Change Password
Your password must have 6 characters or more:.
- a lower case character,
- an upper case character,
- a special character
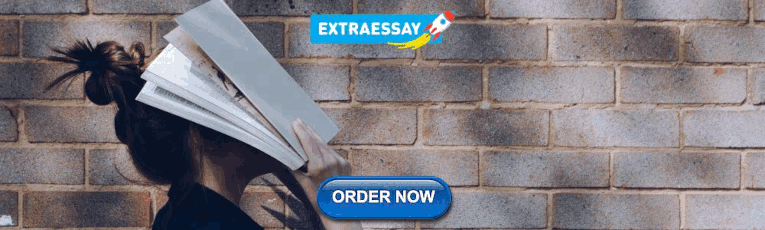
Password Changed Successfully
Your password has been changed
Create your account
Forget yout password.
Enter your email address below and we will send you the reset instructions
If the address matches an existing account you will receive an email with instructions to reset your password
Forgot your Username?
Enter your email address below and we will send you your username
If the address matches an existing account you will receive an email with instructions to retrieve your username

- April 01, 2024 | VOL. 181, NO. 4 CURRENT ISSUE pp.255-346
- March 01, 2024 | VOL. 181, NO. 3 pp.171-254
- February 01, 2024 | VOL. 181, NO. 2 pp.83-170
- January 01, 2024 | VOL. 181, NO. 1 pp.1-82
The American Psychiatric Association (APA) has updated its Privacy Policy and Terms of Use , including with new information specifically addressed to individuals in the European Economic Area. As described in the Privacy Policy and Terms of Use, this website utilizes cookies, including for the purpose of offering an optimal online experience and services tailored to your preferences.
Please read the entire Privacy Policy and Terms of Use. By closing this message, browsing this website, continuing the navigation, or otherwise continuing to use the APA's websites, you confirm that you understand and accept the terms of the Privacy Policy and Terms of Use, including the utilization of cookies.
Anxiety, Depression, and Suicide in Youth
- Ned H. Kalin , M.D.
Search for more papers by this author
Anxiety disorders and depression are among the most common psychiatric illnesses affecting youth. Anxiety disorders typically begin in childhood, whereas the onset of depression frequently occurs later during adolescence or early adulthood. These illnesses are highly comorbid, with pathological anxiety regularly preceding the development of depression. The lifetime prevalence of anxiety disorders when assessed in adolescents is reported to be as high as 32% ( 1 ), whereas the estimated 12-month prevalence of major depression in adolescents is approximately 13% ( 2 ). Prior to adolescence, the incidence of these disorders is the same between boys and girls; however, as girls mature and go through puberty, they are approximately twice as likely as boys to be diagnosed with anxiety and major depression. In addition to causing considerable suffering and impaired functioning, when severe, these illnesses can be life threatening. Tragically, 6,200 suicide deaths were reported in 2017 among U.S. adolescents and young adults from 15 to 24 years of age ( 3 ), and suicide is the second leading cause of death among individuals 10–34 years of age ( 4 ).
As with other psychiatric illnesses, the risks for developing anxiety disorders and major depression are due to interactions between heritable and nonheritable factors. It is estimated that the heritability of anxiety and major depression is between 30% and 40%, leaving a considerable amount of the risk to potentially modifiable environmental factors. Genome-wide association studies with increasingly large sample sizes continue to identify genes that help explain a portion of the heritability for anxiety and depression ( 5 ). However, it is important to note that a recent study has provided evidence questioning the validity of previous findings that have linked a number of the familiar, “usual suspect” candidate genes (e.g., polymorphisms of the gene for the serotonin transporter protein) to be strongly associated with anxiety and depression ( 6 ). Early life trauma, neglect, inadequate parenting, and ongoing stress are among the environmental factors that contribute to the likelihood of developing anxiety, depression, and other stress-related disorders. Adolescence is a particularly vulnerable period, as the psychosocial challenges of adolescence converge with rapid and substantial developmental changes in the brain and in hormones. Prior to the onset of anxiety disorders and major depression, at-risk phenotypes or personality traits such as behavioral inhibition ( 7 ) and neuroticism ( 8 ), which are also partially heritable, can be identified and provide an opportunity for developing early intervention strategies for children at risk.
Two now classic clinical trials have evaluated the efficacy of selective serotonin reuptake inhibitors and cognitive therapies for the treatment of major depression and anxiety disorders in youth. The Treatment for Adolescents With Depression Study was a randomized 12-week trial involving 439 adolescents with major depression in which fluoxetine, cognitive-behavioral therapy (CBT), CBT plus fluoxetine, and placebo were compared ( 9 ). Results demonstrated that fluoxetine plus CBT and fluoxetine alone were significantly better than placebo, with the combination outperforming fluoxetine alone. CBT by itself did not statistically differ from placebo (fluoxetine plus CBT, 71% response; fluoxetine alone, 60.6% response; CBT alone, 43.2% response; placebo, 34.8% response). With continued treatment, rates of response remained high for up to 36 months ( 10 ). The Child/Adolescent Anxiety Multimodal Study was a randomized clinical trial comparing 14 CBT sessions with 12 weeks of sertraline plus CBT, sertraline alone, or placebo in 488 children and adolescents (7–17 years old) with separation anxiety disorder, generalized anxiety disorder, or social phobia ( 11 ). Results demonstrated that all therapies were more effective than placebo and that the combination of sertraline plus CBT was superior to the other active treatments (sertraline plus CBT, 80.7% response; CBT, 59.7% response; sertraline alone, 54.9% response; placebo, 23.7% response). Long-term follow-up of 319 of these children revealed that only 22% were in stable remission, whereas the remainder were either chronically ill or had relapsed ( 12 ). Taken together, these studies highlight the efficacy of relatively short-term interventions and point to the need for treatments that can fundamentally affect childhood developmental trajectories that will enable initial interventions to have long-lasting positive effects.
In this regard, a more complete understanding of the pathophysiology underlying anxiety disorders and major depression in youth is necessary to advance the development of new early intervention strategies. Neuroimaging studies suggest that anxiety and depression share alterations in the function of prefrontal-limbic circuits that underlie the adaptive regulation of emotion and the processing of anxiety ( 13 ), and studies also show alterations in reward-related processing to be associated with both anxiety and depression ( 14 , 15 ). However, to move beyond the associations between brain and behavior that have been identified with neuroimaging, preclinical studies are critical to elucidate potential mechanisms that underlie anxiety- and depression-related pathophysiology. For various reasons, developing valid preclinical animal models of depression has been challenging. In contrast, anxiety and fear can be effectively modeled in rodents and nonhuman primates ( 13 , 16 ), and such research has led to a deep understanding of the circuits, cells, and molecules that are mechanistically involved in mediating adaptive and pathological anxiety. The evolutionary expansion of the primate prefrontal cortex makes nonhuman primates particularly valuable for modeling human anxiety, as the expanded primate prefrontal cortex is prominently involved in mediating internal emotional experiences and cognitive processes that are unique to primate species and that, when aberrant, contribute to psychopathology.
The neural circuitry underlying fear and anxiety includes subcortical structures such as specific amygdala nuclei, the bed nucleus of the stria terminalis, the anterior hippocampus, and brainstem regions such as the periaqueductal gray ( 17 ). These subcortical regions, via their synaptic connectivity, work in concert with the ventromedial prefrontal cortex, the anterior insular cortex, the anterior cingulate cortex, and other regions of the posterior orbitofrontal cortex to regulate and process anxiety. In relation to major depression, the presence of anhedonia is a clinical feature that clearly distinguishes depression from anxiety. This diminished capacity to enjoy and engage with one’s world is in part mediated by altered function of the brain’s reward circuitry. For exam-ple, neuroimaging studies in adolescents with depression demonstrate altered reward-related responsivity of various components of this system, including the nucleus accumbens and striatum, as well as cortical regions such as the insular and the anterior cingulate cortices ( 14 ).
This issue of the Journal focuses on depression and anxiety during childhood and adolescence and importantly includes two articles that address mental health issues in disadvantaged youth living in poverty. We include four research articles that address critical treatment areas, including the use of CBT for treating childhood grief, ketamine for treatment-resistant adolescent depression, the use of neuroimaging in anxious youth to predict treatment response, and a preschool intervention for preventing psychopathology in disadvantaged children. Another article that is relevant to the health of disadvantaged and underresourced populations presents research that combines measures of inflammation with neuroimaging to better understand factors that may underlie physical health problems in children living in poverty. Other articles in this issue are focused on understanding underlying pathophysiology (capitalizing on neuroimaging data from the large Adolescent Brain Cognitive Development [ABCD] database), examining neuroimaging measures associated with suicidal thoughts, and examining reward-related neural processing in relation to disruptive behavior disorders.
Treating Prolonged Grief in Children and Adolescents
The loss of a loved one during childhood is traumatic and increases the risk to develop stress-related psychiatric illnesses such as depression and posttraumatic stress disorder (PTSD). Boelen and coauthors ( 18 ) present data from a randomized clinical trial comparing CBT aimed at coping with grief with an intervention employing supportive counseling in 134 children and adolescents who met criteria for prolonged grief disorder. Prolonged grief disorder, which was recently added to ICD-11, is defined by the presence of significant and interfering grief symptoms that last beyond the first 6 months after a loss. Although it is not in DSM-5, prolonged grief disorder is similar to the DSM-5 diagnosis of persistent complex bereavement disorder. In this study, each participant received nine sessions of the respective therapies, and their parents or caregivers received five therapy sessions focused on supporting their children and strengthening their relationship with their child. Results demonstrated that, when assessed at 3, 6, and 9 months posttreatment, both treatments had positive effects. However, the CBT group demonstrated greater decreases in grief symptoms at all posttreatment time points, and at 6 and 12 months, CBT considerably outperformed counseling in the domains of depression and PTSD symptoms. Margaret Crane and Lesley Norris, Ph.D. candidates, along with Dr. Philip Kendall from Temple University, contribute an editorial that speaks to moving beyond the findings presented in this study toward developing personalized treatment approaches for prolonged grief and modifying current treatment strategies to make them more widely accessible to suffering youth ( 19 ).
An Intervention in Children Living in Poverty Aimed at Reducing the Later Development of Psychopathology
Poverty is associated with numerous factors that are stressful and traumatic. To assess the extent to which an early school intervention can make a difference for impoverished children, Bierman et al. ( 20 ) report data from a randomized clinical trial examining the effects of an evidence-based intervention on the development of psychopathology, when assessed years later during adolescence. In this study, 356 4-year-old children from low-income families received an intervention consisting of a social-emotional learning program combined with an interactive reading program that was compared with usual educational practices. The children were recruited from three counties in Pennsylvania and came from families with a median annual income of $15,000. While differences between the interventions were not apparent when children were in the 7th grade, significant differences were observed when children reached 9th grade. For example, significantly fewer conduct problems, emotional symptoms, and peer problems were present in the 9th graders who, at 4 years of age, had participated in the social-emotional learning program. This study underscores the need to view the societal issue of poverty as stressful and traumatic, the disparities in health care associated with poverty, and the profound effects poverty can have on families and children. The findings are encouraging, with important public health implications, and clearly support early interventions aimed at promoting healthy social, emotional, and cognitive development in children facing the chronic adversity of growing up in poverty.
Enhanced Linkages Between Neural Activation and Inflammation in Impoverished Children
Miller and coauthors present data from a sample of early adolescents supporting an enhanced association between brain activation and peripheral inflammation that is selective to children living in poverty ( 21 ). The findings may shed light onto why underprivileged children have increased vulnerabilities to develop psychiatric and physical illnesses. The study was performed in 207 12- to 14-year-old children from the Chicago area who came from families across the socioeconomic spectrum. To explore a link between peripheral inflammation and neural function, the investigators correlated blood inflammatory markers (C-reactive protein, tumor necrosis factor-α, and interleukins-6, -8, and -10) with functional neuroimaging measures that assessed threat- and reward-related neural activation. First, the authors found that children living in poverty had higher levels of inflammation than children from higher socioeconomic backgrounds. In addition, the results demonstrated that in impoverished children, the inflammatory markers were positively correlated with both threat-related amygdala and reward-related striatal activation. The authors speculate that this enhanced coupling between neural and inflammatory processes may be due to the developmental impact of chronic adversity and may be a mechanism linking poverty to increased stress reactivity and illness. Interestingly, the positive relation between inflammatory markers and striatal activation was not in the predicted direction. Dr. Charles Nemeroff, from the University of Texas at Austin, contributes an editorial that emphasizes the deleterious effects of poverty on poor health and mental illness and further elaborates on the immune and neural alterations found in children who grow up in such impoverished and unfortunate conditions ( 22 ).
Neuroimaging Measures Are Not Good Predictors of Childhood Suicidal Ideation and Behavior
Vidal-Ribas and coworkers ( 23 ) use the large ABCD multimodal imaging database to comprehensively assess the usefulness of structural and functional brain measures in predicting childhood suicidal thoughts and behaviors. In a sample of 7,994 9- to 10-year-old children, the researchers found that 14.3% of the sample, or 1,140 children, had suicidal ideation or behaviors as reported by themselves or by caregivers. The occurrence of suicidal thoughts and behaviors was associated with increased levels of psychopathology and psychosocial adversity. Of the more than 5,000 statistical tests that were performed with multiple imaging measures (to assess cortical thickness, resting-state functional connectivity, and task-related functional activation), only one test survived correction for multiple comparisons. This finding revealed a relation between reduced thickness of the left bank of the superior temporal sulcus and caregiver-reported suicidal thoughts and behaviors. The authors draw the conclusion from these overall negative findings that current neuroimaging methods are not useful in reflecting the biological underpinnings of suicidal ideation and behavior in youth. In their editorial, Dr. Randy Auerbach from Columbia University and Drs. Henry Chase and David Brent from the University of Pittsburgh discuss the comprehensive and thorough nature of the study, the potential meaning of the superior temporal sulcus finding, and other critical aspects worth considering in future studies aimed at understanding the factors underlying youth suicide ( 24 ).
Reward-Related Functional Brain Alterations in Children With Disruptive Behavior Disorders and Callous-Unemotional Traits
Hawes et al. examine the extent to which children with disruptive behavior disorders (DBDs) (e.g., oppositional defiant disorder and conduct disorder) have alterations in neural responses to the anticipation and actual receipt of a reward ( 25 ). As in the Vidal-Ribas et al. study ( 23 ), these investigators used the ABCD neuroimaging database to provide a large sample size. Alterations in reward processing characterized by difficulties in delaying gratification and overvaluation of immediate rewards have been hypothesized to underlie externalizing phenotypes. In this study, reward-related brain activation in response to a monetary incentive delay task was examined in youth with DBDs who were subdivided into those with DBDs only (N=276) and those with DBDs with callous-unemotional traits (N=198), a characteristic that is more likely to be associated with antisocial behavior. The data from these children were compared with neuroimaging data from 693 typically developing youth. The children were, on average, 9.5 years old when studied. The findings from the study demonstrated that regardless of the presence of callous-unemotional traits, youth with DBDs exhibited decreased dorsal anterior cingulate activation in response to reward anticipation and increased orbitofrontal cortical and nucleus accumbens activation during reward receipt. Some neural activation differences between the DBD-only group and the DBD callous-unemotional trait group were also observed. Taken together, these findings shed light on the cortical control systems and subcortical reward-related neural substrates that may underlie the maladaptive behaviors characteristic of youth with DBDs.
Pretreatment Reward-Related Brain Activation Is Associated With Response to Psychotherapy in Youth With Anxiety Disorders
Sequeira and coworkers ( 26 ) explore the use of pretreatment functional imaging measures to predict treatment responses to psychotherapy in 9- to 14-year-old children with anxiety disorders (i.e., separation anxiety disorder, generalized anxiety disorder, or social anxiety disorder). Similar to other articles in this issue, this study probed reward-related brain activation. In this case, activation of brain regions encompassing the medial prefrontal cortex and the striatum was compared between the conditions of winning a reward relative to the experience of losing. The study included 50 children treated with 16 sessions of CBT, 22 children treated with child-centered therapy, and 37 healthy comparison youth. The intervention was effective, as 67% of the patients, regardless of treatment, responded to the intervention. Prior to treatment, greater activation of the medial prefrontal cortex was found in the patients with anxiety compared with the control subjects. However, the authors note that this difference in medial prefrontal activation could be accounted for by the co-occurrence of depressive symptoms in the anxiety group. Importantly, the authors found that as a group, treatment responders compared with nonresponders had increased pretreatment activation of regions encompassing the subgenual anterior cingulate cortex and the nucleus accumbens. These initial findings point to the potential importance of understanding reward-related brain systems in relation to psychotherapeutic outcomes in youth with anxiety. The authors speculate that increased striatal responsivity to rewards prior to treatment could be associated with increased motivation and engagement with therapy.
A Proof-of-Concept Trial Assessing Ketamine for Depression in Adolescents
Dwyer and colleagues ( 27 ) report the results of a small randomized double-blind single-dose crossover study in 17 adolescents with major depression who had not responded to at least one adequate trial of an antidepressant. In this trial, intravenous ketamine (0.5 mg/kg) or intravenous midazolam (0.045 mg/kg) was administered to each patient in a crossover design with a 2-week interval between treatments. Patients remained on their current psychiatric medications throughout the study. Sixteen patients completed both treatments, and the primary outcome was depression severity measured with the Montgomery-Åsberg Depression Rating Scale (MADRS) 24 hours after the infusion. Results demonstrated that 24 hours after infusion, ketamine had a significantly greater effect than midazolam in reducing depressive symptoms. For the midazolam infusion, the average pretreatment MADRS score was 31.88, and 24 hours later it was 24.13. For the ketamine infusion, the average pretreatment MADRS score was 30.56, and 24 hours later it was 15.44. Responders were defined by a 50% reduction in the MADRS score, and it was found that ketamine was associated with a response in 77% of the patients, with 35% of patients responding to midazolam (five of six of these participants also responded to ketamine). Compared with midazolam, the ketamine infusions were associated with reduced MADRS scores at all time points measured up to 14 days postinfusion. Ketamine was associated with dissociative side effects that were transient as well as with transient changes in blood pressure and heart rate. In their editorial ( 28 ), Drs. Parikh and Walkup from Northwestern University discuss the potential importance of this finding in relation to treating adolescent depression, but they also put into context such issues as the small sample size, the difficulty maintaining blinding because of ketamine’s dissociative effects, and concerns raised by others regarding the role of opiates in mediating ketamine’s effects in relation to its addiction potential ( 29 ).
Conclusions
Many psychiatric disorders have their origins early in life, which is clearly the case with anxiety and depression. In addition, the adolescent transition period is a time of increased risk during which psychiatric illnesses, especially depression, tend to emerge. Although there are adequate treatments for youth with anxiety and depressive disorders, many individuals do not respond to current treatments, and it is important to emphasize that many young people with psychiatric illnesses do not have access to available treatments. There is no question that we need better treatments and better access for children suffering from these disorders. This issue of the Journal highlights recent insights and clinical advances related to the treatment of anxiety disorders and major depression. Findings with the potential to directly affect the clinical care of youth include: demonstration of the efficacy of CBT in treating prolonged grief; early school socioemotional and cognitive interventions in disadvantaged children that prevent adolescent psychopathology; the rapid efficacy and safety of ketamine in reducing depressive symptoms in youth with treatment-resistant depression; and the promise of using functional neuroimaging to inform treatment choice and predict outcomes in youth with anxiety disorders. Other articles in this issue address pathophysiology, demonstrating increased coupling between brain and peripheral inflammatory markers in impoverished youth, altered reward-related brain activation in youth with DBDs, and a lack of association between structural and functional neuroimaging measures with suicidal ideation and behavior in youth. Continued research focused on a better understanding of the mechanisms underlying the early life risk to develop anxiety disorders and major depression is critical for the development of novel, improved treatment strategies. Efforts should be devoted to developing treatments that have the potential to positively affect the at-risk neurodevelopmental trajectories of vulnerable children. Such early life interventions provide the hope of moving beyond symptomatic treatment and toward prevention strategies.
Disclosures of Editors’ financial relationships appear in the April 2021 issue of the Journal .
