share this!
April 26, 2024
This article has been reviewed according to Science X's editorial process and policies . Editors have highlighted the following attributes while ensuring the content's credibility:
fact-checked
peer-reviewed publication
trusted source
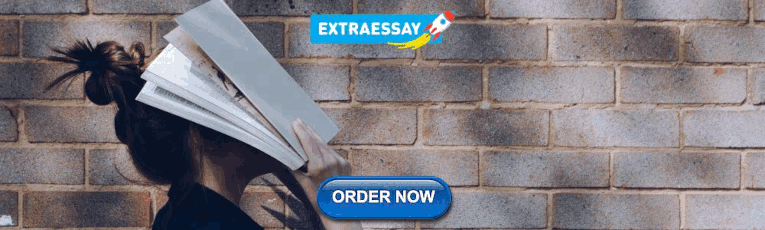
Automated machine learning robot unlocks new potential for genetics research
by University of Minnesota
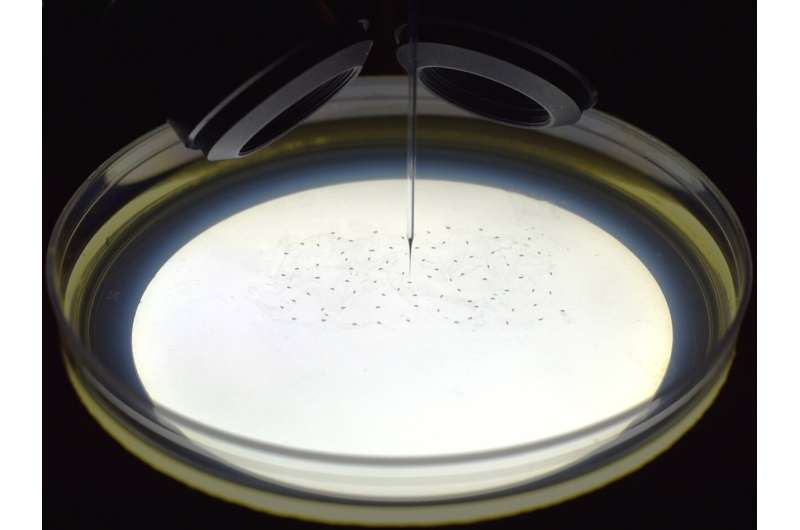
University of Minnesota Twin Cities researchers have constructed a robot that uses machine learning to fully automate a complicated microinjection process used in genetic research.
In their experiments, the researchers were able to use this automated robot to manipulate the genetics of multicellular organisms, including fruit fly and zebrafish embryos . The technology will save labs time and money while enabling them to more easily conduct new, large-scale genetic experiments that were not possible previously using manual techniques
The research, titled "High-throughput genetic manipulation of multicellular organisms using a machine-vision guided embryonic microinjection robot," is featured on the cover of the April 2024 issue of GENETICS , an open access journal. The work was co-led by two University of Minnesota mechanical engineering graduate students Andrew Alegria and Amey Joshi. The team is also working to commercialize this technology to make it widely available through the University of Minnesota start-up company, Objective Biotechnology.
Microinjection is a method for introducing cells, genetic material , or other agents directly into embryos, cells, or tissues using a very fine pipette. The researchers have trained the robot to detect embryos that are one-hundredth the size of a grain of rice. After detection, the machine can calculate a path and automate the process of the injections.
"This new process is more robust and reproducible than manual injections," said Suhasa Kodandaramaiah, a University of Minnesota mechanical engineering associate professor and senior author of the study. "With this model, individual laboratories will be able to think of new experiments that you couldn't do without this type of technology."
Typically, this type of research requires highly skilled technicians to perform the microinjection, which many laboratories do not have. This new technology could expand the ability to perform large experiments in labs, while reducing time and costs.
"This is very exciting for the world of genetics. Writing and reading DNA have drastically improved in recent years, but having this technology will increase our ability to perform large-scale genetic experiments in a wide range of organisms," said Daryl Gohl, a co-author of the study, the group leader of the University of Minnesota Genomics Center's Innovation Lab and research assistant professor in the Department of Genetics, Cell Biology, and Development.
Not only can this technology be used in genetic experiments, but it can also help to preserve endangered species through cryopreservation, a preservation technique conducted at ultra-low temperatures.
"You can use this robot to inject nanoparticles into cells and tissues that helps in cryopreservation and in the process of rewarming afterwards," Kodandaramaiah explained.
Other team members highlighted other applications for the technology that could have even more impact.
"We hope that this technology could eventually be used for in vitro fertilization, where you could detect those eggs on the microscale level," said Andrew Alegria, co-lead author on the paper and University of Minnesota mechanical engineering graduate research assistant in the Biosensing and Biorobotics Lab.
In addition to Kodandaramaiah, Gohl, Alegria, and Joshi, the team included several researchers from the University of Minnesota's College of Science and Engineering and the University of Minnesota Genomics Center's Innovation Lab. The team recently won the University's "Walleye Tank" life science competition. This life science pitch competition provides education and promotional opportunities for emerging and established medical and life science companies.
This research was completed in collaboration with the Engineering Research Center for Advanced Technologies for the Preservation of Biological Systems (ATP-Bio) and the University of Minnesota Zebrafish Core.
Journal information: Genetics
Provided by University of Minnesota
Explore further
Feedback to editors

Research shows baby bird development harmed by sound of cars
8 minutes ago

Bacteria 'nanowires' could help develop green electronics
9 minutes ago

Webb captures iconic Horsehead Nebula in unprecedented detail
12 minutes ago

Orion's erupting star system reveals its secrets

Cartilage healing discovery in animal models could lead to new human therapies
17 minutes ago

Topologically controlled multiskyrmions: Researchers propose a new family of quasiparticles

Tidal disruption event ASASSN-19bt experiences unusual radio evolution, observations show
19 minutes ago

Microgravity-grown crystals reveal new insights into protein structures

Study provides new global accounting of Earth's rivers
27 minutes ago

Researchers achieve electrosynthesis via superwetting organic-solid-water interfaces
28 minutes ago
Relevant PhysicsForums posts
Is 5 milliamps at 240 volts dangerous.
11 hours ago
The Cass Report (UK)
Apr 24, 2024
Major Evolution in Action
Apr 22, 2024
If theres a 15% probability each month of getting a woman pregnant...
Apr 19, 2024
Can four legged animals drink from beneath their feet?
Apr 15, 2024
Mold in Plastic Water Bottles? What does it eat?
Apr 14, 2024
More from Biology and Medical
Related Stories

New method preserves viable fruit fly embryos in liquid nitrogen
Apr 27, 2021

Pioneering robot arm poised to reach new heights in quantum
Nov 17, 2023

Simple liquid-dispensing robot automates experiments to evaluate yeast growth potential
May 11, 2023

Researchers revolutionize vital conservation tool with use of gold nanotechnology and lasers (Update)
Jul 13, 2017

Researchers tune thermal conductivity of materials 'on the fly' for more energy-efficient devices
Jun 8, 2023

Research team achieves near-perfect light absorption in atomic-scale material
Aug 2, 2023
Recommended for you

Getting dynamic information from static snapshots
Apr 27, 2024

Study suggests host response needs to be studied along with other bacteriophage research
Apr 26, 2024

Study details a common bacterial defense against viral infection

Researchers decipher how an enzyme modifies the genetic material in the cell nucleus

Scientists discover higher levels of CO₂ increase survival of viruses in the air and transmission risk

AI deciphers new gene regulatory code in plants and makes accurate predictions for newly sequenced genomes
Let us know if there is a problem with our content.
Use this form if you have come across a typo, inaccuracy or would like to send an edit request for the content on this page. For general inquiries, please use our contact form . For general feedback, use the public comments section below (please adhere to guidelines ).
Please select the most appropriate category to facilitate processing of your request
Thank you for taking time to provide your feedback to the editors.
Your feedback is important to us. However, we do not guarantee individual replies due to the high volume of messages.
E-mail the story
Your email address is used only to let the recipient know who sent the email. Neither your address nor the recipient's address will be used for any other purpose. The information you enter will appear in your e-mail message and is not retained by Phys.org in any form.
Newsletter sign up
Get weekly and/or daily updates delivered to your inbox. You can unsubscribe at any time and we'll never share your details to third parties.
More information Privacy policy
Donate and enjoy an ad-free experience
We keep our content available to everyone. Consider supporting Science X's mission by getting a premium account.
E-mail newsletter

An official website of the United States government
The .gov means it’s official. Federal government websites often end in .gov or .mil. Before sharing sensitive information, make sure you’re on a federal government site.
The site is secure. The https:// ensures that you are connecting to the official website and that any information you provide is encrypted and transmitted securely.
- Publications
- Account settings
Preview improvements coming to the PMC website in October 2024. Learn More or Try it out now .
- Advanced Search
- Journal List
- Springer Nature - PMC COVID-19 Collection

A review on genetic algorithm: past, present, and future
Sourabh katoch.
Computer Science and Engineering Department, National Institute of Technology, Hamirpur, India
Sumit Singh Chauhan
Vijay kumar.
In this paper, the analysis of recent advances in genetic algorithms is discussed. The genetic algorithms of great interest in research community are selected for analysis. This review will help the new and demanding researchers to provide the wider vision of genetic algorithms. The well-known algorithms and their implementation are presented with their pros and cons. The genetic operators and their usages are discussed with the aim of facilitating new researchers. The different research domains involved in genetic algorithms are covered. The future research directions in the area of genetic operators, fitness function and hybrid algorithms are discussed. This structured review will be helpful for research and graduate teaching.
Introduction
In the recent years, metaheuristic algorithms are used to solve real-life complex problems arising from different fields such as economics, engineering, politics, management, and engineering [ 113 ]. Intensification and diversification are the key elements of metaheuristic algorithm. The proper balance between these elements are required to solve the real-life problem in an effective manner. Most of metaheuristic algorithms are inspired from biological evolution process, swarm behavior, and physics’ law [ 17 ]. These algorithms are broadly classified into two categories namely single solution and population based metaheuristic algorithm (Fig. 1 ). Single-solution based metaheuristic algorithms utilize single candidate solution and improve this solution by using local search. However, the solution obtained from single-solution based metaheuristics may stuck in local optima [ 112 ]. The well-known single-solution based metaheuristics are simulated annealing, tabu search (TS), microcanonical annealing (MA), and guided local search (GLS). Population-based metaheuristics utilizes multiple candidate solutions during the search process. These metaheuristics maintain the diversity in population and avoid the solutions are being stuck in local optima. Some of well-known population-based metaheuristic algorithms are genetic algorithm (GA) [ 135 ], particle swarm optimization (PSO) [ 101 ], ant colony optimization (ACO) [ 47 ], spotted hyena optimizer (SHO) [ 41 ], emperor penguin optimizer (EPO) [ 42 ], and seagull optimization (SOA) [ 43 ].

Classification of metaheuristic Algorithms
Among the metaheuristic algorithms, Genetic algorithm (GA) is a well-known algorithm, which is inspired from biological evolution process [ 136 ]. GA mimics the Darwinian theory of survival of fittest in nature. GA was proposed by J.H. Holland in 1992. The basic elements of GA are chromosome representation, fitness selection, and biological-inspired operators. Holland also introduced a novel element namely, Inversion that is generally used in implementations of GA [ 77 ]. Typically, the chromosomes take the binary string format. In chromosomes, each locus (specific position on chromosome) has two possible alleles (variant forms of genes) - 0 and 1. Chromosomes are considered as points in the solution space. These are processed using genetic operators by iteratively replacing its population. The fitness function is used to assign a value for all the chromosomes in the population [ 136 ]. The biological-inspired operators are selection, mutation, and crossover. In selection, the chromosomes are selected on the basis of its fitness value for further processing. In crossover operator, a random locus is chosen and it changes the subsequences between chromosomes to create off-springs. In mutation, some bits of the chromosomes will be randomly flipped on the basis of probability [ 77 , 135 , 136 ]. The further development of GA based on operators, representation, and fitness has diminished. Therefore, these elements of GA are focused in this paper.
The main contribution of this paper are as follows:
- The general framework of GA and hybrid GA are elaborated with mathematical formulation.
- The various types of genetic operators are discussed with their pros and cons.
- The variants of GA with their pros and cons are discussed.
- The applicability of GA in multimedia fields is discussed.
The main aim of this paper is two folds. First, it presents the variants of GA and their applicability in various fields. Second, it broadens the area of possible users in various fields. The various types of crossover, mutation, selection, and encoding techniques are discussed. The single-objective, multi-objective, parallel, and hybrid GAs are deliberated with their advantages and disadvantages. The multimedia applications of GAs are elaborated.
The remainder of this paper is organized as follows: Section 2 presents the methodology used to carry out the research. The classical genetic algorithm and genetic operators are discussed in Section 3 . The variants of genetic algorithm with pros and cons are presented in Section 4 . Section 5 describes the applications of genetic algorithm. Section 6 presents the challenges and future research directions. The concluding remarks are drawn in Section 7 .
Research methodology
PRISMA’s guidelines were used to conduct the review of GA [ 138 ]. A detailed search has been done on Google scholar and PubMed for identification of research papers related to GA. The important research works found during the manual search were also added in this paper. During search, some keywords such as “Genetic Algorithm” or “Application of GA” or “operators of GA” or “representation of GA” or “variants of GA” were used. The selection and rejection of explored research papers are based on the principles, which is mentioned in Table Table1 1 .
Selection criterion for shortlisted research papers
Total 27,64,792 research papers were explored on Google Scholar, PubMed and manual search. The research work related to genetic algorithm for multimedia applications were also included. During the screening of research papers, all the duplicate papers and papers published before 2007 were discarded. 4340 research papers were selected based on 2007 and duplicate entries. Thereafter, 4050 research papers were eliminated based on titles. 220 research papers were eliminated after reading of abstract. 70 research papers were left after third round of screening. 40 more research papers were discarded after full paper reading and facts found in the papers. After the fourth round of screening, final 30 research papers are selected for review.
Based on the relevance and quality of research, 30 papers were selected for evaluation. The relevance of research is decided through some criteria, which is mentioned in Table Table1. 1 . The selected research papers comprise of genetic algorithm for multimedia applications, advancement of their genetic operators, and hybridization of genetic algorithm with other well-established metaheuristic algorithms. The pros and cons of genetic operators are shown in preceding section.
In this section, the basic structure of GA and its genetic operators are discussed with pros and cons.
Classical GA
Genetic algorithm (GA) is an optimization algorithm that is inspired from the natural selection. It is a population based search algorithm, which utilizes the concept of survival of fittest [ 135 ]. The new populations are produced by iterative use of genetic operators on individuals present in the population. The chromosome representation, selection, crossover, mutation, and fitness function computation are the key elements of GA. The procedure of GA is as follows. A population ( Y ) of n chromosomes are initialized randomly. The fitness of each chromosome in Y is computed. Two chromosomes say C1 and C2 are selected from the population Y according to the fitness value. The single-point crossover operator with crossover probability (C p ) is applied on C1 and C2 to produce an offspring say O . Thereafter, uniform mutation operator is applied on produced offspring ( O ) with mutation probability (M p ) to generate O′ . The new offspring O′ is placed in new population. The selection, crossover, and mutation operations will be repeated on current population until the new population is complete. The mathematical analysis of GA is as follows [ 126 ]:
GA dynamically change the search process through the probabilities of crossover and mutation and reached to optimal solution. GA can modify the encoded genes. GA can evaluate multiple individuals and produce multiple optimal solutions. Hence, GA has better global search capability. The offspring produced from crossover of parent chromosomes is probable to abolish the admirable genetic schemas parent chromosomes and crossover formula is defined as [ 126 ]:
where g is the number of generations, and G is the total number of evolutionary generation set by population. It is observed from Eq.( 1 ) that R is dynamically changed and increase with increase in number of evolutionary generation. In initial stage of GA, the similarity between individuals is very low. The value of R should be low to ensure that the new population will not destroy the excellent genetic schema of individuals. At the end of evolution, the similarity between individuals is very high as well as the value of R should be high.
According to Schema theorem, the original schema has to be replaced with modified schema. To maintain the diversity in population, the new schema keep the initial population during the early stage of evolution. At the end of evolution, the appropriate schema will be produced to prevent any distortion of excellent genetic schema [ 65 , 75 ]. Algorithm 1 shows the pseudocode of classical genetic algorithm.
Algorithm 1: Classical Genetic Algorithm (GA)

Genetic operators
GAs used a variety of operators during the search process. These operators are encoding schemes, crossover, mutation, and selection. Figure Figure2 2 depicts the operators used in GAs.

Operators used in GA
Encoding schemes
For most of the computational problems, the encoding scheme (i.e., to convert in particular form) plays an important role. The given information has to be encoded in a particular bit string [ 121 , 183 ]. The encoding schemes are differentiated according to the problem domain. The well-known encoding schemes are binary, octal, hexadecimal, permutation, value-based, and tree.
Binary encoding is the commonly used encoding scheme. Each gene or chromosome is represented as a string of 1 or 0 [ 187 ]. In binary encoding, each bit represents the characteristics of the solution. It provides faster implementation of crossover and mutation operators. However, it requires extra effort to convert into binary form and accuracy of algorithm depends upon the binary conversion. The bit stream is changed according the problem. Binary encoding scheme is not appropriate for some engineering design problems due to epistasis and natural representation.
In octal encoding scheme, the gene or chromosome is represented in the form of octal numbers (0–7). In hexadecimal encoding scheme, the gene or chromosome is represented in the form of hexadecimal numbers (0–9, A-F) [ 111 , 125 , 187 ]. The permutation encoding scheme is generally used in ordering problems. In this encoding scheme, the gene or chromosome is represented by the string of numbers that represents the position in a sequence. In value encoding scheme, the gene or chromosome is represented using string of some values. These values can be real, integer number, or character [ 57 ]. This encoding scheme can be helpful in solving the problems in which more complicated values are used. As binary encoding may fail in such problems. It is mainly used in neural networks for finding the optimal weights.
In tree encoding, the gene or chromosome is represented by a tree of functions or commands. These functions and commands can be related to any programming language. This is very much similar to the representation of repression in tree format [ 88 ]. This type of encoding is generally used in evolving programs or expressions. Table Table2 2 shows the comparison of different encoding schemes of GA.
Comparison of different encoding schemes
Selection techniques
Selection is an important step in genetic algorithms that determines whether the particular string will participate in the reproduction process or not. The selection step is sometimes also known as the reproduction operator [ 57 , 88 ]. The convergence rate of GA depends upon the selection pressure. The well-known selection techniques are roulette wheel, rank, tournament, boltzmann, and stochastic universal sampling.
Roulette wheel selection maps all the possible strings onto a wheel with a portion of the wheel allocated to them according to their fitness value. This wheel is then rotated randomly to select specific solutions that will participate in formation of the next generation [ 88 ]. However, it suffers from many problems such as errors introduced by its stochastic nature. De Jong and Brindle modified the roulette wheel selection method to remove errors by introducing the concept of determinism in selection procedure. Rank selection is the modified form of Roulette wheel selection. It utilizes the ranks instead of fitness value. Ranks are given to them according to their fitness value so that each individual gets a chance of getting selected according to their ranks. Rank selection method reduces the chances of prematurely converging the solution to a local minima [ 88 ].
Tournament selection technique was first proposed by Brindle in 1983. The individuals are selected according to their fitness values from a stochastic roulette wheel in pairs. After selection, the individuals with higher fitness value are added to the pool of next generation [ 88 ]. In this method of selection, each individual is compared with all n-1 other individuals if it reaches the final population of solutions [ 88 ]. Stochastic universal sampling (SUS) is an extension to the existing roulette wheel selection method. It uses a random starting point in the list of individuals from a generation and selects the new individual at evenly spaced intervals [ 3 ]. It gives equal chance to all the individuals in getting selected for participating in crossover for the next generation. Although in case of Travelling Salesman Problem, SUS performs well but as the problem size increases, the traditional Roulette wheel selection performs relatively well [ 180 ].
Boltzmann selection is based on entropy and sampling methods, which are used in Monte Carlo Simulation. It helps in solving the problem of premature convergence [ 118 ]. The probability is very high for selecting the best string, while it executes in very less time. However, there is a possibility of information loss. It can be managed through elitism [ 175 ]. Elitism selection was proposed by K. D. Jong (1975) for improving the performance of Roulette wheel selection. It ensures the elitist individual in a generation is always propagated to the next generation. If the individual having the highest fitness value is not present in the next generation after normal selection procedure, then the elitist one is also included in the next generation automatically [ 88 ]. The comparison of above-mentioned selection techniques are depicted in Table Table3 3 .
Comparison of different selection techniques
Crossover operators
Crossover operators are used to generate the offspring by combining the genetic information of two or more parents. The well-known crossover operators are single-point, two-point, k-point, uniform, partially matched, order, precedence preserving crossover, shuffle, reduced surrogate and cycle.
In a single point crossover, a random crossover point is selected. The genetic information of two parents which is beyond that point will be swapped with each other [ 190 ]. Figure Figure3 3 shows the genetic information after swapping. It replaced the tail array bits of both the parents to get the new offspring.

Swapping genetic information after a crossover point
In a two point and k-point crossover, two or more random crossover points are selected and the genetic information of parents will be swapped as per the segments that have been created [ 190 ]. Figure Figure4 4 shows the swapping of genetic information between crossover points. The middle segment of the parents is replaced to generate the new offspring.

Swapping genetic information between crossover points
In a uniform crossover, parent cannot be decomposed into segments. The parent can be treated as each gene separately. We randomly decide whether we need to swap the gene with the same location of another chromosome [ 190 ]. Figure Figure5 5 depicts the swapping of individuals under uniform crossover operation.

Swapping individual genes
Partially matched crossover (PMX) is the most frequently used crossover operator. It is an operator that performs better than most of the other crossover operators. The partially matched (mapped) crossover was proposed by D. Goldberg and R. Lingle [ 66 ]. Two parents are choose for mating. One parent donates some part of genetic material and the corresponding part of other parent participates in the child. Once this process is completed, the left out alleles are copied from the second parent [ 83 ]. Figure Figure6 6 depicts the example of PMX.

Partially matched crossover (PMX) [ 117 ]
Order crossover (OX) was proposed by Davis in 1985. OX copies one (or more) parts of parent to the offspring from the selected cut-points and fills the remaining space with values other than the ones included in the copied section. The variants of OX are proposed by different researchers for different type of problems. OX is useful for ordering problems [ 166 ]. However, it is found that OX is less efficient in case of Travelling Salesman Problem [ 140 ]. Precedence preserving crossover (PPX) preserves the ordering of individual solutions as present in the parent of offspring before the application of crossover. The offspring is initialized to a string of random 1’s and 0’s that decides whether the individuals from both parents are to be selected or not. In [ 169 ], authors proposed a modified version of PPX for multi-objective scheduling problems.
Shuffle crossover was proposed by Eshelman et al. [ 20 ] to reduce the bias introduced by other crossover techniques. It shuffles the values of an individual solution before the crossover and unshuffles them after crossover operation is performed so that the crossover point does not introduce any bias in crossover. However, the utilization of this crossover is very limited in the recent years. Reduced surrogate crossover (RCX) reduces the unnecessary crossovers if the parents have the same gene sequence for solution representations [ 20 , 139 ]. RCX is based on the assumption that GA produces better individuals if the parents are sufficiently diverse in their genetic composition. However, RCX cannot produce better individuals for those parents that have same composition. Cycle crossover was proposed by Oliver [ 140 ]. It attempts to generate an offspring using parents where each element occupies the position by referring to the position of their parents [ 140 ]. In the first cycle, it takes some elements from the first parent. In the second cycle, it takes the remaining elements from the second parent as shown in Fig. 7 .

