Have a language expert improve your writing
Run a free plagiarism check in 10 minutes, generate accurate citations for free.
- Knowledge Base
Methodology
- Random Assignment in Experiments | Introduction & Examples
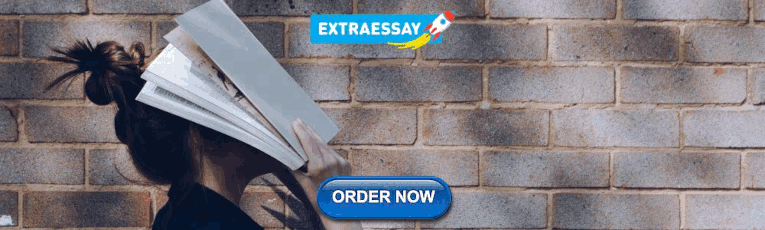
Random Assignment in Experiments | Introduction & Examples
Published on March 8, 2021 by Pritha Bhandari . Revised on June 22, 2023.
In experimental research, random assignment is a way of placing participants from your sample into different treatment groups using randomization.
With simple random assignment, every member of the sample has a known or equal chance of being placed in a control group or an experimental group. Studies that use simple random assignment are also called completely randomized designs .
Random assignment is a key part of experimental design . It helps you ensure that all groups are comparable at the start of a study: any differences between them are due to random factors, not research biases like sampling bias or selection bias .
Table of contents
Why does random assignment matter, random sampling vs random assignment, how do you use random assignment, when is random assignment not used, other interesting articles, frequently asked questions about random assignment.
Random assignment is an important part of control in experimental research, because it helps strengthen the internal validity of an experiment and avoid biases.
In experiments, researchers manipulate an independent variable to assess its effect on a dependent variable, while controlling for other variables. To do so, they often use different levels of an independent variable for different groups of participants.
This is called a between-groups or independent measures design.
You use three groups of participants that are each given a different level of the independent variable:
- a control group that’s given a placebo (no dosage, to control for a placebo effect ),
- an experimental group that’s given a low dosage,
- a second experimental group that’s given a high dosage.
Random assignment to helps you make sure that the treatment groups don’t differ in systematic ways at the start of the experiment, as this can seriously affect (and even invalidate) your work.
If you don’t use random assignment, you may not be able to rule out alternative explanations for your results.
- participants recruited from cafes are placed in the control group ,
- participants recruited from local community centers are placed in the low dosage experimental group,
- participants recruited from gyms are placed in the high dosage group.
With this type of assignment, it’s hard to tell whether the participant characteristics are the same across all groups at the start of the study. Gym-users may tend to engage in more healthy behaviors than people who frequent cafes or community centers, and this would introduce a healthy user bias in your study.
Although random assignment helps even out baseline differences between groups, it doesn’t always make them completely equivalent. There may still be extraneous variables that differ between groups, and there will always be some group differences that arise from chance.
Most of the time, the random variation between groups is low, and, therefore, it’s acceptable for further analysis. This is especially true when you have a large sample. In general, you should always use random assignment in experiments when it is ethically possible and makes sense for your study topic.
Here's why students love Scribbr's proofreading services
Discover proofreading & editing
Random sampling and random assignment are both important concepts in research, but it’s important to understand the difference between them.
Random sampling (also called probability sampling or random selection) is a way of selecting members of a population to be included in your study. In contrast, random assignment is a way of sorting the sample participants into control and experimental groups.
While random sampling is used in many types of studies, random assignment is only used in between-subjects experimental designs.
Some studies use both random sampling and random assignment, while others use only one or the other.
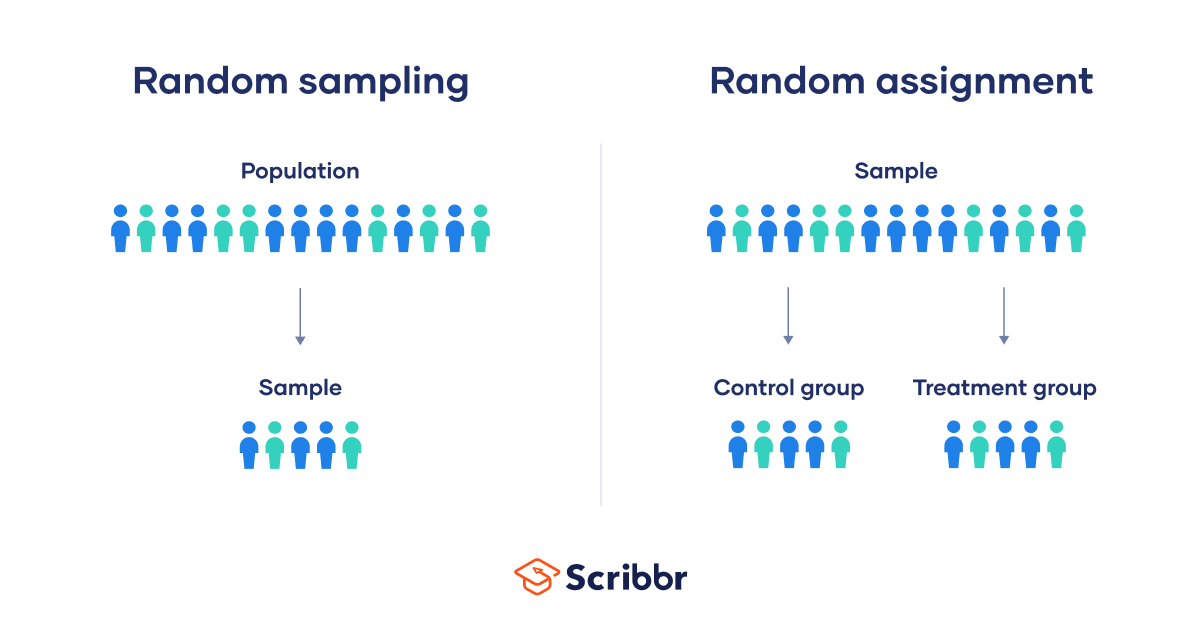
Random sampling enhances the external validity or generalizability of your results, because it helps ensure that your sample is unbiased and representative of the whole population. This allows you to make stronger statistical inferences .
You use a simple random sample to collect data. Because you have access to the whole population (all employees), you can assign all 8000 employees a number and use a random number generator to select 300 employees. These 300 employees are your full sample.
Random assignment enhances the internal validity of the study, because it ensures that there are no systematic differences between the participants in each group. This helps you conclude that the outcomes can be attributed to the independent variable .
- a control group that receives no intervention.
- an experimental group that has a remote team-building intervention every week for a month.
You use random assignment to place participants into the control or experimental group. To do so, you take your list of participants and assign each participant a number. Again, you use a random number generator to place each participant in one of the two groups.
To use simple random assignment, you start by giving every member of the sample a unique number. Then, you can use computer programs or manual methods to randomly assign each participant to a group.
- Random number generator: Use a computer program to generate random numbers from the list for each group.
- Lottery method: Place all numbers individually in a hat or a bucket, and draw numbers at random for each group.
- Flip a coin: When you only have two groups, for each number on the list, flip a coin to decide if they’ll be in the control or the experimental group.
- Use a dice: When you have three groups, for each number on the list, roll a dice to decide which of the groups they will be in. For example, assume that rolling 1 or 2 lands them in a control group; 3 or 4 in an experimental group; and 5 or 6 in a second control or experimental group.
This type of random assignment is the most powerful method of placing participants in conditions, because each individual has an equal chance of being placed in any one of your treatment groups.
Random assignment in block designs
In more complicated experimental designs, random assignment is only used after participants are grouped into blocks based on some characteristic (e.g., test score or demographic variable). These groupings mean that you need a larger sample to achieve high statistical power .
For example, a randomized block design involves placing participants into blocks based on a shared characteristic (e.g., college students versus graduates), and then using random assignment within each block to assign participants to every treatment condition. This helps you assess whether the characteristic affects the outcomes of your treatment.
In an experimental matched design , you use blocking and then match up individual participants from each block based on specific characteristics. Within each matched pair or group, you randomly assign each participant to one of the conditions in the experiment and compare their outcomes.
Sometimes, it’s not relevant or ethical to use simple random assignment, so groups are assigned in a different way.
When comparing different groups
Sometimes, differences between participants are the main focus of a study, for example, when comparing men and women or people with and without health conditions. Participants are not randomly assigned to different groups, but instead assigned based on their characteristics.
In this type of study, the characteristic of interest (e.g., gender) is an independent variable, and the groups differ based on the different levels (e.g., men, women, etc.). All participants are tested the same way, and then their group-level outcomes are compared.
When it’s not ethically permissible
When studying unhealthy or dangerous behaviors, it’s not possible to use random assignment. For example, if you’re studying heavy drinkers and social drinkers, it’s unethical to randomly assign participants to one of the two groups and ask them to drink large amounts of alcohol for your experiment.
When you can’t assign participants to groups, you can also conduct a quasi-experimental study . In a quasi-experiment, you study the outcomes of pre-existing groups who receive treatments that you may not have any control over (e.g., heavy drinkers and social drinkers). These groups aren’t randomly assigned, but may be considered comparable when some other variables (e.g., age or socioeconomic status) are controlled for.
Prevent plagiarism. Run a free check.
If you want to know more about statistics , methodology , or research bias , make sure to check out some of our other articles with explanations and examples.
- Student’s t -distribution
- Normal distribution
- Null and Alternative Hypotheses
- Chi square tests
- Confidence interval
- Quartiles & Quantiles
- Cluster sampling
- Stratified sampling
- Data cleansing
- Reproducibility vs Replicability
- Peer review
- Prospective cohort study
Research bias
- Implicit bias
- Cognitive bias
- Placebo effect
- Hawthorne effect
- Hindsight bias
- Affect heuristic
- Social desirability bias
In experimental research, random assignment is a way of placing participants from your sample into different groups using randomization. With this method, every member of the sample has a known or equal chance of being placed in a control group or an experimental group.
Random selection, or random sampling , is a way of selecting members of a population for your study’s sample.
In contrast, random assignment is a way of sorting the sample into control and experimental groups.
Random sampling enhances the external validity or generalizability of your results, while random assignment improves the internal validity of your study.
Random assignment is used in experiments with a between-groups or independent measures design. In this research design, there’s usually a control group and one or more experimental groups. Random assignment helps ensure that the groups are comparable.
In general, you should always use random assignment in this type of experimental design when it is ethically possible and makes sense for your study topic.
To implement random assignment , assign a unique number to every member of your study’s sample .
Then, you can use a random number generator or a lottery method to randomly assign each number to a control or experimental group. You can also do so manually, by flipping a coin or rolling a dice to randomly assign participants to groups.
Cite this Scribbr article
If you want to cite this source, you can copy and paste the citation or click the “Cite this Scribbr article” button to automatically add the citation to our free Citation Generator.
Bhandari, P. (2023, June 22). Random Assignment in Experiments | Introduction & Examples. Scribbr. Retrieved April 15, 2024, from https://www.scribbr.com/methodology/random-assignment/
Is this article helpful?
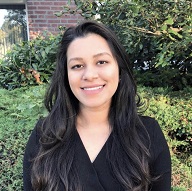
Pritha Bhandari
Other students also liked, guide to experimental design | overview, steps, & examples, confounding variables | definition, examples & controls, control groups and treatment groups | uses & examples, "i thought ai proofreading was useless but..".
I've been using Scribbr for years now and I know it's a service that won't disappoint. It does a good job spotting mistakes”
Random Assignment in Psychology: Definition & Examples
Julia Simkus
Editor at Simply Psychology
BA (Hons) Psychology, Princeton University
Julia Simkus is a graduate of Princeton University with a Bachelor of Arts in Psychology. She is currently studying for a Master's Degree in Counseling for Mental Health and Wellness in September 2023. Julia's research has been published in peer reviewed journals.
Learn about our Editorial Process
Saul Mcleod, PhD
Editor-in-Chief for Simply Psychology
BSc (Hons) Psychology, MRes, PhD, University of Manchester
Saul Mcleod, PhD., is a qualified psychology teacher with over 18 years of experience in further and higher education. He has been published in peer-reviewed journals, including the Journal of Clinical Psychology.
Olivia Guy-Evans, MSc
Associate Editor for Simply Psychology
BSc (Hons) Psychology, MSc Psychology of Education
Olivia Guy-Evans is a writer and associate editor for Simply Psychology. She has previously worked in healthcare and educational sectors.
In psychology, random assignment refers to the practice of allocating participants to different experimental groups in a study in a completely unbiased way, ensuring each participant has an equal chance of being assigned to any group.
In experimental research, random assignment, or random placement, organizes participants from your sample into different groups using randomization.
Random assignment uses chance procedures to ensure that each participant has an equal opportunity of being assigned to either a control or experimental group.
The control group does not receive the treatment in question, whereas the experimental group does receive the treatment.
When using random assignment, neither the researcher nor the participant can choose the group to which the participant is assigned. This ensures that any differences between and within the groups are not systematic at the onset of the study.
In a study to test the success of a weight-loss program, investigators randomly assigned a pool of participants to one of two groups.
Group A participants participated in the weight-loss program for 10 weeks and took a class where they learned about the benefits of healthy eating and exercise.
Group B participants read a 200-page book that explains the benefits of weight loss. The investigator randomly assigned participants to one of the two groups.
The researchers found that those who participated in the program and took the class were more likely to lose weight than those in the other group that received only the book.
Importance
Random assignment ensures that each group in the experiment is identical before applying the independent variable.
In experiments , researchers will manipulate an independent variable to assess its effect on a dependent variable, while controlling for other variables. Random assignment increases the likelihood that the treatment groups are the same at the onset of a study.
Thus, any changes that result from the independent variable can be assumed to be a result of the treatment of interest. This is particularly important for eliminating sources of bias and strengthening the internal validity of an experiment.
Random assignment is the best method for inferring a causal relationship between a treatment and an outcome.
Random Selection vs. Random Assignment
Random selection (also called probability sampling or random sampling) is a way of randomly selecting members of a population to be included in your study.
On the other hand, random assignment is a way of sorting the sample participants into control and treatment groups.
Random selection ensures that everyone in the population has an equal chance of being selected for the study. Once the pool of participants has been chosen, experimenters use random assignment to assign participants into groups.
Random assignment is only used in between-subjects experimental designs, while random selection can be used in a variety of study designs.
Random Assignment vs Random Sampling
Random sampling refers to selecting participants from a population so that each individual has an equal chance of being chosen. This method enhances the representativeness of the sample.
Random assignment, on the other hand, is used in experimental designs once participants are selected. It involves allocating these participants to different experimental groups or conditions randomly.
This helps ensure that any differences in results across groups are due to manipulating the independent variable, not preexisting differences among participants.
When to Use Random Assignment
Random assignment is used in experiments with a between-groups or independent measures design.
In these research designs, researchers will manipulate an independent variable to assess its effect on a dependent variable, while controlling for other variables.
There is usually a control group and one or more experimental groups. Random assignment helps ensure that the groups are comparable at the onset of the study.
How to Use Random Assignment
There are a variety of ways to assign participants into study groups randomly. Here are a handful of popular methods:
- Random Number Generator : Give each member of the sample a unique number; use a computer program to randomly generate a number from the list for each group.
- Lottery : Give each member of the sample a unique number. Place all numbers in a hat or bucket and draw numbers at random for each group.
- Flipping a Coin : Flip a coin for each participant to decide if they will be in the control group or experimental group (this method can only be used when you have just two groups)
- Roll a Die : For each number on the list, roll a dice to decide which of the groups they will be in. For example, assume that rolling 1, 2, or 3 places them in a control group and rolling 3, 4, 5 lands them in an experimental group.
When is Random Assignment not used?
- When it is not ethically permissible: Randomization is only ethical if the researcher has no evidence that one treatment is superior to the other or that one treatment might have harmful side effects.
- When answering non-causal questions : If the researcher is just interested in predicting the probability of an event, the causal relationship between the variables is not important and observational designs would be more suitable than random assignment.
- When studying the effect of variables that cannot be manipulated: Some risk factors cannot be manipulated and so it would not make any sense to study them in a randomized trial. For example, we cannot randomly assign participants into categories based on age, gender, or genetic factors.
Drawbacks of Random Assignment
While randomization assures an unbiased assignment of participants to groups, it does not guarantee the equality of these groups. There could still be extraneous variables that differ between groups or group differences that arise from chance. Additionally, there is still an element of luck with random assignments.
Thus, researchers can not produce perfectly equal groups for each specific study. Differences between the treatment group and control group might still exist, and the results of a randomized trial may sometimes be wrong, but this is absolutely okay.
Scientific evidence is a long and continuous process, and the groups will tend to be equal in the long run when data is aggregated in a meta-analysis.
Additionally, external validity (i.e., the extent to which the researcher can use the results of the study to generalize to the larger population) is compromised with random assignment.
Random assignment is challenging to implement outside of controlled laboratory conditions and might not represent what would happen in the real world at the population level.
Random assignment can also be more costly than simple observational studies, where an investigator is just observing events without intervening with the population.
Randomization also can be time-consuming and challenging, especially when participants refuse to receive the assigned treatment or do not adhere to recommendations.
What is the difference between random sampling and random assignment?
Random sampling refers to randomly selecting a sample of participants from a population. Random assignment refers to randomly assigning participants to treatment groups from the selected sample.
Does random assignment increase internal validity?
Yes, random assignment ensures that there are no systematic differences between the participants in each group, enhancing the study’s internal validity .
Does random assignment reduce sampling error?
Yes, with random assignment, participants have an equal chance of being assigned to either a control group or an experimental group, resulting in a sample that is, in theory, representative of the population.
Random assignment does not completely eliminate sampling error because a sample only approximates the population from which it is drawn. However, random sampling is a way to minimize sampling errors.
When is random assignment not possible?
Random assignment is not possible when the experimenters cannot control the treatment or independent variable.
For example, if you want to compare how men and women perform on a test, you cannot randomly assign subjects to these groups.
Participants are not randomly assigned to different groups in this study, but instead assigned based on their characteristics.
Does random assignment eliminate confounding variables?
Yes, random assignment eliminates the influence of any confounding variables on the treatment because it distributes them at random among the study groups. Randomization invalidates any relationship between a confounding variable and the treatment.
Why is random assignment of participants to treatment conditions in an experiment used?
Random assignment is used to ensure that all groups are comparable at the start of a study. This allows researchers to conclude that the outcomes of the study can be attributed to the intervention at hand and to rule out alternative explanations for study results.
Further Reading
- Bogomolnaia, A., & Moulin, H. (2001). A new solution to the random assignment problem . Journal of Economic theory , 100 (2), 295-328.
- Krause, M. S., & Howard, K. I. (2003). What random assignment does and does not do . Journal of Clinical Psychology , 59 (7), 751-766.

- Bipolar Disorder
- Therapy Center
- When To See a Therapist
- Types of Therapy
- Best Online Therapy
- Best Couples Therapy
- Best Family Therapy
- Managing Stress
- Sleep and Dreaming
- Understanding Emotions
- Self-Improvement
- Healthy Relationships
- Student Resources
- Personality Types
- Guided Meditations
- Verywell Mind Insights
- 2023 Verywell Mind 25
- Mental Health in the Classroom
- Editorial Process
- Meet Our Review Board
- Crisis Support
The Definition of Random Assignment According to Psychology
Kendra Cherry, MS, is a psychosocial rehabilitation specialist, psychology educator, and author of the "Everything Psychology Book."
:max_bytes(150000):strip_icc():format(webp)/IMG_9791-89504ab694d54b66bbd72cb84ffb860e.jpg)
Emily is a board-certified science editor who has worked with top digital publishing brands like Voices for Biodiversity, Study.com, GoodTherapy, Vox, and Verywell.
:max_bytes(150000):strip_icc():format(webp)/Emily-Swaim-1000-0f3197de18f74329aeffb690a177160c.jpg)
Materio / Getty Images
Random assignment refers to the use of chance procedures in psychology experiments to ensure that each participant has the same opportunity to be assigned to any given group in a study to eliminate any potential bias in the experiment at the outset. Participants are randomly assigned to different groups, such as the treatment group versus the control group. In clinical research, randomized clinical trials are known as the gold standard for meaningful results.
Simple random assignment techniques might involve tactics such as flipping a coin, drawing names out of a hat, rolling dice, or assigning random numbers to a list of participants. It is important to note that random assignment differs from random selection .
While random selection refers to how participants are randomly chosen from a target population as representatives of that population, random assignment refers to how those chosen participants are then assigned to experimental groups.
Random Assignment In Research
To determine if changes in one variable will cause changes in another variable, psychologists must perform an experiment. Random assignment is a critical part of the experimental design that helps ensure the reliability of the study outcomes.
Researchers often begin by forming a testable hypothesis predicting that one variable of interest will have some predictable impact on another variable.
The variable that the experimenters will manipulate in the experiment is known as the independent variable , while the variable that they will then measure for different outcomes is known as the dependent variable. While there are different ways to look at relationships between variables, an experiment is the best way to get a clear idea if there is a cause-and-effect relationship between two or more variables.
Once researchers have formulated a hypothesis, conducted background research, and chosen an experimental design, it is time to find participants for their experiment. How exactly do researchers decide who will be part of an experiment? As mentioned previously, this is often accomplished through something known as random selection.
Random Selection
In order to generalize the results of an experiment to a larger group, it is important to choose a sample that is representative of the qualities found in that population. For example, if the total population is 60% female and 40% male, then the sample should reflect those same percentages.
Choosing a representative sample is often accomplished by randomly picking people from the population to be participants in a study. Random selection means that everyone in the group stands an equal chance of being chosen to minimize any bias. Once a pool of participants has been selected, it is time to assign them to groups.
By randomly assigning the participants into groups, the experimenters can be fairly sure that each group will have the same characteristics before the independent variable is applied.
Participants might be randomly assigned to the control group , which does not receive the treatment in question. The control group may receive a placebo or receive the standard treatment. Participants may also be randomly assigned to the experimental group , which receives the treatment of interest. In larger studies, there can be multiple treatment groups for comparison.
There are simple methods of random assignment, like rolling the die. However, there are more complex techniques that involve random number generators to remove any human error.
There can also be random assignment to groups with pre-established rules or parameters. For example, if you want to have an equal number of men and women in each of your study groups, you might separate your sample into two groups (by sex) before randomly assigning each of those groups into the treatment group and control group.
Random assignment is essential because it increases the likelihood that the groups are the same at the outset. With all characteristics being equal between groups, other than the application of the independent variable, any differences found between group outcomes can be more confidently attributed to the effect of the intervention.
Example of Random Assignment
Imagine that a researcher is interested in learning whether or not drinking caffeinated beverages prior to an exam will improve test performance. After randomly selecting a pool of participants, each person is randomly assigned to either the control group or the experimental group.
The participants in the control group consume a placebo drink prior to the exam that does not contain any caffeine. Those in the experimental group, on the other hand, consume a caffeinated beverage before taking the test.
Participants in both groups then take the test, and the researcher compares the results to determine if the caffeinated beverage had any impact on test performance.
A Word From Verywell
Random assignment plays an important role in the psychology research process. Not only does this process help eliminate possible sources of bias, but it also makes it easier to generalize the results of a tested sample of participants to a larger population.
Random assignment helps ensure that members of each group in the experiment are the same, which means that the groups are also likely more representative of what is present in the larger population of interest. Through the use of this technique, psychology researchers are able to study complex phenomena and contribute to our understanding of the human mind and behavior.
Lin Y, Zhu M, Su Z. The pursuit of balance: An overview of covariate-adaptive randomization techniques in clinical trials . Contemp Clin Trials. 2015;45(Pt A):21-25. doi:10.1016/j.cct.2015.07.011
Sullivan L. Random assignment versus random selection . In: The SAGE Glossary of the Social and Behavioral Sciences. SAGE Publications, Inc.; 2009. doi:10.4135/9781412972024.n2108
Alferes VR. Methods of Randomization in Experimental Design . SAGE Publications, Inc.; 2012. doi:10.4135/9781452270012
Nestor PG, Schutt RK. Research Methods in Psychology: Investigating Human Behavior. (2nd Ed.). SAGE Publications, Inc.; 2015.
By Kendra Cherry, MSEd Kendra Cherry, MS, is a psychosocial rehabilitation specialist, psychology educator, and author of the "Everything Psychology Book."
Random Assignment in Psychology (Definition + 40 Examples)

