Thank you for visiting nature.com. You are using a browser version with limited support for CSS. To obtain the best experience, we recommend you use a more up to date browser (or turn off compatibility mode in Internet Explorer). In the meantime, to ensure continued support, we are displaying the site without styles and JavaScript.
- View all journals
- Explore content
- About the journal
- Publish with us
- Sign up for alerts
- Review Article
- Published: 12 September 2023
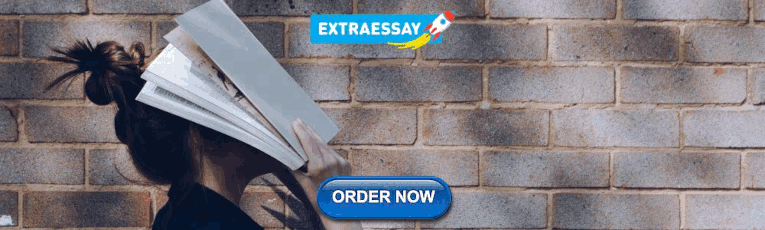
Global river water quality under climate change and hydroclimatic extremes
- Michelle T. H. van Vliet ORCID: orcid.org/0000-0002-2597-8422 1 ,
- Josefin Thorslund ORCID: orcid.org/0000-0001-6111-4819 1 , 2 ,
- Maryna Strokal ORCID: orcid.org/0000-0002-8063-7743 3 ,
- Nynke Hofstra ORCID: orcid.org/0000-0002-0409-5145 3 ,
- Martina Flörke ORCID: orcid.org/0000-0003-2943-5289 4 ,
- Heloisa Ehalt Macedo ORCID: orcid.org/0000-0002-3430-1480 5 ,
- Albert Nkwasa ORCID: orcid.org/0000-0002-8685-8854 6 , 7 ,
- Ting Tang 8 ,
- Sujay S. Kaushal 9 ,
- Rohini Kumar ORCID: orcid.org/0000-0002-4396-2037 10 ,
- Ann van Griensven 6 ,
- Lex Bouwman 11 , 12 &
- Luke M. Mosley 13
Nature Reviews Earth & Environment volume 4 , pages 687–702 ( 2023 ) Cite this article
7096 Accesses
11 Citations
434 Altmetric
Metrics details
- Environmental chemistry
Climate change and extreme weather events (such as droughts, heatwaves, rainstorms and floods) pose serious challenges for water management, in terms of both water resources availability and water quality. However, the responses and mechanisms of river water quality under more frequent and intense hydroclimatic extremes are not well understood. In this Review, we assess the impacts of hydroclimatic extremes and multidecadal climate change on a wide range of water quality constituents to identify the key responses and driving mechanisms. Comparison of 965 case studies indicates that river water quality generally deteriorates under droughts and heatwaves (68% of compiled cases), rainstorms and floods (51%) and under long-term climate change (56%). Also improvements or mixed responses are reported owing to counteracting mechanisms, for example, increased pollutant mobilization versus dilution during flood events. River water quality responses under multidecadal climate change are driven by hydrological alterations, rises in water and soil temperatures and interactions among hydroclimatic, land use and human drivers. These complex interactions synergistically influence the sources, transport and transformation of all water quality constituents. Future research must target tools, techniques and models that support the design of robust water quality management strategies, in a world that is facing more frequent and severe hydroclimatic extremes.
River water quality is generally deteriorating under droughts and heatwaves (68% of case studies), rainstorms and floods (51%) and multidecadal historical and future climate change (56%), although improvements and mixed responses are also reported.
Droughts and heatwaves result in lower dissolved oxygen and increased river temperature, algae, salinity and concentrations of pollutants (such as pharmaceuticals) from point sources owing to lower dilution. By contrast, low flow during these events leads to reduced pollutant transport from agricultural and urban surface runoff, contributing to lower concentrations.
Rainstorms and floods generally increase the mobilization of plastics, suspended solids, absorbed metals, nutrients and other pollutants from agricultural and urban runoff, although high flow can dilute concentrations for salinity and other dissolved pollutants. The sequence of extreme events (such as droughts followed by floods) also impacts the magnitude and drivers of river water quality responses.
Multidecadal climate change is causing water temperatures and algae to generally increase, partly causing a general decrease in dissolved oxygen concentrations. Nutrient and pharmaceutical concentrations are mostly increasing under climate change, whereas biochemical oxygen demand, salinity, suspended sediment, metals and microorganisms show a mixture of increasing and decreasing trends.
The main driving mechanisms for multidecadal water quality changes in response to climate change include hydrological alterations, rises in water and soil temperatures and interactions of hydroclimatic drivers with land use. These impacts are compounded with other human-induced drivers.
Our findings stress the need to improve understanding of the complex hydroclimatic–geographic–human driver feedbacks; water quality constituent fate, transport, interactions and thresholds; and to develop technologies and water quality frameworks that support the design of robust water quality management strategies under increasing hydroclimatic extremes.
This is a preview of subscription content, access via your institution
Access options
Access Nature and 54 other Nature Portfolio journals
Get Nature+, our best-value online-access subscription
24,99 € / 30 days
cancel any time
Subscribe to this journal
Receive 12 digital issues and online access to articles
92,52 € per year
only 7,71 € per issue
Buy this article
- Purchase on Springer Link
- Instant access to full article PDF
Prices may be subject to local taxes which are calculated during checkout
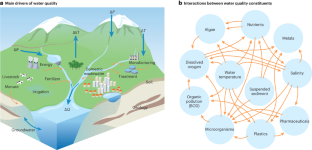
Similar content being viewed by others
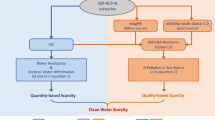
A triple increase in global river basins with water scarcity due to future pollution
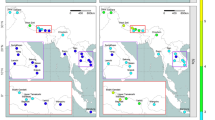
Future hydrology and hydrological extremes under climate change in Asian river basins
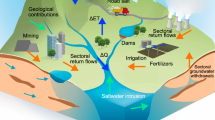
Common irrigation drivers of freshwater salinisation in river basins worldwide
Data availability.
Details on the literature review and reports for each water quality constituent (group) are given in Supplementary Notes 1–12 . The Supplementary Data file includes a spreadsheet with collected meta-data of all literature case studies in the compilation. River water quality monitoring data for Fig. 3 were retrieved from the USGS Water-Quality Data for the Nation database ( https://waterdata.usgs.gov/nwis/qw ) and Rijkswaterstaat Dutch Ministry of Infrastructure and Water database ( https://waterinfo.rws.nl/#!/nav/expert/ ).
Johnson, D. L. et al. Meanings of environmental terms. J. Environ. Qual. 26 , 581–589 (1997).
Article Google Scholar
Boyd, C. E. Water quality: an introduction. Water Quality: An Introduction 1–357 (Springer, 2015); https://doi.org/10.1007/978-3-319-17446-4 .
van Vliet, M. T. H., Flörke, M. & Wada, Y. Quality matters for water scarcity. Nat. Geosci. 10 , 800–802 (2017).
Ma, T. et al. Pollution exacerbates China’s water scarcity and its regional inequality. Nat. Commun. 11 , 650 (2020).
van Vliet, M. T. H. et al. Global water scarcity including surface water quality and expansions of clean water technologies. Environ. Res. Lett. 16 , 24020 (2021).
Whitehead, P. G., Wilby, R. L., Battarbee, R. W., Kernan, M. & Wade, A. J. A review of the potential impacts of climate change on surface water quality. Hydrol. Sci. J.-J. Des. Sci. Hydrol. 54 , 101–123 (2009).
Hrdinka, T., Novický, O., Hanslík, E. & Rieder, M. Possible impacts of floods and droughts on water quality. J. Hydro-Environ. Res. 6 , 145–150 (2012).
Beusen, A. H. W., Bouwman, A. F., Van Beek, L. P. H., Mogollón, J. M. & Middelburg, J. J. Global riverine N and P transport to ocean increased during the twentieth century despite increased retention along the aquatic continuum. Biogeosciences 13 , 2441–2451 (2016).
Thorslund, J., Bierkens, M. F. P., Oude Essink, G. H. P., Sutanudjaja, E. H. & van Vliet, M. T. H. Common irrigation drivers of freshwater salinisation in river basins worldwide. Nat. Commun. 12 , 4232 (2021).
Strokal, M. et al. Global multi-pollutant modelling of water quality: scientific challenges and future directions. Curr. Opin. Environ. Sustain. 36 , 116–125 (2019).
Jones, E. R. et al. Current wastewater treatment targets are insufficient to protect surface water quality. Commun. Earth Environ. 3 , 221 (2022).
UNEP. A Snapshot of the World’s Water Quality — Towards a Global Assessment (United Nations Environment Programme, 2016).
Kaushal, S. S. et al. Five state factors control progressive stages of freshwater salinization syndrome. Limnol. Oceanogr. Lett . https://doi.org/10.1002/LOL2.10248 (2022).
Bouwman, A. F. et al. Lessons from temporal and spatial patterns in global use of N and P fertilizer on cropland. Sci. Rep. 7 , 40366 (2017).
Jones, E. R. et al. DynQual v1.0: a high-resolution global surface water quality model. Geosci. Model Dev. 16 , 4481–4500 (2023).
Gervasio, M. P., Soana, E., Granata, T., Colombo, D. & Castaldelli, G. An unexpected negative feedback between climate change and eutrophication: higher temperatures increase denitrification and buffer nitrogen loads in the Po River (Northern Italy). Environ. Res. Lett. 17 , 84031 (2022).
Paerl, H. W. & Huisman, J. Climate: blooms like it hot. Science 320 , 57–58 (2008).
Conley, D. J. et al. Controlling eutrophication: phosphorus and nitrogen. Science 323 , 1014–1015 (2009).
Cox, B. A. & Whitehead, P. G. Impacts of climate change scenarios on dissolved oxygen in the river Thames, UK. Hydrol. Res. 40 , 138–152 (2009).
Ficklin, D. L., Stewart, I. T. & Maurer, E. P. Effects of climate change on stream temperature, dissolved oxygen, and sediment concentration in the Sierra Nevada in California. Water Resour. Res. 49 , 2765–2782 (2013).
Diamantini, E. et al. Driver detection of water quality trends in three large European river basins. Sci. Total. Environ. 612 , 49–62 (2018).
