-coach themselves through these situations is one approach we could use to achieve this goal.
When students monitored and self-coached, they persisted in their problem-solving
In contrast to the cases of Tiffani and Chandra shared above, we found instances of students self-coaching after acknowledging their lack of understanding about parts of the problem by immediately reassuring themselves that it was okay to not know ( Table 4 ). For example, when exploring the arrows in the Pathway Flux problem figure Ivy states, “I don’t really know what that little negative means, but that’s okay .” After making this self-coaching statement Ivy moves on to thinking about the other arrows in the figure and what they mean to formulate an answer. In a similar vein, when some students were faced with their lack of understanding, one strategy they deployed was not dwelling on their lack of knowledge and pivoting to look for a foothold of something they do know. For example, in the following quote we see Viola acknowledge her initial lack of understanding and familiarity with the Pathway Flux problem and then find a foothold with the term enzymes which she knows she has learned about in the past, “I’m thinking there’s very little here that I recognize or understand. Just… okay. So, talking about enzymes, I know we learned a little bit about that.”
Some students acknowledged this strategy of pivoting to what they do know in their problem reflections. In their problem reflections, Quinn and Gerald expanded that they will rely on what they do know, even if it is not accurate. As Quinn put it, “ taking what I think I know, even if it ’ s wrong , like I kind of have to, you have to go off of something.” Similarly, Gerald acknowledged his strategy of “ it’s okay to get it wrong ” when he doesn’t know and connects this strategy to his experience solving problems on high-stakes exams.
I try to use information that I knew and I didn’t know a lot. So, I had to kind of use my strategy where I’m like, if this was on a test, this is one of the questions that I would either skip and come back to or write down a really quick answer and then come back to . So , my strategy for this one is it ’ s okay to get it wrong. You need to move on and make estimated guess. Like if I wasn’t sure what the arrows meant, so I was like, "okay, make an estimated guess on what you think the arrows mean. And then using the information that you kind of came up with try to get a right answer using that and like, explain your answer so maybe they’ll give you half points…" – Gerald
We also observed students encouraging themselves to persist despite not knowing ( Table 4 ). In the following quote we see Kyle acknowledge a term he doesn’t know at the start of his think aloud and verbally choose to keep going, “So the title is pathway flux problem. I’m not too sure what flux means, but I ’ m going to keep on going .” Sometimes this took the form of persisting to write an answer to the problem despite not knowing. For example, Viola stated, “I’m not even really sure what pathway flux is. So, I’m also not really sure what the little negative sign is and it pointing to B. But I ’ m going to try to type an answer .” Rather than getting stuck on not knowing what the negative feedback loop symbol depicted, she moved past it to come to a solution.
We also saw students use self-coaching to remind themselves of their prior experience ( Table 4 ). In the following example, we see Mila talk herself through the substitution of serine with valine in the Protein X problem: “So, there’s not going to be a hydroxyl anymore, but I don’t know if that even matters, but there, valine, has more to it. I don’t know if that means there would be an effect on the covalent interaction. I haven’t had chemistry in such a long time [pause], but at the same time, this is bio. So , I should still know it. [laughs]” Mila’s tone as she made this statement was very matter-of-fact. Her laugh at the end suggests she did not take what she said too seriously. After making this self-coaching statement, Mila rereads the question a few times and ultimately decides that the noncovalent interaction is affected because of the structural difference in valine and serine. Prior experiences, sometimes called mastery experiences, are one established source of self-efficacy that Mila might have been drawing on when she made this self-coaching statement ( Bandura, 1977 ; Pajares, 1996 ).
Implications for Instruction about Self-Coaching
Students can be encouraged to self-coach by using some of the phrases we identified in our data as prompts ( Table 4 ). However, we would encourage instructors to rephrase some of self-coaching statements in our data by removing the word “should” because this term might make students feel inadequate if they think they are expected to know things they don’t yet know. Instead, we could encourage students to remind themselves of when they’ve successfully solved challenging biology problems in the past by saying things like, “I’ve solved challenging problems like this before, so I can solve this one.” Taken together, we posit that self-coaching could be used by students to decrease anxiety and increase confidence when faced with the feeling of not knowing that can result from monitoring, which could potentially positively impact a student’s self-efficacy and metacognitive regulation. Our results reveal first-year students are monitoring in a myriad of ways. Sometimes when students monitor, they may not act further on the resulting information because it makes them feel bad or uncomfortable. Self-coaching could support students to act on their metacognition or not actively avoid being metacognitive.
LIMITATIONS
Even with the use of in-the-moment methods like think aloud interviews, we are limited to the metacognition that students verbalized. For example, students may have been employing metacognition while solving that they simply did not verbalize. However, using a think aloud approach in this study ensured we were accessing students’ metacognition in use, rather than their remembrance of metacognition they used in the past which is subject to recall bias ( Schellings et al. , 2013 ). Our study, like most education research, may suffer from selection bias where the students who volunteer to participate represent a biased sample ( Collins, 2017 ). To address this potential pitfall, we attempted to ensure our sample represented the student body at each institution by using purposeful sampling based on self-reported demographics and varied responses to the revised Metacognitive Awareness Inventory ( Harrison and Vallin, 2018 ). Lastly, while our sample size is large ( N = 52) for qualitative analyses and includes students from three different institutional types, the data are not necessarily generalizable to contexts beyond the scope of the study.
The goal of this study was to investigate first-year life science students’ metacognition and self-efficacy in-the-moment while they solved challenging problems. Think aloud interviews with 52 students across three institutions revealed that while first-year life science students plan, monitor, and evaluate while solving challenging problems, they predominantly monitor. First-year life science students associated monitoring a lack of conceptual understanding with negative feelings whereas they associated positive feelings with monitoring conceptual understanding. We found that what students chose to do after they monitored a lack of conceptual understanding impacted whether their monitoring moved problem solving forward or not. For example, after monitoring a lack of conceptual understanding, students could either not use a strategy and remain stuck or they could use a strategy to move their problem solving forward. One critical finding revealed in this study was that self-coaching helped students use their metacognition to take action and persist in problem solving. This type of self-efficacy related encouragement helped some students move past the discomfort associated with monitoring a lack of conceptual understanding and enabled them to select and use a strategy. Together these findings about in-the-moment metacognition and self-efficacy offer a positive outlook on ways we can encourage students to couple their developing metacognitive regulation skills and self-efficacy to persist when faced with challenging life science problems.
ACKNOWLEDGMENTS
We would like to thank Dr. Paula Lemons for allowing us to use problems developed in her research program for this study and for her helpful feedback during the writing process, the College Learning Study participants for their willingness to participate in this study, Dr. Mariel Pfeifer for her assistance conducting interviews and continued discussion of the data during the writing of this manuscript, C.J. Zajic for his contribution to preliminary data analysis, and Emily K. Bremers, Rayna Carter, and the UGA BERG community for their thoughtful feedback on earlier versions of this manuscript. We are also grateful for the feedback from the monitoring editor and reviewers at LSE , which strengthened the manuscript. This material is based on work supported by the National Science Foundation under Grant Number 1942318. Any opinions, findings, and conclusions or recommendations expressed in this material are those of the authors and do not necessarily reflect the views of the National Science Foundation.
- Ainscough, L., Foulis, E., Colthorpe, K., Zimbardi, K., Robertson-Dean, M., Chunduri, P., & Lluka, L. ( 2016 ). Changes in biology self-efficacy during a first-year university course . CBE—Life Sciences Education , 15 (2), ar19. https://doi.org/10.1187/cbe.15-04-0092 Link , Google Scholar
- Bandura, A. ( 1977 ). Self-efficacy: Toward a unifying theory of behavioral change . Psychological Review , 84 , 191–215. https://doi.org/10.1037/0033-295X.84.2.191 Medline , Google Scholar
- Bandura, A. ( 1997 ). Self-Efficacy: The Exercise of Control . New York, NY: W H Freeman and Company. Google Scholar
- Bannert, M., & Mengelkamp, C. ( 2008 ). Assessment of metacognitive skills by means of instruction to think aloud and reflect when prompted. Does the verbalisation method affect learning? Metacognition and Learning , 3 (1), 39–58. https://doi.org/10.1007/s11409-007-9009-6 Google Scholar
- Bhatia, K. S., Stack, A., Sensibaugh, C. A., & Lemons, P. P. ( 2022 ). Putting the pieces together: Student thinking about transformations of energy and matter . CBE—Life Sciences Education , 21 (4), ar60. https://doi.org/10.1187/cbe.20-11-0264 Google Scholar
- Blackford, K. A., Greenbaum, J. C., Redkar, N. S., Gaillard, N. T., Helix, M. R., & Baranger, A. M. ( 2023 ). Metacognitive regulation in organic chemistry students: How and why students use metacognitive strategies when predicting reactivity [10.1039/D2RP00208F] . Chemistry Education Research and Practice , 24 (3), 828–851. https://doi.org/10.1039/D2RP00208F Google Scholar
- Carr, M., & Taasoobshirazi, G. ( 2008 ). Metacognition in the gifted: Connections to expertise . In Shaughnessy, M. F.Veenman, M.Kennedy, C. K. (Eds.), Meta-cognition: A recent review of research, theory and perspectives (pp. 109–125). New York, NY: Nova Science Publishers. Google Scholar
- Cartrette, D. P., & Bodner, G. M. ( 2010 ). Non-mathematical problem solving in organic chemistry . Journal of Research in Science Teaching , 47 (6), 643–660. https://doi.org/10.1002/tea.20306 Google Scholar
- Charters, E. ( 2003 ). The use of think-aloud methods in qualitative research: An introduction to think-aloud methods . Brock Education Journal , 12 (2), 68–82. Google Scholar
- Chi, M. T. H., Feltovich, P. J., & Glaser, R. ( 1981 ). Categorization and representation of physics problems by experts and novices . Cognitive Science , 5 (2), 121–152. www.sciencedirect.com/science/article/pii/S0364021381800298 Google Scholar
- Collins, K. M. ( 2017 ). Sampling decisions in educational research . In Wyse, D.Selwyn, N.Smith, E.Suter, L. E. (Eds.), The BERA/SAGE handbook of educational research (pp. 280–292). London, UK: SAGE Publications Ltd. Google Scholar
- Coutinho, S. A., & Neuman, G. ( 2008 ). A model of metacognition, achievement goal orientation, learning style and self-efficacy . Learning Environments Research , 11 (2), 131–151. https://doi.org/10.1007/s10984-008-9042-7 Google Scholar
- Cross, D. R., & Paris, S. G. ( 1988 ). Developmental and instructional analyses of children’s metacognition and reading comprehension . Journal of Educational Psychology , 80 (2), 131–142. https://doi.org/10.1037/0022-0663.80.2.131 Google Scholar
- Davidson, J. E., & Sternberg, R. J. ( 1998 ). Smart problem solving: How metacognition helps . In Douglas, J. H.John, D.Arthur, C. G. (Eds.), Metacognition in Educational Theory and Practice (pp. 30–38). Mahwah, NJ: Routledge. Google Scholar
- de Bruin, A. B. H., Biwer, F., Hui, L., Onan, E., David, L., & Wiradhany, W. ( 2023 ). Worth the effort: The start and stick to desirable difficulties (S2D2) framework . Educational Psychology Review , 35 (2), 41. https://doi.org/10.1007/s10648-023-09766-w Google Scholar
- Dye, K. M., & Stanton, J. D. ( 2017 ). Metacognition in upper-division biology students: Awareness does not always lead to control . CBE—Life Sciences Education , 16 (2), ar31. https://doi.org/10.1187/cbe.16-09-0286 Link , Google Scholar
- Ericsson, K. A., & Simon, H. A. ( 1980 ). Verbal reports as data [Article] . Psychological Review , 87 , 215–251. https://doi.org/10.1037/0033-295X.87.3.215 Google Scholar
- Frey, R. F., Brame, C. J., Fink, A., & Lemons, P. P. ( 2022 ). Teaching discipline-based problem solving . CBE—Life Sciences Education , 21 (2), fe1. https://doi.org/10.1187/cbe.22-02-0030 Medline , Google Scholar
- Halmo, S. M., Bremers, E. K., Fuller, S., & Stanton, J. D. ( 2022 ). “ Oh, that makes sense”: Social metacognition in small-group problem solving . CBE—Life Sciences Education , 21 (3), ar58. https://doi.org/10.1187/cbe.22-01-0009 Medline , Google Scholar
- Halmo, S. M., Sensibaugh, C. A., Bhatia, K. S., Howell, A., Ferryanto, E. P., Choe, B., ... & Lemons, P. P. ( 2018 ). Student difficulties during structure–function problem solving . Biochemistry and Molecular Biology Education , 46 (5), 453–463. https://doi.org/10.1002/bmb.21166 Medline , Google Scholar
- Halmo, S. M., Sensibaugh, C. A., Reinhart, P., Stogniy, O., Fiorella, L., & Lemons, P. P. ( 2020 ). Advancing the guidance debate: Lessons from educational psychology and implications for biochemistry learning . CBE—Life Sciences Education , 19 (3), ar41. https://doi.org/10.1187/cbe.19-11-0260 Link , Google Scholar
- Harrison, G. M., & Vallin, L. M. ( 2018 ). Evaluating the metacognitive awareness inventory using empirical factor-structure evidence . Metacognition and Learning , 13 (1), 15–38. https://doi.org/10.1007/s11409-017-9176-z Google Scholar
- Heidbrink, A., & Weinrich, M. ( 2021 ). Encouraging biochemistry students’ metacognition: Reflecting on how another student might not carefully reflect . Journal of Chemical Education , 98 (9), 2765–2774. https://doi.org/10.1021/acs.jchemed.1c00311 Google Scholar
- Hodges, L. C., Beall, L. C., Anderson, E. C., Carpenter, T. S., Cui, L., Feeser, E., ... & Wagner, C. ( 2020 ). Effect of exam wrappers on student achievement in multiple, large STEM courses . Journal of College Science Teaching , 50 (1), 69–79. www.jstor.org/stable/27119232 Google Scholar
- Huang, X., Bernacki, M. L., Kim, D., & Hong, W. ( 2022 ). Examining the role of self-efficacy and online metacognitive monitoring behaviors in undergraduate life science education . Learning and Instruction , 80 , 101577. https://doi.org/10.1016/j.learninstruc.2021.101577 Google Scholar
- Hurme, T.-R., Palonen, T., & Järvelä, S. ( 2006 ). Metacognition in joint discussions: An analysis of the patterns of interaction and the metacognitive content of the networked discussions in mathematics . Metacognition and Learning , 1 (2), 181–200. https://doi.org/10.1007/s11409-006-9792-5 Google Scholar
- Kelemen, W. L., Frost, P. J., & Weaver, C. A., 3rd. ( 2000 ). Individual differences in metacognition: Evidence against a general metacognitive ability . Memory & Cognition , 28 (1), 92–107. https://doi.org/10.3758/bf03211579 Google Scholar
- Ku, K. Y. L., & Ho, I. T. ( 2010 ). Metacognitive strategies that enhance critical thinking . Metacognition and Learning , 5 (3), 251–267. https://doi.org/10.1007/s11409-010-9060-6 Google Scholar
- Kuhn, D. ( 2000 ). Metacognitive development . Current Directions in Psychological Science , 9 (5), 178–181. https://doi.org/10.1111/1467-8721.00088 Google Scholar
- McGee, E. O. ( 2020 ). Interrogating structural racism in STEM higher education . Educational Researcher , 49 (9), 633–644. https://doi.org/10.3102/0013189×20972718 Google Scholar
- Meijer, J., Veenman, M. V. J., & van Hout-Wolters, B. H. A. M. ( 2006 ). Metacognitive activities in text-studying and problem-solving: Development of a taxonomy . Educational Research and Evaluation , 12 (3), 209–237. https://doi.org/10.1080/13803610500479991 Google Scholar