1 Merikangas KR, He JP, Burstein M, et al. : Lifetime prevalence of mental disorders in U.S. adolescents: results from the National Comorbidity Survey Replication–Adolescent Supplement (NCS-A) . J Am Acad Child Adolesc Psychiatry 2010 ; 49:980–989 Crossref , Medline , Google Scholar
2 Substance Abuse and Mental Health Services Administration (SAMHSA) : National Survey on Drug Use and Health . SAMHSA, Rockville, Md, 2017 Google Scholar
3 America’s Health Rankings : Teen suicide. https://www.americashealthrankings.org/explore/health-of-women-and-children/measure/teen_suicide/state/ALL Google Scholar
4 Centers for Disease Control and Prevention (CDC) : WISQARS Leading Causes of Death Reports . CDC, Atlanta, 2018 Google Scholar
5 Levey DF, Gelernter J, Polimanti R, et al. : Reproducible genetic risk loci for anxiety: results from ∼200,000 participants in the Million Veteran Program . Am J Psychiatry 2020 ; 177:223–232 Link , Google Scholar
6 Border R, Johnson EC, Evans LM, et al. : No support for historical candidate gene or candidate gene-by-interaction hypotheses for major depression across multiple large samples . Am J Psychiatry 2019 ; 176:376–387 Link , Google Scholar
7 Clauss JA, Blackford JU : Behavioral inhibition and risk for developing social anxiety disorder: a meta-analytic study . J Am Acad Child Adolesc Psychiatry 2012 ; 51:1066–1075.e1 Crossref , Medline , Google Scholar
8 Akingbuwa WA, Hammerschlag AR, Jami ES, et al. : Genetic associations between childhood psychopathology and adult depression and associated traits in 42 998 individuals: a meta-analysis . JAMA Psychiatry 2020 ; 77:715–728 Crossref , Medline , Google Scholar
9 March J, Silva S, Petrycki S, et al. : Fluoxetine, cognitive-behavioral therapy, and their combination for adolescents with depression: Treatment for Adolescents With Depression Study (TADS) randomized controlled trial . JAMA 2004 ; 292:807–820 Crossref , Medline , Google Scholar
10 Reinecke MA, Curry JF, March JS : Findings from the Treatment for Adolescents with Depression Study (TADS): what have we learned? What do we need to know? J Clin Child Adolesc Psychol 2009 ; 38:761–767 Crossref , Medline , Google Scholar
11 Walkup JT, Albano AM, Piacentini J, et al. : Cognitive behavioral therapy, sertraline, or a combination in childhood anxiety . N Engl J Med 2008 ; 359:2753–2766 Crossref , Medline , Google Scholar
12 Ginsburg GS, Becker-Haimes EM, Keeton C, et al. : Results from the Child/Adolescent Anxiety Multimodal Extended Long-Term Study (CAMELS): primary anxiety outcomes . J Am Acad Child Adolesc Psychiatry 2018 ; 57:471–480 Crossref , Medline , Google Scholar
13 Kovner R, Oler JA, Kalin NH : Cortico-limbic interactions mediate adaptive and maladaptive responses relevant to psychopathology . Am J Psychiatry 2019 ; 176:987–999 Link , Google Scholar
14 Rappaport BI, Kandala S, Luby JL, et al. : Brain reward system dysfunction in adolescence: current, cumulative, and developmental periods of depression . Am J Psychiatry 2020 ; 177:754–763 Link , Google Scholar
15 Lahat A, Benson BE, Pine DS, et al. : Neural responses to reward in childhood: relations to early behavioral inhibition and social anxiety . Soc Cogn Affect Neurosci 2018 ; 13:281–289 Medline , Google Scholar
16 Fox AS, Kalin NH : A translational neuroscience approach to understanding the development of social anxiety disorder and its pathophysiology . Am J Psychiatry 2014 ; 171:1162–1173 Link , Google Scholar
17 Fox AS, Oler JA, Shackman AJ, et al. : Intergenerational neural mediators of early-life anxious temperament . Proc Natl Acad Sci USA 2015 ; 112:9118–9122 Crossref , Medline , Google Scholar
18 Boelen PA, Lenferink LIM, Spuij M : CBT for prolonged grief in children and adolescents: a randomized clinical trial . Am J Psychiatry 2021 ; 178:294–304 Abstract , Google Scholar
19 Kendall PC, Norris LA, Crane ME : Personalizing and delivering treatment for prolonged grief in youths (editorial). Am J Psychiatry 2021 ; 178:280–281 Abstract , Google Scholar
20 Bierman KL, Heinrichs BS, Welsh JA, et al. : Reducing adolescent psychopathology in socioeconomically disadvantaged children with a preschool intervention: a randomized controlled trial . Am J Psychiatry 2021 ; 178:305–312 Abstract , Google Scholar
21 Miller GE, White SF, Chen E, et al. : Association of inflammatory activity with larger neural responses to threat and reward among children living in poverty . Am J Psychiatry 2021 ; 178:313–320 Abstract , Google Scholar
22 Nemeroff CB : The trifecta of misery and disease vulnerability: poverty, childhood maltreatment, and inflammation (editorial). Am J Psychiatry 2021 ; 178:282–284 Abstract , Google Scholar
23 Vidal-Ribas P, Janiri D, Doucet GE, et al. : Multimodal neuroimaging of suicidal thoughts and behaviors in a U.S. population-based sample of school-age children . Am J Psychiatry 2021 ; 178:321–332 Abstract , Google Scholar
24 Auerbach RP, Chase HW, Brent DA : The elusive phenotype of preadolescent suicidal thoughts and behaviors: can neuroimaging deliver on its promise? (editorial). Am J Psychiatry 2021 ; 178:285–287 Abstract , Google Scholar
25 Hawes SW, Waller R, Byrd AL, et al. : Reward processing in children with disruptive behavior disorders and callous-unemotional traits in the ABCD study . Am J Psychiatry 2021 ; 178:333–342 Abstract , Google Scholar
26 Sequeira SL, Silk JS, Ladouceur CD, et al. : Association of neural reward circuitry function with response to psychotherapy in youths with anxiety disorders . Am J Psychiatry 2021 ; 178:343–351 Link , Google Scholar
27 Dwyer JB, Landeros-Weisenberger A, Johnson JA, et al. : Efficacy of intravenous ketamine in adolescent treatment-resistant depression: a randomized midazolam-controlled trial . Am J Psychiatry 2021 ; 178:352–362 Link , Google Scholar
28 Parikh T, Walkup JT : The future of ketamine in the treatment of teen depression (editorial). Am J Psychiatry 2021 ; 178:288–289 Abstract , Google Scholar
29 Williams NR, Heifets BD, Blasey C, et al. : Attenuation of antidepressant effects of ketamine by opioid receptor antagonism . Am J Psychiatry 2018 ; 175:1205–1215 Link , Google Scholar
- Cited by None
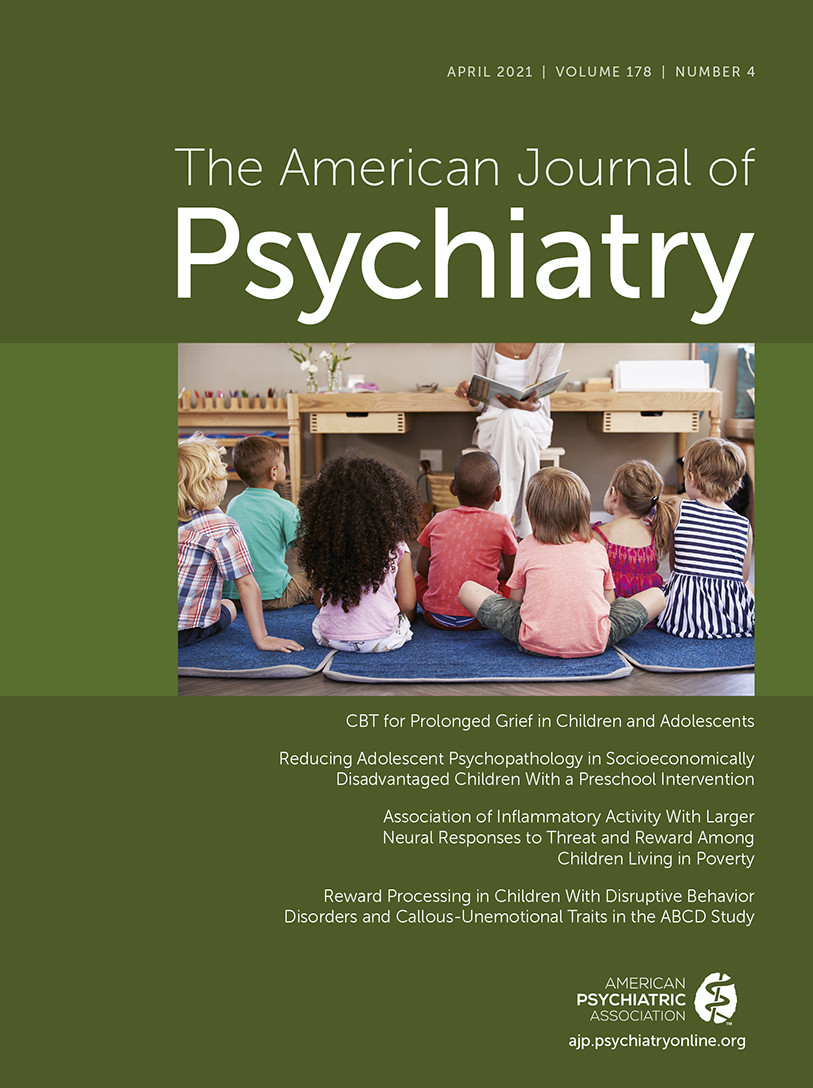
- Anxiety Disorders
- Depressive Disorders
- Suicide and Self-Harm
- Child/Adolescent Psychiatry
Thank you for visiting nature.com. You are using a browser version with limited support for CSS. To obtain the best experience, we recommend you use a more up to date browser (or turn off compatibility mode in Internet Explorer). In the meantime, to ensure continued support, we are displaying the site without styles and JavaScript.
- View all journals
- My Account Login
- Explore content
- About the journal
- Publish with us
- Sign up for alerts
- Open access
- Published: 09 June 2022
Loneliness associates strongly with anxiety and depression during the COVID pandemic, especially in men and younger adults
- Olivier D. Steen 1 ,
- Anil P. S. Ori 1 , 2 ,
- Klaas J. Wardenaar 1 &
- Hanna M. van Loo 1
Scientific Reports volume 12 , Article number: 9517 ( 2022 ) Cite this article
5148 Accesses
13 Citations
41 Altmetric
Metrics details
- Human behaviour
Loneliness is associated with major depressive disorder (MDD), and likely also with generalized anxiety disorder (GAD). It is unclear if these associations are moderated by age, sex, or genetic susceptibility for MDD. We included 75,279 individuals from the Lifelines COVID-19 study, a longitudinal study of a Dutch population-based cohort. Participants completed up to sixteen digital questionnaires between March 2020 and January 2021, yielding a total of 616,129 observations. Loneliness was assessed with the Three-Item Loneliness Scale, and MDD and GAD with the Mini-International Neuropsychiatric Interview. We used generalized estimating equations to investigate the association between loneliness and MDD and GAD, and whether this association varied across time, age, sex and MDD polygenic risk. Loneliness was strongly associated with all MDD and GAD outcomes. Individuals with the highest loneliness scores were around 14 times more likely to have MDD, and 11 times more likely to have GAD, compared to individuals who reported the least loneliness. The association between loneliness and MDD symptoms was stronger in men, younger individuals, and increased across time. While MDD polygenic risk predicted MDD and GAD outcomes, we did not find an interaction effect with loneliness. Our study, which is the largest to date, confirms that loneliness is an important risk factor for MDD, GAD, depressive and anxiety symptoms, especially in men and younger individuals. Future studies should investigate the mechanisms of these associations and explore loneliness-based interventions to prevent and treat MDD and GAD.
Similar content being viewed by others
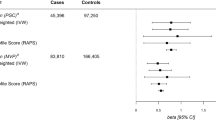
Loneliness and depression: bidirectional mendelian randomization analyses using data from three large genome-wide association studies
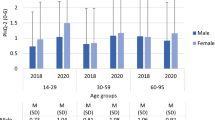
Mental health and loneliness in the German general population during the COVID-19 pandemic compared to a representative pre-pandemic assessment
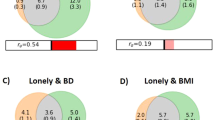
Polygenic overlap and shared genetic loci between loneliness, severe mental disorders, and cardiovascular disease risk factors suggest shared molecular mechanisms
Introduction.
Major depressive disorder (MDD) and generalized anxiety disorder (GAD) are two of the most common mental illnesses worldwide, with substantial morbidity and mortality 1 , 2 . Gaining a better understanding of the mechanisms that underlie their development is important to reduce the burden of these illnesses. The identification of modifiable risk factors is of particular interest because they provide targets for intervention.
The current COVID-19 pandemic has highlighted the importance of loneliness as a modifiable risk factor for MD 3 . Loneliness is the subjective negative experience of deficient social relationships and is distinct from objective social isolation 4 . Research on loneliness and depression indicates a bidirectional relationship between both constructs, with greater experienced loneliness predicting future depressive symptoms, and vice versa 5 . Furthermore, loneliness influences the course of MDD, impeding the chances of recovery 6 . Recent findings furthermore suggest an association between loneliness and GAD as well, but fewer studies have been performed 7 , 8 .
However, it is not clear which groups are most vulnerable to the effect of loneliness on depression and anxiety. Different factors may moderate this association, including sex, age and genetic susceptibility. Findings regarding age are mixed. Some studies suggest an increased vulnerability in younger individuals, but few studies included sufficient numbers of adolescents and children to reliably demonstrate this 9 , 10 . Whether sex moderates the effect of loneliness is also unclear. One study found the effect of loneliness on depression to be more pronounced in men 5 , while others did not observe a moderating effect 9 . Thus, it remains inconclusive whether age or sex moderate the association between loneliness and depression and anxiety outcomes.
Furthermore, the association between loneliness and depressive and anxiety outcomes may be influenced by genetic factors. Loneliness, MDD, and GAD have moderate heritability, which means that genetic factors contribute to their aetiology 11 , 12 , 13 . Genome-wide association studies (GWAS) have identified multiple overlapping regions in the genome which are associated with both experiencing loneliness 14 and developing MDD 15 , 16 . This genetic overlap suggests that loneliness and depression could have a shared genetic aetiology, or that individuals with a higher genetic risk for MDD could be more sensitive to developing depression after exposure to loneliness.
The COVID-19 pandemic offers a unique situation to study the relationship of loneliness with depression and anxiety. The COVID-19 pandemic, and subsequent lockdowns, have had a profound impact on social relationships and increased the prevalence of loneliness 3 , 17 , 18 . This is akin to a natural experiment: a large share of the population is subjected to sustained loneliness, and not only subjects with a psychiatric history or other specific groups. Some studies have investigated the impact of loneliness on anxiety and depression amidst the COVID pandemic, and report an association between loneliness and anxiety and depression 19 , with young women most at risk 20 , but their findings are challenging to interpret due to the use of unrepresentative and smaller samples. The present study makes use of the Lifelines Cohort Study, which represents a large multi-generational population-based study in the North of the Netherlands with repeated and structured assessments of loneliness, MDD and GAD during the COVID-19 pandemic.
Here, we investigate the association of loneliness with depression and anxiety, as well as moderators of this association, in the largest study to date on this relationship.
Lifelines COVID-19 cohort
Data were derived from the Lifelines COVID-19 study, a longitudinal extension of the Lifelines cohort 21 . Lifelines is a large multidisciplinary prospective population-based cohort that monitors the health and health-related behaviours in a detailed manner of 167,729 persons living in the North of the Netherlands. The cohort consists primarily (98%) of white Dutch native people 22 , 23 . All subjects provided written informed consent for participation in Lifelines, and the Medical Ethical Committee of the University Medical Center Groningen approved the study protocol.
Starting on March 30th 2020, Lifelines invited all adult participants of whom an email address was known (n = 139,679) to participate in the first COVID-19 digital questionnaire, which assessed somatic and mental health, COVID-19 infection status, and loneliness, among other domains. The first questionnaire was distributed on March 30, 2020. The first six questionnaires (Q1–Q6) were sent out weekly with items assessing participants' experiences in the 7 days prior to filling out the questionnaire. Starting from Q7, questionnaires were sent biweekly or monthly, with items assessing participants’ experiences in the 14 days prior to assessment (Supplementary Table A ). A further description of the Lifelines COVID-19 cohort can be found elsewhere 24 .
Data from the first nineteen consecutive assessments (March 30th, 2020, to January 29th, 2021) were available. Questionnaires eleven, twelve and thirteen did not include measures of loneliness, so data from these measurements were not included in our analyses. A total of n = 75,279 individuals participated in at least one of the remaining sixteen questionnaires and are included in this study. In some instances (n = 14,763), participants completed multiple different assessments on the same date. In this case, we included only the questionnaire completed first.
Measurements
Depression and anxiety outcomes.
Symptoms of GAD and MDD were assessed with a self-report digital questionnaire based on the Mini-International Neuropsychiatric Interview (MINI) 25 , which assesses all symptoms that are part of the DSM-IV diagnostic criteria. Symptoms that are part of both MDD and GAD (being easily fatigued, difficulty concentrating and sleep disturbance) were assessed once in every questionnaire to avoid repetition.
The presence of current MDD and GAD were established according to the diagnostic criteria of the DSM-IV. For every questionnaire, we also calculated a sum score of MDD (range 0–9) and GAD (range 0–7) criterion symptoms, resulting in two outcomes (symptoms and diagnoses) for both MDD and GAD.
The experience of loneliness was assessed through the previously validated three-item UCLA scale 26 . This scale consists of three items (‘How often do you feel that you lack companionship?’, ‘How often do you feel left out?’ and ‘How often do you feel isolated from others?’), with a three-point (0–2) Likert scale. We calculated a loneliness sum score ranging from 0 to 6, with 6 being the maximum score.
Demographic variables
Age was defined as subjects’ age when completing the first questionnaire. The sex variable refers to biological sex assigned at birth, which was determined by linking the Lifelines data to information stored in personal records of the municipalities in the Netherlands.
Polygenic risk score
We calculated MDD polygenic risk scores (PRS) in 19,128 subjects who had genotype data available. MDD PRS was constructed as a weighted sum of risk alleles for MDD with weights defined as single nucleotide polymorphism (SNP) effect sizes derived from the meta-analysis of the Psychiatric Genomics Consortium (PGC) and UKBiobank GWASs 15 . The Lifelines sample was not included in the base GWAS, but was used as a target sample to calculate PRS for each participant. Details of PRS calculation are discussed in Supplementary Methods .
Missing data
While all subjects were invited to participate in all assessments, few completed all 16 assessments. Out of 75,279 subjects, 11,528 (15.3%) completed one assessment, 54,409 (72.3%) completed > 1 and < 16 assessments, and 9342 (12.4%) completed all assessments. As expected, there were thus a large number of non-responses for different time points.
Some data were missing due to design choices of the questionnaire (e.g., suicidal ideation was not assessed in every instance). As the questionnaire was web-based, some data were missing due to technical glitches (e.g., a failing internet connection on the participants’ end). Further details on missing data are provided in the Supplementary Methods , while rates of missing data are reported in Supplementary Table B .
As missing data were limited, we implemented multivariate imputation by chained equation (MICE) to impute missing data in one dataset 27 . For imputation, all available data, including data on loneliness, lifetime history of MDD and GAD and accompanying age of onset and number of experienced episodes, stressful life events, and the NEO personality inventory neuroticism score that were collected in previous Lifelines assessments, were used as predictors in MICE. Items were only imputed if a participant filled out other items on that specific questionnaire. Questionnaires with a non-response (i.e. complete missing data) were not imputed. Detailed imputation parameters are presented in Supplementary Methods .
Statistical methods
Model specification.
We used generalized estimating equations (GEE) to investigate the association between loneliness and the four depression and anxiety outcomes. GEE is a technique for estimating parameters of a generalized linear model with correlated measurements, yielding population-averaged coefficients. Because our data included repeated measurements within subjects, we used an exchangeable working correlation structure to account for the dependence of measurements within individuals. A further description of GEE can be found elsewhere 28 .
We performed GEE Poisson regression for the sum scores of MDD and GAD symptoms as both of these outcomes were count-variables. We used GEE logistic regression for the dichotomous outcomes of MDD and GAD. The fitted models included a linear and squared effect of time to account for seasonality. Furthermore, we included loneliness, age and sex as main effects. We added an interaction term between loneliness and time to investigate a possible change in the impact of loneliness on MDD and GAD outcomes across the study duration. We furthermore added interaction terms between loneliness and age, and loneliness and sex (main model) to identify possible moderating effects of age and/or sex. For the model including PRS in participants with genetic data available, we also added a main effect for MDD polygenic risk to the model, as well as an interaction term of PRS with loneliness. Furthermore, for the model including PRS, we included ten principal components as model terms to account for population structure confounding.
Multiple testing correction was implemented by Bonferroni correction. We conducted ten hypothesis tests across four outcomes, yielding a total of 40 hypothesis tests and a Bonferroni-corrected alpha of 0.00125. We performed GEE using the ‘geepack’ R package version 1.3.2 29 , and all analyses were performed in R version 4.0.3 30 .
Sensitivity analyses
Attrition bias.
Individuals experiencing much loneliness, or who suffer from mental illnesses, may more likely be lost to attrition in longitudinal studies 31 . To determine if results were sensitive to attrition, we conducted a sensitivity analysis with individuals who participated at least three times across three intervals covering the entire study duration. As assessment dates were variable, we constructed these intervals in such a way that the number of assessments was equal across intervals. The first time interval covered the period March 30, 2020 until 29 April 2020. The second interval covered the interval of 30 April 2020 until 10 July 2020. The third interval covered 10 July 2020 until 31 January 2021. 42,001 (55.8%) participants had completed at least one assessment in each of the three intervals and were included in this sensitivity analysis. Participants in this subsample completed a median of 13 [IQR 10–15] responses.
Family structure
Lifelines is a multi-generational sample and includes family members, which means that our longitudinal data were not only nested within individuals, but also within families. GEE cannot appropriately account for correlated measurements across multiple levels of clustering 28 . Therefore, to test whether our results were influenced by pedigree structures, we conducted a sensitivity analysis in a subsample consisting of one randomly selected subject for every family. As there were 42,089 pedigrees part of the present study, this analysis included 42,089 (55.9%) subjects.
For both sensitivity analyses, we estimated models in the full sample, and in the sample with PRS data available. Because only a limited number of participants had PRS data available, subsamples in the sensitivity analyses are comparatively small (n = 10,955 and n = 7036), and possibly underpowered to detect interaction effects. The latter was therefore only used to test the robustness of a possible interaction term between loneliness and PRS.
Ethics approval and consent to participate
All subjects provided written informed consent for participation in Lifelines, and the Medical Ethical Committee of the University Medical Center Groningen approved the study protocol. All methods were carried out in accordance with relevant guidelines and regulations.
Characteristics of the study population
The 75,279 individuals included in this study completed a total of 616,129 assessments across 16 time points. Out of these individuals, 19,128 (25.4%) had genotype data available. The median number of collected questionnaires per individual was 8 (IQR 3–14) (Table 1 ). The mean age at first assessment was 53.7 (standard deviation 12.9). The majority of participants in the sample were female (60.8%), which is similar to the full Lifelines cohort 23 . MDD was present in 5442 (7.2%) participants and GAD in 6733 (8.9%) participants in the 1/2 weeks preceding at least one questionnaire assessment. Loneliness scores were highest during the start and end of data collection, corresponding to periods of nationwide lockdown as a result of government action in response to the COVID-19 pandemic (Fig. 1 A).
