Machine Learning - CMU

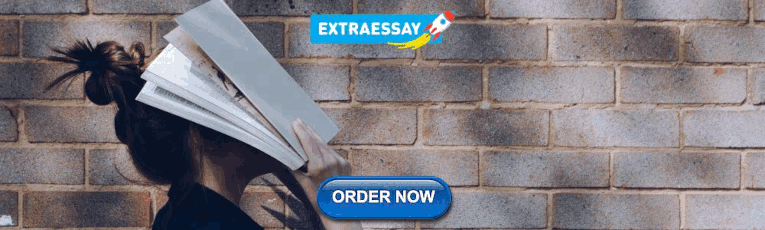
PhD Dissertations
[all are .pdf files].
Learning Models that Match Jacob Tyo, 2024
Improving Human Integration across the Machine Learning Pipeline Charvi Rastogi, 2024
Reliable and Practical Machine Learning for Dynamic Healthcare Settings Helen Zhou, 2023
Automatic customization of large-scale spiking network models to neuronal population activity (unavailable) Shenghao Wu, 2023
Estimation of BVk functions from scattered data (unavailable) Addison J. Hu, 2023
Rethinking object categorization in computer vision (unavailable) Jayanth Koushik, 2023
Advances in Statistical Gene Networks Jinjin Tian, 2023 Post-hoc calibration without distributional assumptions Chirag Gupta, 2023
The Role of Noise, Proxies, and Dynamics in Algorithmic Fairness Nil-Jana Akpinar, 2023
Collaborative learning by leveraging siloed data Sebastian Caldas, 2023
Modeling Epidemiological Time Series Aaron Rumack, 2023
Human-Centered Machine Learning: A Statistical and Algorithmic Perspective Leqi Liu, 2023
Uncertainty Quantification under Distribution Shifts Aleksandr Podkopaev, 2023
Probabilistic Reinforcement Learning: Using Data to Define Desired Outcomes, and Inferring How to Get There Benjamin Eysenbach, 2023
Comparing Forecasters and Abstaining Classifiers Yo Joong Choe, 2023
Using Task Driven Methods to Uncover Representations of Human Vision and Semantics Aria Yuan Wang, 2023
Data-driven Decisions - An Anomaly Detection Perspective Shubhranshu Shekhar, 2023
Applied Mathematics of the Future Kin G. Olivares, 2023
METHODS AND APPLICATIONS OF EXPLAINABLE MACHINE LEARNING Joon Sik Kim, 2023
NEURAL REASONING FOR QUESTION ANSWERING Haitian Sun, 2023
Principled Machine Learning for Societally Consequential Decision Making Amanda Coston, 2023
Long term brain dynamics extend cognitive neuroscience to timescales relevant for health and physiology Maxwell B. Wang, 2023
Long term brain dynamics extend cognitive neuroscience to timescales relevant for health and physiology Darby M. Losey, 2023
Calibrated Conditional Density Models and Predictive Inference via Local Diagnostics David Zhao, 2023
Towards an Application-based Pipeline for Explainability Gregory Plumb, 2022
Objective Criteria for Explainable Machine Learning Chih-Kuan Yeh, 2022
Making Scientific Peer Review Scientific Ivan Stelmakh, 2022
Facets of regularization in high-dimensional learning: Cross-validation, risk monotonization, and model complexity Pratik Patil, 2022
Active Robot Perception using Programmable Light Curtains Siddharth Ancha, 2022
Strategies for Black-Box and Multi-Objective Optimization Biswajit Paria, 2022
Unifying State and Policy-Level Explanations for Reinforcement Learning Nicholay Topin, 2022
Sensor Fusion Frameworks for Nowcasting Maria Jahja, 2022
Equilibrium Approaches to Modern Deep Learning Shaojie Bai, 2022
Towards General Natural Language Understanding with Probabilistic Worldbuilding Abulhair Saparov, 2022
Applications of Point Process Modeling to Spiking Neurons (Unavailable) Yu Chen, 2021
Neural variability: structure, sources, control, and data augmentation Akash Umakantha, 2021
Structure and time course of neural population activity during learning Jay Hennig, 2021
Cross-view Learning with Limited Supervision Yao-Hung Hubert Tsai, 2021
Meta Reinforcement Learning through Memory Emilio Parisotto, 2021
Learning Embodied Agents with Scalably-Supervised Reinforcement Learning Lisa Lee, 2021
Learning to Predict and Make Decisions under Distribution Shift Yifan Wu, 2021
Statistical Game Theory Arun Sai Suggala, 2021
Towards Knowledge-capable AI: Agents that See, Speak, Act and Know Kenneth Marino, 2021
Learning and Reasoning with Fast Semidefinite Programming and Mixing Methods Po-Wei Wang, 2021
Bridging Language in Machines with Language in the Brain Mariya Toneva, 2021
Curriculum Learning Otilia Stretcu, 2021
Principles of Learning in Multitask Settings: A Probabilistic Perspective Maruan Al-Shedivat, 2021
Towards Robust and Resilient Machine Learning Adarsh Prasad, 2021
Towards Training AI Agents with All Types of Experiences: A Unified ML Formalism Zhiting Hu, 2021
Building Intelligent Autonomous Navigation Agents Devendra Chaplot, 2021
Learning to See by Moving: Self-supervising 3D Scene Representations for Perception, Control, and Visual Reasoning Hsiao-Yu Fish Tung, 2021
Statistical Astrophysics: From Extrasolar Planets to the Large-scale Structure of the Universe Collin Politsch, 2020
Causal Inference with Complex Data Structures and Non-Standard Effects Kwhangho Kim, 2020
Networks, Point Processes, and Networks of Point Processes Neil Spencer, 2020
Dissecting neural variability using population recordings, network models, and neurofeedback (Unavailable) Ryan Williamson, 2020
Predicting Health and Safety: Essays in Machine Learning for Decision Support in the Public Sector Dylan Fitzpatrick, 2020
Towards a Unified Framework for Learning and Reasoning Han Zhao, 2020
Learning DAGs with Continuous Optimization Xun Zheng, 2020
Machine Learning and Multiagent Preferences Ritesh Noothigattu, 2020
Learning and Decision Making from Diverse Forms of Information Yichong Xu, 2020
Towards Data-Efficient Machine Learning Qizhe Xie, 2020
Change modeling for understanding our world and the counterfactual one(s) William Herlands, 2020
Machine Learning in High-Stakes Settings: Risks and Opportunities Maria De-Arteaga, 2020
Data Decomposition for Constrained Visual Learning Calvin Murdock, 2020
Structured Sparse Regression Methods for Learning from High-Dimensional Genomic Data Micol Marchetti-Bowick, 2020
Towards Efficient Automated Machine Learning Liam Li, 2020
LEARNING COLLECTIONS OF FUNCTIONS Emmanouil Antonios Platanios, 2020
Provable, structured, and efficient methods for robustness of deep networks to adversarial examples Eric Wong , 2020
Reconstructing and Mining Signals: Algorithms and Applications Hyun Ah Song, 2020
Probabilistic Single Cell Lineage Tracing Chieh Lin, 2020
Graphical network modeling of phase coupling in brain activity (unavailable) Josue Orellana, 2019
Strategic Exploration in Reinforcement Learning - New Algorithms and Learning Guarantees Christoph Dann, 2019 Learning Generative Models using Transformations Chun-Liang Li, 2019
Estimating Probability Distributions and their Properties Shashank Singh, 2019
Post-Inference Methods for Scalable Probabilistic Modeling and Sequential Decision Making Willie Neiswanger, 2019
Accelerating Text-as-Data Research in Computational Social Science Dallas Card, 2019
Multi-view Relationships for Analytics and Inference Eric Lei, 2019
Information flow in networks based on nonstationary multivariate neural recordings Natalie Klein, 2019
Competitive Analysis for Machine Learning & Data Science Michael Spece, 2019
The When, Where and Why of Human Memory Retrieval Qiong Zhang, 2019
Towards Effective and Efficient Learning at Scale Adams Wei Yu, 2019
Towards Literate Artificial Intelligence Mrinmaya Sachan, 2019
Learning Gene Networks Underlying Clinical Phenotypes Under SNP Perturbations From Genome-Wide Data Calvin McCarter, 2019
Unified Models for Dynamical Systems Carlton Downey, 2019
Anytime Prediction and Learning for the Balance between Computation and Accuracy Hanzhang Hu, 2019