- Muncer, G., Higham, P. A., Gosling, C. J., Cortese, S., Wood-Downie, H., & Hadwin, J. A. ( 2022 ). A meta-analysis investigating the association between metacognition and math performance in adolescence . Educational Psychology Review , 34 (1), 301–334. https://doi.org/10.1007/s10648-021-09620-x Google Scholar
- Ohtani, K., & Hisasaka, T. ( 2018 ). Beyond intelligence: A meta-analytic review of the relationship among metacognition, intelligence, and academic performance . Metacognition and Learning , 13 (2), 179–212. https://doi.org/10.1007/s11409-018-9183-8 Google Scholar
- Osterhage, J. L. ( 2021 ). Persistent miscalibration for low and high achievers despite practice test feedback in an introductory biology course . Journal of Microbiology & Biology Education , 22 (2), e00139–e00121. https://doi.org /doi: 10.1128/jmbe.00139-21 Medline , Google Scholar
- Pajares, F. ( 1996 ). Self-efficacy beliefs in academic settings . Review of Educational Research , 66 (4), 543–578. https://doi.org/10.3102/00346543066004543 Google Scholar
- Pajares, F. ( 2002 ). Gender and perceived self-efficacy in self-regulated learning . Theory Into Practice , 41 (2), 116–125. https://doi.org/10.1207/s15430421tip4102_8 Google Scholar
- Pfeifer, M. A., & Dolan, E. L. ( 2023 ). Venturing into qualitative research: A practical guide to getting started . Scholarship and Practice of Undergraduate Research , 7 (1), 10–20. Google Scholar
- Pintrich, P. R., & De Groot, E. V. ( 1990 ). Motivational and self-regulated learning components of classroom academic performance . Journal of Educational Psychology , 82 , 33–40. https://doi.org/10.1037/0022-0663.82.1.33 Google Scholar
- Pintrich, P. R., Smith, D. A. F., Garcia, T., & Mckeachie, W. J. ( 1993 ). Reliability and predictive validity of the motivated strategies for learning questionnaire (MSLQ) . Educational and Psychological Measurement , 53 (3), 801–813. https://doi.org/10.1177/0013164493053003024 Google Scholar
- Pressley, M., Borkowski, J., & Schneider, W. ( 1987 ). Cognitive strategies: Good strategy users coordinate metacognition and knowledge . In: Annals of child development (1987) , 4 , 89–129. Google Scholar
- Prevost, L. B., & Lemons, P. P. ( 2016 ). Step by step: Biology undergraduates' problem-solving procedures during multiple-choice assessment . CBE—Life Science Education , 15 (4). https://doi.org/10.1187/cbe.15-12-0255 Google Scholar
- R Core Team . ( 2021 ). R: A language and environment for statistical computing . Vienna, Austria: R Foundation for Statistical Computing: www.R-project.org Google Scholar
- Reber, R., & Greifeneder, R. ( 2017 ). Processing fluency in education: How metacognitive feelings shape learning, belief formation, and affect . Educational Psychologist , 52 (2), 84–103. https://doi.org/10.1080/00461520.2016.1258173 Google Scholar
- Rickey, D., & Stacy, A. M. ( 2000 ). The role of metacognition in learning chemistry . Journal of Chemical Education , 77 (7), 915. https://doi.org/10.1021/ed077p915 Google Scholar
- Saldaña, J. ( 2021 ). The coding manual for qualitative researchers, 4E ed. Thousand Oaks, CA: SAGE Publications Inc. Google Scholar
- Samuels, S. J., Ediger, K.-A. M., Willcutt, J. R., & Palumbo, T. J. ( 2005 ). Role of automaticity in metacognition and literacy instruction . In Israel, S. E.Block, C. C.Bauserman, K. L.Kinnucan-Welsch, K. (Eds.), Metacognition in Literacy Learning (pp. 41–59). Mahwah, NJ: Lawrence Erlbaum Associates, Inc. Google Scholar
- Sandi-Urena, S., Cooper, M. M., & Stevens, R. H. ( 2011 ). Enhancement of metacognition use and awareness by means of a collaborative intervention . International Journal of Science Education , 33 (3), 323–340. https://doi.org/10.1080/09500690903452922 Google Scholar
- Schellings, G. L. M., van Hout-Wolters, B. H. A. M., Veenman, M. V. J., & Meijer, J. ( 2013 ). Assessing metacognitive activities: The in-depth comparison of a task-specific questionnaire with think-aloud protocols . European Journal of Psychology of Education , 28 (3), 963–990. www.jstor.org/stable/23581531 Google Scholar
- Schraw, G. ( 1998 ). Promoting general metacognitive awareness . Instructional Science , 26 (1), 113–125. https://doi.org/10.1023/A:1003044231033 Google Scholar
- Schraw, G., & Dennison, R. S. ( 1994 ). Assessing metacognitive awareness . Contemporary Educational Psychology , 19 (4), 460–475. https://doi.org/10.1006/ceps.1994.1033 Google Scholar
- Schraw, G., Dunkle, M. E., & Bendixen, L. D. ( 1995 ). Cognitive processes in well-defined and ill-defined problem solving . Applied Cognitive Psychology , 9 (6), 523–538. https://doi.org/10.1002/acp.2350090605 Google Scholar
- Schraw, G., & Moshman, D. ( 1995 ). Metacognitive theories . Educational Psychology Review , 7 (4), 351–371. Google Scholar
- Smith, J. I., Combs, E. D., Nagami, P. H., Alto, V. M., Goh, H. G., Gourdet, M. A. A., ... & Tanner, K. D. ( 2013 ). Development of the biology card sorting task to measure conceptual expertise in biology . CBE—Life Sciences Education , 12 (4), 628–644. https://doi.org/10.1187/cbe.13-05-0096 Link , Google Scholar
- Stanton, J. D., Neider, X. N., Gallegos, I. J., & Clark, N. C. ( 2015 ). Differences in Metacognitive Regulation in Introductory Biology Students: When Prompts Are Not Enough . CBE—Life Sciences Education , 14 (2), ar15. https://doi.org/10.1187/cbe.14-08-0135 Link , Google Scholar
- Stanton, J. D., Dye, K. M., & Johnson, M. S. ( 2019 ). Knowledge of Learning Makes a Difference: A Comparison of Metacognition in Introductory and Senior-Level Biology Students . CBE—Life Sciences Education , 18 (2), ar24. https://doi.org/10.1187/cbe.18-12-0239 Link , Google Scholar
- Stanton, J. D., Sebesta, A. J., & Dunlosky, J. ( 2021 ). Fostering Metacognition to Support Student Learning and Performance . CBE—Life Sciences Education , 20 (2), fe3. https://doi.org/10.1187/cbe.20-12-0289 Link , Google Scholar
- Stewart, J., Henderson, R., Michaluk, L., Deshler, J., Fuller, E., & Rambo-Hernandez, K. ( 2020 ). Using the social cognitive theory framework to chart gender differences in the developmental trajectory of STEM self-efficacy in science and engineering students . Journal of Science Education and Technology , 29 (6), 758–773. https://doi.org/10.1007/s10956-020-09853-5 Google Scholar
- Stylianou-Georgiou, A., & Papanastasiou, E. C. ( 2017 ). Answer changing in testing situations: The role of metacognition in deciding which answers to review . Educational Research and Evaluation , 23 (3-4), 102–118. https://doi.org/10.1080/13803611.2017.1390479 Google Scholar
- Taasoobshirazi, G., & Glynn, S. M. ( 2009 ). College students solving chemistry problems: A theoretical model of expertise . Journal of Research in Science Teaching , 46 (10), 1070–1089. https://doi.org/10.1002/tea.20301 Google Scholar
- Tomanek, D., & Montplaisir, L. ( 2004 ). Students' studying and approaches to learning in introductory biology . Cell Biology Education , 3 (4), 253–262. https://doi.org/10.1187/cbe.04-06-0041 Link , Google Scholar
- Tracy, S. J. ( 2010 ). Qualitative quality: Eight “big-tent” criteria for excellent qualitative research . Qualitative Inquiry , 16 (10), 837–851. https://doi.org/10.1177/1077800410383121 Google Scholar
- Usher, E. L. ( 2009 ). Sources of middle school students’ self-efficacy in mathematics: A qualitative investigation . American Educational Research Journal , 46 (1), 275–314. https://doi.org/10.3102/0002831208324517 Google Scholar
- Veenman, M. V. J., & Spaans, M. A. ( 2005 ). Relation between intellectual and metacognitive skills: Age and task differences . Learning and Individual Differences , 15 (2), 159–176. https://doi.org/10.1016/j.lindif.2004.12.001 Google Scholar
- Veenman, M. V. J., Van Hout-Wolters, B. H. A. M., & Afflerbach, P. ( 2006 ). Metacognition and learning: Conceptual and methodological considerations . Metacognition and Learning , 1 (1), 3–14. https://doi.org/10.1007/s11409-006-6893-0 Google Scholar
- Versteeg, M., Bressers, G., Wijnen-Meijer, M., Ommering, B. W. C., de Beaufort, A. J., & Steendijk, P. ( 2021 ). What were you thinking? Medical students’ metacognition and perceptions of self-regulated learning . Teaching and Learning in Medicine , 33 (5), 473–482. https://doi.org/10.1080/10401334.2021.1889559 Google Scholar
- Villafañe, S. M., Bailey, C. P., Loertscher, J., Minderhout, V., & Lewis, J. E. ( 2011 ). Development and analysis of an instrument to assess student understanding of foundational concepts before biochemistry coursework* . Biochemistry and Molecular Biology Education , 39 (2), 102–109. https://doi.org/10.1002/bmb.20464 Medline , Google Scholar
- Wolters, C. A. ( 1998 ). Self-regulated learning and college students' regulation of motivation . Journal of Educational Psychology , 90 , 224–235. Google Scholar
- Wolters, C. A. ( 2003 ). Regulation of motivation: Evaluating an underemphasized aspect of self-regulated learning . Educational Psychologist , 38 (4), 189–205. Google Scholar
- Yeo, G. B., & Neal, A. ( 2006 ). An examination of the dynamic relationship between self-efficacy and performance across levels of analysis and levels of specificity . Journal of Applied Psychology , 91 (5), 1088–1101. https://doi.org/10.1037/0021-9010.91.5.1088 Google Scholar
- Zepeda, C. D., Martin, R. S., & Butler, A. C. ( 2020 ). Motivational strategies to engage learners in desirable difficulties . Journal of Applied Research in Memory and Cognition , 9 (4), 468–474. https://doi.org/10.1016/j.jarmac.2020.08.007 Google Scholar
- Zheng, J., Xing, W., & Zhu, G. ( 2019 ). Examining sequential patterns of self- and socially shared regulation of STEM learning in a CSCL environment . Computers & Education , 136 , 34–48. https://doi.org/10.1016/j.compedu.2019.03.005 Google Scholar

Submitted: 21 August 2023 Revised: 26 January 2024 Accepted: 9 February 2024
© 2024 S. M. Halmo et al. CBE—Life Sciences Education © 2024 The American Society for Cell Biology. This article is distributed by The American Society for Cell Biology under license from the author(s). It is available to the public under an Attribution–Noncommercial–Share Alike 3.0 Unported Creative Commons License (http://creativecommons.org/licenses/by-nc-sa/3.0).
We would like to thank Dr. Paula Lemons for allowing us to use problems developed in her research program for this study and for her helpful feedback during the writing process, the College Learning Study participants for their willingness to participate in this study, Dr. Mariel Pfeifer for her assistance conducting interviews and continued discussion of the data during the writing of this manuscript, C.J. Zajic for his contribution to preliminary data analysis, and Emily K. Bremers, Rayna Carter, and the UGA BERG community for their thoughtful feedback on earlier versions of this manuscript. We are also grateful for the feedback from the monitoring editor and reviewers at LSE, which strengthened the manuscript. This material is based on work supported by the National Science Foundation under Grant Number 1942318. Any opinions, findings, and conclusions or recommendations expressed in this material are those of the authors and do not necessarily reflect the views of the National Science Foundation.
- Resource Collection
- State Resources
Access Resources for State Adult Education Staff
- Topic Areas
- About the Collection
- Review Process
- Reviewer Biographies
- Federal Initiatives
- COVID-19 Support
- ADVANCE Integrated Education and Training (IET)
- IET Train-the Trainer Resources
- IET Resource Repository
- Program Design
- Collaboration and Industry Engagement
- Curriculum and Instruction
- Policy and Funding
- Program Management - Staffing -Organization Support
- Student Experience and Progress
- Adult Numeracy Instruction 2.0
- Advancing Innovation in Adult Education
- Bridge Practices
- Holistic Approach to Adult Ed
- Integrated Education and Training (IET) Practices
- Secondary Credentialing Practices
- Business-Adult Education Partnerships Toolkit
- Partnerships: Business Leaders
- Partnerships: Adult Education Providers
- Success Stories
- Digital Literacy Initiatives
- Digital Resilience in the American Workforce
- Landscape Scan
- Publications and Resources
- DRAW Professional Development Resources
- Employability Skills Framework
- Enhancing Access for Refugees and New Americans
- English Language Acquisition
- Internationally-Trained Professionals
- Rights and Responsibilities of Citizenship and Civic Participation
- Workforce Preparation Activities
- Workforce Training
- Integrated Education and Training in Corrections
- LINCS ESL PRO
- Integrating Digital Literacy into English Language Instruction
- Meeting the Language Needs of Today's English Language Learner
- Open Educational Resources (OER) for English Language Instruction
- Preparing English Learners for Work and Career Pathways
- Recommendations for Applying These Resources Successfully
- Moving Pathways Forward
- Career Pathways Exchange
- Power in Numbers
- Adult Learner Stories
- Meet Our Experts
- Newsletters
- Reentry Education Tool Kit
- Education Services
- Strategic Partnerships
- Sustainability
- Transition Processes
- Program Infrastructure
- SIA Resources and Professional Development
- Fulfilling the Instructional Shifts
- Observing in Classrooms
- SIA ELA/Literacy Videos
- SIA Math Videos
- SIA ELA Videos
- Conducting Curriculum Reviews
- Boosting English Learner Instruction
- Student Achievement in Reading
- TEAL Just Write! Guide
- Introduction
- Fact Sheet: Research-Based Writing Instruction
- Increase the Amount of Student Writing
- Fact Sheet: Adult Learning Theories
- Fact Sheet: Student-Centered Learning
- Set and Monitor Goals
- Fact Sheet: Self-Regulated Learning
- Fact Sheet: Metacognitive Processes
- Combine Sentences
- Teach Self-Regulated Strategy Development
- Fact Sheet: Self-Regulated Strategy Development
- Teach Summarization
- Make Use of Frames
- Provide Constructive Feedback
- Apply Universal Design for Learning
- Fact Sheet: Universal Design for Learning
- Check for Understanding
- Fact Sheet: Formative Assessment
- Differentiated Instruction
- Fact Sheet: Differentiated Instruction
- Gradual Release of Responsibility
- Join a Professional Learning Community
- Look at Student Work Regularly
- Fact Sheet: Effective Lesson Planning
- Use Technology Effectively
- Fact Sheet: Technology-Supported Writing Instruction
- Project Resources
- Summer Institute
- Teacher Effectiveness in Adult Education
- Adult Education Teacher Induction Toolkit
- Adult Education Teacher Competencies
- Teacher Effectiveness Online Courses
- Teaching Skills that Matter
- Teaching Skills that Matter Toolkit Overview
- Teaching Skills that Matter Civics Education
- Teaching Skills that Matter Digital Literacy
- Teaching Skills that Matter Financial Literacy
- Teaching Skills that Matter Health Literacy
- Teaching Skills that Matter Workforce Preparation
- Teaching Skills that Matter Other Tools and Resources
- Technology-Based Coaching in Adult Education
- Technical Assistance and Professional Development
- About LINCS
- History of LINCS
- LINCS Guide
- Style Guide
TEAL Center Fact Sheet No. 4: Metacognitive Processes
Metacognition is one’s ability to use prior knowledge to plan a strategy for approaching a learning task, take necessary steps to problem solve, reflect on and evaluate results, and modify one’s approach as needed. It helps learners choose the right cognitive tool for the task and plays a critical role in successful learning.
What Is Metacognition?
Metacognition refers to awareness of one’s own knowledge—what one does and doesn’t know—and one’s ability to understand, control, and manipulate one’s cognitive processes (Meichenbaum, 1985). It includes knowing when and where to use particular strategies for learning and problem solving as well as how and why to use specific strategies. Metacognition is the ability to use prior knowledge to plan a strategy for approaching a learning task, take necessary steps to problem solve, reflect on and evaluate results, and modify one’s approach as needed. Flavell (1976), who first used the term, offers the following example: I am engaging in Metacognition if I notice that I am having more trouble learning A than B; if it strikes me that I should double check C before accepting it as fact (p. 232).
Cognitive strategies are the basic mental abilities we use to think, study, and learn (e.g., recalling information from memory, analyzing sounds and images, making associations between or comparing/contrasting different pieces of information, and making inferences or interpreting text). They help an individual achieve a particular goal, such as comprehending text or solving a math problem, and they can be individually identified and measured. In contrast, metacognitive strategies are used to ensure that an overarching learning goal is being or has been reached. Examples of metacognitive activities include planning how to approach a learning task, using appropriate skills and strategies to solve a problem, monitoring one’s own comprehension of text, self-assessing and self-correcting in response to the self-assessment, evaluating progress toward the completion of a task, and becoming aware of distracting stimuli.