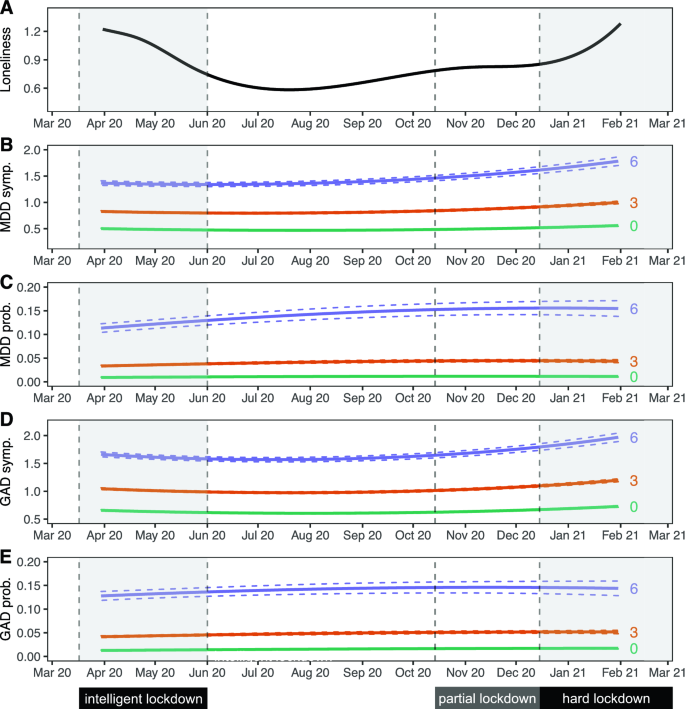
Trajectories of loneliness and MDD and GAD outcomes over time. Shaded areas represent periods during which lockdown measures were enforced in the Netherlands. The first 'intelligent' lockdown consisted of restrictions such as social distancing and bans on public gatherings. During the partial lockdown, some restrictions were reintroduced. The 'hard' lockdown introduced more restrictions and a curfew. ( A ) Marginal GEE effect plot of a GEE model of loneliness scores predicted by a polynomial spline time term. The 95% confidence interval of model-predicted values is not visible because the interval is very small. ( B – E ) Marginal GEE effect plot of predicted counts of MDD symptoms ( B ), odds of MDD diagnoses ( C ), counts of GAD symptoms ( D ) and odds of GAD diagnoses ( E ) across different levels (0/green; lowest, 3/orange; moderate, 6/purple; highest) of loneliness scores. Predicted values represent symptoms counts and diagnosis probabilities, and are based on main model fits (Tables 2 and 3 ). Dashed lines represent a 95% confidence interval of model-predicted values.
Loneliness is associated with MDD and GAD symptoms and diagnoses
We observed an association between loneliness and MDD and GAD. We found that a one-point increase in loneliness was associated with a 55.9% increased odds of MDD, and a 48.9% increased odds of GAD. This means that the group scoring highest on loneliness had a 14.4 times increased odds of MDD and a 10.9 times increased odds of GAD compared to those with the lowest loneliness scores (Tables 2 and 3 , Fig. 1 C, E ). Sensitivity analyses showed that these effects were robust for attrition and family structures (Supplementary Tables D 1-3 and E 1-3).
We found a similar association between loneliness and symptom severity of both MDD and GAD, which was robust across both sensitivity analyses (Tables 2 and 3 ; Fig. 1 B, D ; Supplementary Tables D 1-3 and E 1-3). A one-point increase in loneliness was associated with a 27.5% increase in the number of MDD symptoms, and a 19.9% increase in the number of GAD symptoms. This means that the group scoring highest on loneliness had on average 4.3 times as many MDD symptoms, and 3.0 times as many GAD symptoms, compared to the group with the lowest loneliness score.
We observed a significant interaction between loneliness and time, meaning that the magnitude of the association between loneliness and MDD symptoms increased slightly across time (Tables 2 and 3 , Fig. 1 B,D). A 1 week increment in time was associated with a 0.06% increase in effect size, which corresponds to a 2.4% stronger association between loneliness and MDD symptoms after 10 months (roughly the duration covered by the repeated questionnaires). This interaction effect remained significant in both sensitivity analyses. Taken together, these analyses demonstrate a strong association between loneliness and MDD and GAD outcomes.
Moderators of the association between loneliness and depression
We found a significant interaction effect of loneliness and sex, indicating that the association between loneliness and MDD symptoms was stronger in men than in women (Table 2 , Fig. 2 ). This effect was consistent across both sensitivity analyses. We observed a similar result for GAD symptoms, but this was not robust in the sensitivity analysis for attrition. We performed a sex-stratified analysis for MDD symptoms to estimate effect sizes for men and women separately (Supplementary Table C 5). Results were in agreement with the main analysis, with a point increase in loneliness score being associated with a 32.1% increase in MDD symptoms in men, compared to 22.5% in women. These analyses suggest that the association between loneliness and symptoms of MDD is stronger in men.
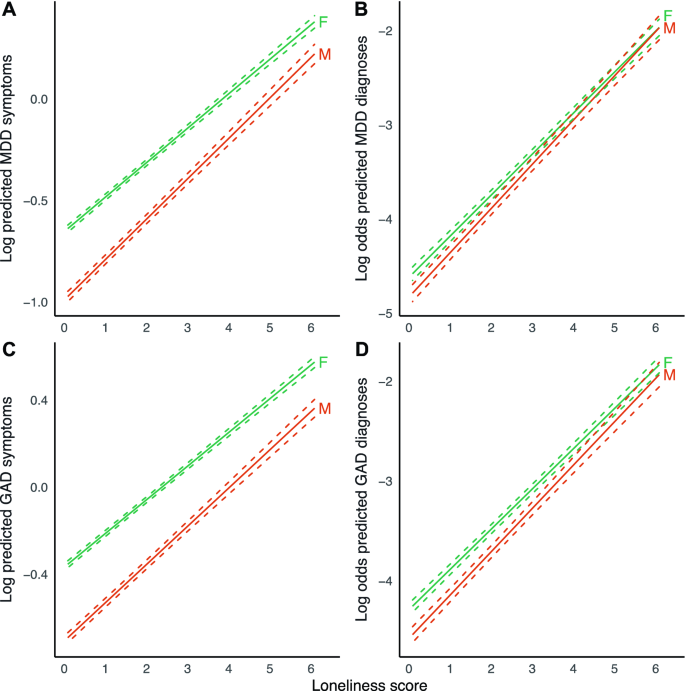
Marginal effect plots of associations between loneliness and MDD and GAD outcomes across sex. Displayed are log predicted counts of MDD symptoms ( A ) and log odds of MDD diagnosis ( B ), as well as log predicted counts of GAD symptoms ( C ) and log odds of GAD ( D ), across different loneliness scores, for men (M) and women (F) (plotted estimates are derived from Tables 2 and 3 , main models). Dashed lines represent 95% confidence intervals of model-predicted values.
We found a significant interaction effect between loneliness and age for MDD symptoms (Table 2 , Fig. 3 ), indicating that the magnitude of the association between loneliness and MDD symptoms increased slightly across age. This finding was robust across both sensitivity analyses. A 1 year increment in age was associated with a 0.08% reduction in effect size, which corresponds to a 4.7% weaker association between loneliness and MDD symptoms with a 60-year age difference. A point increase in loneliness is then associated with an increase in the number of MDD symptoms of 25.5% in 20-year-olds, compared to 19.6% in 80-year-olds. We observed no moderating effect of age for the other outcomes.
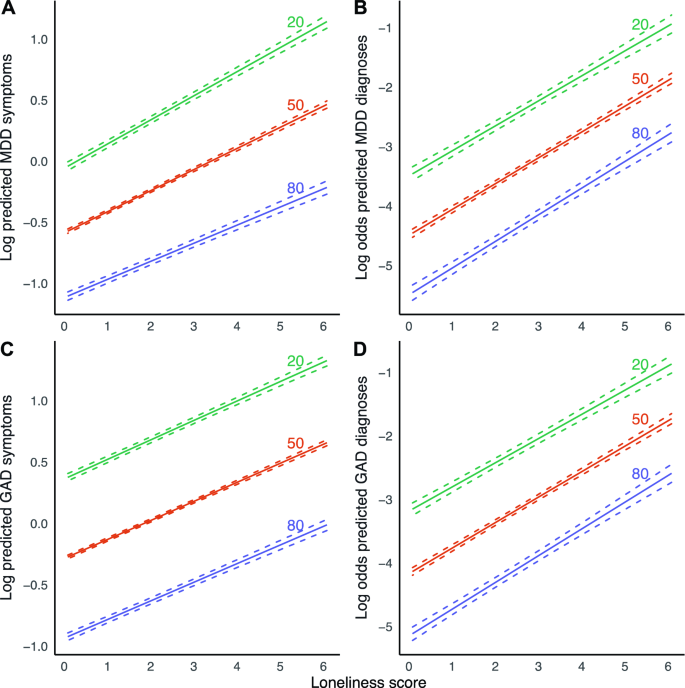
Marginal effect plots of associations between loneliness and MDD and GAD outcomes across age. Displayed are log predicted counts of MDD symptoms ( A ) and log odds of MDD diagnosis ( B ), as well as log predicted counts of GAD symptoms ( C ) and log odds of GAD ( D ), across different loneliness scores, across three levels of age in years (plotted estimates are derived from Tables 2 and 3 , main models). Dashed lines represent 95% confidence intervals of model-predicted values.
We found a significant main effect of PRS on symptoms of both MDD and GAD, as well MDD and GAD diagnoses, with a one standard deviation increase in PRS Z-score being associated with 26.5% increased odds of MDD, and 20.0% increased odds of GAD. We however did not observe a modifying effect of PRS on the association between loneliness and the four outcomes in any of the models.
Summary of main findings
This study investigated the association between loneliness and (symptoms of) MDD and GAD, and whether this association differs across age, sex and MDD PRS. We found significant associations between loneliness and MDD, GAD, MDD symptoms and GAD symptoms. On average, individuals who experienced the most loneliness were about 14 times more likely to report MDD, and around 11 times more likely to report GAD compared to individuals experiencing the least loneliness. For MDD symptoms, the strength of this association increased across time, becoming ~ 2.4% stronger between April 2020 and February 2021.
The association between loneliness and symptoms of MDD was significantly stronger in men than in women. The association of loneliness and MDD symptoms was also stronger in younger individuals. MDD polygenic risk did not modify the association between loneliness and the different outcomes.
Interpretation of findings and comparison with previous studies
We found a robust association between loneliness and MDD symptom severity. We found a similar association between loneliness and MDD. These findings are in line with multiple previous population-based studies 5 , 9 , 32 , which we now replicate in, to our knowledge, the largest study conducted to date. The strength of the association between loneliness and symptoms of MDD, but not MDD diagnoses, increased across the study duration. This could be due to several reasons. It may, for example, suggest that, as the COVID-19 pandemic lingered on, its influence magnified the effect of loneliness on specific depressive symptoms, but that this increase in symptoms did not necessarily cause a participant to develop MDD. Future studies could test this hypothesis, and assess if loneliness associates with only specific depressive symptoms. Alternatively, although the depressive symptom score and MDD are positively associated, they are distinct phenotypic constructs. MDD will likely display less variation across the period of data collection compared to MDD symptoms. Furthermore, the association of the two constructs with loneliness may also be different. Lastly, the present study may be underpowered to detect a significant interaction term for dichotomous outcomes (MDD), compared to continuous outcomes (MDD symptoms). However, we think that our sample of > 75,000 individuals and > 600,000 measurements should be large enough to trace any interaction effect with a relevant effect size.
We also found a robust association between loneliness and symptoms of GAD as well as GAD diagnoses, which is in line with previous studies 7 , 8 . This could mean that loneliness is a risk factor for GAD, or it could mean that GAD is a risk factor for loneliness or both. We however did not find that this association changed over time, suggesting that the course of the COVID-19 pandemic did not impact the relationship between loneliness and (symptoms of) GAD.
We found that the association between loneliness and depressive symptoms was stronger in men than in women. Results in previous studies were inconsistent. Cacioppo et al. found a stronger association in men in a cross-sectional study of 1939 older adults 5 . However, Lee et al. did not find a moderation effect of sex in a longitudinal study with baseline loneliness as a predictor and depressive symptoms at follow-up as an outcome, in a sample of 4211 older adults 9 . The present study assessed loneliness and outcomes across multiple contemporaneous timepoints. It may be that the moderating effect of sex disappears in time-lagged scenarios—possibly, loneliness has a stronger transient impact in men, without having a stronger effect on sustained depressive symptoms or disorders. Another explanation is that previous studies may not have been sufficiently powered to consistently detect a moderating effect of sex. We stress the importance of conducting such analyses in large population-based samples. Furthermore, differences in study populations, such as demographic, social, and environmental factors could contribute to different study outcomes as well.
We found a modifying effect of younger age on the association between loneliness and MDD symptoms. One previous study conducted in 1006 adults observed a significant interaction effect between loneliness and age on both anxiety and depressive symptoms 10 , while this effect was not found in a sample of 4211 adults above 50 years of age 9 . A lack of young adults in these previous studies may have contributed to these mixed findings. As MDD and GAD often first develop in adolescents or young adults, it is warranted that this age group is included in study cohorts, as is the case for the Lifelines cohort.
The present study did not find a significant moderation effect of MDD PRS on the association between loneliness and anxiety or depression. As far as we know, no comparable studies exist. A previous study by Lee et al. did assess the impact of both loneliness and MDD PRS on the association between loneliness and depression, but only as a confounder, not as an interaction term with loneliness. The authors found that the effect of loneliness persisted also in a model adjusted for PRS 9 . PRS for MDD currently explains only a limited amount of variance (Nagelkerke’s R 2 1.5–3.2%) of MDD 15 , thereby capturing only a small proportion of the heritability. As PRS does not capture the complete genetic architecture of MDD, we cannot exclude that genetic factors may impact the relationship between loneliness and MDD and GAD outcomes. Furthermore, while loneliness and depression share genes and heritability, both constructs likely also have phenotype-specific heritability, while the present study only employed genetic susceptibility for MDD. Perhaps, a PRS for loneliness or a PRS calculated from gene variants shared between the two constructs might be a significant moderator. Future work should revisit such analyses as sample sizes of GWASs, continue to increase. Finally, genetic analyses are worthwhile to explore for GAD and loneliness PRS as well, especially now that larger GWASs have been conducted 14 , 33 .
While loneliness is strongly associated with MDD and GAD outcomes, many individuals experiencing significant loneliness do not develop MDD or GAD. While age and sex explain some of these differences, future research should include other variables as well to further understand how loneliness impacts MDD and GAD. These might include socioeconomic variables, specific environmental variables, or psychological traits related to (tolerance of) loneliness and isolation.
Strengths and limitations
A strength of the present study is its large sample size and longitudinal design, with repeated measures of loneliness and depression and anxiety outcomes that are measured using multi-item and well-validated instruments.
As our study was conducted amidst the COVID-19 pandemic in the North of the Netherlands, it raises the question of whether our results are generalizable to populations not or no longer affected by the pandemic or to other regions or countries. We think results may be robust, given that the effect sizes of the association between loneliness and MDD and GAD outcomes are comparable to those found in studies conducted before the pandemic and in other populations 5 , 9 .
Because of the initial weekly and later biweekly/monthly assessments of symptoms, we only assessed MDD and GAD symptoms during the past week or 2 weeks. The DSM requires GAD symptoms to be present for 6 months, which means that our GAD diagnoses likely do not correspond to those of the cases seen in clinical practice. A similar point can be made for MDD diagnoses, which require 14 days of symptoms according to the DSM.
The present study focused on contemporaneous associations but did not investigate temporal relations between loneliness and MDD or GAD. While previous longitudinal studies showed that loneliness predicts (symptoms of) MDD 9 , and vice versa 5 , such studies have not yet been performed for GAD. In addition, studies involving loneliness-targeted interventions can further elucidate the causal relevance of loneliness towards depression and anxiety outcomes, besides evaluating its value as a target for treatment.
As we imputed one single dataset, we have sub-optimally accounted for the uncertainty introduced by our missing data handling approach 34 . However, as we had limited missing data and most data were missing by design (i.e., missing completely at random), we deem it unlikely to have significantly influenced our results.
The present study used a GEE marginal model as opposed to a conditional approach such as generalized linear mixed-effects models. The latter approach also allows the estimation of population-averaged estimates and can adjust for multiple correlation structures (such as for correlation within both individuals and families). This approach however was not feasible as it required excessive computational resources given our large sample size. However, in this study, the estimates from GEE models are likely to be in line with results that would have been derived from conditional models. First, estimates obtained from a GEE model agree closely with those from a conditional model if the right assumptions are being met, as is the case for the present study 35 . Second, our sample size was large, which means that we had sufficient power to trace small effects, even with GEE.
Implications
The current study replicates that loneliness is strongly associated with MDD and demonstrates a significant association in a large population-based sample for GAD. We furthermore observed that the association between loneliness and MDD symptoms became stronger over time. As COVID-19-related restrictions have led to more loneliness in the population, this might precipitate a subsequent increase in diagnoses, possibly even as government restrictions are being loosened. Our findings warrant extra vigilance in groups such as younger individuals, who experienced more loneliness.
Besides its association with MDD and GAD, loneliness is strongly predictive of a myriad of adverse health outcomes, such as cardiovascular disease and mortality 36 . Currently, few effective interventions are used routinely in clinical practice or the community, while effective interventions do exist 37 , 38 . Furthermore, there is a stigma surrounding loneliness 39 , and it has not received significant attention in clinical practice or policy. This is unfortunate, as its amelioration could entail a large health gain across somatic and mental domains. If nationwide restrictions on social relationships can lead to more loneliness and a higher prevalence of depression and anxiety in the population, public health policies aimed at nurturing social interactions may achieve the reverse. Some interventions already exist 37 , 38 , but have not yet been widely employed in clinical or public health settings.
Conclusions
In the largest study on loneliness and mental health to date, we found that loneliness is strongly associated with MDD, GAD and the symptoms thereof during the COVID-19 pandemic in the Netherlands. The association between loneliness and symptoms of MDD was stronger in men and in younger adults. Finally, we found the association between loneliness and symptoms of MDD to become stronger over time during the COVID-19 pandemic.
Data availability
All data is available through the Lifelines Cohort Study. Application for data access can be sent to the Lifelines Research Office: https://www.lifelines.nl/researcher/how-to-apply .
Meier, S. M. et al. Increased mortality among people with anxiety disorders: Total population study. Br. J. Psychiatry 209 , 216–221 (2016).
Article Google Scholar
Cuijpers, P. & Schoevers, R. A. Increased mortality in depressive disorders: A review. Curr. Psychiatry Rep. 6 , 430–437 (2004).
Killgore, W. D. S., Cloonan, S. A., Taylor, E. C. & Dailey, N. S. Loneliness: A signature mental health concern in the era of COVID-19. Psychiatry Res. 290 , 113117 (2020).
Article CAS Google Scholar
Cacioppo, J. T. & Hawkley, L. C. Loneliness. (2009).
Cacioppo, J. T., Hughes, M. E., Waite, L. J., Hawkley, L. C. & Thisted, R. A. Loneliness as a specific risk factor for depressive symptoms: Cross-sectional and longitudinal analyses. Psychol. Aging 21 , 140 (2006).
Van Den Brink, R. H. S. et al. Prognostic significance of social network, social support and loneliness for course of major depressive disorder in adulthood and old age. Epidemiol. Psychiatr. Sci. 27 , 266 (2018).
Beutel, M. E. et al. Loneliness in the general population: Prevalence, determinants and relations to mental health. BMC Psychiatry 17 , 97 (2017).
Domènech-Abella, J., Mundó, J., Haro, J. M. & Rubio-Valera, M. Anxiety, depression, loneliness and social network in the elderly: Longitudinal associations from The Irish Longitudinal Study on Ageing (TILDA). J. Affect. Disord. 246 , 82–88 (2019).
Lee, S. L. et al. The association between loneliness and depressive symptoms among adults aged 50 years and older: A 12-year population-based cohort study. Lancet Psychiatry 8 , 48–57 (2021).
Hubbard, G., den Daas, C., Johnston, M. & Dixon, D. Sociodemographic and psychological risk factors for anxiety and depression: Findings from the Covid-19 health and adherence research in Scotland on mental health (CHARIS-MH) cross-sectional survey. Int. J. Behav. Med. 28 , 788–800 (2021).
Distel, M. A. et al. Familial resemblance for loneliness. Behav. Genet. 40 , 480–494 (2010).
Sullivan, P. F., Neale, M. C. & Kendler, K. S. Genetic epidemiology of major depression: Review and meta-analysis. Am. J. Psychiatry 157 , 1552–1562 (2000).
Hettema, J. M., Neale, M. C. & Kendler, K. S. A review and meta-analysis of the genetic epidemiology of anxiety disorders. Am. J. Psychiatry 158 , 1568–1578 (2001).
Day, F. R., Ong, K. K. & Perry, J. R. B. Elucidating the genetic basis of social interaction and isolation. Nat. Commun. 9 , 2457 (2018).
Article ADS Google Scholar
Howard, D. M. et al. Genome-wide meta-analysis of depression identifies 102 independent variants and highlights the importance of the prefrontal brain regions. Nat. Neurosci. 22 , 343–352 (2019).
Wray, N. R. et al. Genome-wide association analyses identify 44 risk variants and refine the genetic architecture of major depression. Nat. Genet. 50 , 668–681 (2018).
McGinty, E. E., Presskreischer, R., Han, H. & Barry, C. L. Psychological distress and loneliness reported by US adults in 2018 and April 2020. JAMA 324 , 93–94 (2020).
Bu, F., Steptoe, A. & Fancourt, D. Loneliness during a strict lockdown: Trajectories and predictors during the COVID-19 pandemic in 38,217 United Kingdom adults. Soc. Sci. Med. 265 , 113521 (2020).
Robb, C. E. et al. Associations of social isolation with anxiety and depression during the early COVID-19 pandemic: A survey of older adults in London. UK. Front. Psychiatry 11 , 991 (2020).
Google Scholar
McQuaid, R. J., Cox, S. M. L., Ogunlana, A. & Jaworska, N. The burden of loneliness: Implications of the social determinants of health during COVID-19. Psychiatry Res. 296 , 113648 (2021).
McIntyre, K. et al. Lifelines COVID-19 cohort: Investigating COVID-19 infection and its health and societal impacts in a Dutch population-based cohort. BMJ Open 11 , e044474 (2021).
Scholtens, S. et al. Cohort Profile: LifeLines, a three-generation cohort study and biobank. Int. J. Epidemiol. 44 , 1172–1180 (2015).
Klijs, B. et al. Representativeness of the LifeLines cohort study. PLoS ONE 10 , e0137203 (2015).
Mc Intyre, K. et al. Lifelines COVID-19 cohort: Investigating COVID-19 infection and its health and societal impacts in a Dutch population-based cohort. BMJ Open 11 , e044474 (2021).
Sheehan, D. V. et al. The Mini-International Neuropsychiatric Interview (MINI): The development and validation of a structured diagnostic psychiatric interview for DSM-IV and ICD-10. J. Clin. Psychiatry 59 , 22–33 (1998).
PubMed Google Scholar
Hughes, M. E., Waite, L. J., Hawkley, L. C. & Cacioppo, J. T. A short scale for measuring loneliness in large surveys: Results from two population-based studies. Res. Aging 26 , 655–672 (2004).
Azur, M. J., Stuart, E. A., Frangakis, C. & Leaf, P. J. Multiple imputation by chained equations: What is it and how does it work?. Int. J. Methods Psychiatr. Res. 20 , 40–49 (2011).