Statistical and Computational Properties of Some "User-Friendly" Methods for High-Dimensional Estimation Alnur Ali, 2019
Nonparametric Methods with Total Variation Type Regularization Veeranjaneyulu Sadhanala, 2019
New Advances in Sparse Learning, Deep Networks, and Adversarial Learning: Theory and Applications Hongyang Zhang, 2019
Gradient Descent for Non-convex Problems in Modern Machine Learning Simon Shaolei Du, 2019
Selective Data Acquisition in Learning and Decision Making Problems Yining Wang, 2019
Anomaly Detection in Graphs and Time Series: Algorithms and Applications Bryan Hooi, 2019
Neural dynamics and interactions in the human ventral visual pathway Yuanning Li, 2018
Tuning Hyperparameters without Grad Students: Scaling up Bandit Optimisation Kirthevasan Kandasamy, 2018
Teaching Machines to Classify from Natural Language Interactions Shashank Srivastava, 2018
Statistical Inference for Geometric Data Jisu Kim, 2018
Representation Learning @ Scale Manzil Zaheer, 2018
Diversity-promoting and Large-scale Machine Learning for Healthcare Pengtao Xie, 2018
Distribution and Histogram (DIsH) Learning Junier Oliva, 2018
Stress Detection for Keystroke Dynamics Shing-Hon Lau, 2018
Sublinear-Time Learning and Inference for High-Dimensional Models Enxu Yan, 2018
Neural population activity in the visual cortex: Statistical methods and application Benjamin Cowley, 2018
Efficient Methods for Prediction and Control in Partially Observable Environments Ahmed Hefny, 2018
Learning with Staleness Wei Dai, 2018
Statistical Approach for Functionally Validating Transcription Factor Bindings Using Population SNP and Gene Expression Data Jing Xiang, 2017
New Paradigms and Optimality Guarantees in Statistical Learning and Estimation Yu-Xiang Wang, 2017
Dynamic Question Ordering: Obtaining Useful Information While Reducing User Burden Kirstin Early, 2017
New Optimization Methods for Modern Machine Learning Sashank J. Reddi, 2017
Active Search with Complex Actions and Rewards Yifei Ma, 2017
Why Machine Learning Works George D. Montañez , 2017
Source-Space Analyses in MEG/EEG and Applications to Explore Spatio-temporal Neural Dynamics in Human Vision Ying Yang , 2017
Computational Tools for Identification and Analysis of Neuronal Population Activity Pengcheng Zhou, 2016
Expressive Collaborative Music Performance via Machine Learning Gus (Guangyu) Xia, 2016
Supervision Beyond Manual Annotations for Learning Visual Representations Carl Doersch, 2016
Exploring Weakly Labeled Data Across the Noise-Bias Spectrum Robert W. H. Fisher, 2016
Optimizing Optimization: Scalable Convex Programming with Proximal Operators Matt Wytock, 2016
Combining Neural Population Recordings: Theory and Application William Bishop, 2015
Discovering Compact and Informative Structures through Data Partitioning Madalina Fiterau-Brostean, 2015
Machine Learning in Space and Time Seth R. Flaxman, 2015
The Time and Location of Natural Reading Processes in the Brain Leila Wehbe, 2015
Shape-Constrained Estimation in High Dimensions Min Xu, 2015
Spectral Probabilistic Modeling and Applications to Natural Language Processing Ankur Parikh, 2015 Computational and Statistical Advances in Testing and Learning Aaditya Kumar Ramdas, 2015
Corpora and Cognition: The Semantic Composition of Adjectives and Nouns in the Human Brain Alona Fyshe, 2015
Learning Statistical Features of Scene Images Wooyoung Lee, 2014
Towards Scalable Analysis of Images and Videos Bin Zhao, 2014
Statistical Text Analysis for Social Science Brendan T. O'Connor, 2014
Modeling Large Social Networks in Context Qirong Ho, 2014
Semi-Cooperative Learning in Smart Grid Agents Prashant P. Reddy, 2013
On Learning from Collective Data Liang Xiong, 2013
Exploiting Non-sequence Data in Dynamic Model Learning Tzu-Kuo Huang, 2013
Mathematical Theories of Interaction with Oracles Liu Yang, 2013
Short-Sighted Probabilistic Planning Felipe W. Trevizan, 2013
Statistical Models and Algorithms for Studying Hand and Finger Kinematics and their Neural Mechanisms Lucia Castellanos, 2013
Approximation Algorithms and New Models for Clustering and Learning Pranjal Awasthi, 2013
Uncovering Structure in High-Dimensions: Networks and Multi-task Learning Problems Mladen Kolar, 2013
Learning with Sparsity: Structures, Optimization and Applications Xi Chen, 2013
GraphLab: A Distributed Abstraction for Large Scale Machine Learning Yucheng Low, 2013
Graph Structured Normal Means Inference James Sharpnack, 2013 (Joint Statistics & ML PhD)
Probabilistic Models for Collecting, Analyzing, and Modeling Expression Data Hai-Son Phuoc Le, 2013
Learning Large-Scale Conditional Random Fields Joseph K. Bradley, 2013
New Statistical Applications for Differential Privacy Rob Hall, 2013 (Joint Statistics & ML PhD)
Parallel and Distributed Systems for Probabilistic Reasoning Joseph Gonzalez, 2012
Spectral Approaches to Learning Predictive Representations Byron Boots, 2012
Attribute Learning using Joint Human and Machine Computation Edith L. M. Law, 2012
Statistical Methods for Studying Genetic Variation in Populations Suyash Shringarpure, 2012
Data Mining Meets HCI: Making Sense of Large Graphs Duen Horng (Polo) Chau, 2012
Learning with Limited Supervision by Input and Output Coding Yi Zhang, 2012
Target Sequence Clustering Benjamin Shih, 2011
Nonparametric Learning in High Dimensions Han Liu, 2010 (Joint Statistics & ML PhD)
Structural Analysis of Large Networks: Observations and Applications Mary McGlohon, 2010
Modeling Purposeful Adaptive Behavior with the Principle of Maximum Causal Entropy Brian D. Ziebart, 2010
Tractable Algorithms for Proximity Search on Large Graphs Purnamrita Sarkar, 2010
Rare Category Analysis Jingrui He, 2010
Coupled Semi-Supervised Learning Andrew Carlson, 2010
Fast Algorithms for Querying and Mining Large Graphs Hanghang Tong, 2009
Efficient Matrix Models for Relational Learning Ajit Paul Singh, 2009
Exploiting Domain and Task Regularities for Robust Named Entity Recognition Andrew O. Arnold, 2009
Theoretical Foundations of Active Learning Steve Hanneke, 2009
Generalized Learning Factors Analysis: Improving Cognitive Models with Machine Learning Hao Cen, 2009
Detecting Patterns of Anomalies Kaustav Das, 2009
Dynamics of Large Networks Jurij Leskovec, 2008
Computational Methods for Analyzing and Modeling Gene Regulation Dynamics Jason Ernst, 2008
Stacked Graphical Learning Zhenzhen Kou, 2007
Actively Learning Specific Function Properties with Applications to Statistical Inference Brent Bryan, 2007
Approximate Inference, Structure Learning and Feature Estimation in Markov Random Fields Pradeep Ravikumar, 2007
Scalable Graphical Models for Social Networks Anna Goldenberg, 2007
Measure Concentration of Strongly Mixing Processes with Applications Leonid Kontorovich, 2007
Tools for Graph Mining Deepayan Chakrabarti, 2005
Automatic Discovery of Latent Variable Models Ricardo Silva, 2005
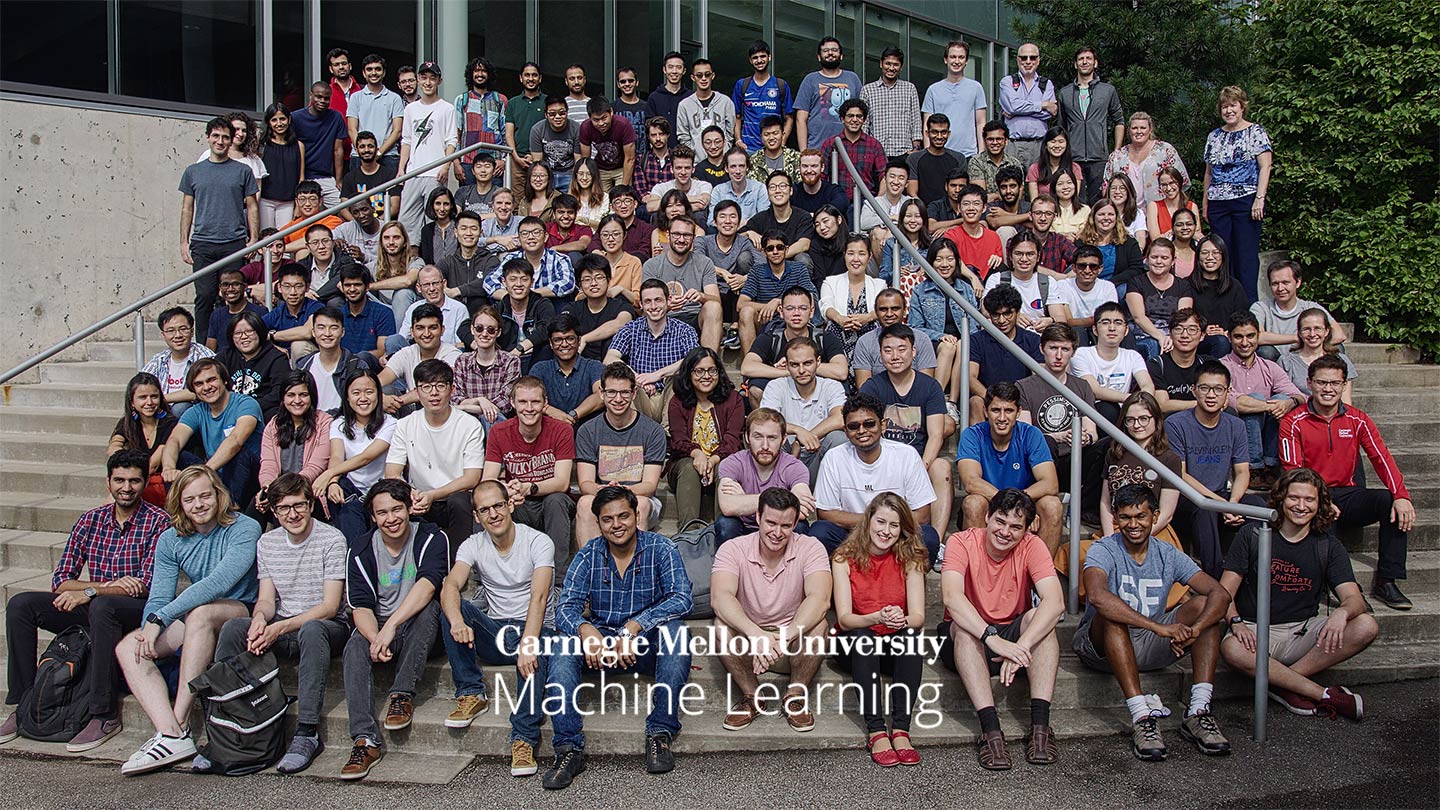
- Warning : Invalid argument supplied for foreach() in /home/customer/www/opendatascience.com/public_html/wp-includes/nav-menu.php on line 95 Warning : array_merge(): Expected parameter 2 to be an array, null given in /home/customer/www/opendatascience.com/public_html/wp-includes/nav-menu.php on line 102
- ODSC EUROPE
- AI+ Training
- Speak at ODSC