Elements of Metacognition
Researchers distinguish between metacognitive knowledge and metacognitive regulation (Flavell, 1979, 1987; Schraw & Dennison, 1994). Metacognitive knowledge refers to what individuals know about themselves as cognitive processors, about different approaches that can be used for learning and problem solving, and about the demands of a particular learning task. Metacognitive regulation refers to adjustments individuals make to their processes to help control their learning, such as planning, information management strategies, comprehension monitoring, de-bugging strategies, and evaluation of progress and goals. Flavell (1979) further divides metacognitive knowledge into three categories:
- Person variables: What one recognizes about his or her strengths and weaknesses in learning and processing information.
- Task variables: What one knows or can figure out about the nature of a task and the processing demands required to complete the task—for example, knowledge that it will take more time to read, comprehend, and remember a technical article than it will a similar-length passage from a novel.
- Strategy variables: The strategies a person has “at the ready” to apply in a flexible way to successfully accomplish a task; for example, knowing how to activate prior knowledge before reading a technical article, using a glossary to look up unfamiliar words, or recognizing that sometimes one has to reread a paragraph several times before it makes sense.
Livingston (1997) provides an example of all three variables: “I know that I ( person variable ) have difficulty with word problems ( task variable ), so I will answer the computational problems first and save the word problems for last ( strategy variable ).”
Why Teach Metacognitive Skills?
Research shows that metacognitive skills can be taught to students to improve their learning (Nietfeld & Shraw, 2002; Thiede, Anderson, & Therriault, 2003).
Constructing understanding requires both cognitive and metacognitive elements. Learners “construct knowledge” using cognitive strategies, and they guide, regulate, and evaluate their learning using metacognitive strategies. It is through this “thinking about thinking,” this use of metacognitive strategies, that real learning occurs. As students become more skilled at using metacognitive strategies, they gain confidence and become more independent as learners.
Individuals with well-developed metacognitive skills can think through a problem or approach a learning task, select appropriate strategies, and make decisions about a course of action to resolve the problem or successfully perform the task. They often think about their own thinking processes, taking time to think about and learn from mistakes or inaccuracies (North Central Regional Educational Laboratory, 1995). Some instructional programs encourage students to engage in “metacognitive conversations” with themselves so that they can “talk” with themselves about their learning, the challenges they encounter, and the ways in which they can self-correct and continue learning.
Moreover, individuals who demonstrate a wide variety of metacognitive skills perform better on exams and complete work more efficiently—they use the right tool for the job, and they modify learning strategies as needed, identifying blocks to learning and changing tools or strategies to ensure goal attainment. Because Metacognition plays a critical role in successful learning, it is imperative that instructors help learners develop metacognitively.
What’s the Research?
Metacognitive strategies can be taught (Halpern, 1996), they are associated with successful learning (Borkowski, Carr, & Pressley, 1987). Successful learners have a repertoire of strategies to select from and can transfer them to new settings (Pressley, Borkowski, & Schneider, 1987). Instructors need to set tasks at an appropriate level of difficulty (i.e., challenging enough so that students need to apply metacognitive strategies to monitor success but not so challenging that students become overwhelmed or frustrated), and instructors need to prompt learners to think about what they are doing as they complete these tasks (Biemiller & Meichenbaum, 1992). Instructors should take care not to do the thinking for learners or tell them what to do because this runs the risk of making students experts at seeking help rather than experts at thinking about and directing their own learning. Instead, effective instructors continually prompt learners, asking “What should you do next?”
McKeachie (1988) found that few college instructors explicitly teach strategies for monitoring learning. They assume that students have already learned these strategies in high school. But many have not and are unaware of the metacognitive process and its importance to learning. Rote memorization is the usual—and often the only—learning strategy employed by high school students when they enter college (Nist, 1993). Simpson and Nist (2000), in a review of the literature on strategic learning, emphasize that instructors need to provide explicit instruction on the use of study strategies. The implication for ABE programs is that it is likely that ABE learners need explicit instruction in both cognitive and metacognitive strategies. They need to know that they have choices about the strategies they can employ in different contexts, and they need to monitor their use of and success with these strategies.
Recommended Instructional Strategies
Instructors can encourage ABE learners to become more strategic thinkers by helping them focus on the ways they process information. Self-questioning, reflective journal writing, and discussing their thought processes with other learners are among the ways that teachers can encourage learners to examine and develop their metacognitive processes.
Fogarty (1994) suggests that Metacognition is a process that spans three distinct phases, and that, to be successful thinkers, students must do the following:
- Develop a plan before approaching a learning task, such as reading for comprehension or solving a math problem.
- Monitor their understanding; use “fix-up” strategies when meaning breaks down.
- Evaluate their thinking after completing the task.
Instructors can model the application of questions, and they can prompt learners to ask themselves questions during each phase. They can incorporate into lesson plans opportunities for learners to practice using these questions during learning tasks, as illustratetd in the following examples:
- During the planning phase, learners can ask, What am I supposed to learn? What prior knowledge will help me with this task? What should I do first? What should I look for in this reading? How much time do I have to complete this? In what direction do I want my thinking to take me?
- During the monitoring phase, learners can ask, How am I doing? Am I on the right track? How should I proceed? What information is important to remember? Should I move in a different direction? Should I adjust the pace because of the difficulty? What can I do if I do not understand?
- During the evaluation phase, learners can ask, H ow well did I do? What did I learn? Did I get the results I expected? What could I have done differently? Can I apply this way of thinking to other problems or situations? Is there anything I don’t understand—any gaps in my knowledge? Do I need to go back through the task to fill in any gaps in understanding? How might I apply this line of thinking to other problems?
Rather than viewing reading, writing, science, social studies, and math only as subjects or content to be taught, instructors can see them as opportunities for learners to reflect on their learning processes. Examples follow for each content area:
- Reading: Teach learners how to ask questions during reading and model “think-alouds.” Ask learners questions during read-alouds and teach them to monitor their reading by constantly asking themselves if they understand what the text is about. Teach them to take notes or highlight important details, asking themselves, “Why is this a key phrase to highlight?” and “Why am I not highlighting this?”
- Writing: Model prewriting strategies for organizing thoughts, such as brainstorming ideas using a word web, or using a graphic organizer to put ideas into paragraphs, with the main idea at the top and the supporting details below it.
- Social Studies and Science: Teach learners the importance of using organizers such as KWL charts, Venn diagrams, concept maps , and anticipation/reaction charts to sort information and help them learn and understand content. Learners can use organizers prior to a task to focus their attention on what they already know and identify what they want to learn. They can use a Venn diagram to identify similarities and differences between two related concepts.
- Math: Teach learners to use mnemonics to recall steps in a process, such as the order of mathematical operations. Model your thought processes in solving problems—for example, “This is a lot of information; where should I start? Now that I know____, is there something else I know?”
The goal of teaching metacognitive strategies is to help learners become comfortable with these strategies so that they employ them automatically to learning tasks, focusing their attention, deriving meaning, and making adjustments if something goes wrong. They do not think about these skills while performing them but, if asked what they are doing, they can usually accurately describe their metacognitive processes.
Biemiller, A., & Meichenbaum, D. (1992). The nature and nurture of the self-directed learner. Educational Leadership, 50, 75–80.
Borkowski, J., Carr, M., & Pressely, M. (1987). “Spontaneous” strategy use: Perspectives from metacognitive theory. Intelligence, 11, 61–75.
Flavell, J. H. (1979). Metacognition and cognitive monitoring: A new area of cognitive-developmental inquiry. American Psychologist, 34, 906–911.
Flavell, J. H. (1976). Metacognitive aspects of problem solving. In L. B. Resnick (Ed.), The nature of intelligence (pp. 231–236). Hillsdale, NJ: Lawrence Erlbaum Associates.
Flavell, J. H. (1987). Speculations about the nature and development of metacognition. In F. E. Weinert & R. H. Kluwe (Eds.), Metacognition, motivation, and understanding (pp. 21–29). Hillside, NJ: Lawrence Erlbaum Associates.
Fogarty, R. (1994). How to teach for metacognition. Palatine, IL: IRI/Skylight Publishing.
Halpern, D. F. (1996). Thought and knowledge: An introduction to critical thinking. Mahwah, NJ: Lawrence Erlbaum Associates.
Livingston, J. A. (1997). Metacognition: An overview. Retrieved December 27, 2011 from http://gse.buffalo.edu/fas/shuell/CEP564/Metacog.htm
McKeachie, W. J. (1988). The need for study strategy training. In C. E. Weinstein, E. T. Goetz, & P. A. Alexander (Eds.), Learning and study strategies: Issues in assessment, instruction, and evaluation (pp. 3–9). New York: Academic Press.
Meichenbaum, D. (1985). Teaching thinking: A cognitive-behavioral perspective. In S. F., Chipman, J. W. Segal, & R. Glaser (Eds.), Thinking and learning skills, Vol. 2: Research and open questions. Hillsdale, NJ: Lawrence Erlbaum Associates.
North Central Regional Educational Laboratory. (1995). Strategic teaching and reading project guidebook. Retrieved December 27, 2011
Nietfeld, J. L., & Shraw, G. (2002). The effect of knowledge and strategy explanation on monitoring accuracy. Journal of Educational Research, 95, 131–142.
Nist, S. (1993). What the literature says about academic literacy. Georgia Journal of Reading, Fall-Winter, 11–18.
Pressley, M., Borkowski, J. G., & Schneider, W. (1987). Cognitive strategies: Good strategy users coordinate metacognition and knowledge. In R. Vasta, & G. Whitehurst (Eds.), Annals of child development, 4, 80–129. Greenwich, CT: JAI Press.
Schraw, G., & Dennison, R. S. (1994). Assessing metacognitive awareness. Contemporary Educational Psychology, 19, 460–475.
Simpson, M. L., & Nist, S. L. (2000). An update on strategic learning: It’s more than textbook reading strategies. Journal of Adolescent and Adult Literacy, 43 (6) 528–541.
Thiede, K. W., Anderson, M. C., & Therriault, D. (2003). Accuracy of metacognitive monitoring affects learning of texts. Journal of Educational Psychology, 95, 66–73.
Authors: TEAL Center staff
Reviewed by: David Scanlon, Boston College
About the TEAL Center: The Teaching Excellence in Adult Literacy (TEAL) Center is a project of the U.S. Department of Education, Office of Career, Technical, and Adult Education (OCTAE), designed to improve the quality of teaching in adult education in the content areas.
Strategies for teaching metacognition in classrooms
Subscribe to the center for universal education bulletin, david owen and do david owen history and politics teacher - melbourne high school - victoria, australia alvin vista alvin vista former brookings expert @alvin_vista.
November 15, 2017
This is the third piece in a six-part blog series on teaching 21st century skills , including problem solving , metacognition , critical thinking , and collaboration , in classrooms.
Metacognition is thinking about thinking. It is an increasingly useful mechanism to enhance student learning, both for immediate outcomes and for helping students to understand their own learning processes. So metacognition is a broad concept that refers to the knowledge and thought processes regarding one’s own learning. Importantly, there is research evidence (e.g., Moely and colleagues, 1995 ; Schraw, 1998 ) that metacognition is a teachable skill that is central to other skills sets such as problem solving, decisionmaking, and critical thinking. Reflective thinking, as a component of metacognition, is the ability to reflect critically on learning experiences and processes in order to inform future progress.
David Owen, who teaches history and politics at Melbourne High School in Victoria, Australia, discusses a simple but effective approach to encourage student self-reflection:
I have rethought some of my classroom strategies this year. I teach at a secondary school which prides itself on its high level of student achievement, and I had always believed my students performed accordingly. They always ask for help before, during, and after class. Their varied queries could be superficial knowledge-based questions or more general questions about their progress, but I’d always read this habit as a sign that my students had an open mindset: they were inquisitive, cared about their learning, and charted their progress.
But having students asking a million questions of the teacher poses another challenge entirely, which can be framed: “Why aren’t students asking these questions of themselves?”
Recent shifts in pedagogy have emphasized the importance of encouraging students to figure out how to be independent, self-regulated learners. The teacher cannot be there to hold their hand beyond school. This demands that students reflect on their learning in meaningful ways. It also requires students be critical analysts of their own thinking in order to overcome complex or unexpected problems.
I’ve begun to highlight strategies which might better encourage this kind of metacognition. For younger adolescents, I’ve found that “Exit Tickets” are an opportunity for students to reflect on what they have accomplished and what they could improve on. Exit Tickets are a family of feedback tools that students complete for a few minutes at the end of each lesson. They prompt students to think about how and what they learn, as well as what challenges they are still facing.
“Traffic Lights” is a simple yet effective Exit Ticket which emphasizes three key factors:
- When students encountered a challenge;
- When students had thought differently about something; and
- When students were learning well.
Over time I’ve found myself more interested in student responses to the Yellow Light, because it requires students to think about how they were thinking, rather than when (the emphasis of the Red and Green lights). The Yellow Light encourages reflective thinking as well as “thinking about thinking, or what is known as metacognition.” The possibilities for Exit Tickets are numerous and easily adaptable to the content and specific skills taught in any lesson.
Another example of reflective, self-directed learning which is suited to group work is setting a classroom rule that groups ask a question together , rather than individually. What this means is that rather than immediately ask the teacher for help, a student who has encountered a problem must consult with their group first. If the group cannot collectively find the solution, they can raise their hands simultaneously—a sign that the question has been fielded to the group already. There are various ways to modify this rule: highlighting with traffic-light colors, like in the Exit Ticket activity, is one such example.
For older students, setting a few rules before requesting aid from the teacher has seen their self-directed learning—and my feedback—improve markedly. I have emphasized that students seek specific feedback concerning their trial exams. I ask students to ensure they have highlighted and annotated their responses before seeing me. This approach shifts student thinking from the simplistic “submission to feedback” principle towards a more involved process, where students must consider what feedback they would want, what advice they would give themselves, and where they think they need to improve. This approach encourages the students to independently exercise control over their learning and progress, thereby making them more independent and self-directed learners.
Evidence supporting the impact of metacognition suggests that students applying metacognitive strategies to learning tasks outperform those who do not ( Mason, Boldrin & Ariasi, 2010 ; Dignath & Buettner, 2008 ). The classroom approaches that David Owen uses in his classroom demonstrates one way of developing parts of this important complex skill. Interestingly, although these skills are so important in our modern world, the approaches discussed here are practical, do not require 21st century technology or resources, and can be applied in almost any classroom setting.
Related Content
Esther Care, Helyn Kim, Alvin Vista
October 17, 2017
Kate Mills, Helyn Kim
October 31, 2017
Global Education K-12 Education
Global Economy and Development
Center for Universal Education
Katharine Meyer
May 7, 2024
Jamie Klinenberg, Jon Valant, Nicolas Zerbino
Thinley Choden
May 3, 2024

How it works
Transform your enterprise with the scalable mindsets, skills, & behavior change that drive performance.
Explore how BetterUp connects to your core business systems.
We pair AI with the latest in human-centered coaching to drive powerful, lasting learning and behavior change.
Build leaders that accelerate team performance and engagement.
Unlock performance potential at scale with AI-powered curated growth journeys.
Build resilience, well-being and agility to drive performance across your entire enterprise.
Transform your business, starting with your sales leaders.
Unlock business impact from the top with executive coaching.
Foster a culture of inclusion and belonging.
Accelerate the performance and potential of your agencies and employees.
See how innovative organizations use BetterUp to build a thriving workforce.
Discover how BetterUp measurably impacts key business outcomes for organizations like yours.
A demo is the first step to transforming your business. Meet with us to develop a plan for attaining your goals.

- What is coaching?
Learn how 1:1 coaching works, who its for, and if it's right for you.
Accelerate your personal and professional growth with the expert guidance of a BetterUp Coach.
Types of Coaching
Navigate career transitions, accelerate your professional growth, and achieve your career goals with expert coaching.
Enhance your communication skills for better personal and professional relationships, with tailored coaching that focuses on your needs.
Find balance, resilience, and well-being in all areas of your life with holistic coaching designed to empower you.
Discover your perfect match : Take our 5-minute assessment and let us pair you with one of our top Coaches tailored just for you.

Research, expert insights, and resources to develop courageous leaders within your organization.
Best practices, research, and tools to fuel individual and business growth.
View on-demand BetterUp events and learn about upcoming live discussions.
The latest insights and ideas for building a high-performing workplace.
- BetterUp Briefing
The online magazine that helps you understand tomorrow's workforce trends, today.
Innovative research featured in peer-reviewed journals, press, and more.