Pekár, S. & Brabec, M. Generalized estimating equations: A pragmatic and flexible approach to the marginal GLM modelling of correlated data in the behavioural sciences. Ethology 124 , 86–93 (2018).
Højsgaard, S., Halekoh, U. & Yan, J. The R package geepack for generalized estimating equations. J. Stat. Softw. 15 , 1–11 (2005).
R Core Team. R: A Language and Environment for Statistical Computing. (2019).
Nunan, D., Aronson, J. & Bankhead, C. Catalogue of bias: Attrition bias. BMJ Evid.-Based Med. 23 , 21–22 (2018).
Wang, J., Mann, F., Lloyd-Evans, B., Ma, R. & Johnson, S. Associations between loneliness and perceived social support and outcomes of mental health problems: A systematic review. BMC Psychiatry 18 , 1–16 (2018).
Levey, D. F. et al. Reproducible genetic risk loci for anxiety: Results from ∼ 200,000 participants in the Million Veteran Program. Am. J. Psychiatry 177 , 223–232 (2020).
Pedersen, A. B. et al. Missing data and multiple imputation in clinical epidemiological research. Clin. Epidemiol. 9 , 157 (2017).
Hedeker, D., du Toit, S. H. C., Demirtas, H. & Gibbons, R. D. A note on marginalization of regression parameters from mixed models of binary outcomes. Biometrics 74 , 354–361 (2018).
Article MathSciNet Google Scholar
Holt-Lunstad, J., Smith, T. B., Baker, M., Harris, T. & Stephenson, D. Loneliness and social isolation as risk factors for mortality: A meta-analytic review. Perspect. Psychol. Sci. 10 , 227–237 (2015).
Masi, C. M., Chen, H.-Y., Hawkley, L. C. & Cacioppo, J. T. A meta-analysis of interventions to reduce loneliness. Pers. Soc. Psychol. Rev. 15 , 219–266 (2011).
Kahlon, M. K. et al. Effect of layperson-delivered, empathy-focused program of telephone calls on loneliness, depression, and anxiety among adults during the COVID-19 pandemic: A randomized clinical trial. JAMA Psychiat. 78 , 616–622 (2021).
Rotenberg, K. J. & MacKie, J. Stigmatization of social and intimacy loneliness. Psychol. Rep. 84 , 147–148 (1999).
Download references
Acknowledgements
We acknowledge funding for the Lifelines Corona Research project from the University of Groningen and the University Medical Centre Groningen. The authors wish to acknowledge the efforts of the Lifelines Corona Research Initiative and the following initiative participants:
H. M. Boezen 1 , Jochen O. Mierau 2,3 , Lude H. Franke 4 , Jackie Dekens 4,6 , Patrick Deelen 4 , Pauline Lanting 4 , Judith M. Vonk 1 , Ilja Nolte 1 , Anil P.S. Ori 4,5 , Annique Claringbould 4 , Floranne Boulogne 4 , Marjolein X.L. Dijkema 4 , Henry H. Wiersma 4 , Robert Warmerdam 4 , Soesma A. Jankipersadsing 4 , Irene van Blokland 4,7 .
1 Department of Epidemiology, University of Groningen, University Medical Center Groningen, Groningen, The Netherlands. 2 Faculty of Economics and Business, University of Groningen, Groningen, The Netherlands. 3 Aletta Jacobs School of Public Health, Groningen, The Netherlands. 4 Department of Genetics, University of Groningen, University Medical Center Groningen, Groningen, The Netherlands. 5 Department of Psychiatry, University of Groningen, University Medical Center Groningen, Groningen, The Netherlands. 6 Center of Development and Innovation, University of Groningen, University Medical Center Groningen, Groningen, The Netherlands. 7 Department of Cardiology, University of Groningen, University Medical Center Groningen, Groningen, The Netherlands.
The generation and management of GWAS genotype data for the Lifelines Cohort Study is supported by the UMCG Genetics Lifelines Initiative (UGLI). The authors wish to acknowledge the services of the Lifelines Cohort Study, the contributing research centres delivering data to Lifelines:
Raul Aguirre-Gamboa 1 , Patrick Deelen 1 , Lude Franke 1 , Jan A. Kuivenhoven 2 , Esteban A. Lopera Maya 1 , Ilja M Nolte 3 , Serena Sanna 1 , Harold Snieder 3 , Morris A. Swertz 1 , Judith M. Vonk 3 , Cisca Wijmenga 1 .
1 Department of Genetics, University of Groningen, University Medical Center Groningen, The Netherlands. 2 Department of Paediatrics, University of Groningen, University Medical Center Groningen, The Netherlands. 3 Department of Epidemiology, University of Groningen, University Medical Center Groningen, The Netherlands
As well as all the study participants.
We thank the UMCG Genomics Coordination Center, the UG Center for Information Technology and their sponsors BBMRI-NL & TarGet for storage and computing infrastructure.
We thank Lian Beijers, Aranka Ballering, Judith Rosmalen and Nord van den Bos for their collaboration and feedback as part of our COVID Psychiatry working group.
The Lifelines Biobank initiative has been made possible by funding from the Dutch Ministry of Health, Welfare and Sport, the Dutch Ministry of Economic Affairs, the University Medical Center Groningen (UMCG the Netherlands), the University of Groningen, the Northern Provinces of the Netherlands, FES (Fonds Economische Structuurversterking), SNN (Samenwerkingsverband Noord Nederland) and REP (Ruimtelijk Economisch Programma). Hanna M. van Loo was supported by a NARSAD Young Investigator Grant from the Brain & Behavior Research Foundation and a VENI grant from the Talent Programme of the Netherlands Organization of Scientific Research (NWO-ZonMW 09150161810021).
Author information
Authors and affiliations.
Department of Psychiatry, University Medical Center Groningen, University of Groningen, Hanzeplein 1, PO Box 30.001, 9700 RB, Groningen, The Netherlands
Olivier D. Steen, Anil P. S. Ori, Klaas J. Wardenaar & Hanna M. van Loo
Department of Genetics, University Medical Center Groningen, University of Groningen, Groningen, The Netherlands
Anil P. S. Ori
You can also search for this author in PubMed Google Scholar
Contributions
O.D.S., A.P.S.O., H.M.v.L. designed the study. A.P.S.O. and H.M.v.L. were involved in data collection. O.D.S. performed the statistical analyses and primary drafting of the manuscript with critical input from A.P.S.O., K.J.W., and H.M.v.L. A.P.S.O. and H.M.v.L. oversaw the study. All authors contributed to and approved the final manuscript.
Corresponding author
Correspondence to Hanna M. van Loo .
Ethics declarations
Competing interests.
The authors declare no competing interests.
Additional information
Publisher's note.
Springer Nature remains neutral with regard to jurisdictional claims in published maps and institutional affiliations.
Supplementary Information
Supplementary information., rights and permissions.
Open Access This article is licensed under a Creative Commons Attribution 4.0 International License, which permits use, sharing, adaptation, distribution and reproduction in any medium or format, as long as you give appropriate credit to the original author(s) and the source, provide a link to the Creative Commons licence, and indicate if changes were made. The images or other third party material in this article are included in the article's Creative Commons licence, unless indicated otherwise in a credit line to the material. If material is not included in the article's Creative Commons licence and your intended use is not permitted by statutory regulation or exceeds the permitted use, you will need to obtain permission directly from the copyright holder. To view a copy of this licence, visit http://creativecommons.org/licenses/by/4.0/ .
Reprints and permissions
About this article
Cite this article.
Steen, O.D., Ori, A.P.S., Wardenaar, K.J. et al. Loneliness associates strongly with anxiety and depression during the COVID pandemic, especially in men and younger adults. Sci Rep 12 , 9517 (2022). https://doi.org/10.1038/s41598-022-13049-9
Download citation
Received : 09 November 2021
Accepted : 09 May 2022
Published : 09 June 2022
DOI : https://doi.org/10.1038/s41598-022-13049-9
Share this article
Anyone you share the following link with will be able to read this content:
Sorry, a shareable link is not currently available for this article.
Provided by the Springer Nature SharedIt content-sharing initiative
This article is cited by
Loneliness, belonging and psychosomatic complaints across late adolescence and young adulthood: a swedish cohort study.
- Karina Grigorian
- Viveca Östberg
- Sara Brolin Låftman
BMC Public Health (2024)
The Association Between Emotion Recognition and Internalizing Problems in Children and Adolescents: A Three-Level Meta-Analysis
- Heting Liang
Journal of Youth and Adolescence (2024)
Het beloop van suïcidaliteit tijdens de coronapandemie in Nederland
- Lizanne J. S. Schweren
- Marjolein Veerbeek
- Renske Gilissen
TSG - Tijdschrift voor gezondheidswetenschappen (2023)
By submitting a comment you agree to abide by our Terms and Community Guidelines . If you find something abusive or that does not comply with our terms or guidelines please flag it as inappropriate.
Quick links
- Explore articles by subject
- Guide to authors
- Editorial policies
Sign up for the Nature Briefing newsletter — what matters in science, free to your inbox daily.

Depression and anxiety
Affiliation.
- 1 University of Melbourne, Melbourne, VIC, Australia. [email protected].
- PMID: 25370281
- DOI: 10.5694/mja12.10628
Comorbid depression and anxiety disorders occur in up to 25% of general practice patients. About 85% of patients with depression have significant anxiety, and 90% of patients with anxiety disorder have depression. Symptomatology may initially seem vague and non-specific. A careful history and examination with relevant investigations should be used to make the diagnosis. Once the diagnosis is made, rating scales may identify illness severity and help in monitoring treatment progress. Both the depression disorder and the specific anxiety disorder require appropriate treatment. Psychological therapies, such as cognitive behaviour therapy, and antidepressants, occasionally augmented with antipsychotics, have proven benefit for treating both depression and anxiety. Benzodiazepines may help alleviate insomnia and anxiety but not depression. They have dependency and withdrawal issues for some people, and may increase the risk of falls in older people. Despite the availability of treatments, 40% of patients with depression or anxiety do not seek treatment, and of those who do, less than half are offered beneficial treatment.
- Anxiety / drug therapy
- Anxiety / epidemiology*
- Anxiety / therapy
- Benzodiazepines / adverse effects
- Benzodiazepines / therapeutic use
- Cognitive Behavioral Therapy
- Comorbidity
- Depression / drug therapy
- Depression / epidemiology*
- Depression / therapy
- Depressive Disorder / psychology
- Depressive Disorder / therapy
- Benzodiazepines
- U.S. Department of Health & Human Services

- Virtual Tour
- Staff Directory
- En Español
You are here
Nih research matters.
April 23, 2024
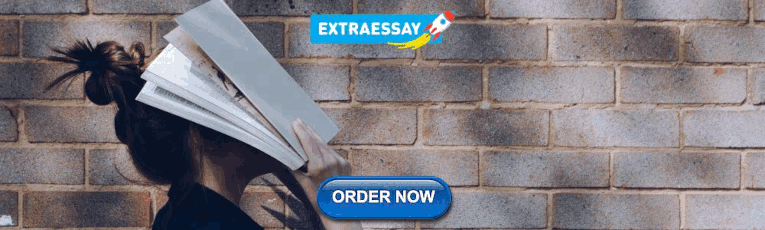
Research in Context: Treating depression
Finding better approaches.
While effective treatments for major depression are available, there is still room for improvement. This special Research in Context feature explores the development of more effective ways to treat depression, including personalized treatment approaches and both old and new drugs.

Everyone has a bad day sometimes. People experience various types of stress in the course of everyday life. These stressors can cause sadness, anxiety, hopelessness, frustration, or guilt. You may not enjoy the activities you usually do. These feelings tend to be only temporary. Once circumstances change, and the source of stress goes away, your mood usually improves. But sometimes, these feelings don’t go away. When these feelings stick around for at least two weeks and interfere with your daily activities, it’s called major depression, or clinical depression.
In 2021, 8.3% of U.S. adults experienced major depression. That’s about 21 million people. Among adolescents, the prevalence was much greater—more than 20%. Major depression can bring decreased energy, difficulty thinking straight, sleep problems, loss of appetite, and even physical pain. People with major depression may become unable to meet their responsibilities at work or home. Depression can also lead people to use alcohol or drugs or engage in high-risk activities. In the most extreme cases, depression can drive people to self-harm or even suicide.
The good news is that effective treatments are available. But current treatments have limitations. That’s why NIH-funded researchers have been working to develop more effective ways to treat depression. These include finding ways to predict whether certain treatments will help a given patient. They're also trying to develop more effective drugs or, in some cases, find new uses for existing drugs.
Finding the right treatments
The most common treatments for depression include psychotherapy, medications, or a combination. Mild depression may be treated with psychotherapy. Moderate to severe depression often requires the addition of medication.
Several types of psychotherapy have been shown to help relieve depression symptoms. For example, cognitive behavioral therapy helps people to recognize harmful ways of thinking and teaches them how to change these. Some researchers are working to develop new therapies to enhance people’s positive emotions. But good psychotherapy can be hard to access due to the cost, scheduling difficulties, or lack of available providers. The recent growth of telehealth services for mental health has improved access in some cases.
There are many antidepressant drugs on the market. Different drugs will work best on different patients. But it can be challenging to predict which drugs will work for a given patient. And it can take anywhere from 6 to 12 weeks to know whether a drug is working. Finding an effective drug can involve a long period of trial and error, with no guarantee of results.
If depression doesn’t improve with psychotherapy or medications, brain stimulation therapies could be used. Electroconvulsive therapy, or ECT, uses electrodes to send electric current into the brain. A newer technique, transcranial magnetic stimulation (TMS), stimulates the brain using magnetic fields. These treatments must be administered by specially trained health professionals.
“A lot of patients, they kind of muddle along, treatment after treatment, with little idea whether something’s going to work,” says psychiatric researcher Dr. Amit Etkin.
One reason it’s difficult to know which antidepressant medications will work is that there are likely different biological mechanisms that can cause depression. Two people with similar symptoms may both be diagnosed with depression, but the causes of their symptoms could be different. As NIH depression researcher Dr. Carlos Zarate explains, “we believe that there’s not one depression, but hundreds of depressions.”
Depression may be due to many factors. Genetics can put certain people at risk for depression. Stressful situations, physical health conditions, and medications may contribute. And depression can also be part of a more complicated mental disorder, such as bipolar disorder. All of these can affect which treatment would be best to use.
Etkin has been developing methods to distinguish patients with different types of depression based on measurable biological features, or biomarkers. The idea is that different types of patients would respond differently to various treatments. Etkin calls this approach “precision psychiatry.”
One such type of biomarker is electrical activity in the brain. A technique called electroencephalography, or EEG, measures electrical activity using electrodes placed on the scalp. When Etkin was at Stanford University, he led a research team that developed a machine-learning algorithm to predict treatment response based on EEG signals. The team applied the algorithm to data from a clinical trial of the antidepressant sertraline (Zoloft) involving more than 300 people.

EEG data for the participants were collected at the outset. Participants were then randomly assigned to take either sertraline or an inactive placebo for eight weeks. The team found a specific set of signals that predicted the participants’ responses to sertraline. The same neural “signature” also predicted which patients with depression responded to medication in a separate group.
Etkin’s team also examined this neural signature in a set of patients who were treated with TMS and psychotherapy. People who were predicted to respond less to sertraline had a greater response to the TMS/psychotherapy combination.
Etkin continues to develop methods for personalized depression treatment through his company, Alto Neuroscience. He notes that EEG has the advantage of being low-cost and accessible; data can even be collected in a patient’s home. That’s important for being able to get personalized treatments to the large number of people they could help. He’s also working on developing antidepressant drugs targeted to specific EEG profiles. Candidate drugs are in clinical trials now.
“It’s not like a pie-in-the-sky future thing, 20-30 years from now,” Etkin explains. “This is something that could be in people's hands within the next five years.”
New tricks for old drugs
While some researchers focus on matching patients with their optimal treatments, others aim to find treatments that can work for many different patients. It turns out that some drugs we’ve known about for decades might be very effective antidepressants, but we didn’t recognize their antidepressant properties until recently.
One such drug is ketamine. Ketamine has been used as an anesthetic for more than 50 years. Around the turn of this century, researchers started to discover its potential as an antidepressant. Zarate and others have found that, unlike traditional antidepressants that can take weeks to take effect, ketamine can improve depression in as little as one day. And a single dose can have an effect for a week or more. In 2019, the FDA approved a form of ketamine for treating depression that is resistant to other treatments.
But ketamine has drawbacks of its own. It’s a dissociative drug, meaning that it can make people feel disconnected from their body and environment. It also has the potential for addiction and misuse. For these reasons, it’s a controlled substance and can only be administered in a doctor’s office or clinic.
Another class of drugs being studied as possible antidepressants are psychedelics. These include lysergic acid diethylamide (LSD) and psilocybin, the active ingredient in magic mushrooms. These drugs can temporarily alter a person’s mood, thoughts, and perceptions of reality. Some have historically been used for religious rituals, but they are also used recreationally.
In clinical studies, psychedelics are typically administered in combination with psychotherapy. This includes several preparatory sessions with a therapist in the weeks before getting the drug, and several sessions in the weeks following to help people process their experiences. The drugs are administered in a controlled setting.
Dr. Stephen Ross, co-director of the New York University Langone Health Center for Psychedelic Medicine, describes a typical session: “It takes place in a living room-like setting. The person is prepared, and they state their intention. They take the drug, they lie supine, they put on eye shades and preselected music, and two therapists monitor them.” Sessions last for as long as the acute effects of the drug last, which is typically several hours. This is a healthcare-intensive intervention given the time and personnel needed.
In 2016, Ross led a clinical trial examining whether psilocybin-assisted therapy could reduce depression and anxiety in people with cancer. According to Ross, as many as 40% of people with cancer have clinically significant anxiety and depression. The study showed that a single psilocybin session led to substantial reductions in anxiety and depression compared with a placebo. These reductions were evident as soon as one day after psilocybin administration. Six months later, 60-80% of participants still had reduced depression and anxiety.
Psychedelic drugs frequently trigger mystical experiences in the people who take them. “People can feel a sense…that their consciousness is part of a greater consciousness or that all energy is one,” Ross explains. “People can have an experience that for them feels more ‘real’ than regular reality. They can feel transported to a different dimension of reality.”
About three out of four participants in Ross’s study said it was among the most meaningful experiences of their lives. And the degree of mystical experience correlated with the drug’s therapeutic effect. A long-term follow-up study found that the effects of the treatment continued more than four years later.
If these results seem too good to be true, Ross is quick to point out that it was a small study, with only 29 participants, although similar studies from other groups have yielded similar results. Psychedelics haven’t yet been shown to be effective in a large, controlled clinical trial. Ross is now conducting a trial with 200 people to see if the results of his earlier study pan out in this larger group. For now, though, psychedelics remain experimental drugs—approved for testing, but not for routine medical use.
Unlike ketamine, psychedelics aren’t considered addictive. But they, too, carry risks, which certain conditions may increase. Psychedelics can cause cardiovascular complications. They can cause psychosis in people who are predisposed to it. In uncontrolled settings, they have the risk of causing anxiety, confusion, and paranoia—a so-called “bad trip”—that can lead the person taking the drug to harm themself or others. This is why psychedelic-assisted therapy takes place in such tightly controlled settings. That increases the cost and complexity of the therapy, which may prevent many people from having access to it.
Better, safer drugs
Despite the promise of ketamine or psychedelics, their drawbacks have led some researchers to look for drugs that work like them but with fewer side effects.
Depression is thought to be caused by the loss of connections between nerve cells, or neurons, in certain regions of the brain. Ketamine and psychedelics both promote the brain’s ability to repair these connections, a quality called plasticity. If we could understand how these drugs encourage plasticity, we might be able to design drugs that can do so without the side effects.

Dr. David Olson at the University of California, Davis studies how psychedelics work at the cellular and molecular levels. The drugs appear to promote plasticity by binding to a receptor in cells called the 5-hydroxytryptamine 2A receptor (5-HT2AR). But many other compounds also bind 5-HT2AR without promoting plasticity. In a recent NIH-funded study, Olson showed that 5-HT2AR can be found both inside and on the surface of the cell. Only compounds that bound to the receptor inside the cells promoted plasticity. This suggests that a drug has to be able to get into the cell to promote plasticity.
Moreover, not all drugs that bind 5-HT2AR have psychedelic effects. Olson’s team has developed a molecular sensor, called psychLight, that can identify which compounds that bind 5-HT2AR have psychedelic effects. Using psychLight, they identified compounds that are not psychedelic but still have rapid and long-lasting antidepressant effects in animal models. He’s founded a company, Delix Therapeutics, to further develop drugs that promote plasticity.
Meanwhile, Zarate and his colleagues have been investigating a compound related to ketamine called hydroxynorketamine (HNK). Ketamine is converted to HNK in the body, and this process appears to be required for ketamine’s antidepressant effects. Administering HNK directly produced antidepressant-like effects in mice. At the same time, it did not cause the dissociative side effects and addiction caused by ketamine. Zarate’s team has already completed phase I trials of HNK in people showing that it’s safe. Phase II trials to find out whether it’s effective are scheduled to begin soon.
“What [ketamine and psychedelics] are doing for the field is they’re helping us realize that it is possible to move toward a repair model versus a symptom mitigation model,” Olson says. Unlike existing antidepressants, which just relieve the symptoms of depression, these drugs appear to fix the underlying causes. That’s likely why they work faster and produce longer-lasting effects. This research is bringing us closer to having safer antidepressants that only need to be taken once in a while, instead of every day.
—by Brian Doctrow, Ph.D.