Chapra, S. C., Camacho, L. A. & McBride, G. B. Impact of global warming on dissolved oxygen and bod assimilative capacity of the world’s rivers: modeling analysis. Water ( Switzerland ) 13 , 2408 (2021).
Sjerps, R. M. A., ter Laak, T. L. & Zwolsman, G. J. J. G. Projected impact of climate change and chemical emissions on the water quality of the European rivers Rhine and Meuse: a drinking water perspective. Sci. Total Environ. 601–602 , 1682–1694 (2017).
Wolff, E. & van Vliet, M. T. H. Impact of the 2018 drought on pharmaceutical concentrations and general water quality of the Rhine and Meuse rivers. Sci. Total Environ. 778 , 146182 (2021).
Hofstra, N. Quantifying the impact of climate change on enteric waterborne pathogen concentrations in surface water. Environ. Sustain. 3 , 471–479 (2011).
Google Scholar
Hunter, P. R. Climate change and waterborne and vector-borne disease. J. Appl. Microbiol. 94 , 37S–46S (2003).
DeVilbiss, S. E., Steele, M. K., Krometis, L. A. H. & Badgley, B. D. Freshwater salinization increases survival of Escherichia coli and risk of bacterial impairment. Water Res. 191 , 116812 (2021).
Leyden, E., Farkas, J., Hutson, J. & Mosley, L. M. Short-term seawater inundation induces metal mobilisation in freshwater and acid sulfate soil environments. Chemosphere 299 , 134383 (2022).
Zhang, Y. et al. How climate change and eutrophication interact with microplastic pollution and sediment resuspension in shallow lakes: a review. Sci. Total Environ. 705 , 135979 (2020).
Naqash, N., Prakash, S., Kapoor, D. & Singh, R. Interaction of freshwater microplastics with biota and heavy metals: a review. Environ. Chem. Lett. 18 , 1813–1824 (2020).
Mei, W. et al. Interactions between microplastics and organic compounds in aquatic environments: a mini review. Sci. Total Environ. 736 , 139472 (2020).
Wang, Y. et al. Interaction of microplastics with antibiotics in aquatic environment: distribution, adsorption, and toxicity. Environ. Sci. Technol. 55 , 15579–15595 (2021).
Caretta, M. A. et al. Water . in Climate Change 2022: Impacts, Adaptation, and Vulnerability. Contribution of Working Group II to the Sixth Assessment Report of the Intergovernmental Panel on Climate Change . https://doi.org/10.1017/9781009325844.006.552 (2022).
Prudhomme, C. et al. Hydrological droughts in the 21st century, hotspots and uncertainties from a global multimodel ensemble experiment. Proc. Natl Acad. Sci. USA 111 , 3262–3267 (2014).
Trenberth, K. E. et al. Global warming and changes in drought. Nat. Clim. Change 4 , 17–22 (2014).
Christidis, N., Jones, G. S. & Stott, P. A. Dramatically increasing chance of extremely hot summers since the 2003 European heatwave. Nat. Clim. Change 5 , 46–50 (2015).
Perkins-Kirkpatrick, S. E. & Lewis, S. C. Increasing trends in regional heatwaves. Nat. Commun. 11 , 3357 (2020).
Ye, L., Shi, K., Xin, Z., Wang, C. & Zhang, C. Compound droughts and heat waves in china. Sustainability 11 , 3270 (2019).
Dankers, R. & Feyen, L. Flood hazard in Europe in an ensemble of regional climate scenarios. J. Geophys. Res . https://doi.org/10.1029/2008JD011523 (2009).
Tabari, H. Climate change impact on flood and extreme precipitation increases with water availability. Sci. Rep. 10 , 13768 (2020).
Blöschl, G. et al. Changing climate both increases and decreases European river floods. Nature 573 , 108–111 (2019).
Mosley, L. M. Drought impacts on the water quality of freshwater systems; review and integration. Earth-Sci. Rev. 140 , 203–214 (2015).
Murdoch, P. S., Baron, J. S. & Miller, T. L. Potential effects of climate chance on surface-water quality in North America. J. Am. Water Resour. Assoc. 36 , 347–366 (2000).
Mishra, A., Alnahit, A. & Campbell, B. Impact of land uses, drought, flood, wildfire, and cascading events on water quality and microbial communities: a review and analysis. J. Hydrol. 596 , 125707 (2021).
Wada, Y., van Beek, L. P. H., Wanders, N. & Bierkens, M. F. P. Human water consumption intensifies hydrological drought worldwide. Environ. Res. Lett. 8 , 34036 (2013).
Gu, L. et al. Intensification of global hydrological droughts under anthropogenic climate warming. Water Resour. Res. 59 , e2022WR032997 (2023).
Fischer, E. M. & Schär, C. Consistent geographical patterns of changes in high-impact European heatwaves. Nat. Geosci. 3 , 398–403 (2010).
Arias, P.A. et al in Climate Change 2021: The Physical Science Basis (eds. Masson-Delmotte, V. et al.) 33−144 (Cambridge Univ. Press, 2021).
Tassone, S. J. et al. Increasing heatwave frequency in streams and rivers of the United States. Limnol. Oceanogr. Lett. 8 , 295–304 (2022).
Woolway, R. I. et al. Lake heatwaves under climate change. Nature 589 , 402–407 (2021).
van Vliet, M. T. H. & Zwolsman, J. J. G. Impact of summer droughts on the water quality of the Meuse river. J. Hydrol. 353 , 1–17 (2008).
Hellwig, J., Stahl, K. & Lange, J. Patterns in the linkage of water quantity and quality during low-flows. Hydrol. Process. 31 , 4195–4205 (2017).
Jones, E. & van Vliet, M. T. H. Drought impacts on river salinity in the southern US: implications for water scarcity. Sci. Total Environ. 644 , 844–853 (2018).
Anderson, T. T. et al. Catchment-scale groundwater-flow and recharge paradox revealed from base flow analysis during the Australian Millennium Drought (Mt Lofty Ranges, South Australia). Hydrogeol. J. 29 , 963–983 (2021).
Abdullah, A. D. et al. Predicting the salt water intrusion in the Shatt al-Arab estuary using an analytical approach. Hydrol. Earth Syst. Sci. 20 , 4031–4042 (2016).
Garcés-Vargas, J. et al. Tidally forced saltwater intrusions might impact the quality of drinking water, the Valdivia River (40° S), Chile Estuary Case. Water 12 , 1 (2020).
Thorslund, J., Bierkens, M. F. P., Scaini, A., Sutanudjaja, E. H. & van Vliet, M. T. H. Salinity impacts on irrigation water-scarcity in food bowl regions of the US and Australia. Environ. Res. Lett. 17 , 84002 (2022).
Méndez-Freire, V., Villaseñor, T. & Mellado, C. Spatial and temporal changes in suspended sediment fluxes in Central Chile induced by the mega drought: the case of the Itata River Basin (36°–37°S). J. South Am. Earth Sci . https://doi.org/10.1016/j/jsames.2022/103930 (2022).
Mosley, L. M. et al. The impact of extreme low flows on the water quality of the Lower Murray River and lakes (South Australia). Water Resour. Manag. 26 , 3923–3946 (2012).
Murphy, S. F., McCleskey, R. B., Martin, D. A., Writer, J. H. & Ebel, B. A. Fire, flood, and drought: extreme climate events alter flow paths and stream chemistry. J. Geophys. Res. Biogeosci. 123 , 2513–2526 (2018).
Scanlon, B. R., Fakhreddine, S., Reedy, R. C., Yang, Q. & Malito, J. G. Drivers of spatiotemporal variability in drinking water quality in the United States. Environ. Sci. Technol. 2022 , 12965–12974 (2022).
Rozemeijer, J. et al. Climate variability effects on eutrophication of groundwater, lakes, rivers, and coastal waters in the Netherlands. Sci. Total Environ. 771 , 145366 (2021).
Yu, L. et al. Groundwater impacts on surface water quality and nutrient loads in lowland polder catchments: monitoring the greater Amsterdam area. Hydrol. Earth Syst. Sci. 22 , 487–508 (2018).
Zhou, X. et al. Exploring the relations between sequential droughts and stream nitrogen dynamics in central Germany through catchment-scale mechanistic modelling. J. Hydrol. 614 , 128615 (2022).
Huisman, J. et al. Cyanobacterial blooms. Nat. Rev. Microbiol. 16 , 471–483 (2018).
Cox, B. A. A review of dissolved oxygen modelling techniques for lowland rivers. Sci. Total Environ. 314–316 , 303–334 (2003).
Paerl, H. W. Mitigating harmful cyanobacterial blooms in a human- and climatically-impacted world. Life 4 , 988–1012 (2014).
Chen, Y. J., Nicholson, E. & Cheng, S. T. Using machine learning to understand the implications of meteorological conditions for fish kills. Sci. Rep. 10 , 17003 (2020).
Mosley, L. M., Wallace, T., Rahman, J., Roberts, T. & Gibbs, M. An integrated model to predict and prevent hypoxia in floodplain-river systems. J. Environ. Manage. 286 , 112213 (2021).
Koehn, J. D. & Koehn, J. D. Key steps to improve the assessment, evaluation and management of fish kills: lessons from the Murray–Darling river system, Australia. Mar. Freshw. Res. 73 , 269–281 (2021).
Cheng, B. et al. Characterization and causes analysis for algae blooms in large river system. Sustain. Cities Soc. 51 , 101707 (2019).
Xia, R. et al. River algal blooms are well predicted by antecedent environmental conditions. Water Res. 185 , 116221 (2020).
Yang, N. et al. Dam-induced flow velocity decrease leads to the transition from heterotrophic to autotrophic system through modifying microbial food web dynamics. Environ. Res. 212 , 113568 (2022).
Lürling, M., Eshetu, F., Faassen, E. J., Kosten, S. & Huszar, V. L. M. Comparison of cyanobacterial and green algal growth rates at different temperatures. Freshw. Biol. 58 , 552–559 (2013).
Hallegraeff, G. M. et al. Perceived global increase in algal blooms is attributable to intensified monitoring and emerging bloom impacts. Commun. Earth Environ. 2 , 1–10 (2021).
Johnk, K. D. et al. Summer heatwaves promote blooms of harmful cyanobacteria. Glob. Change Biol. 14 , 495–512 (2008).