Cycle Crossover (CX) [ 140 ]
Table Table4 4 shows the comparison of crossover techniques. It is observed from Table Table4 4 that single and k-point crossover techniques are easy to implement. Uniform crossover is suitable for large subsets. Order and cycle crossovers provide better exploration than the other crossover techniques. Partially matched crossover provides better exploration. The performance of partially matched crossover is better than the other crossover techniques. Reduced surrogate and cycle crossovers suffer from premature convergence.
Comparison of different crossover techniques
Mutation operators
Mutation is an operator that maintains the genetic diversity from one population to the next population. The well-known mutation operators are displacement, simple inversion, and scramble mutation. Displacement mutation (DM) operator displaces a substring of a given individual solution within itself. The place is randomly chosen from the given substring for displacement such that the resulting solution is valid as well as a random displacement mutation. There are variants of DM are exchange mutation and insertion mutation. In Exchange mutation and insertion mutation operators, a part of an individual solution is either exchanged with another part or inserted in another location, respectively [ 88 ].
The simple inversion mutation operator (SIM) reverses the substring between any two specified locations in an individual solution. SIM is an inversion operator that reverses the randomly selected string and places it at a random location [ 88 ]. The scramble mutation (SM) operator places the elements in a specified range of the individual solution in a random order and checks whether the fitness value of the recently generated solution is improved or not [ 88 ]. Table Table5 5 shows the comparison of different mutation techniques.
Comparison of different mutation operators
Table Table6 6 shows the best combination of encoding scheme, mutation, and crossover techniques. It is observed from Table Table6 6 that uniform and single-point crossovers can be used with most of encoding and mutation operators. Partially matched crossover is used with inversion mutation and permutation encoding scheme provides the optimal solution.
Best combination of various operators under optimal Environment
Variants of GA
Various variants of GA’s have been proposed by researchers. The variants of GA are broadly classified into five main categories namely, real and binary coded, multiobjective, parallel, chaotic, and hybrid GAs. The pros and cons of these algorithms with their application has been discussed in the preceding subsections.
Real and binary coded GAs
Based on the representation of chromosomes, GAs are categorized in two classes, namely binary and real coded GAs.
Binary coded GAs
The binary representation was used to encode GA and known as binary GA. The genetic operators were also modified to carry out the search process. Payne and Glen [ 153 ] developed a binary GA to identify the similarity among molecules. They used binary representation for position of molecule and their conformations. However, this method has high computational complexity. Longyan et al. [ 203 ] investigated three different method for wind farm design using binary GA (BGA). Their method produced better fitness value and farm efficiency. Shukla et al. [ 185 ] utilized BGA for feature subset selection. They used mutual information maximization concept for selecting the significant features. BGAs suffer from Hamming cliffs, uneven schema, and difficulty in achieving precision [ 116 , 199 ].
Real-coded GAs
Real-coded GAs (RGAs) have been widely used in various real-life applications. The representation of chromosomes is closely associated with real-life problems. The main advantages of RGAs are robust, efficient, and accurate. However, RGAs suffer from premature convergence. Researchers are working on RGAs to improve their performance. Most of RGAs are developed by modifying the crossover, mutation and selection operators.
The searching capability of crossover operators are not satisfactory for continuous search space. The developments in crossover operators have been done to enhance their performance in real environment. Wright [ 210 ] presented a heuristics crossover that was applied on parents to produce off-spring. Michalewicz [ 135 ] proposed arithmetical crossover operators for RGAs. Deb and Agrawal [ 34 ] developed a real-coded crossover operator, which is based on characteristics of single-point crossover in BGA. The developed crossover operator named as simulated binary crossover (SBX). SBX is able to overcome the Hamming cliff, precision, and fixed mapping problem. The performance of SBX is not satisfactory in two-variable blocked function. Eshelman et al. [ 53 ] utilized the schemata concept to design the blend crossover for RGAs. The unimodal normal distribution crossover operator (UNDX) was developed by Ono et al. [ 144 ]. They used ellipsoidal probability distribution to generate the offspring. Kita et al. [ 106 ] presented a multi-parent UNDX (MP-UNDX), which is the extension of [ 144 ]. However, the performance of RGA with MP-UNDX is much similar to UNDX. Deep and Thakur [ 39 ] presented a Laplace crossover for RGAs, which is based on Laplacian distribution. Chuang et al. [ 27 ] developed a direction based crossover to further explore the all possible search directions. However, the search directions are limited. The heuristic normal distribution crossover operator was developed by Wang et al. [ 207 ]. It generates the cross-generated offspring for better search operation. However, the better individuals are not considered in this approach. Subbaraj et al. [ 192 ] proposed Taguchi self-adaptive RCGA. They used Taguchi method and simulated binary crossover to exploit the capable offspring.
Mutation operators generate diversity in the population. The two main challenges have to tackle during the application of mutation. First, the probability of mutation operator that was applied on population. Second, the outlier produced in chromosome after mutation process. Michalewicz [ 135 ] presented uniform and non-uniform mutation operators for RGAs. Michalewicz and Schoenauer [ 136 ] developed a special case of uniform mutation. They developed boundary mutation. Deep and Thakur [ 38 ] presented a novel mutation operator based on power law and named as power mutation. Das and Pratihar [ 30 ] presented direction-based exponential mutation operator. They used direction information of variables. Tang and Tseng [ 196 ] presented a novel mutation operator for enhancing the performance of RCGA. Their approach was fast and reliable. However, it stuck in local optima for some applications. Deb et al. [ 35 ] developed polynomial mutation that was used in RCGA. It provides better exploration. However, the convergence speed is slow and stuck in local optima. Lucasius et al. [ 129 ] proposed a real-coded genetic algorithm (RCGA). It is simple and easy to implement. However, it suffers from local optima problem. Wang et al. [ 205 ] developed multi-offspring GA and investigated their performance over single point crossover. Wang et al. [ 206 ] stated the theoretical basis of multi-offspring GA. The performance of this method is better than non-multi-offspring GA. Pattanaik et al. [ 152 ] presented an improvement in the RCGA. Their method has better convergence speed and quality of solution. Wang et al. [ 208 ] proposed multi-offspring RCGA with direction based crossover for solving constrained problems.
Table Table7 7 shows the mathematical formulation of genetic operators in RGAs.
Mathematical formulation of genetic operators in RGAs
Multiobjective GAs
Multiobjective GA (MOGA) is the modified version of simple GA. MOGA differ from GA in terms of fitness function assignment. The remaining steps are similar to GA. The main motive of multiobjective GA is to generate the optimal Pareto Front in the objective space in such a way that no further enhancement in any fitness function without disturbing the other fitness functions [ 123 ]. Convergence, diversity, and coverage are main goal of multiobjective GAs. The multiobjective GAs are broadly categorized into two categories namely, Pareto-based, and decomposition-based multiobjective GAs [ 52 ]. These techniques are discussed in the preceding subsections.
Pareto-based multi-objective GA
The concept of Pareto dominance was introduced in multiobjective GAs. Fonseca and Fleming [ 56 ] developed first multiobjective GA (MOGA). The niche and decision maker concepts were proposed to tackle the multimodal problems. However, MOGA suffers from parameter tuning problem and degree of selection pressure. Horn et al. [ 80 ] proposed a niched Pareto genetic algorithm (NPGA) that utilized the concept of tournament selection and Pareto dominance. Srinivas and Deb [ 191 ] developed a non-dominated sorting genetic algorithm (NSGA). However, it suffers from lack of elitism, need of sharing parameter, and high computation complexity. To alleviate these problems, Deb et al. [ 36 ] developed a fast elitist non-dominated sorting genetic algorithm (NSGA-II). The performance of NSGA-II may be deteriorated for many objective problems. NSGA-II was unable to maintain the diversity in Pareto-front. To alleviate this problem, Luo et al. [ 130 ] introduced a dynamic crowding distance in NSGA-II. Coello and Pulido [ 28 ] developed a multiobjective micro GA. They used an archive for storing the non-dominated solutions. The performance of Pareto-based approaches may be deteriorated in many objective problems [ 52 ].
Decomposition-based multiobjective GA
Decomposition-based MOGAs decompose the given problem into multiple subproblems. These subproblems are solved simultaneously and exchange the solutions among neighboring subproblems [ 52 ]. Ishibuchi and Murata [ 84 ] developed a multiobjective genetic local search (MOGLS). In MOGLS, the random weights were used to select the parents and local search for their offspring. They used generation replacement and roulette wheel selection method. Jaszkiewicz [ 86 ] modified the MOGLS by utilizing different selection mechanisms for parents. Murata and Gen [ 141 ] proposed a cellular genetic algorithm for multiobjective optimization (C-MOGA) that was an extension of MOGA. They added cellular structure in MOGA. In C-MOGA, the selection operator was performed on the neighboring of each cell. C-MOGA was further extended by introducing an immigration procedure and known as CI-MOGA. Alves and Almeida [ 11 ] developed a multiobjective Tchebycheffs-based genetic algorithm (MOTGA) that ensures convergence and diversity. Tchebycheff scalar function was used to generate non-dominated solution set. Patel et al. [ 151 ] proposed a decomposition based MOGA (D-MOGA). They integrated opposition based learning in D-MOGA for weight vector generation. D-MOGA is able to maintain the balance between diversity of solutions and exploration of search space.
Parallel GAs
The motivation behind the parallel GAs is to improve the computational time and quality of solutions through distributed individuals. Parallel GAs are categorized into three broad categories such as master-slave parallel GAs, fine grained parallel GAs, and multi-population coarse grained parallel Gas [ 70 ]. In master-slave parallel GA, the computation of fitness functions is distributed over the several processors. In fine grained GA, parallel computers are used to solve the real-life problems. The genetic operators are bounded to their neighborhood. However, the interaction is allowed among the individuals. In coarse grained GA, the exchange of individuals among sub-populations is performed. The control parameters are also transferred during migration. The main challenges in parallel GAs are to maximize memory bandwidth and arrange threads for utilizing the power of GPUs [ 23 ]. Table Table8 8 shows the comparative analysis of parallel GAs in terms of hardware and software. The well-known parallel GAs are studied in the preceding subsections.
Analysis of parallel GAs in terms of hardware and software
Master slave parallel GA
The large number of processors are utilized in master-slave parallel GA (MS-PGA) as compared to other approaches. The computation of fitness functions may be increased by increasing the number of processors. Hong et al. [ 79 ] used MS-PGA for solving data mining problems. Fuzzy rules are used with parallel GA. The evaluation of fitness function was performed on slave machines. However, it suffers from high computational time. Sahingzo [ 174 ] implemented MS-PGA for UAV path finding problem. The genetic operators were executed on processors. They used multicore CPU with four cores. Selection and fitness evaluation was done on slave machines. MS-PGA was applied on traffic assignment problem in [ 127 ]. They used thirty processors to solve this problem at National University of Singapore. Yang et al. [ 213 ] developed a web-based parallel GA. They implemented the master slave version of NSGA-II in distributed environment. However, the system is complex in nature.
Fine grained parallel GA
In last few decades, researchers are working on migration policies of fine grained parallel GA (FG-PGA). Porta et al. [ 161 ] utilized clock-time for migration frequency, which is independent of generations. They used non-uniform structure and static configuration. The best solution was selected for migration and worst solution was replaced with migrant solution. Kurdi [ 115 ] used adaptive migration frequency. The migration procedure starts until there is no change in the obtained solutions after ten successive generations. The non-uniform and dynamic structure was used. In [ 209 ], local best solutions were synchronized and formed a global best solutions. The global best solutions were transferred to all processors for father execution. The migration frequency depends upon the number of generation. They used uniform structure with fixed configuration. Zhang et al. [ 220 ] used parallel GA to solve the set cover problem of wireless networks. They used divide-and-conquer strategy to decompose the population into sub-populations. Thereafter, the genetic operators were applied on local solutions and Kuhn-Munkres was used to merge the local solutions.
Coarse grained parallel GA
Pinel et al. [ 158 ] proposed a GraphCell. The population was initialized with random values and one solution was initialized with Min-min heuristic technique. 448 processors were used to implement the proposed approach. However, coarse grained parallel GAs are less used due to complex in nature. The hybrid parallel GAs are widely used in various applications. Shayeghi et al. [ 182 ] proposed a pool-based Birmingham cluster GA. Master node was responsible for managing global population. Slave node selected the solutions from global population and executed it. 240 processors are used for computation. Roberge et al. [ 170 ] used hybrid approach to optimize switching angle of inverters. They used four different strategies for fitness function computation. Nowadays, GPU, cloud, and grid are most popular hardware for parallel GAs [ 198 ].
Chaotic GAs
The main drawback of GAs is premature convergence. The chaotic systems are incorporated into GAs to alleviate this problem. The diversity of chaos genetic algorithm removes premature convergence. Crossover and mutation operators can be replaced with chaotic maps. Tiong et al. [ 197 ] integrated the chaotic maps into GA for further improvement in accuracy. They used six different chaotic maps. The performance of Logistic, Henon and Ikeda chaotic GA performed better than the classical GA. However, these techniques suffer from high computational complexity. Ebrahimzadeh and Jampour [ 48 ] used Lorenz chaotic for genetic operators of GA to eliminate the local optima problem. However, the proposed approach was unable to find relationship between entropy and chaotic map. Javidi and Hosseinpourfard [ 87 ] utilized two chaotic maps namely logistic map and tent map for generating chaotic values instead of random selection of initial population. The proposed chaotic GA performs better than the GA. However, this method suffers from high computational complexity. Fuertes et al. [ 60 ] integrated the entropy into chaotic GA. The control parameters are modified through chaotic maps. They investigated the relationship between entropy and performance optimization.
Chaotic systems have also used in multiobjective and hybrid GAs. Abo-Elnaga and Nasr [ 5 ] integrated chaotic system into modified GA for solving Bi-level programming problems. Chaotic helps the proposed algorithm to alleviate local optima and enhance the convergence. Tahir et al. [ 193 ] presented a binary chaotic GA for feature selection in healthcare. The chaotic maps were used to initialize the population and modified reproduction operators were applied on population. Xu et al. [ 115 ] proposed a chaotic hybrid immune GA for spectrum allocation. The proposed approach utilizes the advantages of both chaotic and immune operator. However, this method suffers from parameter initialization problem.
Genetic Algorithms can be easily hybridized with other optimization methods for improving their performance such as image denoising methods, chemical reaction optimization, and many more. The main advantages of hybridized GA with other methods are better solution quality, better efficiency, guarantee of feasible solutions, and optimized control parameters [ 51 ]. It is observed from literature that the sampling capability of GAs is greatly affected from population size. To resolve this problem, local search algorithms such as memetic algorithm, Baldwinian, Lamarckian, and local search have been integrated with GAs. This integration provides proper balance between intensification and diversification. Another problem in GA is parameter setting. Finding appropriate control parameters is a tedious task. The other metaheuristic techniques can be used with GA to resolve this problem. Hybrid GAs have been used to solve the issues mentioned in the preceding subsections [ 29 , 137 , 186 ].
Enhance search capability
GAs have been integrated with local search algorithms to reduce the genetic drift. The explicit refinement operator was introduced in local search for producing better solutions. El-Mihoub et al. [ 54 ] established the effect of probability of local search on the population size of GA. Espinoza et al. [ 50 ] investigated the effect of local search for reducing the population size of GA. Different search algorithms have been integrated with GAs for solving real-life applications.
Generate feasible solutions
In complex and high-dimensional problems, the genetic operators of GA generate infeasible solutions. PMX crossover generates the infeasible solutions for order-based problems. The distance preserving crossover operator was developed to generate feasible solutions for travelling salesman problem [ 58 ]. The gene pooling operator instead of crossover was used to generate feasible solution for data clustering [ 19 ]. Konak and Smith [ 108 ] integrated a cut-saturation algorithm with GA for designing the communication networks. They used uniform crossover to produce feasible solutions.
Replacement of genetic operators
There is a possibility to replace the genetic operators which are mentioned in Section 3.2 with other search techniques. Leng [ 122 ] developed a guided GA that utilizes the penalties from guided local search. These penalties were used in fitness function to improve the performance of GA. Headar and Fukushima [ 74 ] used simplex crossover instead of standard crossover. The standard mutation operator was replaced with simulated annealing in [ 195 ]. The basic concepts of quantum computing are used to improve the performance of GAs. The heuristic crossover and hill-climbing operators can be integrated into GA for solving three-matching problem.
Optimize control parameters
The control parameters of GA play a crucial role in maintaining the balance between intensification and diversification. Fuzzy logic has an ability to estimate the appropriate control parameters of GA [ 167 ]. Beside this, GA can be used to optimize the control parameters of other techniques. GAs have been used to optimize the learning rate, weights, and topology of neutral networks [ 21 ]. GAs can be used to estimate the optimal value of fuzzy membership in controller. It was also used to optimize the control parameters of ACO, PSO, and other metaheuristic techniques [ 156 ]. The comparative analysis of well-known GAs are mentioned in Table Table9 9 .
Comparative study of GA’s variants in terms of pros and cons
Applications
Genetic Algorithms have been applied in various NP-hard problems with high accuracy rates. There are a few application areas in which GAs have been successfully applied.
Operation management
GA is an efficient metaheuristic for solving operation management (OM) problems such as facility layout problem (FLP), supply network design, scheduling, forecasting, and inventory control.
Facility layout
Datta et al. [ 32 ] utilized GA for solving single row facility layout problem (SRFLP). For SRFLP, the modified crossover and mutation operators of GA produce valid solutions. They applied GA to large sized problems that consists of 60–80 instances. However, it suffers from parameter dependency problem. Sadrzadeh [ 173 ] proposed GA for multi-line FLP have multi products. The facilities were clustered using mutation and heuristic operators. The total cost obtained from the proposed GA was decreased by 7.2% as compared to the other algorithms. Wu et al. [ 211 ] implemented hierarchical GA to find out the layout of cellular manufacturing system. However, the performance of GA is greatly affected from the genetic operators. Aiello et al. [ 7 ] proposed MOGA for FLP. They used MOGA on the layout of twenty different departments. Palomo-Romero et al. [ 148 ] proposed an island model GA to solve the FLP. The proposed technique maintains the population diversity and generates better solutions than the existing techniques. However, this technique suffers from improper migration strategy that can be utilized for improving the population. GA and its variants has been successfully applied on FLP [ 103 , 119 , 133 , 201 ].
GA shows the superior performance for solving the scheduling problems such as job-shop scheduling (JSS), integrated process planning and scheduling (IPPS), etc. [ 119 ]. To improve the performance in the above-mentioned areas of scheduling, researchers developed various genetic representation [ 12 , 159 , 215 ], genetic operators, and hybridized GA with other methods [ 2 , 67 , 147 , 219 ].
Inventory control
Besides the scheduling, inventory control plays an important role in OM. Backordering and lost sales are two main approaches for inventory control [ 119 ]. Hiassat et al. [ 76 ] utilized the location-inventory model to find out the number and location of warehouses. Various design constraints have been added in the objective functions of GA and its variants for solving inventory control problem [].
Forecasting and network design
Forecasting is an important component for OM. Researchers are working on forecasting of financial trading, logistics demand, and tourist arrivals. GA has been hybridized with support vector regression, fuzzy set, and neural network (NN) to improve their forecasting capability [ 22 , 78 , 89 , 178 , 214 ]. Supply network design greatly affect the operations planning and scheduling. Most of the research articles are focused on capacity constraints of facilities [ 45 , 184 ]. Multi-product multi-period problems increases the complexity of supply networks. To resolve the above-mentioned problem, GA has been hybridized with other techniques [ 6 , 45 , 55 , 188 , 189 ]. Multi-objective GAs are also used to optimize the cost, profit, carbon emissions, etc. [ 184 , 189 ].
GAs have been applied in various fields of multimedia. Some of well-known multimedia fields are encryption, image processing, video processing, medical imaging, and gaming.
Information security
Due to development in multimedia applications, images, videos and audios are transferred from one place to another over Internet. It has been found in literature that the images are more error prone during the transmission. Therefore, image protection techniques such as encryption, watermarking and cryptography are required. The classical image encryption techniques require the input parameters for encryption. The wrong selection of input parameters will generate inadequate encryption results. GA and its variants have been used to select the appropriate control parameters. Kaur and Kumar [ 96 ] developed a multi-objective genetic algorithm to optimize the control parameters of chaotic map. The secret key was generated using beta chaotic map. The generated key was use to encrypt the image. Parallel GAs were also used to encrypt the image [ 97 ].
Image processing
The main image processing tasks are preprocessing, segmentation, object detection, denoising, and recognition. Image segmentation is an important step to solve the image processing problems. Decomposing/partitioning an image requires high computational time. To resolve this problem, GA is used due to their better search capability [ 26 , 102 ]. Enhancement is a technique to improve the quality and contrast of an image. The better image quality is required to analyze the given image. GAs have been used to enhance natural contrast and magnify image [ 40 , 64 , 99 ]. Some researchers are working on hybridization of rough set with adaptive genetic algorithm to merge the noise and color attributes. GAs have been used to remove the noise from the given image. GA can be hybridized with fuzzy logic to denoise the noisy image. GA based restoration technique can be used to remove haze, fog and smog from the given image [ 8 , 110 , 146 , 200 ]. Object detection and recognition is a challenging issue in real-world problem. Gaussian mixture model provides better performance during detection and recognition process. The control parameters are optimized through GA [ 93 ].
Video processing
Video segmentation has been widely used in pattern recognition, and computer vision. There are some critical issues that are associated with video segmentation. These are distinguishing object from the background and determine accurate boundaries. GA can be used to resolve these issues [ 9 , 105 ]. GAs have been implemented for gesture recognition successfully by Chao el al. [ 81 ] used GA for gesture recognition. They applied GAs and found an accuracy of 95% in robot vision. Kaluri and Reddy [ 91 ] proposed an adaptive genetic algorithm based method along with fuzzy classifiers for sign gesture recognition. They reported an improved recognition rate of 85% as compared to the existing method that provides 79% accuracy. Beside the gesture recognition, face recognition play an important role in criminal identification, unmanned vehicles, surveillance, and robots. GA is able to tackle the occlusion, orientations, expressions, pose, and lighting condition [ 69 , 95 , 109 ].
Medical imaging
Genetic algorithms have been applied in medical imaging such as edge detection in MRI and pulmonary nodules detection in CT scan images [ 100 , 179 ]. In [ 120 ], authors used a template matching technique with GA for detecting nodules in CT images. Kavitha and Chellamuthu [ 179 ] used GA based region growing method for detecting the brain tumor. GAs have been applied on medical prediction problems captured from pathological subjects. Sari and Tuna [ 176 ] used GA used to solve issues arises in biomechanics. It is used to predict pathologies during examination. Ghosh and Bhattachrya [ 62 ] implemented sequential GA with cellular automata for modelling the coronavirus disease 19 (COVID-19) data. GAs can be applied in parallel mode to find rules in biological datasets [ 31 ]. The authors proposed a parallel GA that runs by dividing the process into small sub-generations and evaluating the fitness of each individual solution in parallel. Genetic algorithms are used in medicine and other related fields. Koh et al. [ 61 ] proposed a genetic algorithm based method for evaluation of adverse effects of a given drug.
Precision agriculture
GAs have been applied on various problems that are related to precision agriculture. The main issues are crop yield, weed detection, and improvement in farming equipment. Pachepsky and Acock [ 145 ] implemented GA to analyze the water capacity in soil using remote sensing images. The crop yield can be predicted through the capacity of water present in soil. The weed identification was done through GA in [ 142 ]. They used aerial image for classification of plants. In [ 124 ], color image segmentation was used to discriminate the weed and plant. Peerlink et al. [ 154 ] determined the appropriate rate of fertilizer for various portions of agriculture field. They GA for determining the nitrogen in wheat field. The energy requirements in water irrigation systems can be optimized by viewing it as a multi-objective optimization problem. The amount of irrigation required and thus power requirements change continuously in a SMART farm. Therefore, GA can be applied in irrigation systems to reduce the power requirements [ 33 ].
GAs have been successfully used in games such as gomoku. In [ 202 ], the authors shown that the GA based approach finds the solution having the highest fitness than the normal tree based methods. However, in real-time strategy based games, GA based solutions become less practical to implement [ 82 ]. GAs have been implemented for path planning problems considering the environment constraints as well as avoiding the obstacles to reach the given destination. Burchardt and Salomon [ 18 ] described an implementation for path planning for soccer games. GA can encode the path planning problems via the coordinate points of a two-dimensional playing field, hence resulting in a variable length solution. The fitness function in path planning considers length of path as well as the collision avoiding terms for soccer players.
Wireless networking
Due to adaptive, scalable, and easy implementation of GA, it has been used to solve the various issues of wireless networking. The main issues of wireless networking are routing, quality of service, load balancing, localization, bandwidth allocation and channel assignment [ 128 , 134 ]. GA has been hybridized with other metaheuristics for solving the routing problems. Hybrid GA not only producing the efficient routes among pair of nodes, but also used for load balancing [ 24 , 212 ].
Load balancing
Nowadays, multimedia applications require Quality-of-Service (QoS) demand for delay and bandwidth. Various researchers are working on GAs for QoS based solutions.GA produces optimal solutions for complex networks [ 49 ]. Roy et al. [ 172 ] proposed a multi-objective GA for multicast QoS routing problem. GA was used with ACO and other search algorithms for finding optimal routes with desired QoS metrics. Load balancing is another issue in wireless networks. Scully and Brown [ 177 ] used MicroGAs and MacroGAs to distribute the load among various components of networks. He et al. [ 73 ] implemented GA to determine the balance load in wireless sensor networks. Cheng et al. [ 25 ] utilized distributed GA with multi-population scheme for load balancing. They used load balancing metric as a fitness function in GA.
Localization
The process of determining the location of wireless nodes is called as localization. It plays an important role in disaster management and military services. Yun et al. [ 216 ] used GA with fuzzy logic to find out the weights, which are assigned according to the signal strength. Zhang et al. [ 218 ] hybridized GA with simulated annealing (SA) to determine the position of wireless nodes. SA is used as local search to eliminate the premature convergence.
Bandwidth and channel allocation
The appropriate bandwidth allocation is a complex task. GAs and its variants have been developed to solve the bandwidth allocation problem [ 92 , 94 , 107 ]. GAs were used to investigate the allocation of bandwidth with QoS constraints. The fitness function of GAs may consists of resource utilization, bandwidth distribution, and computation time [ 168 ]. The channel allocation is an important issue in wireless networks. The main objective of channel allocation is to simultaneously optimize the number of channels and reuse of allocated frequency. Friend et al. [ 59 ] used distributed island GA to resolve the channel allocation problem in cognitive radio networks. Zhenhua et al. [ 221 ] implemented a modified immune GA for channel assignment. They used different encoding scheme and immune operators. Pinagapany and Kulkarni [ 157 ] developed a parallel GA to solve both static and dynamic channel allocation problem. They used decimal encoding scheme. Table Table10 10 summarizes the applications of GA and its variants.
Applications of GA
Challenges and future possibilities
In this section, the main challenges faced during the implementation of GAs are discussed followed by the possible research directions.
Despite the several advantages, there are some challenges that need to be resolved for future advancements and further evolution of genetic algorithms. Some major challenges are given below:
Selection of initial population
Initial population is always considered as an important factor for the performance of genetic algorithms. The size of population also affects the quality of solution [ 160 ]. The researchers argue that if a large population is considered, then the algorithm takes more computation time. However, the small population may lead to poor solution [ 155 ]. Therefore, finding the appropriate population size is always a challenging issue. Harik and Lobo [ 71 ] investigated the population using self-adaption method. They used two approaches such as (1) use of self-adaption prior to execution of algorithm, in which the size of population remains the same and (2) in which the self-adaption used during the algorithm execution where the population size is affected by fitness function.
Premature convergence
Premature convergence is a common issue for GA. It can lead to the loss of alleles that makes it difficult to identify a gene [ 15 ]. Premature convergence states that the result will be suboptimal if the optimization problem coincides too early. To avoid this issue, some researchers suggested that the diversity should be used. The selection pressure should be used to increase the diversity. Selection pressure is a degree which favors the better individuals in the initial population of GA’s. If selection pressure (SP1) is greater than some selection pressure (SP2), then population using SP1 should be larger than the population using SP2. The higher selection pressure can decrease the population diversity that may lead to premature convergence [ 71 ].
Convergence property has to be handled properly so that the algorithm finds global optimal solution instead of local optimal solution (see Fig. Fig.8). 8 ). If the optimal solution lies in the vicinity of an infeasible solution, then the global nature of GA can be combined with local nature of other algorithms such as Tabu search and local search. The global nature of genetic algorithms and local nature of Tabu search provide the proper balance between intensification and diversification.