Have you ever wondered how researchers discover new ways to help people learn, make decisions, or overcome challenges? A hidden hero in this adventure of discovery is a method called random assignment, a cornerstone in psychological research that helps scientists uncover the truths about the human mind and behavior.
Random Assignment is a process used in research where each participant has an equal chance of being placed in any group within the study. This technique is essential in experiments as it helps to eliminate biases, ensuring that the different groups being compared are similar in all important aspects.
By doing so, researchers can be confident that any differences observed are likely due to the variable being tested, rather than other factors.
In this article, we’ll explore the intriguing world of random assignment, diving into its history, principles, real-world examples, and the impact it has had on the field of psychology.
History of Random Assignment
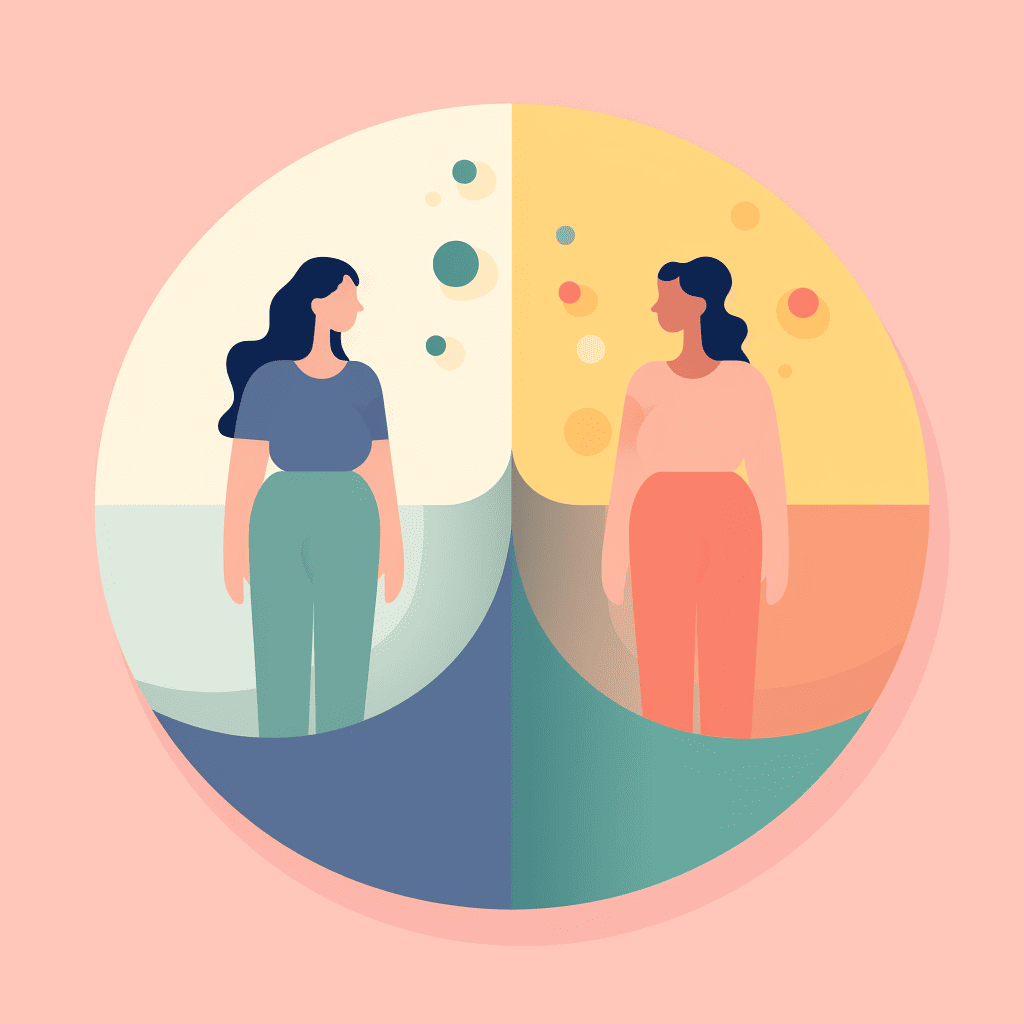
Stepping back in time, we delve into the origins of random assignment, which finds its roots in the early 20th century.
The pioneering mind behind this innovative technique was Sir Ronald A. Fisher , a British statistician and biologist. Fisher introduced the concept of random assignment in the 1920s, aiming to improve the quality and reliability of experimental research .
His contributions laid the groundwork for the method's evolution and its widespread adoption in various fields, particularly in psychology.
Fisher’s groundbreaking work on random assignment was motivated by his desire to control for confounding variables – those pesky factors that could muddy the waters of research findings.
By assigning participants to different groups purely by chance, he realized that the influence of these confounding variables could be minimized, paving the way for more accurate and trustworthy results.
Early Studies Utilizing Random Assignment
Following Fisher's initial development, random assignment started to gain traction in the research community. Early studies adopting this methodology focused on a variety of topics, from agriculture (which was Fisher’s primary field of interest) to medicine and psychology.
The approach allowed researchers to draw stronger conclusions from their experiments, bolstering the development of new theories and practices.
One notable early study utilizing random assignment was conducted in the field of educational psychology. Researchers were keen to understand the impact of different teaching methods on student outcomes.
By randomly assigning students to various instructional approaches, they were able to isolate the effects of the teaching methods, leading to valuable insights and recommendations for educators.
Evolution of the Methodology
As the decades rolled on, random assignment continued to evolve and adapt to the changing landscape of research.
Advances in technology introduced new tools and techniques for implementing randomization, such as computerized random number generators, which offered greater precision and ease of use.
The application of random assignment expanded beyond the confines of the laboratory, finding its way into field studies and large-scale surveys.
Researchers across diverse disciplines embraced the methodology, recognizing its potential to enhance the validity of their findings and contribute to the advancement of knowledge.
From its humble beginnings in the early 20th century to its widespread use today, random assignment has proven to be a cornerstone of scientific inquiry.
Its development and evolution have played a pivotal role in shaping the landscape of psychological research, driving discoveries that have improved lives and deepened our understanding of the human experience.
Principles of Random Assignment
Delving into the heart of random assignment, we uncover the theories and principles that form its foundation.
The method is steeped in the basics of probability theory and statistical inference, ensuring that each participant has an equal chance of being placed in any group, thus fostering fair and unbiased results.
Basic Principles of Random Assignment
Understanding the core principles of random assignment is key to grasping its significance in research. There are three principles: equal probability of selection, reduction of bias, and ensuring representativeness.
The first principle, equal probability of selection , ensures that every participant has an identical chance of being assigned to any group in the study. This randomness is crucial as it mitigates the risk of bias and establishes a level playing field.
The second principle focuses on the reduction of bias . Random assignment acts as a safeguard, ensuring that the groups being compared are alike in all essential aspects before the experiment begins.
This similarity between groups allows researchers to attribute any differences observed in the outcomes directly to the independent variable being studied.
Lastly, ensuring representativeness is a vital principle. When participants are assigned randomly, the resulting groups are more likely to be representative of the larger population.
This characteristic is crucial for the generalizability of the study’s findings, allowing researchers to apply their insights broadly.
Theoretical Foundation
The theoretical foundation of random assignment lies in probability theory and statistical inference .
Probability theory deals with the likelihood of different outcomes, providing a mathematical framework for analyzing random phenomena. In the context of random assignment, it helps in ensuring that each participant has an equal chance of being placed in any group.
Statistical inference, on the other hand, allows researchers to draw conclusions about a population based on a sample of data drawn from that population. It is the mechanism through which the results of a study can be generalized to a broader context.
Random assignment enhances the reliability of statistical inferences by reducing biases and ensuring that the sample is representative.
Differentiating Random Assignment from Random Selection
It’s essential to distinguish between random assignment and random selection, as the two terms, while related, have distinct meanings in the realm of research.
Random assignment refers to how participants are placed into different groups in an experiment, aiming to control for confounding variables and help determine causes.
In contrast, random selection pertains to how individuals are chosen to participate in a study. This method is used to ensure that the sample of participants is representative of the larger population, which is vital for the external validity of the research.
While both methods are rooted in randomness and probability, they serve different purposes in the research process.
Understanding the theories, principles, and distinctions of random assignment illuminates its pivotal role in psychological research.
This method, anchored in probability theory and statistical inference, serves as a beacon of reliability, guiding researchers in their quest for knowledge and ensuring that their findings stand the test of validity and applicability.
Methodology of Random Assignment
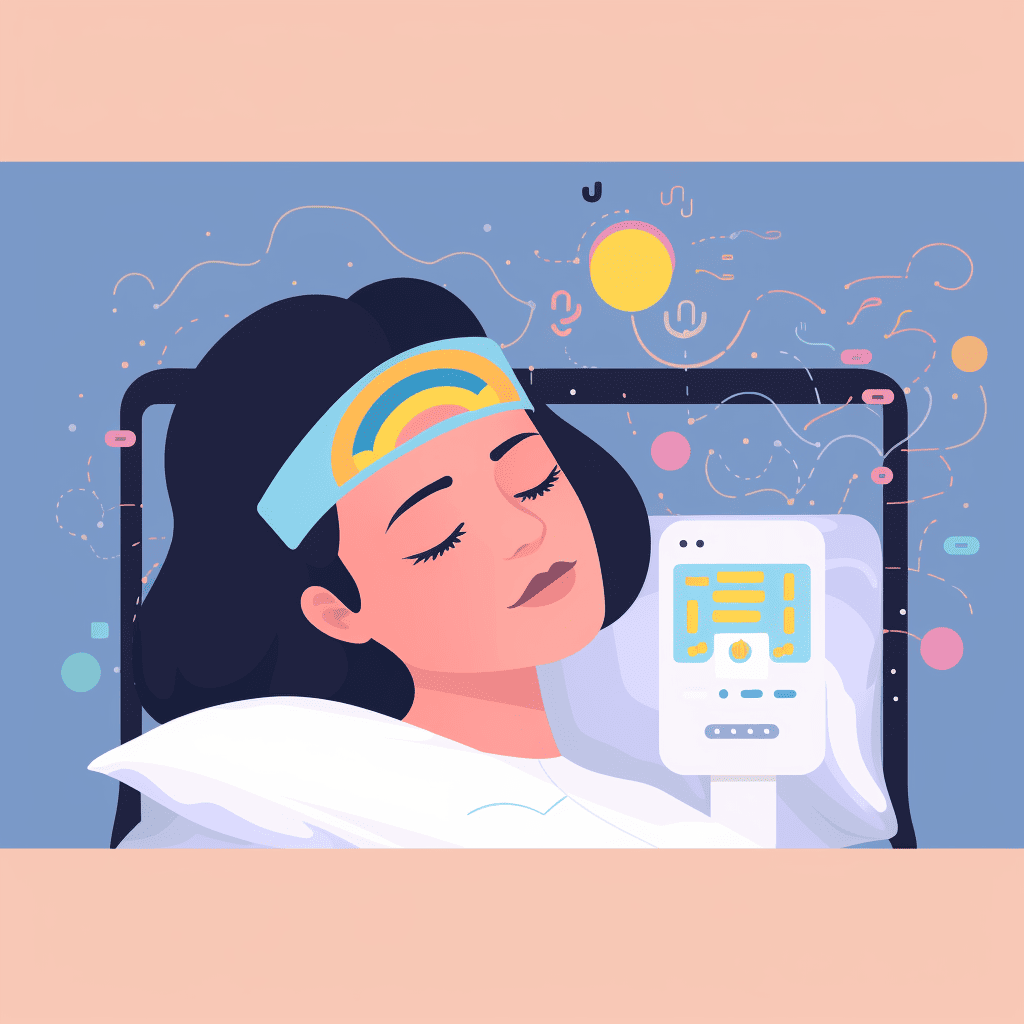
Implementing random assignment in a study is a meticulous process that involves several crucial steps.
The initial step is participant selection, where individuals are chosen to partake in the study. This stage is critical to ensure that the pool of participants is diverse and representative of the population the study aims to generalize to.
Once the pool of participants has been established, the actual assignment process begins. In this step, each participant is allocated randomly to one of the groups in the study.
Researchers use various tools, such as random number generators or computerized methods, to ensure that this assignment is genuinely random and free from biases.
Monitoring and adjusting form the final step in the implementation of random assignment. Researchers need to continuously observe the groups to ensure that they remain comparable in all essential aspects throughout the study.
If any significant discrepancies arise, adjustments might be necessary to maintain the study’s integrity and validity.
Tools and Techniques Used
The evolution of technology has introduced a variety of tools and techniques to facilitate random assignment.
Random number generators, both manual and computerized, are commonly used to assign participants to different groups. These generators ensure that each individual has an equal chance of being placed in any group, upholding the principle of equal probability of selection.
In addition to random number generators, researchers often use specialized computer software designed for statistical analysis and experimental design.
These software programs offer advanced features that allow for precise and efficient random assignment, minimizing the risk of human error and enhancing the study’s reliability.
Ethical Considerations
The implementation of random assignment is not devoid of ethical considerations. Informed consent is a fundamental ethical principle that researchers must uphold.
Informed consent means that every participant should be fully informed about the nature of the study, the procedures involved, and any potential risks or benefits, ensuring that they voluntarily agree to participate.
Beyond informed consent, researchers must conduct a thorough risk and benefit analysis. The potential benefits of the study should outweigh any risks or harms to the participants.
Safeguarding the well-being of participants is paramount, and any study employing random assignment must adhere to established ethical guidelines and standards.
Conclusion of Methodology
The methodology of random assignment, while seemingly straightforward, is a multifaceted process that demands precision, fairness, and ethical integrity. From participant selection to assignment and monitoring, each step is crucial to ensure the validity of the study’s findings.
The tools and techniques employed, coupled with a steadfast commitment to ethical principles, underscore the significance of random assignment as a cornerstone of robust psychological research.
Benefits of Random Assignment in Psychological Research
The impact and importance of random assignment in psychological research cannot be overstated. It is fundamental for ensuring the study is accurate, allowing the researchers to determine if their study actually caused the results they saw, and making sure the findings can be applied to the real world.
Facilitating Causal Inferences
When participants are randomly assigned to different groups, researchers can be more confident that the observed effects are due to the independent variable being changed, and not other factors.
This ability to determine the cause is called causal inference .
This confidence allows for the drawing of causal relationships, which are foundational for theory development and application in psychology.
Ensuring Internal Validity
One of the foremost impacts of random assignment is its ability to enhance the internal validity of an experiment.
Internal validity refers to the extent to which a researcher can assert that changes in the dependent variable are solely due to manipulations of the independent variable , and not due to confounding variables.
By ensuring that each participant has an equal chance of being in any condition of the experiment, random assignment helps control for participant characteristics that could otherwise complicate the results.
Enhancing Generalizability
Beyond internal validity, random assignment also plays a crucial role in enhancing the generalizability of research findings.
When done correctly, it ensures that the sample groups are representative of the larger population, so can allow researchers to apply their findings more broadly.
This representative nature is essential for the practical application of research, impacting policy, interventions, and psychological therapies.
Limitations of Random Assignment
Potential for implementation issues.
While the principles of random assignment are robust, the method can face implementation issues.
One of the most common problems is logistical constraints. Some studies, due to their nature or the specific population being studied, find it challenging to implement random assignment effectively.
For instance, in educational settings, logistical issues such as class schedules and school policies might stop the random allocation of students to different teaching methods .
Ethical Dilemmas
Random assignment, while methodologically sound, can also present ethical dilemmas.
In some cases, withholding a potentially beneficial treatment from one of the groups of participants can raise serious ethical questions, especially in medical or clinical research where participants' well-being might be directly affected.
Researchers must navigate these ethical waters carefully, balancing the pursuit of knowledge with the well-being of participants.
Generalizability Concerns
Even when implemented correctly, random assignment does not always guarantee generalizable results.
The types of people in the participant pool, the specific context of the study, and the nature of the variables being studied can all influence the extent to which the findings can be applied to the broader population.
Researchers must be cautious in making broad generalizations from studies, even those employing strict random assignment.
Practical and Real-World Limitations
In the real world, many variables cannot be manipulated for ethical or practical reasons, limiting the applicability of random assignment.
For instance, researchers cannot randomly assign individuals to different levels of intelligence, socioeconomic status, or cultural backgrounds.
This limitation necessitates the use of other research designs, such as correlational or observational studies , when exploring relationships involving such variables.
Response to Critiques
In response to these critiques, people in favor of random assignment argue that the method, despite its limitations, remains one of the most reliable ways to establish cause and effect in experimental research.
They acknowledge the challenges and ethical considerations but emphasize the rigorous frameworks in place to address them.
The ongoing discussion around the limitations and critiques of random assignment contributes to the evolution of the method, making sure it is continuously relevant and applicable in psychological research.
While random assignment is a powerful tool in experimental research, it is not without its critiques and limitations. Implementation issues, ethical dilemmas, generalizability concerns, and real-world limitations can pose significant challenges.
However, the continued discourse and refinement around these issues underline the method's enduring significance in the pursuit of knowledge in psychology.
By being careful with how we do things and doing what's right, random assignment stays a really important part of studying how people act and think.
Real-World Applications and Examples
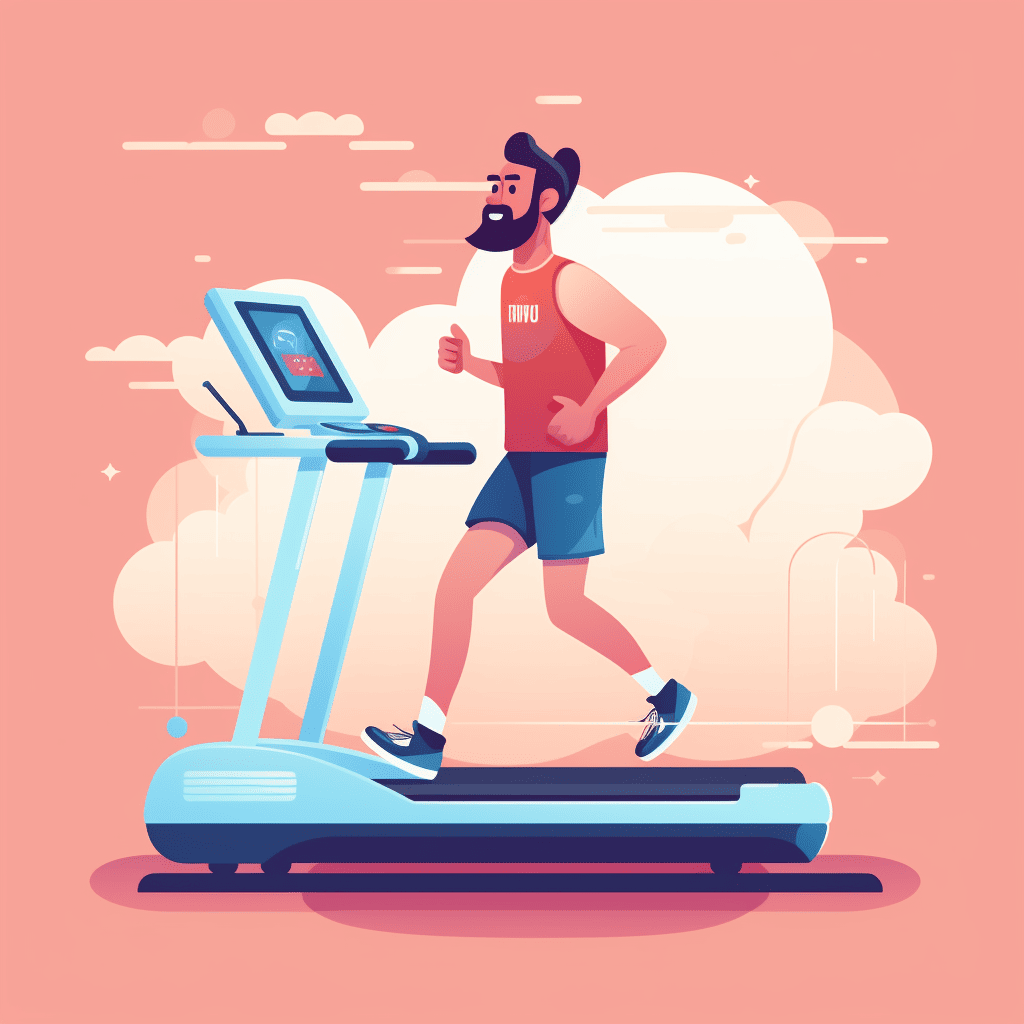
Random assignment has been employed in many studies across various fields of psychology, leading to significant discoveries and advancements.
Here are some real-world applications and examples illustrating the diversity and impact of this method:
- Medicine and Health Psychology: Randomized Controlled Trials (RCTs) are the gold standard in medical research. In these studies, participants are randomly assigned to either the treatment or control group to test the efficacy of new medications or interventions.
- Educational Psychology: Studies in this field have used random assignment to explore the effects of different teaching methods, classroom environments, and educational technologies on student learning and outcomes.
- Cognitive Psychology: Researchers have employed random assignment to investigate various aspects of human cognition, including memory, attention, and problem-solving, leading to a deeper understanding of how the mind works.
- Social Psychology: Random assignment has been instrumental in studying social phenomena, such as conformity, aggression, and prosocial behavior, shedding light on the intricate dynamics of human interaction.
Let's get into some specific examples. You'll need to know one term though, and that is "control group." A control group is a set of participants in a study who do not receive the treatment or intervention being tested , serving as a baseline to compare with the group that does, in order to assess the effectiveness of the treatment.
- Smoking Cessation Study: Researchers used random assignment to put participants into two groups. One group received a new anti-smoking program, while the other did not. This helped determine if the program was effective in helping people quit smoking.
- Math Tutoring Program: A study on students used random assignment to place them into two groups. One group received additional math tutoring, while the other continued with regular classes, to see if the extra help improved their grades.
- Exercise and Mental Health: Adults were randomly assigned to either an exercise group or a control group to study the impact of physical activity on mental health and mood.
- Diet and Weight Loss: A study randomly assigned participants to different diet plans to compare their effectiveness in promoting weight loss and improving health markers.
- Sleep and Learning: Researchers randomly assigned students to either a sleep extension group or a regular sleep group to study the impact of sleep on learning and memory.
- Classroom Seating Arrangement: Teachers used random assignment to place students in different seating arrangements to examine the effect on focus and academic performance.
- Music and Productivity: Employees were randomly assigned to listen to music or work in silence to investigate the effect of music on workplace productivity.
- Medication for ADHD: Children with ADHD were randomly assigned to receive either medication, behavioral therapy, or a placebo to compare treatment effectiveness.
- Mindfulness Meditation for Stress: Adults were randomly assigned to a mindfulness meditation group or a waitlist control group to study the impact on stress levels.
- Video Games and Aggression: A study randomly assigned participants to play either violent or non-violent video games and then measured their aggression levels.
- Online Learning Platforms: Students were randomly assigned to use different online learning platforms to evaluate their effectiveness in enhancing learning outcomes.
- Hand Sanitizers in Schools: Schools were randomly assigned to use hand sanitizers or not to study the impact on student illness and absenteeism.
- Caffeine and Alertness: Participants were randomly assigned to consume caffeinated or decaffeinated beverages to measure the effects on alertness and cognitive performance.
- Green Spaces and Well-being: Neighborhoods were randomly assigned to receive green space interventions to study the impact on residents’ well-being and community connections.
- Pet Therapy for Hospital Patients: Patients were randomly assigned to receive pet therapy or standard care to assess the impact on recovery and mood.
- Yoga for Chronic Pain: Individuals with chronic pain were randomly assigned to a yoga intervention group or a control group to study the effect on pain levels and quality of life.
- Flu Vaccines Effectiveness: Different groups of people were randomly assigned to receive either the flu vaccine or a placebo to determine the vaccine’s effectiveness.
- Reading Strategies for Dyslexia: Children with dyslexia were randomly assigned to different reading intervention strategies to compare their effectiveness.
- Physical Environment and Creativity: Participants were randomly assigned to different room setups to study the impact of physical environment on creative thinking.
- Laughter Therapy for Depression: Individuals with depression were randomly assigned to laughter therapy sessions or control groups to assess the impact on mood.
- Financial Incentives for Exercise: Participants were randomly assigned to receive financial incentives for exercising to study the impact on physical activity levels.
- Art Therapy for Anxiety: Individuals with anxiety were randomly assigned to art therapy sessions or a waitlist control group to measure the effect on anxiety levels.
- Natural Light in Offices: Employees were randomly assigned to workspaces with natural or artificial light to study the impact on productivity and job satisfaction.
- School Start Times and Academic Performance: Schools were randomly assigned different start times to study the effect on student academic performance and well-being.
- Horticulture Therapy for Seniors: Older adults were randomly assigned to participate in horticulture therapy or traditional activities to study the impact on cognitive function and life satisfaction.
- Hydration and Cognitive Function: Participants were randomly assigned to different hydration levels to measure the impact on cognitive function and alertness.
- Intergenerational Programs: Seniors and young people were randomly assigned to intergenerational programs to study the effects on well-being and cross-generational understanding.
- Therapeutic Horseback Riding for Autism: Children with autism were randomly assigned to therapeutic horseback riding or traditional therapy to study the impact on social communication skills.
- Active Commuting and Health: Employees were randomly assigned to active commuting (cycling, walking) or passive commuting to study the effect on physical health.
- Mindful Eating for Weight Management: Individuals were randomly assigned to mindful eating workshops or control groups to study the impact on weight management and eating habits.
- Noise Levels and Learning: Students were randomly assigned to classrooms with different noise levels to study the effect on learning and concentration.
- Bilingual Education Methods: Schools were randomly assigned different bilingual education methods to compare their effectiveness in language acquisition.
- Outdoor Play and Child Development: Children were randomly assigned to different amounts of outdoor playtime to study the impact on physical and cognitive development.
- Social Media Detox: Participants were randomly assigned to a social media detox or regular usage to study the impact on mental health and well-being.
- Therapeutic Writing for Trauma Survivors: Individuals who experienced trauma were randomly assigned to therapeutic writing sessions or control groups to study the impact on psychological well-being.
- Mentoring Programs for At-risk Youth: At-risk youth were randomly assigned to mentoring programs or control groups to assess the impact on academic achievement and behavior.
- Dance Therapy for Parkinson’s Disease: Individuals with Parkinson’s disease were randomly assigned to dance therapy or traditional exercise to study the effect on motor function and quality of life.
- Aquaponics in Schools: Schools were randomly assigned to implement aquaponics programs to study the impact on student engagement and environmental awareness.
- Virtual Reality for Phobia Treatment: Individuals with phobias were randomly assigned to virtual reality exposure therapy or traditional therapy to compare effectiveness.
- Gardening and Mental Health: Participants were randomly assigned to engage in gardening or other leisure activities to study the impact on mental health and stress reduction.
Each of these studies exemplifies how random assignment is utilized in various fields and settings, shedding light on the multitude of ways it can be applied to glean valuable insights and knowledge.
Real-world Impact of Random Assignment
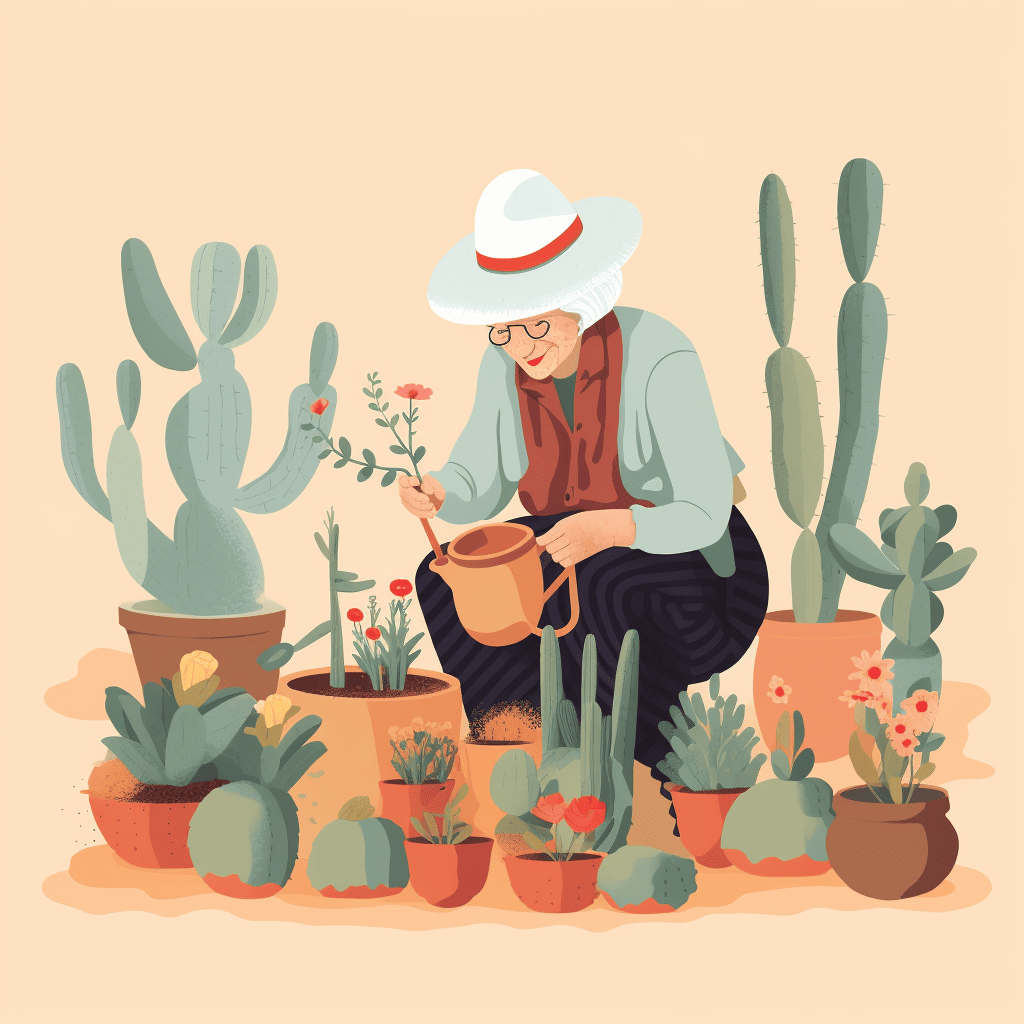
Random assignment is like a key tool in the world of learning about people's minds and behaviors. It’s super important and helps in many different areas of our everyday lives. It helps make better rules, creates new ways to help people, and is used in lots of different fields.
Health and Medicine
In health and medicine, random assignment has helped doctors and scientists make lots of discoveries. It’s a big part of tests that help create new medicines and treatments.
By putting people into different groups by chance, scientists can really see if a medicine works.
This has led to new ways to help people with all sorts of health problems, like diabetes, heart disease, and mental health issues like depression and anxiety.
Schools and education have also learned a lot from random assignment. Researchers have used it to look at different ways of teaching, what kind of classrooms are best, and how technology can help learning.
This knowledge has helped make better school rules, develop what we learn in school, and find the best ways to teach students of all ages and backgrounds.
Workplace and Organizational Behavior
Random assignment helps us understand how people act at work and what makes a workplace good or bad.
Studies have looked at different kinds of workplaces, how bosses should act, and how teams should be put together. This has helped companies make better rules and create places to work that are helpful and make people happy.
Environmental and Social Changes
Random assignment is also used to see how changes in the community and environment affect people. Studies have looked at community projects, changes to the environment, and social programs to see how they help or hurt people’s well-being.
This has led to better community projects, efforts to protect the environment, and programs to help people in society.
Technology and Human Interaction
In our world where technology is always changing, studies with random assignment help us see how tech like social media, virtual reality, and online stuff affect how we act and feel.
This has helped make better and safer technology and rules about using it so that everyone can benefit.
The effects of random assignment go far and wide, way beyond just a science lab. It helps us understand lots of different things, leads to new and improved ways to do things, and really makes a difference in the world around us.
From making healthcare and schools better to creating positive changes in communities and the environment, the real-world impact of random assignment shows just how important it is in helping us learn and make the world a better place.
So, what have we learned? Random assignment is like a super tool in learning about how people think and act. It's like a detective helping us find clues and solve mysteries in many parts of our lives.
From creating new medicines to helping kids learn better in school, and from making workplaces happier to protecting the environment, it’s got a big job!
This method isn’t just something scientists use in labs; it reaches out and touches our everyday lives. It helps make positive changes and teaches us valuable lessons.
Whether we are talking about technology, health, education, or the environment, random assignment is there, working behind the scenes, making things better and safer for all of us.
In the end, the simple act of putting people into groups by chance helps us make big discoveries and improvements. It’s like throwing a small stone into a pond and watching the ripples spread out far and wide.
Thanks to random assignment, we are always learning, growing, and finding new ways to make our world a happier and healthier place for everyone!
Related posts:
- 19+ Experimental Design Examples (Methods + Types)
- Cluster Sampling vs Stratified Sampling
- 41+ White Collar Job Examples (Salary + Path)
- 47+ Blue Collar Job Examples (Salary + Path)
- McDonaldization of Society (Definition + Examples)
Reference this article:
About The Author
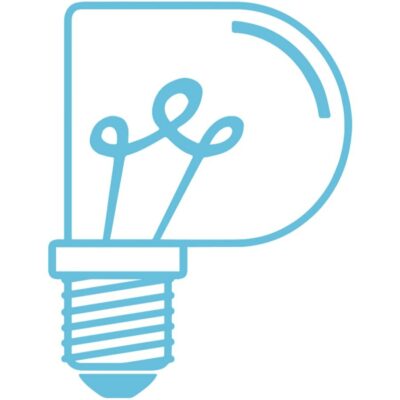
Free Personality Test
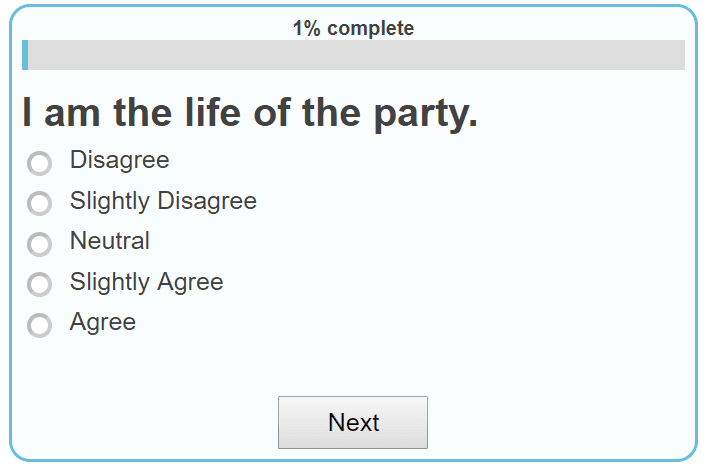
Free Memory Test
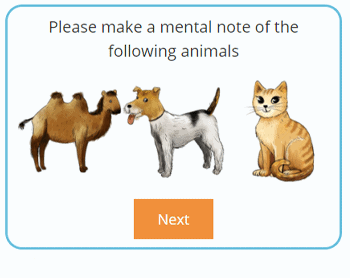
Free IQ Test
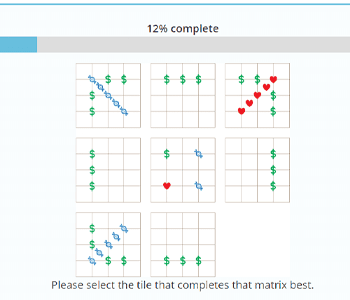
PracticalPie.com is a participant in the Amazon Associates Program. As an Amazon Associate we earn from qualifying purchases.
Follow Us On:
Youtube Facebook Instagram X/Twitter
Psychology Resources
Developmental
Personality
Relationships
Psychologists
Serial Killers
Psychology Tests
Personality Quiz
Memory Test
Depression test
Type A/B Personality Test
© PracticalPsychology. All rights reserved
Privacy Policy | Terms of Use
If you're seeing this message, it means we're having trouble loading external resources on our website.
If you're behind a web filter, please make sure that the domains *.kastatic.org and *.kasandbox.org are unblocked.
To log in and use all the features of Khan Academy, please enable JavaScript in your browser.
AP®︎/College Statistics
Course: ap®︎/college statistics > unit 6.
- Statistical significance of experiment
Random sampling vs. random assignment (scope of inference)
- Conclusions in observational studies versus experiments
- Finding errors in study conclusions
- (Choice A) Just the residents involved in Hilary's study. A Just the residents involved in Hilary's study.
- (Choice B) All residents in Hilary's town. B All residents in Hilary's town.
- (Choice C) All residents in Hilary's country. C All residents in Hilary's country.
- (Choice A) Yes A Yes
- (Choice B) No B No
- (Choice A) Just the residents in Hilary's study. A Just the residents in Hilary's study.
Want to join the conversation?
- Upvote Button navigates to signup page
- Downvote Button navigates to signup page
- Flag Button navigates to signup page

Apply to be a D-Lab Data Science Fellow! Applications are due May 5.
Introduction to Field Experiments and Randomized Controlled Trials