Related Links
- How Psychedelic Drugs May Help with Depression
- Biosensor Advances Drug Discovery
- Neural Signature Predicts Antidepressant Response
- How Ketamine Relieves Symptoms of Depression
- Protein Structure Reveals How LSD Affects the Brain
- Predicting The Usefulness of Antidepressants
- Depression Screening and Treatment in Adults
- Serotonin Transporter Structure Revealed
- Placebo Effect in Depression Treatment
- When Sadness Lingers: Understanding and Treating Depression
- Psychedelic and Dissociative Drugs
References: An electroencephalographic signature predicts antidepressant response in major depression. Wu W, Zhang Y, Jiang J, Lucas MV, Fonzo GA, Rolle CE, Cooper C, Chin-Fatt C, Krepel N, Cornelssen CA, Wright R, Toll RT, Trivedi HM, Monuszko K, Caudle TL, Sarhadi K, Jha MK, Trombello JM, Deckersbach T, Adams P, McGrath PJ, Weissman MM, Fava M, Pizzagalli DA, Arns M, Trivedi MH, Etkin A. Nat Biotechnol. 2020 Feb 10. doi: 10.1038/s41587-019-0397-3. Epub 2020 Feb 10. PMID: 32042166. Rapid and sustained symptom reduction following psilocybin treatment for anxiety and depression in patients with life-threatening cancer: a randomized controlled trial. Ross S, Bossis A, Guss J, Agin-Liebes G, Malone T, Cohen B, Mennenga SE, Belser A, Kalliontzi K, Babb J, Su Z, Corby P, Schmidt BL. J Psychopharmacol . 2016 Dec;30(12):1165-1180. doi: 10.1177/0269881116675512. PMID: 27909164. Long-term follow-up of psilocybin-assisted psychotherapy for psychiatric and existential distress in patients with life-threatening cancer. Agin-Liebes GI, Malone T, Yalch MM, Mennenga SE, Ponté KL, Guss J, Bossis AP, Grigsby J, Fischer S, Ross S. J Psychopharmacol . 2020 Feb;34(2):155-166. doi: 10.1177/0269881119897615. Epub 2020 Jan 9. PMID: 31916890. Psychedelics promote neuroplasticity through the activation of intracellular 5-HT2A receptors. Vargas MV, Dunlap LE, Dong C, Carter SJ, Tombari RJ, Jami SA, Cameron LP, Patel SD, Hennessey JJ, Saeger HN, McCorvy JD, Gray JA, Tian L, Olson DE. Science . 2023 Feb 17;379(6633):700-706. doi: 10.1126/science.adf0435. Epub 2023 Feb 16. PMID: 36795823. Psychedelic-inspired drug discovery using an engineered biosensor. Dong C, Ly C, Dunlap LE, Vargas MV, Sun J, Hwang IW, Azinfar A, Oh WC, Wetsel WC, Olson DE, Tian L. Cell . 2021 Apr 8: S0092-8674(21)00374-3. doi: 10.1016/j.cell.2021.03.043. Epub 2021 Apr 28. PMID: 33915107. NMDAR inhibition-independent antidepressant actions of ketamine metabolites. Zanos P, Moaddel R, Morris PJ, Georgiou P, Fischell J, Elmer GI, Alkondon M, Yuan P, Pribut HJ, Singh NS, Dossou KS, Fang Y, Huang XP, Mayo CL, Wainer IW, Albuquerque EX, Thompson SM, Thomas CJ, Zarate CA Jr, Gould TD. Nature . 2016 May 26;533(7604):481-6. doi: 10.1038/nature17998. Epub 2016 May 4. PMID: 27144355.
Connect with Us
- More Social Media from NIH
This paper is in the following e-collection/theme issue:
Published on 25.4.2024 in Vol 26 (2024)
Digital Therapeutic (Mika) Targeting Distress in Patients With Cancer: Results From a Nationwide Waitlist Randomized Controlled Trial
Authors of this article:

Original Paper
- Franziska Springer 1 * , MSc ;
- Ayline Maier 2 * , PhD ;
- Michael Friedrich 1 , PhD ;
- Jan Simon Raue 2 , PhD ;
- Gandolf Finke 2 , PhD ;
- Florian Lordick 3, 4 , Prof Dr ;
- Guy Montgomery 5 , Prof Dr ;
- Peter Esser 1 , PhD ;
- Hannah Brock 1 , MSc ;
- Anja Mehnert-Theuerkauf 1 , Prof Dr
1 Department of Medical Psychology and Medical Sociology, Comprehensive Cancer Center Central Germany, University Medical Center Leipzig, Leipzig, Germany
2 Fosanis GmbH, Berlin, Germany
3 Department of Medicine II, University Medical Center Leipzig, Leipzig, Germany
4 University Cancer Center Leipzig, Comprehensive Cancer Center Central Germany, Leipzig, Germany
5 Center for Behavioral Oncology, Department of Population Health Science and Policy, Icahn School of Medicine at Mount Sinai, New York, NY, United States
*these authors contributed equally
Corresponding Author:
Anja Mehnert-Theuerkauf, Prof Dr
Department of Medical Psychology and Medical Sociology, Comprehensive Cancer Center Central Germany, University Medical Center Leipzig
Philipp-Rosenthal-Str. 55, Haus W
Leipzig, 04103
Phone: 49 341 97 18800
Email: [email protected]
Background: Distress is highly prevalent among patients with cancer, but supportive care needs often go unmet. Digital therapeutics hold the potential to overcome barriers in cancer care and improve health outcomes.
Objective: This study conducted a randomized controlled trial to investigate the efficacy of Mika, an app-based digital therapeutic designed to reduce distress across the cancer trajectory.
Methods: This nationwide waitlist randomized controlled trial in Germany enrolled patients with cancer across all tumor entities diagnosed within the last 5 years. Participants were randomized into the intervention (Mika plus usual care) and control (usual care alone) groups. The participants completed web-based assessments at baseline and at 2, 6, and 12 weeks. The primary outcome was the change in distress from baseline to week 12, as measured by the National Comprehensive Cancer Network Distress Thermometer. Secondary outcomes included depression, anxiety (Hospital Anxiety and Depression Scale), fatigue (Functional Assessment of Chronic Illness Therapy-Fatigue), and quality of life (Clinical Global Impression-Improvement Scale). Intention-to-treat and per-protocol analyses were performed. Analyses of covariance were used to test for outcome changes over time between the groups, controlling for baseline.
Results: A total of 218 patients (intervention: n=99 and control: n=119) were included in the intention-to-treat analysis. Compared with the control group, the intervention group reported greater reductions in distress ( P =.03; ηp²=0.02), depression ( P< .001; ηp²=0.07), anxiety ( P= .03; ηp²=0.02), and fatigue ( P= .04; ηp²=0.02). Per-protocol analyses revealed more pronounced treatment effects, with the exception of fatigue. No group difference was found for quality of life.
Conclusions: Mika effectively diminished distress in patients with cancer. As a digital therapeutic solution, Mika offers accessible, tailored psychosocial and self-management support to address the unmet needs in cancer care.
Trial Registration: German Clinical Trials Register (DRKS) DRKS00026038; https://drks.de/search/en/trial/DRKS00026038
Introduction
In addition to somatic symptoms such as pain [ 1 ], patients with cancer report elevated levels of distress, anxiety, and depression [ 2 , 3 ]. Epidemiological data show that the prevalence of clinically substantial psychological distress typically ranges from 30% to 60% among patients with cancer [ 2 , 4 ]. Psychological distress can persist long after the end of treatment and is associated with reduced quality of life (QoL), lower cancer treatment adherence, and lower survival rates [ 5 ].
Supportive care interventions to prevent and manage the adverse psychological and physical effects of cancer across the cancer trajectory effectively improve outcomes such as emotional distress, QoL, and fatigue [ 6 ]. Optimal supportive care is holistic and patient centered, that is, based on the needs of each individual patient [ 7 ]. However, access to supportive care is often limited by a lack of specialist staff, organizational deficiencies, and barriers that cause patients to avoid or delay their treatment [ 8 - 10 ]. Thus, emerging or persistent supportive care needs across the cancer trajectory often go unmet, with detrimental psychosocial and emotional impacts on patients with cancer [ 11 ]. Moreover, the number of patients living with cancer has increased rapidly in recent years [ 12 ] due to improved early detection, diagnosis, and oncological treatments, posing a growing challenge to health systems worldwide to ensure adequate and long-term care for all patients with cancer [ 13 ].
The increasing use of digital health has ushered in a new era of patient-centered cancer care due to its potential for cancer care delivery [ 14 ]. Digital health interventions provide multiple benefits: they facilitate easy and low-threshold access to care, can overcome barriers to care (eg, location, time, and health status), may enhance symptom management through real-time symptom assessment, are scalable, and provide cost-effective and efficient information sharing [ 14 ]. Growing literature suggests that digital therapeutics, a subset of digital health interventions providing evidence-based treatments driven by software, play a useful role in addressing the unmet needs of patients with cancer [ 15 ]. For instance, various mobile apps have proven to be effective in catering to specific needs of patients with cancer, such as pain, anxiety, or QoL, by using different types of interventions, such as psychoeducation, physical exercises, or coping skills training (eg, [ 16 - 19 ]). Moreover, large analyses such as systematic reviews and meta-analyses evaluating the efficacy of app-based interventions for patients with cancer show positive effects on patient-relevant outcomes, such as distress, QoL, anxiety, depression, pain, and fatigue [ 20 - 23 ].
Existing app-based supportive care interventions provide various intervention modules, such as symptom monitoring, psychoeducation, mindfulness exercises, physical exercises, and cognitive behavioral therapy (CBT) techniques [ 24 ]. However, most of these apps are limited in their scope, targeting only specific symptoms (eg, fatigue) [ 25 ] and health behaviors (eg, physical activity) [ 26 ], or provide only a single function (eg, mindfulness training or symptom tracking) [ 27 - 29 ]. Furthermore, some of these apps were originally developed for non–oncology patient populations and have only been slightly adapted for patients with cancer [ 30 ]. Only a few apps offer a broader range of intervention modules [ 25 , 31 ], but they target specific subgroups of patients with cancer (eg, patients with 1 tumor entity or with specific symptoms).
Despite the evident need, there is yet no digital therapeutic that comprehensively addresses the problems faced by all patients with cancer and simultaneously offers tailored support for each individual patient. Therefore, we investigated the efficacy of Mika (developed by Fosanis GmbH), an app-based digital therapeutic that addresses all patients with cancer transdiagnostically and provides a holistic supportive care intervention. The app incorporates evidence-based supportive care elements, such as distress and symptom monitoring [ 32 ], CBT-based coping skills training [ 33 ], mindfulness-based stress reduction (MBSR) [ 34 , 35 ], strength and flexibility training [ 36 ], and patient education [ 37 ], thus targeting different aspects of psychological distress. An artificial intelligence algorithm individually tailors the content of the app to patients’ needs, considering cancer type, cancer treatment stage, and use behavior. A previously conducted pilot study of 70 patients with gynecological cancer indicated Mika’s feasibility and potential efficacy [ 38 ]. Considering the significant prevalence and impact of psychological distress among patients with cancer, this condition was selected as the primary end point of our study. This is underscored by the app’s integrated features for distress tracking and management alongside the widespread recommendation for distress screening in routine clinical care. Distress is recognized as a crucial clinical marker for assessing the efficacy of interventions across various tumor types and catering to the immediate and long-term supportive care needs of this patient group.
The primary aim of this waitlist randomized controlled trial (RCT) was to examine the efficacy of the Mika app for general distress in patients with cancer. The secondary aim was to assess the efficacy of the Mika app on anxiety, depression, fatigue, and QoL. We hypothesized that participants receiving access to the Mika app plus usual care (UC) for 12 weeks would report greater reductions in distress, anxiety, depression, and fatigue and greater improvements in QoL compared to participants receiving UC only.
Study Design
This nationwide unblinded 2-arm waitlist RCT evaluated the efficacy of the app-based digital therapeutic Mika in reducing distress in patients with cancer and was conducted fully decentralized in Germany, that is, participant recruitment, delivery of the study intervention, and outcome data collection were conducted without involving in-person contact between the study team and the participants. In this RCT, participants were assigned to either (1) access to the Mika app plus UC (intervention group [IG]), or (2) UC alone (control group [CG]). Participants were assessed at baseline (t0), 2 weeks (t1), 6 weeks (t2), and 12 weeks (t3) using self-report questionnaires. Once the participants in the CG completed the 12-week questionnaire, they also received access to the Mika app.
Ethical Considerations
The trial was approved by the Ethics Committee of the Medical Faculty of Leipzig University (404/21-ek) and was registered at the German Clinical Trials Register (DRKS00026038) in October 2021. All participants provided written informed consent prior to their participation in the study and retained the autonomy to withdraw from the study at any time. All personal data collected and used for this study underwent deidentification to safeguard the anonymity of participants. Monetary compensation was not provided to participants for their involvement in the study.
Participants
Textbox 1 shows the inclusion and exclusion criteria for this study. We only included patients who had been diagnosed with cancer or relapse within the last 5 years as they are likely to feel burdened by the physical and psychological effects of the disease and its treatment and therefore require supportive care. Epidemiological data indicate that supportive care needs typically decline in the years of long-term survivorship (cancer or relapse diagnosis ≥5 years ago) [ 39 ]. Participants were required to confirm their cancer diagnosis during the course of the study by submitting a letter from their treating physician. The study team enrolled patients after they had provided written informed consent, which had to be completed at home and submitted by email or mail.
Inclusion criteria
- Age≥18 years
- Cancer diagnosis or relapse diagnosis within the last 5 years (10th revision of the International Statistical Classification of Diseases and Related Health Problems: C00-C97)
- Access to a smartphone or tablet
- Ability to provide informed consent
Exclusion criteria
- Insufficient German language skills
- Inability to use a smartphone or tablet
- Prior use of the investigated digital therapeutic
Random Assignment
Participants were randomly assigned (1:1) to either the IG or CG using permuted block randomization with blocks of 4 based on an a priori created randomization list. The allocation sequence was concealed from the study investigators until assignment. Due to the nature of the intervention, it was not feasible to blind participants or the study team to the group assignment.
Recruitment and Procedure
Between September and November 2021, patients were recruited via social media advertising campaigns (Facebook and Instagram, Meta Inc) and informational emails to cancer support groups that directed patients to the trial website with a contact form for study registration. In addition, patients were recruited from a participant pool consisting of participants from previous independent studies at the University Medical Center Leipzig. Patients from the participant pool were approached directly by the study team via phone.
All interested patients were screened by phone to determine eligibility. To identify patients who were already users of the digital therapeutic, the study team asked participants about their use of digital support, however, without referring to the publicly available digital therapeutic by name to prevent CG patients from accessing the digital therapeutic before their enrollment in the study. Eligible patients received study information in the form of a video and text via email. Patients were informed that they were required to submit a physician’s letter confirming their cancer diagnosis via a secure cloud data-sharing service (TeamDrive, Crunchbase) during the course of their study participation. After providing informed consent, the participants were randomized into the IG or CG and completed the baseline questionnaires. Participants were informed about their group assignment following a completed baseline assessment. IG participants received a study access code to activate the app after downloading it from the app stores for either Android or iOS smartphones, allowing free use. The questionnaire battery was administered electronically using LimeSurvey (LimeSurvey GmbH). All participants received email invitations and reminders at 2, 6, and 12 weeks to complete the questionnaire. This RCT focused on changes in outcomes from baseline (t0) to week 12 (t3). The 2 assessments in between (t1 and t2) were not part of the analysis; an analysis of the trajectory of the symptoms is planned for the future. Once the CG participants completed the 12-week questionnaire, they also received a study access code that could be used to activate the app. All the participants received information about the app’s content and technical application via a standardized telephone introduction to the app. All participants were contacted for an exploratively structured telephone interview after completing the 12-week questionnaire. During this interview, the use of psychotherapeutic support during study participation was assessed. Data collection ended in March 2022.
Data monitoring was performed via standardized phone calls following questionnaire completion of each participant across all measurement time points to ensure data validity. These phone calls served to ask participants to provide missing questionnaire data, to allow participants to clarify difficulties in understanding single questionnaire items, and to provide assistance with limited app functionality. Missing questionnaire data were entered directly into the database by the study team, with a study team member reading the unanswered questions and associated response options to participants verbatim, prompting them to select their response option.
Self-reported adverse reactions and side effects of the investigated digital therapeutic were assessed at each measurement time point as part of the web-based questionnaire battery.
Intervention
Mika is an app-based digital therapeutic that provides a personalized supportive intervention aiming to reduce distress associated with cancer and its medical treatment, thus improving patients’ QoL. Mika comprises 3 modules: Check-Up , Discover, and Journeys . The Check-Up module allows for the monitoring of distress and symptom monitoring with electronic patient-reported outcomes that can be shared and discussed with the attending physician. The Discover module delivers coaching via articles and videos on cancer types and medical treatments, psychological well-being, physical activity, diet, and social and financial issues, which are based on scientific evidence and presented in a clear and understandable manner for patients. The Journeys module provides users with evidence-based, resource-activating training courses combining psychoeducation and exercises to help patients cope with the mental and physical effects of cancer, for example, coping with stress and fatigue, making decisions, or living with immunotherapy (for more details on the app modules, refer to Table 1 and Figure 1 ). An artificial intelligence algorithm within the app customizes the content for each patient. This includes personalized recommendations based on cancer type, cancer treatment stage, and crucially; the nature and severity of reported symptoms; and ensuring personalized support for each individual. This customization process not only accounts for general patient information but also actively incorporates real-time symptom tracking data and user reading behavior using an attentional factorization machine that predicts a patient’s likelihood of engaging with specific content. This approach focuses on important feature interactions related to content consumption [ 40 ], ensuring that recommendations are dynamically adjusted as patients report changes in symptoms and interact with the content. In addition, the algorithm uses a Dirichlet loss function to estimate the uncertainty in predictions [ 41 ], allowing the content to be ranked and presented based on the estimated read probability. The model undergoes monthly updates using historical data, optimizing through hyperparameter tuning evaluated by 7-fold time series cross-validation.
It is hypothesized that the digital therapeutic empowers patients with cancer by improving their health literacy and self-management along the cancer trajectory using evidence-based methods, such as symptom monitoring, patient education, MBSR, strength and flexibility training, acceptance and commitment therapy, and CBT-based coping skills training.
The Mika app was developed by Fosanis GmbH in collaboration with leading research institutions, such as the Charité University Hospital Berlin, University Hospital Leipzig, and the National Center for Tumor Diseases Heidelberg. All content of the app was carefully reviewed by experts (eg, oncologists, psychotherapists, nutritionists, and physiotherapists) before publication. The feasibility and preliminary efficacy of Mika were investigated in a previously conducted randomized pilot study involving 70 patients with gynecological cancer [ 38 ]. Mika is available for download free of charge in German and the United Kingdom app stores for Android and iOS smartphones.
IG participants could freely choose the modules to work on. While regular app use was recommended, participants were instructed to use the app at least 3 times a week.
a PRO: patient-reported outcome.

UC Condition
UC consisted of all health care that patients in Germany usually receive. There were no restrictions on health care use.
Outcome Assessment
Primary outcome.
The primary outcome was the change in psychological distress from baseline to 12 weeks, measured using the validated German version of the National Comprehensive Cancer Network Distress Thermometer [ 42 ]. Distress Thermometer is a well-established single-item self-report measure that assesses the global level of distress on a 0 (no distress) to 10 (extreme distress)-point Likert scale. It shows excellent psychometric properties across various cancer populations worldwide and is recommended as a clinical tool for routine clinical care [ 43 ]. A score ≥5 indicates clinically significant levels of distress.
Secondary Outcomes
The secondary outcomes included changes in anxiety and depression symptoms, fatigue from baseline to 12 weeks, and QoL at 12 weeks. Anxiety and depression symptoms were measured using the Hospital Anxiety and Depression Scale [ 44 ], a 14-item self-report measure of anxiety and depression, with 7 items measuring each subscale. Scores for each subscale range from 0 to 21, with a higher score indicating higher levels of anxiety or depression and a cutoff score of ≥8 for each subscale. Fatigue was assessed using the Functional Assessment of Chronic Illness Therapy-Fatigue [ 45 ], a 13-item measure that assesses self-reported tiredness, weakness, and difficulty in performing usual activities due to fatigue. The Functional Assessment of Chronic Illness Therapy-Fatigue score ranges from 0 to 52, with higher scores representing less fatigue. Self-reported QoL was measured using an adapted version of the Clinical Global Impression-Improvement Scale [ 46 ], a single-item 7-point measure that assesses the overall improvement of a patient’s disease relative to a baseline state at the beginning of the intervention. In this trial, the Clinical Global Impression-Improvement Scale measured improvement in QoL relative to the beginning of the study, with a value of 4 indicating no change, <4 indicating improvement, and >4 indicating deterioration in QoL.
Intervention Safety
The safety of the digital therapeutic was assessed by the number and type of self-reported adverse reactions and side effects during the trial duration.
Intervention Adherence and Engagement
Adherence to the intervention was assessed by tracking app activities. IG participants were considered active once they activated the app using the study access code and consented to the Mika app’s privacy terms. Subsequently, their pseudonymized in-app activities were automatically recorded as log data. These log data facilitated the evaluation of intervention adherence, defined as the number of days with ≥1 app activity during each of the three 4-week periods (0-4, 5-8, and 9-12 weeks) within the 12-week intervention. Such an approach enabled us to capture the frequency and diversity of app engagement, thus embodying a comprehensive definition of adherence. In addition, engagement across the app’s 3 modules—Check-Up, Discover, and Journeys—was analyzed.
Statistical Analysis
Given an estimated dropout rate of 20% (50/250), a priori sample calculations showed that a sample of 2×125 (N=250) at baseline was needed to detect a change of 1 scale point (SD 2; α=.05; 1−β=.8) in the primary outcome.
Primary analyses were performed using the intention-to-treat (ITT) principle, which included all randomized participants with a confirmed cancer diagnosis by a physician’s letter. Analyses were also performed per-protocol (PP), which was restricted to participants who (1) completed the self-report questionnaire at all measurement time points, (2) did not receive psychotherapeutic support during study participation, (3) did not use the investigated digital therapeutic before receiving access during study participation, and (4) used the investigated digital therapeutic at least 1 time per period up to the 5- to 8-week period of the 12-week intervention period (only IG).
Analysis of covariance was used to examine changes in distress, depression, anxiety, and fatigue outcomes between the trial arms from baseline to 12 weeks, controlling for baseline scores. Exploratory regression analyses were conducted to investigate potential variables influencing the primary outcome. These analyses focused exclusively on sociodemographic and clinical factors that showed differences between the IG and CG in the initial group comparison. Partial eta–squared was reported as the effect size for all analyses of covariance, with effect sizes interpreted as small, medium, and large at ≥0.01, ≥0.06, and ≥0.14 [ 47 ], respectively. Differences in QoL between trial arms at follow-up (12 weeks) were analyzed with a 2-tailed 2-sample t test, using Hedges g ' as a measure of effect size (≥0.2=small effect, ≥0.5=medium effect, and ≥0.8=large effect [ 47 ]).