- Data Analytics
- Data Engineering
- Data Visualization
- Deep Learning
- Generative AI
- Machine Learning
- NLP and LLMs
- Business & Use Cases
- Career Advice
- Write for us
- ODSC Community Slack Channel
- Upcoming Webinars

10 Compelling Machine Learning Ph.D. Dissertations for 2020
Machine Learning Modeling Research posted by Daniel Gutierrez, ODSC August 19, 2020 Daniel Gutierrez, ODSC
As a data scientist, an integral part of my work in the field revolves around keeping current with research coming out of academia. I frequently scour arXiv.org for late-breaking papers that show trends and reveal fertile areas of research. Other sources of valuable research developments are in the form of Ph.D. dissertations, the culmination of a doctoral candidate’s work to confer his/her degree. Ph.D. candidates are highly motivated to choose research topics that establish new and creative paths toward discovery in their field of study. Their dissertations are highly focused on a specific problem. If you can find a dissertation that aligns with your areas of interest, consuming the research is an excellent way to do a deep dive into the technology. After reviewing hundreds of recent theses from universities all over the country, I present 10 machine learning dissertations that I found compelling in terms of my own areas of interest.
[Related article: Introduction to Bayesian Deep Learning ]
I hope you’ll find several that match your own fields of inquiry. Each thesis may take a while to consume but will result in hours of satisfying summer reading. Enjoy!
1. Bayesian Modeling and Variable Selection for Complex Data
As we routinely encounter high-throughput data sets in complex biological and environmental research, developing novel models and methods for variable selection has received widespread attention. This dissertation addresses a few key challenges in Bayesian modeling and variable selection for high-dimensional data with complex spatial structures.
2. Topics in Statistical Learning with a Focus on Large Scale Data
Big data vary in shape and call for different approaches. One type of big data is the tall data, i.e., a very large number of samples but not too many features. This dissertation describes a general communication-efficient algorithm for distributed statistical learning on this type of big data. The algorithm distributes the samples uniformly to multiple machines, and uses a common reference data to improve the performance of local estimates. The algorithm enables potentially much faster analysis, at a small cost to statistical performance.
Another type of big data is the wide data, i.e., too many features but a limited number of samples. It is also called high-dimensional data, to which many classical statistical methods are not applicable.
This dissertation discusses a method of dimensionality reduction for high-dimensional classification. The method partitions features into independent communities and splits the original classification problem into separate smaller ones. It enables parallel computing and produces more interpretable results.
3. Sets as Measures: Optimization and Machine Learning
The purpose of this machine learning dissertation is to address the following simple question:
How do we design efficient algorithms to solve optimization or machine learning problems where the decision variable (or target label) is a set of unknown cardinality?
Optimization and machine learning have proved remarkably successful in applications requiring the choice of single vectors. Some tasks, in particular many inverse problems, call for the design, or estimation, of sets of objects. When the size of these sets is a priori unknown, directly applying optimization or machine learning techniques designed for single vectors appears difficult. The work in this dissertation shows that a very old idea for transforming sets into elements of a vector space (namely, a space of measures), a common trick in theoretical analysis, generates effective practical algorithms.
4. A Geometric Perspective on Some Topics in Statistical Learning
Modern science and engineering often generate data sets with a large sample size and a comparably large dimension which puts classic asymptotic theory into question in many ways. Therefore, the main focus of this dissertation is to develop a fundamental understanding of statistical procedures for estimation and hypothesis testing from a non-asymptotic point of view, where both the sample size and problem dimension grow hand in hand. A range of different problems are explored in this thesis, including work on the geometry of hypothesis testing, adaptivity to local structure in estimation, effective methods for shape-constrained problems, and early stopping with boosting algorithms. The treatment of these different problems shares the common theme of emphasizing the underlying geometric structure.
5. Essays on Random Forest Ensembles
A random forest is a popular machine learning ensemble method that has proven successful in solving a wide range of classification problems. While other successful classifiers, such as boosting algorithms or neural networks, admit natural interpretations as maximum likelihood, a suitable statistical interpretation is much more elusive for a random forest. The first part of this dissertation demonstrates that a random forest is a fruitful framework in which to study AdaBoost and deep neural networks. The work explores the concept and utility of interpolation, the ability of a classifier to perfectly fit its training data. The second part of this dissertation places a random forest on more sound statistical footing by framing it as kernel regression with the proximity kernel. The work then analyzes the parameters that control the bandwidth of this kernel and discuss useful generalizations.
6. Marginally Interpretable Generalized Linear Mixed Models
A popular approach for relating correlated measurements of a non-Gaussian response variable to a set of predictors is to introduce latent random variables and fit a generalized linear mixed model. The conventional strategy for specifying such a model leads to parameter estimates that must be interpreted conditional on the latent variables. In many cases, interest lies not in these conditional parameters, but rather in marginal parameters that summarize the average effect of the predictors across the entire population. Due to the structure of the generalized linear mixed model, the average effect across all individuals in a population is generally not the same as the effect for an average individual. Further complicating matters, obtaining marginal summaries from a generalized linear mixed model often requires evaluation of an analytically intractable integral or use of an approximation. Another popular approach in this setting is to fit a marginal model using generalized estimating equations. This strategy is effective for estimating marginal parameters, but leaves one without a formal model for the data with which to assess quality of fit or make predictions for future observations. Thus, there exists a need for a better approach.
This dissertation defines a class of marginally interpretable generalized linear mixed models that leads to parameter estimates with a marginal interpretation while maintaining the desirable statistical properties of a conditionally specified model. The distinguishing feature of these models is an additive adjustment that accounts for the curvature of the link function and thereby preserves a specific form for the marginal mean after integrating out the latent random variables.
7. On the Detection of Hate Speech, Hate Speakers and Polarized Groups in Online Social Media
The objective of this dissertation is to explore the use of machine learning algorithms in understanding and detecting hate speech, hate speakers and polarized groups in online social media. Beginning with a unique typology for detecting abusive language, the work outlines the distinctions and similarities of different abusive language subtasks (offensive language, hate speech, cyberbullying and trolling) and how we might benefit from the progress made in each area. Specifically, the work suggests that each subtask can be categorized based on whether or not the abusive language being studied 1) is directed at a specific individual, or targets a generalized “Other” and 2) the extent to which the language is explicit versus implicit. The work then uses knowledge gained from this typology to tackle the “problem of offensive language” in hate speech detection.
8. Lasso Guarantees for Dependent Data
Serially correlated high dimensional data are prevalent in the big data era. In order to predict and learn the complex relationship among the multiple time series, high dimensional modeling has gained importance in various fields such as control theory, statistics, economics, finance, genetics and neuroscience. This dissertation studies a number of high dimensional statistical problems involving different classes of mixing processes.
9. Random forest robustness, variable importance, and tree aggregation
Random forest methodology is a nonparametric, machine learning approach capable of strong performance in regression and classification problems involving complex data sets. In addition to making predictions, random forests can be used to assess the relative importance of feature variables. This dissertation explores three topics related to random forests: tree aggregation, variable importance, and robustness.
10. Climate Data Computing: Optimal Interpolation, Averaging, Visualization and Delivery
This dissertation solves two important problems in the modern analysis of big climate data. The first is the efficient visualization and fast delivery of big climate data, and the second is a computationally extensive principal component analysis (PCA) using spherical harmonics on the Earth’s surface. The second problem creates a way to supply the data for the technology developed in the first. These two problems are computationally difficult, such as the representation of higher order spherical harmonics Y400, which is critical for upscaling weather data to almost infinitely fine spatial resolution.
I hope you enjoyed learning about these compelling machine learning dissertations.
Editor’s note: Interested in more data science research? Check out the Research Frontiers track at ODSC Europe this September 17-19 or the ODSC West Research Frontiers track this October 27-30.

Daniel Gutierrez, ODSC
Daniel D. Gutierrez is a practicing data scientist who’s been working with data long before the field came in vogue. As a technology journalist, he enjoys keeping a pulse on this fast-paced industry. Daniel is also an educator having taught data science, machine learning and R classes at the university level. He has authored four computer industry books on database and data science technology, including his most recent title, “Machine Learning and Data Science: An Introduction to Statistical Learning Methods with R.” Daniel holds a BS in Mathematics and Computer Science from UCLA.

Meta Sees Free Models as its Future
AI and Data Science News posted by ODSC Team Apr 27, 2024 A few days ago, Meta introduced Llama 3, its latest advanced AI model, to the public...

ODSC’s AI Weekly Recap: Week of April 26th
AI and Data Science News posted by Jorge Arenas Apr 26, 2024 Every week, the ODSC team researches the latest advancements in AI. We review a selection of...

New AI Models From Apple May Find Home in Future iPhones
AI and Data Science News posted by ODSC Team Apr 25, 2024 In a report from the Independent AI, Apple researchers have introduced a series of new AI...