Have you ever been curious about the methods researchers employ to determine causal relationships among various factors, ultimately leading to significant breakthroughs and progress in numerous fields? In this article, we offer an overview of field experimentation and its importance in discerning cause and effect relationships. We outline how randomized experiments represent an unbiased method for determining what works. Furthermore, we discuss key aspects of experiments, such as intervention, excludability, and non-interference. To illustrate these concepts, we present a hypothetical example of a randomized controlled trial evaluating the efficacy of an experimental drug called Covi-Mapp.
Why experiments?
Every day, we find ourselves faced with questions of cause and effect. Understanding the driving forces behind outcomes is crucial, ranging from personal decisions like parenting strategies to organizational challenges such as effective advertising. This blog aims to provide a systematic introduction to experimentation, igniting enthusiasm for primary research and highlighting the myriad of experimental applications and opportunities available.
The challenge for those who seek to answer causal questions convincingly is to develop a research methodology that doesn't require identifying or measuring all potential confounders. Since no planned design can eliminate every possible systematic difference between treatment and control groups, random assignment emerges as a powerful tool for minimizing bias. In the contentious world of causal claims, randomized experiments represent an unbiased method for determining what works. Random assignment means participants are assigned to different groups or conditions in a study purely by chance. Basically, each participant has an equal chance to be assigned to a control group or a treatment group.
Field experiments, or randomized studies conducted in real-world settings, can take many forms. While experiments on college campuses are often considered lab studies, certain experiments on campus – such as those examining club participation – may be regarded as field experiments, depending on the experimental design. Ultimately, whether a study is considered a field experiment hinges on the definition of "the field."
Researchers may employ two main scenarios for randomization. The first involves gathering study participants and randomizing them at the time of the experiment. The second capitalizes on naturally occurring randomizations, such as the Vietnam draft lottery.
Intervention, Excludability, and Non-Interference
Three essential features of any experiment are intervention, excludability, and non-interference. In a general sense, the intervention refers to the treatment or action being tested in an experiment. The excludability principle is satisfied when the only difference between the experimental and control groups is the presence or absence of the intervention. The non-interference principle holds when the outcome of one participant in the study does not influence the outcomes of other participants. Together, these principles ensure that the experiment is designed to provide unbiased and reliable results, isolating the causal effect of the intervention under study.
Omitted Variables and Non-Compliance
To ensure unbiased results, researchers must randomize as much as possible to minimize omitted variable bias. Omitted variables are factors that influence the outcome but are not measured or are difficult to measure. These unmeasured attributes, sometimes called confounding variables or unobserved heterogeneity, must be accounted for to guarantee accurate findings.
Non-compliance can also complicate experiments. One-sided non-compliance occurs when individuals assigned to a treatment group don't receive the treatment (failure to treat), while two-sided non-compliance occurs when some subjects assigned to the treatment group go untreated or individuals assigned to the control group receive the treatment. Addressing these issues at the design level by implementing a blind or double-blind study can help mitigate potential biases.
Achieving Precision through Covariate Balance
To ensure the control and treatment groups are comparatively similar in all relevant aspects, particularly when the sample size (n) is small, it is essential to achieve covariate balance. Covariance measures the association between two variables, while a covariate is a factor that influences the outcome variable. By balancing covariates, we can more accurately isolate the effects of the treatment, leading to improved precision in our findings.
Fictional Example of Randomized Controlled Trial of Covi-Mapp for COVID-19 Management
Let's explore a fictional example to better understand experiments: a one-week randomized controlled trial of the experimental drug Covi-Mapp for managing Covid. In this case, the control group receives the standard care for Covid patients, while the treatment group receives the standard care plus Covi-Mapp. The outcome of interest is whether patients have cough symptoms on day 7, as subsidizing cough symptoms is an encouraging sign in Covid recovery. We'll measure the presence of cough on day 0 and day 7, as well as temperature on day 0 and day 7. Gender is also tracked. The control represents the standard care for COVID-19 patients, while the treatment includes standard care plus the experimental drug.
In this Covi-Mapp example, the intervention is the Covi-Mapp drug, the excludability principle is satisfied if the only difference in patient care between the groups is the drug administration, and the non-interference principle holds if one patient's outcome doesn't affect another's.
First, let's assume we have a dataset containing the relevant information for each patient, including cough status on day 0 and day 7, temperature on day 0 and day 7, treatment assignment, and gender. We'll read the data and explore the dataset:
Simple treatment effect of the experimental drug
Without any covariates, let's first look at the estimated effect of the treatment on the presence of cough on day 7. The estimated proportion of patients with a cough on day 7 for the control group (not receiving the experimental drug) is 0.847458. In other words, about 84.7% of patients in the control group are expected to have a cough on day 7, all else being equal. The estimated effect of the experimental drug on the presence of cough on day 7 is -0.23. This means that, on average, receiving the experimental drug reduces the proportion of patients with a cough on day 7 by 23.8% compared to the control group.
We know that a patient's initial condition would affect the final outcome. If the patient has a cough and a fever on day 0, they might not fare well with the treatment. To better understand the treatment's effect, let's add these covariates:
The output shows the results of a linear regression model, estimating the effect of the experimental drug (treat_covid_mapp) on the presence of cough on day 7, adjusting for cough on day 0 and temperature on day 0. The experimental drug significantly reduces the presence of cough on day 7 by approximately 16.6% compared to the control group (p-value = 0.046242). The presence of cough on day 0 does not significantly predict the presence of cough on day 7 (p-value = 0.717689). A one-unit increase in temperature on day 0 is associated with a 20.6% increase in the presence of cough on day 7, and this effect is statistically significant (p-value = 0.009859).
Should we add day 7 temperature as a covariate? By including it, we might find that the treatment is no longer statistically significant since the temperature on day 7 could be affected by the treatment itself. It is a post-treatment variable, and by including it, the experiment loses value as we used something that was affected by intervention as our covariate.
However, we'd like to investigate if the treatment affects men or women differently. Since we collected gender as part of the study, we could check for Heterogeneous Treatment Effect (HTE) for male vs. female. The experimental drug has a marginally significant effect on the outcome variable for females, reducing it by approximately 23.1% (p-value = 0.05391).
Which group, those coded as male == 0 or male == 1, have better health outcomes (cough) in control? What about in treatment? How does this help to contextualize any heterogeneous treatment effect that might have been estimated?
Stargazer is a popular R package that enables users to create well-formatted tables and reports for statistical analysis results.
Looking at this regression report, we see that males in control have a temperature of 102; females in control have a temperature of 98.6 (which is very nearly a normal temperature). So, in control, males are worse off. In treatment, males have a temperature of 102 - 2.59 = 99.41. While this is closer to a normal temperature, this is still elevated. Females in treatment have a temperature of 98.5 - .32 = 98.18, which is slightly lower than a normal temperature, and is better than an elevated temperature. It appears that the treatment is able to have a stronger effect among male participants than females because males are *more sick* at baseline.
In conclusion, experimentation offers a fascinating and valuable avenue for primary research, allowing us to address causal questions and enhance our understanding of the world around us. Covariate control helps to isolate the causal effect of the treatment on the outcome variable, ensuring that the observed effect is not driven by confounding factors. Proper control of covariates enhances the internal validity of the study and ensures that the estimated treatment effect is an accurate representation of the true causal relationship. By exploring and accounting for sub groups in data, researchers can identify whether the treatment has different effects on different groups, such as men and women or younger and older individuals. This information can be critical for making informed policy decisions and developing targeted interventions that maximize the benefits for specific groups. The ongoing investigation of experimental methodologies and their potential applications represents a compelling and significant area of inquiry.
Gerber, A. S., & Green, D. P. (2012). Field Experiments: Design, Analysis, and Interpretation . W. W. Norton.
“DALL·E 2.” OpenAI , https://openai.com/product/dall-e-2
“Data Science 241. Experiments and Causal Inference.” UC Berkeley School of Information , https://www.ischool.berkeley.edu/courses/datasci/241
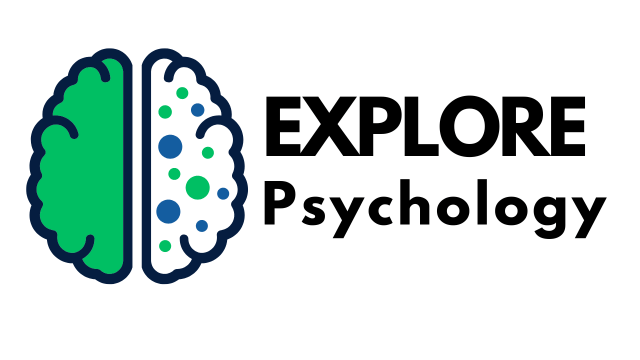
What Is Random Assignment in Psychology?
Categories Research Methods
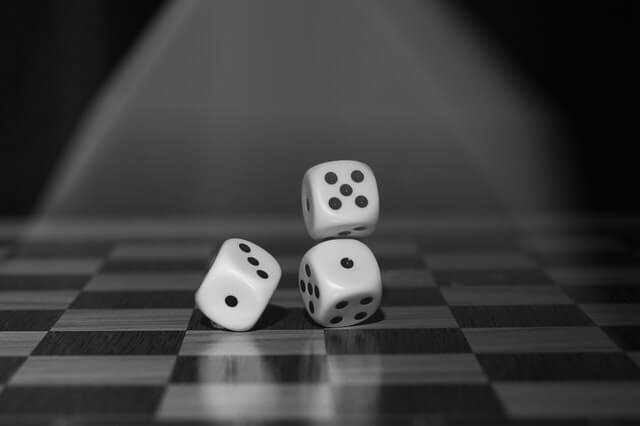
Sharing is caring!
Random assignment means that every participant has the same chance of being chosen for the experimental or control group. It involves using procedures that rely on chance to assign participants to groups. Doing this means that every participant in a study has an equal opportunity to be assigned to any group.
For example, in a psychology experiment, participants might be assigned to either a control or experimental group. Some experiments might only have one experimental group, while others may have several treatment variations.
Using random assignment means that each participant has the same chance of being assigned to any of these groups.
Table of Contents
How to Use Random Assignment
So what type of procedures might psychologists utilize for random assignment? Strategies can include:
- Flipping a coin
- Assigning random numbers
- Rolling dice
- Drawing names out of a hat
How Does Random Assignment Work?
A psychology experiment aims to determine if changes in one variable lead to changes in another variable. Researchers will first begin by coming up with a hypothesis. Once researchers have an idea of what they think they might find in a population, they will come up with an experimental design and then recruit participants for their study.
Once they have a pool of participants representative of the population they are interested in looking at, they will randomly assign the participants to their groups.
- Control group : Some participants will end up in the control group, which serves as a baseline and does not receive the independent variables.
- Experimental group : Other participants will end up in the experimental groups that receive some form of the independent variables.
By using random assignment, the researchers make it more likely that the groups are equal at the start of the experiment. Since the groups are the same on other variables, it can be assumed that any changes that occur are the result of varying the independent variables.
After a treatment has been administered, the researchers will then collect data in order to determine if the independent variable had any impact on the dependent variable.
Random Assignment vs. Random Selection
It is important to remember that random assignment is not the same thing as random selection , also known as random sampling.
Random selection instead involves how people are chosen to be in a study. Using random selection, every member of a population stands an equal chance of being chosen for a study or experiment.
So random sampling affects how participants are chosen for a study, while random assignment affects how participants are then assigned to groups.
Examples of Random Assignment
Imagine that a psychology researcher is conducting an experiment to determine if getting adequate sleep the night before an exam results in better test scores.
Forming a Hypothesis
They hypothesize that participants who get 8 hours of sleep will do better on a math exam than participants who only get 4 hours of sleep.
Obtaining Participants
The researcher starts by obtaining a pool of participants. They find 100 participants from a local university. Half of the participants are female, and half are male.
Randomly Assign Participants to Groups
The researcher then assigns random numbers to each participant and uses a random number generator to randomly assign each number to either the 4-hour or 8-hour sleep groups.
Conduct the Experiment
Those in the 8-hour sleep group agree to sleep for 8 hours that night, while those in the 4-hour group agree to wake up after only 4 hours. The following day, all of the participants meet in a classroom.
Collect and Analyze Data
Everyone takes the same math test. The test scores are then compared to see if the amount of sleep the night before had any impact on test scores.
Why Is Random Assignment Important in Psychology Research?
Random assignment is important in psychology research because it helps improve a study’s internal validity. This means that the researchers are sure that the study demonstrates a cause-and-effect relationship between an independent and dependent variable.
Random assignment improves the internal validity by minimizing the risk that there are systematic differences in the participants who are in each group.
Key Points to Remember About Random Assignment
- Random assignment in psychology involves each participant having an equal chance of being chosen for any of the groups, including the control and experimental groups.
- It helps control for potential confounding variables, reducing the likelihood of pre-existing differences between groups.
- This method enhances the internal validity of experiments, allowing researchers to draw more reliable conclusions about cause-and-effect relationships.
- Random assignment is crucial for creating comparable groups and increasing the scientific rigor of psychological studies.
5.2 Experimental Design
Learning objectives.
- Explain the difference between between-subjects and within-subjects experiments, list some of the pros and cons of each approach, and decide which approach to use to answer a particular research question.
- Define random assignment, distinguish it from random sampling, explain its purpose in experimental research, and use some simple strategies to implement it
- Define several types of carryover effect, give examples of each, and explain how counterbalancing helps to deal with them.
In this section, we look at some different ways to design an experiment. The primary distinction we will make is between approaches in which each participant experiences one level of the independent variable and approaches in which each participant experiences all levels of the independent variable. The former are called between-subjects experiments and the latter are called within-subjects experiments.
Between-Subjects Experiments
In a between-subjects experiment , each participant is tested in only one condition. For example, a researcher with a sample of 100 university students might assign half of them to write about a traumatic event and the other half write about a neutral event. Or a researcher with a sample of 60 people with severe agoraphobia (fear of open spaces) might assign 20 of them to receive each of three different treatments for that disorder. It is essential in a between-subjects experiment that the researcher assigns participants to conditions so that the different groups are, on average, highly similar to each other. Those in a trauma condition and a neutral condition, for example, should include a similar proportion of men and women, and they should have similar average intelligence quotients (IQs), similar average levels of motivation, similar average numbers of health problems, and so on. This matching is a matter of controlling these extraneous participant variables across conditions so that they do not become confounding variables.
Random Assignment
The primary way that researchers accomplish this kind of control of extraneous variables across conditions is called random assignment , which means using a random process to decide which participants are tested in which conditions. Do not confuse random assignment with random sampling. Random sampling is a method for selecting a sample from a population, and it is rarely used in psychological research. Random assignment is a method for assigning participants in a sample to the different conditions, and it is an important element of all experimental research in psychology and other fields too.
In its strictest sense, random assignment should meet two criteria. One is that each participant has an equal chance of being assigned to each condition (e.g., a 50% chance of being assigned to each of two conditions). The second is that each participant is assigned to a condition independently of other participants. Thus one way to assign participants to two conditions would be to flip a coin for each one. If the coin lands heads, the participant is assigned to Condition A, and if it lands tails, the participant is assigned to Condition B. For three conditions, one could use a computer to generate a random integer from 1 to 3 for each participant. If the integer is 1, the participant is assigned to Condition A; if it is 2, the participant is assigned to Condition B; and if it is 3, the participant is assigned to Condition C. In practice, a full sequence of conditions—one for each participant expected to be in the experiment—is usually created ahead of time, and each new participant is assigned to the next condition in the sequence as he or she is tested. When the procedure is computerized, the computer program often handles the random assignment.
One problem with coin flipping and other strict procedures for random assignment is that they are likely to result in unequal sample sizes in the different conditions. Unequal sample sizes are generally not a serious problem, and you should never throw away data you have already collected to achieve equal sample sizes. However, for a fixed number of participants, it is statistically most efficient to divide them into equal-sized groups. It is standard practice, therefore, to use a kind of modified random assignment that keeps the number of participants in each group as similar as possible. One approach is block randomization . In block randomization, all the conditions occur once in the sequence before any of them is repeated. Then they all occur again before any of them is repeated again. Within each of these “blocks,” the conditions occur in a random order. Again, the sequence of conditions is usually generated before any participants are tested, and each new participant is assigned to the next condition in the sequence. Table 5.2 shows such a sequence for assigning nine participants to three conditions. The Research Randomizer website ( http://www.randomizer.org ) will generate block randomization sequences for any number of participants and conditions. Again, when the procedure is computerized, the computer program often handles the block randomization.
Random assignment is not guaranteed to control all extraneous variables across conditions. The process is random, so it is always possible that just by chance, the participants in one condition might turn out to be substantially older, less tired, more motivated, or less depressed on average than the participants in another condition. However, there are some reasons that this possibility is not a major concern. One is that random assignment works better than one might expect, especially for large samples. Another is that the inferential statistics that researchers use to decide whether a difference between groups reflects a difference in the population takes the “fallibility” of random assignment into account. Yet another reason is that even if random assignment does result in a confounding variable and therefore produces misleading results, this confound is likely to be detected when the experiment is replicated. The upshot is that random assignment to conditions—although not infallible in terms of controlling extraneous variables—is always considered a strength of a research design.
Matched Groups
An alternative to simple random assignment of participants to conditions is the use of a matched-groups design . Using this design, participants in the various conditions are matched on the dependent variable or on some extraneous variable(s) prior the manipulation of the independent variable. This guarantees that these variables will not be confounded across the experimental conditions. For instance, if we want to determine whether expressive writing affects people’s health then we could start by measuring various health-related variables in our prospective research participants. We could then use that information to rank-order participants according to how healthy or unhealthy they are. Next, the two healthiest participants would be randomly assigned to complete different conditions (one would be randomly assigned to the traumatic experiences writing condition and the other to the neutral writing condition). The next two healthiest participants would then be randomly assigned to complete different conditions, and so on until the two least healthy participants. This method would ensure that participants in the traumatic experiences writing condition are matched to participants in the neutral writing condition with respect to health at the beginning of the study. If at the end of the experiment, a difference in health was detected across the two conditions, then we would know that it is due to the writing manipulation and not to pre-existing differences in health.
Within-Subjects Experiments
In a within-subjects experiment , each participant is tested under all conditions. Consider an experiment on the effect of a defendant’s physical attractiveness on judgments of his guilt. Again, in a between-subjects experiment, one group of participants would be shown an attractive defendant and asked to judge his guilt, and another group of participants would be shown an unattractive defendant and asked to judge his guilt. In a within-subjects experiment, however, the same group of participants would judge the guilt of both an attractive and an unattractive defendant.
The primary advantage of this approach is that it provides maximum control of extraneous participant variables. Participants in all conditions have the same mean IQ, same socioeconomic status, same number of siblings, and so on—because they are the very same people. Within-subjects experiments also make it possible to use statistical procedures that remove the effect of these extraneous participant variables on the dependent variable and therefore make the data less “noisy” and the effect of the independent variable easier to detect. We will look more closely at this idea later in the book . However, not all experiments can use a within-subjects design nor would it be desirable to do so.
One disadvantage of within-subjects experiments is that they make it easier for participants to guess the hypothesis. For example, a participant who is asked to judge the guilt of an attractive defendant and then is asked to judge the guilt of an unattractive defendant is likely to guess that the hypothesis is that defendant attractiveness affects judgments of guilt. This knowledge could lead the participant to judge the unattractive defendant more harshly because he thinks this is what he is expected to do. Or it could make participants judge the two defendants similarly in an effort to be “fair.”
Carryover Effects and Counterbalancing
The primary disadvantage of within-subjects designs is that they can result in order effects. An order effect occurs when participants’ responses in the various conditions are affected by the order of conditions to which they were exposed. One type of order effect is a carryover effect. A carryover effect is an effect of being tested in one condition on participants’ behavior in later conditions. One type of carryover effect is a practice effect , where participants perform a task better in later conditions because they have had a chance to practice it. Another type is a fatigue effect , where participants perform a task worse in later conditions because they become tired or bored. Being tested in one condition can also change how participants perceive stimuli or interpret their task in later conditions. This type of effect is called a context effect (or contrast effect) . For example, an average-looking defendant might be judged more harshly when participants have just judged an attractive defendant than when they have just judged an unattractive defendant. Within-subjects experiments also make it easier for participants to guess the hypothesis. For example, a participant who is asked to judge the guilt of an attractive defendant and then is asked to judge the guilt of an unattractive defendant is likely to guess that the hypothesis is that defendant attractiveness affects judgments of guilt.
Carryover effects can be interesting in their own right. (Does the attractiveness of one person depend on the attractiveness of other people that we have seen recently?) But when they are not the focus of the research, carryover effects can be problematic. Imagine, for example, that participants judge the guilt of an attractive defendant and then judge the guilt of an unattractive defendant. If they judge the unattractive defendant more harshly, this might be because of his unattractiveness. But it could be instead that they judge him more harshly because they are becoming bored or tired. In other words, the order of the conditions is a confounding variable. The attractive condition is always the first condition and the unattractive condition the second. Thus any difference between the conditions in terms of the dependent variable could be caused by the order of the conditions and not the independent variable itself.
There is a solution to the problem of order effects, however, that can be used in many situations. It is counterbalancing , which means testing different participants in different orders. The best method of counterbalancing is complete counterbalancing in which an equal number of participants complete each possible order of conditions. For example, half of the participants would be tested in the attractive defendant condition followed by the unattractive defendant condition, and others half would be tested in the unattractive condition followed by the attractive condition. With three conditions, there would be six different orders (ABC, ACB, BAC, BCA, CAB, and CBA), so some participants would be tested in each of the six orders. With four conditions, there would be 24 different orders; with five conditions there would be 120 possible orders. With counterbalancing, participants are assigned to orders randomly, using the techniques we have already discussed. Thus, random assignment plays an important role in within-subjects designs just as in between-subjects designs. Here, instead of randomly assigning to conditions, they are randomly assigned to different orders of conditions. In fact, it can safely be said that if a study does not involve random assignment in one form or another, it is not an experiment.
A more efficient way of counterbalancing is through a Latin square design which randomizes through having equal rows and columns. For example, if you have four treatments, you must have four versions. Like a Sudoku puzzle, no treatment can repeat in a row or column. For four versions of four treatments, the Latin square design would look like:
You can see in the diagram above that the square has been constructed to ensure that each condition appears at each ordinal position (A appears first once, second once, third once, and fourth once) and each condition preceded and follows each other condition one time. A Latin square for an experiment with 6 conditions would by 6 x 6 in dimension, one for an experiment with 8 conditions would be 8 x 8 in dimension, and so on. So while complete counterbalancing of 6 conditions would require 720 orders, a Latin square would only require 6 orders.
Finally, when the number of conditions is large experiments can use random counterbalancing in which the order of the conditions is randomly determined for each participant. Using this technique every possible order of conditions is determined and then one of these orders is randomly selected for each participant. This is not as powerful a technique as complete counterbalancing or partial counterbalancing using a Latin squares design. Use of random counterbalancing will result in more random error, but if order effects are likely to be small and the number of conditions is large, this is an option available to researchers.
There are two ways to think about what counterbalancing accomplishes. One is that it controls the order of conditions so that it is no longer a confounding variable. Instead of the attractive condition always being first and the unattractive condition always being second, the attractive condition comes first for some participants and second for others. Likewise, the unattractive condition comes first for some participants and second for others. Thus any overall difference in the dependent variable between the two conditions cannot have been caused by the order of conditions. A second way to think about what counterbalancing accomplishes is that if there are carryover effects, it makes it possible to detect them. One can analyze the data separately for each order to see whether it had an effect.
When 9 Is “Larger” Than 221
Researcher Michael Birnbaum has argued that the lack of context provided by between-subjects designs is often a bigger problem than the context effects created by within-subjects designs. To demonstrate this problem, he asked participants to rate two numbers on how large they were on a scale of 1-to-10 where 1 was “very very small” and 10 was “very very large”. One group of participants were asked to rate the number 9 and another group was asked to rate the number 221 (Birnbaum, 1999) [1] . Participants in this between-subjects design gave the number 9 a mean rating of 5.13 and the number 221 a mean rating of 3.10. In other words, they rated 9 as larger than 221! According to Birnbaum, this difference is because participants spontaneously compared 9 with other one-digit numbers (in which case it is relatively large) and compared 221 with other three-digit numbers (in which case it is relatively small).
Simultaneous Within-Subjects Designs
So far, we have discussed an approach to within-subjects designs in which participants are tested in one condition at a time. There is another approach, however, that is often used when participants make multiple responses in each condition. Imagine, for example, that participants judge the guilt of 10 attractive defendants and 10 unattractive defendants. Instead of having people make judgments about all 10 defendants of one type followed by all 10 defendants of the other type, the researcher could present all 20 defendants in a sequence that mixed the two types. The researcher could then compute each participant’s mean rating for each type of defendant. Or imagine an experiment designed to see whether people with social anxiety disorder remember negative adjectives (e.g., “stupid,” “incompetent”) better than positive ones (e.g., “happy,” “productive”). The researcher could have participants study a single list that includes both kinds of words and then have them try to recall as many words as possible. The researcher could then count the number of each type of word that was recalled.
Between-Subjects or Within-Subjects?
Almost every experiment can be conducted using either a between-subjects design or a within-subjects design. This possibility means that researchers must choose between the two approaches based on their relative merits for the particular situation.
Between-subjects experiments have the advantage of being conceptually simpler and requiring less testing time per participant. They also avoid carryover effects without the need for counterbalancing. Within-subjects experiments have the advantage of controlling extraneous participant variables, which generally reduces noise in the data and makes it easier to detect a relationship between the independent and dependent variables.
A good rule of thumb, then, is that if it is possible to conduct a within-subjects experiment (with proper counterbalancing) in the time that is available per participant—and you have no serious concerns about carryover effects—this design is probably the best option. If a within-subjects design would be difficult or impossible to carry out, then you should consider a between-subjects design instead. For example, if you were testing participants in a doctor’s waiting room or shoppers in line at a grocery store, you might not have enough time to test each participant in all conditions and therefore would opt for a between-subjects design. Or imagine you were trying to reduce people’s level of prejudice by having them interact with someone of another race. A within-subjects design with counterbalancing would require testing some participants in the treatment condition first and then in a control condition. But if the treatment works and reduces people’s level of prejudice, then they would no longer be suitable for testing in the control condition. This difficulty is true for many designs that involve a treatment meant to produce long-term change in participants’ behavior (e.g., studies testing the effectiveness of psychotherapy). Clearly, a between-subjects design would be necessary here.
Remember also that using one type of design does not preclude using the other type in a different study. There is no reason that a researcher could not use both a between-subjects design and a within-subjects design to answer the same research question. In fact, professional researchers often take exactly this type of mixed methods approach.
Key Takeaways
- Experiments can be conducted using either between-subjects or within-subjects designs. Deciding which to use in a particular situation requires careful consideration of the pros and cons of each approach.
- Random assignment to conditions in between-subjects experiments or counterbalancing of orders of conditions in within-subjects experiments is a fundamental element of experimental research. The purpose of these techniques is to control extraneous variables so that they do not become confounding variables.
- You want to test the relative effectiveness of two training programs for running a marathon.
- Using photographs of people as stimuli, you want to see if smiling people are perceived as more intelligent than people who are not smiling.
- In a field experiment, you want to see if the way a panhandler is dressed (neatly vs. sloppily) affects whether or not passersby give him any money.
- You want to see if concrete nouns (e.g., dog ) are recalled better than abstract nouns (e.g., truth).
- Birnbaum, M.H. (1999). How to show that 9>221: Collect judgments in a between-subjects design. Psychological Methods, 4 (3), 243-249. ↵

Share This Book
- Increase Font Size
2.3 Analyzing Findings
Learning objectives.
By the end of this section, you will be able to:
- Explain what a correlation coefficient tells us about the relationship between variables
- Recognize that correlation does not indicate a cause-and-effect relationship between variables
- Discuss our tendency to look for relationships between variables that do not really exist
- Explain random sampling and assignment of participants into experimental and control groups
- Discuss how experimenter or participant bias could affect the results of an experiment
- Identify independent and dependent variables
Did you know that as sales in ice cream increase, so does the overall rate of crime? Is it possible that indulging in your favorite flavor of ice cream could send you on a crime spree? Or, after committing crime do you think you might decide to treat yourself to a cone? There is no question that a relationship exists between ice cream and crime (e.g., Harper, 2013), but it would be pretty foolish to decide that one thing actually caused the other to occur.
It is much more likely that both ice cream sales and crime rates are related to the temperature outside. When the temperature is warm, there are lots of people out of their houses, interacting with each other, getting annoyed with one another, and sometimes committing crimes. Also, when it is warm outside, we are more likely to seek a cool treat like ice cream. How do we determine if there is indeed a relationship between two things? And when there is a relationship, how can we discern whether it is attributable to coincidence or causation?
Correlational Research
Correlation means that there is a relationship between two or more variables (such as ice cream consumption and crime), but this relationship does not necessarily imply cause and effect. When two variables are correlated, it simply means that as one variable changes, so does the other. We can measure correlation by calculating a statistic known as a correlation coefficient. A correlation coefficient is a number from -1 to +1 that indicates the strength and direction of the relationship between variables. The correlation coefficient is usually represented by the letter r .
The number portion of the correlation coefficient indicates the strength of the relationship. The closer the number is to 1 (be it negative or positive), the more strongly related the variables are, and the more predictable changes in one variable will be as the other variable changes. The closer the number is to zero, the weaker the relationship, and the less predictable the relationship between the variables becomes. For instance, a correlation coefficient of 0.9 indicates a far stronger relationship than a correlation coefficient of 0.3. If the variables are not related to one another at all, the correlation coefficient is 0. The example above about ice cream and crime is an example of two variables that we might expect to have no relationship to each other.
The sign—positive or negative—of the correlation coefficient indicates the direction of the relationship ( Figure 2.12 ). A positive correlation means that the variables move in the same direction. Put another way, it means that as one variable increases so does the other, and conversely, when one variable decreases so does the other. A negative correlation means that the variables move in opposite directions. If two variables are negatively correlated, a decrease in one variable is associated with an increase in the other and vice versa.
The example of ice cream and crime rates is a positive correlation because both variables increase when temperatures are warmer. Other examples of positive correlations are the relationship between an individual’s height and weight or the relationship between a person’s age and number of wrinkles. One might expect a negative correlation to exist between someone’s tiredness during the day and the number of hours they slept the previous night: the amount of sleep decreases as the feelings of tiredness increase. In a real-world example of negative correlation, student researchers at the University of Minnesota found a weak negative correlation ( r = -0.29) between the average number of days per week that students got fewer than 5 hours of sleep and their GPA (Lowry, Dean, & Manders, 2010). Keep in mind that a negative correlation is not the same as no correlation. For example, we would probably find no correlation between hours of sleep and shoe size.
As mentioned earlier, correlations have predictive value. Imagine that you are on the admissions committee of a major university. You are faced with a huge number of applications, but you are able to accommodate only a small percentage of the applicant pool. How might you decide who should be admitted? You might try to correlate your current students’ college GPA with their scores on standardized tests like the SAT or ACT. By observing which correlations were strongest for your current students, you could use this information to predict relative success of those students who have applied for admission into the university.
Link to Learning
Manipulate this interactive scatterplot to practice your understanding of positive and negative correlation.
Correlation Does Not Indicate Causation
Correlational research is useful because it allows us to discover the strength and direction of relationships that exist between two variables. However, correlation is limited because establishing the existence of a relationship tells us little about cause and effect . While variables are sometimes correlated because one does cause the other, it could also be that some other factor, a confounding variable , is actually causing the systematic movement in our variables of interest. In the ice cream/crime rate example mentioned earlier, temperature is a confounding variable that could account for the relationship between the two variables.
Even when we cannot point to clear confounding variables, we should not assume that a correlation between two variables implies that one variable causes changes in another. This can be frustrating when a cause-and-effect relationship seems clear and intuitive. Think back to our discussion of the research done by the American Cancer Society and how their research projects were some of the first demonstrations of the link between smoking and cancer. It seems reasonable to assume that smoking causes cancer, but if we were limited to correlational research , we would be overstepping our bounds by making this assumption.
Unfortunately, people mistakenly make claims of causation as a function of correlations all the time. Such claims are especially common in advertisements and news stories. For example, research found that people who eat certain breakfast cereal may have a reduced risk of heart disease (Anderson, Hanna, Peng, & Kryscio, 2000). Cereal companies are likely to share this information in a way that maximizes and perhaps overstates the positive aspects of eating cereal. But does cereal really cause better health, or are there other possible explanations for the health of those who eat cereal? While correlational research is invaluable in identifying relationships among variables, a major limitation is the inability to establish causality. Psychologists want to make statements about cause and effect, but the only way to do that is to conduct an experiment to answer a research question. The next section describes how scientific experiments incorporate methods that eliminate, or control for, alternative explanations, which allow researchers to explore how changes in one variable cause changes in another variable.
Illusory Correlations
The temptation to make erroneous cause-and-effect statements based on correlational research is not the only way we tend to misinterpret data. We also tend to make the mistake of illusory correlations, especially with unsystematic observations. Illusory correlations , or false correlations, occur when people believe that relationships exist between two things when no such relationship exists. One well-known illusory correlation is the supposed effect that the moon’s phases have on human behavior. Many people passionately assert that human behavior is affected by the phase of the moon, and specifically, that people act strangely when the moon is full ( Figure 2.14 ).
There is no denying that the moon exerts a powerful influence on our planet. The ebb and flow of the ocean’s tides are tightly tied to the gravitational forces of the moon. Many people believe, therefore, that it is logical that we are affected by the moon as well. After all, our bodies are largely made up of water. A meta-analysis of nearly 40 studies consistently demonstrated, however, that the relationship between the moon and our behavior does not exist (Rotton & Kelly, 1985). While we may pay more attention to odd behavior during the full phase of the moon, the rates of odd behavior remain constant throughout the lunar cycle.
Why are we so apt to believe in illusory correlations like this? Often we read or hear about them and simply accept the information as valid. Or, we have a hunch about how something works and then look for evidence to support that hunch, ignoring evidence that would tell us our hunch is false; this is known as confirmation bias . Other times, we find illusory correlations based on the information that comes most easily to mind, even if that information is severely limited. And while we may feel confident that we can use these relationships to better understand and predict the world around us, illusory correlations can have significant drawbacks. For example, research suggests that illusory correlations—in which certain behaviors are inaccurately attributed to certain groups—are involved in the formation of prejudicial attitudes that can ultimately lead to discriminatory behavior (Fiedler, 2004).
Causality: Conducting Experiments and Using the Data
As you’ve learned, the only way to establish that there is a cause-and-effect relationship between two variables is to conduct a scientific experiment . Experiment has a different meaning in the scientific context than in everyday life. In everyday conversation, we often use it to describe trying something for the first time, such as experimenting with a new hair style or a new food. However, in the scientific context, an experiment has precise requirements for design and implementation.
The Experimental Hypothesis
In order to conduct an experiment, a researcher must have a specific hypothesis to be tested. As you’ve learned, hypotheses can be formulated either through direct observation of the real world or after careful review of previous research. For example, if you think that the use of technology in the classroom has negative impacts on learning, then you have basically formulated a hypothesis—namely, that the use of technology in the classroom should be limited because it decreases learning. How might you have arrived at this particular hypothesis? You may have noticed that your classmates who take notes on their laptops perform at lower levels on class exams than those who take notes by hand, or those who receive a lesson via a computer program versus via an in-person teacher have different levels of performance when tested ( Figure 2.15 ).
These sorts of personal observations are what often lead us to formulate a specific hypothesis, but we cannot use limited personal observations and anecdotal evidence to rigorously test our hypothesis. Instead, to find out if real-world data supports our hypothesis, we have to conduct an experiment.
Designing an Experiment
The most basic experimental design involves two groups: the experimental group and the control group. The two groups are designed to be the same except for one difference— experimental manipulation. The experimental group gets the experimental manipulation—that is, the treatment or variable being tested (in this case, the use of technology)—and the control group does not. Since experimental manipulation is the only difference between the experimental and control groups, we can be sure that any differences between the two are due to experimental manipulation rather than chance.
In our example of how the use of technology should be limited in the classroom, we have the experimental group learn algebra using a computer program and then test their learning. We measure the learning in our control group after they are taught algebra by a teacher in a traditional classroom. It is important for the control group to be treated similarly to the experimental group, with the exception that the control group does not receive the experimental manipulation.
We also need to precisely define, or operationalize, how we measure learning of algebra. An operational definition is a precise description of our variables, and it is important in allowing others to understand exactly how and what a researcher measures in a particular experiment. In operationalizing learning, we might choose to look at performance on a test covering the material on which the individuals were taught by the teacher or the computer program. We might also ask our participants to summarize the information that was just presented in some way. Whatever we determine, it is important that we operationalize learning in such a way that anyone who hears about our study for the first time knows exactly what we mean by learning. This aids peoples’ ability to interpret our data as well as their capacity to repeat our experiment should they choose to do so.
Once we have operationalized what is considered use of technology and what is considered learning in our experiment participants, we need to establish how we will run our experiment. In this case, we might have participants spend 45 minutes learning algebra (either through a computer program or with an in-person math teacher) and then give them a test on the material covered during the 45 minutes.
Ideally, the people who score the tests are unaware of who was assigned to the experimental or control group, in order to control for experimenter bias. Experimenter bias refers to the possibility that a researcher’s expectations might skew the results of the study. Remember, conducting an experiment requires a lot of planning, and the people involved in the research project have a vested interest in supporting their hypotheses. If the observers knew which child was in which group, it might influence how they interpret ambiguous responses, such as sloppy handwriting or minor computational mistakes. By being blind to which child is in which group, we protect against those biases. This situation is a single-blind study , meaning that one of the groups (participants) are unaware as to which group they are in (experiment or control group) while the researcher who developed the experiment knows which participants are in each group.
In a double-blind study , both the researchers and the participants are blind to group assignments. Why would a researcher want to run a study where no one knows who is in which group? Because by doing so, we can control for both experimenter and participant expectations. If you are familiar with the phrase placebo effect , you already have some idea as to why this is an important consideration. The placebo effect occurs when people's expectations or beliefs influence or determine their experience in a given situation. In other words, simply expecting something to happen can actually make it happen.
The placebo effect is commonly described in terms of testing the effectiveness of a new medication. Imagine that you work in a pharmaceutical company, and you think you have a new drug that is effective in treating depression. To demonstrate that your medication is effective, you run an experiment with two groups: The experimental group receives the medication, and the control group does not. But you don’t want participants to know whether they received the drug or not.
Why is that? Imagine that you are a participant in this study, and you have just taken a pill that you think will improve your mood. Because you expect the pill to have an effect, you might feel better simply because you took the pill and not because of any drug actually contained in the pill—this is the placebo effect.
To make sure that any effects on mood are due to the drug and not due to expectations, the control group receives a placebo (in this case a sugar pill). Now everyone gets a pill, and once again neither the researcher nor the experimental participants know who got the drug and who got the sugar pill. Any differences in mood between the experimental and control groups can now be attributed to the drug itself rather than to experimenter bias or participant expectations ( Figure 2.16 ).
Independent and Dependent Variables
In a research experiment, we strive to study whether changes in one thing cause changes in another. To achieve this, we must pay attention to two important variables, or things that can be changed, in any experimental study: the independent variable and the dependent variable. An independent variable is manipulated or controlled by the experimenter. In a well-designed experimental study, the independent variable is the only important difference between the experimental and control groups. In our example of how technology use in the classroom affects learning, the independent variable is the type of learning by participants in the study ( Figure 2.17 ). A dependent variable is what the researcher measures to see how much effect the independent variable had. In our example, the dependent variable is the learning exhibited by our participants.
We expect that the dependent variable will change as a function of the independent variable. In other words, the dependent variable depends on the independent variable. A good way to think about the relationship between the independent and dependent variables is with this question: What effect does the independent variable have on the dependent variable? Returning to our example, what is the effect of being taught a lesson through a computer program versus through an in-person instructor?
Selecting and Assigning Experimental Participants
Now that our study is designed, we need to obtain a sample of individuals to include in our experiment. Our study involves human participants so we need to determine whom to include. Participants are the subjects of psychological research, and as the name implies, individuals who are involved in psychological research actively participate in the process. Often, psychological research projects rely on college students to serve as participants. In fact, the vast majority of research in psychology subfields has historically involved students as research participants (Sears, 1986; Arnett, 2008). But are college students truly representative of the general population? College students tend to be younger, more educated, more liberal, and less diverse than the general population. Although using students as test subjects is an accepted practice, relying on such a limited pool of research participants can be problematic because it is difficult to generalize findings to the larger population.
Our hypothetical experiment involves high school students, and we must first generate a sample of students. Samples are used because populations are usually too large to reasonably involve every member in our particular experiment ( Figure 2.18 ). If possible, we should use a random sample (there are other types of samples, but for the purposes of this chapter, we will focus on random samples). A random sample is a subset of a larger population in which every member of the population has an equal chance of being selected. Random samples are preferred because if the sample is large enough we can be reasonably sure that the participating individuals are representative of the larger population. This means that the percentages of characteristics in the sample—sex, ethnicity, socioeconomic level, and any other characteristics that might affect the results—are close to those percentages in the larger population.
In our example, let’s say we decide our population of interest is algebra students. But all algebra students is a very large population, so we need to be more specific; instead we might say our population of interest is all algebra students in a particular city. We should include students from various income brackets, family situations, races, ethnicities, religions, and geographic areas of town. With this more manageable population, we can work with the local schools in selecting a random sample of around 200 algebra students who we want to participate in our experiment.
In summary, because we cannot test all of the algebra students in a city, we want to find a group of about 200 that reflects the composition of that city. With a representative group, we can generalize our findings to the larger population without fear of our sample being biased in some way.
Now that we have a sample, the next step of the experimental process is to split the participants into experimental and control groups through random assignment. With random assignment , all participants have an equal chance of being assigned to either group. There is statistical software that will randomly assign each of the algebra students in the sample to either the experimental or the control group.
Random assignment is critical for sound experimental design . With sufficiently large samples, random assignment makes it unlikely that there are systematic differences between the groups. So, for instance, it would be very unlikely that we would get one group composed entirely of males, a given ethnic identity, or a given religious ideology. This is important because if the groups were systematically different before the experiment began, we would not know the origin of any differences we find between the groups: Were the differences preexisting, or were they caused by manipulation of the independent variable? Random assignment allows us to assume that any differences observed between experimental and control groups result from the manipulation of the independent variable.
Use this online random number generator to learn more about random sampling and assignments.
Issues to Consider
While experiments allow scientists to make cause-and-effect claims, they are not without problems. True experiments require the experimenter to manipulate an independent variable, and that can complicate many questions that psychologists might want to address. For instance, imagine that you want to know what effect sex (the independent variable) has on spatial memory (the dependent variable). Although you can certainly look for differences between males and females on a task that taps into spatial memory, you cannot directly control a person’s sex. We categorize this type of research approach as quasi-experimental and recognize that we cannot make cause-and-effect claims in these circumstances.
Experimenters are also limited by ethical constraints. For instance, you would not be able to conduct an experiment designed to determine if experiencing abuse as a child leads to lower levels of self-esteem among adults. To conduct such an experiment, you would need to randomly assign some experimental participants to a group that receives abuse, and that experiment would be unethical.
Interpreting Experimental Findings
Once data is collected from both the experimental and the control groups, a statistical analysis is conducted to find out if there are meaningful differences between the two groups. A statistical analysis determines how likely it is that any difference found is due to chance (and thus not meaningful). For example, if an experiment is done on the effectiveness of a nutritional supplement, and those taking a placebo pill (and not the supplement) have the same result as those taking the supplement, then the experiment has shown that the nutritional supplement is not effective. Generally, psychologists consider differences to be statistically significant if there is less than a five percent chance of observing them if the groups did not actually differ from one another. Stated another way, psychologists want to limit the chances of making “false positive” claims to five percent or less.
The greatest strength of experiments is the ability to assert that any significant differences in the findings are caused by the independent variable. This occurs because random selection, random assignment, and a design that limits the effects of both experimenter bias and participant expectancy should create groups that are similar in composition and treatment. Therefore, any difference between the groups is attributable to the independent variable, and now we can finally make a causal statement. If we find that watching a violent television program results in more violent behavior than watching a nonviolent program, we can safely say that watching violent television programs causes an increase in the display of violent behavior.
Reporting Research
When psychologists complete a research project, they generally want to share their findings with other scientists. The American Psychological Association (APA) publishes a manual detailing how to write a paper for submission to scientific journals. Unlike an article that might be published in a magazine like Psychology Today, which targets a general audience with an interest in psychology, scientific journals generally publish peer-reviewed journal articles aimed at an audience of professionals and scholars who are actively involved in research themselves.
The Online Writing Lab (OWL) at Purdue University can walk you through the APA writing guidelines.
A peer-reviewed journal article is read by several other scientists (generally anonymously) with expertise in the subject matter. These peer reviewers provide feedback—to both the author and the journal editor—regarding the quality of the draft. Peer reviewers look for a strong rationale for the research being described, a clear description of how the research was conducted, and evidence that the research was conducted in an ethical manner. They also look for flaws in the study's design, methods, and statistical analyses. They check that the conclusions drawn by the authors seem reasonable given the observations made during the research. Peer reviewers also comment on how valuable the research is in advancing the discipline’s knowledge. This helps prevent unnecessary duplication of research findings in the scientific literature and, to some extent, ensures that each research article provides new information. Ultimately, the journal editor will compile all of the peer reviewer feedback and determine whether the article will be published in its current state (a rare occurrence), published with revisions, or not accepted for publication.
Peer review provides some degree of quality control for psychological research. Poorly conceived or executed studies can be weeded out, and even well-designed research can be improved by the revisions suggested. Peer review also ensures that the research is described clearly enough to allow other scientists to replicate it, meaning they can repeat the experiment using different samples to determine reliability. Sometimes replications involve additional measures that expand on the original finding. In any case, each replication serves to provide more evidence to support the original research findings. Successful replications of published research make scientists more apt to adopt those findings, while repeated failures tend to cast doubt on the legitimacy of the original article and lead scientists to look elsewhere. For example, it would be a major advancement in the medical field if a published study indicated that taking a new drug helped individuals achieve better health without changing their behavior. But if other scientists could not replicate the results, the original study’s claims would be questioned.
In recent years, there has been increasing concern about a “replication crisis” that has affected a number of scientific fields, including psychology. Some of the most well-known studies and scientists have produced research that has failed to be replicated by others (as discussed in Shrout & Rodgers, 2018). In fact, even a famous Nobel Prize-winning scientist has recently retracted a published paper because she had difficulty replicating her results (Nobel Prize-winning scientist Frances Arnold retracts paper, 2020 January 3). These kinds of outcomes have prompted some scientists to begin to work together and more openly, and some would argue that the current “crisis” is actually improving the ways in which science is conducted and in how its results are shared with others (Aschwanden, 2018).
The Vaccine-Autism Myth and Retraction of Published Studies
Some scientists have claimed that routine childhood vaccines cause some children to develop autism, and, in fact, several peer-reviewed publications published research making these claims. Since the initial reports, large-scale epidemiological research has indicated that vaccinations are not responsible for causing autism and that it is much safer to have your child vaccinated than not. Furthermore, several of the original studies making this claim have since been retracted.
A published piece of work can be rescinded when data is called into question because of falsification, fabrication, or serious research design problems. Once rescinded, the scientific community is informed that there are serious problems with the original publication. Retractions can be initiated by the researcher who led the study, by research collaborators, by the institution that employed the researcher, or by the editorial board of the journal in which the article was originally published. In the vaccine-autism case, the retraction was made because of a significant conflict of interest in which the leading researcher had a financial interest in establishing a link between childhood vaccines and autism (Offit, 2008). Unfortunately, the initial studies received so much media attention that many parents around the world became hesitant to have their children vaccinated ( Figure 2.19 ). Continued reliance on such debunked studies has significant consequences. For instance, between January and October of 2019, there were 22 measles outbreaks across the United States and more than a thousand cases of individuals contracting measles (Patel et al., 2019). This is likely due to the anti-vaccination movements that have risen from the debunked research. For more information about how the vaccine/autism story unfolded, as well as the repercussions of this story, take a look at Paul Offit’s book, Autism’s False Prophets: Bad Science, Risky Medicine, and the Search for a Cure.
Reliability and Validity
Reliability and validity are two important considerations that must be made with any type of data collection. Reliability refers to the ability to consistently produce a given result. In the context of psychological research, this would mean that any instruments or tools used to collect data do so in consistent, reproducible ways. There are a number of different types of reliability. Some of these include inter-rater reliability (the degree to which two or more different observers agree on what has been observed), internal consistency (the degree to which different items on a survey that measure the same thing correlate with one another), and test-retest reliability (the degree to which the outcomes of a particular measure remain consistent over multiple administrations).
Unfortunately, being consistent in measurement does not necessarily mean that you have measured something correctly. To illustrate this concept, consider a kitchen scale that would be used to measure the weight of cereal that you eat in the morning. If the scale is not properly calibrated, it may consistently under- or overestimate the amount of cereal that’s being measured. While the scale is highly reliable in producing consistent results (e.g., the same amount of cereal poured onto the scale produces the same reading each time), those results are incorrect. This is where validity comes into play. Validity refers to the extent to which a given instrument or tool accurately measures what it’s supposed to measure, and once again, there are a number of ways in which validity can be expressed. Ecological validity (the degree to which research results generalize to real-world applications), construct validity (the degree to which a given variable actually captures or measures what it is intended to measure), and face validity (the degree to which a given variable seems valid on the surface) are just a few types that researchers consider. While any valid measure is by necessity reliable, the reverse is not necessarily true. Researchers strive to use instruments that are both highly reliable and valid.
Everyday Connection
How valid are the sat and act.
Standardized tests like the SAT and ACT are supposed to measure an individual’s aptitude for a college education, but how reliable and valid are such tests? Research conducted by the College Board suggests that scores on the SAT have high predictive validity for first-year college students’ GPA (Kobrin, Patterson, Shaw, Mattern, & Barbuti, 2008). In this context, predictive validity refers to the test’s ability to effectively predict the GPA of college freshmen. Given that many institutions of higher education require the SAT or ACT for admission, this high degree of predictive validity might be comforting.
However, the emphasis placed on SAT or ACT scores in college admissions is changing based on a number of factors. For one, some researchers assert that these tests are biased, and students from historically marginalized populations are at a disadvantage that unfairly reduces the likelihood of being admitted into a college (Santelices & Wilson, 2010). Additionally, some research has suggested that the predictive validity of these tests is grossly exaggerated in how well they are able to predict the GPA of first-year college students. In fact, it has been suggested that the SAT’s predictive validity may be overestimated by as much as 150% (Rothstein, 2004). Many institutions of higher education are beginning to consider de-emphasizing the significance of SAT scores in making admission decisions (Rimer, 2008).
Recent examples of high profile cheating scandals both domestically and abroad have only increased the scrutiny being placed on these types of tests, and as of March 2019, more than 1000 institutions of higher education have either relaxed or eliminated the requirements for SAT or ACT testing for admissions (Strauss, 2019, March 19).
As an Amazon Associate we earn from qualifying purchases.
This book may not be used in the training of large language models or otherwise be ingested into large language models or generative AI offerings without OpenStax's permission.
Want to cite, share, or modify this book? This book uses the Creative Commons Attribution License and you must attribute OpenStax.
Access for free at https://openstax.org/books/psychology-2e/pages/1-introduction
- Authors: Rose M. Spielman, William J. Jenkins, Marilyn D. Lovett
- Publisher/website: OpenStax
- Book title: Psychology 2e
- Publication date: Apr 22, 2020
- Location: Houston, Texas
- Book URL: https://openstax.org/books/psychology-2e/pages/1-introduction
- Section URL: https://openstax.org/books/psychology-2e/pages/2-3-analyzing-findings
© Jan 6, 2024 OpenStax. Textbook content produced by OpenStax is licensed under a Creative Commons Attribution License . The OpenStax name, OpenStax logo, OpenStax book covers, OpenStax CNX name, and OpenStax CNX logo are not subject to the Creative Commons license and may not be reproduced without the prior and express written consent of Rice University.