Local and global optima [ 149 ]
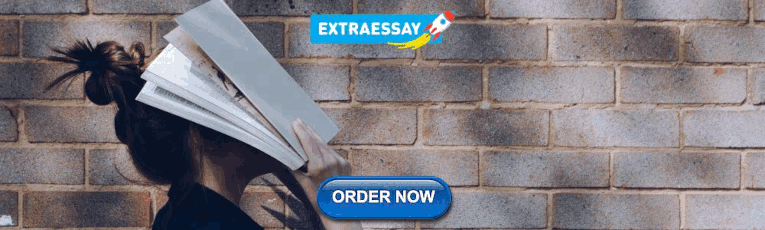
Selection of efficient fitness functions
Fitness function is the driving force, which plays an important role in selecting the fittest individual in every iteration of an algorithm. If the number of iterations are small, then a costly fitness function can be adjusted. The number of iterations increases may increase the computational cost. The selection of fitness function depends upon the computational cost as well as their suitability. In [ 46 ], the authors used Davies-Bouldin index for classification of documents.
Degree of mutation and crossover
Crossover and mutation operators are the integral part of GAs. If the mutation is not considered during evolution, then there will be no new information available for evolution. If crossover is not considered during evolution, then the algorithm can result in local optima. The degree of these operators greatly affect the performance of GAs [ 72 ]. The proper balance between these operators are required to ensure the global optima. The probabilistic nature cannot determine the exact degree for an effective and optimal solution.
Selection of encoding schemes
GAs require a particular encoding scheme for a specific problem. There is no general methodology for deciding whether the particular encoding scheme is suitable for any type of real-life problem. If there are two different problems, then two different encoding schemes are required. Ronald [ 171 ] suggested that the encoding schemes should be designed to overwhelm the redundant forms. The genetic operators should be implemented in a manner that they are not biased towards the redundant forms.
Future research directions
GAs have been applied in different fields by modifying the basic structure of GA. The optimality of a solution obtained from GA can be made better by overcoming the current challenges. Some future possibilities for GA are as follows:
- There should be some way to choose the appropriate degree of crossover and mutation operators. For example Self-Organizing GA adapt the crossover and mutation operators according to the given problem. It can save computation time that make it faster.
- Future work can also be considered for reducing premature convergence problem. Some researchers are working in this direction. However, it is suggested that new methods of crossover and mutation techniques are required to tackle the premature convergence problem.
- Genetic algorithms mimic the natural evolution process. There can be a possible scope for simulating the natural evolution process such as the responses of human immune system and the mutations in viruses.
- In real-life problems, the mapping from genotype to phenotype is complex. In this situation, the problem has no obvious building blocks or building blocks are not adjacent groups of genes. Hence, there is a possibility to develop novel encoding schemes to different problems that does not exhibit same degree of difficulty.
Conclusions
This paper presents the structured and explained view of genetic algorithms. GA and its variants have been discussed with application. Application specific genetic operators are discussed. Some genetic operators are designed for representation. However, they are not applicable to research domains. The role of genetic operators such as crossover, mutation, and selection in alleviating the premature convergence is studied extensively. The applicability of GA and its variants in various research domain has been discussed. Multimedia and wireless network applications were the main attention of this paper. The challenges and issues mentioned in this paper will help the practitioners to carry out their research. There are many advantages of using GAs in other research domains and metaheuristic algorithms.
The intention of this paper is not only provide the source of recent research in GAs, but also provide the information about each component of GA. It will encourage the researchers to understand the fundamentals of GA and use the knowledge in their research problems.
Publisher’s note
Springer Nature remains neutral with regard to jurisdictional claims in published maps and institutional affiliations.
- Headed to a festival or outdoor event soon? Here is what you should know
- Healthcare on wheels: Using mobile clinics to reach children all over Houston
Research method finds new use in diagnosis of genetic disorders
- How the hand muscles have evolved
- Patient’s leg saved by Baylor Medicine surgeon
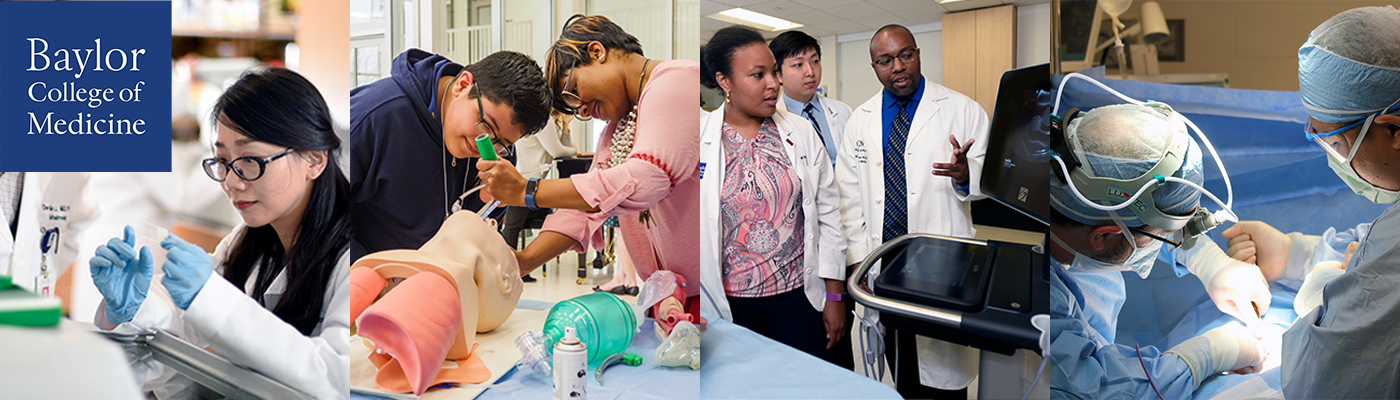
Researchers at Baylor College of Medicine have tested the feasibility of using human cell transdifferentiation with RNA sequencing to facilitate diagnoses of Mendelian disorders. The approach generated an overall diagnostic yield of 25.4% in a cohort of Undiagnosed Diseases Network cases. The findings are published in the American Journal of Human Genetics .
RNA sequencing, which reads the transcriptome or gene expression in a cell, is often needed to support a genetic diagnosis obtained through exome sequencing or whole genome sequencing. However, the effectiveness of RNA sequencing is limited by expression of disease-associated genes in clinically accessible tissues like blood or skin fibroblasts, cells that contribute to the formation of connective tissue.
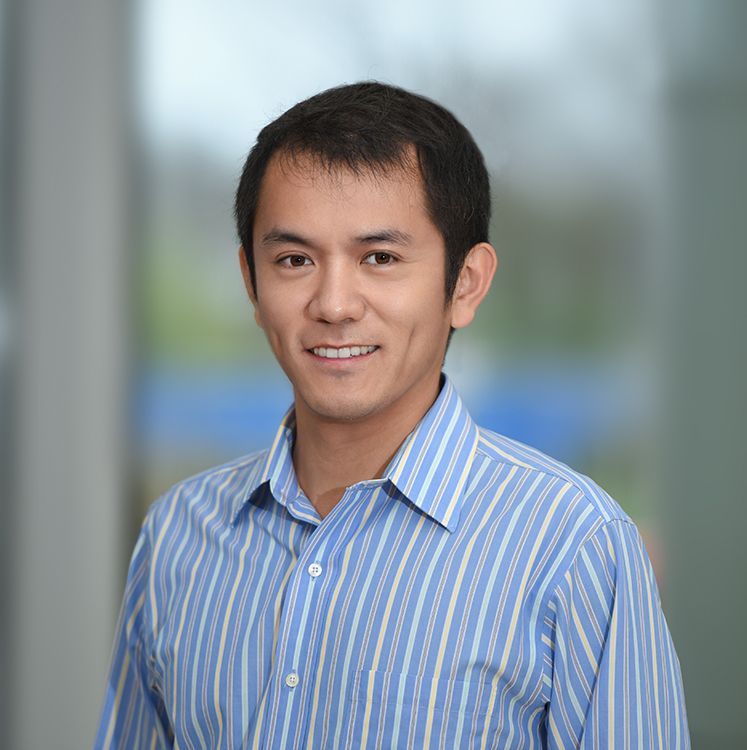
“This is especially problematic in neurological diseases because the gene causing the disorder may not be expressed in blood and skin cells,” said corresponding author Dr. Pengfei Liu , associate professor of molecular and human genetics and director of the ACGME-accredited Laboratory Genetics and Genomics Fellowship Program at Baylor.
Research method helps overcome obstacles to genetic diagnoses
To overcome this challenge, researchers led by first author Dr. Shenglan Li , staff scientist in Liu’s lab at Baylor, converted fibroblasts obtained in skin biopsies to neurons in a process called transdifferentiation. “This method activates neuron-specific gene expression and increases the probability that we can accurately characterize disease-causing mutations in these cells,” Li said.
For clinical validation, researchers generated these induced neurons for a cohort of 71 individuals with neurological characteristics in the Undiagnosed Diseases Network. RNA sequencing of the induced neurons led to a diagnosis in 18 individuals (25.4%); five of those cases could not have been diagnosed with RNA sequencing of fibroblasts alone.
This study shows that fibroblast-to-neuron transdifferentiation followed by RNA sequencing is a simple, low-cost and reproducible approach with a reasonable turnaround time, making it feasible for clinical implementation,” Liu said. “This new testing method represents a paradigm shift in laboratory genetics, moving from the traditional DNA-centric approach to one that focuses on the patient’s cells.”
“It’s exciting to apply this well-established technique, which previously has been used to study mechanisms of neurodegenerative diseases, for a new use in genetic diagnostics,” Li said.
Other authors of this work include Sen Zhao, Jefferson C. Sinson, Aleksandar Bajic, Jill A. Rosenfeld, Matthew B. Neeley, Mezthly Pena, Kim C. Worley, Lindsay C. Burrage, Monika Weisz-Hubshman, Shamika Ketkar, William J. Craigen, Gary D. Clark, Seema Lalani, Carlos A. Bacino, Keren Machol, Hsiao-Tuan Chao, Lorraine Potocki, Lisa Emrick, Jennifer Sheppard, My T.T. Nguyen, Anahita Khoramnia, Paula Patricia Hernandez, Sandesh C. S. Nagamani, Zhandong Liu, Christine M. Eng and Brendan Lee. They are affiliated with one or more of the following institutions: Baylor College of Medicine, Jan and Dan Duncan Neurological Research Institute at Texas Children’s Hospital, Texas Children’s Hospital, McNair Medical Institute at the Robert and Janice McNair Foundation and Baylor Genetics.
The research was supported by the National Institutes of Health Common Fund (U01HG007709 and U01HG007942), the National Human Genome Research Institute (R35HG011311) and the BCM Intellectual and Developmental Disabilities Research Center funded by the Eunice Kennedy Shriver National Institute of Child Health & Human Development (P50HD103555). For a full list of funding sources, see the publication .
By Molly Chiu
Follow From the Labs on X @BCMFromtheLabs and Instagram !
Receive From the Labs via email
Enter your email address to subscribe to this blog and receive notifications of new posts by email.
Email Address
Share this:
- Click to share on Facebook (Opens in new window)
- Click to share on Twitter (Opens in new window)
- Click to share on LinkedIn (Opens in new window)
- Click to email a link to a friend (Opens in new window)
You May Also Like
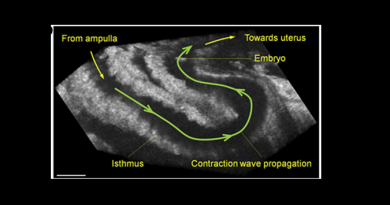
How the egg and the embryo actually travel through the fallopian tube
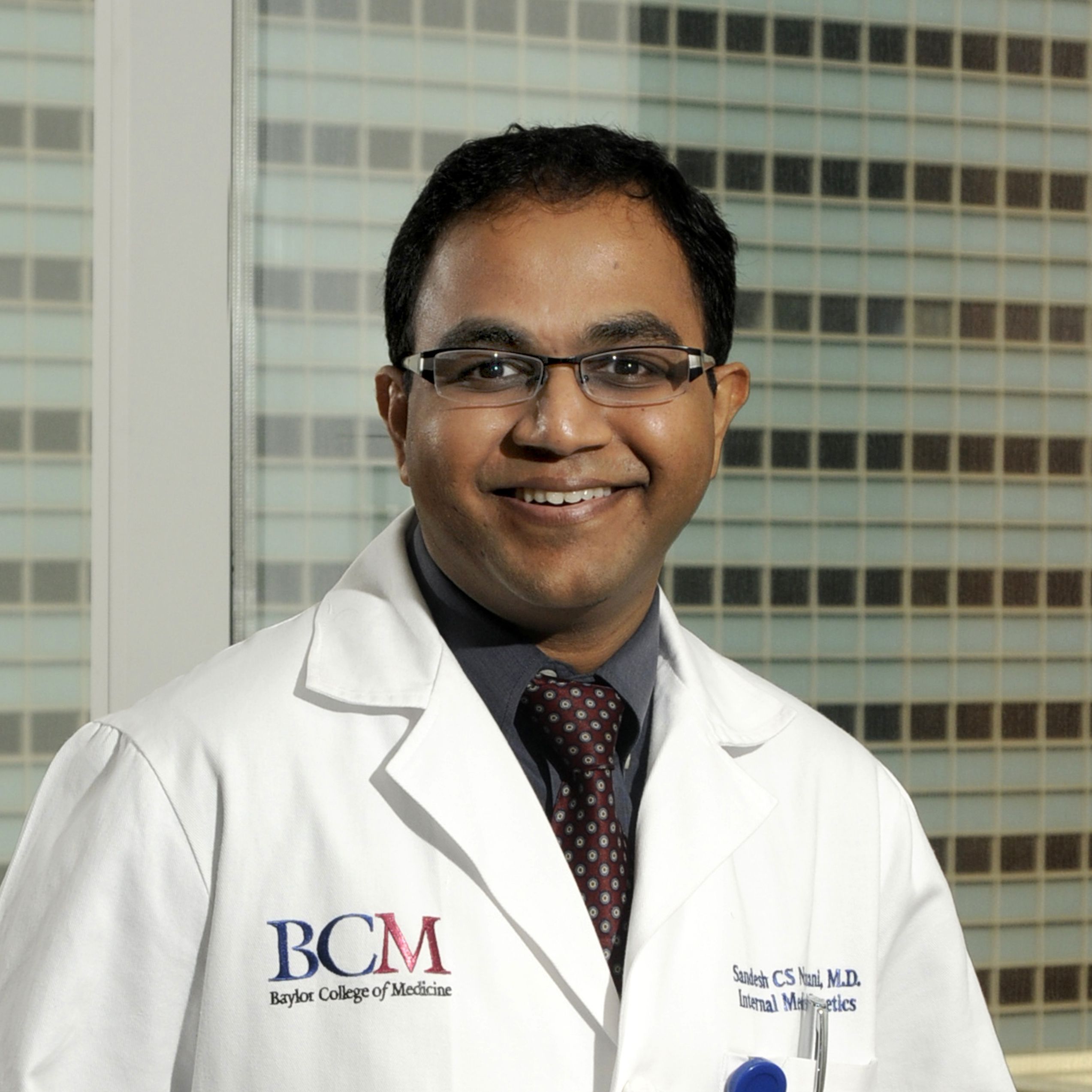
C-section delivery does not decrease at-birth fracture rates in infants with rare bone disease
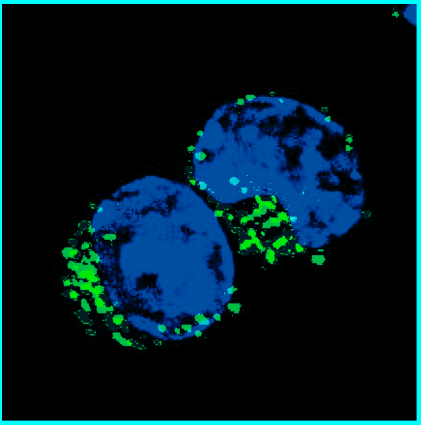
Image of the Month: Memory B Cells
Leave a reply cancel reply.
Your email address will not be published. Required fields are marked *
Notify me of follow-up comments by email.
Notify me of new posts by email.
Advertisement
New developments in the field of genomic technologies and their relevance to conservation management
- Review Article
- Open access
- Published: 11 November 2021
- Volume 23 , pages 217–242, ( 2022 )
Cite this article
You have full access to this open access article
- Gernot Segelbacher ORCID: orcid.org/0000-0002-8024-7008 1 ,
- Mirte Bosse ORCID: orcid.org/0000-0003-2433-2483 2 ,
- Pamela Burger ORCID: orcid.org/0000-0002-6941-0257 3 ,
- Peter Galbusera ORCID: orcid.org/0000-0001-9516-8196 4 ,
- José A. Godoy ORCID: orcid.org/0000-0001-7502-9471 5 ,
- Philippe Helsen ORCID: orcid.org/0000-0002-0178-077X 4 ,
- Christina Hvilsom ORCID: orcid.org/0000-0001-7870-6888 6 ,
- Laura Iacolina ORCID: orcid.org/0000-0001-5504-6549 7 ,
- Adla Kahric ORCID: orcid.org/0000-0002-5224-7502 8 ,
- Chiara Manfrin ORCID: orcid.org/0000-0002-4979-8557 9 ,
- Marina Nonic ORCID: orcid.org/0000-0003-4894-1371 10 ,
- Delphine Thizy ORCID: orcid.org/0000-0001-6325-8138 11 ,
- Ivaylo Tsvetkov ORCID: orcid.org/0000-0001-9957-7050 12 ,
- Nevena Veličković ORCID: orcid.org/0000-0003-0797-269X 13 ,
- Carles Vilà ORCID: orcid.org/0000-0002-4206-5246 5 ,
- Samantha M. Wisely ORCID: orcid.org/0000-0003-1748-4518 14 &
- Elena Buzan ORCID: orcid.org/0000-0003-0714-5301 7
15k Accesses
24 Citations
50 Altmetric
Explore all metrics
Recent technological advances in the field of genomics offer conservation managers and practitioners new tools to explore for conservation applications. Many of these tools are well developed and used by other life science fields, while others are still in development. Considering these technological possibilities, choosing the right tool(s) from the toolbox is crucial and can pose a challenging task. With this in mind, we strive to inspire, inform and illuminate managers and practitioners on how conservation efforts can benefit from the current genomic and biotechnological revolution. With inspirational case studies we show how new technologies can help resolve some of the main conservation challenges, while also informing how implementable the different technologies are. We here focus specifically on small population management, highlight the potential for genetic rescue, and discuss the opportunities in the field of gene editing to help with adaptation to changing environments. In addition, we delineate potential applications of gene drives for controlling invasive species. We illuminate that the genomic toolbox offers added benefit to conservation efforts, but also comes with limitations for the use of these novel emerging techniques.
Similar content being viewed by others
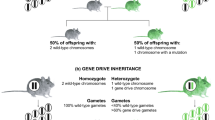
Population management using gene drive: molecular design, models of spread dynamics and assessment of ecological risks
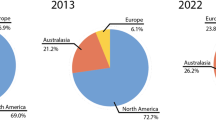
Capturing conservation in the post-genomics era: a book review of “Conservation and the Genomics of Populations.”
A Fresh Look at Conservation Genetics in the Neotropics
Avoid common mistakes on your manuscript.
Introduction
Despite ongoing activities to halt biodiversity loss, we are still losing many species at an alarming rate (Turvey and Crees 2019 ; WWF 2020 ). Targets to stop this loss, defined by the Convention of Biological Diversity (CBD) to be achieved by 2020, have clearly not been met (CBD 2020 ). Currently the post-2020 targets for CBD are developed and the EU biodiversity strategy discussed, both phrasing ambitious goals and milestones. However, it is less clear how these could be achieved. Meanwhile, conservationists seek to identify tools to improve and solve many of the conservation challenges globally, such as managing small populations or controlling invasive species. At the same time a technological revolution is taking place in the field of molecular biology, granting novel opportunities to screen, manipulate and even edit genomic material. These technological advancements have been seen as opportunities for conservation by some (Breed et al. 2019 ; Hohenlohe et al. 2021 ), and highly controversial among others (e.g. see motion 75 of the IUCN World Conservation Congress 2020 on synthetic biology https://www.iucncongress2020.org/motion/075 ). While the field of synthetic biology (see glossary) has been a centre of debate (Piaggio et al. 2017 ), conservation projects can benefit from the existing knowledge in other areas of biotechnology such as animal or plant breeding approaches applied in agriculture and livestock (McLean-Rodríguez et al. 2021 ).
With this perspective, we aim to provide an overview on the latest biotechnological developments for conservation practitioners and managers and especially highlight their feasibility for present and future potential conservation applications. This manuscript is a result of a G-BiKE COST Action (https://g-bikegenetics.eu ) workshop on novel genomic tools for conservation. The aim of the workshop was to bring practitioners and researchers together and help bridging the gap between researchers and managers, which has been described earlier (Shafer et al. 2015 ). Most attendees of the workshop had a background in animal conservation, thus our overview is likely to be biased towards animals, but we state that many topics are similar also for plants and other organisms.
Here, we focus on four important conservation areas: (1) management of small populations, (2) restoring and increasing genetic diversity, (3) support of adaptation to changing environments, and (4) invasive species management. We discuss the underlying techniques of genomic screening, cloning, gene editing and gene drive , highlighting examples from different plant and animal species. We also provide some key questions which need to be asked before starting a conservation project (Fig. 1 and end of each section) and present an overview on the feasibility and challenges of those techniques for the different conservation areas (Fig. 2 ). With this overview, we hope to inspire, inform and illuminate if and how these technologies can be used by managers and practitioners in their conservation projects.
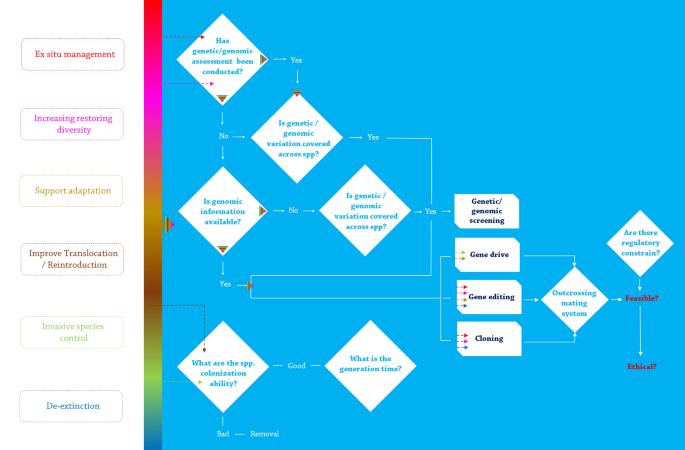
Genomic and biotechnological toolkit for conservation. Main conservation problems (left side, each box marked with a different colour) and most relevant questions when starting a genomic study. The flow chart connects conservation problems with techniques already available or under development. Arrow colours link the technologies with the conservation tasks (the same colour is used for the arrows as in the boxes on the left)
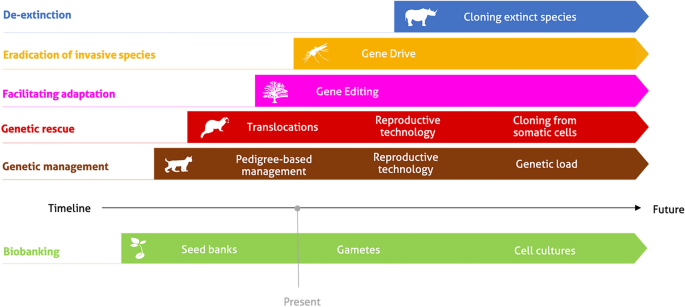
Feasibility of conservation areas and the different genomic and biotechnological tools. Each line and colour presents one of the main conservation problems (same colours as in Fig. 1 ). The timeline indicates the feasibility of the listed tools for current and future potential conservation applications
Small population management using genomic tools
Small population management is a critical tool to safeguard populations and has evolved at a rapid pace over the last decades, as a response to the escalating biodiversity crisis (see also CBD 2020 , Target 12). Many populations have become so small and fragmented that they are vulnerable to genetic and demographic stochasticity and unable to recover on their own, even when the anthropogenic threats they face can be successfully mitigated (Gilpin and Soulé 1986 ). Due to their limited (effective) population size, small populations lose genetic diversity over time, and as such adaptive potential, making them prone to inbreeding. In these situations, effective conservation requires a strategy that incorporates the most appropriate population management activities, selected from a range of options across ex situ and in situ conditions (Traylor-Holzer et al. 2019 ). Whether ex situ or in situ , the work follows a multi-step procedure starting from identifying the need and defining the role of the management actions, setting measurable goals, evaluating alternative (genetic) management actions and methods, monitoring progress towards the set genetic goals and adjust as needed (see the IUCN guidelines on ex situ management https://portals.iucn.org/library/node/44952 and Westgate et al. 2013 , McGowan et al. 2017 ). Genomics now has the potential to revolutionise how we approach these steps (Russello and Jensen 2018 ; Supple and Shapiro 2018 ) by evaluating the genetic history and status of the in situ and any ex situ populations in more detail.
Defining ex situ populations and their relationship to in situ populations using genomics
Today, ex situ conservation programs often rely completely or in part on existing collections of living individuals, gametes, plant seeds, or tissue and blood samples. Genomic techniques allow us to detect DNA sequence variation at single nucleotides (i.e. single-nucleotide polymorphism: SNP, see glossary) that can be used to evaluate the effects of historic founder selection (Gomes Viana et al. 2018 ; Frandsen et al 2020 ; Ogden et al. 2020 ; Wei and Jiang 2021 ) and set guidance on how to increase levels of genetic diversity and minimise genetic similarity (Bragg et al. 2020 ) via selective addition of unrepresented lines (Wildt et al. 2019 ; Galla et al. 2020 ; Miller et al. 2010 ). The efficiency of ex situ management partly relies on the profound knowledge of a species’ taxonomic status and recent demographic history. For many species of conservation concern, such information is either missing or incomplete, and genomics has proven key in filling knowledge gaps (e.g. Frandsen et al. 2020 ; Robinson et al. 2021a ) and delineating management units (Barbosa et al. 2018 ) starting from almost any type of sample (e.g. museum collections and biobanks; Baveja et al. 2020 ). Likewise, the inclusion of species specific genomic analysis generates unseen possibilities to reach predefined long-term conservation goals whether that is to e.g. increase genetic diversity or minimize inbreeding, even if little is known about relatedness of individuals. Genome wide SNP data are starting to be used to complement or even replace missing or incomplete pedigrees to provide robust data for guiding breeding efforts (Galla et al. 2020 ). Simultaneously, genomic data will guide optimal management (e.g. balancing hybridisation or the potential of adaptation) with the in situ population as a benchmark. As such, modern population management can and should foster a tight link between conservation efforts of ex situ populations and their wild counterparts (Boscari et al. 2021 ) and be integrated into the overall conservation strategy for a single or multiple species (the One Plan Approach coined by the Conservation Planning Specialist Group of the IUCN; Pritchard et al. 2012 ; Byers et al. 2013 ).
Small in situ populations, either remnant or reintroduced, are affected by the same negative processes that affect ex situ populations (e.g. loss of genetic diversity and accumulation of inbreeding), so the same general principles used for genetic management in the latter could be applied in the former. These include the maximization of founder population size and the management of gene flow; however, managing precise breeding is often out of reach. The same applies to ex situ management of group living species such as many aquatic species, some primate species or hoof stock living in large herds, where individual pedigrees are unknown and breeding recommendations are difficult. For plants, seed banks, i.e. storage of seeds, is the most popular way of ex situ conservation due to ease of storage, low costs and low risks of infestation or predation (Schoen and Brown 2001 ). However, seed banking can be problematic for species with low seed longevity, persistent dormancy or low germination rates. For these species, living collections for example in botanical gardens are often implemented as ex situ method. In addition to the same negative genetic processes of small and isolated in situ populations, ex situ living collections of plants risk local adaptation to non-natural, ‘garden’ conditions. To minimize the risk of adaptation to non-natural conditions, ‘ quasi in situ’ conservation—ex situ collections that are grown in natural environments—can be suggested as a compromise between ex situ and in situ conservation (Volis and Blecher 2010 ). In combination with genomic techniques to monitor genetic variation in next generations this may provide a successful integrated conservation strategy for plants.
Improving population management and monitoring through genomics
Minimizing overall genetic relatedness within populations (i.e. pedigree based kinship breeding) significantly reduces the pace at which diversity is lost and became the method of choice to manage small populations (Lacy et al. 2012 ; Che-Castaldo et al. 2021 ). Out of necessity, the pedigree approach was built on assumptions such as no founder relatedness and the absence of selection, knowing that this is not always the case (Frankham 2008 ; Hogg et al. 2019 ). Hence, many breeding programs will benefit from genetic screenings to (i) compensate for differences between theoretical and realized kinships (Grueber et al. 2021 ), (ii) resolve origin and screening for hybridisation (Howard-McCombe et al. 2021 ), (iii) detect clonal reproduction (e.g. many plants and apomictic species (Brown and Marshall 1995 ), (iv) guide reproduction or translocations in species for which individual record keeping, and as such pedigree reconstruction and/or kinship estimation, is (historically) missing, inaccurate or difficult e.g. group managed species (McLennan et al. 2020 ), (v) optimize health via direct screening for genetic or heritable diseases e.g. Devil Facial Tumor Disease (Storfer et al. 2018 ; Hohenlohe et al. 2019 ) or maintaining overall immunity (Savage and Zamudio 2011 ) and (vi) ensure that the genetic diversity captured within the ex situ populations represents that of the in situ population (Kleinman-Ruiz et al. 2019 ; Wei and Jiang 2021 ). Given the existing similarity of the general management aims in plant and animal ex situ collections, botanic gardens could benefit by adapting and broadly using the well-established scientific approaches by the zoo community (Fant et al. 2016 ; Wood et al. 2020 ).
Genomic tools as drivers for future management and biobanking
The largest gain in genomic analyses, over more traditional genetic tools, is their power to get additional information on genomic regions under selection, [e.g. runs of homozygosity (ROH) and deleterious mutations, Hohenlohe et al. 2021 ; see also Fig. 3 and glossary]. These could be introgressed genomic segments stemming from hybridisation or the lack hereof, or parts of the genome that are under selection. Additionally, population structure within a population can be detected and, depending on the conservation goals, should or could lead to a population management in separate groups based on evolutionary and genomic divergence. The genomic revolution also allows for direct identification of desirable genes and alleles, as well as the transfer of these adaptive genes to deliver new varieties, as has been proven effective for crop and livestock improvement (Wambugu et al. 2018 ). In addition, management of ex situ collections of plant genetic resources can benefit from genome-wide screening and the availability of reference-genome assemblies for almost all major crop species, overcoming the reproducibility issues related to earlier markers (Mascher et al. 2019 ).
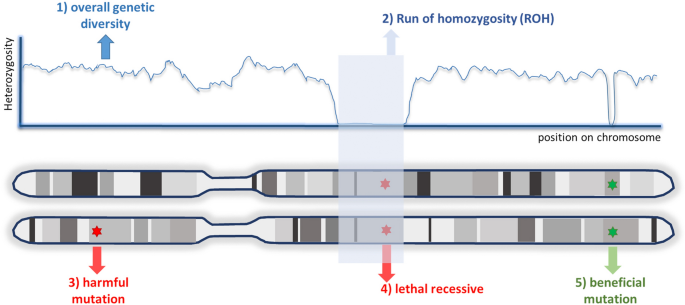
Genomic measures estimating genetic diversity within the genome of an organism
Broad applicability of genomics to improve conservation management is, however, often limited by a lack of samples from current populations and their (wild) founders. Biobanking techniques are well suited for animals and plants (Heywood and Iriondo 2003 ), including seed banks, in vitro storage (slow growth and cryopreservation), pollen banks, tissue cryopreservation, DNA storage, and botanic gardens living collections (Thormann et al. 2006 ). As such, regional zoo and aquarium biobanks [e.g. the European Association of Zoos and Aquaria (EAZA) Biobank] and seed banking initiatives (e.g. Millenium Seed Bank) are very valuable for both research and population management by maintaining genetic diversity (e.g. Howell et al. 2021 ) or even reviving lost genetic lineages. The addition of genomic information, e.g. SNP genotypes, to the information linked to records of stored samples could complement and correct traditional metadata and constitute a molecular fingerprint (Digital Sequence Information (DSI), for more background and discussion see the White Paper on DSI at https://www.dsmz.de/fileadmin/user_upload/Collection_allg/Final_WiLDSI_White_Paper_Oct7_2020.pdf ). Such a unique fingerprint would complement the conventional sample banks with bio-digital resource centres combining the storage of the preserved materials with their genomic and molecular characterization. Alongside improving the management of the collections, the dense genotyping information for all accessions would highlight duplicates and sampling coverage gaps (Mascher et al. 2019 ). Some novel initiatives within this context (e.g. the DivSeek project https://divseekintl.org ), and the potential of genomics-based technologies, could generate a paradigm shift in the usability of bio- and cryobank collections and bridge the gap between different data types.
Questions to be considered for managing small populations
For some (mostly iconic) species, genomic data already provided useful insights to guide ex situ population management. While the list of species that can benefit from genomic screenings increases, the shortage of available resources, such as reference genomes and samples from both ex situ and in situ populations, is often hindering the evaluation of how current levels of genetic diversity compares to historic or current in situ diversity.
Which genomic tools will help secure the data needed (e.g. coding, non-coding, whole genome to scan for inbreeding levels and adaptive potential) to answer the management questions in an in- and ex situ framework?
Several populations and research questions have been or are currently generating data on wild plants and animals that potentially could be used to help improve population management. However, one of the challenges to overcome is how to integrate and allow comparisons among data collected over different time periods or geographic ranges with various technologies.
How can we ensure that different data types can be integrated and comparisons with suitable reference populations be made?
As more data isn’t always better and the data required might be very much case by base dependent;
What is the ideal amount of data (e.g. number of SNPs) needed to answer questions that are challenging a conservation breeding program over time, e.g. high mean kinship within a population requires an increased need for informative SNPs?
Depending on the conservation question(s) to be answered, the population size, management strategy and resources, it is important to consider sampling;
How many and which extant populations and/or individuals as well as historical collections need to be sampled to provide answers that can help guide the conservation action?
And explore if samples are linked to metadata;
Is demographic, medical or other relevant data linked to samples/individuals through databases (e.g. Zoological Information Management System (ZIMS) or BGCI PlantSearch), which could be used to better understand a given population?
And lastly, how can future considerations and needs be incorporated into a species management plan;
Will cryopreservation of cell lines or gametes be of future use?
The National Academy of Sciences Engineering and Medicine (NASEM) defines gene drive as “a system of biased inheritance in which the ability of a genetic element to pass from a parent to its offspring through sexual reproduction is enhanced. Thus, the result of a gene drive is the preferential increase of a specific genotype, the genetic makeup of an organism that determines a specific phenotype (trait), from one generation to the next, and potentially throughout the population” (NASEM 2016 ). In summary, it can be described as a mechanism to bias inheritance through sexual reproduction (for a more detailed overview see Rode et al. 2019 ). Gene drive systems are naturally occurring, but in the last decades, researchers have been studying how to develop gene drives to modify species in order to address challenges in public health, conservation or agricultural pest control. The advances of gene editing tools have increased the ability of researchers to develop such mechanisms and the recent years have seen a multiplication of successful cage experiments, in particular in mosquitoes (Gantz et al. 2015 ; Hammond et al. 2016 ; Kyrou et al. 2018 ; Simoni et al. 2020 , Oh et al. 2021 ). While the overall definition is quite simple, it fails to represent the diversity of gene drive mechanisms and applications. For instance, in some cases (e.g. human malaria), there is a need to develop a mechanism that can spread through large populations while releasing a relatively small number of individuals carrying the modification (Garrood et al. 2021 ). Those mechanisms are called “low threshold” as opposed to “high threshold” mechanisms that require a high frequency of individuals carrying the modification to spread and modify the population. As such the risks and benefits might be different, and the choice of gene drive mechanism depends on the specific context. For instance, high threshold gene drives can be considered as one method of molecular confinement, and can be beneficial for interventions on invasive alien species that need to be eradicated in a location in contact with another location where the species is not invasive. Risk assessment and evaluation need to be done on a case-by-case basis to take into account those specificities (Redford et al. 2019 ).
To develop an engineered gene drive (as opposed to naturally occurring ones), researchers use gene-editing tools. Different platforms can be used depending on the type of gene drive to be developed. One of the most common types of gene drives is based on endonucleases, which will break the DNA and allow for the integration of the new sequence (Hammond et al. 2016 ). CRISPR-Cas9 has been a commonly used endonuclease for this, but other systems have also been developed. All engineered gene drive mechanisms are a result of gene editing but not all gene editing events would result in a gene drive. In most cases, gene-edited traits are inherited in a normal Mendelian way: that is, for any given gene editing event there is a 50% chance that an offspring will inherit it.