MIT Libraries home DSpace@MIT
- DSpace@MIT Home
- MIT Libraries
- Doctoral Theses
On optimization and scalability in deep learning
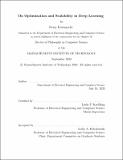
Other Contributors
Terms of use, description, date issued, collections.
- Faculty of Arts and Sciences
- FAS Theses and Dissertations
- Communities & Collections
- By Issue Date
- FAS Department
- Quick submit
- Waiver Generator
- DASH Stories
- Accessibility
- COVID-related Research
Terms of Use
- Privacy Policy
- By Collections
- By Departments
Enabling High Performance, Efficient, and Sustainable Deep Learning Systems At Scale
Citable link to this page
Collections.
- FAS Theses and Dissertations [6136]
Contact administrator regarding this item (to report mistakes or request changes)
Uncertainty in Deep Learning (PhD Thesis)
October 13th, 2016 (Updated: June 4th, 2017)
Tweet Share

Function draws from a dropout neural network. This new visualisation technique depicts the distribution over functions rather than the predictive distribution (see demo below ).
Thesis: uncertainty in deep learning.
- some discussions : a discussion of AI safety and model uncertainty ( §1.3 ), a historical survey of Bayesian neural networks ( §2.2 ),
- some theoretical analysis : a theoretical analysis of the variance of the re-parametrisation trick and other Monte Carlo estimators used in variational inference (the re-parametrisation trick is not a universal variance reduction technique! §3.1.1 – §3.1.2 ), a survey of measures of uncertainty in classification tasks ( §3.3.1 ),
- some empirical results : an empirical analysis of different Bayesian neural network priors ( §4.1 ) and posteriors with various approximating distributions ( §4.2 ), new quantitative results comparing dropout to existing techniques ( §4.3 ), tools for heteroscedastic model uncertainty in Bayesian neural networks ( §4.6 ),
- some applications : a survey of recent applications in language, biology, medicine, and computer vision making use of the tools presented in this thesis ( §5.1 ), new applications in active learning with image data ( §5.2 ),
- and more theoretical results : a discussion of what determines what our model uncertainty looks like ( §6.1 – §6.2 ), an analytical analysis of the dropout approximating distribution in Bayesian linear regression ( §6.3 ), an analysis of ELBO-test log likelihood correlation ( §6.4 ), discrete prior models ( §6.5 ), an interpretation of dropout as a proxy posterior in spike and slab prior models ( §6.6 , relating dropout to works by MacKay, Nowlan, and Hinton from 1992), as well as a procedure to optimise the dropout probabilities based on the variational interpretation to separate the different sources of uncertainty ( §6.7 ).
- Contents ( PDF , 36K)
- Chapter 1: The Importance of Knowing What We Don't Know ( PDF , 393K)
- Chapter 2: The Language of Uncertainty ( PDF , 136K)
- Chapter 3: Bayesian Deep Learning ( PDF , 302K)
- Chapter 4: Uncertainty Quality ( PDF , 2.9M)
- Chapter 5: Applications ( PDF , 648K)
- Chapter 6: Deep Insights ( PDF , 939K)
- Chapter 7: Future Research ( PDF , 28K)
- Bibliography ( PDF , 72K)
- Appendix A: KL condition ( PDF , 71K)
- Appendix B: Figures ( PDF , 2M)
- Appendix C: Spike and slab prior KL ( PDF , 28K)
Function visualisation
$ \newcommand{\N}{\mathcal{N}} \newcommand{\x}{\mathbf{x}} \newcommand{\y}{\mathbf{y}} \newcommand{\X}{\mathbf{X}} \newcommand{\Y}{\mathbf{Y}} \newcommand{\train}{{\text{train}}} \newcommand{\bo}{\text{$\omega$}} \newcommand{\yh}{\hat{\y}} \newcommand{\boh}{\text{$\hat{\omega}$}} \newcommand{\liminfT}{\xrightarrow[T \to \infty]{}} $
There are two factors at play when visualising uncertainty in dropout Bayesian neural networks: the dropout masks and the dropout probability of the first layer. Uncertainty depictions in my previous blog posts drew new dropout masks for each test point—which is equivalent to drawing a new prediction from the predictive distribution for each test point $-2 \leq \x \leq 2$. More specifically, for each test point $\x_i$ we drew a set of network parameters from the dropout approximate posterior $\boh_{i} \sim q_\theta(\bo)$, and conditioned on these parameters we drew a prediction from the likelihood $\y_i \sim p(\y | \x_i, \boh_{i})$. Since the predictive distribution has \begin{align*} p&(\y_i | \x_i, \X_\train, \Y_\train) \\ &= \int p(\y_i | \x_i, \bo) p(\bo | \X_\train, \Y_\train) \text{d} \bo \\ &\approx \int p(\y_i | \x_i, \bo) q_\theta(\bo) \text{d} \bo \\ &=: q_\theta(\y_i | \x_i) \end{align*} we have that $\y_i$ is a draw from an approximation to the predictive distribution.

Figure A: In black is a draw from the predictive distribution of a dropout neural network $\yh \sim q_\theta(\y | \x)$ for each test point $-2 \leq \x \leq 2$, compared to the function draws in figure B.

Figure B: Each solid black line is a function drawn from a dropout neural network posterior over functions, induced by a draw from the approximate posterior over the weights $\boh \sim q_\theta(\bo)$.
Another important factor affecting visualisation is the dropout probability of the first layer. In the previous posts we depicted scalar functions and set all dropout probabilities to $0.1$. As a result, with probability $0.1$, the sampled functions from the posterior would be identically zero. This is because a zero draw from the Bernoulli distribution in the first layer together with a scalar input leads the model to completely drop its input (explaining the points where the function touches the $x$-axis in figure A). This is a behaviour we might not believe the posterior should exhibit (especially when a single set of masks is drawn for the entire test set), and could change this by setting a different probability for the first layer. Setting $p_1 = 0$ for example is identical to placing a delta approximating distribution over the first weight layer.
In the demo below we use $p_1 = 0$, and depict draws from the approximate predictive distribution evaluated on the entire test set $q_{\theta_i}(\Y | \X, \boh_i)$ ($\boh_i \sim q_{\theta_i}(\bo)$, function draws ), as the variational parameters $\theta_i$ change and adapt to minimise the divergence to the true posterior (with old samples disappearing after 20 optimisation steps). You can change the function the data is drawn from (with two functions, one from the last blog post and one from the appendix in this paper ), and the model used (a homoscedastic model or a heteroscedastic model, see section §4.6 in the thesis for example or this blog post ).
- Show Uncertainty
- Heteroscedastic
- $y = x \sin x$
- $y = x + \sin 4 (x + \epsilon) + \sin 13 (x + \epsilon) + \epsilon$, $\epsilon \sim \N(0, 0.03^2)$
Last 20 function draws (together with predictive mean and predictive variance) for a dropout neural network , as the approximate posterior is transformed to fit the true posterior. This is done for two functions, both for a homoscedastic model as well as for a heteroscedastic one. You can add new points by left clicking.
Acknowledgements.
To finish this blog post I would like to thank the people that helped through comments and discussions during the writing of the various papers composing the thesis above. I would like to thank (in alphabetical order) Christof Angermueller, Yoshua Bengio, Phil Blunsom, Yutian Chen, Roger Frigola, Shane Gu, Alex Kendall, Yingzhen Li, Rowan McAllister, Carl Rasmussen, Ilya Sutskever, Gabriel Synnaeve, Nilesh Tripuraneni, Richard Turner, Oriol Vinyals, Adrian Weller, Mark van der Wilk, Yan Wu, and many other reviewers for their helpful comments and discussions. I would further like to thank my collaborators Rowan McAllister, Carl Rasmussen, Richard Turner, Mark van der Wilk, and my supervisor Zoubin Ghahramani.
Lastly, I would like to thank Google for supporting three years of my PhD with the Google European Doctoral Fellowship in Machine Learning, and Qualcomm for supporting my fourth year with the Qualcomm Innovation Fellowship.
PS. there might be some easter eggs hidden in the introduction :)