Whitworth, K. L. & Baldwin, D. S. Improving our capacity to manage hypoxic blackwater events in lowland rivers: the Blackwater Risk Assessment Tool. Ecol. Modell. 320 , 292–298 (2016).
Veraart, A. J., de Klein, J. J. M. & Scheffer, M. Warming can boost denitrification disproportionately due to altered oxygen dynamics. PLoS ONE 6 , e18508 (2011).
Hirabayashi, Y., Kanae, S., Emori, S., Oki, T. & Kimoto, M. Global projections of changing risks of floods and droughts in a changing climate. Hydrol. Sci. J.-J. Des. Sci. Hydrol. 53 , 754–772 (2008).
Breinl, K., Di Baldassarre, G., Mazzoleni, M., Lun, D. & Vico, G. Extreme dry and wet spells face changes in their duration. Environ. Res. Lett. 15 , ab7d05 (2020).
Blöschl, G. Three hypotheses on changing river flood hazards. Hydrol. Earth Syst. Sci. 26 , 5015–5033 (2022).
Carroll, R. W. H., Warwick, J. J., James, A. I. & Miller, J. R. Modeling erosion and overbank deposition during extreme flood conditions on the Carson River, Nevada. J. Hydrol. 297 , 1–21 (2004).
Dennis, I. A., Macklin, M. G., Coulthard, T. J. & Brewer, P. A. The impact of the October–November 2000 floods on contaminant metal dispersal in the River Swale catchment, North Yorkshire, UK. Hydrol. Process. 17 , 1641–1657 (2003).
Milačič, R., Zuliani, T., Vidmar, J., Oprčkal, P. & Ščančar, J. Potentially toxic elements in water and sediments of the Sava River under extreme flow events. Sci. Total Environ. 605–606 , 894–905 (2017).
Nábělková, J., Šťastná, G. & Komínková, D. Flood impact on water quality of small urban streams. Water Sci. Technol. 52 , 267–274 (2005).
Saha, A. et al. Impacts of a massive flood event on the physico-chemistry and water quality of River Pampa in Western Ghats of India. Int. J. Environ. Anal. Chem. 102 , 7969–7987 (2020).
van Emmerik, T. et al. Hydrology as a driver of floating river plastic transport. Earth’s Future 10 , e2022EF002811 (2022).
Roebroek, C. T. J. et al. Plastic in global rivers: are floods making it worse? Environ. Res. Lett. 16 , 25003 (2021).
Scircle, A., Cizdziel, J. V., Missling, K., Li, L. & Vianello, A. Single-pot method for the collection and preparation of natural water for microplastic analyses: microplastics in the Mississippi River System during and after historic flooding. Environ. Toxicol. Chem. 39 , 986–995 (2020).
Eppehimer, D. E. et al. Impacts of baseflow and flooding on microplastic pollution in an effluent-dependent arid land river in the USA. Environ. Sci. Pollut. Res. Int. 28 , 45375–45389 (2021).
Costa, I. Dda et al. Is the Paraíba do Sul river colourful? Prevalence of microplastics in freshwater, south-eastern Brazil. Mar. Freshw. Res. 73 , 1439–1449 (2022).
Constant, M. et al. Microplastic fluxes in a large and a small Mediterranean river catchments: the Têt and the Rhône, Northwestern Mediterranean Sea. Sci. Total Environ. 716 , 136984 (2020).
de Carvalho, A. R., Riem-Galliano, L., ter Halle, A. & Cucherousset, J. Interactive effect of urbanization and flood in modulating microplastic pollution in rivers. Environ. Pollut . https://doi.org/10.2139/ssrn.4045864 (2022).
Treilles, R. et al. Microplastic and microfiber fluxes in the Seine River: flood events versus dry periods. Sci. Total Environ. 805 , 150123 (2022).
Zhdanov, I. et al. Assessment of seasonal variability of input of microplastics from the Northern Dvina river to the Arctic Ocean. Mar. Pollut. Bull. 175 , 113370 (2022).
Xu, D., Gao, B., Wan, X., Peng, W. & Zhang, B. Influence of catastrophic flood on microplastics organization in surface water of the Three Gorges Reservoir, China. Water Res. 211 , 118018 (2022).
Han, N., Ao, H., Mai, Z., Zhao, Q. & Wu, C. Characteristics of (micro)plastic transport in the upper reaches of the Yangtze river. Sci. Total Environ. 855 , 158887 (2023).
Hitchcock, J. N. Storm events as key moments of microplastic contamination in aquatic ecosystems. Sci. Total Environ. 734 , 139436 (2020).
Yang, C. P., Yu, Y. T. & Kao, C. M. Impact of climate change on Kaoping river water quality. Appl. Mech. Mater. 212–213 , 137–140 (2012).
Krein, A. et al. Concentrations and loads of dissolved xenobiotics and hormones in two small river catchments of different land use in Luxembourg. Hydrol. Process. 27 , 284–296 (2013).
Zouboulis, A. & Tolkou, A. Effect of climate change in wastewater treatment plants: reviewing the problems and solutions. Manag. Water Resour. Under Clim. Uncertain . https://doi.org/10.1007/978-3-319-10467-6_10/FIGURES/10 (2015).
Biswas, T. K. & Mosley, L. M. From mountain ranges to sweeping plains, in droughts and flooding rains; river Murray water quality over the last four decades. Water Resour. Manag. 33 , 1087–1101 (2019).
Rodrigues, M. T. et al. Human adenovirus spread, rainfalls, and the occurrence of gastroenteritis cases in a Brazilian basin. Environ. Monit. Assess. 187 , 720 (2015).
Whitworth, K. L., Baldwin, D. S. & Kerr, J. L. Drought, floods and water quality: drivers of a severe hypoxic blackwater event in a major river system (the southern Murray–Darling Basin, Australia). J. Hydrol. 450–451 , 190–198 (2012).
Lee, M., Shevliakova, E., Malyshev, S., Milly, P. C. D. & Jaffé, P. R. C. G. L. Climate variability and extremes, interacting with nitrogen storage, amplify eutrophication risk. Geophys. Res. Lett . https://doi.org/10.1002/2016gl069254 (2016).
Lee, M., Stock, C. A., Shevliakova, E., Malyshev, S. & Milly, P. C. D. Globally prevalent land nitrogen memory amplifies water pollution following drought years. Environ. Res. Lett. 16 , 014049 (2021).
Kaushal, S. S. et al. Interaction between urbanization and climate variability amplifies watershed nitrate export in Maryland. Environ. Sci. Technol. 42 , 5872–5878 (2008).
Kaushal, S. S. et al. Land use and climate variability amplify carbon, nutrient, and contaminant pulses: a review with management implications 1. J. Am. Water Resour. Assoc. 50 , 585–614 (2014).
Zhou, M. et al. Dilution or enrichment: the effects of flood on pollutants in urban rivers. Environ. Sci. Eur. 34 , 61 (2022).
Pailler, J. Y. et al. Behaviour and fluxes of dissolved antibiotics, analgesics and hormones during flood events in a small heterogeneous catchment in the grand Duchy of Luxembourg. Water Air Soil Pollut. 203 , 79–98 (2009).
Xie, H. & Ringler, C. Agricultural nutrient loadings to the freshwater environment: the role of climate change and socioeconomic change. Environ. Res. Lett. 12 , 104008 (2017).
Wang, M., Kroeze, C., Strokal, M., van Vliet, M. T. H. & Ma, L. Global change can make coastal eutrophication control in China more difficult. Earth’s Future 8 , e2019EF001280 (2020).
van Vliet, M. T. H. et al. Global river discharge and water temperature under climate change. Glob. Environ. Change Policy Dimens. 23 , 450–464 (2013).
Eisner, S. et al. An ensemble analysis of climate change impacts on streamflow seasonality across 11 large river basins. Clim. Change 141 , 401–417 (2017).
Abily, M. et al. Climate change impact on EU rivers’ dilution capacity and ecological status. Water Res. 199 , 117166 (2021).
Knapp, J. L. A., Von Freyberg, J., Studer, B., Kiewiet, L. & Kirchner, J. W. Concentration–discharge relationships vary among hydrological events, reflecting differences in event characteristics. Hydrol. Earth Syst. Sci. 24 , 2561–2576 (2020).
Kaushal, S. S. et al. Freshwater salinization syndrome on a continental scale. Proc. Natl Acad. Sci. USA 115 , E574–E583 (2018).
Bartholow, J. M. Recent water temperature trends in the lower Klamath river, California. North Am. J. Fish. Manag. 25 , 152–162 (2005).
Kaushal, S. S. et al. Rising stream and river temperatures in the United States. Front. Ecol. Environ. 8 , 461–466 (2010).
Pekarova, P. et al. Long-term trend and multi-annual variability of water temperature in the pristine Bela River basin (Slovakia). J. Hydrol. 400 , 333–340 (2011).
Webb, B. W. & Nobilis, F. Long-term changes in river temperature and the influence of climatic and hydrological factors. Hydrol. Sci. J.-J. Des. Sci. Hydrol. 52 , 74–85 (2007).
Lammers, R. B., Pundsack, J. W. & Shiklomanov, A. I. Variability in river temperature, discharge, and energy flux from the Russian pan-Arctic landmass. J. Geophys. Res. 112 , 1–15, G04S59 (2007).
Liu, B. Z., Yang, D. Q., Ye, B. S. & Berezovskaya, S. Long-term open-water season stream temperature variations and changes over Lena River basin in Siberia. Glob. Planet. Change 48 , 96–111 (2005).
Wanders, N., van Vliet, M. T. H., Wada, Y., Bierkens, M. F. P. & van Beek, L. P. H. High-resolution global water temperature modeling. Water Resou. Res. 55 , 2760–2778 (2019).
Bosmans, J. et al. FutureStreams, a global dataset of future streamflow and water temperature. Sci. Data 9 , 307 (2022).
Punzet, M., Voß, F., Voß, A., Kynast, E. & Bärlund, I. A global approach to assess the potential impact of climate change on stream water temperatures and related in-stream first-order decay rates. J. Hydrometeorol. 13 , 1052–1065 (2012).
Delpla, I., Jung, A.-V., Baures, E., Clement, M. & Thomas, O. Impacts of climate change on surface water quality in relation to drinking water production. Environ. Int. 35 , 1225–1233 (2009).