What genomics can teach us?
The availability of complete genomes of a range of species has opened up a treasure-trove of information for conservation biologists. Tools originally developed for human and model species such as laboratory animals or livestock, we can now use also for non-model species (Ekblom and Galindo 2011 ) and thus unlock a wealth of valuable data about the genetic history and current status of a species (Primmer 2009 ; Ouborg et al. 2010 ; Shafer et al. 2015 ; Supple and Shapiro 2018 ). Data from genomic approaches can now readily be used to improve conservation management. One very recent example where policy for breeding and reinforcement programmes have been gained from such an approach, was assessing the potential introgression to the endangered lesser white-fronted goose ( Anser erythropus ) in Sweden (Díez-Del-Molino et al. 2020 ).
What parameters obtained by full genome sequencing are relevant for conservation? (see also Fig. 3 and supplementary Table 1).
Overall genetic diversity , measured from re-sequence data as the number of heterozygous variants in an individual, can be seen as a proxy for long-term adaptive potential. Naturally, most of the genetic variation will prove not useful in the long run, but the measure is thought to reflect the potential variants in a population that may become adaptive in the future. Especially when the environment is changing rapidly, adaptive potential can define whether a species will persist or go extinct. But in a stable environment individual heterozygosity can also determine translocation success (Scott et al. 2020 ).
Inbreeding levels , measured as Runs Of Homozygosity (ROH) in the genome of an individual, reflects the extent of consanguinity in a population under the assumption of random mating. Numerous and long ROH are a warning that the population is undergoing inbreeding as a consequence of population decline, which may result in inbreeding depression (see also Fig. 3 ). As such, ROH could be an estimator of genetic health in a population.
Genetic load , measured as the amount of predicted harmful mutations in the genome of an individual, reflects how many variants with a potentially negative effect on fitness are present in a population. Most of such variants are recessive, therefore their harmful effect is only exposed during inbreeding (known as inbreeding depression). This measure can predict the severity of fitness reduction and population decline if inbreeding will occur, and can therefore be seen as an early warning system and also be considered in translocations (Hansson et al. 2021 ).
Lethal recessives are particularly damaging mutations that can be problematic in small populations when they are at high frequency. Note that this is different from the genetic load, which is thought to consist of many variants with small effects. These lethal mutations can be pinpointed in a genome and included in a breeding program or serve as a target for gene editing.
Locally adapted genes can be pinpointed as selective sweeps or highly differentiated parts of the genome between related populations or species. Local adaptation is important for a population to persist, and therefore such genetic variants should be maintained in a population, especially if individuals are re-introduced into their original habitat. Also, outbreeding with another population may not be desirable if many differences in local adaptation exist.
Cloning in animals
Cloning animals produces genetically identical copies of individuals via a process called somatic cell nuclear transfer (SCNT). During this process the nucleus of a cell from the animal of interest is merged with the enucleated oocyte of another animal. The resulting embryo is implanted into a surrogate mother. The resulting offspring is genetically identical to the nucleus donor. The process of producing this animal is novel because gametic cells (eggs or sperm) are not used. Instead, somatic cells, often skin cells called fibroblasts, are harvested from animals and their nuclei are inserted into the donated oocytes. Harvesting fibroblasts is less invasive than harvesting eggs or sperm which is desirable from an animal welfare standpoint (Ryder and Onuma 2018 ). Fibroblasts are relatively easy to cryopreserve and thaw for downstream applications. Thus, animals that have been dead for decades but have cryopreserved biomaterial can be cloned and reproduced. Biorepositories around the globe have preserved hundreds of species over the last 40 years, and many of the preserved samples would be suitable materials for cloning.
Cloning endangered species requires a modified approach to SCNT because of inefficiencies in the process. Many oocytes need to be harvested from animals to produce an embryo that develops into viable offspring. Therefore many oocyte donors are needed to produce a single clone. For endangered species, available oocyte donors are typically not available, therefore interspecific SCNT (iSCNT) has been proposed. iSCNT uses oocytes donated from individuals of closely related species. This mismatch of species results in greater inefficiencies still. To date, only a few attempts at iSCNT have been successful at producing healthy clones (Borges and Pereira 2019 ). iSCNT is a useful and often used experimental design in developmental biology and biomedicine, with rapid strides being made at overcoming these inefficiencies and developmental mismatches. Currently, cloning is limited to use in biomedicine and research in the European Union. Cloning animals for food or related commodities is banned under an EU directive ( https://ec.europa.eu/food/sites/food/files/animals/docs/aw_other_aspects_novel-cloning_com2013-894_final_en.pdf ).
Utilizing biotechnology developed on domestic species to conservation of wildlife
Genomic research on domestic animals has paved the way for the study of their wild relatives. These studies have applications for both ex situ and in situ population management. For example, in domestic species different breeds can become admixed over time and ancestral breeds lost. Genomic information can assist in the design of breeding programmes aimed at recovering original genetic variability from currently admixed breeds (Amador et al. 2014 ; Fernández et al. 2016 ). Such approaches have been useful in the conservation of domestic populations and, particularly, local breeds (Kristensen et al. 2015 ), but have the potential to support conservation efforts of wildlife as well (Hedrick 2010 ; Miller et al. 2017 ). For example, wild ( Felis silvestris silvestris ) and domestic ( Felis silvestris catus ) cats interbreed in Portugal (Oliveira et al. 2008 ), wild and domestic pigs (both Sus scrofa ) interbreed on the Iberian Peninsula (Gama et al. 2013 ) and cultivated walnut populations mix with native walnut trees (Hoban et al. 2012 ). In each case, the domestic population threatens the wild population with genetic swamping. While identifying hybrids has been possible already with microsatellites or SNPs (e.g. Soto et al. 2003 ) new genomic resources could help to identify hybrid individuals and to distinguish between past and recent hybridisation events in previously unknown accuracy (Mattucci et al. 2019 ). Additionally simulations can be useful for predicting outcomes of hybrid gene flow (e.g. DiFazio et al. 2012 ). This has been shown to be especially relevant for estimating gene flow from transgenic plants and crops into their wild relatives.
Research into domestic animals has also allowed the development of tools and baseline knowledge, as exemplified by the large number of studies on wolves ( Canis lupus ) genomics, which take advantage of resources developed for dogs (e.g. Pendleton et al. 2018 ). These tools have allowed, for example, the identification of the origin of the black pigmentation of some American wolves as a result of ancient hybridization with dogs (Anderson et al. 2009 ), the identification of genes associated with altitude adaptation in central Asian wolves (Zhang et al. 2014 ) or the characterization of inbreeding at Isle Royale (Robinson et al. 2019 ).
Importantly, the management of domestic populations has also allowed the advancement of reproductive schemes and approaches that could also be applied to wild species. These involve the design of mating strategies that minimize inbreeding or maximize allelic diversity (Fernández et al. 2016 ; López-Cortegano et al. 2019 ) applicable to closely monitored and managed wild and captive populations. Similarly, research on domestic species enabled the development of assisted reproductive technologies allowing the introduction of allelic variants from one population to another without the need to move individuals, and even allowing the recovery of genetic variants from long-dead individuals (for examples see Table 1 ). Biomedical research that advances biotechnology also utilizes domestic species such as the domestic ferret ( Mustela putorius furo ), horse, and sheep, and these models have paved the way for reproductive technologies, such as cloning of wild counterparts like the black-footed ferret ( Mustela nigripes ), Przewalski’s horse ( Equus ferus przwalski ) and mouflon ( Ovis aries musimon ) (see references in Sandler et al. 2021 , Box C and Table 1 ). These genomic and reproductive biotechnological advances in domestic species may also be combined in the future to design genomes with an increased variation, or that display more fit phenotypes (see Sect. “ Facilitating adaptation to changing environments through biotechnological applications ” for details). However, the strict selection of animal or plant breeding specimen for particular traits comes at the price of an additional reduction in the size of the founding population, with accompanying reduction in the effective population size (Amador et al. 2014 ; Toro et al. 2014 ; Fernández et al. 2016 ). Selective breeding of admixed populations can only aspire to restore the variation that is still segregating, but part of the original variation may no longer be available. In these cases, it may be possible to use cryopreserved biological material or seed banks to reintroduce some of the lost genetic variants into the population (Table 1 ).
A potentially new source of information derived from studies of biomedical models of domestic species, comes from studies on epigenetic variation. Epigenetics refers to changes in the gene products and ultimately phenotypes of individuals that are heritable but not associated with changes in the nucleic acid sequence. Rapid adaptations to changing environments can be brought by epigenetic mechanisms. Epigenetic modifications represent an extra-molecular component of biodiversity that links genomes to the environment, and ultimately improves the identification of conservation units by estimating the adaptive potential of organisms (Rey et al. 2020 ). Epigenetics has been put forward as a biomarker for physical conditions, and methylation levels that cells use to control gene expression might be of importance for guarding against the expression of harmful alleles under specific conditions (Marin et al. 2020 ). In plants, DNA methylation has been shown to regulate multiple processes, such as gene expression, genome stability and gene imprinting (Zhang et al. 2018 ; Gallego-Bartolomé 2020 ) and is postulated to play an important role in phenotypic plasticity and fast responses to sudden environmental changes (Colicchio and Herman 2020 ). Ultimately epigenetics may be valuable to the conservation practitioner as a new tool to delimit evolutionary significant units that take into account the capacity for organisms to adapt to rapidly changing environments (Rey et al. 2020 ).
When might domestic animals be good surrogate models for conservation of wildlife counterparts?
Domestic species, particularly those used for agriculture or biomedicine, are frequently well-studied and have genomic and reproductive science resources that are on the cutting edge of technology. When those domestic model species are congeners and closely related to species of concern, they can be powerful resources for understanding the target species. Genomes of domestic species have been used as a reference for unannotated genomes of wildlife (Hohenlohe et al. 2021 ), and reproductive techniques once applied to domestic species have been utilized in wildlife (Comizzoli and Wildt 2017 ). While it is important to understand that each species is ecologically, physiologically and behaviourally unique, domestic species can provide important information about evolutionarily similar species. For example, domestic ferrets have been used to advance the reproductive capabilities of both European mink ( Mustela lutreola ) and black-footed ferrets (Amstislavsky et al. 2008 ). The domestic dog has been used as a reference genome for multiple canid species, including the highly inbred Channel Island Fox ( Urocyon littoralis ; Robinson et al. 2016 ).
Advanced genomic and reproductive sciences have enhanced reintroductions, translocations and genetic rescue
Small populations that have become inbred due to matings between relatives are at risk of inbreeding depression , which results from the expression of deleterious alleles that can accumulate over time simply by chance ( genetic load ; for an overview see: van Oosterhout 2020 ). Animals with inbreeding depression show reduced fitness that manifests as reduced survivorship or fecundity (Hedrick and Fredrickson 2010 ). For example, inbred Scandinavian wolves bred less frequently and produced less offspring resulting in a population that declined over time (Åkesson et al. 2016 ). Two strategies are often suggested to reduce the impact of inbreeding depression in natural populations: purging and genetic rescue (Hedrick and Garcia-Dorado 2016 ; Bell et al. 2019 , see also glossary). At least, in theory, maintaining a population at a small size should contribute to removing deleterious variation through purging, thus reducing the amount of genetic load and inbreeding depression. However, simulations have shown that methods aimed at enhancing purging by intentional inbreeding should not be generally advised in conservation programmes because this implies important reductions in effective population size leading to severe inbreeding depression in the short term (Caballero et al. 2017 ). Inbreeding is alleviated by mating of individuals in the inbred population with other individuals characterised by alleles not found in that population. The resulting offspring of that mating will be likely more fit than their parents and then survive longer or have more offspring. The population recovers and grows as a result of the addition of genetically unique individuals, defined as genetic rescue (Ralls et al. 2020 for a detailed overview) . Genetic rescue has been observed to occur naturally when immigrants from a distant population integrate into an inbred one (reviewed in Frankham 2015 ). Genetic rescue has also been used successfully as a management tool for inbred populations, using translocated individuals e.g. in adders ( Vipera berus, Madsen et al. 1999 ), Florida Panther ( Puma concolor coryi, Johnson et al. 2010 ) or greater prairie chicken ( Tympanuchus cupido pinnatus, Westemeier et al. 1998 ), or translocations or interpopulation crosses in rare plant species (e.g. Pinus torrevana , Hamilton et al. 2017 ; Rutidosis leptorrhnynchoides , Pickup and Young 2008 ; Ranunculus reptans , Willi et al. 2007 ). In arctic foxes ( Vulpes lagopus, Hasselgren et al. 2021 ) and Isle Royale wolves ( Canis lupus, Robinson et al. 2019 ) inbreeding could also be reduced through genetic rescue, although this effect was only significant in the first generation. The goal for conservation practitioners and wildlife managers is to determine (i) which individuals can provide genetic rescue, (ii) and where a source of those individuals can be found.
Determining which individuals to use for genetic rescue
If translocated or reintroduced individuals are too genetically similar to the target population in need of genetic rescue, then inbreeding will not be alleviated and an increase in fitness will not be observed. On the other hand, if translocated individuals are too genetically dissimilar from the target population, then outbreeding depression can occur. Outbreeding depression reduces fitness in the next generation as a result of mismatches in genetic compatibility between two individuals and/or the introduction of maladapted genes (Flanagan et al. 2018 ). Recent advances in genomics allow conservation practitioners to better understand if candidate individuals for translocation are a good fit for genetic rescue. First, a genomic screening is suggested in which genetic diversity, inbreeding and harmful mutations are assessed for both the population in need of genetic rescue as well as potential donor individuals (see also Fig. 3 ). Ideally, these donor individuals come from different source populations as this greatly enhances the genetic diversity that can be used for rescue. As this approach could increase the genetic load in the target population, some authors suggested to source individuals from partially-inbred populations (Robinson et al. 2018 , 2019 ; Kyriazis et al. 2021 ). However, Ralls et al. ( 2020 ) recently highlight the limitations of these studies and argue to use source populations with high genetic diversity. In any case, intensive monitoring and rigorous evaluations will be needed to develop best practices for implementing genetic rescue measures (see Robinson et al. 2021b ).
Determining the source of individuals to use for genetic rescue
The selection of suitable source populations is key for any reintroduction program (Houde et al. 2015 ). For this purpose, genomic data can provide very valuable and previously inaccessible insights into the evolutionary and demographic history of target and source populations (Hohenlohe et al. 2021 ). Relocation programs, while aiming at increasing genetic diversity, should also consider source populations from environments as similar as possible to the population to be augmented (e.g. Hedrick and Fredrickson 2010 ) to decrease the possibility of maladaptation/outbreeding depression. Such a situation arises when donor and recipient populations have developed different environmental adaptations and their offspring are less adapted to either habitat (Marshall and Spalton 2000 ). Identifying potential functional fitness-related variation enables direct assessments of candidate source population for preadaptation to the target environment, and for their potential for acclimation to the new environments through phenotypic plasticity (Cauwelier et al. 2018 ; Muchero et al. 2018 ). Despite the need to preserve adaptive variants and avoid the introduction of deleterious alleles in translocations (Kristensen et al. 2015 ), the general recommendation has been to prioritize overall genetic diversity and evolutionary potential more than specific variants or adaptations (Weeks et al. 2011 ; Kardos and Shafer 2018 ). Careful consideration of alternative source populations is thus required when planning translocation or reintroduction programs, so that closely related and ecologically similar populations are favoured and large populations with high genetic load are avoided when alternatives are available.
Genetic rescue has typically been conducted using live individuals that are translocated from either a wild or a captive population. Assisted reproduction technologies like artificial insemination or in vitro fertilization have been used in captive wildlife management for decades and have the possibility of being used for genetic rescue of wild populations (Comizzoli and Wildt 2017 ). In this case sperm, ova, or embryos from genetically valuable individuals would be used instead of live individuals to increase the genetic diversity of the recipient gene pool. Although technologically feasible, this technique has not been used for genetic rescue of in situ populations. Finally, instead of using germplasm, such as sperm or eggs, it has been proposed that non-reproductive somatic cells could be used to clone genetically valuable individuals that could then be used for genetic rescue. While this idea has been proposed earlier (Wisely et al. 2015 ), efforts have now led to successful protocols (Borges and Pereira 2019 ), which have only recently been used for genetic rescue in the Przewalski horse ( Equus przewalskii; Hernandez 2020 ) and the black-footed ferret ( Mustela nigripes; Imbler 2021 ). For potential ethical considerations of such approaches see Sandler et al. ( 2021 ).
A typical example of a ‘genetic rescue’ approach is the case of the California endemic Torrey pine ( Pinus torreyana Parry). The species is one of the rarest pines in the world and of conservation concern with only two populations, occupying a mainland and an island (Hamilton et al. 2017 ). Based on a progeny trial for evaluation of phenotypic differences, the authors found genetic population-specific differences, with the island population having reduced genetic variability compared to the mainland population. The F 1 hybrids (a cross between both populations) exhibited increased fitness (height and higher fecundity) compared to the parents. In this context, the authors suggest that intraspecific hybridization might help restore genetic variation capable of coping with environmental changes, thus preserving the evolutionary potential in rare species and realising the concept for ‘genetic rescue’.
Reintroduced populations are particularly susceptible to genetic erosion due to intense founder effects caused by usually a small number of released individuals, low survival and large variance in reproductive success during the establishment phase. As in the foundation of a captive population, the selection of animals for release should be focused on minimizing inbreeding and relatedness to allow optimal founder diversity and to avoid inbreeding accumulation in the first few generations. Any post-release genetic management will require accurate information on the survival and reproduction of the released individuals. Fortunately, this is greatly facilitated by the genetic profiling of scats, hair, moulted feathers, and other material obtained through non-invasive genetic sampling, suboptimal materials that allow, nevertheless, for the genotyping of an increasing number of genome-wide markers (von Thaden et al. 2020 ) and even the access to targeted (Perry et al. 2010 ) or whole genome sequences (Taylor et al. 2020 ). Intensive monitoring of reintroduced populations would then allow for an individual-based genetic management of subsequent releases during the establishment phase by selecting individuals that are minimally related to the current population.
The global Iberian lynx ( Lynx pardinus ) population in 2002 was less than 100 individuals distributed in two isolated remnant populations in Southern Spain. Whole-genome sequences revealed the Iberian lynx as one of the species with the lowest species- and genome-wide genetic diversity (Abascal et al. 2016 ), resulting from long-term small population sizes and recent bottlenecks. Historical and ancient genetic data suggested that currently observed genetic differentiation between the two remnant populations was driven by genetic drift and isolation, hence validating their admixture and the management of the species as a single management unit (Casas-Marce et al. 2013 , 2017 ). The holistic conservation program included the establishment of an ex situ population and an intensive in situ program, which eventually incorporated translocations and an ambitious reintroduction program. Molecular marker data became an integral part of the management of the ex situ population since its conception, by contributing an empirical founder kinship matrix and by allowing the comparison of the genetic compositions of wild and captive populations and the monitoring of genetic diversity and inbreeding through time (Kleinman-Ruiz et al. 2019 ). Genetic management has been based on the principle of minimizing average kinship, so the priority for reproduction, pairings and releases are determined annually from the marker-assisted kinship matrix. Ongoing genome wide studies seek to identify potentially deleterious mutations relevant for inbreeding depression, as well as particular genetic variants associated with specific traits, information that could eventually be implemented in the management of the species. Once one of the most endangered felids in the world, the recent 2019 census yielded > 800 wild individuals living in the two remnants and six reintroduction areas, illustrating a very successful species recovery assisted by the application of genomic information and molecular tools.
How can genomic information help?
For the target population, it is important to know which specific mutations are causing the reduction in fitness when exposed in a homozygous state (i.e. which mutations contribute to the genetic load). Then the potential donor animals are genetically screened for the five important genomic measures as indicated in Box B “Genomic tools’ (see also Fitzpatrick and Funk 2019 ; Fitzpatrick et al. 2020 ). These genomic measures can be used to accurately estimate distinct genetic areas and assess inbreeding in populations, they have been used to design breeding schemes to select against deleterious alleles or to increase heterozygosity, and they have the potential to increase the long-term survival of breeds and wild populations in view of climate change (for an overview see Kristensen et al. 2015 ). For evaluating overall adaptive potential whole genome, or at least genome-wide screenings, may remain the preferred option (Flanagan et al. 2018 ; Bourgeois and Warren 2021 ).
Genomic analyses of source populations could also allow the identification of genetic variation underlying (or simply linked to) traits that increase fitness in particular environments. Although there are a few examples of successful identification of the genetic basis of particular traits in natural populations (e.g. Anderson et al. 2009 ; Fulgione et al. 2016 ; Benazzo et al. 2017 ; Christmas et al. 2019 ), this remains a challenging task, despite the increasing availability of annotated reference genomes (Kardos et al. 2016 ). Such a problem is particularly daring for endangered species, as they present severe limitations in sample sizes, phenotypic data, and experimental approaches. Here ex situ populations can be of help, as they offer ample opportunities for combining e.g. behavioural and demographic data with genomic profiles, and access to samples either directly from zoos, aquariums and botanic gardens or from large collections in biobanks (such as the EAZA Biobank or the Millenium Seed Bank). Finally, transcriptomic analyses can inform on the ability of particular individuals, or populations, to change expression levels in response to environmental stressors anticipated at the reintroduction area, either at candidate response genes when these have been previously identified, or globally when these remain unknown (He et al. 2016 ).
A similar challenge to variants under positive selection due to local adaptation exists for the detection of variants that have detrimental effects. Recent genomics methodologies have been developed to assess the potential impact of mutations that are present in genomes, without the need of phenotypes to test their harmful nature. Such bioinformatic identification of deleterious alleles rely on evolutionary conservation (Cooper et al. 2005 ; Pollard et al. 2010 ), physicochemical properties and their putative effect on the functionality of genes (Ng and Henikoff 2003 ) or combined approaches (Kircher et al. 2014 ; Wang et al. 2010 ). Although the deleteriousness of single variants remains notoriously difficult to predict from sequence data alone, when combined they can provide a reliable estimate of genetic load in a comparative framework (van der Valk et al. 2019 ; von Seth et al. 2021 ).
Questions to consider prior to management action
Prior to initiating a genetic rescue program, conservation practitioners should consider several questions. As with any conservation action, defining the problem that needs to be solved is paramount. Thus for genetic rescue, a first question to consider is:
Does the population decline have a genetic cause, i.e. is there reduced fitness in the population?
Previous studies have conducted time series analysis of population size and inbreeding (Åkesson et al. 2016 ), or assessed correlations of fitness and levels of inbreeding (Santymire et al. 2019 ). Genomic methods increasingly provide a robust method for identifying maladaptations or recessive alleles.
Have genomes been sequenced for the target species so that different populations can be analysed and potential maladaptations be assessed?
Historically, living individuals or seeds from different populations have been the source of genomes for genetic rescue. If genomes of living individuals are available we can use bioinformatic tools to pinpoint potential deleterious mutations within the genome and choose individuals with the lowest load. As an alternative to such wild or domestic individuals, advanced reproductive science makes it possible to use cryopreserved semen or embryos (Comizzoli and Wildt 2017 ) or even cryopreserved fibroblasts (Wisely et al. 2015 ). Ultimately, source material may come in the form of living organisms or biobank samples from ex situ or in situ populations.
Are the source genomes compatible with the gene pool of the recipient population and do they have the potential to increase fitness?
Advances in genomic analyses will allow for better matching between source and recipient gene pools so that inbreeding depression is alleviated yet outbreeding depression or other genomic pitfalls are avoided. In addition, individuals with high genetic load could be identified as unsuitable as a source for translocation (Kyriazis et al. 2021 ). Therefore screening genomic samples not only from the recipient population, but also the source population is desirable.
What technical expertise is required to integrate source and recipient gene pools?
It is important to understand the technical feasibility of a genetic rescue operation. Wild animal or plant translocations have requirements that would be different from using animals or plants from captive breeding facilities or botanical gardens. Genetic rescue from cryopreserved samples is technologically complicated and requires feasibility tests on different levels (from fertilization of a single individual to implementing such information in a wild population). For example, advanced reproductive science techniques such as cryopreservation, artificial insemination, in vitro fertilization and cloning would need to be optimised for each species for which the specific technology is being considered.
How will success be measured?
Genetic rescue implies that success is not solely an increase in population size, but also increased reproduction, survival, and adaptation potential in the target population. Therefore monitoring of recipient populations for population vital rates is key. However, genomic and biotechnological tools are only a small part of the overall toolbox.
Facilitating adaptation to changing environments through biotechnological applications
