Home Blog Design Understanding Data Presentations (Guide + Examples)
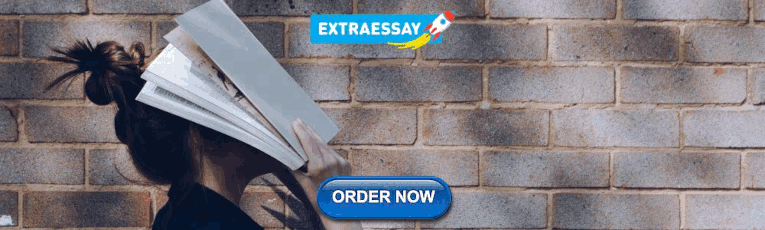
Understanding Data Presentations (Guide + Examples)
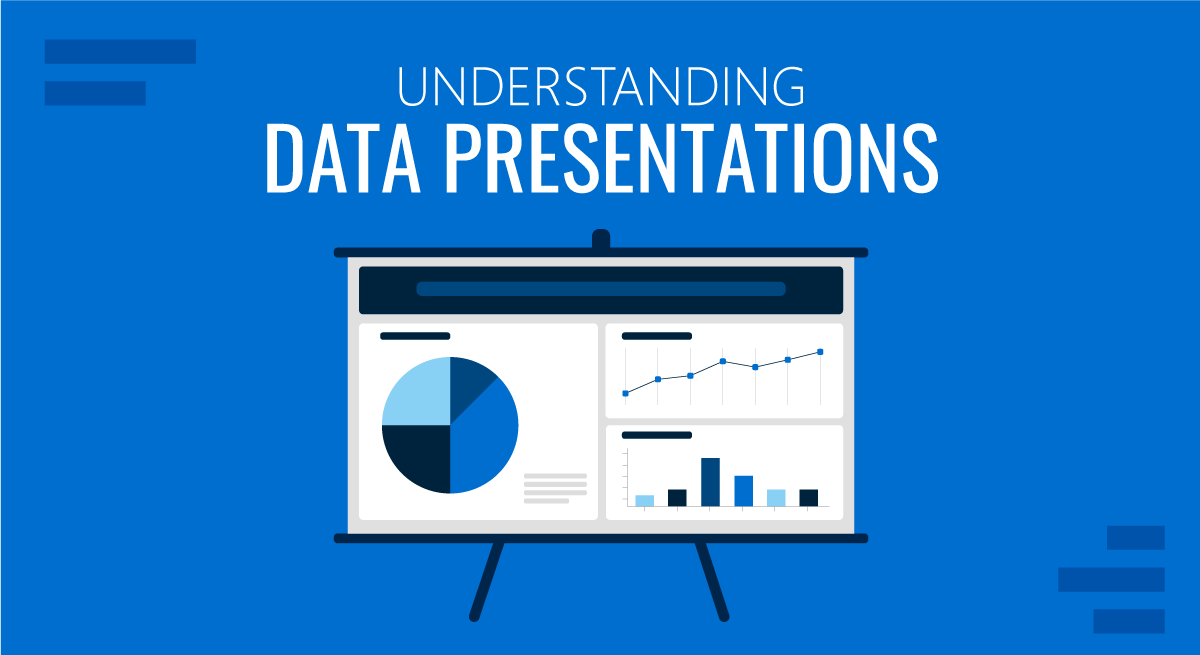
In this age of overwhelming information, the skill to effectively convey data has become extremely valuable. Initiating a discussion on data presentation types involves thoughtful consideration of the nature of your data and the message you aim to convey. Different types of visualizations serve distinct purposes. Whether you’re dealing with how to develop a report or simply trying to communicate complex information, how you present data influences how well your audience understands and engages with it. This extensive guide leads you through the different ways of data presentation.
Table of Contents
What is a Data Presentation?
What should a data presentation include, line graphs, treemap chart, scatter plot, how to choose a data presentation type, recommended data presentation templates, common mistakes done in data presentation.
A data presentation is a slide deck that aims to disclose quantitative information to an audience through the use of visual formats and narrative techniques derived from data analysis, making complex data understandable and actionable. This process requires a series of tools, such as charts, graphs, tables, infographics, dashboards, and so on, supported by concise textual explanations to improve understanding and boost retention rate.
Data presentations require us to cull data in a format that allows the presenter to highlight trends, patterns, and insights so that the audience can act upon the shared information. In a few words, the goal of data presentations is to enable viewers to grasp complicated concepts or trends quickly, facilitating informed decision-making or deeper analysis.
Data presentations go beyond the mere usage of graphical elements. Seasoned presenters encompass visuals with the art of data storytelling , so the speech skillfully connects the points through a narrative that resonates with the audience. Depending on the purpose – inspire, persuade, inform, support decision-making processes, etc. – is the data presentation format that is better suited to help us in this journey.
To nail your upcoming data presentation, ensure to count with the following elements:
- Clear Objectives: Understand the intent of your presentation before selecting the graphical layout and metaphors to make content easier to grasp.
- Engaging introduction: Use a powerful hook from the get-go. For instance, you can ask a big question or present a problem that your data will answer. Take a look at our guide on how to start a presentation for tips & insights.
- Structured Narrative: Your data presentation must tell a coherent story. This means a beginning where you present the context, a middle section in which you present the data, and an ending that uses a call-to-action. Check our guide on presentation structure for further information.
- Visual Elements: These are the charts, graphs, and other elements of visual communication we ought to use to present data. This article will cover one by one the different types of data representation methods we can use, and provide further guidance on choosing between them.
- Insights and Analysis: This is not just showcasing a graph and letting people get an idea about it. A proper data presentation includes the interpretation of that data, the reason why it’s included, and why it matters to your research.
- Conclusion & CTA: Ending your presentation with a call to action is necessary. Whether you intend to wow your audience into acquiring your services, inspire them to change the world, or whatever the purpose of your presentation, there must be a stage in which you convey all that you shared and show the path to staying in touch. Plan ahead whether you want to use a thank-you slide, a video presentation, or which method is apt and tailored to the kind of presentation you deliver.
- Q&A Session: After your speech is concluded, allocate 3-5 minutes for the audience to raise any questions about the information you disclosed. This is an extra chance to establish your authority on the topic. Check our guide on questions and answer sessions in presentations here.
Bar charts are a graphical representation of data using rectangular bars to show quantities or frequencies in an established category. They make it easy for readers to spot patterns or trends. Bar charts can be horizontal or vertical, although the vertical format is commonly known as a column chart. They display categorical, discrete, or continuous variables grouped in class intervals [1] . They include an axis and a set of labeled bars horizontally or vertically. These bars represent the frequencies of variable values or the values themselves. Numbers on the y-axis of a vertical bar chart or the x-axis of a horizontal bar chart are called the scale.
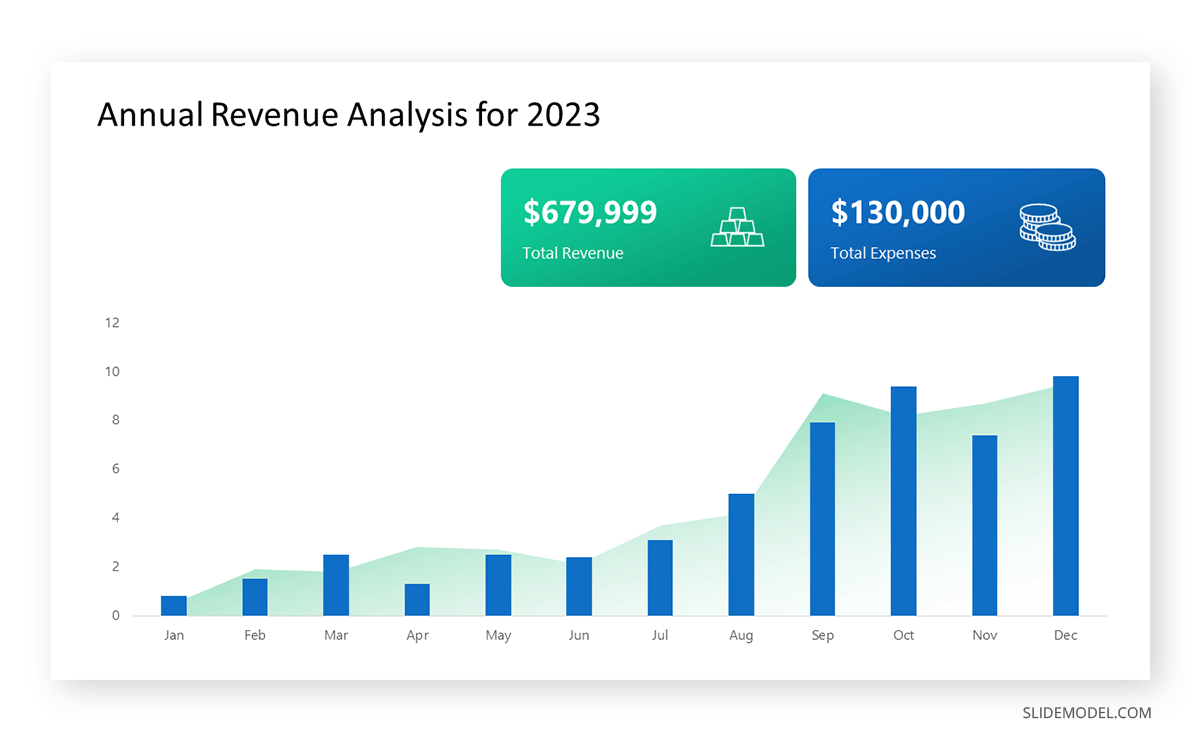
Real-Life Application of Bar Charts
Let’s say a sales manager is presenting sales to their audience. Using a bar chart, he follows these steps.
Step 1: Selecting Data
The first step is to identify the specific data you will present to your audience.
The sales manager has highlighted these products for the presentation.
- Product A: Men’s Shoes
- Product B: Women’s Apparel
- Product C: Electronics
- Product D: Home Decor
Step 2: Choosing Orientation
Opt for a vertical layout for simplicity. Vertical bar charts help compare different categories in case there are not too many categories [1] . They can also help show different trends. A vertical bar chart is used where each bar represents one of the four chosen products. After plotting the data, it is seen that the height of each bar directly represents the sales performance of the respective product.
It is visible that the tallest bar (Electronics – Product C) is showing the highest sales. However, the shorter bars (Women’s Apparel – Product B and Home Decor – Product D) need attention. It indicates areas that require further analysis or strategies for improvement.
Step 3: Colorful Insights
Different colors are used to differentiate each product. It is essential to show a color-coded chart where the audience can distinguish between products.
- Men’s Shoes (Product A): Yellow
- Women’s Apparel (Product B): Orange
- Electronics (Product C): Violet
- Home Decor (Product D): Blue
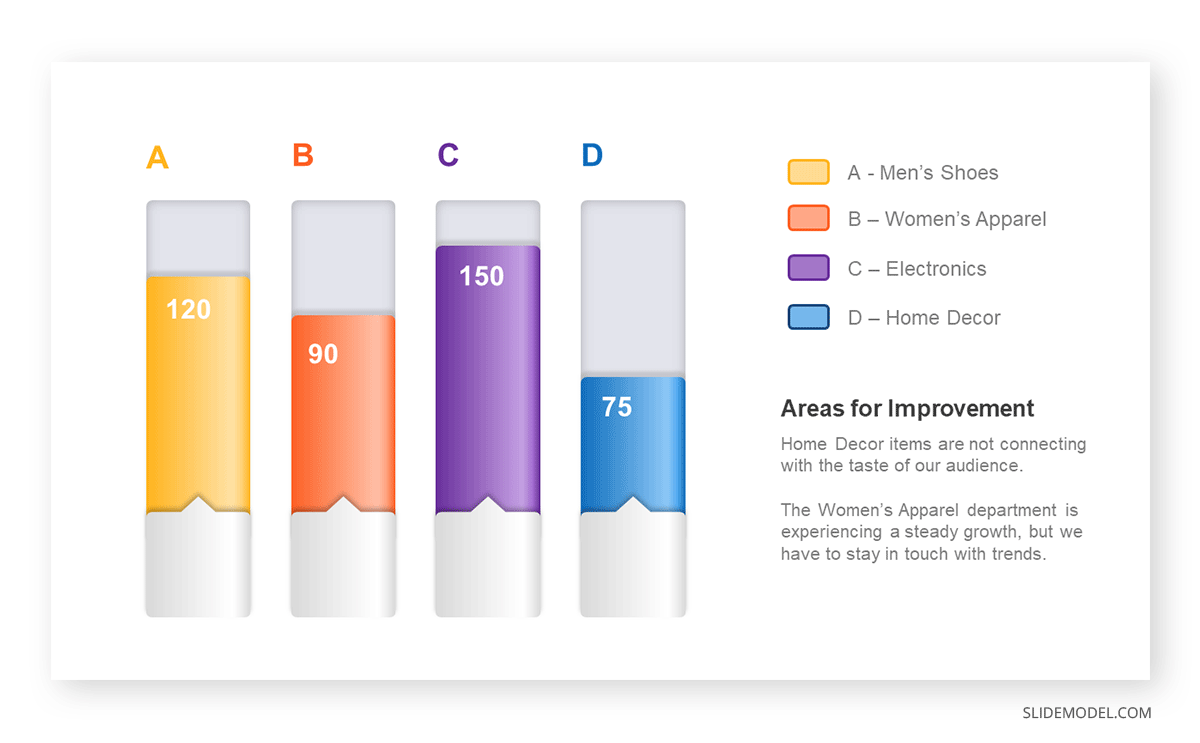
Bar charts are straightforward and easily understandable for presenting data. They are versatile when comparing products or any categorical data [2] . Bar charts adapt seamlessly to retail scenarios. Despite that, bar charts have a few shortcomings. They cannot illustrate data trends over time. Besides, overloading the chart with numerous products can lead to visual clutter, diminishing its effectiveness.
For more information, check our collection of bar chart templates for PowerPoint .
Line graphs help illustrate data trends, progressions, or fluctuations by connecting a series of data points called ‘markers’ with straight line segments. This provides a straightforward representation of how values change [5] . Their versatility makes them invaluable for scenarios requiring a visual understanding of continuous data. In addition, line graphs are also useful for comparing multiple datasets over the same timeline. Using multiple line graphs allows us to compare more than one data set. They simplify complex information so the audience can quickly grasp the ups and downs of values. From tracking stock prices to analyzing experimental results, you can use line graphs to show how data changes over a continuous timeline. They show trends with simplicity and clarity.
Real-life Application of Line Graphs
To understand line graphs thoroughly, we will use a real case. Imagine you’re a financial analyst presenting a tech company’s monthly sales for a licensed product over the past year. Investors want insights into sales behavior by month, how market trends may have influenced sales performance and reception to the new pricing strategy. To present data via a line graph, you will complete these steps.
First, you need to gather the data. In this case, your data will be the sales numbers. For example:
- January: $45,000
- February: $55,000
- March: $45,000
- April: $60,000
- May: $ 70,000
- June: $65,000
- July: $62,000
- August: $68,000
- September: $81,000
- October: $76,000
- November: $87,000
- December: $91,000
After choosing the data, the next step is to select the orientation. Like bar charts, you can use vertical or horizontal line graphs. However, we want to keep this simple, so we will keep the timeline (x-axis) horizontal while the sales numbers (y-axis) vertical.
Step 3: Connecting Trends
After adding the data to your preferred software, you will plot a line graph. In the graph, each month’s sales are represented by data points connected by a line.
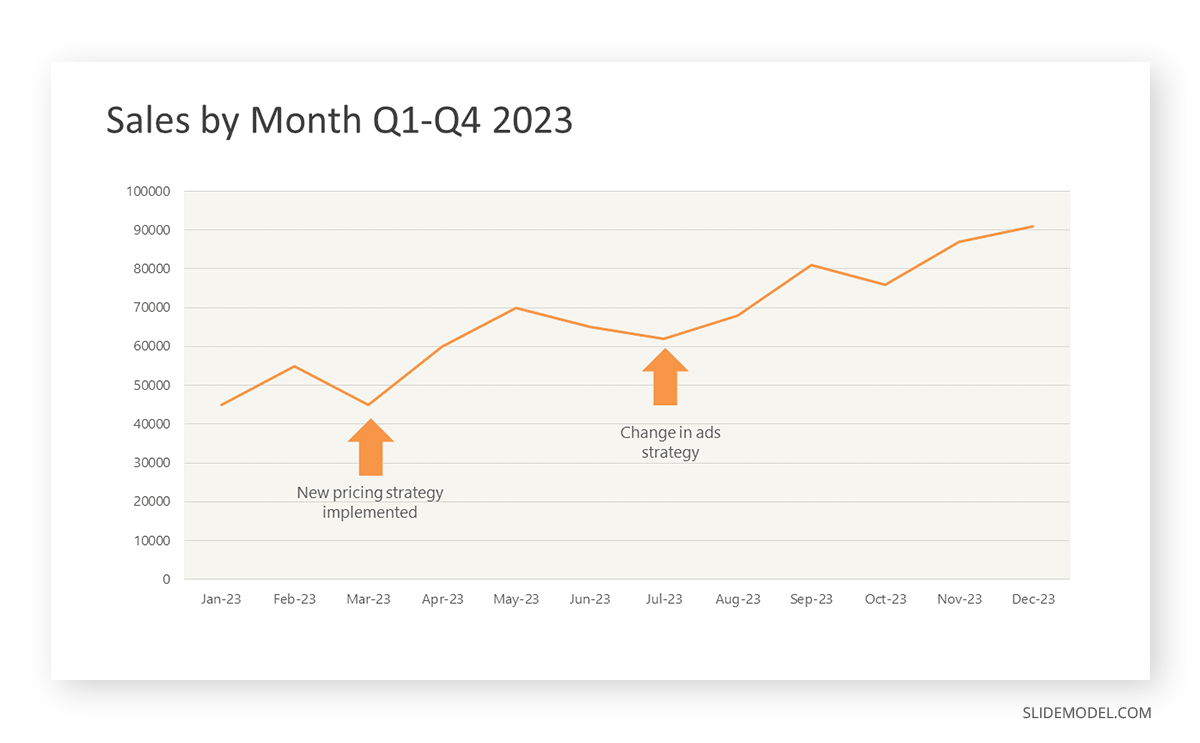
Step 4: Adding Clarity with Color
If there are multiple lines, you can also add colors to highlight each one, making it easier to follow.
Line graphs excel at visually presenting trends over time. These presentation aids identify patterns, like upward or downward trends. However, too many data points can clutter the graph, making it harder to interpret. Line graphs work best with continuous data but are not suitable for categories.
For more information, check our collection of line chart templates for PowerPoint and our article about how to make a presentation graph .
A data dashboard is a visual tool for analyzing information. Different graphs, charts, and tables are consolidated in a layout to showcase the information required to achieve one or more objectives. Dashboards help quickly see Key Performance Indicators (KPIs). You don’t make new visuals in the dashboard; instead, you use it to display visuals you’ve already made in worksheets [3] .
Keeping the number of visuals on a dashboard to three or four is recommended. Adding too many can make it hard to see the main points [4]. Dashboards can be used for business analytics to analyze sales, revenue, and marketing metrics at a time. They are also used in the manufacturing industry, as they allow users to grasp the entire production scenario at the moment while tracking the core KPIs for each line.
Real-Life Application of a Dashboard
Consider a project manager presenting a software development project’s progress to a tech company’s leadership team. He follows the following steps.
Step 1: Defining Key Metrics
To effectively communicate the project’s status, identify key metrics such as completion status, budget, and bug resolution rates. Then, choose measurable metrics aligned with project objectives.
Step 2: Choosing Visualization Widgets
After finalizing the data, presentation aids that align with each metric are selected. For this project, the project manager chooses a progress bar for the completion status and uses bar charts for budget allocation. Likewise, he implements line charts for bug resolution rates.
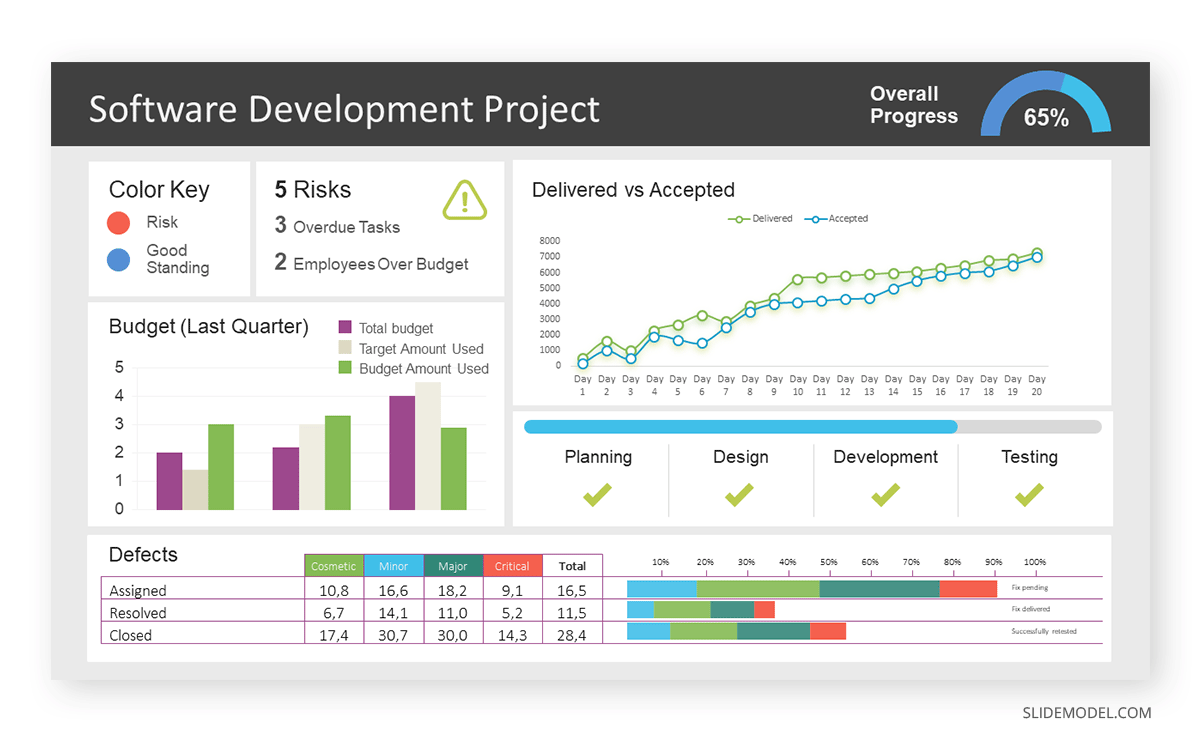
Step 3: Dashboard Layout
Key metrics are prominently placed in the dashboard for easy visibility, and the manager ensures that it appears clean and organized.
Dashboards provide a comprehensive view of key project metrics. Users can interact with data, customize views, and drill down for detailed analysis. However, creating an effective dashboard requires careful planning to avoid clutter. Besides, dashboards rely on the availability and accuracy of underlying data sources.
For more information, check our article on how to design a dashboard presentation , and discover our collection of dashboard PowerPoint templates .
Treemap charts represent hierarchical data structured in a series of nested rectangles [6] . As each branch of the ‘tree’ is given a rectangle, smaller tiles can be seen representing sub-branches, meaning elements on a lower hierarchical level than the parent rectangle. Each one of those rectangular nodes is built by representing an area proportional to the specified data dimension.
Treemaps are useful for visualizing large datasets in compact space. It is easy to identify patterns, such as which categories are dominant. Common applications of the treemap chart are seen in the IT industry, such as resource allocation, disk space management, website analytics, etc. Also, they can be used in multiple industries like healthcare data analysis, market share across different product categories, or even in finance to visualize portfolios.
Real-Life Application of a Treemap Chart
Let’s consider a financial scenario where a financial team wants to represent the budget allocation of a company. There is a hierarchy in the process, so it is helpful to use a treemap chart. In the chart, the top-level rectangle could represent the total budget, and it would be subdivided into smaller rectangles, each denoting a specific department. Further subdivisions within these smaller rectangles might represent individual projects or cost categories.
Step 1: Define Your Data Hierarchy
While presenting data on the budget allocation, start by outlining the hierarchical structure. The sequence will be like the overall budget at the top, followed by departments, projects within each department, and finally, individual cost categories for each project.
- Top-level rectangle: Total Budget
- Second-level rectangles: Departments (Engineering, Marketing, Sales)
- Third-level rectangles: Projects within each department
- Fourth-level rectangles: Cost categories for each project (Personnel, Marketing Expenses, Equipment)
Step 2: Choose a Suitable Tool
It’s time to select a data visualization tool supporting Treemaps. Popular choices include Tableau, Microsoft Power BI, PowerPoint, or even coding with libraries like D3.js. It is vital to ensure that the chosen tool provides customization options for colors, labels, and hierarchical structures.
Here, the team uses PowerPoint for this guide because of its user-friendly interface and robust Treemap capabilities.
Step 3: Make a Treemap Chart with PowerPoint
After opening the PowerPoint presentation, they chose “SmartArt” to form the chart. The SmartArt Graphic window has a “Hierarchy” category on the left. Here, you will see multiple options. You can choose any layout that resembles a Treemap. The “Table Hierarchy” or “Organization Chart” options can be adapted. The team selects the Table Hierarchy as it looks close to a Treemap.
Step 5: Input Your Data
After that, a new window will open with a basic structure. They add the data one by one by clicking on the text boxes. They start with the top-level rectangle, representing the total budget.
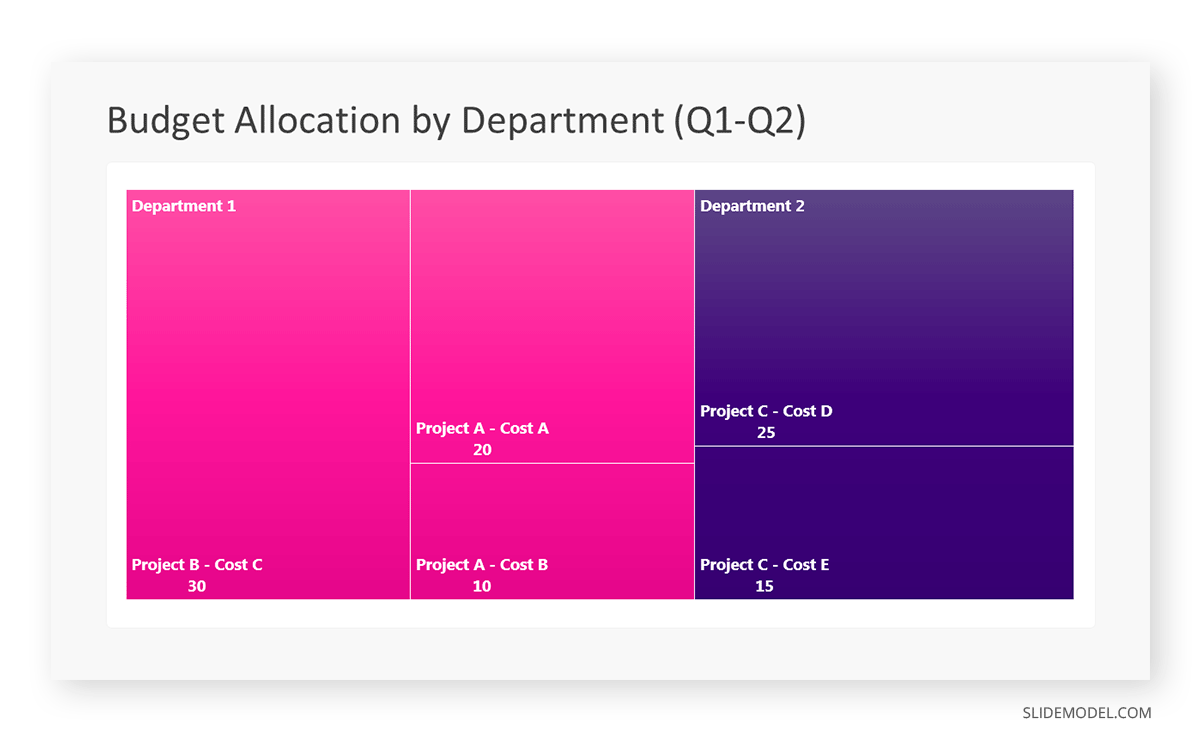
Step 6: Customize the Treemap
By clicking on each shape, they customize its color, size, and label. At the same time, they can adjust the font size, style, and color of labels by using the options in the “Format” tab in PowerPoint. Using different colors for each level enhances the visual difference.