Soong, J. L., Phillips, C. L., Ledna, C., Koven, C. D. & Torn, M. S. CMIP5 models predict rapid and deep soil warming over the 21st century. J. Geophys. Res. Biogeosci. 125 , e2019JG005266 (2020).
Zhang, Q., Qin, W., Feng, J. & Zhu, B. Responses of soil microbial carbon use efficiency to warming: review and prospects. Soil. Ecol. Lett. 4 , 307–318 (2022).
Melillo, J. M. et al. Long-term pattern and magnitude of soil carbon feedback to the climate system in a warming world. Science 358 , 101–105 (2017).
Koch, O., Tscherko, D. & Kandeler, E. Temperature sensitivity of microbial respiration, nitrogen mineralization, and potential soil enzyme activities in organic alpine soils. Glob. Biogeochem. Cycles 21 , 4017 (2007).
Hicks Pries, C. E., Castanha, C., Porras, R. C. & Torn, M. S. The whole-soil carbon flux in response to warming. Science 355 , 1420–1423 (2017).
Bai, E. et al. A meta-analysis of experimental warming effects on terrestrial nitrogen pools and dynamics. N. Phytol. 199 , 441–451 (2013).
Turner, M. M. & Henry, H. A. L. Net nitrogen mineralization and leaching in response to warming and nitrogen deposition in a temperate old field: the importance of winter temperature. Oecologia 162 , 227–236 (2010).
Valenca, R., Ramnath, K., Dittrich, T. M., Taylor, R. E. & Mohanty, S. K. Microbial quality of surface water and subsurface soil after wildfire. Water Res. 175 , 115672 (2020).
Abbott, B. W. et al. Tundra wildfire triggers sustained lateral nutrient loss in Alaskan Arctic. Glob. Change Biol. 27 , 1408–1430 (2021).
Santos, R. M. B., Sanches Fernandes, L. F., Pereira, M. G., Cortes, R. M. V. & Pacheco, F. A. L. Water resources planning for a river basin with recurrent wildfires. Sci. Total Environ. 526 , 1–13 (2015).
Sterling, S. M., Ducharne, A. & Polcher, J. The impact of global land-cover change on the terrestrial water cycle. Nat. Clim. Change 3 , 385–390 (2012).
Cao, Q., Sun, N., Yearsley, J., Nijssen, B. & Lettenmaier, D. P. Climate and land cover effects on the temperature of puget sound streams. Hydrol. Process. 30 , 2286–2304 (2016).
Green, P. et al. Pre-industrial and contemporary fluxes of nitrogen through rivers: a global assessment based on typology. Biogeochemistry 68 , 71–105 (2004).
Meybeck, M. Global analysis of river systems: from Earth system controls to Anthropocene syndromes. Philos. Trans. R. Soc. B Biol. Sci. 358 , 1935–1955 (2003).
Stets, E. G. et al. Landscape drivers of dynamic change in water quality of U.S. rivers. Environ. Sci. Technol. 54 , 4336–4343 (2020).
Bouraoui, F. & Grizzetti, B. Long term change of nutrient concentrations of rivers discharging in European seas. Sci. Total Environ. 409 , 4899–4916 (2011).
Miller, J. D. & Hutchins, M. The impacts of urbanisation and climate change on urban flooding and urban water quality: a review of the evidence concerning the United Kingdom. J. Hydrol. Reg. Stud. 12 , 345–362 (2017).
Marlow, T., Elliott, J. R. & Frickel, S. Future flooding increases unequal exposure risks to relic industrial pollution. Environ. Res. Lett. 17 , 074021 (2022).
Ntelekos, A. A., Oppenheimer, M., Smith, J. A. & Miller, A. J. Urbanization, climate change and flood policy in the United States. Clim. Change 103 , 597–616 (2010).
Koop, S. H. A. The challenges of water, waste and climate change in cities. Environ. Dev. Sustain. 19 , 385–418 (2017).
Strokal, M. et al. Urbanization: an increasing source of multiple pollutants to rivers in the 21st century. npj Urban Sustain. 1 , 24 (2021).
Bloomfield, J. P., Williams, R. J., Gooddy, D. C., Cape, J. N. & Guha, P. Impacts of climate change on the fate and behaviour of pesticides in surface and groundwater — a UK perspective. Sci. Total Environ. 369 , 163–177 (2006).
Jones, E. R. et al. Sub-Saharan Africa will increasingly become the dominant hotspot of surface water pollution. Nat. Water 1 , 602–613 (2023).
Morin-Crini, N. et al. Emerging contaminants: analysis, aquatic compartments and water pollution. Emerging Contaminants Vol. 1 https://doi.org/10.1007/978-3-030-69079-3_1 (2021).
Pérez, C. J., Vega-Rodríguez, M. A., Reder, K. & Flörke, M. A multi-objective artificial bee colony-based optimization approach to design water quality monitoring networks in river basins. J. Clean. Prod. 166 , 579–589 (2017).
Ryberg, K. R. & Chanat, J. G. Climate extremes as drivers of surface-water-quality trends in the United States. Sci. Total Environ. 809 , 152165 (2022).
Mengel, M., Treu, S., Lange, S. & Frieler, K. ATTRICI v1.1 — counterfactual climate for impact attribution. Geosci. Model Dev. 14 , 5269–5284 (2021).
Michalak, A. M. Study role of climate change in extreme threats to water quality. Nature 535 , 349–350 (2016).
Sinha, E., Michalak, A. M. & Balaji, V. Eutrophication will increase during the 21st century as a result of precipitation changes. Science 357 , 405–408 (2017).
Sinha, E. et al. Modeling perennial bioenergy crops in the E3SM land model (ELMv2). J. Adv. Model. Earth Syst . https://doi.org/10.1029/2022MS003171 (2023).
van Vliet, M. T. H. et al. Model inter-comparison design for large-scale water quality models. Curr. Opin. Environ. Sustain. 36 , 59–67 (2019).
WWQA. A Partnership Effort . https://www.unep.org/explore-topics/water/what-we-do/improving-and-assessing-world-water-quality-partnership-effort (accessed 2023).
ISIMIP. Inter-Sectoral Impact Model Intercomparison Project . https://www.isimip.org/ (accessed 2023).
Chen, X. et al. Multi-scale modeling of nutrient pollution in the rivers of China. Environ. Sci. Technol. 53 , 9614–9625 (2019).
Tang, T. et al. Bridging global, basin and local-scale water quality modeling towards enhancing water quality management worldwide. Curr. Opin. Environ. Sustain. 36 , 39–48 (2019).
Hofstra, N., Kroeze, C., Flörke, M. & van Vliet, M. T. H. Editorial overview: water quality: a new challenge for global scale model development and application. Curr. Opin. Environ. Sustain. 36 , A1–A5 (2019).
Strokal, M. et al. Cost-effective management of coastal eutrophication: a case study for the Yangtze River basin. Resour. Conserv. Recycl. 154 , 104635 (2020).
Wang, M. et al. Accounting for interactions between sustainable development goals is essential for water pollution control in China. Nat. Commun. 13 , 730 (2022).
Jarosiewicz, P., Fazi, S. & Zalewski, M. How to boost ecohydrological nature-based solutions in water quality management. Ecohydrol. Hydrobiol. 22 , 226–233 (2022).
Copernicus Global Land Surface — Lake Water Quality (Copernicus, 2023); https://land.copernicus.eu/global/products/lwq .
IIWQ World Water Quality Information and Capacity Building Portal (UNESCO & EOMAP, 2023); http://www.worldwaterquality.org/ .
Dekker, A. & Hestir, E. Evaluating the Feasibility of Systematic Inland Water Quality Monitoring with Satellite Remote Sensing, CSIRO: Water for a Healthy Country National Research Flagship (CSIRO, 2012).
Lymburner, L. et al. Landsat 8: providing continuity and increased precision for measuring multi-decadal time series of total suspended matter. Remote Sens. Environ. 185 , 108–118 (2016).
Mi, H., Fagherazzi, S., Qiao, G., Hong, Y. & Fichot, C. G. Climate change leads to a doubling of turbidity in a rapidly expanding Tibetan lake. Sci. Total Environ. 688 , 952–959 (2019).
Baltodano, A., Agramont, A., Reusen, I. & van Griensven, A. Land cover change and water quality: how remote sensing can help understand driver–impact relations in the Lake Titicaca basin. Water 14 , 1021 (2022).
Odermatt, D., Giardino, C. & Heege, T. Chlorophyll retrieval with MERIS Case-2-Regional in perialpine lakes. Remote Sens. Environ. 114 , 607–617 (2010).
Heege, T., Kiselev, V., Wettle, M. & Hung, N. N. Operational multi-sensor monitoring of turbidity for the entire Mekong Delta. Int. J. Remote Sens. 35 , 2910–2926 (2014).
Lisle, S., D, P. J. & H, M. W. An evaluation of nitrate, fDOM, and turbidity sensors in New Hampshire streams. Water Resour. Res. 54 , 2466–2479 (2018).
Handcock, R. N. et al. Accuracy and uncertainty of thermal-infrared remote sensing of stream temperatures at multiple spatial scales. Remote Sens. Environ. 100 , 427–440 (2006).
Chen, C. Q., Shi, P. & Mao, Q. W. Application of remote sensing techniques for monitoring the thermal pollution of cooling-water discharge from nuclear power plant. J. Environ. Sci. Heal. A 38 , 1659–1668 (2003).
Ling, F. et al. Monitoring thermal pollution in rivers downstream of dams with Landsat ETM+ thermal infrared images. Remote Sens. 9 , 1175 (2017).
Luo, L. et al. A novel early warning system (EWS) for water quality, integrating a high-frequency monitoring database with efficient data quality control technology at a large and deep lake (Lake Qiandao), China. Water 14 , 602 (2022).
Kirschke, S. et al. Citizen science projects in freshwater monitoring. From individual design to clusters? J. Environ. Manage 309 , 114714 (2022).
Deltares. Nitrate App. https://publicwiki.deltares.nl/display/wqapp (2023).
Beusen, A. H. W. et al. Exploring river nitrogen and phosphorus loading and export to global coastal waters in the shared socio-economic pathways. Glob. Environ. Change 72 , 102426 (2022).
Jones, E. R., van Vliet, M. T. H., Qadir, M. & Bierkens, M. F. P. Country-level and gridded estimates of wastewater production, collection, treatment and reuse. Earth Syst. Sci. Data 13 , 237–254 (2021).