Genetic diversity is the underlying prerequisite for all species to adapt to changing environmental conditions. Ecosystem resilience thus highly depends on genetic variation (see Hoban et al. 2021 ; Stange et al. 2021 ). Genomic tools now enable us to assess the adaptive potential of species and identify particular loci underlying adaptation. Such genomic information is adopted in commercial animal and plant breeding to predict the phenotype of individuals and identify potential shifts in phenotypes (Meuwissen et al. 2001 ; Georges et al. 2019 ). Only recently has it been applied in wild populations, e.g. to identify the basis of the shift in egg laying date in great tits ( Parus major ) (Gienapp et al. 2019 ) or multiple morphological traits such as weight, horn length or coat color in soay sheep ( Ovis aries ) (Ashraf et al. 2020 ) or drought, freezing tolerance, warmer temperatures or salt stress in plants and trees (Exposito-Alonso et al. 2018 ; Browne et al. 2019 ). Genomic knowledge of specific genes can not only be used to identify specific variants of interest (e.g. Shryock et al. 2021 ) but the genetic code can also be altered by targeted changes of an organism's genome, also known as genome editing (see glossary). This approach encompasses a wide variety of tools using site-specific enzymes. Currently, the CRISPR/Cas9 nuclease system (clustered regularly interspaced short palindromic repeat/CRISPR-associated protein) is commonly used in many research fields (such as agriculture and medicine) and the attempts to alter or introduce genes with the goal of enhancing species survival against specific threats, such as disease or climate change are discussed widely (for an overview see Piaggio et al. 2017 ; Redford et al. 2019 ). The versatility, simplicity, and efficacy of the CRISPR technology offer the researchers previously unattainable levels of precision and control over genomic modifications, making it the most widely used among the genome editing tools (Bewg et al. 2018 ). While the application of genome editing tools in many wild animal species is still in its infancy, it has already been demonstrated in forest trees such as poplars ( Populus ) (Elorriaga et al. 2018 ) and woody perennials (Tsai and Xue 2015 ) and we can expect an increasing genomic knowledge to develop strategies for disease resistance (Naidoo et al. 2019 ; Dort et al. 2020 ).
Changing environmental conditions such as increasing sea temperatures are threatening marine ecosystems and especially corals that suffer from mass bleaching. Thus, thermal adaptation is crucial for their persistence. Heat tolerance can be inherited and natural variation in temperature tolerance may thus facilitate adaptation (Dixon et al. 2015 ). Specific genes which are responsible for heat tolerance have already been identified through gene editing techniques (Cleves et al. 2020 ). This knowledge helps to understand which genetic traits are controlling heat tolerance of corals and might thus be useful targets for future genetic engineering and enhancing thermal tolerance (van Oppen et al. 2017 ).
The American chestnut ( Castanea dentata ) is a fast-growing and long-lived keystone tree species in the eastern United States and Canada (Powell et al. 2019 ). An invasive fungal pathogen Chestnut blight ( Cryphonectria parasitica ) has been introduced from Asia into the United States in the late 1800s. Since then it has been spreading throughout the species range killing around 90% of the population (Merkle et al. 2007 ; Powell et al. 2019 ). The American Chestnut Foundation ( https://www.acf.org ) has implemented a breeding program that tries to incorporate the blight resistance of Chinese chestnut ( Castanea mollissima ) into the American chestnut trees by backcross breeding. While this has been partly promising, inheritance by future generations is inconsistent and will likely require many generations of breeding (Steiner et al. 2017 ). Thus genetically engineered blight-tolerant American chestnut trees have been developed and those first transgenic American chestnut trees were planted in field trials in 2006 (Steiner et al. 2017 ). While transgenic trees are mostly developed for economic purposes, the chestnut trees represent the first example of genome editing as part of a restoration strategy for conservation purposes (Merkle et al. 2007 ). The US Department of Agriculture has started a regulatory review of the proposed transgenic American chestnut in August 2020, including a period of public comment.
Questions to consider prior to further integration of genome editing for management
Genome editing and the introduction of additional variation into the genome of wild organisms are based on the genomic information available in different populations of the target species. In case a genome editing approach might be considered several key questions need to be answered ahead:
Is full genome/transcriptome information for the target species available?
Is that information available across different populations or subspecies?
Is the target species related to a model species?
If genome information is accessible then we need to identify if and how this information relates to specific phenotypes.
Can target genes potentially be identified?
Are the traits which should be altered controlled by a single gene or multiple genes?
By identifying the underlying genomic basis for specific traits we can start experiments. For this, we need to have additional information.
What is the breeding system of the organism?
Can these organisms be kept in an experimental setting under different environments?
Managing/eradication of invasive species
Invasive species are within the five key drivers of biodiversity loss and extinction with 20% of extinctions being attributed to invasive alien species and 54% partially caused by them (Clavero and García-Berthou 2005 ). However, the management and eradication of invasive alien species is challenging and needs case by base evaluations due to different ways of spreading, life cycles and reproduction strategies. Currently managing invasives is mostly done through mechanical, chemical and biological controls, bio pesticides or the mass release of sterile individuals (Wittenberg and Cock 2001 ; Teem et al. 2020 ). While those tools have shown to be partly successful, they are labour and cost-intensive and, therefore, difficult to scale up to larger areas (Glen et al. 2013 ). There can also be negative side effects on non-target species that can result as bycatch products, or when using chemicals and introducing allochthonous natural predators (Messing and Wright 2006 ; Glen et al. 2007 ). Genetic information can complement the existing integrated invasive alien species management programmes by providing relevant information on, e.g. hybridisation mechanisms (van de Crommenacker et al. 2015 ) and physiological adaptation to different conditions (i.e. transcriptomic studies) (Luo et al. 2020 ; Clark et al. 2021 ). Increasing numbers of genomes are currently being sequenced or already available for many invasive species, providing key information on their biology, evolution and invasive spread (Prentis et al. 2008 ; North et al. 2021 ).
The processes during an invasion can be tracked through molecular data and understanding the history of invasions can inform management of current and future introductions (Hamelin and Roe 2020 ). Information on the temporal and spatial dynamics of an invasion also helps to develop a biosurveillance system (Westfall et al. 2020 ), where pests and pathogens could be identified and pathways of spread assessed (Hamelin and Roe 2020 ).
Genomic information can give valuable insight whether genetic variability in native species can be linked to invasiveness. The emerald ash borer ( Agrilus planipennis ) is a phloem-feeding beetle that is native to Asia but invasive in North America where it has been identified to be an extremely destructive pest for ash trees ( Fraxinus spp.) (Global Invasive Species Database 2020 ). Studies comparing the genomes of the most susceptible North American ashes ( F. americana, F. pennsylvanica, F. nigra ) with the resistant species F. mandshurica in Asia mapped defence related genes and identified candidate genes linked to resistance (Lane et al. 2016 ; Kelly et al. 2019 ). This ultimately can lead to improved future ash breeding programmes (Bai et al. 2011 ).
A different approach is used when the genomic composition of the invasive species itself is analysed by identifying the genes and causative mutations that play an important role in the evolution of invasiveness. Studying the actual function of such genes can be combined with phenotypic traits of interest (Prentis and Pavasovic 2013 ). Identified resistance genes could then be monitored and potentially increased for sexually reproductive species. The introduction or modification of a genetic trait that would negatively affect the reproductive ability of the invasive alien species could be achieved by a so-called gene drive (see Box A ; Alphey et al. 2020 ). This mechanism can be observed in nature in fungi, yeast and other organisms, and allows a gene to be passed to the progeny through sexual reproduction at a higher inheritance rate, even if it does not confer a competitive advantage (Burt and Trivers 2006 ). Possibilities to apply gene drive for invasive species eradication management plans involve targeting specific genes involved in main physiological functions or reproduction (i.e., for arthropods). Recently, the potential use of gene drives for the management of populations has been discussed and described in detail in Rode et al. ( 2019 ). However, the development of gene drives for conservation applications is still at a very early stage, and further investigations are required to evaluate their feasibility as well as potential impacts both positive and negative (for a recent review see Price et al. 2020 ).
Case studies of biotechnology in wild organisms
Avian malaria.
Similar to efforts taking place to tackle human malaria using gene drive approaches (Burt et al. 2018 ), researchers are investigating potential new tools to eliminate avian malaria threatening Hawaiian birds. Avian malaria is the driver for the native bird population extinction and decline (Atkinson and Lapointe 2009 ). The avian malaria vector mosquito ( Culex quinquefasciatus ) is an alien invasive species in Hawaii (Kenis et al. 2009 ). Different interventions involving genetic approaches are being considered. For instance, using a gene drive approach to make the invasive mosquitoes refractory to avian malaria (gene drive population alteration approach) or reducing the population of invasive mosquitoes by driving infertility traits or biasing the sex ratio (gene drive population suppression approach) (National Academies Science, Engineering, Medicine 2016 ). The US Fish and Wildlife Service, Hawaiʻi Department of Land and Natural Resources, and the American Bird Conservancy are also looking at the potential use of Wolbachia , a naturally-occurring bacteria that can lead to the absence of viable progeny (Redford et al. 2019 ). This approach has been used to control another invasive mosquito species Aedes aegypti, which is one of the key vectors for dengue and Zika viruses (Hoffmann et al. 2011 ). This research on new approaches to tackle avian malaria is still at an early stage and is expected to benefit from some of the discoveries made in human malaria research, where the research is more advanced, partly because of higher attention from researchers, funders and the public.
Potential of suppression of invasive social wasps
The invasive common wasp ( Vespula vulgaris ) originally native to Eurasia is listed among the 100 world worst invasive alien species (Lowe et al. 2000 ). In New Zealand the common wasp predates 0.8–4.8 million loads of prey/ha, exerting an annual cost of about NZ$133 million. Due to the high densities of wasp populations that cover over more than a million hectares of native forest, the environmental impact of using pesticides is prohibitive and risky for biodiversity. Modellers have demonstrated the potential of a CRISPR-based gene drive mechanism that would target spermatogenesis genes with likely male-specific expression to obtain modified-queens (Lester et al. 2020 ). When a modified-queen mates with wild-type males it will produce fertile workers carrying modified genes and thus propagate it to the next generation, but if it mates with a genetically modified male, fertilization will fail and all offspring will be male. This nest will fail and die in spring or early summer as males do not forage or aid in nest maintenance. A spermatogenesis gene drive could thus potentially reduce the wasp population and depending on population dynamics, locally eradicate those invasive wasps (Rode et al. 2019 ).
Invasive rodent eradication on islands
Since the 1960s, conservation efforts have focused on restoring the islands' biodiversity by eradicating invasive rodents (particularly because they are destroying native fauna and flora). The use of gene drive to eradicate rodents requires special knowledge and precautions to ensure that the intervention remains on the target populations and does not affect populations of rodents where they are native species. The proposed approaches (Lindholm et al. 2016 ; Harvey-Samuel et al. 2017 ; Piaggo et al. 2017; Campbell et al. 2019 ) are to bias sex ratio of the invasive rodents’ offspring, and to get this modification inherited at a high ratio, which would reduce or completely suppress the population due to lack of reproduction and natural attrition. This is still under development in the laboratories and the release of modified mice into the wild is likely to be in the distant future. This gene drive approach offers a potentially attractive alternative to the use of toxicants for eradication and can serve as an important tool to reduce invasive rodent pests, island predators, or zoonotic disease hosts (e.g. house mice, Mus musculus , and three rat species; Rattus norvegicus, R. rattus and R. exulans ).
Questions to consider prior to further integration of gene drives for targeting invasive species
While some conservationists already discuss the use of gene drives for conservation management the technologies are still in development and will not be readily applicable in the near future. For practitioners and managers that means that gene drives will need further rigorous research—both in containment and potentially with small-scale field evaluations—before they can be applied to address specific challenges. Several key questions have to be asked for potential projects:
What is the success rate of previous control attempts?
Is there any genomic information available for the target species and the target genes?
What are the benefits of using a potential gene drive approach?
What is the timeframe and scale on implementing invasive disease control?
Is there a risk of the gene drive technology spreading to a non-target population (either in a location where the species is not invasive, or to a non-invasive related species through hybridisation).
Would gene drive technology obtain social, ethical, and regulatory acceptability? And what are stakeholder perceptions/views for acceptance and engagement in genetic biocontrol?
In cases where gene drives could be established in model organisms successfully some additional points have to be asked.
Is the generation length short enough for a gene drive approach to have an effect in a relevant timescale for humans and nature?
Does the target organism reproduce sexually?
Currently it is very clear that gene drives cannot be uniformly applied to all organisms. Short generation times and high offspring rates such as in mosquitoes can make the intervention relevant. Before planning field trials one has to consider:
Are there co-occurring native species at the target site which might be affected through hybridisation?
Can the risk assessment of field trials be done on the basis of a deployment hypothesis?
What are the potential risks for the accidental or deliberate release of genetically modified organisms into non-target populations?
The crux of almost all management actions is to obtain comprehensive knowledge of the target species to inform decision making, including data on population size and habitat use, behavioural data like colonisation ability, generation time, and reproductive mode. Many of these questions can be confidently addressed using microsatellites or a few hundred SNPs. However, with genomic information being increasingly available for many species (Hohenlohe et al. 2021 ), we gain additional insight, as on the demographic history or introgression patterns, thus providing relevant information for species management. Genomic technologies are increasingly being applied in ex situ and in situ management and genomic screenings are necessary to identify the most suitable individuals for breeding programs, translocations and genetic rescue in terms of genetic relatedness and to avoid increasing genetic load in the target population. Genomic variation also helps to more efficiently fight potential diseases and combat climate change by identifying genetic variation of specific traits that increase fitness in particular environments. In addition, knowledge from genomes helps us to compare contemporary populations as well as allowing temporal comparisons in more detail and thus enables us to estimate the magnitude of change across populations and time. Genomic information is equally important for cloning representatives of highly endangered or even extinct species, where the first achievements have been made. In this context, a state-of-the-art collection and storage of seeds, tissues, and cells in biobanks are invaluable. Comprehensive genomic knowledge is also essential for gene editing of organisms in support of their adaptation to environmental changes or for combating pathogens. This practice of genome modification has already been applied in plant taxa, model species, and even in humans, and holds great potential for conservation applications at the individual level. On the population level gene drives are currently developed to be applied on pest species, for which other eradication interventions have not succeeded. The broad range of biotechnological and genomic tools can help conservation practitioners and managers to resolve some of the most pressing conservation challenges, whilst at the same time actions should be made to secure habitat and provide species protection. However, a careful case-by-case risk assessment for potential applications is required, and ethical and political aspects need to be considered (Breed et al. 2019 ; Redford et al. 2019 ). While some tools are already well established, others still need to be tested and will only be applicable in the future (Fig. 2 ).
Data Availability
Not applicable.
Abascal F, Corvelo A, Cruz F, Villanueva-Cañas JL, Vlasova A, Marcet-Houben M, Martínez-Cruz B, Cheng JY, Prieto P, Quesada V, Quilez J, Li G, García F, Rubio-Camarillo M, Frias L, Ribeca P, Capella-Gutiérrez S, Rodríguez JM, Câmara F, Lowy E, Cozzuto L, Erb I, Tress ML, Rodriguez-Ales JL, Ruiz-Orera J, Reverter F, Casas-Marce M, Soriano L, Arango JR, Derdak S, Galán B, Blanc J, Gut M, Lorente-Galdos B, Andrés-Nieto M, López-Otín C, Valencia A, Gut I, García JL, Guigó R, Murphy WJ, Ruiz-Herrera A, Marques-Bonet T, Roma G, Notredame C, Mailund T, Albà MM, Gabaldón T, Alioto T, Godoy JA (2016) Extreme genomic erosion after recurrent demographic bottlenecks in the highly endangered Iberian lynx. Genome Biol 17:251. https://doi.org/10.1186/s13059-016-1090-1
Article CAS PubMed PubMed Central Google Scholar
Åkesson M, Liberg O, Sand H, Wabakken P, Bensch S, Flagstad Ø (2016) Genetic rescue in a severely inbred wolf population. Mol Ecol 25(19):4745–4756. https://doi.org/10.1111/mec.13797
Article PubMed PubMed Central Google Scholar
Alphey LS, Crisanti A, Randazzo FF, Akbari OS (2020) Opinion: standardizing the definition of gene drive. Proc Natl Acad Sci USA 117(49):30864–30867. https://doi.org/10.1073/pnas.2020417117
Amador C, Hayes BJ, Daetwyler HD (2014) Genomic selection for recovery of original genetic background from hybrids of endangered and common breeds. Evol Appl 7:227–237. https://doi.org/10.1111/eva.12113
Article CAS PubMed Google Scholar
Amstislavsky S, Lindeberg H, Alto JA, Kennedy MW (2008) Conservation of the European mink ( Mustela lutreola ): Focus on reproduction and reproductive technologies. Reprod Domest Anim 43:502–513. https://doi.org/10.1111/j.1439-0531.2007.00950.x
Anderson TM, von Holdt BM, Candille SI, Musiani M, Greco C, Stahler DR, Smith DW, Padhukasahasram B, Randi E, Leonard JA, Bustamante CD, Ostrander EA, Tang H, Wayne RK, Barsh GS (2009) Molecular and evolutionary history of melanism in North American gray wolves. Science 323(5919):1339–1343. https://doi.org/10.1126/science.1165448
Ashraf B, Hunter DC, Bérénos C, Ellis PA, Johnston SE, Pilkington JG, Pemberton JM, Slate J (2020) Genomic prediction in the wild: a case study in Soay sheep. bioRxiv. https://doi.org/10.1101/2020.07.15.205385
Article Google Scholar
Atkinson CT, LaPointe DA (2009) Introduced avian diseases, climate change, and the future of Hawaiian honeycreepers. J Avian Med Surg 23(1):53–63. https://doi.org/10.1647/2008-059.1
Article PubMed Google Scholar
Bai X, Rivera-Vega L, Mamidala P, Bonello P, Herms DA (2011) Transcriptomic signatures of Ash ( Fraxinus spp.) phloem. PLoS ONE 6(1):e16368. https://doi.org/10.1371/journal.pone.0016368
Barbosa S, Mestre F, White T, Paupério J, Alves P, Searle J (2018) Integrative approaches to guide conservation decisions: using genomics to define conservation units and functional corridors. Mol Ecol 27:3452–3465. https://doi.org/10.1111/mec.14806
Bard NW, Miller CS, Bruederle LP (2021) High genomic diversity maintained by populations of Carex scirpoidea subsp. convoluta, a paraphyletic Great Lakes ecotype. Conserv Genet 22:169–185. https://doi.org/10.1007/s10592-020-01326-x
Baveja P, Garg KM, Chattopadhyay B, Sadanandan KR, Prawiradilaga DM, Yuda P, Lee JGH, Rheindt FE (2020) Using historical genome-wide DNA to unravel the confused taxonomy in a songbird lineage that is extinct in the wild. Evol Appl 14(3):698–709. https://doi.org/10.1111/eva.13149
Bell D, Robinson Z, Funk C, Fitzpatrick S, Allendorf F, Tallmon D, Whiteley A (2019) The exciting potential and remaining uncertainties of genetic rescue. Trends Ecol Evol 34(12):1070–1079. https://doi.org/10.1016/j.tree.2019.06.006
Benazzo A, Trucchi E, Cahill JA, Maisano Delser P, Mona S, Fumagalli M, Bunnefeld L, Cornetti L, Ghirotto S, Girardi M, Ometto L, Panziera A, Rota-Stabelli O, Zanetti E, Karamanlidis A, Groff C, Paule L, Gentile L, Vilà C, Vicario S, Boitani L, Orlando L, Fuselli S, Vernesi C, Shapiro B, Ciucci P, Bertorelle G (2017) Survival and divergence in a small group: the extraordinary genomic history of the endangered Apennine brown bear stragglers. Proc Natl Acad Sci USA 114:E9589–E9597. https://doi.org/10.1073/pnas.1707279114
Bewg WP, Ci D, Tsai C-J (2018) Genome editing in trees: from multiple repair pathways to long-term stability. Front Plant Sci 9:1732. https://doi.org/10.3389/fpls.2018.01732
Borges AA, Pereira AF (2019) Potential role of intraspecific and interspecific cloning in the conservation of wild mammals. Zygote 27:111–117. https://doi.org/10.1017/S0967199419000170
Boscari E, Marino IAM, Caruso C, Gessner J, Lari M, Mugue N, Barmintseva A, Suciu R, Onara D, Zane L, Congiu L (2021) Defining criteria for the reintroduction of locally extinct populations based on contemporary and ancient genetic diversity: the case of the Adriatic Beluga sturgeon ( Huso huso ). Divers Distrib 27:816–827. https://doi.org/10.1111/ddi.13230
Bourgeois YX, Warren BH (2021) An overview of current population genomics methods for the analysis of whole-genome resequencing data in eukaryotes. Mol Ecol. https://doi.org/10.1111/mec.15989
Bragg JG, Cuneo P, Sherieff A, Rossetto M (2020) Optimizing the genetic composition of a translocation population: Incorporating constraints and conflicting objectives. Mol Ecol Resour 20:54–65. https://doi.org/10.1111/1755-0998.13074
Breed MF, Harrison PA, Blyth C, Byrne M, Gaget V, Gellie NJC, Groom SVC, Hodgson R, Mills JG, Prowse TAA, Steane DA, Mohr JJ (2019) The potential of genomics for restoring ecosystems and biodiversity. Nat Rev Genet 20(10):615–628. https://doi.org/10.1038/s41576-019-0152-0
Brown AHD, Marshall DR (1995) A basic sampling strategy: theory and practice. Collecting plant genetic diversity: technical guidelines, vol 75. CAB International, Wallingford, p 91
Google Scholar
Browne L, Wright JW, Fitz-Gibbon S, Gugger PF, Sork VL (2019) Adaptational lag to temperature in valley oak ( Quercus lobata ) can be mitigated by genome-informed assisted gene flow. Proc Natl Acad Sci 116:25179–25185. https://doi.org/10.1073/pnas.1908771116
Burt A, Trivers R (2006) Genes in conflict—the biology of selfish genetic elements. Harvard University Press, Cambridge. https://doi.org/10.4159/9780674029118
Book Google Scholar
Burt A, Coulibaly M, Crisanti A, Diabate A, Kayondo JK (2018) Gene drive to reduce malaria transmission in sub-Saharan Africa. J Responsib Innov 5:66–80. https://doi.org/10.1080/23299460.2017.1419410
Byers O, Lees C, Wilcken J, Schwitzer C (2013) The one plan approach: the philosophy and implementation of CBSG’s approach to integrated species conservation planning. WAZA Magazine 14:2–5
Caballero A, Bravo I, Wang J (2017) Inbreeding load and purging: implications for the short-term survival and the conservation management of small populations. Heredity 118:177–185. https://doi.org/10.1038/hdy.2016.80
Campbell KJ, Saah JR, Brown PR, Godwin J, Gould F, Howald GR, Piaggio A, Thomas P, Tompkins DM, Threadgill D, Delborne J, Kanavy DM, Kuiken T, Packard H, Serr M, Shiels A (2019) A potential new tool for the toolbox: assessing gene drives for eradicating invasive rodent populations. In: Veitch CR, Clout MN, Martin AR, Russell JC, West CJ (eds) Island invasives: scaling up to meet the challenge. International Union for Conservation of Nature and Natural Resources, Gland, pp 6–14
Caplancq T, Fitzpatrick MC, Bay RA, Exposito-Alonso M, Keller SR (2020) Genomic prediction of (Mal) adaptation across current and future climatic landscapes. Annu Rev Ecol Evol Syst 51:245–269. https://doi.org/10.1146/annurev-ecolsys-020720-042553
Carvalho CS, Forester BR, Mitre SK, Alves R, Imperatriz-Fonseca VL, Ramos SJ, Resende-Mpreira LC, Siqueira JO, Trevelin LC, Caldeira CF, Gastauer M, Jaffé R (2021) Combining genotype, phenotype, and environmental data to delineate site-adjusted provenance strategies for ecological restoration. Mol Ecol Resour 21:44–58. https://doi.org/10.1111/1755-0998.13191
Casas-Marce M, Soriano L, López-Bao JV, Godoy JA (2013) Genetics at the verge of extinction: insights from the Iberian lynx. Mol Ecol 22:5503–5515. https://doi.org/10.1111/mec.12498
Casas-Marce M, Marmesat E, Soriano L, Martínez-Cruz B, Lucena-Perez M, Nocete F, Rodríguez-Hidalgo A, Canals A, Nadal J, Detry C, Bernáldez-Sánchez E, Fernández-Rodríguez C, Pérez-Ripoll M, Stiller M, Hofreiter M, Rodríguez A, Revilla E, Delibes M, Godoy JA (2017) Spatiotemporal dynamics of genetic variation in the Iberian lynx along its path to extinction reconstructed with ancient DNA. Mol Biol Evol 34(11):2893–2907. https://doi.org/10.1093/molbev/msx222
Cauwelier E, Gilbey J, Sampayo J, Stradmeyer L, Sj M (2018) Identification of a single genomic region associated with seasonal river return timing in adult Scottish Atlantic salmon ( Salmo salar ), using a genome-wide association study. Can J Fish Aquat Sci 75:1427–1435. https://doi.org/10.1139/CJFAS-2017-0293
Article CAS Google Scholar
CBD (2020) Secretariat of the Convention on Biological Diversity. Global biodiversity outlook 5 – Summary for policy makers
Ceccarelli V, Fremout T, Zavaleta D, Lastra S, Correa SI, Arévalo-Gardini E, Rodriguez CA, Cruz Hilacondo W, Thomas E (2021) Climate change impact on cultivated and wild cacao in Peru and the search of climate change-tolerant genotypes. Divers Distrib 2021(00):1–15. https://doi.org/10.1111/ddi.13294
Charlesworth D, Charlesworth B (1999) The genetic basis of inbreeding depression. Genet Res 74:329–340. https://doi.org/10.1017/S0016672399004152
Che-Castaldo J, Gray SM, Rodriguez-Clark KM, Schad Eebes K, Faust LJ (2021) Expected demographic and genetic declines not found in most zoo and aquarium populations. Front Ecol Environ 2021:1–8. https://doi.org/10.1002/fee.2362
Christmas MJ, Wallberg A, Bunikis I, Olsson A, Wallerman O, Webster MT (2019) Chromosomal inversions associated with environmental adaptation in honeybees. Mol Ecol 28:1358–1374. https://doi.org/10.1111/mec.14944
Clark MS, Peck LS, Thyrring J (2021) Resilience in greenland intertidal mytilus: the hidden stress defense. Sci Total Environ 767(9):144366. https://doi.org/10.1016/j.scitotenv.2020.144366
Clavero M, García-Berthou E (2005) Invasive species are a leading cause of animal extinctions. Trends Ecol Evol 20(3):110. https://doi.org/10.1016/j.tree.2005.01.003
Cleves AP, Tinoco AI, Bradford J, Perrin D, Bay LK, Pringle JR (2020) Reduced thermal tolerance in a coral carrying CRISPR-induced mutations in the gene for a heat-shock transcription factor. Proc Natl Acad Sci USA 117(46):28899–28905. https://doi.org/10.1073/pnas.1920779117
Colicchio JM, Herman J (2020) Empirical patterns of environmental variation favor adaptive transgenerational plasticity. Ecol Evol 10:1648–1665
Comizzoli P, Wildt DE (2017) Cryobanking biomaterials from wild animal species to conserve genes and biodiversity: relevance to human biobanking and biomedical research. In: Hainaut P, Vaught J, Zatloukal K, Pasterk M (eds) Biobanking of human biospecimens. Springer, Cham, pp 217–235. https://doi.org/10.1007/978-3-319-55120-3_13
Chapter Google Scholar
Cooper GM, Stone EA, Asimenos G, Green ED, Batzoglou S, Sidow A (2005) Distribution and intensity of constraint in mammalian genomic sequence. Genome Res 15:901–913. https://doi.org/10.1101/gr.3577405
De Villemereuli P, Rutschmann A, Lee KD, Ewen JG, Brekke P, Santure AW (2019) Little adaptive potential in a threatened passerine bird. Curr Biol 29:889–894. https://doi.org/10.1016/j.cub.2019.01.072
Díez-Del-Molino D, von Seth J, Gyllenstrand N, Widemo F, Liljebäck N, Svensson M, Sjögren-Gulve P, Dalén L (2020) Population genomics reveals lack of greater white-fronted introgression into the Swedish lesser white-fronted goose. Sci Rep 10:18347. https://doi.org/10.1038/s41598-020-75315-y
DiFazio SP, Leonardi S, Slavov GT, Garman SL, Adams WT, Strauss SH (2012) Gene flow and simulation of transgene dispersal from hybrid poplar plantations. New Phytol 193:903–915
Dixon G, Davies SW, Aglyamova G, Meyer E, Bay LK, Matz M (2015) Genomic determinants of coral heat tolerance across latitudes. Science 348:1460–1462
Dort EN, Tanguay Ph, Hamelin RC (2020) CRISPR/Cas9 gene editing: an unexplored frontier for forest pathology. Front Plant Sci 11:1126. https://doi.org/10.3389/fpls.2020.01126
Dussex N, von Seth J, Robertson BC, Dalén L (2018) Full mitogenomes in the critically endangered Kākāpō reveal major post-glacial and anthropogenic effects on neutral genetic diversity. Genes (basel) 9(4):220. https://doi.org/10.3390/genes9040220
Ekblom R, Galindo J (2011) Applications of next generation sequencing in molecular ecology of nonmodel organisms. Heredity 107:1–15. https://doi.org/10.1038/hdy.2010.152
Elorriaga E, Klocko AL, Ma C, Strauss SH (2018) Variation in mutation spectra among CRISPR/Cas9 mutagenized poplars. Front Plant Sci 9:594. https://doi.org/10.3389/fpls.2018.00594
Exposito-Alonso M, Vasseur F, Ding W, Wang G, Burbano HA, Weigel D (2018) Genomic basis and evolutionary potential for extreme drought adaptation in Arabidopsis thaliana . Nat Ecol Evol 2:352–358. https://doi.org/10.1038/s41559-017-0423-0
Fant JB, Havens K, Kramer AT, Walsh SK, Calicrate T, Lacy RC, Maunder M, Meyer AH, Smith PP (2016) What to do when we can’t bank on seeds: what botanic gardens can learn from the zoo community about conserving plants in living collections. Am J Bot 103(9):1541–1543. https://doi.org/10.3732/ajb.1600247
Fernández J, Toro MA, Gómez-Romano F, Villanueva B (2016) The use of genomic information can enhance the efficiency of conservation programs. Anim Front 6:59–64. https://doi.org/10.2527/af.2016-0009
Fitzpatrick SW, Funk WC (2019) Genomics for genetic rescue. In: Rajora OP (ed) Population genomics. Springer, Cham, pp 1–35. https://doi.org/10.1007/13836_2019_64