Missing outcome data at random were imputed using the expectation-maximization algorithm. For dropouts, the last observation carried forward was used. For deceased participants, the worst possible values were assumed. Dropouts were participants who failed to complete the baseline or follow-up questionnaires or failed to provide a physician’s letter confirming their cancer diagnosis. A dropout analysis was performed to compare the variables of age, sex, and baseline distress between study noncompleters (dropouts) and study completers using chi-square and t tests. Furthermore, to model the robustness of the primary efficacy analysis under different assumptions for missing data mechanisms, an explorative sensitivity analysis using reference-based multiple imputation (jump-to-reference) [ 48 ] was performed in the extended ITT population (all randomized participants). For this purpose, monotone missing values were replaced using the jump-to-reference approach, whereas sporadic missing values were replaced under the assumption of missing at random. For jump-to-control and jump-to-reference imputation, 50 data sets were generated to minimize the loss of statistical power. The results were then aggregated across the imputed data sets [ 49 ].
All statistical tests were 2-tailed, with a significance level of 5%. Analyses were performed using R (version 4.1.0; R Foundation for Statistical Computing) [ 50 ].
Study Sample
Over the 3-month recruitment period, 517 persons were screened for eligibility and 321 were determined eligible. Of the 321 participants, 248 (77.3%) gave informed consent and were randomly assigned to the IG and the CG ( Figure 2 ). Of the 248 participants, 37 (14.9%) were considered dropouts because they did not complete baseline or follow-up assessments (n=7), failed to confirm their cancer diagnosis by submission of a physician’s letter (n=7), or both (n=23). Age and sex of study dropouts and study completers did not differ ( P age =.89 and P sex =.23), but participants who dropped out showed higher distress levels at baseline compared to study completers ( P =.02). Participants without a verified cancer diagnosis (30/248, 12.1%) were excluded from the ITT analysis, resulting in an ITT population of 218 participants (n=99, 45.4% IG and n=119, 54.6% CG). Of the 218 participants, 173 (79%) were recruited via social media advertisements and cancer support groups and 45 (21%) were recruited using the participant pool of prior studies.

Baseline characteristics were balanced between the groups ( Table 2 ), but participants in the IG were younger compared with those in the CG ( P =.02). No baseline differences in the primary and secondary outcome parameters were observed between the groups, with P values as follows: P =.99 (distress), P =.25 (depression), P =.47 (anxiety), and P =.21 (fatigue). On average, participants were 56 (SD 11) years old, and 60.6% (132/218) of the participants were female and had been diagnosed with cancer 25 (SD 17) months earlier. The most frequently reported cancer types were breast cancer (74/218, 33.9%) and hematological cancer (61/218, 28%), with 8.7% (19/218) of participants reporting a diagnosis of relapsed cancer. The PP population comprised 124 participants, following the exclusion of 94 participants. The primary reasons for exclusion were psychotherapeutic support during study participation and prior use of the investigated digital therapeutic.
a Intervention=12-week access to digital therapeutic app intervention+usual care.
b Control=usual care.
c NCCN Distress Thermometer: National Comprehensive Cancer Network Distress Thermometer (at baseline, clinically significant level of distress≥5).
d HADS-A: Hospital Anxiety and Depression Scale, anxiety subscale (German version, at baseline, cutoff score ≥8).
e HADS-D: Hospital Anxiety and Depression Scale, depression subscale (German version, at baseline, cutoff score ≥8).
f Multiple reasons are possible within 1 patient, and cases do not add up to the total number.
After 12 weeks, participants in the IG reported a reduced level of distress compared to participants in the CG in the ITT population ( F 1,215 =4.7; P =.03; ηp²=0.02; Table 3 ). The observed treatment effect was more pronounced in the PP population ( F 1,121 =6.9; P =.01; ηp²=0.05). The analysis revealed that higher levels of baseline distress predicted a greater change in distress after 12 weeks in the IG. An exploratory regression analysis yielded no predictive effect of age on the change in distress. The explorative sensitivity analysis among all randomized participants (n=248) showed comparable treatment effects (jump-to-control: F 1,19375.1 =5.3; P =.02; ηp²=0.02 and jump-to-intervention: F 1,15314.8 =5.9; P =.02; ηp²=0.02).
a An analysis of covariance was used to test for differences in change in distress levels between groups from baseline to follow-up (12 weeks), controlling for baseline. The partial eta–squared is the reported standardized effect size for the mean difference. The effect sizes can be interpreted as small, medium, or large at ≥0.01, ≥0.06, and ≥0.14, respectively. The results of the intention-to-treat and per-protocol analysis are reported.
b Intervention=12-week access to digital therapeutic app intervention+usual care.
c Control=usual care.
d N/A: not applicable.
In the ITT population, symptoms of anxiety ( F 1,215 =4.8; P =.03; ηp²=0.02), depression ( F 1,215 =15.5; P <.001; ηp²=0.07), and fatigue ( F 1,215 =4.4; P =.04; ηp²=0.02) improved in participants in the IG from baseline to 12 weeks compared to participants in the CG ( Table 4 ). The observed treatment effects on anxiety and depression were more pronounced in the PP population (anxiety: F 1,121 =7.2; P =.01; ηp²=0.06 and depression: F 1,121 =14.9; P <.001; ηp²=0.11). A trend-to-significant treatment effect was observed for fatigue symptoms in the PP population ( F 1,121 =3.8; P =.05; ηp²=0.03). QoL did not differ significantly between the groups at 12 weeks (ITT: t 216 =0.88; P =.38; g=0.12 and PP: t 122 =1.63; P =.11; g=0.30).
c HADS-A: Hospital Anxiety and Depression Scale, anxiety subscale (German version, at baseline, cutoff score ≥8).
d ITT: intention-to-treat.
e N/A: not applicable.
f Italicized values are significant at P <.05.
g PP: per-protocol.
h HADS-D: Hospital Anxiety and Depression Scale, depression subscale (German version, at baseline, cutoff score ≥8).
i FACIT-F Functional Assessment of Chronic Illness Therapy–Fatigue.
j CGI-I: Clinical Global Impression Improvement.
Safety Outcomes
IG participants reported no adverse reactions or side effects of digital therapeutic during the study.
Of the 99 participants in the IG (ITT), 98 (99%), 78 (79%), and 67 (68%) used the digital therapeutic intervention at 0- to 4-, 5- to 8-, and 9- to 12-week periods of the 12-week intervention, respectively, demonstrating good initial adherence to the intervention, which decreased moderately over time. App use (module use and days spent on the app) decreased over time ( Table 5 ). IG participants accessed content from various categories at different frequencies. The most accessed categories were cancer therapy, symptoms and side effects, and nutrition in cancer, with 80% (79/99), 83% (82/99), and 80% (79/99) of users accessing the content in these categories, respectively. Conversely, partnership and family, relaxation, and recipes were accessed less, with 29% (28/99), 34% (33/99), and 32% (31/99) of users, respectively.
Principal Findings
This nationwide waitlist RCT examined the efficacy of Mika, an app-based digital therapeutic that provides a personalized supportive intervention for patients with cancer. Participants who had access to the Mika app for 12 weeks showed significant improvements in perceived distress (ie, the primary outcome) and symptoms of anxiety, depression, and fatigue (ie, the secondary outcomes) compared to participants who received UC. The observed treatment effects were similar in the ITT and PP populations but more pronounced in the PP population, indicating the overall robustness of the findings. We observed no group difference in the QoL after 12 weeks. Intervention adherence was good, and no adverse reactions or side effects of the investigated digital therapeutic were reported.
Comparison With Prior Work
While a growing body of research shows evidence of the efficacy of app-based interventions for oncological populations on distress, fatigue, anxiety, and depression [ 20 , 25 , 27 , 31 , 51 ], this is the first study to examine the efficacy of a single holistic app-based digital therapeutic based on multiple intervention modules on these patient-relevant outcomes. Although the improvement in the primary outcome was modest, it reflects the nuanced nature of psycho-oncological interventions, where even modest changes can have significant clinical relevance. Furthermore, we conducted comprehensive testing of the effects of the investigated digital therapeutic on patients with cancer across all tumor entities, using a larger sample size compared to most previous studies [ 25 , 27 , 31 , 51 ].
In contrast to the findings of this study, however, other studies found an effect of app-based supportive interventions on QoL [ 23 , 27 , 31 ]. This difference in findings could be due to differences in the operationalization and measurement of QoL. In this study, participants’ global QoL was assessed using a single-item questionnaire after a 12-week intervention period. However, global QoL has been shown to be less affected in patients with cancer compared to specific components of QoL, such as social or cognitive functioning and symptom burden from fatigue or insomnia [ 52 ]. Further research using different QoL assessment tools could provide more insights into the efficacy of the investigated digital therapeutic on specific aspects of QoL.
A significant level of intervention adherence and engagement with the digital therapeutic, with varying degrees of interaction across the different app modules, indicates good acceptability and perceived subjective benefit of the investigated digital therapeutic and allows for reliable conclusions about its efficacy in oncological settings. The broad range of engagement, as illustrated by the IQRs, underscores the personalized nature of app use, catering to diverse participant needs and preferences. The variability in engagement levels across different app modules highlights the importance of personalizing digital therapeutics to increase adherence and maximize therapeutic effects.
As we evaluated the app intervention holistically, future studies should examine the impact of the app’s individual components.
While the dropout rate in the IG was slightly higher than that in the CG, the dropout rate in the IG as well as the overall dropout rate was low compared to other app-based supportive interventions [ 25 , 30 ]. Considering that patients with cancer have been found to have a positive attitude toward digital health [ 53 , 54 ], the findings of this study add to the notion that digital health interventions have the potential to overcome barriers associated with access to supportive care in oncological populations [ 55 ].
We found a positive effect of the investigated digital therapeutic on general psychological distress and a broad range of specific distress-associated parameters. Importantly, improvements in psychological symptoms, that is, depression and anxiety, can also have a positive tertiary preventive effect on cancer progression [ 5 ]. The effect sizes in this study ranged from small (ηp²=0.02) to medium (ηp²=0.07) in the ITT population and were more pronounced in the PP population (ηp²=0.05-0.11). The primary outcome improvement, while subtle, aligns with the expected outcomes in psycho-oncological interventions, highlighting the importance of considering the broad spectrum of therapeutic impacts. The medium to large effects observed in secondary end points, together with the primary outcome, illustrate the broad therapeutic impact and highlight the digital therapeutic’s capacity to significantly improve key aspects of psychological well-being in patients with cancer. Small-to-medium effect sizes are common in in-person supportive care interventions [ 6 ]. Our results also compare well with other app-based supportive care interventions, such as small effect sizes reported for a CBT and psychoeducation self-management apps on fatigue [ 25 ] or small to medium effects of a web-based mindfulness-based intervention on anxiety and depression [ 56 ]. This is further supported by the results of several systematic reviews [ 20 , 21 ]. The fact that such effect sizes can be achieved with minimal cost and personnel effort via a digital approach further supports the significant potential for accessibility, reach, and impact of digital therapeutics.
Clinical Implications
The multifaceted intervention modules of the investigated digital therapeutic aim to support patients holistically. The investigated digital therapeutic hereby translates widely used evidence-based intervention methods within supportive care, such as symptom monitoring; patient education; modules of CBT, MBSR, and acceptance and commitment therapy; and strength and flexibility training, into a digital format. The intervention modules of the app are designed to help patients learn about their disease and prepare for discussions with clinicians in an informed decision-making process. This may reduce anxiety and insecurities across the cancer trajectory, while empowering patients and strengthening their self-efficacy.
While it is acknowledged that digital therapeutic interventions might not fully replicate the “in-person” experience, the scope and utility of these tools in the realm of oncology are substantial. For instance, a study evaluating a mobile app designed for tracking patient-reported daily activities found that when supervised by a physician, the data collected were more accurate than when used without guidance [ 57 ]. Conversely, a music app was equally effective in alleviating pain and anxiety in emergency department patients irrespective of supervision [ 58 ]. This suggests that certain interventions, such as symptom tracking, might be more prone to inaccuracies without proper guidance than passive activities, such as listening to music. In addition, CBT, which is traditionally the most effective in face-to-face settings, has generated interest in the digital domain. A study on the digital adaptation of mindfulness-based cognitive therapy for patients with cancer experiencing distress found the therapeutic connection between therapist and patient to be as potent as in in-person sessions [ 59 ]. This underlines the evolving role of digital therapeutics and its potential to reshape therapeutic avenues in oncology, thus paving the way for enhanced patient care.
Furthermore, considering the increasing number of patients with cancer experiencing psychosocial distress and the limited availability of health care professionals, digital therapeutics could present scalable and cost-effective solutions. These solutions can address symptoms and bolster the quality and accessibility of supportive care [ 55 , 60 , 61 ]. Recognizing patients’ diverse needs, tools such as the Mika app leverage artificial intelligence to deliver real-time, tailored support. This has the potential to benefit a broad spectrum of patients with cancer globally while also reducing the pressure on health care infrastructure and professionals. Therefore, digital therapeutics offer a patient-focused approach that is adaptable to specific clinical and lifestyle challenges such as disease management, emotional support, and health-related determinants. They might also further enhance medication adherence, tolerance to chemotherapy, and overall survival rate in the cancer care continuum [ 15 ]. Incorporating these digital tools into routine oncological supportive care can augment patient-centric care and enrich patient experience, safety, and interactions with clinicians [ 15 , 61 ]. However, while there is a consensus among medical professionals and stakeholders regarding the revolutionary potential of digital health in addressing cancer treatment challenges, the path to universal adoption remains intricate. Future studies should delve into the assimilation of digital therapeutics, such as Mika, into standard care across varied clinical environments and evaluate hurdles such as digital literacy and the acceptance of digital tools by both patients and health care professionals [ 62 - 64 ].
Strengths and Limitations
The main strength of this study was the app itself. It addresses the overreaching problem areas faced by all patients with cancer while providing tailored support for population-specific areas of burden (ie, cancer type, treatment status, and use behavior). Its flexible and easily accessible use allows for seamless integration into patients’ daily lives and continuity of supportive treatment. In addition, the low overall dropout rate and data monitoring led to very little missing data. Similar findings in the ITT, PP, and extended ITT populations suggest overall robustness of the results.
This study has several limitations. First, the web-based recruitment procedure may have led to study registration from patients with cancer who were particularly motivated, digitally literate, and highly functioning in seeking support during their cancer journey, which may limit the generalizability of the study. However, the use of additional recruitment pathways (support groups and participant pool) likely resulted in the recruitment of a more heterogeneous sample, possibly compensating for potential selection bias. Future studies might investigate the impact of various recruitment channels on the efficacy of digital therapeutics, and thus, which population may be particularly responsive to digital interventions. Second, the higher number of dropouts in the IG compared to the CG may reflect treatment dissatisfaction or lost interest in the treatment of some participants, potentially confounding the study’s results. Dropouts, who are more likely to show elevated levels of distress, may have been made aware of the increased need for support through the intervention modules. Patients with clinically significant levels of distress or mental disorders might have accessed support services with more guidance from a health care professional, such as psychotherapy or psycho-oncological counseling. However, no side effects or adverse events were reported in the IG, and the overall robust pattern of results in the ITT, PP, and extended ITT populations suggests a low risk of attrition bias. The fact that participants who dropped out of the study showed higher baseline distress levels may have led to an underestimation of the intervention effect as higher baseline distress levels predicted a greater change in outcome after treatment. Third, due to the nature of the intervention, the group allocation could not be blinded. While experimenter bias was reduced due to a predefined, standardized monitoring procedure and statistical analysis plan, IG participants may have anticipated potential effects. Fourth, the intervention, along with its adherence, was assessed as a whole, which requires the evaluation of specific modules and any potential dose-response relationship in the future. In addition, there was no specific measure to evaluate the subjective usefulness or satisfaction with the digital therapeutic under investigation. Incorporating such a measure could have provided targeted insights into the participants’ perceptions and experiences with the app. However, the observed use behavior, characterized by participants repeatedly accessing the app and actively engaging with its content, may serve as an indirect indicator of the app’s value to the participants. Future studies should aim to validate this interpretation. Finally, the study sample included participants with a wide variety of cancer diagnoses, which did not allow for the examination of diagnosis-specific intervention effects. However, the sample composition is consistent with the target population of the investigated digital therapeutic, which includes patients with cancer of all entities, and strengthens the study’s generalizability and clinical utility. Moreover, a large body of data shows that while variables such as cancer type, treatment status, disease progression, and sex may influence the magnitude of treatment response to supportive therapy, the beneficial effects of supportive therapy are present across various cancer subpopulations [ 65 - 67 ]. In addition, there is a consensus that psychosocial support needs to be integrated into routine cancer care for all cancer types [ 68 , 69 ].
Conclusions
In summary, this RCT demonstrated that Mika, an app-based digital therapeutic that provides a personalized supportive care intervention, can effectively reduce psychological distress and further alleviate symptoms of anxiety, depression, and fatigue in patients with cancer. Digital therapeutics, such as Mika, deliver easily accessible, patient-centered, and effective psychosocial and self-management support for patients with cancer across the course of the disease. Digital therapeutics may present scalable solutions to support patients with cancer worldwide and thus help fill the supportive care gap. Further research is needed to explore the integration of Mika into routine cancer care and its efficacy in diverse clinical settings.
Acknowledgments
The clinical trial was funded by Fosanis GmbH, Berlin.
Data Availability
The data set generated during and analyzed during this study, including individual participant data that underlie the results reported in this article after deidentification (text, tables, and figures), clinical study report, informed consent form, and analytic code, are available from AMT beginning 3 months and ending 5 years following article publication. Access to the data will be granted to investigators whose proposed use of the data has been approved by an independent review committee identified for this purpose, for individual patient data meta-analysis. Proposals for accessing the data may be submitted up to 36 months following article publication.
Authors' Contributions
JSR and GF provided financial support. FS, AM, and HB provided administrative support, with FS also contributing to the collection and assembly of data. MF contributed to data curation. FS, AM, and MF contributed to data analysis and interpretation. FS and AM equally contributed to writing the original draft. All authors contributed to reviewing and editing the draft and provided final approval of the manuscript. AMT, FS, and JSR contributed to the conception and design.
Conflicts of Interest
FS, MF, and HB received research funding for this trial from Fosanis GmbH, which was paid to their institution. AM is an employee at the company Fosanis GmbH. JSR and GF work for the company Fosanis GmbH. They are the managing directors and board members of Fosanis GmbH and own shares of Fosanis GmbH. All other authors declare no other conflicts of interest.
CONSORT-eHEALTH checklist (V 1.6.1).