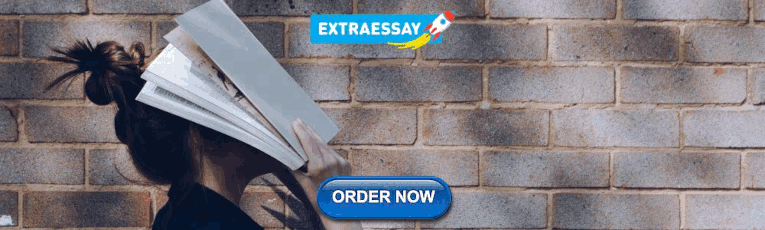
Random Assignment in Psychology (Intro for Students)
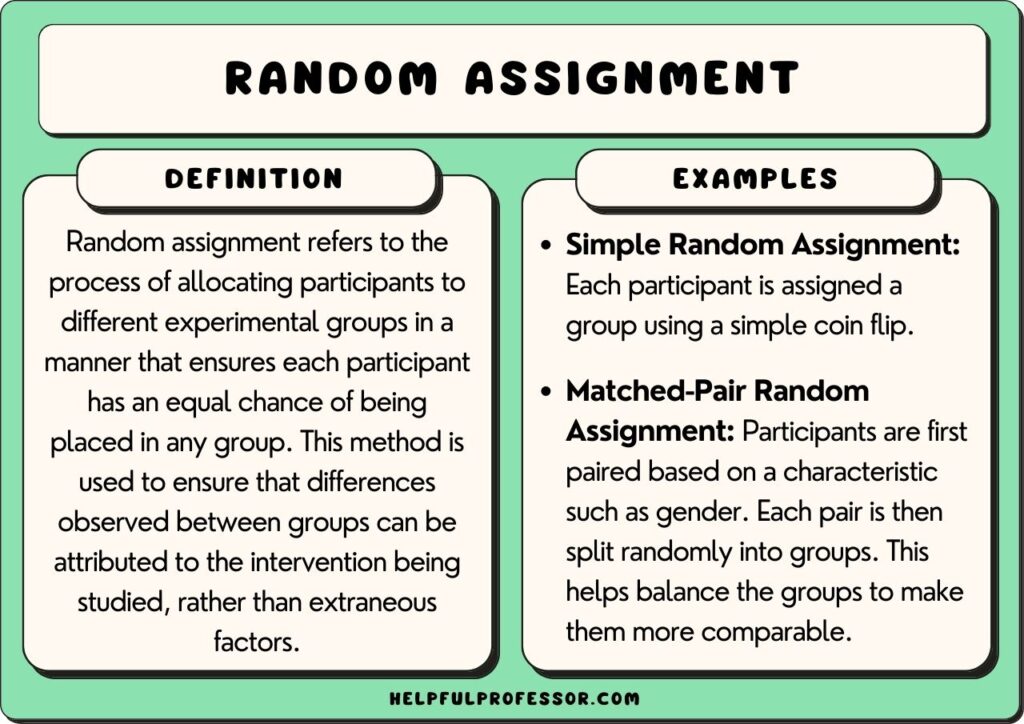
Random assignment is a research procedure used to randomly assign participants to different experimental conditions (or ‘groups’). This introduces the element of chance, ensuring that each participant has an equal likelihood of being placed in any condition group for the study.
It is absolutely essential that the treatment condition and the control condition are the same in all ways except for the variable being manipulated.
Using random assignment to place participants in different conditions helps to achieve this.
It ensures that those conditions are the same in regards to all potential confounding variables and extraneous factors .
Why Researchers Use Random Assignment
Researchers use random assignment to control for confounds in research.
Confounds refer to unwanted and often unaccounted-for variables that might affect the outcome of a study. These confounding variables can skew the results, rendering the experiment unreliable.
For example, below is a study with two groups. Note how there are more ‘red’ individuals in the first group than the second:
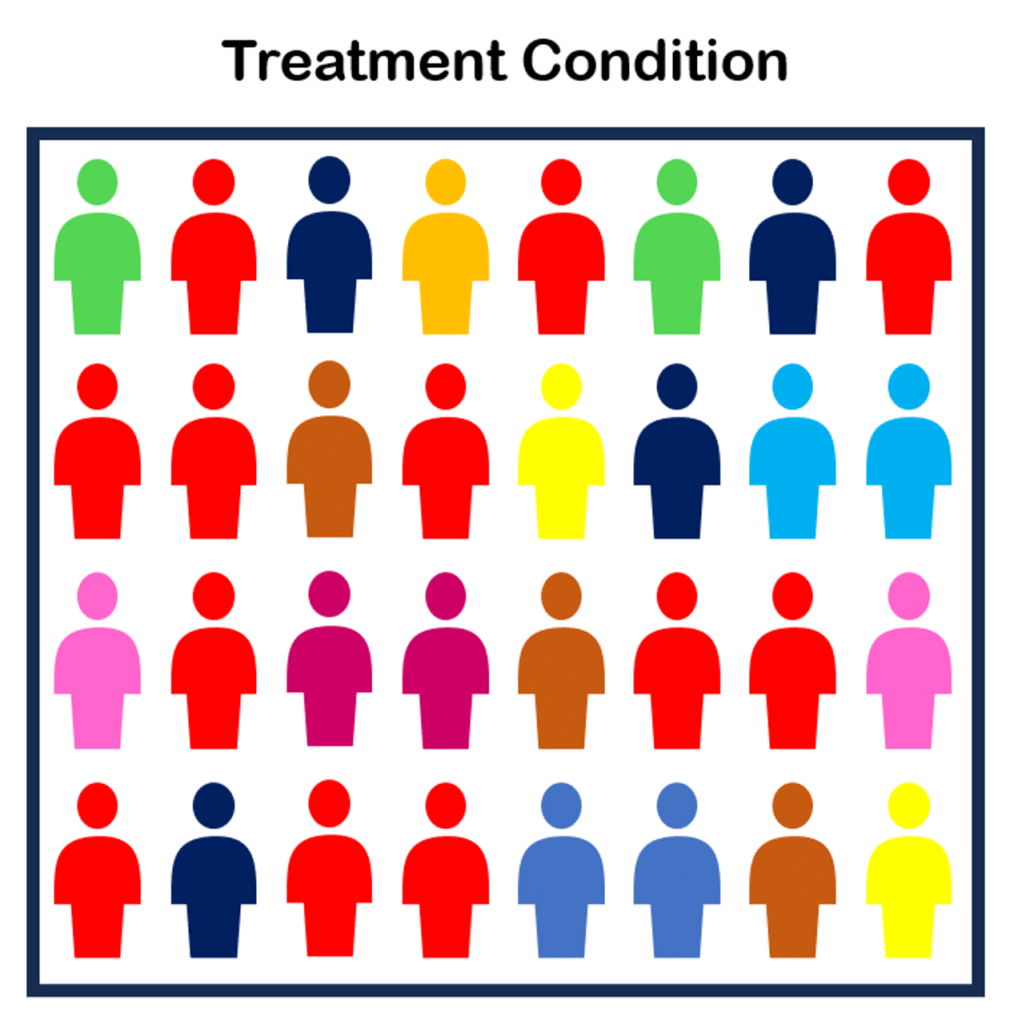
There is likely a confounding variable in this experiment explaining why more red people ended up in the treatment condition and less in the control condition. The red people might have self-selected, for example, leading to a skew of them in one group over the other.
Ideally, we’d want a more even distribution, like below:
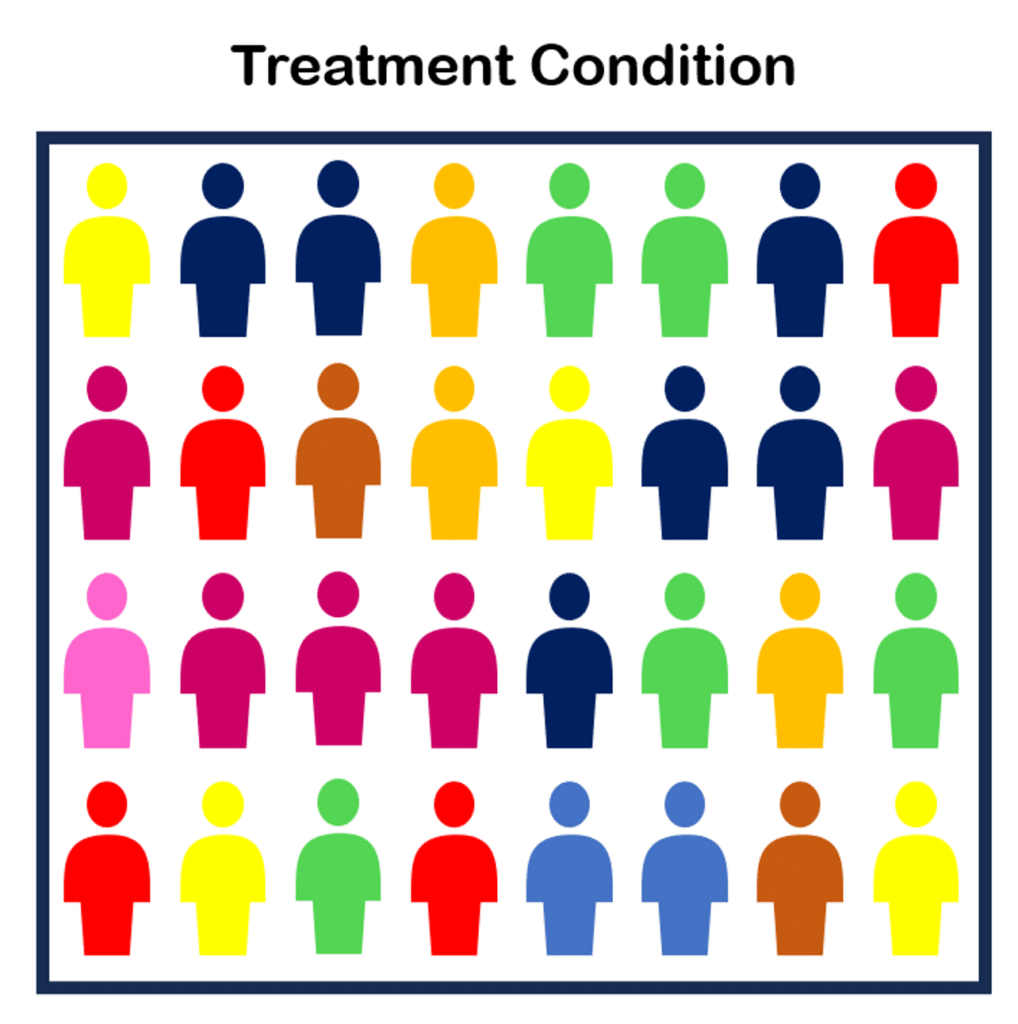
To achieve better balance in our two conditions, we use randomized sampling.
Fact File: Experiments 101
Random assignment is used in the type of research called the experiment.
An experiment involves manipulating the level of one variable and examining how it affects another variable. These are the independent and dependent variables :
- Independent Variable: The variable manipulated is called the independent variable (IV)
- Dependent Variable: The variable that it is expected to affect is called the dependent variable (DV).
The most basic form of the experiment involves two conditions: the treatment and the control .
- The Treatment Condition: The treatment condition involves the participants being exposed to the IV.
- The Control Condition: The control condition involves the absence of the IV. Therefore, the IV has two levels: zero and some quantity.
Researchers utilize random assignment to determine which participants go into which conditions.
Methods of Random Assignment
There are several procedures that researchers can use to randomly assign participants to different conditions.
1. Random number generator
There are several websites that offer computer-generated random numbers. Simply indicate how many conditions are in the experiment and then click. If there are 4 conditions, the program will randomly generate a number between 1 and 4 each time it is clicked.
2. Flipping a coin
If there are two conditions in an experiment, then the simplest way to implement random assignment is to flip a coin for each participant. Heads means being assigned to the treatment and tails means being assigned to the control (or vice versa).
3. Rolling a die
Rolling a single die is another way to randomly assign participants. If the experiment has three conditions, then numbers 1 and 2 mean being assigned to the control; numbers 3 and 4 mean treatment condition one; and numbers 5 and 6 mean treatment condition two.
4. Condition names in a hat
In some studies, the researcher will write the name of the treatment condition(s) or control on slips of paper and place them in a hat. If there are 4 conditions and 1 control, then there are 5 slips of paper.
The researcher closes their eyes and selects one slip for each participant. That person is then assigned to one of the conditions in the study and that slip of paper is placed back in the hat. Repeat as necessary.
There are other ways of trying to ensure that the groups of participants are equal in all ways with the exception of the IV. However, random assignment is the most often used because it is so effective at reducing confounds.
Read About More Methods and Examples of Random Assignment Here
Potential Confounding Effects
Random assignment is all about minimizing confounding effects.
Here are six types of confounds that can be controlled for using random assignment:
- Individual Differences: Participants in a study will naturally vary in terms of personality, intelligence, mood, prior knowledge, and many other characteristics. If one group happens to have more people with a particular characteristic, this could affect the results. Random assignment ensures that these individual differences are spread out equally among the experimental groups, making it less likely that they will unduly influence the outcome.
- Temporal or Time-Related Confounds: Events or situations that occur at a particular time can influence the outcome of an experiment. For example, a participant might be tested after a stressful event, while another might be tested after a relaxing weekend. Random assignment ensures that such effects are equally distributed among groups, thus controlling for their potential influence.
- Order Effects: If participants are exposed to multiple treatments or tests, the order in which they experience them can influence their responses. Randomly assigning the order of treatments for different participants helps control for this.
- Location or Environmental Confounds: The environment in which the study is conducted can influence the results. One group might be tested in a noisy room, while another might be in a quiet room. Randomly assigning participants to different locations can control for these effects.
- Instrumentation Confounds: These occur when there are variations in the calibration or functioning of measurement instruments across conditions. If one group’s responses are being measured using a slightly different tool or scale, it can introduce a confound. Random assignment can ensure that any such potential inconsistencies in instrumentation are equally distributed among groups.
- Experimenter Effects: Sometimes, the behavior or expectations of the person administering the experiment can unintentionally influence the participants’ behavior or responses. For instance, if an experimenter believes one treatment is superior, they might unconsciously communicate this belief to participants. Randomly assigning experimenters or using a double-blind procedure (where neither the participant nor the experimenter knows the treatment being given) can help control for this.
Random assignment helps balance out these and other potential confounds across groups, ensuring that any observed differences are more likely due to the manipulated independent variable rather than some extraneous factor.
Limitations of the Random Assignment Procedure
Although random assignment is extremely effective at eliminating the presence of participant-related confounds, there are several scenarios in which it cannot be used.
- Ethics: The most obvious scenario is when it would be unethical. For example, if wanting to investigate the effects of emotional abuse on children, it would be unethical to randomly assign children to either received abuse or not. Even if a researcher were to propose such a study, it would not receive approval from the Institutional Review Board (IRB) which oversees research by university faculty.
- Practicality: Other scenarios involve matters of practicality. For example, randomly assigning people to specific types of diet over a 10-year period would be interesting, but it would be highly unlikely that participants would be diligent enough to make the study valid. This is why examining these types of subjects has to be carried out through observational studies . The data is correlational, which is informative, but falls short of the scientist’s ultimate goal of identifying causality.
- Small Sample Size: The smaller the sample size being assigned to conditions, the more likely it is that the two groups will be unequal. For example, if you flip a coin many times in a row then you will notice that sometimes there will be a string of heads or tails that come up consecutively. This means that one condition may have a build-up of participants that share the same characteristics. However, if you continue flipping the coin, over the long-term, there will be a balance of heads and tails. Unfortunately, how large a sample size is necessary has been the subject of considerable debate (Bloom, 2006; Shadish et al., 2002).
“It is well known that larger sample sizes reduce the probability that random assignment will result in conditions that are unequal” (Goldberg, 2019, p. 2).
Applications of Random Assignment
The importance of random assignment has been recognized in a wide range of scientific and applied disciplines (Bloom, 2006).
Random assignment began as a tool in agricultural research by Fisher (1925, 1935). After WWII, it became extensively used in medical research to test the effectiveness of new treatments and pharmaceuticals (Marks, 1997).
Today it is widely used in industrial engineering (Box, Hunter, and Hunter, 2005), educational research (Lindquist, 1953; Ong-Dean et al., 2011)), psychology (Myers, 1972), and social policy studies (Boruch, 1998; Orr, 1999).
One of the biggest obstacles to the validity of an experiment is the confound. If the group of participants in the treatment condition are substantially different from the group in the control condition, then it is impossible to determine if the IV has an affect or if the confound has an effect.
Thankfully, random assignment is highly effective at eliminating confounds that are known and unknown. Because each participant has an equal chance of being placed in each condition, they are equally distributed.
There are several ways of implementing random assignment, including flipping a coin or using a random number generator.
Random assignment has become an essential procedure in research in a wide range of subjects such as psychology, education, and social policy.
Alferes, V. R. (2012). Methods of randomization in experimental design . Sage Publications.
Bloom, H. S. (2008). The core analytics of randomized experiments for social research. The SAGE Handbook of Social Research Methods , 115-133.
Boruch, R. F. (1998). Randomized controlled experiments for evaluation and planning. Handbook of applied social research methods , 161-191.
Box, G. E., Hunter, W. G., & Hunter, J. S. (2005). Design of experiments: Statistics for Experimenters: Design, Innovation and Discovery.
Dehue, T. (1997). Deception, efficiency, and random groups: Psychology and the gradual origination of the random group design. Isis , 88 (4), 653-673.
Fisher, R.A. (1925). Statistical methods for research workers (11th ed. rev.). Oliver and Boyd: Edinburgh.
Fisher, R. A. (1935). The Design of Experiments. Edinburgh: Oliver and Boyd.
Goldberg, M. H. (2019). How often does random assignment fail? Estimates and recommendations. Journal of Environmental Psychology , 66 , 101351.
Jamison, J. C. (2019). The entry of randomized assignment into the social sciences. Journal of Causal Inference , 7 (1), 20170025.
Lindquist, E. F. (1953). Design and analysis of experiments in psychology and education . Boston: Houghton Mifflin Company.
Marks, H. M. (1997). The progress of experiment: Science and therapeutic reform in the United States, 1900-1990 . Cambridge University Press.
Myers, J. L. (1972). Fundamentals of experimental design (2nd ed.). Allyn & Bacon.
Ong-Dean, C., Huie Hofstetter, C., & Strick, B. R. (2011). Challenges and dilemmas in implementing random assignment in educational research. American Journal of Evaluation , 32 (1), 29-49.
Orr, L. L. (1999). Social experiments: Evaluating public programs with experimental methods . Sage.
Shadish, W. R., Cook, T. D., & Campbell, D. T. (2002). Quasi-experiments: interrupted time-series designs. Experimental and quasi-experimental designs for generalized causal inference , 171-205.
Stigler, S. M. (1992). A historical view of statistical concepts in psychology and educational research. American Journal of Education , 101 (1), 60-70.
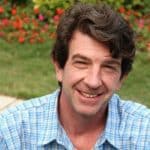
Dave Cornell (PhD)
Dr. Cornell has worked in education for more than 20 years. His work has involved designing teacher certification for Trinity College in London and in-service training for state governments in the United States. He has trained kindergarten teachers in 8 countries and helped businessmen and women open baby centers and kindergartens in 3 countries.
- Dave Cornell (PhD) https://helpfulprofessor.com/author/dave-cornell-phd/ 25 Positive Punishment Examples
- Dave Cornell (PhD) https://helpfulprofessor.com/author/dave-cornell-phd/ 25 Dissociation Examples (Psychology)
- Dave Cornell (PhD) https://helpfulprofessor.com/author/dave-cornell-phd/ 15 Zone of Proximal Development Examples
- Dave Cornell (PhD) https://helpfulprofessor.com/author/dave-cornell-phd/ Perception Checking: 15 Examples and Definition
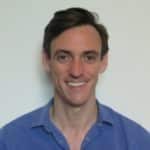
Chris Drew (PhD)
This article was peer-reviewed and edited by Chris Drew (PhD). The review process on Helpful Professor involves having a PhD level expert fact check, edit, and contribute to articles. Reviewers ensure all content reflects expert academic consensus and is backed up with reference to academic studies. Dr. Drew has published over 20 academic articles in scholarly journals. He is the former editor of the Journal of Learning Development in Higher Education and holds a PhD in Education from ACU.
- Chris Drew (PhD) #molongui-disabled-link 25 Positive Punishment Examples
- Chris Drew (PhD) #molongui-disabled-link 25 Dissociation Examples (Psychology)
- Chris Drew (PhD) #molongui-disabled-link 15 Zone of Proximal Development Examples
- Chris Drew (PhD) #molongui-disabled-link Perception Checking: 15 Examples and Definition
Leave a Comment Cancel Reply
Your email address will not be published. Required fields are marked *
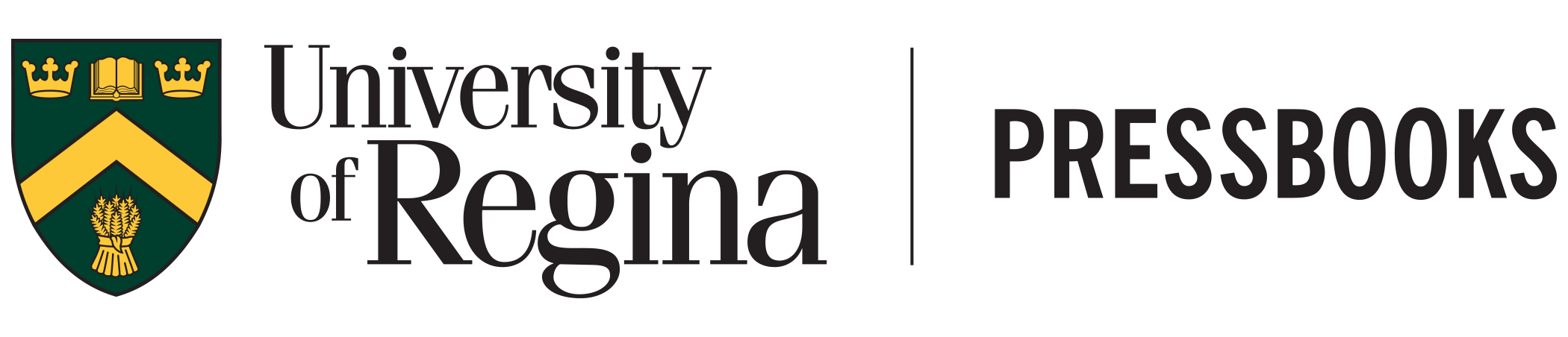
Want to create or adapt books like this? Learn more about how Pressbooks supports open publishing practices.
30 8.1 Experimental design: What is it and when should it be used?
Learning objectives.
- Define experiment
- Identify the core features of true experimental designs
- Describe the difference between an experimental group and a control group
- Identify and describe the various types of true experimental designs
Experiments are an excellent data collection strategy for social workers wishing to observe the effects of a clinical intervention or social welfare program. Understanding what experiments are and how they are conducted is useful for all social scientists, whether they actually plan to use this methodology or simply aim to understand findings from experimental studies. An experiment is a method of data collection designed to test hypotheses under controlled conditions. In social scientific research, the term experiment has a precise meaning and should not be used to describe all research methodologies.
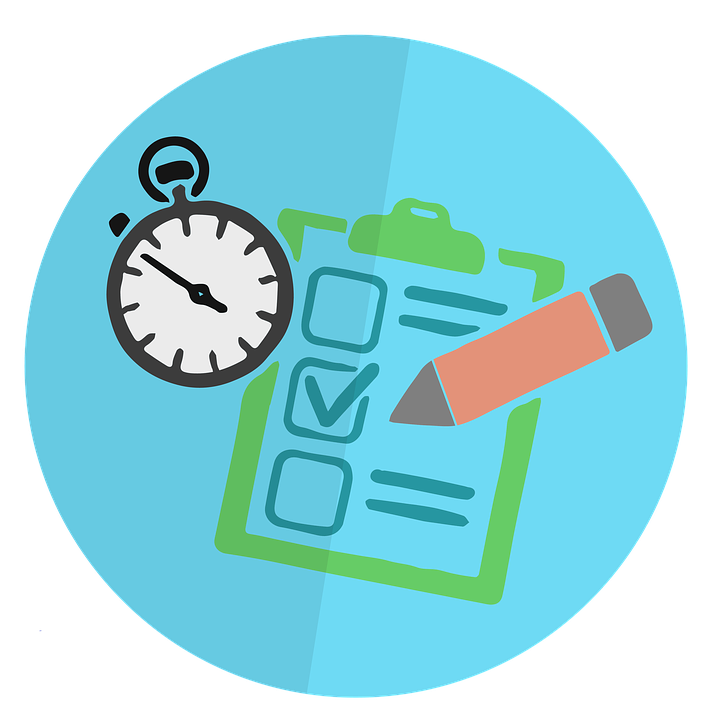
Experiments have a long and important history in social science. Behaviorists such as John Watson, B. F. Skinner, Ivan Pavlov, and Albert Bandura used experimental design to demonstrate the various types of conditioning. Using strictly controlled environments, behaviorists were able to isolate a single stimulus as the cause of measurable differences in behavior or physiological responses. The foundations of social learning theory and behavior modification are found in experimental research projects. Moreover, behaviorist experiments brought psychology and social science away from the abstract world of Freudian analysis and towards empirical inquiry, grounded in real-world observations and objectively-defined variables. Experiments are used at all levels of social work inquiry, including agency-based experiments that test therapeutic interventions and policy experiments that test new programs.
Several kinds of experimental designs exist. In general, designs considered to be true experiments contain three basic key features:
- random assignment of participants into experimental and control groups
- a “treatment” (or intervention) provided to the experimental group
- measurement of the effects of the treatment in a post-test administered to both groups
Some true experiments are more complex. Their designs can also include a pre-test and can have more than two groups, but these are the minimum requirements for a design to be a true experiment.
Experimental and control groups
In a true experiment, the effect of an intervention is tested by comparing two groups: one that is exposed to the intervention (the experimental group , also known as the treatment group) and another that does not receive the intervention (the control group ). Importantly, participants in a true experiment need to be randomly assigned to either the control or experimental groups. Random assignment uses a random number generator or some other random process to assign people into experimental and control groups. Random assignment is important in experimental research because it helps to ensure that the experimental group and control group are comparable and that any differences between the experimental and control groups are due to random chance. We will address more of the logic behind random assignment in the next section.
Treatment or intervention
In an experiment, the independent variable is receiving the intervention being tested—for example, a therapeutic technique, prevention program, or access to some service or support. It is less common in of social work research, but social science research may also have a stimulus, rather than an intervention as the independent variable. For example, an electric shock or a reading about death might be used as a stimulus to provoke a response.
In some cases, it may be immoral to withhold treatment completely from a control group within an experiment. If you recruited two groups of people with severe addiction and only provided treatment to one group, the other group would likely suffer. For these cases, researchers use a control group that receives “treatment as usual.” Experimenters must clearly define what treatment as usual means. For example, a standard treatment in substance abuse recovery is attending Alcoholics Anonymous or Narcotics Anonymous meetings. A substance abuse researcher conducting an experiment may use twelve-step programs in their control group and use their experimental intervention in the experimental group. The results would show whether the experimental intervention worked better than normal treatment, which is useful information.
The dependent variable is usually the intended effect the researcher wants the intervention to have. If the researcher is testing a new therapy for individuals with binge eating disorder, their dependent variable may be the number of binge eating episodes a participant reports. The researcher likely expects her intervention to decrease the number of binge eating episodes reported by participants. Thus, she must, at a minimum, measure the number of episodes that occur after the intervention, which is the post-test . In a classic experimental design, participants are also given a pretest to measure the dependent variable before the experimental treatment begins.
Types of experimental design
Let’s put these concepts in chronological order so we can better understand how an experiment runs from start to finish. Once you’ve collected your sample, you’ll need to randomly assign your participants to the experimental group and control group. In a common type of experimental design, you will then give both groups your pretest, which measures your dependent variable, to see what your participants are like before you start your intervention. Next, you will provide your intervention, or independent variable, to your experimental group, but not to your control group. Many interventions last a few weeks or months to complete, particularly therapeutic treatments. Finally, you will administer your post-test to both groups to observe any changes in your dependent variable. What we’ve just described is known as the classical experimental design and is the simplest type of true experimental design. All of the designs we review in this section are variations on this approach. Figure 8.1 visually represents these steps.