Fitzpatrick SW, Bradburd GS, Kremer CT, Salerno PE, Angeloni LM, Funk WC (2020) Genomic and fitness consequences of genetic rescue in wild populations. Curr Biol 30(3):517–522. https://doi.org/10.1016/j.cub.2019.11.062
Flanagan SP, Forester BR, Latch EK, Aitken SN, Hoban S (2018) Guidelines for planning genomic assessment and monitoring of locally adaptive variation to inform species conservation. Evol Appl 11:1035–1052. https://doi.org/10.1111/eva.12569
Frandsen P, Fontsere C, Nielsen SV, Hanghøj K, Castejon-Fernandez N, Lizano E, Hughes D, Hernandez-Rodriguez J, Korneliussen TS, Carlsen F, Siegismund HR, Mailund T, Marques-Bonet T, Hvilsom C (2020) Targeted conservation genetics of the endangered chimpanzee. Heredity 125:15–27. https://doi.org/10.1038/s41437-020-0313-0
Frankham R (2008) Genetic adaptation to captivity in species conservation programs. Mol Ecol 17(1):325–333. https://doi.org/10.1111/j.1365-294X.2007.03399.x
Frankham R (2015) Genetic rescue of small inbred populations: meta-analysis reveals large and consistent benefits of gene flow. Mol Ecol 24(11):2610–2618. https://doi.org/10.1111/mec.13139
Fulgione D, Rippa D, Buglione M et al (2016) Unexpected but welcome. artificially selected traits may increase fitness in wild boar. Evol Appl 9:769–776
Galla SJ, Moraga R, Brown L, Cleland S, Hoeppner MP, Maloney RF, Richardson A, Slater L, Santure AW, Steeves TE (2020) A comparison of pedigree, genetic and genomic estimates of relatedness for informing pairing decisions in two critically endangered birds: Implications for conservation breeding programmes worldwide. Evol Appl 2020(13):991–1008. https://doi.org/10.1111/eva.12916
Gallego-Bartolomé J (2020) DNA methylation in plants: mechanisms and tools for targeted manipulation. New Phytol 227:38–44. https://doi.org/10.1111/nph.16529
Gama MAM, Carolino I, Landi V, Delgado JV, Vicente AA et al (2013) Genetic structure, relationships and admixture with wild relatives in native pig breeds from Iberia and its islands. Genet Sel Evol 45:18
Gantz VM, Jasinskiene N, Tatarenkova O, Fazekas A, Macias VM, Bier E, James AA (2015) Highly efficient Cas9-mediated gene drive for population modification of the malaria vector mosquito Anopheles stephensi . Proc Natl Acad Sci USA 112(49):E6736-6743. https://doi.org/10.1073/pnas.1521077112
Garrood WT, Kranjc N, Petri K, Kim DY, Guo JA, Hammond AM, Morianou I, Pattanayak V, Joung JK, Crisanti A, Simoni A (2021) Analysis of off-target effects in CRISPR-based gene drives in the human malaria mosquito. Proc Natl Acad Sci USA 118(22):e2004838117. https://doi.org/10.1073/pnas.2004838117
Georges M, Charlier C, Hayes B (2019) Harnessing genomic information for livestock improvement. Nat Rev Genet 20(3):135–156. https://doi.org/10.1038/s41576-018-0082-2
Gienapp PG, Calus MPL, Laine VN, Visser ME (2019) Genomic selection on breeding time in a wild bird population. Evol Lett 3:142–151. https://doi.org/10.1002/evl3.103
Gilpin ME, Soulé ME (1986) Minimal viable populations: process of species extinction. In: Soulé M (ed) Conservation biology: the science of scarcity and diversity. Sinauer, Sunderland, pp 10–34
Glen AS, Dickman CR, Soule ME, Mackey BG (2007) Evaluating the role of the dingo as a trophic regulator in Australian ecosystems. Austral Ecol 32:492–501. https://doi.org/10.1111/j.1442-9993.2007.01721.x
Glen AS, Atkinson R, Campbell KJ, Hagen E, Holmes ND, Keitt BS, Parkes JP, Saunders A, Sawyer J, Torres H (2013) Eradicating multiple invasive species on inhabited islands: the next big step in island restoration? Biol Invasions 15:2589–2603. https://doi.org/10.1007/s10530-013-0495-y
Global Invasive Species Database (2020) Species profile: Agrilus planipennis . http://www.iucngisd.org/gisd/species.php?sc=722 . Accessed 17 Dec 2020
Gomes Viana JP, Bohrer Monteiro Siqueira MV, Araujo FL, Grando C, Sanae Sujii P, Silvestre EDA et al (2018) Genomic diversity is similar between Atlantic Forest restorations and natural remnants for the native tree Casearia sylvestris . PLoS ONE 13:e0192165
Grueber CE, Farquharson KA, Wright BR, Wallis GP, Hogg CJ, Belov K (2021) First evidence of deviation from Mendelian proportions in a conservation program. Mol Ecol. https://doi.org/10.1111/mec.16004
Hamelin RC, Roe AD (2020) Genomic biosurveillance of forest invasive alien enemies: a story written in code. Evol Appl 13:95–115. https://doi.org/10.1111/eva.12853
Hamilton JA, Royauté R, Wright JW, Hodgskiss P, Ledig FT (2017) Genetic conservation and management of the California endemic, Torrey pine ( Pinus torreyana Parry): implications of genetic rescue in a genetically depauperate species. Ecol Evol 7(18):7370–7381. https://doi.org/10.1002/ece3.3306
Hammond A, Galizi R, Kyrou K et al (2016) A CRISPR-Cas9 gene drive system targeting female reproduction in the malaria mosquito vector Anopheles gambiae . Nat Biotechnol 34:78–83. https://doi.org/10.1038/nbt.3439
Hansson B, Morales HE, Van Oosterhout C (2021) Comment on “individual heterozygosity predicts translocation success in threatened desert tortoises. Science 372:eabh1105. https://doi.org/10.1126/science.abh1105
Harvey-Samuel T, Ant T, Alphey L (2017) Towards the genetic control of invasive species. Biol Invasions 19(6):1683–1703. https://doi.org/10.1007/s10530-017-1384-6
Hasselgren M, Dussex N, von Seth J, Angerbjörn A, Olsen R-A, Dalén L, Norén K (2021) Genomic and fitness consequences of inbreeding in an endangered carnivore. Mol Ecol 30:2790–2799. https://doi.org/10.1111/mec.15943
He XP, Johansson ML, Heath DD (2016) Role of genomics and transcriptomics in selection of reintroduction source populations. Conserv Biol 30:1010–1018
Hedrick PW (2010) Cattle ancestry in bison: explanations for higher mtDNA than autosomal ancestry. Mol Ecol 19:3328–3335
Hedrick PW, Fredrickson R (2010) Genetic rescue guidelines with examples from Mexican wolves and Florida panthers. Conserv Genet 11:615–626. https://doi.org/10.1007/s10592-009-9999-5
Hedrick PW, Garcia-Dorado A (2016) Understanding inbreeding depression, purging, and genetic rescue. Trends Ecol Evol 31(12):940–952. https://doi.org/10.1016/j.tree.2016.09.005
Hernandez D (2020) Scientists cloned the most endangered horse in the world—from 40-year-old DNA. Popular mechanic. https://www.popularmechanics.com/science/animals/a34362850/baby-horse-cloned-40-year-old-dna/ . Accessed 8 June 2021
Heywood VH, Iriondo JM (2003) Plant conservation: old problems, new perspectives. Biol Conserv 113(3):321–335. https://doi.org/10.1016/S0006-3207(03)00121-6
Hoban SM, McCleary TS, Schlarbaum SE, Anagnostakis SL, Romero-Severson J (2012) Human-impacted landscapes facilitate hybridization between a native and an introduced tree. Evol Appl 5:720–731
Hoban S et al (2021) Global commitments to conserving and monitoring genetic diversity are now necessary and feasible. Bioscience. https://doi.org/10.1093/biosci/biab054
Hoffmann AA, Montgomery BL, Popovici I, Iturbe-Ormaetxe I, John-son PH, Muzzi F, Greenfield M, Durkan M, Leong YS, Dong Y, CookH AJ, Callahan AG, Kenny N, Omodei C, McGraw EA, Ryan PA, Ritchie SA, Turelli M, O’Neill SL (2011) Successful establishment of Wolbachia in Aedes populations to suppress dengue transmission. Nature 476:454–457
Hoffmann AA, Miller AD, Weeks AR (2021) Genetic mixing for population management: from genetic rescue to provenancing. Evol Appl 14:634–652. https://doi.org/10.1111/eva.13154
Hogg CJ, Wright B, Morris KM, Lee AV, Ivy JA, Grueber CE, Belov K (2019) Founder relationships and conservation management: empirical kinships reveal the effect on breeding programmes when founders are assumed to be unrelated. Anim Conserv 22:348–361. https://doi.org/10.1111/acv.12463
Hohenlohe PA, McCallum H, Jones M, Lawrance M, Hamede RK, Storfer A (2019) Conserving adaptive potential: lessons from Tasmanian devils and their transmissible cancer. Conserv Genet 20:81–87. https://doi.org/10.1007/s10592-019-01157-5
Hohenlohe PA, Funk C, Rajora OP (2021) Population genomics for wildlife conservation and management. Mol Ecol 30(1):62–82. https://doi.org/10.1111/mec.15720
Houde ALS, Garner SR, Neff BD (2015) Restoring species through reintroductions: strategies for source population selection. Restor Ecol 23:746–753
Howard-McCombe J, Ward D, Kitchener A, Lawson D, Senn H, Beaumont M (2021) On the use of genome-wide data to model and date the time of anthropogenic hybridisation: an example from the Scottish wildcat. Mol Ecol. https://doi.org/10.22541/au.161466378.84293954/v1
Howell LG, Frankham R, Rodger JC, Witt RR, Clulow S, Upton RMO, Clulow J (2021) Integrating biobanking minimises inbreeding and produces significant cost benefits for a threatened frog captive breeding programme. Conserv Lett 14(2):1–9. https://doi.org/10.1111/conl.12776
Imbler S (2021) Meet Elizabeth Ann, the first cloned black-footed ferret. The New York Times. https://www.nytimes.com/2021/02/18/science/black-footed-ferret-clone.html . Accessed 8 June 2021
Johnson WE, Onorato DP, Roelke ME, Land ED, Cunningham M, Belden RC, McBride D, Jansen M, Lotz D, Shindle J, Howard DE, Wildt LM, Penfold JA, Hostetler R, Oli MK, O’Brien SJ (2010) Genetic restoration of the Florida panther. Science 329(5999):1641–1645. https://doi.org/10.1126/science.1192891
Kardos M, Shafer ABA (2018) The peril of gene-targeted conservation. Trends Ecol Evol 33:827–839. https://doi.org/10.1016/j.tree.2018.08.011
Kardos M, Husby A, McFarlane SE, Qvarnström A, Ellegren H (2016) Whole-genome resequencing of extreme phenotypes in collared flycatchers highlights the difficulty of detecting quantitative trait loci in natural populations. Mol Ecol Res 16:727–741
Kelly L, Plumb W, Carey D, Mason M, Cooper E, Crowther W, Whittemore A, St R, Koch J, Buggs R (2019) Genes for ash tree resistance to an insect pest identified via comparative genomics. Nat Ecol Evol. https://doi.org/10.1101/772913
Kenis M, Auger-Rozenberg MA, Roques A et al (2009) Ecological effects of invasive alien insects. Biol Invasions 11:21–45. https://doi.org/10.1007/s10530-008-9318-y
Kijowska-Oberc J, Staszak AM, Kamiński J, Ratajczak E (2020) Adaptation of forest trees to rapidly changing climate. Forests 11:123. https://doi.org/10.3390/f11020123
Kircher M, Witten DM, Jain P, O’Roak BJ, Cooper GM, Shendure J (2014) A general framework for estimating the relative pathogenicity of human genetic variants. Nat Genet 46(3):310–315. https://doi.org/10.1038/ng.2892
Kleinman-Ruiz D, Soriano L, Casas-Marce M, Szychta C, Sánchez I, Fernández J, Godoy JA (2019) Genetic evaluation of the Iberian lynx ex situ conservation programme. Heredity (edinb) 123(5):647–661. https://doi.org/10.1038/s41437-019-0217-z
Kristensen TN, Hoffmann AA, Pertoldi C, Stronen AV (2015) What can livestock breeders learn from conservation genetics and vice versa? Front Genet 6:38
Kyriazis CC, Wayne RK, Lohmueller KE (2021) Strongly deleterious mutations are a primary determinant of extinction risk due to inbreeding depression. Evol Lett 5:33–47. https://doi.org/10.1002/evl3.209
Kyrou K, Hammond AM, Galizi R, Kranjc N, Burt A, Beaghton AK, Nolan T, Crisanti A (2018) A CRISPR–Cas9 gene drive targeting doublesex causes complete population suppression in caged Anopheles gambiae mosquitoes. Nat Biotechnol 36:1062–1066. https://doi.org/10.1038/nbt.4245
Lacy RC, Ballou JD, Pollack JP (2012) PMx: Software package for the demographic and genetic analysis and management of pedigreed populations. Methods Ecol Evol 3:433–437. https://doi.org/10.1111/j.2041-210X.2011.00148.x
Lane T, Best T, Zembower N, Davitt JN, Xu Y, Koch J, Liang H, McGraw J, Schuster S, Shim D, Coggeshall MV, Carlson JE, Staton ME (2016) The green ash transcriptome and identification of genes responding to abiotic and biotic stress. BMC Genom. https://doi.org/10.1186/s12864-016-3052-0
Lester PJ, Bulgarella M, Baty JW, Dearden PK, Guhlin J, Kean JM (2020) The potential for a CRISPR gene drive to eradicate or suppress globally invasive social wasps. Sci Rep 10(1):12398. https://doi.org/10.1038/s41598-020-69259-6
Lindholm AK, Dyer KA, Firman RC, Fishman L, Forstmeier W, Holman L, Johannesson H, Knief U, Kokko H, Larracuente AM, Manser A, Montchamp-Moreau C, Petrosyan VG, Pomiankowski A, Presgraves DC, Safronova LD, Sutter A, Unckless R, Verspoor RL, Wedell N, Wilkinson G, Price T (2016) The ecology and evolutionary dynamics of meiotic drive. Trends Ecol Evol 31(4):315–326. https://doi.org/10.1016/j.tree.2016.02.001
Liu L, Bosse M, Megens HJ, de Visser M, Groenen MAM, Madsen O (2020) Genetic consequences of long-term small effective population size in the critically endangered pygmy hog. Evol Appl 14(3):710–720. https://doi.org/10.1111/eva.13150
Loi P, Ptak G, Barboni B, Fulka J, Cappai P, Clinton M (2001) Genetic rescue of an endangered mammal by cross-species nuclear transfer using post-mortem somatic cells. Nat Biotechnol 19:962–964. https://doi.org/10.1038/nbt1001-962
López-Cortegano E, Pouso R, Labrador A, Pérez-Figueroa A, Fernández J, Caballero A (2019) Optimal management of genetic diversity in subdivided populations. Front Genet 10:843. https://doi.org/10.3389/fgene.2019.00843
Lowe S, Browne M, Boudjelas S, De Poorter M (2000) 100 of the world’s worst invasive alien species a selection from the global invasive species database. The Invasive Species Specialist Group (ISSG)
Luo L, Kong X, Gao Z, Zheng Y, Yang Y, Li X, Yang D, Geng Y, Yang Y (2020) Comparative transcriptome analysis reveals ecological adaption of cold tolerance in northward invasion of Alternanthera philoxeroides . BMC Genom 21(1):532. https://doi.org/10.1186/s12864-020-06941-z
Madsen T, Shine R, Olsson M, Wittzell H (1999) Restoration of an inbred adder population. Nature 402(6757):34–35. https://doi.org/10.1038/46941
Marin P, Genitoni J, Barloy D, Maury S, Gibert P, Ghalambor CK, Vieira C (2020) Biological invasion: the influence of the hidden side of the (epi) genome. Funct Ecol 34(2):385–400. https://doi.org/10.1111/1365-2435.13317
Marshall TC, Spalton JA (2000) Simultaneous inbreeding and outbreeding depression in reintroduced Arabian oryx. Anim Conserv 3:241–248. https://doi.org/10.1111/j.1469-1795.2000.tb00109.x
Mascher M, Schreiber M, Scholz U, Graner A, Jochen C, Reif JC, Stein N (2019) Genebank genomics bridges the gap between the conservation of crop diversity and plant breeding. Nat Genet 51:1076–1081. https://doi.org/10.1038/s41588-019-0443-6
Mattucci F, Galaverni M, Lyons LA, Alves PC, Randi E, Velli E, Pagani L, Caniglia R (2019) Genomic approaches to identify hybrids and estimate admixture times in European wildcat populations. Sci Rep 9(1):11612. https://doi.org/10.1038/s41598-019-48002-w
McGowan PJ, Traylor-Holzer K, Leus K (2017) IUCN guidelines for determining when and how ex situ management should be used in species conservation. Conserv Lett 10:361–366. https://doi.org/10.1111/conl.12285
McLean-Rodríguez FD, Costich DE, Camacho-Villa TC, Pè ME, Dell’Acqua M (2021) Genetic diversity and selection signatures in maize landraces compared across 50 years of in situ and ex situ conservation. Heredity 126:913–928. https://doi.org/10.1038/s41437-021-00423-y
McLennan EA, Grueber CE, Wise P, Belov K, Hogg CJ (2020) Mixing genetically differentiated populations successfully boosts diversity of an endangered carnivore. Anim Conserv 23:700–712. https://doi.org/10.1111/acv.12589
Merkle SA, Andrade GM, Nairn CJ, Powell WA, Maynard CA (2007) Restoration of threatened species: a noble cause for transgenic trees. Tree Genet Genom 3(2):111–118. https://doi.org/10.1007/s11295-006-0050-4
Messing RH, Wright MG (2006) Biological control of invasive species: solution or pollution? Front Ecol Environ 4:132–140. https://doi.org/10.1890/1540-9295(2006)004[0132:BCOISS]2.0.CO;2
Meuwissen THE, Hayes BJ, Goddard ME (2001) Prediction of total genetic value using genome wide dense marker maps. Genetics 157(4):1819–1829
Miller JM, Quinzin MC, Poulakakis N, Gibbs JP, Beheregaray LB, Garrick RC, Russello MA, Ciofi C, Edwards DL, Hunter EA, Tapia W, Rueda D, Carrión J, Valdivieso AA, Caccone A (2017) Identification of genetically important individuals of the rediscovered Floreana Galapagos giant tortoise ( Chelonoidis elephantopus ) provide founders for species restoration program. Sci Rep 7:11471. https://doi.org/10.1038/s41598-017-11516-2
Miller W, Wright SJ, Zhang Y, Schuster SC, Hayes VM (2010) Optimization methods for selecting founder individuals for captive breeding or reintroduction of endangered species. Pac Symp Biocomput 2010:43–53. https://doi.org/10.1142/9789814295291_0006
Muchero W, Sondreli KL, Chen J-G et al (2018) Association mapping, transcriptomics, and transient expression identify candidate genes mediating plant–pathogen interactions in a tree. PNAS 115:11573–11578
Naidoo S, Slippers B, Plett JM, Coles D, Oates CN (2019) The road to resistance in forest trees. Front Plant Sci 10:273. https://doi.org/10.3389/fpls.2019.00273
National Academies Science, Engineering, Medicine (2016) Gene drives on the horizon. National Academies Press, Washington, D.C.
Ng PC, Henikoff S (2003) SIFT: predicting amino acid changes that affect protein function. Nucleic Acids Res 31(13):3812–3814. https://doi.org/10.1093/nar/gkg509
North HL, McGaughran A, Jiggins C (2021) Insights into invasive species from whole-genome resequencing. Mol Ecol. https://doi.org/10.1111/mec.15999
Ogden R, Chuven J, Gilbert T, Hosking C, Gharbi K, Craig M, Dhaheri SA, Senn H (2020) Benefits and pitfalls of captive conservation genetic management: evaluating diversity in scimitar-horned oryx to support reintroduction planning. Biol Conserv 241:108244. https://doi.org/10.1016/j.biocon.2019.108244
Oh KP, Shiels AB, Shiels L, Blondel DV, Campbell KJ, Saah JR, Lloyd AL, Thomas PQ, Gould F, Abdo Z, Godwin JR, Piaggio AJ (2021) Population genomics of invasive rodents on islands: genetic consequences of colonization and prospects for localized synthetic gene drive. Evol Appl 14(5):1421–1435. https://doi.org/10.1111/eva.13210
Oliveira R, Godinho R, Randi E, Alves PC (2008) Hybridization versus conservation: are domestic cats threatening the genetic integrity of wildcats ( Felis silvestris silvestris) in Iberian Peninsula. Phil Trans R Soc Lond B 363:2953–2961. https://doi.org/10.1098/rstb.2008.0052
Ortego J, Gugger PF, Sork VL (2018) Genomic data reveal cryptic lineage diversification and introgression in Californian golden cup oaks (section Protobalanus). New Phytol 218:804–818
Ouborg NJ, Pertoldi C, Loeschcke V, Bijlsma RK, Hedrick PW (2010) Conservation genetics in transition to conservation genomics. Trends Genet 26(4):177–187. https://doi.org/10.1016/j.tig.2010.01.001
Ouédraogo D, Ouédraogo-Koné S, Yougbaré B, Soudré A, Zoma-Traoré B, Mészáros G, Khayatzadeh N, Traoré A, Sanou M, Mwai OA, Wurzinger M, Burger PA, Sölkner J (2021) Population structure, inbreeding and admixture in local cattle populations managed by community-based breeding programs in Burkina Faso. J Anim Breed Genet 138(3):379–388. https://doi.org/10.1111/jbg.12529
Pendleton AL, Shen F, Taravella AM, Emery S, Veeramah KR, Boyko AR, Kidd JM (2018) Comparison of village dog and wolf genomes highlights the role of the neural crest in dog domestication. BMC Biol 16(1):64. https://doi.org/10.1186/s12915-018-0535-2
Perry GH, Marioni JC, Melsted P, Gilad Y (2010) Genomic-scale capture and sequencing of endogenous DNA from feces. Mol Ecol 19:5332–5344
Piaggio AJ, Segelbacher G, Seddon PJ, Alphey L, Bennett EL, Carlson RH, Friedman RM, Kanavy D, Phelan R, Redford KH, Rosales M, Slobodian L, Wheeler K (2017) Is it time for synthetic biodiversity conservation? Trends Ecol Evol 32(2):97–107. https://doi.org/10.1016/j.tree.2016.10.016
Pickup M, Young AG (2008) Population size, self-incompatibility and genetic rescue in diploid and tetraploid races of Rutidosis leptorrhynchoides (Asteraceae). Heredity 100:268–274
Pollard KS, Hubisz MJ, Rosenbloom KR, Siepel A (2010) Detection of nonneutral substitution rates on mammalian phylogenies. Genome Res 20(1):110–121. https://doi.org/10.1101/gr.097857.109
Powell W, Newhouse A, Coffey V (2019) Developing blight-tolerant American chestnut trees. Cold Spring Harb Perspect Biol 11(7):a034587. https://doi.org/10.1101/cshperspect.a034587
Prentis PJ, Pavasovic A (2013) Understanding the genetic basis of invasiveness. Mol Ecol 22:2366–2368. https://doi.org/10.1111/mec.12277
Prentis PJ, Wilson JR, Dormontt EE, Richardson DM, Lowe AJ (2008) Adaptive evolution in invasive species. Trends Plant Sci 13(6):288–294. https://doi.org/10.1016/j.tplants.2008.03.004
Price TAR, Windbichler N, Unckless RL, Sutter A, Runge JN, Ross PA, Pomiankowski A, Nuckolls NL, Montchamp-Moreau C, Mideo N, Martin OY, Manser A, Legros M, Larracuente AM, Holman L, Godwin J, Gemmell N, Courret C, Buchman A, Barrett LG, Lindholm AK (2020) Resistance to natural and synthetic gene drive systems. J Evol Biol 33(10):1345–1360. https://doi.org/10.1111/jeb.13693
Primmer CR (2009) From conservation genetics to conservation genomics. Ann N Y Acad Sci 1162:357–368. https://doi.org/10.1111/j.1749-6632.2009.04444.x
Pritchard D, Fa J, Oldfield S, Harrop S (2012) Bring the captive closer to the wild: redefining the role of ex situ conservation. Oryx 46(1):18–23. https://doi.org/10.1017/S0030605310001766
Ralls K, Sunnucks P, Lacy RC, Frankham R (2020) Genetic rescue: a critique of the evidence supports maximizing genetic diversity rather than minimizing the introduction of putatively harmful genetic variation. Biol Conserv 251:108784. https://doi.org/10.1016/j.biocon.2020.108784
Redford KH, Brooks TM, Macfarlane NBW, Adams JS (eds) (2019) Genetic frontiers for conservation: An assessment of synthetic biology and biodiversity conservation. Technical assessment.Gland, Switzerland: IUCN. xiv + 166pp.
Rey O, Eizaguirre C, Angers B, Baltazar-Soares M, Sagonas K, Prunier JG, Blanchet S (2020) Linking epigenetics and biological conservation: towards a conservation epigenetics perspective. Funct Ecol 34:414–427. https://doi.org/10.1111/1365-2435.13429
Robinson JA, Ortega-Del Vecchyo D, Fan Z, Kim BY, vonHoldt BM, Marsden CD, Lohmueller KE, Wayne RK (2016) Genomic flatlining in the Endangered Island Fox. Curr Biol 26:1183–1189. https://doi.org/10.1016/j.cub.2016.02.062
Robinson JA, Brown C, Kim BY, Lohmueller KE, Wayne RK (2018) Purging of strongly deleterious mutations explains long-term persistence and absence of inbreeding depression in island foxes. Curr Biol 28(21):3487–3494. https://doi.org/10.1016/j.cub.2018.08.066
Robinson JA, Räikkönen J, Vucetich LM et al (2019) Genomic signatures of extensive inbreeding in Isle Royale wolves, a population on the threshold of extinction. Sci Adv 5(5):eaau0757. https://doi.org/10.1126/sciadv.aau0757
Robinson JA, Bowie RCK, Dudchenko O, Aiden EL, Hendrickson SL, Steiner CC, Ryder OA, Mindell DP, Wall JD (2021a) Genome-wide diversity in the California condor tracks its prehistoric abundance and decline. Curr Biol S0960–9822(21):00548. https://doi.org/10.1016/j.cub.2021.04.035
Robinson ZL, Bell DA, Dhendup T, Luikart G, Whiteley AR, Kardos M (2021b) Evaluating the outcomes of genetic rescue attempts. Conserv Biol 35(2):666–677. https://doi.org/10.1111/cobi.13596
Rochat E, Selmoni O, Joost S (2021) Spatial areas of genotype probability: Predicting the spatial distribution of adaptive genetic variants under future climatic conditions. Divers Distrib 27:1076–1090. https://doi.org/10.1111/ddi.13256
Rode NO, Estoup A, Bourguet D, Courtier-Orgogozo V, Débarre F (2019) Population management using gene drive: molecular design, models of spread dynamics and assessment of ecological risks. Conserv Genet 20:671–690. https://doi.org/10.1007/s10592-019-01165-5
Russello MA, Jensen EL (2018) Ex situ wildlife conservation in the age of population genomics. In: Hohenlohe PA, Rajora OP (eds) Population genomics: wildlife. Population genomics. Springer, Cham. https://doi.org/10.1007/13836_2018_44
Ryder OA, Onuma M (2018) Viable cell culture banking for biodiversity characterization and conservation. Annu Rev Anim Biosci 6:83–98. https://doi.org/10.1146/annurev-animal-030117-014556
Sandler RL, Moses L, Wisely SM (2021) An ethical analysis of cloning for genetic rescue: case study of the black-footed ferret. Biol Conserv 257:109118. https://doi.org/10.1016/j.biocon.2021.109118
Santymire RM, Lonsdorf EV, Lynch CM, Wildt DE, Marinari PE, Kreeger JS, Howard JG (2019) Inbreeding causes decreased seminal quality affecting pregnancy and litter size in the endangered black-footed ferret. Anim Conserv 22:331–340. https://doi.org/10.1111/acv.12466
Savage AE, Zamudio KR (2011) MHC genotypes associate with resistance to a frog-killing fungus. Proc Natl Acad Sci USA 108(40):16705–16710. https://doi.org/10.1073/pnas.1106893108
Schoen DJ, Brown AH (2001) The conservation of wild plant species in seed banks: attention to both taxonomic coverage and population biology will improve the role of seed banks as conservation tools. Bioscience 51(11):960–966
Scott PA, Allison LJ, Field KJ, Averill-Murray RC, Shaffer HB (2020) Individual heterozygosity predicts translocation success in threatened desert tortoises. Science 370(6520):1086–1089. https://doi.org/10.1126/science.abb0421
Seaborn T, Andrews KR, Applestein CV, Breech TM, Garrett MJ, Zaiats A, Caughlin TT (2021) Integrating genomics in population models to forecast translocation success. Restor Ecol 29:e13395. https://doi.org/10.1111/rec.13395
Shafer ABA, Wolf JBW, Alves PC, Bergström L, Bruford MW, Brännström I, Colling G, Dalén L, De Meester L, Ekblom R, Fawcett KD, Fior S, Hajibabaei M, Hill JA, Rus Hoezel A, Höglund J, Jensen EL, Krause J, Kristensen TN, Krützen M, McKay JK, Norman AJ, Ogden R, Österling EM, Ouborg NJ, Piccolo J, Popović D, Primmer CR, Reed FA, Roumet M, Salmona J, Schenekar T, Schwartz MK, Segelbacher G, Senn H, Thaulow J, Valtonen M, Veale A, Vergeer Ph, Vijay N, Vilà C, Weissensteiner M, Wennerström L, Wheat CW, Zieliński P (2015) Genomics and the challenging translation into conservation practice. Trends Ecol Evol 30:78–87. https://doi.org/10.1016/j.tree.2014.11.009
Shryock DF, Washburn LK, DeFalco LA, Esque TC (2021) Harnessing landscape genomics to identify future climate resilient genotypes in a desert annual. Mol Ecol 30(3):698–717. https://doi.org/10.1111/mec.15672
Simoni A, Hammond AM, Beaghton AK et al (2020) A male-biased sex-distorter gene drive for the human malaria vector Anopheles gambiae . Nat Biotechnol 38:1054–1060. https://doi.org/10.1038/s41587-020-0508-1
Soto A, Lorenzo Z, Gil L (2003) Nuclear microsatellite markers for the identification of Quercus ilex L. and Q. suber L. hybrids. Silvae Genet 52:63–66
Stange M, Barrett RDH, Hendry AP (2021) The importance of genomic variation for biodiversity, ecosystems and people. Nat Rev Genet 22(2):89–105. https://doi.org/10.1038/s41576-020-00288-7
Steiner KC, Westbrook JW, Hebard FV, Georgi LL, Powell WA, Fitzsimmons SF (2017) Rescue of American chestnut with extraspecific genes following its destruction by a naturalized pathogen. New for 48(2):317–336. https://doi.org/10.1007/s11056-016-9561-5
Storfer A, Hohenlohe PA, Margres MJ, Patton A, Fraik AK, Lawrance M, Ricci LE, Stahlke AR, McCallum HI, Jones ME (2018) The devil is in the details: genomics of transmissible cancers in Tasmanian devils. PLoS Pathog 14(8):e1007098. https://doi.org/10.1371/journal.ppat.1007098
Supple MA, Shapiro B (2018) Conservation of biodiversity in the genomics era. Genome Biol 19(1):131. https://doi.org/10.1186/s13059-018-1520-3
Taylor SS, Kaila-Sharma P, Weng JH, Aoto P, Schmidt SH, Knapp S, Mathea S, Herberg FW (2020) Kinase domain is a dynamic hub for driving LRRK2 allostery. Front Mol Neurosci 13:538219. https://doi.org/10.3389/fnmol.2020.538219
Teem JL, Alphey L, Descamps S, Edgington MP, Edwards O, Gemmell N, Harvey-Samuel T, Melnick RL, Oh KP, Piaggio AJ, Saah JR, Schill D, Thomas P, Smith T, Roberts A (2020) Genetic biocontrol for invasive species. Front Bioeng Biotechnol 8:452. https://doi.org/10.3389/fbioe.2020.00452
Thormann I, Dulloo M, Engels J (2006) Techniques for ex situ plant conservation. In: Henry RJ (ed) Plant conservation genetics. Haworth Press, Philadelphia, pp 7–36
Toro MA, Villanueva B, Fernández J (2014) Genomics applied to management strategies in conservation programmes. Livest Sci 166:48–53. https://doi.org/10.1016/j.livsci.2014.04.020
Traylor-Holzer K, Leus K, Byers O (2019) Ex situ management for conservation. In: Leal Filho W, Azul A, Brandli L, Özuyar P, Wall T (eds) Life on land. Encyclopedia of the UN sustainable development goals. Springer, Cham
Tsai CJ, Xue LJ (2015) CRISPRing into the woods. GM Crops Food 6:206–215. https://doi.org/10.1080/21645698.2015.1091553
Turvey ST, Crees JJ (2019) Extinction in the anthropocene. Curr Biol 29(19):982–986. https://doi.org/10.1016/j.cub.2019.07.040
van de Crommenacker J, Bourgeois YXC, Warren BH, Jackson H, Fleischer-Dogley F, Groombridge J, Bunbury N (2015) Using molecular tools to guide management of invasive alien species: assessing the genetic impact of a recently introduced island bird population. Divers Distrib 21:1414–1427. https://doi.org/10.1111/ddi.12364
van der Valk T, Díez-del-Molino D, Marques-Bonet T, Guschanski K, Dalén L (2019) Historical genomes reveal the genomic consequences of recent population decline in eastern gorillas. Curr Biol 29(1):165–170. https://doi.org/10.2139/ssrn.3254908
van Oosterhout C (2020) Mutation load is the spectre of species conservation. Nat Ecol Evol 4:1004–1006. https://doi.org/10.1038/s41559-020-1204-8
van Oppen MJH, Gates RD, Blackall LL, Chakravarti LJ, Chan WY, Cormick C, Crean A, Damjanovic K, Epstein H, Harrison PL, Jones TA, Miller M, Pears RJ, Peplow LM, Raftos DA, Schaffelke B, Stewart K, Torda G, Wachenfeld D, Weeks AR, Putnam HM (2017) Shifting paradigms in restoration of the world’s coral reefs. Glob Change Biol 23(9):3437–3448. https://doi.org/10.1111/gcb.13647
Volis S, Blecher M (2010) Quasi in situ: a bridge between ex situ and in situ conservation of plants. Biodivers Conserv 19:2441–2454
von Seth J, Dussex N, Díez-Del-Molino D, van der Valk T, Kutschera VE, Kierczak M, Steiner CC, Liu S, Gilbert MTP, Sinding MS, Prost S, Guschanski K, Nathan SKSS, Brace S, Chan YL, Wheat CW, Skoglund P, Ryder OA, Goossens B, Götherström A, Dalén L (2021) Genomic insights into the conservation status of the world’s last remaining Sumatran rhinoceros populations. Nat Commun 12(1):2393. https://doi.org/10.1038/s41467-021-22386-8
von Thaden A, Nowak C, Tiesmeyer A et al (2020) Applying genomic data in wildlife monitoring: development guidelines for genotyping degraded samples with reduced single nucleotide polymorphism (SNP) panels. Mol Ecol Resour. https://doi.org/10.1111/1755-0998.13136
Wambugu PW, Ndjiondjop MN, Henry RJ (2018) Role of genomics in promoting the utilization of plant genetic resources in genebanks. Brief Funct Genom 17(3):198–206. https://doi.org/10.1093/bfgp/ely014
Wang K, Li M, Hakonarson H (2010) ANNOVAR: functional annotation of genetic variants from high-throughput sequencing data. Nucleic Acids Res 38(16):e164. https://doi.org/10.1093/nar/gkq603
Weeks AR, Sgro CM, Young AG, Frankham R, Mitchell NJ, Miller KA, Byrne M, Coates DJ, Eldridge MD, Sunnucks P, Breed MF, James EA, Hoffmann AA (2011) Assessing the benefits and risks of translocations in changing environments: a genetic perspective. Evol Appl 4:709–725. https://doi.org/10.1111/j.1752-4571.2011.00192.x
Wei X, Jiang M (2021) Meta-analysis of genetic representativeness of plant populations under ex situ conservation in contrast to wild source populations. Conserv Biol 35(1):12–23. https://doi.org/10.1111/cobi.13617
Westemeier RL, Brawn JD, Simpson SA, Esker TL, Jansen RW, Walk JW, Kershner EL, Bouzat JL, Paige KN (1998) Tracking the long-term decline and recovery of an isolated population. Science 282(5394):1695–1698. https://doi.org/10.1126/science.282.5394.1695
Westfall KM, Therriault TW, Abbott CL (2020) A new approach to molecular biosurveillance of invasive species using DNA metabarcoding. Glob Change Biol 26(2):1012–1022. https://doi.org/10.1111/gcb.14886
Westgate MJ, Likens GE, Lindenmayer DB (2013) Adaptive management of biological systems: a review. Biol Conserv 158:128–139. https://doi.org/10.1016/j.biocon.2012.08.016