Treemaps excel at illustrating hierarchical structures. These charts make it easy to understand relationships and dependencies. They efficiently use space, compactly displaying a large amount of data, reducing the need for excessive scrolling or navigation. Additionally, using colors enhances the understanding of data by representing different variables or categories.
In some cases, treemaps might become complex, especially with deep hierarchies. It becomes challenging for some users to interpret the chart. At the same time, displaying detailed information within each rectangle might be constrained by space. It potentially limits the amount of data that can be shown clearly. Without proper labeling and color coding, there’s a risk of misinterpretation.
A heatmap is a data visualization tool that uses color coding to represent values across a two-dimensional surface. In these, colors replace numbers to indicate the magnitude of each cell. This color-shaded matrix display is valuable for summarizing and understanding data sets with a glance [7] . The intensity of the color corresponds to the value it represents, making it easy to identify patterns, trends, and variations in the data.
As a tool, heatmaps help businesses analyze website interactions, revealing user behavior patterns and preferences to enhance overall user experience. In addition, companies use heatmaps to assess content engagement, identifying popular sections and areas of improvement for more effective communication. They excel at highlighting patterns and trends in large datasets, making it easy to identify areas of interest.
We can implement heatmaps to express multiple data types, such as numerical values, percentages, or even categorical data. Heatmaps help us easily spot areas with lots of activity, making them helpful in figuring out clusters [8] . When making these maps, it is important to pick colors carefully. The colors need to show the differences between groups or levels of something. And it is good to use colors that people with colorblindness can easily see.
Check our detailed guide on how to create a heatmap here. Also discover our collection of heatmap PowerPoint templates .
Pie charts are circular statistical graphics divided into slices to illustrate numerical proportions. Each slice represents a proportionate part of the whole, making it easy to visualize the contribution of each component to the total.
The size of the pie charts is influenced by the value of data points within each pie. The total of all data points in a pie determines its size. The pie with the highest data points appears as the largest, whereas the others are proportionally smaller. However, you can present all pies of the same size if proportional representation is not required [9] . Sometimes, pie charts are difficult to read, or additional information is required. A variation of this tool can be used instead, known as the donut chart , which has the same structure but a blank center, creating a ring shape. Presenters can add extra information, and the ring shape helps to declutter the graph.
Pie charts are used in business to show percentage distribution, compare relative sizes of categories, or present straightforward data sets where visualizing ratios is essential.
Real-Life Application of Pie Charts
Consider a scenario where you want to represent the distribution of the data. Each slice of the pie chart would represent a different category, and the size of each slice would indicate the percentage of the total portion allocated to that category.
Step 1: Define Your Data Structure
Imagine you are presenting the distribution of a project budget among different expense categories.
- Column A: Expense Categories (Personnel, Equipment, Marketing, Miscellaneous)
- Column B: Budget Amounts ($40,000, $30,000, $20,000, $10,000) Column B represents the values of your categories in Column A.
Step 2: Insert a Pie Chart
Using any of the accessible tools, you can create a pie chart. The most convenient tools for forming a pie chart in a presentation are presentation tools such as PowerPoint or Google Slides. You will notice that the pie chart assigns each expense category a percentage of the total budget by dividing it by the total budget.
For instance:
- Personnel: $40,000 / ($40,000 + $30,000 + $20,000 + $10,000) = 40%
- Equipment: $30,000 / ($40,000 + $30,000 + $20,000 + $10,000) = 30%
- Marketing: $20,000 / ($40,000 + $30,000 + $20,000 + $10,000) = 20%
- Miscellaneous: $10,000 / ($40,000 + $30,000 + $20,000 + $10,000) = 10%
You can make a chart out of this or just pull out the pie chart from the data.
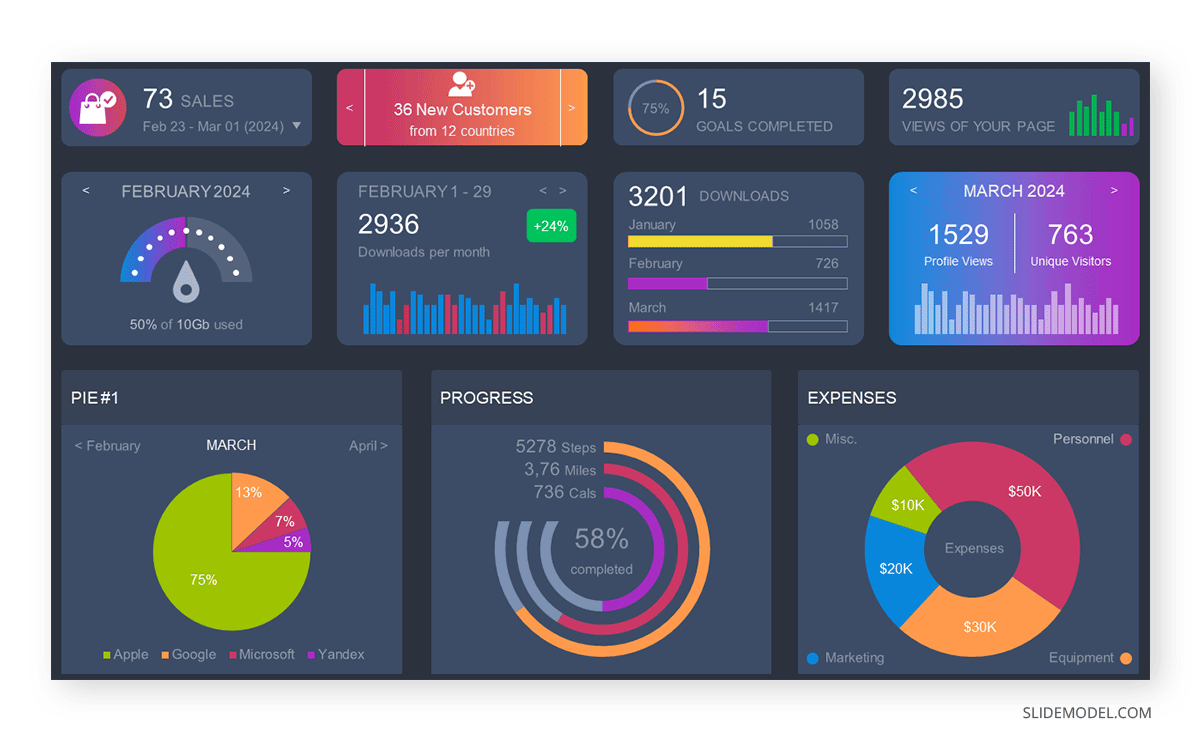
3D pie charts and 3D donut charts are quite popular among the audience. They stand out as visual elements in any presentation slide, so let’s take a look at how our pie chart example would look in 3D pie chart format.
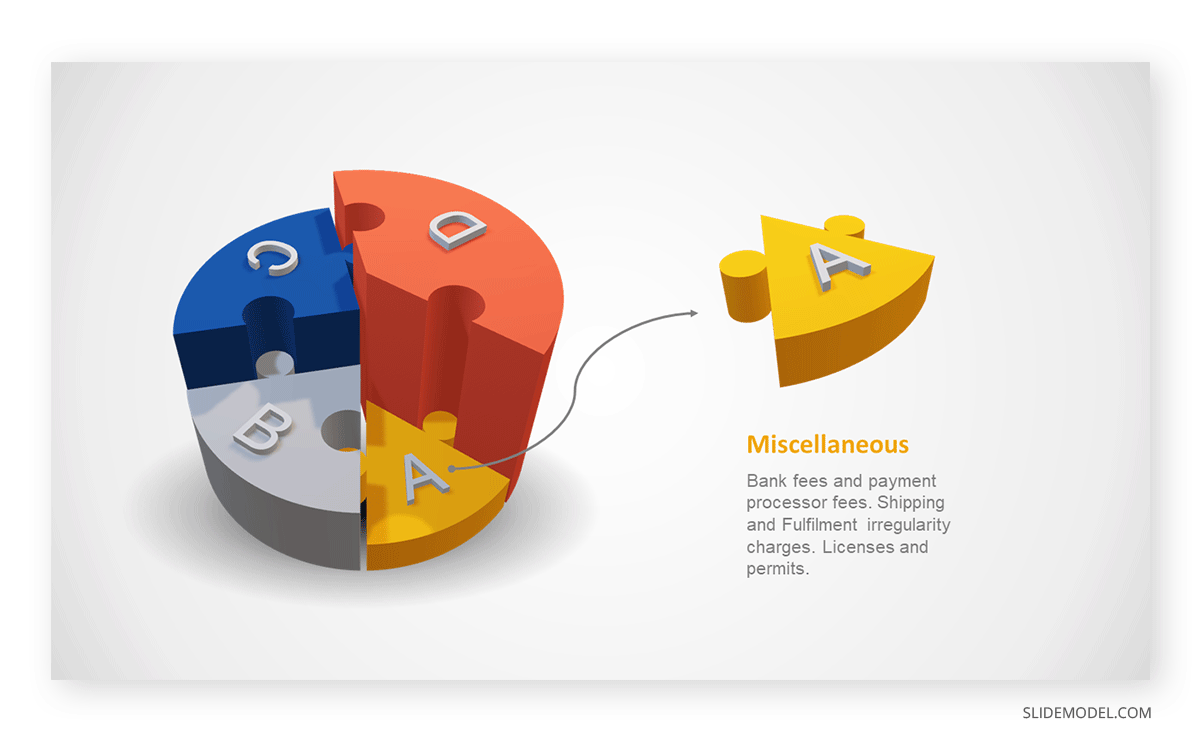
Step 03: Results Interpretation
The pie chart visually illustrates the distribution of the project budget among different expense categories. Personnel constitutes the largest portion at 40%, followed by equipment at 30%, marketing at 20%, and miscellaneous at 10%. This breakdown provides a clear overview of where the project funds are allocated, which helps in informed decision-making and resource management. It is evident that personnel are a significant investment, emphasizing their importance in the overall project budget.
Pie charts provide a straightforward way to represent proportions and percentages. They are easy to understand, even for individuals with limited data analysis experience. These charts work well for small datasets with a limited number of categories.
However, a pie chart can become cluttered and less effective in situations with many categories. Accurate interpretation may be challenging, especially when dealing with slight differences in slice sizes. In addition, these charts are static and do not effectively convey trends over time.
For more information, check our collection of pie chart templates for PowerPoint .
Histograms present the distribution of numerical variables. Unlike a bar chart that records each unique response separately, histograms organize numeric responses into bins and show the frequency of reactions within each bin [10] . The x-axis of a histogram shows the range of values for a numeric variable. At the same time, the y-axis indicates the relative frequencies (percentage of the total counts) for that range of values.
Whenever you want to understand the distribution of your data, check which values are more common, or identify outliers, histograms are your go-to. Think of them as a spotlight on the story your data is telling. A histogram can provide a quick and insightful overview if you’re curious about exam scores, sales figures, or any numerical data distribution.
Real-Life Application of a Histogram
In the histogram data analysis presentation example, imagine an instructor analyzing a class’s grades to identify the most common score range. A histogram could effectively display the distribution. It will show whether most students scored in the average range or if there are significant outliers.
Step 1: Gather Data
He begins by gathering the data. The scores of each student in class are gathered to analyze exam scores.
After arranging the scores in ascending order, bin ranges are set.
Step 2: Define Bins
Bins are like categories that group similar values. Think of them as buckets that organize your data. The presenter decides how wide each bin should be based on the range of the values. For instance, the instructor sets the bin ranges based on score intervals: 60-69, 70-79, 80-89, and 90-100.
Step 3: Count Frequency
Now, he counts how many data points fall into each bin. This step is crucial because it tells you how often specific ranges of values occur. The result is the frequency distribution, showing the occurrences of each group.
Here, the instructor counts the number of students in each category.
- 60-69: 1 student (Kate)
- 70-79: 4 students (David, Emma, Grace, Jack)
- 80-89: 7 students (Alice, Bob, Frank, Isabel, Liam, Mia, Noah)
- 90-100: 3 students (Clara, Henry, Olivia)
Step 4: Create the Histogram
It’s time to turn the data into a visual representation. Draw a bar for each bin on a graph. The width of the bar should correspond to the range of the bin, and the height should correspond to the frequency. To make your histogram understandable, label the X and Y axes.
In this case, the X-axis should represent the bins (e.g., test score ranges), and the Y-axis represents the frequency.
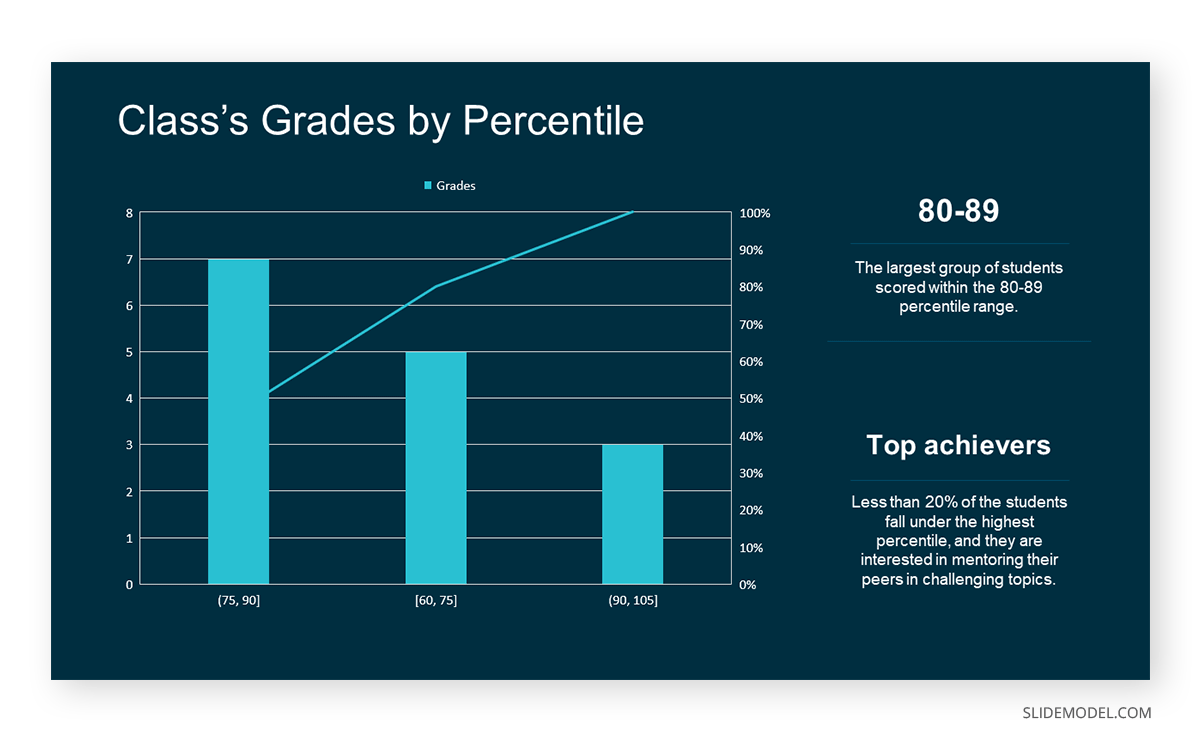
The histogram of the class grades reveals insightful patterns in the distribution. Most students, with seven students, fall within the 80-89 score range. The histogram provides a clear visualization of the class’s performance. It showcases a concentration of grades in the upper-middle range with few outliers at both ends. This analysis helps in understanding the overall academic standing of the class. It also identifies the areas for potential improvement or recognition.
Thus, histograms provide a clear visual representation of data distribution. They are easy to interpret, even for those without a statistical background. They apply to various types of data, including continuous and discrete variables. One weak point is that histograms do not capture detailed patterns in students’ data, with seven compared to other visualization methods.
A scatter plot is a graphical representation of the relationship between two variables. It consists of individual data points on a two-dimensional plane. This plane plots one variable on the x-axis and the other on the y-axis. Each point represents a unique observation. It visualizes patterns, trends, or correlations between the two variables.
Scatter plots are also effective in revealing the strength and direction of relationships. They identify outliers and assess the overall distribution of data points. The points’ dispersion and clustering reflect the relationship’s nature, whether it is positive, negative, or lacks a discernible pattern. In business, scatter plots assess relationships between variables such as marketing cost and sales revenue. They help present data correlations and decision-making.
Real-Life Application of Scatter Plot
A group of scientists is conducting a study on the relationship between daily hours of screen time and sleep quality. After reviewing the data, they managed to create this table to help them build a scatter plot graph:
In the provided example, the x-axis represents Daily Hours of Screen Time, and the y-axis represents the Sleep Quality Rating.
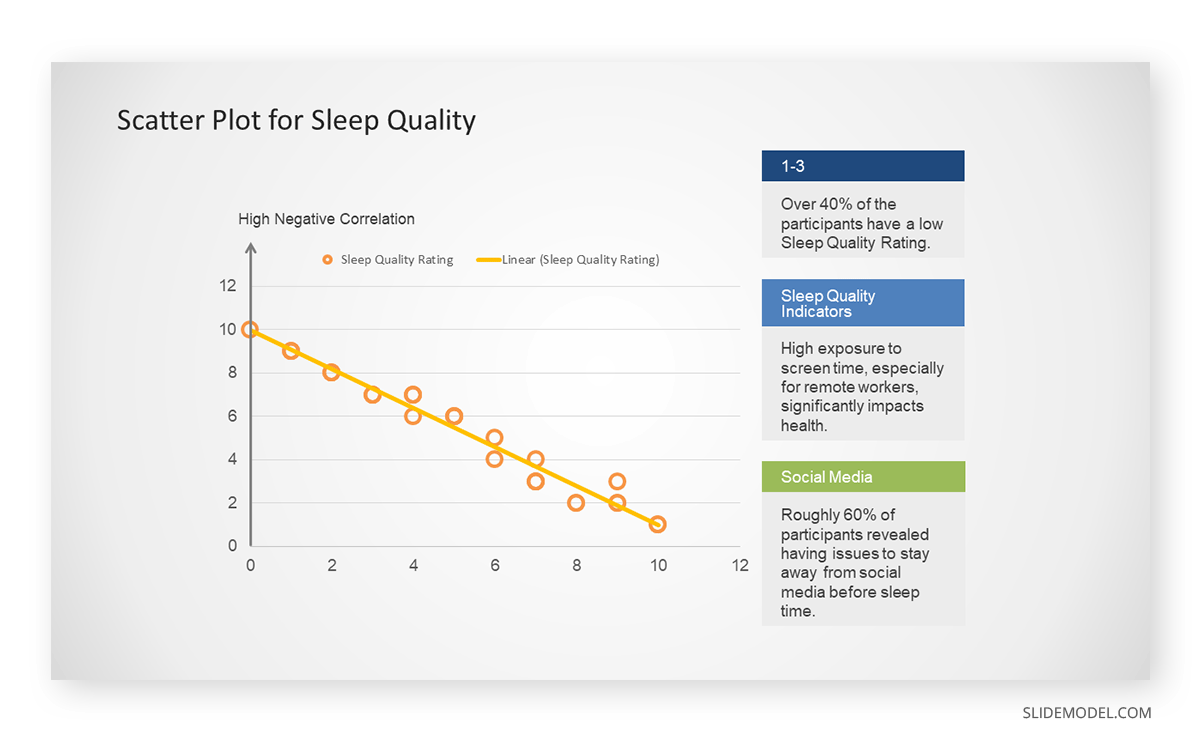
The scientists observe a negative correlation between the amount of screen time and the quality of sleep. This is consistent with their hypothesis that blue light, especially before bedtime, has a significant impact on sleep quality and metabolic processes.
There are a few things to remember when using a scatter plot. Even when a scatter diagram indicates a relationship, it doesn’t mean one variable affects the other. A third factor can influence both variables. The more the plot resembles a straight line, the stronger the relationship is perceived [11] . If it suggests no ties, the observed pattern might be due to random fluctuations in data. When the scatter diagram depicts no correlation, whether the data might be stratified is worth considering.
Choosing the appropriate data presentation type is crucial when making a presentation . Understanding the nature of your data and the message you intend to convey will guide this selection process. For instance, when showcasing quantitative relationships, scatter plots become instrumental in revealing correlations between variables. If the focus is on emphasizing parts of a whole, pie charts offer a concise display of proportions. Histograms, on the other hand, prove valuable for illustrating distributions and frequency patterns.
Bar charts provide a clear visual comparison of different categories. Likewise, line charts excel in showcasing trends over time, while tables are ideal for detailed data examination. Starting a presentation on data presentation types involves evaluating the specific information you want to communicate and selecting the format that aligns with your message. This ensures clarity and resonance with your audience from the beginning of your presentation.
1. Fact Sheet Dashboard for Data Presentation
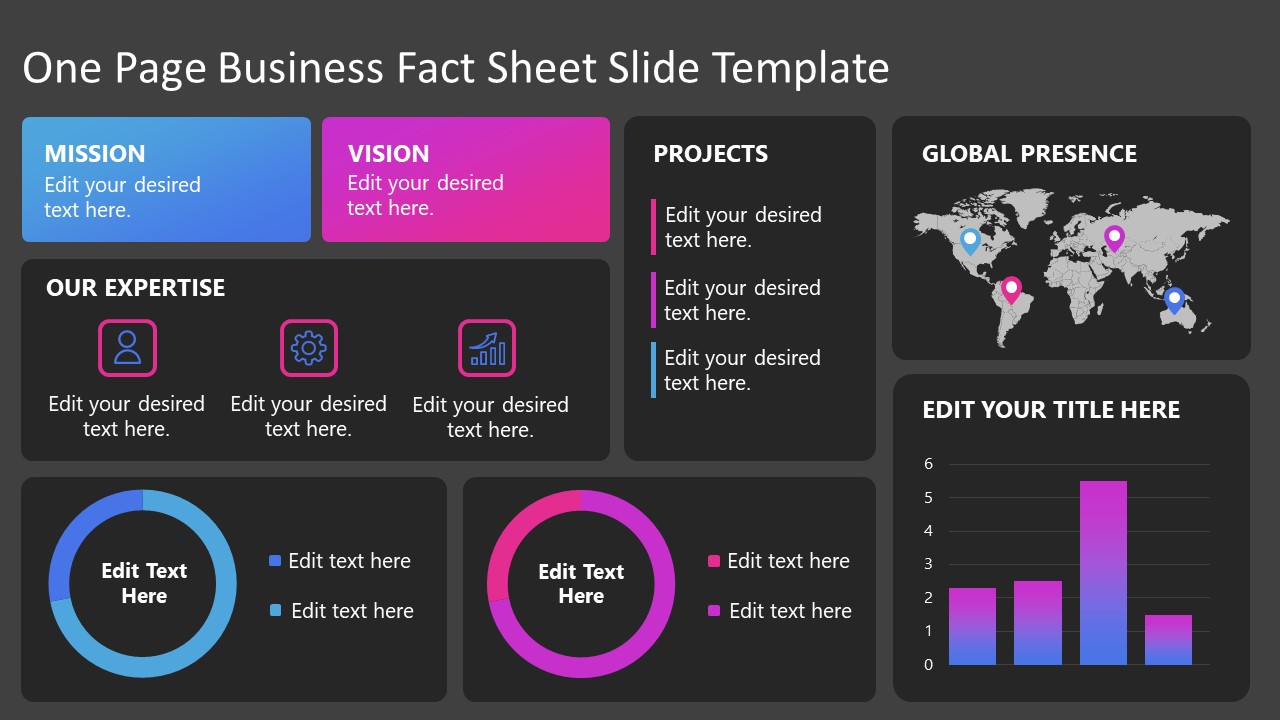
Convey all the data you need to present in this one-pager format, an ideal solution tailored for users looking for presentation aids. Global maps, donut chats, column graphs, and text neatly arranged in a clean layout presented in light and dark themes.
Use This Template
2. 3D Column Chart Infographic PPT Template
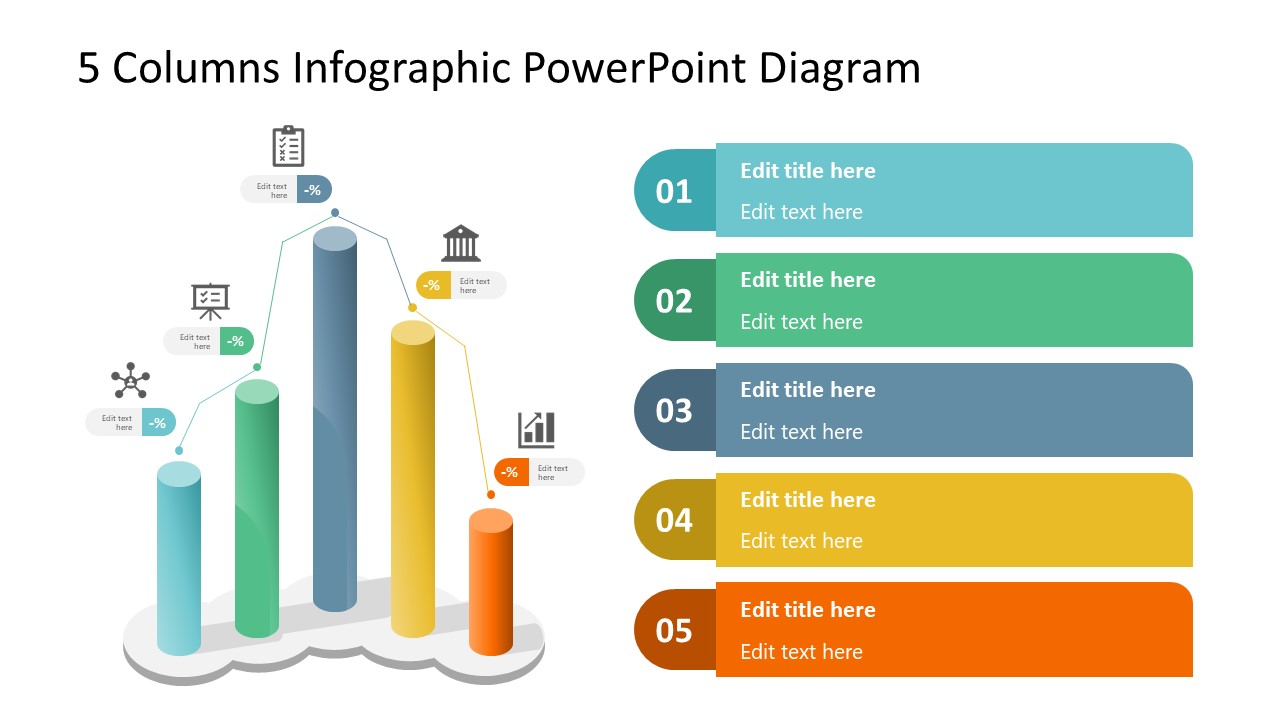
Represent column charts in a highly visual 3D format with this PPT template. A creative way to present data, this template is entirely editable, and we can craft either a one-page infographic or a series of slides explaining what we intend to disclose point by point.