Founded in 2022 to deepen the understanding of the intersection of well-being, purpose, and performance
We're on a mission to help everyone live with clarity, purpose, and passion.
Join us and create impactful change.
Read the buzz about BetterUp.
Meet the leadership that's passionate about empowering your workforce.

For Business
For Individuals
What are metacognitive skills? Examples in everyday life
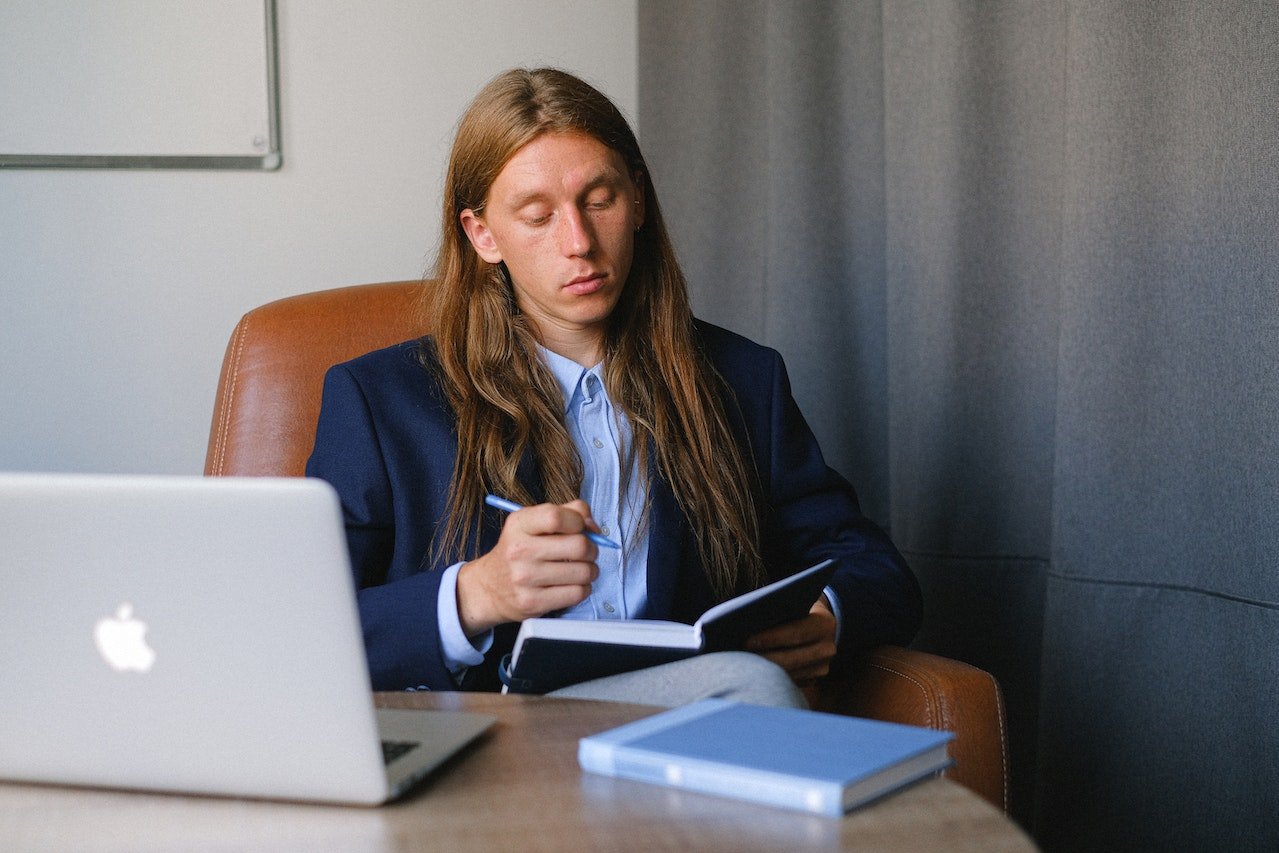
Jump to section
What are metacognitive skills?
Examples of metacognitive skills, how to improve metacognitive skills, take charge of your mind.
When facing a career change or deciding to switch jobs, you might update the hard and soft skills on your resume. You could even take courses to upskill and expand your portfolio.
But some growth happens off the page. Your metacognitive skills contribute to your learning process and help you look inward to self-reflect and monitor your growth. They’re like a golden ticket toward excellence in both academia and your career path , always pushing you further.
A deeper understanding of metacognition, along with effective strategies for developing related skills, opens the door to heightened personal and professional development . Metacognitive thinking might just be the tool you need to reach your academic and career goals
Metacognitive skills are the soft skills you use to monitor and control your learning and problem-solving processes , or your thinking about thinking. This self-understanding is known as metacognition theory, a term that the American developmental psychologist John H. Flavell coined in the 1970s .
It might sound abstract, but these skills are mostly about self-awareness , learning, and organizing your thoughts. Metacognitive strategies include thinking out loud and answering reflective questions. They’re often relevant for students who need to memorize concepts fast or absorb lots of information at once.
But metacognition is important for everyone because it helps you retain information more efficiently and feel more confident about what you know. One meta-analysis of many studies showed that being aware of metacognitive strategies has a strong positive impact on teaching and learning , and that knowing how to plan ahead was a key indicator of future success.
Understanding your cognition and how you learn is a fundamental step in optimizing your educational process. To make the concept more tangible, here are a few cognitive skills examples:
Goal setting
One of the foremost metacognitive skills is knowing how to set goals — recognizing what your ambitions are and fine-tuning them into manageable and attainable objectives. The SMART goal framework is a good place to start because it dives deeper into what you know you can realistically achieve.
Whether it's a personal goal of grasping a complex concept, a professional goal of developing a new skill set, or a financial goal of achieving a budgeting milestone , setting a concrete goal helps you know what you’re working toward. It’s the first step to self-directed learning and achievement, giving you a destination for your path.
Planning and organization
Planning is an essential metacognition example because it sketches out the route you'll take to reach your goal, as well as identifying and collecting the specific strategies, resources, and support mechanisms you'll need along the way. It’s an in-demand skill for many jobs, but it also helps you learn new things.
Creating and organizing a plan is where you contemplate the best methods for learning, evaluate the materials and resources at your disposal, and determine the most efficient time management strategies. Even though it’s a concrete skill, it falls under the umbrella of metacognition because it involves self-awareness about your learning style and abilities.

Problem-solving
Central to metacognition is problem-solving, a higher-order cognitive process requiring both creative and critical thinking skills . Solving problems both at work and during learning begins with recognizing the issue at hand, analyzing the details, and considering potential solutions. The next step is selecting the most promising solution from the pool of possibilities and evaluating the results after implementation.
The problem-solving process gives you the opportunity to grow from your mistakes and practice trial and error. It also helps you reflect and refine your approach for future endeavors. These qualities make it central to metacognition’s inward-facing yet action-oriented processes.
Concentration
Concentration allows you to fully engage with the information you’re processing and retain new knowledge. It involves a high degree of mental fitness , which you can develop with metacognition. Most tasks require the ability to ignore distractions , resist procrastination , and maintain a steady focus on the task at hand.
This skill is paramount when it comes to work-from-home settings or jobs with lots of moving parts where countless distractions are constantly vying for your attention. And training your mind to focus better in general can also increase your learning efficacy and overall productivity.
Self-reflection
The practice of self-reflection involves continually assessing your performance, cognitive strategies, and experiences to foster self-improvement . It's a type of mental debriefing where you look back on your actions and outcomes, examining them critically to gain insight and experience valuable lessons.
Reflective practice can help you identify what worked well, what didn't, and why, giving you the opportunity to make necessary adjustments for future actions. This continuous process enhances your learning and helps you adapt to new changes and strategies.

Metacognition turns you into a self-aware problem solver, empowering you to take control of your education and become a more efficient thinker. Although it’s helpful for students, you can also apply it in the workplace while brainstorming and discovering new ways to fulfill your roles and responsibilities .
Here are some examples of metacognitive strategies and how to cultivate your abilities:
1. Determine your learning style
Are you a visual learner who thrives on images, diagrams, and color-coded notes? Are you an auditory learner who benefits more from verbal instructions, podcasts , or group discussions? Or are you a kinesthetic learner who enjoys hands-on experiences, experiments, or physical activities?
Metacognition in education is critical because it teaches you to recognize the way you intake information — the first step to effective strategies that help you truly retain information. By identifying your learning style, you can tailor your goals and study strategies to suit your strengths, maximizing your cognitive potential and improving your understanding of new material.
2. Find deeper meaning in what you read
Merely skimming the surface of the text you read won't lead to profound understanding or long-term retention. Instead, dive deep into the material. Employ reading strategies like note-taking, highlighting, and summarizing to help information enter your brain.
If that process doesn’t work for you, try using brainstorming techniques like mind mapping to tease out the underlying themes and messages. This depth of processing enhances comprehension and allows you to connect new information to prior knowledge, promoting meaningful learning.

3. Write organized plans
Deconstruct your tasks into manageable units and create a comprehensive, step-by-step plan. Having a detailed guide breaks down large, intimidating tasks into bite-sized, achievable parts, reduces the risk of procrastination, and helps manage cognitive load. This process frees up your mental energy for higher-order thinking.
4. Ask yourself open-ended questions
Metacognitive questioning is a powerful tool for fostering self-awareness. Asking good questions like “What am I trying to achieve?” and “Why did this approach work or not work?” facilitates a deeper understanding of your education style, promotes critical thinking, and enables self-directed learning. Your answers will pave the way for improved processes.
5. Ask for feedback
External perspectives offer valuable insights into your thinking patterns and strategies. Seek feedback from teachers, peers, or mentors and earn the metacognitive knowledge you need to identify strengths to harness and weaknesses to address. Remember, the objective isn’t to nitpick or micromanage. It’s constructive criticism to help refine your learning process.
6. Self-evaluate
Cultivate a habit of self-assessment and self-monitoring, whether you’re experiencing something new or working on an innovative project. Check in on progress regularly, and compare current performance with your goals. This continuous self-evaluation helps you maintain focus on your objectives and identify when you're going off track, allowing for timely adjustments when necessary.
Introspection is a powerful tool, and you can’t overstate the importance of knowing yourself . After all, building your metacognitive skills begins with a strong foundation of self-awareness and accountability .
7. Focus on solutions
It's easy to let problems and obstacles discourage you during the learning process. But metacognitive skills encourage a solutions-oriented mindset. Instead of fixating on the challenges, shift your focus to identifying, analyzing, and implementing creative solutions .
This proactive approach fosters resilience and adaptability skills in the face of adversity, helping you overcome whatever comes your way. Cultivating this mindset — sometimes known as a growth mindset — also boosts your problem-solving prowess and transforms challenges into opportunities for growth.
The simple act of writing about your learning experiences can heighten your metacognitive awareness. Journaling provides a space to reflect on your thought processes, emotions, and struggles, which can reveal patterns and trends in your behavior. It’s a springboard for improvement that helps you recognize and solve problems as they come.

In the journey of learning and career advancement, metacognitive skills are your compass toward improvement. They empower you to understand your cognitive processes, enhance your strategies, and become a more effective thinker. They’re useful whether you’re just starting a master’s degree or upskilling to earn a promotion.
Remember, the journey to gain metacognitive skills isn’t a race. It’s a personal voyage of self-discovery and growth. Each stride you take toward honing your metacognitive skills is a step toward a more successful, fulfilling, and self-aware life.
Cultivate your creativity
Foster creativity and continuous learning with guidance from our certified Coaches.
Elizabeth Perry, ACC
Elizabeth Perry is a Coach Community Manager at BetterUp. She uses strategic engagement strategies to cultivate a learning community across a global network of Coaches through in-person and virtual experiences, technology-enabled platforms, and strategic coaching industry partnerships. With over 3 years of coaching experience and a certification in transformative leadership and life coaching from Sofia University, Elizabeth leverages transpersonal psychology expertise to help coaches and clients gain awareness of their behavioral and thought patterns, discover their purpose and passions, and elevate their potential. She is a lifelong student of psychology, personal growth, and human potential as well as an ICF-certified ACC transpersonal life and leadership Coach.
What’s convergent thinking? How to be a better problem-solver
Self directed learning is the key to new skills and knowledge, what we can learn from “pandemic thrivers”, what i didn't know before working with a coach: the power of reflection, why asynchronous learning is the key to successful upskilling, how to develop critical thinking skills, 18 excellent educational podcasts to fuel your love of learning, discover 4 types of innovation and how to encourage them, looking inward can make you a better leader, similar articles, 10 problem-solving strategies to turn challenges on their head, curious wanting to learn more is key to career success, learn what process mapping is and how to create one (+ examples), how experiential learning encourages employees to own their learning, what are analytical skills examples and how to level up, critical thinking is the one skillset you can't afford not to master, stay connected with betterup, get our newsletter, event invites, plus product insights and research..
3100 E 5th Street, Suite 350 Austin, TX 78702
- Platform Overview
- Integrations
- Powered by AI
- BetterUp Lead
- BetterUp Manage™
- BetterUp Care™
- Sales Performance
- Diversity & Inclusion
- Case Studies
- Why BetterUp?
- About Coaching
- Find your Coach
- Career Coaching
- Communication Coaching
- Life Coaching
- News and Press
- Leadership Team
- Become a BetterUp Coach
- BetterUp Labs
- Center for Purpose & Performance
- Leadership Training
- Business Coaching
- Contact Support
- Contact Sales
- Privacy Policy
- Acceptable Use Policy
- Trust & Security
- Cookie Preferences
“Quasi-Metacognitive Machines: Why We Don’t Need Morally Trustworthy AI and Communicating Reliability is Enough”
- Research Article
- Open access
- Published: 03 May 2024
- Volume 37 , article number 62 , ( 2024 )
Cite this article
You have full access to this open access article
- John Dorsch ORCID: orcid.org/0000-0002-9974-1487 1 &
- Ophelia Deroy ORCID: orcid.org/0000-0001-9431-3136 1
391 Accesses
2 Altmetric
Explore all metrics
Many policies and ethical guidelines recommend developing “trustworthy AI”. We argue that developing morally trustworthy AI is not only unethical, as it promotes trust in an entity that cannot be trustworthy, but it is also unnecessary for optimal calibration. Instead, we show that reliability, exclusive of moral trust, entails the appropriate normative constraints that enable optimal calibration and mitigate the vulnerability that arises in high-stakes hybrid decision-making environments, without also demanding, as moral trust would, the anthropomorphization of AI and thus epistemically dubious behavior. The normative demands of reliability for inter-agential action are argued to be met by an analogue to procedural metacognitive competence (i.e., the ability to evaluate the quality of one’s own informational states to regulate subsequent action). Drawing on recent empirical findings that suggest providing reliability scores (e.g., F1-scores) to human decision-makers improves calibration in the AI system, we argue that reliability scores provide a good index of competence and enable humans to determine how much they wish to rely on the system.
Similar content being viewed by others
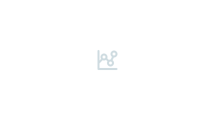
In AI We Trust: Ethics, Artificial Intelligence, and Reliability
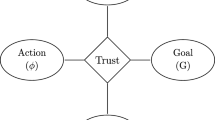
Trust and Trust-Engineering in Artificial Intelligence Research: Theory and Praxis
Can machines be trustworthy.
Avoid common mistakes on your manuscript.
1 Introduction
Trust is an essential factor determining human decisions, one that shapes and constrains our actions towards individuals, organizations, and institutions. Crucially, this role may now be extending to certain sufficiently sophisticated artifacts. Since the emergence of automated systems (AS) and, more recently, systems of artificial intelligence (AI) as integral parts to the decision-making process, questions concerning trust in AS/AI have been at the forefront of research (for a review, see Glikson & Woolley, 2022 ). Footnote 1 This steadily increasing and encompassing role of intelligent machines has culminated in calls from both industry leaders and regulative bodies to develop so-called “trustworthy AI” (EU Guidelines, 2019). This paper aims to expose the wrongheadedness of this call and argues that reliability ought to be a more appropriate goal.
To understand the call for trustworthy AI, it is essential to recognize it as downstream from the earlier push for developing trustworthy automation (Sheridan & Hennessy, 1984 ), which led to the development of models describing the dynamics of human-machine interaction, models that remain deeply influential today (Lee & See, 2004 ). Moreover, careful analysis of the literature on trust in AI reveals its conceptual indebtedness to various models of interpersonal trust , such as those that result from Deutsch’s ( 1977 ) groundbreaking research on conflict resolution. Such influences expose the underlying assumption that continues to shape contemporary discourse in AI ethics: trust not only mediates interpersonal relationships, but also relationships between people and automation. That said, it is unclear what it means to say an automated system is or should be trustworthy.