An interesting example of experimental research can be found in Shannon K. McCoy and Brenda Major’s (2003) study of people’s perceptions of prejudice. In one portion of this multifaceted study, all participants were given a pretest to assess their levels of depression. No significant differences in depression were found between the experimental and control groups during the pretest. Participants in the experimental group were then asked to read an article suggesting that prejudice against their own racial group is severe and pervasive, while participants in the control group were asked to read an article suggesting that prejudice against a racial group other than their own is severe and pervasive. Clearly, these were not meant to be interventions or treatments to help depression, but were stimuli designed to elicit changes in people’s depression levels. Upon measuring depression scores during the post-test period, the researchers discovered that those who had received the experimental stimulus (the article citing prejudice against their same racial group) reported greater depression than those in the control group. This is just one of many examples of social scientific experimental research.
In addition to classic experimental design, there are two other ways of designing experiments that are considered to fall within the purview of “true” experiments (Babbie, 2010; Campbell & Stanley, 1963). The posttest-only control group design is almost the same as classic experimental design, except it does not use a pretest. Researchers who use posttest-only designs want to eliminate testing effects , in which participants’ scores on a measure change because they have already been exposed to it. If you took multiple SAT or ACT practice exams before you took the real one you sent to colleges, you’ve taken advantage of testing effects to get a better score. Considering the previous example on racism and depression, participants who are given a pretest about depression before being exposed to the stimulus would likely assume that the intervention is designed to address depression. That knowledge could cause them to answer differently on the post-test than they otherwise would. In theory, as long as the control and experimental groups have been determined randomly and are therefore comparable, no pretest is needed. However, most researchers prefer to use pretests in case randomization did not result in equivalent groups and to help assess change over time within both the experimental and control groups.
Researchers wishing to account for testing effects but also gather pretest data can use a Solomon four-group design. In the Solomon four-group design , the researcher uses four groups. Two groups are treated as they would be in a classic experiment—pretest, experimental group intervention, and post-test. The other two groups do not receive the pretest, though one receives the intervention. All groups are given the post-test. Table 8.1 illustrates the features of each of the four groups in the Solomon four-group design. By having one set of experimental and control groups that complete the pretest (Groups 1 and 2) and another set that does not complete the pretest (Groups 3 and 4), researchers using the Solomon four-group design can account for testing effects in their analysis.
Solomon four-group designs are challenging to implement in the real world because they are time- and resource-intensive. Researchers must recruit enough participants to create four groups and implement interventions in two of them.
Overall, true experimental designs are sometimes difficult to implement in a real-world practice environment. It may be impossible to withhold treatment from a control group or randomly assign participants in a study. In these cases, pre-experimental and quasi-experimental designs–which we will discuss in the next section–can be used. However, the differences in rigor from true experimental designs leave their conclusions more open to critique.
Experimental design in macro-level research
You can imagine that social work researchers may be limited in their ability to use random assignment when examining the effects of governmental policy on individuals. For example, it is unlikely that a researcher could randomly assign some states to implement decriminalization of recreational marijuana and some states not to in order to assess the effects of the policy change. There are, however, important examples of policy experiments that use random assignment, including the Oregon Medicaid experiment. In the Oregon Medicaid experiment, the wait list for Oregon was so long, state officials conducted a lottery to see who from the wait list would receive Medicaid (Baicker et al., 2013). Researchers used the lottery as a natural experiment that included random assignment. People selected to be a part of Medicaid were the experimental group and those on the wait list were in the control group. There are some practical complications macro-level experiments, just as with other experiments. For example, the ethical concern with using people on a wait list as a control group exists in macro-level research just as it does in micro-level research.
Key Takeaways
- True experimental designs require random assignment.
- Control groups do not receive an intervention, and experimental groups receive an intervention.
- The basic components of a true experiment include a pretest, posttest, control group, and experimental group.
- Testing effects may cause researchers to use variations on the classic experimental design.
- Classic experimental design- uses random assignment, an experimental and control group, as well as pre- and posttesting
- Control group- the group in an experiment that does not receive the intervention
- Experiment- a method of data collection designed to test hypotheses under controlled conditions
- Experimental group- the group in an experiment that receives the intervention
- Posttest- a measurement taken after the intervention
- Posttest-only control group design- a type of experimental design that uses random assignment, and an experimental and control group, but does not use a pretest
- Pretest- a measurement taken prior to the intervention
- Random assignment-using a random process to assign people into experimental and control groups
- Solomon four-group design- uses random assignment, two experimental and two control groups, pretests for half of the groups, and posttests for all
- Testing effects- when a participant’s scores on a measure change because they have already been exposed to it
- True experiments- a group of experimental designs that contain independent and dependent variables, pretesting and post testing, and experimental and control groups
Image attributions
exam scientific experiment by mohamed_hassan CC-0
Foundations of Social Work Research Copyright © 2020 by Rebecca L. Mauldin is licensed under a Creative Commons Attribution-NonCommercial-ShareAlike 4.0 International License , except where otherwise noted.
Share This Book
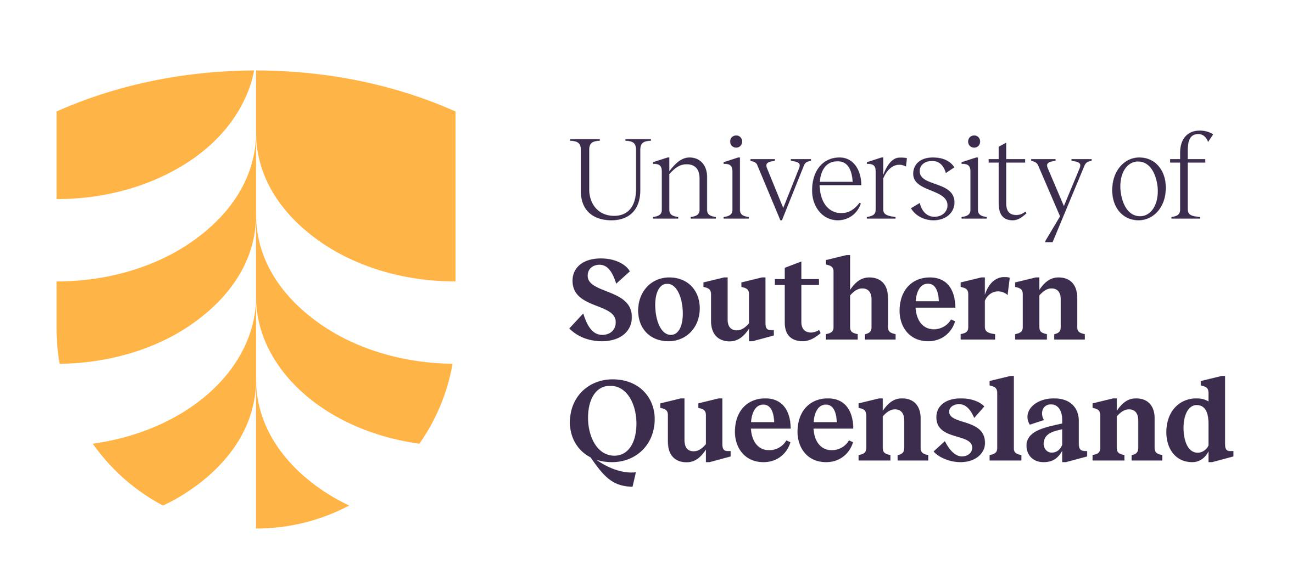
Want to create or adapt books like this? Learn more about how Pressbooks supports open publishing practices.
10 Experimental research
Experimental research—often considered to be the ‘gold standard’ in research designs—is one of the most rigorous of all research designs. In this design, one or more independent variables are manipulated by the researcher (as treatments), subjects are randomly assigned to different treatment levels (random assignment), and the results of the treatments on outcomes (dependent variables) are observed. The unique strength of experimental research is its internal validity (causality) due to its ability to link cause and effect through treatment manipulation, while controlling for the spurious effect of extraneous variable.
Experimental research is best suited for explanatory research—rather than for descriptive or exploratory research—where the goal of the study is to examine cause-effect relationships. It also works well for research that involves a relatively limited and well-defined set of independent variables that can either be manipulated or controlled. Experimental research can be conducted in laboratory or field settings. Laboratory experiments , conducted in laboratory (artificial) settings, tend to be high in internal validity, but this comes at the cost of low external validity (generalisability), because the artificial (laboratory) setting in which the study is conducted may not reflect the real world. Field experiments are conducted in field settings such as in a real organisation, and are high in both internal and external validity. But such experiments are relatively rare, because of the difficulties associated with manipulating treatments and controlling for extraneous effects in a field setting.
Experimental research can be grouped into two broad categories: true experimental designs and quasi-experimental designs. Both designs require treatment manipulation, but while true experiments also require random assignment, quasi-experiments do not. Sometimes, we also refer to non-experimental research, which is not really a research design, but an all-inclusive term that includes all types of research that do not employ treatment manipulation or random assignment, such as survey research, observational research, and correlational studies.
Basic concepts
Treatment and control groups. In experimental research, some subjects are administered one or more experimental stimulus called a treatment (the treatment group ) while other subjects are not given such a stimulus (the control group ). The treatment may be considered successful if subjects in the treatment group rate more favourably on outcome variables than control group subjects. Multiple levels of experimental stimulus may be administered, in which case, there may be more than one treatment group. For example, in order to test the effects of a new drug intended to treat a certain medical condition like dementia, if a sample of dementia patients is randomly divided into three groups, with the first group receiving a high dosage of the drug, the second group receiving a low dosage, and the third group receiving a placebo such as a sugar pill (control group), then the first two groups are experimental groups and the third group is a control group. After administering the drug for a period of time, if the condition of the experimental group subjects improved significantly more than the control group subjects, we can say that the drug is effective. We can also compare the conditions of the high and low dosage experimental groups to determine if the high dose is more effective than the low dose.
Treatment manipulation. Treatments are the unique feature of experimental research that sets this design apart from all other research methods. Treatment manipulation helps control for the ‘cause’ in cause-effect relationships. Naturally, the validity of experimental research depends on how well the treatment was manipulated. Treatment manipulation must be checked using pretests and pilot tests prior to the experimental study. Any measurements conducted before the treatment is administered are called pretest measures , while those conducted after the treatment are posttest measures .
Random selection and assignment. Random selection is the process of randomly drawing a sample from a population or a sampling frame. This approach is typically employed in survey research, and ensures that each unit in the population has a positive chance of being selected into the sample. Random assignment, however, is a process of randomly assigning subjects to experimental or control groups. This is a standard practice in true experimental research to ensure that treatment groups are similar (equivalent) to each other and to the control group prior to treatment administration. Random selection is related to sampling, and is therefore more closely related to the external validity (generalisability) of findings. However, random assignment is related to design, and is therefore most related to internal validity. It is possible to have both random selection and random assignment in well-designed experimental research, but quasi-experimental research involves neither random selection nor random assignment.
Threats to internal validity. Although experimental designs are considered more rigorous than other research methods in terms of the internal validity of their inferences (by virtue of their ability to control causes through treatment manipulation), they are not immune to internal validity threats. Some of these threats to internal validity are described below, within the context of a study of the impact of a special remedial math tutoring program for improving the math abilities of high school students.
History threat is the possibility that the observed effects (dependent variables) are caused by extraneous or historical events rather than by the experimental treatment. For instance, students’ post-remedial math score improvement may have been caused by their preparation for a math exam at their school, rather than the remedial math program.
Maturation threat refers to the possibility that observed effects are caused by natural maturation of subjects (e.g., a general improvement in their intellectual ability to understand complex concepts) rather than the experimental treatment.
Testing threat is a threat in pre-post designs where subjects’ posttest responses are conditioned by their pretest responses. For instance, if students remember their answers from the pretest evaluation, they may tend to repeat them in the posttest exam.
Not conducting a pretest can help avoid this threat.
Instrumentation threat , which also occurs in pre-post designs, refers to the possibility that the difference between pretest and posttest scores is not due to the remedial math program, but due to changes in the administered test, such as the posttest having a higher or lower degree of difficulty than the pretest.
Mortality threat refers to the possibility that subjects may be dropping out of the study at differential rates between the treatment and control groups due to a systematic reason, such that the dropouts were mostly students who scored low on the pretest. If the low-performing students drop out, the results of the posttest will be artificially inflated by the preponderance of high-performing students.
Regression threat —also called a regression to the mean—refers to the statistical tendency of a group’s overall performance to regress toward the mean during a posttest rather than in the anticipated direction. For instance, if subjects scored high on a pretest, they will have a tendency to score lower on the posttest (closer to the mean) because their high scores (away from the mean) during the pretest were possibly a statistical aberration. This problem tends to be more prevalent in non-random samples and when the two measures are imperfectly correlated.
Two-group experimental designs

Pretest-posttest control group design . In this design, subjects are randomly assigned to treatment and control groups, subjected to an initial (pretest) measurement of the dependent variables of interest, the treatment group is administered a treatment (representing the independent variable of interest), and the dependent variables measured again (posttest). The notation of this design is shown in Figure 10.1.

Statistical analysis of this design involves a simple analysis of variance (ANOVA) between the treatment and control groups. The pretest-posttest design handles several threats to internal validity, such as maturation, testing, and regression, since these threats can be expected to influence both treatment and control groups in a similar (random) manner. The selection threat is controlled via random assignment. However, additional threats to internal validity may exist. For instance, mortality can be a problem if there are differential dropout rates between the two groups, and the pretest measurement may bias the posttest measurement—especially if the pretest introduces unusual topics or content.
Posttest -only control group design . This design is a simpler version of the pretest-posttest design where pretest measurements are omitted. The design notation is shown in Figure 10.2.

The treatment effect is measured simply as the difference in the posttest scores between the two groups:
![Rendered by QuickLaTeX.com \[E = (O_{1} - O_{2})\,.\]](https://usq.pressbooks.pub/app/uploads/quicklatex/quicklatex.com-90f2ce47275e7b35def5c5b52b7d7d45_l3.png)
The appropriate statistical analysis of this design is also a two-group analysis of variance (ANOVA). The simplicity of this design makes it more attractive than the pretest-posttest design in terms of internal validity. This design controls for maturation, testing, regression, selection, and pretest-posttest interaction, though the mortality threat may continue to exist.

Because the pretest measure is not a measurement of the dependent variable, but rather a covariate, the treatment effect is measured as the difference in the posttest scores between the treatment and control groups as:
Due to the presence of covariates, the right statistical analysis of this design is a two-group analysis of covariance (ANCOVA). This design has all the advantages of posttest-only design, but with internal validity due to the controlling of covariates. Covariance designs can also be extended to pretest-posttest control group design.
Factorial designs
Two-group designs are inadequate if your research requires manipulation of two or more independent variables (treatments). In such cases, you would need four or higher-group designs. Such designs, quite popular in experimental research, are commonly called factorial designs. Each independent variable in this design is called a factor , and each subdivision of a factor is called a level . Factorial designs enable the researcher to examine not only the individual effect of each treatment on the dependent variables (called main effects), but also their joint effect (called interaction effects).

In a factorial design, a main effect is said to exist if the dependent variable shows a significant difference between multiple levels of one factor, at all levels of other factors. No change in the dependent variable across factor levels is the null case (baseline), from which main effects are evaluated. In the above example, you may see a main effect of instructional type, instructional time, or both on learning outcomes. An interaction effect exists when the effect of differences in one factor depends upon the level of a second factor. In our example, if the effect of instructional type on learning outcomes is greater for three hours/week of instructional time than for one and a half hours/week, then we can say that there is an interaction effect between instructional type and instructional time on learning outcomes. Note that the presence of interaction effects dominate and make main effects irrelevant, and it is not meaningful to interpret main effects if interaction effects are significant.
Hybrid experimental designs
Hybrid designs are those that are formed by combining features of more established designs. Three such hybrid designs are randomised bocks design, Solomon four-group design, and switched replications design.
Randomised block design. This is a variation of the posttest-only or pretest-posttest control group design where the subject population can be grouped into relatively homogeneous subgroups (called blocks ) within which the experiment is replicated. For instance, if you want to replicate the same posttest-only design among university students and full-time working professionals (two homogeneous blocks), subjects in both blocks are randomly split between the treatment group (receiving the same treatment) and the control group (see Figure 10.5). The purpose of this design is to reduce the ‘noise’ or variance in data that may be attributable to differences between the blocks so that the actual effect of interest can be detected more accurately.
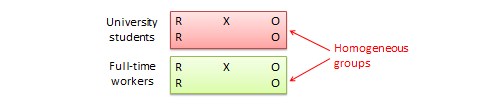
Solomon four-group design . In this design, the sample is divided into two treatment groups and two control groups. One treatment group and one control group receive the pretest, and the other two groups do not. This design represents a combination of posttest-only and pretest-posttest control group design, and is intended to test for the potential biasing effect of pretest measurement on posttest measures that tends to occur in pretest-posttest designs, but not in posttest-only designs. The design notation is shown in Figure 10.6.
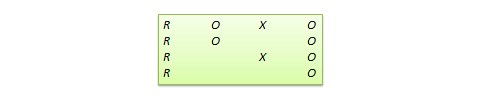
Switched replication design . This is a two-group design implemented in two phases with three waves of measurement. The treatment group in the first phase serves as the control group in the second phase, and the control group in the first phase becomes the treatment group in the second phase, as illustrated in Figure 10.7. In other words, the original design is repeated or replicated temporally with treatment/control roles switched between the two groups. By the end of the study, all participants will have received the treatment either during the first or the second phase. This design is most feasible in organisational contexts where organisational programs (e.g., employee training) are implemented in a phased manner or are repeated at regular intervals.

Quasi-experimental designs
Quasi-experimental designs are almost identical to true experimental designs, but lacking one key ingredient: random assignment. For instance, one entire class section or one organisation is used as the treatment group, while another section of the same class or a different organisation in the same industry is used as the control group. This lack of random assignment potentially results in groups that are non-equivalent, such as one group possessing greater mastery of certain content than the other group, say by virtue of having a better teacher in a previous semester, which introduces the possibility of selection bias . Quasi-experimental designs are therefore inferior to true experimental designs in interval validity due to the presence of a variety of selection related threats such as selection-maturation threat (the treatment and control groups maturing at different rates), selection-history threat (the treatment and control groups being differentially impacted by extraneous or historical events), selection-regression threat (the treatment and control groups regressing toward the mean between pretest and posttest at different rates), selection-instrumentation threat (the treatment and control groups responding differently to the measurement), selection-testing (the treatment and control groups responding differently to the pretest), and selection-mortality (the treatment and control groups demonstrating differential dropout rates). Given these selection threats, it is generally preferable to avoid quasi-experimental designs to the greatest extent possible.

In addition, there are quite a few unique non-equivalent designs without corresponding true experimental design cousins. Some of the more useful of these designs are discussed next.
Regression discontinuity (RD) design . This is a non-equivalent pretest-posttest design where subjects are assigned to the treatment or control group based on a cut-off score on a preprogram measure. For instance, patients who are severely ill may be assigned to a treatment group to test the efficacy of a new drug or treatment protocol and those who are mildly ill are assigned to the control group. In another example, students who are lagging behind on standardised test scores may be selected for a remedial curriculum program intended to improve their performance, while those who score high on such tests are not selected from the remedial program.

Because of the use of a cut-off score, it is possible that the observed results may be a function of the cut-off score rather than the treatment, which introduces a new threat to internal validity. However, using the cut-off score also ensures that limited or costly resources are distributed to people who need them the most, rather than randomly across a population, while simultaneously allowing a quasi-experimental treatment. The control group scores in the RD design do not serve as a benchmark for comparing treatment group scores, given the systematic non-equivalence between the two groups. Rather, if there is no discontinuity between pretest and posttest scores in the control group, but such a discontinuity persists in the treatment group, then this discontinuity is viewed as evidence of the treatment effect.
Proxy pretest design . This design, shown in Figure 10.11, looks very similar to the standard NEGD (pretest-posttest) design, with one critical difference: the pretest score is collected after the treatment is administered. A typical application of this design is when a researcher is brought in to test the efficacy of a program (e.g., an educational program) after the program has already started and pretest data is not available. Under such circumstances, the best option for the researcher is often to use a different prerecorded measure, such as students’ grade point average before the start of the program, as a proxy for pretest data. A variation of the proxy pretest design is to use subjects’ posttest recollection of pretest data, which may be subject to recall bias, but nevertheless may provide a measure of perceived gain or change in the dependent variable.

Separate pretest-posttest samples design . This design is useful if it is not possible to collect pretest and posttest data from the same subjects for some reason. As shown in Figure 10.12, there are four groups in this design, but two groups come from a single non-equivalent group, while the other two groups come from a different non-equivalent group. For instance, say you want to test customer satisfaction with a new online service that is implemented in one city but not in another. In this case, customers in the first city serve as the treatment group and those in the second city constitute the control group. If it is not possible to obtain pretest and posttest measures from the same customers, you can measure customer satisfaction at one point in time, implement the new service program, and measure customer satisfaction (with a different set of customers) after the program is implemented. Customer satisfaction is also measured in the control group at the same times as in the treatment group, but without the new program implementation. The design is not particularly strong, because you cannot examine the changes in any specific customer’s satisfaction score before and after the implementation, but you can only examine average customer satisfaction scores. Despite the lower internal validity, this design may still be a useful way of collecting quasi-experimental data when pretest and posttest data is not available from the same subjects.
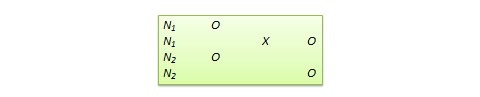
An interesting variation of the NEDV design is a pattern-matching NEDV design , which employs multiple outcome variables and a theory that explains how much each variable will be affected by the treatment. The researcher can then examine if the theoretical prediction is matched in actual observations. This pattern-matching technique—based on the degree of correspondence between theoretical and observed patterns—is a powerful way of alleviating internal validity concerns in the original NEDV design.

Perils of experimental research
Experimental research is one of the most difficult of research designs, and should not be taken lightly. This type of research is often best with a multitude of methodological problems. First, though experimental research requires theories for framing hypotheses for testing, much of current experimental research is atheoretical. Without theories, the hypotheses being tested tend to be ad hoc, possibly illogical, and meaningless. Second, many of the measurement instruments used in experimental research are not tested for reliability and validity, and are incomparable across studies. Consequently, results generated using such instruments are also incomparable. Third, often experimental research uses inappropriate research designs, such as irrelevant dependent variables, no interaction effects, no experimental controls, and non-equivalent stimulus across treatment groups. Findings from such studies tend to lack internal validity and are highly suspect. Fourth, the treatments (tasks) used in experimental research may be diverse, incomparable, and inconsistent across studies, and sometimes inappropriate for the subject population. For instance, undergraduate student subjects are often asked to pretend that they are marketing managers and asked to perform a complex budget allocation task in which they have no experience or expertise. The use of such inappropriate tasks, introduces new threats to internal validity (i.e., subject’s performance may be an artefact of the content or difficulty of the task setting), generates findings that are non-interpretable and meaningless, and makes integration of findings across studies impossible.
The design of proper experimental treatments is a very important task in experimental design, because the treatment is the raison d’etre of the experimental method, and must never be rushed or neglected. To design an adequate and appropriate task, researchers should use prevalidated tasks if available, conduct treatment manipulation checks to check for the adequacy of such tasks (by debriefing subjects after performing the assigned task), conduct pilot tests (repeatedly, if necessary), and if in doubt, use tasks that are simple and familiar for the respondent sample rather than tasks that are complex or unfamiliar.
In summary, this chapter introduced key concepts in the experimental design research method and introduced a variety of true experimental and quasi-experimental designs. Although these designs vary widely in internal validity, designs with less internal validity should not be overlooked and may sometimes be useful under specific circumstances and empirical contingencies.
Social Science Research: Principles, Methods and Practices (Revised edition) Copyright © 2019 by Anol Bhattacherjee is licensed under a Creative Commons Attribution-NonCommercial-ShareAlike 4.0 International License , except where otherwise noted.
Share This Book
Statistical Thinking: A Simulation Approach to Modeling Uncertainty (UM STAT 216 edition)
3.6 causation and random assignment.
Medical researchers may be interested in showing that a drug helps improve people’s health (the cause of improvement is the drug), while educational researchers may be interested in showing a curricular innovation improves students’ learning (the curricular innovation causes improved learning).
To attribute a causal relationship, there are three criteria a researcher needs to establish:
- Association of the Cause and Effect: There needs to be a association between the cause and effect.
- Timing: The cause needs to happen BEFORE the effect.
- No Plausible Alternative Explanations: ALL other possible explanations for the effect need to be ruled out.
Please read more about each of these criteria at the Web Center for Social Research Methods .
The third criterion can be quite difficult to meet. To rule out ALL other possible explanations for the effect, we want to compare the world with the cause applied to the world without the cause. In practice, we do this by comparing two different groups: a “treatment” group that gets the cause applied to them, and a “control” group that does not. To rule out alternative explanations, the groups need to be “identical” with respect to every possible characteristic (aside from the treatment) that could explain differences. This way the only characteristic that will be different is that the treatment group gets the treatment and the control group doesn’t. If there are differences in the outcome, then it must be attributable to the treatment, because the other possible explanations are ruled out.
So, the key is to make the control and treatment groups “identical” when you are forming them. One thing that makes this task (slightly) easier is that they don’t have to be exactly identical, only probabilistically equivalent . This means, for example, that if you were matching groups on age that you don’t need the two groups to have identical age distributions; they would only need to have roughly the same AVERAGE age. Here roughly means “the average ages should be the same within what we expect because of sampling error.”
Now we just need to create the groups so that they have, on average, the same characteristics … for EVERY POSSIBLE CHARCTERISTIC that could explain differences in the outcome.
It turns out that creating probabilistically equivalent groups is a really difficult problem. One method that works pretty well for doing this is to randomly assign participants to the groups. This works best when you have large sample sizes, but even with small sample sizes random assignment has the advantage of at least removing the systematic bias between the two groups (any differences are due to chance and will probably even out between the groups). As Wikipedia’s page on random assignment points out,
Random assignment of participants helps to ensure that any differences between and within the groups are not systematic at the outset of the experiment. Thus, any differences between groups recorded at the end of the experiment can be more confidently attributed to the experimental procedures or treatment. … Random assignment does not guarantee that the groups are matched or equivalent. The groups may still differ on some preexisting attribute due to chance. The use of random assignment cannot eliminate this possibility, but it greatly reduces it.
We use the term internal validity to describe the degree to which cause-and-effect inferences are accurate and meaningful. Causal attribution is the goal for many researchers. Thus, by using random assignment we have a pretty high degree of evidence for internal validity; we have a much higher belief in causal inferences. Much like evidence used in a court of law, it is useful to think about validity evidence on a continuum. For example, a visualization of the internal validity evidence for a study that employed random assignment in the design might be:

The degree of internal validity evidence is high (in the upper-third). How high depends on other factors such as sample size.
To learn more about random assignment, you can read the following:
- The research report, Random Assignment Evaluation Studies: A Guide for Out-of-School Time Program Practitioners
3.6.1 Example: Does sleep deprivation cause an decrease in performance?
Let’s consider the criteria with respect to the sleep deprivation study we explored in class.
3.6.1.1 Association of cause and effect
First, we ask, Is there an association between the cause and the effect? In the sleep deprivation study, we would ask, “Is sleep deprivation associated with an decrease in performance?”
This is what a hypothesis test helps us answer! If the result is statistically significant , then we have an association between the cause and the effect. If the result is not statistically significant, then there is not sufficient evidence for an association between cause and effect.
In the case of the sleep deprivation experiment, the result was statistically significant, so we can say that sleep deprivation is associated with a decrease in performance.
3.6.1.2 Timing
Second, we ask, Did the cause come before the effect? In the sleep deprivation study, the answer is yes. The participants were sleep deprived before their performance was tested. It may seem like this is a silly question to ask, but as the link above describes, it is not always so clear to establish the timing. Thus, it is important to consider this question any time we are interested in establishing causality.
3.6.1.3 No plausible alternative explanations
Finally, we ask Are there any plausible alternative explanations for the observed effect? In the sleep deprivation study, we would ask, “Are there plausible alternative explanations for the observed difference between the groups, other than sleep deprivation?” Because this is a question about plausibility, human judgment comes into play. Researchers must make an argument about why there are no plausible alternatives. As described above, a strong study design can help to strengthen the argument.
At first, it may seem like there are a lot of plausible alternative explanations for the difference in performance. There are a lot of things that might affect someone’s performance on a visual task! Sleep deprivation is just one of them! For example, artists may be more adept at visual discrimination than other people. This is an example of a potential confounding variable. A confounding variable is a variable that might affect the results, other than the causal variable that we are interested in.
Here’s the thing though. We are not interested in figuring out why any particular person got the score that they did. Instead, we are interested in determining why one group was different from another group. In the sleep deprivation study, the participants were randomly assigned. This means that the there is no systematic difference between the groups, with respect to any confounding variables. Yes—artistic experience is a possible confounding variable, and it may be the reason why two people score differently. BUT: There is no systematic difference between the groups with respect to artistic experience, and so artistic experience is not a plausible explanation as to why the groups would be different. The same can be said for any possible confounding variable. Because the groups were randomly assigned, it is not plausible to say that the groups are different with respect to any confounding variable. Random assignment helps us rule out plausible alternatives.
3.6.1.4 Making a causal claim
Now, let’s see about make a causal claim for the sleep deprivation study:
- Association: There is a statistically significant result, so the cause is associated with the effect
- Timing: The participants were sleep deprived before their performance was measured, so the cause came before the effect
- Plausible alternative explanations: The participants were randomly assigned, so the groups are not systematically different on any confounding variable. The only systematic difference between the groups was sleep deprivation. Thus, there are no plausible alternative explanations for the difference between the groups, other than sleep deprivation
Thus, the internal validity evidence for this study is high, and we can make a causal claim. For the participants in this study, we can say that sleep deprivation caused a decrease in performance.
Key points: Causation and internal validity
To make a cause-and-effect inference, you need to consider three criteria:
- Association of the Cause and Effect: There needs to be a association between the cause and effect. This can be established by a hypothesis test.
Random assignment removes any systematic differences between the groups (other than the treatment), and thus helps to rule out plausible alternative explanations.
Internal validity describes the degree to which cause-and-effect inferences are accurate and meaningful.
Confounding variables are variables that might affect the results, other than the causal variable that we are interested in.
Probabilistic equivalence means that there is not a systematic difference between groups. The groups are the same on average.
How can we make "equivalent" experimental groups?