3. Data Circles Infographic PowerPoint Template
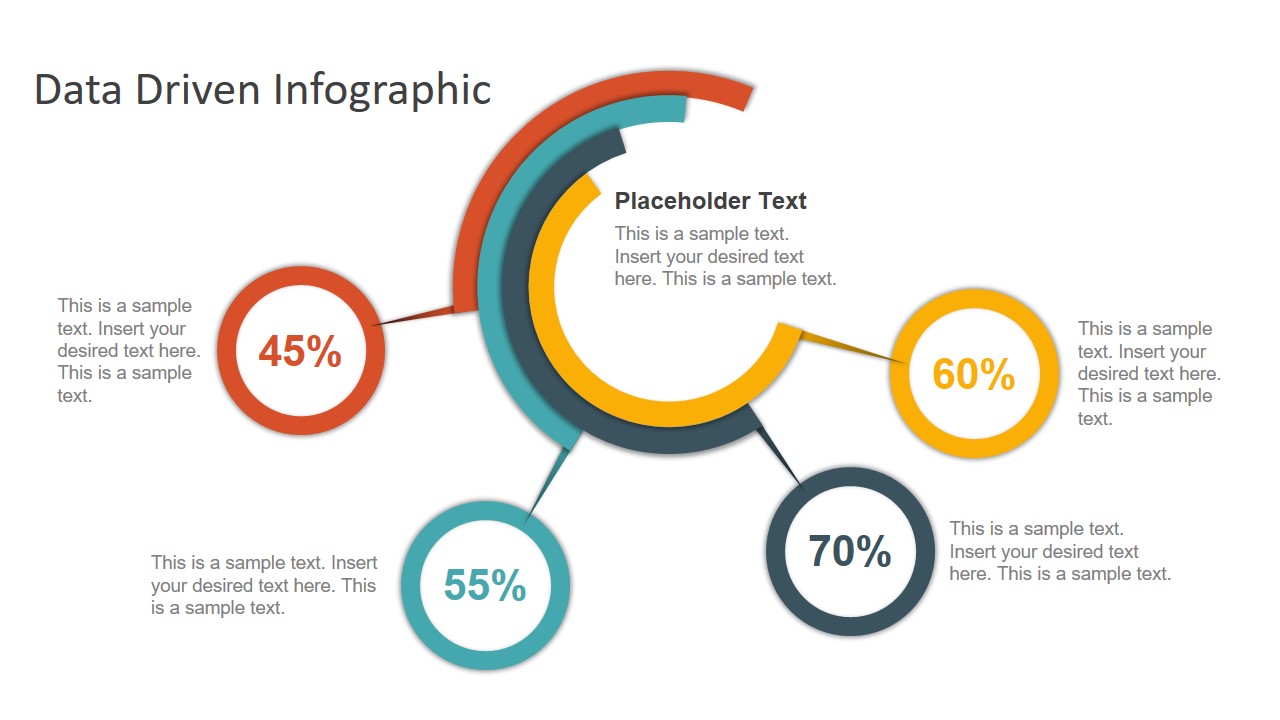
An alternative to the pie chart and donut chart diagrams, this template features a series of curved shapes with bubble callouts as ways of presenting data. Expand the information for each arch in the text placeholder areas.
4. Colorful Metrics Dashboard for Data Presentation
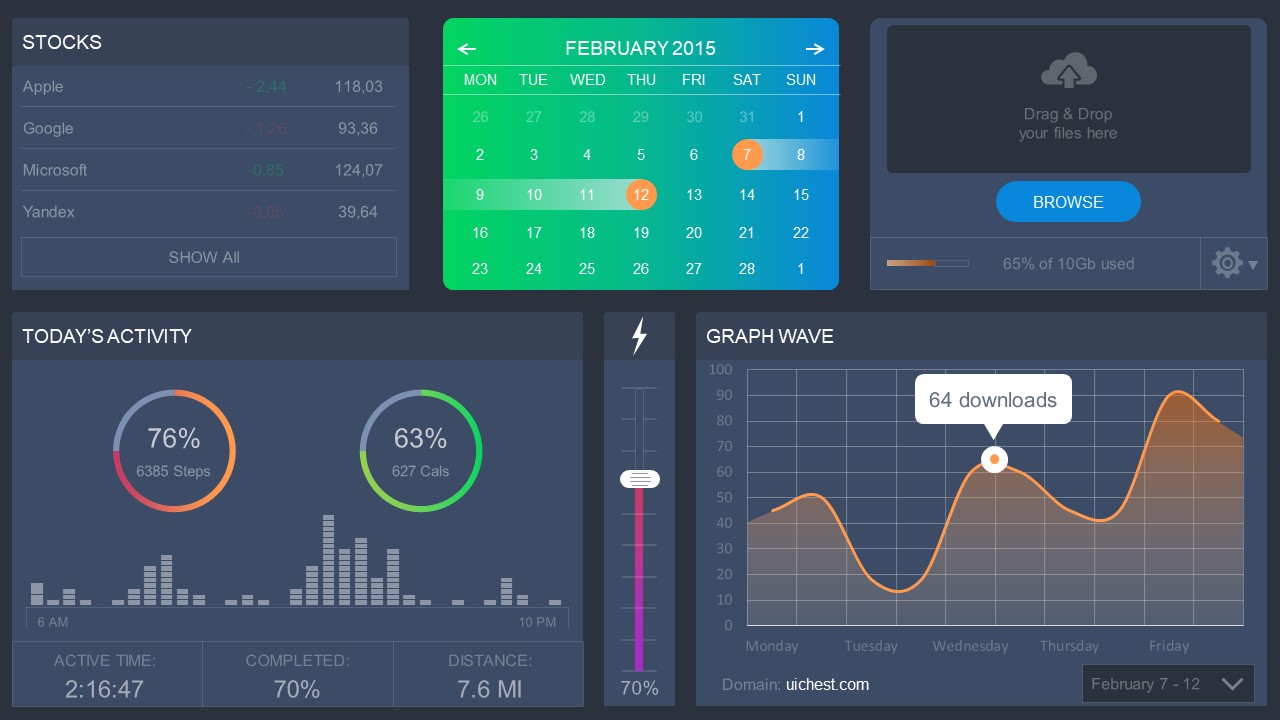
This versatile dashboard template helps us in the presentation of the data by offering several graphs and methods to convert numbers into graphics. Implement it for e-commerce projects, financial projections, project development, and more.
5. Animated Data Presentation Tools for PowerPoint & Google Slides
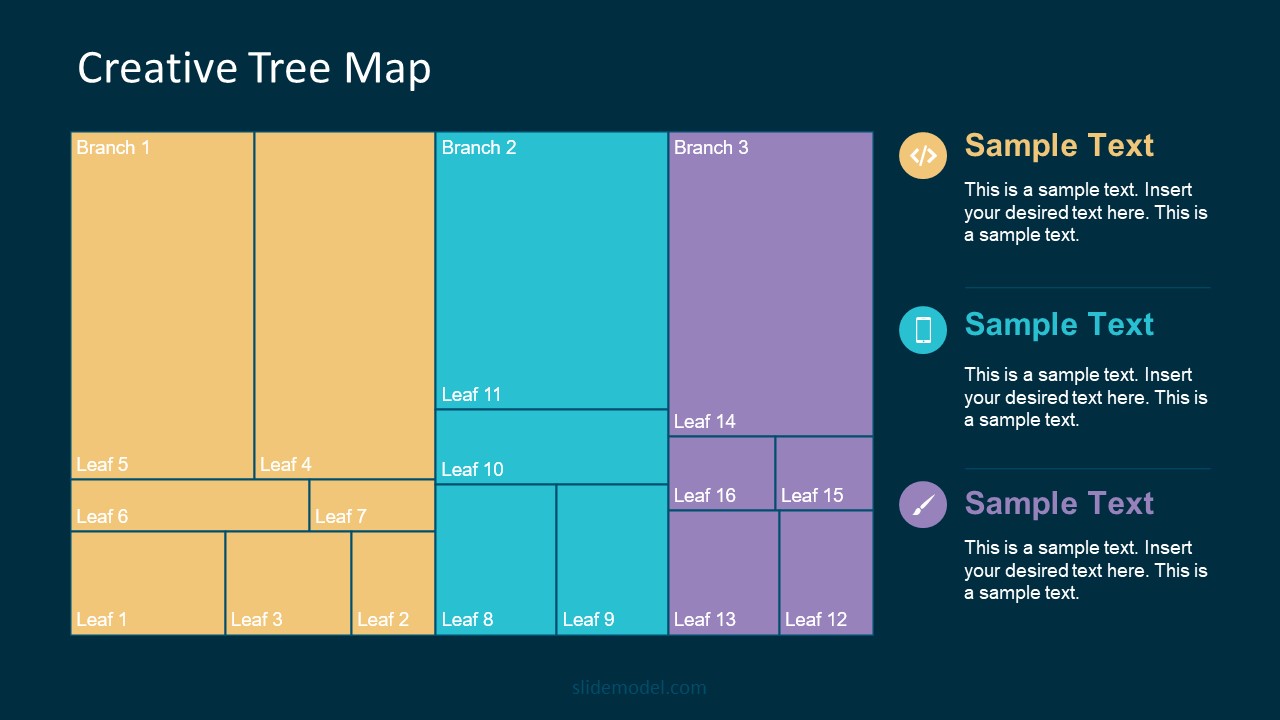
A slide deck filled with most of the tools mentioned in this article, from bar charts, column charts, treemap graphs, pie charts, histogram, etc. Animated effects make each slide look dynamic when sharing data with stakeholders.
6. Statistics Waffle Charts PPT Template for Data Presentations
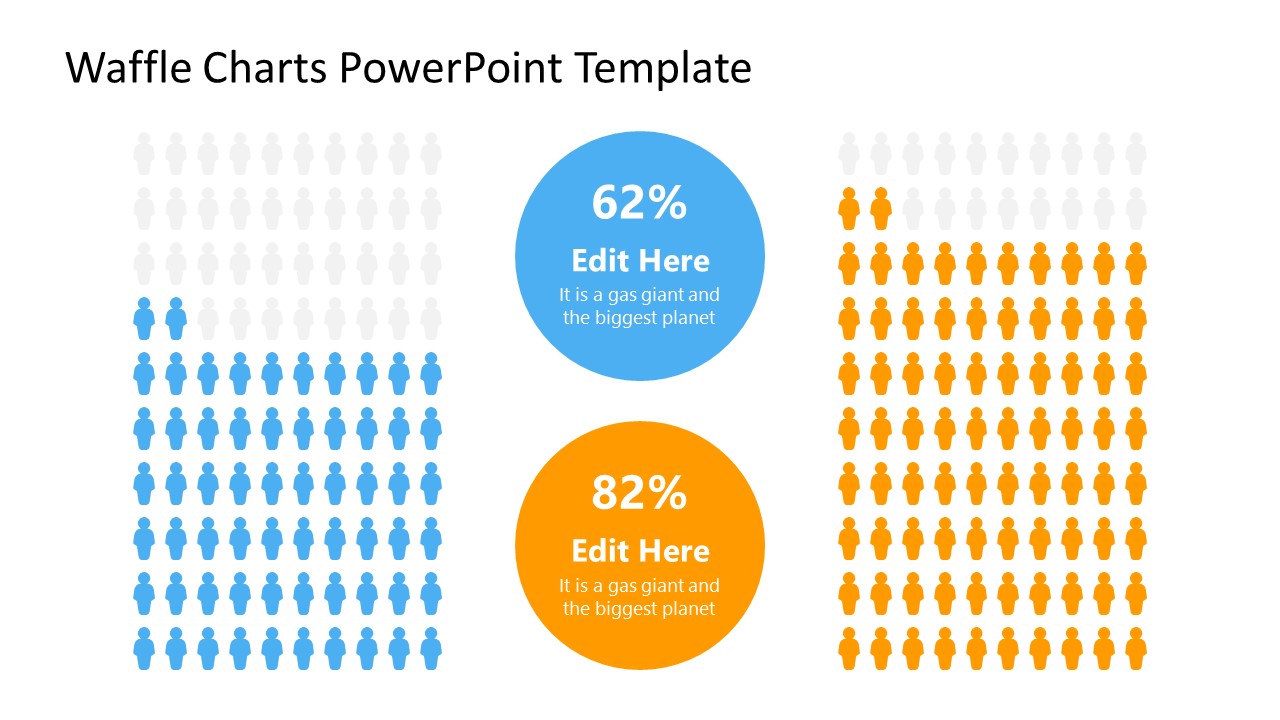
This PPT template helps us how to present data beyond the typical pie chart representation. It is widely used for demographics, so it’s a great fit for marketing teams, data science professionals, HR personnel, and more.
7. Data Presentation Dashboard Template for Google Slides
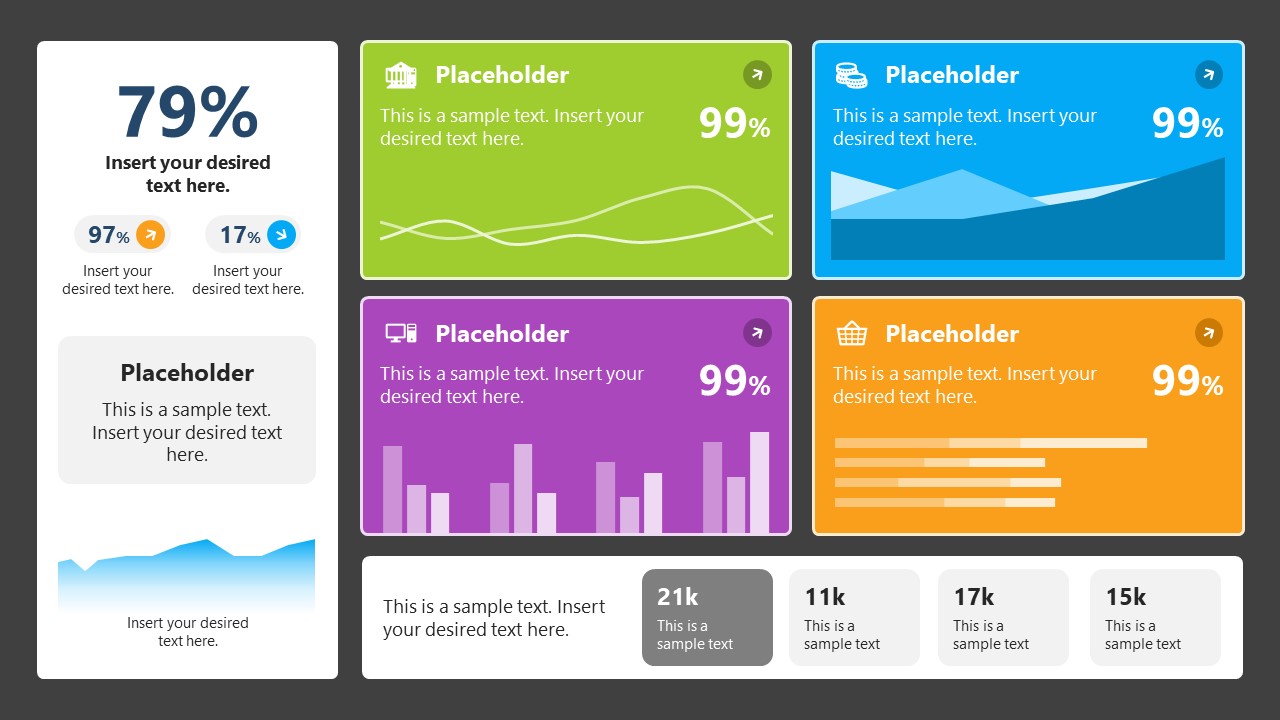
A compendium of tools in dashboard format featuring line graphs, bar charts, column charts, and neatly arranged placeholder text areas.
8. Weather Dashboard for Data Presentation
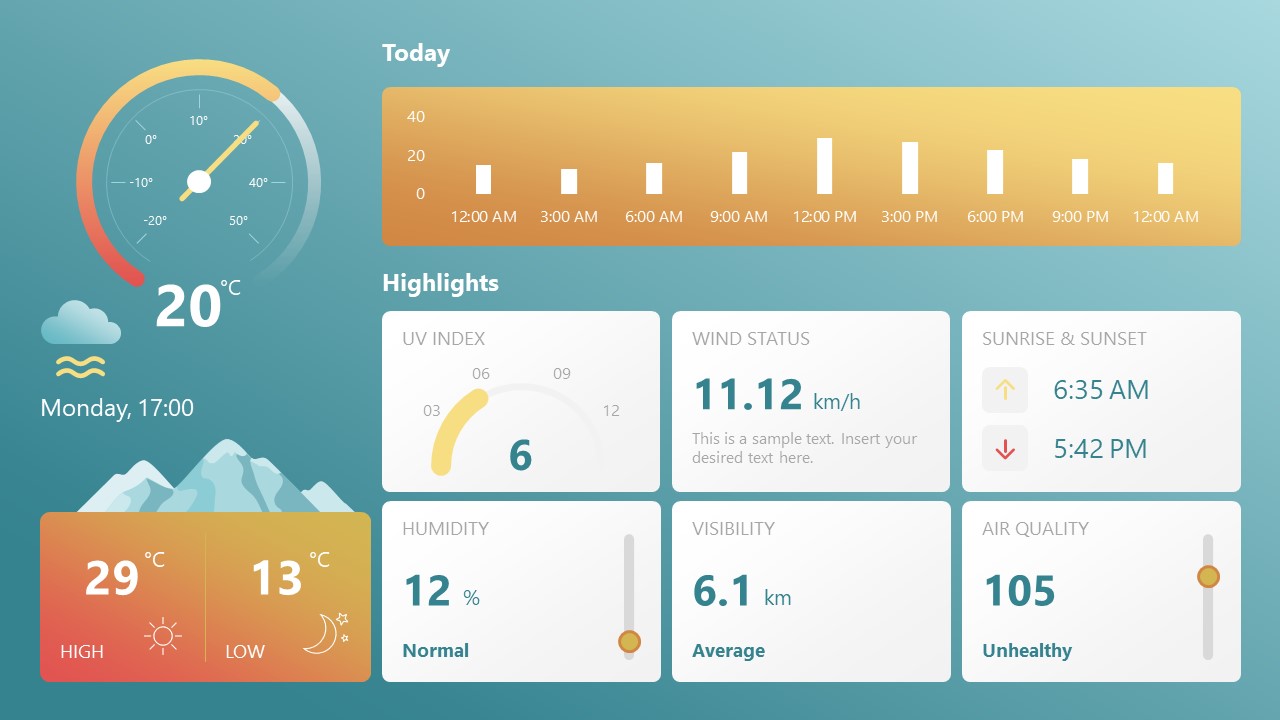
Share weather data for agricultural presentation topics, environmental studies, or any kind of presentation that requires a highly visual layout for weather forecasting on a single day. Two color themes are available.
9. Social Media Marketing Dashboard Data Presentation Template
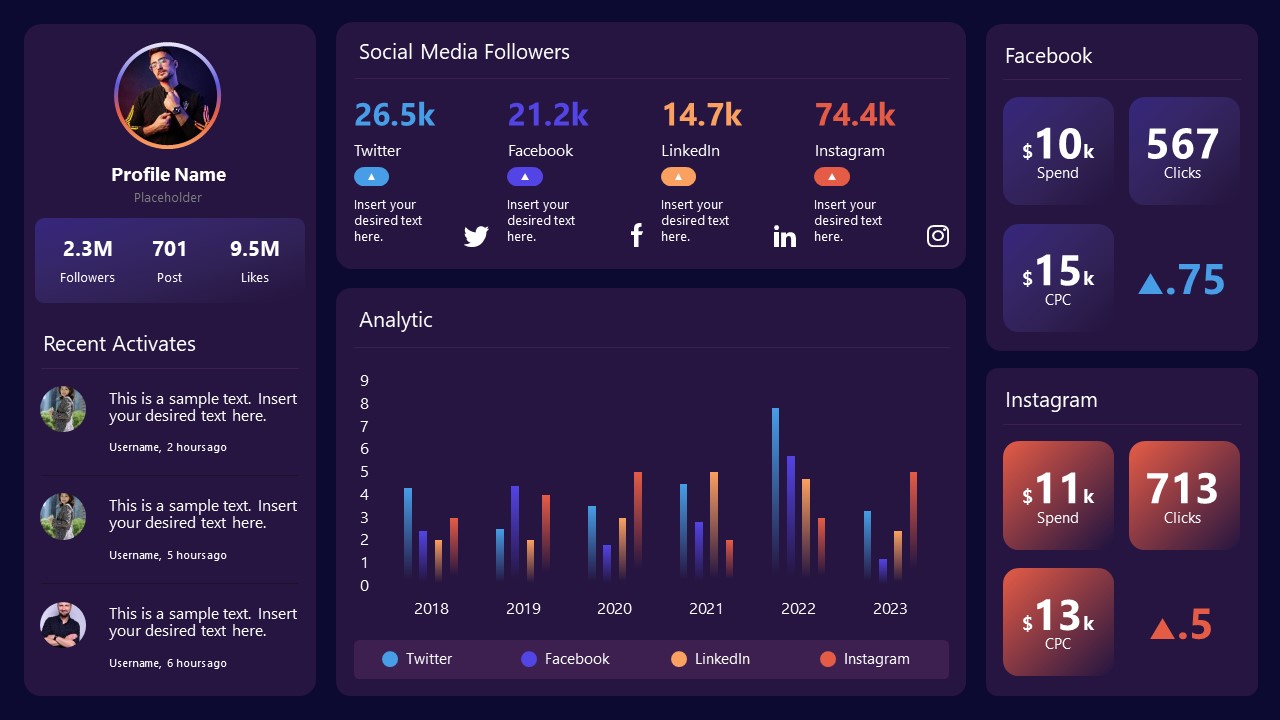
Intended for marketing professionals, this dashboard template for data presentation is a tool for presenting data analytics from social media channels. Two slide layouts featuring line graphs and column charts.
10. Project Management Summary Dashboard Template
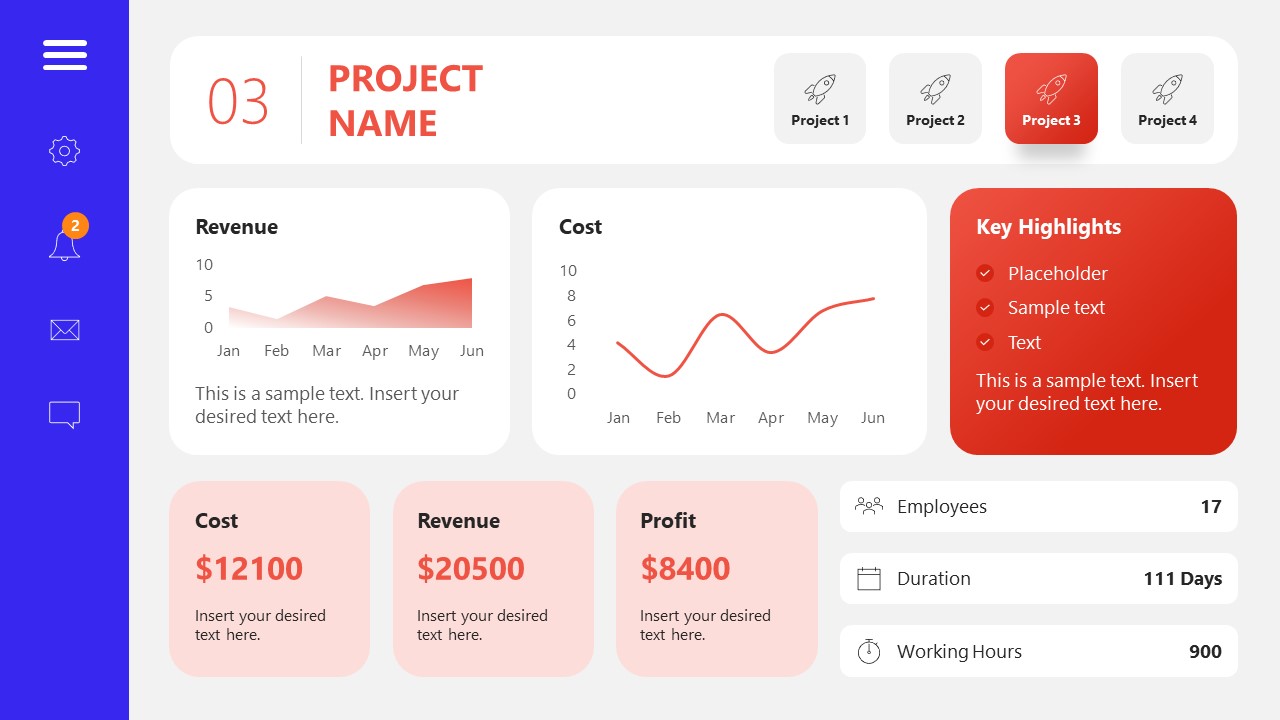
A tool crafted for project managers to deliver highly visual reports on a project’s completion, the profits it delivered for the company, and expenses/time required to execute it. 4 different color layouts are available.
11. Profit & Loss Dashboard for PowerPoint and Google Slides
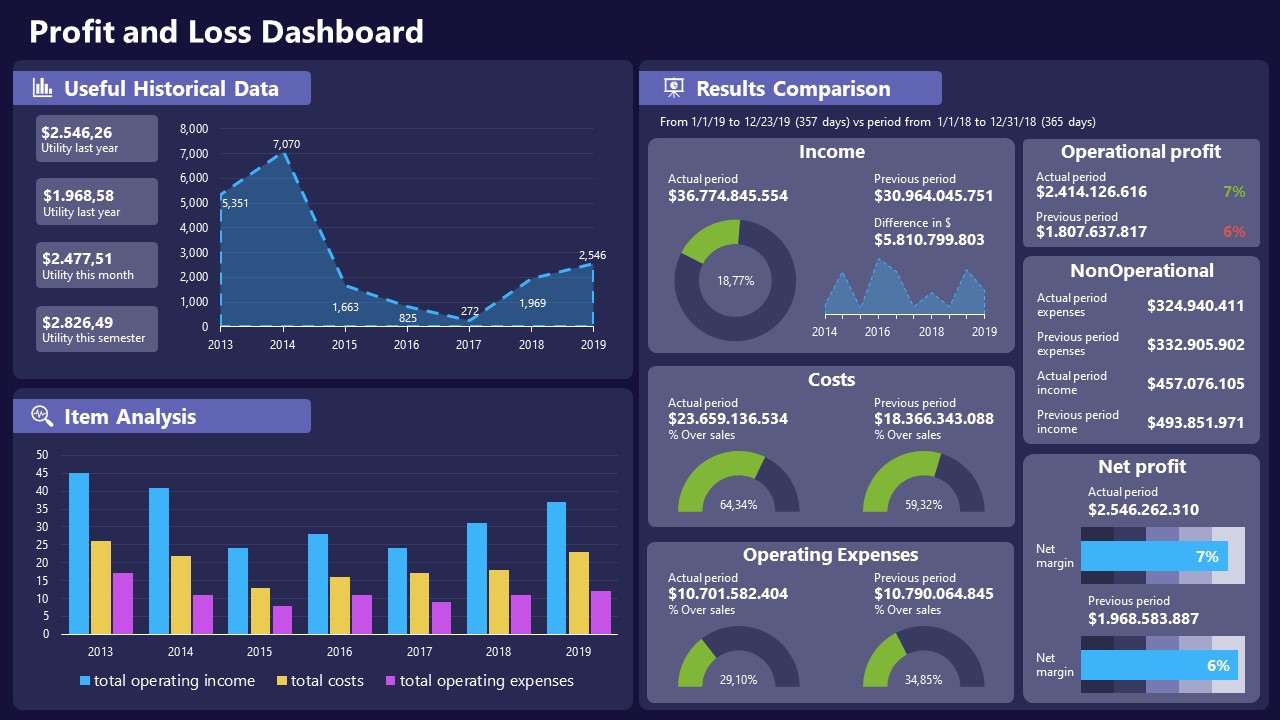
A must-have for finance professionals. This typical profit & loss dashboard includes progress bars, donut charts, column charts, line graphs, and everything that’s required to deliver a comprehensive report about a company’s financial situation.