Wildt D, Miller P, Koepfli K, Pukazhenthi B, Palfrey K, Livingston G, Beetem D, Shurter S, Gregory J, Takacs M, Snodgrass K (2019) Breeding centers, private ranches, and genomics for creating sustainable wildlife populations. Bioscience 69:928–943. https://doi.org/10.1093/biosci/biz091
Willi Y, Kleunen MV, Dietrich S, Fischer M (2007) Genetic rescue persists beyond first-generation outbreeding in small populations of a rare plant. Proc R Soc B 274:2357–2364
Wisely SM, Ryder OA, Santymire RM, Engelhardt JF, Novak BJ (2015) A road map for 21st century genetic restoration: gene pool enrichment of the black-footed ferret. J Hered 106(5):581–592. https://doi.org/10.1093/jhered/esv041
Wittenberg R, Cock MJW (eds) (2001) Invasive alien species: a toolkit of best prevention and management practices. CAB International, Wallingford, p 228
Wood J, Ballou JD, Callicrate T, Fant JB, Griffith MP, Kramer AT, Lacy RC, Meyer A, Sullivan S, Traylor-Holzer K, Walsh SK, Havens K (2020) Applying the zoo model to conservation of threatened exceptional plant species. Conserv Biol. https://doi.org/10.1111/cobi.13503
WWF (2020) Living planet report 2020—bending the curve of biodiversity loss. Almond REA, Grooten M, Petersen T (eds). WWF, Gland Switzerland
Zhang W, Fan Z, Han E, Hou R, Zhang L, Galaverni M, Huang J, Liu H, Silva P, Li P, Pollinger JP, Du L, Zhang X, Yue B, Wayne RK, Zhang Z (2014) Hypoxia adaptations in the grey wolf ( Canis lupus chanco) from Qinghai-Tibet Plateau. PLoS Genet 10(7):e1004466. https://doi.org/10.1371/journal.pgen.1004466
Zhang H, Lang Z, Zhu JK (2018) Dynamics and function of DNA methylation in plants. Nat Rev Mol Cell Biol 19:489–506
Download references
Acknowledgements
This article is a result of a workshop organised by COST Action G-Bike (CA18134), supported by COST (European Cooperation in Science and Technology). We appreciate the feedback of three anonymous reviewers, the editor and Philippine Vergeer and Maria Balcazar.
Open Access funding enabled and organized by Projekt DEAL.
Author information
Authors and affiliations.
Wildlife Ecology and Management, University Freiburg, Tennenbacher Str. 4, 79106, Freiburg, Germany
Gernot Segelbacher
Wageningen University & Research - Animal Breeding and Genomics, Droevendaalsesteeg 1, 6708, Wageningen, The Netherlands
Mirte Bosse
Vetmeduni Vienna, Research Institute of Wildlife Ecology, Savoyenstrasse 1, 1160, Vienna, Austria
Pamela Burger
Centre for Research and Conservation, Antwerp Zoo Society, Koningin Astridplein 20, 2018, Antwerp, Belgium
Peter Galbusera & Philippe Helsen
Doñana Biological Station (EBD-CSIC), Avd. Americo Vespucio 26, 41092, Seville, Spain
José A. Godoy & Carles Vilà
Copenhagen Zoo, Roskildevej 38, 2000, Frederiksberg, Denmark
Christina Hvilsom
Department of Biodiversity, Faculty of Mathematics, Natural Sciences and Information Technologies, University of Primorska, Glagoljaška 8, 6000, Koper, Slovenia
Laura Iacolina & Elena Buzan
Center for Marine and Freshwater Biology Sharklab ADRIA, Paromlinska 16, 71000, Sarajevo, Bosnia and Herzegovina
Adla Kahric
Department of Life Sciences, University of Trieste, via L. Giorgieri, 5, 34127, Trieste, Italy
Chiara Manfrin
Faculty of Forestry, University of Belgrade, Kneza Višeslava 1, 11030, Belgrade, Serbia
Marina Nonic
Imperial College London, Exhibition Rd, South Kensington, London, SW7 2BU, UK
Delphine Thizy
Forest Research Institute, Bulgarian Academy of Sciences, 132, Kl. Ohridski Blvd., 1756, Sofia, Bulgaria
Ivaylo Tsvetkov
Department of Biology and Ecology, Faculty of Sciences, University of Novi Sad, Trg Dositeja Obradovica 2, 21000, Novi Sad, Serbia
Nevena Veličković
Department of Wildlife Ecology and Conservation, University of Florida, Gainesville, FL, 32611, USA
Samantha M. Wisely
You can also search for this author in PubMed Google Scholar
Contributions
Corresponding author.
Correspondence to Gernot Segelbacher .
Ethics declarations
Conflict of interest.
The authors declare that there is no conflict of interest.
Ethical Approval
Consent to participate, consent for publications, additional information, publisher's note.
Springer Nature remains neutral with regard to jurisdictional claims in published maps and institutional affiliations.
Supplementary Information
Below is the link to the electronic supplementary material.
Supplementary file1 (DOCX 22 kb)
Two populations/genetic lineages of a species which have been previously isolated mix which each other. Admixture introduces new genetic information into a given population.
One, two, or more versions of a specific locus (gene) on the chromosome. An individual inherits one allele from each of its parents, if both alleles are the same/different the individual is homozygous/heterozygous.
Pre-specified gene of interest, where the biological function is known and which is associated with a specific phenotype or disease status.
Coding DNA is providing instructions for making proteins, whereas non-coding DNA does not code for any amino acids.
Changes in heritable phenotypes that are not associated with DNA sequence changes. Epigenetic modifications alter the accessibility of DNA to the transcription process and thus influence gene expression.
Genetic variation which is causing phenotypic variation.
Site-specific DNA modifications, that involves cutting the DNA at specific sites and changing/ adding/removing the DNA where it was cut.
A gene drive is a system of biased inheritance in which the ability of a genetic element to pass from a parent to its offspring through sexual reproduction is enhanced. Thus, the result of a gene drive is the preferential increase of a specific genotype, the genetic makeup of an organism that determines a specific phenotype (trait), from one generation to the next, and potentially throughout the population (NASEM 2016 ).
Lowered mean population fitness due to detrimental mutations with only small selective disadvantages that occur in high frequency, or become fixed in a population by genetic drift (Hedrick and Fredrickson 2010 ).
Unrelated individuals from a genetically close population are introduced into a population with low fitness (Hedrick and Fredrickson 2010 ).
An individual has inherited two identical/different alleles for a particular gene or genes from both parents.
Lower fitness of an individual due to an increased homozygosity of detrimental (deleterious) alleles from mating between close relatives (Charlesworth and Charlesworth 1999 ).
Transfer of genes/alleles from one species into another distinct species.
Also called third-generation sequencing produces DNA reads in excess of 10 kb. Long reads improve de novo assembly, mapping certainty, transcript isoform identification, and detection of structural variants.
Genetic variation which is unaffected by natural selection.
Massive parallel amplification of DNA sequence reads, also called massive parallel sequencing or second generation sequencing; generates DNA reads in excess of 600 bp.
Lower fitness of a hybrid individual resulting from mating between individuals with mixed backgrounds, due to genomic incompatibilities, maladaptation or disrupting pleiotropic gene complexes.
Genetic purging means the reduction of the frequency of a deleterious allele. As deleterious alleles have a negative effect (many alleles are only harmful when homozygous) such offspring will be removed from the gene pool.
Genomic regions where identical haplotypes (or identical DNA sequences) are inherited from each parent.
Different variants of a single nucleotide at a given position in the genome. Measures variability within populations or species.
Cloning technique in which the nucleus of an oocyte (egg cell) is replaced with the nucleus of a somatic (body) cell.
Synthetic biology is a further development and new dimension of modern biotechnology that combines science, technology and engineering to facilitate and accelerate the understanding, design, redesign, manufacture and/or modification of genetic materials, living organisms and biological systems.
Genes which can be identified to be expressed under changing environmental conditions (e.g. salt tolerance, temperature tolerance).
Complete set of RNA transcribed from a specific genome.
Rights and permissions
Open Access This article is licensed under a Creative Commons Attribution 4.0 International License, which permits use, sharing, adaptation, distribution and reproduction in any medium or format, as long as you give appropriate credit to the original author(s) and the source, provide a link to the Creative Commons licence, and indicate if changes were made. The images or other third party material in this article are included in the article's Creative Commons licence, unless indicated otherwise in a credit line to the material. If material is not included in the article's Creative Commons licence and your intended use is not permitted by statutory regulation or exceeds the permitted use, you will need to obtain permission directly from the copyright holder. To view a copy of this licence, visit http://creativecommons.org/licenses/by/4.0/ .
Reprints and permissions
About this article
Segelbacher, G., Bosse, M., Burger, P. et al. New developments in the field of genomic technologies and their relevance to conservation management. Conserv Genet 23 , 217–242 (2022). https://doi.org/10.1007/s10592-021-01415-5
Download citation
Received : 19 December 2020
Accepted : 22 August 2021
Published : 11 November 2021
Issue Date : April 2022
DOI : https://doi.org/10.1007/s10592-021-01415-5
Share this article
Anyone you share the following link with will be able to read this content:
Sorry, a shareable link is not currently available for this article.
Provided by the Springer Nature SharedIt content-sharing initiative
- Genomic tools
- Biotechnology
- Genetic rescue
- Gene editing
- Find a journal
- Publish with us
- Track your research
BRIEF RESEARCH REPORT article
Application of whole exome sequencing in the diagnosis of muscular disorders: a study of taiwanese pediatric patients.
- 1 Pediatric, Mackay Memorial Hospital, Taipei, Taipei County, Taiwan
- 2 Mackay Memorial Hospital, Taipei, Taipei County, Taiwan
The final, formatted version of the article will be published soon.
Select one of your emails
You have multiple emails registered with Frontiers:
Notify me on publication
Please enter your email address:
If you already have an account, please login
You don't have a Frontiers account ? You can register here
Background: Muscular dystrophies and congenital myopathies encompass various inherited muscular disorders that present diagnostic challenges due to clinical complexity and genetic heterogeneity.Methods: This study aimed to investigate the use of whole exome sequencing (WES) in diagnosing muscular disorders in pediatric patients in Taiwan. Out of 161 pediatric patients suspected to have genetic/inherited myopathies, 115 received a molecular diagnosis through conventional tests, single gene testing, and gene panels. The remaining 46 patients were divided into three groups: Group 1 (multiplex ligationdependent probe amplification-negative Duchenne muscular dystrophy) with three patients (6.5%), Group 2 (various forms of muscular dystrophies) with 21 patients (45.7%), and Group 3 (congenital myopathies) with 22 patients (47.8%).Results: WES analysis of these groups found pathogenic variants in 100.0% (3/3), 57.1% (12/21), and 68.2% (15/22) of patients in Groups 1 to 3, respectively. WES had a diagnostic yield of 65.2% (30 patients out of 46), detecting 30 pathogenic or potentially pathogenic variants across 28 genes.WES enables the diagnosis of rare diseases with symptoms and characteristics similar to congenital myopathy and muscular dystrophy, such as muscle weakness. Consequently, this approach facilitates targeted therapy implementation and appropriate genetic counseling.
Keywords: Muscular disorders, muscular dystrophy, Congenital myopathy, whole exome sequencing, Mutational diversity
Received: 04 Jan 2024; Accepted: 23 Apr 2024.
Copyright: © 2024 Lee, Chuang, Chiu, Chang, Tu, Lo, Lin and Lin. This is an open-access article distributed under the terms of the Creative Commons Attribution License (CC BY) . The use, distribution or reproduction in other forums is permitted, provided the original author(s) or licensor are credited and that the original publication in this journal is cited, in accordance with accepted academic practice. No use, distribution or reproduction is permitted which does not comply with these terms.
* Correspondence: Chih-Kuang Chuang, Mackay Memorial Hospital, Taipei, Taipei County, Taiwan Huei-Ching Chiu, Mackay Memorial Hospital, Taipei, Taipei County, Taiwan Ya-Hui Chang, Mackay Memorial Hospital, Taipei, Taipei County, Taiwan Yuan- Rong Tu, Mackay Memorial Hospital, Taipei, Taipei County, Taiwan Yun-Ting Lo, Mackay Memorial Hospital, Taipei, Taipei County, Taiwan Hsiang-Yu Lin, Mackay Memorial Hospital, Taipei, Taipei County, Taiwan Shuan-Pei Lin, Mackay Memorial Hospital, Taipei, Taipei County, Taiwan
Disclaimer: All claims expressed in this article are solely those of the authors and do not necessarily represent those of their affiliated organizations, or those of the publisher, the editors and the reviewers. Any product that may be evaluated in this article or claim that may be made by its manufacturer is not guaranteed or endorsed by the publisher.
- Introduction to Genomics
- Educational Resources
- Policy Issues in Genomics
- The Human Genome Project
- Funding Opportunities
- Funded Programs & Projects
- Division and Program Directors
- Scientific Program Analysts
- Contact by Research Area
- News & Events
- Research Areas
- Research investigators
- Research Projects
- Clinical Research
- Data Tools & Resources
- Genomics & Medicine
- Family Health History
- For Patients & Families
- For Health Professionals
- Jobs at NHGRI
- Training at NHGRI
- Funding for Research Training
- Professional Development Programs
- NHGRI Culture
- Social Media
- Broadcast Media
- Image Gallery
- Press Resources
- Organization
- NHGRI Director
- Mission & Vision
- Policies & Guidance
- Institute Advisors
- Strategic Vision
- Leadership Initiatives
- Diversity, Equity, and Inclusion
- Partner with NHGRI
- Staff Search
Current Clinical Studies
Researchers at the National Human Genome Research Institute (NHGRI) are working with patients and families to better understand of how genes can cause or influence diseases and develop new and more effective diagnostics and treatments.
Value of Clinical Studies
Clinical studies give us a better understanding of how genes can cause or influence diseases. NHGRI researchers are working with patients, and with families with a history of inherited diseases, to learn more about the genetic components of common and rare disorders, and to develop new and more effective tests and treatments.
Deciding whether to participate in a clinical study is an important and personal process. Some reasons people choose to participate include:
- Participants in clinical studies help current and future generations. Through these studies, researchers develop new diagnostic tests, more effective treatments, and better ways of managing diseases with genetic components.
- Participants in studies are actively involved in understanding their disorder and current research.
- Participants in some studies gain access to new tests and treatments before they are widely available.
Featured Clinical Studies
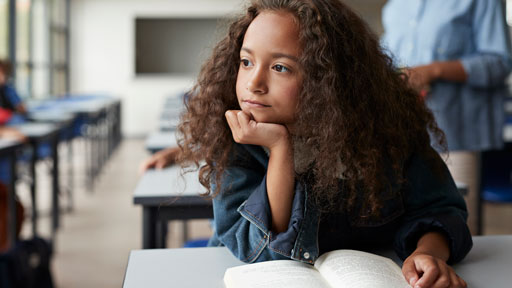
Metabolism, Infection and Immunity (MINI) Section
The MINI section aims to define the relationship between infection, immunity and clinical decline in individuals with mitochondrial disease.
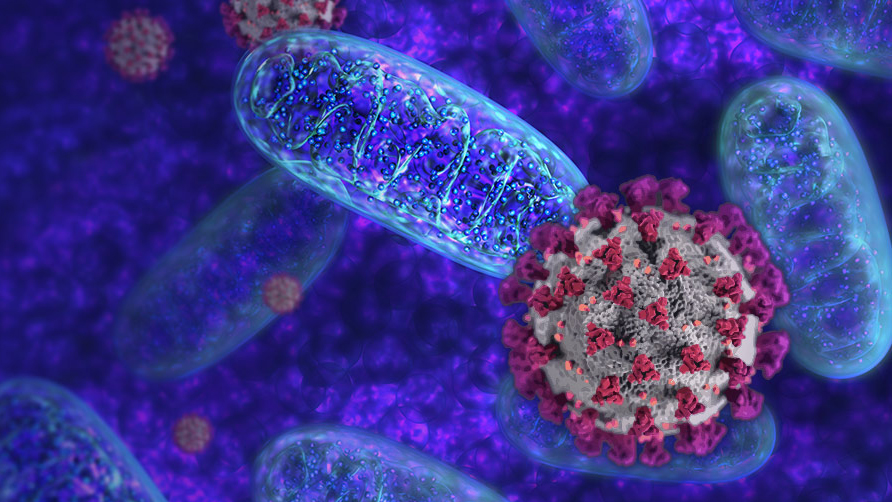
Other Clinical Studies
The following are conducted by NHGRI researchers. For eligibility requirements and contact information, visit the study on clinicaltrials.gov.
Last updated: January 12, 2023
Recent advances in the application of gene therapy to human disease
Affiliation.
- 1 Department of Hematology, University of Texas M.D. Anderson Cancer Center, Houston, USA.
- PMID: 7485213
- DOI: 10.1016/s0002-9343(99)80232-0
Purpose: To review the recent advances in the application of genetic modification strategies to the therapy of human diseases for which a molecular defect is known.
Methods: A computerized data bank search, the minutes of the National Institutes of Health (NIH) Recombinant DNA Advisory Committee published in the Federal Record, and reports of human clinical trials were used as data sources for this review. Clinical trials included in this review were published in the literature or approved by the NIH Recombinant DNA Advisory Committee.
Study selection: Evaluations of the efficacy of genetic modification strategies in clinical trials in human and in animal models are summarized. The design and outcome of the genetic modification strategies employed are reviewed for 16 marking trials, 16 gene replacement trials for molecular deficiency diseases, 3 chemoprotection and 4 chemotherapy sensitization trials, 11 cancer vaccine trials, 2 antisense oligonucleotide trials, and 3 molecular immunotherapy trials.
Data synthesis: The marking trials have shown that residual leukemia cells in the infused autologous marrow can contribute to relapse following autologous bone marrow transplants. The use of genetic modification for the replacement of missing or deficient genes in severe combined immunodeficiency, familial hypercholesterolemia, and cystic fibrosis has been associated with encouraging results so far. Clinical genetic therapy trials involving cancer vaccines, antisense oligonucleotides, adoptive immunotherapy with genetically modified T cells, delivery vectors containing interleukin-1 receptor inhibitor for arthritis, replacement strategies for storage diseases, and genetic suppression of human immunodeficiency viral replication are just commencing.
Conclusions: The clinical application of genetic modification techniques has thus far been successful in the beginning phases of this field. These early results suggest that continuation of gene therapy trials designed to correct the molecular changes that lead to disease states in humans is warranted. Evaluation of such clinical trials in the future may be based on the analysis of assays for short-term surrogate endpoints, as well as on the therapeutic outcomes of the trial, such as survival or remission.
Publication types
- Research Support, Non-U.S. Gov't
- Research Support, U.S. Gov't, P.H.S.
- Bone Marrow Transplantation
- Clinical Trials as Topic
- Genetic Therapy* / methods
- HIV Infections / therapy
- Immunotherapy
- Neoplasms / therapy
- Treatment Outcome
Grants and funding
- P01 CA49639/CA/NCI NIH HHS/United States
- P01 CA55164/CA/NCI NIH HHS/United States
Thank you for visiting nature.com. You are using a browser version with limited support for CSS. To obtain the best experience, we recommend you use a more up to date browser (or turn off compatibility mode in Internet Explorer). In the meantime, to ensure continued support, we are displaying the site without styles and JavaScript.
- View all journals
- Explore content
- About the journal
- Publish with us
- Sign up for alerts
- Published: 13 September 2023
The reuse of genetic information in research and informed consent
- David Lorenzo 1 , 2 ,
- Montse Esquerda ORCID: orcid.org/0000-0002-5781-8298 1 , 3 ,
- Margarita Bofarull ORCID: orcid.org/0000-0001-8215-4115 1 ,
- Victoria Cusi 1 ,
- Helena Roig 1 ,
- Joan Bertran ORCID: orcid.org/0000-0001-8553-0534 1 ,
- Joan Carrera 1 ,
- Francesc Torralba 1 ,
- Francisco José Cambra 1 , 4 ,
- Martí Vila 1 ,
- Martina Garriga 1 &
- Francesc Palau ORCID: orcid.org/0000-0002-8635-5421 5 , 6 , 7 , 8
European Journal of Human Genetics volume 31 , pages 1393–1397 ( 2023 ) Cite this article
374 Accesses
1 Citations
5 Altmetric
Metrics details
- Medical ethics
Important advances in genetics research have been made in recent years. Such advances have facilitated the availability of huge amounts of genetic information that could potentially be reused beyond the original purpose for which such information was obtained. Any such reuse must meet certain ethical criteria to ensure that the dignity, integrity, and autonomy of the individual from whom that information was obtained are protected. The aim of this paper is to reflect on these criteria through a critical analysis of the literature. To guarantee these values, ethical criteria need to be established in several respects. For instance, the question must be posed whether the information requires special attention and protection (so-called genetic exceptionalism ). Another aspect to bear in mind is the most appropriate type of consent to be given by the person involved, on the one hand favouring research and the reuse of genetic information while on the other protecting the autonomy of that person. Finally, there is a need to determine what protection such reuse should have in order to avoid detrimental consequences and protect the rights of the individual. The main conclusions are that genetic information requires special care and protection (genetic exceptionalism) and that broad consent is the most practical and trustworthy type of consent for the reuse of genetic information.
This is a preview of subscription content, access via your institution
Access options
Subscribe to this journal
Receive 12 print issues and online access
251,40 € per year
only 20,95 € per issue
Buy this article
- Purchase on Springer Link
- Instant access to full article PDF
Prices may be subject to local taxes which are calculated during checkout
Similar content being viewed by others
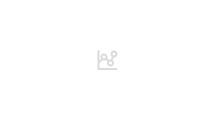
Rethinking the ethical principles of genomic medicine services
Willingness to donate genomic and other medical data: results from germany.
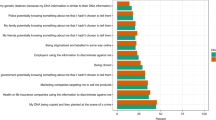
Members of the public in the USA, UK, Canada and Australia expressing genetic exceptionalism say they are more willing to donate genomic data
Pereira S, Robinson JO, McGuire AL. Return of individual genomic research results: what do consent forms tell participants? Eur J Hum Genet. 2016;24:1524–9.
PubMed PubMed Central Google Scholar
McGuire AL, Beskow LM. Informed consent in genomics and genetic research. Annu Rev Genomics Hum Genet. 2010;11:361–81.
CAS PubMed PubMed Central Google Scholar
Martani A, Genevieve LD, Pauli-Magnus, McLennan S, Elger BS. Regulating the secondary use of data for research: arguments against genetic exceptionalism. Front Genet. 2019;10:1254.
Evans JP, Burke W. Genetic exceptionalism. Too much of a good thing? Genet Med. 2008;10:500–1.
PubMed Google Scholar
McGuire AL, Fisher R, Cusenza P, Hudson K, Rothstein MA, McGraw D, et al. Confidentiality, privacy, and security of genetic and genomic test information in electronic health records: points to consider. Genet Med. 2008;10:495–9.
Slokenberga S. You can’t put the genie back in the bottle: on the legal and conceptual understanding of genetic privacy in the era of personal data protection in Europe. BioLaw J. 2021;21:223–50.
Google Scholar
Mascalzoni D, Hicks A, Pramstaller P, Wjst M. Informed consent in the genomics era. PLoS Med. 2008;5:e192.
Lin Z, Altman RB, Owen AB. Confidentiality in genome research. Science. 2006;313:441–2.
CAS PubMed Google Scholar
Byrd JB, Greene AC, Prasar DV, Jiang X, Greene C. Responsible, practical genomic data sharing that accelerates research. Nat Rev Genet. 2020;21:615–29.
Stauton C, Slokenberga S, Parziale A, Mascalzoni D. Appropriate safeguards and article 89 of the GDPR: considerations for biobank, databank and genetic research. Front Genet. 2022;13:719317.
Lemke AA, Wolf WA, Herbert-Beirne J, Smith ME. Public and biobank participant attitudes toward genetic research participation and data sharing. Public Health Genom. 2010;13:368–77.
CAS Google Scholar
Haga SB, O’Daniel J. Public perspectives regarding data-sharing practices in genomics research. Public Health Genom. 2011;14:319–24.
Shabani M, Borry P. Challenges of web-based personal genomic data Sharing. Life Sci Soc Policy. 2015;11:3.
Khan A, Capps B, Sum MY, Kuswanto C, Sim K. Informed consent for human genetic and genomic studies: a systematic review. Clin Genet. 2014;86:199–206.
Hofmann B. Broadening consent–and diluting ethics?”. J Med Ethics. 2009;35:125–9.
Clayton EW, Halverson CMN, Sathe NA, Malin BA. A systematic literature review of individuals’ perspectives on privacy and genetic information in the United States. PLoS ONE. 2018;13:e0204417.
Gille F, Brall C. Can we know if donor trust expires? About trust relationships and time in the context of open consent for future data use. J Med Ethics. 2022;48:184–8.
Knoppers BM, Joly Y, Simard J, Durocher F. The emergence of an ethical duty to disclose genetic research results: international perspectives. Eur J Hum Genet. 2006;14:1170–8.
Martin-Sanchez FJ, Aguiar-Pulido V, Lopez-Campos GH, Peek KN, Sacchi L. Secondary use and analysis of big data collected for patient care. Yearb Med Inf. 2017;26:28–37.
Wendler D, Emanuel E. The debate over research on stored biological samples: what do sources think? Arch Int Med. 2002;162:1457–62.
Petrini C. Broad consent, exceptions to consent and the question of using biological samples for research purposes different from the initial collection purpose”. Soc Sci Med. 2010;70:217–20.
Johnsson L, Eriksson S. Autonomy is a right, not a feat: how theoretical misconceptions have muddled the debate on dynamic consent to biobank research. Bioethics 2016;30:471–8.
Budin-Ljosnel I, Teare H, Kaye J, Beck S, Bentzen HB, Caenazzo L, et al. Dynamic consent: a potential solution to some of the challenges of modern biomedical research. BMC Med Ethics. 2017;18:4.
Hallinan D. Broad consent under the GDPR: an optimistic perspective on a bright future”. Life Sci Soc Policy. 2020;16:1.
Then SN, Lipworth W, Stewart C, Kerridge I. A framework for ethics review of applications to store, reuse and share tissue samples. Monash Bioeth Rev. 2021;39:115–24.
Teare HJ, Prictor M, Kaye J. Reflections on dynamic consent in biomedical research: the story so far. Eur J Hum Genet. 2021;29:649–56.
Gille F, Vayena E, Blasimme A. Future-proofing biobanks’ governance. Eur J Hum Genet. 2020;28:989–96.
Caulfield T. Biobanks and blanket consent: the proper place of the public good and public perception rationales. King’s Law J 2007;18:209–26.
Clayton EW. Informed consent and biobanks. J Law Med Ethics. 2005;3:15–21.
Grady C, Eckstein L, Berkman B, Brock D, Cook-Deegan R, Fullerton SM, et al. Broad consent for research with biological samples: workshop conclusions. Am J Bioeth. 2015;15:34–42.
McKeown A, Mourby M, Harrison P, Walker S, Sheehan M, Singh I. Ethical issues in consent for the reuse of data in health data platforms. Sci Eng Ethics 2021;27:9.
Hansson MG, Dillner J, Bartram CR, Carlson JA, Helgesson G. Should donors be allowed to give broad consent to future biobank research? Lancet Oncol. 2006;7:266–9.
Regidor E. The use of personal data from medical records and biological materials: ethical perspectives and the basis for legal restrictions in health research. Soc Sci Med. 2004;59:1975–84.
Mascalzoni D, Melotti R, Pattaro C, Pramstaller PP, Gögele M, De Grandi A, et al. Ten years of dynamic consent in the CHRIS study: informed consent as a dynamic process. Eur J Hum Genet. 2022;30:1391–7.
Kaye J, Whitley EA, Lund D, Morrison M, Teare H, Melham K. Dynamic consent : a patient interface for twenty-first century research networks. Eur J Hum Genet. 2014;23:141–6. https://doi.org/10.1038/ejhg.2014.71 .
Article PubMed PubMed Central Google Scholar
Austin MA, Hardings S, McElroy C. Genebanks: a comparison of eight proposed international genetic databases. Community Genet. 2003;6:37–45.
Salter B, Jones M. Biobanks and bioethics: the politics of legitimation. J Eur Public Policy. 2005;12:710–32.
Hewitt R. Biobanking: The foundation of personalized medicine. Curr Opin Oncol. 2011;23:112–9.
D’Abramo F. Biobank research, informed consent and society. Towards a new alliance? J Epidemiol Community Health. 2015;69:1125–8.
Shalowitz DI, Miller FG. The search for clarity in communicating research results to study participants. J Med Ethics. 2008;34:e17.
Fernandez CV, Kodish E, Weijer C. Informing study participants of research results: an ethical imperative. Ethics Hum Res. 2003;25:12–19.
Parker M, Lucasse AM. Genetic information: a joint account? BMJ. 2004;329:165–7.
Meggiolaro N, Barlow-Stewart K, Dunlop K, Newson AJ, Fleming J. Disclosure to genetic relatives without consent–Australian genetic professionals’ awareness of the health privacy law. BMC Med Ethics. 2020;21:1–10.
Geissbuhler A, Safran C, Buchan I, Bellazzi R, Labkoff S, Eilenberg K, et al. Trustworthy reuse of health data: a transnational perspective. Int J Med Inform 2013;82:1–9 A.
Langhof H, Schwietering J, Strech D. Practice evaluation of biobank ethics and governance: current needs and future perspectives. J Med Genet. 2019;56:176–85.
Helgersson G, Dillner J, Carlson J, Bartram CR, Hansson MG. Ethical framework for previously collected biobank samples. Nat Biotechnol. 2007;25:973–6.
Wiertz S, Boldt J. Evaluating models of consent in changing health research environments. Med Health Care Philos. 2022;25:269–80.
Laurie G. Evidence of support for biobanking practices. BMJ. 2008;337:a337.
Download references
No funding was needed.
Author information
Authors and affiliations.
Institut Borja de Bioetica, Universitat Ramon Llull, Barcelona, Spain
David Lorenzo, Montse Esquerda, Margarita Bofarull, Victoria Cusi, Helena Roig, Joan Bertran, Joan Carrera, Francesc Torralba, Francisco José Cambra, Martí Vila & Martina Garriga
EUI San Joan de Deu, Barcelona, Spain
David Lorenzo
Sant Joan de Deu Terres de Lleida, Lleida, Spain
Montse Esquerda
Hospital Universitari Sant Joan de Déu Barcelona, Barcelona, Spain
Francisco José Cambra
Department of Genetic Medicine and Pediatric Institut of Rare Diseases, Hospital Sant Joan de Déu, Barcelona, Spain
Francesc Palau
Institut de Recerca Sant Joan de Déu, Barcelona, Spain
CIBER de Enfermedades Raras, ISCIII, Madrid, Spain
Division of Pediatrics, University of Barcelona School of Medicine and Health Sciences, Barcelona, Spain
You can also search for this author in PubMed Google Scholar
Contributions
DL, ME, VC and FP developed the research project and wrote the manuscript. MB, HR, JC, JB, FC, MV, MG participated in the discussion, drafted the paper and all authors provided critical revisions.
Corresponding author
Correspondence to Montse Esquerda .
Ethics declarations
Competing interests.
The authors declare no competing interests.
Additional information
Publisher’s note Springer Nature remains neutral with regard to jurisdictional claims in published maps and institutional affiliations.
Rights and permissions
Springer Nature or its licensor (e.g. a society or other partner) holds exclusive rights to this article under a publishing agreement with the author(s) or other rightsholder(s); author self-archiving of the accepted manuscript version of this article is solely governed by the terms of such publishing agreement and applicable law.
Reprints and permissions
About this article
Cite this article.
Lorenzo, D., Esquerda, M., Bofarull, M. et al. The reuse of genetic information in research and informed consent. Eur J Hum Genet 31 , 1393–1397 (2023). https://doi.org/10.1038/s41431-023-01457-y
Download citation
Received : 05 December 2022
Revised : 28 May 2023
Accepted : 31 August 2023
Published : 13 September 2023
Issue Date : December 2023
DOI : https://doi.org/10.1038/s41431-023-01457-y
Share this article
Anyone you share the following link with will be able to read this content:
Sorry, a shareable link is not currently available for this article.
Provided by the Springer Nature SharedIt content-sharing initiative
This article is cited by
Ambivalence and regret in genome sequencing.
- Alisdair McNeill
European Journal of Human Genetics (2023)
Quick links
- Explore articles by subject
- Guide to authors
- Editorial policies