PhD Thesis: Geometry and Uncertainty in Deep Learning for Computer Vision
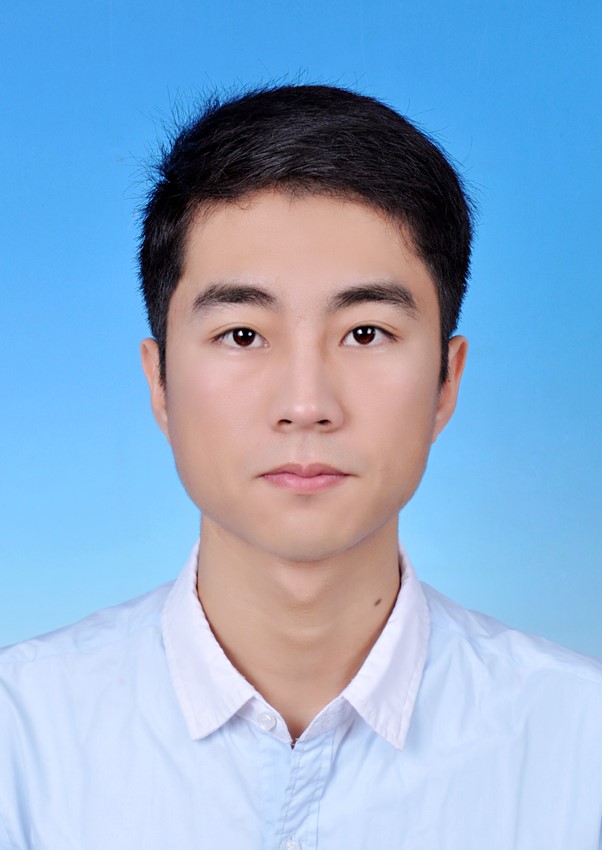
Chaoqiang Zhao
Monocular Depth Estimation, VO
- University of Bologna
- Google Scholar
Today I can share my final PhD thesis, which I submitted in November 2017. It was examined by Dr. Joan Lasenby and Prof. Andrew Zisserman in February 2018 and has just been approved for publication. This thesis presents the main narrative of my research at the University of Cambridge, under the supervision of Prof Roberto Cipolla. It contains 206 pages, 62 figures, 24 tables and 318 citations. You can download the complete .pdf here .
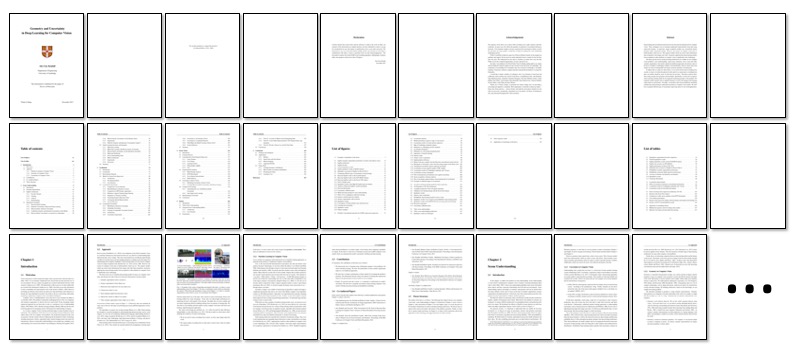
My thesis presents contributions to the field of computer vision, the science which enables machines to see. This blog post introduces the work and tells the story behind this research.
This thesis presents deep learning models for an array of computer vision problems: semantic segmentation , instance segmentation , depth prediction , localisation , stereo vision and video scene understanding .
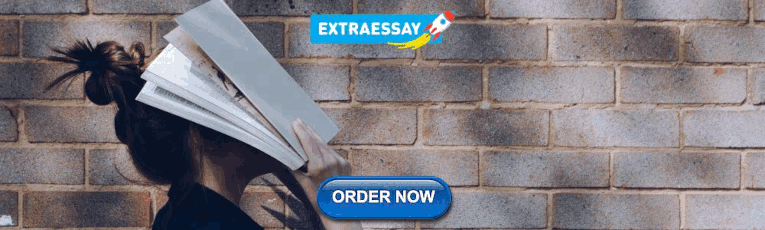
The abstract
Deep learning and convolutional neural networks have become the dominant tool for computer vision. These techniques excel at learning complicated representations from data using supervised learning. In particular, image recognition models now out-perform human baselines under constrained settings. However, the science of computer vision aims to build machines which can see. This requires models which can extract richer information than recognition, from images and video. In general, applying these deep learning models from recognition to other problems in computer vision is significantly more challenging.
This thesis presents end-to-end deep learning architectures for a number of core computer vision problems; scene understanding, camera pose estimation, stereo vision and video semantic segmentation. Our models outperform traditional approaches and advance state-of-the-art on a number of challenging computer vision benchmarks. However, these end-to-end models are often not interpretable and require enormous quantities of training data.
To address this, we make two observations: (i) we do not need to learn everything from scratch, we know a lot about the physical world, and (ii) we cannot know everything from data, our models should be aware of what they do not know. This thesis explores these ideas using concepts from geometry and uncertainty. Specifically, we show how to improve end-to-end deep learning models by leveraging the underlying geometry of the problem. We explicitly model concepts such as epipolar geometry to learn with unsupervised learning, which improves performance. Secondly, we introduce ideas from probabilistic modelling and Bayesian deep learning to understand uncertainty in computer vision models. We show how to quantify different types of uncertainty, improving safety for real world applications.
I began my PhD in October 2014, joining the controls research group at Cambridge University Engineering Department. Looking back at my original research proposal, I said that I wanted to work on the ‘engineering questions to control autonomous vehicles… in uncertain and challenging environments.’ I spent three months or so reading literature, and quickly developed the opinion that the field of robotics was most limited by perception. If you could obtain a reliable state of the world, control was often simple. However, at this time, computer vision was very fragile in the wild. After many weeks of lobbying Prof. Roberto Cipolla (thanks!), I was able to join his research group in January 2015 and begin a PhD in computer vision.
When I began reading computer vision literature, deep learning had just become popular in image classification, following inspiring breakthroughs on the ImageNet dataset. But it was yet to become ubiquitous in the field and be used in richer computer vision tasks such as scene understanding. What excited me about deep learning was that it could learn representations from data that are too complicated to hand-design.
I initially focused on building end-to-end deep learning models for computer vision tasks which I thought were most interesting for robotics, such as scene understanding (SegNet) and localisation (PoseNet) . However, I quickly realised that, while it was a start, applying end-to-end deep learning wasn’t enough. In my thesis, I argue that we can do better than naive end-to-end convolutional networks. Especially with limited data and compute, we can form more powerful computer vision models by leveraging our knowledge of the world. Specifically, I focus on two ideas around geometry and uncertainty.
- Geometry is all about leveraging structure of the world. This is useful for improving architectures and learning with self-supervision.
- Uncertainty understands what our model doesn’t know. This is useful for robust learning, safety-critical systems and active learning.
Over the last three years, I have had the pleasure of working with some incredibly talented researchers, studying a number of core computer vision problems from localisation to segmentation to stereo vision.
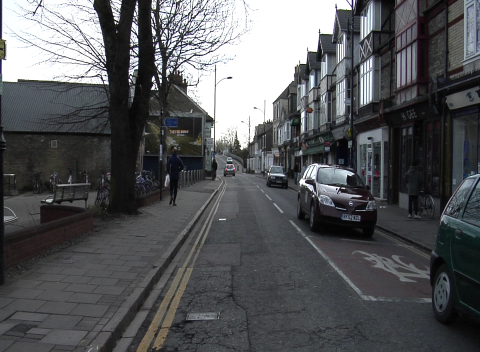
The science
This thesis consists of six chapters. Each of the main chapters introduces an end-to-end deep learning model and discusses how to apply the ideas of geometry and uncertainty.
Chatper 1 - Introduction. Motivates this work within the wider field of computer vision.
Chapter 2 - Scene Understanding. Introduces SegNet, modelling aleatoric and epistemic uncertainty and a method for learning multi-task scene understanding models for geometry and semantics.
Chapter 3 - Localisation. Describes PoseNet for efficient localisation, with improvements using geometric reprojection error and estimating relocalisation uncertainty.
Chapter 4 - Stereo Vision. Designs an end-to-end model for stereo vision, using geometry and shows how to leverage uncertainty and self-supervised learning to improve performance.
Chapter 5 - Video Scene Understanding. Illustrates a video scene understanding model for learning semantics, motion and geometry.
Chapter 6 - Conclusions. Describes limitations of this research and future challenges.
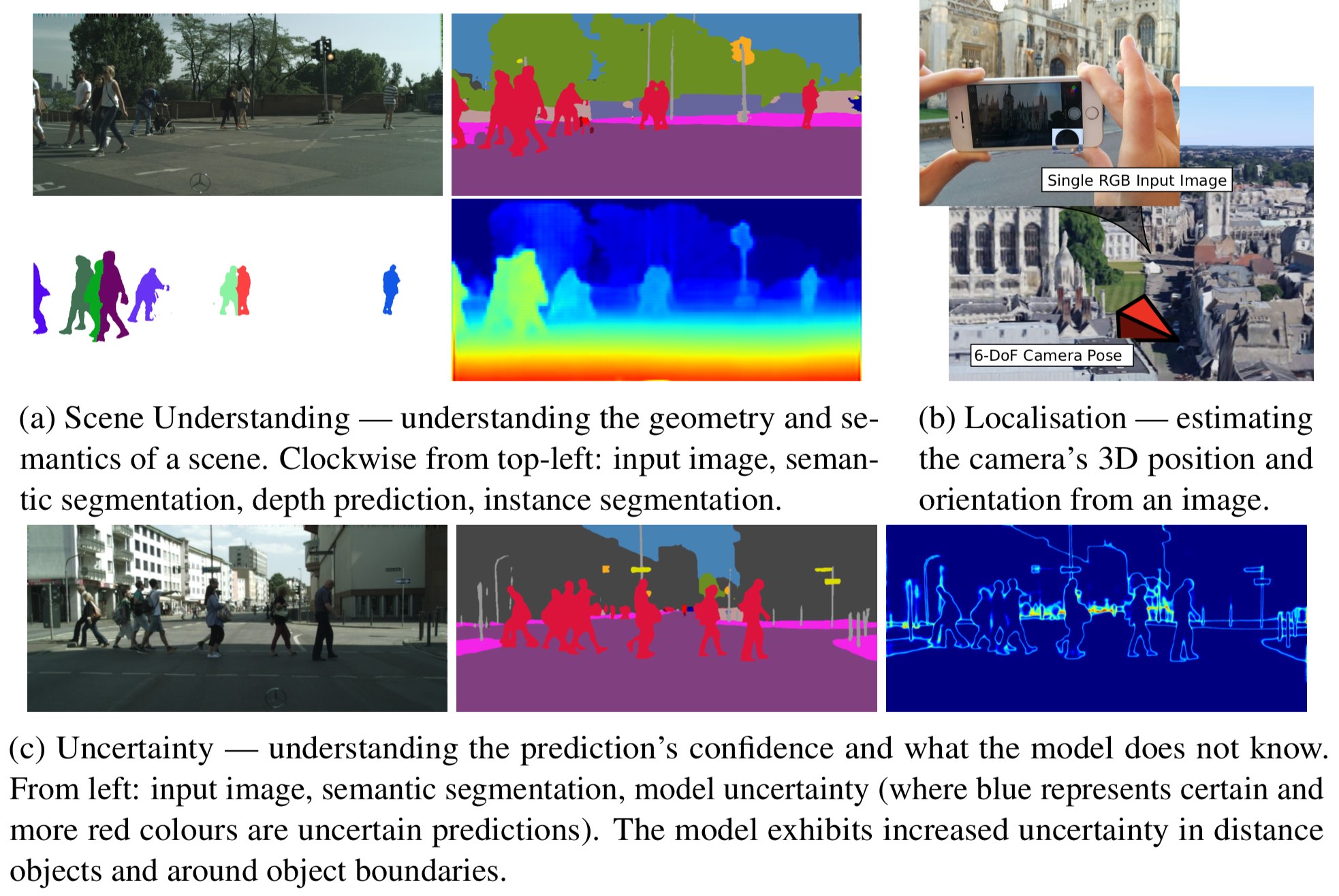
As for what’s next?
This thesis explains how to extract a robust state of the world – semantics, motion and geometry – from video. I’m now excited about applying these ideas to robotics and learning to reason from perception to action. I’m working with an amazing team on autonomous driving, bringing together the worlds of robotics and machine learning. We’re using ideas from computer vision and reinforcement learning to build the most data-efficient self-driving car. And, we’re hiring, come work with me! wayve.ai/careers
I’d like to give a huge thank you to everyone who motivated, distracted and inspired me while writing this thesis.
Here’s the bibtex if you’d like to cite this work.
And the source code for the latex document is here .
- Help & FAQ
PhD thesis: Foundations and advances in deep learning
- Computer Science
Research output : Book/Report › Other report
T1 - PhD thesis
T2 - Foundations and advances in deep learning
AU - Cho, Kyunghyun
M3 - Other report
BT - PhD thesis
PB - Aalto University
Probabilistic Machine Learning in the Age of Deep Learning: New Perspectives for Gaussian Processes, Bayesian Optimization and Beyond (PhD Thesis)
Advances in artificial intelligence (AI) are rapidly transforming our world, with systems now matching or surpassing human capabilities in areas ranging from game-playing to scientific discovery. Much of this progress traces back to machine learning (ML), particularly deep learning and its ability to uncover meaningful patterns and representations in data. However, true intelligence in AI demands more than raw predictive power; it requires a principled approach to making decisions under uncertainty. This highlights the necessity of probabilistic ML, which offers a systematic framework for reasoning about the unknown through probability theory and Bayesian inference. Gaussian processes (GPs) stand out as a quintessential probabilistic model, offering flexibility, data efficiency, and well-calibrated uncertainty estimates. They are integral to many sequential decision-making algorithms, notably Bayesian optimisation (BO), which has emerged as an indispensable tool for optimising expensive and complex black-box objective functions. While considerable efforts have focused on improving gp scalability, performance gaps persist in practice when compared against neural networks (NNs) due in large to its lack of representation learning capabilities. This, among other natural deficiencies of GPs, has hampered the capacity of BO to address critical real-world optimisation challenges. This thesis aims to unlock the potential of deep learning within probabilistic methods and reciprocally lend probabilistic perspectives to deep learning. The contributions include improving approximations to bridge the gap between GPs and NNs, providing a new formulation of BO that seamlessly accommodates deep learning methods to tackle complex optimisation problems, as well as a probabilistic interpretation of a powerful class of deep generative models for image style transfer. By enriching the interplay between deep learning and probabilistic ML, this thesis advances the foundations of AI and facilitates the development of more capable and dependable automated decision-making systems.
The full text is available as a single PDF file
You can also find a list of contents and PDFs corresponding to each individual chapter below:
Table of Contents
- Chapter 1: Introduction
- Chapter 2: Background
- Chapter 3: Orthogonally-Decoupled Sparse Gaussian Processes with Spherical Neural Network Activation Features
- Chapter 4: Cycle-Consistent Generative Adversarial Networks as a Bayesian Approximation
- Chapter 5: Bayesian Optimisation by Classification with Deep Learning and Beyond
- Chapter 6: Conclusion
- Appendix A: Numerical Methods for Improved Decoupled Sampling of Gaussian Processes
- Bibliography
Please find Chapter 1: Introduction reproduced in full below:
Introduction
PhD Candidate (AI & Machine Learning)
Thanks for stopping by! Let’s connect – drop me a message or follow me
DAVID STUTZ
Phd thesis: uncertainty and robustness.
- Download and Citing
Defense Talk
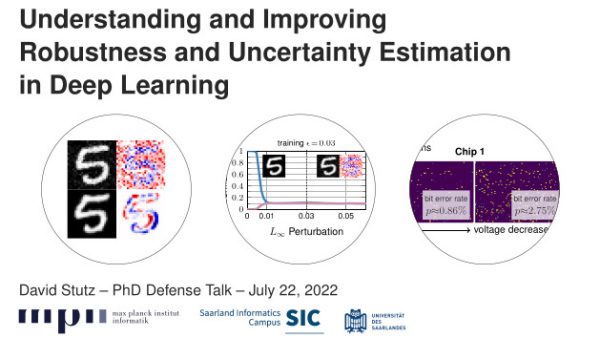
Quick links: Thesis | Defense | Thesis Template | Defense Template