Through its conceptual analysis of trust, moral philosophy has yielded a relative consensus surrounding the proposal that trustworthiness is constituted chiefly by two components, reliability, on the one hand, and some additional X factor, on the other, with controversy over the exact complement to reliability (Simon, 2020 ). Thus, what might be called, the “trust equation” construes trustworthiness as essentially solving the sum of reliability plus X. But despite decades of debate hardly any agreement has emerged about the correct solution, with prominent views solving X in various ways by appealing to goodwill (Baier, 1986 ), a behavioral disposition to cooperate (Deutsch, 1977 ), an emergent property of social interaction (Luhmann, 1979 ), an emotional sensitivity to moral values (Lahno, 2001 ), or the encapsulation of the trustor’s interests in those of the trustee (Hardin, 2002 ).
While it is unclear whether competing views can be reconciled into one vision of trustworthiness, we suggest that one common thread that strings them together is that moral trust, over and above mere reliance, involves a moral appraisal on the part of the trustor about the trustee. That is, moral trust involves either an affective evaluation or a cognitive judgement about the trustee as either morally agential, responsible, accountable, or rational. Footnote 2 As such, an entity is morally trustworthy if this appraisal is correct relative to a context. For this reason, developing trustworthy AI is routinely regarded as a solution to the “the alignment problem” (Christian, 2020 ), namely, how to ensure that AS/AI, does not operate contrary to our values or facilitates actions that violate them.
That said, it is crucial to ensure that two undoubtedly orthogonal dimensions remain distinct regarding the assumption that trust mediates human relationships to automata. There is the descriptive dimension, how we actually behave toward AI, and there is the prescriptive dimension, how we ought to behave toward AI. If the goal is to describe and predict human behavior, then one might appeal to facts about socially enculturated agents who cannot help but employ trust when dealing with sufficiently sophisticated systems because trust is infused into the fabric of social life (Coeckelbergh, 2012 ). But if the goal is to develop ethical policies for the development and implementation of such systems, we ought to question whether this assumption is justified and ask whether moral trust ought to be employed in our relationship to machines or whether a related, but ultimately distinct concept, such as reliability (exclusive of moral trust), ought to modulate behavior toward automation. Footnote 3
It is thus a matter of deep controversy whether trustworthy AI is part of the solution to the alignment problem or rather carves a larger cleft between technology and our values. While some researchers focus primarily on describing – and to limited degrees, justifying – our tendency to treat AI as trustworthy, often in an appeal to “diffuse forms of trust” that describe the blurring of lines between artificial agents and the humans responsible (e.g., Buechner & Tavani, 2011 ), some philosophers hold a positive stance toward trusting AI, offering minimal notions of moral agency and accountability, respectively (Floridi & Sanders, 2004 ). Meanwhile, other philosophers are critical of the call for trustworthy AI (Moor, 2006 ; Bryson, 2018 ; Ryan, 2020 ; Deroy, 2023 ), arguing this would result in normalizing anthropomorphism, a category mistake with disastrous consequences in the age of misinformation (O’Connor & Weatherall, 2019 ), wherein AI becomes deployed as a tool for societal, economic, and political manipulation.
For our criticism, we are solely concerned with autonomous systems, whose purpose is to facilitate human decision-making by taking autonomous action that assists the human in reaching her goal, which, in virtue of its automation, places the human (and potentially other people) in a position of vulnerability, wherein concerns of trustworthiness and reliability become central to her use of the application. To that end, we argue that automated systems cannot be morally trustworthy. As it regards our proposal that AI does not need to be morally trustworthy because it can be designed to be reliable, we are exclusively concerned with machine learning applications that have been trained on a dataset about which there is a ground truth which is instrumental in establishing the reliability of the application.
Though reliability has been previously proposed as the correct target for ethical AI (Ryan, 2020 ), this paper aims to complement this research by conducting a normative analysis of reliability (Section 2 ), defending the claim that morally trustworthy AI is unnecessary (Section 3 ), and ultimately offering a framework for thinking about how reliability can mitigate vulnerability for humans in hybrid decision-making scenarios (Section 4 ). We argue that reliability is constituted by the consistency of competence, which justifies claims about the reliability of agents, biological or artificial, while demonstrating that consistent competence demands a capacity for procedural metacognition. We conclude with a summary and avenues for future research ( Conclusion ).
2 Reliability, Trustworthiness, and Vulnerability
In the technology ethics literature, there is no consensus around what reliability means. In developing his reliabilitst approach to epistemology, Goldman ( 1976 ) articulates reliability as a specific kind of tendency: “a cognitive mechanism or process is reliable if it not only produces true beliefs in actual situations, but would produce true beliefs […] in relevant counterfactual situations” (p. 771). Though Goldman’s framing of reliability is epistemological, it can be modified to provide a condition for a reliable agent or entity : an agent/entity is reliable at accomplishing a task if she/it tends not only to be successful in the completion of the task in actual situations, but would also be successful in relevant counterfactual situations. This notion provides a definition of reliability that articulates its normative conditions as it entails correctness conditions: certain conditions must hold for an agent to be reliable, while a judgment about the reliability of an agent is only justified if such conditions hold.
This normative analysis of reliability discloses that reliability is a matter of consistent competence, competence in the past, into the future, and competence in relevant counterfactual situations. For example, Kawakami et al.’s ( 2022 ) qualitative study on the perceptions and experiences of users of AI-based decision support tools (for more on such tools, see Section 3 below) discusses a case wherein users perceive the tool as unreliable due to how its screening score appeared to change at random when the algorithm was run successively without any obvious change to its inputs. This is a clear case of reliability being undermined by inconsistency.
Notice that the fulfillment of these normative conditions establishes a prima facie reason for trusting an agent as an agent . So, before the relationship between reliability and trustworthiness can be articulated in detail, it will help to clarify what it means to say that AI can be an agent. Here, conditions on the part of agency are minimal, such that entities need only be capable of resolving “many-many problems” that arise when acting (Wu, 2013 ). Agents are faced with having many inputs to act on and many ways to act on those inputs. Agency means having the capacity to resolve such problems by monitoring inputs and outputs and exercising control over which go with which. A typical action on the part of the AS/AI is making a recommendation or a prediction, which the human agent considers in her decision-making (see Section 3 ).
An agent’s reliability thus serves as a pro tanto reason for trusting her, since knowing her to be reliable will justify, as far as it goes, making oneself vulnerable to her (for a discussion of the importance of vulnerability in trust-based relationships, see Baier, 1986 ). For example, if I know my cousin to be a reliable person, then I am justified in trusting her to perform some action, such as picking me up from the airport, unless, that is, I have a specific reason not to trust her. While reliability has some justificatory force in manners of trust, it is by no means sufficient, a point demonstrated by the trust equation: trustworthiness is reliability plus an additional X- factor. Even though I might know my cousin to be reliable, if I also have a reason to appraise her as immoral, her reliability can no longer serve as justification for the decision to be vulnerable to her. In fact, her reliability (as a stable character trait) might become a reason not to trust her as she might be relied upon to behave immorally.
To understand what justifies making oneself vulnerable to another agent, we need to place a richer gloss on the moral appraisal distinguishing moral trustworthiness from mere reliability. In line with much of the philosophical literature cited above, we understand that trusting entails an expectation on the part of the trustor that the trustee will do (or has done) the right thing for the right reasons relative to a context. Clearly then, an agent’s reliability (either context-specific or as a general character trait), is insufficient to warrant trust, as this would demand knowing her behavior is constrained by “the right thing” and “the right reasons”. For example, to believe that a data scientist is trustworthy is to believe that, in the context of data science, you expect she will do the right thing (e.g., ensuring the collected data is representative of the target population) for the right reasons (e.g., as this avoids bias), and to say that she is trustworthy is to say she does these things for these reasons in this context.
As it concerns AI, however, we are obviously not dealing with an agent that can do the right thing for the right reasons simply because, to the degree that AI can be said to have an intention Footnote 4 , its intention cannot be grounded in the recognition of rational constraints, an appreciation of what is right versus what is wrong. Put differently, the intentions of AI cannot be grounded in moral rationality . Thus, the contribution of the present proposal is revisioning the rationality requirement typically demanded of artificial moral agency (e.g., Johnson, 2006 ) as applying to moral trustworthiness. This means, until such time as they can be designed to exhibit moral rationality, AI will never manifest moral trustworthiness. Footnote 5 Of course, this does not mean that vulnerability to these systems somehow disappears. On the contrary, the vulnerability is often exacerbated specifically because these systems are incapable of forming intentions that conform to normative knowledge.
If AI cannot manifest trustworthiness, then we ought to pursue all appropriate means to mitigate the vulnerability incurred by human-machine interaction. This is because vulnerability to AI can never be justified since its actions only ever support a pro tanto reason to trust (namely, reliability), which is inevitably defeated by knowledge that AI’s intentions cannot be grounded in a recognition of rational constraints, so that vulnerability is a necessary consequence of hybrid decision-making situations. Below we discuss this problem in more detail (particularly, how to mitigate it by developing machine learning applications to meet the normative conditions of reliability), but for now, we need to expose why designing systems to manifest moral trustworthiness would not actually mitigate the vulnerability that arises in high-stakes decision-making environments.
3 Why Morally Trustworthy AI is Unnecessary
For the purposes of our argument for why morally trustworthy AI is unnecessary, we are exclusively concerned with machine learning applications. Footnote 6 In particular, we are focused on those applications deployed in medium to high-stakes environments, exemplified by the real-world case of decision support tools in child welfare services to assist child maltreatment screening decisions (Kawakami et al., 2022 ), specifically the Allegheny Family Screening Tool (AFST) (Vaithianathan et al., 2017 ). Such applications promise to bring more balanced results to this decision-making process by mitigating biases in human decision-making (Lebreton et al., 2019 ). But since machine learning algorithms are susceptible to their own biases, ones quite distinct from those of humans (De-Arteaga et al., 2020 ), a growing body of research has called for human-AI partnerships (Kamar, 2016 ). The core program is that humans and AI ought to build upon each other’s strengths and compensate for their weaknesses (Bansal et al., 2021 ; cf. Green & Chen, 2019 ). Such hybrid environments are the target of our analysis which exposes how moral trustworthiness is unnecessary and reliability is sufficient.
AFST is a predictive risk modeling tool that generates a screening score for incoming calls of child mistreatment allegations by integrating and analyzing hundreds of data elements. The score aims to predict the long-term likelihood that child welfare services will be involved in a particular case. Should the score meet a specific threshold, an investigation is mandatory. But in all other cases, the decision is left to the human agent, “the screener”, and the score serves as an additional piece of information to assist the decision of whether to open an investigation (Samant et al., 2021 ), thus making clear the vulnerability caused by such environments. Since an inaccurate score has the potential to affect the screener’s decision on whether to begin an investigation, the screener, the affected children, and their caretakers, are vulnerable to the AI’s contribution to the decision space. This is not to say that the worry is that the screeners over-rely on the tool. Given how screeners consider details that AFST does not have access to, such as the potential motives of callers, cultural misunderstandings, and so-called “retaliation reports”, wherein parents in custody battles repeatedly report one another during a dispute (Kawakami et al., 2022 ), studies have consistently shown that overreliance is not an issue. The point is that screeners must calibrate their reliance on the AI’s recommendations that are not always accurate (more on this calibration process below).
Thus, the people involved are in a position of severe vulnerability that can be diminished by designing AFST to be as reliable as possible in analyzing, integrating, and summarizing the relevant data elements. One might wonder, however, whether reliability is sufficient to deal with this problem of vulnerability or whether AFST ought to be designed to be trustworthy. At first glance, this certainly seems like a noteworthy goal, since trustworthiness is a categorical reason to trust, one which would justify this vulnerability. But, given what was discussed above, what exactly does it mean to say that a machine is trustworthy? Recall that the consensus in the philosophical literature is that trust entails a moral appraisal on the part of the trustor about the trustee and trustworthiness entails this appraisal being correct relative to some context. Though attempts to instill a sense of morality into AFST would likely be in vain, it might still be designed to at least simulate degrees of moral sensitivity, and doing so could be in line with developing trustworthy AI (i.e., so long as simulation is part of the agenda).
How is that possible considering the above discussion about the nature of trust? We can list at least four possibilities: an AI might be designed to simulate (a) moral agency, (b) moral responsibility, (c) moral accountability, or (d) moral rationality – either of which, it might be argued, would lend support to the proposal that the AI is morally trustworthy. Concerning (a) moral agency, if this is understood in a minimal sense, that of being the source of moral consequences (Floridi & Sanders, 2004 ), then systems like AFST are already moral agents, since they produce the screening score, which, once used, has moral consequences. Thus construed, moral agency is better thought of as a precondition on the problem of vulnerability introduced by use, rather than one of its solutions. Of course, more robust notions of moral agency exist, wherein, for instance, the agent must act on behalf of moral values (Cherkowski et al., 2015 ), but in the framework deployed here, the capacity to act on behalf of moral values is a criterion for moral responsibility.
Another avenue for making AI trustworthy would be to develop it to simulate (b) moral responsibility, which would entail, given how responsibility is distinguished from accountability, that human agents are justified in evaluating AI behavior as either morally blameworthy or morally praiseworthy (see Hieronymi, 2004 for this distinction as it applies generally). Note, however, that until AI can be designed to exhibit an awareness of right and wrong, it would be a conceptual mistake to transfer our praise or blame onto an artificial agent. The argument here is not that our practices of blame and praise are biased or flawed (see, e.g., Longin et al., 2023 and Porsdam et al., 2023 , for empirical and normative discussion of this point). The argument is that our practices demand that the goal of moral evaluation is to shape the recipient’s conscience, structure her cognitive repertoire to better align with shared values. Until AI possesses an emotional and social self, AI cannot be an appropriate target of such rich sociocultural mind-shaping practices (for research into such practices, see Zawidzki, 2013 in philosophy and Heyes et al., 2020 in cognitive science). Setting aside whether it is feasible to build an AI that can possess such a complex self, the question arises whether having such a self would indeed contribute to mitigating the problem of vulnerability that arises in high-stakes decision-making situations. This issue will be dealt with below, after we have made sense of how an emotional self is entwined with moral rationality (see Section 3.4 ).
Thirdly, we might design AI to simulate (c) moral accountability, which would entail it possess the capacity to correct its behavior to comply with moral norms. In the case of AFST, the application might be designed to update its predictions due to mistakes of a moral kind, which might be controlled by the screener. For example, one common issue that screeners see with how AFST’s produces its score is its tendency to increase the severity of the case as more allegations are made. In the event of escalating retaliation reports, this will have a pronounced negative effect on the AI’s performance. If the system were to learn not to increase severity due to retaliation calls, then, proponents of trustworthy AI would claim, the AI would be simulating a minimal form of moral accountability.
If this is all there is to moral accountability, then, like moral agency above, moral accountability is already implemented in AFST: as a statistical model, it employs representations of its predictive error and utilizes them to update its predictions. But despite being so designed, it is hard to make sense of how this autocorrection process, ubiquitous in machine-learning applications, makes AFST trustworthy in a manner distinct from how it simply makes AFST more reliable. This process only ensures AFST is a consistently competent agent (see above, Section 2 ).
Consequently, two takeaways emerge. First, designing AIs to exhibit a minimal notion of moral accountability only makes AIs more trustworthy in virtue of how it makes them more reliable. If this is correct, questions arise about why the scientific and political community ought to call AIs trustworthy if reliability is a sufficient descriptor, especially if this might bias people into believing moral appraisals of AI are justified. To prevent such epistemically dubious behavior, we wish to introduce a principle to guide communication:
The Principle of the Non-equivalence of Trustworthiness and Reliability (PNTR)
To avoid unnecessary moral entailments or implications that come with speaking about trust, one ought to speak of trustworthiness (in manners of communication) only when trustworthiness means something over and above reliability.
PNTR say that if the notions “trust”, “trustworthy”, and “trustworthiness” can be replaced without semantic loss by the notions “rely”, “reliable”, and “reliability” respectively, then one ought only to employ the latter for the purposes of scientific and policy communication. Footnote 7
Second, since notions of minimal moral accountability only make AI more trustworthy in virtue of how it makes it more reliable, it is too thin to be of any use to make sense of how AI might mitigate vulnerability in a distinctly trust-involving manner. To examine whether this would be possible, we need to turn to a more robust notion of moral accountability. By “distinctly trust-involving”, we mean to say the AI would be capable of simulating moral trustworthiness, as it is understood above as simulating (d) moral rationality (i.e., doing the right thing for the right reasons), so that talk of such forms of trustworthy AI would not fall under the jurisdiction of the above principle. The question then becomes whether designing AI to exhibit moral rationality would assist in mitigating the vulnerability that arises in high-stakes hybrid decision-making environments. But before this issue can be addressed, we must consider underlying details concerning what it would mean for an agent to possess moral rationality.