Ehalt Macedo, H. et al. Distribution and characteristics of wastewater treatment plants within the global river network. Earth Syst. Sci. Data 14 , 559–577 (2022).
Desbureaux, S. et al. Mapping global hotspots and trends of water quality (1992–2010): a data driven approach. Environ. Res. Lett. 17 , 114048 (2022).
Hameed, M. et al. Application of artificial intelligence (AI) techniques in water quality index prediction: a case study in tropical region, Malaysia. Neural Comput. Appl. 28 , 893–905 (2017).
Chau, K. A review on integration of artificial intelligence into water quality modelling. Mar. Pollut. Bull. 52 , 726–733 (2006).
Khan, Y. & Chai, S. S. Ensemble of ANN and ANFIS for water quality prediction and analysis — a data driven approach. J. Telecommun. Electron. Comput. Eng. 9 , 117–122 (2017).
Heddam, S. & Kisi, O. Modelling daily dissolved oxygen concentration using least square support vector machine, multivariate adaptive regression splines and M5 model tree. J. Hydrol. 559 , 499–509 (2018).
Wang, X., Zhang, F. & Ding, J. Evaluation of water quality based on a machine learning algorithm and water quality index for the Ebinur Lake Watershed, China. Sci. Rep. 7 , 12858 (2017).
Yajima, H. & Derot, J. Application of the random forest model for chlorophyll- a forecasts in fresh and brackish water bodies in Japan, using multivariate long-term databases. J. Hydroinformatics 20 , jh2017010 (2017).
Download references
Acknowledgements
The authors kindly acknowledge J. Banken of Wageningen University and K. Schweden of Ruhr University Bochum for their assistance with collecting water quality literature. The authors thank M. Stoete of Utrecht University for her assistance in designing some figures. The authors also acknowledge the World Water Quality Alliance (WWQA), ISI-MIP and EU COST-Action PROCLIAS initiatives. M.T.H.v.V. was financially supported by the European Union (ERC Starting Grant, B-WEX, Project 101039426) and Netherlands Scientific Organisation (NWO) by a VIDI grant (VI.Vidi.193.019). M.S. was supported by the Netherlands Scientific Organisation (NWO) by a VENI grant (016.Veni.198.001). J.T. was financially supported by The Swedish Research Council Formas (Project No. 2018-00812).
Author information
Authors and affiliations.
Department of Physical Geography, Faculty of Geosciences, Utrecht University, Utrecht, The Netherlands
Michelle T. H. van Vliet & Josefin Thorslund
Department of Physical Geography and the Bolin Centre for Climate Research, Stockholm University, Stockholm, Sweden
Josefin Thorslund
Water Systems and Global Change Group, Wageningen University, Wageningen, The Netherlands
Maryna Strokal & Nynke Hofstra
Institute of Engineering Hydrology and Water Resources Management, Ruhr University Bochum, Bochum, Germany
Martina Flörke
Department of Geography, McGill University, Montréal, Quebec, Canada
Heloisa Ehalt Macedo
Vrije Universiteit Brussel, Brussels, Belgium
Albert Nkwasa & Ann van Griensven
Directorate of Water Resources Management, Ministry of Water and Environment, Kampala, Uganda
Albert Nkwasa
International Institute for Applied Systems Analysis, Laxenburg, Austria
Department of Geology and Earth System Science Interdisciplinary Center, University of Maryland, UMD, College Park, MD, USA
Sujay S. Kaushal
Helmholtz Centre for Environmental Research — UFZ, Leipzig, Germany
Rohini Kumar
Department of Earth Sciences — Geochemistry, Faculty of Geosciences, Utrecht University, Utrecht, The Netherlands
Lex Bouwman
PBL Netherlands Environmental Assessment Agency, The Hague, The Netherlands
School of Biological Sciences, Faculty of Science, Engineering and Technology, University of Adelaide, Adelaide, SA, Australia
Luke M. Mosley
You can also search for this author in PubMed Google Scholar
Contributions
M.T.H.v.V. designed and led the study and manuscript effort. J.T. contributed to the design of the literature review. J.T., M.S., N.H., M.F., H.E.M., A.N., T.T. and M.T.H.v.V. collected literature for the analyses and wrote reports for specific water quality constituents for the supplementary information. L.M.M., S.S.K. and R.K. contributed to the writing of specific sections. All authors contributed to the writing of the manuscript.
Corresponding author
Correspondence to Michelle T. H. van Vliet .
Ethics declarations
Competing interests.
The authors declare no competing interests.
Peer review
Peer review information.
Nature Reviews Earth & Environment thanks J. Rozemeijer, D. Barceló, E. Douglas and the other, anonymous, reviewer(s) for their contribution to the peer review of this work.
Additional information
Publisher’s note Springer Nature remains neutral with regard to jurisdictional claims in published maps and institutional affiliations.
Supplementary information
Supplementary information, supplementary data, rights and permissions.
Springer Nature or its licensor (e.g. a society or other partner) holds exclusive rights to this article under a publishing agreement with the author(s) or other rightsholder(s); author self-archiving of the accepted manuscript version of this article is solely governed by the terms of such publishing agreement and applicable law.
Reprints and permissions
About this article
Cite this article.
van Vliet, M.T.H., Thorslund, J., Strokal, M. et al. Global river water quality under climate change and hydroclimatic extremes. Nat Rev Earth Environ 4 , 687–702 (2023). https://doi.org/10.1038/s43017-023-00472-3
Download citation
Accepted : 19 July 2023
Published : 12 September 2023
Issue Date : October 2023
DOI : https://doi.org/10.1038/s43017-023-00472-3
Share this article
Anyone you share the following link with will be able to read this content:
Sorry, a shareable link is not currently available for this article.
Provided by the Springer Nature SharedIt content-sharing initiative
This article is cited by
River water quality shaped by land–river connectivity in a changing climate.
- Julia L. A. Knapp
Nature Climate Change (2024)
Complex interplay of water quality and water use affects water scarcity under droughts and heatwaves
- Michelle T. H. van Vliet
Nature Water (2023)
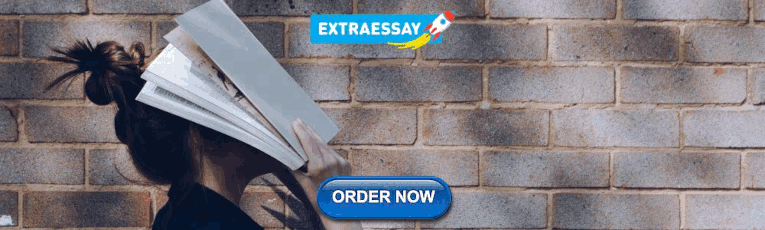
Quick links
- Explore articles by subject
- Guide to authors
- Editorial policies
Sign up for the Nature Briefing newsletter — what matters in science, free to your inbox daily.

Estimating turbidity concentrations in highly dynamic rivers using Sentinel-2 imagery in Google Earth Engine: Case study of the Godavari River, India
- Research Article
- Published: 01 May 2024
Cite this article
- Meena Kumari Kolli 1 , 2 &
- Pennan Chinnasamy ORCID: orcid.org/0000-0002-3184-2134 1 , 2 , 3 , 4 , 5 , 6
Turbidity is an essential biogeochemical parameter for water quality management because it shapes the physical landscape and regulates ecological systems. It varies spatially and temporally across large water bodies, but monitoring based on point-source field observations remains a difficult task in developing countries due to the need for logistics and costs. In this study, we present a novel semi-analytical approach for estimating turbidity from remote sensing reflectance \(({R}_{{\text{rs}}})\) in moderate to highly turbid waters in the lower part of the Godavari River (i.e., locations near Rajahmundry). The proposed method includes two sub-algorithms—Normalized Difference Turbidity Index (NDTI) and semi-empirical single-band turbidity ( \({T}_{{\text{s}}}\) ) algorithm—to retrieve spectral reflectance information corresponding to the study locations for turbidity modeling. Sentinel-2 Multi-Spectral Imager data have been used to quantify the turbidity in the Google Earth Engine (GEE) platform. The correlation analysis was observed between spectral reflectance values and in situ turbidity data using cubic polynomial regression equations. The results indicated that the \({T}_{{\text{s}}}\) , which uses the only red-edge wavelength, identified turbidity as the most accurate across all locations (highest R 2 = 0.91, lowest RMSE = 0.003), followed by NDTI (highest R 2 = 0.85, lowest RMSE = 0.05), respectively. The remote sensing data application provides a better way to monitor turbidity at large spatio-temporal scales in attaining the water quality standards of the Godavari River.
This is a preview of subscription content, log in via an institution to check access.
Access this article
Price includes VAT (Russian Federation)
Instant access to the full article PDF.
Rent this article via DeepDyve
Institutional subscriptions
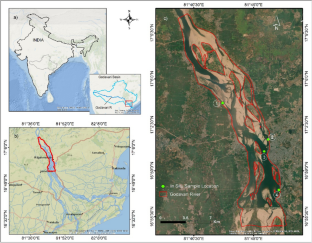
Data availability
Sources of all the data have been described properly. Derived data supporting the findings of this study are available based on the request to the corresponding author.