Figure 1: Problems tackled in my PhD thesis, ranging from 3D reconstruction in the context of autonomous driving to adversarial robustness, bit error robustness and uncertainty estimation.
Deep learning is becoming increasingly relevant for many high-stakes applications such as autonomous driving or medical diagnosis where wrong decisions can have massive impact on human lives. Unfortunately, deep neural networks are typically assessed solely based on generalization, e.g., accuracy on a fixed test set. However, this is clearly insufficient for safe deployment as potential malicious actors and distribution shifts or the effects of quantization and unreliable hardware are disregarded. Thus, recent work additionally evaluates performance on potentially manipulated or corrupted inputs as well as after quantization and deployment on specialized hardware. In such settings, it is also important to obtain reasonable estimates of the model's confidence alongside its predictions. This thesis studies robustness and uncertainty estimation in deep learning along three main directions: First, we consider so-called adversarial examples, slightly perturbed inputs causing severe drops in accuracy. Second, we study weight perturbations, focusing particularly on bit errors in quantized weights. This is relevant for deploying models on special-purpose hardware for efficient inference, so-called accelerators. Finally, we address uncertainty estimation to improve robustness and provide meaningful statistical performance guarantees for safe deployment.
In detail, we study the existence of adversarial examples with respect to the underlying data manifold. In this context, we also investigate adversarial training which improves robustness by augmenting training with adversarial examples at the cost of reduced accuracy. We show that regular adversarial examples leave the data manifold in an almost orthogonal direction. While we find no inherent trade-off between robustness and accuracy, this contributes to a higher sample complexity as well as severe overfitting of adversarial training. Using a novel measure of flatness in the robust loss landscape with respect to weight changes, we also show that robust overfitting is caused by converging to particularly sharp minima. In fact, we find a clear correlation between flatness and good robust generalization.
Further, we study random and adversarial bit errors in quantized weights. In accelerators, random bit errors occur in the memory when reducing voltage with the goal of improving energy-efficiency. Here, we consider a robust quantization scheme, use weight clipping as regularization and perform random bit error training to improve bit error robustness, allowing considerable energy savings without requiring hardware changes. In contrast, adversarial bit errors are maliciously introduced through hardware- or software-based attacks on the memory, with severe consequences on performance. We propose a novel adversarial bit error attack to study this threat and use adversarial bit error training to improve robustness and thereby also the accelerator's security.
Finally, we view robustness in the context of uncertainty estimation. By encouraging low-confidence predictions on adversarial examples, our confidence-calibrated adversarial training successfully rejects adversarial, corrupted as well as out-of-distribution examples at test time. Thereby, we are also able to improve the robustness-accuracy trade-off compared to regular adversarial training. However, even robust models do not provide any guarantee for safe deployment. To address this problem, conformal prediction allows the model to predict confidence sets with user-specified guarantee of including the true label. Unfortunately, as conformal prediction is usually applied after training, the model is trained without taking this calibration step into account. To address this limitation, we propose conformal training which allows training conformal predictors end-to-end with the underlying model. This not only improves the obtained uncertainty estimates but also enables optimizing application-specific objectives without losing the provided guarantee.
Besides our work on robustness or uncertainty, we also address the problem of 3D shape completion of partially observed point clouds. Specifically, we consider an autonomous driving or robotics setting where vehicles are commonly equipped with LiDAR or depth sensors and obtaining a complete 3D representation of the environment is crucial. However, ground truth shapes that are essential for applying deep learning techniques are extremely difficult to obtain. Thus, we propose a weakly-supervised approach that can be trained on the incomplete point clouds while offering efficient inference.
In summary, this thesis contributes to our understanding of robustness against both input and weight perturbations. To this end, we also develop methods to improve robustness alongside uncertainty estimation for safe deployment of deep learning methods in high-stakes applications. In the particular context of autonomous driving, we also address 3D shape completion of sparse point clouds.
Download & Citing
The thesis is available on the webpage of Saarland University's library:
Table for contents:
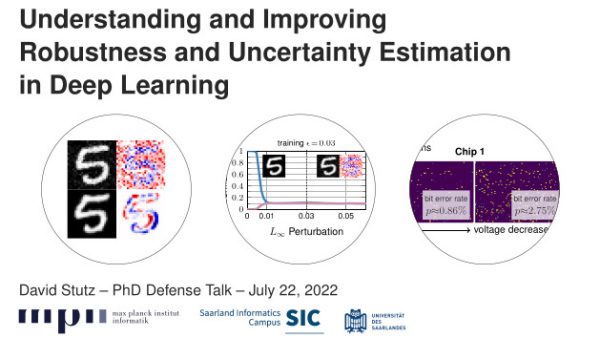
If you are interested in the LaTeX templates used for my thesis and defense, you can find them on GitHub:
Thesis Template Defense Template
SEARCH THEBLOG
- 2024 —
- 2023 —
- 2022 —
- 2021 —
- 2020 —
- 2019 —
- 2018 —
- 2017 —
- 2016 —
- 2015 —
- 2014 —
- 2013 —
- ADVERSARIAL MACHINE LEARNING
- ARTIFICIAL INTELLIGENCE
- COMPRESSED SENSING
- COMPUTER GRAPHICS
- COMPUTER SCIENCE
- COMPUTER VISION
- DATA MINING
- DEEP LEARNING
- DNN ACCELERATORS
- GAME THEORY
- IMAGE PROCESSING
- MACHINE LEARNING
- MATHEMATICS
- MEDIA COVERAGE
- MEDICAL IMAGE PROCESSING
- NATURAL LANGUAGE PROCESSING
- NUMERICAL ANALYSIS
- OPTIMIZATION
- PUBLICATION
- RASPBERRY PI
- SECURITY AND PRIVACY
- SOCIAL NETWORKS
- SOFTWARE ENGINEERING
- TWITTER BOOTSTRAP
- UNCERTAINTY ESTIMATION
- WEB SECURITY
- sci.aalto.fi
- cs.aalto.fi
Deep Learning