3.1 The Cognitive Conditions for Moral Rationality
Philosophers have long emphasized that capacities for rule acquisition and compliance, even rules that ought to be interpreted as moral, are insufficient for moral rationality as this requires a sensitivity to moral norms , which are irreducible to rules (for a review, see Haugeland ( 1990 ); and for the relationship between accountability, normativity, and rationality see Strawson, 1962 ). By drawing clear lines between mere rule-following and the sophisticated norm-following behavior, Gibbard ( 1990 ) develops a naturalistic theory on the origin of normativity, proposing two distinct cognitive systems that enable (i.e., are sufficient for) normative behavior to manifest: (i) an emotional system and (ii) a conceptual system.
To illustrate what distinguishes the two systems, Gibbard introduces the elaborate ritual that ensues whenever two dogs meet on neutral ground. Their interaction follows regular patterns that Gibbard believes exhibits a form of rationale that emerged through the pressure of natural selection for coordinating behavior among conspecifics: “…in this special sense, the beasts ‘follow [norms]’ for social interaction. They have not, of course, decided to conduct themselves by these [norms]…” (p. 69). For (ii) the conceptual system, which underpins the capacity to decide to conduct oneself according to a norm, requires a capacity to represent a norm as such, which, according to Gibbard, depends upon language and propositional thought, which produces mental representations as representations (i.e., representations whose content is determined by correctness conditions, e.g., Peacocke, 1992 ). Essentially, conceptual capacities, according to Gibbard, have the effect of enriching an agent’s decision-making repertoire, allowing for motivation based on norms as such .
While conceptual capacities distinguish the normative behavior of humans from the normative behavior of non-human animals, it is (i) the emotional system that distinguishes the behavior of non-human and human animals from that of non-normative, mere rule-following entities. Gibbard describes the emotional system as enabling the agent to “be in the grip of norms”, a process of internalization, constituted as much by a specific behavioral profile as it is by a species of emotional receptivity: “a norm prescribes a pattern of behavior, and to internalize a norm is to have a motivational tendency of a particular kind to act on that pattern” (p. 70). For Gibbard, tendencies are evolutionary adaptations for coordination, while emotion (or affect, more generally) explains the motivation to perform the coordinated act: “When a person’s emotions tend to follow a pattern in this way, we can say that the person internalizes the norm that prescribes the pattern” (p. 71).
Consequently, Gibbard draws two sharp lines, one between normative and non-normative entities and another between mere normative entities and human agents. To be a normative entity, one must be capable of internalizing a rule, thereby transforming the rule into a norm, which requires possessing either a motivational tendency to enact specific behavioral patterns that evolved for social coordination or the conceptual capacity to represent norms as such. In other words, normative agency is about having the right kind of intentional state driving the rule-following behavior, namely either (i) an affective state of attunement to the sociocultural environment or (ii) a belief state constituted by the correctness conditions describing the normative behavior. Thus, Gibbard’s theory of normativity is particularly useful for present purposes because it helps articulate boundaries around moral rationality. To be capable of exhibiting moral rationality, one must be sensitive to moral norms, a receptivity which is irreducible to a capacity for following rules: for rules to qualify as norms, they must be either felt or understood .
3.2 AI Is Not Morally Rational
Applying Gibbard’s naturalistic theory on the origin of normativity, we can safely say human behavior is guided by norms in a manner distinct from how AI might be said to be guided by rules. Our responsiveness to norms arises from conceptual capacities that enable the representation of norms as such, sometimes referred to as capacities for “representing as” (Dretske, 1988 ; Fodor, 2008 ). It is widely believed that these capacities hold a special status as exclusively human, making possible a form of metacognition known as “metarepresentation” (Proust, 2013 ), that is, the capacity to represent representations as representations, as mental entities with correctness conditions that can misrepresent, and thus represent beliefs as beliefs, which could be false; perceptions as perceptions, which could be inaccurate or illusory; and norms as norms, which could be inapt (Evans, 1982 ; Peacocke, 1983 ; McDowell, 1994 ). Importantly, the capacity to metarepresent is much more sophisticated than the capacity to explicitly represent (for representing rules explicitly in machines, see Sharkey, 2020 ), since metarepresentation is often argued to enable reflective self-knowledge (see Heyes et al., 2020 for an account of this). To be clear, the day may come when AI acquires the capacity to reflect and acquire self-knowledge, but the AI of today is a far cry from such sophistication, and so describing AI as capable of accepting norms, and thereby exhibiting moral rationality in this sense, is clearly a conceptual mistake.
Analogous to the issue above of claiming AI has metarepresentational capacities, the claim that the AI of today can undergo emotion, such that it would be warranted to say it is motivated to comply with moral norms (thus exhibiting morally rational in this sense) would be a conceptual error. Despite the progress that affective psychology has made in recent years, for example, with the explanatory power of the neo-Jamesian interoception-based theory of emotion (Tsakiris & De Preester, 2018 ), an exhaustive account of the antecedents of emotion remains to be discovered. That said, a relevant consensus surrounds the multiple components view of emotion, wherein emotion is composed of various essential parts, one of which being the physiological component (Scarantino & de Sousa, 2018 ). Thus, we can say (without invoking feelings) that emotion demands an internal milieu, whose regulation is essential for the agent’s survival, requiring a delicate balancing of metabolic variables – or, simply put, molecular turnover – something which AI does not do. Footnote 8
As a result, we have convincing reasons to consider thinking of AIs as accountable in a trust-involving sense as a conceptual mistake. Though AI can be designed to monitor and adjust predictive weights to comply with moral rules, rule compliance is not norm compliance. Put simply: a fundamental difference exists between Jupiter obeying the laws of physics as a consequence of natural law and the young child choosing not to steal for the reason that it is morally wrong, the former of which is closer to artificial systems updating their models as a consequence of operational rules . Of course, in between these extremes is the norm-guided behavior of animals that enact behavioral tendencies in virtue of being motivated by emotional sensitivity to regulatory patterns, something which, of course, AIs cannot do.
3.3 Even Morally Rational Artificial Agents Do Not Help
The specific issue that our discussion aims to shed light on is how it is deeply unclear whether designing AI to be morally rational, such that they possess either (i) the emotional system or (ii) the conceptual system and so would be deserving of the appellation “trustworthy AI”, would mitigate vulnerability arising in high-stakes hybrid decision-making environments. Below we begin with (ii) and then move on to (i), arguing that neither system implemented in AI will mitigate the relevant vulnerability.
To begin exposing this issue, we first need to make sense of what this vulnerability entails. The relevant vulnerability arises because of the need, on the part of the human agent, to calibrate her reliance on the AI’s performance (see Lee and See ( 2004 ), who refer to this as “trust calibration”). Put simply: optimal reliance calibration means the human relies on the AI when its advice is correct (or more correct if the outcome is not categorical) and deviates from the AI’s advice whenever incorrect (or more incorrect). Were this optimum realized, the relevant vulnerability would disappear. Obviously then, solutions to this problem of vulnerability ought to aim at facilitating optimal calibration of reliance, such that the screener can more easily distinguish correct from incorrect cases.
Starting with (ii) the conceptual control system and sticking with AFST, we might ask whether the AI’s capacity to represent norms as such would facilitate the screener in determining whether the AI’s score is an accurate representation of the actual likelihood that intervention is needed. Though it is difficult to imagine what such a system would look like, we might provide a simple answer by pivoting to how humans are able to represent norms as such, and so, for the sake of the argument, we might envision simply replacing AFST with a human. However, replacing the AI with a full-blooded moral agent, even a perfect one, will not necessarily facilitate the screener’s decision about whether the score should be relied upon or deviated from.
This is because, of course, having a capacity to regulate your behavior around moral norms as norms does not assist another agent in determining whether you are providing accurate recommendations. It does not even entail an increase in reliability, since morality-based regulatory capacities do not offer any advice on how to analyze and interpret the factors that determine whether children are at risk of abuse, and so this capacity does not facilitate the screener’s calibration. Rather, what is needed for this task is detailed knowledge about the relevant causal antecedents predictive of child maltreatment and neglect. Indeed, we can envision an amoral agent that is proficient at determining the relevant causal factors and assigning appropriate weights based on statistical regularities, and hence why AI is usefully deployed in these environments.
Because the AI would be capable of representing norms as such, we can envision AFST as providing information about the moral norm that it adhered to in producing the screening score (e.g., “Good parents ought not to have criminal records”). While this might be helpful in determining whether the score should be relied upon, and so might assist in mitigating the vulnerability for all stakeholders, the AI can be designed to yield this information without thereby having the capacity to base its decision on moral norms as such. That is, the AI might be able to offer this “reason” without a capacity for metarepresentation. It could simply be designed to highlight the information relevant for its determination or, somewhat more sophisticated, be designed to generate a written summary of the relevant factors influencing its decision, a summary that might be fine-tuned to appear like a reason (or might be fine-tuned to be a reason to the right kind of human agent).
Moving on to (ii) the emotional system, which enables agents to be motivated by norms, we might likewise ask whether designing an AI to be morally rational in this manner would help alleviate the problem of vulnerability in high-stakes hybrid decision-making environments. As above, the question is whether this would lead to optimal calibration, that is, facilitate the screener’s ability to discriminate accurate from inaccurate screening scores. But, once again, it is unclear how equipping AI, such as AFST, with emotional sensitivity will facilitate determining whether the screening score should be relied upon.
For example, if AFST were designed to report the emotion that motivated its screening score, the screener would be tasked with speculating about the explanation behind the emotion. If the screener knew it was fear, say, rather than sadness, that guided AFST to score a particular case as 15 out of 20, it would be hard to make sense of how exactly this is useful other than as a prompt to initiate some detective work about why fear was influential. Rather, the AI might be designed to provide an explanation, rather than an emotional abstraction, which, as above, the AI could be designed to do, without undergoing or even reporting an emotion.
3.4 Where Does This Leave Us? The Significance of Trustworthiness and Why AI Still Does Not Need To Be Morally Trustworthy
Before discussing conceptual details around the distinct societal role of trustworthiness compared to reliability, let us illustrate this key notion with a quotidian example. Imagine asking your partner to give you a haircut even though they are not professionally trained and have never cut hair before. Obviously, it makes little sense to say they are reliable at giving haircuts, since they have never attempted to cut hair. Now, let us say your partner is morally trustworthy. What does this mean? It means, though they are not reliable at cutting hair, they are nonetheless reliable at conforming their behavior to certain moral norms, either by way of explicitly representing those norms via conceptual capacities or by way of being motivated to adhere to those norms via emotional capacities (and usually by way of both). Footnote 9 For instance, they will cut your hair carefully for the reason that they respect how you would feel if they acted carelessly. In these cases, we can talk about trustworthiness that is irreducible to reliability, since it makes little sense to say the trustee is reliable at performing the task, but it does make sense to say that the trustee’s character – her trustworthiness – justifies the trustor’s decision to have her perform the task. Footnote 10 Put differently, trustworthiness is society’s solution to the problem of how to bridge the gap in task-specific reliability, and it tends to work because moral goodness occasionally functions as a proxy for goodness in specific tasks.
Hence, the distinct societal role of trust is most obvious in situations characterized by an epistemic asymmetry that obtains in virtue of task novelty . In the above example, there is a crucial difference between what you ought to know (i.e., whether your partner is reliable at giving good haircuts) and what you actually know (i.e., they, a good person, have never cut her before), and this difference ought to serve as a counterweight when deciding whether to let her perform the action. By deciding to trust, one can attempt to compensate for this counterweight by appealing to the agent’s moral trustworthiness in lieu of her task-relevant reliability. Continuing with the above example, you know your partner is a good person and so will do their best, and this, you believe, will be sufficient for them to produce an acceptable outcome. Intuitively, such a compensation strategy will reap societal benefits should it ultimately turn out to be justified (and which it will do, so long as, by and large, people are good people). Notice, however, that such cases of trust are not relevant for the machine learning applications in focus here, since they are always trained on a dataset about which there is a ground truth instrumental in establishing reliability.
One of the chief aims of the present discussion is to explicate how this epistemic asymmetry does not need to arise with certain machine learning applications, since these can be designed to be highly reliable at accomplishing tasks (and ought to be so designed). Thus, so long as certain features are in place (see below), there will be no gap between what you know (the AI is reliable at its tasks) and what you ought to know when interacting with the AI in these specific tasks. Also, it is our aim to make clear how the vulnerability associated with its deployment would not be alleviated if the AI were designed to be morally trustworthy. This is because the relevant vulnerability arises not from epistemic asymmetry, but from the possibility that the AI’s prediction is wrong about the data it has been trained on, that is, from the possibility that the AI is unreliable. Footnote 11
To sum up, our discussion unpacks what it means to say we should develop morally trustworthy AI. It means developing morally rational AI endowed with a responsiveness to moral norms. But as this discussion makes clear, such machines would not help mitigate the vulnerability that deploying such technology creates. To the extent that it does, it means developing the AI to offer various kinds of explanations for its decisions, capacities which do not entail moral rationality. Thus, it is wrongheaded to call for morally trustworthy AI. In the penultimate section below, we discuss how meeting the normative conditions of reliability can be done by developing quasi-metacognitive machines and how this strategy can be coupled with another one about designing them to be quasi-partners that offer explanations. These two features will mitigate vulnerability incurred by deploying them in medium to high-stakes hybrid decision-making environments.
4 Quasi-Metacognitive Machines and Alleviating the Problem of Vulnerability
Crucial for present purposes, calling for reliable AI, reliability understood as consistent competence, avoids the category mistake that skeptics of trustworthy AI are concerned about, since competence is completely orthogonal to morality (e.g., it is possible to be a perfectly competent liar). Thus, reliability, independent of trust, possesses unique normative conditions, conceptualized above as features of agents (see Section 2 ). Equipped with these notions of reliability and minimal agency, we can now ask about the capacities that AI needs to be consistently competent.
First, AI needs a capacity to process its decision as input; otherwise, it will have no way of monitoring its decision to ensure it exhibits consistency. Second, it needs to develop an internal model of its decisions, so that it can control its performance. Third, it requires a comparative function that contrasts performance to its internal model to determine whether decisions remain consistent. Fourth, this comparator function needs the capacity to produce an evaluation of performance that serves as the means by which the AI controls its behavior. Fifth, it needs a capacity to process this evaluation as input, so that the information carried by the evaluation can be used to update internal models and thereby control future performance. Finally, its decisions need to be a product of this complex process of monitoring, evaluating, and revising of internal models; otherwise, it will be unable to ensure competence in the event of error and in relevant counterfactual situations.
Interestingly, the architecture described above is analogous to, what has been called, “procedural metacognition”, in which feedback signals inform the system implicitly about its own performance, signals which are used to update internal models and regulate the system’s behavior (Proust, 2013 ). Procedural metacognition (also known as “system 1 metacognition”, “evaluative metacognition”, and “implicit metacognition”) can be defined as, “the ability to evaluate the quality of one’s own informational states, and the efficiency of one’s own learning attempts, in order to regulate subsequent cognitive activities and behavior” (Goupil & Proust, 2023 ). Capacities for monitoring and evaluating one’s own performance is a form of metacognitive sensitivity, and capacities for regulating behavior according to the evaluations of self-monitoring mechanisms is a form of metacognitive calibration and control. As such, one solution to the alignment problem is not developing machines to simulate moral rationality, but to simulate metacognition, and thus the goal of developing reliable AI is about developing quasi-metacognitive machines. Footnote 12
As it turns out, a simulation of metacognitive architecture comes at little cost in many AI applications. In machine learning, what is known as the “F1-score” (also known as “model confidence”) is a metric used to evaluate the performance of a classification model. It is the harmonic mean of precision and recall into a single score ranging from 0 to 1, which makes it useful for comparing the model’s competence. Precision is the proportion of true positive results out of all predicted positive results (i.e., TP/(TP + FP)), while recall is the proportion of true positive results out of all actual positive results (i.e., TP/(TP + FN)). A higher F1-score indicates better performance, and it is routinely regarded as a measure of the confidence from the system in the generated classification or prediction. Moreover, several machine learning algorithms, such as logistic regression (which AFST employs) and Naïve Bayes, provide probability estimations for each class as part of their output. There are also Accuracy and Area Under the Curve scores. Thus, what ought to be called, “reliability scores” (rather than the anthropomorphic “confidence scores”) inform about the reliability of the underlying process that determine the classification score.