Overwhelming visuals
One of the mistakes related to using data-presenting methods is including too much data or using overly complex visualizations. They can confuse the audience and dilute the key message.
Inappropriate chart types
Choosing the wrong type of chart for the data at hand can lead to misinterpretation. For example, using a pie chart for data that doesn’t represent parts of a whole is not right.
Lack of context
Failing to provide context or sufficient labeling can make it challenging for the audience to understand the significance of the presented data.
Inconsistency in design
Using inconsistent design elements and color schemes across different visualizations can create confusion and visual disarray.
Failure to provide details
Simply presenting raw data without offering clear insights or takeaways can leave the audience without a meaningful conclusion.
Lack of focus
Not having a clear focus on the key message or main takeaway can result in a presentation that lacks a central theme.
Visual accessibility issues
Overlooking the visual accessibility of charts and graphs can exclude certain audience members who may have difficulty interpreting visual information.
In order to avoid these mistakes in data presentation, presenters can benefit from using presentation templates . These templates provide a structured framework. They ensure consistency, clarity, and an aesthetically pleasing design, enhancing data communication’s overall impact.
Understanding and choosing data presentation types are pivotal in effective communication. Each method serves a unique purpose, so selecting the appropriate one depends on the nature of the data and the message to be conveyed. The diverse array of presentation types offers versatility in visually representing information, from bar charts showing values to pie charts illustrating proportions.
Using the proper method enhances clarity, engages the audience, and ensures that data sets are not just presented but comprehensively understood. By appreciating the strengths and limitations of different presentation types, communicators can tailor their approach to convey information accurately, developing a deeper connection between data and audience understanding.
[1] Government of Canada, S.C. (2021) 5 Data Visualization 5.2 Bar Chart , 5.2 Bar chart . https://www150.statcan.gc.ca/n1/edu/power-pouvoir/ch9/bargraph-diagrammeabarres/5214818-eng.htm
[2] Kosslyn, S.M., 1989. Understanding charts and graphs. Applied cognitive psychology, 3(3), pp.185-225. https://apps.dtic.mil/sti/pdfs/ADA183409.pdf
[3] Creating a Dashboard . https://it.tufts.edu/book/export/html/1870
[4] https://www.goldenwestcollege.edu/research/data-and-more/data-dashboards/index.html
[5] https://www.mit.edu/course/21/21.guide/grf-line.htm
[6] Jadeja, M. and Shah, K., 2015, January. Tree-Map: A Visualization Tool for Large Data. In GSB@ SIGIR (pp. 9-13). https://ceur-ws.org/Vol-1393/gsb15proceedings.pdf#page=15
[7] Heat Maps and Quilt Plots. https://www.publichealth.columbia.edu/research/population-health-methods/heat-maps-and-quilt-plots
[8] EIU QGIS WORKSHOP. https://www.eiu.edu/qgisworkshop/heatmaps.php
[9] About Pie Charts. https://www.mit.edu/~mbarker/formula1/f1help/11-ch-c8.htm
[10] Histograms. https://sites.utexas.edu/sos/guided/descriptive/numericaldd/descriptiven2/histogram/ [11] https://asq.org/quality-resources/scatter-diagram
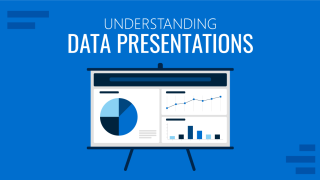
Like this article? Please share
Data Analysis, Data Science, Data Visualization Filed under Design
Related Articles
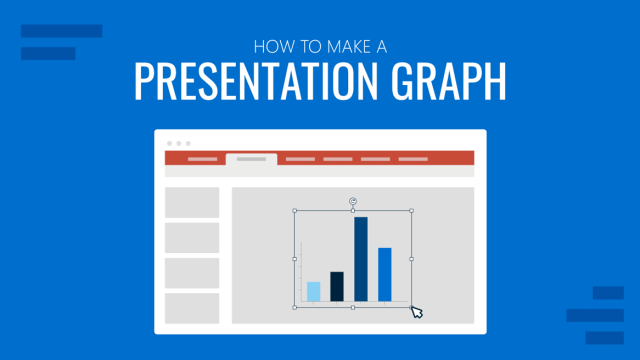
Filed under Design • March 27th, 2024
How to Make a Presentation Graph
Detailed step-by-step instructions to master the art of how to make a presentation graph in PowerPoint and Google Slides. Check it out!
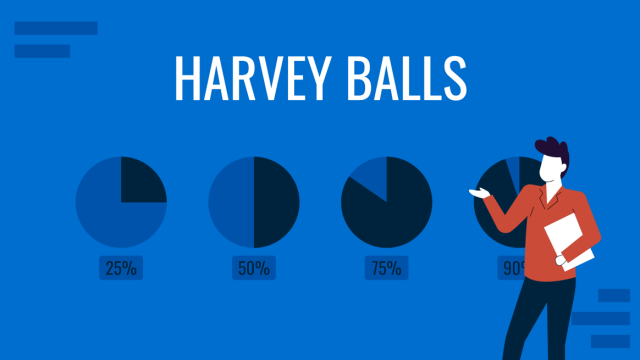
Filed under Presentation Ideas • January 6th, 2024
All About Using Harvey Balls
Among the many tools in the arsenal of the modern presenter, Harvey Balls have a special place. In this article we will tell you all about using Harvey Balls.
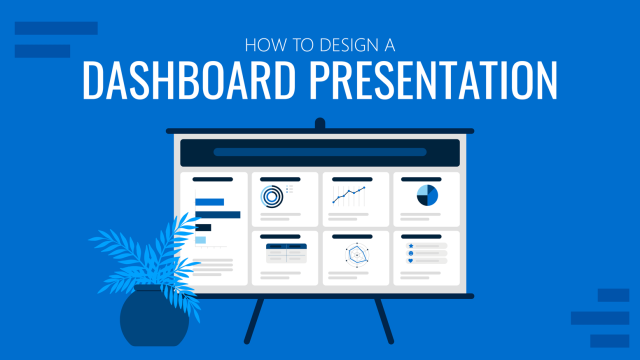
Filed under Business • December 8th, 2023
How to Design a Dashboard Presentation: A Step-by-Step Guide
Take a step further in your professional presentation skills by learning what a dashboard presentation is and how to properly design one in PowerPoint. A detailed step-by-step guide is here!
Leave a Reply
- Slidesgo School
- Presentation Tips
How to Present Data Effectively
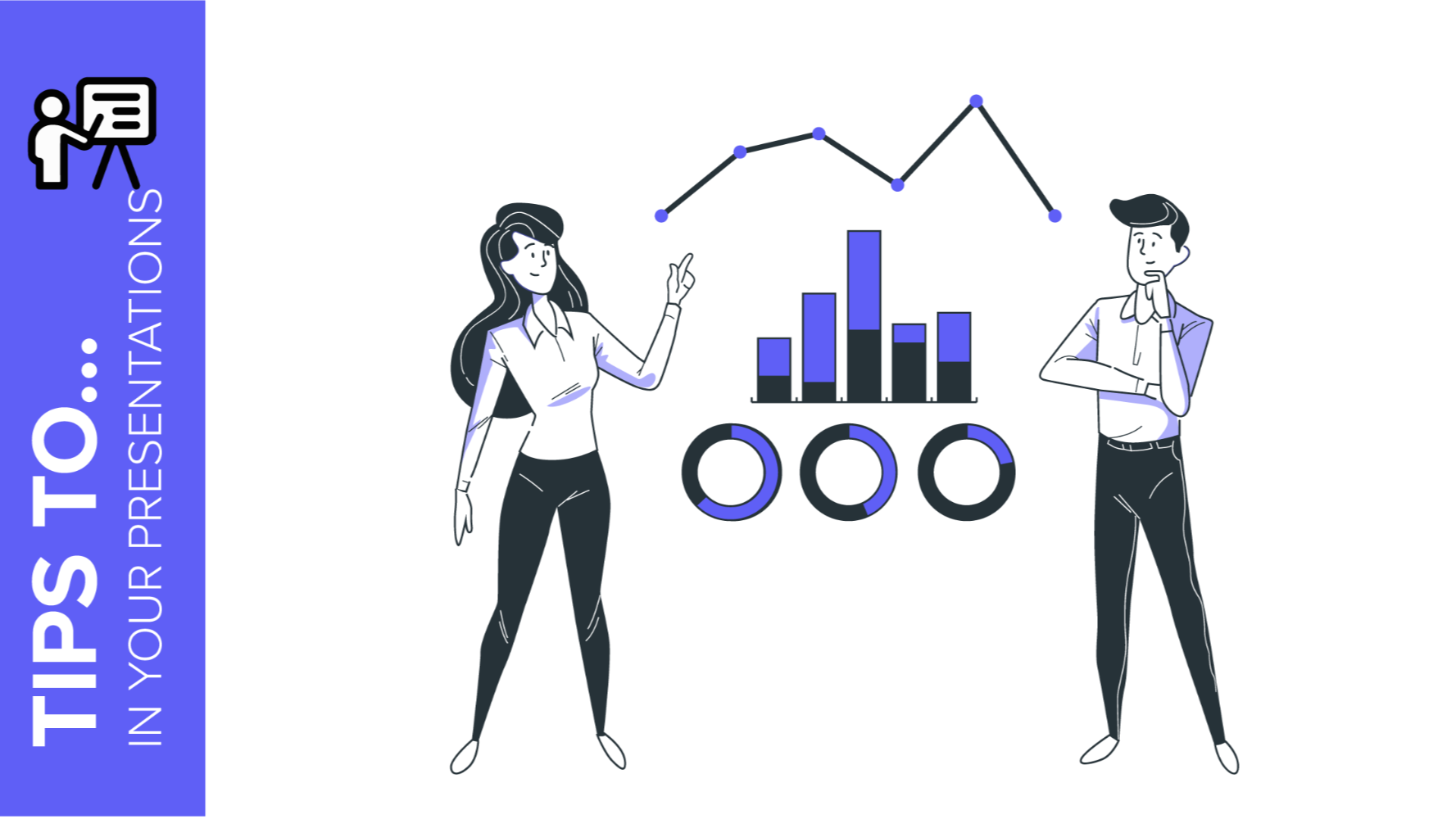
You’re sitting in front of your computer and ready to put together a presentation involving data. The numbers stare at you from your screen, jumbled and raw. How do you start? Numbers on their own can be difficult to digest. Without any context, they’re just that—numbers. But organize them well and they tell a story. In this blog post, we’ll go into the importance of structuring data in a presentation and provide tips on how to do it well. These tips are practical and applicable for all sorts of presentations—from marketing plans and medical breakthroughs to project proposals and portfolios.
What is data presentation?
3 essential tips on data presentation, use the right chart, keep it simple, use text wisely and sparingly.
In many ways, data presentation is like storytelling—only you do them with a series of graphs and charts. One of the most common mistakes presenters make is being so submerged in the data that they fail to view it from an outsider’s point of view. Always keep this in mind: What makes sense to you may not make sense to your audience. To portray figures and statistics in a way that’s comprehensible to your viewers, step back, put yourself in their shoes, and consider the following:
- How much do they know about the topic?
- How much information will they need?
- What data will impress them?
Providing a context helps your audience visualize and understand the numbers. To help you achieve that, here are three tips on how to represent data effectively.
Whether you’re using Google Slides or PowerPoint, both come equipped with a range of design tools that help you help your viewers make sense of your qualitative data. The key here is to know how to use them and how to use them well. In these tips, we’ll cover the basics of data presentation that are often overlooked but also go beyond basics for more professional advice.
The downside of having too many tools at your disposal is that it makes selecting an uphill task. Pie and bar charts are by far the most commonly used methods as they are versatile and easy to understand.
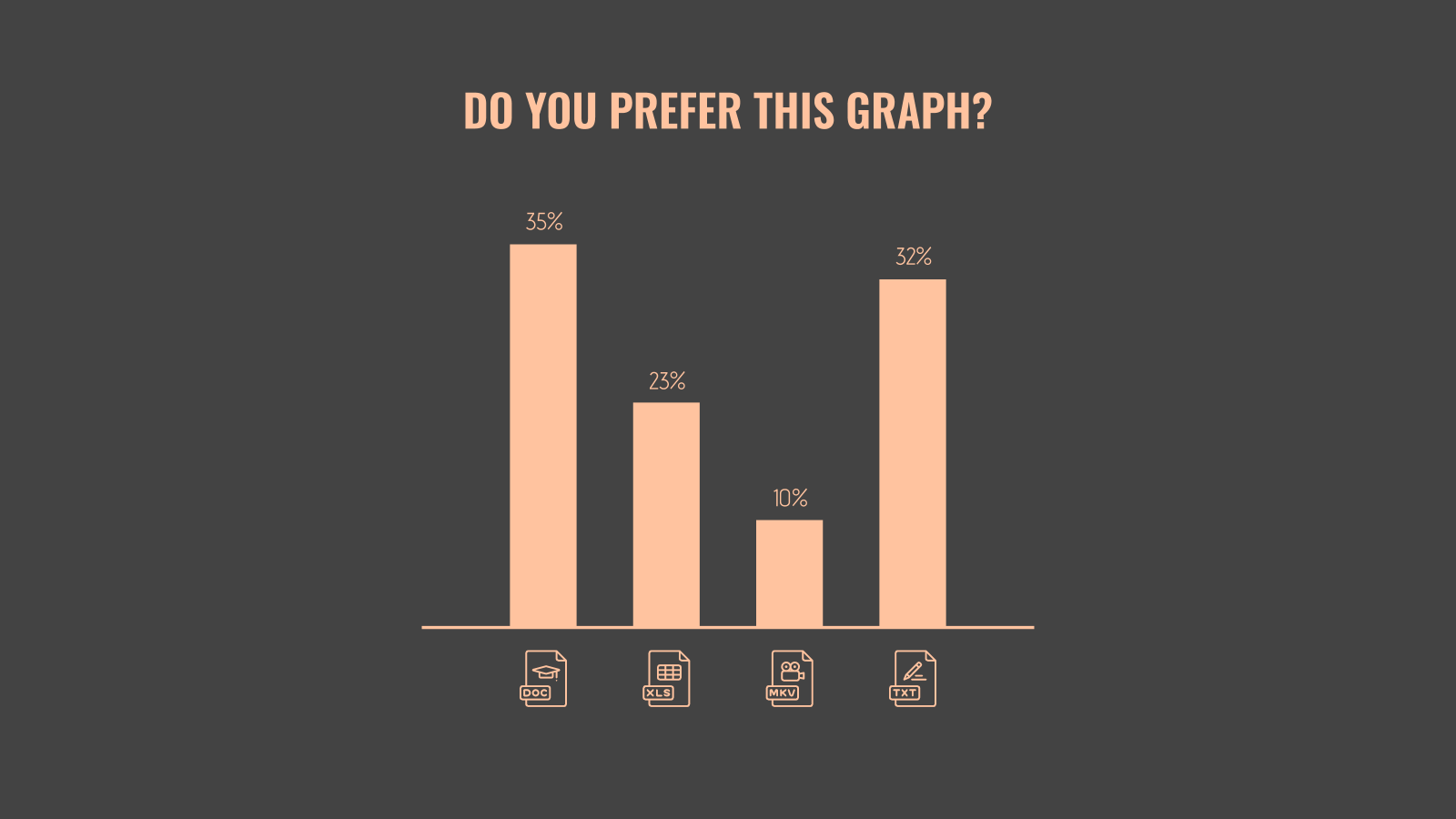
If you’re looking to kick things up a notch, think outside the box. When the numbers allow for it, opt for something different. For example, donut charts can sometimes be used to execute the same effect as pie charts.
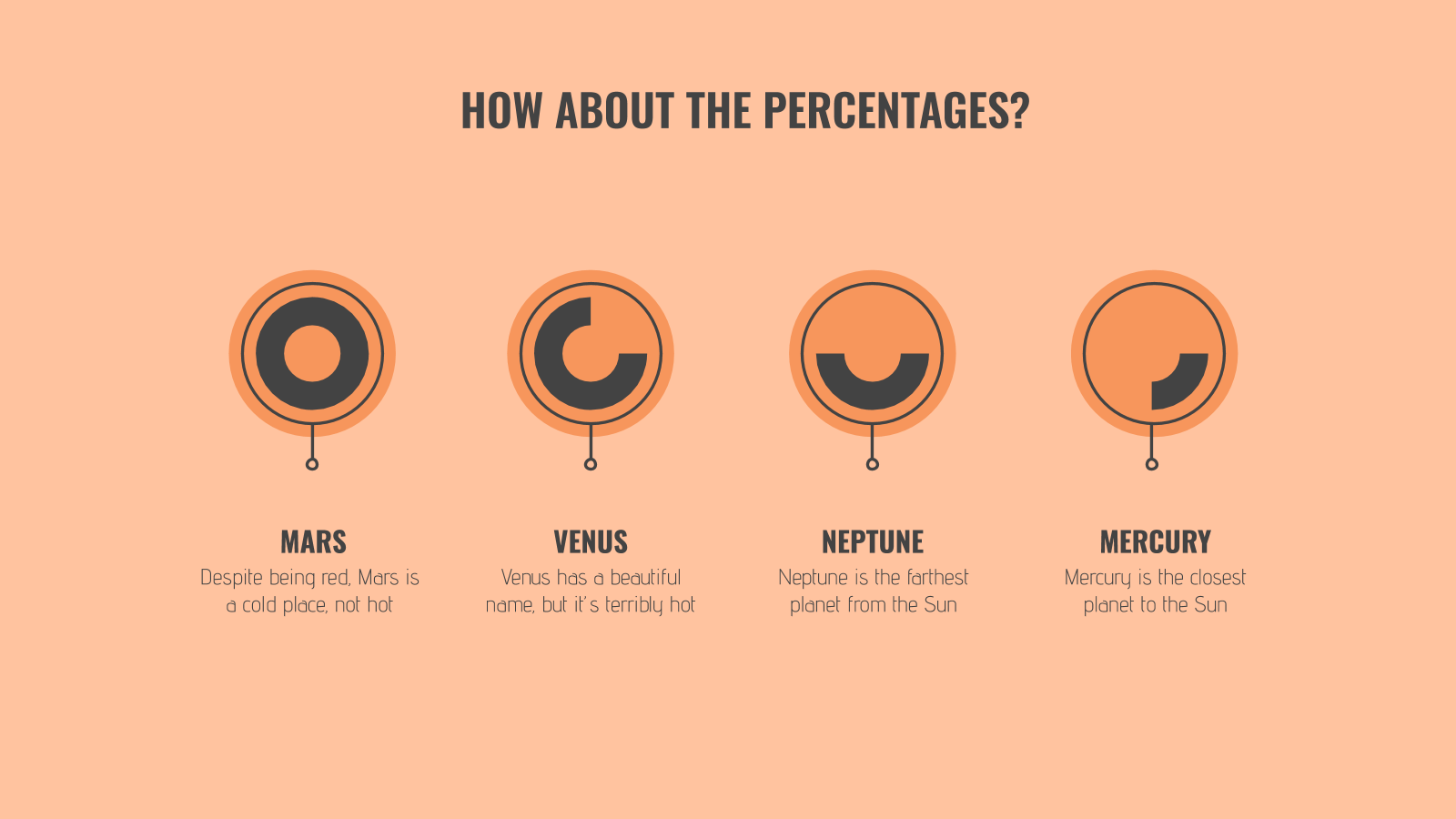
But these conventional graphs and charts aren’t applicable to all types of data. For example, if you’re comparing numerous variables and factors, a bar chart would do no good. A table, on the other hand, offers a much cleaner look.
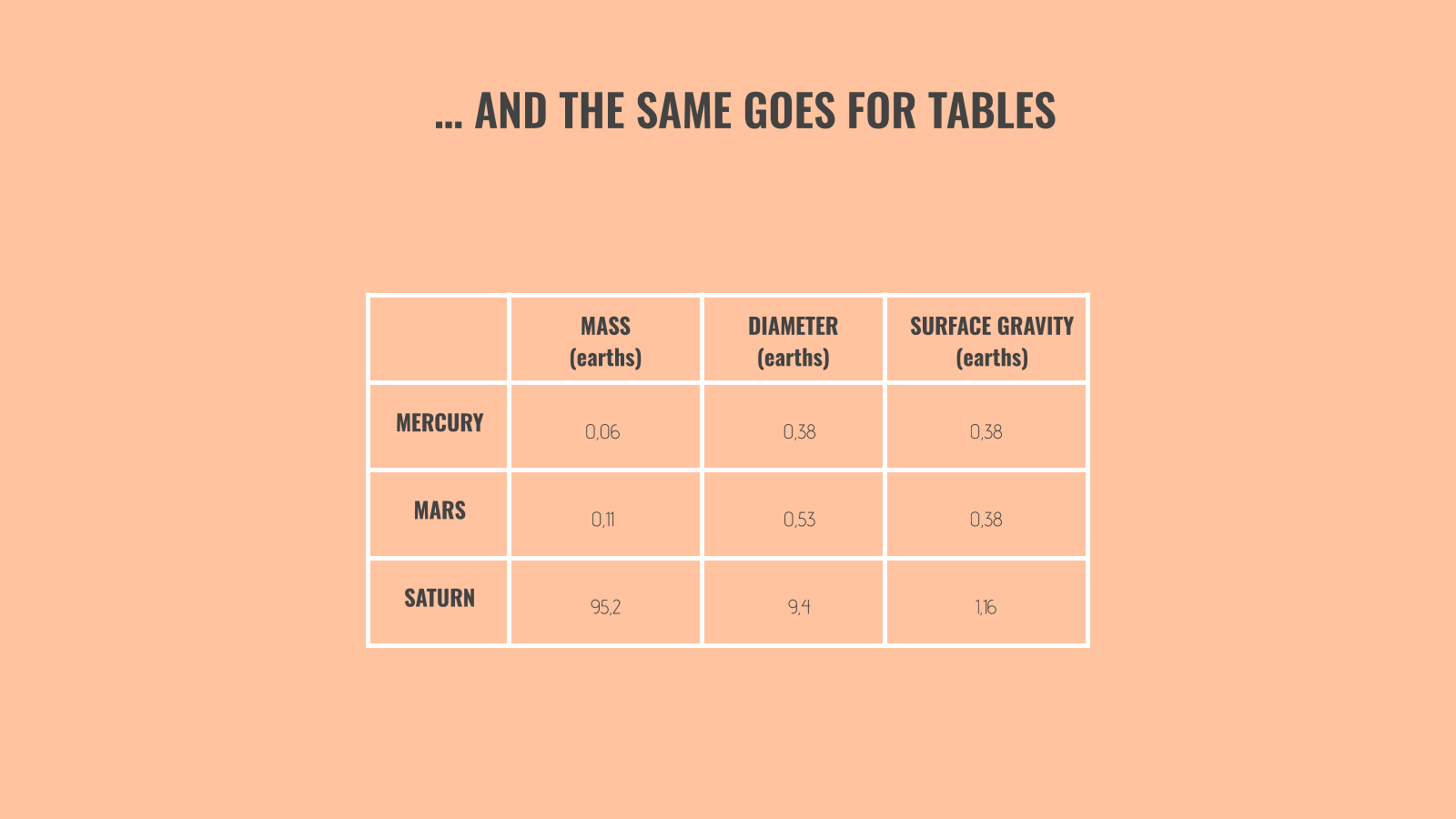
Pro tip : If you want to go beyond basics, create your own shapes and use their sizes to reflect proportion, as seen in this next image.
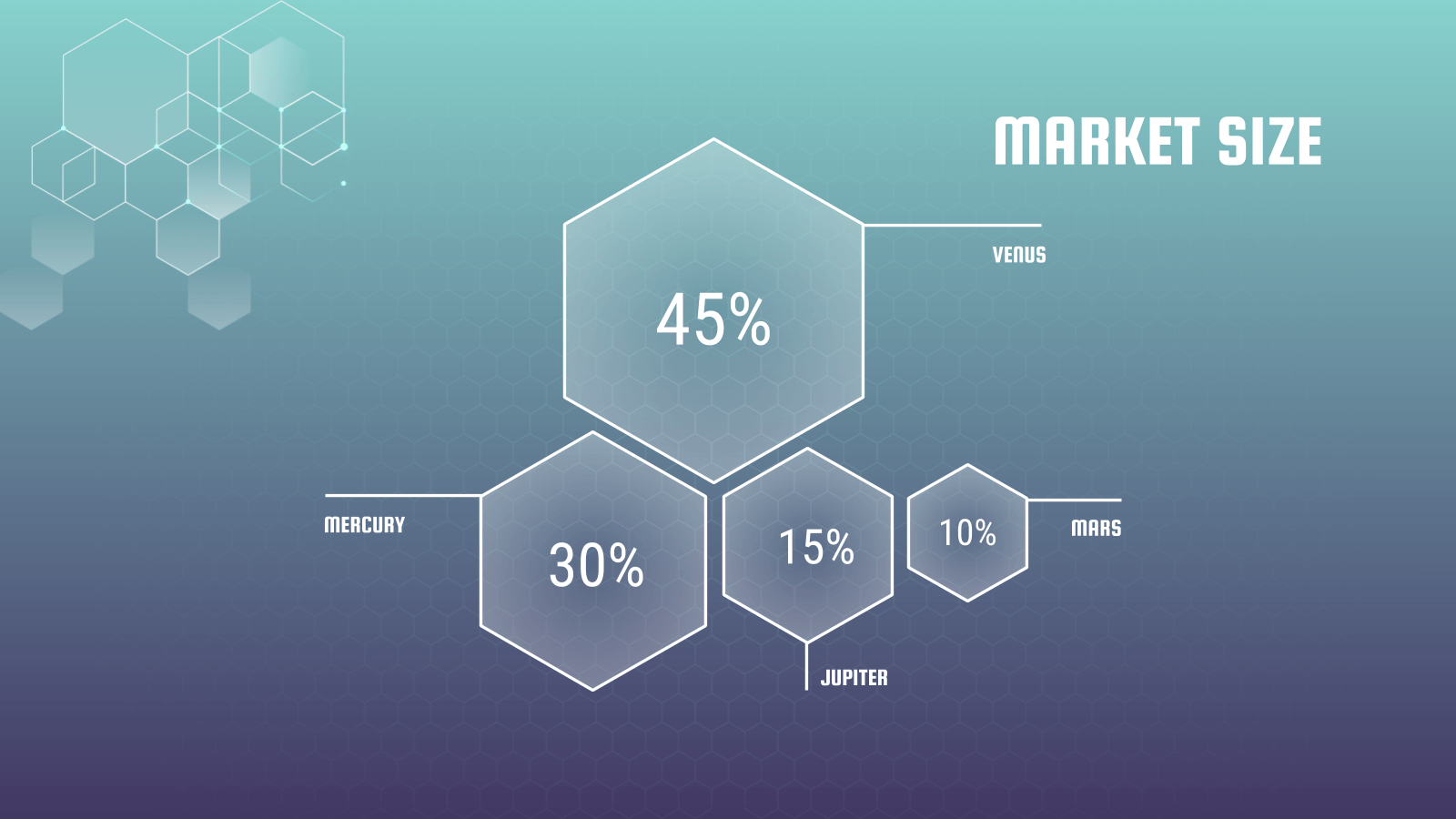
Their sizes don’t have to be an exact reflection of their proportions. What’s important here is that they’re discernible and are of the same shape so that your viewers can grasp its concept at first glance. Note that this should only be used for comparisons with large enough contrasts. For instance, it’d be difficult to use this to compare two market sizes of 25 percent and 26 percent.
When it comes to making qualitative data digestible, simplicity does the trick. Limit the number of elements on the slide as much as possible and provide only the bare essentials.