Ahmed U, Mumtaz R, Anwar H, Shah AA, Irfan R, García-Nieto J (2019) Efficient water quality prediction using supervised machine learning. Water 11:2210
Article CAS Google Scholar
Alka S, Sushma P, Singh TS, Patel JG, Tanwar H (2014) Wetland information system using remote sensing and GIS in Himachal Pradesh, India. Asian J Geoinform 14(4):13–22
Google Scholar
Anderson CW (2006) Turbidity (ver. 2.1): US Geological survey techniques of water-resources investigations. Book 9, chap. A6., sec. 6.7., from: http://pubs.water.usgs.gov/twri9A6/ . Accessed 10 Jul 2022
Bosire AS, Nyantika D, Mamboleo D (2021) Evaluation of the effects of human activities on water resources in Masaba North, Nyamira County. Int J Res Sch Commun 4(2):85–98
Bui DT, Khosravi K, Tiefenbacher J, Nguyen H, Kazakis N (2020) Improving prediction of water quality indices using novel hybrid machine-learning algorithms. Sci Total Environ 721:137612
Bustamante J, Pacios F, Diaz-Delgado R, Aragones D (2009) Predictive models of turbidity and water depth in the Donana marshes using LANDSAT TM and ETM + images. J Environ Manage 90:2219–2225
Article Google Scholar
Chander S, Gujrati A, Hakeem KA, Garg V, Issac AM, Dhote PR, Kumar V, Sahay A (2019) Water quality assessment of River Ganga and Chilika lagoon using AVIRIS-NG hyperspectral data. Curr Sci 116(7):1172–1181
Chen Z, Muller-Karger F, Hu C (2007) Remote sensing of water clarity in Tampa Bay. Remote Sens Environ 109:249–259
Chen X, Liu L, Zhang X, Li J, Wang S, Liu D, Duan H, Song K (2021) An assessment of water color for inland water in China using a Landsat 8-Derived Forel-Ule Index and the Google Earth Engine platform. IEEE J Sel Top Appl Earth Obs Remote Sens 14:5773–5785
Chinnasamy P, Honap VU (2023) Spatiotemporal variations in soil loss across the biodiversity hotspots of Western Ghats Region, India. J Earth Syst Sci 132(2):90
Chinnasamy P, Shah Z, Shahid S (2023) Impact of lockdown on air quality during COVID-19 pandemic: a case study of India. J Indian Soc Remote Sens 51(1):103–120
Choubey VK (1992) Correlation of turbidity with Indian Remote Sensing Satellite-1A data. Hydrol Sci 37(2):129–140
DeLuca N, Zaitchik B, Curriero F (2018) Can multispectral information improve remotely sensed estimates of total suspended solids? A statistical study in Chesapeake bay. Rem Sens 10:1393
Dogliotti AI, Ruddick KG, Nechad B, Doxaran D, Knaeps E (2015) A single algorithm to retrieve turbidity from remotely-sensed data in all coastal and estuarine waters. Remote Sens Environ 156:157–168
Garg V, Kumar AS, Aggarwal SP, Kumar V, Dhote PR, Thakur PK, Nikam BR, Sambare RS, Siddiqui A, Muduli PR, Rastogi G (2017) Spectral similarity approach for mapping turbidity of an inland waterbody. J Hydrol 550:527–537
Garg V, Aggarwal SP, Chauhan P (2020) Changes in turbidity along Ganga River using Sentinel-2 satellite data during lockdown associated with COVID-19. Geomat Nat Hazards Risks 11:1175–1195
Gholizadeh MH, Melesse AM, Reddi L (2016) A comprehensive review on water quality parameters estimation using remote sensing techniques. Sensors 16:1298
Gorelick N, Hancher M, Dixon M, Ilyushchhenko S, Thau D, Moore R (2017) Google Earth Engine: Planetary-scale geospatial analysis for everyone. Remote Sens Environ 202:18–27
Hasmadi MI, Norsaliza U (2010) Analysis of SPOT-5 data for mapping turbidity level of River Klang, Peninsular Malaysia. Appl Remote Sens J 1:14–18
Hussain J, Husain I, Arif M, Gupta N (2017) Studies on heavy metal contamination in the Godavari river basin. Appl Water Sci 7:4539–4548
Islam M, Sado K (2006) Analyses of ASTER and Spectroradiometer data with in situ measurements for turbidity and transparency study of lake Abashri. Int J Geoinf 2:31–45
Konik M, Kowalczuk P, Zablocka M, Makarewicz A, Meler J, Zdun A, Darecki M (2020) Empirical relationships between remote-sensing reflectance and selected inherent optical properties in Nordic Sea surface water for the MODIS and OLCI Ocean Colour Sensors. Remote Sens 12:2774
Lacaux JP, Tourre YM, Vignolles C, Ndione JA, Lafaye M (2007) Classification of ponds from high-spatial resolution remote sensing: application to Rift Valley fever epidemics in Senegal. Remote Sens Environ 106(1):66–74
Lobo FDL, Nagel GW, Maciel DA, de Carvalho LAS, Martins VS, Barbosa CCF, de Moraes Novo EML (2021) AlgaeMAp: algae bloom monitoring application for inland waters in Latin America. Remote Sens 13:2874
Lonare A, Maheshwari B, Chinnasamy P (2022) Village level identification of sugarcane in Sangali, Maharashtra using open source data. J Agrometeorol 24(3):249–254
Luis KM, Rheuban JE, Kavanaugh MT, Glover DM, Wei J, Lee Z, Doney SC (2019) Capturing coastal water clarity variability with Landsat 8. Mar Pollut Bull 145:96–104
Mahato LL, Pathak AK, Kapoor D, Patel N, Murthy M (2004) Surface water monitoring and evaluation of Indravati reservoir using the application of principal component analysis using satellite remote sensing technology. In: Proceedings of Map Asia, Beijing, China, 26–29 August 2004
Maltese A, Capodici F, Ciraolo G, La Loggia G (2013) Coastal zone water quality: calibration of a water-turbidity equation for MODIS data. Eur J Remote Sens 46:333–347
Markert KN, Schmidt CM, Griffin RE, Flores AI, Poortinga A, Saah DS, Muench RE, Clinton NE, Chishtie F, Kityuttachai K, Someth P, Anderson ER, Aekakkararungroj A, Ganz DJ (2018) Historical and operational monitoring of surface sediments in the Lower Mekong Basin using Landsat and Google Earth Engine Cloud Computing. Remote Sens 10:909
Martinez E, Gorgues T, Lengaigne M, Fontana C, Sauzede R, Menkes C, Uitz J, Di Lorenzo E, Fablet R (2020) Reconstructing global chlorophyll-a variations using a non-linear statistical approach. Front Mar Sci 7:464
McCarthy FMG, Riddick NL, Volik O, Danesh DC, Krueger AM (2018) Algal palynomorphs as proxies of human impact on freshwater resources in the Great Lakes region. Anthropocene 21:16–31
McFeeters SK (1996) The use of the Normalized Difference Water Index (NDWI) in the delineation of open water features. Int J Remote Sens 17(7):1425–1432
Nechad B, Ruddick KG, Park Y (2010) Calibration and validation of a generic multisensor algorithm for mapping of total suspended matter in turbid waters. Remote Sens Environ 114:854–866
Nechad B, Ruddick KG, Neukermans G (2009) Calibration and validation of a generic multisensor algorithm for mapping of turbidity in coastal waters. Proc. SPIE 7473, Remote Sensing of the Ocean, Sea Ice, and Large Water Regions 2009, 74730H. https://doi.org/10.1117/12.830700
Chapter Google Scholar
Papoutsa C, Retalis A, Toulios L, Hadjimitsis DG (2014) Defining the Landsat TM/ETM+ and CHRIS/PROBA spectral regions in which turbidity can be retrieved in inland waterbodies using field spectroscopy. Int J Remote Sens 35:1674–1692
Peterson KT, Sagan V, Sloan JJ (2020) Deep learning-based water quality estimation and anomaly detection using Landsat-8/Sentinel-2 virtual constellation and cloud computing. Giscience Remote Sens 57:510–525
Pote SK, Singal SK (2012) Srivastava DK (2012) Assessment of surface water quality of Godavari River at Aurangabad. Asian J Water Environ Pollut 9(1):117–122
CAS Google Scholar
Potes M, Costa MJ, Salgado R (2012) Satellite remote sensing of water turbidity in Aiqueva reservoir and implications on lake modeling. Hydrol Earth Syst Sci Discuss 16:1623–1633
Quang NH, Sasaki J, Higa H, Huan NH (2017) Spatiotemporal variation of turbidity based on Landsat 8 OLI in Cam Ranh Bay and Thuy Trieu Lagoon, Vietnam. Water 9(8):570
Rodríguez-López L, Duran-Llacer I, González-Rodríguez L, Cardenas R, Urrutia R (2021) Retrieving water turbidity in Araucanian lakes (South-Central Chile) based on Multispectral Landsat Imagery. Remote Sens 13:3133
Rodriguez-Perez J, Leigh C, Liquet B, Kermorvant C, Peterson E, Sous D, Mengersen K (2020) Detecting technical anomalies in high-frequency water-quality data using artificial neural networks. Environ Sci Technol 54:13719–13730
Sebastiá-Frasquet MT, Aguilar-Maldonado JA, Santamaría-Del-Ángel E, Estornell J (2019) Sentinel 2 analysis of turbidity patterns in a coastal lagoon. Remote Sens 11(24):2926
Simpson ZP, Haggard BE (2018) Optimizing the flow adjustment of constituent concentrations via LOESS for trend analysis. Environ Monit Assess 190:103. https://doi.org/10.1007/s10661-018-6461-5
Singh N, Nalgire SM, Gupta M, Chinnasamy P (2022) Potential of open source remote sensing data for improved spatiotemporal monitoring of inland water quality in India: case study of Gujarat. Photogramm Eng Remote Sens 88:155–163
Traganos D, Poursanidis D, Aggarwal B, Chrysoulakis N, Reinartz P (2018) Estimating satellite-derived bathymetry (SDB) with the Google Earth Engine and Sentinel-2. Remote Sens 10:859
Union E (2008) Directive 2008/56/EC of the European Parliament and of the council of June 17 2008 establishing a framework for community action in the field of marine environmental policy (Marine Strategy Framework Directive). Off J Eur Union 164:19–40
Vos K, Splinter KD, Harley MD, Simmons JA, Turner IL (2019) CoastSat: A Google Earth Engine-enabled Python toolkit to extract shorelines from publicly available satellite imagery. Environ Model Softw 122:104528
Wang L, Xu M, Liu Y, Liu H, Beck R, Reif M, Emery E, Young J, Wu Q (2020) Mapping freshwater chlorophyll-a concentrations at a regional scale integrating multi-sensor satellite observations with Google Earth Engine. Remote Sens 12:3278
Wickham H (2016) ggplot2: elegant graphics for data analysis. Springer-Verlag, New York, New York, USA. https://ggplot2.tidyverse.org/ . Accessed 22 Aug 2022
Wu JL, Ho CR, Huang CC, Srivastav AL, Tzeng JH, Lin YT (2014) Hyperspectral sensing for turbid water quality monitoring in freshwater rivers: empirical relationship between reflectance and turbidity and total solids. Sensors 14:22670–22688
Yin F, Lewis PE, Gomez-Dans J, Wu Q (2019) A sensor-invariant atmospheric correction method: application toSentinel-2/MSI and Landsat 8/OLI. https://doi.org/10.31223/osf.io/ps957
Yunus AP, Masago Y, Hijioka Y (2020) COVID-19 and surface water quality: improved lake water quality during the lockdown. Sci Total Environ 731:139012
Yunus AP, Masago Y, Hijioka Y (2021) Analysis of long-term (2002–2020) trends and peak events in total suspended solids concentrations in the Chesapeake Bay using MODIS imagery. J Environ Manage 299:113550
Zhang C, Di L, Yang Z, Lin L, Hao P (2020) AgKit4EE: A toolkit for agricultural land use modeling of the conterminous United States based on Google Earth Engine. Environ Model Softw 129:104694
Zheng G, DiGiacomo PM (2022) A simple water-clarity turbidity index for the Great Lakes. J Great Lakes Res 48:684–694
Download references
Acknowledgements
This work is partially supported by the Institute Post-doctoral Fellowship at IIT Bombay. The authors would like to thank the European Space Agency for free access to the Sentinel-2 data. We are grateful to Google for the GEE platform, which provided an efficient and powerful computing platform.