In the context of experimental psychology, metacognitive competence refers to an individual’s ability to accurately assess and evaluate their own cognitive processes, such as their memory, perception, or problem-solving abilities (Fleming & Lau, 2014 ). This is measured by contrasting metacognitive sensitivity to metacognitive bias. Metacognitive sensitivity refers to the ability to accurately detect and discriminate between one’s own correct and incorrect judgments or performance, while metacognitive bias, on the other hand, refers to systematic deviations or distortions in metacognitive judgments, representing the tendency to make consistent errors in self-assessment, independent of one’s actual performance or knowledge. While products of a fundamentally different mechanism, reliability scores can be the machine analogue to metacognitive sensitivity, with specific scores being analogues to calibrated confidence.
In other words, it would be advantageous for AFST to offer two scores. In addition to the already-present screening score about the likelihood that intervention is needed, there would also be a second score about the AI’s reliability regarding the process that formed the screening score. At first glance, the reliability score might sound redundant, but comparing these two statements makes it clear that this is not the case: “I am 80% confident this case scores 18 out 20” versus “I am 20% confident this case scores 18 out of 20”. Clearly, reliability scores will provide the screener with another useful piece of information to consider when deciding whether to rely on or deviate from the AI’s recommendation.
This proposal is supported by recent empirical results. For example, Zhang et al. ( 2020 ) found that providing reliability scores not only led to the collective benefit of higher accuracy in discrimination and classification tasks, but it also facilitated human decision-makers in calibrating their reliance on the AI (i.e., mitigating the problem of vulnerability). This result is also supported by Rechkemmer and Yin ( 2022 ) that showed that providing reliability scores positively influences whether people believe an AI’s predictions, so long as these scores are well-calibrated with performance (i.e., so long as the model exhibits consistent competence). Though the process by which reliability scores are calculated is vastly different from how confidence signals of procedural metacognition are produced, there are important functional similarities in terms of both. Both inform about the degree to which performance is sensitive to the relevant constraints, and both can be used to determine whether strategies are effective or whether ought to change.
As introduced above, many machine learning applications are already quasi-metacognitive in the manner described here, and so the demand of developing reliable AI is already met in many cases. What is left to understand is how to turn reliable AIs into reliable quasi-partners (only “quasi” because genuine partnership arguably requires joint responsibility; see Schmidt and Loidolt ( 2023 ) for a relevant taxonomy of partnerships), so that we might make sense of how it can be deployed to mitigate the problem of vulnerability for all relevant stakeholders. As a baseline enabling condition for partnering with AI (however simulated), it helps to recognize that AI advice is often constitutive of the final decision, such that the final decision ought to be regarded as a species of collective decision and the AI regarded as a contributor to this decision.
One plausible answer to the question of what enables a reliable agent to become a reliable partner is that the agent communicates her confidence to the other agents involved in the collective decision-making process. Not only is there a wealth of evidence that demonstrates that confidence communication incurs collective and collaborative benefits in collective decision-making (Bahrami et al., 2012 ), but there are conceptual reasons for linking the communication of confidence with the enabling conditions of a reliable partner. Recall from above that reliability is chiefly about consistent competence; indeed, having access to an agent’s confidence clearly facilitates relying on them, since when confidence is well-calibrated, it serves as an approximation of the agent’s competence. In other words, if I am confident that I can perform some action, then my confidence approximates the likelihood that I will be successful, and the inverse goes for when I am not confident.
As it regards providing explanations, our discussion above demonstrates that, rather than conceptual or emotional capacities (i.e., what it would really take to make AI morally trustworthy), it is the capacity to provide explanations that makes a meaningful contribution to mitigating the problem of vulnerability. In combination with the capacity to report reliability scores (i.e., the machine analogue to confidence), the AI should also be designed to provide explanations for the reliability scores. In the case of AFST, an explanation for a reliability score of 90 (out of 100) for a classification score of 18 (out of 20) might be, for instance, a parent’s recent substance abuse charges. This would enable the AI to be a more reliable quasi-partner in the collective decision of whether to open an investigation. This is because, as our discussion has shown, confidence, when well-calibrated, is an indication of competence, and reliability, what we ought to be aiming toward, should be following competence.
Furthermore, explanations might be provided in counterfactual terms to facilitate the role of the AI as a quasi-partner. As the above normative analysis shows, reliability is also about competence in relevant counterfactual situations, and a responsiveness, when scoring, to counterfactual possibilities demonstrates this competence. For example, AFST might be designed to report that were the recent substance abuse charges absent in the case file, the reliability score would drop to 20% for a classification score of 18. Crucially, access to explanations of a counterfactual stripe is high on screeners’ wishlist of AFST improvements (Kawakami et a., 2022), and counterfactual explanations have been shown to increase understanding of the reliability scores (Le et al., 2022 ). Moreover, the proposal is highly feasible since various machine learning methods exist for providing counterfactual descriptions (Erasmus et al., 2021 ).
5 Conclusion and Future Directions
The prevailing notion of developing morally trustworthy AI is fundamentally wrongheaded. What trustworthy AI ultimately amounts to is morally rational AI, which, feasibility aside, is unnecessary for promoting optimal calibration of the human decision-maker on AI assistance. Instead, reliability, exclusive of trust, ought to be the appropriate goal of ethical AI, as it entails the normative constraints that, when met, are effective in mitigating the vulnerability created by having AI make meaningful contributions in high-stakes decision-making environments. These normative demands can be met by developing AI to exhibit quasi-metacognitive competence as quasi-partners, which is largely already in place in machine learning systems. Crucially, the AI ought to be designed to report reliability scores to the human decision-makers due to how such scores enable the human to calibrate her reliance on the AI’s advice. Moreover, these scores could be more effective if accompanied by reasons and counterfactual explanations. By highlighting the importance of such explanations in promoting optimal calibration, without any need for moral trust, we have pointed the way to developing more ethical applications by way of developing quasi-metacognitive machines.
Data Availability
Not Applicable.
Though questions arise about how to delineate the terms “artificial intelligence”, “machine learning algorithms”, and “automated systems”, they are treated as subsets of each other (AS - AI - ML). Until Section 3 , we use the term “AI” to refer to any sufficiently sophisticated automated system that makes the human agent operating it vulnerable, so that questions of trustworthiness and reliability become a central concern to her use of the application.
It might be useful here to introduce common definitions for these crucial terms morally “agential”, “accountable” and “responsible” (Floridi & Sanders, 2004 ). Moral agency is about being the source of moral action. Moral accountability is described by the capacity to change behavior to correct for moral wrongdoings. Moral responsibility, on the other hand, is about being the subject of moral evaluation and exhibiting the right intentional (mental) state. Finally, moral rationality is about being constrained by moral reasons (see Section 2 for more).
As it concerns the issue of whether the distinction between trustworthy and reliable is a mere linguistic one, two forms of vulnerability remain nonetheless crucial for the practical philosophy of cooperation: one related to task-specific competence and another related to ethical norms (see Section 2 ). To argue for general applicability of this distinction, one might demonstrate (for example) that these are manifestations of the Kantian means/ends distinction, such that trustworthiness describes what agents ought to possess in the as ends in themselves mode, with reliability describing the as means mode (which, for humans at least, should be read as “never merely, but sometimes primarily” as means mode). Such an argument, if sound, would ground this distinction in fundamental principles of moral philosophy.
In the classical theory of action (Davidson, 1963 ), intentions are beliefs plus pro-attitudes. In the minimal account of agency articulated above, AS/AI can be said to have intentions if they derive solutions to many-many problems (minimal beliefs) and have programming for solving such problems or similar problems (minimal pro-attitudes).
It is important to note the possibility of restricting trust solely to epistemic matters, wherein an argument could be made it is potentially justified to trust AS/AI solely as an epistemic source or epistemic tool (see Alvarado, 2023 ). But here we are interested in trust in general, which, as argued above, is an implicitly moral notion. Henceforth, trust is always meant as moral trust.
We are no longer concerned with all AS, since non-machine-learning AS (lacking, e.g., processes for updating internal models and minimizing error), cannot meet the normative requirements for reliability established in Section 2 . Henceforth, by “AI” we mean autonomous machine learning systems for decision support. As our argument concerns AS in general, it follows from our view that we should design any AS that raises similar vulnerability issues to realize quasi-metacognition and to communicate its reliability to the relevant human agents because this would be sufficient to mitigate the vulnerability incurred by its deployment (see Section 4 ).
Thus, we respond to the “so-what objection” (Mainz, 2023 ) by rejecting the claim that it is trivial to point out that AI cannot be trustworthy. Simply put, what we say matters : calling AI trustworthy, when it is not, propagates misinformation and engenders miscommunication that could result in mistrust in science (National Academies of Sciences, 2017 ).
Notice that an appeal to metabolic processes is not necessarily opposed to a functionalist account of emotion. A functionalist could accommodate this demand by appealing to functions, such that properties of the environment serve as input, while properties of the boundaries that constitute the agent serve as output. One would then need to provide a convincing case for how these processes are constitutive of the AI’s basic interaction with the environment. Of course, it is unclear whether this would be sufficient for claiming the agent’s emotions are constituted by feelings, as many theorists would demand, but, at the very least, it could theoretically implement physiological functions.
An astute observer will detect two kinds of reliability in play: one that fails to apply to the task at hand (here, cutting hair) and another which does apply to the agent’s consistency in conforming to moral norms. Recall that trustworthiness is reliability plus an additional X- factor, so every trustworthy person is also reliable in the sense that she has reliability as an enduring character trait. However, this does not mean, of course, that every trustworthy person is reliable at all tasks.
For readers familiar with Mayer et al.’s ( 1995 ) influential model of trust based on three dimensions (ability, integrity, and benevolence), this example is about the agent scoring near zero in ability but high in integrity and benevolence.
Considering the above interpretation of the significance of trustworthiness, one could interject that designing AI to be morally trustworthy would mean that we would be justified in deploying it in novel situations, which, in this context, would mean deploying it without the required training. To our knowledge, this would violate ethical codes as well as be simply a bad idea from an engineering standpoint, and so the point is somewhat moot, since its practical significance is unclear.
Why quasi? Bayne et al. ( 2019 ), a review article in Current Biology entitled “What is Cognition?”, features answers to the titular question from 11 experts, ranging from philosophers of mind to cognitive neuroscientists and professors of artificial intelligence. The majority position is characterized by adaptive information processing, involving high degrees of flexibility, that enables stimulus-independence and causal reasoning. While the information processing of the AI systems for decision support admits of high degrees of flexibility, it is unclear how its processing is stimulus independent and produces genuine causal reasoning. Having said that, we deploy the term “ quasi -metacognitive” to refer only to current AS/AI systems, which lacks stimulus independence and genuine causal reasoning. We do not wish to draw an a priori line on whether some future AI might manifest genuine cognition or even metacognition.
Alvarado, R. (2023). What kind of trust does AI deserve, if any? AI and Ethics , 3 (4), 1169–1183. https://doi.org/10.1007/s43681-022-00224-x .
Article Google Scholar
Bahrami, B., Olsen, K., Bang, D., Roepstorff, A., Rees, G., & Frith, C. (2012). Together, slowly but surely: The role of social interaction and feedback on the build-up of benefit in collective decision-making. Journal of Experimental Psychology: Human Perception and Performance , 38 (1), 3–8. https://doi.org/10.1037/a0025708 .
Baier, A. (1986). Trust and Antitrust. Ethics , 96 (2), 231–260. https://doi.org/10.1086/292745 .
Bansal, G., Nushi, B., Kamar, E., Horvitz, E., & Weld, D. S. (2021). Is the most accurate AI the best teammate? Optimizing AI for Teamwork. Proceedings of the AAAI Conference on Artificial Intelligence , 35 (13), 11405–11414. https://doi.org/10.1609/aaai.v35i13.17359 .
Bayne, T., Brainard, D., Byrne, R. W., Chittka, L., Clayton, N., Heyes, C., Mather, J., Ölveczky, B., Shadlen, M., Suddendorf, T., & Webb, B. (2019). What is cognition? Current Biology , 29 (13), R608–r615. https://doi.org/10.1016/j.cub.2019.05.044 .
Bryson, J. (2018). AI & global governance: no one should trust AI. United Nations Centre for Policy Research . Retrieved April, 27, 2023: https://cpr.unu.edu/publications/articles/ai-global-governance-no-one-should-trust-ai.html#:~:text=We%20should%20focus%20on%20AI,of%20our%20institutions%20and%20ourselves .
Buechner, J., & Tavani, H. T. (2011). Trust and multi-agent systems: Applying the diffuse, default model of trust to experiments involving artificial agents. Ethics and Information Technology , 13 (1), 39–51. https://doi.org/10.1007/s10676-010-9249-z .
Cherkowski, S., Walker, K. D., & Kutsyuruba, B. (2015). Principals’ Moral Agency and ethical Decision-Making: Toward a transformational Ethics. International Journal of Education Policy and Leadership , 10 (5), n5. https://eric.ed.gov/?id=EJ1138586 .
Christian, B. (2020). The Alignment Problem: Machine learning and human values . WW Norton & Company.
Coeckelbergh, M. (2012). Can we trust robots? Ethics and Information Technology , 14 (1), 53–60. https://doi.org/10.1007/s10676-011-9279-1 .
Davidson, D. (1963). Actions, reasons and causes. Journal of Philosophy , 60 , 685–670.
De-Arteaga, M., Fogliato, R., & Chouldechova, A. (2020). A case for humans-in-the-loop: Decisions in the presence of erroneous algorithmic scores. Proceedings of the 2020 CHI Conference on Human Factors in Computing Systems . https://doi.org/10.1145/3313831.3376638
Deroy, O. (2023). The Ethics of Terminology: Can we use human terms to describe AI? Topoi , 42 (3), 881–889. https://doi.org/10.1007/s11245-023-09934-1 .
Deutsch, M. (1977). The resolution of conflict: Constructive and destructive processes . Yale University Press. https://doi.org/10.12987/9780300159356 .
Dretske, F. (1988). Explaining Behavior . MIT Press.
Erasmus, A., Brunet, T. D. P., & Fisher, E. (2021). What is Interpretability? Philosophy & Technology , 34 (4), 833–862. https://doi.org/10.1007/s13347-020-00435-2 .
European Commission (2019). Communication from the Commission to the European Parliament, the Council and the European Economic and Social Committee and the Committee of the Regions , Building trust in human-centric artificial intelligence. COM(2019) 168 final (8 April 2019).
Evans, G. (1982). The Varieties of Reference. Oxford: Oxford University Press.
Fleming, S. M., & Lau, H. C. (2014). How to measure metacognition. Frontiers in Human Neuroscience . https://doi.org/10.3389/fnhum.2014.00443 . 8.
Floridi, L., & Sanders, J. W. (2004). On the morality of Artificial agents. Minds and Machines , 14 (3), 349–379. https://doi.org/10.1023/B:MIND.0000035461.63578.9d .
Fodor, J. (2008). LOT2: The language of thought revisited . Oxford University Press.
Gibbard, A. (1990). Wise choices, apt feelings: A theory of normative Judgment . Harvard University Press.
Glikson, E., & Woolley, A. W. (2020). Human Trust in Artificial Intelligence: Review of empirical research. Academy of Management Annals , 14 (2), 627–660. https://doi.org/10.5465/annals.2018.0057 .
Goldman, A. I. (1976). Discrimination and perceptual knowledge. The Journal of Philosophy , 73 (20), 771–791. https://doi.org/10.2307/2025679 .
Goupil, L., & Proust, J. (2023). Curiosity as a metacognitive feeling. Cognition , 231 , 105325. https://doi.org/10.1016/j.cognition.2022.105325 .
Green, B., & Chen, Y. (2019). The principles and limits of Algorithm-in-the-Loop decision making. Proceedings of ACM Human Computer Interactions , 3 (CSCW), Article50. https://doi.org/10.1145/3359152 .
Hardin, R. (2002). Trust and Trustworthiness . Russell Sage Foundation.
Haugeland, J. (1990). The Intentionality All-Stars. Philosophical Perspectives , 4 , 383–427. https://doi.org/10.2307/2214199 .
Heyes, C., Bang, D., Shea, N., Frith, C. D., & Fleming, S. M. (2020). Knowing ourselves together: The cultural origins of metacognition. Trends in Cognitive Sciences , 24 , 349–362. https://doi.org/10.1016/j.tics.2020.02.007 .
Hieronymi, P. (2004). The Force and Fairness of blame. Philosophical Perspectives , 18 (1), 115–148. https://doi.org/10.1111/j.1520-8583.2004.00023.x .