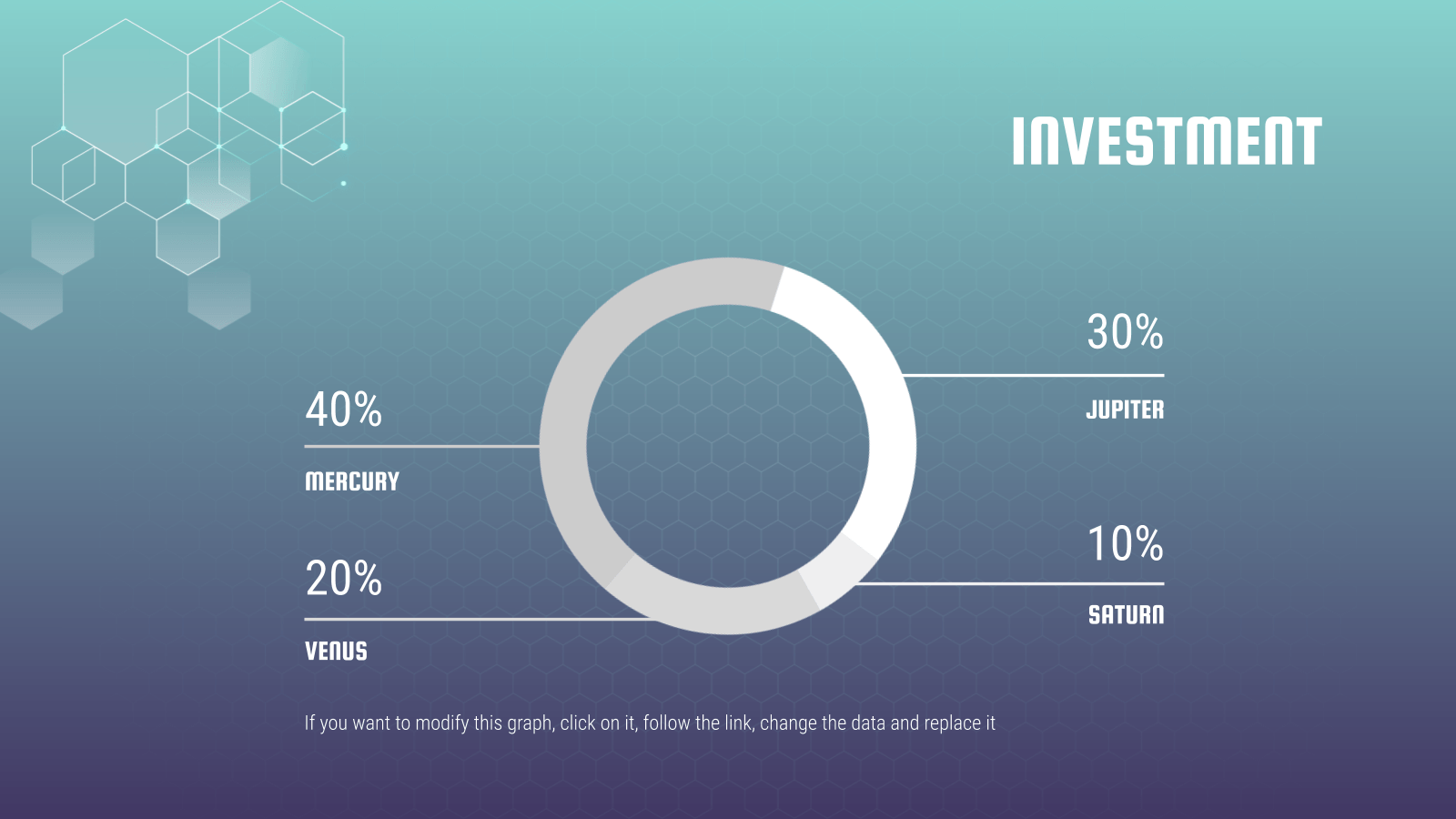
See how simple this slide is? In one glance, your eye immediately goes to the percentages of the donut because there are no text boxes, illustrations, graphics, etc. to distract you. Sometimes, more context is needed for your numbers to make sense. In the spirit of keeping your slides neat, you may be tempted to spread the data across two slides. But that makes it complicated, so putting it all on one slide is your only option. In such cases, our mantra of “keep it simple” still applies. The trick lies in neat positioning and clever formatting.
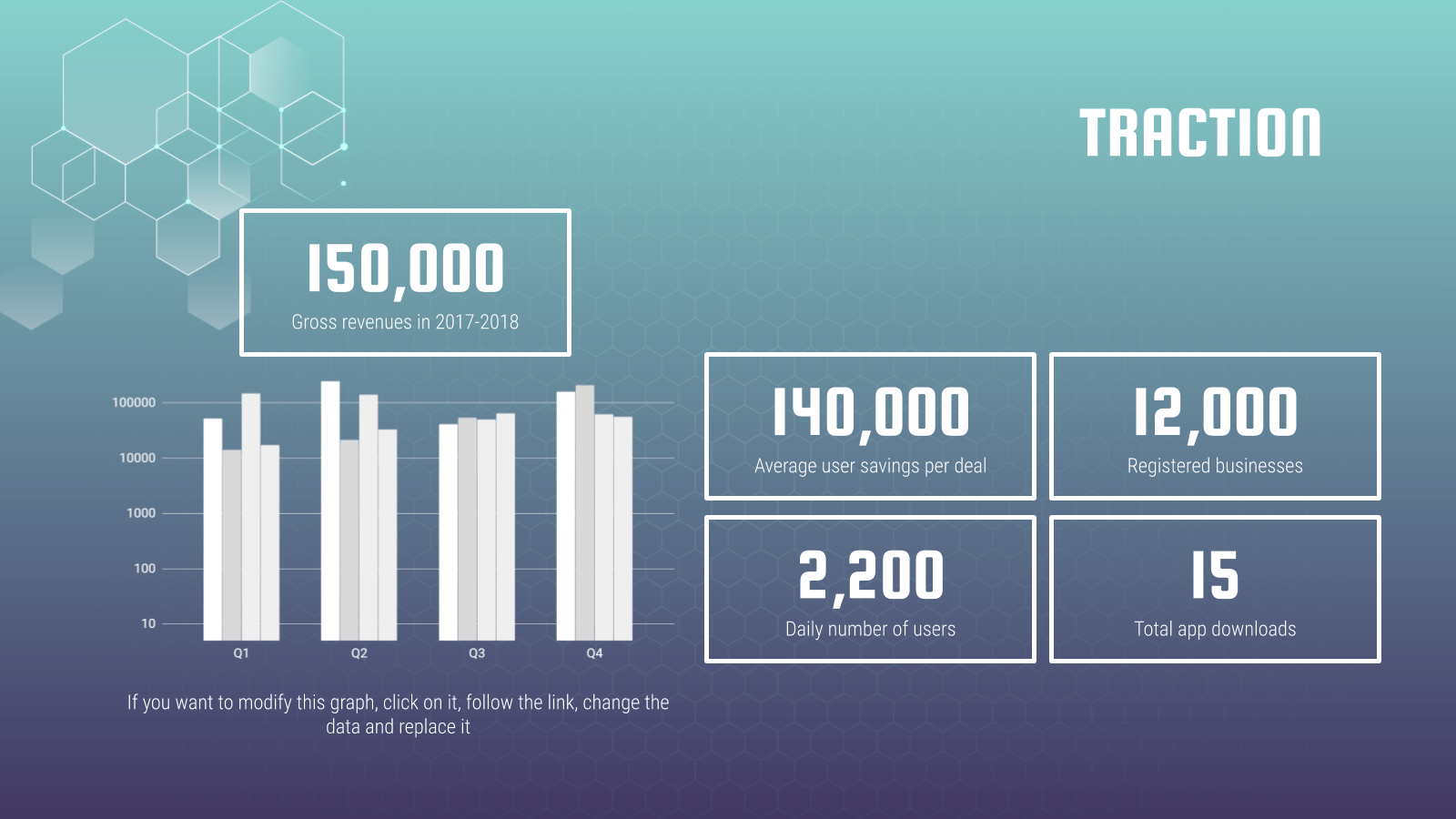
In the above slides, we’ve used boxes to highlight supporting figures while giving enough attention to the main chart. This separates them visually and helps the audience focus better. With the slide already pretty full, it’s crucial to use a plain background or risk overwhelming your viewers.
Last but certainly not least, our final tip involves the use of text. Just because you’re telling a story with numbers doesn’t mean text cannot be used. In fact, the contrary proves true: Text plays a vital role in data presentation and should be used strategically. To highlight a particular statistic, do not hesitate to go all out and have that be the focal point of your slide for emphasis. Keep text to a minimum and as a supporting element.
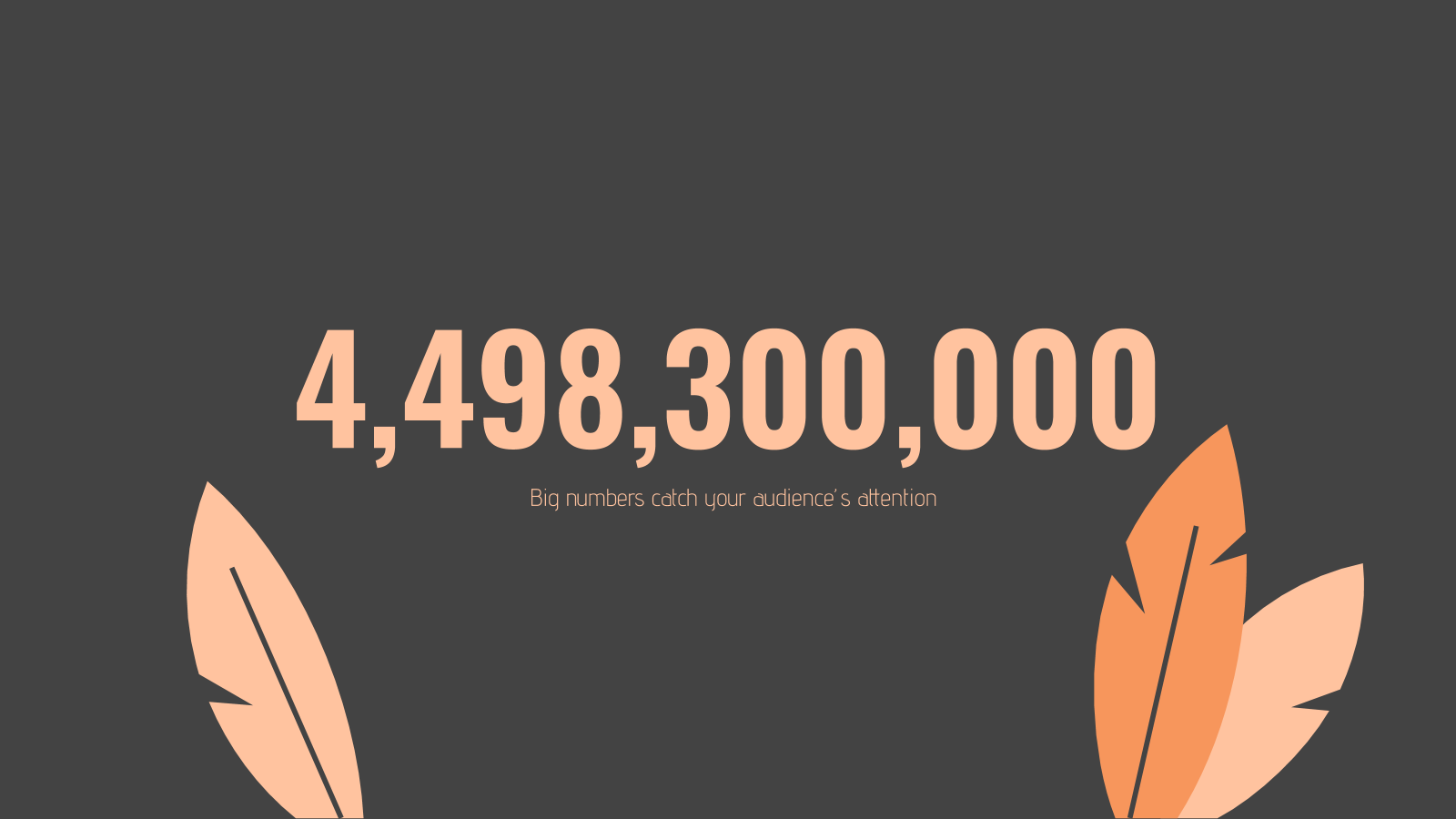
Make sure your numbers are formatted clearly. Large figures should have thousands separated with commas. For example, 4,498,300,000 makes for a much easier read than “4498300000”. Any corresponding units should also be clear. With data presentation, don’t forget that numbers are still your protagonist, so they must be highlighted with a larger or bolder font. Where there are numbers and graphics, space is scarce so every single word must be chosen wisely. The key here is to ensure your viewers understand what your data represents in one glance but to leave it sufficiently vague, like a teaser, so that they pay attention to your speech for more information. → Slidesgo’s free presentation templates come included with specially designed and created charts and graphs that you can easily personalize according to your data. Give them a try now!
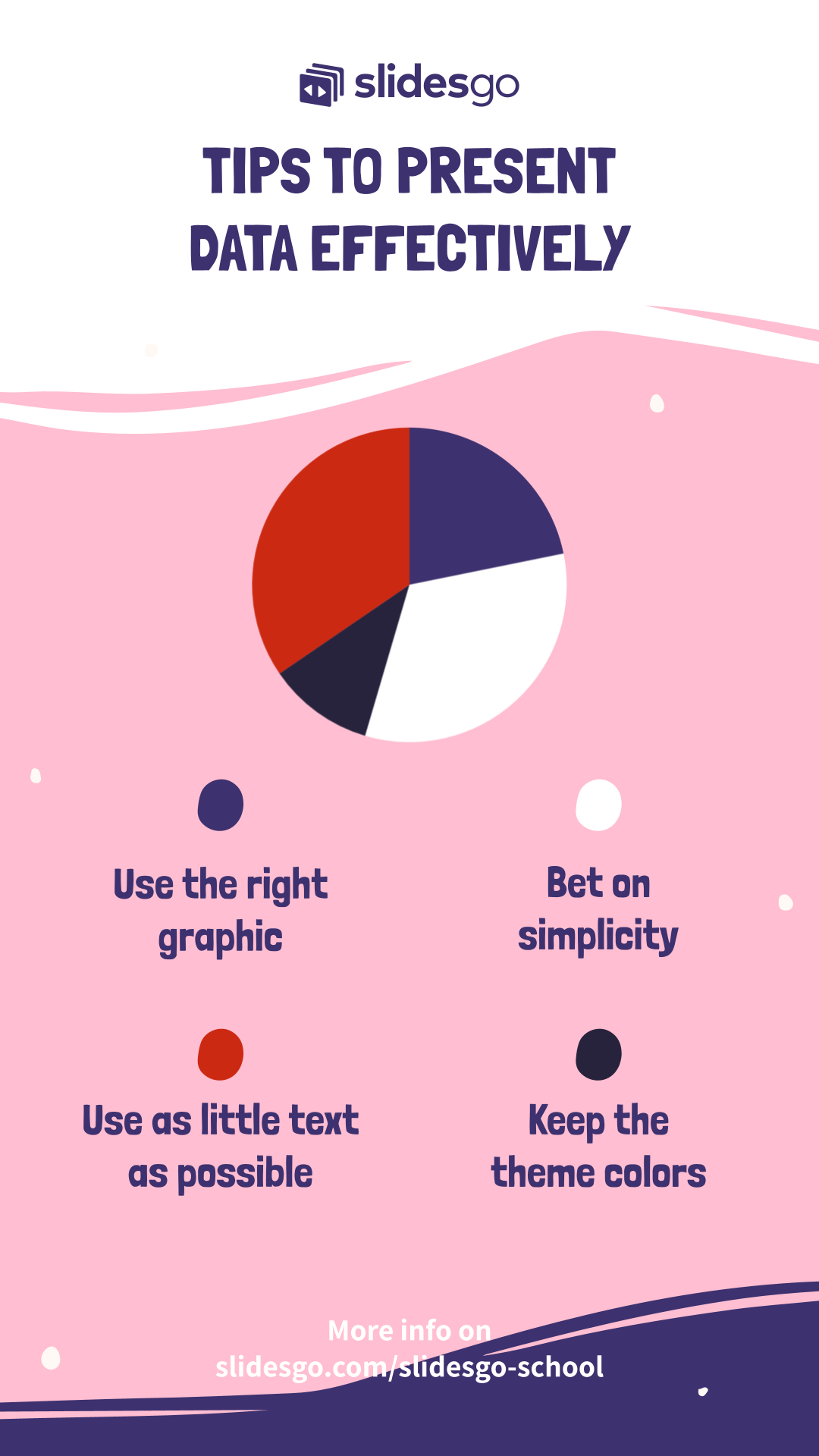
Do you find this article useful?
Related tutorials.
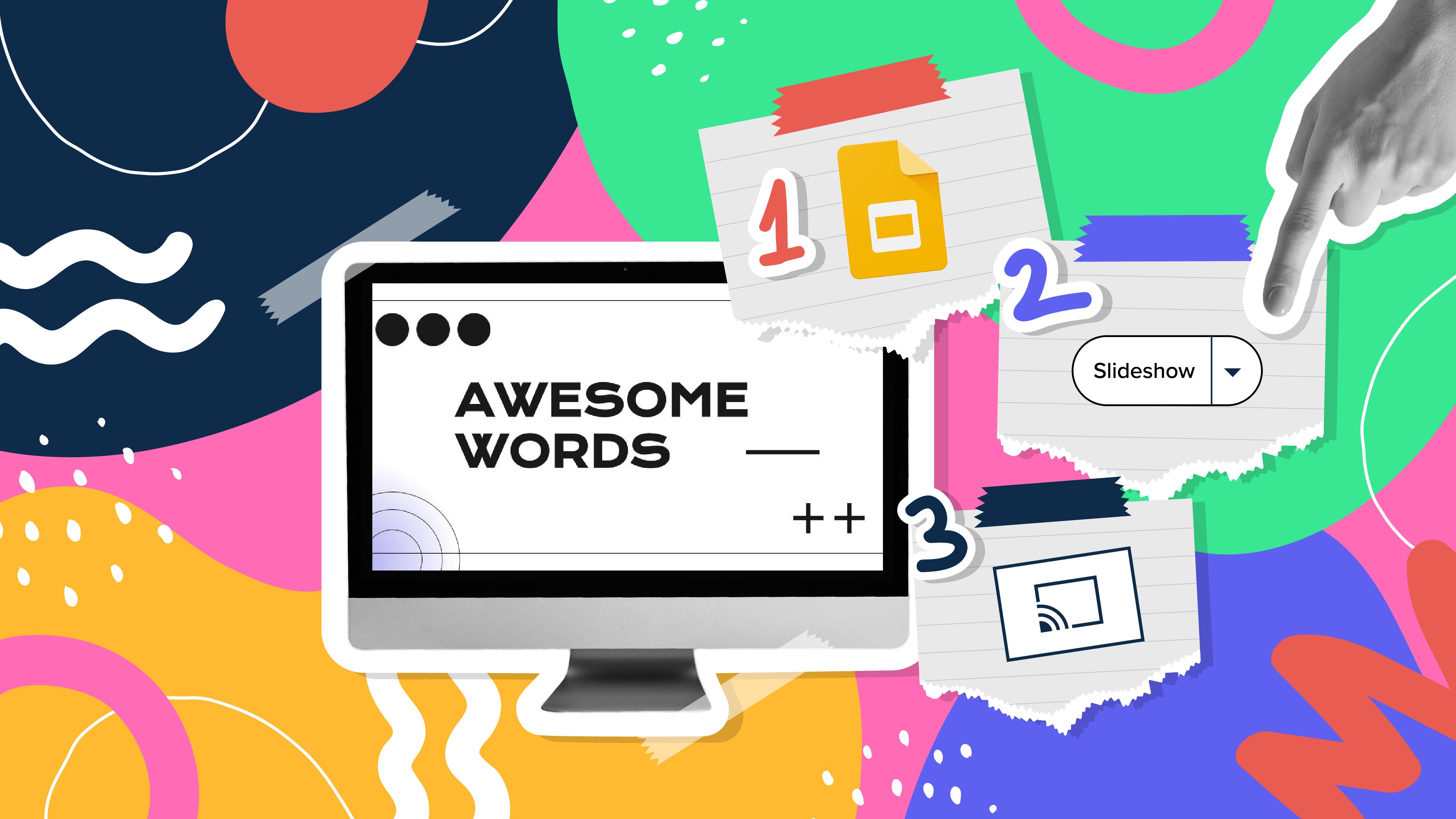
How to Use the Presenter View in Google Slides
Google Slides, like PowerPoint, has different presentation modes that can come in handy when you’re presenting and you want your slideshow to look smooth. Whether you’re looking for slides only, speaker notes or the Q&A feature, in this new Google Slides tutorial, you’ll learn about these and their respective settings. Ready? Then let’s explore the presenter view!
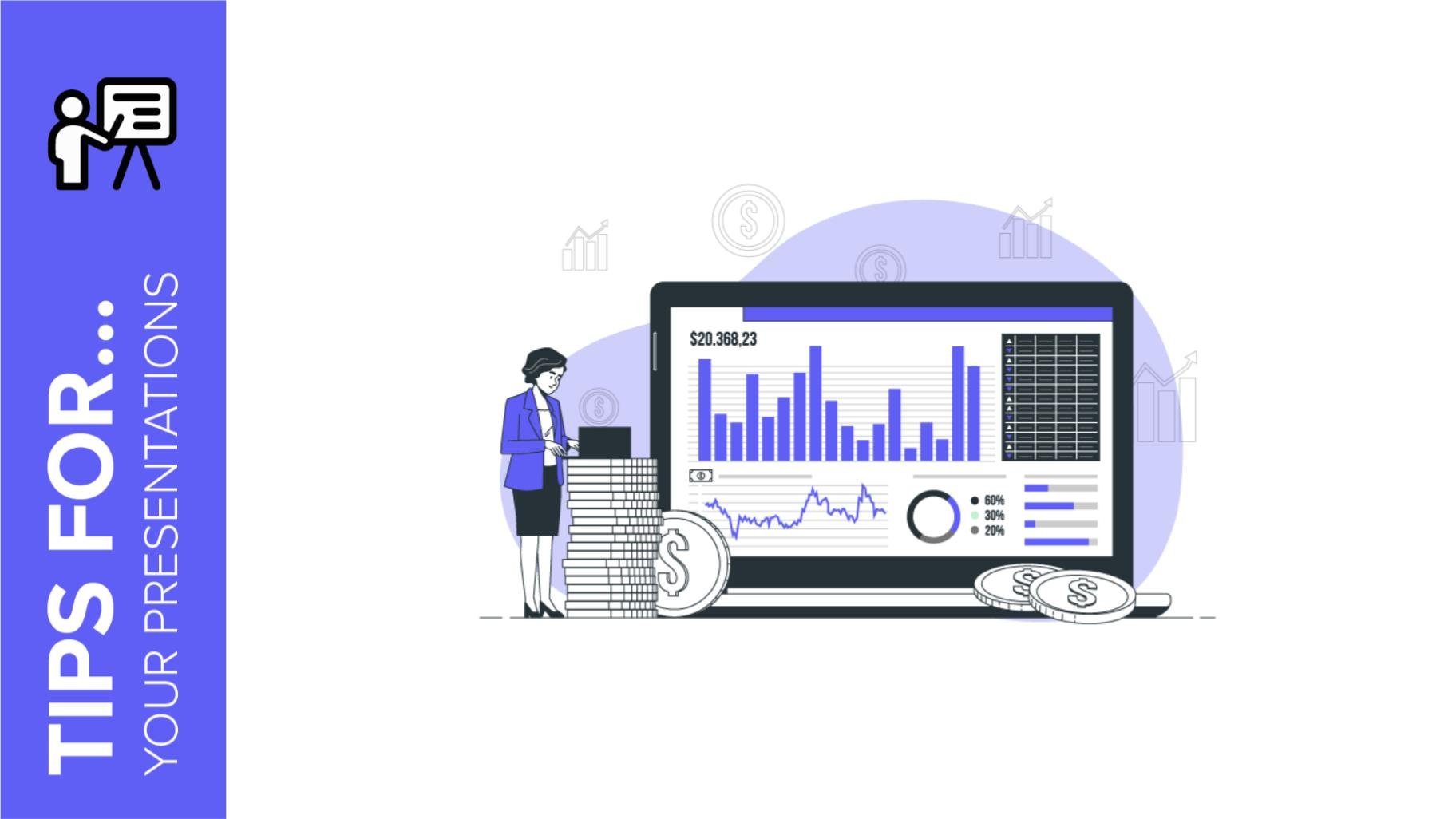
Top 10 tips and tricks for creating a business presentation!
Slidesgo is back with a new post! We want your presentations and oral expositions to never be the same again, but to go to the next level of presentations. Success comes from a combination of two main ingredients: a presentation template suitable for the topic and a correct development of the spoken part. For templates, just take a look at the Slidesgo website, where you are sure to find your ideal design. For tips and tricks on how to make a presentation, our blog contains a lot of information, for example, this post. We have focused these tips on business presentations, so that, no matter what type of company or...
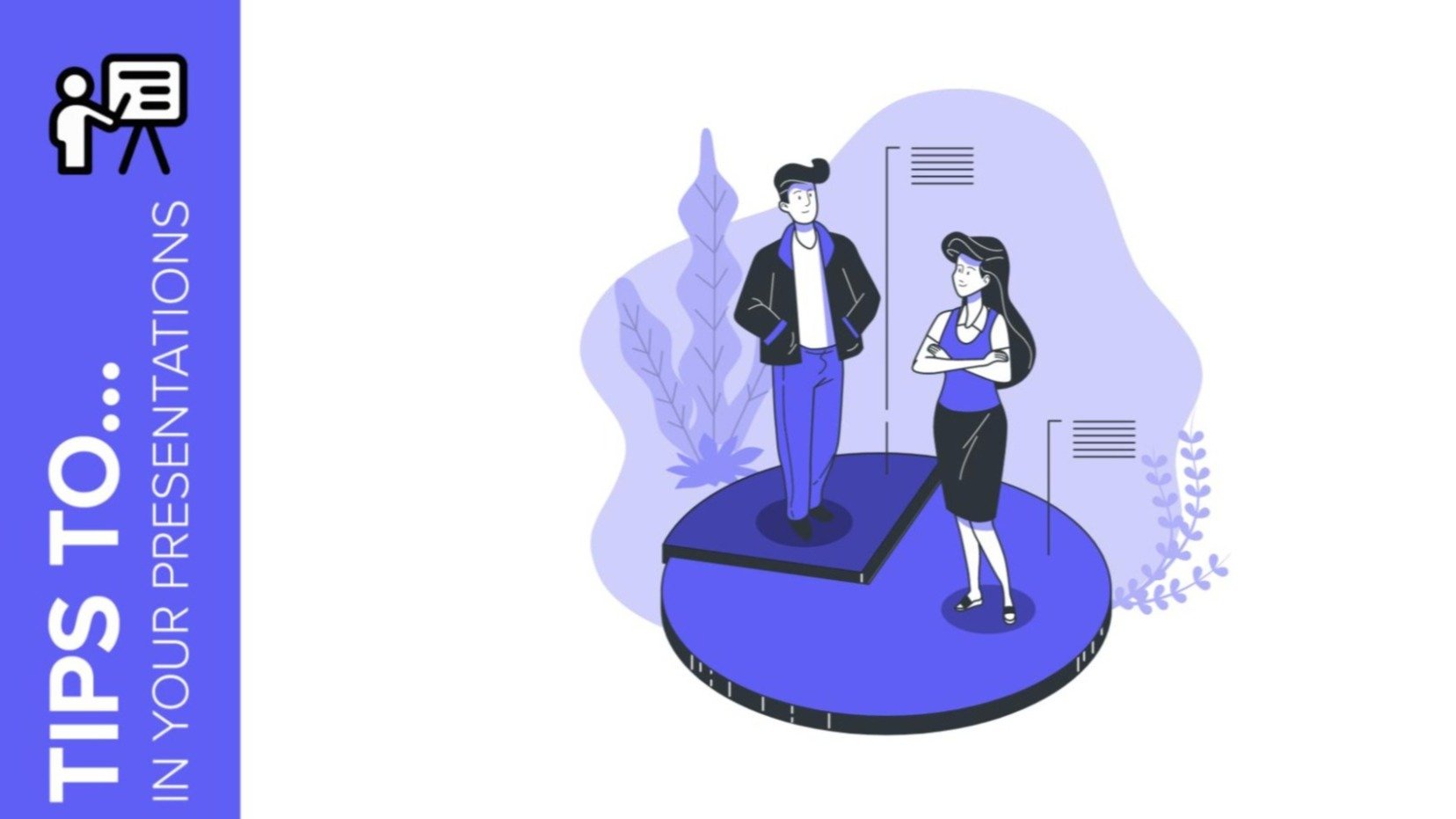
How to present survey results in PowerPoint or Google Slides
A survey is a technique that is applied by conducting a questionnaire to a significant sample of a group of people. When we carry out the survey, we start from a hypothesis and it is this survey activity that will allow us to confirm the hypothesis or to see where the problem and solution of what we are investigating lies.We know: fieldwork is hard work. Many hours collecting data, analyzing and organizing it until we have our survey results.Well, we don't want to discourage you (at Slidesgo we stand for positivism) but this is only 50% of the survey work....