An official website of the United States government
The .gov means it’s official. Federal government websites often end in .gov or .mil. Before sharing sensitive information, make sure you’re on a federal government site.
The site is secure. The https:// ensures that you are connecting to the official website and that any information you provide is encrypted and transmitted securely.
- Publications
- Account settings
Preview improvements coming to the PMC website in October 2024. Learn More or Try it out now .
- Advanced Search
- Journal List
- J Hum Reprod Sci
- v.4(1); Jan-Apr 2011
This article has been retracted.
An overview of randomization techniques: an unbiased assessment of outcome in clinical research.
Department of Biostatics, National Institute of Animal Nutrition & Physiology (NIANP), Adugodi, Bangalore, India
Randomization as a method of experimental control has been extensively used in human clinical trials and other biological experiments. It prevents the selection bias and insures against the accidental bias. It produces the comparable groups and eliminates the source of bias in treatment assignments. Finally, it permits the use of probability theory to express the likelihood of chance as a source for the difference of end outcome. This paper discusses the different methods of randomization and use of online statistical computing web programming ( www.graphpad.com /quickcalcs or www.randomization.com ) to generate the randomization schedule. Issues related to randomization are also discussed in this paper.
INTRODUCTION
A good experiment or trial minimizes the variability of the evaluation and provides unbiased evaluation of the intervention by avoiding confounding from other factors, which are known and unknown. Randomization ensures that each patient has an equal chance of receiving any of the treatments under study, generate comparable intervention groups, which are alike in all the important aspects except for the intervention each groups receives. It also provides a basis for the statistical methods used in analyzing the data. The basic benefits of randomization are as follows: it eliminates the selection bias, balances the groups with respect to many known and unknown confounding or prognostic variables, and forms the basis for statistical tests, a basis for an assumption of free statistical test of the equality of treatments. In general, a randomized experiment is an essential tool for testing the efficacy of the treatment.
In practice, randomization requires generating randomization schedules, which should be reproducible. Generation of a randomization schedule usually includes obtaining the random numbers and assigning random numbers to each subject or treatment conditions. Random numbers can be generated by computers or can come from random number tables found in the most statistical text books. For simple experiments with small number of subjects, randomization can be performed easily by assigning the random numbers from random number tables to the treatment conditions. However, in the large sample size situation or if restricted randomization or stratified randomization to be performed for an experiment or if an unbalanced allocation ratio will be used, it is better to use the computer programming to do the randomization such as SAS, R environment etc.[ 1 – 6 ]
REASON FOR RANDOMIZATION
Researchers in life science research demand randomization for several reasons. First, subjects in various groups should not differ in any systematic way. In a clinical research, if treatment groups are systematically different, research results will be biased. Suppose that subjects are assigned to control and treatment groups in a study examining the efficacy of a surgical intervention. If a greater proportion of older subjects are assigned to the treatment group, then the outcome of the surgical intervention may be influenced by this imbalance. The effects of the treatment would be indistinguishable from the influence of the imbalance of covariates, thereby requiring the researcher to control for the covariates in the analysis to obtain an unbiased result.[ 7 , 8 ]
Second, proper randomization ensures no a priori knowledge of group assignment (i.e., allocation concealment). That is, researchers, subject or patients or participants, and others should not know to which group the subject will be assigned. Knowledge of group assignment creates a layer of potential selection bias that may taint the data.[ 9 ] Schul and Grimes stated that trials with inadequate or unclear randomization tended to overestimate treatment effects up to 40% compared with those that used proper randomization. The outcome of the research can be negatively influenced by this inadequate randomization.
Statistical techniques such as analysis of covariance (ANCOVA), multivariate ANCOVA, or both, are often used to adjust for covariate imbalance in the analysis stage of the clinical research. However, the interpretation of this post adjustment approach is often difficult because imbalance of covariates frequently leads to unanticipated interaction effects, such as unequal slopes among subgroups of covariates.[ 1 ] One of the critical assumptions in ANCOVA is that the slopes of regression lines are the same for each group of covariates. The adjustment needed for each covariate group may vary, which is problematic because ANCOVA uses the average slope across the groups to adjust the outcome variable. Thus, the ideal way of balancing covariates among groups is to apply sound randomization in the design stage of a clinical research (before the adjustment procedure) instead of post data collection. In such instances, random assignment is necessary and guarantees validity for statistical tests of significance that are used to compare treatments.
TYPES OF RANDOMIZATION
Many procedures have been proposed for the random assignment of participants to treatment groups in clinical trials. In this article, common randomization techniques, including simple randomization, block randomization, stratified randomization, and covariate adaptive randomization, are reviewed. Each method is described along with its advantages and disadvantages. It is very important to select a method that will produce interpretable and valid results for your study. Use of online software to generate randomization code using block randomization procedure will be presented.
Simple randomization
Randomization based on a single sequence of random assignments is known as simple randomization.[ 3 ] This technique maintains complete randomness of the assignment of a subject to a particular group. The most common and basic method of simple randomization is flipping a coin. For example, with two treatment groups (control versus treatment), the side of the coin (i.e., heads - control, tails - treatment) determines the assignment of each subject. Other methods include using a shuffled deck of cards (e.g., even - control, odd - treatment) or throwing a dice (e.g., below and equal to 3 - control, over 3 - treatment). A random number table found in a statistics book or computer-generated random numbers can also be used for simple randomization of subjects.
This randomization approach is simple and easy to implement in a clinical research. In large clinical research, simple randomization can be trusted to generate similar numbers of subjects among groups. However, randomization results could be problematic in relatively small sample size clinical research, resulting in an unequal number of participants among groups.
Block randomization
The block randomization method is designed to randomize subjects into groups that result in equal sample sizes. This method is used to ensure a balance in sample size across groups over time. Blocks are small and balanced with predetermined group assignments, which keeps the numbers of subjects in each group similar at all times.[ 1 , 2 ] The block size is determined by the researcher and should be a multiple of the number of groups (i.e., with two treatment groups, block size of either 4, 6, or 8). Blocks are best used in smaller increments as researchers can more easily control balance.[ 10 ]
After block size has been determined, all possible balanced combinations of assignment within the block (i.e., equal number for all groups within the block) must be calculated. Blocks are then randomly chosen to determine the patients’ assignment into the groups.
Although balance in sample size may be achieved with this method, groups may be generated that are rarely comparable in terms of certain covariates. For example, one group may have more participants with secondary diseases (e.g., diabetes, multiple sclerosis, cancer, hypertension, etc.) that could confound the data and may negatively influence the results of the clinical trial.[ 11 ] Pocock and Simon stressed the importance of controlling for these covariates because of serious consequences to the interpretation of the results. Such an imbalance could introduce bias in the statistical analysis and reduce the power of the study. Hence, sample size and covariates must be balanced in clinical research.
Stratified randomization
The stratified randomization method addresses the need to control and balance the influence of covariates. This method can be used to achieve balance among groups in terms of subjects’ baseline characteristics (covariates). Specific covariates must be identified by the researcher who understands the potential influence each covariate has on the dependent variable. Stratified randomization is achieved by generating a separate block for each combination of covariates, and subjects are assigned to the appropriate block of covariates. After all subjects have been identified and assigned into blocks, simple randomization is performed within each block to assign subjects to one of the groups.
The stratified randomization method controls for the possible influence of covariates that would jeopardize the conclusions of the clinical research. For example, a clinical research of different rehabilitation techniques after a surgical procedure will have a number of covariates. It is well known that the age of the subject affects the rate of prognosis. Thus, age could be a confounding variable and influence the outcome of the clinical research. Stratified randomization can balance the control and treatment groups for age or other identified covariates. Although stratified randomization is a relatively simple and useful technique, especially for smaller clinical trials, it becomes complicated to implement if many covariates must be controlled.[ 12 ] Stratified randomization has another limitation; it works only when all subjects have been identified before group assignment. However, this method is rarely applicable because clinical research subjects are often enrolled one at a time on a continuous basis. When baseline characteristics of all subjects are not available before assignment, using stratified randomization is difficult.[ 10 ]
Covariate adaptive randomization
One potential problem with small to moderate size clinical research is that simple randomization (with or without taking stratification of prognostic variables into account) may result in imbalance of important covariates among treatment groups. Imbalance of covariates is important because of its potential to influence the interpretation of a research results. Covariate adaptive randomization has been recommended by many researchers as a valid alternative randomization method for clinical research.[ 8 , 13 ] In covariate adaptive randomization, a new participant is sequentially assigned to a particular treatment group by taking into account the specific covariates and previous assignments of participants.[ 7 ] Covariate adaptive randomization uses the method of minimization by assessing the imbalance of sample size among several covariates.
Using the online randomization http://www.graphpad.com/quickcalcs/index.cfm , researcher can generate randomization plan for treatment assignment to patients. This online software is very simple and easy to implement. Up to 10 treatments can be allocated to patients and the replication of treatment can also be performed up to 9 times. The major limitations of this software is that once the randomization plan is generated, same randomization plan cannot be generated as this uses the seed point of local computer clock and is not displayed for further use. Other limitation of this online software Maximum of only 10 treatments can be assigned to patients. Entering the web address http://www.graphpad.com/quickcalcs/index.cfm on address bar of any browser, the page of graphpad appears with number of options. Select the option of “Random Numbers” and then press continue, Random Number Calculator with three options appears. Select the tab “Randomly assign subjects to groups” and press continue. In the next page, enter the number of subjects in each group in the tab “Assign” and select the number of groups from the tab “Subjects to each group” and keep number 1 in repeat tab if there is no replication in the study. For example, the total number of patients in a three group experimental study is 30 and each group will assigned to 10 patients. Type 10 in the “Assign” tab and select 3 in the tab “Subjects to each group” and then press “do it” button. The results is obtained as shown as below (partial output is presented)
Another randomization online software, which can be used to generate randomization plan is http://www.randomization.com . The seed for the random number generator[ 14 , 15 ] (Wichmann and Hill, 1982, as modified by McLeod, 1985) is obtained from the clock of the local computer and is printed at the bottom of the randomization plan. If a seed is included in the request, it overrides the value obtained from the clock and can be used to reproduce or verify a particular plan. Up to 20 treatments can be specified. The randomization plan is not affected by the order in which the treatments are entered or the particular boxes left blank if not all are needed. The program begins by sorting treatment names internally. The sorting is case sensitive, however, so the same capitalization should be used when recreating an earlier plan. Example of 10 patients allocating to two groups (each with 5 patients), first the enter the treatment labels in the boxes, and enter the total number of patients that is 10 in the tab “Number of subjects per block” and enter the 1 in the tab “Number of blocks” for simple randomization or more than one for Block randomization. The output of this online software is presented as follows.
The benefits of randomization are numerous. It ensures against the accidental bias in the experiment and produces comparable groups in all the respect except the intervention each group received. The purpose of this paper is to introduce the randomization, including concept and significance and to review several randomization techniques to guide the researchers and practitioners to better design their randomized clinical trials. Use of online randomization was effectively demonstrated in this article for benefit of researchers. Simple randomization works well for the large clinical trails ( n >100) and for small to moderate clinical trials ( n <100) without covariates, use of block randomization helps to achieve the balance. For small to moderate size clinical trials with several prognostic factors or covariates, the adaptive randomization method could be more useful in providing a means to achieve treatment balance.
Source of Support: Nil
Conflict of Interest: None declared.
The Effect of Mindset Interventions on Stress and Academic Motivation in College Students
- Open access
- Published: 10 April 2024
Cite this article
You have full access to this open access article
- Heidi H. Meyer 1 &
- Lauren A. Stutts ORCID: orcid.org/0000-0002-5014-1907 1
241 Accesses
6 Altmetric
Explore all metrics
Stress levels are high among college students in the United States. Growth mindset and stress-is-enhancing mindset interventions offer ways to reduce stress, but minimal research has examined them. This study’s aim was to examine the effect of mindset interventions on mindsets, stress, academic motivation, and responses to hypothetical academic scenarios. Participants included 210 college students who were randomized to one of four groups: growth mindset (intelligence is malleable), stress mindset (stress is beneficial), synergistic (intelligence is malleable, and stress is beneficial), or control (brain functions). The growth mindset and the synergistic mindset group increased in growth mindset, and the growth mindset group had higher growth mindset than the stress mindset and control group post-intervention. The stress mindset and the synergistic group increased in stress-is-enhancing mindset, and both groups had higher stress-is-enhancing mindsets than the growth mindset and control group post-intervention. All groups decreased in stress and increased in academic motivation. The synergistic group was the only group to improve on all the main outcomes, and students in this group were less likely to want to withdraw from a course in both negative hypothetical academic scenarios (if they failed an assignment or were faced with a professor with a fixed mindset). Our findings suggest that students would benefit from increased access to mindset interventions.
Similar content being viewed by others
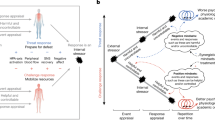
A synergistic mindsets intervention protects adolescents from stress
David S. Yeager, Christopher J. Bryan, … Jeremy P. Jamieson
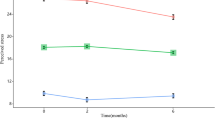
Trajectories of Perceived Stress among Students in Transition to College: Mindset Antecedents and Adjustment Outcomes
Shan Zhao, Yanjia Zhang, … Danhua Lin
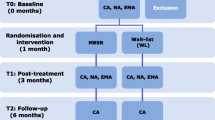
Study protocol for a randomised controlled trial investigating the effects of Mindfulness Based Stress Reduction on stress regulation and associated neurocognitive mechanisms in stressed university students: the MindRest study
Nikos Kogias, Dirk E. M. Geurts, … Erno J. Hermans
Avoid common mistakes on your manuscript.
The American College Health Association ( 2022 ) reported that approximately 50% of college students in the United States (U.S.) reported experiencing a moderate level of stress, and approximately 30% of students reported experiencing a high level of stress. Stress has been found to be strongly associated with psychological disorders (e.g., depression) and suicidality in college students (Liu et al., 2019 ). Therefore, it is critical to examine what factors are related to stress and how stress can be reduced.
One key factor that influences stress and resilience is one’s mindset (Yeager & Dweck, 2012 ). Mindsets are defined as one’s implicit theories about the flexibility of characteristics such as intelligence. There are two main types of mindsets regarding intelligence: growth and fixed (Dweck & Leggett, 1988 ). A growth mindset believes intelligence can be improved through effort, while a fixed mindset believes that intelligence is set and cannot change despite someone’s effort. Individuals are theorized to be on a continuum between these two mindsets. A meta-analysis found that growth mindsets are negatively correlated with psychological distress and positively correlated with active coping (Burnette et al., 2020 ). This association may be because a growth mindset buffers against maladaptive cognitions that can lead to maladaptive coping and/or adverse psychological outcomes.
A growth mindset is a skill that can be learned through interventions. For example, Smith and Capuzzi ( 2019 ) examined the effect of a single-session growth mindset intervention on anxiety and grades for U.S. college students in a statistics class (Smith & Capuzzi, 2019 ). They randomly assigned students to either a growth mindset intervention (e.g., a 75-minute interactive class session with exercises) or a control group (no intervention). They measured the students’ mindset and anxiety level at baseline and six months after completing the intervention. They found that students in the intervention group had an increased growth mindset, whereas mindset did not change in the control group. While they did not find significant changes in anxiety in either group, they found that a higher growth mindset was associated with lower anxiety and a higher course grade. Another study found that a single-session growth mindset intervention reduced depressive symptoms in U.S. adolescent girls four months after the intervention (Heaman et al., 2023 ).
Furthermore, a growth mindset is related to positive educational outcomes. A meta-analysis across 10 studies concluded that teaching students about a growth mindset increases their motivation and achievement (Sarrasin et al., 2018 ). For example, one study randomized adolescents in the U.S. to a growth mindset group (8-session intervention with information about the brain and growth mindset) or a control group (8-session intervention with information about the brain) (Blackwell et al., 2007 ). Students in the growth mindset group had significantly more positive change in classroom motivation three weeks after the intervention than the control group.
While a growth mindset is associated with lower stress (Burnette et al., 2020 ), two mindsets directly related to stress have been studied as well: stress-is-enhancing and stress-is-debilitating (Crum et al., 2013 ). A stress-is-enhancing mindset views stress as beneficial, whereas a stress-is-debilitating mindset views stress as harmful (Crum et al., 2013 ). Similar to growth and fixed mindsets, individuals are thought to be on a continuum between these two mindsets. Keech et al. ( 2018 ) found that having a stress-is-enhancing mindset was negatively correlated with perceived stress and was positively correlated with proactive behavior. Another study found that a stress-is-enhancing mindset served as a moderator between perceived stress and depressive symptoms in U.S. college students (Huebschmann & Sheets, 2020 ). The stress mindset is theorized to be beneficial in part because it changes how one psychologically experiences stress and how one behaviorally copes with stress (Crum et al., 2013 ).
Similar to a growth mindset, a stress-is-enhancing mindset can be developed through interventions. For example, one study with Australian university students found significant benefits of a stress mindset intervention (Keech et al., 2021 ). Students were randomized to one of two groups: an intervention group who watched videos about the consequences and benefits of stress and completed mental imagery tasks applying the benefits of stress in their own lives (one session of an unspecified length of time), or a control group who completed a mental imagery task unrelated to reframing stress. Two weeks after the intervention, the intervention group increased in stress-is-enhancing mindset from pre- to post-intervention and had a higher stress-is-enhancing mindset than the control group. In participants with high perceived stress, those in the intervention group also had lower distress and more proactive behavior than the control group. Another study using college students in the U.K. found that short videos about the stress-is-enhancing mindset was effective in increasing the stress-is-enhancing mindset immediately after the intervention (Williams & Ginty, 2023 ). However, no previous studies to our knowledge have examined the effect of a stress-is-enhancing intervention on academic motivation.
Yeager et al. ( 2022 ) proposed a mindset that combines the growth mindset and stress-is-enhancing mindset, which they called a synergistic mindset. They created a single-session 30-minute online module that contained information and exercises related to improving both mindsets. In a sample of U.S. college students, they found that this synergistic mindset intervention was more effective than a growth mindset intervention alone, a stress-is-enhancing mindset intervention alone, or a control intervention (learned about brain functions) in reducing cardiovascular reactivity when experiencing a social stressor shortly after the intervention (Yeager et al., 2022 ). In another sample of college students who received the intervention, they found that students made less negative stress appraisals about a quiz one to three days after the intervention and three weeks afterward (Yeager et al., 2022 ).
Having single-session interventions can be valuable as they are time- and cost-effective, but it’s important that they have lasting effects. A meta-analysis on single-session interventions on psychiatric problems in adolescents found that the interventions had the highest effect size for lasting effects on anxiety, which is similar to the present study’s focus on stress in young adults (Schleider & Weisz, 2017 ). Another study specifically examined the longitudinal effects of a single-session synergistic mindset intervention (Hecht et al., 2023 ). At the beginning of the semester, U.S. college students were randomly assigned to receive a 30-minute synergistic mindset or a control intervention (lesson about the brain). Subsequently, half of the students in each group received four 5-minute supportive messages related to the synergistic mindset whereas the other half received 5-minute neutral messages about assessing their learning progress periodically over the remainder of the semester (15-week semester). They found that the synergistic mindset intervention was effective in reducing fixed mindset beliefs and increasing stress-is-enhancing beliefs immediately after the intervention and at 3-weeks post-intervention similar to previous findings (Yeager et al., 2022 ); however, they found that receiving brief continued supportive messages made the initial intervention’s effects stronger over time (Hecht et al., 2023 ). Overall, single-session interventions are promising low-cost, accessible options that need further exploration.
Present Study
The present study is novel in two main ways. First, we examined the impact of mindset interventions on academic motivation and on responses to hypothetical academic scenarios to examine how mindsets could affect one’s potential behaviors, which has not been explored previously to our knowledge. Second, we assessed the efficacy of a shorter and simpler intervention (5 to 10 min) than was used previously (Yeager et al., 2022 ), which could make the intervention even more accessible for students.
Our main study aim was to assess the effect of mindset interventions on growth mindset, stress-is-enhancing mindset, stress, and academic motivation. We randomly assigned participants to one of four groups: growth mindset, stress mindset, synergistic mindset, or control. Participants’ growth mindset, stress-is-enhancing mindset, stress, and academic motivation were measured before and after the intervention. We hypothesized that the synergistic group would have increased growth mindset, stress-is-enhancing mindset, and academic motivation, and decreased stress from pre- to post-intervention. We also predicted that the synergistic group would have lower stress and higher academic motivation post-intervention compared to the other groups. In contrast, we predicted that the control group would have lower growth mindset, stress-is-enhancing mindset, and academic motivation and higher stress compared to the other groups post-intervention. Our second aim was to assess the effect of mindset interventions on responses to hypothetical academic scenarios to examine how mindsets could affect one’s potential behaviors. We did not create hypotheses for the second aim as it was exploratory.
Participants
Any currently enrolled students at a southeastern college who were at least 18 years of age were eligible to participate in the study. According to a G*power analysis using an effect size f 2 of 0.25, a power level of 0.80, and a significance value of 0.05, 136 participants were required for the main analysis (2 × 4 mixed ANOVA) to have sufficient statistical power (Faul et al., 2009 ). Although 272 participants began the survey and provided consent, we removed data from 62 participants because they stopped the study before finishing the pre-post measures ( n = 58), or they did not follow the instructions in their written responses ( n = 4). The majority of individuals who stopped early quit on the first survey ( n = 26) or during the intervention ( n = 21). There was not a clear trend that one intervention resulted in more drop-out than others ( n = 4 growth mindset; n = 4 synergistic; n = 6 stress-is-enhancing mindset; n = 7 control group. Our final sample contained 210 participants, which was sufficient for our analyses.
We received approval from the Institutional Review Board of the college and collected data in January 2023 online. Participants were recruited through a campus-wide email. After indicating consent, participants completed a baseline stress scale. Next, they completed measures of growth mindset, stress-is-enhancing mindset, state stress, and academic motivation. Subsequently, they were randomly assigned to one of four groups: growth mindset, stress mindset, synergistic mindset, or control. All groups completed three activities designed to shift their mindset to the group they were assigned. After completing the activities, participants completed the same assessments of growth mindset, stress-is-enhancing mindset, state stress, and academic motivation. Subsequently, participants responded to behavioral questions about hypothetical academic scenarios. Finally, participants completed demographic information. The study took approximately 17 min, and each participant was compensated with a $10 Amazon gift card.
Group Interventions
Our interventions were modeled after Yeager et al. ( 2022 ) but were adapted to be shorter and simpler. Each group first read research on their assigned mindset. The growth mindset group read about the malleability of the brain. The stress mindset group read about the adaptive purposes of stress. The synergistic mindset group read about both of those topics, and the control group read about the functional areas of the brain. Each group then read practical strategies about how to implement the mindsets in their life. Next, participants read an example story about how a student applied the assigned mindset to an academic challenge. Finally, participants were asked to write a short paragraph about how they planned to use a similar mindset and strategies on a future academic setback/challenge. They were prompted to write at least 500 characters (100 words). Each group’s procedure is detailed in the Supplemental Materials.
Baseline Stress
Baseline stress was measured with the Perceived Stress Scale, which asked participants questions to rate their stress levels over the past month (Cohen et al., 1983 ). Participants rated 10 items (e.g., “In the last month, how often have you been upset because of something that happened unexpectedly?”) on a scale from 0 ( never ) to 4 ( very often ). After reverse scoring the necessary items, a total score was calculated. The possible total score ranged from 0 to 40, with higher scores representing more stress. Cronbach’s α was 0.86.
Pre-Post Measures
Growth mindset.
The Growth Mindset Scale assessed whether participants believe they can learn and improve (Dweck et al., 1995 ). Participants indicated their agreement with three items (e.g., “You have a certain amount of intelligence, and you can’t really change it”) on a Likert scale from 1 ( strongly agree ) to 6 ( strongly disagree ). Items were reverse-scored and averaged; higher scores indicated a higher growth mindset. Cronbach’s α was 0.88 for pre- and post-intervention.
Stress Mindset
The Stress Mindset Measure assessed whether participants view stress as enhancing or debilitating (Crum et al., 2013 ). Participants indicated their agreement to eight items (e.g., “Experiencing stress facilitates my learning and growth”) on a Likert scale from 0 ( strongly disagree ) to 4 ( strongly agree ). Scores were averaged, and higher scores indicated a higher stress-is-enhancing mindset. Cronbach’s α was 0.83 for pre-intervention and 0.88 for post-intervention.
State Stress
State stress was measured through a visual analog scale where participants indicated how much they felt stress currently from 0 ( not at all ) to 100 ( extremely ).
Academic Motivation
Academic motivation was measured through a visual analog scale where participants indicated how motivated they felt to complete their coursework currently from 0 ( not at all ) to 100 ( extremely ).
Academic Scenarios
Participants read and responded to three hypothetical academic scenarios. The first scenario involved failing an assignment: “Imagine you fail the first assignment of the semester after completing the work and trying your best.” The second scenario included a professor’s fixed mindset response: “Imagine that you talk to the professor about your failed assignment. Your professor tries to make you feel better by telling you that this subject is hard and isn’t for everyone.” The third scenario demonstrated a professor’s growth mindset response: “Imagine that you talk to the professor about your failed assignment. Your professor tries to make you feel better by telling you that they will help you, and they believe in your ability to improve.” In each scenario, participants were asked to rate how much they would want to withdraw from the course if they could on a visual analogue scale from 0 ( not at all ) to 100 ( extremely ).
Demographic Questions
Participants reported their age, gender, race, ethnicity, and class year.
Manipulation Check
We examined all the written responses to the interventions to make sure they followed instructions of their group assignment. We removed four individuals for not following those instructions.
Statistical Analyses
SPSS version 28 was used for all analyses. Descriptive statistics and reliability analyses were calculated for all main variables. Chi-squared analyses were conducted to assess if there were differences in demographic variables among the four groups. A one-way (group) ANOVA was conducted for baseline stress to determine any baseline differences among the four groups. For aim 1, we conducted 2 (time) x 4 (group) mixed ANOVAs on growth-mindset, stress-is-enhancing mindset, stress, and academic motivation. For aim 2, we conducted one-way between-subjects (4 groups) ANOVAs on desire to withdraw from a course based on three hypothetical scenarios. Post-hoc tests using Bonferroni were conducted to determine where the significant differences occurred.
Descriptive Statistics of Participants
Descriptive statistics including gender, race, ethnicity, class year, and age for participants are displayed in Table 1 . Most participants were White, non-Hispanic/Latinx women. The mean age of participants was 20 years old and ranged from 18 to 22. There were no significant differences by demographic variables among the four groups (Table 1 ).
There was a significant interaction between time and group for growth mindset, F (3, 206) = 10.65, p < .001, η p 2 = 0.13 (Fig. 1 ). Growth mindset significantly increased from pre-intervention ( M = 4.21, SD = 1.05) to post-intervention ( M = 4.67, SD = 1.06) in the synergistic group, p < .001. Additionally, growth mindset significantly increased from pre-intervention ( M = 4.14, SD = 1.05) to post-intervention ( M = 4.78, SD = 1.07) in the growth mindset group, p < .001. At post-intervention, the growth mindset group ( M = 4.78, SD = 1.07) had significantly higher growth mindset than the control group ( M = 4.18, SD = 1.06), p = .028, and the stress mindset group ( M = 4.22, SD = 1.06), p = .048.
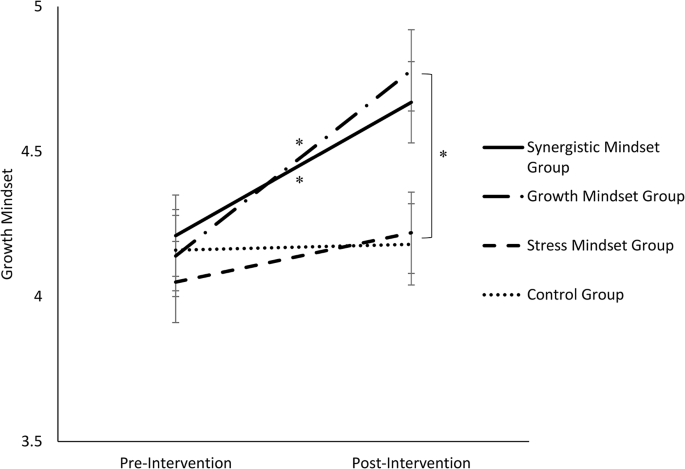
Growth Mindset by Group and Time. Note. Vertical axis was abridged for clarity. The bracket indicates there is a significant difference between the growth mindset group and the stress mindset and control group. Error bars represent standard error. * p < .05
Stress-is-Enhancing Mindset
There was a significant interaction between time and group for stress-is-enhancing mindset, F (3, 206) = 17.74, p < .001, η p 2 = 0.21 (Fig. 2 ). Stress-is-enhancing mindset significantly increased from pre-intervention ( M = 1.76, SD = 0.67) to post-intervention ( M = 2.18, SD = 0.66) in the synergistic group, p < .001. Additionally, stress-is-enhancing mindset significantly increased from pre-intervention ( M = 1.72, SD = 0.65) to post-intervention ( M = 2.25, SD = 0.63) in the stress mindset group, p < .001. At post-intervention, the synergistic group ( M = 2.18, SD = 0.69) had significantly higher stress-is-enhancing mindset than the control group ( M = 1.82, SD = 0.69), p = .048, and the growth mindset group ( M = 1.73, SD = 0.69), p = .005. Also at post-intervention, the stress mindset group ( M = 2.25, SD = 0.69) had significantly higher stress-is-enhancing mindset than the control group ( M = 1.82, SD = 0.69), p = .011, and the growth mindset group ( M = 1.73, SD = 0.69), p < .001.
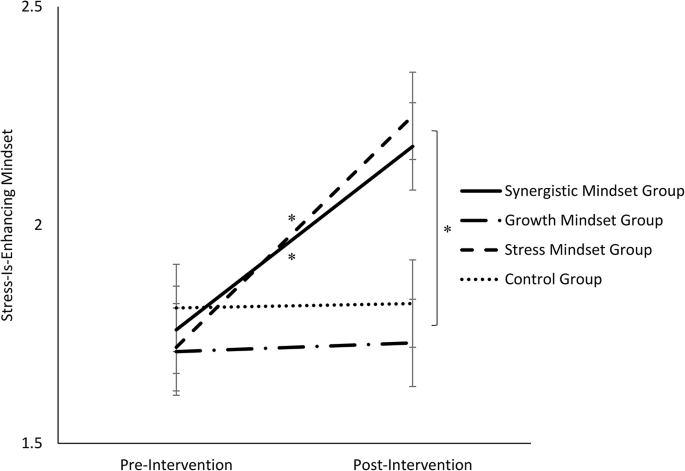
Stress Mindset by Group and Time. Note. Vertical axis was abridged for clarity. The bracket indicates there is a significant difference between both the synergistic and stress mindset group and the growth mindset and control group. Error bars represent standard error. * p < .01
There was a significant main effect by time, F (1, 206) = 21.80, p < .001, η p 2 = 0.10, such that stress decreased across all groups from pre-exposure ( M = 46.41, SD = 23.59) to post-exposure ( M = 41.76, SD = 25.04), p < .001 (Fig. 3 ). However, there was not a significant main effect by group, F (3, 206) = 0.37, p = .77, η p 2 = 0.005, or a significant interaction by time and group, F (3, 206) = 1.42, p = .24, η p 2 = 0.02.
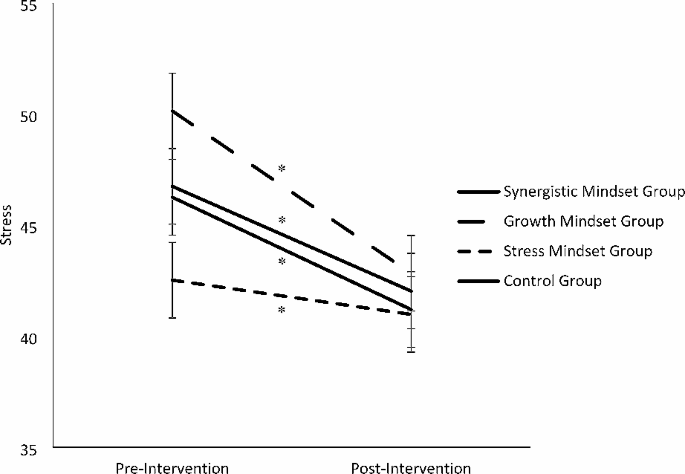
Stress by Group and Time. Note. Vertical axis was abridged for clarity. Error bars represent standard error. * p < .01
There was a significant main effect by time, F (1, 206) = 13.74, p < .001, η p 2 = 0.063, such that academic motivation increased across all groups from pre-exposure ( M = 51.74, SD = 25.94) to post-exposure ( M = 55.19, SD = 25.98) (Fig. 4 ). However, there was not a significant main effect by group, F (3, 206) = 0.31, p = .82, η p 2 = 0.004, or a significant interaction by time and group, F (3, 206) = 0.05, p = .99, η p 2 = 0.001.
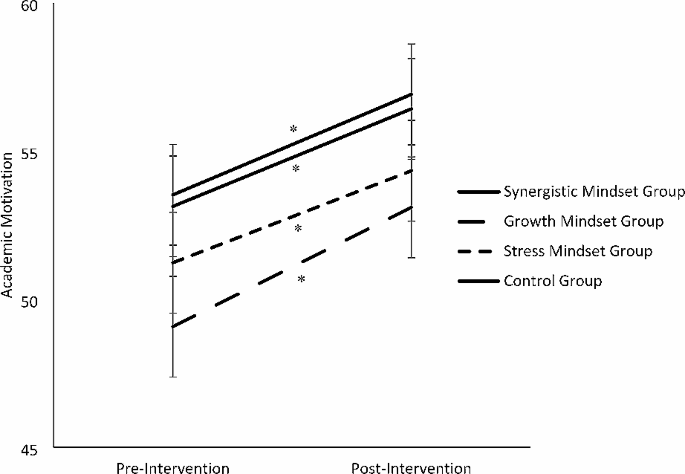
Academic Motivation by Group and Time. Note. Vertical axis was abridged for clarity. Error bars represent standard error. * p < .01
There was a significant main effect for group about wanting to withdraw from a course if they could when they failed the first assignment after trying their best, F (3, 206) = 5.99, p < .001, η p 2 = 0.08. The synergistic group ( M = 40.54, SD = 33.60) had a significantly lower likelihood of wanting to withdraw from the course compared to the control group ( M = 67.15, SD = 33.59), p < .001. In addition, the stress mindset group ( M = 46.22, SD = 33.64) had a significantly lower likelihood of wanting to withdraw from the course compared to the control group ( M = 67.15, SD = 33.59), p = .01.
There was also a significant main effect for group about wanting to withdraw when participants were faced with a professor with a fixed mindset, F (3, 206) = 2.88, p = .037, η p 2 = 0.04. Participants in the synergistic group ( M = 51.37, SD = 35.52) were significantly less likely to want to withdraw from the course than those in the control group ( M = 71.48, SD = 35.57), p = .03. When a professor had a growth mindset, participants did not differ significantly in their likelihood to want to withdraw from the course based on their mindset group, F (3, 206) = 1.08, p = .36, η p 2 = 0.02.
This study’s main aim was to examine the effect of mindset interventions on mindsets, state stress, and academic motivation. Our hypotheses were partially supported. Each mindset intervention improved mindsets as intended. In addition, state stress decreased, and academic motivation increased from pre- to post-intervention in the mindset groups as predicted, but we did not predict that state stress would decrease and academic motivation would increase in the control group as well. In addition, we did not find that the synergistic group had better outcomes compared to the other three groups for stress and academic motivation. The study’s second aim was to examine the effect of the mindset interventions on hypothetical academic scenarios. We found that students in the synergistic group were the only students who were less likely to want to withdraw from a course in both negative hypothetical academic scenarios (if they failed an assignment or were faced with a professor with a fixed mindset), suggesting the benefits of the synergistic mindset.
Our results showed that the growth mindset intervention (in both the growth mindset group and synergistic group) was successful in increasing a growth mindset. This finding aligns with previous research (Smith & Capuzzi, 2019 ). However, our study also found that the growth mindset intervention reduced stress, whereas Smith and Capuzzi ( 2019 ) did not find a change in anxiety, though they found a negative relationship between growth mindset and anxiety. Perhaps the difference in the construct of anxiety versus stress and the different measures used in those studies played a role in those varying results. However, our study’s intervention was notably shorter (5 to 10 min) than Smith and Capuzzi’s ( 2019 ) 75-minute intervention, which suggests that a growth mindset can be induced more efficiently. Moreover, we found that the growth mindset intervention increased academic motivation, which parallels previous research (Blackwell et al., 2007 ; Sarrasin et al., 2018 ). That said, it is important to note that while participants in the growth mindset group had significantly higher growth mindset than the control group, there were not differences between the groups for stress and academic motivation, indicating a lack of treatment effect for those variables. That lack of difference may be because those variables were more distal outcomes. A meta-analysis found that the largest effects for growth mindset interventions are on more proximal outcomes such as mindset than more distal outcomes such as psychological health (Burnette et al., 2023 ).