This research has been supported by the Water Productivity Improvement in Practice (Water-PIP) project, which is funded by the IHE Delft Water and Development Partnership Programme (WDPP) under the programmatic cooperation between the Directorate General for International Cooperation (DGIS) of the Ministry of Foreign Affairs of the Netherlands and IHE Delft (ID: DGIS Activity DME0121369).
Award Number: 111349 (WATERPIP project) | Recipient: Pennan Chinnasamy, Ph.D.
Author information
Authors and affiliations.
Centre for Technology Alternatives for Rural Areas, Indian Institute of Technology (IITB), Powai, Mumbai, Maharashtra, 400076, India
Meena Kumari Kolli & Pennan Chinnasamy
Rural Data Research and Analysis Lab (RuDRA), IIT Bombay, Mumbai, India
Interdisciplinary Programme in Climate Studies (IDPCS), IIT Bombay, Mumbai, India
Pennan Chinnasamy
Centre for Machine Intelligence and Data Science(C‑MInDS), IIT Bombay, Mumbai, India
Ashank Desai Centre for Policy Studies, IIT Bombay, Mumbai, India
Nebraska Water Center, University of Nebraska, Lincoln, NE, USA
You can also search for this author in PubMed Google Scholar
Contributions
Ideation, discussion, data sources identification, draft preparation, editing, and finalization—Prof. Pennan Chinnasamy.
Data collection, GIS, analysis, draft preparation, writing, and maps—Meena Kumari Kolli.
Corresponding author
Correspondence to Pennan Chinnasamy .
Ethics declarations
Ethical approval.
Not applicable.
Consent to participate
Consent for publication, competing interests.
The authors declare no competing interests.
Additional information
Responsible Editor: Philippe Garrigues
Publisher's Note
Springer Nature remains neutral with regard to jurisdictional claims in published maps and institutional affiliations.
Rights and permissions
Springer Nature or its licensor (e.g. a society or other partner) holds exclusive rights to this article under a publishing agreement with the author(s) or other rightsholder(s); author self-archiving of the accepted manuscript version of this article is solely governed by the terms of such publishing agreement and applicable law.
Reprints and permissions
About this article
Kolli, M.K., Chinnasamy, P. Estimating turbidity concentrations in highly dynamic rivers using Sentinel-2 imagery in Google Earth Engine: Case study of the Godavari River, India. Environ Sci Pollut Res (2024). https://doi.org/10.1007/s11356-024-33344-4
Download citation
Received : 06 November 2022
Accepted : 11 April 2024
Published : 01 May 2024
DOI : https://doi.org/10.1007/s11356-024-33344-4
Share this article
Anyone you share the following link with will be able to read this content:
Sorry, a shareable link is not currently available for this article.
Provided by the Springer Nature SharedIt content-sharing initiative
- Sentinel-2 Multi-Spectral Image
- Normalized Difference Turbidity Index
- Single-band turbidity
- Regression analysis
- Godavari River
- Google Earth Engine
- Find a journal
- Publish with us
- Track your research
Academia.edu no longer supports Internet Explorer.
To browse Academia.edu and the wider internet faster and more securely, please take a few seconds to upgrade your browser .
Enter the email address you signed up with and we'll email you a reset link.
- We're Hiring!
- Help Center
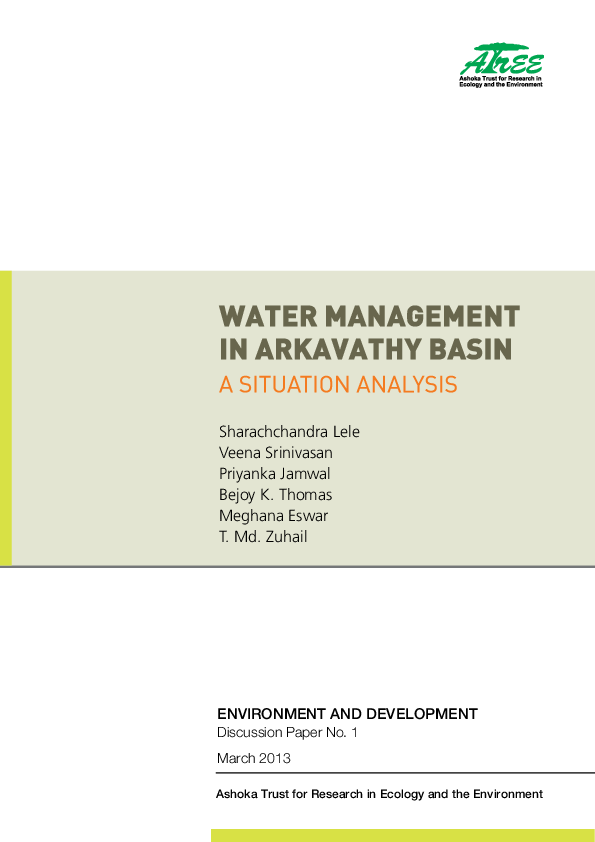
Water management in Arkavathy basin: A situation analysis

Related Papers
E&D Discussion Paper
Veena Srinivasan , Priyanka Jamwal
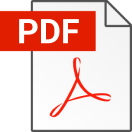
Dr. Amartya Kumar Bhattacharya
Dr.Aravinda P T
Vrishabhavathi Watershed is a constituent of the Arkavathi River Basin, Bangalore Urban and Ramanagara District and covers an area of 360.62 Km2, representing seasonally dry tropical climate. In Vrishabhavathi catchment Vrishabhavathi River is the main source of surface water source which is tributary of River Arkavathy, which joins the Cauvery River at Mekedatu near sangama. Earlier this holy water was mainly used for agricultural purpose and drinking purpose. The Catchment lies in Bangalore urban and Bangalore rural area. The stream which flows in urban region receives a lot of waste water released from industrial and domestic areas. The water is highly polluted and generates lot of foul smell in the flow stretch in urban region. An attempt has been made to identify the potential sites for detention ponds along the Vrishabhavathi River which will be used to maintain the acceptable water quality for sewage Irrigation. For this analysis we need the Base map and thematic maps such as Drainage map, Land use /land cover map and Vrishabhavathi River geometry map. Remote sensing and GIS is useful tool to identify the suitable sites for detention ponds along the River. By assigning the weights to point feature in Vrishabhavathi River geometry map, then calculating suitability indices potential sites for detention ponds are identified .The water stored in the detention ponds are continuously treated with minimum cost and allowed to flow in the downstream for further oxidation. At suitable location water can be lifted up to suitable near water body and used for sewage irrigation.
Geological Society of India Special Publication No. 3
Sunando Bandyopadhyay , Sayantan Das , Nabendu Sekhar Kar , Jayanta Sen
West Bengal (88,752 km²) is the only Indian state that stretches from the Himalaya to the Bay of Bengal. It can be divided into nine physiographic provinces of which the Himalayas, the western plateaus, the northern and western alluvial fans and the Ganga delta are most important. The development of the river system of the state was largely governed by tectonic evolution of the eastern Himalaya and western edges of the Bengal basin. The characteristics of the rivers as well as a number of aspects of water resources of the state can be linked to its physiographic regions. The principal issues associated with water in West Bengal include river degeneration, channel shifting, flood, urban waterlogging, drought, pollution, groundwater depletion and inland navigation. The management of water is practised by river impoundment projects in various scales and by drainage schemes. It is estimated that the western and eastern parts of the state are most water stressed regions due to climatic and human factors, respectively. The solution to many of the water-related problems of West Bengal, progressive or cyclic, can be addressed by putting emphasis on participatory management besides government intervention.
Sharachchandra (Sharad) Lele
SUSANTA BISWAS
Sharachchandra (Sharad) Lele , Veena Srinivasan
Water planning decisions are only as good as our ability to explain historical trends and make reasonable predictions of future water availability. But predicting water availability can be a challenge in rapidly growing regions, where human modifications of land and waterscapes are changing the hydrologic system. Yet, many regions of the world lack the long-term hydrologic monitoring records needed to understand past changes and predict future trends. We investigated this “predictions under change” problem in the data-scarce Thippagondanahalli (TG Halli) catchment of the Arkavathy sub-basin in southern India. Inflows into TG Halli reservoir have declined sharply since the 1970s. The causes of the drying are poorly understood, resulting in misdirected or counter-productive management responses. Five plausible hypotheses that could explain the decline were tested using data from field surveys and secondary sources: (1) changes in rainfall amount, seasonality and intensity; (2) increases in temperature; (3) groundwater extraction; (4) expansion of eucalyptus plantations; and (5) fragmentation of the river channel. Our results suggest that groundwater pumping, expansion of eucalyptus plantations and, to a lesser extent, channel fragmentation are much more likely to have caused the decline in surface flows in the TG Halli catchment than changing climate. The multiple-hypothesis approach presents a systematic way to quantify the relative contributions of proximate anthropogenic and climate drivers to hydrological change. The approach not only makes a meaningful contribution to the policy debate but also helps prioritize and design future research. The approach is a first step to conducting use-inspired socio-hydrologic research in a watershed.
adikant Pradhan , dinesh marothia
ISSN: 2319-7706 Volume 8 Number 01 (2019) Journal homepage: http://www.ijcmas.com Topographical and other constraints further limit the utilizable quantity of water which rapid growth in the demand for water due to rise in population, increased pace of urbanization and industrialization is posing serious challenges in providing food and water security. King Dalpatdev Kaktiya was a king from the Kakatiya dynasty who made reservoir in north-west part of Jagdalpur and named Dalpatsagar is now being affected badly by city expansion and draining sewage into the reservoir. Earlier the people settled at top of the toposequence which supported for run off flow but expansion of city on catchment areas created problem. For assessing effect of Dalpatsagar on water resource, the data on 121 dugwells and borewells were taken under consideration of depth pre (April-May) and post (October-November) monsoon observations during 2015-16 along with locations in Jagdalpur city. In which 121 dugwells, 6...