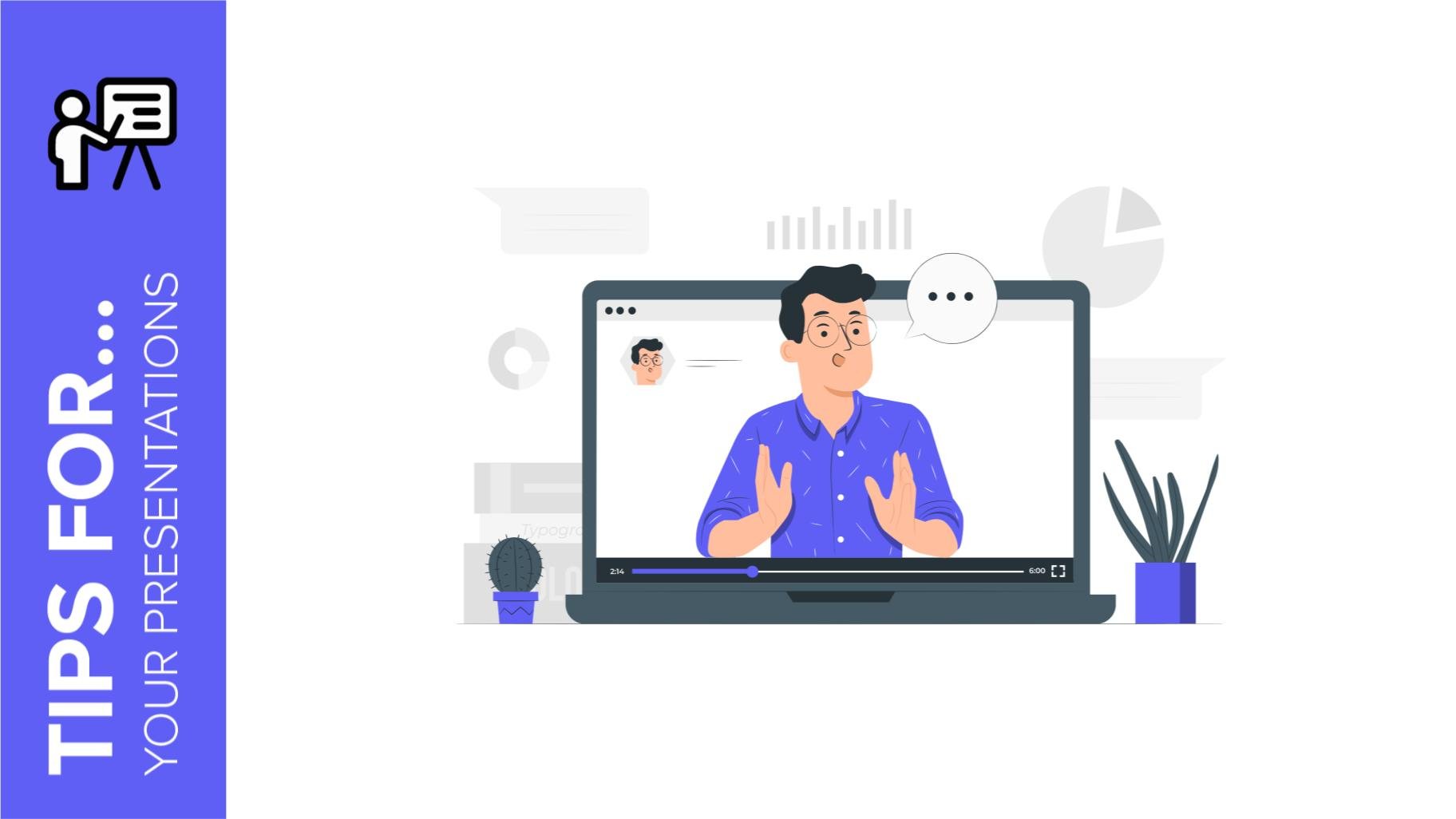
Best 10 tips for webinar presentations
During the last couple of years, the popularity of webinars has skyrocketed. Thousands of people have taken advantage of the shift to online learning and have prepared their own webinars where they have both taught and learned new skills while getting to know more people from their fields. Thanks to online resources like Google Meet and Slidesgo, now you can also prepare your own webinar. Here are 10 webinar presentation tips that will make your speech stand out!
Have a language expert improve your writing
Run a free plagiarism check in 10 minutes, automatically generate references for free.
- Knowledge Base
- Methodology
- How to Write a Strong Hypothesis | Guide & Examples
How to Write a Strong Hypothesis | Guide & Examples
Published on 6 May 2022 by Shona McCombes .
A hypothesis is a statement that can be tested by scientific research. If you want to test a relationship between two or more variables, you need to write hypotheses before you start your experiment or data collection.
Table of contents
What is a hypothesis, developing a hypothesis (with example), hypothesis examples, frequently asked questions about writing hypotheses.
A hypothesis states your predictions about what your research will find. It is a tentative answer to your research question that has not yet been tested. For some research projects, you might have to write several hypotheses that address different aspects of your research question.
A hypothesis is not just a guess – it should be based on existing theories and knowledge. It also has to be testable, which means you can support or refute it through scientific research methods (such as experiments, observations, and statistical analysis of data).
Variables in hypotheses
Hypotheses propose a relationship between two or more variables . An independent variable is something the researcher changes or controls. A dependent variable is something the researcher observes and measures.
In this example, the independent variable is exposure to the sun – the assumed cause . The dependent variable is the level of happiness – the assumed effect .
Prevent plagiarism, run a free check.
Step 1: ask a question.
Writing a hypothesis begins with a research question that you want to answer. The question should be focused, specific, and researchable within the constraints of your project.
Step 2: Do some preliminary research
Your initial answer to the question should be based on what is already known about the topic. Look for theories and previous studies to help you form educated assumptions about what your research will find.
At this stage, you might construct a conceptual framework to identify which variables you will study and what you think the relationships are between them. Sometimes, you’ll have to operationalise more complex constructs.
Step 3: Formulate your hypothesis
Now you should have some idea of what you expect to find. Write your initial answer to the question in a clear, concise sentence.
Step 4: Refine your hypothesis
You need to make sure your hypothesis is specific and testable. There are various ways of phrasing a hypothesis, but all the terms you use should have clear definitions, and the hypothesis should contain:
- The relevant variables
- The specific group being studied
- The predicted outcome of the experiment or analysis
Step 5: Phrase your hypothesis in three ways
To identify the variables, you can write a simple prediction in if … then form. The first part of the sentence states the independent variable and the second part states the dependent variable.
In academic research, hypotheses are more commonly phrased in terms of correlations or effects, where you directly state the predicted relationship between variables.
If you are comparing two groups, the hypothesis can state what difference you expect to find between them.
Step 6. Write a null hypothesis
If your research involves statistical hypothesis testing , you will also have to write a null hypothesis. The null hypothesis is the default position that there is no association between the variables. The null hypothesis is written as H 0 , while the alternative hypothesis is H 1 or H a .
Hypothesis testing is a formal procedure for investigating our ideas about the world using statistics. It is used by scientists to test specific predictions, called hypotheses , by calculating how likely it is that a pattern or relationship between variables could have arisen by chance.
A hypothesis is not just a guess. It should be based on existing theories and knowledge. It also has to be testable, which means you can support or refute it through scientific research methods (such as experiments, observations, and statistical analysis of data).
A research hypothesis is your proposed answer to your research question. The research hypothesis usually includes an explanation (‘ x affects y because …’).
A statistical hypothesis, on the other hand, is a mathematical statement about a population parameter. Statistical hypotheses always come in pairs: the null and alternative hypotheses. In a well-designed study , the statistical hypotheses correspond logically to the research hypothesis.
Cite this Scribbr article
If you want to cite this source, you can copy and paste the citation or click the ‘Cite this Scribbr article’ button to automatically add the citation to our free Reference Generator.
McCombes, S. (2022, May 06). How to Write a Strong Hypothesis | Guide & Examples. Scribbr. Retrieved 14 May 2024, from https://www.scribbr.co.uk/research-methods/hypothesis-writing/
Is this article helpful?
Shona McCombes
Other students also liked, operationalisation | a guide with examples, pros & cons, what is a conceptual framework | tips & examples, a quick guide to experimental design | 5 steps & examples.

How to Develop a Good Research Hypothesis
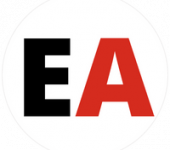
The story of a research study begins by asking a question. Researchers all around the globe are asking curious questions and formulating research hypothesis. However, whether the research study provides an effective conclusion depends on how well one develops a good research hypothesis. Research hypothesis examples could help researchers get an idea as to how to write a good research hypothesis.
This blog will help you understand what is a research hypothesis, its characteristics and, how to formulate a research hypothesis
Table of Contents
What is Hypothesis?
Hypothesis is an assumption or an idea proposed for the sake of argument so that it can be tested. It is a precise, testable statement of what the researchers predict will be outcome of the study. Hypothesis usually involves proposing a relationship between two variables: the independent variable (what the researchers change) and the dependent variable (what the research measures).
What is a Research Hypothesis?
Research hypothesis is a statement that introduces a research question and proposes an expected result. It is an integral part of the scientific method that forms the basis of scientific experiments. Therefore, you need to be careful and thorough when building your research hypothesis. A minor flaw in the construction of your hypothesis could have an adverse effect on your experiment. In research, there is a convention that the hypothesis is written in two forms, the null hypothesis, and the alternative hypothesis (called the experimental hypothesis when the method of investigation is an experiment).
Characteristics of a Good Research Hypothesis
As the hypothesis is specific, there is a testable prediction about what you expect to happen in a study. You may consider drawing hypothesis from previously published research based on the theory.
A good research hypothesis involves more effort than just a guess. In particular, your hypothesis may begin with a question that could be further explored through background research.
To help you formulate a promising research hypothesis, you should ask yourself the following questions:
- Is the language clear and focused?
- What is the relationship between your hypothesis and your research topic?
- Is your hypothesis testable? If yes, then how?
- What are the possible explanations that you might want to explore?
- Does your hypothesis include both an independent and dependent variable?
- Can you manipulate your variables without hampering the ethical standards?
- Does your research predict the relationship and outcome?
- Is your research simple and concise (avoids wordiness)?
- Is it clear with no ambiguity or assumptions about the readers’ knowledge
- Is your research observable and testable results?
- Is it relevant and specific to the research question or problem?
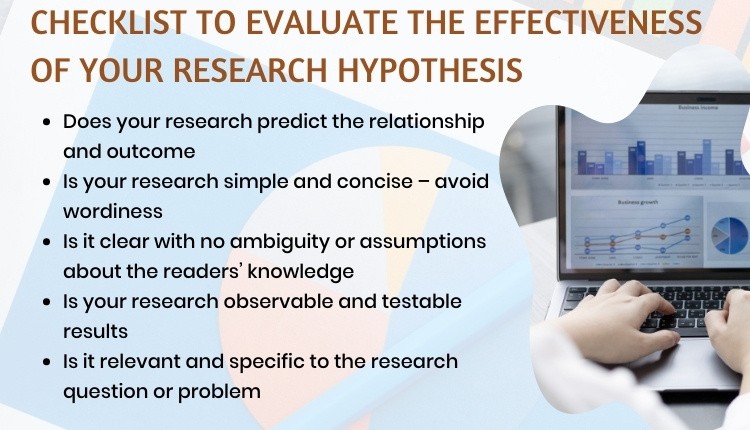
The questions listed above can be used as a checklist to make sure your hypothesis is based on a solid foundation. Furthermore, it can help you identify weaknesses in your hypothesis and revise it if necessary.
Source: Educational Hub
How to formulate a research hypothesis.
A testable hypothesis is not a simple statement. It is rather an intricate statement that needs to offer a clear introduction to a scientific experiment, its intentions, and the possible outcomes. However, there are some important things to consider when building a compelling hypothesis.
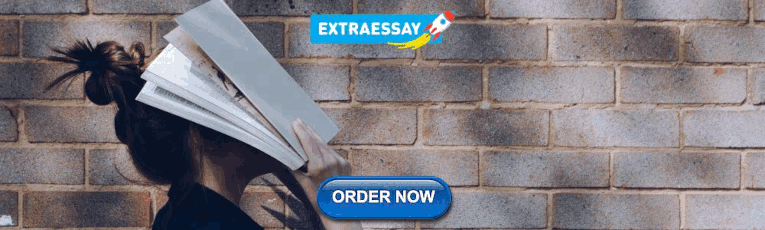
1. State the problem that you are trying to solve.
Make sure that the hypothesis clearly defines the topic and the focus of the experiment.
2. Try to write the hypothesis as an if-then statement.
Follow this template: If a specific action is taken, then a certain outcome is expected.
3. Define the variables
Independent variables are the ones that are manipulated, controlled, or changed. Independent variables are isolated from other factors of the study.
Dependent variables , as the name suggests are dependent on other factors of the study. They are influenced by the change in independent variable.
4. Scrutinize the hypothesis
Evaluate assumptions, predictions, and evidence rigorously to refine your understanding.
Types of Research Hypothesis
The types of research hypothesis are stated below:
1. Simple Hypothesis
It predicts the relationship between a single dependent variable and a single independent variable.
2. Complex Hypothesis
It predicts the relationship between two or more independent and dependent variables.
3. Directional Hypothesis
It specifies the expected direction to be followed to determine the relationship between variables and is derived from theory. Furthermore, it implies the researcher’s intellectual commitment to a particular outcome.
4. Non-directional Hypothesis
It does not predict the exact direction or nature of the relationship between the two variables. The non-directional hypothesis is used when there is no theory involved or when findings contradict previous research.
5. Associative and Causal Hypothesis
The associative hypothesis defines interdependency between variables. A change in one variable results in the change of the other variable. On the other hand, the causal hypothesis proposes an effect on the dependent due to manipulation of the independent variable.
6. Null Hypothesis
Null hypothesis states a negative statement to support the researcher’s findings that there is no relationship between two variables. There will be no changes in the dependent variable due the manipulation of the independent variable. Furthermore, it states results are due to chance and are not significant in terms of supporting the idea being investigated.
7. Alternative Hypothesis
It states that there is a relationship between the two variables of the study and that the results are significant to the research topic. An experimental hypothesis predicts what changes will take place in the dependent variable when the independent variable is manipulated. Also, it states that the results are not due to chance and that they are significant in terms of supporting the theory being investigated.
Research Hypothesis Examples of Independent and Dependent Variables
Research Hypothesis Example 1 The greater number of coal plants in a region (independent variable) increases water pollution (dependent variable). If you change the independent variable (building more coal factories), it will change the dependent variable (amount of water pollution).
Research Hypothesis Example 2 What is the effect of diet or regular soda (independent variable) on blood sugar levels (dependent variable)? If you change the independent variable (the type of soda you consume), it will change the dependent variable (blood sugar levels)
You should not ignore the importance of the above steps. The validity of your experiment and its results rely on a robust testable hypothesis. Developing a strong testable hypothesis has few advantages, it compels us to think intensely and specifically about the outcomes of a study. Consequently, it enables us to understand the implication of the question and the different variables involved in the study. Furthermore, it helps us to make precise predictions based on prior research. Hence, forming a hypothesis would be of great value to the research. Here are some good examples of testable hypotheses.
More importantly, you need to build a robust testable research hypothesis for your scientific experiments. A testable hypothesis is a hypothesis that can be proved or disproved as a result of experimentation.
Importance of a Testable Hypothesis
To devise and perform an experiment using scientific method, you need to make sure that your hypothesis is testable. To be considered testable, some essential criteria must be met:
- There must be a possibility to prove that the hypothesis is true.
- There must be a possibility to prove that the hypothesis is false.
- The results of the hypothesis must be reproducible.
Without these criteria, the hypothesis and the results will be vague. As a result, the experiment will not prove or disprove anything significant.
What are your experiences with building hypotheses for scientific experiments? What challenges did you face? How did you overcome these challenges? Please share your thoughts with us in the comments section.
Frequently Asked Questions
The steps to write a research hypothesis are: 1. Stating the problem: Ensure that the hypothesis defines the research problem 2. Writing a hypothesis as an 'if-then' statement: Include the action and the expected outcome of your study by following a ‘if-then’ structure. 3. Defining the variables: Define the variables as Dependent or Independent based on their dependency to other factors. 4. Scrutinizing the hypothesis: Identify the type of your hypothesis
Hypothesis testing is a statistical tool which is used to make inferences about a population data to draw conclusions for a particular hypothesis.
Hypothesis in statistics is a formal statement about the nature of a population within a structured framework of a statistical model. It is used to test an existing hypothesis by studying a population.
Research hypothesis is a statement that introduces a research question and proposes an expected result. It forms the basis of scientific experiments.
The different types of hypothesis in research are: • Null hypothesis: Null hypothesis is a negative statement to support the researcher’s findings that there is no relationship between two variables. • Alternate hypothesis: Alternate hypothesis predicts the relationship between the two variables of the study. • Directional hypothesis: Directional hypothesis specifies the expected direction to be followed to determine the relationship between variables. • Non-directional hypothesis: Non-directional hypothesis does not predict the exact direction or nature of the relationship between the two variables. • Simple hypothesis: Simple hypothesis predicts the relationship between a single dependent variable and a single independent variable. • Complex hypothesis: Complex hypothesis predicts the relationship between two or more independent and dependent variables. • Associative and casual hypothesis: Associative and casual hypothesis predicts the relationship between two or more independent and dependent variables. • Empirical hypothesis: Empirical hypothesis can be tested via experiments and observation. • Statistical hypothesis: A statistical hypothesis utilizes statistical models to draw conclusions about broader populations.

Wow! You really simplified your explanation that even dummies would find it easy to comprehend. Thank you so much.
Thanks a lot for your valuable guidance.
I enjoy reading the post. Hypotheses are actually an intrinsic part in a study. It bridges the research question and the methodology of the study.
Useful piece!
This is awesome.Wow.
It very interesting to read the topic, can you guide me any specific example of hypothesis process establish throw the Demand and supply of the specific product in market
Nicely explained
It is really a useful for me Kindly give some examples of hypothesis
It was a well explained content ,can you please give me an example with the null and alternative hypothesis illustrated
clear and concise. thanks.
So Good so Amazing
Good to learn
Thanks a lot for explaining to my level of understanding
Explained well and in simple terms. Quick read! Thank you
It awesome. It has really positioned me in my research project
Rate this article Cancel Reply
Your email address will not be published.
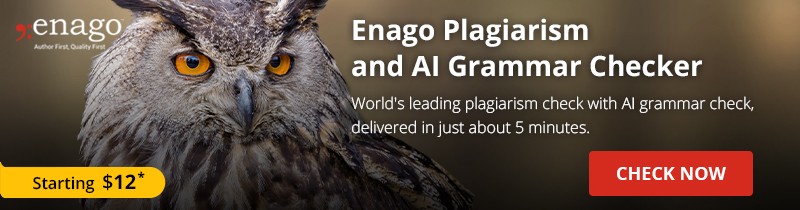
Enago Academy's Most Popular Articles
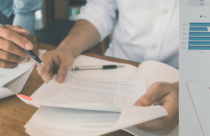
- Reporting Research
Choosing the Right Analytical Approach: Thematic analysis vs. content analysis for data interpretation
In research, choosing the right approach to understand data is crucial for deriving meaningful insights.…
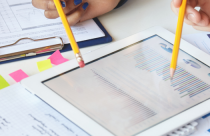
Comparing Cross Sectional and Longitudinal Studies: 5 steps for choosing the right approach
The process of choosing the right research design can put ourselves at the crossroads of…
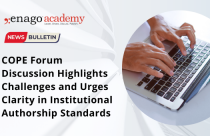
- Industry News
COPE Forum Discussion Highlights Challenges and Urges Clarity in Institutional Authorship Standards
The COPE forum discussion held in December 2023 initiated with a fundamental question — is…
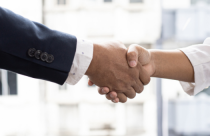
- Career Corner
Unlocking the Power of Networking in Academic Conferences
Embarking on your first academic conference experience? Fear not, we got you covered! Academic conferences…
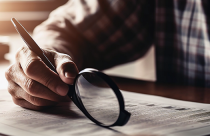
Research Recommendations – Guiding policy-makers for evidence-based decision making
Research recommendations play a crucial role in guiding scholars and researchers toward fruitful avenues of…
Choosing the Right Analytical Approach: Thematic analysis vs. content analysis for…
Comparing Cross Sectional and Longitudinal Studies: 5 steps for choosing the right…
How to Design Effective Research Questionnaires for Robust Findings

Sign-up to read more
Subscribe for free to get unrestricted access to all our resources on research writing and academic publishing including:
- 2000+ blog articles
- 50+ Webinars
- 10+ Expert podcasts
- 50+ Infographics
- 10+ Checklists
- Research Guides
We hate spam too. We promise to protect your privacy and never spam you.
I am looking for Editing/ Proofreading services for my manuscript Tentative date of next journal submission:
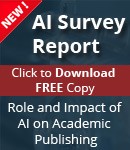
As a researcher, what do you consider most when choosing an image manipulation detector?
- Bipolar Disorder
- Therapy Center
- When To See a Therapist
- Types of Therapy
- Best Online Therapy
- Best Couples Therapy
- Best Family Therapy
- Managing Stress
- Sleep and Dreaming
- Understanding Emotions
- Self-Improvement
- Healthy Relationships
- Student Resources
- Personality Types
- Guided Meditations
- Verywell Mind Insights
- 2024 Verywell Mind 25
- Mental Health in the Classroom
- Editorial Process
- Meet Our Review Board
- Crisis Support
How to Write a Great Hypothesis
Hypothesis Definition, Format, Examples, and Tips
Kendra Cherry, MS, is a psychosocial rehabilitation specialist, psychology educator, and author of the "Everything Psychology Book."
:max_bytes(150000):strip_icc():format(webp)/IMG_9791-89504ab694d54b66bbd72cb84ffb860e.jpg)
Amy Morin, LCSW, is a psychotherapist and international bestselling author. Her books, including "13 Things Mentally Strong People Don't Do," have been translated into more than 40 languages. Her TEDx talk, "The Secret of Becoming Mentally Strong," is one of the most viewed talks of all time.
:max_bytes(150000):strip_icc():format(webp)/VW-MIND-Amy-2b338105f1ee493f94d7e333e410fa76.jpg)
Verywell / Alex Dos Diaz
- The Scientific Method
Hypothesis Format
Falsifiability of a hypothesis.
- Operationalization
Hypothesis Types
Hypotheses examples.
- Collecting Data
A hypothesis is a tentative statement about the relationship between two or more variables. It is a specific, testable prediction about what you expect to happen in a study. It is a preliminary answer to your question that helps guide the research process.
Consider a study designed to examine the relationship between sleep deprivation and test performance. The hypothesis might be: "This study is designed to assess the hypothesis that sleep-deprived people will perform worse on a test than individuals who are not sleep-deprived."
At a Glance
A hypothesis is crucial to scientific research because it offers a clear direction for what the researchers are looking to find. This allows them to design experiments to test their predictions and add to our scientific knowledge about the world. This article explores how a hypothesis is used in psychology research, how to write a good hypothesis, and the different types of hypotheses you might use.
The Hypothesis in the Scientific Method
In the scientific method , whether it involves research in psychology, biology, or some other area, a hypothesis represents what the researchers think will happen in an experiment. The scientific method involves the following steps:
- Forming a question
- Performing background research
- Creating a hypothesis
- Designing an experiment
- Collecting data
- Analyzing the results
- Drawing conclusions
- Communicating the results
The hypothesis is a prediction, but it involves more than a guess. Most of the time, the hypothesis begins with a question which is then explored through background research. At this point, researchers then begin to develop a testable hypothesis.
Unless you are creating an exploratory study, your hypothesis should always explain what you expect to happen.
In a study exploring the effects of a particular drug, the hypothesis might be that researchers expect the drug to have some type of effect on the symptoms of a specific illness. In psychology, the hypothesis might focus on how a certain aspect of the environment might influence a particular behavior.
Remember, a hypothesis does not have to be correct. While the hypothesis predicts what the researchers expect to see, the goal of the research is to determine whether this guess is right or wrong. When conducting an experiment, researchers might explore numerous factors to determine which ones might contribute to the ultimate outcome.
In many cases, researchers may find that the results of an experiment do not support the original hypothesis. When writing up these results, the researchers might suggest other options that should be explored in future studies.
In many cases, researchers might draw a hypothesis from a specific theory or build on previous research. For example, prior research has shown that stress can impact the immune system. So a researcher might hypothesize: "People with high-stress levels will be more likely to contract a common cold after being exposed to the virus than people who have low-stress levels."
In other instances, researchers might look at commonly held beliefs or folk wisdom. "Birds of a feather flock together" is one example of folk adage that a psychologist might try to investigate. The researcher might pose a specific hypothesis that "People tend to select romantic partners who are similar to them in interests and educational level."
Elements of a Good Hypothesis
So how do you write a good hypothesis? When trying to come up with a hypothesis for your research or experiments, ask yourself the following questions:
- Is your hypothesis based on your research on a topic?
- Can your hypothesis be tested?
- Does your hypothesis include independent and dependent variables?
Before you come up with a specific hypothesis, spend some time doing background research. Once you have completed a literature review, start thinking about potential questions you still have. Pay attention to the discussion section in the journal articles you read . Many authors will suggest questions that still need to be explored.
How to Formulate a Good Hypothesis
To form a hypothesis, you should take these steps:
- Collect as many observations about a topic or problem as you can.
- Evaluate these observations and look for possible causes of the problem.
- Create a list of possible explanations that you might want to explore.
- After you have developed some possible hypotheses, think of ways that you could confirm or disprove each hypothesis through experimentation. This is known as falsifiability.
In the scientific method , falsifiability is an important part of any valid hypothesis. In order to test a claim scientifically, it must be possible that the claim could be proven false.
Students sometimes confuse the idea of falsifiability with the idea that it means that something is false, which is not the case. What falsifiability means is that if something was false, then it is possible to demonstrate that it is false.
One of the hallmarks of pseudoscience is that it makes claims that cannot be refuted or proven false.