Similarly, we found that the stress mindset intervention (in both the stress mindset group and synergistic group) was successful in increasing a stress-is-enhancing mindset. This finding is in concert with previous research (Keech et al., 2021 ). Furthermore, we found that stress decreased in those groups, whereas Keech et al. ( 2021 ) only found decreased stress in participants who had overall higher stress than the other participants. Our sample had high averages of baseline stress across all groups, which may be why we found an overall difference for everyone. We also found that the stress mindset intervention increased academic motivation, which has not been studied previously to our knowledge. Perhaps rethinking academic stress reminded students of the benefits of learning rather than viewing it as threatening, which may have led to an increased desire to complete academic work. However, similar to growth mindset, we only found differences between the stress mindset group and the control group for the proximal outcome of stress-is-enhancing mindset, which indicates there was not a treatment effect for stress and academic motivation.
The control group did not change in growth mindset or stress-is-enhancing mindset over time, which aligns with expectations. Surprisingly, though, the control group had decreased stress and increased academic motivation after learning about the brain, despite us using a similar control group design as Yeager et al. ( 2022 ). It is possible that learning about the function of different brain parts and writing about them led to increased feelings of appreciation of one’s brain. Completing this activity also served as a break from their academic work, which may also have led to students feeling less stressed and more energized for their work.
While the synergistic group was the only group to have improvements in all four outcomes, the synergistic group did not show significantly better results in stress reduction and academic motivation than the other groups. Therefore, it appears that learning about both mindsets does not necessarily have additive effects as they potentially affect stress and academic motivation in a similar way. However, the mechanisms of how those mindsets are related to different outcomes needs to be explored further.
For our second aim, we found that the interventions affected projected behavioral responses to hypothetical academic scenarios. The synergistic and stress mindset groups were significantly less likely to want to withdraw from a course compared to the control group when faced with failing the first assignment in a course after trying their best. Perhaps they were applying what they learned and practiced in the intervention to view the failure as a challenge rather than a stressor.
In addition, participants in the synergistic group were less likely to want to withdraw from a course compared to the control group when faced with a professor who expressed a fixed mindset. This finding is in line with previous research that shows that a professor’s mindset can affect students’ course engagement and dropout intentions (Muenks et al., 2020 ). The synergistic group may have applied both ideas from the growth mindset and stress mindset intervention; they may have trusted their own mindset rather than the professor’s mindset and reframed the situation as a challenge rather than a stressor. These behavioral responses suggest that the synergistic group may confer benefits above and beyond the single mindset groups.
Our sample had multiple limitations regarding participants. For example, the sample was mostly white, educated women, which limits the generalizability of our findings. The demographics of this study’s sample also could have influenced the familiarity of participants with the concepts taught. For example, the concept of a growth mindset is now commonly taught in many college courses, especially psychology courses. Therefore, participants may have already been aware of some of the research about mindsets.
Furthermore, the intervention was limited in several ways. We do not know if participants read and absorbed each piece of information. In addition, twenty-one participants quit the study during the intervention, which could indicate that they were not interested in it or engaged with it. There was not a clear difference in drop-out across intervention groups, so it’s possible it was due to all of them requiring writing. However, further research is needed to determine why some individuals would choose not to continue this type of intervention. A few individuals also had negative responses to the stress mindset intervention as they thought that it was invalidating to the negative impact of stressors. Yeager et al. ( 2022 ) highlighted that mindset interventions are not meant for reappraising traumatic stressors; therefore, it’s possible that the individuals who had a negative response may have had those types of experiences and felt invalidated. In turn, feeling emotionally invalidated has been found to predict negative affect and stress (Schreiber & Veilleux, 2022 ; Zielinski et al., 2023 ). Recent research has discovered that applying a meta-cognitive approach that highlights both the stress-is-enhancing mindset and addresses how to handle society’s messaging around the negative aspects of stress was more effective than a stress-is-enhancing mindset intervention alone (Crum et al., 2023 ). Future research should continue to explore how these interventions can balance providing the benefit of mindset change while not invalidating an individual’s stress.
Moreover, there were limitations to our measures and the timing of them. State stress and academic motivation were single item sliders, which did not allow us to assess reliability. We also administered the intervention at the beginning of the semester, which could have influenced engagement and perceived relevance of the information. Furthermore, since we only assessed participants at one time point, we do not know how long the effects of the short intervention lasted. While single-session mindset interventions have been shown to have long-term effects (e.g., Heaman et al., 2023 ; Hecht et al., 2023 ; Smith & Capuzzi, 2019 ), it is possible that this shorter intervention will not have sustained effects over time. Including brief “booster” sessions after the intervention similar to Hecht et al. ( 2023 ) would potentially be valuable and should be examined in future research.
The main implication of our study is that brief mindset interventions can be effective in shifting student mindsets. Our results also showed that the synergistic mindset intervention provided the most benefits to students; it was the only group that improved on all the main outcomes, and students in this group were less likely to want to withdraw from a course in both negative hypothetical academic scenarios (if they failed an assignment or were faced with a professor with a fixed mindset). This type of brief (5–10 min) mindset intervention could be easily applied online by students or led by teachers in the classroom. Future research should further examine the mechanisms by which these mindsets are effective on various outcomes. In addition, research should examine the longitudinal effects of brief interventions and if students apply them behaviorally. It also would be valuable for studies to examine how individuals’ characteristics (e.g., age, gender) influence the effectiveness of mindset interventions. Overall, continuing this research can have benefits on reducing stress and improving educational outcomes in young adults.
Data Availability
Data can be made available upon request.
American College Health Association (2022). National College Health Assessment: Spring 2022 reference group executive summary . https://www.acha.org/documents/ncha/NCHA-III_SPRING_2022_REFERENCE_GROUP_EXECUTIVE_SUMMARY.pdf
Blackwell, L. S., Trzesniewski, K. H., & Dweck, C. S. (2007). Implicit theories of intelligence predict achievement across an adolescent transition: A longitudinal study and an intervention. Child Development , 78 (1), 246–263. https://doi.org/10.1111/j.1467-8624.2007.00995.x
Article Google Scholar
Burnette, J. L., Knouse, L. E., Vavra, D. T., O’Boyle, E., & Brooks, M. A. (2020). Growth mindsets and psychological distress: A meta-analysis. Clinical Psychology Review , 77 , 101816. https://doi.org/10.1016/j.cpr.2020.101816
Burnette, J. L., Billingsley, J., Banks, G. C., Knouse, L. E., Hoyt, C. L., Pollack, J. M., & Simon, S. (2023). A systematic review and meta-analysis of growth mindset interventions: For whom, how, and why might such interventions work? Psychological Bulletin , 149 (3–4), 174–205. https://doi.org/10.1037/bul0000368
Cohen, S., Kamarck, T., & Mermelstein, R. (1983). A global measure of perceived stress. Journal of Health and Social Behavior , 24 (4), 385–396. https://doi.org/10.2307/2136404
Crum, A. J., Salovey, P., & Achor, S. (2013). Rethinking stress: The role of mindsets in determining the stress response. Journal of Personality and Social Psychology , 104 (4), 716–733. https://doi.org/10.1037/a0031201
Crum, A. J., Santoro, E., Handley-Miner, I., Smith, E. N., Evans, K., Moraveji, N., Achor, S., & Salovey, P. (2023). Evaluation of the rethink stress mindset intervention: A metacognitive approach to changing mindsets. Journal of Experimental Psychology: General , 152 (9), 2603–2622. https://doi.org/10.1037/xge0001396
Dweck, C. S., & Leggett, E. L. (1988). A social-cognitive approach to motivation and personality. Psychological Review , 95 , 256–273. https://doi.org/10.1037/0033-295X.95.2.256
Dweck, C. S., Chiu, C., & Hong, Y. (1995). Implicit theories and their role in judgments and reactions: A world from two perspectives. Psychological Inquiry , 6 (4), 267–285. https://doi.org/10.1207/s15327965pli0604_1
Faul, F., Erdfelder, E., Buchner, A., & Lang, A. G. (2009). Statistical power analyses using G*Power 3.1: Tests for correlation and regression analyses. Behavior Research Methods , 41 (4), 1149–1160. https://doi.org/10.3758/BRM.41.4.1149
Heaman, J. A. L., Cherry, K. M., McMurtry, C. M., Giguère, B., & Lumley, M. N. (2023). Changing minds: An rct of a growth mindset intervention on depressive symptoms and well-being in adolescent girls and boys. International Journal of Applied Positive Psychology . https://doi.org/10.1007/s41042-023-00128-z
Hecht, C. A., Gosling, S. D., Bryan, C. J., Jamieson, J. P., Murray, J. S., & Yeager, D. S. (2023). When do the effects of single-session interventions persist? Testing the mindset + supportive context hypothesis in a longitudinal randomized trial. JCPP Advances , 3 (4), e12191. https://doi.org/10.1002/jcv2.12191
Huebschmann, N. A., & Sheets, E. S. (2020). The right mindset: Stress mindset moderates the association between perceived stress and depressive symptoms. Anxiety Stress & Coping: An International Journal , 33 (3), 248–255. https://doi.org/10.1080/10615806.2020.1736900
Keech, J. J., Hagger, M. S., O’Callaghan, F. V., & Hamilton, K. (2018). The influence of university students’ stress mindsets on health and performance outcomes. Annals of Behavioral Medicine , 52 (12), 1046–1059. https://doi.org/10.1093/abm/kay008
Keech, J. J., Hagger, M. S., & Hamilton, K. (2021). Changing stress mindsets with a novel imagery intervention: A randomized controlled trial. Emotion , 21 (1), 123–136. https://doi.org/10.1037/emo0000678.supp
Liu, C. H., Stevens, C., Wong, S. H. M., Yasui, M., & Chen, J. A. (2019). The prevalence and predictors of mental health diagnoses and suicide among U.S. college students: Implications for addressing disparities in service use. Depression and Anxiety , 36 (1), 8–17. https://doi.org/10.1002/da.22830
Muenks, K., Canning, E. A., LaCosse, J., Green, D. J., Zirkel, S., Garcia, J. A., & Murphy, M. C. (2020). Does my professor think my ability can change? Students’ perceptions of their STEM professors’ mindset beliefs predict their psychological vulnerability, engagement, and performance in class. Journal of Experimental Psychology: General , 149 (11), 2119–2144. https://doi.org/10.1037/xge0000763.supp
Sarrasin, J. B., Nenciovici, L., Foisy, L. M. B., Allaire-Duquette, G., Riopel, M., & Masson, S. (2018). Effects of teaching the concept of neuroplasticity to induce a growth mindset on motivation, achievement, and brain activity: A meta-analysis. Trends in Neuroscience and Education , 12 , 22–31. https://doi.org/10.1016/j.tine.2018.07.003
Schleider, J. L., & Weisz, J. R. (2017). Little treatments, promising effects? Meta-analysis of single-session interventions for youth psychiatric problems. Journal of the American Academy of Child and Adolescent Psychiatry , 56 (2), 107–115. https://doi.org/10.1016/j.jaac.2016.11.007
Schreiber, R. E., & Veilleux, J. C. (2022). Perceived invalidation of emotion uniquely predicts affective distress: Implications for the role of interpersonal factors in emotional experience. Personality and Individual Differences , 184 , 111191. https://doi.org/10.1016/j.paid.2021.111191
Smith, T. F., & Capuzzi, G. (2019). Using a mindset intervention to reduce anxiety in the statistics classroom. Psychology Learning & Teaching , 18 (3), 326–336. https://doi.org/10.1177/1475725719836641
Williams, S. E., & Ginty, A. T. (2023). Improving stress mindset through education and imagery. Anxiety Stress and Coping , 1–9. https://doi.org/10.1080/10615806.2023.2279663
Yeager, D. S., & Dweck, C. S. (2012). Mindsets that promote resilience: When students believe that personal characteristics can be developed. Educational Psychologist , 47 (4), 302–314. https://doi.org/10.1080/00461520.2012.722805
Yeager, D. S., Bryan, C. J., Gross, J. J., Murray, J. S., Cobb, D. K., Santos, P. H. F., Gravelding, H., Johnson, M., & Jamieson, J. P. (2022). A synergistic mindsets intervention protects adolescents from stress. Nature , 607 (7919), 512–520. https://doi.org/10.1038/s41586-022-04907-7
Zielinski, M. J., Veilleux, J. C., Fradley, M. F., & Skinner, K. D. (2023). Perceived emotion invalidation predicts daily affect and stressors. Anxiety Stress and Coping , 36 (2), 214–228. https://doi.org/10.1080/10615806.2022.2033973
Download references
Open access funding provided by the Carolinas Consortium. This work was supported by the George L. Abernethy Endowment at Davidson College.
Author information
Authors and affiliations.
Public Health Department, Davidson College, 209 Ridge Rd, PO Box 5000, Davidson, NC, 28035, USA
Heidi H. Meyer & Lauren A. Stutts
You can also search for this author in PubMed Google Scholar
Contributions
Heidi Meyer and Lauren Stutts designed the study aims and procedures, obtained IRB approval, conducted literature searches, provided summaries of previous research studies, collected the data, analyzed the data, and wrote the paper. All authors have contributed to and approve the final manuscript.
Corresponding author
Correspondence to Lauren A. Stutts .
Ethics declarations
Financial interests.
The authors declare they have no financial interests.
Non-Financial Interests
Research involving human participants.
This study was approved by the Institutional Review Board at Davidson College.
Informed Consent
All participants provided informed consent before they began the study.
Additional information
Publisher’s note.
Springer Nature remains neutral with regard to jurisdictional claims in published maps and institutional affiliations.
Electronic Supplementary Material
Below is the link to the electronic supplementary material.
Supplementary Material 1
Rights and permissions.
Open Access This article is licensed under a Creative Commons Attribution 4.0 International License, which permits use, sharing, adaptation, distribution and reproduction in any medium or format, as long as you give appropriate credit to the original author(s) and the source, provide a link to the Creative Commons licence, and indicate if changes were made. The images or other third party material in this article are included in the article’s Creative Commons licence, unless indicated otherwise in a credit line to the material. If material is not included in the article’s Creative Commons licence and your intended use is not permitted by statutory regulation or exceeds the permitted use, you will need to obtain permission directly from the copyright holder. To view a copy of this licence, visit http://creativecommons.org/licenses/by/4.0/ .
Reprints and permissions
About this article
Meyer, H.H., Stutts, L.A. The Effect of Mindset Interventions on Stress and Academic Motivation in College Students. Innov High Educ (2024). https://doi.org/10.1007/s10755-024-09706-8
Download citation
Accepted : 04 March 2024
Published : 10 April 2024
DOI : https://doi.org/10.1007/s10755-024-09706-8
Share this article
Anyone you share the following link with will be able to read this content:
Sorry, a shareable link is not currently available for this article.
Provided by the Springer Nature SharedIt content-sharing initiative
- Growth mindset
- Stress-is-enhancing mindset
- Synergistic mindset
- Academic motivation
- Find a journal
- Publish with us
- Track your research
- Study Protocol
- Open access
- Published: 17 April 2024
Study protocol: exercise training for treating major depressive disorder in multiple sclerosis
- Robert W. Motl 1 ,
- Charles H. Bombardier 2 ,
- Jennifer Duffecy 3 ,
- Brooks Hibner 1 ,
- Alison Wathen 1 ,
- Michael Carrithers 4 &
- Gary Cutter 5
BMC Neurology volume 24 , Article number: 131 ( 2024 ) Cite this article
Metrics details
Major depressive disorder (MDD) is prevalent, yet sub-optimally treated among persons with multiple sclerosis (MS). We propose that exercise training may be a promising approach for treating depression in persons with MS who have MDD. Our primary hypothesis predicts a reduction in depression severity immediately after an exercise training intervention compared with minimal change in an attention control condition, and the reduction will be maintained during a follow-up period.
This study involves a parallel-group, assessor-blinded RCT that examines the effect of a 4-month home-based exercise training intervention on depression severity in a sample of persons with MS who have MDD based on the MINI International Neuropsychiatric Interview. The primary outcomes of depression severity are the Patient Health Questionnaire-9 and Hamilton Depression Rating Scale. Participants ( N = 146) will be recruited from within 200 miles of the University of Illinois at Chicago and randomized (1:1) into either a home-based exercise training condition or control condition with concealed allocation. The exercise training and social-contact, attention control (i.e., stretching) conditions will be delivered remotely over a 4-month period and supported through eight, 1:1 Zoom-based behavioral coaching sessions guided by social-cognitive theory and conducted by persons who are uninvolved in screening, recruitment, random assignment, and outcome assessment. We will collect outcome data at 0, 4 and 8 months using treatment-blinded assessors, and data analyses will involve intent-to-treat principles.
If successful, the proposed study will provide the first Class I evidence supporting a home-based exercise training program for treating MDD in persons with MS. This is critical as exercise training would likely have positive secondary effects on symptoms, cognition, and quality of life, and provide a powerful, behavioral approach for managing the many negative outcomes of MDD in MS. The program in the proposed research is accessible and scalable for broad treatment of depression in MS, and provides the potential for integration in the clinical management of MS.
Trial registration
The trial was registered on September 10, 2021 at clinicaltrials.gov with the identifier NCT05051618. The registration occurred before we initiated recruitment on June 2, 2023
Peer Review reports
Introduction
Multiple sclerosis (MS) is an immune-mediated, neurodegenerative disease of the central nervous system (CNS). There are an estimated one million adults living with MS in the United States [ 1 ]. This disease is characterized by demyelination and transection of axons and loss of neurons in the CNS [ 2 ]. The extent and location of CNS damage results in consequences including motor and cognitive dysfunction, fatigue, and major depressive disorder (MDD) [ 3 ].
MDD is characterized by persistently depressed mood or loss of interest in usual activities plus the presence of at least 5 of 9 symptoms that cause significant impairment in daily life [ 4 ]. The prevalence of MDD in persons with MS is nearly 1.7 times higher than the general population [ 5 ]. One recent systematic review reported the prevalence of MDD among persons with MS as 23.7% [ 6 ] and this translates into an estimated 250,000 people living with MS and MDD in the United States.
MDD has widespread, negative effects on the lives of people with MS [ 3 ]. The presence of MDD is associated with worsening of other symptoms such as fatigue, poorer neuropsychological functioning, and lower health-related quality of life (HRQOL) [ 3 ].
The prevalence and burden of MDD in MS underscore the critical importance of efficacious antidepressant treatments, yet such treatments are sorely lacking in MS. For example, the American Academy of Neurology concluded that there is insufficient evidence from randomized controlled trials (RCTs) for recommending antidepressants for treating MDD in MS [ 7 ]. One meta-analysis [ 8 ] of RCTs concluded that “CBT can be an effective intervention for reducing moderate depression, over the short term in patients with MS.” Yet, nearly 50% of participants do not benefit from CBT [ 9 ].
Exercise training is a promising therapy for improving depressive symptomology and managing MDD in MS [ 10 ]. Exercise training has yielded a moderate-to-large antidepressant effect in persons from the general population who have MDD [ 11 , 12 , 13 , 14 ]. Exercise training further has improved depressive symptomology in MS [ 15 , 16 , 17 ], and those meta-analyses offer critical insights for informing the exercise training parameters for treating MDD. The first meta-analysis indicated that both aerobic and resistance exercise training can yield a reduction in depressive symptoms for people with MS [ 15 ]. The second meta-analysis quantified the effect of exercise on depression in adults with neurologic disorders, including MS [ 16 ], and noted that interventions meeting physical activity guidelines yielded a reduction in depression that was two-times larger than interventions that did not meet physical activity guidelines. The third meta-analysis examined variables that moderate the effects of exercise on depressive symptoms among people with MS [ 17 ], and there was a dose–response effect for frequency (days/week) of exercise on reductions in depressive symptoms with the largest effect occurring for three days/week of exercise training.
The aforementioned meta-analyses identified four major limitations of previous research on exercise training for treating depression in MS [ 15 , 16 , 17 ]. The most pressing limitation is that the samples of persons with MS were not pre-screened for MDD [ 15 , 16 , 17 ]. Another limitation is that the exercise training programs were administered in supervised, center-based settings that present barriers associated with accessibility (e.g., distance, transportation, and costs) that likely influence adoption and maintenance of exercise behavior. An additional limitation is the lack of standardization of the exercise training prescription included in RCTs. The final limitation is the lack of follow-up regarding the durability of changes in depressive symptoms following exercise training.
We designed a RCT that is based on sound scientific rationale established through critical review and analysis of the relevant literature [ 10 , 15 , 16 , 17 ], and further capitalizes on our experiences with home-based delivery of exercise training programs in MS [ 18 , 19 , 20 , 21 , 22 ]. To that end, we propose a parallel group, RCT for examining the efficacy of a home-based, exercise training program informed by prescriptive guidelines [ 23 , 24 ] and guided by social cognitive theory (SCT)-based remote behavior coaching compared with a social-contact, attention control condition (i.e., stretching) for yielding immediate and sustained reductions in the severity of depressive symptoms among persons with MS who have MDD.
Methods/design
There is only one protocol version and it will follow the Standard Protocol Items: Recommendations for Interventional Trials (SPIRIT) guidelines.
Aims, design, and setting of the study
The primary aim examines the efficacy of a 4-month, home-based aerobic and resistance exercise training intervention compared with a 4-month, home-based stretching and flexibility intervention (i.e., social contact, attention control condition) for immediate and sustained (i.e., 4-months post-intervention) reductions in depression severity among persons with MS who have impaired MDD.
The secondary aim examines the efficacy of the exercise training intervention compared with the control condition for immediate and sustained improvements in fatigue, cognition, and HRQOL among persons with MS who have impaired MDD.
The tertiary aim involves a manipulation check and examines the efficacy of the exercise training intervention compared with control condition for immediate and sustained improvements in exercise behavior, physical activity, aerobic fitness, and muscle strength for persons with MS who have impaired MDD.
The study aims will be tested using a parallel-group, assessor-blinded RCT design. This study does not include a data safety monitoring board, but there is a data safety monitoring plan and safety monitor for oversight.
Participants
Recruitment.
We will recruit participants residing within 200 miles of the University of Illinois Chicago (UIC) campus located in Chicago, IL USA through distribution of study materials (flyers, business and post cards, and advertisements) among the Greater Illinois and other regional Chapters of the National MS Society; North American Research Committee on Multiple Sclerosis and iCONQUER MS Registries; waiting rooms of 10 + local MS Centers and Neurology offices; local community centers, churches, libraries, and physical therapy clinics; community events and MS support group meetings; focal study website ( https://metsforms.ahs.uic.edu ); UIC and professional listservs; and social media.
Inclusion/exclusion
We will assess inclusion/exclusion during a scripted phone screening by the project coordinator, and this will involve a two-stage process with the inclusion and exclusion criteria listed in Table 1 .
We have experienced low attrition (10%) in our previous RCTs of the exercise training protocol in this proposal [ 19 ], and we note recent data from a meta-analysis suggesting an attrition rate of ~ 10% across 40 RCTs of exercise training in MS [ 33 ]. We believe attrition could be higher in this RCT based on depressive symptomology in MDD resulting in poor motivation and adherence for exercise engagement. We conservatively planned for a higher attrition rate of 20% for the proposed RCT, and recognize that retention might be a challenge, although we are including SCT-based content and 1:1 zoom-based behavioral coaching for maximizing retention and adherence [ 34 ] as is appropriate for persons with MS who have elevated depressive symptoms [ 35 ].
Power analysis and sample size
The power analysis was conducted in G*Power, Version 3.1 using F test for Test family and ANOVA: Repeated measures, within-between interaction for Statistical test. We estimated the sample necessary for detecting a Condition (2 levels of between-subjects factor: Intervention vs. Control) × Time (2 levels of within-subjects factor: 0 and 4 months) interaction on the primary outcomes of depression severity (i.e., 9-item Patient Health Questionnaire (PHQ-9; [ 36 ]) and Hamilton Depression Rating Scale (HDRS-17; [ 37 ]). We did not include 3 time-points as this assumes linear change across all 3 time points in G*Power 3.1, and we expected change between 0 and 4 months for the intervention condition, followed by stability between 4 and 8 months. The effect size (Cohen’s f = 0.18) was from our previous meta-analyses [ 15 ] regarding the effect of exercise training on depressive symptoms in persons with MS. The power analysis included assumptions of reliability for the within-subjects factor of ICC = 0.50, two-tailed α = 0.025, and β = 0.05 (i.e., 95% power); the α = 0.025 was selected based on two primary outcomes. The power analysis indicated the minimal total sample size for testing the Time × Condition interaction of 122 participants (61 per group), and we anticipate a dropout rate of ~ 20% resulting in a projected recruitment of 146 participants.
The primary outcomes are the PHQ-9 [ 36 ] and HDRS-17 [ 37 ] as measures of depression symptom severity appropriate for MDD, whereas the secondary outcomes include measures of fatigue, cognitive performance, and HRQOL. The tertiary outcomes are exercise behavior, accelerometry as a device-based measure of free-living PA, and aerobic and muscle fitness. All outcomes will be assessed at baseline (0 months), immediate follow-up (4 months), and long-term follow-up (8 months) by treatment-blinded assessors. The assessors will not be involved in random assignment or delivery of the conditions, and will not directly communicate with the behavior coaches about participants. Participants themselves will be instructed not to discuss exercise routines with assessors, and why it might bias the evaluators.
Primary outcome measures
We include two outcomes for depression severity, as one is self-reported (primary) and the other is a semi-structured, interviewer-rated measure (secondary). The logic is that the self-report change in depression severity should be confirmed with the semi-structured, interviewer-rated change, as change in the former is more likely, but could represent a self-report bias associated with participants not being blinded regarding treatment condition.
Self-reported depression severity
The PHQ-9 is a brief, patient-reported depression severity measure [ 36 ]. The PHQ-9 is unidimensional [ 38 ], has good test–retest reliability [ 39 ], and has validated thresholds of mild, moderate, moderately severe and severe depression [ 36 ]. The PHQ-9 accurately discriminates differential treatment response among groups independently judged to have persistent MDD, partial remission, and full remission [ 39 ]. The PHQ-9 has a valid threshold for determining depression remission (less than 5) [ 39 ], and an established threshold for minimal clinically significant difference for individual change (5 points on 0–27 point scale) [ 39 ].
Interviewer-rated depression severity
The 6-item Maier subscale [ 40 ] of the HDRS-17 [ 37 ] is a semi-structured, interviewer-rated measure that is administered by treatment-blinded assessors. The Maier subscale was developed using Rasch analyses and provides a unidimensional subscale that has equivalent or greater sensitivity to treatment effects compared with the HDRS-17 [ 41 , 42 ]. The Maier subscale has been recommended specifically for depression treatment trials in patients with medical comorbidities because the measure includes no somatic items [ 42 ]. The Maier has a valid cutoff for remission (4 or less) [ 42 ].
Secondary outcome measures
We will include the secondary end-points of fatigue [ 43 ], cognitive performance [ 44 ], and HRQOL [ 45 ], as changes in depression are often accompanied by changes in fatigue, neuropsychological function, and HRQOL [ 3 ]. These outcomes will anchor depression changes with other clinical end-points of substantial relevance for persons with MS who have MDD.
The perception of fatigue severity will be measured using the Fatigue Severity Scale (FSS) [ 43 ]. The FSS has 9 items rated on a 7-point scale regarding the severity of fatigue symptoms during the past 7 days. The item scores are averaged into a measure of fatigue severity that ranges between 1 and 7. FSS scores of 4 or above are indicative of severe MS-related fatigue [ 43 ], and the MDC for the FSS is 1.9 points [ 46 ]. There is evidence for the internal consistency, test–retest reliability, and validity of FSS scores as a measure of fatigue severity in MS [ 43 ].
Cognitive performance
Cognitive performance is a secondary outcome that will be assessed using the Brief International Cognitive Assessment for MS (BICAMS) [ 44 ]. The BICAMS battery includes the Symbol Digit Modalities Test (SDMT), first five learning trials of the California Verbal Learning Test-II (CVLT-II), and first three learning trials of the Brief Visuospatial Memory Test-Revised (BVMT-R) for measuring information processing speed, verbal learning and memory, and visuospatial learning and memory, respectively [ 44 , 47 ]. The SDMT involves pairing 9 abstract geometric symbols with single digit numbers in a key, and orally stating the correct numbers for unpaired symbols as rapidly as possible for 90 s. The primary outcome of the SDMT is the number of correct responses provided in 90 s (i.e., raw score). The CVLT-II involves an examiner reading aloud a list of 16 words (four items belonging to four categories such as vegetables, animals, furniture, modes of transportation) that are randomly arranged; this is done five times in the same order at a rate of approximately one word per second. Participants recall as many items as possible, in any order, following each reading of list. The primary outcome of the CVLT-II is the total number of correct words identified over the five trials (i.e., raw score). The BVMT-R involves three trials of the examiner presenting a 2 × 3 array of abstract geometric figures approximately 15 inches in front of the participant for 10 s. The array is then removed and participants draw the array as precisely as possible with the figures in the correct location. Each drawing is scored based on accurately portraying each figure and its correct location using a 0–2 scale. The primary outcome of the BVMT-R is the total raw score across the three trials. There are benchmark scores for the cognitive tests included in the BICAMS that are associated with specific degrees of impairment in work status [ 48 ].
The 29-item Multiple Sclerosis Impact Scale (MSIS-29) [ 45 ] provides a disease-specific measure of physical (20 items) and psychological (nine items) HRQOL. The scores range between 0 and 100 with lower MSIS-29 scores representing higher HRQOL. There is evidence for the reliability and validity of the MSIS-29 in samples of persons with MS [ 45 , 49 ].
Tertiary outcome measures
We will measure change in exercise behavior using the Godin Leisure-Time Exercise Questionnaire (GLTEQ) [ 50 ] and minutes/day of moderate-to-vigorous physical activity (MVPA) from accelerometry as a measure of free-living physical activity. We will measure aerobic capacity and muscle strength using accepted measures and protocols in MS [ 51 ]; this permits an additional check on the manipulation of performing the GEMS exercise-training protocol.
Self-reported exercise behavior
The GLTEQ measures the frequency of strenuous, moderate, and mild physical activity performed for periods of 15 min or more over a 7-day period [ 50 , 52 ], and it will be scored as the Health Contribution Score (HCS) [ 28 ]. The HCS only includes strenuous and moderate physical activity. The HCS is computed by multiplying the frequencies of strenuous and moderate activities by 9 and 5 METs, respectively, and then summing the weighted scores. The HCS can be converted into one of three categories, namely, insufficiently active (i.e., score < 14 units), moderately active (i.e., score between 14 and 23 units), and active (i.e., score ≥ 24 units).
Device-measured free-living physical activity
The ActiGraph model GT3X + accelerometer (Actigraph Corporation, FL) worn during a seven-day period will provide a measure of free-living physical activity as minutes/day of MVPA. The ActiGraph accelerometer will be placed on an elastic belt that is worn snuggly around the waist over the non-dominant hip during the waking hours of a seven-day period. The data from the ActiGraph accelerometer will be downloaded and processed using the low frequency extension (i.e., filter for increasing the devices sensitivity) into one-minute epochs using ActiLife software (Actigraph Corporation, FL), and then scored for wear time and minutes/day of MVPA using MS-specific cut-points [ 53 ]. Only data from valid days (wear time ≥ 600 min) will be included in the analyses [ 53 ] and this will be confirmed with the compliance log. We will average data over two or more valid days for the outcome of minutes/day of MVPA, as this provides a reliable estimate of free-living physical activity behavior over a seven-day period [ 53 ]. Other measures such as steps/day and minutes/day spent in light physical activity and sedentary behavior [ 54 ] can be generated as additional end-points for understanding change in free-living physical activity.
Aerobic capacity
Cardiorespiratory fitness will be operationalized as peak oxygen consumption (VO 2peak ) and peak power output (watts or W) derived from a maximal, incremental exercise test on an electronically-braked, computer-driven cycle ergometer (Lode BV, Groningen, The Netherlands) and a calibrated open-circuit spirometry system (TrueOne, Parvo Medics, Sandy, UT) for analyzing expired gases [ 55 , 56 ]. The incremental exercise test initially involves a brief, 3-min warm-up at 0 W. The initial work rate for the incremental exercise test is 0 W, and the work rate continuously increases at a rate of 15 W/min (0.25 W/sec) until participants reach maximal exertion defined as volitional fatigue. Oxygen consumption (VO 2 ), respiratory exchange ratio (RER), and W are measured continuously by the open-circuit spirometry system and expressed as 20-s averages. Heart rate (HR) is displayed using a Polar HR monitor (Polar Electro Oy, Finland), and HR and rating of perceived exertion (RPE) are recorded every minute. VO 2peak is expressed in ml kg −1 min −1 and peak power output is expressed in W based on the highest recorded 20-s values when two or more of the following criteria are satisfied: (1) VO 2 plateau with increasing W; (2) RER ≥ 1.10; (3) peak HR within 10 beats per minute of age-predicted maximum (i.e., ~ 1 SD); or (4) peak RPE ≥ 17 [ 55 , 56 ].
Muscle strength
Bilateral, isometric knee extensor (KE) and knee flexor (KF) peak torque will be measured using an isokinetic dynamometer (Biodex System 3 Dynamometer, Shirley, NY) [ 51 , 57 ]. Participants will be seated on the dynamometer consistent with the manufacturer's recommendations. Isometric torque will be assessed at 3 joint angles of 45°, 60° and 75°. Per joint angle, participants perform three, 5-s maximal knee extensions and one, 5-s maximal knee flexion. There is a rest period of 5-s between contractions within a set, and the rest period is 1 min between sets. The highest recorded peak torque for the stronger leg, regardless of joint angle, represents KE and KF isometric strength (N·m) [ 51 , 57 ].
Random assignment
After collection of baseline data, participants will be randomly assigned into either the exercise training condition or the control condition using a computerized process based on a random numbers sequence, and group allocation will be concealed. Participants will not be informed directly that the exercise training condition represents the experimental treatment condition and the stretching condition (i.e., attention and social contact control condition) represents the control condition, as both conditions are based on guidelines and likely have benefits in MS. To do this, the study will be advertised as comparing two different exercise approaches for managing consequences of MS and improving health indicators among persons with MS. We will measure treatment credibility after the first assigned treatment session using an adaptation of the Reaction to Treatment Questionnaire (RTQ) [ 58 ].
Intervention condition – home-based aerobic and resistance exercise training
The proposed trial will deliver the Guidelines for Exercise in MS (GEMS) program, as fully described in our previous research [ 18 , 19 , 20 , 21 , 22 ], within a remotely coached/guided, home-based setting using telerehabilitation (i.e., Zoom). The schematic of the main program components is provided in Fig. 1 and the components are summarized in Table 2 . The intervention condition consists of six main components: (1) three different progressive trajectories of aerobic/resistance exercise prescriptions for individualization (Orange, Blue, and Green; Table 3 ) that are based on current guidelines for adults with MS who have mild-to-moderate disability (i.e., defined as EDSS 0–7) [ 23 , 24 ], (2) appropriate exercise equipment including a CW-300 pedometer (NEW-LIFESTYLES, INC., Lee’s Summit, MO) and set of elastic resistance bands (Black Mountain Products, McHenry, IL), (3) one-on-one coaching, (4) action-planning via calendars, (5) log books for self-monitoring, and (6) SCT-based newsletters. Of note, the current exercise guidelines specify 30 + minutes of moderate-intensity aerobic exercise 3 time per week and resistance training targeting major muscle groups 3 times per week [ 23 , 24 ]. Walking is the aerobic exercise modality based on it being the most commonly reported mode of exercise among people with mild MS [ 59 ] and the intensity walking is controlled based on a step rate of 100 steps per minute as this corresponds with moderate-intensity exercise in persons with MS [ 60 ]. The resistance training stimulus consists of 1–2 sets involving 10–15 repetitions of 5–10 exercises that target the lower body, upper body, and core muscle groups. The specific lower body exercises are the chair raise, calf raise, knee flexion, knee extension, and the lunge; the specific upper body resistance exercises are the shoulder row, shoulder raise, elbow flexion, and elbow extension; and the specific core exercise is the abdominal curl. The one-on-one coaching (i.e. weeks 1, 2, 3, 4, 5, 7, 11, and 15) focuses on three main components: (1) exercise training guidance and oversight, (2) discussion of the behavioral strategies of action planning and self-monitoring, and (3) presentation and discussion of newsletters based on SCT constructs (i.e., outcome expectations, self-monitoring, goal-setting, self-efficacy, barriers, and facilitators) for optimizing adherence and compliance (Table 4 ) [ 18 , 19 , 20 , 21 , 22 ]. We further provide all participants with an NMSS educational packet “Minimizing your risk for falls: A guide for people with MS”, and a study-specific instruction sheet on fall prevention. Participants are instructed to document any falls and other concerns or adverse events in the exercise adherence log and report these during one-on-one coaching, and all adverse events will be documented and reported per UIC IRB guidelines.