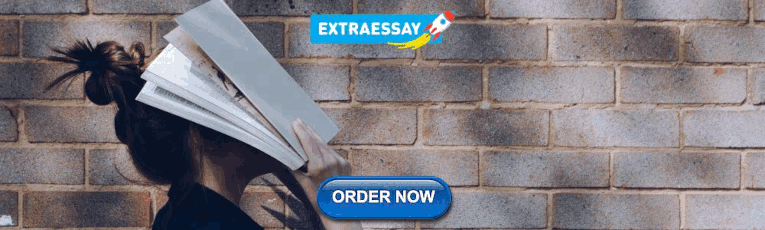
IMAGES
VIDEO
COMMENTS
The widening scope and scale of genetic tests are posing new challenges, and the need to address these challenges is becoming immediately relevant for all clinicians, not just genetics experts.
Genetics articles from across Nature Portfolio. Genetics is the branch of science concerned with genes, heredity, and variation in living organisms. It seeks to understand the process of trait ...
In 1987, the New York Times Magazine characterized the Human Genome Project as the "biggest, costliest, most provocative biomedical research project in history." 2 But in the years between the ...
The research, titled "High-throughput genetic manipulation of multicellular organisms using a machine-vision guided embryonic microinjection robot," is featured on the cover of the April 2024 ...
Genetics research is the scientific discipline concerned with the study of the role of genes in traits such as the development of disease. It has a key role in identifying potential targets for ...
Read the latest Research articles in Genetics from Scientific Reports. Skip to main content. ... Genetics articles within Scientific Reports. Featured. Article 26 April 2024 | Open Access.
Recent genetic discoveries offer a new lens through which to study cognitive, emotional, behavioral, and social processes that are foundational to children's development. In this article, we review the latest advances in genomics—genome‐wide association studies and the polygenic scores that have come out of them—and discuss how these techniques can be leveraged to shed light on ...
A recent study in a neonatal intensive care unit in Texas studied outcomes for 278 infants who were referred for clinical exome sequencing, and found that 36.7% received a genetic diagnosis, and medical management was affected for 52% of infants with diagnoses [ ].
1. Introduction. The past two decades have witnessed major advances in technologies and clinical applications of genetics and genomics. Twenty years ago, the human genome project [1-2] provided the first glimpses into the human genome sequence and launched a new era of human genetics: biologists and geneticists for the first time had got almost the entire human genome at their fingertips ...
In this paper, the analysis of recent advances in genetic algorithms is discussed. The genetic algorithms of great interest in research community are selected for analysis. ... Applications: Research involves on multimedia, operation management and wireless networks: Research involves on engineering design, data mining, software applications ...
FROM THE LABS: Research method finds new use in diagnosis of genetic disorders April 25, 2024 genetic diagnosis, Rare Diseases, Research, ... McNair Medical Institute at the Robert and Janice McNair Foundation and Baylor Genetics. The research was supported by the National Institutes of Health Common Fund (U01HG007709 and U01HG007942), the ...
Psychiatric genetics has made substantial progress in the last decade, providing new insights into the genetic etiology of psychiatric disorders, and paving the way for precision psychiatry, in which individual genetic profiles may be used to personalize risk assessment and inform clinical decision- …
In this paper, the analysis of recent advances in genetic algorithms is discussed. The genetic algorithms of great interest in research community are selected for analysis. This review will help the new and demanding researchers to provide the wider vision of genetic algorithms. The well-known algorithms and their implementation are presented with their pros and cons. The genetic operators and ...
In the 'Clinical Application of MAVE Data' workshop, held on 12th July 2023 at the Wellcome Connecting Science Conference Centre in between two relevant research meetings, 'Curating the ...
05 Feb 2024. 23 Jan 2024. Genetics Research is a fully open access journal providing a key forum for original research on all aspects of human and animal genetics, reporting key findings on genomes, genes, mutations, developmental, evolutionary, and population genetics as well as ethical, legal and social aspects.
Recent technological advances in the field of genomics offer conservation managers and practitioners new tools to explore for conservation applications. Many of these tools are well developed and used by other life science fields, while others are still in development. Considering these technological possibilities, choosing the right tool(s) from the toolbox is crucial and can pose a ...
BRIEF RESEARCH REPORT article. Front. Genet. Sec. Genetics of Common and Rare Diseases Volume 15 - 2024 | doi: 10.3389/fgene.2024.1365729 Application of Whole Exome Sequencing in the Diagnosis of Muscular Disorders: A Study of Taiwanese Pediatric Patients ... Out of 161 pediatric patients suspected to have genetic/inherited myopathies, 115 ...
Over the past few decades, basic and applied research on wheat has lagged behind other cereal crops due to the complex and polyploid genome and difficulties in genetic transformation. A breakthrough called as PureWheat was made in the genetic transformation of wheat in 2014 in Asia, leading to a noticeable progress of wheat genome editing.
Participants in clinical studies help current and future generations. Through these studies, researchers develop new diagnostic tests, more effective treatments, and better ways of managing diseases with genetic components. Participants in studies are actively involved in understanding their disorder and current research.
Genetic engineering articles from across Nature Portfolio. Genetic engineering is the act of modifying the genetic makeup of an organism. Modifications can be generated by methods such as gene ...
Genetic engineering r elated to the living environment and sustainable development is. brief ly touched upon in several sections of chapters 2.6.50, 2.7 and 2.8.74 of the 1/2018. report. Recent ...
TARRYTOWN, N.Y., April 22, 2024 (GLOBE NEWSWIRE) -- Regeneron Pharmaceuticals, Inc. (NASDAQ: REGN) today announced that new and updated data across its genetic medicines portfolio will be presented at the American Society of Gene and Cell Therapy (ASGCT) annual conference in Baltimore, Maryland, from May 7 to 11, 2024. Data from 10 abstracts ...
Purpose: To review the recent advances in the application of genetic modification strategies to the therapy of human diseases for which a molecular defect is known. Methods: A computerized data bank search, the minutes of the National Institutes of Health (NIH) Recombinant DNA Advisory Committee published in the Federal Record, and reports of human clinical trials were used as data sources for ...
Recent developments in base editing, prime editing and other CRISPR-associated systems have laid a solid technological foundation to enable plant basic research and precise molecular breeding.
Genetic engineering is a group of techniques used for. direct genetic modification of organisms or population of. organisms using recombination of DNA. Now it is. possible to alter directly a ...
In celebration of the 20th anniversary of Nature Reviews Genetics, we asked 12 leading researchers to reflect on the key challenges and opportunities faced by the field of genetics and genomics.
A-report-on-the-recent-application-of-genetic-research - Free download as PDF File (.pdf), Text File (.txt) or read online for free.
Important advances in genetics research have been made in recent years. Such advances have facilitated the availability of huge amounts of genetic information that could potentially be reused ...