- Publications

Theses done in the research group
Doctoral theses.
J. Luttinen (2015). Bayesian Latent Gaussian Spatio-Temporal Models . PhD thesis, Aalto University, Espoo, Finland.
K. Cho (2014). Foundations and Advances in Deep Learning . PhD thesis, Aalto University, Espoo, Finland.
M. Harva (2008). Algorithms for Approximate Bayesian Inference with Applications to Astronomical Data Analysis . PhD thesis, Helsinki University of Technology, Espoo, Finland.
T. Raiko (2006). Bayesian Inference in Nonlinear and Relational Latent Variable Models . PhD thesis, Helsinki University of Technology, Espoo, Finland.
A. Ilin (2006). Advanced Source Separation Methods with Applications to Spatio-Temporal Datasets . PhD thesis, Helsinki University of Technology, Espoo, Finland.
A. Honkela (2005). Advances in Variational Bayesian Nonlinear Blind Source Separation . PhD thesis, Helsinki University of Technology, Espoo, Finland.
Master's Theses
R. Boney (2018). Fast Adaptation of Neural Networks . Master's thesis, Aalto University, Helsinki, Finland.
G. van den Broeke (2016). What auto-encoders could learn from brains - Generation as feedback in unsupervised deep learning and inference . Master's thesis, Aalto University, Helsinki, Finland.
M. Perello Nieto (2015). Merging chrominance and luminance in an early, medium and late fusion using Convolutional Neural Networks . Master's thesis, Aalto University, Helsinki, Finland.
P. Noeva (2012). Sampling Methods for Missing Value Reconstruction. Master's thesis, Aalto University, Helsinki, Finland.
T. Hao (2012). Gated Boltzmann Machine in Texture Modelling . Master's thesis, Aalto University, Helsinki, Finland.
K. Cho (2011). Improved Learning Algorithms for Restricted Boltzmann Machines . Master's thesis, Aalto University, Helsinki, Finland.
N. Korsakova (2010). Feature extraction and dynamical modelling of spatio-temporal data. Master's thesis, Aalto University, Finland.
M. Tornio (2010). Natural Gradient for Variational Bayesian Learning . Master's thesis, Aalto University, Helsinki, Finland.
J. Luttinen (2009). Gaussian-process factor analysis for modeling spatio-temporal data . Master's thesis, Helsinki University of Technology, Espoo, Finland.
M. Harva (2004). Hierarchical Variance Models of Image Sequences . Master's thesis, Helsinki University of Technology, Espoo, Finland.
T. Raiko (2001). Hierarchical Nonlinear Factor Analysis . Master's thesis, Helsinki University of Technology, Espoo, Finland.
A. Honkela (2001). Nonlinear Switching State-Space Models . Master's thesis, Helsinki University of Technology, Espoo, Finland.
Latest update 2018-10-10 15:31 EEST Maintained by Alexander Ilin
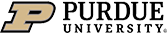
File(s) under embargo
Reason: Parts of the thesis are not published yet.
until file(s) become available
DISCOVERY OF NOVEL DISEASE BIOMARKERS AND THERAPEUTICS USING MACHINE LEARNING MODELS
In the fields of computational biology and bioinformatics, the identification of novel disease biomarkers and therapeutic strategies, especially for cancer diseases, remains a crucial challenge. The advancement in computer science, particularly machine learning techniques, has greatly empowered the study of computational biology and bioinformatics for their unprecedented prediction power. This thesis explores how to utilize classic and advanced machine learning models to predict prognostic pathways, biomarkers, and therapeutics associated with cancers.
Firstly, this thesis presents a comprehensive overview of computational biology and bioinformatics, covering past milestones to current groundbreaking advancements, providing context for the research. A centerpiece of this thesis is the introduction of the Pathway Ensemble Tool and Benchmark, an original methodology designated for the unbiased discovery of cancer-related pathways and biomarkers. This toolset not only enhances the identification of crucial prognostic components distinguishing clinical outcomes in cancer patients but also guides the development of targeted drug treatments based on these signatures. Inspired by Benchmark, we extended the methodology to single-cell technologies and proposed scBenchmark and PathPCA, which provides insights into the potential and limitations of how novel techniques can benefit biomarker and therapeutic discovery. Next, the research progresses to the development of DREx, a deep learning model trained on large-scale transcriptome data, for predicting gene expression responses to drug treatments across multiple cell lines. DREx highlights the potential of advanced machine learning models in drug repurposing using a genomics-centric approach, which could significantly enhance the efficiency of initial drug selection.
The thesis concludes by summarizing these findings and highlighting their importance in advancing cancer-related biomarkers and drug discovery. Various computational predictions in this work have already been experimentally validated, showcasing the real-life impact of these methodologies. By integrating machine learning models with computational biology and bioinformatics, this research pioneers new standards for novel biomarker and therapeutics discovery.
Degree Type
- Doctor of Philosophy
- Computer Science
Campus location
- West Lafayette
Advisor/Supervisor/Committee Chair
Additional committee member 2, additional committee member 3, additional committee member 4, usage metrics.
- Other information and computing sciences not elsewhere classified

Help | Advanced Search
Astrophysics > High Energy Astrophysical Phenomena
Title: population synthesis of galactic pulsars with machine learning.
Abstract: This thesis work represents the first efforts to combine population synthesis studies of the Galactic isolated neutron stars with deep-learning techniques with the aim of better understanding neutron-star birth properties and evolution. In particular, we develop a flexible population-synthesis framework to model the dynamical and magneto-rotational evolution of neutron stars, their emission in radio and their detection with radio telescopes. We first study the feasibility of using deep neural networks to infer the dynamical properties at birth and then explore a simulation-based inference approach to predict the birth magnetic-field and spin-period distributions and the late-time magnetic-field decay for the observed radio pulsar population. Our results for the birth magneto-rotational properties agree with the findings of previous works while we constrain the late-time evolution of the magnetic field in neutron stars for the first time. Moreover, this thesis also studies possible scenarios to explain the puzzling nature of recently discovered periodic radio sources with very long periods of the order of thousands of seconds. In particular, by assuming a neutron-star origin, we study the spin-period evolution of a newborn neutron star interacting with a supernova fallback disk and find that the combination of strong, magnetar-like magnetic fields and moderate accretion rates can lead to very large spin periods on timescales of ten thousands of years. Moreover, we perform population synthesis studies to assess the possibility for these sources to be either neutron stars or magnetic white dwarfs emitting coherently through magnetic dipolar losses. These discoveries have opened up a new perspective on the neutron-star population and have started to question our current understanding of how coherent radio emission is produced in pulsar magnetospheres.
Submission history
Access paper:.
- HTML (experimental)
- Other Formats

References & Citations
- INSPIRE HEP
- Google Scholar
- Semantic Scholar
BibTeX formatted citation