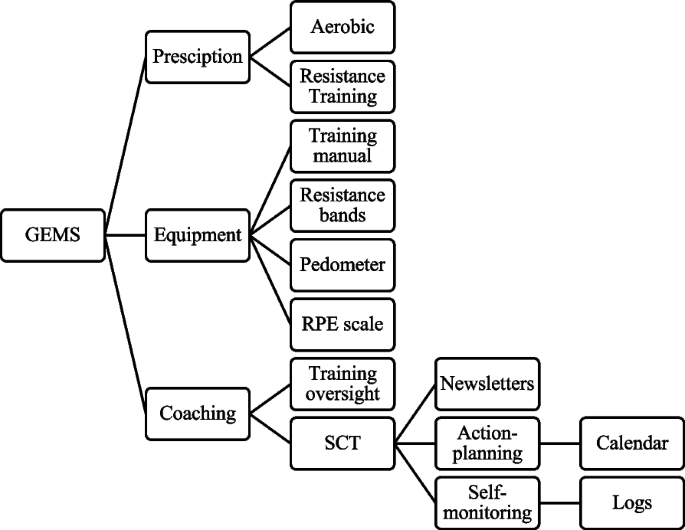
Outline of the Guideline for Exercise in Multiple Sclerosis (GEMS) program
Control condition – home-based stretching and flexibility program
This program has been described in our previous research [ 22 ] and was developed based on a RCT of exercise training for improving mobility in MS [ 51 ] and two RCTs of exercise training for cognitive dysfunction in MS [ 61 , 62 ]. The program itself has identical components as the GEMS program for aerobic and resistance exercise training, but focuses on stretching for improving flexibility and range of motion as important components of fitness. The program itself is based on Stretching for People with MS: An Illustrated Manuel from the National MS Society (Table 5 ), as this is MS specific and enhances the credibility of the control condition. Participants will be provided with a yoga pad (i.e., exercise equipment) and a manual, log-book, calendar, and prescription for the stretching program. This program includes newsletters focusing on SCT for behavior change, and video-chats with behavioral coaches that provide motivation and social accountability. The video-chats occur on the same timeline and frequency as the GEMS exercise training program in the intervention condition, but focus on the SCT constructs applied for stretching. We further monitor safety and compliance as done in the intervention condition, and provide resources and instruction on safety. Importantly, this condition accounts for the possible influences of social-contact and attention associated with the GEMS program on the study outcomes, and this represents a major advancement over waitlist control and standard of care conditions in previous RCTs of exercise training and depression in MS [ 10 , 15 , 16 , 17 ].
The study procedure is administered by a project coordinator with oversight by the PI and Co-Is, and monitored through a fidelity monitoring plan (Table 6 ). As done in our previous research [ 18 , 19 , 20 , 21 , 22 ], the project coordinator will contact interested participants via telephone, describe the study and its requirements, and then conduct the screening for inclusion/exclusion criteria. The project coordinator will then distribute the informed consent document electronically among participants who meet inclusion criteria further information about the study. This will be followed by a telephone call that ensures participants received the document and understand the study and research procedures. The project coordinator will further work with participants in obtaining physician approval for participation and verification of MS diagnosis as a final step in enrollment.
The project coordinator will schedule baseline data collection, and provide written and verbal instructions regarding the baseline testing procedures. The project coordinator will send the participant document with directions and parking information, and contact the participant electronically and through telephone 24-h before the appointment as a reminder. Upon arrival, the project coordinator will review the study procedures with the participant, obtain written informed consent, and then initiate the baseline data collection.
The baseline data collection will be undertaken by treatment-blinded researchers who will start with a PAR-Q for ensuring safety and then administer measures of depression severity (i.e., primary outcomes) followed by the BICAMS and measures of fatigue and HRQOL (i.e., secondary outcomes). The participant will then undertake the maximal exercise test and muscle strength testing with a 15-min break between the measures of fitness. The competition of those measures will take ~ 120 min based on our previous experiences.
The treatment-blinded researchers will provide the participant with a packet containing an accelerometer along with GLTEQ. This packet will include instructions regarding the importance of wearing the accelerometer as instructed every day during the seven-day period, and provide a pre-stamped and pre-addressed envelope for return postal service. The participants will wear the accelerometer for a seven-day period and then complete the GLTEQ. The project coordinator will send brief, scripted e-mails for reminding participants about wearing the accelerometer in the middle of the seven-day period. This will be followed by a telephone call verifying that participants wore the accelerometer daily during the seven-day period and returned it along with the GLTEQ through the United States Postal Service.
Of note, demographic and disease-related characteristics will be collected from participant interviews and verification forms from the treating Neurologist, respectively. The patients will further provide a list of current medications and ongoing treatments for MDD and other symptoms of MS.
Once the baseline assessment is completed, participants will be randomly assigned into either the intervention or control conditions using a random numbers sequence with concealed allocation. The project coordinator will receive information on allocation, record it in a database, and communicate the condition of assignment with the participant and behavioral coaches. Importantly, several strategies will be adopted for maintaining blinded conditions. The behavioral coaches and other study staff are located in a separate lab space from where the treatment-blinded researchers administer outcomes. The behavioral coaches will emphsize among participants the importance of not revealing what type of exercise is being undertaken when interactiong with outcome assessors. The study staff will remind participants about not revealing the type of exercise being undertaken before the outset of follow-up outcome assessments.
The intervention/control conditions will be delivered by behavioral coaches who are univolved in outcome assessments in 12, partially overlapping waves of ~ 12 participants per wave, and the conditions will be delivered across a 4-month period. This use of waves will afford additional time for behavioral coaching during the one-on-one chat sessions than if enrolling 146 in one recruitment wave. This should permit greater penetration of the study materials. Participants will be asked to contact the project coordinator via the dedicated toll-free telephone number or e-mail in the occurrence of an adverse event or any other problem; this information will further be collected during video chats with behavioral coaches. The project coordinator will administer the PHQ-9 on the same weeks as the behavioral chats for ongoing monitoring of the mood status of participants.
The participants will complete the same measurement procedures immediately (i.e., immediate follow-up; 4 months) and 4-months (i.e., long-term follow-up; 8 months) after initiating the intervention/control conditions. There will be no behavioral coaching session during the long-term follow-up period for examining sustainability.
Participants will receive $100 USD remuneration for completing the measures per assessment period, including baseline, for a total of $300 USD. We will collect formative feedback using a Qualtrics survey for identifying opportunities for intervention improvement and refinement; this will be undertaken by participants after completion of the study.
Data analyses
The data analyses will be overseen by a biostatistician and follow intent-to-treat (ITT) principles (i.e., include all persons regardless of dropout). We will perform exploratory data analyses only among those who complete immediate and long-term follow-up testing (i.e., completer’s or per protocol analysis). We will check the data for errors and outliers, and lock the data set before analyses. The analytic plan will account for potential confounders of the intervention effect on the outcomes. The confounders may include MS duration, BMI, age, sex, disease-modifying therapy, and relapse rate. We will include any of those variables and others that differ between conditions as covariates in the following analyses.
Data analysis – aim 1
The first analysis tests the hypothesis that those who are randomly assigned into the intervention condition (i.e., exercise training) will demonstrate (a) reductions from baseline in depression severity that (b) are sustained over 4-months of follow-up compared with those in the control condition (i.e., stretching). The hypothesis will be tested using a linear mixed model in JMP Pro 16.0. The linear mixed model will include condition and time as fixed effects, and subject nested within condition as a random effect using unbounded variance components and the REML method ( https://www.jmp.com/content/dam/jmp/documents/en/academic/learning-library/08-repeated-measures-analysis-(mixed-model).pdf ). The hypothesized interaction term will be decomposed with follow-up tests, and differences in comparison of mean scores will be expressed as Cohen’s d with standard guidelines for interpretation. The final models for PHQ-9 and HDRS-17 scores will be adjusted for covariates. The overall Type I error will be controlled based on an adjustment of alpha (two-tailed α = 0.025) given the two primary outcomes in Aim 1.
Data analysis – aim 2
The second set of analyses test the hypotheses that those who are randomly assigned into the intervention condition (i.e., exercise training) will report (a) improvements from baseline in fatigue, cognitive performance, and QOL that (b) are sustained over 4-months of follow-up compared with those in the control condition (i.e., stretching). Those hypotheses will be tested with the same modeling approach described for Aim 1. The overall Type I error will be controlled using a step-down procedure testing first fatigue, followed by domains of cognitive performance (SDMT, CVLT-II, and then BVMT-R), and lastly HRQOL [ 63 ].
Data analysis – aim 3
The third set of analyses test the hypotheses that those who are randomly assigned into the intervention condition (i.e., exercise training) will report (a) improvements from baseline in exercise behavior, free-living PA, and aerobic capacity and muscle strength that (b) are sustained over 4-months of follow-up compared with those in the control condition (i.e., stretching). Those hypotheses will be tested with the same modeling approach described for Aim 1. The overall Type I error will be controlled using a step-down procedure testing first exercise behavior and free-living PA, followed by aerobic capacity and muscle strength as outcomes [ 63 ].
Current trial status
As of February 13, 2024 and reported in our quarterly report for the funder, we have enrolled 18 persons into the trial, and these persons have been equally randomized into the intervention and control conditions (9 per condition). There were 6 other persons scheduled for baseline testing and ready for randomization.
We are proposing a Phase-II RCT of exercise training for treating depression severity in persons with MS who have MDD. If successful based on statistically significant and clinically meaningful improvements in depression symptom outcomes (e.g., ½ SD improvement for exercise compared with control) [ 64 ] as well as retention exceeding 20% (primary decision rules), we will proceed with the design of a Phase-III clinical trial of exercise training compared with CBT alone and combined with exercise training for treating depression severity in persons with MS who have MDD. We propose the addition of CBT as it has been considered a “possibly efficacious” treatment for depression in MS [ 7 , 8 ] and can be delivered remotely [ 9 ]. This is a logical next step, as the data gathered herein would power such a clinical trial and provide necessary experiences for a presumed larger trial. We further have experience in the conduct of Phase-III trials of exercise and physical activity in MS [ 21 , 65 ], and our ongoing PCORI trial provides a benchmark for conducting a Phase-III clinical trial of exercise training compared with CBT for managing depression severity in persons with MS who have MDD. Such a Phase-III clinical trial would provide definitive evidence for transition into clinical care and practice of persons with MS who have MDD, perhaps serve as a benchmark for studying exercise training as a treatment of other outcomes in persons with MS – this is a major stumbling block in all MS research involving exercise training [ 66 ], including depressive symptoms in MDD [ 10 ].
We may experience problems with the participants adhering with the intervention and control conditions based on the lack of interest/pleasure in activities, sadness, tiredness/fatigue, or physical problems (e.g., pain) as part of MDD. We are minimizing this by using SCT-based content and strategies and 1:1 remote behavioral coaching for maximizing adherence with both conditions. We further are managing this by enrolling a smaller number of persons (n ~ 12) over 12, partially overlapping recruitment waves (i.e., 12 waves of ~ 12 participants per wave), and thereby having the behavior coaches devote a greater amount of time with the participants during the one-on-one chat sessions. This should permit greater penetration of the study materials and a larger change in behavior for both conditions. The power analysis was based on meta-analyses for the effect of exercise interventions on depressive symptoms in samples that were not prescreened for MDD, and the preliminary data might not represent the treatment effect for those with MDD. Of note, our secondary analysis of previously published data suggested that effect of a physical activity intervention on depressive symptoms was stronger in those with elevated scores [ 67 ], and this would suggest that our power analysis and sample size should be appropriate for detecting an intervention effect on depression in those with MDD. There may be some attrition during the 4-month follow-up period wherein there is no planned coaching/contact, but this has been minimal in our previous [ 68 ] and ongoing [ 21 ] trials using the sample general approach; this is expected as the conditions are designed around teaching people skills, techniques, and strategies for sustainable behavior change.
If successful, the proposed study will provide the first and only Class I evidence for a home-based exercise training program as a treatment of depression in persons with MS who have MDD. This is critical as exercise training would likely have secondary effects on symptoms, cognition, and HRQOL, and provide a powerful, behavioral approach for managing the many negative outcomes of MDD in MS. The program in the proposed research is accessible and scalable for broad-scale treatment of depression in MS, and provides the potential for integration in the clinical management of this disease.
Availability of data and materials
No datasets were generated or analysed during the current study.
Abbreviations
Adverse Events
Brief International Cognitive Assessment for MS
Brief Visuospatial Memory Test-Revised
California Verbal Learning Test-II
Central Nervous System
Cognitive Behavioral Therapy
Co-Investigators
Comparative Effectiveness Research
Diagnostic Manual of Mental Disorders V
Disease Modifying Therapies
Effect Size
Exercise Neuroscience Research Laboratory
Fatigue Severity Scale
Guidelines for Exercise in Multiple Sclerosis
Godin Leisure-Time Exercise Questionnaire
Hamilton Depression Rating Scale
Health Contribution Score
Health-Related Quality of Life
Institutional Review Board
Knee Extensor
Knee Flexor
Major Depressive Disorder
Modified Fatigue Impact Scale
Multiple Sclerosis
Multiple Sclerosis Impact Scale
National MS Society
Oxygen Consumption
Patient-Determined Disease Steps
Patient Health Questionnaire-9
Peak Oxygen Consumption
Peak Power Output
Physical Activity
Physical Activity Readiness Questionnaire
Principal Investigator
Randomized Controlled Trial
Rating of Perceived Exertion
Relapsing-Remitting MS
Respiratory Exchange Ratio
Serious Adverse Events
Social-Cognitive Theory
Symbol Digit Modalities Test
Telephone Interview for Cognitive Status
United States Dollar
Wallin MT, Culpepper WJ, Campbell JD, et al. The prevalence of MS in the United States: a population-based estimate using health claims data. Neurology. 2019;92:1029–40.
Article Google Scholar
Trapp BD, Nave K. Multiple sclerosis: an immune or neurodegenerative disorder? Ann Rev Neurosci. 2008;31:247–69.
Article CAS PubMed Google Scholar
Feinstein A, Magalhaes S, Richard JF, et al. The link between multiple sclerosis and depression. Nat Rev Neurol. 2014;10:507–17.
Article PubMed Google Scholar
American Psychiatric Association DSM-5 Taskforce. Diagnostic and statistical manual of mental disorders. 5th ed. Arlington: American Psychiatric Association; 2013.
Book Google Scholar
Marrie RA, Fisk JD, Tremlett H, et al. Differences in the burden of psychiatric comorbidity in MS vs the general population. Neurology. 2015;85:1972–9.
Article PubMed PubMed Central Google Scholar
Marrie RA, Reingold S, Cohen J, et al. The incidence and prevalence of psychiatric disorders in multiple sclerosis: a systematic review. Mult Scler. 2015;21:305–17.
Minden SL, Feinstein A, Kalb RC, et al. Evidence-based guideline: assessment and management of psychiatric disorders in individuals with MS: report of the Guideline Development Subcommittee of the American Academy of Neurology. Neurology. 2014;82:174–81.
Hind D, Cotter J, Thake A, et al. Cognitive behavioural therapy for the treatment of depression in people with multiple sclerosis: a systematic review and meta-analysis. BMC Psychiatry. 2014;14.
Mohr DC, Hart SL, Julian L, et al. Telephone-administered psychotherapy for depression. Arch Gen Psychiatry. 2005;62:1007–14.
Feinstein A, Rector N, Motl R. Exercising away the blues: can it help multiple sclerosis-related depression? Mult Scler. 2013;19:1815–9.
Kvam S, Kleppe CL, Nordhus IH, et al. Exercise as a treatment for depression: A meta-analysis. J Affect Disord. 2016;202:67–86.
Miller KJ, Goncalves-Bradley DC, Areerob P, et al. Comparative effectiveness of three exercise types to treat clinical depression in older adults: a systematic review and network meta-analysis of randomised controlled trials. Ageing Res Rev. 2020;58:100999.
Morres ID, Hatzigeorgiadis A, Stathi A, et al. Aerobic exercise for adult patients with major depressive disorder in mental health services: a systematic review and meta-analysis. Depress Anxiety. 2019;36:39–53.
Schuch FB, Vancampfort D, Richards J, et al. Exercise as a treatment for depression: a meta-analysis adjusting for publication bias. J Psychiatr Res. 2016;77:42–51.
Ensari I, Motl RW, Pilutti LA. Exercise training improves depressive symptoms in people with multiple sclerosis: results of a meta-analysis. J Psychosom Res. 2014;76:465–71.
Adamson BC, Ensari I, Motl RW. The effect of exercise on depressive symptoms in adults with neurological disorders: a systematic review and meta-analysis. Arch Phys Med Rehabil. 2015;96:1329–38.
Herring MP, Fleming KM, Hayes SP, et al. Moderators of exercise effects on depression symptoms in multiple sclerosis. Am J Prev Med. 2017;53:508–18.
Adamson BC, Learmonth YC, Kinnett-Hopkins D, et al. Feasibility study design and methods for project GEMS: guidelines for exercise in multiple sclerosis. Contemp Clin Trials. 2016;47:32–9.
Learmonth YC, Adamson BC, Kinnett-Hopkins D, et al. Results of a feasibility randomized controlled study of the guidelines for exercise in multiple sclerosis project. Contemp Clin Trials. 2017;54:84–97.
Kinnett-Hopkins D, Motl RW. Results of a feasibility study of a patient informed, racially tailored home-based exercise program for black persons with multiple sclerosis. Contemp Clin Trials. 2018;75:1–8.
Motl RW, Backus D, Neal WN, et al. Rationale and design of the STEP for MS Trial: comparative effectiveness of supervised versus telerehabilitation exercise programs for multiple sclerosis. Contemp Clin Trials. 2019;81:110–22.
Motl RW, Kinnett-Hopkins D, Neal W, et al. Targeted exercise for African-Americans with multiple sclerosis: project TEAAMS. Contemp Clin Trials. 2023;126:107088.
Kalb R, Brown TR, Coote S, et al. Exercise and lifestyle physical activity recommendations for people with multiple sclerosis throughout the disease course. Mult Scler. 2020. https://doi.org/10.1177/1352458520915629 . in press.
Kim Y, Lai B, Mehta T, et al. Exercise training guidelines for multiple sclerosis, stroke, and Parkinson disease: Rapid review and synthesis. Am J Phys Med Rehabil. 2019;98:613–21.
Thomas S, Reading J, Shephard RJ. Revision of the physical activity readiness questionnaire. Can J Sport Sci. 1992;17:338–45.
CAS PubMed Google Scholar
de Jager CA, Budge MM, Clarke R. Utility of the TICS-M for the assessment of cognitive function in older adults. Int J Geriatr Psychiatry. 2003;8(4):318–24.
Posner K, Brown GK, Stanley B, et al. The Columbia-Suicide Severity Rating Scale (C-SSRS): initial validity and internal consistency findings from three multisite studies with adolescents and adults. Am J Psychiatry. 2011;168:1266–77.
Motl RW, Bollaert RE, Sandroff BM. Validation of the Godin Leisure-Time Exercise Questionnaire classification coding system using accelerometry in multiple sclerosis. Rehabil Psychol. 2018;63:77–82.
Learmonth YC, Motl RW, Sandroff BM, et al. Validation of patient determined disease steps (PDDS) scale scores in persons with multiple sclerosis. BMC Neurol. 2013;13: 37.
Beck AT, Steer RA, Brown GK. BDI – FastScreen for medical patients, manual. San Antonio: The Psychological Corporation, A Harcourt Assessment Company; 2000.
Google Scholar
Benedict RHB, Fishman MM, McClellan R, et al. Validity of the beck depression inventory – fast screen in multiple sclerosis. Mult Scler. 2003;9:393–6.
Sheehan DV, Lecrubier Y, Sheehan KH, et al. The Mini-International Neuropsychiatric Interview (M.I.N.I.): the development and validation of a structured diagnostic psychiatric interview for DSM-IV and ICD-10. J Clin Psychiatry. 1998;59:22–33.
PubMed Google Scholar
Motl RW, Russell DI, Pilutt LA, et al. Drop-out, adherence, and compliance in randomized controlled trials of exercise training in multiple sclerosis: short report. Multi Scler J. 2024;30:605–11.
Motl RW, Pekmezi D, Wingo BC. Promotion of physical activity and exercise in multiple sclerosis: importance of behavioral science and theory. Mult Scler J Exp Transl Clin. 2018;4:2055217318786745.
PubMed PubMed Central Google Scholar
Ensari I, Kinnett-Hopkins D, Motl RW. Social cognitive correlates of physical activity among persons with multiple sclerosis: Influence of depressive symptoms. Disabil Health J. 2017;10:580–6.
Kroenke K, Spitzer RL, Williams JB. The PHQ-9: Validity of a brief depression severity measure. J Gen Intern Med. 2001;16:606–13.
Article CAS PubMed PubMed Central Google Scholar
Hamilton M. A rating scale for depression. J Neurol Neurosurg Psychiatry. 1960;23:56–62.
Gunzler DD, Perzynski A, Morris N, et al. Disentangling multiple sclerosis and depression: an adjusted depression screening score for patient-centered care. J Behav Med. 2015;38:237–50.
Löwe B, Kroenke K, Herzog W, et al. Measuring depression outcome with a brief self-report instrument: sensitivity to change of the Patient Health Questionnaire (PHQ-9). J Affect Disord. 2004;81:61–6.
Maier W, Heuser I, Philipp M, et al. Improving depression severity assessment - i. Reliability, internal validity and sensitivity to change of three observer depression scales. J Psychiatr Res. 1988;22:3–12.
Faries D, Herrera J, Rayamajhi J, et al. The responsiveness of the hamilton depression rating scale. J Psychiatr Res. 2000;34:3–10.
Ruhe HG, Dekker JJ, Peen J, et al. Clinical use of the hamilton depression rating scale: Is increased efficiency possible? A post hoc comparison of hamilton depression rating scale, maier and bech subscales, clinical global impression, and symptom chicklist-90 scores. Compr Psychiatry. 2005;46:417–27.
Krupp LB, LaRocca NG, Muir-Nash J, et al. The fatigue severity scale: application to patients with multiple sclerosis and systemic lupus erythematosus. Arch Neurol. 1989;46:1121–3.
Langdon DW, Amato MP, Boringa J, et al. Recommendations for a brief international cognitive assessment for multiple sclerosis. Mult Scler. 2012;18:891–8.
Hobart J, Lamping D, Fitzpatrick R, et al. The Multiple Sclerosis Impact Scale (MSIS-29): a new patient-based outcome measure. Brain. 2001;124:962–73.
Learmonth YC, Dlugonski D, Pilutti LA, et al. Psychometric properties of the fatigue severity scale and the modified fatigue impact scale. J Neurol Sci. 2013;331(1–2):102–7.
Corfield F, Langdon D. A systematic review and meta-analysis of the brief cognitive assessment for multiple sclerosis (BICAMS). Neurol Ther. 2018;7:287–306.
Benedict RHB, Drake AS, Irwin LN, et al. Benchmarks of meaningful impairment on the MSFC and BICAMS. Mult Scler. 2016;22:1874–82.
Riazi A, Hobart JC, Lamping DL, et al. Multiple Sclerosis Impact Scale (MSIS-29): reliability and validity in hospital based samples. J Neurol Neurosurg Psychiatry. 2002;73:701–4.
Godin G. The Godin-Shephard leisure-time physical activity questionnaire. Health Fitness J Can. 2011;4:18–22.
Sandroff BM, Bollaert RE, Pilutti LA, et al. Multimodal exercise training in multiple sclerosis: a randomized controlled trial in persons with substantial mobility disability. Contemp Clin Trials. 2017;61:39–47.
Sikes EM, Richardson EV, Cederberg KJ, et al. Use of the Godin leisure-time exercise questionnaire in multiple sclerosis research: a comprehensive narrative review. Disabil Rehabil. 2019;41:1243–67.
Sasaki JE, Sandroff BM, Bamman MM, et al. Motion sensors in multiple sclerosis: narrative review and update of applications. Expert Rev Med Devices. 2017;14:891–900.
Sandroff BM, Motl RW, Suh Y. Accelerometer output and its association with energy expenditure in persons with multiple sclerosis. J Rehabil Res Dev. 2012;49:467–75.
Klaren RE, Sandroff BM, Fernhall B, et al. Comprehensive profile of cardiopulmonary exercise testing in ambulatory persons with multiple sclerosis. Sports Med. 2016;46:1365–79.
Motl RW, Fernhall B. Accurate prediction of cardiorespiratory fitness using cycle ergometry in minimally disabled persons with relapsing-remitting multiple sclerosis. Arch Phys Med Rehabil. 2012;93:490–5.
Sandroff BM, Sosnoff JJ, Motl RW. Physical fitness, walking performance, and gait in multiple sclerosis. J Neurol Sci. 2013;328:70–6.
Holt CS, Heimberg RG. The reaction to treatment questionnaire: measuring treatment credibility and outcome expectancies. Behav Ther. 1990;13(213–214):222.
Weikert M, Dlugonski D, Balantrapu S, et al. Most common types of physical activity self-selected by people with multiple sclerosis. Int J MS Care. 2011;13:16–20.
Agiovlasitis S, Sandroff BM, Motl RW. Step-rate cut-points for physical activity intensity in patients with multiple sclerosis: the effect of disability status. J Neurol Sci. 2016;361:95–100.
Sandroff BM, Motl RW, Bamman M, et al. Rationale and design of a single-blind, randomized controlled trial of exercise training for managing learning and memory impairment in persons with multiple sclerosis. BMJ Open. 2018;8:e023231.
Sandroff BM, Diggs MD, Bamman MM, et al. Protocol for a systematically-developed, Phase I/II, single-blind, randomized controlled trial of treadmill walking exercise training effects on cognition and brain function in persons with multiple sclerosis. Contemp Clin Trials. 2019;87:105878.
Benjamini Y, Liu W. A step-down multiple hypotheses testing procedure that controls the false discovery rate under independence. J Stat Planning Inference. 1999;82(1–2):163–70.
Norman GR, Sloan JA, Wyrwich KW. The truly remarkable universality of half a standard deviation: Confirmation through another look. Expert Rev Pharmacoecon Outcomes Res. 2004;4:581–5.
Motl RW, Sandroff BM, Wingo BC, et al. Phase-III randomized controlled trial of the Behavioral Intervention for increasing physical activity in multiple sclerosis: project BIPAMS. Contemp Clin Trials. 2018;71:154–61.
Motl RW, Sandroff BM, Kwakkel G, et al. Exercise in patients with multiple sclerosis. Lancet Neurol. 2017;16:848–56.
Motl RW, Sandroff BM. Randomized controlled trial of physical activity intervention effects on fatigue and depression in multiple sclerosis: secondary analysis of data from persons with elevated symptom status. Contemp Clin Trials Commun. 2020;17:100521.
Dlugonski D, Motl RW, Mohr DC, et al. Internet-delivered behavioral intervention to increase physical activity in persons with multiple sclerosis: sustainability and secondary outcomes. Psychol Health Med. 2012;17:636–51.
Download references
Acknowledgements
The authors recognize the critical role of the behavioral coaches in the delivery of the intervention and control conditions.
This project was funded by a grant from the Congressionally Directed Medical Research Programs – Multiple Sclerosis Research Program (W81XWH2110952). The study sponsor had no role in study design.
Author information
Authors and affiliations.
Department of Kinesiology and Nutrition, University of Illinois at Chicago, Chicago, IL, 60612, USA
Robert W. Motl, Brooks Hibner & Alison Wathen
Department of Rehabilitation Medicine, University of Washington, Seattle, WA, USA
Charles H. Bombardier
Department of Psychiatry, University of Illinois at Chicago, Chicago, IL, USA
Jennifer Duffecy
Department of Neurology and Rehabilitation, University of Illinois at Chicago, Chicago, IL, USA
Michael Carrithers
Department of Biostatistics, University of Alabama at Birmingham, Birmingham, AL, USA
Gary Cutter
You can also search for this author in PubMed Google Scholar
Contributions
The manuscript was drafted by RWM. All other authors read and critically evaluated the manuscript, and approved it before submission. The PI of the study is RWM. The project coordinator is AW. Data management and analysis is guided by GC. CHB, JD, and BH provide ongoing insights on study management and quality control. CHB and JD provide guidance on all cases of suicidal risk. BH provides oversite of intervention delivery and outcome assessments. MC is the study neurologist and safety officer.
Corresponding author
Correspondence to Robert W. Motl .
Ethics declarations
Ethics approval and consent to participate.
The protocol received approval on December 14, 2022 from the UIC Institutional Review Board (2022–1354) and all participants provide written informed consent.
Consent for publication
Competing interests.
The authors declare no competing interests.
Additional information
Publisher’s note.
Springer Nature remains neutral with regard to jurisdictional claims in published maps and institutional affiliations.
Supplementary Information
Supplementary material 1., rights and permissions.
Open Access This article is licensed under a Creative Commons Attribution 4.0 International License, which permits use, sharing, adaptation, distribution and reproduction in any medium or format, as long as you give appropriate credit to the original author(s) and the source, provide a link to the Creative Commons licence, and indicate if changes were made. The images or other third party material in this article are included in the article's Creative Commons licence, unless indicated otherwise in a credit line to the material. If material is not included in the article's Creative Commons licence and your intended use is not permitted by statutory regulation or exceeds the permitted use, you will need to obtain permission directly from the copyright holder. To view a copy of this licence, visit http://creativecommons.org/licenses/by/4.0/ . The Creative Commons Public Domain Dedication waiver ( http://creativecommons.org/publicdomain/zero/1.0/ ) applies to the data made available in this article, unless otherwise stated in a credit line to the data.
Reprints and permissions
About this article
Cite this article.
Motl, R.W., Bombardier, C.H., Duffecy, J. et al. Study protocol: exercise training for treating major depressive disorder in multiple sclerosis. BMC Neurol 24 , 131 (2024). https://doi.org/10.1186/s12883-024-03634-y
Download citation
Received : 05 March 2024
Accepted : 10 April 2024
Published : 17 April 2024
DOI : https://doi.org/10.1186/s12883-024-03634-y
Share this article
Anyone you share the following link with will be able to read this content:
Sorry, a shareable link is not currently available for this article.
Provided by the Springer Nature SharedIt content-sharing initiative
- Physical activity
- Mental health
- Behavior change
- Multiple sclerosis
- Neurological disease
BMC Neurology
ISSN: 1471-2377
- General enquiries: [email protected]
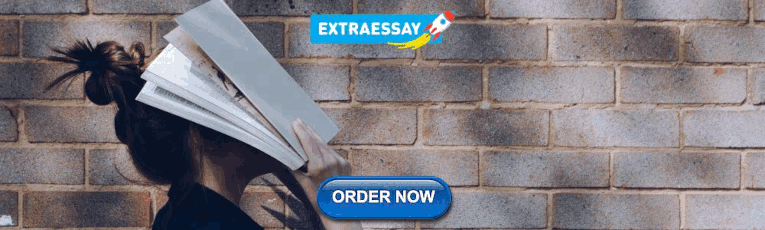
IMAGES
VIDEO
COMMENTS
Revised on June 22, 2023. In experimental research, random assignment is a way of placing participants from your sample into different treatment groups using randomization. With simple random assignment, every member of the sample has a known or equal chance of being placed in a control group or an experimental group.
Random selection (also called probability sampling or random sampling) is a way of randomly selecting members of a population to be included in your study. On the other hand, random assignment is a way of sorting the sample participants into control and treatment groups. Random selection ensures that everyone in the population has an equal ...
Random assignment helps you separation causation from correlation and rule out confounding variables. As a critical component of the scientific method, experiments typically set up contrasts between a control group and one or more treatment groups. The idea is to determine whether the effect, which is the difference between a treatment group ...
Random assignment refers to the use of chance procedures in psychology experiments to ensure that each participant has the same opportunity to be assigned to any given group in a study to eliminate any potential bias in the experiment at the outset. Participants are randomly assigned to different groups, such as the treatment group versus the control group.
Random assignment or random placement is an experimental technique for assigning human participants or animal subjects to different groups in an experiment (e.g., a treatment group versus a control group) using randomization, such as by a chance procedure (e.g., flipping a coin) or a random number generator. This ensures that each participant or subject has an equal chance of being placed in ...
A control group is a set of participants in a study who do not receive the treatment or intervention being tested, serving as a baseline to compare with the group that does, in order to assess the effectiveness of the treatment. Smoking Cessation Study: Researchers used random assignment to put participants into two groups.
Control group: Does not consume vitamin supplements; Treatment group: Regularly consumes vitamin supplements.; In this experiment, we randomly assign subjects to the two groups. Because we use random assignment, the two groups start with similar characteristics, including healthy habits, physical attributes, medical conditions, and other factors affecting the outcome.
No random assignment: Can detect relationships in population, but cannot determine causality. ... There are some unstated assumptions, for instance that the treatment and control groups are similar in terms of demographic makeup, health, health-related habits, etc. To the extent the assumptions hold true, however, the differentiating factor ...
Random assignment means participants are assigned to different groups or conditions in a study purely by chance. Basically, each participant has an equal chance to be assigned to a control group or a treatment group. Field experiments, or randomized studies conducted in real-world settings, can take many forms.
Random assignment in psychology involves each participant having an equal chance of being chosen for any of the groups, including the control and experimental groups. It helps control for potential confounding variables, reducing the likelihood of pre-existing differences between groups. This method enhances the internal validity of experiments ...
control group and random assignment. This is an improvement over the one-shot case study because the researcher measures the de-pendent variable both before and after the treatment. But it lacks a control group. The researcher cannot know whether something other than the treatment occurred between the pretest and the posttest to cause the ...
Random assignment is a method for assigning participants in a sample to the different conditions, and it is an important element of all experimental research in psychology and other fields too. In its strictest sense, random assignment should meet two criteria. One is that each participant has an equal chance of being assigned to each condition ...
With random assignment, all participants have an equal chance of being assigned to either group. There is statistical software that will randomly assign each of the algebra students in the sample to either the experimental or the control group. Random assignment is critical for sound experimental design. With sufficiently large samples, random ...
Why Researchers Use Random Assignment. Researchers use random assignment to control for confounds in research. Confounds refer to unwanted and often unaccounted-for variables that might affect the outcome of a study. These confounding variables can skew the results, rendering the experiment unreliable. For example, below is a study with two groups.
Random assignment uses a random number generator or some other random process to assign people into experimental and control groups. Random assignment is important in experimental research because it helps to ensure that the experimental group and control group are comparable and that any differences between the experimental and control groups ...
These designs are often depicted using a standardised design notation, where represents random assignment of subjects to groups, ... testing, and regression, since these threats can be expected to influence both treatment and control groups in a similar (random) manner. The selection threat is controlled via random assignment. However ...
Random assignment of participants helps to ensure that any differences between and within the groups are not systematic at the outset of the experiment. Thus, any differences between groups recorded at the end of the experiment can be more confidently attributed to the experimental procedures or treatment. … Random assignment does not ...
Comparison of assignment to groups using (a) variance minimisation and (b) random assignment.When a new participant joins a study, variance minimisation assigns the participant to the group that minimises the variance between groups along with the pre-defined variables (i.e., V); in this case intelligence (IQ), executive functions (EFs), attentional performance (AP), and gender, while keeping ...
In this video, Dr. Kushner outlines how to conduct a psychology experiment. The experimental method is a powerful tool for psychologists because it is the on...
This technique maintains complete randomness of the assignment of a subject to a particular group. The most common and basic method of simple randomization is flipping a coin. For example, with two treatment groups (control versus treatment), the side of the coin (i.e., heads - control, tails - treatment) determines the assignment of each subject.
Without random assignment of treatments, treated and control groups may not have been comparable prior to treatment, so differing outcomes may reflect either biases from these pretreatment differences or else effects actually caused by the treatment. The difficulty in distinguishing treatment effects from biases is the key consequence of the ...
So, to summarize, random sampling refers to how you select individuals from the population to participate in your study. Random assignment refers to how you place those participants into groups (such as experimental vs. control). Knowing this distinction will help you clearly and accurately describe the methods you use to collect your data and ...
Chapter 7: Experimental Research Designs. experiment. Click the card to flip 👆. a research method which can confidently assert a causal relation between the independent and dependent variables; includes manipulation and random assignment; tend to be very high in internal validity. Click the card to flip 👆.
Students were randomized to one of two groups: an intervention group who watched videos about the consequences and benefits of stress and completed mental imagery tasks applying the benefits of stress in their own lives (one session of an unspecified length of time), or a control group who completed a mental imagery task unrelated to reframing ...
Random assignment. After collection of baseline data, participants will be randomly assigned into either the exercise training condition or the control condition using a computerized process based on a random numbers sequence, and group allocation will be concealed.