The Importance of Operational Definitions
A variable is a factor or element that can be changed and manipulated in ways that are observable and measurable. However, the researcher must also define how the variable will be manipulated and measured in the study.
Operational definitions are specific definitions for all relevant factors in a study. This process helps make vague or ambiguous concepts detailed and measurable.
For example, a researcher might operationally define the variable " test anxiety " as the results of a self-report measure of anxiety experienced during an exam. A "study habits" variable might be defined by the amount of studying that actually occurs as measured by time.
These precise descriptions are important because many things can be measured in various ways. Clearly defining these variables and how they are measured helps ensure that other researchers can replicate your results.
Replicability
One of the basic principles of any type of scientific research is that the results must be replicable.
Replication means repeating an experiment in the same way to produce the same results. By clearly detailing the specifics of how the variables were measured and manipulated, other researchers can better understand the results and repeat the study if needed.
Some variables are more difficult than others to define. For example, how would you operationally define a variable such as aggression ? For obvious ethical reasons, researchers cannot create a situation in which a person behaves aggressively toward others.
To measure this variable, the researcher must devise a measurement that assesses aggressive behavior without harming others. The researcher might utilize a simulated task to measure aggressiveness in this situation.
Hypothesis Checklist
- Does your hypothesis focus on something that you can actually test?
- Does your hypothesis include both an independent and dependent variable?
- Can you manipulate the variables?
- Can your hypothesis be tested without violating ethical standards?
The hypothesis you use will depend on what you are investigating and hoping to find. Some of the main types of hypotheses that you might use include:
- Simple hypothesis : This type of hypothesis suggests there is a relationship between one independent variable and one dependent variable.
- Complex hypothesis : This type suggests a relationship between three or more variables, such as two independent and dependent variables.
- Null hypothesis : This hypothesis suggests no relationship exists between two or more variables.
- Alternative hypothesis : This hypothesis states the opposite of the null hypothesis.
- Statistical hypothesis : This hypothesis uses statistical analysis to evaluate a representative population sample and then generalizes the findings to the larger group.
- Logical hypothesis : This hypothesis assumes a relationship between variables without collecting data or evidence.
A hypothesis often follows a basic format of "If {this happens} then {this will happen}." One way to structure your hypothesis is to describe what will happen to the dependent variable if you change the independent variable .
The basic format might be: "If {these changes are made to a certain independent variable}, then we will observe {a change in a specific dependent variable}."
A few examples of simple hypotheses:
- "Students who eat breakfast will perform better on a math exam than students who do not eat breakfast."
- "Students who experience test anxiety before an English exam will get lower scores than students who do not experience test anxiety."
- "Motorists who talk on the phone while driving will be more likely to make errors on a driving course than those who do not talk on the phone."
- "Children who receive a new reading intervention will have higher reading scores than students who do not receive the intervention."
Examples of a complex hypothesis include:
- "People with high-sugar diets and sedentary activity levels are more likely to develop depression."
- "Younger people who are regularly exposed to green, outdoor areas have better subjective well-being than older adults who have limited exposure to green spaces."
Examples of a null hypothesis include:
- "There is no difference in anxiety levels between people who take St. John's wort supplements and those who do not."
- "There is no difference in scores on a memory recall task between children and adults."
- "There is no difference in aggression levels between children who play first-person shooter games and those who do not."
Examples of an alternative hypothesis:
- "People who take St. John's wort supplements will have less anxiety than those who do not."
- "Adults will perform better on a memory task than children."
- "Children who play first-person shooter games will show higher levels of aggression than children who do not."
Collecting Data on Your Hypothesis
Once a researcher has formed a testable hypothesis, the next step is to select a research design and start collecting data. The research method depends largely on exactly what they are studying. There are two basic types of research methods: descriptive research and experimental research.
Descriptive Research Methods
Descriptive research such as case studies , naturalistic observations , and surveys are often used when conducting an experiment is difficult or impossible. These methods are best used to describe different aspects of a behavior or psychological phenomenon.
Once a researcher has collected data using descriptive methods, a correlational study can examine how the variables are related. This research method might be used to investigate a hypothesis that is difficult to test experimentally.
Experimental Research Methods
Experimental methods are used to demonstrate causal relationships between variables. In an experiment, the researcher systematically manipulates a variable of interest (known as the independent variable) and measures the effect on another variable (known as the dependent variable).
Unlike correlational studies, which can only be used to determine if there is a relationship between two variables, experimental methods can be used to determine the actual nature of the relationship—whether changes in one variable actually cause another to change.
The hypothesis is a critical part of any scientific exploration. It represents what researchers expect to find in a study or experiment. In situations where the hypothesis is unsupported by the research, the research still has value. Such research helps us better understand how different aspects of the natural world relate to one another. It also helps us develop new hypotheses that can then be tested in the future.
Thompson WH, Skau S. On the scope of scientific hypotheses . R Soc Open Sci . 2023;10(8):230607. doi:10.1098/rsos.230607
Taran S, Adhikari NKJ, Fan E. Falsifiability in medicine: what clinicians can learn from Karl Popper [published correction appears in Intensive Care Med. 2021 Jun 17;:]. Intensive Care Med . 2021;47(9):1054-1056. doi:10.1007/s00134-021-06432-z
Eyler AA. Research Methods for Public Health . 1st ed. Springer Publishing Company; 2020. doi:10.1891/9780826182067.0004
Nosek BA, Errington TM. What is replication ? PLoS Biol . 2020;18(3):e3000691. doi:10.1371/journal.pbio.3000691
Aggarwal R, Ranganathan P. Study designs: Part 2 - Descriptive studies . Perspect Clin Res . 2019;10(1):34-36. doi:10.4103/picr.PICR_154_18
Nevid J. Psychology: Concepts and Applications. Wadworth, 2013.
By Kendra Cherry, MSEd Kendra Cherry, MS, is a psychosocial rehabilitation specialist, psychology educator, and author of the "Everything Psychology Book."
6.4.3.Weekly challenge 4 - sj50179/Google-Data-Analytics-Professional-Certificate GitHub Wiki
Weekly challenge 4.
LATEST SUBMISSION GRADE 100%
A data analyst gives a presentation about predicting upcoming investment opportunities. How does establishing a hypothesis help the audience understand their predictions?
- It summarizes the findings succinctly
- It provides context about the presentation’s purpose
- It visualizes the data clearly and concisely
- It describes the data thoroughly
Correct. Establishing a hypothesis provides the audience with context about the analyst’s presentation. In this scenario, it establishes what the analyst wants to prove or disprove about which investment opportunities are most promising.
What is the final step and "so what?" moment when presenting a data visualization with the McCandless Method?
- Answer obvious questions before they’re asked
- Call out data to support that insight
- Describe why the graphic matters
- State the insight of the graphic
Correct. The most complex part of the McCandless Method is telling the audience why your graphic matters. When presenting to stakeholders, this is when you discuss the clear actions they can take and the impact of your findings.
An analyst introduces a graph to their audience to explain an analysis they performed. Which strategy would allow the audience to absorb the data visualizations? Select all that apply.
- Practicing breathing exercises
- Improving body language
- Using the five-second rule
- Starting with broad ideas
Correct. When introducing a data visualization, an analyst can use the five-second rule to allow their audience to absorb the data visualizations presented. They can also start with broad ideas to simplify the explanation about the visualization’s purpose.
You are preparing to present in front of a large audience. Which of the following is a best practice for speaking to an audience?
- Speak at a relaxed pace in short sentences
- Take as few pauses as possible
- Take long pauses between sentences
- Speak as quickly as possible
Correct. Speaking at a relaxed pace in short sentences is a best practice for a presentation. A large audience will have an easier time following your points if you use public speaking best practices.
You are running a colleague test with your coworkers. One coworker points out that she doesn’t understand one of your graphs. What can you do to prepare for presenting to your stakeholders? Select all that apply.
- Redesign the graph
- Move the graph to a later slide
- Remove the graph
- Elaborate on the data from the graph
Correct. If you receive a concern from a colleague about one of your data visualizations, you can redesign it or elaborate on the data it displays. By running a Colleague Test, you can catch and fix issues with your presentation before getting in front of your audience.
Your stakeholders express concern that the results of your analysis are very different from the predictions they made last year. Which kind of objection are they making?
- Presentation skills
Correct. When a stakeholder is concerned about the results of your analysis, they are making an objection about your findings. This is when someone objects to problems or inconsistencies in your final results.
You are on a team of analysts presenting to your stakeholders. Your teammate responds to an objection about your steps of analysis by repeating the steps and then getting defensive when the stakeholders don’t seem to understand. What could they have done to respond to the objection more appropriately? Select all that apply.
- Promise to investigate your analysis question further
- Remind the stakeholders of your successes
- Acknowledge that the objection is valid
- Describe the approach you took in your analysis
Correct. Instead of getting defensive, your coworker should have acknowledged that the objection was valid. Simply repeating the steps you took may not be helpful. By describing your approach and promising to investigate the matter further, your coworker can respond to an objection appropriately and reassure the stakeholders.
You notice that your audience is not as engaged as you’d like during your Q&A. Which of the following are ways to get them more involved?
- Ask them for insights
- Repeat your key findings
- Wait longer for the audience to ask questions
- Keep your pitch level
Correct. One way to engage your audience is to ask them if they know anything about the topic you’re presenting about. You can enrich the discussion if they do and would like to share their insights.
You are giving a presentation to an audience that knows very little about your subject. Which concept in the McCandless Method offers you the opportunity to avoid people getting distracted by something they don't understand?
- Tell the audience why the graphic matters
- Introduce the graphic by name
Correct. According to the McCandless Method, the earliest opportunity to provide context to your audience is when you answer obvious questions before they’re asked.
Fill in the blank: You are working as a data analyst at a gas company and want to learn more about top-performing staff members. You create a _____, which states that employee success hinges on a successful onboarding process when people are first hired. You hope to either prove or disprove this theory with your data.
- business task
Correct. You create a hypothesis. A hypothesis is the theory you try to prove or disprove with data.
You are introducing a data visualization during your presentation and are concerned that it may overwhelm your audience. How can you help your audience when you first introduce it?
- Wait five seconds
- Define each parameter
- Thoroughly explain the context
- Describe each graph quickly
Correct. It’s helpful to wait five seconds after you first introduce a data visualization. This gives your audience time to process your data before you discuss it, reducing the chance of overwhelming them.
You are preparing for a presentation and want to make sure your nerves don’t distract you from your presentation. Which practices can help you stay focused on an audience? Select all that apply.
- Keep the pitch of your voice level
- Speak as quickly and briefly as possible
- Be mindful of nervous habits
- Use short sentences
Correct. Some helpful ways to focus on an audience include being mindful of nervous habits, using short sentences, and keeping an even pitch. By using these strategies, you can reduce the risk of getting distracted during your presentation.
You are presenting to your stakeholders and want to convey confidence. How should your body language reflect your composure? Select all that apply.
- Pace as you speak to the audience
- Gesture enthusiastically to illustrate each point
- Stand up straight and be still
- Make eye contact with audience members
Correct. If you want to seem confident to your stakeholders, try to keep your body language poised while presenting. Standing straight, keeping still, and making eye contact will also help you avoid distracting your audience.
You run a colleague test on your presentation before getting in front of an audience. Your coworker asks a question about a section of your analysis, but addressing their concern would mean adding information you didn’t plan to include. How should you proceed with building your presentation?
- Remove the section of the analysis that prompted the question
- Leave the presentation as-is
- Keep the concern in mind and anticipate that stakeholders may ask the same question
- Expand your presentation by including the information
Correct. In this scenario, adding the information can help elaborate on important information. If your colleague has a question about your presentation, it is likely that your audience will too. Addressing concerns brought up during a Colleague Test can help you improve your presentation in ways you might not have anticipated.
Your stakeholders are concerned about the source of your data. They are unfamiliar with the organization that ran the analyses you referenced in your presentation. Which kind of objection are they making?
Correct. When a stakeholder is concerned about the source of your data, they are making an objection about your data. This is when someone objects to the source or relevance of the data you use.
A stakeholder objects to the steps of your analysis. What are some appropriate ways to respond to this objection? Select all that apply.
- Explain why you think any discrepancies exist
- Defend the results of your analysis
- Take steps to investigate your analysis question further
- Communicate the assumptions you made in your analysis
Correct. When responding to a concerned or objecting stakeholder, you can communicate the assumptions you made to clarify if they are accurate. You can also explain why you think the discrepancies exist and promise to investigate the matter further.
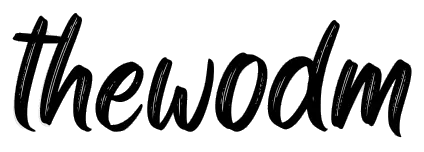
Week 4 – Developing presentations and slideshows – Shuffle Q/A 1
- Post author: Devendra Kumar
- Post published:
- Post comments: 0 Comments
11. An analyst introduces a graph to their audience to explain an analysis they performed. Which strategy would allow the audience to absorb the data visualizations? Select all that apply.
- Practicing breathing exercises
- Improving body language
- Using the five second rule
- Starting with broad ideas
12. During a presentation, one of your stakeholders expresses concern that you did not control for differences in the data. Which kind of objection are they making?
- Presentation Skills
13. During a meeting, a colleague on your team points out a flaw in your analysis that you had not noticed before. What steps should you take to respond to their objection? Select all that apply.
- Hide evidence that you were incorrect
- Follow up with your colleague
- Investigate the issue
- Acknowledge that their objection is valid
14. You are presenting to a large audience and want to keep everyone engaged during your Q&A. What can you do to ensure your audience doesn’t grow disinterested despite its size?
- Ask your audience for insights
- Wait longer for the audience to ask questions
- Repeat your key findings
- Keep your pitch level
15. Which of the following statements is true about using a hypothesis in your data presentation?
- Include the hypothesis in a summary at the end of your presentation
- Choose a hypothesis your audience will like
- Include a new hypothesis before every data visualization
- Present the hypothesis early in your presentation
16. Why is it important to state the insights from your graphic when using the McCandless method?
- To get everyone on the same page before you give supporting details
- To make sure your audience understands why the data matters
- To ensure that you establish credibility as a serious data analyst
- To add a strong finish to your presentation
17. A researcher is presenting the data for their study. What can they do to ensure their presentation is impactful?
- Ensure their delivery is as well executed as their analysis
- Suppress their excitement to remain passive and neutral
- Start with really narrow ideas and works towards broad ideas
- Focus on the data instead of focusing on presentation skills
18. You run a colleague test on your presentation before getting in front of an audience. Your coworker asks a question about a section of your analysis, but addressing their concern would mean adding information you didn’t plan to include. How should you proceed with building your presentation? Select all that apply.
- Leave the presentation as-is
- Keep the concern in mind and anticipate that stakeholders may ask the same question
- Remove the section of the analysis that prompted the question
- Expand your presentation by including the information
Shuffle Q/A 2
19. one of your stakeholders tried to reproduce the work you presented by using a copy of your scripts and was unable to get the same results. which kind of objection are they making.
- Presentation skills
20. One of your co-workers is giving a presentation on the results of an analysis the two of you have been working on. Someone in the audience points out that the data system you used has frequent errors. How should you deal with this comment?
- Assume you were given valid data
- Tell them they should have looked at the appendix
- Explain how you cleaned and formatted the data
- Ignore the question and move on
Please Share This Share this content
- Opens in a new window
Devendra Kumar
You might also like, week 3 – managing budgeting and procurement – shuffle q/a 2, week 5 – documentation and reports – shuffle q/a 2, leave a reply cancel reply.
Save my name, email, and website in this browser for the next time I comment.


- SUGGESTED TOPICS
- The Magazine
- Newsletters
- Managing Yourself
- Managing Teams
- Work-life Balance
- The Big Idea
- Data & Visuals
- Reading Lists
- Case Selections
- HBR Learning
- Topic Feeds
- Account Settings
- Email Preferences
How to Present to an Audience That Knows More Than You
- Deborah Grayson Riegel

Lean into being a facilitator — not an expert.
What happens when you have to give a presentation to an audience that might have some professionals who have more expertise on the topic than you do? While it can be intimidating, it can also be an opportunity to leverage their deep and diverse expertise in service of the group’s learning. And it’s an opportunity to exercise some intellectual humility, which includes having respect for other viewpoints, not being intellectually overconfident, separating your ego from your intellect, and being willing to revise your own viewpoint — especially in the face of new information. This article offers several tips for how you might approach a roomful of experts, including how to invite them into the discussion without allowing them to completely take over, as well as how to pivot on the proposed topic when necessary.
I was five years into my executive coaching practice when I was invited to lead a workshop on “Coaching Skills for Human Resource Leaders” at a global conference. As the room filled up with participants, I identified a few colleagues who had already been coaching professionally for more than a decade. I felt self-doubt start to kick in: Why were they even here? What did they come to learn? Why do they want to hear from me?

- Deborah Grayson Riegel is a professional speaker and facilitator, as well as a communication and presentation skills coach. She teaches leadership communication at Duke University’s Fuqua School of Business and has taught for Wharton Business School, Columbia Business School’s Women in Leadership Program, and Peking University’s International MBA Program. She is the author of Overcoming Overthinking: 36 Ways to Tame Anxiety for Work, School, and Life and the best-selling Go To Help: 31 Strategies to Offer, Ask for, and Accept Help .
Partner Center
Join our free digital event: Marketing Workshop: Create Impact with Stunning Presentations with Adobe Express
Reserve your spot today by completing this form.
May 30, 2024 | Time: 1pm (PT) / 4pm (ET) Get hands-on training with Adobe Express to uplevel your production skills and learn to generate ideas for digital assets, social campaigns, and more in this monthly marketing workshop series. Effective data visualization does a lot more than relay information — it also tells helpful stories that contextualize ideas, concepts, and numbers. With Adobe Express, marketers can easily build beautiful and compelling data narratives. Join this hands-on workshop to learn how to use Adobe Express to:
- Create stunning presentations and data visualizations using easy-to-master tools and templates.
- Incorporate branded elements, such as colors, logos, and imagery, to assemble cohesive data narratives.
- Build stunning visuals to express data in easy-to-understand ways for presentations, reports, newsletters, white papers, and more.
- Participate in a fun and interactive challenge to receive a $10 gift card.
Demo Artist
Jamie Nuzbach, Strategic Development Manager, Adobe
Technical Level
Beginner to Intermediate
Language Navigation

A .gov website belongs to an official government organization in the United States.
A lock ( ) or https:// means you've safely connected to the .gov website. Share sensitive information only on official, secure websites.
- Contact Information
- Guidelines for Examining Unusual Patterns of Cancer and Environmental Concerns
- Resources and Tools
Phased Approach to Respond to Community Inquiries: Phase 3
- Conduct a feasibility assessment to determine whether an epidemiologic study would provide meaningful answers.
- Establish a community advisory committee (CAC) to create a link back to the community for valuable information.
- Consider different aspects to include in the study design and protocol development.
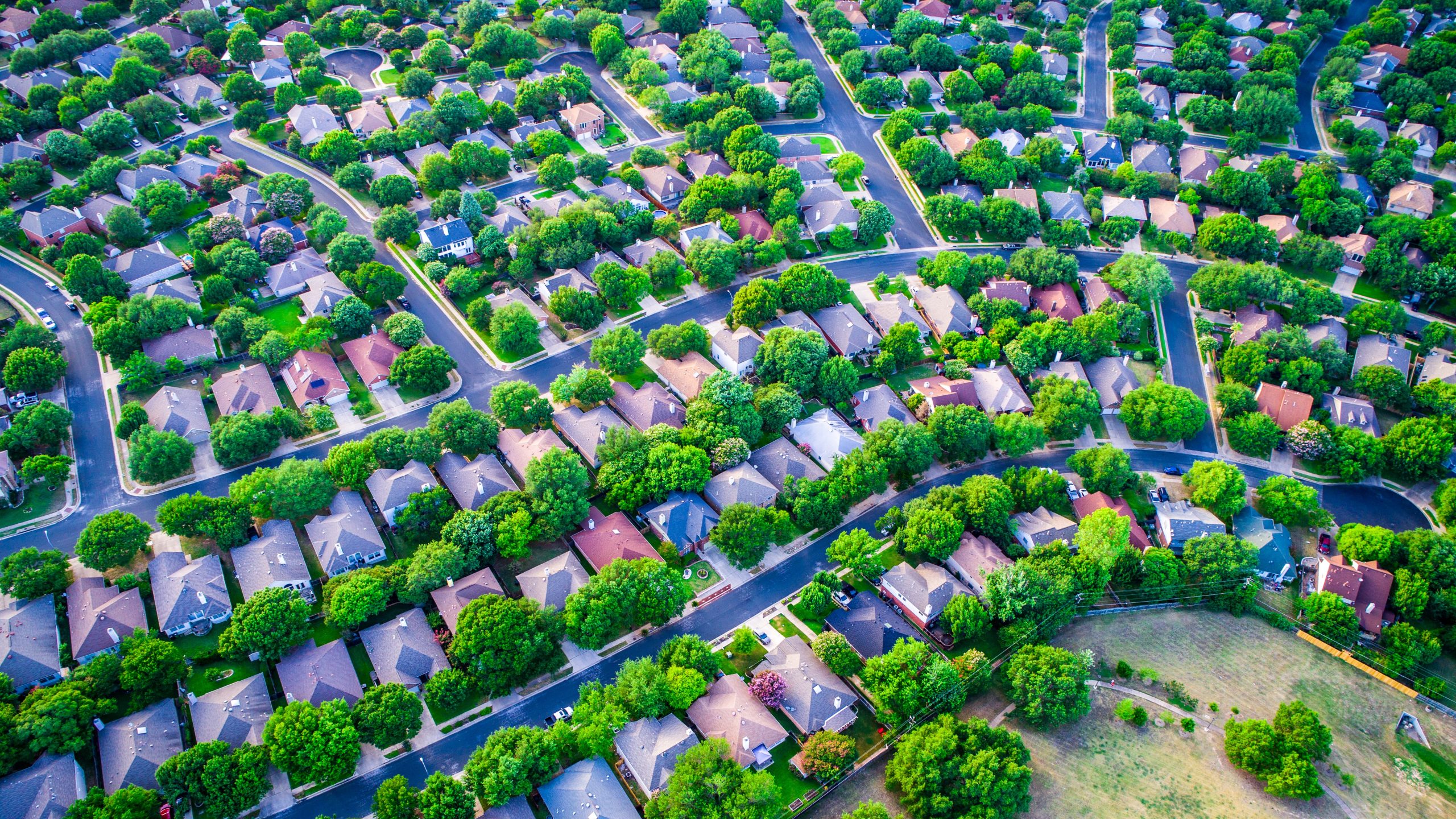
Phase 3: Considerations for epidemiologic studies
Feasibility assessment.
The feasibility of designing and conducting an epidemiologic study is dependent upon a variety of factors. For that reason, a feasibility assessment should be conducted to determine whether it is practical to conduct a study that would provide meaningful answers. The feasibility assessment should address multiple issues, including, but not limited to, data availability, adequate sample size to detect meaningful differences or associations, staff capacity, and other resources. In addition, to support studies and investigations it may be important for STLT health departments to consider all sources of funding and resources that may be available to them, including funding at the federal and state or local level, as well as nontraditional sources of funding.
When it has been determined that an epidemiologic study is feasible, do the following:
- Schedule meetings to discuss the initial findings and proposed approach for further assessment with the initial inquirer and other community members.
- Provide a detailed description of the next steps for evaluation. These next steps should be based on summary findings from the analysis of the 10 criteria in Phase 2 .
- Develop a summary that includes a rationale for continuing the investigation. For example, the assessment identified a potential spatial cluster or environmental factors that warrant further exploration.
Establish a community advisory committee
A community advisory committee (CAC) can contribute valuable sources of information and liaise with other community members. A CAC can provide specific details on the community, including help with cultural sensitivities or knowledge of current and/or historical environmental concerns in the area. A CAC can serve as a communication link back to the community at large.
Open communication will be needed to set goals, establish timelines, and discuss other issues (e.g., possible study limitations) so that expectations are clear. As previously mentioned, it may be important to also discuss issues related to resource requirements and other barriers that may impact conducting an epidemiologic study. Every community is different; however, agencies may be able to work with some existing organizations (e.g., neighborhood or religious organizations) to establish a CAC.
CAC and other community members can provide important insight and assist investigations:
- Help promote participation in studies and conduct outreach
- Identify specific tasks for community members that would contribute to the success of the investigation
- Identify additional data sources within the community (e.g., local lists that may be maintained which would identify previous residents)
- Identify population mobility patterns and housing development considerations
Consider the following for study design and protocol development
The following sections highlight the most important considerations for study design and protocol development. Consider developing a peer review committee with external partners to assess study design issues. Committee members should have a breadth of related skill sets in epidemiology, cancer, biostatistics, toxicology, and environmental health. The final draft of the protocol should be shared with the CAC in advance of initiating the study.
Case definition
Generate a formal case definition ahead of further analyses. Include the type of cancer, the population, the geographic area of concern, and the time period of interest. Review the study area and revise (if necessary) before starting additional analyses. For example, early assessments may have indicated that a particular contaminant was of concern and that more populations were found to be at potential risk for exposure to this contaminant. Thus, the study area would be expanded to include the other populations who were at potential risk for the exposure of concern.
A hypothesis regarding cancer cases or potential environmental contaminants was likely already generated during the assessment phase. However, hypotheses can evolve during an investigation, particularly if a potential exposure source has not been identified 1 . Re-review and update hypotheses regarding the cancer cases and contaminant(s) of interest (i.e., based on observations within the assessment phase to formulate analyses a priori). The hypothesis helps to guide the collection of data, the analysis plan, and interpretation of results.
Study population
Define the population of interest for the study. Ask the following questions to help define the study population as well as the time period of interest:
- How are the boundaries defined for the geographic area of interest?
- What are the characteristics of the population? Are there specific characteristics that are noted for the people with cancer (i.e., age group, race/ethnicity)?
- What is the latency period for the particular cancers of interest? What years of residence would need to be included to reflect the latency/development period assumed for the cancers of concern?
- How long have the residents lived in the area? What is the mobility and migration of former residents?
- Is there a potential for in utero exposures for the cancer(s) of concern based on where the mother lived during pregnancy?
Latency and change of residence add to the complexity of these investigations. Because residential history data are generally not available, collecting such data is critical as part of any epidemiologic study. Given the long latency period associated with cancers in adults, behaviors and exposures that might have contributed to the development of cancer in a person typically occur years to decades before the diagnosis. For example, malignant mesothelioma, a tumor of the lining of the lung, is associated with asbestos exposure, and the latency period between first exposure to asbestos and death from mesothelioma is often 30 years or longer 2 .
Latency in an epidemiologic investigation influences the exposure period relevant to the investigation. For example, if a person with cancer did not live in the suspected area of cancer concern during the relevant exposure period, then that person's cancer would not likely be related to an exposure in the area of concern. Conversely, the latency period might limit the ability to detect unusual patterns of cancer or identify cancers related to an environmental exposure that occurred in the past. In a mobile population, patterns resulting from an environmental contamination occurring years or even decades earlier might go undetected because exposed residents may have moved away from the community before the cancer developed. Thus, as persons move in and out of different communities, their cumulative exposure profile will change.
Because childhood cancers generally have shorter latency periods than cancers in adults, changes of residence might be less of an issue in the investigation of unusual patterns of childhood cancers. However, childhood cancer investigations may have the same limitations as adult counterparts. For example, in one California study of 380 children with a diagnosis of leukemia, approximately 65% of the study participants changed residence between birth and diagnosis 3 , indicating that even among cancers with short latency periods, migration might be an important factor. Account for latency when designing any additional analyses or studies.