Bibliographic and Citation Tools
Code, data and media associated with this article, recommenders and search tools.
- Institution
arXivLabs: experimental projects with community collaborators
arXivLabs is a framework that allows collaborators to develop and share new arXiv features directly on our website.
Both individuals and organizations that work with arXivLabs have embraced and accepted our values of openness, community, excellence, and user data privacy. arXiv is committed to these values and only works with partners that adhere to them.
Have an idea for a project that will add value for arXiv's community? Learn more about arXivLabs .
Department of Computer Science
- Department of Computer Science DIKU
- Event Calendar 2024
PhD defence by Lei Li
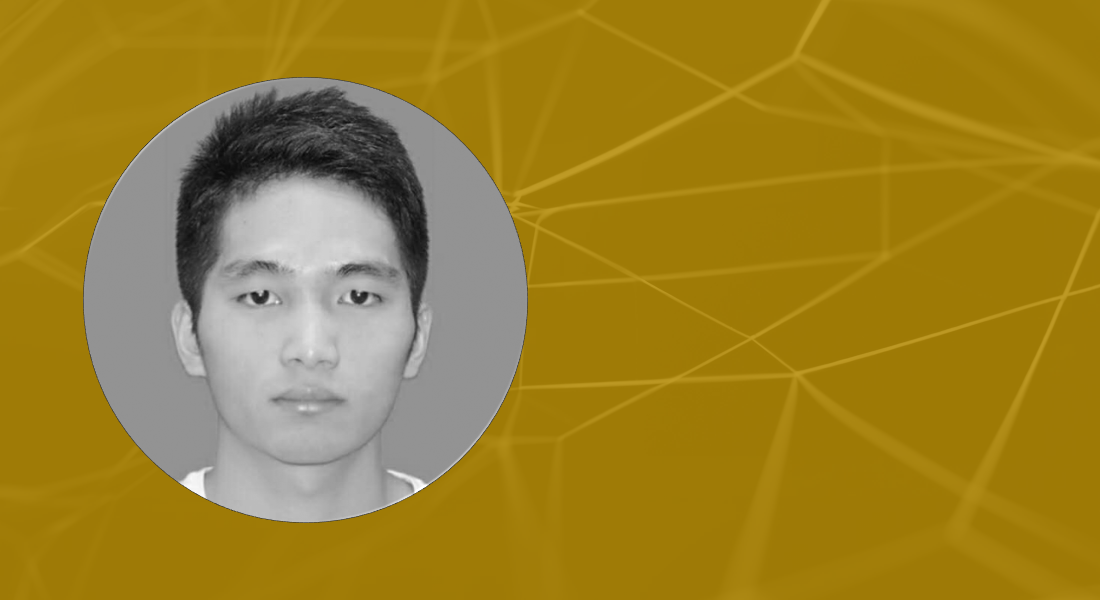
Follow this link to participate on Zoom
Contributions to deep learning for computer vision applied to environmental remote sensing and human face and pose analysis
This thesis presents research in deep learning for computer vision with applications to remote sensing data and human face and pose analysis. The focus is on 3D data, and various input modalities are considered: images, 3D point clouds, and natural language.
The first part of the thesis considers remote sensing of the environment. The first contribution in this domain is the use of Chain-of-Thought language prompting to enhance semantic image segmentation accuracy, particularly in challenging scenarios like flood disasters. This approach fuses visual and linguistic elements.
The second study considers aligning and fusing diverse modalities for the segmentation of buildings in satellite imagery.
The third study considers the prediction of aboveground forest biomass based on 3D point clouds from airborne LiDAR, for example for measuring carbon sequestration. We suggest replacing the current analysis of handcrafted statistical features derived from the point clouds by applying point cloud neural networks for regression directly to the 3D data. Then edgeaware learning for 3D point clouds is proposed, which addresses the challenges of noise in point cloud data by focusing on edge features to improve classification and segmentation.
The second part of the thesis focuses on the analysis of data from humans. The first work presented involves systems capable of real-time face segmentation, which includes accurate face detection, alignment, and parsing. These systems leverage 3D facial features and can handle occlusions and diverse facial expressions. The final study addresses human pose estimation, which finds extensive application in fields such as augmented reality/virtual reality (AR/VR), live broadcasting, and interactive media. It enhances the user experience by providing more realistic and responsive interactions. The proposed method leverages zero-shot learning algorithms to accurately capture and analyze human movements and poses with diffusion generation methods in uncontrolled environments.
We propose various methods for a range of applied computer vision tasks, utilizing different data modalities. Our research encompasses several scenarios, unified by a core challenge: effectively employing deep learning networks for the analysis of varied modalities.
Supervisors
Principal Supervisor Christian Igel
Assessment Committee
Professor Kim Steenstrup Pedersen, Computer Science Professor Yifang Ban, KTH, Sweden Professor Daniel Sonntag, Oldenburg University, Germany
For an electronic copy of the thesis, please visit the PhD Programme page .
Time: 3 May 2024, 13:00-16:00
Place: Zoom
Organizer: Department of Computer Science
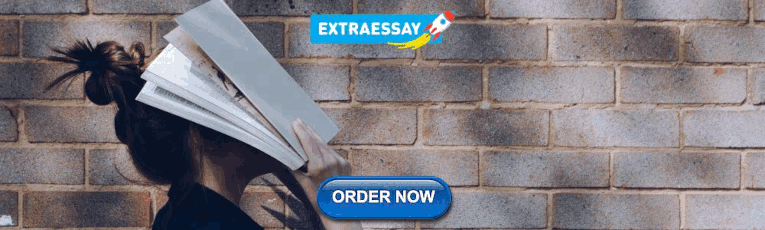
IMAGES
VIDEO
COMMENTS
PhD Dissertations [All are .pdf files] Probabilistic Reinforcement Learning: Using Data to Define Desired Outcomes, and Inferring How to Get There Benjamin Eysenbach, 2023. Data-driven Decisions - An Anomaly Detection Perspective Shubhranshu Shekhar, 2023. METHODS AND APPLICATIONS OF EXPLAINABLE MACHINE LEARNING Joon Sik Kim, 2023. Applied Mathematics of the Future Kin G. Olivares, 2023
If you can find a dissertation that aligns with your areas of interest, consuming the research is an excellent way to do a deep dive into the technology. After reviewing hundreds of recent theses from universities all over the country, I present 10 machine learning dissertations that I found compelling in terms of my own areas of interest.
Lastly, I would like to thank Google for supporting three years of my PhD with the Google European Doctoral Fellowship in Machine Learning, and Qualcomm for ... The most basic model in deep learning can be described as a hierarchy of these parametrised basis functions ...
The new model family introduced in this thesis is summarized under the term Recursive Deep Learning. The models in this family are variations and extensions of unsupervised and supervised recursive neural networks (RNNs) which generalize deep and feature learning ideas to hierarchical structures. The RNN models of this thesis
This thesis studies the non-convex optimization of various architectures of deep neural networks by focusing on some fundamental bottlenecks in the scalability, such as suboptimal local minima and saddle points. In particular, for deep neural networks, we present various guarantees for the values of local minima and critical points, as well as ...
In this thesis, we take a ``natural sciences'' approach towards building a theory for deep learning. We begin by identifying various empirical properties that emerge in practical deep networks across a variety of different settings. Then, we discuss how these empirical findings can be used to inform theory. Specifically, we show the following ...
This thesis considers deep learning theories of brain function, and in particular biologically plausible deep learning. The idea is to treat a standard deep network as a high-level model of a neural circuit (e.g., the visual stream), adding biological constraints to some clearly artificial features. Two big questions are possible. First,
This dissertation investigates how to enable high performance, efficient, and sustainable deep learning systems at scale. The thesis first identifies deep learning-based personalized recommendation engines as the dominating consumer of AI training and inference cycles in production data centers; the high infrastructure demands not only impede ...
During the PhD course, I explore and establish theoretical foundations for deep learning. In this thesis, I present my contributions positioned upon existing literature: (1) analysing the generalizability of the neural networks with residual connections via complexity and capacity-based hypothesis complexity measures; (2) modeling stochastic ...
The thesis can be obtained as a Single PDF (9.1M), or as individual chapters (since the single file is fairly large): Contents ( PDF, 36K) Chapter 1: The Importance of Knowing What We Don't Know ( PDF, 393K) Chapter 2: The Language of Uncertainty ( PDF, 136K) Chapter 3: Bayesian Deep Learning ( PDF, 302K) Chapter 4: Uncertainty Quality ( PDF, 2.9M)
Thesis Abstract Thesis Abstract "Deep Learning"/"Deep Neural Nets" is a technological marvel that is now increasingly deployed at the cutting-edge of artificial intelligence tasks. This ongoing revolution can be said to have been ignited by the iconic 2012 paper from the University of Toronto titled "ImageNet Classification with
Go 1.0 was released in March 2012 [22]. The focus of this thesis is to integrate GPU computation with the Go language for the purpose of developing deep learning models. This chapter includes a review of some of the packages that were developed for GPU computation with Go, the applications that use them, and other deep learning frameworks. 2.1 ...
This thesis consists of six chapters. Each of the main chapters introduces an end-to-end deep learning model and discusses how to apply the ideas of geometry and uncertainty. Chatper 1 - Introduction. Motivates this work within the wider field of computer vision. Chapter 2 - Scene Understanding.
Abstract of thesis entitled: Fast and Efficient Deep Learning Deployments via Learning- based Methods Submitted by SUN, Qi for the degree of Doctor of Philosophy at The Chinese University of Hong Kong in July 2022 The past few years witnessed the significant success of deep learning (DL) algorithms and the increasing deployment effi- ciency and ...
diversity in contextual learning and the initial stage of explainability, respectively. This thesis aims to explore and develop novel deep learning techniques escorted by uncertainty quantification for developing actionable automated grading and di-agnosis systems. More specifically, the thesis provides the following three main contributions.
T1 - PhD thesis. T2 - Foundations and advances in deep learning. AU - Cho, Kyunghyun. PY - 2014. Y1 - 2014. M3 - Other report. BT - PhD thesis. PB - Aalto University. ER - Powered by Pure, Scopus & Elsevier Fingerprint Engine ...
Advancements in machine learning techniques have encouraged scholars to focus on convolutional neural network (CNN) based solutions for object detection and pose estimation tasks. Most … Year: 2020 Contributor: Derman, Can Eren (creator) Bahar, Iris (thesis advisor) Taubin, Gabriel (reader) Brown University. School of Engineering (sponsor ...
This thesis explores the intersection of deep learning and probabilistic machine learning to enhance the capabilities of artificial intelligence. It addresses the limitations of Gaussian processes (GPs) in practical applications, particularly in comparison to neural networks (NNs), and proposes advancements such as improved approximations and a novel formulation of Bayesian optimization (BO ...
This work develops tools to obtain practical uncertainty estimates in deep learning, casting recent deep learning tools as Bayesian models without changing either the models or the optimisation, and develops the theory for such tools. Deep learning has attracted tremendous attention from researchers in various fields of information engineering such as AI, computer vision, and language ...
In March this year I finally submitted my PhD thesis and successfully defended in July. Now, more than 6 months later, my thesis is finally available in the university's library. During my PhD, I worked on various topics surrounding robustness and uncertainty in deep learning, including adversarial robustness, robustness to bit errors, out-of-distribution detection and conformal prediction. In ...
This thesis studies robustness and uncertainty estimation in deep learning along three main directions: First, we consider so-called adversarial examples, slightly perturbed inputs causing severe drops in accuracy. Second, we study weight perturbations, focusing particularly on bit errors in quantized weights.
Unsupervised Networks, Stochasticity and Optimization in Deep Learning. PhD thesis, Aalto University, Department of Computer Science, Espoo, Finland, April 2017. J. Luttinen (2015). Bayesian Latent Gaussian Spatio-Temporal Models. PhD thesis, Aalto University, Espoo, Finland. K. Cho (2014). Foundations and Advances in Deep Learning.
than recognition, from images and video. In general, applying these deep learning models from recognition to other problems in computer vision is significantly more challenging. This thesis presents end-to-end deep learning architectures for a number of core computer vision problems; scene understanding, camera pose estimation, stereo vision ...
A centerpiece of this thesis is the introduction of the Pathway Ensemble Tool and Benchmark, an original methodology designated for the unbiased discovery of cancer-related pathways and biomarkers. ... Next, the research progresses to the development of DREx, a deep learning model trained on large-scale transcriptome data, for predicting gene ...
This thesis work represents the first efforts to combine population synthesis studies of the Galactic isolated neutron stars with deep-learning techniques with the aim of better understanding neutron-star birth properties and evolution. In particular, we develop a flexible population-synthesis framework to model the dynamical and magneto-rotational evolution of neutron stars, their emission in ...
This thesis presents research in deep learning for computer vision with applications to remote sensing data and human face and pose analysis. The focus is on 3D data, and various input modalities are considered: images, 3D point clouds, and natural language. The first part of the thesis considers remote sensing of the environment.