Johnson, D. (2006). Computer systems: Moral entities but not moral agents. Ethics of Information Technology , 8 , 195–204. https://doi.org/10.1007/s10676-006-9111-5 .
Kamar, E. (2016). Directions in Hybrid Intelligence: Complementing AI Systems with Human Intelligence. Proceedings of the 25th International Joint Conference on Artificial Intelligence , New York, 9–15 July 2016, 4070–4073.
Kawakami, A., Sivaraman, V., Cheng, H. F., Stapleton, L., Cheng, Y., Qing, D., Perer, A., Wu, Z. S., Zhu, H., & Holstein, K. (2022). Improving Human-AI Partnerships in Child Welfare: Understanding Worker Practices, Challenges, and Desires for Algorithmic Decision Support. Proceedings of the 2022 CHI Conference on Human Factors in Computing Systems , New Orleans, LA, USA. https://doi.org/10.1145/3491102.3517439 .
Lahno, B. (2001). On the emotional character of Trust. Ethical Theory and Moral Practice , 4 (2), 171–189. https://doi.org/10.1023/A:1011425102875 .
Le, T., Miller, T., Singh, R., & Sonenberg, L. (2022). Improving model understanding and trust with counterfactual explanations of Model confidence. arXiv Preprint arXiv :220602790.
Lebreton, M., Bacily, K., Palminteri, S., & Engelmann, J. B. (2019). Contextual influence on confidence judgments in human reinforcement learning. PLOS Computational Biology , 15 (4), e1006973. https://doi.org/10.1371/journal.pcbi.1006973 .
Lee, J. D., & See, K. A. (2004). Trust in automation: Designing for Appropriate Reliance. Human Factors , 46 (1), 50–80. https://doi.org/10.1518/hfes.46.1.50_30392 .
Longin, L., Bahrami, B., & Deroy, O. (2023). Intelligence brings responsibility - even smart AI-assistants are held responsible. iScience , 107494. https://doi.org/10.1016/j.isci.2023.107494 .
Luhmann, N. (1979). Trust and Power . Wiley.
Mainz, J. T. (2023). Medical AI: Is trust really the issue? Journal of Medical Ethics . https://doi.org/10.1136/jme-2023-109414 .
Mayer, R. C., Davis, J. H., & Schoorman, F. D. (1995). An Integrative Model of Organizational Trust. The Academy of Management Review , 20 (3), 709–734. https://doi.org/10.2307/258792 .
McDowell, J. (1994). Mind and world . Harvard University Press.
Moor, J. H. (2006). The Nature, Importance, and Difficulty of Machine Ethics. IEEE Intelligent Systems , 21 (4), 18–21. https://doi.org/10.1109/MIS.2006.80 .
National Academies of Sciences. (2017). Communicating Science effectively: A Research Agenda. National Academies Press . https://doi.org/10.17226/23674 .
O’Connor, C., & Weatherall, J. O. (2019). The misinformation age: How false beliefs spread . Yale University Press.
Peacocke, C. (1983). Sense and Content: Experience, Thought and Their Relations. Oxford: Oxford University Press.
Peacocke, C. (1992). A study of concepts . The MIT.
Porsdam Mann, S., Earp, B. D., Nyholm, S., Danaher, J., Møller, N., Bowman-Smart, H., Hatherley, J., Koplin, J., Plozza, M., Rodger, D., Treit, P. V., Renard, G., McMillan, J., & Savulescu, J. (2023). Generative AI entails a credit–blame asymmetry. Nature Machine Intelligence , 5 (5), 472–475. https://doi.org/10.1038/s42256-023-00653-1 .
Proust, J. (2013). The philosophy of Metacognition: Mental Agency and Self-Awareness . Oxford University Press.
Rechkemmer, A., & Yin, M. (2022). When Confidence Meets Accuracy: Exploring the Effects of Multiple Performance Indicators on Trust in Machine Learning Models. Proceedings of the 2022 CHI Conference on Human Factors in Computing Systems , New Orleans, LA, USA. https://doi.org/10.1145/3491102.3501967 .
Ryan, M. (2020). In AI we trust: Ethics, Artificial Intelligence, and reliability. Science and Engineering Ethics , 26 (5), 2749–2767. https://doi.org/10.1007/s11948-020-00228-y .
Samant, A., Horowitz, A., Xu, K., & Beiers, S. (2021). Family surveillance by algorithm. American Civil Liberties Union . https://www.aclu.org/fact-sheet/family-surveillance-algorithm
Scarantino, A. (2018). & de Sousa. R. Emotion. The Stanford Encyclopedia of Philosophy (Summer 2021 Edition) . Edward N. Zalta (Ed.). https://plato.stanford.edu/archives/sum2021/entries/emotion .
Schmidt, P., & Loidolt, S. (2023). Interacting with machines: Can an Artificially Intelligent Agent be a Partner? Philosophy & Technology , 36 (3), 55. https://doi.org/10.1007/s13347-023-00656-1 .
Sharkey, A. (2020). Can we Program or Train Robots to be good? Ethics and Information Technology , 22 (4), 283–295. https://doi.org/10.1007/s10676-017-9425-5 .
Sheridan, T. B., & Hennessy, R. T. (1984). Research and modeling of supervisory control behavior. Report of a workshop . National Research Council Washington DC Committee on Human Factors.
Simon, J. (Ed.). (2020). The Routledge Handbook of Trust and Philosophy (1st ed.). Routledge. https://doi.org/10.4324/9781315542294 .
Strawson, P. (1962). Freedom and Resentment. Proceedings of the British Academy , 48 , 187–211.
Google Scholar
Tsakiris, M., & De Preester, H. (Eds.). (2018). The interoceptive mind: From homeostasis to awareness . Oxford University Press.
Vaithianathan, R., Putnam-Hornstein, E., Jiang, N., Nand, P., & Maloney, T. (2017). Developing predictive models to support child maltreatment hotline screening decisions: Allegheny County methodology and implementation . Center for Social data Analytics.
Wu, W. (2013). Mental Action and the threat of Automaticity. In A. Clark, J. Kiverstein, & T. Vierkant (Eds.), Decomposing the Will (pp. 244–261). Oxford University Press.
Zawidzki, T. W. (2013). Mindshaping: A new framework for understanding human social cognition . MIT Press.
Zhang, Y., Liao, Q. V., & Bellamy, R. K. E. (2020). Effect of confidence and explanation on accuracy and trust calibration in AI-assisted decision making. Proceedings of the 2020 Conference on Fairness, Accountability, and Transparency , Barcelona, Spain. https://doi.org/10.1145/3351095.3372852 .
Download references
Acknowledgements
We would like to thank the anonymous reviewer for their helpful and valuable feedback that greatly improved the quality of this manuscript.
This work was supported by Bayerisches Forschungsinstitut für Digitale Transformation (bidt) Co-Learn Award Number KON-21-0000048 and HORIZON EUROPE European Innovation Council (EIC) EMERGE Grant agreement ID: 101070918.
Open Access funding enabled and organized by Projekt DEAL.
Author information
Authors and affiliations.
Faculty of Philosophy, Philosophy of Science and Religious Studies, Ludwig Maximilian University, Munich, Germany
John Dorsch & Ophelia Deroy
You can also search for this author in PubMed Google Scholar
Contributions
The first draft and peer review revisions of the manuscript were written by John Dorsch, and Ophelia Deroy commented on drafts of the manuscript. All authors read and approved the final manuscript.
Corresponding author
Correspondence to John Dorsch .
Ethics declarations
Ethics approval and consent to participate, consent for publication.
We hereby give our consent for the submitted manuscript to be published in the journal Philosophy & Technology and bestow onto the publisher all rights that come with this.
Competing Interests
The authors declare that they have no competing interests.
Additional information
Publisher’s note.
Springer Nature remains neutral with regard to jurisdictional claims in published maps and institutional affiliations.
Rights and permissions
Open Access This article is licensed under a Creative Commons Attribution 4.0 International License, which permits use, sharing, adaptation, distribution and reproduction in any medium or format, as long as you give appropriate credit to the original author(s) and the source, provide a link to the Creative Commons licence, and indicate if changes were made. The images or other third party material in this article are included in the article’s Creative Commons licence, unless indicated otherwise in a credit line to the material. If material is not included in the article’s Creative Commons licence and your intended use is not permitted by statutory regulation or exceeds the permitted use, you will need to obtain permission directly from the copyright holder. To view a copy of this licence, visit http://creativecommons.org/licenses/by/4.0/ .
Reprints and permissions
About this article
Dorsch, J., Deroy, O. “Quasi-Metacognitive Machines: Why We Don’t Need Morally Trustworthy AI and Communicating Reliability is Enough”. Philos. Technol. 37 , 62 (2024). https://doi.org/10.1007/s13347-024-00752-w
Download citation
Received : 09 January 2024
Accepted : 25 April 2024
Published : 03 May 2024
DOI : https://doi.org/10.1007/s13347-024-00752-w
Share this article
Anyone you share the following link with will be able to read this content:
Sorry, a shareable link is not currently available for this article.
Provided by the Springer Nature SharedIt content-sharing initiative
- Ethics of AI
- Trustworthy AI
- Trustworthiness
- Reliability
- Science communication
- Metacognition
- Find a journal
- Publish with us
- Track your research
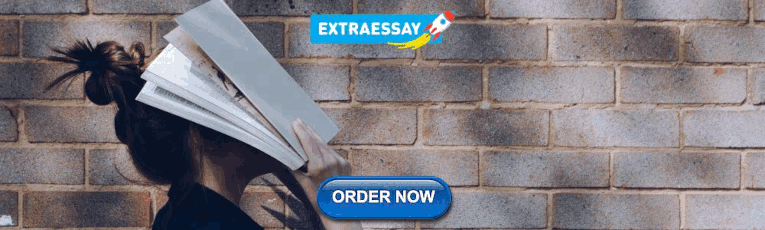
IMAGES
VIDEO
COMMENTS
Metacognition is defined as "thinking about thinking" or the ability to monitor and control one's cognitive processes 1 and plays an important role in learning and education 2,3,4.For ...
Metacognition is the practice of being aware of one's own thinking. Some scholars refer to it as "thinking about thinking.". Fogarty and Pete give a great everyday example of metacognition ...
1. Introduction. Metacognition is a multi-faceted phenomenon that involves both the awareness and regulation of one's cognitions (Flavell 1979).Past research has shown that metacognitive regulation, or the skills learners use to manage their cognitions, is positively related to effective problem-solving (Berardi-Coletta et al. 1995), transfer (Lin and Lehman 1999), and self-regulated ...
To solve problems, there is a need not only for carrying out the stages of problem-solving but also for following, regulating, and controlling these stages consciously. Therefore, metacognition is an essential element of the problem-solving process (Schoenfeld, 2005). Although various studies have revealed the positive relationship
develop their metacognitive processes. Fogarty (1994) suggests that metacognition is a process that spans three distinct phases, and that, to be successful thinkers, students must do the follow-ing: 1. Develop a . plan. before approaching a learning task, such as reading for comprehension or solv-ing a math problem. 2. Monitor
Even when there is evidence of the relation between metacognitive processes and critical thinking, there are still few initiatives which seek to clarify which process determines which other one, or whether there is interdependence between both. ... " Metacognitive aspects of problem solving," in The Nature of Intelligence. ed. Resnik L. B ...
Metacognition and Problem Solving. Metacognition, or one's awareness and control of their own thinking for the purpose of learning (Cross and Paris, 1988), is linked to improved problem-solving performance and academic achievement.In one meta-analysis of studies that spanned developmental stages from elementary school to adulthood, metacognition predicted academic performance when ...
Metacognition is the ability of learners to monitor and control their cognitive process (Young & Fry, 2008) for improving academic achievements (Tanner, 2012; van der Stel & Veenman, 2014), fostering reading and comprehension skills (Reeve & Brown, 1984), advancing critical thinking skills (Ku & Ho, 2010) and enhancing problem solving and ...
There are various cognitive theories of creativity. Some involve basic process, such as memory and information processing, and some focus on higher-order thought, including problem solving, metaphor, and divergent thinking. Metacognitive processes are frequently involved in cognitive theories of creativity.
Fogarty (1994) suggests that Metacognition is a process that spans three distinct phases, and that, to be successful thinkers, students must do the following: Develop a plan before approaching a learning task, such as reading for comprehension or solving a math problem. Monitor their understanding; use "fix-up" strategies when meaning ...
Metacognition is an awareness of one's thought processes and an understanding of the patterns behind them. The term comes from the root word meta, meaning "beyond", or "on top of". Metacognition can take many forms, such as reflecting on one's ways of thinking and knowing when and how to use particular strategies for problem-solving. There are generally two components of metacognition: (1 ...
Strategies for teaching metacognition in classrooms. This is the third piece in a six-part blog series on teaching 21st century skills, including problem solving , metacognition, critical thinking ...
We did this in the context of a problem-solving task based on literature indicating that individuals tend to display higher levels of metacognitive and executive processes while problem-solving (Antonietti et al. 2000; Aşık and Erktin 2019). We had two overarching aims: 1) to examine early years Mc and compare results across different ...
Metacognition comprises both the ability to be aware of one's cognitive processes (metacognitive knowledge) and to regulate them (metacognitive control). ... subjects are asked to verbalise their thoughts while performing a problem-solving task 80. Each of these instruments can be used to study meta-knowledge and meta-control. For instance, ...
Metacognition in Collaborative Problem Solving. CPS is a joint activity in which multiple individuals combine their resources, skills, and efforts to transform a problem state into a desired state (Roschelle & Teasley, 1995).CPS offers a rich source to support or prompt metacognition in cognitive and social processes.
Almost everything students do in school is a problem. This chapter describes some of the metacognitive knowledge and processes that help individuals efficiently handle the givens, goals, and obstacles found in problem solving. There are five sections. The first three focus on the metacognitive knowledge and processes related to the three parts of a problem. In particular, the first section ...
Metacognitive skills are the soft skills you use to monitor and control your learning and problem-solving processes, or your thinking about thinking. This self-understanding is known as metacognition theory, a term that the American developmental psychologist John H. Flavell coined in the 1970s. It might sound abstract, but these skills are ...
Metacognition, Problem, and Problem-Solving . Metacognition, problem, and problem-solving processes are of great importance and are the basic skills that should be acquired in mathematics education (Artzt and Armor-Thomas, 1992; Lucangeli et al., 2019; Jacobse and Harskamp, 2012; Posamentier and Krulik, 2008; Smith and Mancy, 2018).
Mathematical problem solving is a process involving metacognitive (e.g., judging progress), cognitive (e.g., working memory), and affective (e.g., math anxiety) factors. Recent research encourages researchers who study math cognition to consider the role that the interaction between metacognition and math anxiety plays in mathematical problem solving. Problem solvers can make many ...
reasoning and problem-solving (Ader, 2019). Metacognitive skills play a critical role in monitoring and. regulating cognitive processes (Chan & Mansoor, 2007) and help stude nts to understand when ...
The strategies suggested by Montague (1992) for problem solving according to metacognitive process consist of seven stages: 1) study the problem to understand it, 2) paraphrase the problem (in your own words), 3) visualize the problem, 4) hypothesize (a program to solve the problem), 5) estimate (predict the problem), 6) compute (computational ...
In this study, the first three of the six metacognitive processes are identified and clustered as. the problem solving task-related metacognition. On the other hand, the next three are grouped ...
Metacognition, the ability to understand and regulate one's cognitive processes, is a fundamental aspect of human learning, decision making and problem solving. Traditionally viewed as a conscious process, metacognition involves activities such as planning, monitoring, and evaluating one's performance during cognitive tasks.
Insights into the process of mathematical problem posing is a central concern in mathematics education research. However, little is known about regulative or metacognitive behaviors that are essential to understanding this process. In this study, we investigate metacognitive behavior in problem posing. We aim at (1) identifying problem-posing-specific metacognitive behaviors and (2) applying ...
A systematic problem-solving process may be used for any child suspected of being an eligible individual, and nothing in this chapter nor in Part B of the Act shall be construed to limit the applicability of a systematic problem-solving process to children suspected of having a certain type of disability. Iowa Admin. Code r. 281-41.313
In the context of experimental psychology, metacognitive competence refers to an individual's ability to accurately assess and evaluate their own cognitive processes, such as their memory, perception, or problem-solving abilities (Fleming & Lau, 2014). This is measured by contrasting metacognitive sensitivity to metacognitive bias.
A systematic problem-solving process may be used for any child suspected of being an eligible individual, and nothing in this chapter nor in Part B of the Act is to be construed to limit the applicability of a systematic problem-solving process to children suspected of having a certain type of disability. Iowa Admin. Code rr. 281-41.313