Additional environmental data
If additional environmental data are needed, partner with state or local environmental regulatory agencies. ATSDR, including the regional offices, can also provide technical assistance, such as review of environmental sampling plans. Outline the types of sampling needed and resources available or necessary to conduct such sampling. Also, consider the following:
- Potential sources and routes of exposure (e.g., through air inhalation, water ingestion, in utero )
- Contaminant transport (e.g., if exposure occurs via ambient air, meteorological factors such as wind direction and speed are important)
- Other contributing sources of exposure
- Boundaries of potential spread/movement (important to understand the potential at-risk population)
- Any prior or ongoing remediation or reduction activities related to the environmental concern of interest
- Role of participating agencies in data collection and identification of resources to support the collection of environmental data.
Existing health data for case finding
Primarily, cases of cancer among the study population are identified from a state’s cancer registry, using the case definition. Consider multiple existing data sources for use to help identify cases. Forming partnerships with health information exchange or network entities may help with identifying other existing data sources. For additional support and guidance, CDC/ATSDR can provide technical assistance.
Contributing risk factors
An environmental factor may have been identified as a potential risk factor for the cancer of interest; however, cancers may be caused by several different risk factors or through multiple causal mechanisms 4 . Review additional risk factors for the type(s) of cancer under investigation, such as social determinants of health, behavioral risks, occupational exposures, and in some cases genetic factors.
Types of epidemiologic studies to consider
Methods used in environmental epidemiology are observational, not experimental. This makes the process of identifying a causative agent more difficult because researchers may not have access to information about other exposures or other variables that can create bias in the analysis 1 5 6 . However, additional studies can potentially help make inferences about cancer cases and potential associations. Consider the following types of descriptive or analytical studies:
- Case-series study : A case-series study is a descriptive analysis of persons with a similar cancer diagnosis. This study can be particularly helpful when there are small numbers of cases and it is not feasible to pursue other studies (e.g., statistical challenges associated with a small number of individuals diagnosed with the disease). Case-series studies are often designed to collect more information about each person to identify any commonalities. For these studies, identify as many relevant cases as possible to avoid selection bias. Depending on the findings, a further analytical study may be possible or warranted (see case-control or cohort below).
- Ecological study : An ecological study compares aggregated environmental data to aggregated cancer data to examine general associations. Exposures and outcomes are generally grouped to a geographic area, such as a census tract, for comparison. Individual exposures are not included in an ecological analysis. Ecological studies are most often done initially to explore potential associations but alone cannot determine causality. Epidemiologists must use caution when interpreting this type of analysis because the association with a particular environmental contaminant might not be true for individual cases, especially if there is heterogeneous distribution of the exposure over the geographic area. The related bias is known as ecological inference fallacy and could result in exposure misclassification.
- Cross-sectional study : A cross-sectional study is another descriptive study that measures health outcomes and exposure factors during a specific point in time. This type of study is helpful in describing the frequency of different characteristics in a study population during the time frame of interest. However, it may not be the ideal study design for investigating unusual patterns of cancer because this type of study design only looks at data distributions in a specific time frame, and temporal patterns cannot be established. In instances when biomarker data are available, a cross-sectional study may provide insights about key exposures among the population.
- Case-control study : Consider a case-control study when the etiology of the cancer is unknown. This type of analytical study can also be used to assess the association between cancer and a presumed exposure while being able to control for confounding factors. A case-control study collects information from cancer cases meeting the case-definition and controls within the same study area. Exposures and risk factors are compared between the two groups, for example using regression analysis methods. Case-control studies are suggested for rare cancer outcomes. Unlike the ecological study, case-control studies allow for the collection of individual-level data and risk factors to assess within the analyses. The primary disadvantages of a case-control study are the inability for individuals to recall historic events (e.g., exposures) and the difficulty of providing a direct estimate of risk. Risk is often estimated as an odds ratio, showing the odds for a particular cancer to have occurred given a particular exposure, compared to odds of the same cancer without the exposure. For this type of study, the sample size needed should be calculated ahead of time considering the power needed to detect statistical differences between the populations ( Appendix A ).
- Cohort (retrospective or prospective) : Consider a cohort study when the exposure source is known or being investigated. Select people who have varying levels of exposure for the study. Prospective cohort studies, while one of the strongest types of studies to examine exposure-disease relationships, are very time consuming and expensive. Depending on the latency of the cancer(s) of concern, a prospective cohort study may need to be 10–20 years or longer to collect enough data for analyses. The length of time needed often must account for study attrition and thus require a very large sample size. A retrospective cohort study may be feasible, but also is reliant on time-related information. For example, a cohort study may want to compare a population exposed to chemicals from Factory X, which was operating in the early 1990s. To establish a study cohort, a population within that community would have to have been in the study area ~30 years previously. Because of the length of time needed for these studies, some challenges may arise that need to be accounted for within the study design. For example, a community may have experienced a substantial percentage of movement or migration. Also, as previously mentioned, the latency of the cancer will also need to be accounted for within the study design. For example, the average latency for mesothelioma is generally thought to be decades 7 . More recently, data from the World Trade Center Health Program suggests that the minimum latency for mesothelioma is about 11 years and the minimum latency for thyroid cancer is 2.5 years 4 . Studies assessing these types of cancers would have to account for the number of years that specific types of cancers develop following exposures. Additionally, limitations exist with quantifying historic exposures for retrospective cohort studies.
Ongoing technical and scientific advancements in areas such as data science, analytic and geospatial methods, and cancer genomics may be available in the future (e.g., genomic research, gene-environment interaction).
Other considerations for your study
When evaluating the feasibility of different epidemiologic studies, consider the following:
- Funding : Funding may be needed to help with the investigation. Seek state and federal opportunities to assist with funding the investigation or with providing technical assistance. In addition, academic partnerships may help to support the investigation.
- Outreach : For more robust analyses, reach out to other states to discuss case-finding and/or environmental sampling activities, specifically in neighboring states if suspected environmental contaminants cross state borders.
- Communication : Ultimately, the goal of an epidemiologic investigation of unusual patterns or excesses of cancer is to understand the potential relationship between environmental and other risk factors identified and observed cases of cancer. Some epidemiologic investigations have identified potential associations between certain cancers and risk factors including exposures to certain chemicals 8 9 10 . However, methodological limitations and data limitations (such as unknown levels of exposure) often limit the ability to demonstrate that such relationships exist. Regardless of the outcome of an epidemiologic investigation, continuing to communicate with individuals most concerned about exposures and health outcomes is important.
- Merrill R. Environmental Epidemiology, Principles and Methods. Sudbury, MA: Jones and Bartlett Publishers, Inc.; 2008.
- Lanphear B, Buncher C. Latent period for malignant mesothelioma of occupational origin – PubMed. J Occup Med [Internet]. 1992 [cited 2022 Jan 7];34(7):718–21. Available from: https://pubmed.ncbi.nlm.nih.gov/1494965/
- Urayama KY, Von Behren J, Reynolds P, Hertz A, Does M, Buffler PA. Factors associated with residential mobility in children with leukemia: implications for assigning exposures. Ann Epidemiol [Internet]. 2009 Nov [cited 2022 Jan 7];19(11):834–40. Available from: https://pubmed.ncbi.nlm.nih.gov/19364662/
- CDC. World Trade Center Health Program [Internet]. Available from: https://www.cdc.gov/wtc/pdfs/policies/WTCHP-Minimum-Cancer-Latency-PP-01062015-508.pdf
- Gordis L. Epidemiology. Philadelphia, PA: Elsevier Saunders; 2014.
- Dicker R, Coronado F, Koo D, Parrish R. Principles of Epidemiology in Public Health Practice: An Introduction to Applied Epidemiology and Biostatics. CDC Office of Workforce and Career Development; 2006.
- Frost G. The latency period of mesothelioma among a cohort of British asbestos workers (1978–2005). Br J Cancer. 2013 Oct 29;109(7):1965–73.
- Costas K, Knorr RS, Condon SK. A case-control study of childhood leukemia in Woburn, Massachusetts: The relationship between leukemia incidence and exposure to public drinking water. Sci Total Environ. 2002;300(1–3):23–35.
- Massachusetts Department of Public Health. The Wilmington Childhood Cancer Study: An Epidemiologic Investigation of Childhood Cancer from 1990–2000. 2021.
- Vieira VM, Hoffman K, Shin HM, Weinberg JM, Webster TF, Fletcher T. Perfluorooctanoic acid exposure and cancer outcomes in a contaminated community: A geographic analysis. Environ Health Perspect. 2013;121(3):318–23.
Unusual Cancer Patterns
Guidelines and resources for examining unusual patterns of cancer and environmental concerns.
For Everyone
Public health.
- Get 7 Days Free
EHA 2024 | Results from Five Studies of Ascentage Pharma's Key Drug Candidates Selected for Presentations at 2024 European Hematology Association Hybrid Congress
PR Newswire
SUZHOU, China and ROCKVILLE, Md., May 15, 2024
SUZHOU, China and ROCKVILLE, Md., May 15, 2024 /PRNewswire/ -- Ascentage Pharma (6855.HK), a global biopharmaceutical company engaged in developing novel therapies for cancer, chronic hepatitis B (CHB), and age-related diseases, announced today that the latest results from five studies have been selected for Poster Presentations at the 2024 European Hematology Association Hybrid Congress (EHA 2024). These posters will feature olverembatinib (HQP1351), the first and only China-approved third-generation BCL-ABL1 inhibitor; investigational lisaftoclax (APG-2575), a Bcl-2 selective inhibitor; and investigational APG-5918, an EED selective inhibitor.

The European Hematology Association (EHA) Hybrid Congress is the largest gathering of the hematology field in Europe. It showcases the most cutting-edge research and state-of-the-art innovative therapies, attracting over 10,000 clinical experts and researchers from more than 100 countries every year. This year, the EHA Hybrid Congress will take place on June 13 - 16, 2024, in Madrid, Spain.
"I am delighted to showcase the strength and progress of Ascentage Pharma in hematology at this year's congress, especially the therapeutic potential and clinical value of its drug candidates in chronic myeloid leukemia (CML), Philadelphia chromosome-positive acute lymphoblastic leukemia (Ph+ ALL), multiple myeloma (MM), immunoglobulin light-chain (AL) amyloidosis, and anemia diseases," said Dr. Yifan Zhai, Chief Medical Officer of Ascentage Pharma . "We look forward to sharing the detailed results at the congress. In the future, we will continue to advance those development programs in efforts to bring more treatment options to patients around the world."
The five studies to be presented at EHA 2024 are as follows:
- Olverembatinib
Olverembatinib Overcomes Ponatinib and Asciminib Resistance in Patients (Pts) with Heavily Pretreated Chronic Myeloid Leukemia (CML) and Philadelphia-Positive Acute Lymphoblastic Leukemia (Ph ⁺ ALL)
• Abstract#: P722 • Presentation Type: Poster presentation • Topic: Chronic myeloid leukemia – Clinical • Date & Time: Friday June 14, 2024, 18:00 - 19:00 CEST • Presenting Author: Dr. Elias Jabbour, The University of Texas MD Anderson Cancer Center
Combination of Third Generation TKI Olverembatinib and Chemotherapy or Blinatumomab for New Diagnosed Adult Ph + ALL Patients
• Abstract#: P427 • Presentation Type: Poster presentation • Topic: Acute lymphoblastic leukemia – Clinical • Date & Time: Friday June 14, 2024, 18:00 - 19:00 CEST • Presenting Author: Junjie Chen, Nanfang Hospital, Southern Medical University
Patient Reported Outcomes in Adults with TKI-Resistant Chronic Myeloid Leukemia Receiving Olverembatinib-Therapy
• Abstract#: P1862 • Presentation Type: e-Poster presentation • Topic: Chronic myeloid leukemia – Clinical • Date & Time: Friday June 14, 2024, 18:00 - 19:00 CEST • Presenting Author: Lu Yu, Peking University People's Hospital
- Lisaftoclax
Lisaftoclax (APG-2575) Combined with Novel Therapeutic Regimens in Patients (Pts) with Relapsed or Refractory (R/R) Multiple Myeloma (MM) or Immunoglobulin Light-Chain (AL) Amyloidosis
• Abstract#: P917 • Presentation Type: Poster presentation • Topic: Myeloma and other monoclonal gammopathies – Clinical • Date & Time: Friday June 14, 2024, 18:00 - 19:00 CEST • Presenting Author: Dr. Sikander Ailawadhi, Mayo Clinic Florida
Embryonic Ectoderm Development (EED) Inhibitor APG-5918 Improves Chronic Kidney Disease- (CKD)-Induced Hemoglobin (HB) Insufficiency in Preclinical Models of Anemia
• Abstract#: P1550 • Presentation Type: Poster presentation • Topic: Enzymopathies, membranopathies and other anemias • Date & Time: Friday June 14, 2024, 18:00 - 19:00 CEST • Presenting Author: Dr. Eric Liang, Ascentage Pharma Group Inc.
About Ascentage Pharma
Ascentage Pharma (6855.HK) is a globally focused biopharmaceutical company engaged in developing novel therapies for cancers, chronic hepatitis B, and age-related diseases. On October 28, 2019, Ascentage Pharma was listed on the Main Board of the Stock Exchange of Hong Kong Limited with the stock code 6855.HK.
Ascentage Pharma focuses on developing therapeutics that inhibit protein-protein interactions to restore apoptosis, or programmed cell death. The company has built a pipeline of 9 clinical drug candidates, including novel, highly potent Bcl-2, and dual Bcl-2/Bcl-xL inhibitors, as well as candidates aimed at IAP and MDM2-p53 pathways, and next-generation tyrosine kinase inhibitors (TKIs). Ascentage Pharma is also the only company in the world with active clinical programs targeting all three known classes of key apoptosis regulators. The company is conducting more than 40 Phase I/II clinical trials, including 5 global registrational phase III studies, in the US, Australia, Europe, and China. Ascentage Pharma has been designated for multiple Major National R&D Projects, including five Major New Drug Projects, one New Drug Incubator status, four Innovative Drug Programs, and one Major Project for the Prevention and Treatment of Infectious Diseases.
Olverembatinib, the company's core drug candidate developed for the treatment of drug-resistant chronic myeloid leukemia (CML) and the company's first approved product, has been granted Priority Review Designations and Breakthrough Therapy Designations by the Center for Drug Evaluation (CDE) of China National Medical Products Administration (NMPA). To date, the drug had been included into the China 2022 National Reimbursement Drug List (NRDL). Furthermore, olverembatinib has been granted an Orphan Drug Designation (ODD) and a Fast Track Designation (FTD) by the US FDA, and an Orphan Designation by the EMA of the EU. To date, Ascentage Pharma has obtained a total of 16 ODDs from the US FDA and 1 Orphan Designation from the EMA of the EU for 4 of the company's investigational drug candidates.
Leveraging its robust R&D capabilities, Ascentage Pharma has built a portfolio of global intellectual property rights and entered into global partnerships with numerous renowned biotechnology and pharmaceutical companies and research institutes such as UNITY Biotechnology, MD Anderson Cancer Center, Mayo Clinic, Dana-Farber Cancer Institute, MSD, and AstraZeneca. The company has built a talented team with global experience in the discovery and development of innovative drugs and is setting up its world-class commercial manufacturing and Sales & Marketing teams. One pivotal aim of Ascentage Pharma is to continuously strengthen its R&D capabilities and accelerate its clinical development programs, in order to fulfil its mission of addressing unmet clinical needs in China and around the world for the benefit of more patients.
Forward-Looking Statements
The forward-looking statements made in this article relate only to the events or information as of the date on which the statements are made in this article. Except as required by law, Ascentage Pharma undertakes no obligation to update or revise publicly any forward-looking statements, whether as a result of new information, future events, or otherwise, after the date on which the statements are made or to reflect the occurrence of unanticipated events. You should read this article completely and with the understanding that our actual future results or performance may be materially different from what we expect. In this article, statements of, or references to, our intentions or those of any of our Directors or our Company are made as of the date of this article. Any of these intentions may alter in light of future development.

SOURCE Ascentage Pharma
Search by keyword
Gdp up by 0.3% and employment up by 0.3% in the euro area, gdp growth in the euro area and eu.
In the first quarter of 2024, seasonally adjusted GDP increased by 0.3% in both the euro area and the EU , compared with the previous quarter, according to a flash estimate published by Eurostat, the statistical office of the European Union . In the fourth quarter of 2023, GDP had declined by 0.1% in the euro area and had remained stable in the EU .
Compared with the same quarter of the previous year, seasonally adjusted GDP increased by 0.4% both in the euro area and in the EU in the first quarter of 2024, after +0.1% in the euro area and +0.2% in the EU in the previous quarter.
During the first quarter of 2024, GDP in the United States increased by 0.4% compared to the previous quarter (after +0.8% in the fourth quarter of 2023). Compared with the same quarter of the previous year, GDP increased by 3.0% (after +3.1% in the previous quarter).
Employment growth in the euro area and EU
The number of employed persons increased by 0.3% in the euro area and by 0.2% in the EU in the first quarter of 2024, compared with the previous quarter. These are the same trends as observed in the fourth quarter of 2023.
Compared with the same quarter of the previous year, employment increased by 1.0% in the euro area and by 0.7% in the EU in the first quarter of 2024, after +1.2% in the euro area and +1.0% in the EU in the fourth quarter of 2023.
These data provide a picture of labour input consistent with the output and income measures of national accounts.
Notes for users
The reliability of GDP and employment flash estimates was tested by dedicated working groups and revisions of subsequent estimates are continuously monitored. Further information can be found on Eurostat website .
With these flash estimates, euro area and EU employment and GDP figures for earlier quarters are not revised.
The flash GDP estimates of the first quarter 2024 are based on Member States’ data covering 99% of the EA and the EU GDP, while flash employment estimates are based on Member States’ data covering 96% of the EA and 93% of the EU total employment.
A preliminary flash estimate of GDP growth was published in News Release issued on 30 April 2024. This was based on GDP estimates for eighteen Member States.
The EA and EU estimates for the last quarter were revised as presented in the following table:
All figures presented in this release may be revised with Eurostat’s regular estimates of GDP and main aggregates (including employment) scheduled for 7 June 2024 and 19 July 2024.
Release schedule
Comprehensive estimates of European main aggregates (including GDP and employment) are based on countries regular transmissions and published around 65 and 110 days after the end of each quarter. To improve the timeliness of key indicators, Eurostat also publishes flash estimates for GDP (after around 30 and 45 days) and employment (after around 45 days). Their compilation is based on estimates provided by EU Member States on a voluntary basis.
This news release presents flash estimates for euro area and EU GDP and employment growth after around 45 days.
Methods and definitions
European quarterly national accounts are compiled in accordance with the European System of Accounts 2010 (ESA 2010). They include key policy indicators of GDP and employment.
Gross domestic product (GDP) at market prices measures the production activity of resident production units. Growth rates are based on chain-linked volumes.
Employment covers employees and self-employed working in resident production units (domestic concept). While employment flash estimates are limited to total employment in persons, regular estimates also cover hours worked and industry breakdowns.
The method used for compilation of European GDP and employment estimates is the same as for previous releases.
Geographical information
Euro area (EA20): Belgium, Germany, Estonia, Ireland, Greece, Spain, France, Croatia, Italy, Cyprus, Latvia, Lithuania, Luxembourg, Malta, the Netherlands, Austria, Portugal, Slovenia, Slovakia and Finland.
European Union (EU27): Belgium, Bulgaria, Czechia, Denmark, Germany, Estonia, Ireland, Greece, Spain, France, Croatia, Italy, Cyprus, Latvia, Lithuania, Luxembourg, Hungary, Malta, the Netherlands, Austria, Poland, Portugal, Romania, Slovenia, Slovakia, Finland and Sweden.
For more information
Website section on national accounts , notably information on European GDP and employment estimates
Database section on national accounts and metadata on quarterly national accounts
Statistics Explained articles on measuring quarterly GDP and presentation of updated quarterly estimates
Country specific metadata
Country specific metadata on the recording of Ukrainian refugees in main aggregates of national accounts
European System of Accounts 2010
Euro indicators dashboard
Release calendar for Euro indicators
European Statistics Code of Practice
Get in touch
Media requests
Eurostat Media Support
Phone: (+352) 4301 33 408
E-mail: [email protected]
Further information on data
Véronique DENEUVILLE (GDP)
E-mail: [email protected]
Jenny RUNESSON (Employment)
Share the release
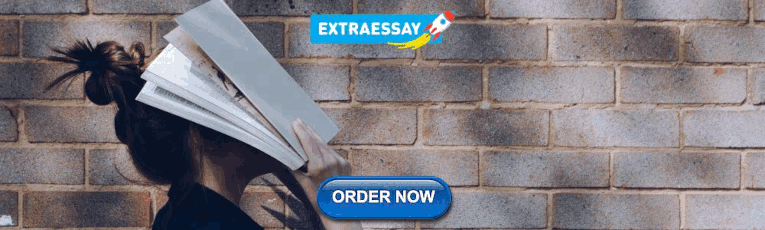
IMAGES
VIDEO
COMMENTS
Structured Narrative: Your data presentation must tell a coherent story. This means a beginning where you present the context, a middle section in which you present the data, and an ending that uses a call-to-action. ... This is consistent with their hypothesis that blue light, especially before bedtime, has a significant impact on sleep ...
Table of contents. Step 1: State your null and alternate hypothesis. Step 2: Collect data. Step 3: Perform a statistical test. Step 4: Decide whether to reject or fail to reject your null hypothesis. Step 5: Present your findings. Other interesting articles. Frequently asked questions about hypothesis testing.
5. Phrase your hypothesis in three ways. To identify the variables, you can write a simple prediction in if…then form. The first part of the sentence states the independent variable and the second part states the dependent variable. If a first-year student starts attending more lectures, then their exam scores will improve.
Be the first to add your personal experience. 6. Deliver your presentation. 7. Here's what else to consider. Be the first to add your personal experience. Data analysis is a valuable skill, but ...
1. Know your audience. Be the first to add your personal experience. 2. Choose the right data. Be the first to add your personal experience. 3. Visualize your data. Be the first to add your ...
TheJoelTruth. While a good presentation has data, data alone doesn't guarantee a good presentation. It's all about how that data is presented. The quickest way to confuse your audience is by ...
Storytelling with data is a highly valued skill in the workforce today and translating data and insights for a non-technical audience is rare to see than it is expected. Here's my five-step routine to make and deliver your data presentation right where it is intended —. 1. Understand Your Data & Make It Seen.
Given your alpha level, if the p <= alpha, the null hypothesis is rejected in favour of the alternative hypothesis with confidence level 1-alpha. If the p-value is greater than the alpha-level, the null hypothesis is accepted. Summary. In hypothesis testing, two mutually exclusive statements about a population are tested using a random data sample.
Large figures should have thousands separated with commas. For example, 4,498,300,000 makes for a much easier read than "4498300000". Any corresponding units should also be clear. With data presentation, don't forget that numbers are still your protagonist, so they must be highlighted with a larger or bolder font.
Step 5: Phrase your hypothesis in three ways. To identify the variables, you can write a simple prediction in if … then form. The first part of the sentence states the independent variable and the second part states the dependent variable. If a first-year student starts attending more lectures, then their exam scores will improve.
Since the hypothesis is an increase of 5% in user spend, this means that you expect the new landing page to make $10.50 on average. You want to be extra careful, so you also hypothesize that the new landing page will have a higher standard deviation ($9 instead of $8). To sum up, your hypothesis is:
3. Simple hypothesis. A simple hypothesis is a statement made to reflect the relation between exactly two variables. One independent and one dependent. Consider the example, "Smoking is a prominent cause of lung cancer." The dependent variable, lung cancer, is dependent on the independent variable, smoking. 4.
A question or problem your data analysis answers; A way to give your audience context about your data; Correct. An initial hypothesis is a theory you're trying to prove or disprove with data. You want to establish your hypothesis early in the presentation. Step-by-step critique of a presentation Messy data presentation
In framing a hypothesis, the data scientist must not know the outcome of the hypothesis that has been generated based on any evidence. "A hypothesis may be simply defined as a guess. A scientific hypothesis is an intelligent guess." - Isaac Asimov. Hypothesis generation is a crucial step in any data science project.
The steps to write a research hypothesis are: 1. Stating the problem: Ensure that the hypothesis defines the research problem. 2. Writing a hypothesis as an 'if-then' statement: Include the action and the expected outcome of your study by following a 'if-then' structure. 3.
The most common way of presentation of data is in the form of statements. This works best for simple observations, such as: "When viewed by light microscopy, all of the cells appeared dead." When data are more quantitative, such as- "7 out of 10 cells were dead", a table is the preferred form. Tables.
It seeks to explore and understand a particular aspect of the research subject. In contrast, a research hypothesis is a specific statement or prediction that suggests an expected relationship between variables. It is formulated based on existing knowledge or theories and guides the research design and data analysis. 7.
A hypothesis is a tentative statement about the relationship between two or more variables. It is a specific, testable prediction about what you expect to happen in a study. It is a preliminary answer to your question that helps guide the research process. Consider a study designed to examine the relationship between sleep deprivation and test ...
It summarizes the findings succinctly. It provides context about the presentation's purpose. It visualizes the data clearly and concisely. It describes the data thoroughly. Correct. Establishing a hypothesis provides the audience with context about the analyst's presentation. In this scenario, it establishes what the analyst wants to prove ...
Study with Quizlet and memorize flashcards containing terms like A data analyst gives a presentation about predicting upcoming investment opportunities. How does establishing a hypothesis help the audience understand their predictions? Provides context about the presentation's purpose Summarizes the findings succinctly Visualizes the data clearly and concisely Describes the data thoroughly ...
Shuffle Q/A 2. 19. One of your stakeholders tried to reproduce the work you presented by using a copy of your scripts and was unable to get the same results. Which kind of objection are they making? Answers. 20. One of your co-workers is giving a presentation on the results of an analysis the two of you have been working on. Someone in the ...
Summary. What happens when you have to give a presentation to an audience that might have some professionals who have more expertise on the topic than you do? While it can be intimidating, it can ...
With Adobe Express, marketers can easily build beautiful and compelling data narratives. Join this hands-on workshop to learn how to use Adobe Express to: Create stunning presentations and data visualizations using easy-to-master tools and templates. Incorporate branded elements, such as colors, logos, and imagery, to assemble cohesive data ...
Asked by shahinurislam. Which of the following statements is true about using a hypothesis in your data presentation? 1 point Include the hypothesis in a summary at the end of your presentation Include a new hypothesis before every data visualization Choose a hypothesis your audience will like Present the hypothesis early in your presentation.
Using a hypothesis in data presentation provides a clear framework for testing and analyzing specific predictions derived from the collected data, enhancing the rigor and interpretability of the findings. Hypotheses are unnecessary in data presentation; data can be presented without any specific predictions. Hypotheses restrict the flexibility ...
Re-review and update hypotheses regarding the cancer cases and contaminant(s) of interest (i.e., based on observations within the assessment phase to formulate analyses a priori). The hypothesis helps to guide the collection of data, the analysis plan, and interpretation of results. Study population. Define the population of interest for the study.
• Presentation Type: Poster presentation • Topic: Acute lymphoblastic leukemia - Clinical • Date & Time: Friday June 14, 2024, 18:00 - 19:00 CEST
Prior to GPT-4o, you could use Voice Mode to talk to ChatGPT with latencies of 2.8 seconds (GPT-3.5) and 5.4 seconds (GPT-4) on average. To achieve this, Voice Mode is a pipeline of three separate models: one simple model transcribes audio to text, GPT-3.5 or GPT-4 takes in text and outputs text, and a third simple model converts that text back to audio.
GDP growth in the euro area and EU In the first quarter of 2024, seasonally adjusted GDP increased by 0.3% in both the euro area and the EU, compared with the previous quarter, according to a flash estimate published by Eurostat, the statistical office of the European Union. In the fourth quarter of 2023, GDP had declined by 0.1% in the euro area and had remained stable in the EU. Compared ...