- Pachman DR, Barton DL, Swetz KM, Loprinzi CL. Troublesome symptoms in cancer survivors: fatigue, insomnia, neuropathy, and pain. J Clin Oncol. Oct 20, 2012;30(30):3687-3696. [ CrossRef ] [ Medline ]
- Mehnert A, Hartung TJ, Friedrich M, Vehling S, Brähler E, Härter M, et al. One in two cancer patients is significantly distressed: prevalence and indicators of distress. Psychooncology. Jan 16, 2018;27(1):75-82. [ CrossRef ] [ Medline ]
- Mitchell AJ, Chan M, Bhatti H, Halton M, Grassi L, Johansen C, et al. Prevalence of depression, anxiety, and adjustment disorder in oncological, haematological, and palliative-care settings: a meta-analysis of 94 interview-based studies. Lancet Oncol. Feb 2011;12(2):160-174. [ CrossRef ] [ Medline ]
- Meggiolaro E, Berardi MA, Andritsch E, Nanni MG, Sirgo A, Samorì E, et al. Cancer patients' emotional distress, coping styles and perception of doctor-patient interaction in European cancer settings. Pall Supp Care. Jul 09, 2015;14(3):204-211. [ CrossRef ] [ Medline ]
- Brown KW, Levy AR, Rosberger Z, Edgar L. Psychological distress and cancer survival: a follow-up 10 years after diagnosis. Psychosom Med. 2003;65(4):636-643. [ CrossRef ] [ Medline ]
- Faller H, Schuler M, Richard M, Heckl U, Weis J, Küffner R. Effects of psycho-oncologic interventions on emotional distress and quality of life in adult patients with cancer: systematic review and meta-analysis. J Clin Oncol. Feb 20, 2013;31(6):782-793. [ CrossRef ] [ Medline ]
- Epstein RM, Street RL. Patient-centered communication in cancer care: promoting healing and reducing suffering. National Cancer Institute. 2007. URL: https://cancercontrol.cancer.gov/sites/default/files/2020-06/pcc_monograph.pdf [accessed 2024-03-05]
- Alcalde Castro M, Chavarri Guerra Y, Ramos-Lopez WA, Covarrubias-Gómez A, Sanchez S, Quiroz P, et al. Patient-reported barriers for accessing supportive care among patients with metastatic cancer treated at a public cancer center in Mexico. J Clin Oncol. Dec 01, 2018;36(34_suppl):124. [ CrossRef ]
- Carrieri D, Peccatori FA, Boniolo G. Supporting supportive care in cancer: the ethical importance of promoting a holistic conception of quality of life. Crit Rev Oncol Hematol. Nov 2018;131:90-95. [ CrossRef ] [ Medline ]
- Kumar P, Casarett D, Corcoran A, Desai K, Li Q, Chen J, et al. Utilization of supportive and palliative care services among oncology outpatients at one academic cancer center: determinants of use and barriers to access. J Palliat Med. Aug 2012;15(8):923-930. [ FREE Full text ] [ CrossRef ] [ Medline ]
- Bellas O, Kemp E, Edney L, Oster C, Roseleur J. The impacts of unmet supportive care needs of cancer survivors in Australia: a qualitative systematic review. Eur J Cancer Care (Engl). Nov 12, 2022;31(6):e13726. [ CrossRef ] [ Medline ]
- Siegel RL, Miller KD, Jemal A. Cancer statistics, 2019. CA Cancer J Clin. Jan 08, 2019;69(1):7-34. [ FREE Full text ] [ CrossRef ] [ Medline ]
- Atun R, Cavalli F. The global fight against cancer: challenges and opportunities. Lancet. Feb 2018;391(10119):412-413. [ CrossRef ]
- Penedo FJ, Oswald LB, Kronenfeld JP, Garcia SF, Cella D, Yanez B. The increasing value of eHealth in the delivery of patient-centred cancer care. Lancet Oncol. May 2020;21(5):e240-e251. [ FREE Full text ] [ CrossRef ] [ Medline ]
- Gussoni G, Ravot E, Zecchina M, Recchia G, Santoro E, Ascione R, et al. Digital therapeutics in oncology: findings, barriers and prospects. A narrative review. Ann Res Oncol. Feb 2022;02(01):55. [ FREE Full text ] [ CrossRef ]
- Yang J, Weng L, Chen Z, Cai H, Lin X, Hu Z, et al. Development and testing of a mobile app for pain management among cancer patients discharged from hospital treatment: randomized controlled trial. JMIR Mhealth Uhealth. May 29, 2019;7(5):e12542. [ FREE Full text ] [ CrossRef ] [ Medline ]
- Ghanbari E, Yektatalab S, Mehrabi M. Effects of psychoeducational interventions using mobile apps and mobile-based online group discussions on anxiety and self-esteem in women with breast cancer: randomized controlled trial. JMIR Mhealth Uhealth. May 18, 2021;9(5):e19262. [ FREE Full text ] [ CrossRef ] [ Medline ]
- Hou IC, Lin HY, Shen SH, Chang KJ, Tai HC, Tsai AJ, et al. Quality of life of women after a first diagnosis of breast cancer using a self-management support mHealth app in Taiwan: randomized controlled trial. JMIR Mhealth Uhealth. Mar 04, 2020;8(3):e17084. [ FREE Full text ] [ CrossRef ] [ Medline ]
- Keum J, Chung MJ, Kim Y, Ko H, Sung MJ, Jo JH, et al. Usefulness of smartphone apps for improving nutritional status of pancreatic cancer patients: randomized controlled trial. JMIR Mhealth Uhealth. Aug 31, 2021;9(8):e21088. [ FREE Full text ] [ CrossRef ] [ Medline ]
- Hernandez Silva E, Lawler S, Langbecker D. The effectiveness of mHealth for self-management in improving pain, psychological distress, fatigue, and sleep in cancer survivors: a systematic review. J Cancer Surviv. Feb 2019;13(1):97-107. [ CrossRef ] [ Medline ]
- Matis J, Svetlak M, Slezackova A, Svoboda M, Šumec R. Mindfulness-based programs for patients with cancer via eHealth and mobile health: systematic review and synthesis of quantitative research. J Med Internet Res. Nov 16, 2020;22(11):e20709. [ FREE Full text ] [ CrossRef ] [ Medline ]
- Adriaans DJ, Dierick-van Daele AT, van Bakel MJ, Nieuwenhuijzen GA, Teijink JA, Heesakkers FF, et al. Digital self-management support tools in the care plan of patients with cancer: review of randomized controlled trials. J Med Internet Res. Jun 29, 2021;23(6):e20861. [ FREE Full text ] [ CrossRef ] [ Medline ]
- Qin M, Chen B, Sun S, Liu X. Effect of mobile phone app-based interventions on quality of life and psychological symptoms among adult cancer survivors: systematic review and meta-analysis of randomized controlled trials. J Med Internet Res. Dec 19, 2022;24(12):e39799. [ FREE Full text ] [ CrossRef ] [ Medline ]
- Springer F, Mehnert-Theuerkauf A. Content features and its implementation in novel app-based psycho-oncological interventions for cancer survivors: a narrative review. Curr Opin Oncol. Jul 01, 2022;34(4):313-319. [ CrossRef ] [ Medline ]
- Spahrkäs SS, Looijmans A, Sanderman R, Hagedoorn M. Beating cancer-related fatigue with the Untire mobile app: results from a waiting-list randomized controlled trial. Psychooncology. Nov 2020;29(11):1823-1834. [ FREE Full text ] [ CrossRef ] [ Medline ]
- Mayer DK, Landucci G, Awoyinka L, Atwood AK, Carmack CL, Demark-Wahnefried W, et al. SurvivorCHESS to increase physical activity in colon cancer survivors: can we get them moving? J Cancer Surviv. Feb 9, 2018;12(1):82-94. [ FREE Full text ] [ CrossRef ] [ Medline ]
- Lengacher CA, Reich RR, Ramesar S, Alinat CB, Moscoso M, Cousin L, et al. Feasibility of the mobile mindfulness-based stress reduction for breast cancer (mMBSR(BC)) program for symptom improvement among breast cancer survivors. Psychooncology. Feb 2018;27(2):524-531. [ FREE Full text ] [ CrossRef ] [ Medline ]
- Mikolasek M, Witt CM, Barth J. Effects and implementation of a mindfulness and relaxation app for patients with cancer: mixed methods feasibility study. JMIR Cancer. Jan 13, 2021;7(1):e16785. [ FREE Full text ] [ CrossRef ] [ Medline ]
- Gustafson DH, DuBenske LL, Atwood AK, Chih MY, Johnson RA, McTavish F, et al. Reducing symptom distress in patients with advanced cancer using an e-alert system for caregivers: pooled analysis of two randomized clinical trials. J Med Internet Res. Nov 14, 2017;19(11):e354. [ FREE Full text ] [ CrossRef ] [ Medline ]
- Chung IY, Jung M, Park YR, Cho D, Chung H, Min YH, et al. Exercise promotion and distress reduction using a mobile app-based community in breast cancer survivors. Front Oncol. 2019;9:1505. [ FREE Full text ] [ CrossRef ] [ Medline ]
- Greer JA, Jacobs J, Pensak N, MacDonald JJ, Fuh C, Perez GK, et al. Randomized trial of a tailored cognitive-behavioral therapy mobile application for anxiety in patients with incurable cancer. Oncologist. Aug 25, 2019;24(8):1111-1120. [ FREE Full text ] [ CrossRef ] [ Medline ]
- Basch E, Deal AM, Kris MG, Scher HI, Hudis CA, Sabbatini P, et al. Symptom monitoring with patient-reported outcomes during routine cancer treatment: a randomized controlled trial. J Clin Oncol. Feb 20, 2016;34(6):557-565. [ FREE Full text ] [ CrossRef ] [ Medline ]
- Greer JA, Park ER, Prigerson HG, Safren SA. Tailoring cognitive-behavioral therapy to treat anxiety comorbid with advanced cancer. J Cogn Psychother. Jan 01, 2010;24(4):294-313. [ FREE Full text ] [ CrossRef ] [ Medline ]
- Carlson LE, Speca M, Faris P, Patel KD. One year pre-post intervention follow-up of psychological, immune, endocrine and blood pressure outcomes of mindfulness-based stress reduction (MBSR) in breast and prostate cancer outpatients. Brain Behav Immun. Nov 2007;21(8):1038-1049. [ CrossRef ] [ Medline ]
- Speca M, Carlson LE, Goodey E, Angen M. A randomized, wait-list controlled clinical trial: the effect of a mindfulness meditation-based stress reduction program on mood and symptoms of stress in cancer outpatients. Psychosom Med. 2000;62(5):613-622. [ CrossRef ] [ Medline ]
- Winters-Stone KM, Dobek J, Nail L, Bennett JA, Leo MC, Naik A, et al. Strength training stops bone loss and builds muscle in postmenopausal breast cancer survivors: a randomized, controlled trial. Breast Cancer Res Treat. Jun 19, 2011;127(2):447-456. [ FREE Full text ] [ CrossRef ] [ Medline ]
- Adam R, Bond C, Murchie P. Educational interventions for cancer pain. A systematic review of systematic reviews with nested narrative review of randomized controlled trials. Patient Educ Couns. Mar 2015;98(3):269-282. [ CrossRef ] [ Medline ]
- Wolff J, Stupin J, Olschewski J, Pirmorady Sehouli A, Maier A, Fofana M, et al. Digital therapeutic to improve cancer-related well-being: a pilot randomized controlled trial. Int J Gynecol Cancer. Jul 03, 2023;33(7):1118-1124. [ CrossRef ] [ Medline ]
- Harrison SE, Watson EK, Ward AM, Khan NF, Turner D, Adams E, et al. Primary health and supportive care needs of long-term cancer survivors: a questionnaire survey. J Clin Oncol. May 20, 2011;29(15):2091-2098. [ CrossRef ] [ Medline ]
- Xiao J, Ye H, He X, Zhang H, Wu F, Chua TS. Attentional factorization machines: learning the weight of feature interactions via attention networks. arXiv. Preprint posted online August 15, 2017. 2017. [ FREE Full text ] [ CrossRef ]
- Sensoy M, Kaplan L, Kandemir M. Evidential deep learning to quantify classification uncertainty. In: Proceedings of the 32nd Conference on Neural Information Processing Systems. 2018. Presented at: NeurIPS '18; December 3-8, 2018; Montreal, QC. URL: https://www.proceedings.com/48413.html
- Mehnert A, Müller D, Lehmann C, Koch U. Die Deutsche version des NCCN distress-thermometers. Z Für Psychiatr Psychol Psychother. Jan 2006;54(3):213-223. [ CrossRef ]
- National Comprehensive Cancer Network. Distress management. Clinical practice guidelines. J Natl Compr Canc Netw. Jul 01, 2003;1(3):344-374. [ CrossRef ] [ Medline ]
- Petermann F. Hospital anxiety and depression scale, Deutsche version (HADS-D). Z Für Psychiatr Psychol Psychother. Jul 2011;59(3):251-253. [ CrossRef ]
- Lai J, Cella D, Chang C, Bode RK, Heinemann AW. Item banking to improve, shorten and computerize self-reported fatigue: an illustration of steps to create a core item bank from the FACIT-Fatigue Scale. Qual Life Res. Aug 2003;12(5):485-501. [ CrossRef ] [ Medline ]
- Guy W. Clinical global impressions scale. Psychiatry. 1976. [ CrossRef ]
- Cohen J. Statistical Power Analysis for the Behavioral Sciences. Oxfordshire, UK. Routledge; 2013.
- Carpenter JR, Roger JH, Kenward MG. Analysis of longitudinal trials with protocol deviation: a framework for relevant, accessible assumptions, and inference via multiple imputation. J Biopharm Stat. Oct 18, 2013;23(6):1352-1371. [ CrossRef ] [ Medline ]
- Enders CK. Applied Missing Data Analysis. New York, NY. The Guilford Press; 2022.
- R Core Team. R: a language and environment for statistical computing. R Foundation for Statistical Computing. 2021. URL: https://www.R-project.org/ [accessed 2024-03-05]
- Ham K, Chin S, Suh YJ, Rhee M, Yu ES, Lee HJ, et al. Preliminary results from a randomized controlled study for an app-based cognitive behavioral therapy program for depression and anxiety in cancer patients. Front Psychol. Jul 25, 2019;10:1592. [ FREE Full text ] [ CrossRef ] [ Medline ]
- Hinz A, Mehnert A, Dégi C, Reissmann DR, Schotte D, Schulte T. The relationship between global and specific components of quality of life, assessed with the EORTC QLQ-C30 in a sample of 2019 cancer patients. Eur J Cancer Care (Engl). Mar 16, 2017;26(2):e12416. [ CrossRef ] [ Medline ]
- Jansen F, van Uden-Kraan CF, van Zwieten V, Witte BI, Verdonck-de Leeuw IM. Cancer survivors' perceived need for supportive care and their attitude towards self-management and eHealth. Support Care Cancer. Jun 26, 2015;23(6):1679-1688. [ CrossRef ] [ Medline ]
- Kessel KA, Vogel MM, Kessel C, Bier H, Biedermann T, Friess H, et al. Mobile health in oncology: a patient survey about app-assisted cancer care. JMIR Mhealth Uhealth. Jun 14, 2017;5(6):e81. [ FREE Full text ] [ CrossRef ] [ Medline ]
- Marthick M, McGregor D, Alison J, Cheema B, Dhillon H, Shaw T. Supportive care interventions for people with cancer assisted by digital technology: systematic review. J Med Internet Res. Oct 29, 2021;23(10):e24722. [ FREE Full text ] [ CrossRef ] [ Medline ]
- Nissen ER, O'Connor M, Kaldo V, Højris I, Borre M, Zachariae R, et al. Internet-delivered mindfulness-based cognitive therapy for anxiety and depression in cancer survivors: a randomized controlled trial. Psychooncology. Jan 18, 2020;29(1):68-75. [ FREE Full text ] [ CrossRef ] [ Medline ]
- Egbring M, Far E, Roos M, Dietrich M, Brauchbar M, Kullak-Ublick GA, et al. A mobile app to stabilize daily functional activity of breast cancer patients in collaboration with the physician: a randomized controlled clinical trial. J Med Internet Res. Sep 06, 2016;18(9):e238. [ FREE Full text ] [ CrossRef ] [ Medline ]
- Chai PR, Schwartz E, Hasdianda MA, Azizoddin DR, Kikut A, Jambaulikar GD, et al. A brief music app to address pain in the emergency department: prospective study. J Med Internet Res. May 20, 2020;22(5):e18537. [ FREE Full text ] [ CrossRef ] [ Medline ]
- Bisseling E, Cillessen L, Spinhoven P, Schellekens M, Compen F, van der Lee M, et al. Development of the therapeutic alliance and its association with internet-based mindfulness-based cognitive therapy for distressed cancer patients: secondary analysis of a multicenter randomized controlled trial. J Med Internet Res. Oct 18, 2019;21(10):e14065. [ FREE Full text ] [ CrossRef ] [ Medline ]
- Parikh RB, Basen-Enquist KM, Bradley C, Estrin D, Levy M, Lichtenfeld JL, et al. Digital health applications in oncology: an opportunity to seize. J Natl Cancer Inst. Oct 06, 2022;114(10):1338-1339. [ FREE Full text ] [ CrossRef ] [ Medline ]
- Aapro M, Bossi P, Dasari A, Fallowfield L, Gascón P, Geller M, et al. Digital health for optimal supportive care in oncology: benefits, limits, and future perspectives. Support Care Cancer. Oct 12, 2020;28(10):4589-4612. [ FREE Full text ] [ CrossRef ] [ Medline ]
- Rankin NM, Butow PN, Thein T, Robinson T, Shaw JM, Price MA, et al. Everybody wants it done but nobody wants to do it: an exploration of the barrier and enablers of critical components towards creating a clinical pathway for anxiety and depression in cancer. BMC Health Serv Res. Jan 22, 2015;15(1):28. [ FREE Full text ] [ CrossRef ] [ Medline ]
- Leader AE, Capparella LM, Waldman LB, Cammy RB, Petok AR, Dean R, et al. Digital literacy at an urban cancer center: implications for technology use and vulnerable patients. JCO Clinical Cancer Informatics. Dec 2021;(5):872-880. [ CrossRef ]
- den Bakker CM, Schaafsma FG, Huirne JA, Consten EC, Stockmann HB, Rodenburg CJ, et al. Cancer survivors' needs during various treatment phases after multimodal treatment for colon cancer - is there a role for eHealth? BMC Cancer. Dec 04, 2018;18(1):1207. [ FREE Full text ] [ CrossRef ] [ Medline ]
- Cillessen L, Johannsen M, Speckens AE, Zachariae R. Mindfulness-based interventions for psychological and physical health outcomes in cancer patients and survivors: a systematic review and meta-analysis of randomized controlled trials. Psychooncology. Dec 11, 2019;28(12):2257-2269. [ FREE Full text ] [ CrossRef ] [ Medline ]
- Tauber NM, O'Toole MS, Dinkel A, Galica J, Humphris G, Lebel S, et al. Effect of psychological intervention on fear of cancer recurrence: a systematic review and meta-analysis. J Clin Oncol. Nov 01, 2019;37(31):2899-2915. [ FREE Full text ] [ CrossRef ] [ Medline ]
- Merluzzi TV, Pustejovsky JE, Philip EJ, Sohl SJ, Berendsen M, Salsman JM. Interventions to enhance self-efficacy in cancer patients: a meta-analysis of randomized controlled trials. Psychooncology. Sep 09, 2019;28(9):1781-1790. [ FREE Full text ] [ CrossRef ] [ Medline ]
- Jacobsen PB, Wagner LI. A new quality standard: the integration of psychosocial care into routine cancer care. J Clin Oncol. Apr 10, 2012;30(11):1154-1159. [ CrossRef ] [ Medline ]
- Fann JR, Ell K, Sharpe M. Integrating psychosocial care into cancer services. J Clin Oncol. Apr 10, 2012;30(11):1178-1186. [ CrossRef ] [ Medline ]
Abbreviations
Edited by YH Lin; submitted 21.08.23; peer-reviewed by F Denis, A Haussmann, N Schaeffeler, P Chow; comments to author 24.01.24; accepted 23.02.24; published 25.04.24.
©Franziska Springer, Ayline Maier, Michael Friedrich, Jan Simon Raue, Gandolf Finke, Florian Lordick, Guy Montgomery, Peter Esser, Hannah Brock, Anja Mehnert-Theuerkauf. Originally published in the Journal of Medical Internet Research (https://www.jmir.org), 25.04.2024.
This is an open-access article distributed under the terms of the Creative Commons Attribution License (https://creativecommons.org/licenses/by/4.0/), which permits unrestricted use, distribution, and reproduction in any medium, provided the original work, first published in the Journal of Medical Internet Research, is properly cited. The complete bibliographic information, a link to the original publication on https://www.jmir.org/, as well as this copyright and license information must be included.

An official website of the United States government
The .gov means it’s official. Federal government websites often end in .gov or .mil. Before sharing sensitive information, make sure you’re on a federal government site.
The site is secure. The https:// ensures that you are connecting to the official website and that any information you provide is encrypted and transmitted securely.
- Publications
- Account settings
Preview improvements coming to the PMC website in October 2024. Learn More or Try it out now .
- Advanced Search
- Journal List
- Front Neurol
The Effect of Anxiety and Depression on Sleep Quality of Individuals With High Risk for Insomnia: A Population-Based Study
Chang-myung oh.
1 Division of Endocrinology and Metabolism, CHA Bundang Medical Center, School of Medicine CHA University, Seongnam-si, South Korea
2 Biostatistics Collaboration Unit, Yonsei University College of Medicine, Seoul, South Korea
3 Department of Neurology, Yonsei University College of Medicine, Seoul, South Korea
Kyoo Ho Cho
Min kyung chu, associated data.
The datasets analyzed in this manuscript are not publicly available. Requests to access the datasets should be directed to ca.shuy@kmuhc .
Introduction: One of the most common sleep disorders, insomnia is a significant public health concern. Several psychiatric disorders, such as anxiety disorders and depression, have shown strong relationships with insomnia. However, the clinical impact of the combination of these two conditions on insomnia severity and sleep quality remains unknown. We investigated the relationship between sleep disturbance and psychiatric comorbidities in subjects with high risk for insomnia.
Methods: We analyzed data from a nation-wide cross-sectional survey of Korean adults aged 19 ~ 69 years conducted from November 2011 to January 2012. The survey was performed via face-to-face interviews using a structured questionnaire. We used the insomnia severity index (ISI) to evaluate insomnia and defined respondents with ISI scores of ≥10 were considered to be at high risk for insomnia. To diagnose anxiety and depression, we used the Goldberg anxiety scale (GAS) and Patient Health Questionnaire-9 (PHQ-9), respectively.
Results: Of the 2,762 respondents, 290 (10.5%) were classified as subjects with high risk for insomnia; anxiety [odds ratio (OR), 9.8; 95% confidence interval (CI), 7.3–13.1] and depression (OR, 19.7; 95% CI, 13.1–29.6) were more common in this population than in participants without insomnia. Of the participants with insomnia, 152 (52.4%) had neither anxiety nor depression, 63 (21.7%) only had anxiety, 21 (7.2%) only had depression, and 54 (18.6%) had both anxiety and depression. The group with both anxiety and depression was associated with worse scores on sleep-related scales than the other groups [high ISI, Pittsburgh Sleep Quality Index (PSQI), and Epworth Sleepiness Scale]. The relationship between outcome measures (ISI and PSQI) and psychiatric problems was significant only when anxiety and depression were present. The PSQI has a significant mediation effect on the relationship between psychiatric comorbidities and insomnia severity.
Conclusion: Among the respondents with insomnia, psychiatric comorbidities may have a negative impact on daytime alertness, general sleep quality, and insomnia severity, especially when the two conditions are present at the same time. Clinicians should, therefore, consider psychiatric comorbidities when treating insomnia.
Introduction
As one of the most common sleep disorders, insomnia has become a significant public health problem. While the prevalence of insomnia varies considerably across countries, its global prevalence of insomnia as defined by the Diagnostic and Statistical Manual of Mental Disorders, Fourth Edition (DSM-IV) criteria is estimated to be ~6–10% ( 1 – 3 ).
Insomnia reportedly increases the rate of car accidents ( 4 ), decreases job performance, results in self-medication with alcohol as well as socio-economic problems ( 5 – 7 ), and has been associated with the onset of cardiovascular diseases ( 8 ).
Although a previous (second) edition of the International Classification of Sleep Disorders (ICSD-2) distinguished psychophysiological insomnia from mental-illness induced insomnia ( 9 ), there are substantial overlap features between the two: e.g., conditioned arousal, poor sleep hygiene, and excessive worry about sleep ( 10 ). Also, many subjects with insomnia have psychiatric comorbidities, rendering the discrimination of insomnia subtypes difficult. Because of these issues (overlapping primary and secondary insomnia), in the next version, ICSD-3, insomnia was recategorized according to time course ( 10 ). Nevertheless, it is still of great value to recognize the most common psychiatric comorbidities such as anxiety disorders and depression in patients with insomnia ( 11 ). Among patients with insomnia, the prevalence of anxiety disorder, including generalized anxiety disorder, panic disorder, post-traumatic stress disorder, and phobia, is 24–36% ( 11 , 12 ), while that of major depression is 14–31% ( 11 , 12 ). Conversely, about 90% of patients with depression complain of sleep disturbance ( 13 , 14 ). Similarly, sleep problems are much more common among individuals with anxiety disorders ( 15 , 16 ).
Multiple studies have investigated the relationship between sleep disorders and psychiatric comorbidity. A study that used data collected by the national survey in the United States revealed that individuals with any comorbid sleep problem (especially non-restorative sleep) are more prone to impairments of daytime activities ( 17 ). Using data obtained by the same survey, another investigation reported that the rate of insomnia complaints was highest among individuals with anxiety and mood disorders (42–63%) ( 18 ). However, to date, it remains unclear whether the combination of anxiety and mood (depression) problems aggravate the severity of insomnia itself and the sleep quality of people at high risk for insomnia.
This study explored the relationships among insomnia severity, sleep quality and daytime sleepiness, and comorbidities with anxiety and depression among individuals at high risk for insomnia. In addition, we used mediation modeling to evaluate whether insomnia severity is mediated by changes in general sleep quality.
Survey Procedure
We used data from a nationwide, cross-sectional survey of headache and anxiety in the general Korean population conducted from November 2011 to January 2012 ( 19 ). Trained interviewers performed structured, face-to-face interviews that included questionnaires regarding sleep and headache disorders and mood problems (anxiety and depression). Adults between the ages of 19 and 69 years were included. Besides, we collected the respondents' demographic and geographic information. The target area, sampling method, and detailed survey procedures were same as the previously documented process ( 19 ). The distribution in this study and the total population were not significantly different in sex, age groups, size of a residential area, and educational level.