Strategic Analyses of the …
Luna Bharati
IOSR Journals
Background: Urbanization as a structural process of change is generally related to the concentration of large-scale and small scale industrial and commercial, financial and administrative set up in the cities (Newman, 2011). Accelerated urbanization and industrialization are two of the main processes responsible for increased pressure and deterioration of water resources. Naihati Municipality in Barrackpore Sub division is highly urbanized area and the population pressure is also very high which affects the quality and also quantity of ground water sources. The people of this municipality depend on the underground water sources for their daily domestic water use and also drinking purposes. Besides, due to the rapid growth of industrialization mainly, different small and medium scales industries the whole required water withdrawals from the underground water sources. This study reveals the affects of urban industrial expansion on water resources both the ground water and also the surface water sources. Materials and Methods: Naihati Municipality has a strong industrial background and a huge population pressure, to understand the present situation of water sources both the surface and ground water source so this study consist both the Primary survey (including GPS survey) and secondary data collections methods. Primary data were collected through household survey by interview (face to face) method and the secondary data was collected from different secondary sources like Barrackpore Subdivision Office, Naihati Municipality, District Census hand book and Census of India. All the collected data were tabulated and computed through MS ward 2007 and MS Excel for constructing different charts and diagrams by using various cartographic techniques. Maps are prepared through (QGIS, Arc GIS) open source software. Results: In the Naihati Municipality there are 31 no of wards with a density of population 18891 person/sq km (2011). Due to the urban growth and also industrial development a high water demand is noticed here. But the municipal water supply does not satisfy this high demand of water among the urban people and create a negative water balance. Besides, the only water source is from underground water so naturally the ground water levels decline day by day. This study represents the above scenario of Naihati Municipality and introduced some possible suggestions and recommendations. Conclusion: From the study it is identified that urbanization is highly related the industrialization and also modernization. A rapid growth of industrialization and increasing population are the two main features in this municipality along the bank of the river Hugly, for easy availability of water and transport.
RELATED PAPERS
Isabel Izquierdo
Innocent Musonda
distributor kaligrafi
distributorkaligrafi blitar
CRISTIAN JAVIER DOMÍNGUEZ MALPARTIDA
feloussi messaoud
The Journal of Immunology
Farzin Farzaneh
Brazilian Journal of Development
Eduardo Henrique de Oliveira Barbosa
International Journal of Academic Medicine and Pharmacy
Erhan Bozkurt
Eko Aribowo
Hepatitis Monthly
Shima Salimi
Physics Letters B
Eliezer Piasetzky
proxy.furb.br
Gabriel Barreto
Nadiyah Wahyu Ananda
nadiyah wahyu ananda
Zahra Sanat
Jurnal Ekonomi dan Bisnis
Ahmad Nur rosyid
IEEE Transactions on Information Theory
Vaneet Aggarwal
Reseach Publisher
Archives of Internal Medicine
Ivanka Matoušková
Fitness & Performance Journal
Estelio H. M. Dantas
Olga Arnaudova
Journal on Education
Mas'ud Muhammadiah
Revista de Enfermedades no Transmisibles Finlay
Ahmed Jose Pomares Avalos
IEEE Transactions on Computational Social Systems
Dimitris Askounis
Rose Duroux
- We're Hiring!
- Help Center
- Find new research papers in:
- Health Sciences
- Earth Sciences
- Cognitive Science
- Mathematics
- Computer Science
- Academia ©2024
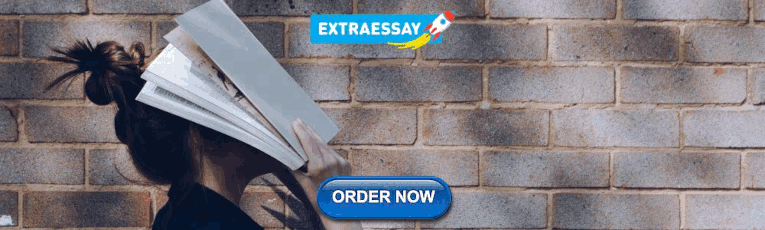
IMAGES
VIDEO
COMMENTS
Changes in hydrology and hydrometeorology of the Arkavathy Basin, 1970-2010. (a) Annual inflows into the TG Halli reservoir . The 1938-1975, 1975-2000 and 2000-2010 median and mean annual ...
The case study shows that direct human interventions play a significant role in alter-ing the hydrology of watersheds. The multiple working hypotheses approach presents 25 a systematic way to quantify the relative contributions of anthropogenic drivers to hy-drologic change. The approach not only yields a meaningful contribution to the policy
affected by nitrate pollution, which is not surprising, given that this tank is situated on the highly polluted Vrishabhavathy River. In another study also, nitrate levels Table 4: Nitrate levels in groundwater in talukas overlapping with the Arkavathy sub-basin Taluka Anekal Bangalore North Bangalore South Doddaballapur Kanakapura Magadi ...
the Arkavathy watershed in southern India. Five possible hy-potheses that link anthropogenic and climatic changes to the water scarcity in the watershed are outlined and investigated. 2 The problem: drying of TG Halli reservoir 2.1 Description of study area The Arkavathy River is located in the state of Karnataka in southern India (Fig.1).
The paper asks why the Arkavathy River in southern India is drying. The study results indicate that anthropogenic drivers like groundwater pumping, eucalyptus plantations and channel fragmentation are much more likely to have caused the decline than changing climate.
Water planning decisions are only as good as our ability to explain historical trends and make reasonable predictions of future water availability. But predicting water availability can be a challenge in rapidly growing regions, where human
30 The case study shows that direct human interventions play a significant role in altering the hydrology of watersheds. The ... Why is the Arkavathy River drying? 3 ad-hoc decisions. There is an ...
In this study, the water samples are collected from 8 selected sampling stations of Arkavathi River during the study period of post monsoon month in February 2020 for physio-chemical analysis and ...
Most of the global population will live in urban areas in the 21st century. We study impacts of urbanization on future river pollution taking a multi-pollutant approach. We quantify combined point ...
Comparison of 965 case studies indicates that river water quality generally deteriorates under droughts and heatwaves (68% of compiled cases), rainstorms and floods (51%) and under long-term ...
5 This case study of the data-scarce, upper Arkavathy watershed, near the city of Bengaluru in south- ... and north east (Oct-Dec) monsoons. The main stem of the Arkavathy River has its headwaters in the Nandi Hills north of Bengaluru and is joined by its first major tributary, the Ku-80 mudavathy River at Thippagondanahalli village, where the ...
Enter the email address you signed up with and we'll email you a reset link.
The Arkavathy sub-basin, which is part of the Cauvery basin, is a highly stressed, rapidly urbanising watershed on the outskirts of the city of Bengaluru. The purpose of this situation analysis document is to summarise the current state of knowledge on water management in the Arkavathy sub-basin and identify critical knowledge gaps to inform future researchers in the basin.
A one-day "Action plan workshop for the rejuvenation and sustenance of Arkavathi river basin", was organised on 25th July 2009, by the Global Academy of Technology and Geological Society of India at Bangalore, to discuss the future of the dying Arkavathi river and Bangalore's precarious water situation, and to develop concurrent implementable action plans to address the problem.
The Arkavathy River feeds a series of cascading tanks . ... is illustrated by a set of case studies of Tataguni, ... monitored the level of pollution in groundwater of the .
Arkavathi River. / 13.368689; 77.681335. / 12.287986; 77.432141. The Arkavati is an important mountain river in Karnataka, India, originating at Nandi Hills of Chikkaballapura district. [1] It is a tributary of the Kaveri, which it joins at 34 km south of Kanakapura, Ramanagara District called Sangama in Kannada, after flowing through ...
Figure 1. Map of the upper Arkavathy Watershed, showing regional context within (a) India and (b) KarnatakaBengaluru state, (c) the TG Halli watershed, and (d,e) two intensively-studied milliwatersheds . The Bengaluru (Banga- lore) urban area is shown in red on the eastern boundary of the watershed. 2 Study Area The upper Arkavathy (TG Halli ...
The surface water quality monitoring of the Godavari River is managed by the Central Pollution Control Board (CPCB), and the data is maintained by the India Water Resources Information System (WRIS); thus, the in situ turbidity data were obtained from the WRIS in this study. ... Case study of the Godavari River, India. Environ Sci Pollut Res ...
It also shows a cluster of villages Two studies (Ramesh et al. 2012; Shashirekha 2009) located downstream of Byramangala tank being monitored the level of pollution in groundwater of the affected by nitrate pollution, which is not surprising, Peenya industrial area in the northern Arkavathy basin. given that this tank is situated on the highly ...
So, we focus here on the quality of surface water used for irrigation. Faecal coliforms : Monitoring studies conducted in the Arkavathy sub-basin indicates the presence of FCs in water samples both from Byramangala tank and Arkavathy River downstream of Kanakapura town (CPCB 2012; Prakash and Somashekar 2006; Singh et al. 2009).
In this study, seasonal variations of physico-chemical and bacteriological characteristics of water quality in Tunga river was assessed in Shimoga town in Karnataka. A. Study Area. Shimoga is town, situated between the North and South branches of river Tunga. It is located on the Bangalore - Honnavar highway.Though it is a town of medium ...
The causes of the drying are poorly understood, resulting in misdirected or counter-productive management responses. Five plausible hypotheses that could explain the decline were tested using data from field surveys and secondary sources: (1) changes in rainfall amount, seasonality and intensity; (2) increases in temperature; (3) groundwater ...
"Correlation Study for Assessment of Water Quality and Its Parameters of Par River Valsad, Gujarat, India", International Journal of Innovative and Emerging Research in Engineering, 150-156. [5] Leslie Danquah, (2010) "The Causes and Health Effects Of River Pollution- A Case Study of Aboabo River Kumasi".