Diagnosis of Insomnia, Anxiety, and Depression
We used the insomnia severity index (ISI) to define high risk for insomnia. Subjects who received an ISI score of ≥10 were classified as high risk for insomnia according to a previous community-based study ( 20 ). Using an ISI score of 10 as the cutoff, insomnia was detected with 86.1% sensitivity and 87.7% specificity ( 20 ).
For the diagnosis of anxiety and depression, we used the Goldberg anxiety scale (GAS) and Patient Health Questionnaire-9 (PHQ-9), respectively. The GAS consists of four screening and five supplementary questions. The validated Korean version of the GAS features a sensitivity of 82.0% and a specificity of 94.4% ( 21 ). At least two positive answers to screening and five or more positive answers to complementary questions in GAS questions indicated anxiety. The PHQ-9 was used to diagnose depression ( 22 ). The Korean version of the PHQ-9 features 81% sensitivity and 89.9% specificity ( 23 ). Participants with a PHQ-9 score of ≥10 were considered to have depression. We classified the respondents into four groups: individuals (1) without anxiety or depression, (2) with anxiety but without depression, (3) without anxiety but with depression, and (4) with both anxiety and depression. The demographic data of the participants are shown in Table 1 .
Sociodemographic distribution of all survey participants, the total Korean population, and of cases identified as insomnia, anxiety, and depression.
Total subjects: 2,695; P, p-value;
Measures Related to Sleep Quality
Each individual was asked to complete questionnaires, including the Pittsburgh Sleep Quality Index (PSQI), which accesses the multifactorial construct of sleep dysfunction with strong reliability and validity ( 24 ), and the Epworth Sleepiness Scale (ESS). which assesses each participant's daytime sleepiness ( 25 ). The subjective and objective sleep qualities measured with ISI feature a high concordance with daytime disability ( 26 ). Even young adults with insomnia frequently complain of daytime sleepiness ( 27 ). Additionally, each subject's ISI score was categorized into one of three groups (mild insomnia, 10 ≤ ISI < 15; moderate, 15 ≤ ISI < 20; severe, ISI > 20).
This study was approved by the institutional review board/ethics committee of the Severance Hospital, and written informed consent was obtained from each participant.
Statistical Analysis
We used the chi-square test to examine whether the number of diagnoses differed according to sex, age, size of a residential area, educational level, and types of work shift. We compared group differences in sleep time and ESS and PSQI scores using the Kruskal-Wallis test followed by Bonferroni's multiple comparison correction. Group differences in ISI severity and insomnia characteristics were compared with Jonckheere's trend test. Univariate and multivariate logistic regression analysis was performed to evaluate the odds ratio (OR) of anxiety and depression in individuals with high risk for insomnia compared to those without high risk for insomnia. The same procedure was used to compare the PSQI and ISI scores stratified by the presence of depression or anxiety between groups; sex, age group, size of residential area, and education level were included in the multivariate analysis. To examine whether the association between anxiety and depression comorbidities and insomnia risk is mediated by sleep quality, we performed a mediation analysis based on a previously developed method ( 28 ). In all the statistical analyses, two-tailed p <0.05 were considered statistically significant.
Demographic Data of the Subjects
Of the total 7,430 interviewees, 3,114 completed the survey (acceptance rate of 41.9%), and 352 subjects suspended the interview. The final sample that completed the survey and the interview included 2,762 individuals ( 19 ). The enrollment flow chart of this study is presented in Figure 1 . The prevalence of insomnia, anxiety, and depression according to sex, age distribution, size of the residential area, and education level is depicted in Table 1 . Insomnia, depression, and anxiety were significantly more prevalent among women. Participants with the lowest educational levels were associated with a higher prevalence of insomnia and anxiety than were those who had completed high school, college, or graduate school.

Flow chart of participants in the Korean Headache-Sleep study. Whether individuals had anxiety (A) or depression (D) are denoted by positive (+) and negative (–). A–/D–, without anxiety or depression; A+/D–, with anxiety only; A–/D+, with depression only; A+/D+, with both anxiety and depression.
Prevalence of Insomnia, Anxiety, and Depression and Mean Scores on Sleep-Related Scales
Of the 2,762 participants, 290 (10.5%) were classified as having a high risk for insomnia. The frequencies of insomnia, anxiety, and depression are presented in Table 1 according to sex, age group, size of the residential area, and education level. The mean ISI, ESS, and PSQI scores of individuals with high risk for insomnia were 14.37 ± 4.39, 7.73 ± 4.87, and 7.42 ± 2.59, respectively. Of the 290 subjects with high risk for insomnia, 152 (52.4%) had neither anxiety nor depression (Group 1), 63 (21.7 %) had anxiety only (Group 2), 21 (7.2%) had depression only (Group 3), and 54 (18.6%) had both anxiety and depression (Group 4). Anxiety was more common among individuals who had a high risk for insomnia than among those who did not [40.3% vs. 6.1%; OR, 10.1; 95% confidence interval (CI), 7.6–13.4]; this finding remained consistent after adjustment for demographic variables (OR, 9.8; 95% CI, 7.3–13.1). Depression was also more common among individuals who had a high risk for insomnia than those who did not (25.9% vs. 1.7%; OR, 20.1; 95% CI, 13.4–30.2); this finding also remained consistent after adjustment for demographic variables (OR, 19.7l 95% CI, 13.1–29.6) ( Table 2 ).
Logistic regression analysis.
Insomnia vs. non-insomnia population. OR, odds ratio; CI, 95% confidence interval;
Difference in Sleep-Related Scales Among the Four Groups
Participants at high risk for insomnia with both anxiety and depression were associated with significantly higher ESS and PSQI scores relative to the anxiety-only group ( Figure 2 ). Meanwhile, individuals with no psychiatric comorbidities were associated with lower PSQI scores relative to the three other groups. ISI scores were significantly higher among participants with both anxiety and depression than those with any psychiatric comorbidities. When stratified by the presence of anxiety and depression, severe ISI tended to increase from Groups 1–4 ( Figure 3 ).

Differences in scores on sleep-related scale across four groups. (A) Epworth sleepiness scale and (B) Pittsburg sleep quality index is depicted in box plots. (C) Proportion of each insomnia severity index category is shown in a stacked bar plot. A–/D–, without anxiety or depression; A+/D–, with anxiety only; A–/D+, with depression only; A+/D+, with both anxiety and depression; * p < 0.05, ** p < 0.01, and *** p < 0.001.

Stacked bar plot showing the proportion of each insomnia characteristic scale 1–5 across groups. A–/D–, without anxiety or depression; A+/D–, with anxiety only; A–/D+, with depression only; A+/D+, with both anxiety and depression. Insomnia characteristic scale (ICS) was graded on a scale of 1–5.
Insomnia Characteristics According to the Presence of Anxiety or Depression
No statistical differences were observed among the 4 groups in average sleep time during workdays, weekends, and overall sleep. The three insomnia-related symptom scales of each group are presented in Figure 3 . Regarding the difficulty in initiating sleep and feelings of impaired quality of life, only Groups 1 and 4 featured a statistically significant difference. Group 4 was more likely to have difficulties in maintaining sleep than were Groups 1 and 2. The groups did not differ in their scores on the frequent awakening scale.
Mediation Model Analysis
When insomnia was comorbid with both anxiety and depression, PSQI and ISI were significantly correlated with each other ( Tables 3 , ,4). 4 ). The relationship between anxiety and ISI (beta = 4.21) was attenuated when PSQI was used as a mediator ( Figure 4 ). The Sobel test revealed the significance of the indirect effect of PSQI in mediating both anxiety and depression and ISI ( Table 5 ).
Mediation analysis.
ISI, insomnia severity index; PSQI, Pittsburgh Sleep Quality Index; SE, standard error; P, p-value .
Linear regression analysis, endpoint: ISI.
ISI, insomnia severity index;

Mediation model: the indirect effect of combined anxiety and depression on ISI scores through PSQI. Unstandardized regression coefficients are presented for each path *** p < 0.001.
Linear regression analysis, endpoint: PSQI.
PSQI, Pittsburgh Sleep Quality Index;
We found the prevalence of high risk for insomnia in the Korean adult population to be 10.5%, which is significantly different from previously reported rates in the Korean population (about 20%). Differences between the methodology of our study and those of previous investigations, which used telephone-based interviews without validated questionnaires, may account for the divergent findings ( 29 , 30 ). Measuring residential noise levels in addition to employing a structured questionnaire, a population-based study conducted in Japan, reported a crude prevalence of insomnia of 8.8% ( 31 ), which better accords with our results. The prevalence of overall anxiety and depression observed in our study was also similar to or slightly higher than the rates reported by prior Korean epidemiologic studies ( 32 , 33 ). Similar to a previous study ( 34 ), we found that the prevalence of insomnia to be higher among women and individuals with low levels of education. We also found no association between age and the prevalence of insomnia. This discrepancy may be due to the changes in the lifestyles of young Korean adults, which might cause higher rates of insomnia among adults below the age of 30 ( 35 ).
We observed that 47.6% of individuals with high risks for insomnia had comorbidities of anxiety or depression. This finding in the Korean population was similar to a previously reported rate: 40% of individuals with insomnia had comorbid psychiatric disorders ( 11 ), among which depression and anxiety disorders were reportedly the most common.
As expected, Group 1 had lower PSQI and ISI scores than those with anxiety-only, depression-only, or both (Groups 2, 3, and 4). The ESS scores of subjects with anxiety-only or depression-only (Groups 2 and 3), but not both (Group 4), did not differ significantly from those of individuals without any anxiety or depression (Group 1) as depicted in Figure 1 . This might be due to individuals with insomnia having excessive hyperarousal continuing throughout the day time rather than daytime sleepiness. Group 4 was consistently associated with poorer sleep-related indices than was Group 2. While Groups 3 and 4 differed significantly in the proportion of participants who had higher ISI scores ( Figure 3C ), they did not differ significantly in ESS and PSQI scores. This result might be due to a lack of statistical power associated with sample sizes (Group 3 was relatively small).
Individuals with high risk for insomnia are associated with an increased incidence of anxiety and depression relative to those without insomnia. After controlling for possible confounding variables, subjects with insomnia were found to be 9.8 times more likely to have anxiety than subjects without insomnia and 19.7 times more likely to have depression. In addition, we found Groups 2, 3, and 4, in order, had significantly higher rates of insomnia symptoms.
Numerous studies have reported an association between insomnia and depression and anxiety ( 36 – 40 ). Indeed, patients with persistent insomnia are reportedly predisposed to developing psychiatric illness and are more prone to the recurrence of depression ( 41 , 42 ). Among patients with partially treated depression, residual symptoms such as anxiety and insomnia are among the most powerful predictors for relapse ( 41 ). Additionally, insomnia and anxiety share a pathogenetic mechanism: hyperarousal caused by dysregulation of neurotransmitter systems including cholinergic and GABA (gamma-aminobutyric acid) eric mechanisms ( 43 ). Hyperarousal and insufficient sleep disrupt the function of corticolimbic circuitry, which leads to impaired affective reactivity and regulation ( 44 ). Genetic studies also showed a strong overlap between genetic influences on insomnia, depression, and anxiety ( 44 ). This is reflected in high comorbidity of the two conditions (70–90% of patients with anxiety report insomnia) ( 45 ).
The mediational model revealed that the effect of combined anxiety and depression on insomnia severity is mediated by poor sleep behaviors. Considering that the essential feature of insomnia is conditioned arousal affected by dysfunctional efforts to sleep and negative expectations, patients with insomnia become anxious and frustrated as insomnia symptoms persist. The mediator PSQI covers general sleep problems including sleep latency; sleep duration and efficiency; and sleep-disturbing factors, such as nocturia, nocturnal breathing problems, pain, feeling too hot or cold, use of sleeping pills, and daytime dysfunction. Even in the absence of direct insomnia-related factors, nearly all of these components are associated with somatic symptoms of depression ( 46 ), and more than half of patients with anxiety disorders report those somatic complaints ( 47 ). These observations are in agreement with the findings of our mediation analysis: depression and anxiety have indirect relationships with the degree of insomnia severity and poor sleep-related somatic symptoms, which in turn aggravates insomnia severity.
There was a systemic review on the relationships among anxiety, depression, and sleep disturbance. They showed that insomnia and sleep quality features have “bidirectional” relationships with anxiety and depression, respectively ( 48 ). Moreover, regarding treatment, cognitive behavioral therapy that focuses on attenuating anxiety and depression reduces insomnia severity and the symptoms of the two psychiatric conditions ( 49 ).
Our study is subject to several limitations. First, we did not consider comorbidities of anxiety and depression with other sleep disorders, including obstructive sleep apnea, narcolepsy, and restless legs syndrome. The presence of any pain or other significant medical illnesses was also not investigated. Second, even though we performed a population-based study with a low sampling error, the statistical power for the examination of subgroups might have been diminished due to the small samples (especially Group 3). Third, in the mediation analysis, we did not conduct a detailed investigation to elucidate the component of PSQI that features a close relationship with insomnia severity. Third, we did not analyze longitudinal data which causes a problem to establish causality, despite a quite large sample. Last, we didn't consider the impact of medication use when we analyzed our data. The medication status is an important factor that can change the subjects' mental status. Unfortunately, our research target was the general population. So, it was not easy to obtain optimal medical information from the survey method.
This report is, to the best of our knowledge, the first to evaluate how anxiety and depression affect sleep quality and the severity of insomnia. The prevalence of high risk for insomnia and the comorbidities with anxiety and depression is comparable to the findings of previous reports. Daytime sleepiness, general sleep quality, and insomnia severity were consistently poorer in subjects with both depression and anxiety. Also, we found that the effect of the combination of both psychiatric conditions was mediated by general sleep quality indices, which encompass insomnia and related somatic symptoms. We surmise that anxiety and depression affect insomnia in a supra-additive manner. When treating insomnia patients, clinicians should look for underlying comorbid psychiatric conditions to determine the appropriate therapy and enhance the therapeutic effect.
Data Availability
Author contributions.
C-MO, KC, and MC: study concept and design. C-MO, HK, KC, and MC: acquisition, analysis, and interpretation of data. C-MO and KC: drafting of the manuscript. HK, KC, and HN: statistical analysis. C-MO: obtained funding. KC and MC: study supervision. All authors read and approved the manuscript.
Conflict of Interest Statement
The authors declare that the research was conducted in the absence of any commercial or financial relationships that could be construed as a potential conflict of interest.
Acknowledgments
We would like to thank the Biostatistics Collaboration Unit members of Yonsei Medical University for their assistance in data analysis.
Funding. This research was supported by grant provided to C-MO by the Basic Science Research Program through the National Research Foundation of Korea (NRF), which was funded by the Ministry of Education (Grant No. 2016R1A6A3A04010466). KC was supported by a faculty research grant from Yonsei University College of Medicine 6-2018-0092.
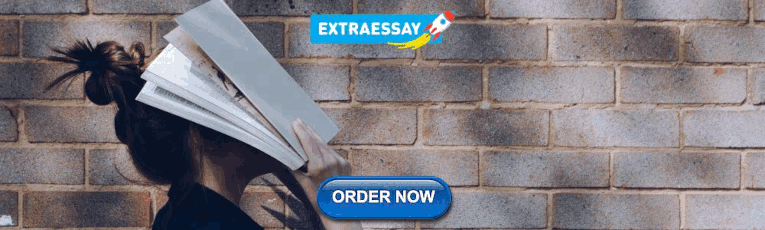
IMAGES
VIDEO
COMMENTS
The findings revealed a 19% concurrent comorbidity between these disorders, and in 65% of the cases, social phobia preceded major depressive disorder by at least 2 years. In addition, initial presentation with social phobia was associated with a 5.7-fold increased risk of developing major depressive disorder. These associations between anxiety ...
This article is a systematic review of the literature on the impact of anxiety and depression on health-related quality of life (HRQoL) in patients with various medical conditions. It summarizes the evidence from 42 studies and discusses the possible mechanisms, implications and limitations of the findings. The article aims to provide a comprehensive overview of the topic and suggest ...
This review may be used as an evidence base by those in public health, clinical practice, and research. This paper discusses key areas in depression research; however, an exhaustive discussion of all the risk factors and determinants linked to depression and their mechanisms is not possible in one journal article—which, by its very nature, a ...
At the same time, studies of trait anxiety suggest that moderate (vs. low) ELS is associated with greater self-reported anxiety. This study tested the hypothesis that stress inoculation effects are evident for implicit (nonconscious) but not explicit (conscious) aspects of anxiety. Methods: Ninety-seven healthy women were assessed for ELS and ...
Empirical evidence on the predictive relationships between anxiety and depression. There is research evidence supporting the claim that anxiety is a precursor of depression. Pine et al. (1998) ... LT contributed to the drafts at different stages and proof-read the paper. All authors contributed to the article and approved the submitted version.
Depression and Anxiety welcomes original research and synthetic review articles covering neurobiology (genetics and neuroimaging), epidemiology, experimental psychopathology, and treatment (psychotherapeutic and pharmacologic) aspects of mood and anxiety disorders and related phenomena in humans. Read the full Aims and Scope here.
Authors of papers were contacted for clarification when data was missing or unclear. ... with most research on depression focusing on the 5-HT ... The serotonin transporter gene and depression ...
Even though there are few studies at the single level on depression, anxiety, and psychological distress, to the investigator's knowledge, this systematic review and meta-analysis study was the first of its kind that assessed the average prevalence of anxiety, depression, and psychological distress in the global community during the COVID-19 ...
population aged 16-85 years, 14.4% have an anxiety. disorder. The pre valence of depression is 6.2%, with the. prevalence of unipolar depressive episodes being 4.1%, dysthymia, 1.3%, and bipolar ...
Anxiety is widely conceptualized as a state of heightened distress, arousal, and vigilance that can be elicited by potential threat (1, 2).When extreme or pervasive, anxiety can be debilitating ().Anxiety disorders are among the leading cause of years lived with disability, afflicting ∼300 million individuals annually ().In the United States, nearly 1 in 3 individuals will experience an ...
Our lack of knowledge cannot be put down to a scarcity of research in existing treatments. In the past decades, more than 500 randomised trials have examined the effects of antidepressant medications, and more than 600 trials have examined the effects of psychotherapies for depression (although comparatively few are conducted for early-onset depression).
Tragically, 6,200 suicide deaths were reported in 2017 among U.S. adolescents and young adults from 15 to 24 years of age ( 3 ), and suicide is the second leading cause of death among individuals 10-34 years of age ( 4 ). As with other psychiatric illnesses, the risks for developing anxiety disorders and major depression are due to ...
Research on loneliness and depression indicates a bidirectional relationship between both constructs, with greater experienced loneliness predicting future depressive symptoms, and vice versa 5.
Abstract. Comorbid depression and anxiety disorders occur in up to 25% of general practice patients. About 85% of patients with depression have significant anxiety, and 90% of patients with anxiety disorder have depression. Symptomatology may initially seem vague and non-specific. A careful history and examination with relevant investigations ...
Depression is a prevalent psychiatric disorder that often leads to poor quality of life and impaired functioning. Treatment during the acute phase of a major depressive episode aims to help the patient reach a remission state and eventually return to their baseline level of functioning. Pharmacotherapy, especially selective serotonin reuptake ...
Most research has examined stress generation in the context of depression (e.g., Liu and Alloy, 2010) with considerably less research on anxiety (Meyer and Curry, 2017). This gap is surprising given that depression and anxiety share etiologies ( Kendler et al., 1992 ) and symptoms ( Clark and Watson, 1991 ) that might partially explain stress ...
We review the interdisciplinary evidence of the bidirectional causal relationship between poverty and common mental illnesses—depression and anxiety—and the underlying mechanisms. Research shows that mental illness reduces employment and therefore income, and that psychological interventions generate economic gains.
In the 13 studies, depression was the most commonly measured outcome. The prominent risk factors for depression, anxiety and psychological distress emerging from this review comprised time spent on social media, activities such as repeated checking for messages, personal investment, and addictive or problematic use.
Methods . In order to explore the influencing factors and prevalence of anxiety and depression among healthcare workers, a cross-sectional research design was used to survey 500 healthcare workers using the 14-item Hospital Anxiety Depression Scale (HADS), the Rosenberg Self-Esteem Scale (RSES), the Multidimensional Scale of Perceived Social Support (MSPSS), and the Survey of Perceived ...
In this paper, we explored whether social psychological strains are related to depression, anxiety, and stress in non-clinical populations. Methods 6,305 college students (39.3% men; 60.7% women) from six Chinese provincial-level jurisdictions completed a paper-and-pencil survey with Psychological Strain Scales (PSS-40) and Depression, Anxiety ...
RESEARCH ARTICLES. no. ... Dynamics of daily positive and negative affect and relations to anxiety and depression symptoms in a transdiagnostic clinical sample. Joyce Y. Zhu, André Plamondon, Abby L. Goldstein, Ivar Snorrason, Jasmin Katz, Thröstur Björgvinsson, Pages: 932-943;
This special Research in Context feature explores the development of more effective ways to treat depression, including personalized treatment approaches and both old and new drugs. ... In 2016, Ross led a clinical trial examining whether psilocybin-assisted therapy could reduce depression and anxiety in people with cancer. According to Ross ...
Our paper describes the prevalence of stress, anxiety, and depression among a sample of undergraduate students in a public research university during an early phase of the COVID-19 outbreak. Using well-established clinical tools, we find that stress, anxiety, and depression were the pervasive problems for college student population during the ...
This paper is in the following e-collection/theme issue: Web-based and Mobile Health Interventions (2972) Innovations and Technology in Cancer Care (389) Emotional, Social, Psychological Support for Cancer (181) Mobile Apps for Cancer Care and Cancer Prevention and Screening (189) Depression and Mood Disorders; Suicide Prevention (1234) Anxiety and Stress Disorders (970) Supporting Partners ...
Please use one of the following formats to cite this article in your essay, paper or report: APA. Thomas, Liji. (2024, April 22). Academic pressure linked to higher depression risk in teens. News ...
Data were collected using a questionnaire. DASS (Depression, Anxiety and Stress Scale) Questionnaire (short form) was used, which is a different, simple, and approved instrument for assessing depression, anxiety, and stress both in clinical settings and in the community (21, 22). DASS is a short screening tool that measures depression, anxiety ...
Introduction: One of the most common sleep disorders, insomnia is a significant public health concern. Several psychiatric disorders, such as anxiety disorders and depression, have shown strong relationships with insomnia. However, the clinical impact of the combination of these two conditions on insomnia severity and sleep quality remains unknown.